
To achieve the automation of the sewing industry,
we are digitally transforming the center of production.
To achieve the automation
of the sewing industry,
we are digitally transforming
the center of production.

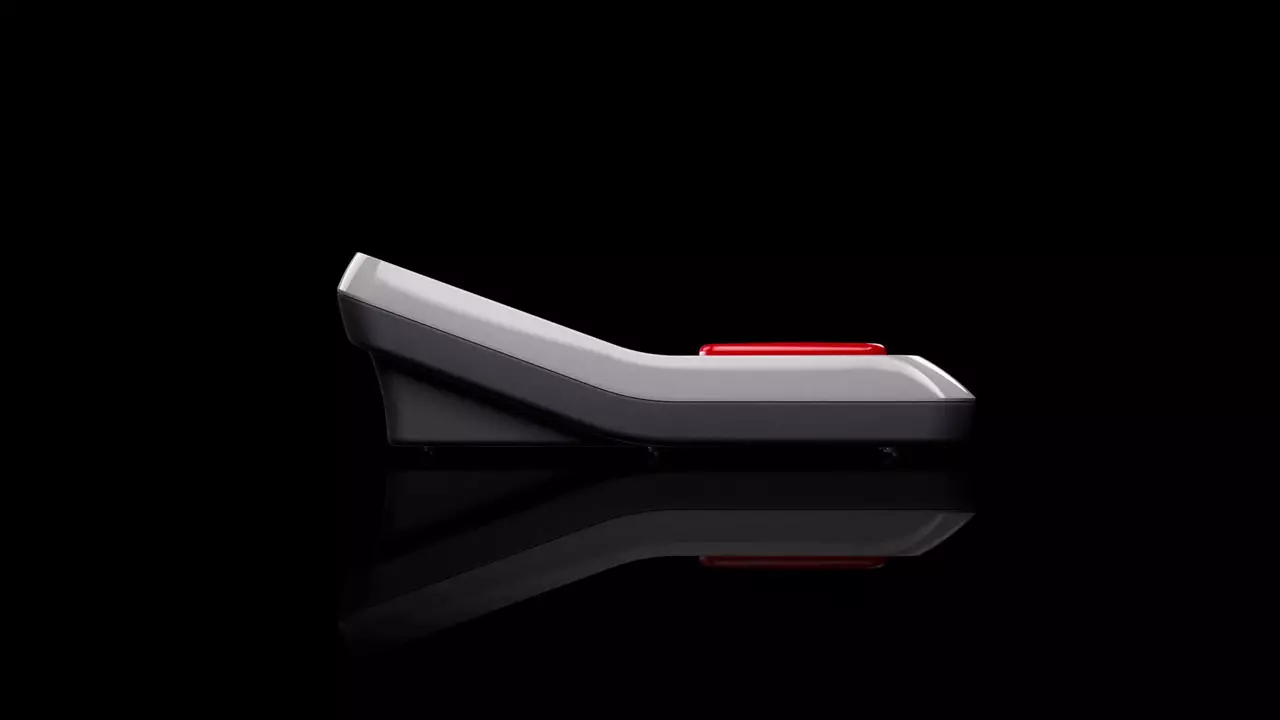
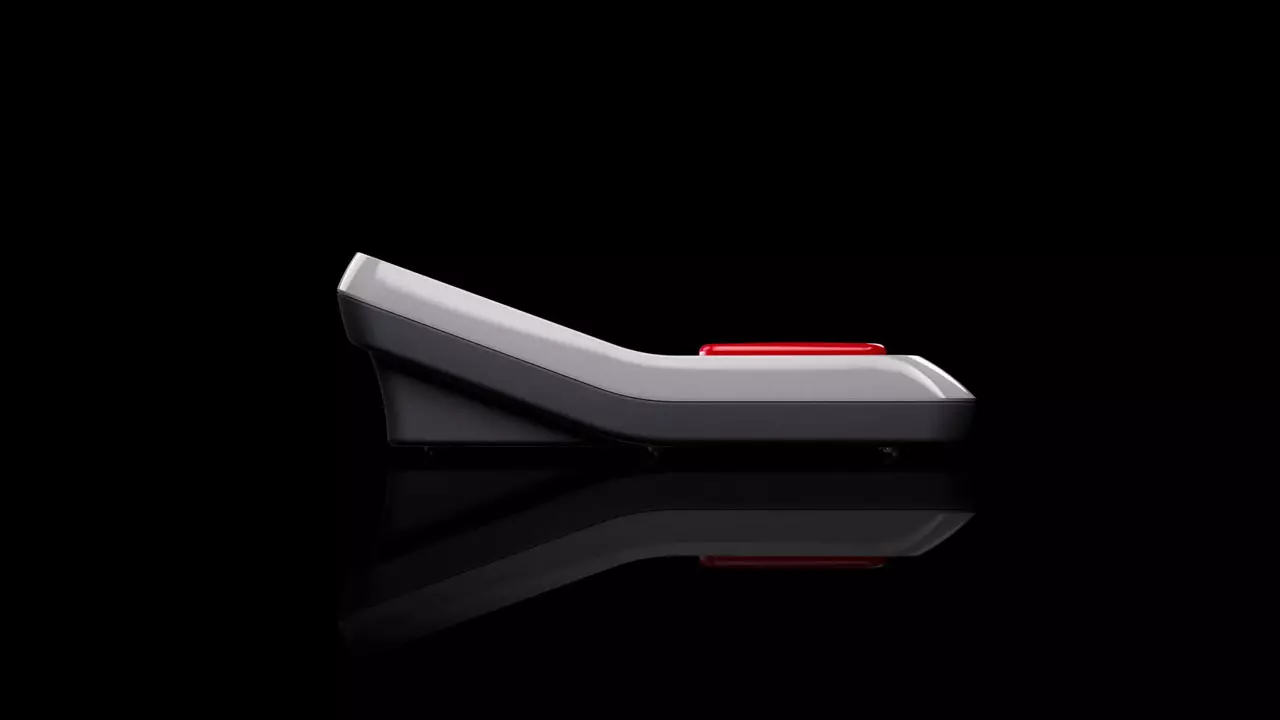
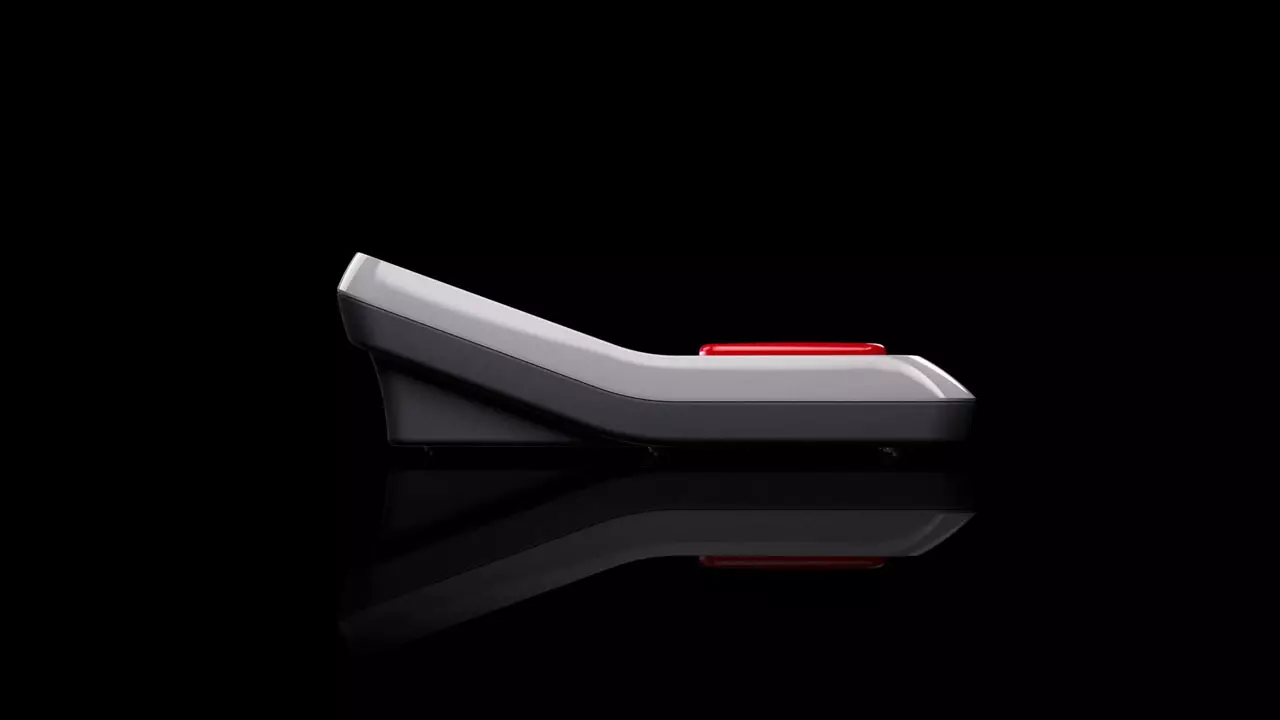
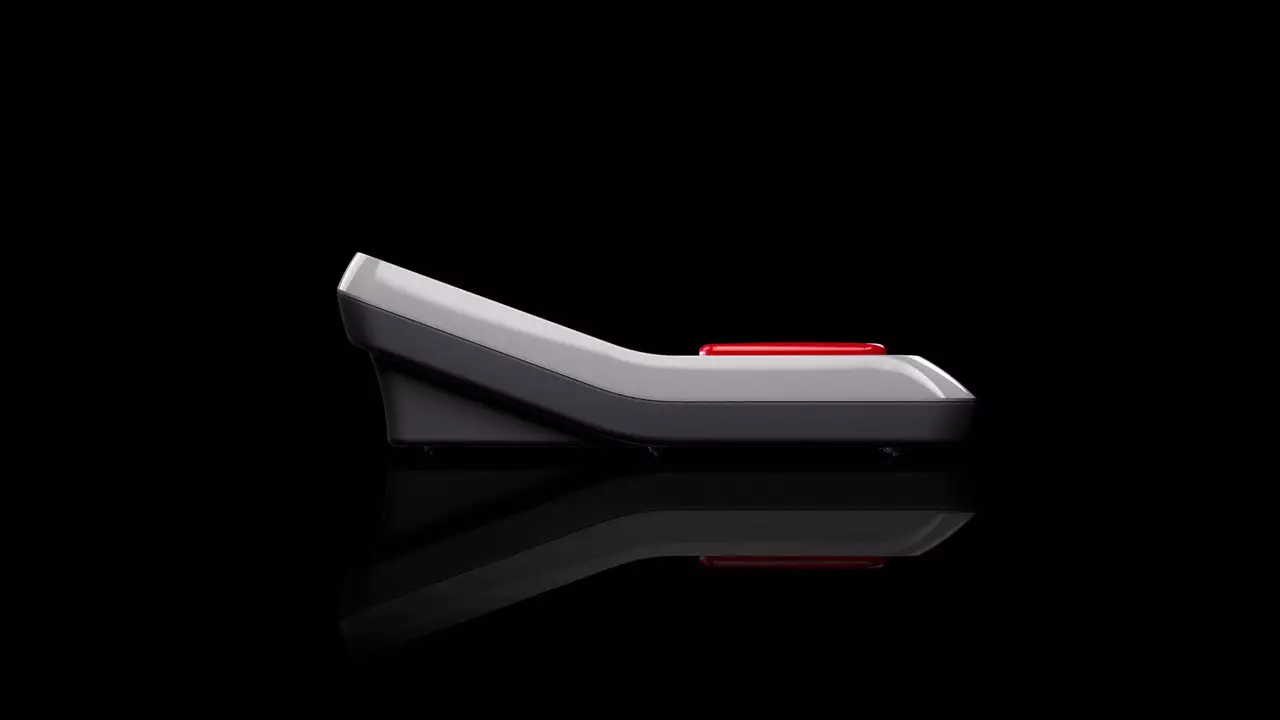
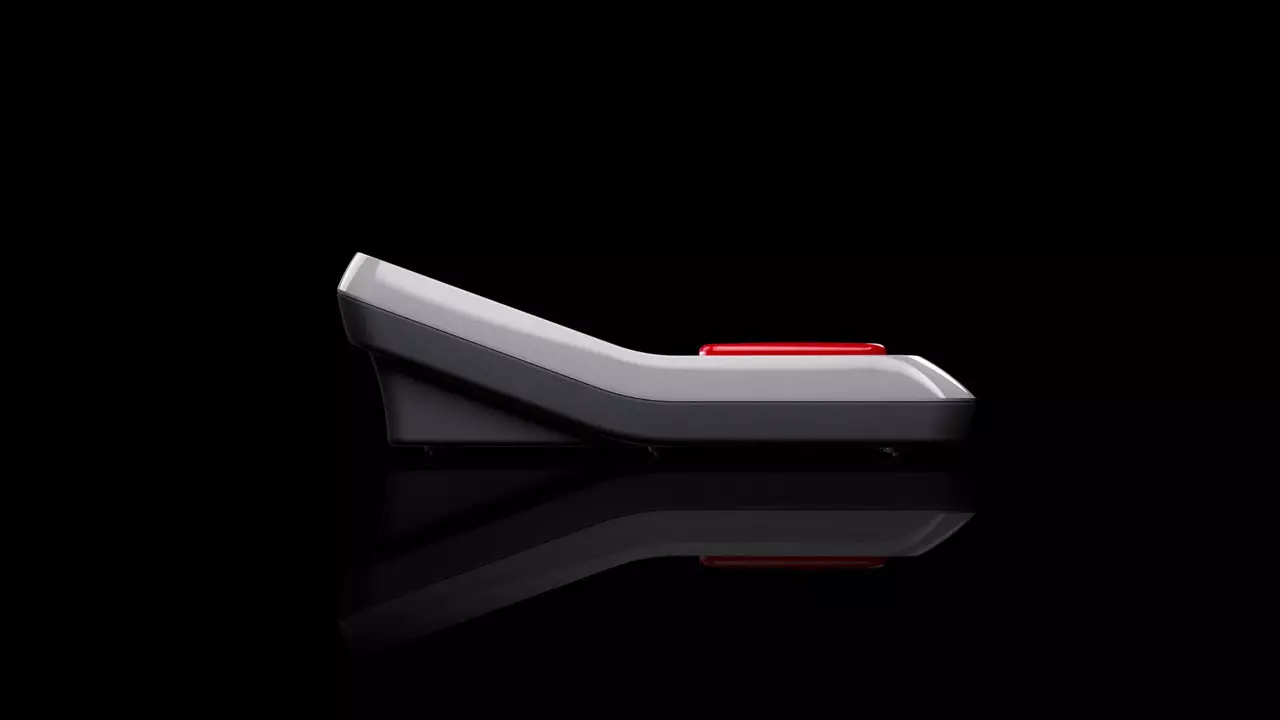
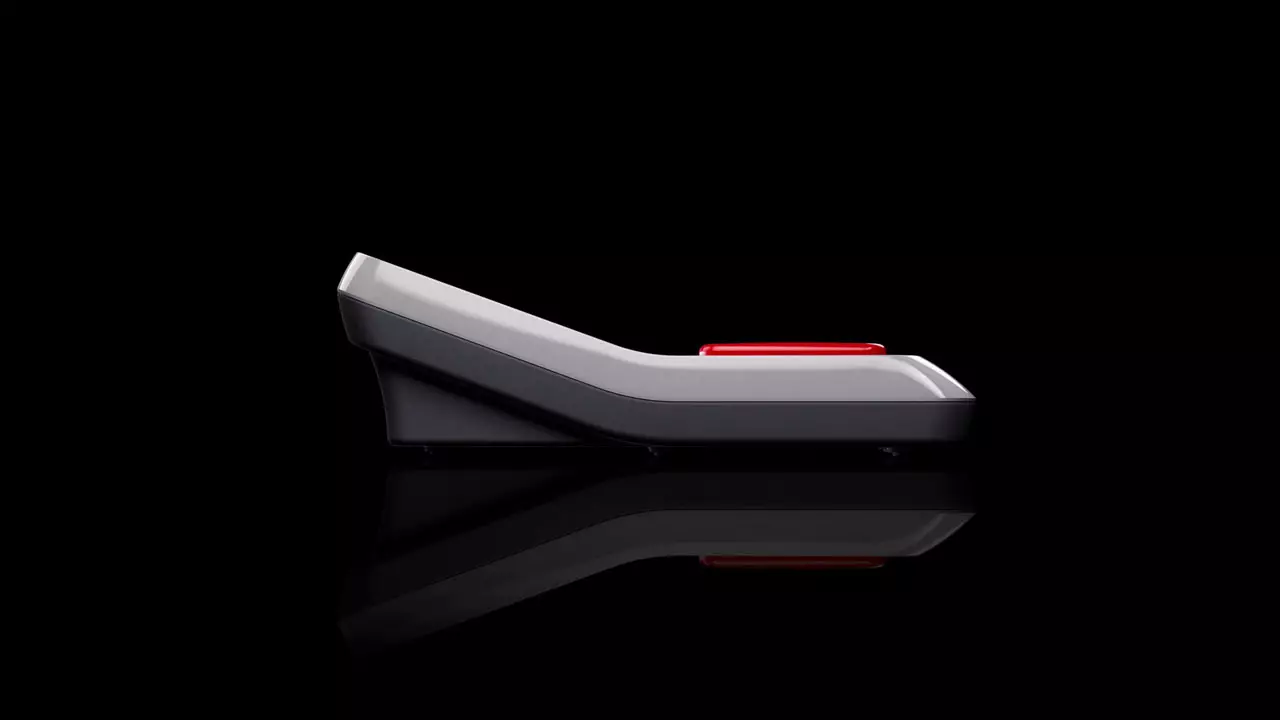
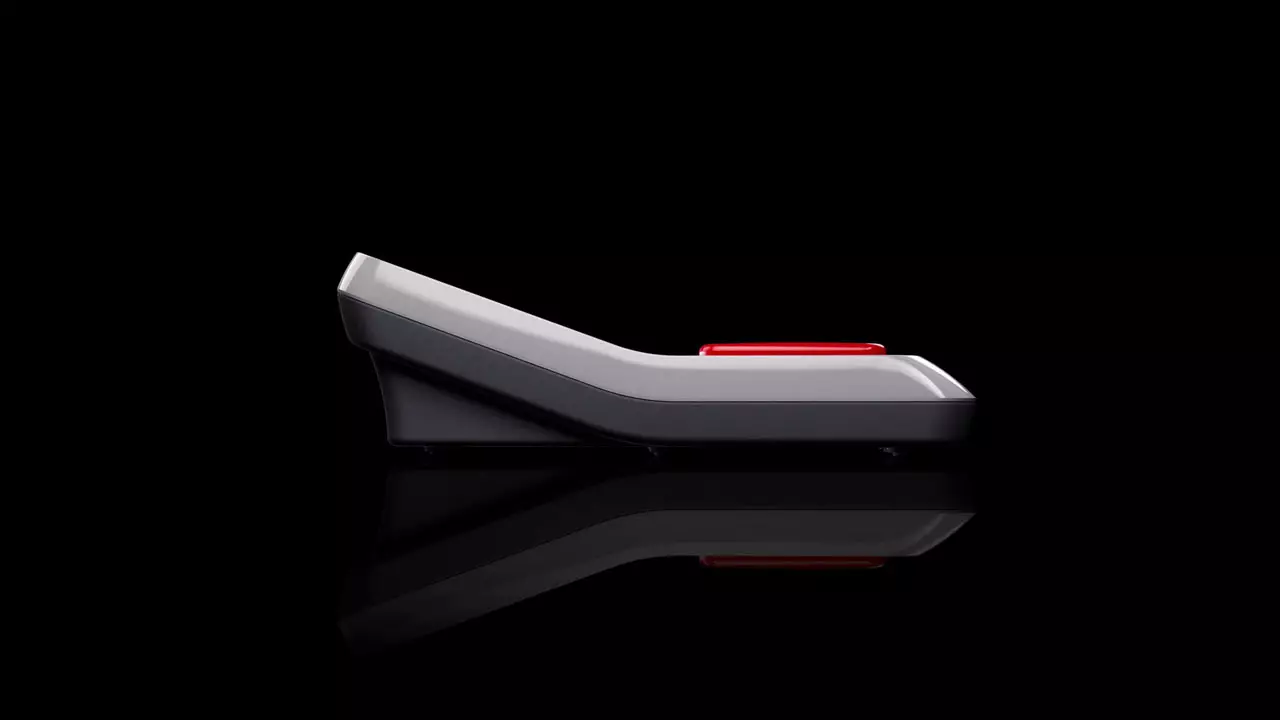
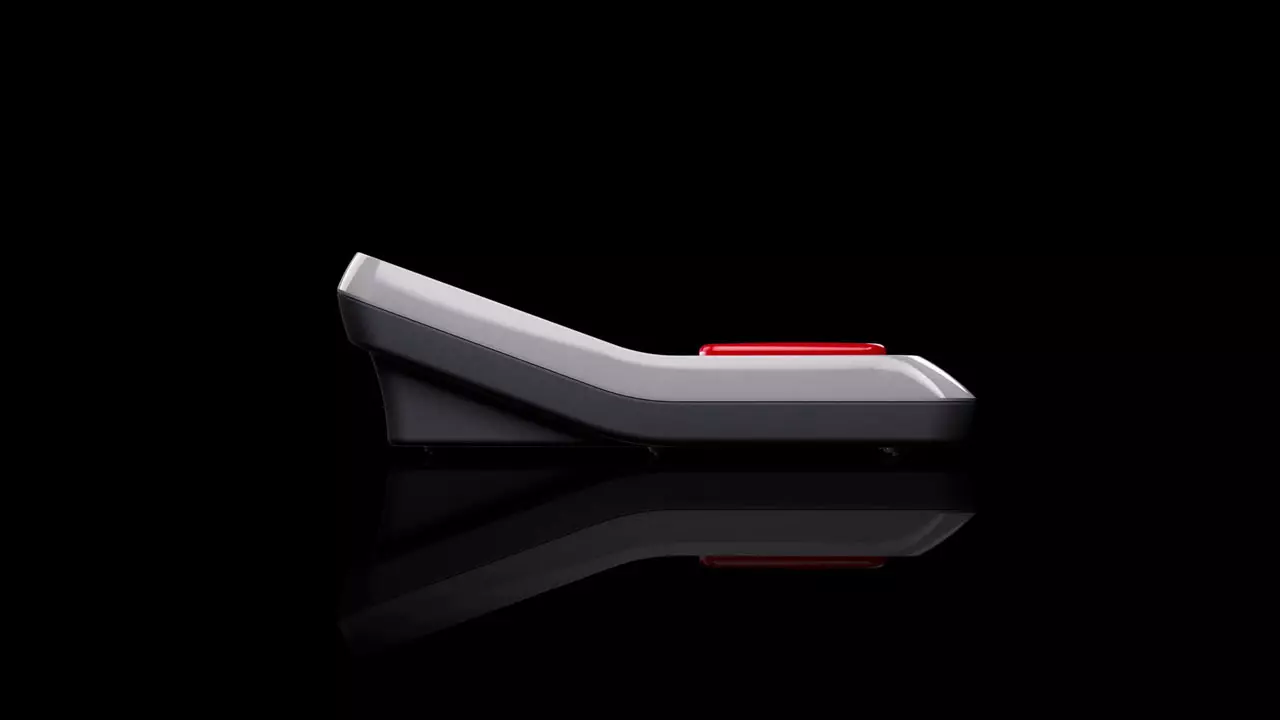
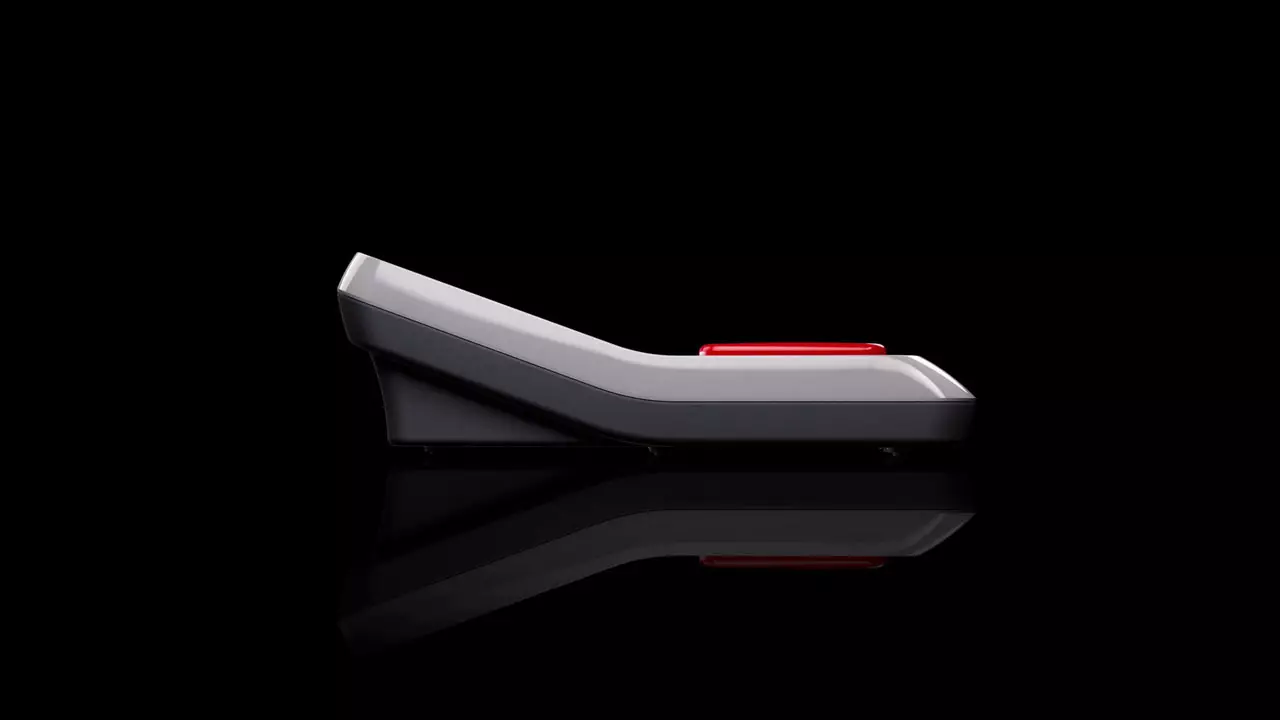
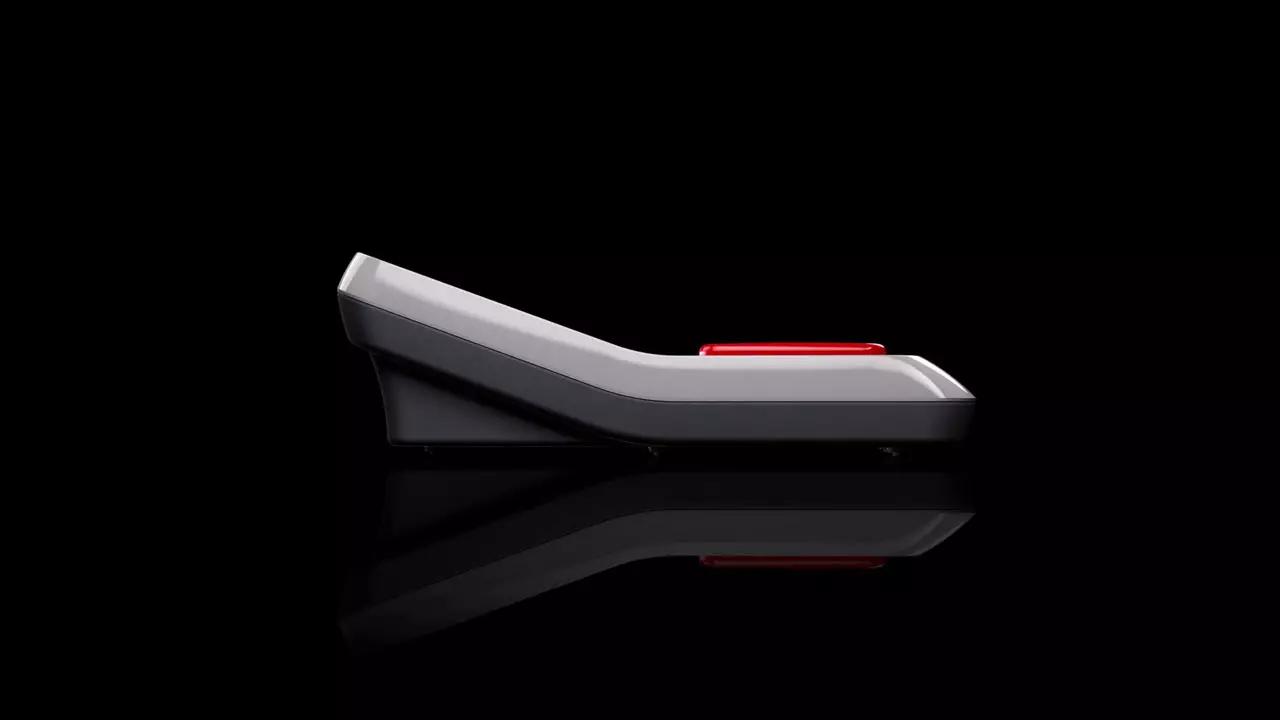
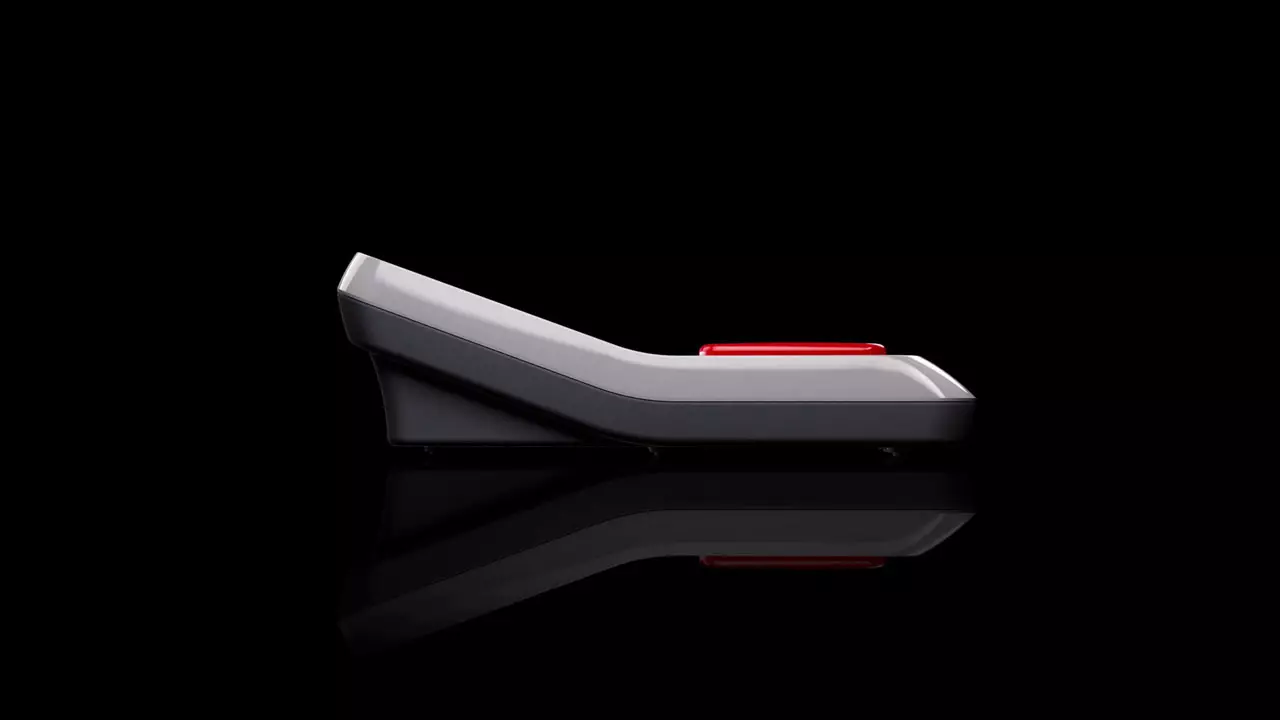
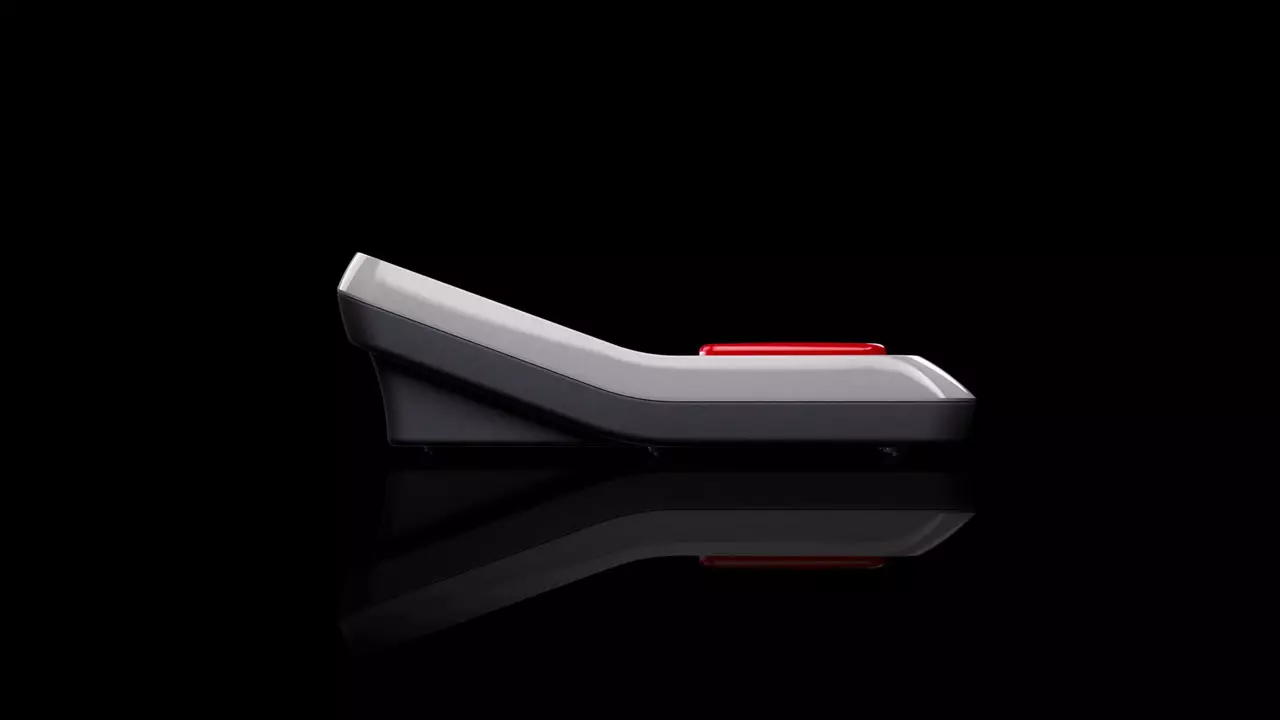
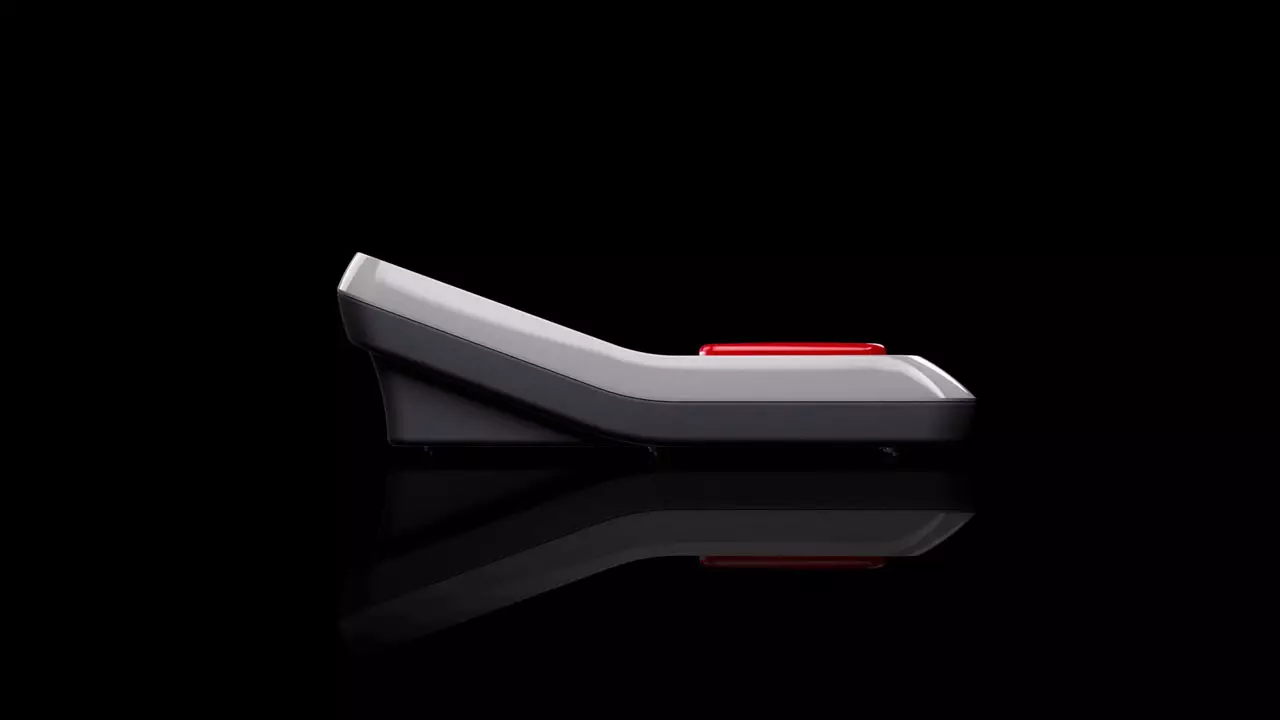
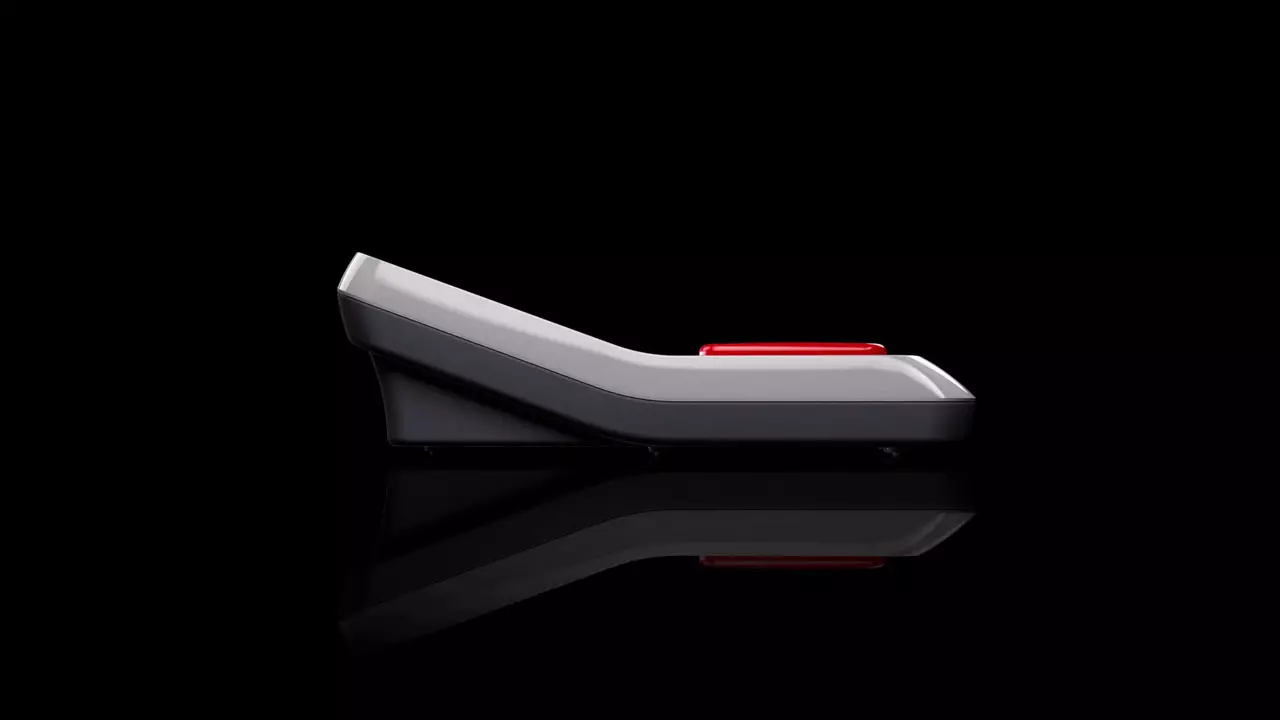
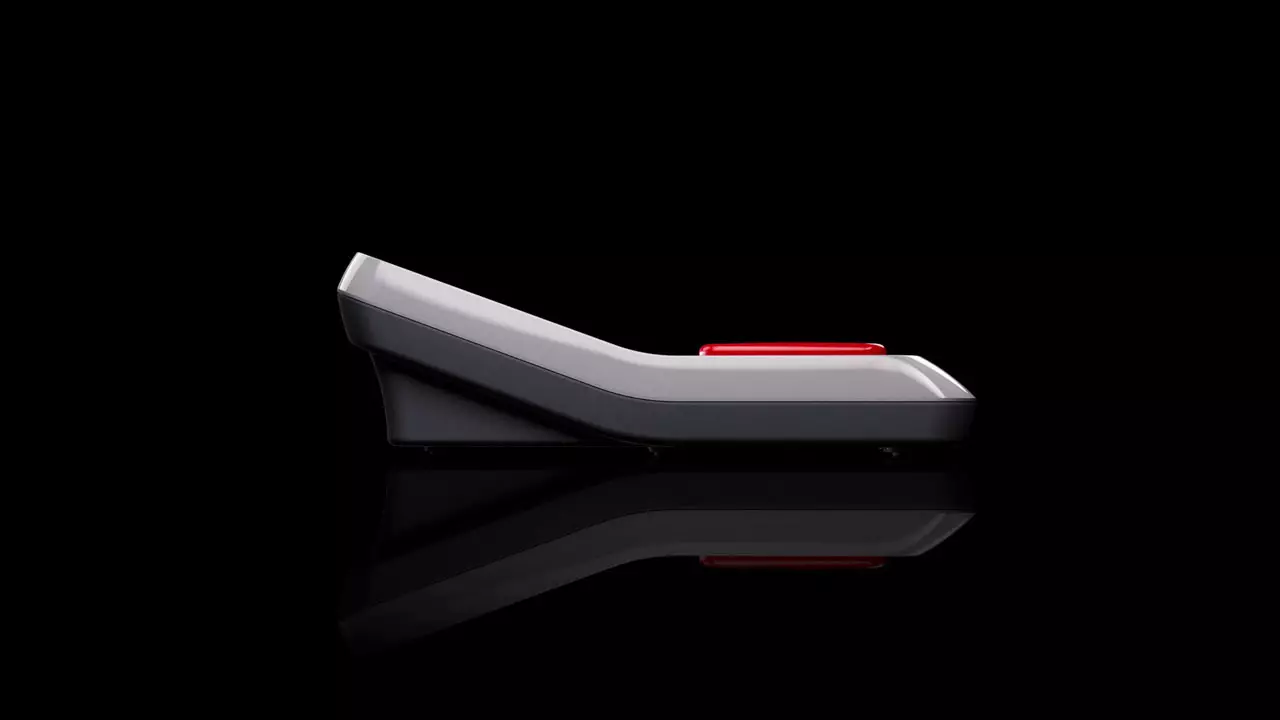
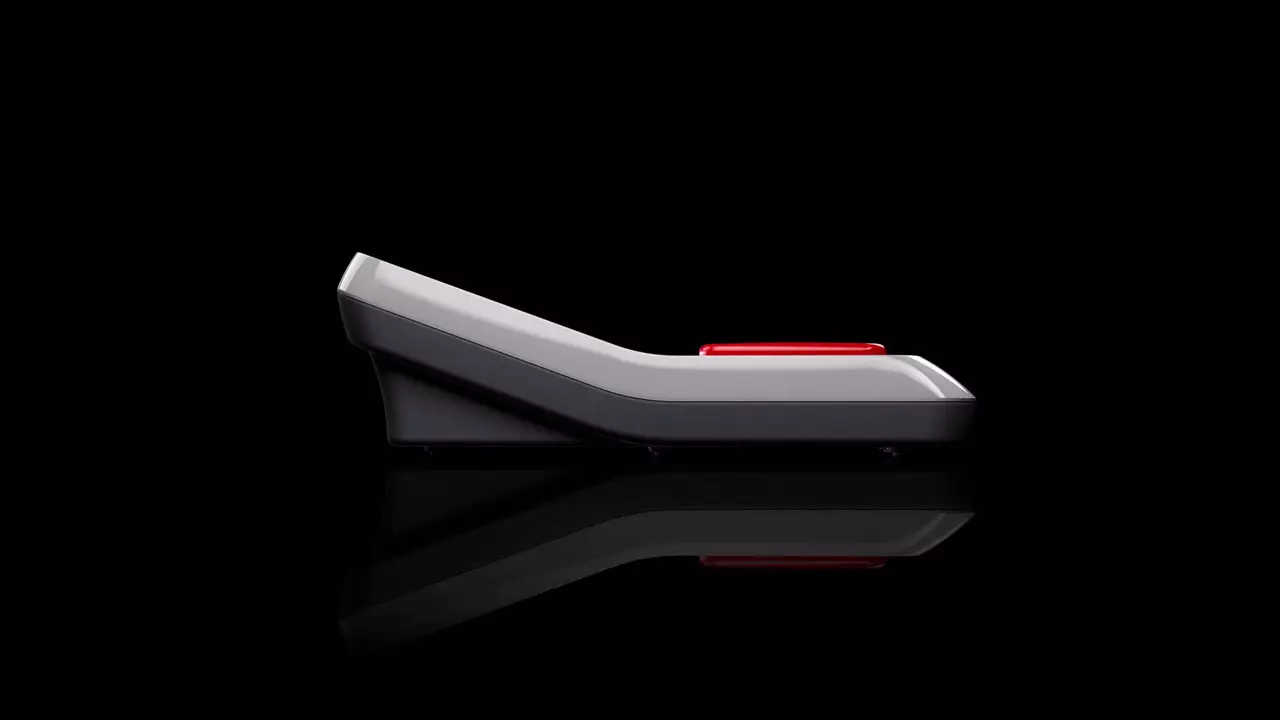
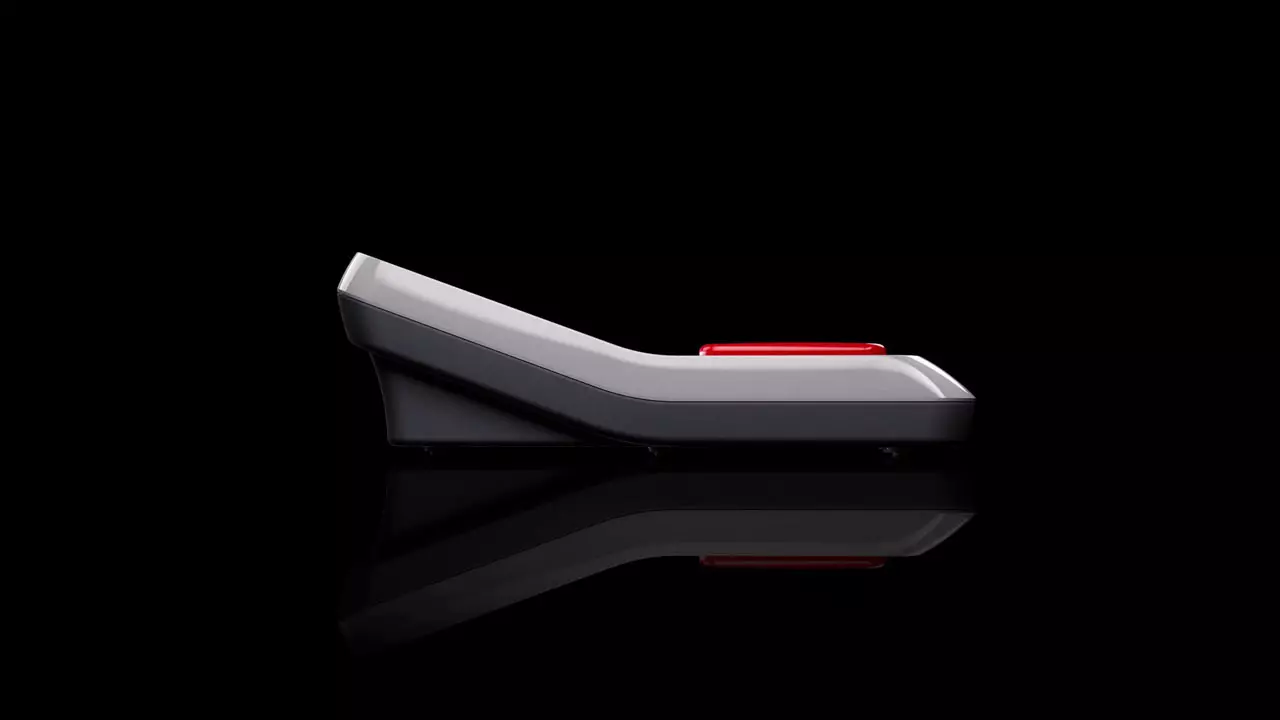
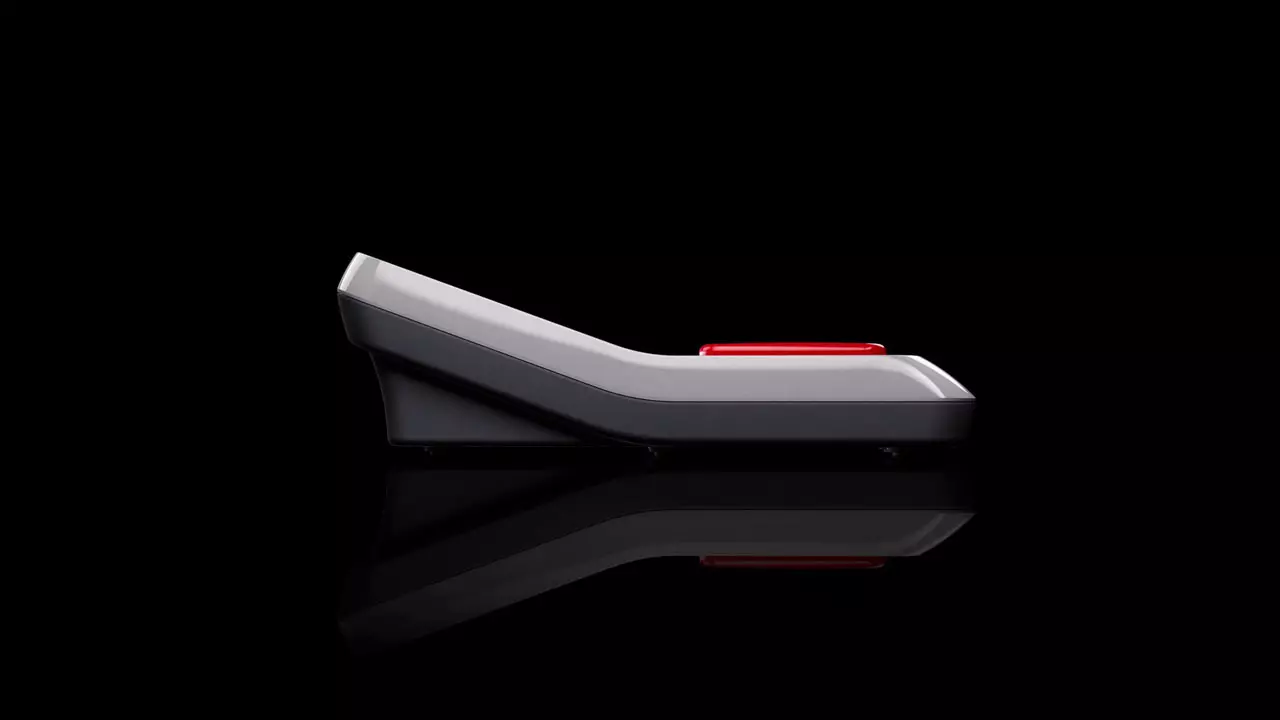
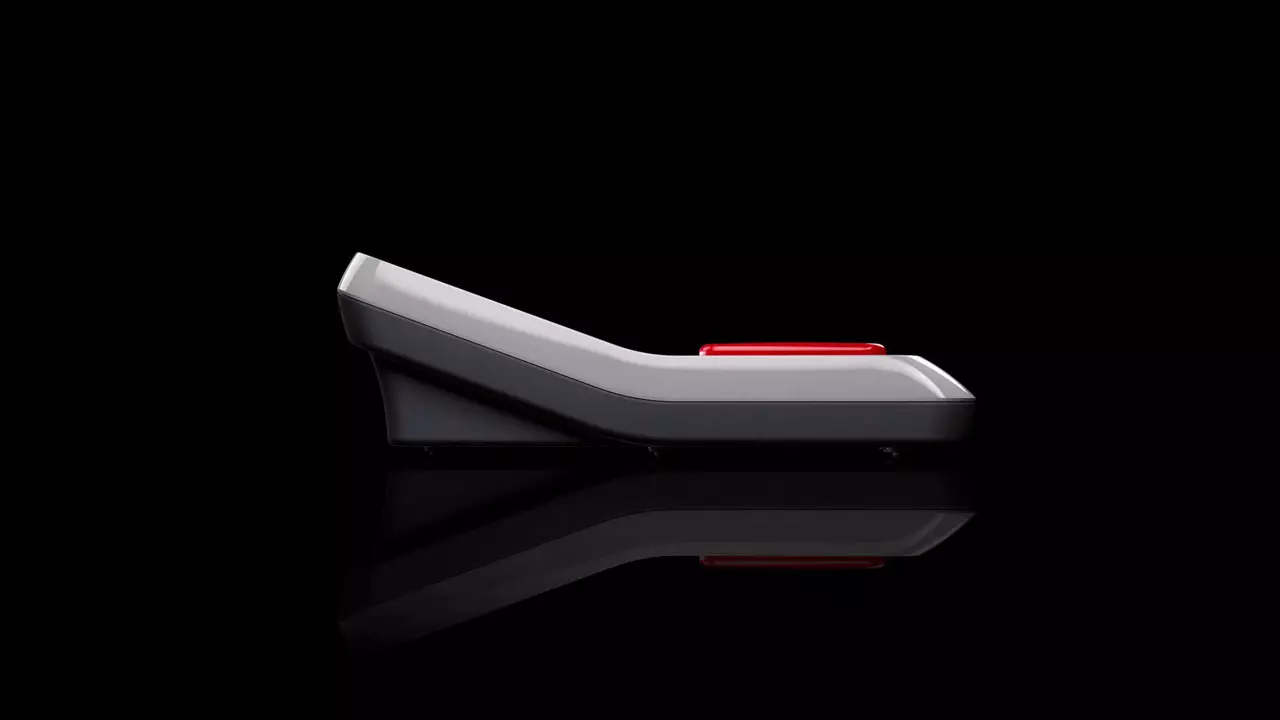
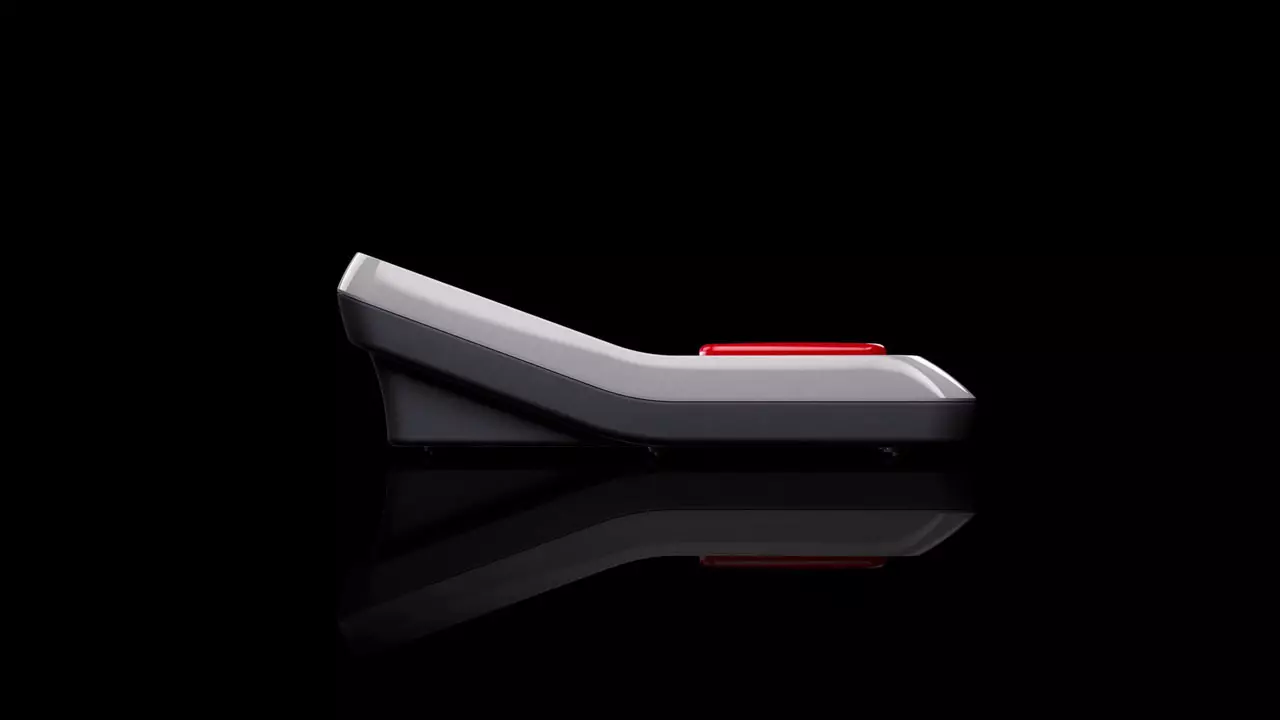
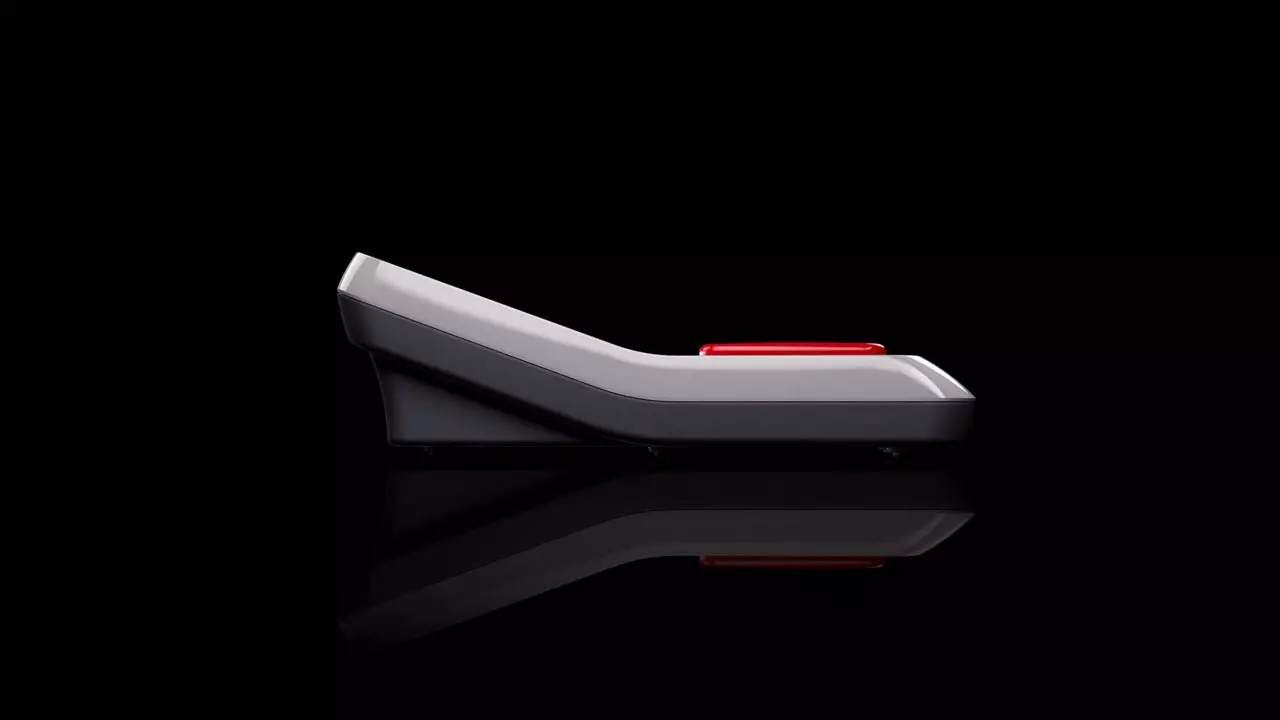
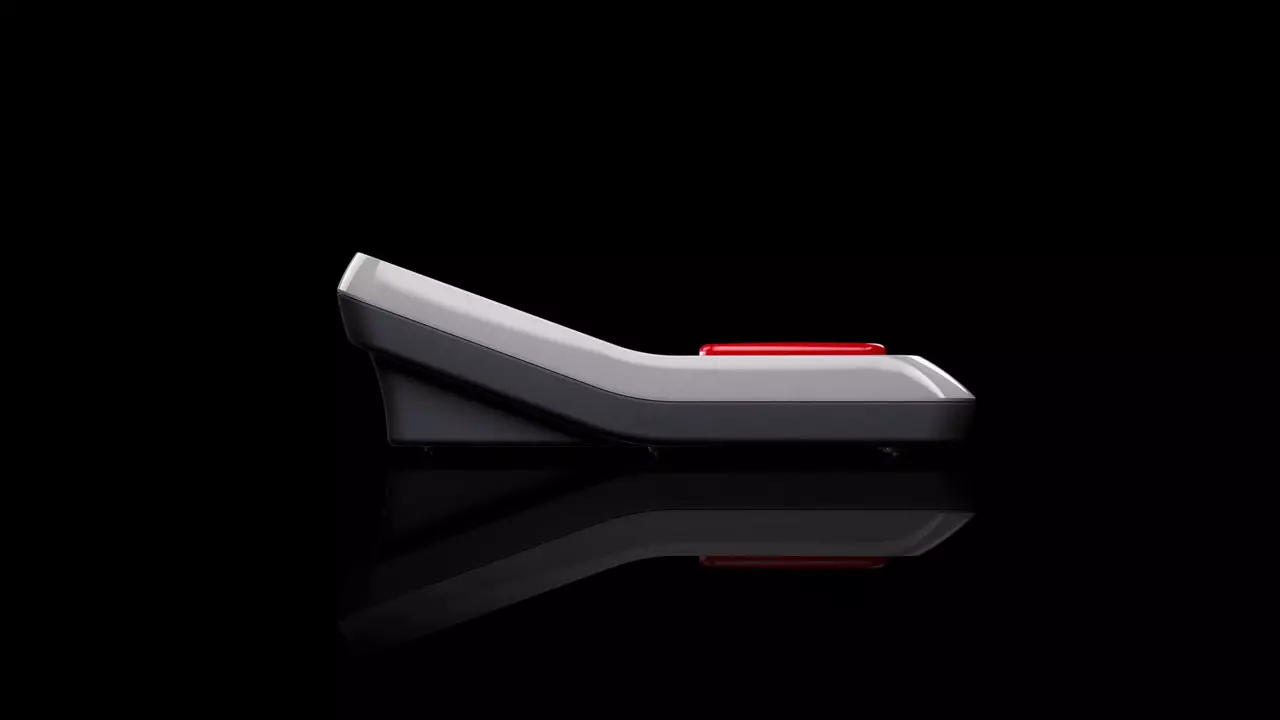
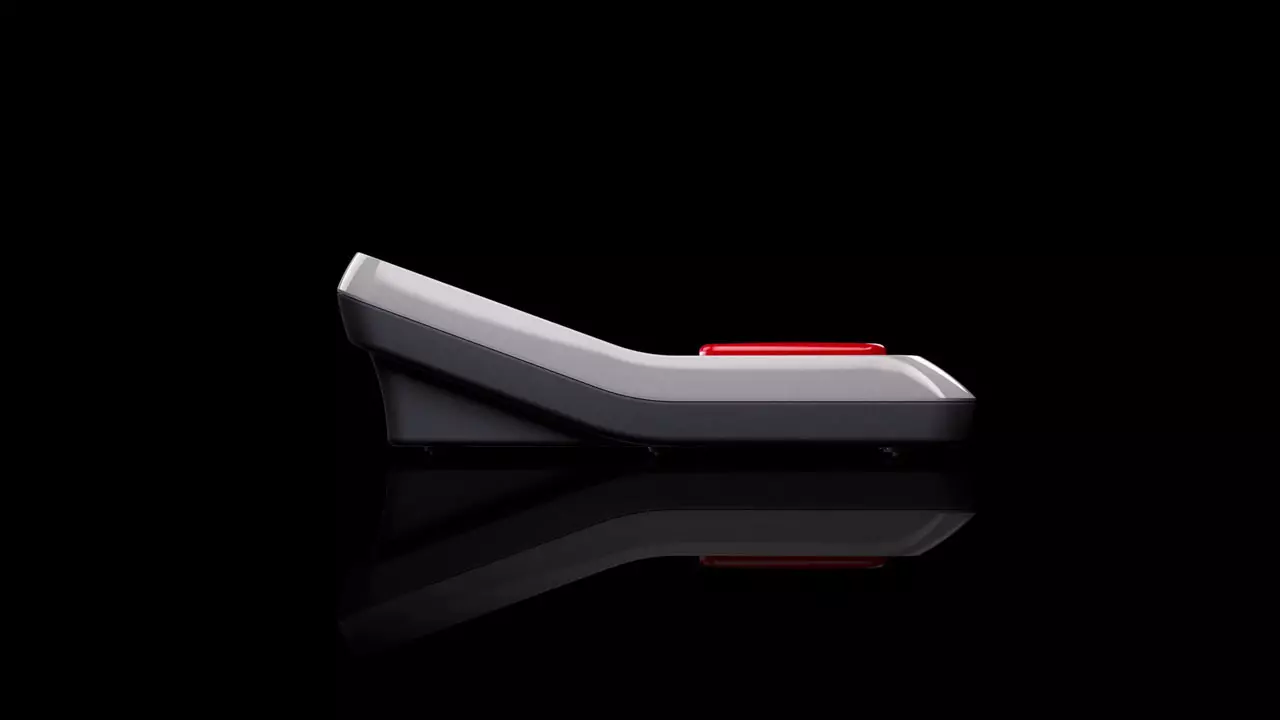
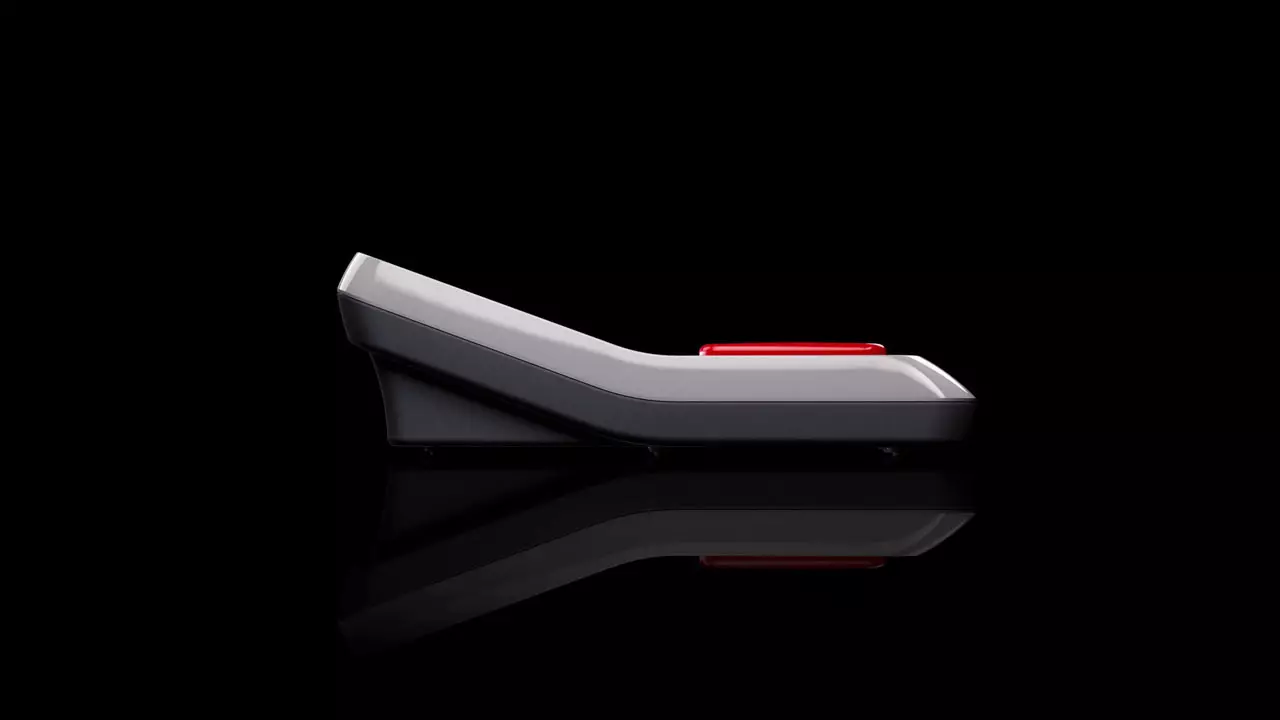
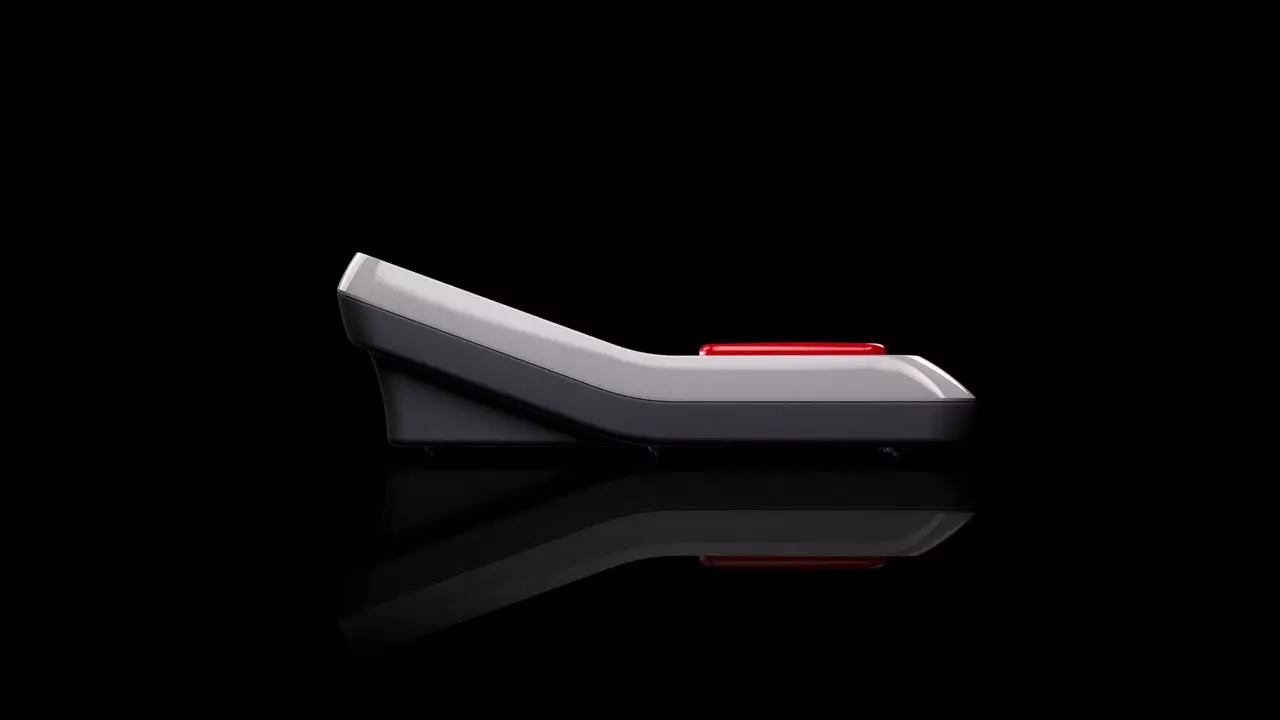
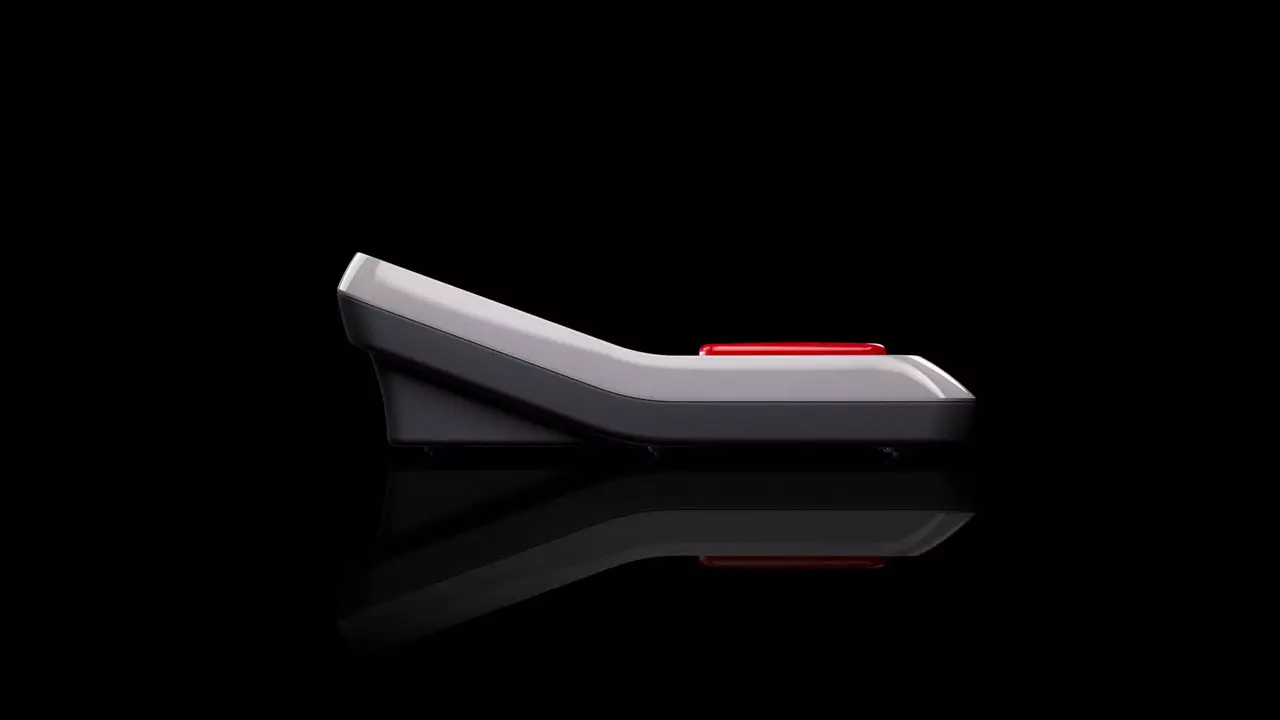
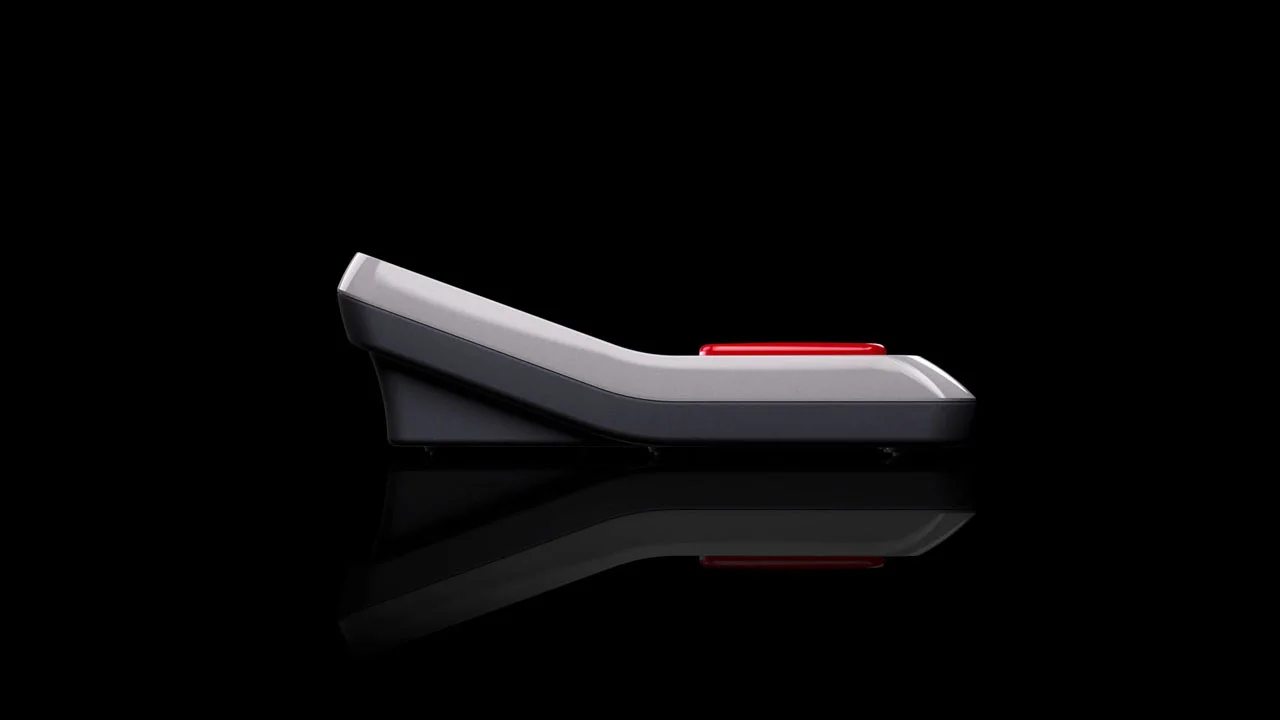
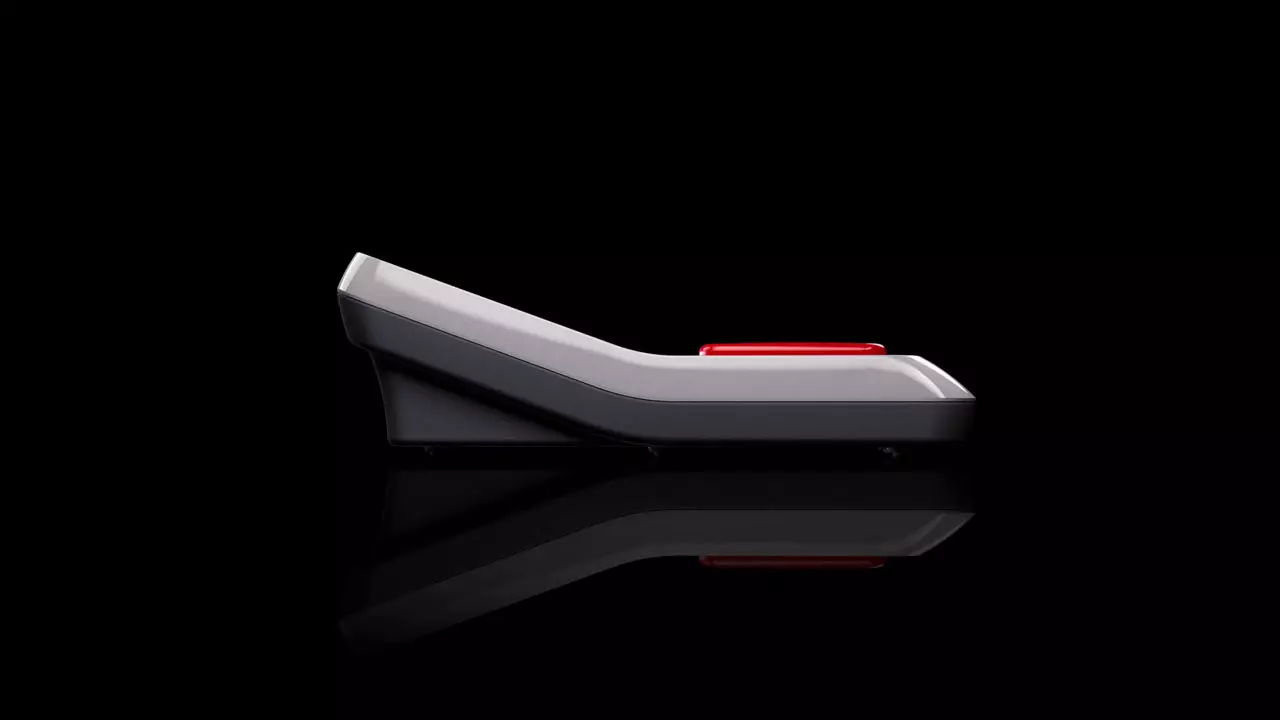
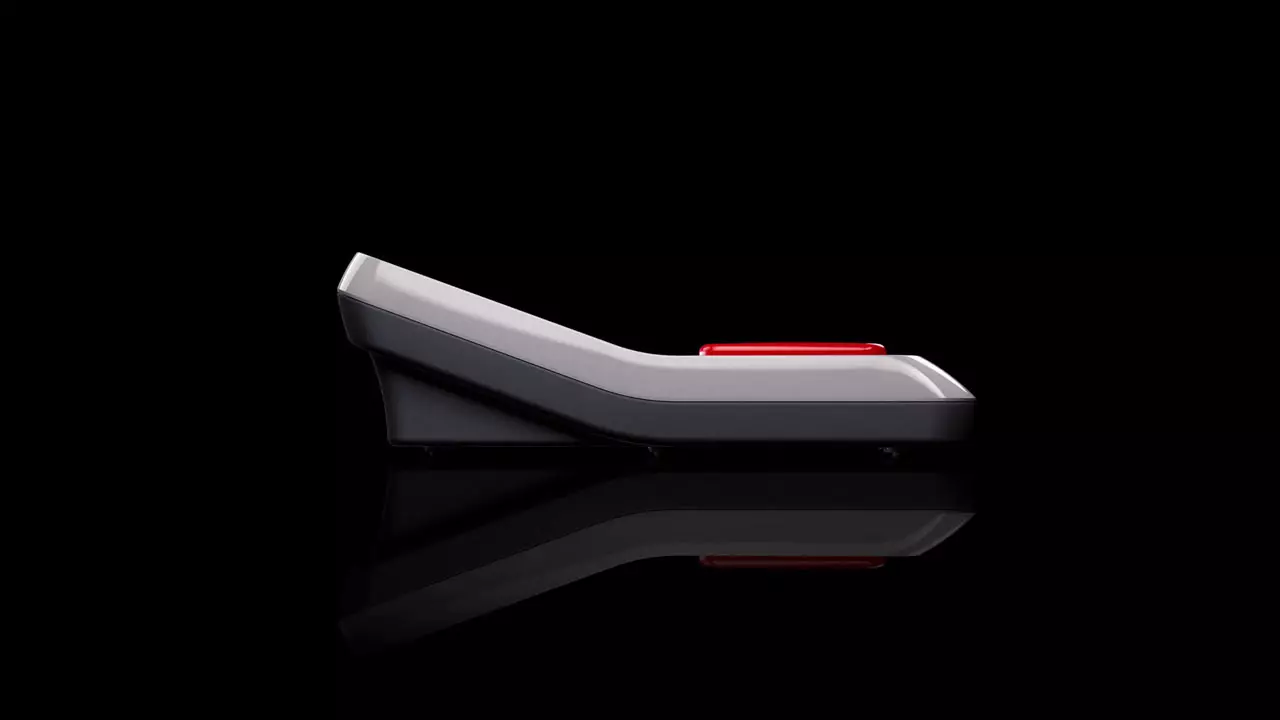
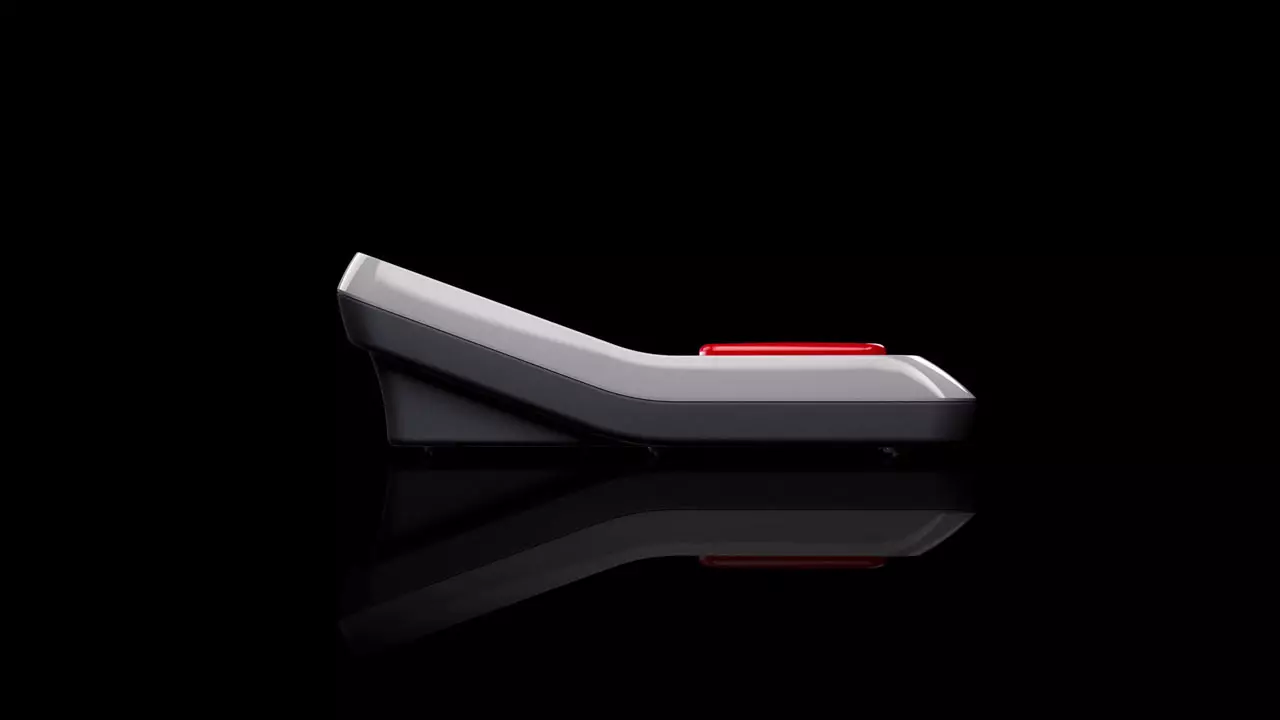
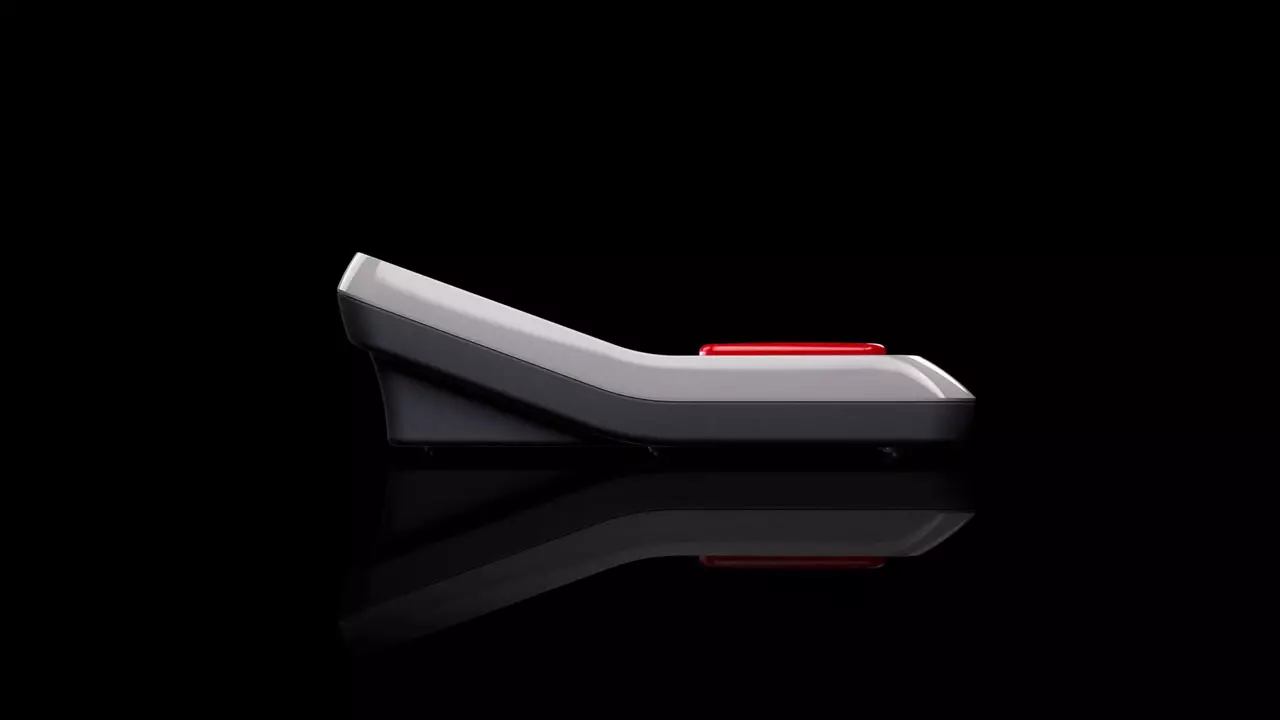
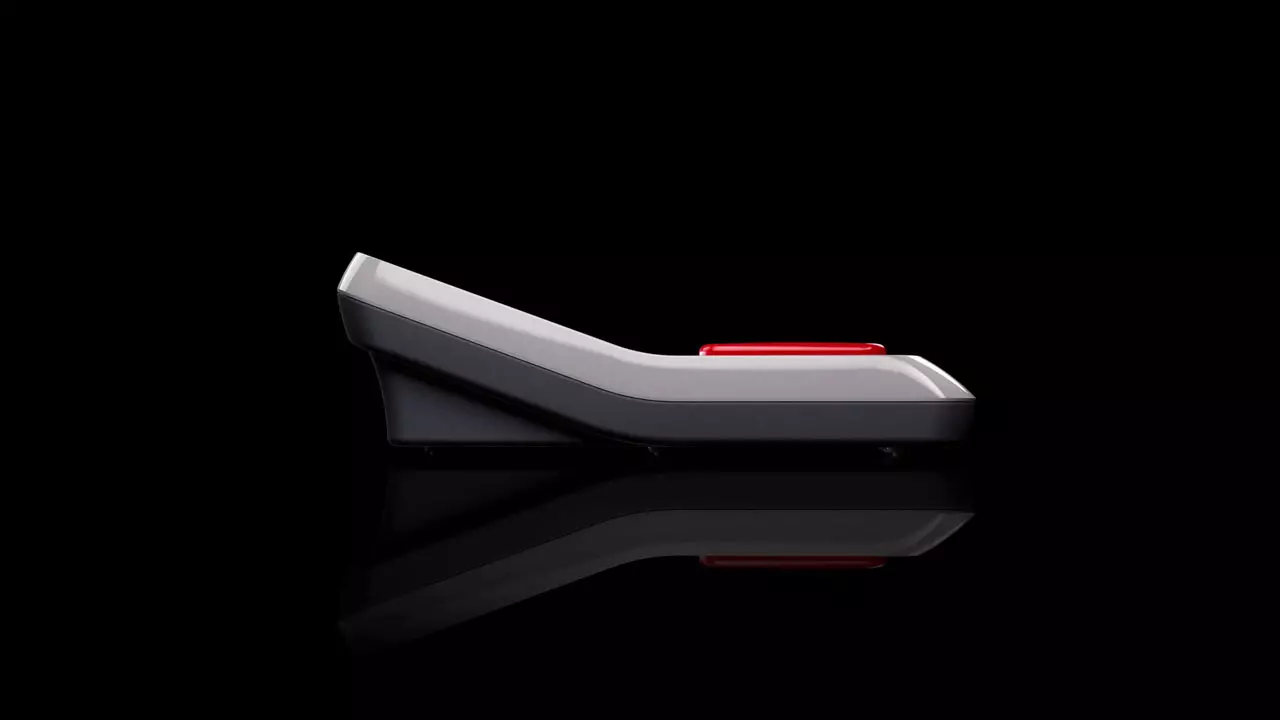
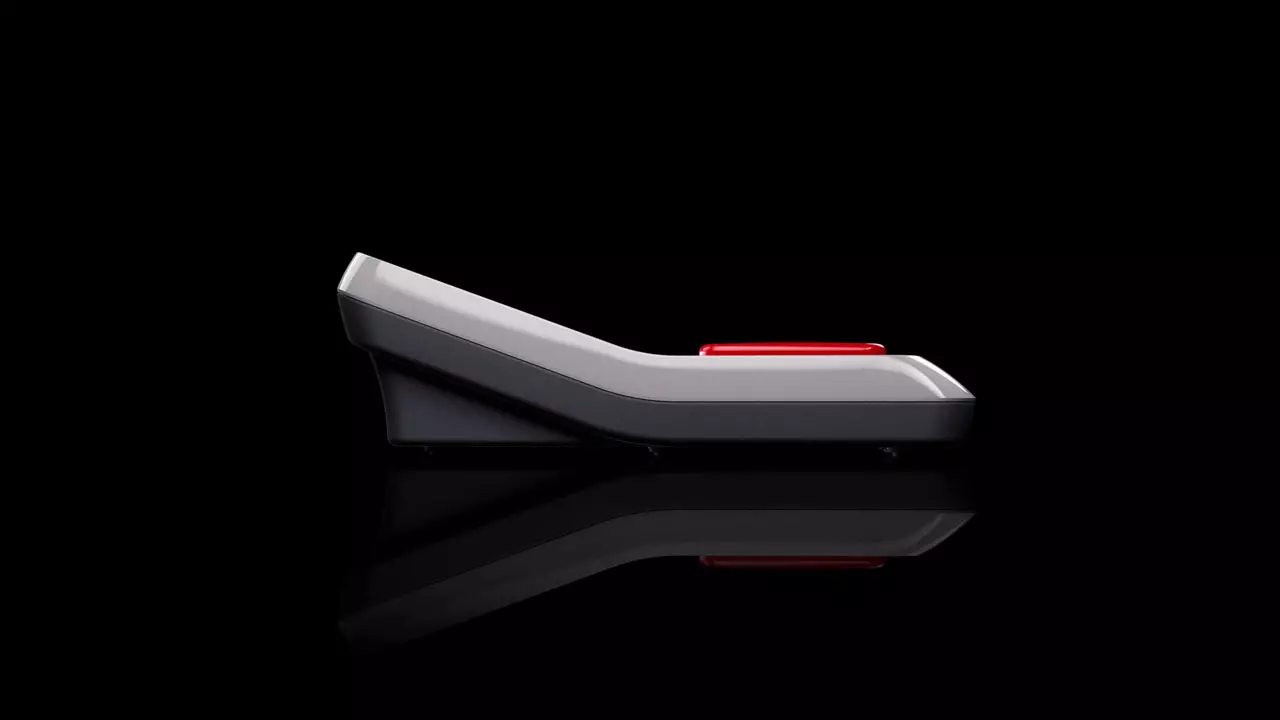
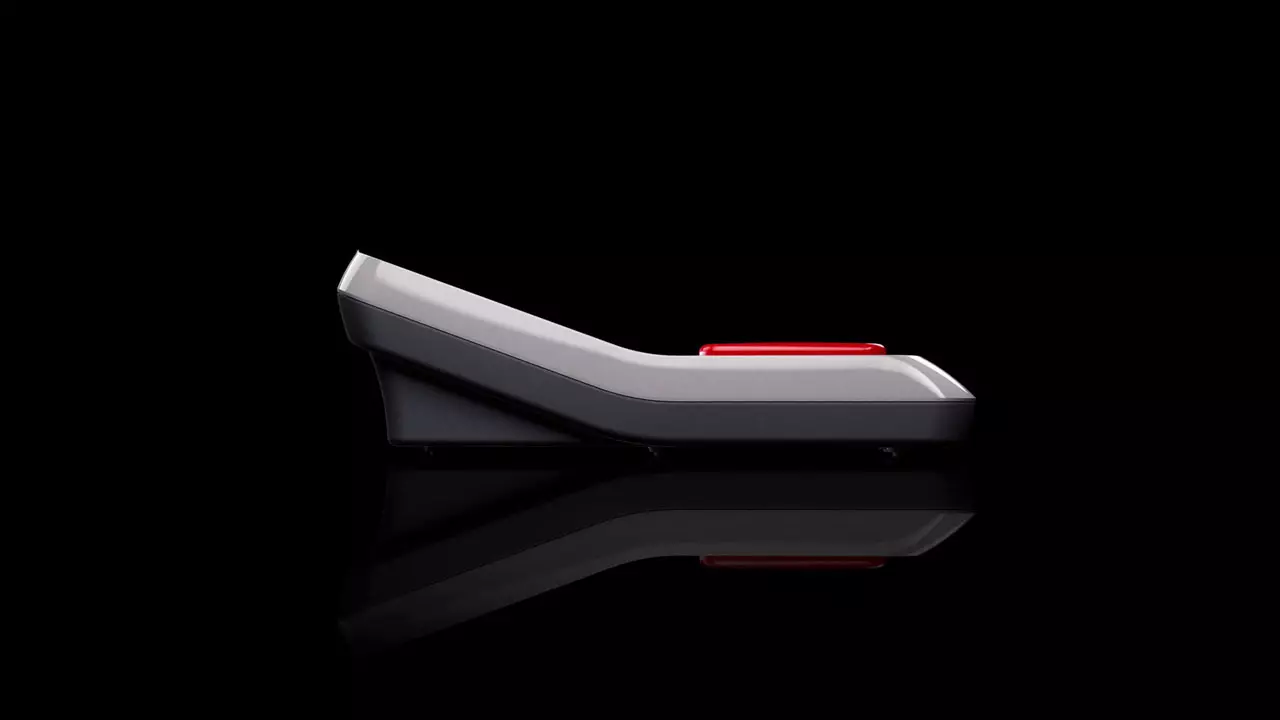
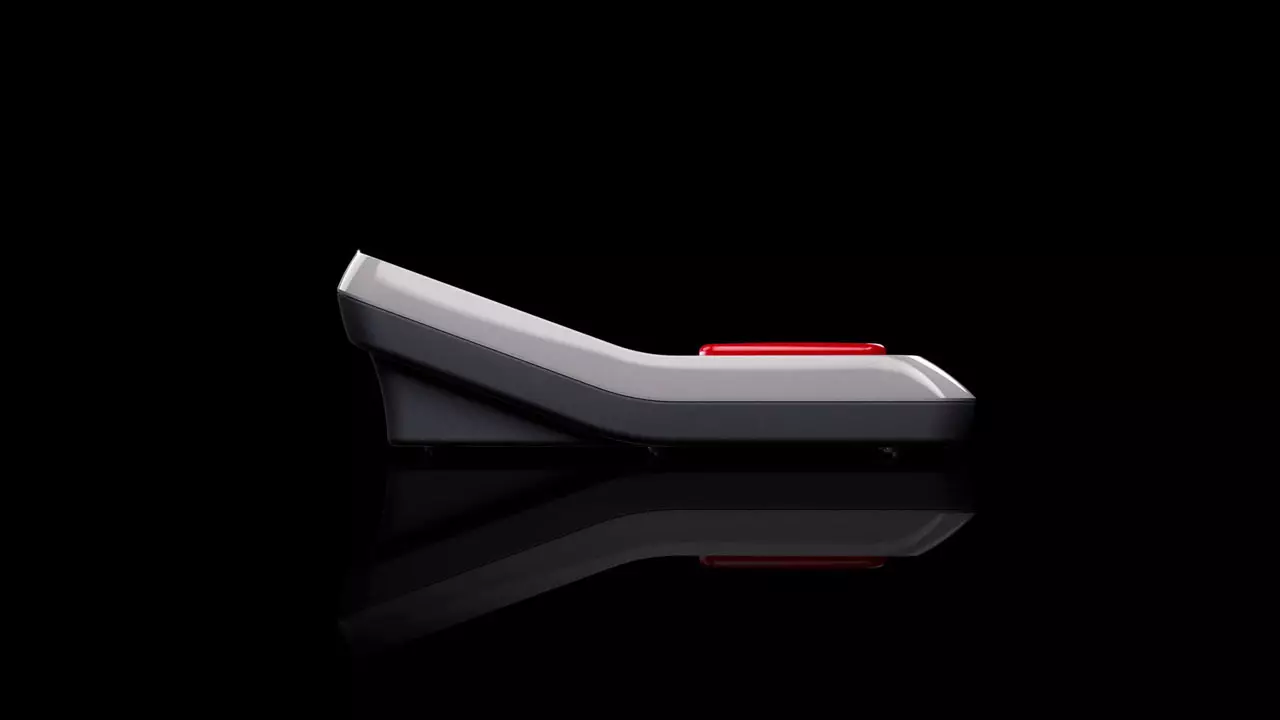
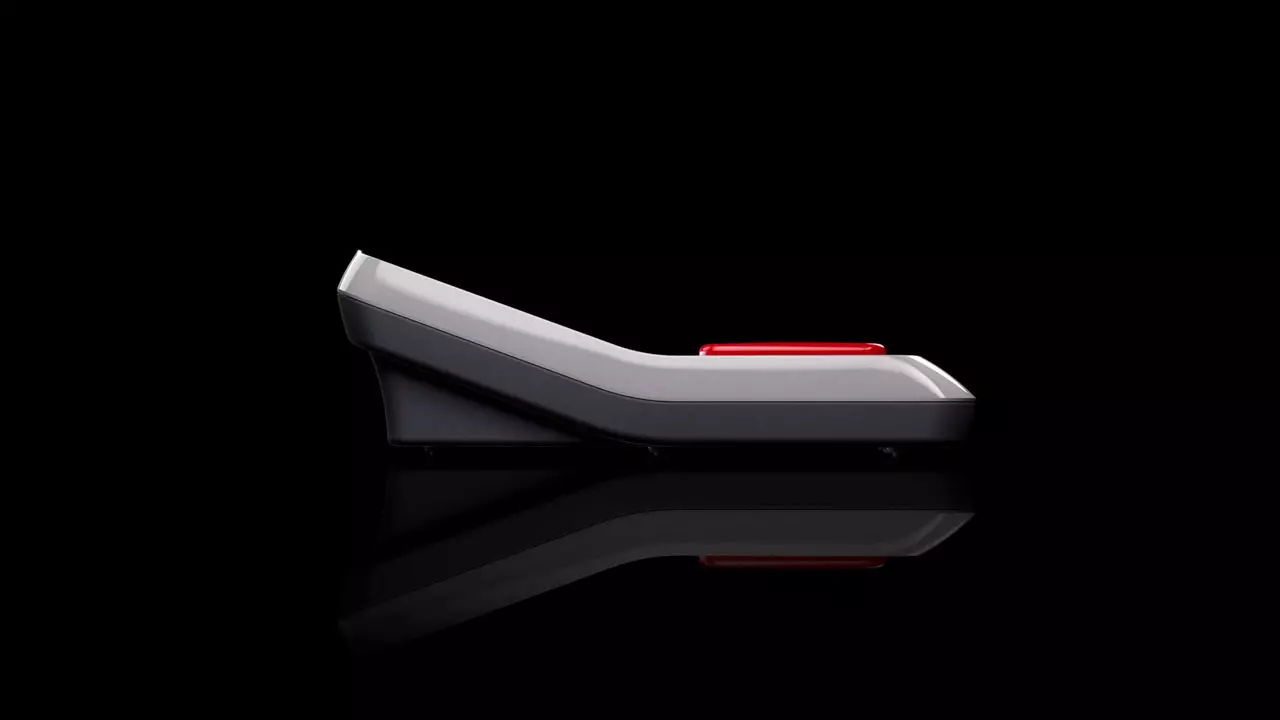
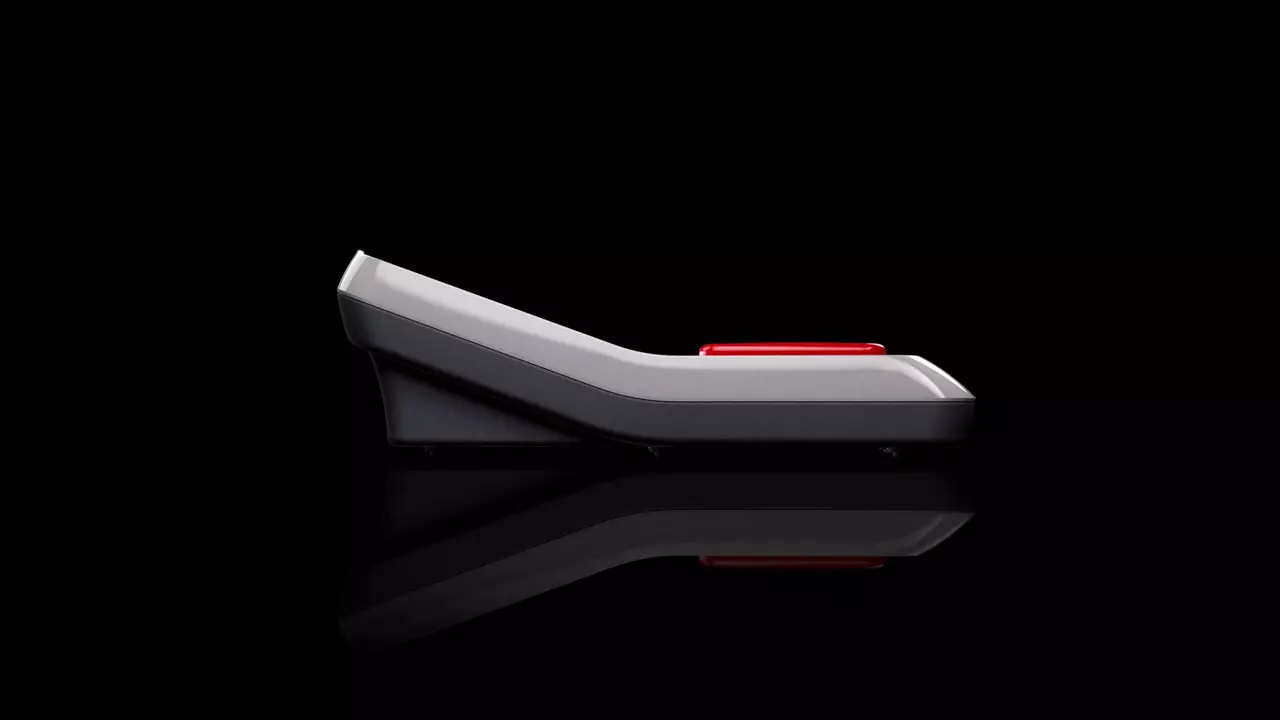
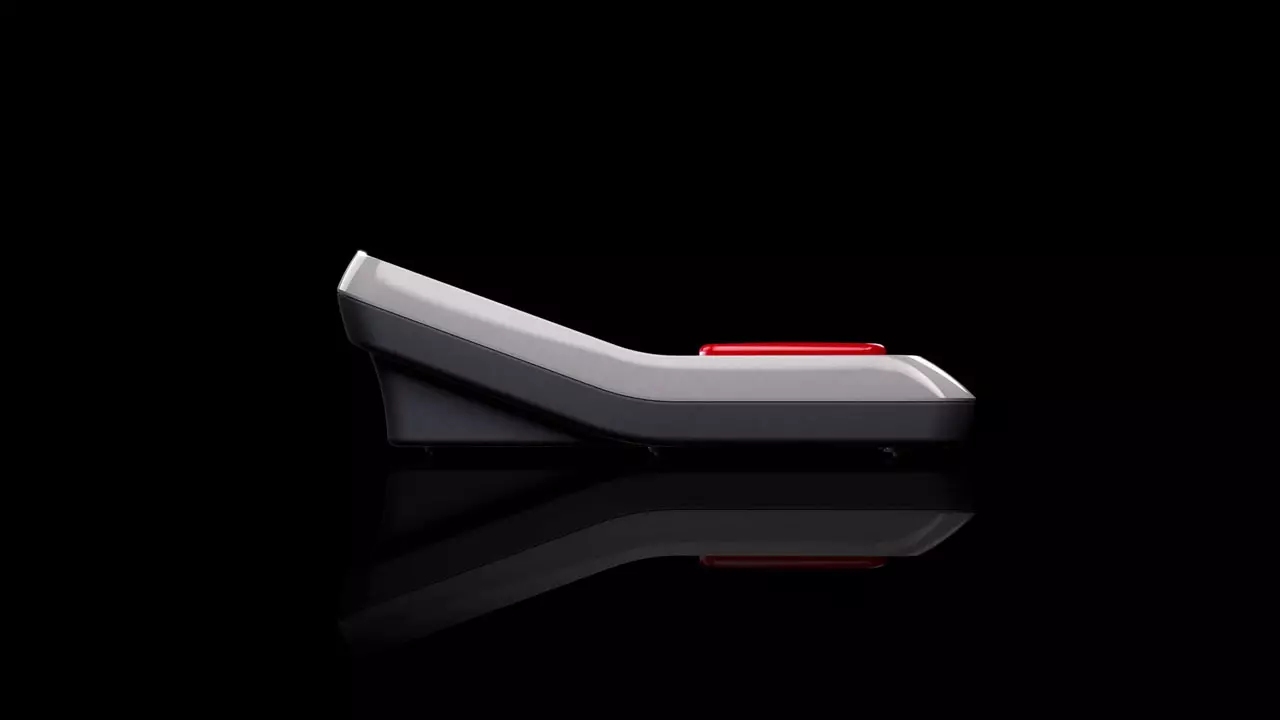
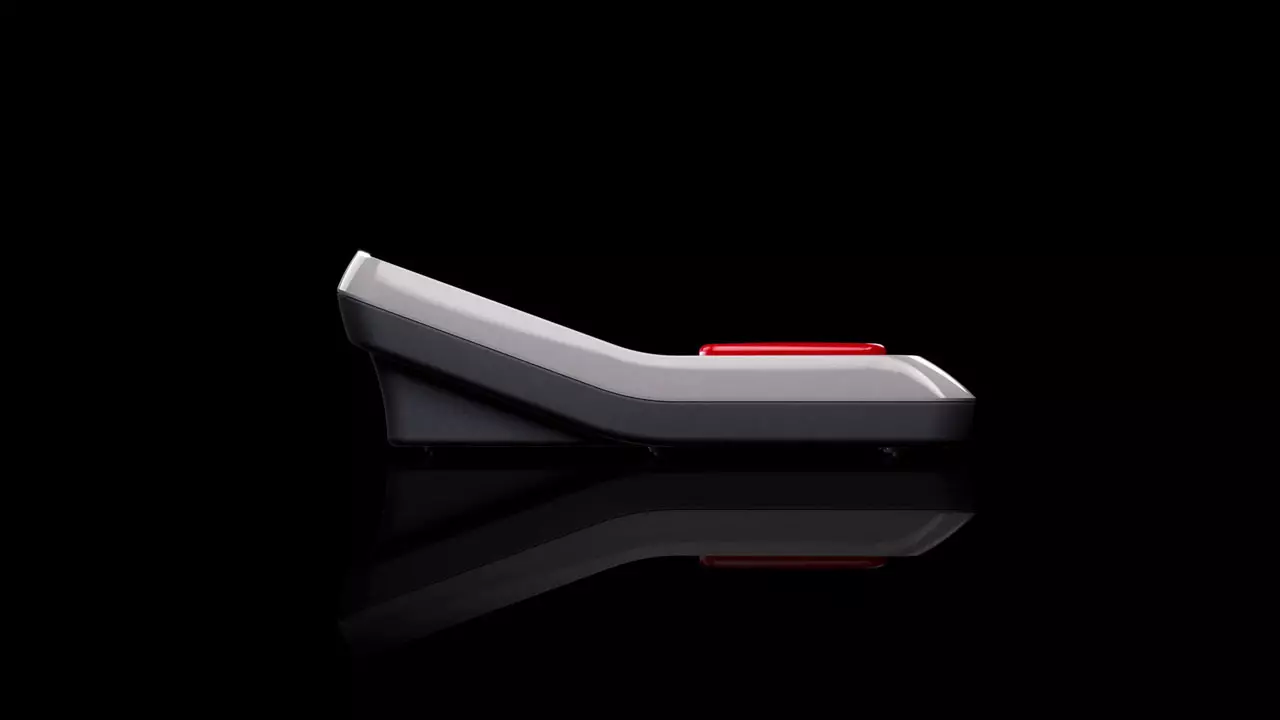
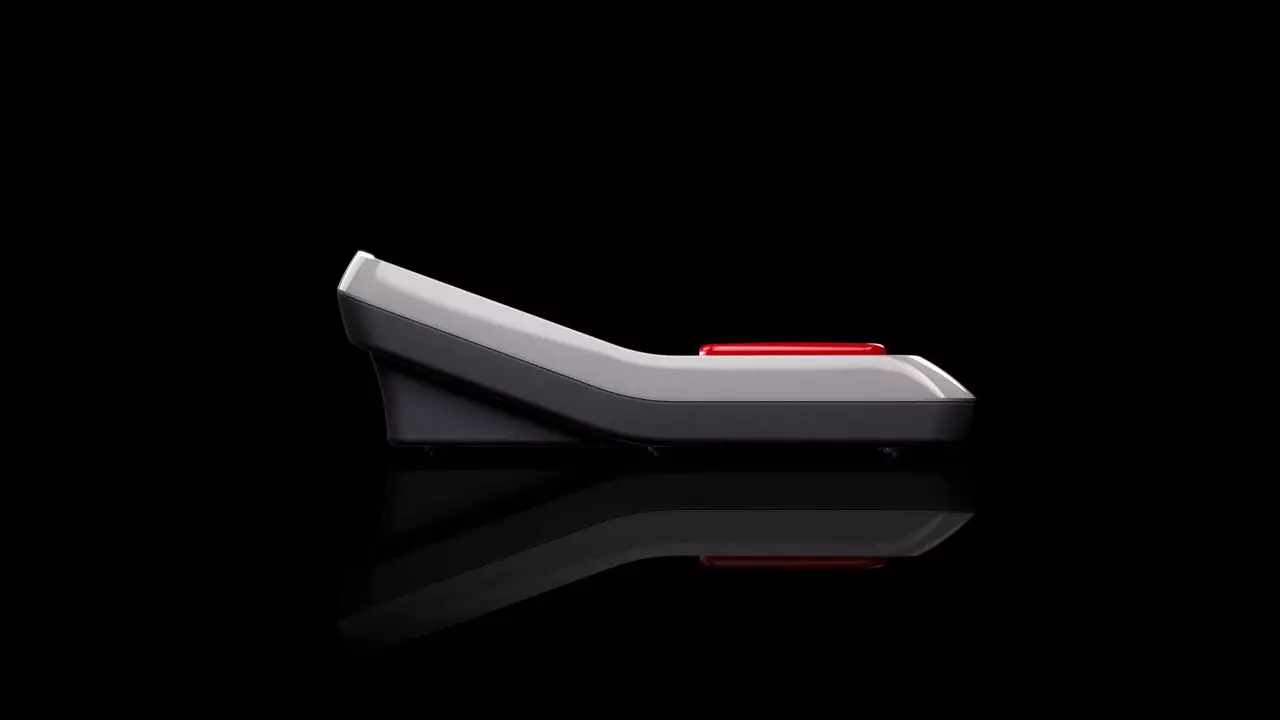
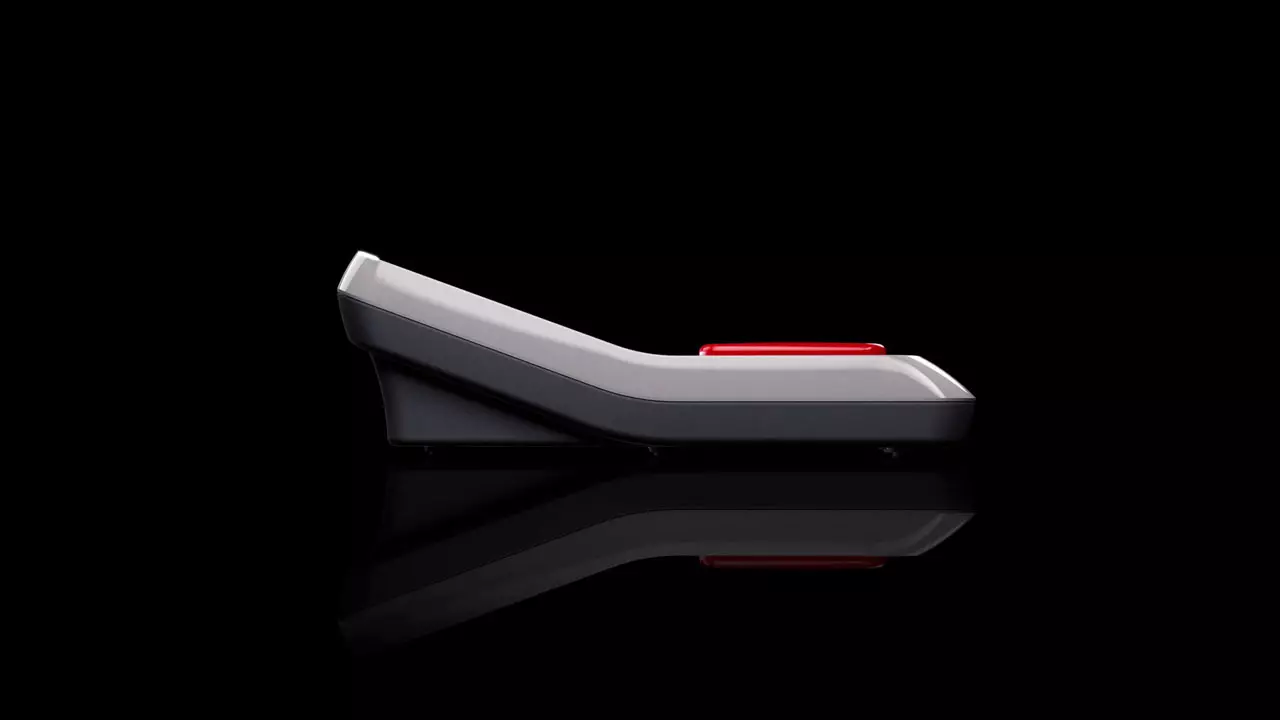
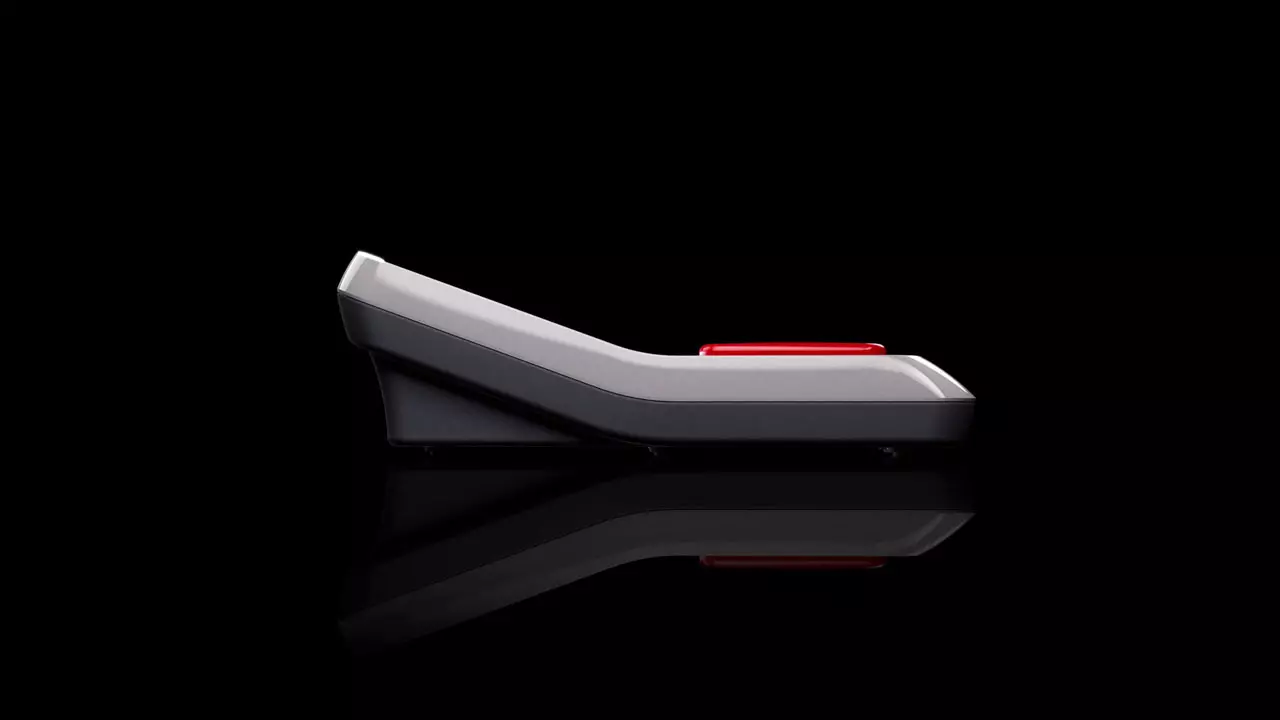
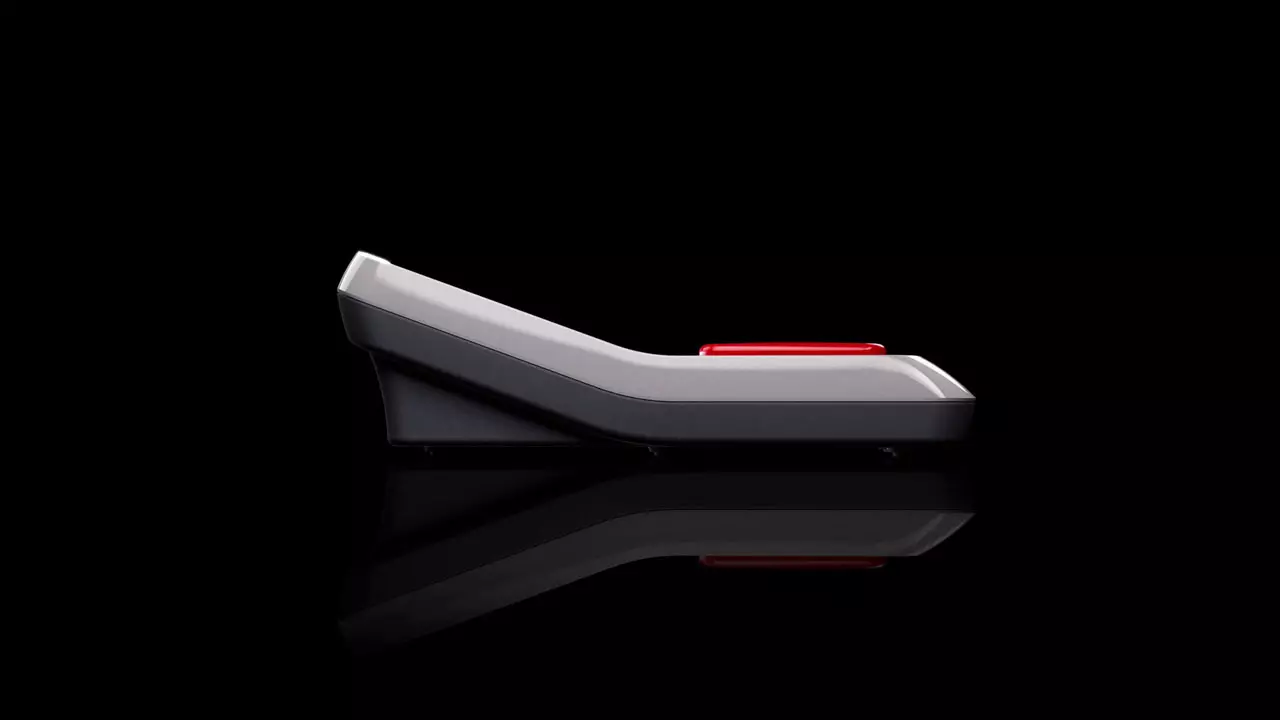
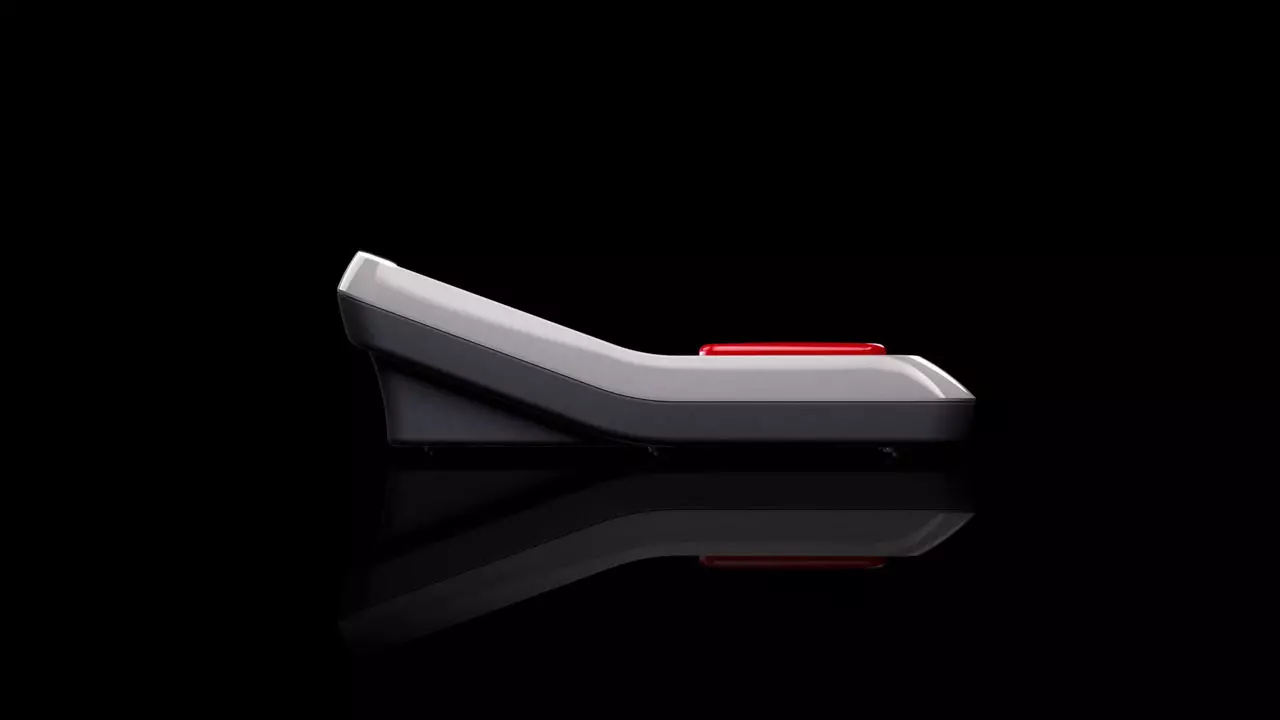
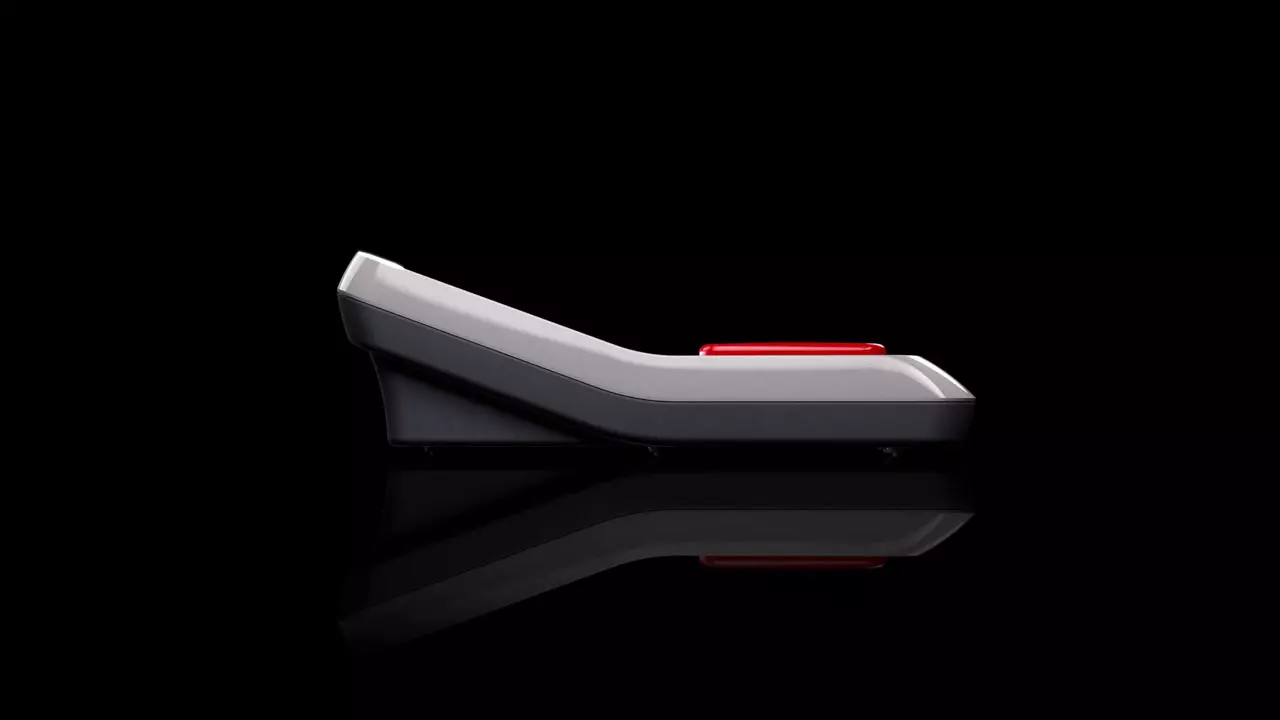
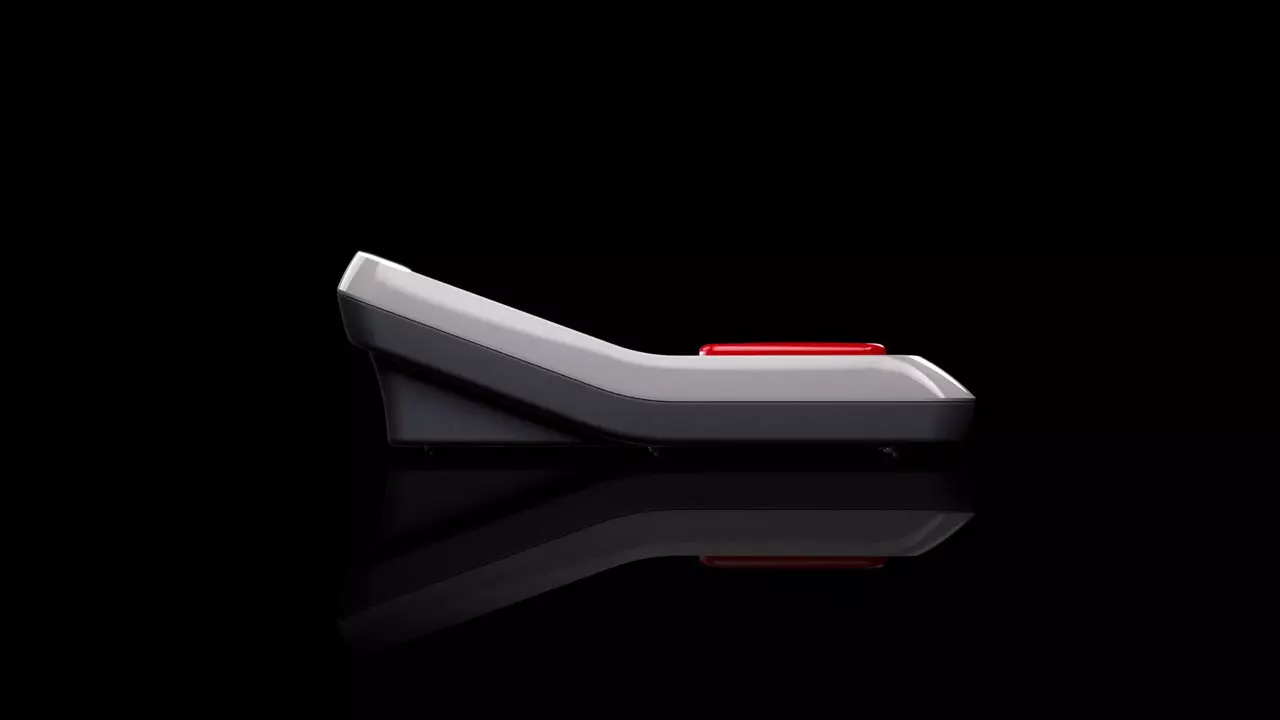
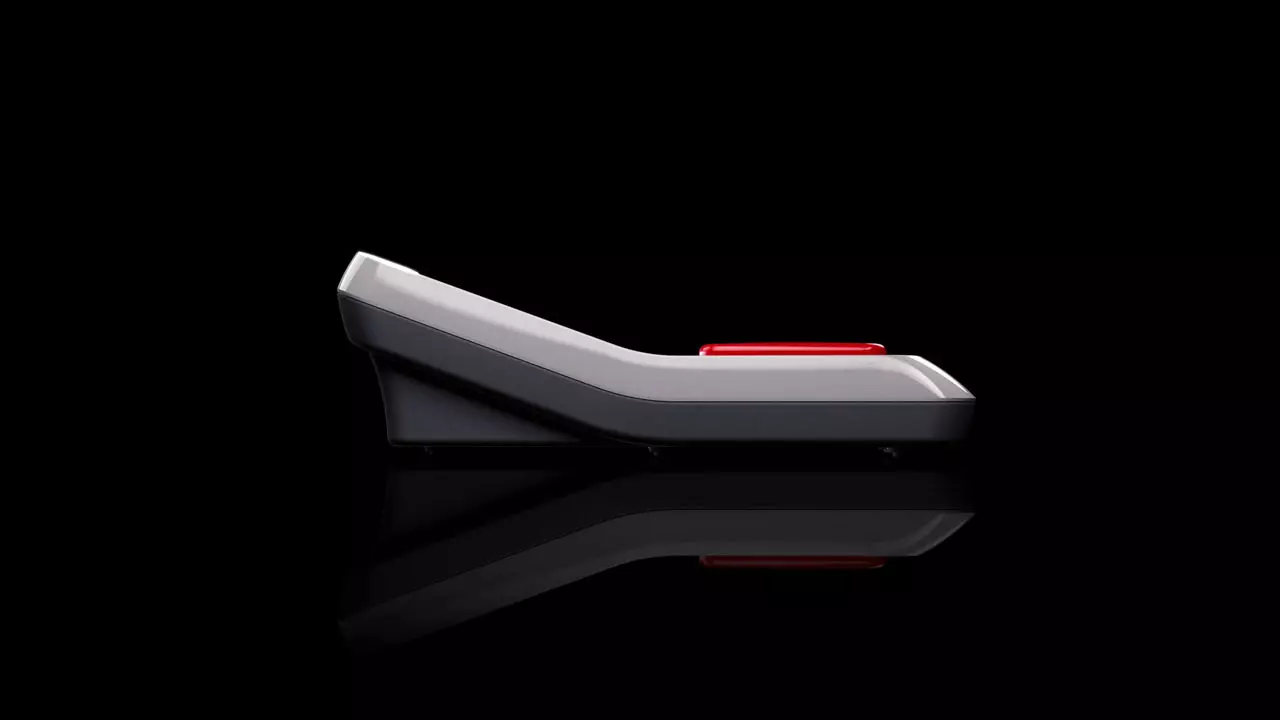
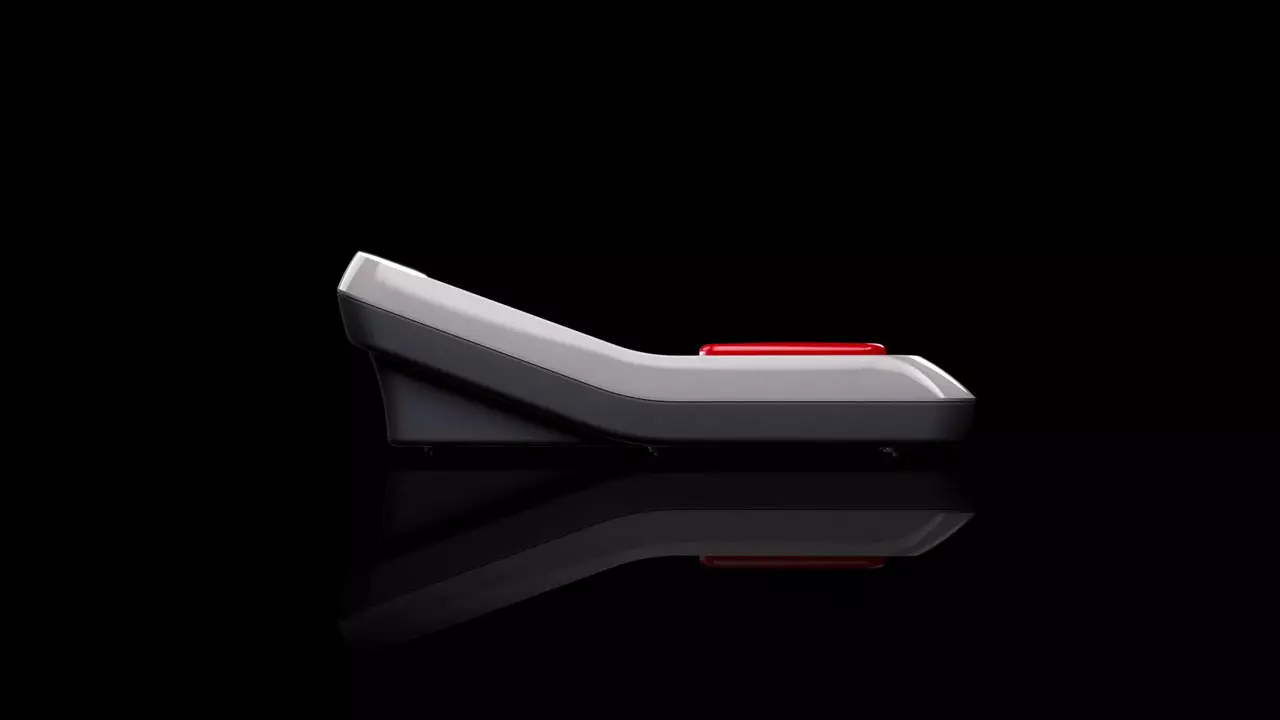
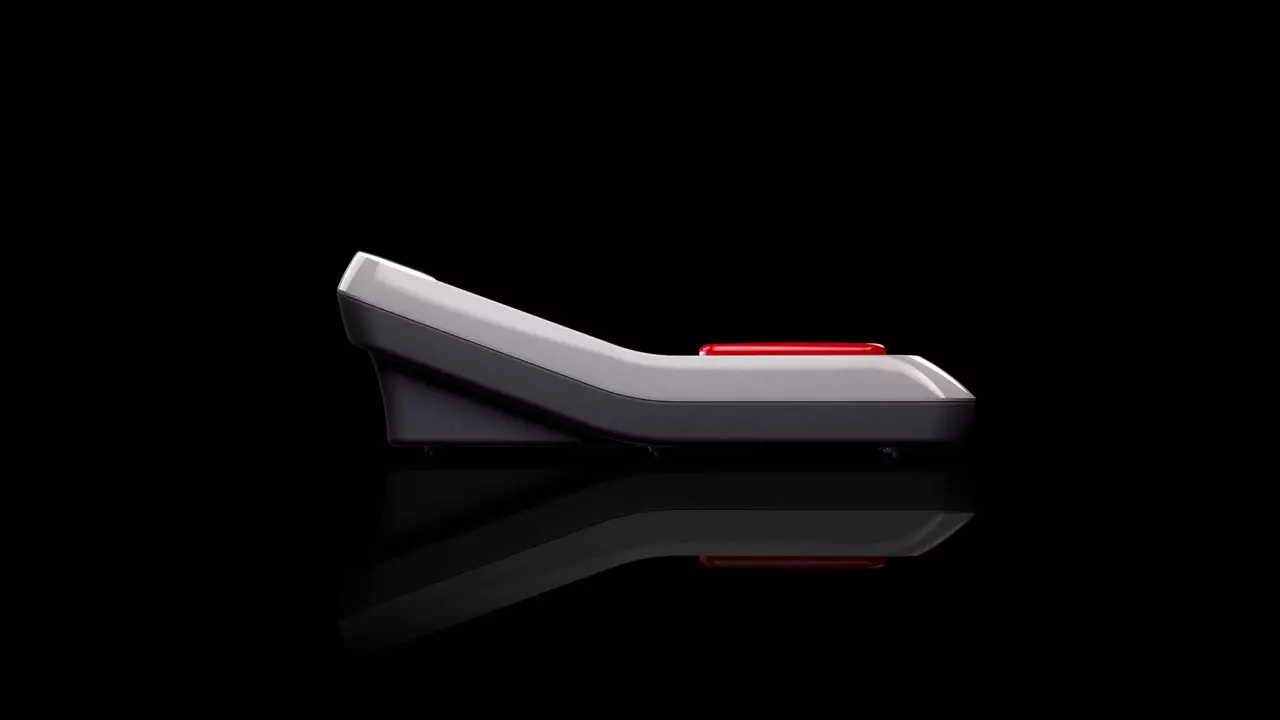
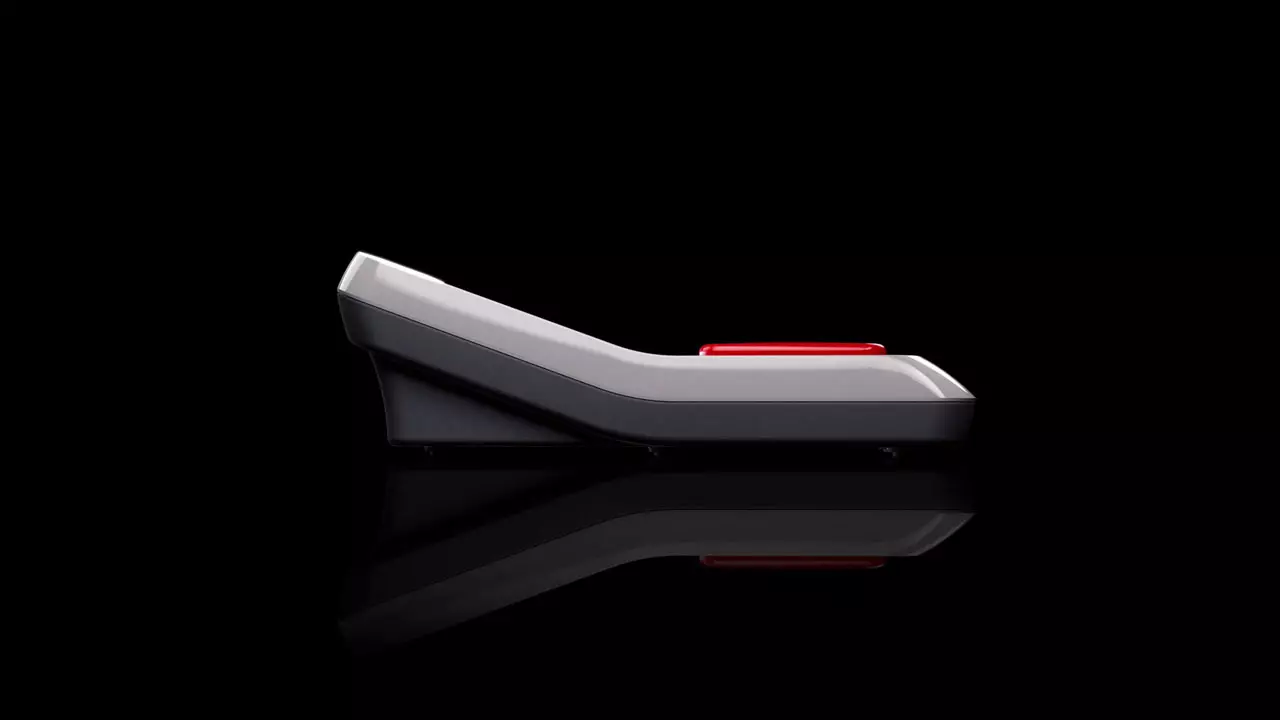
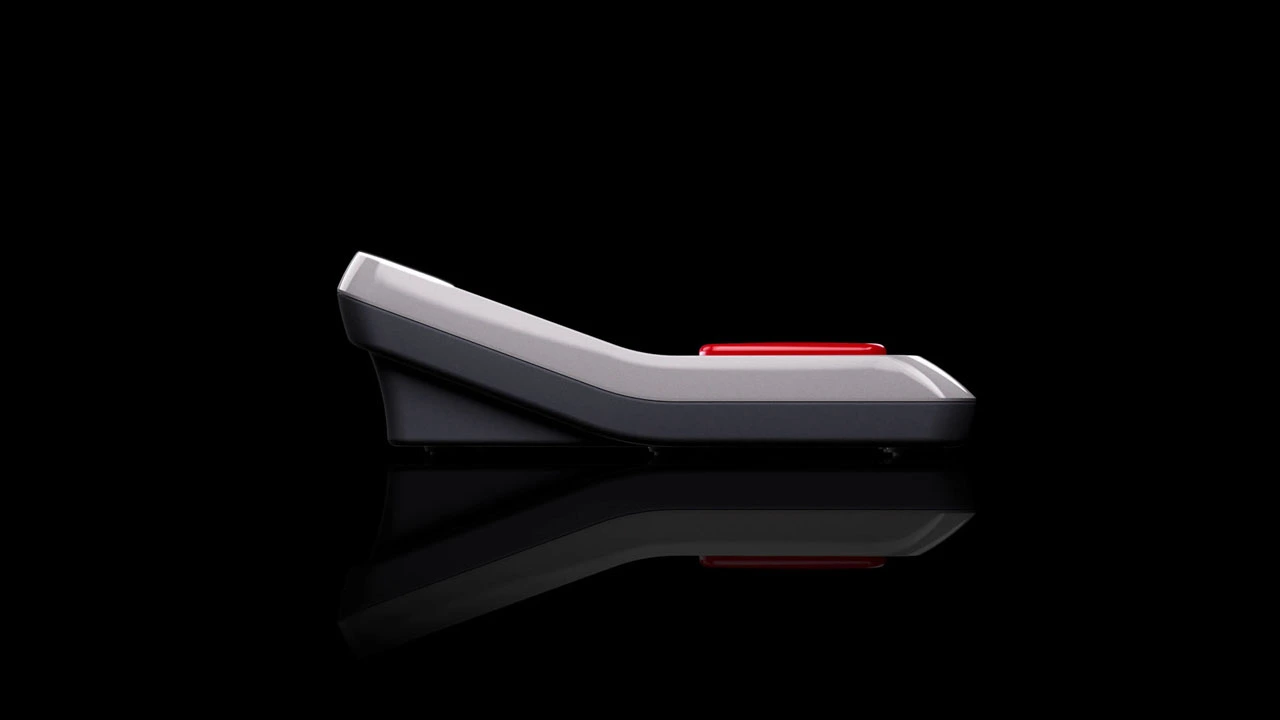
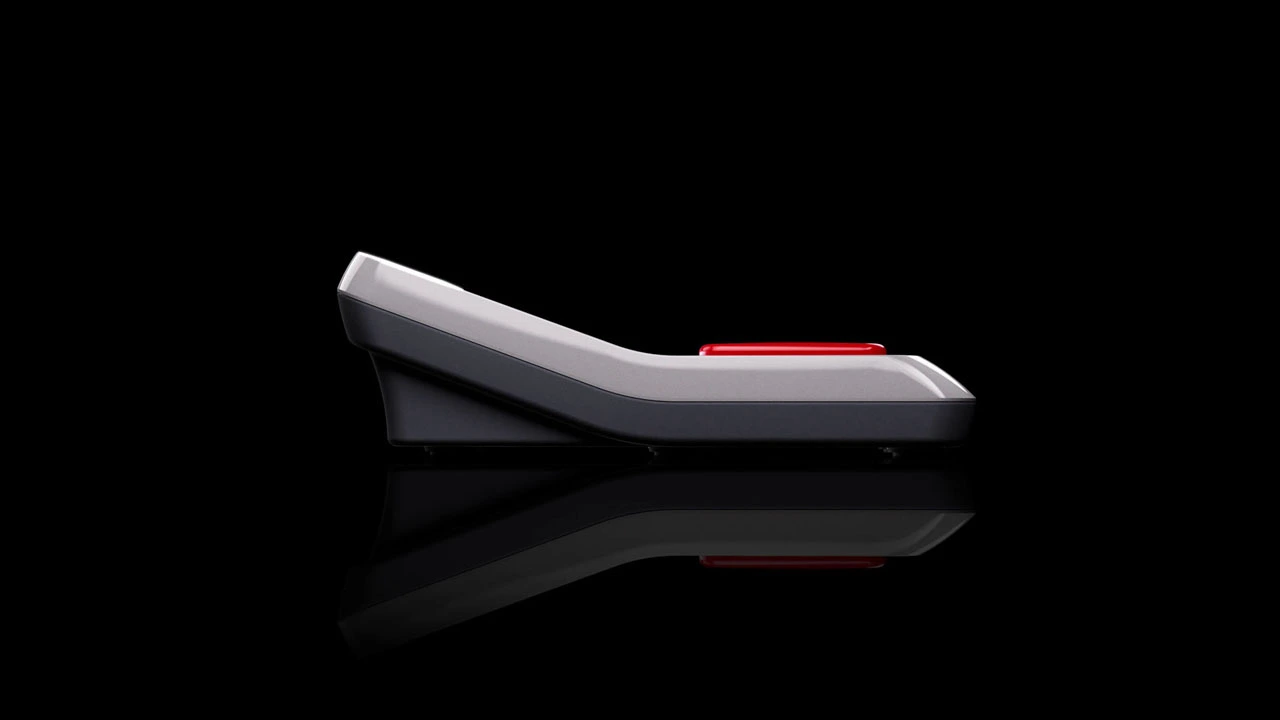
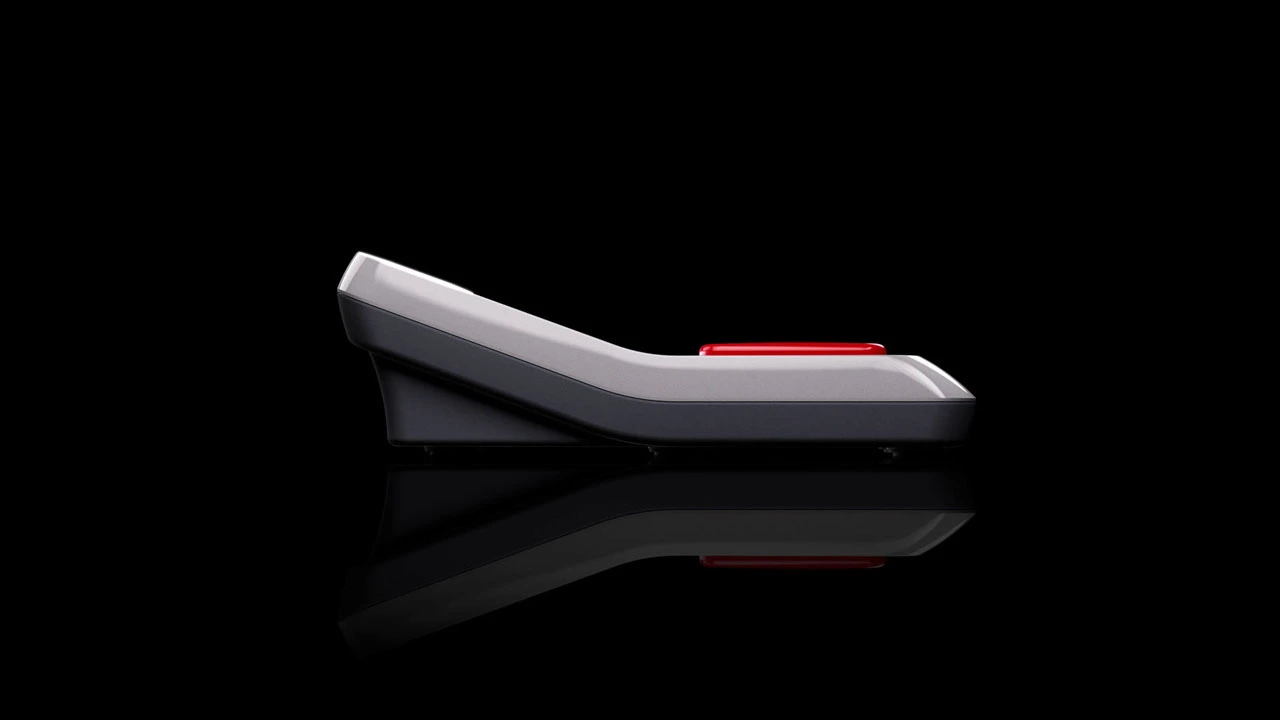
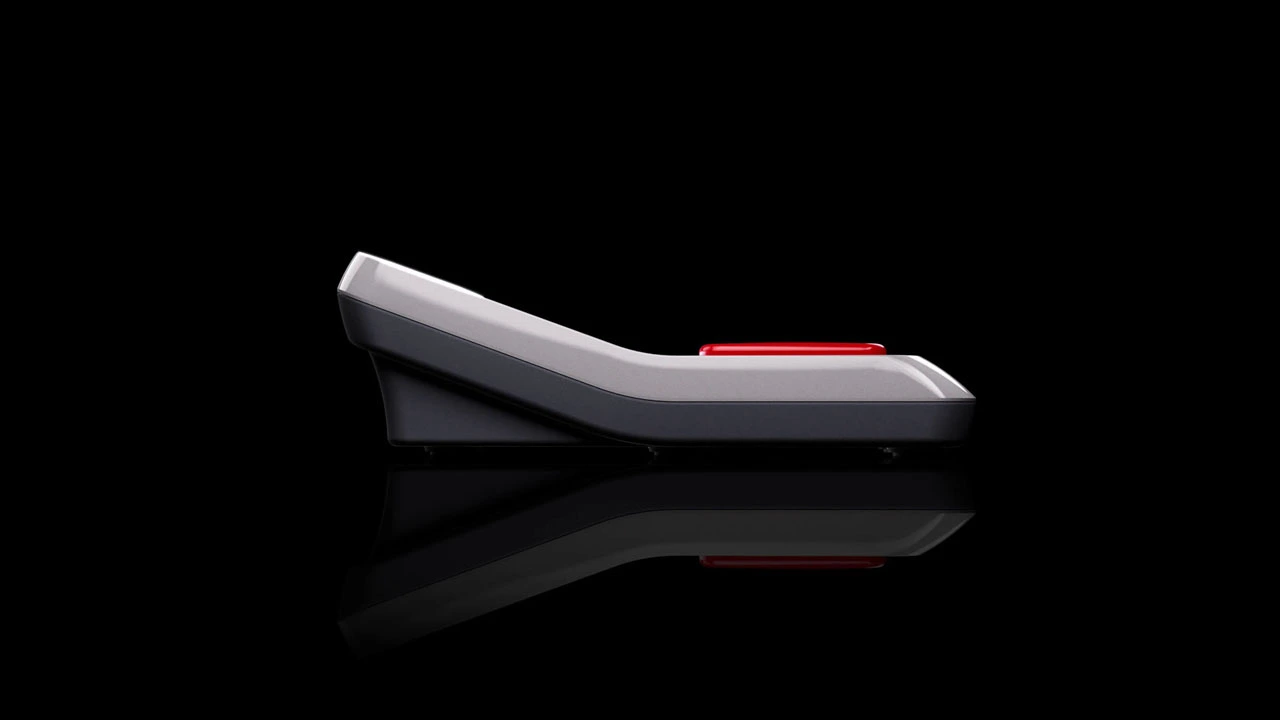
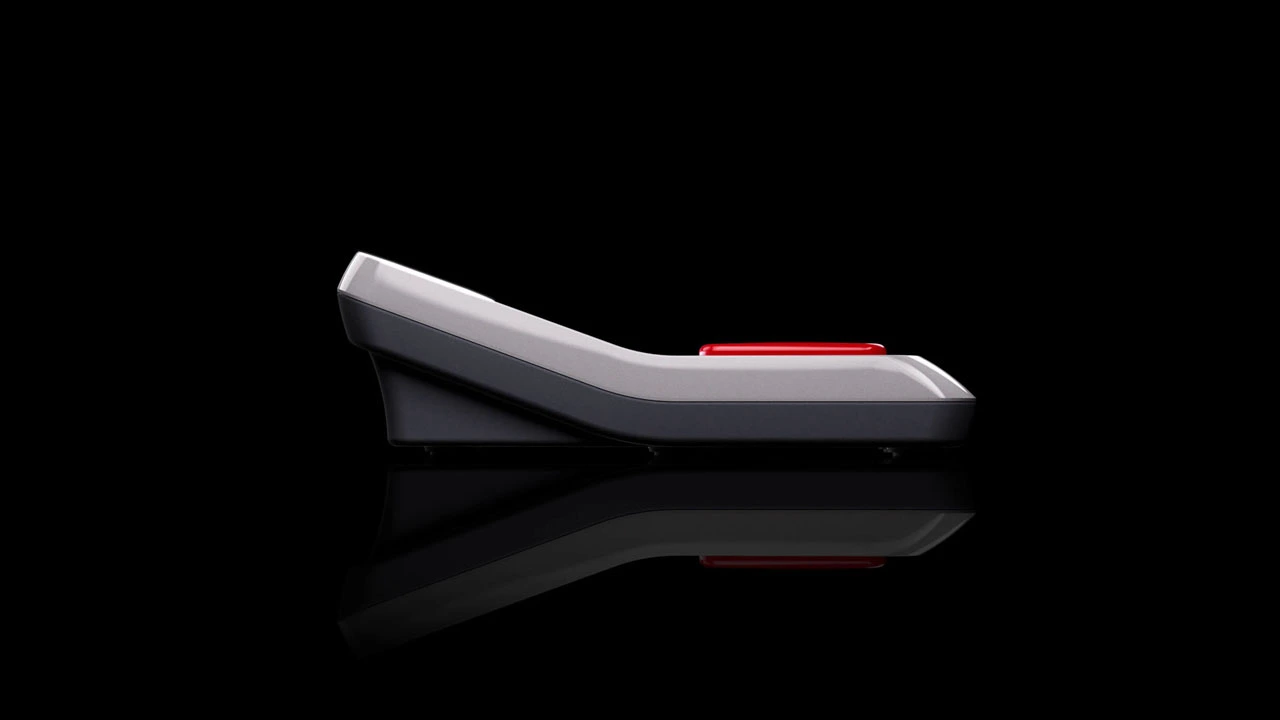
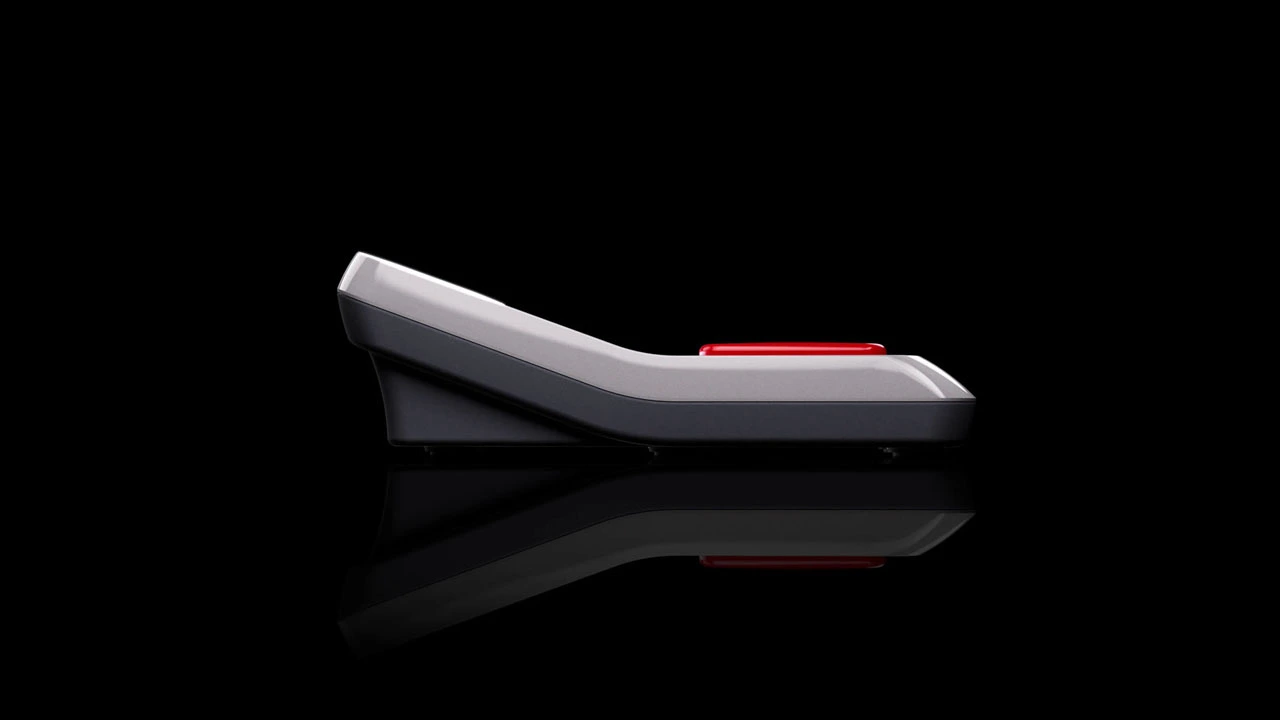
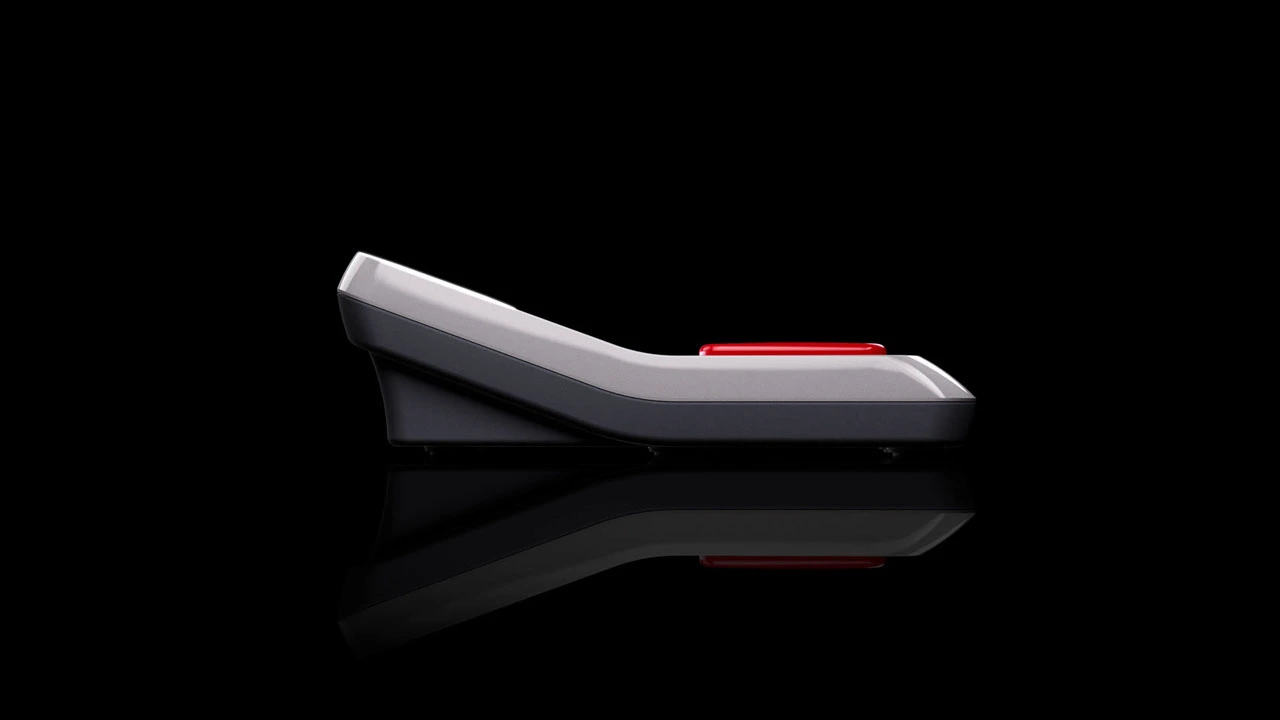
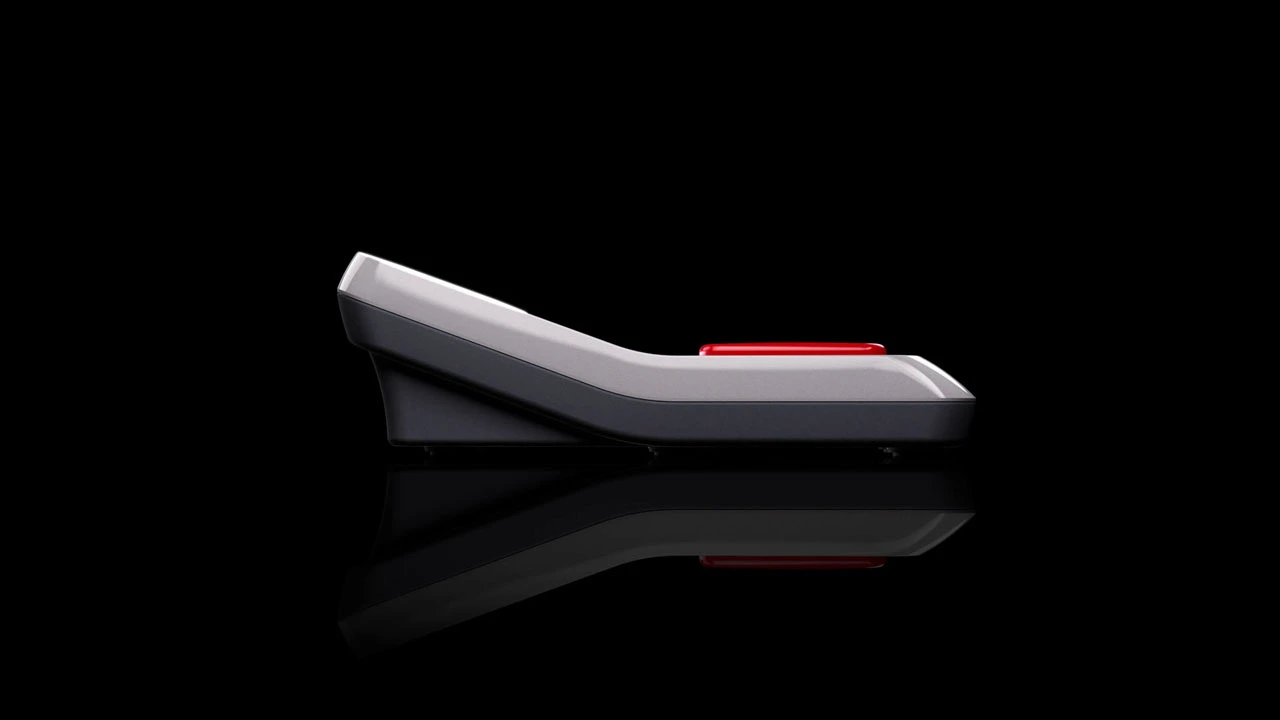
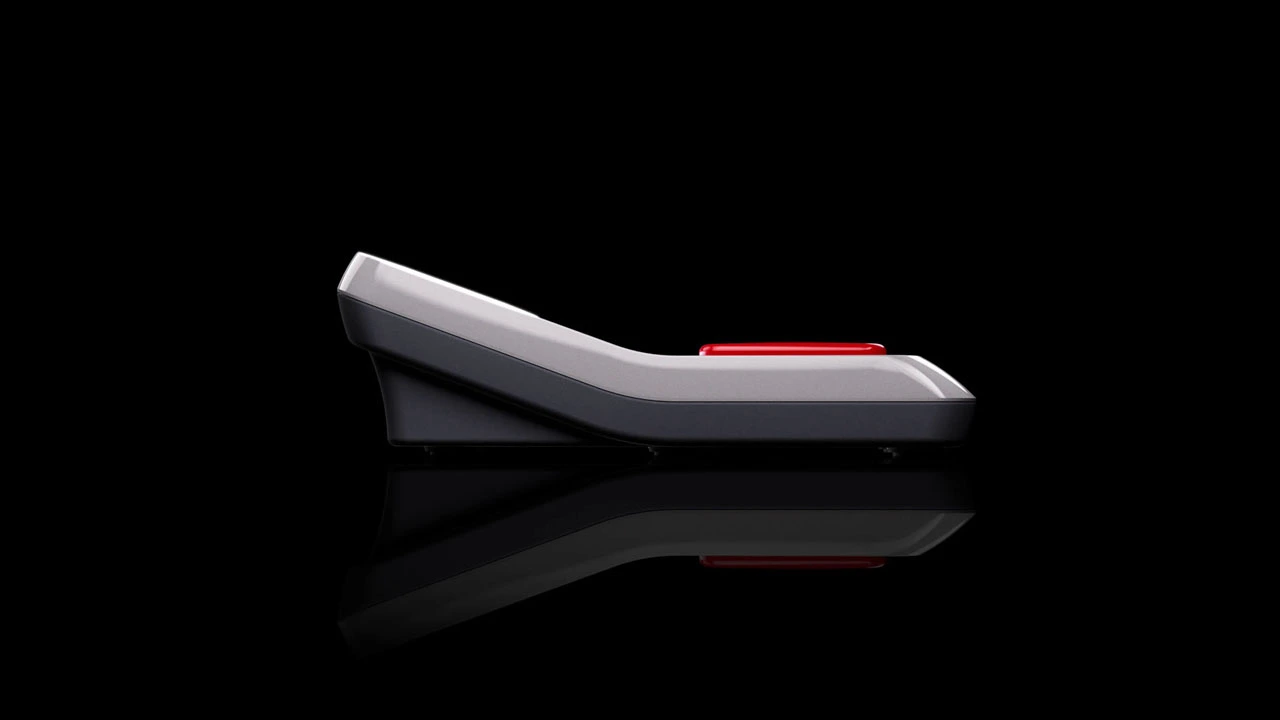
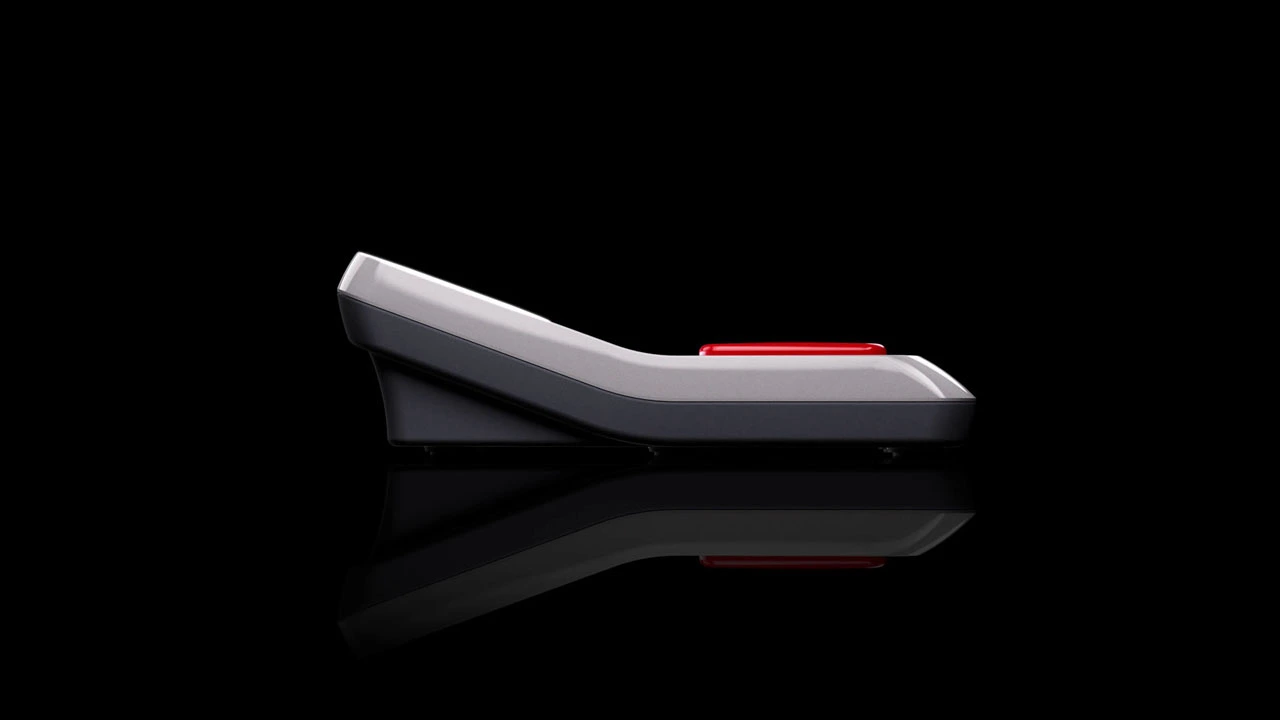
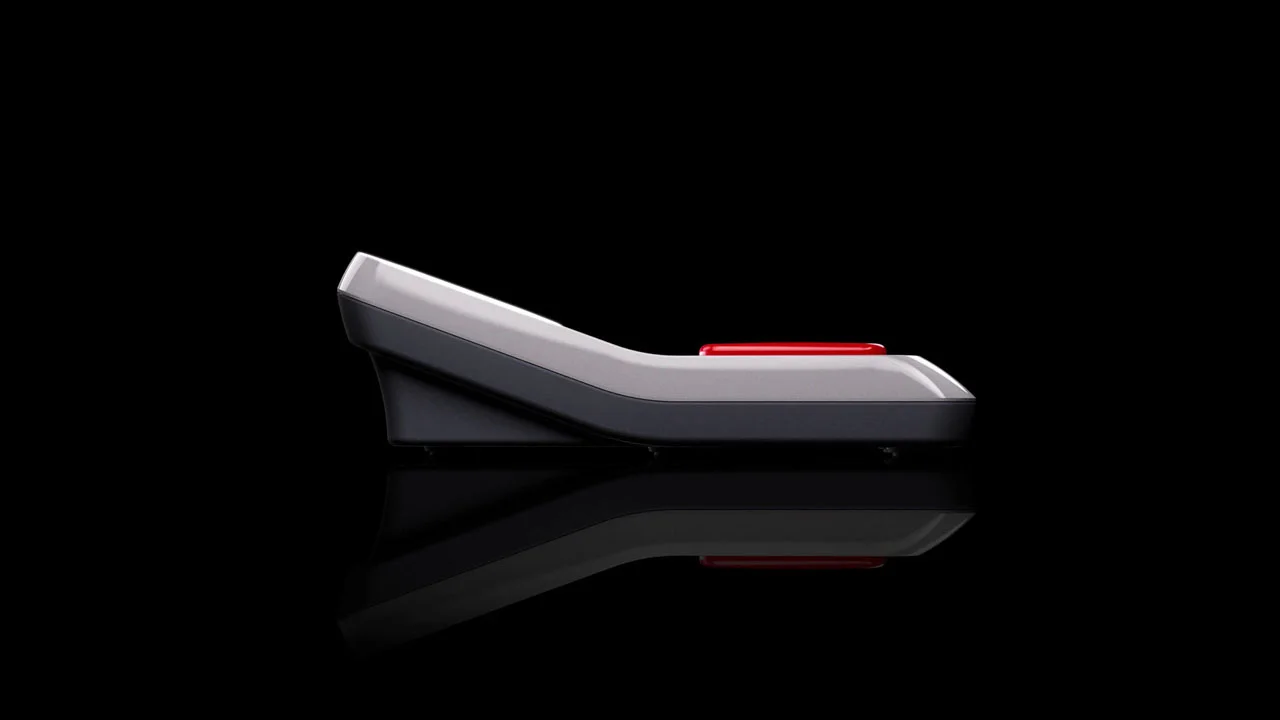
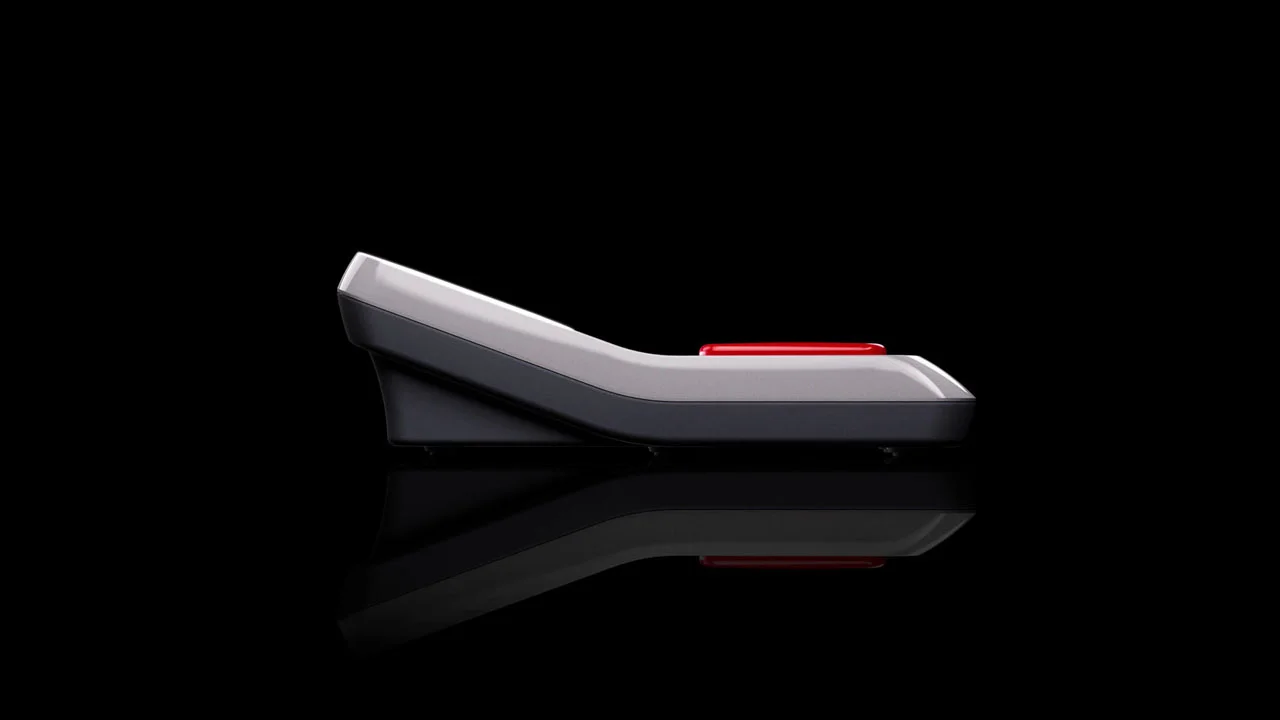
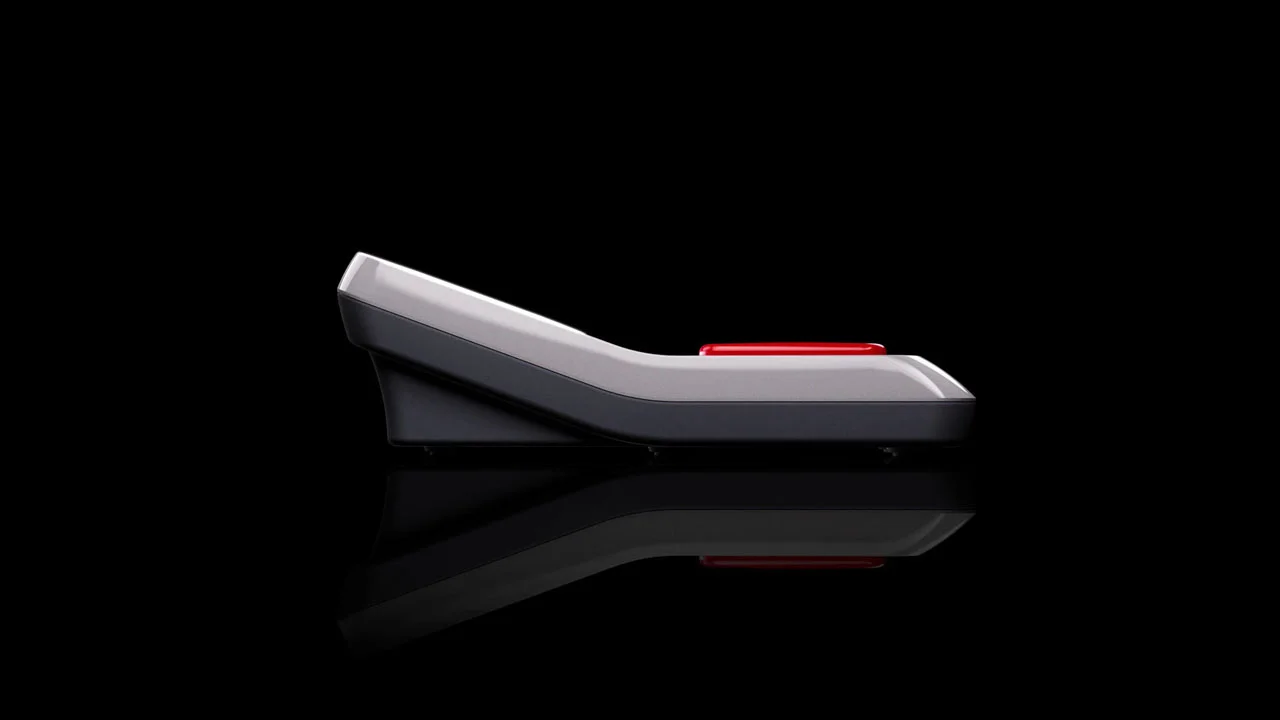
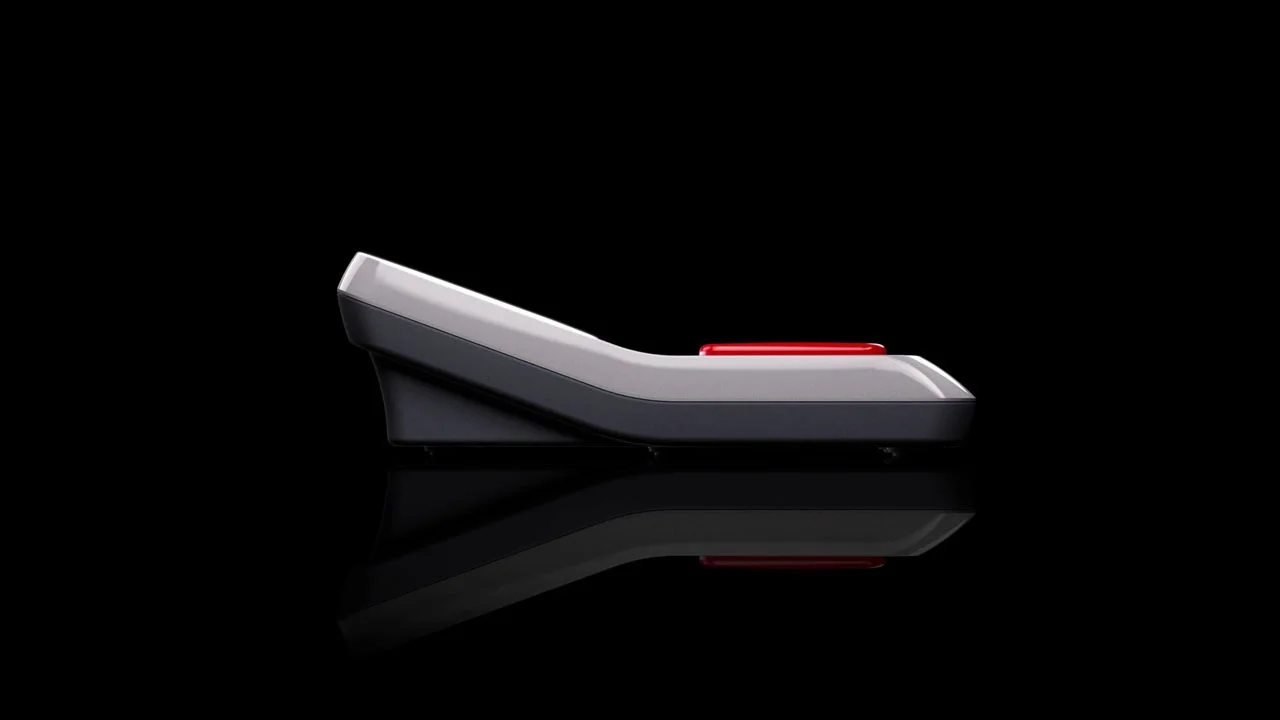
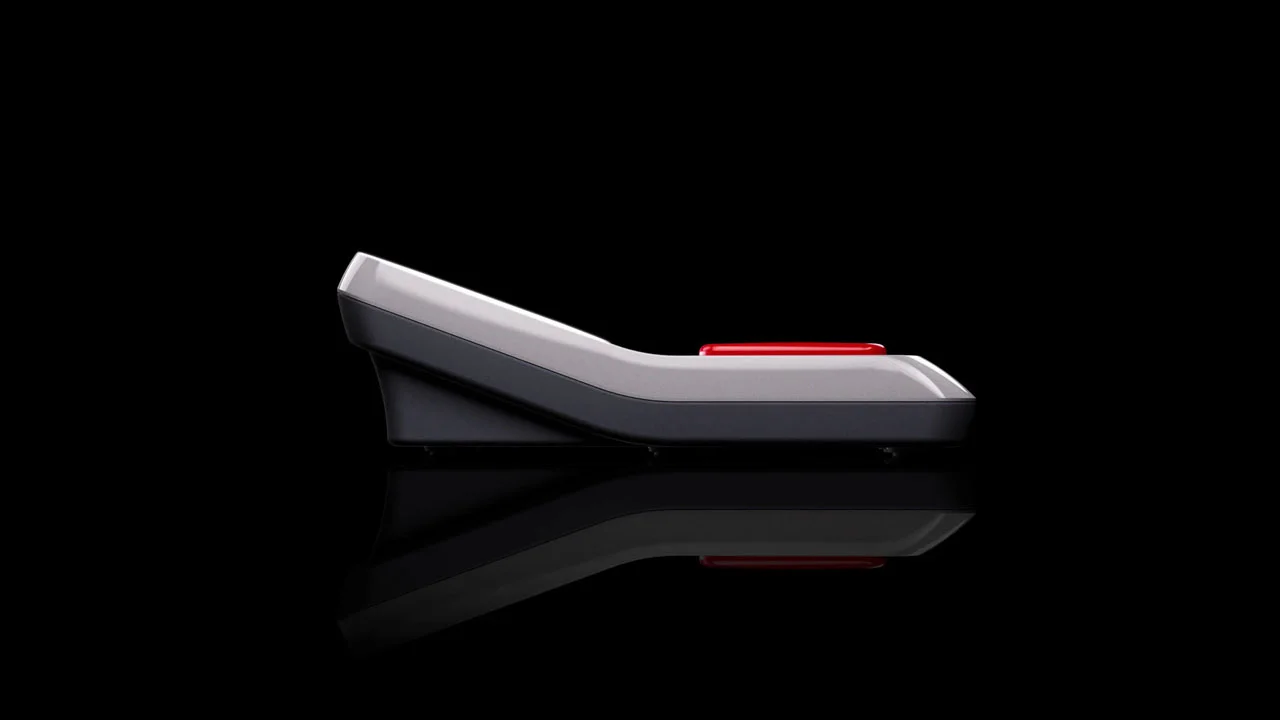
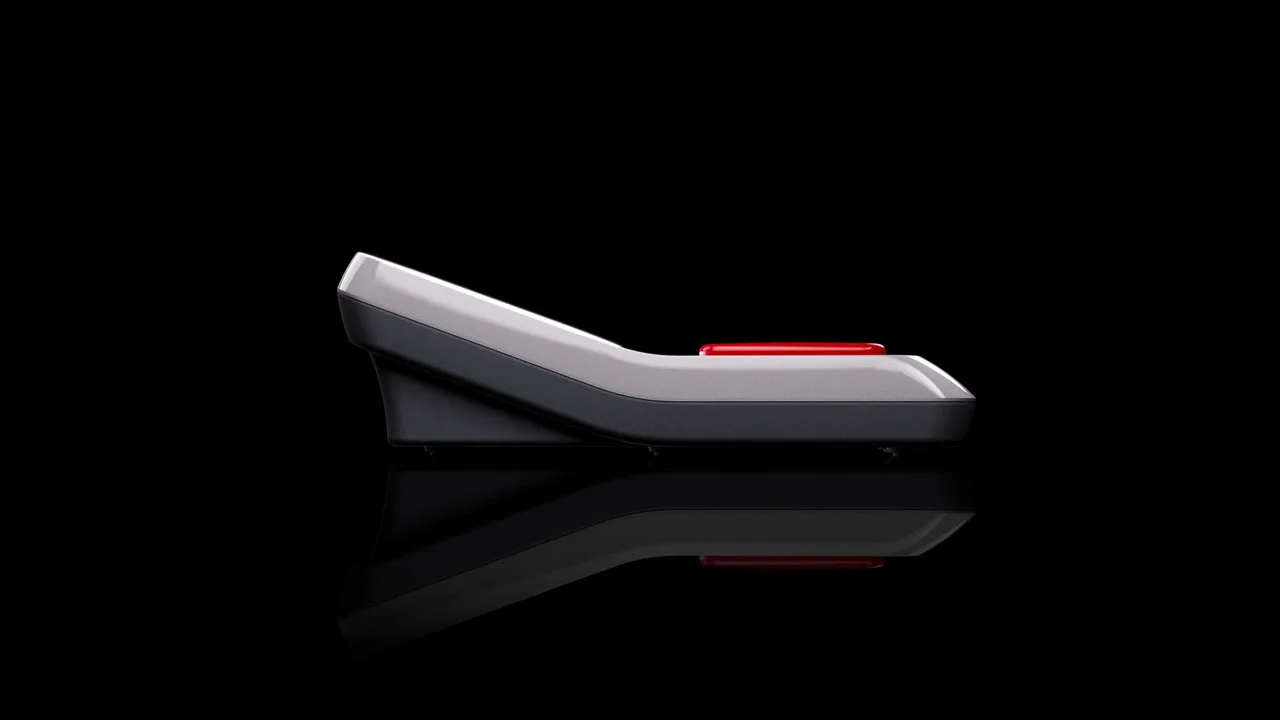
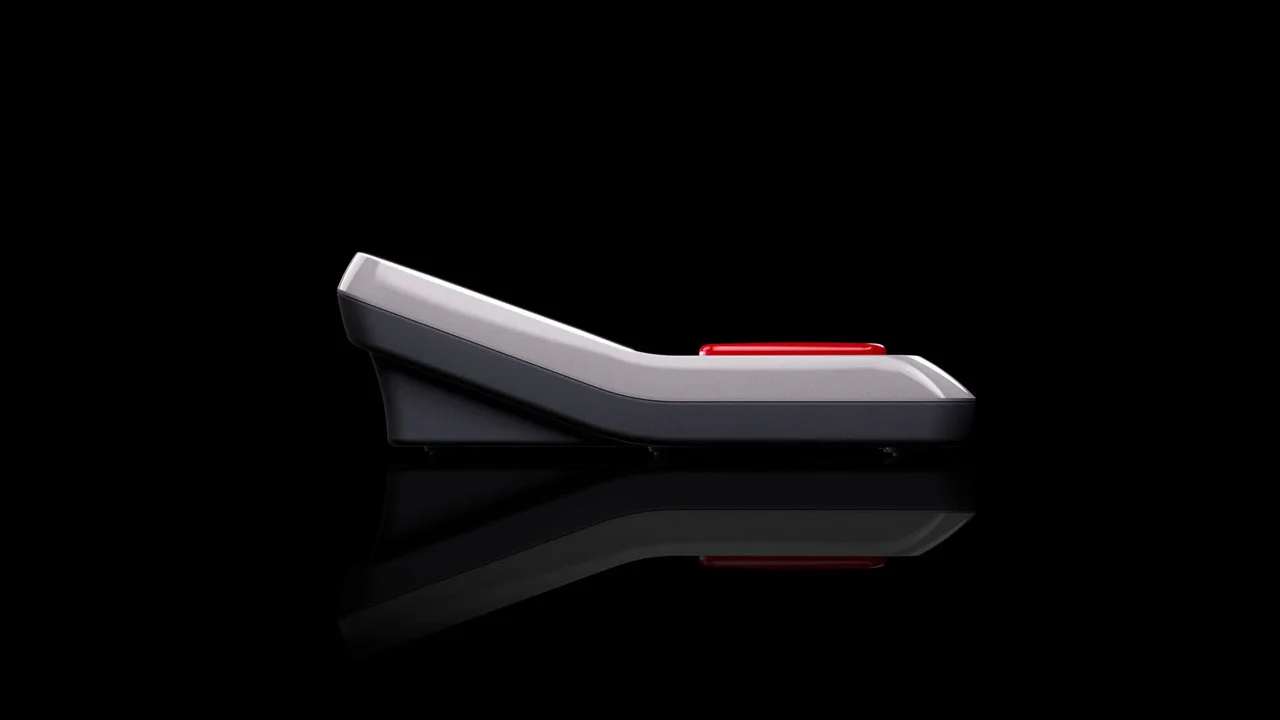
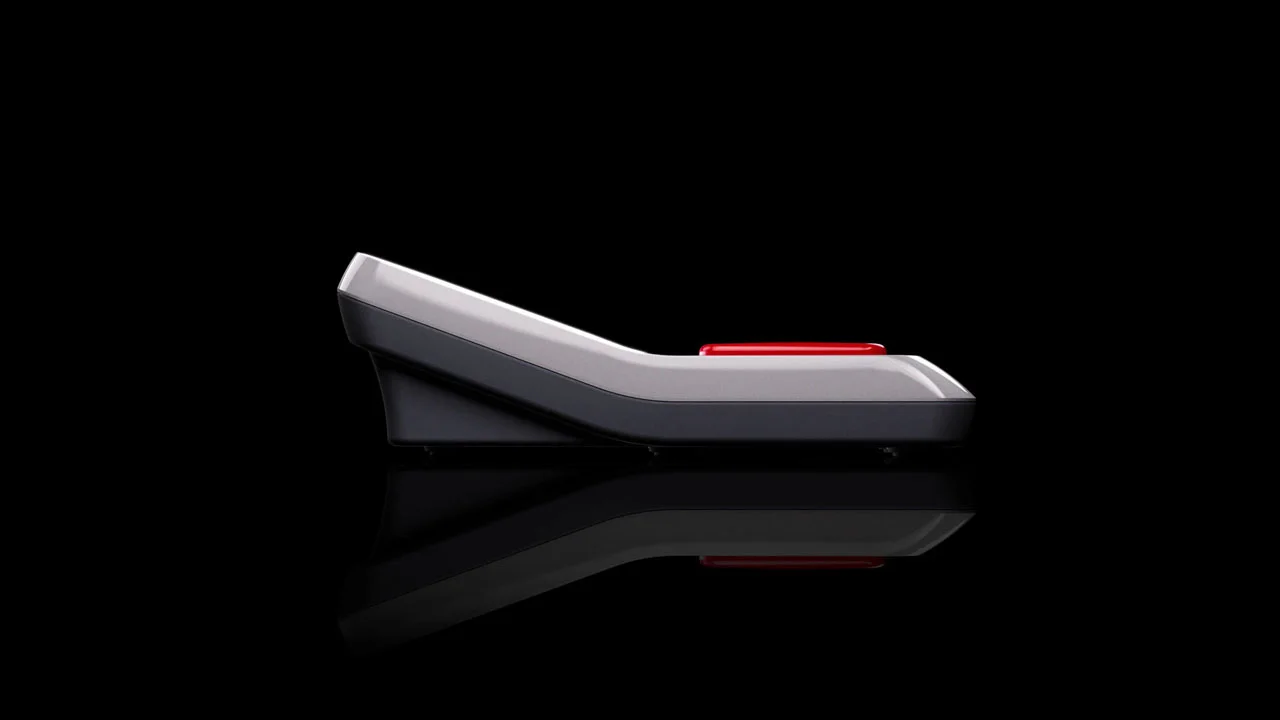
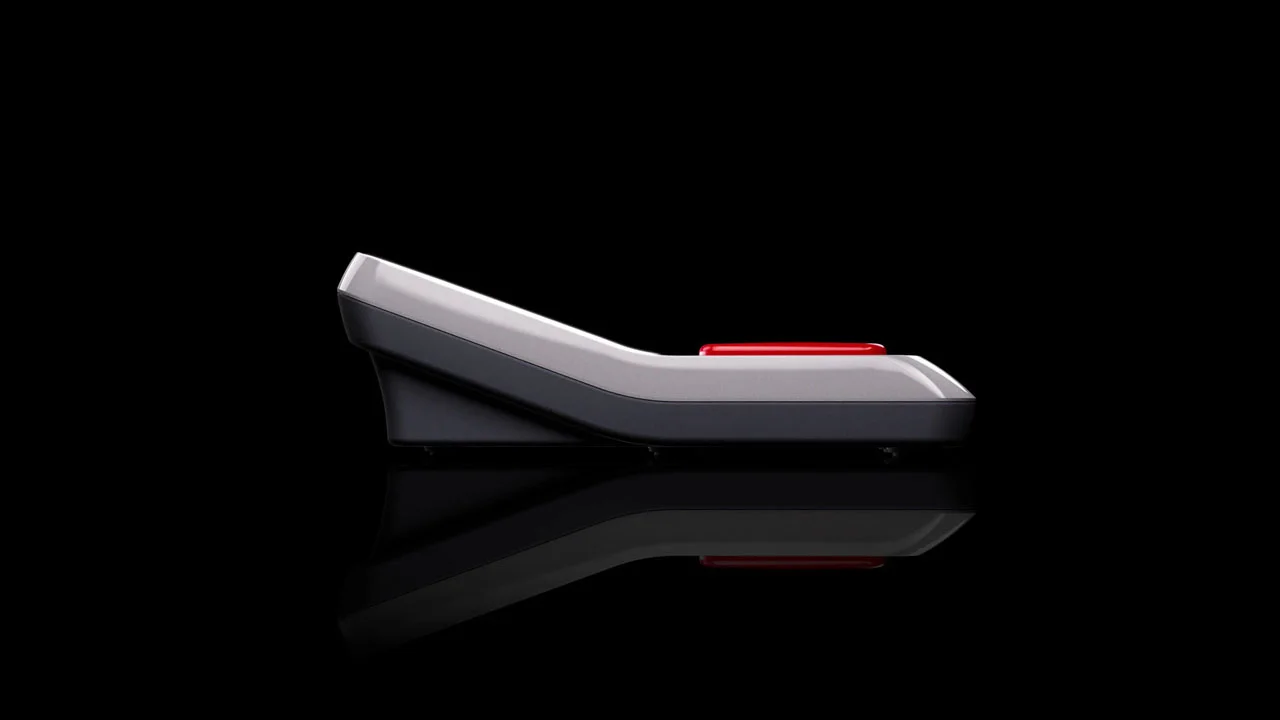
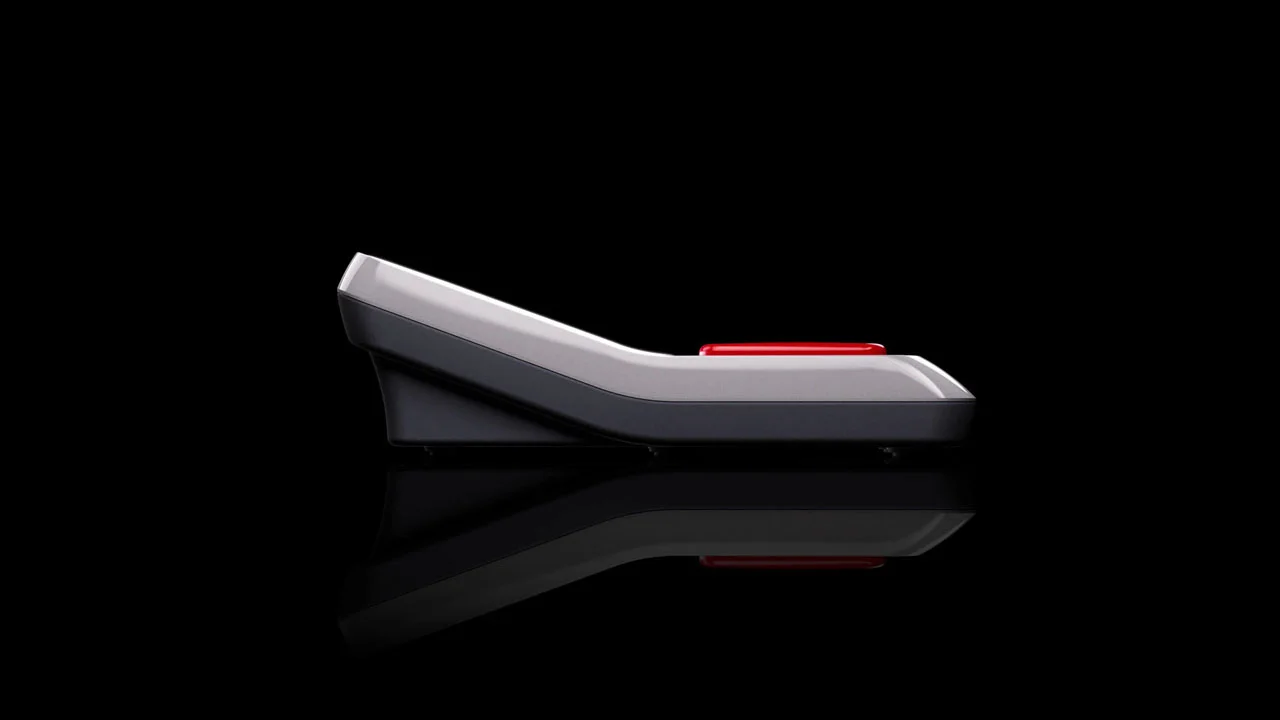
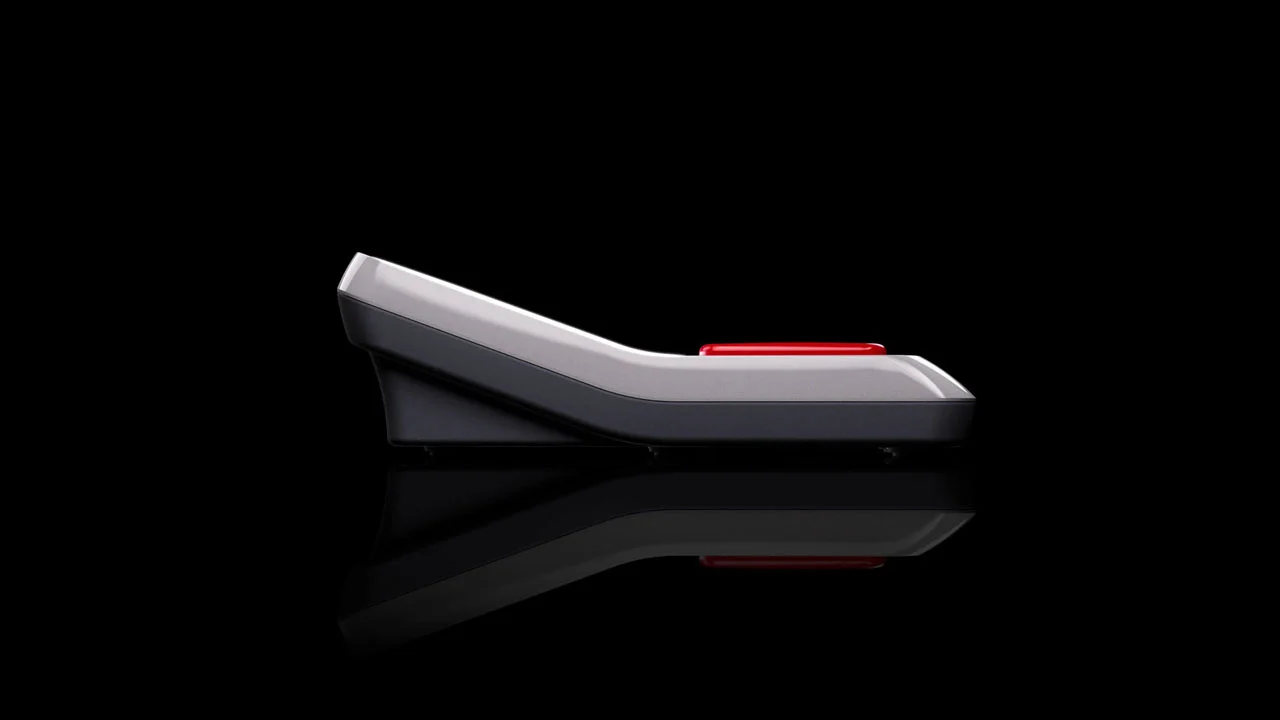
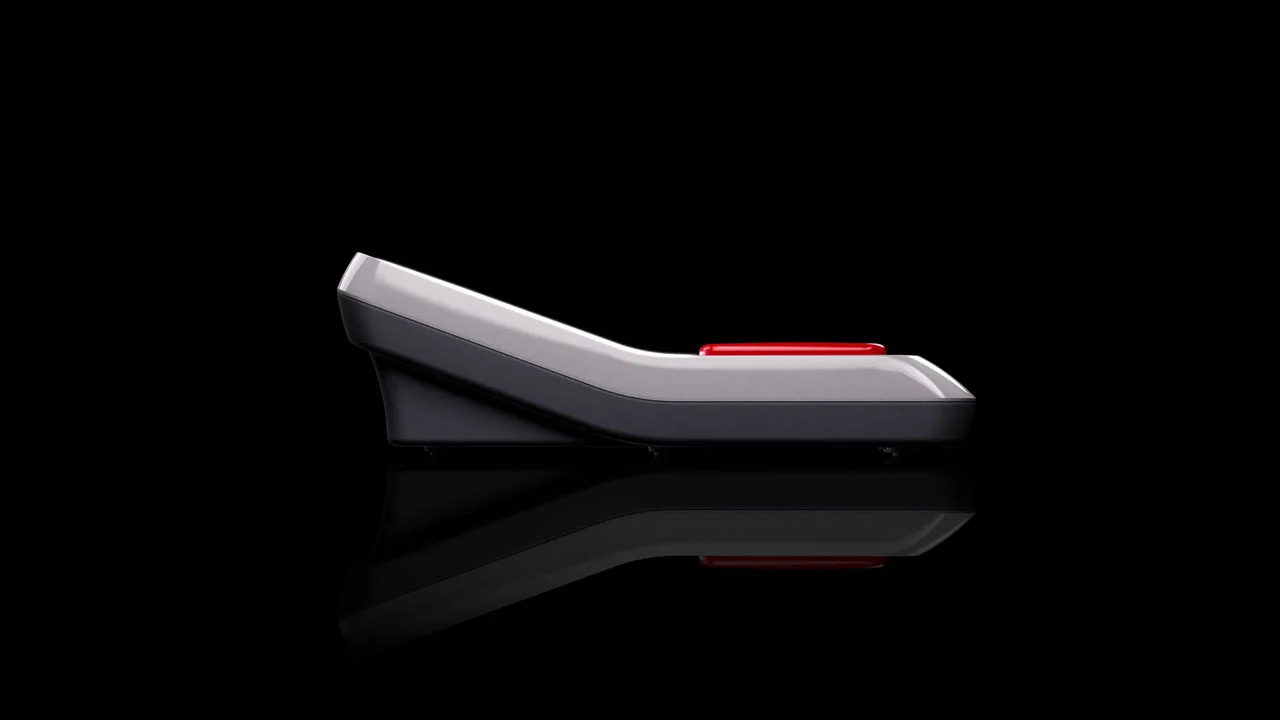
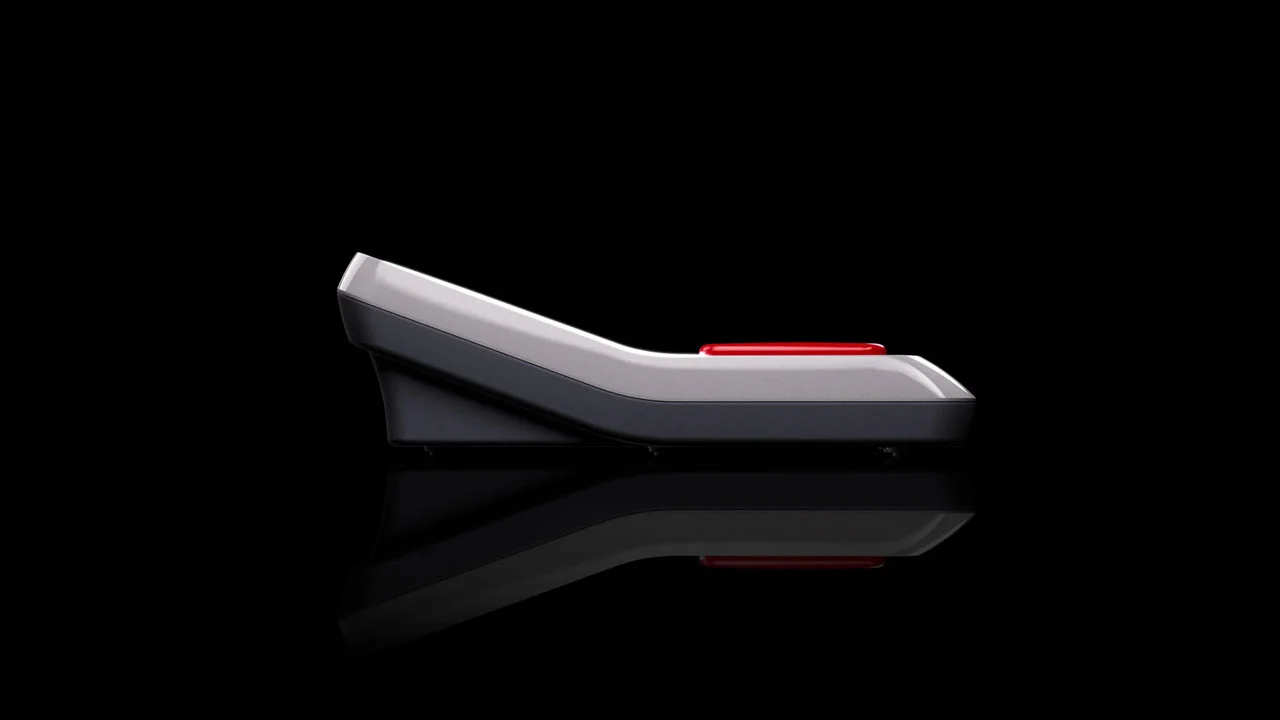
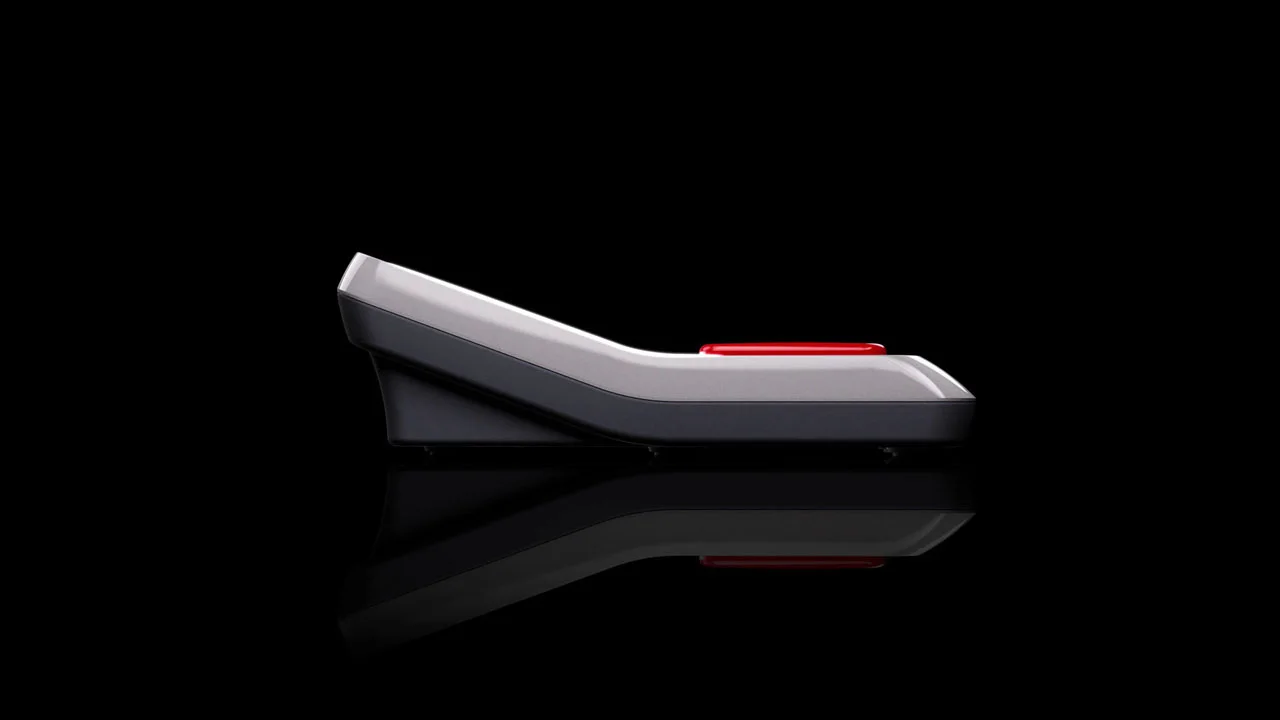
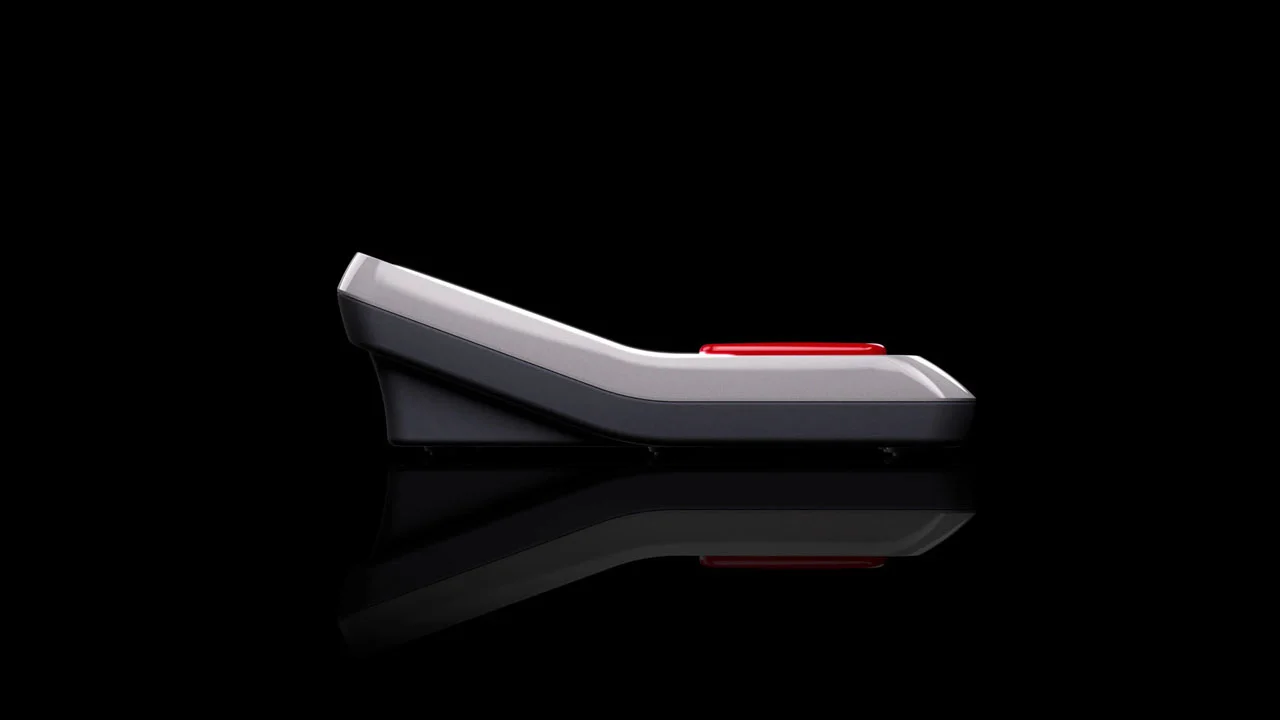
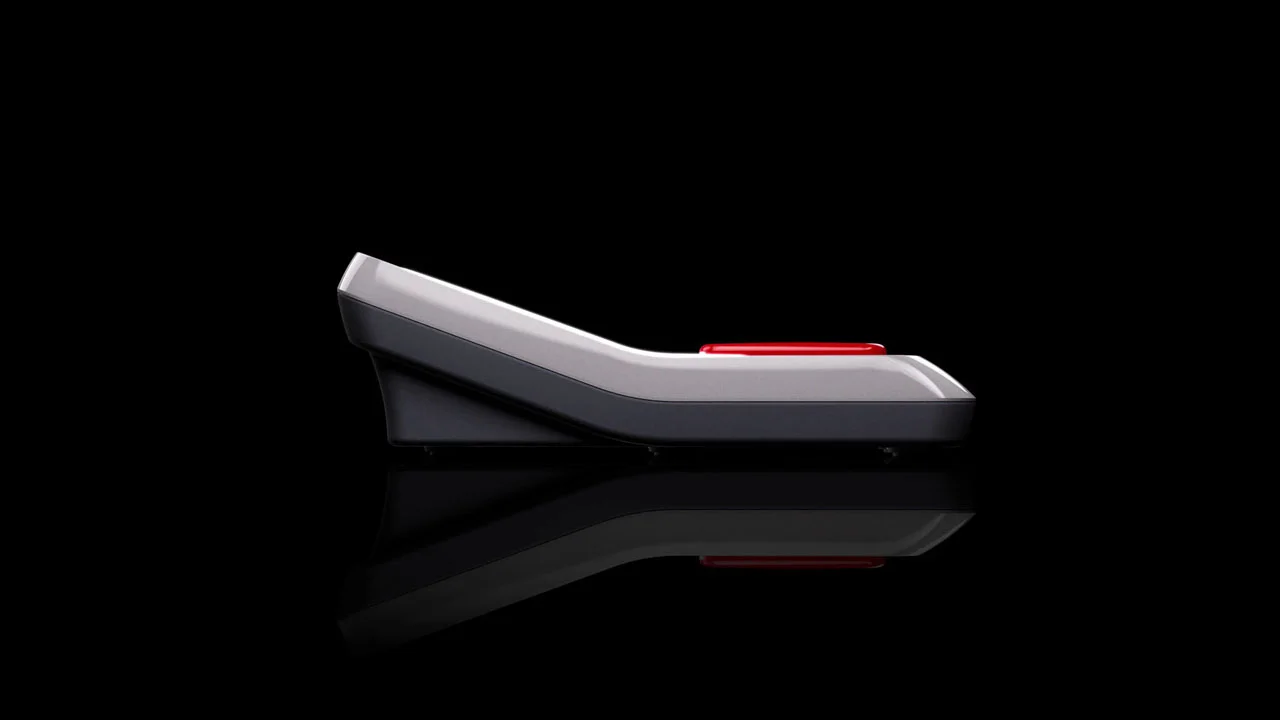
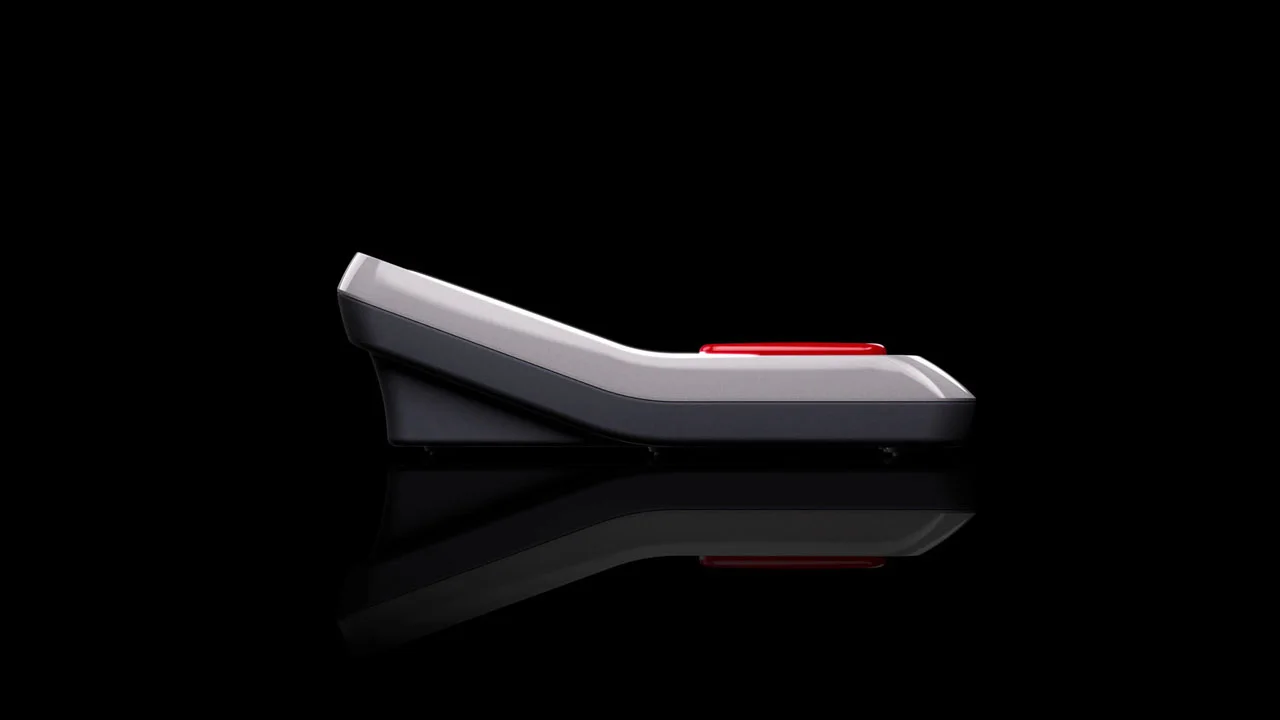
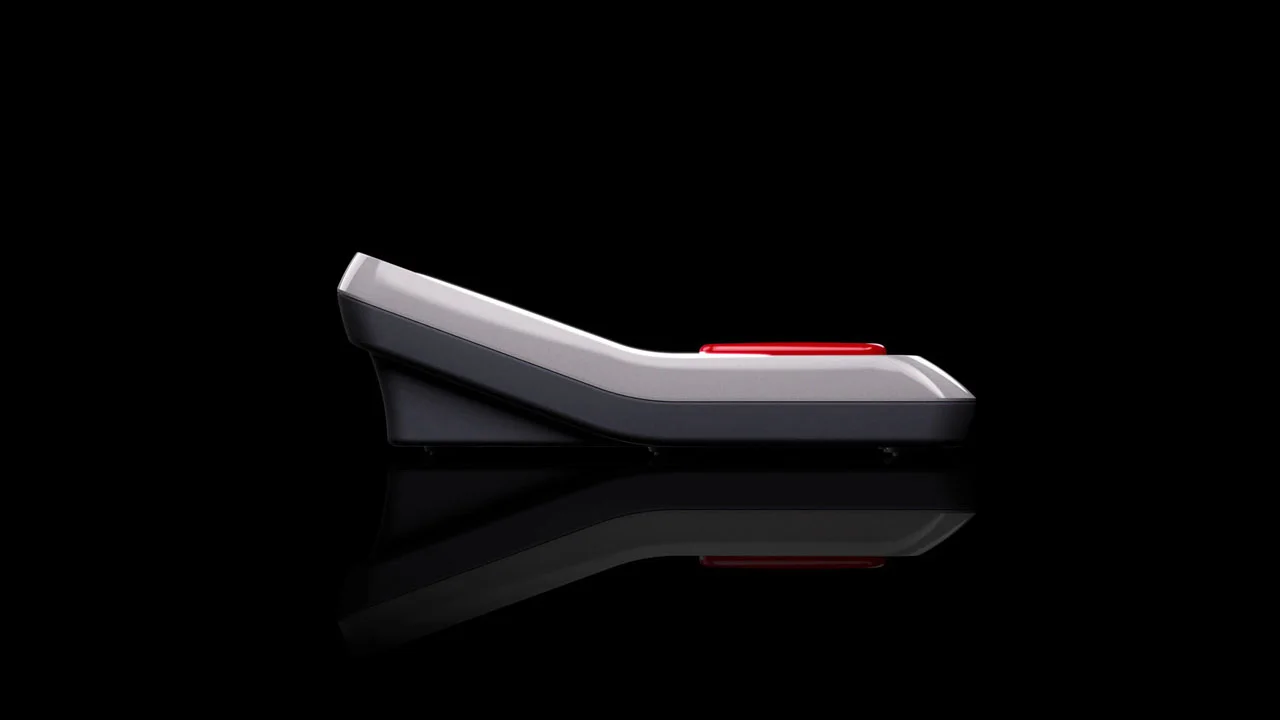
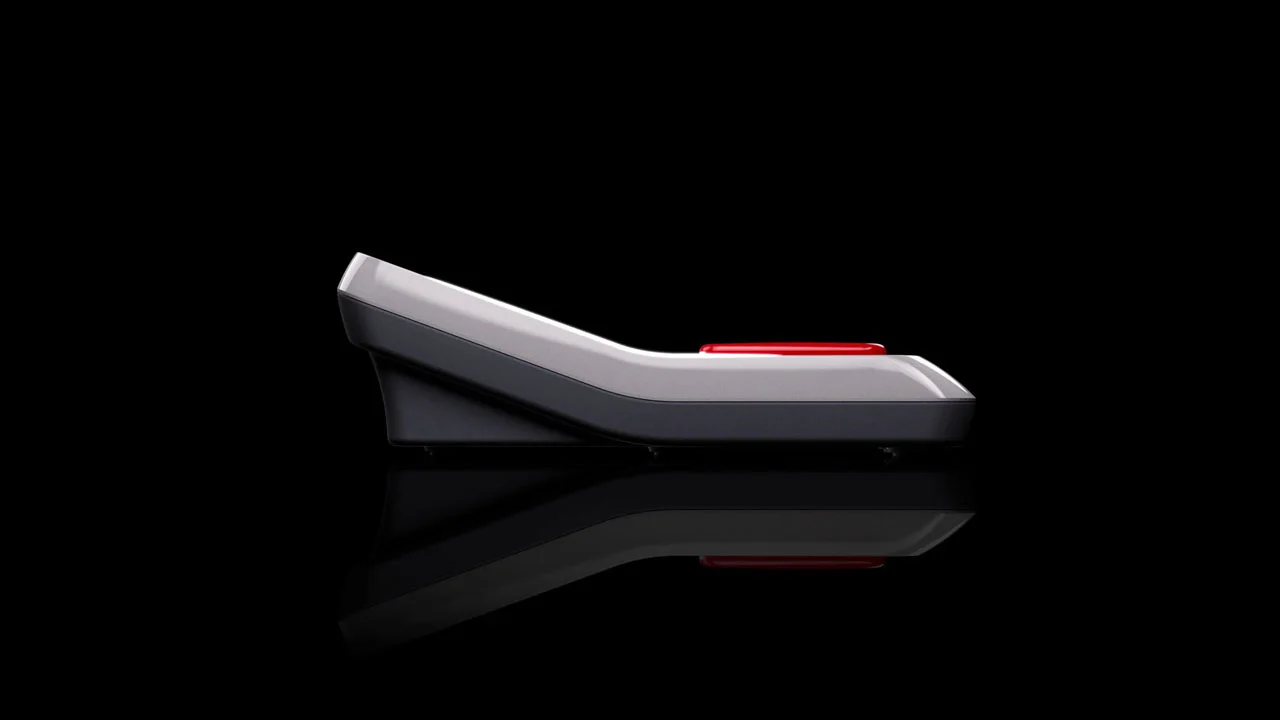
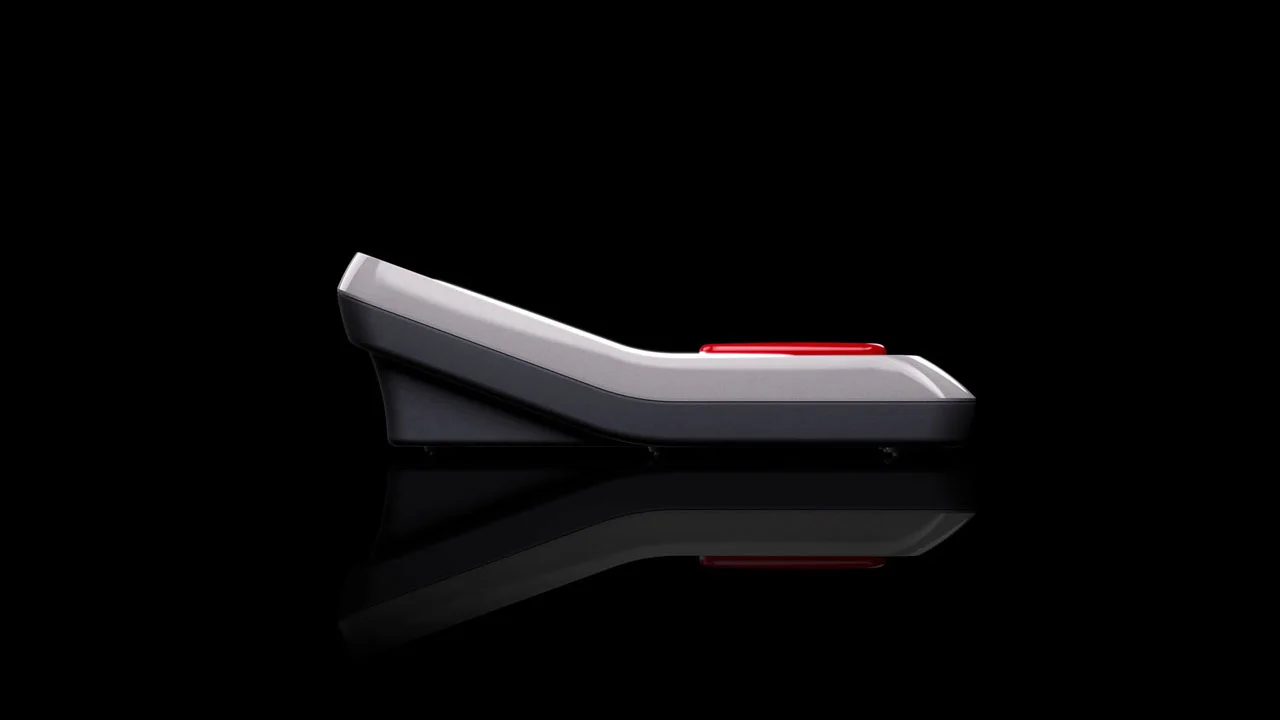
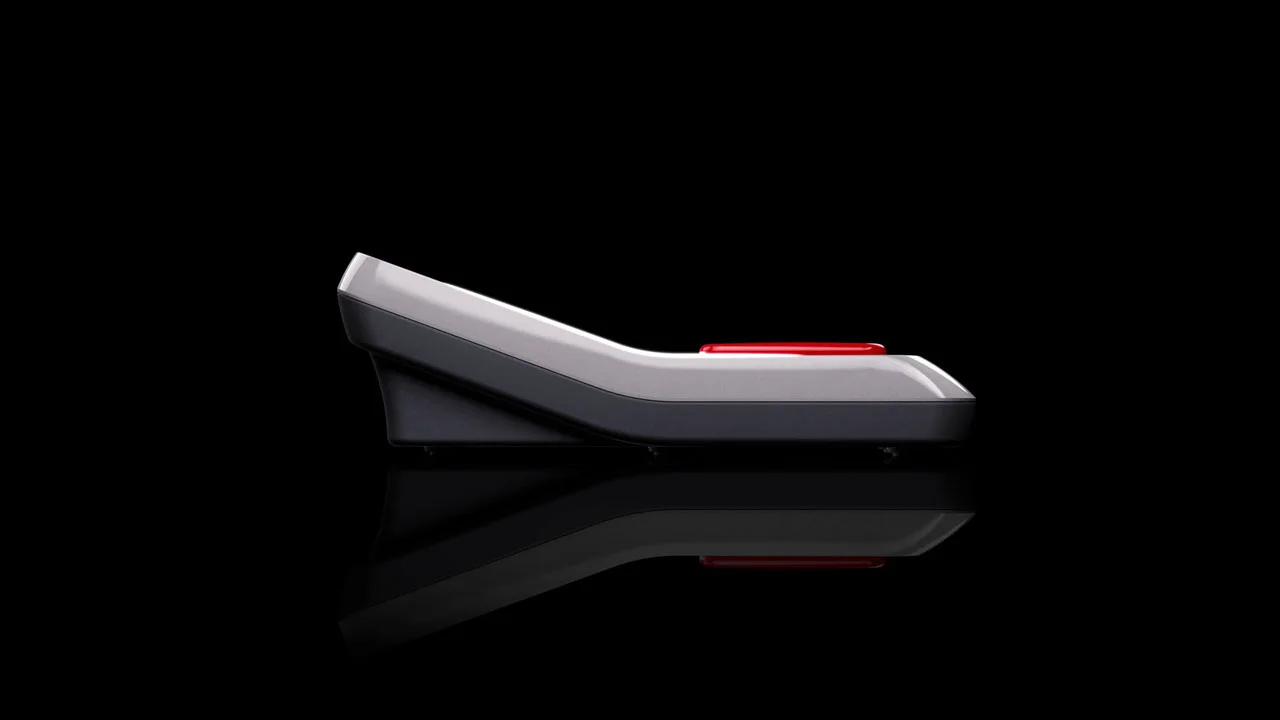
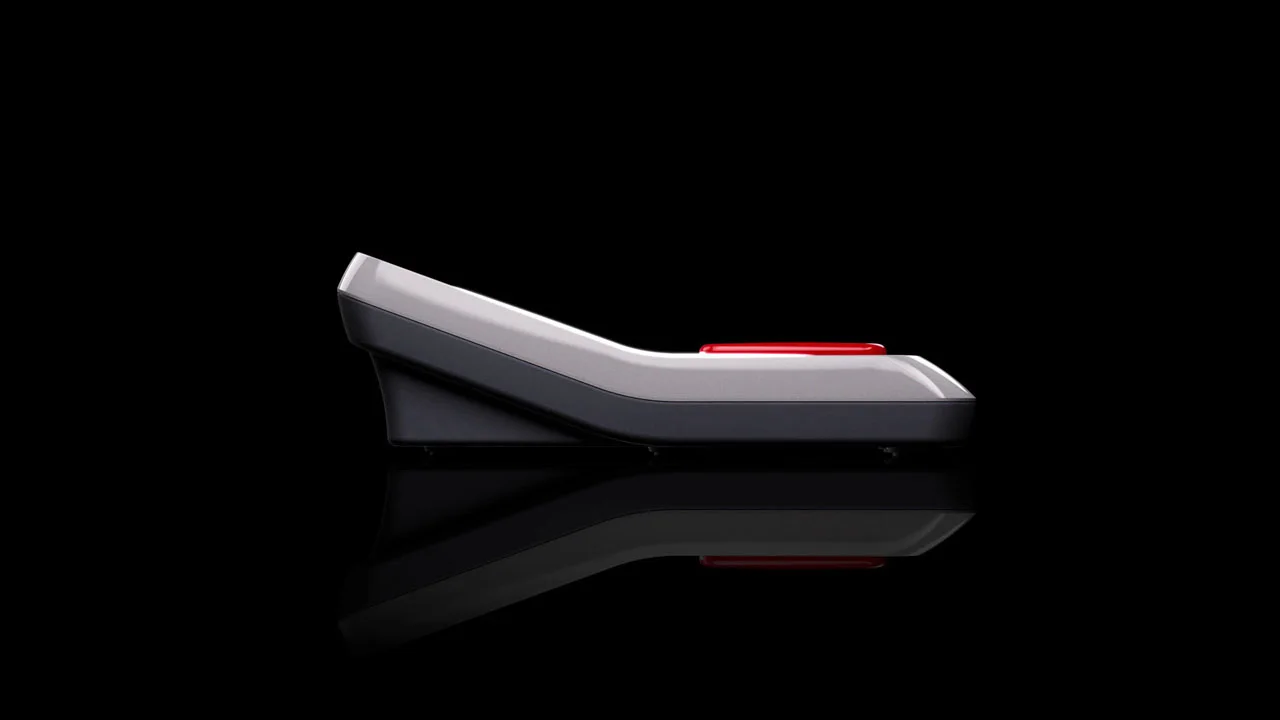
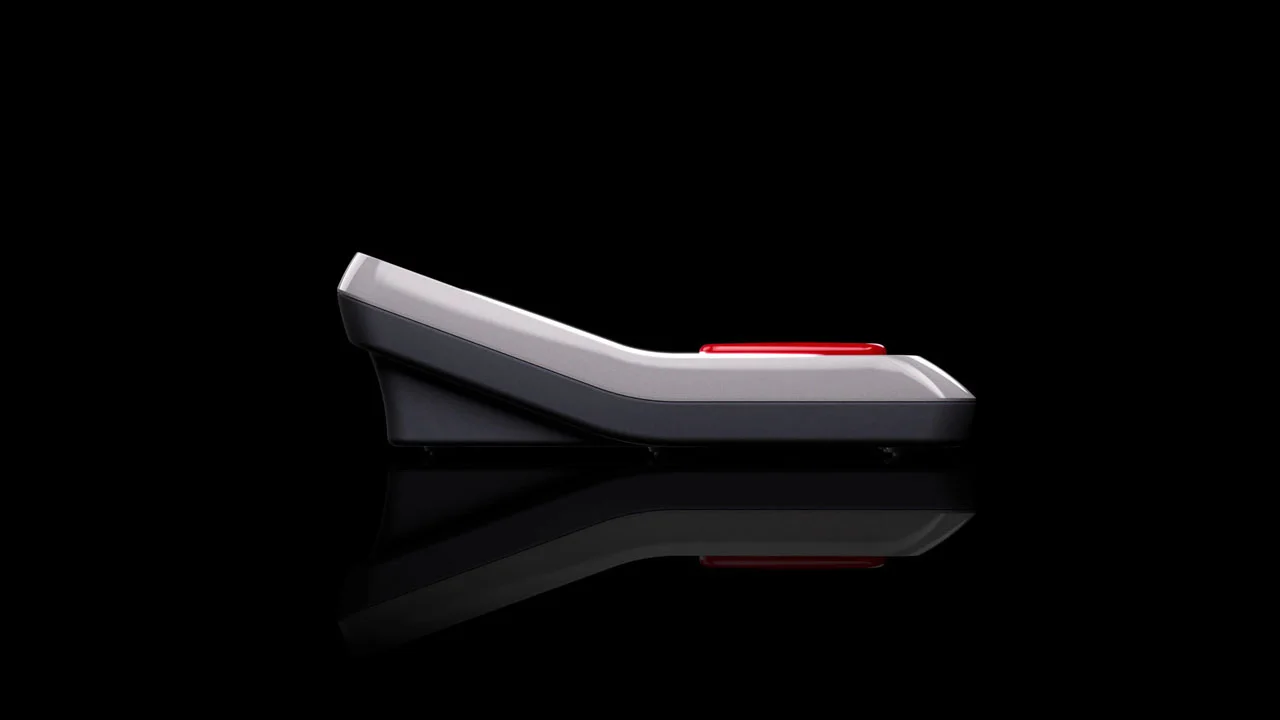
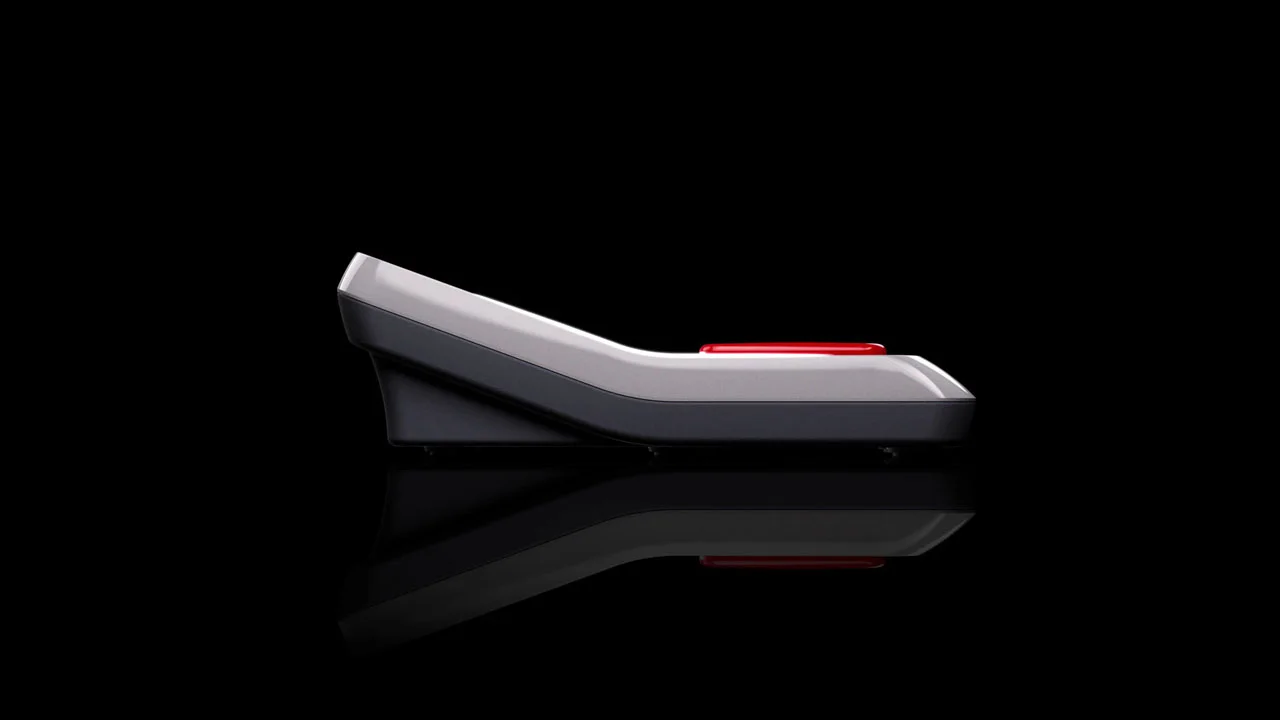
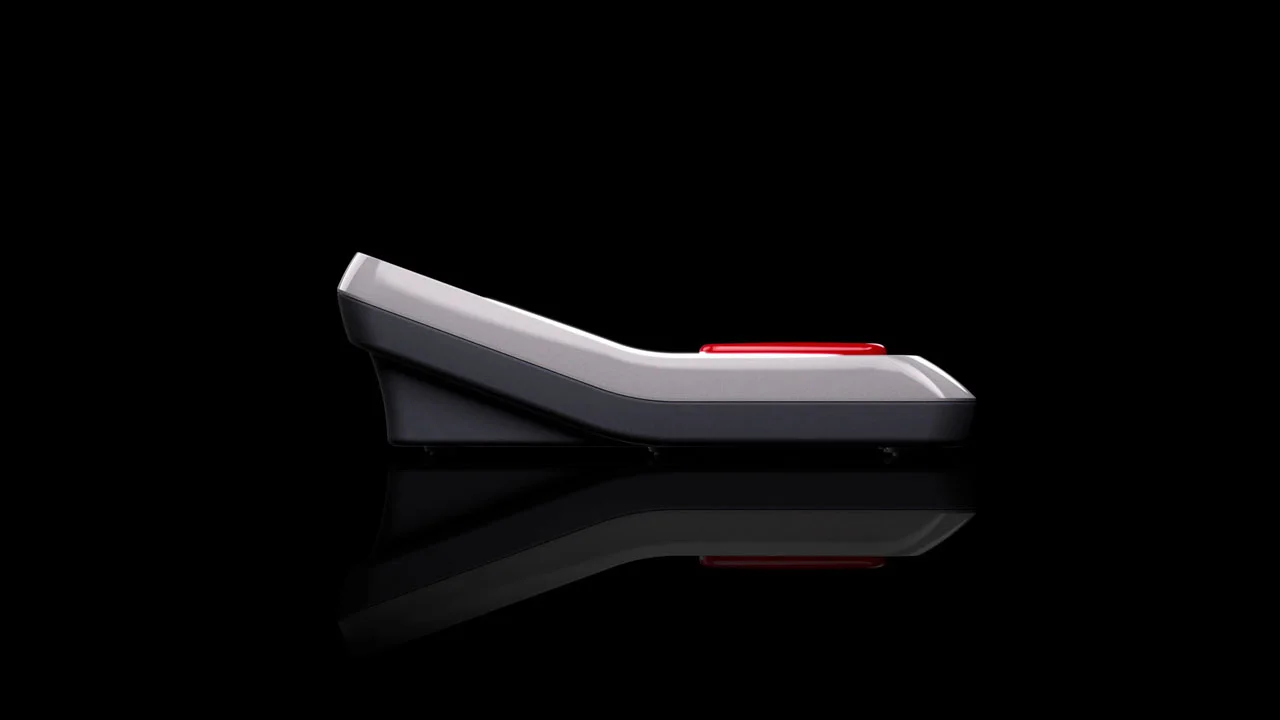
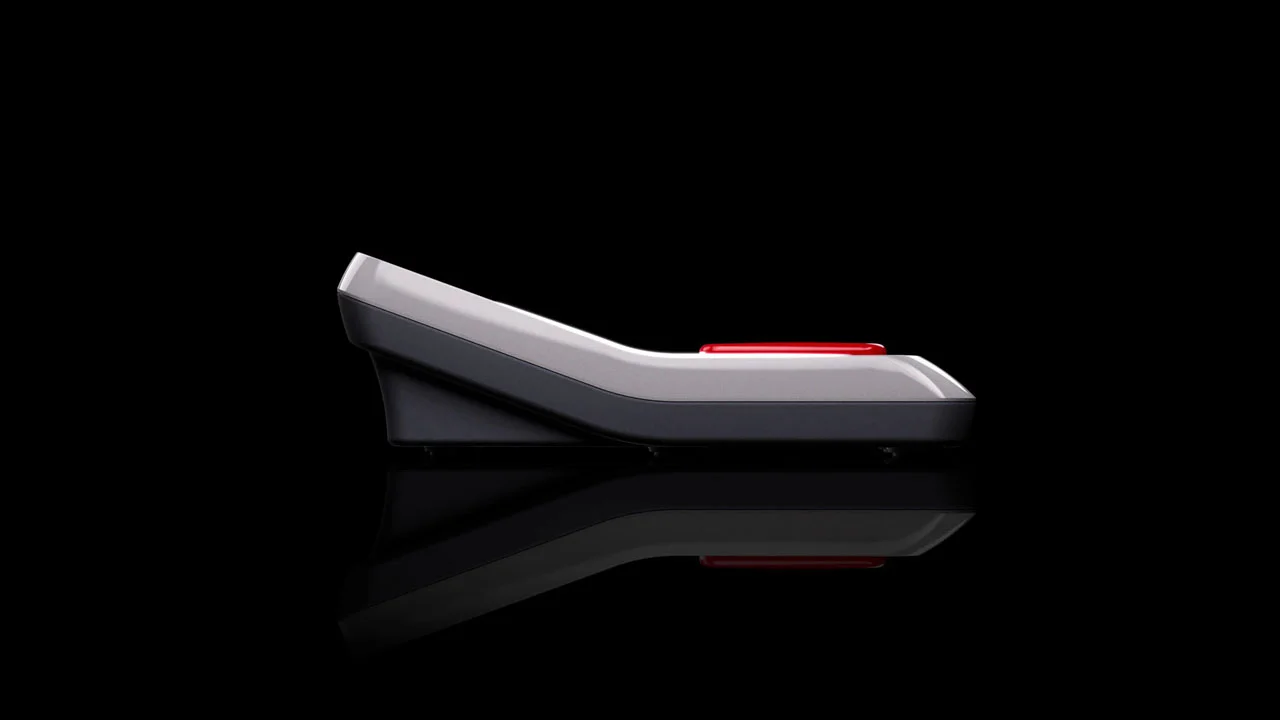
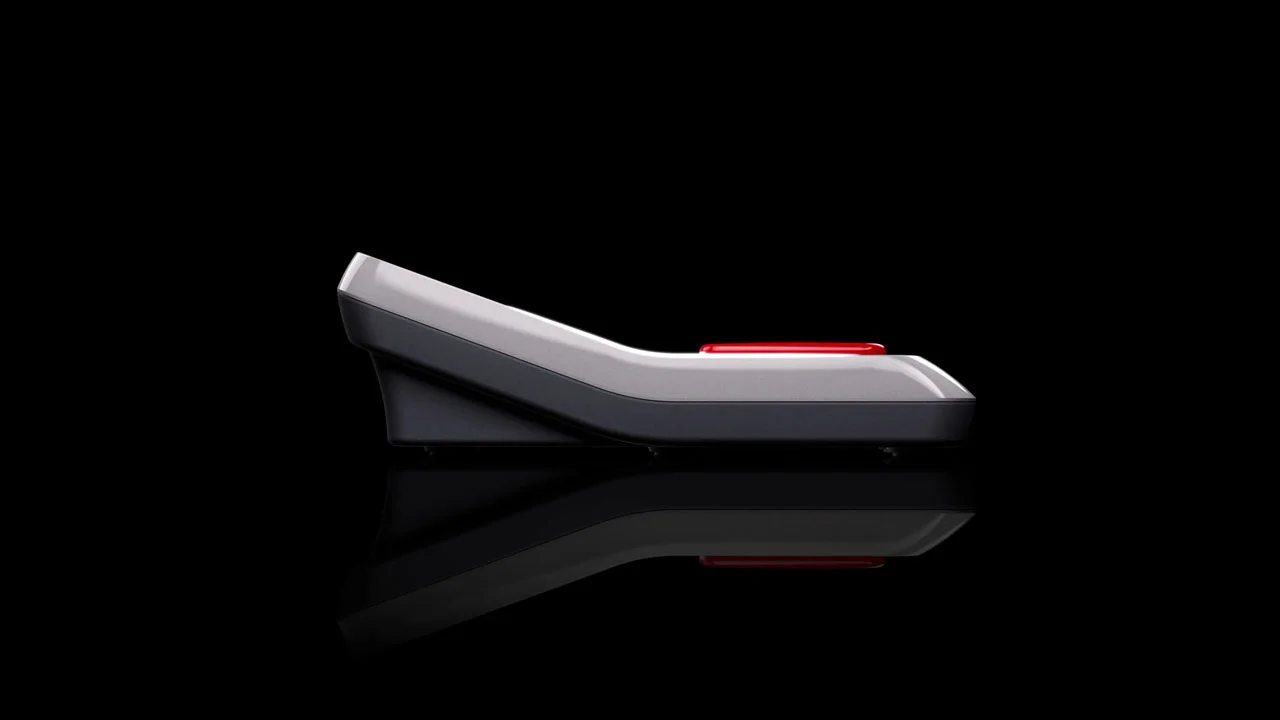
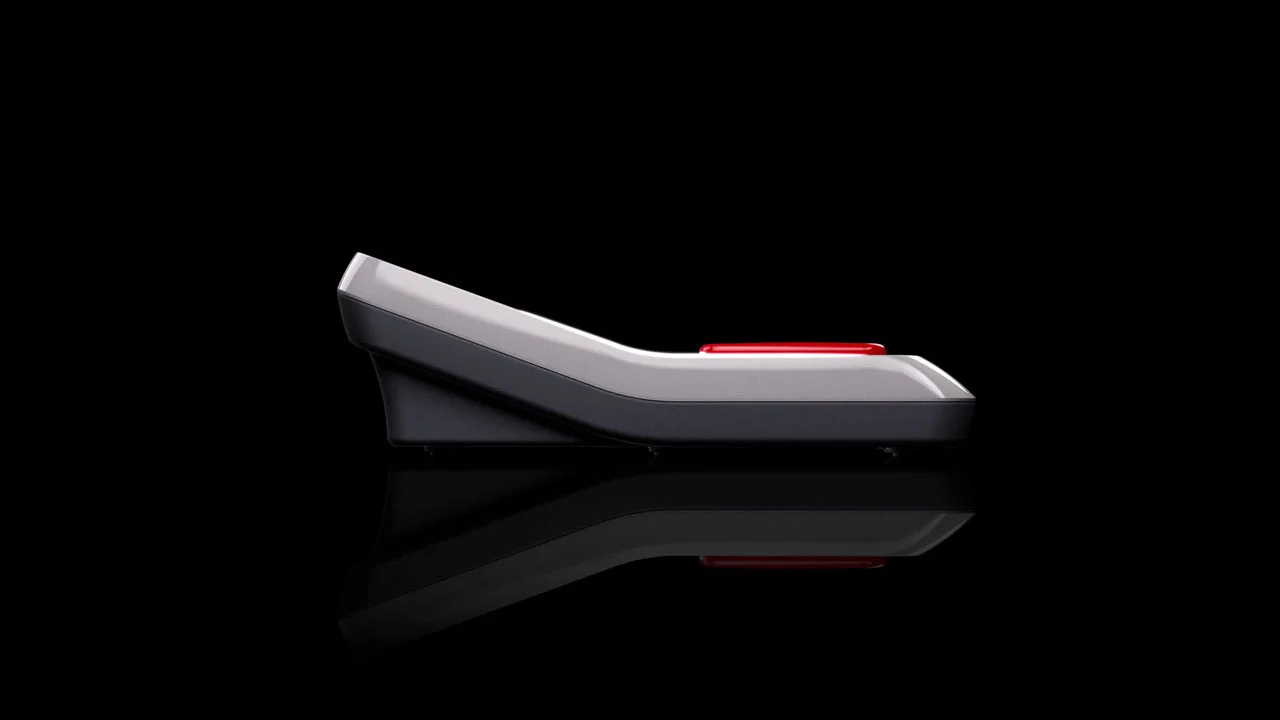
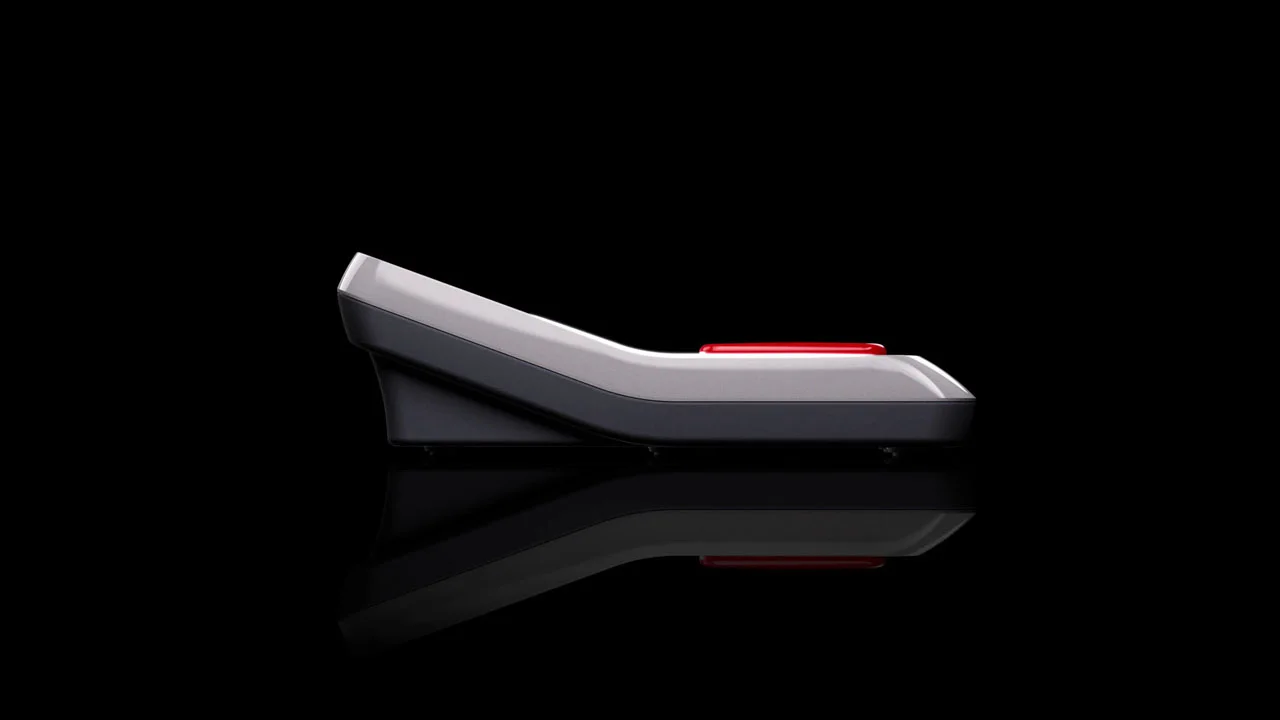
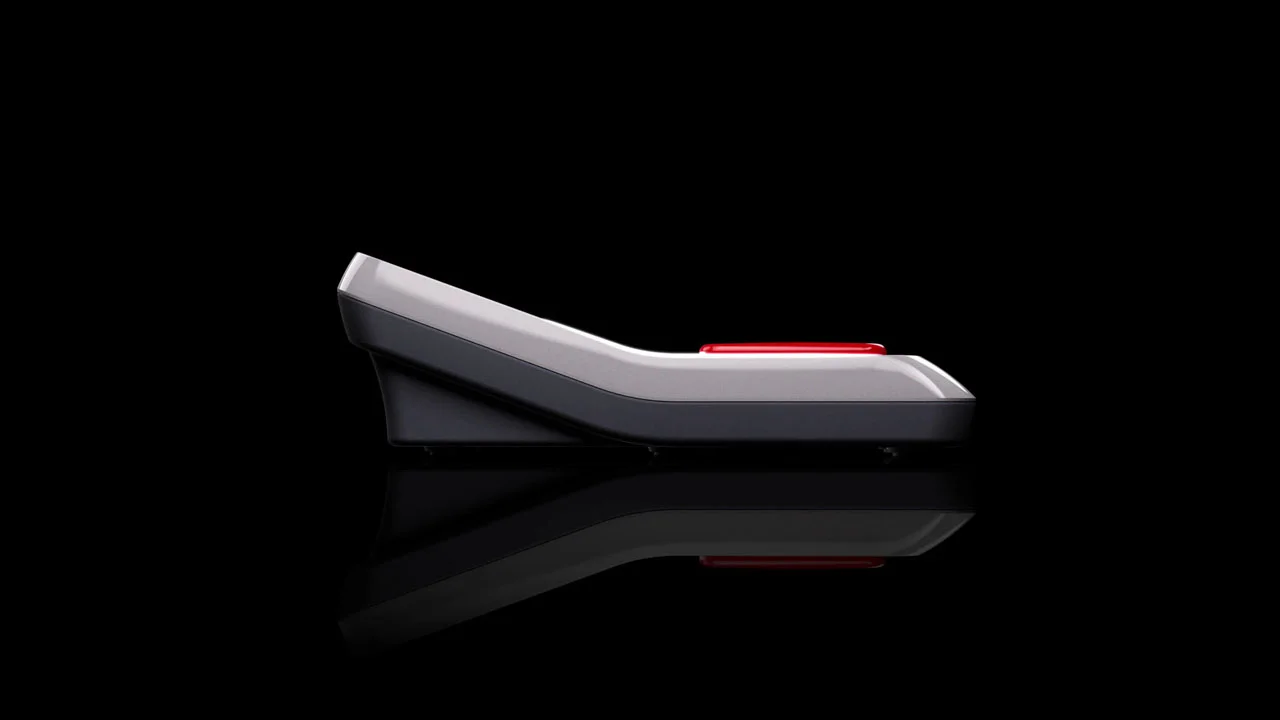
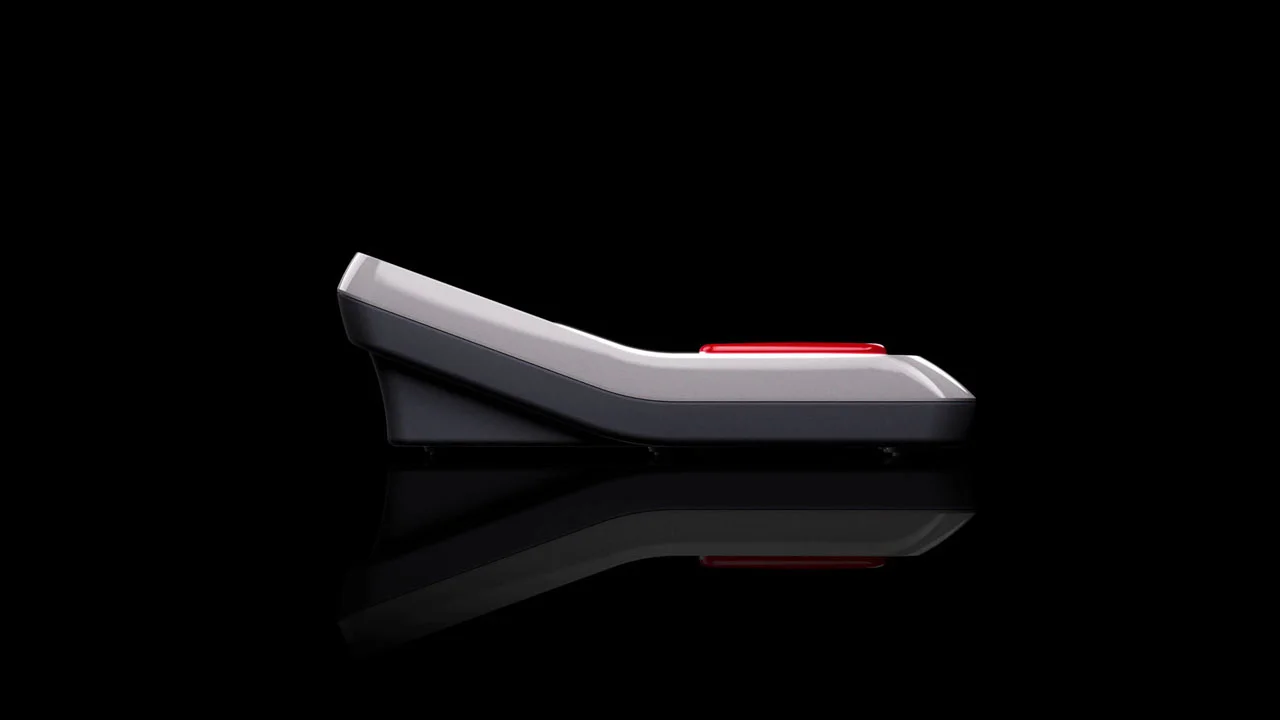
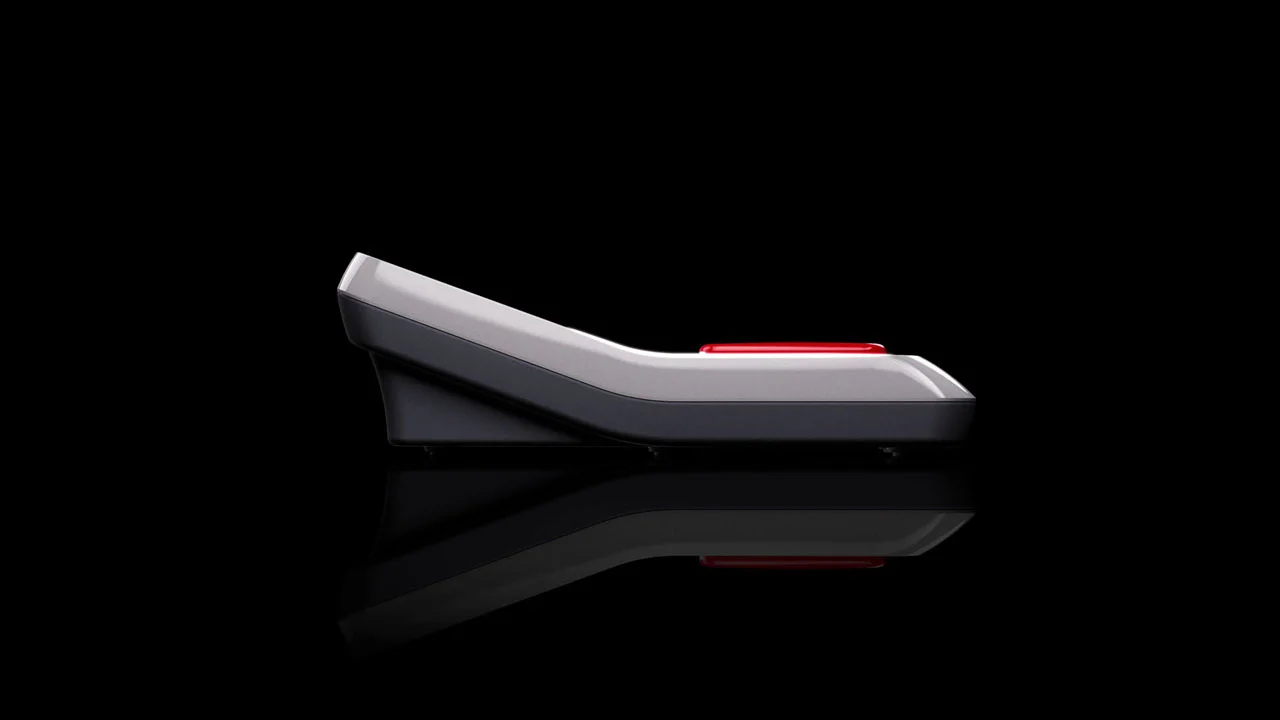
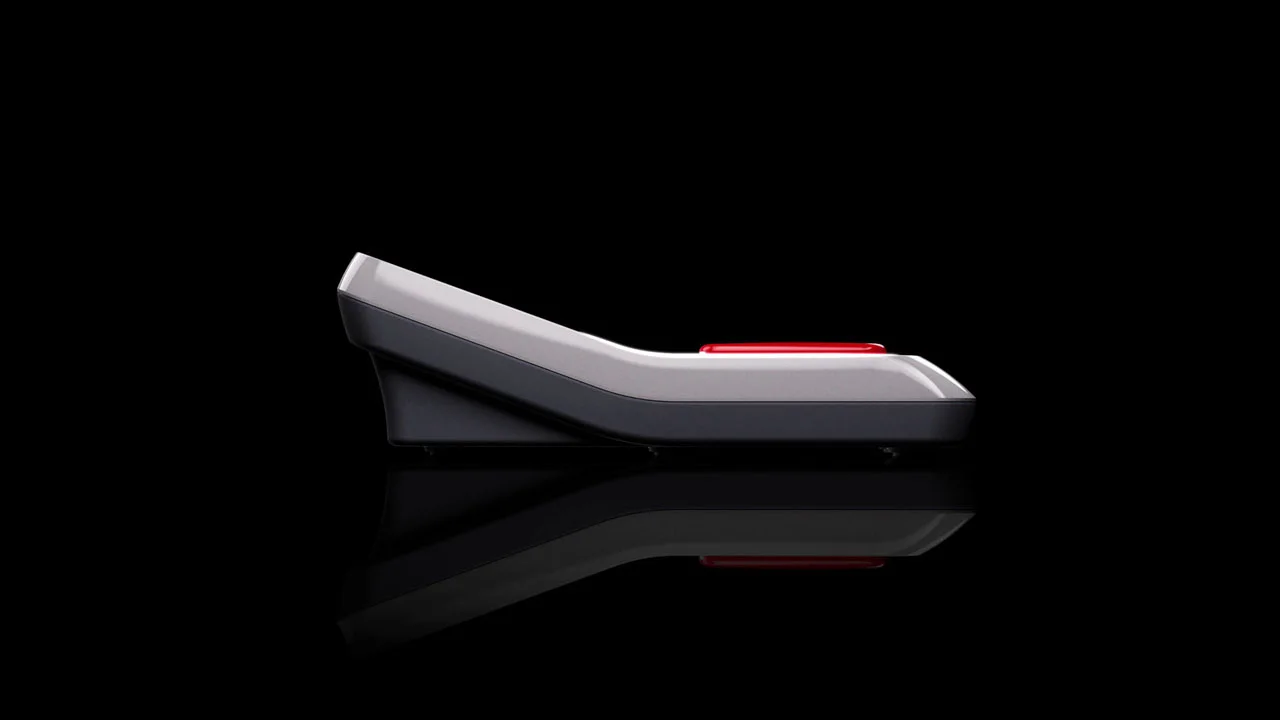
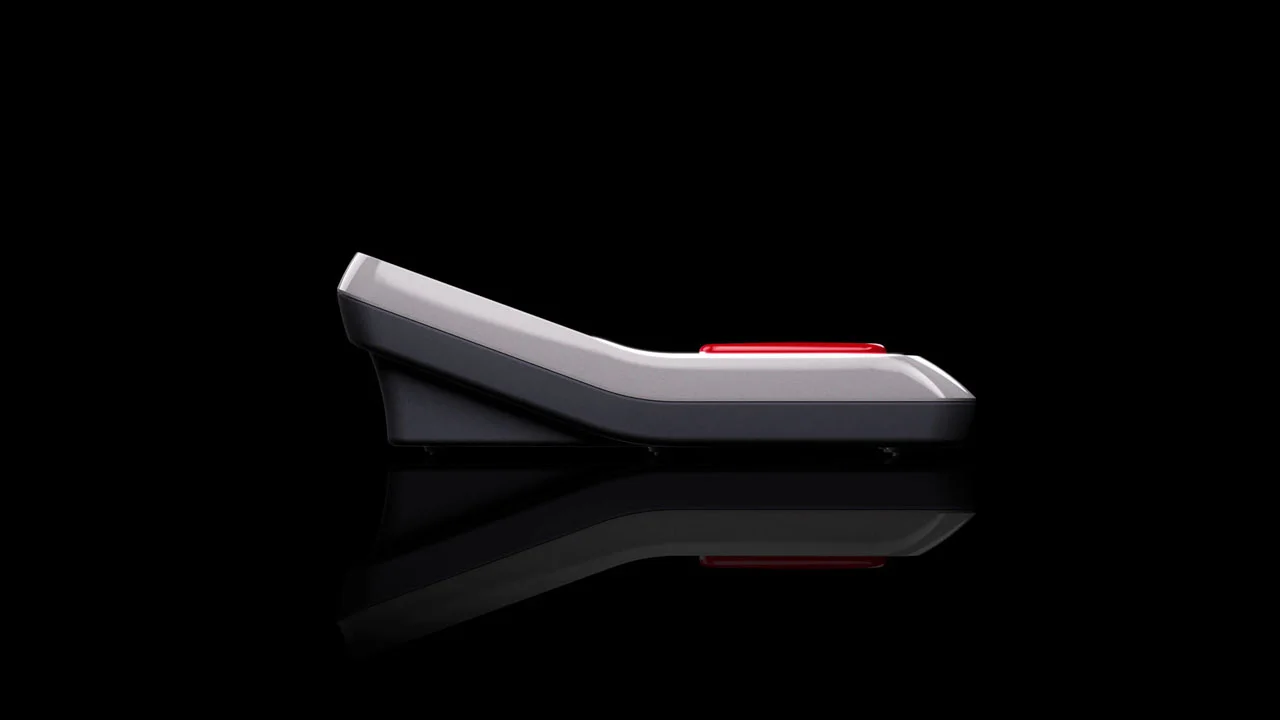
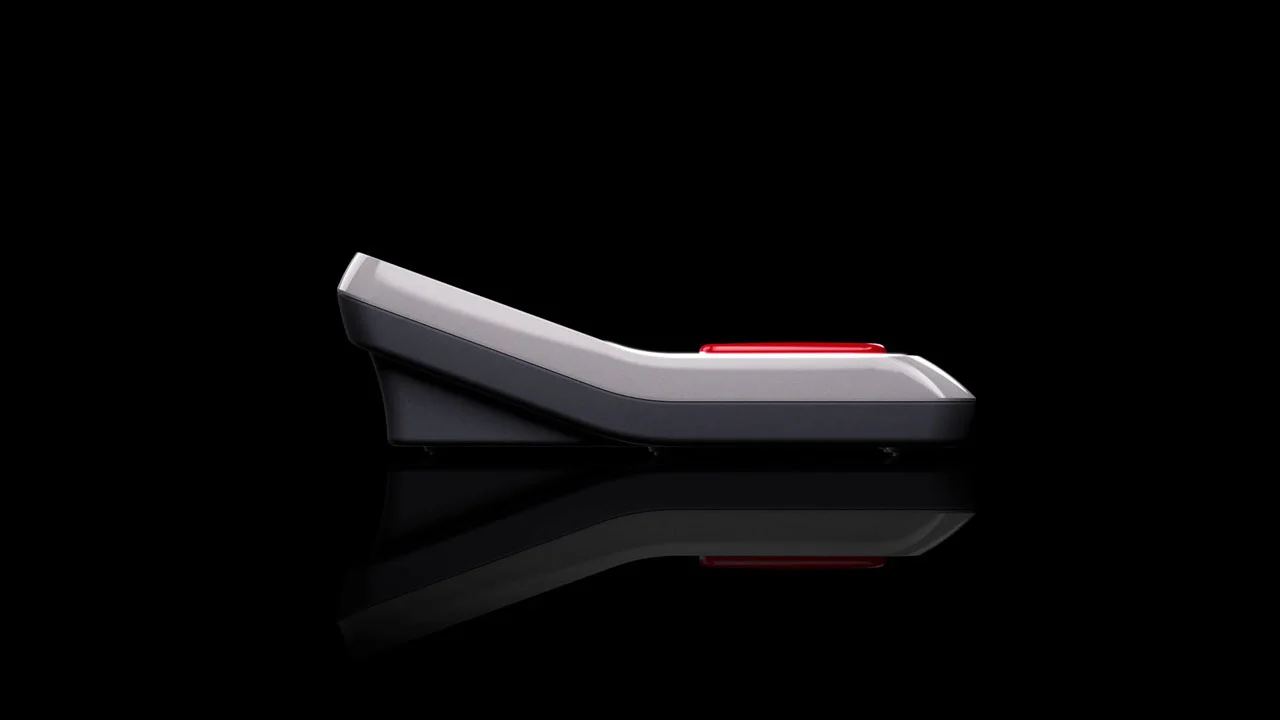
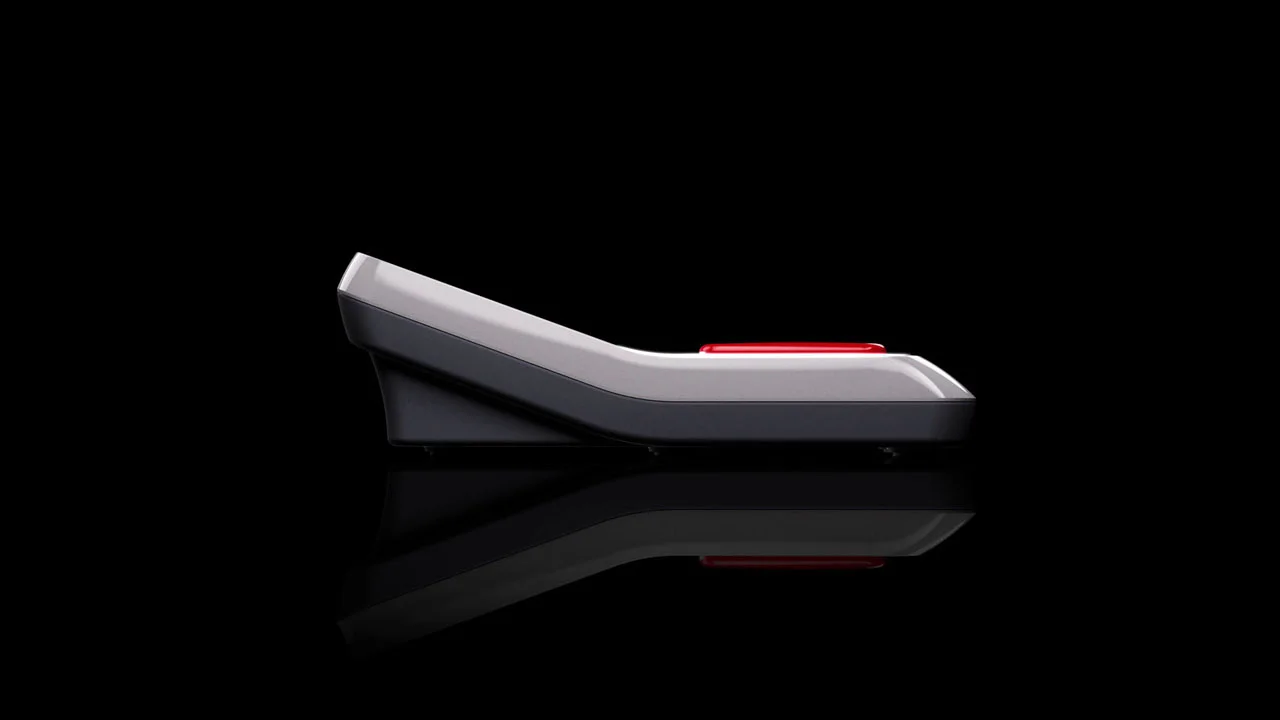
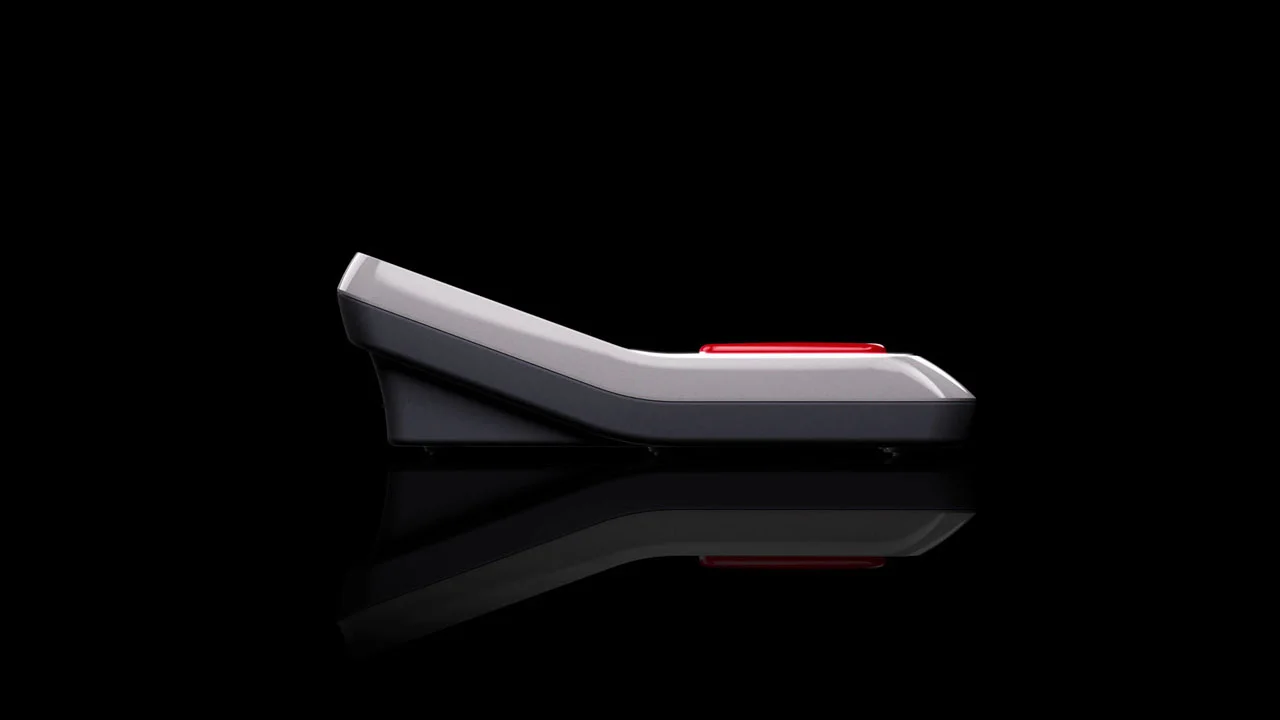
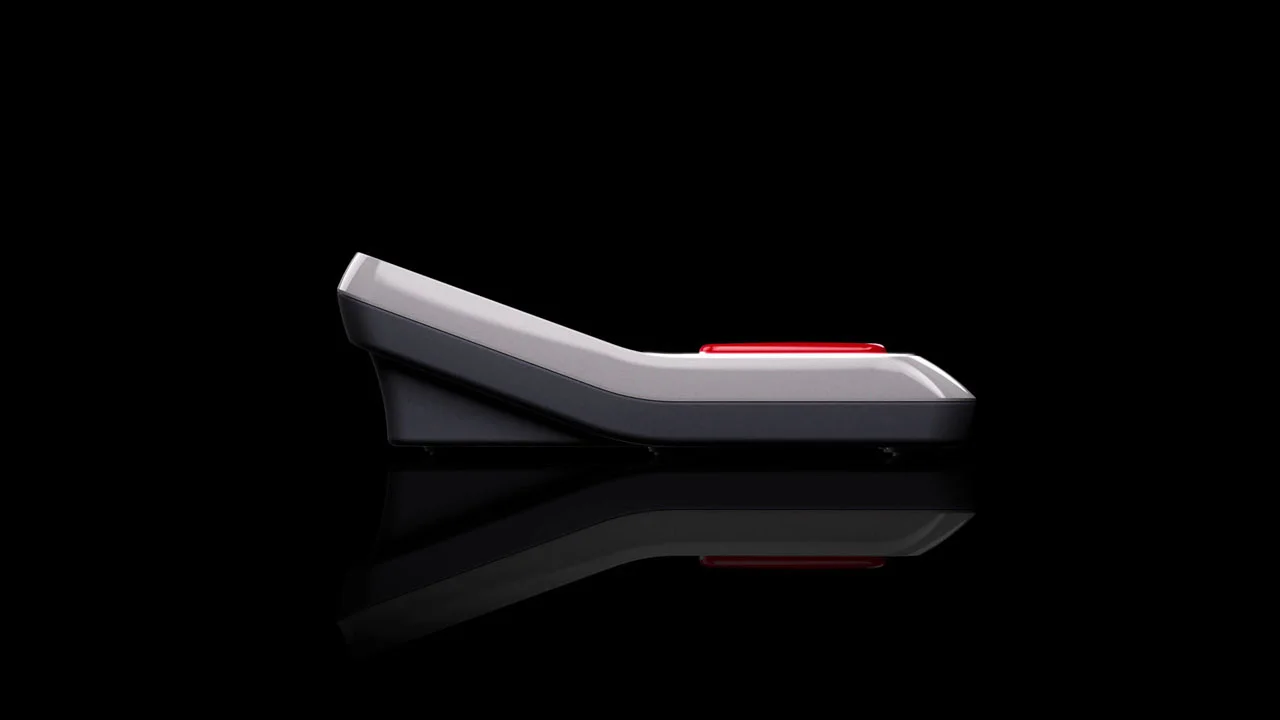
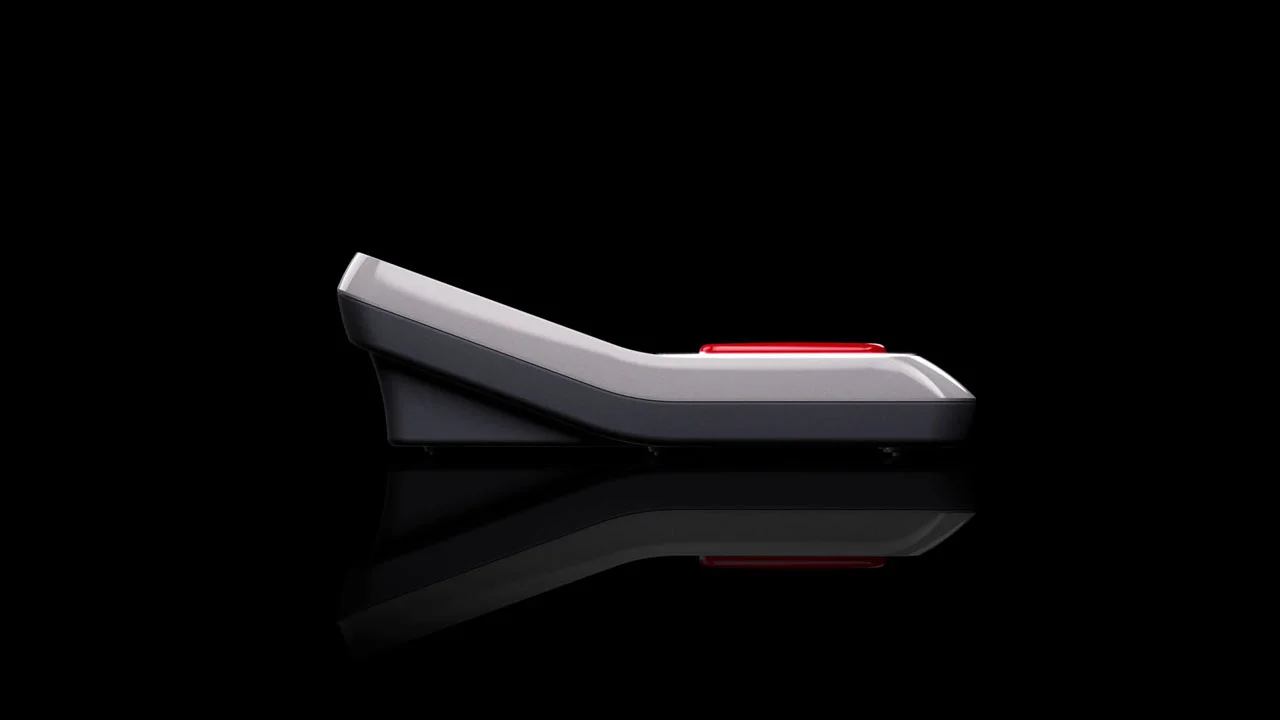
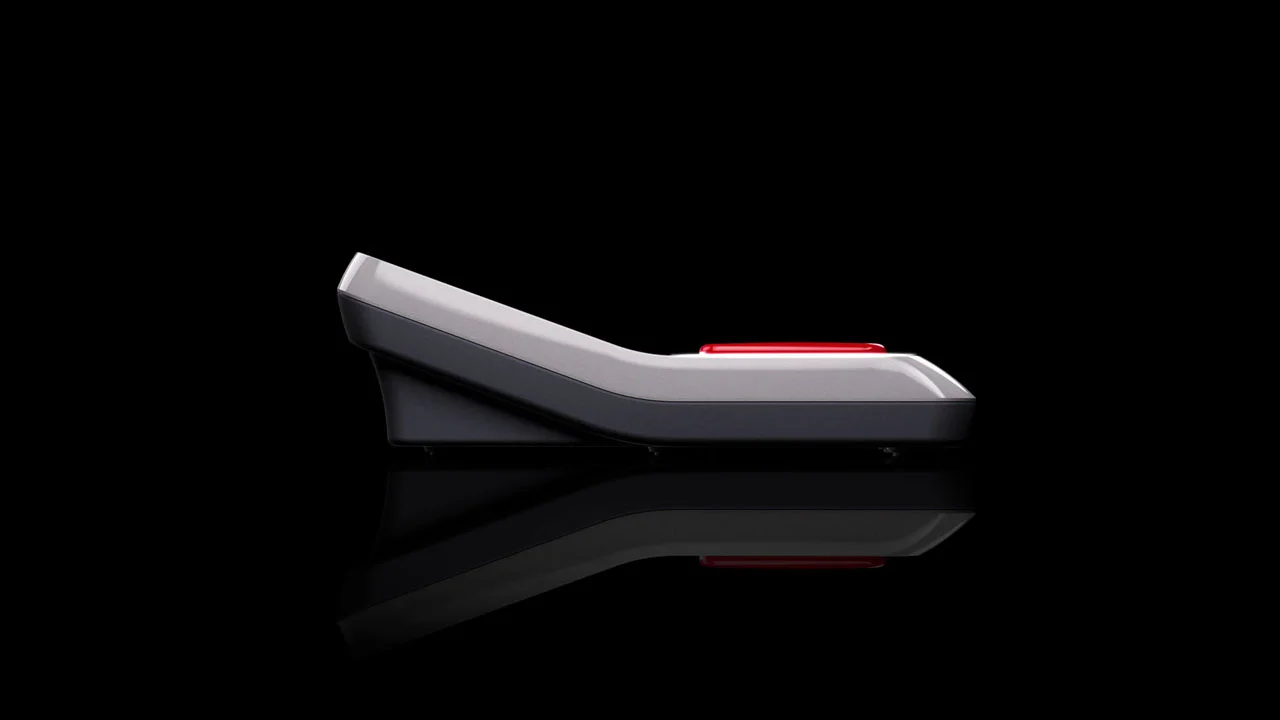
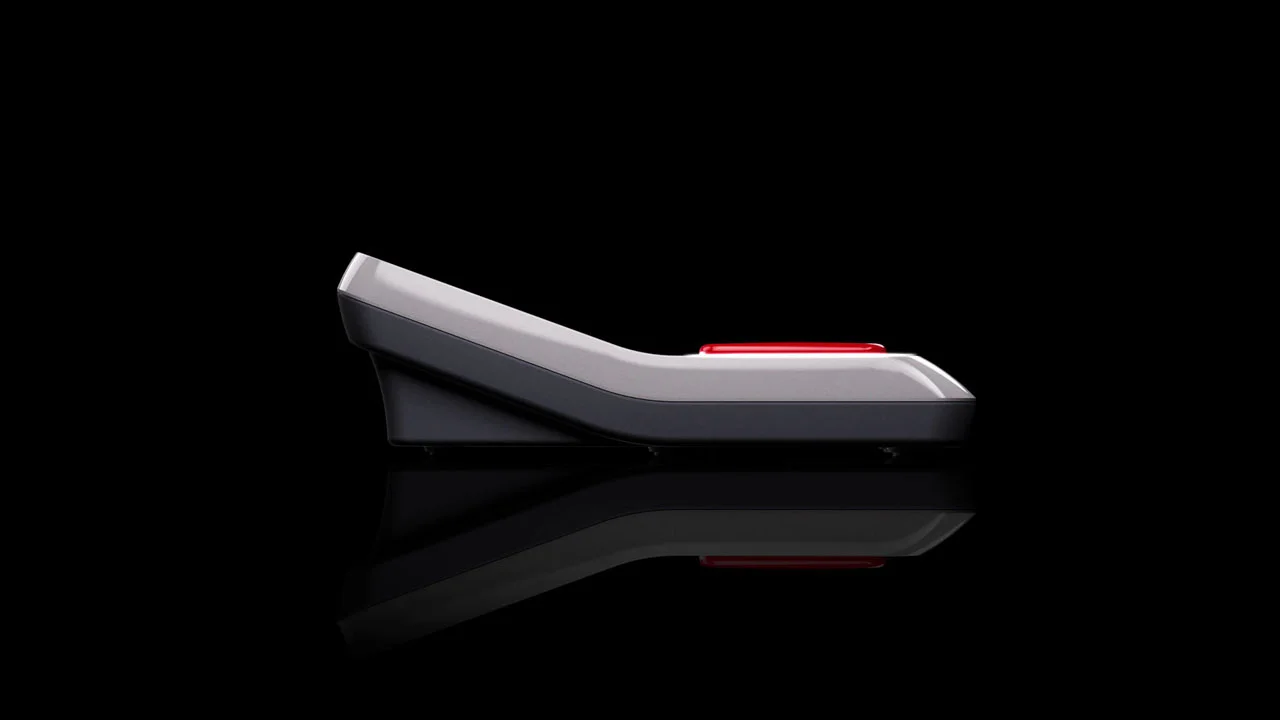
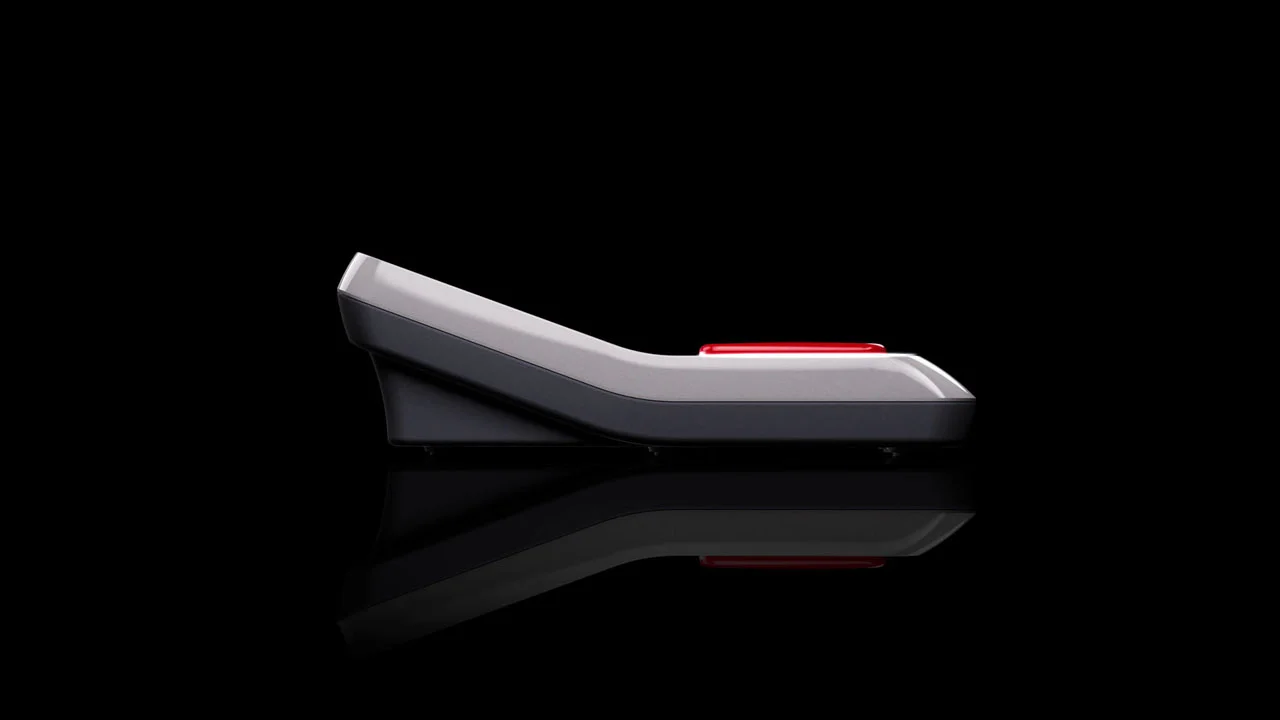
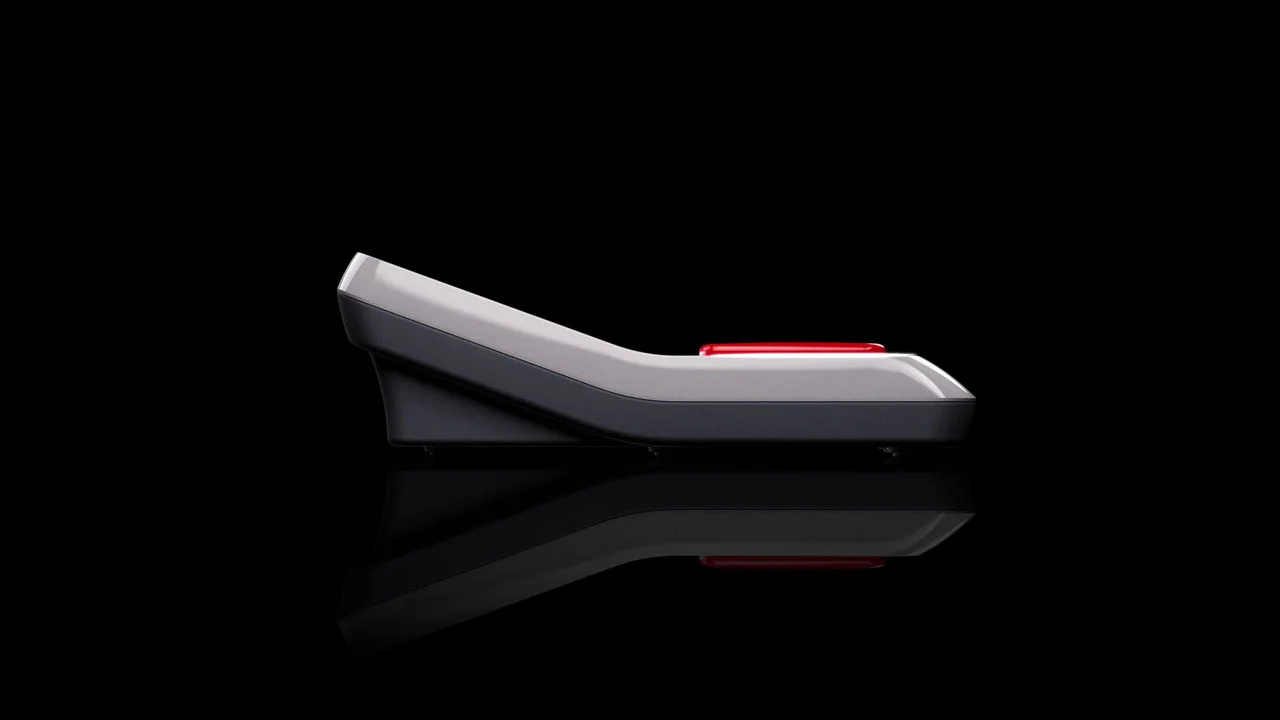
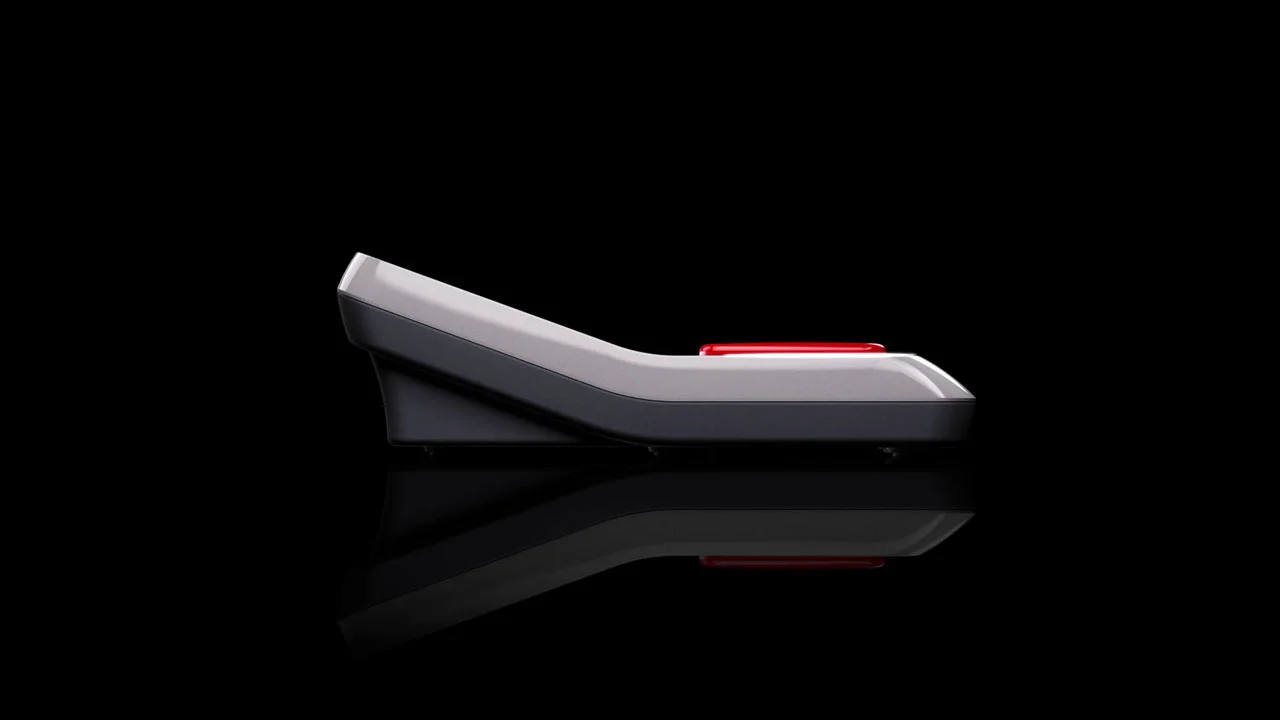
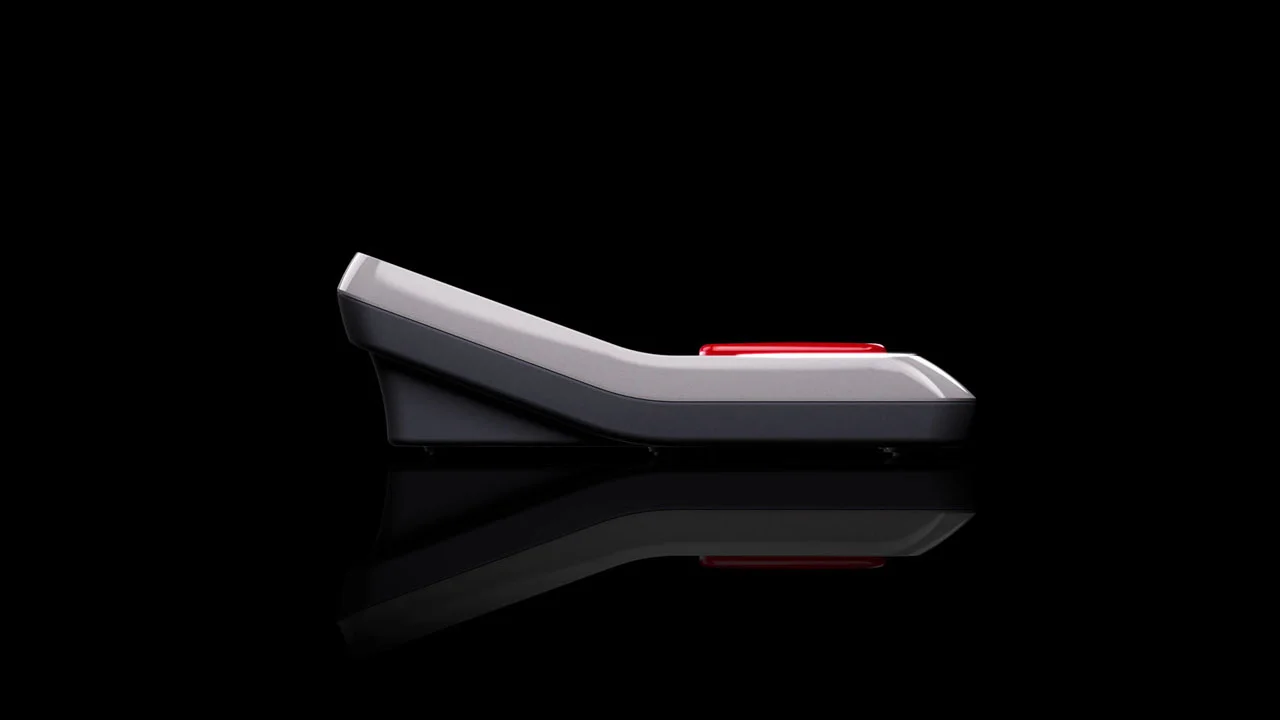
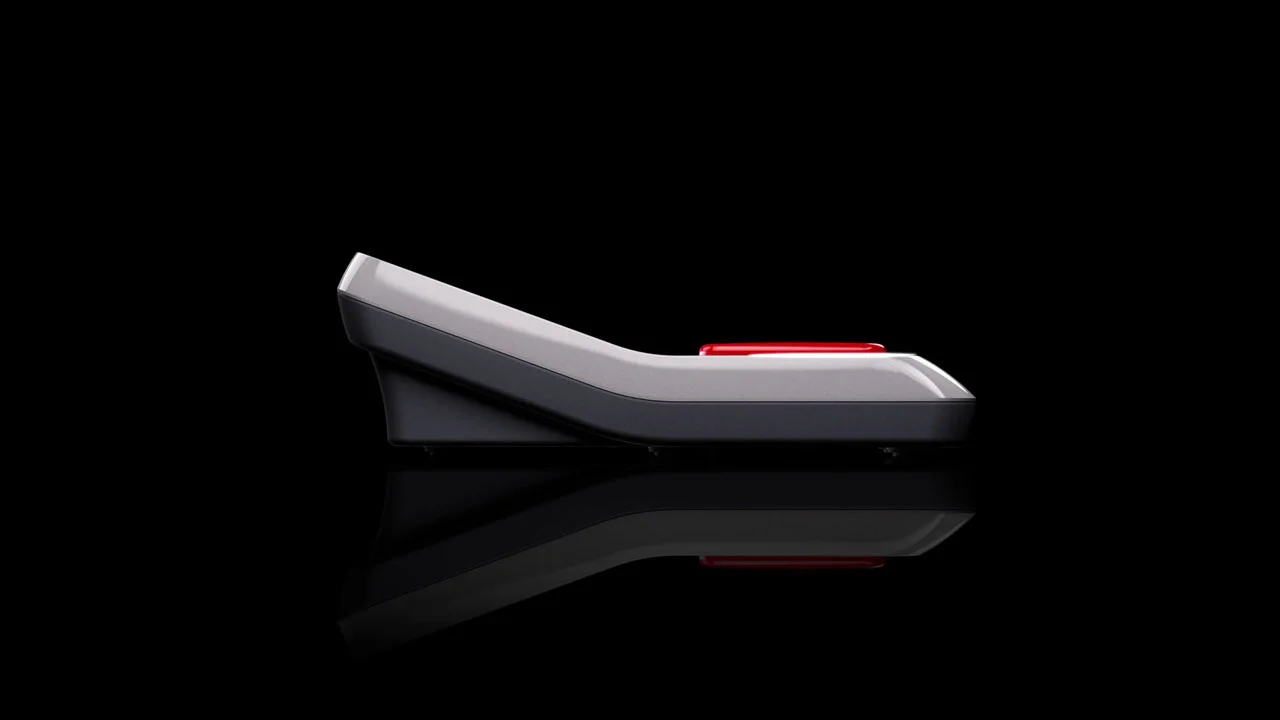
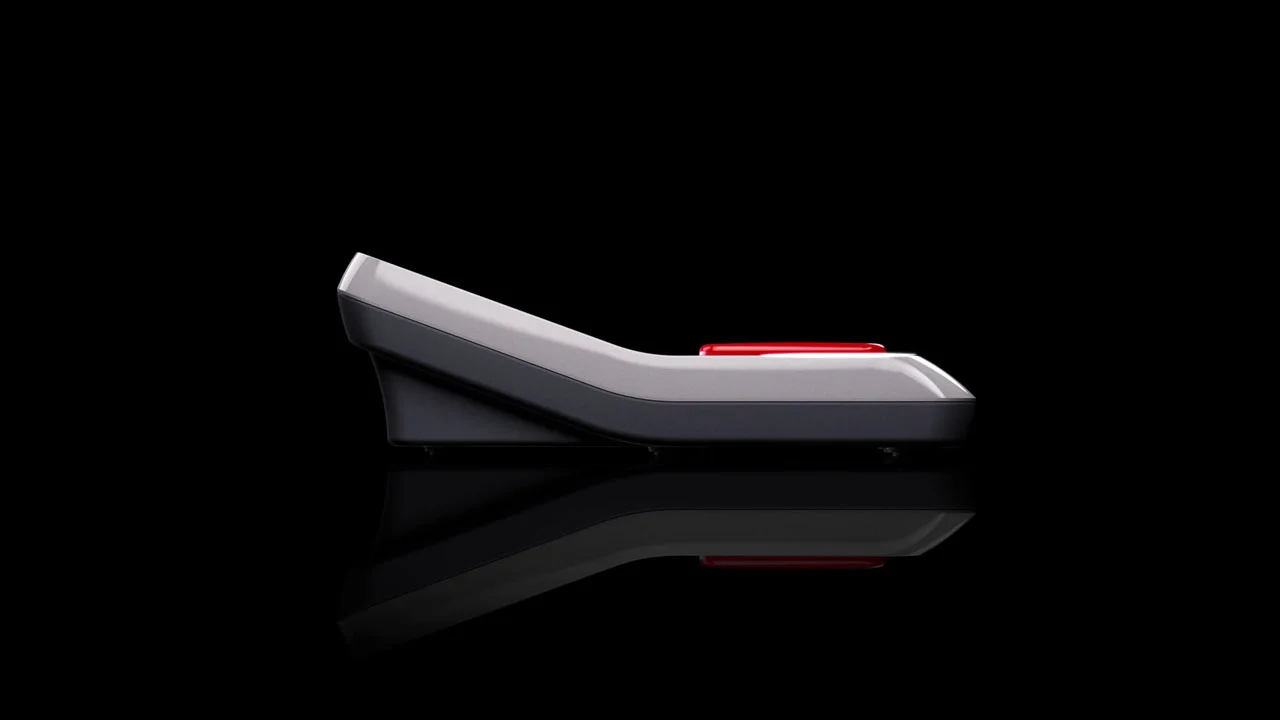
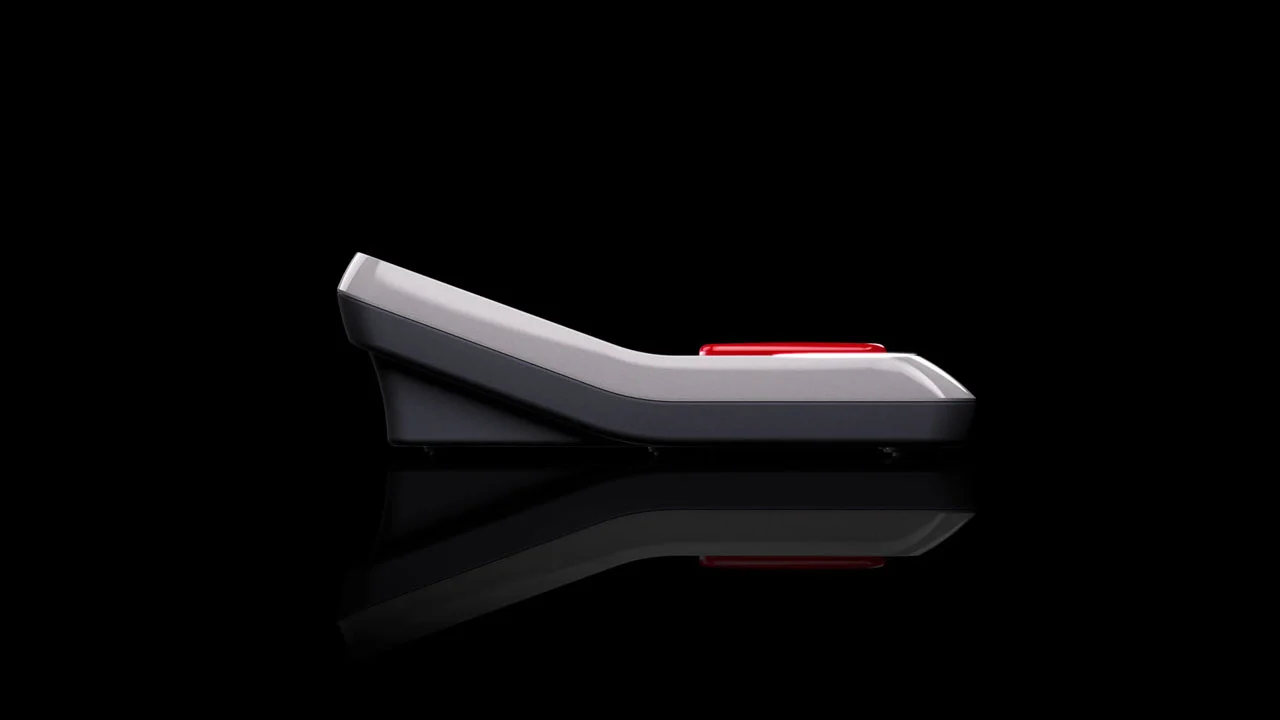
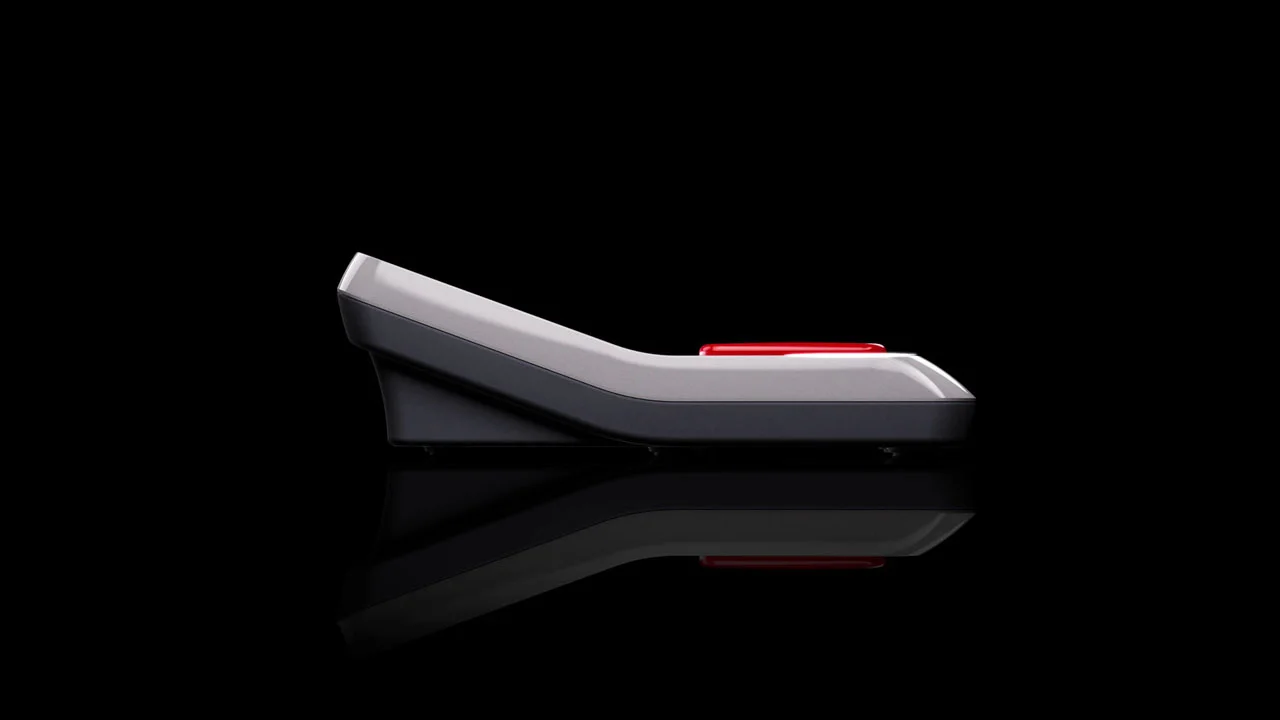
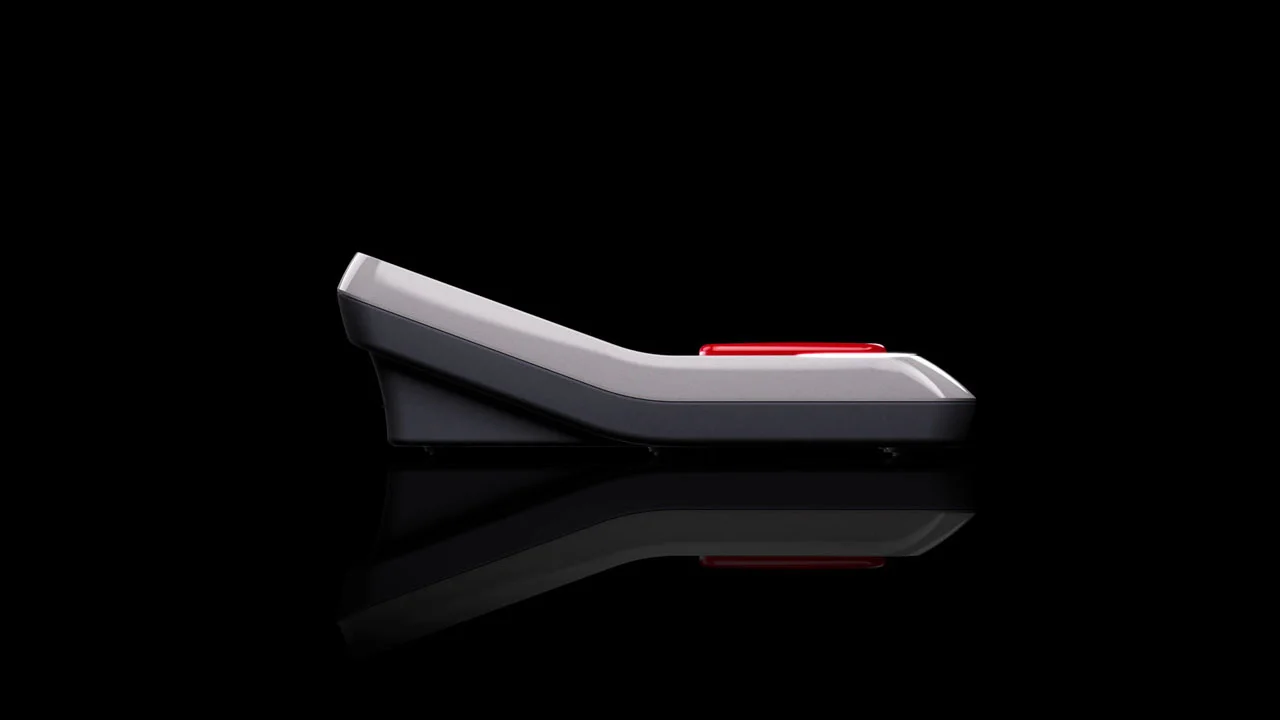
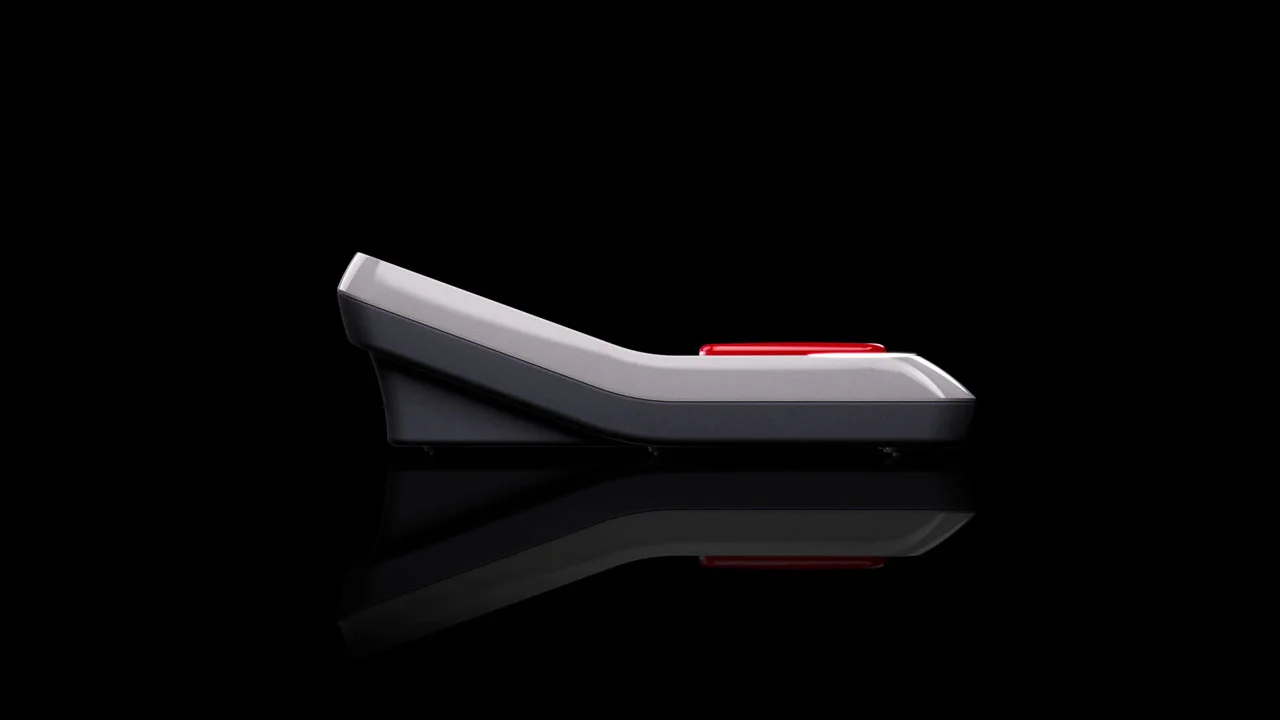
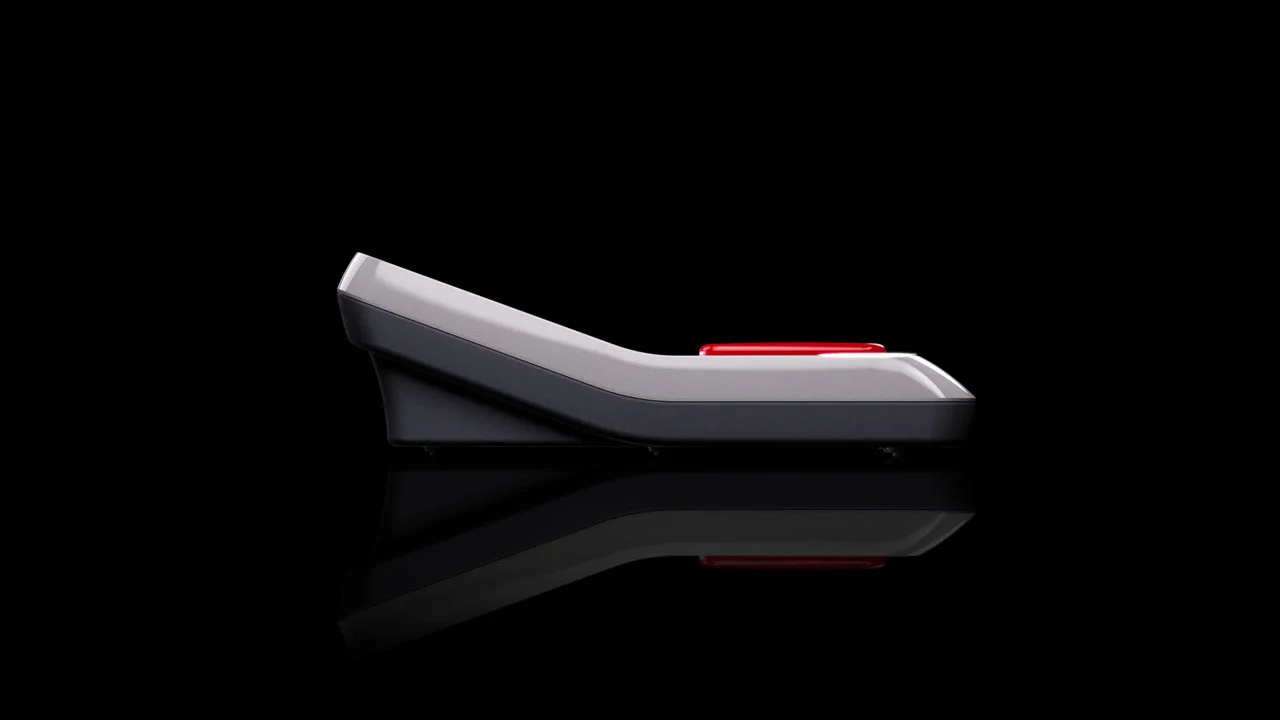
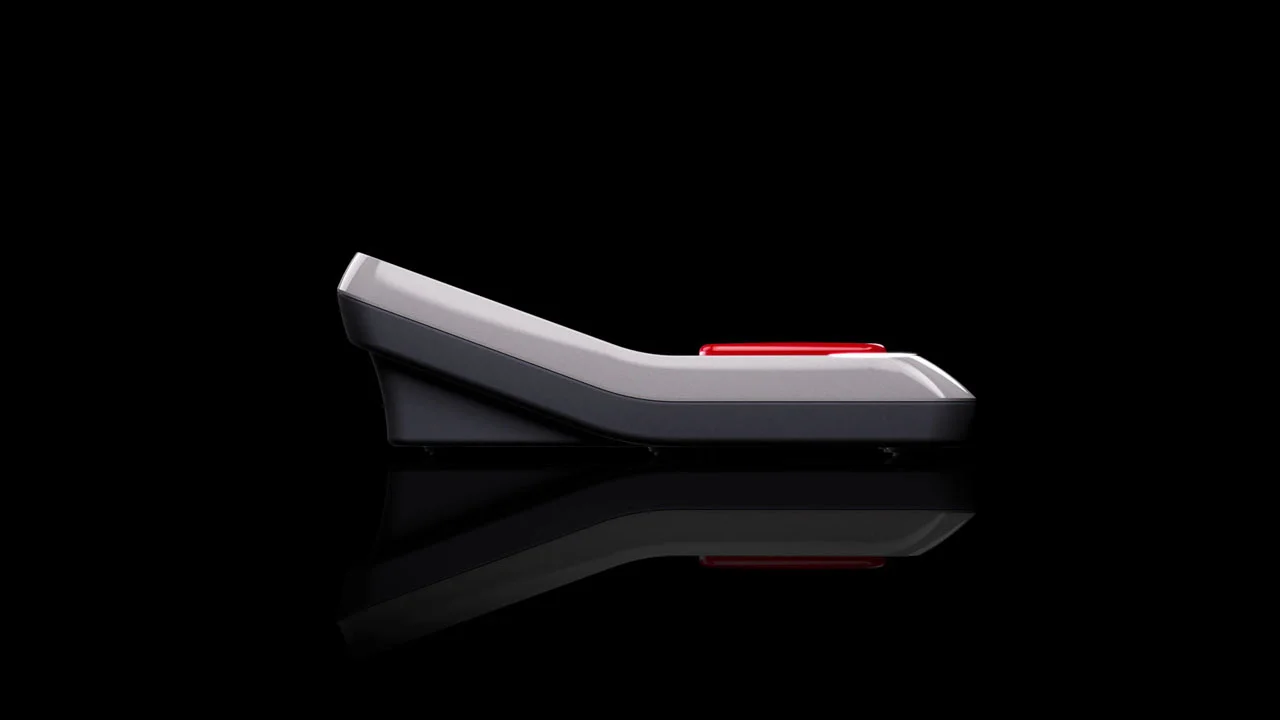
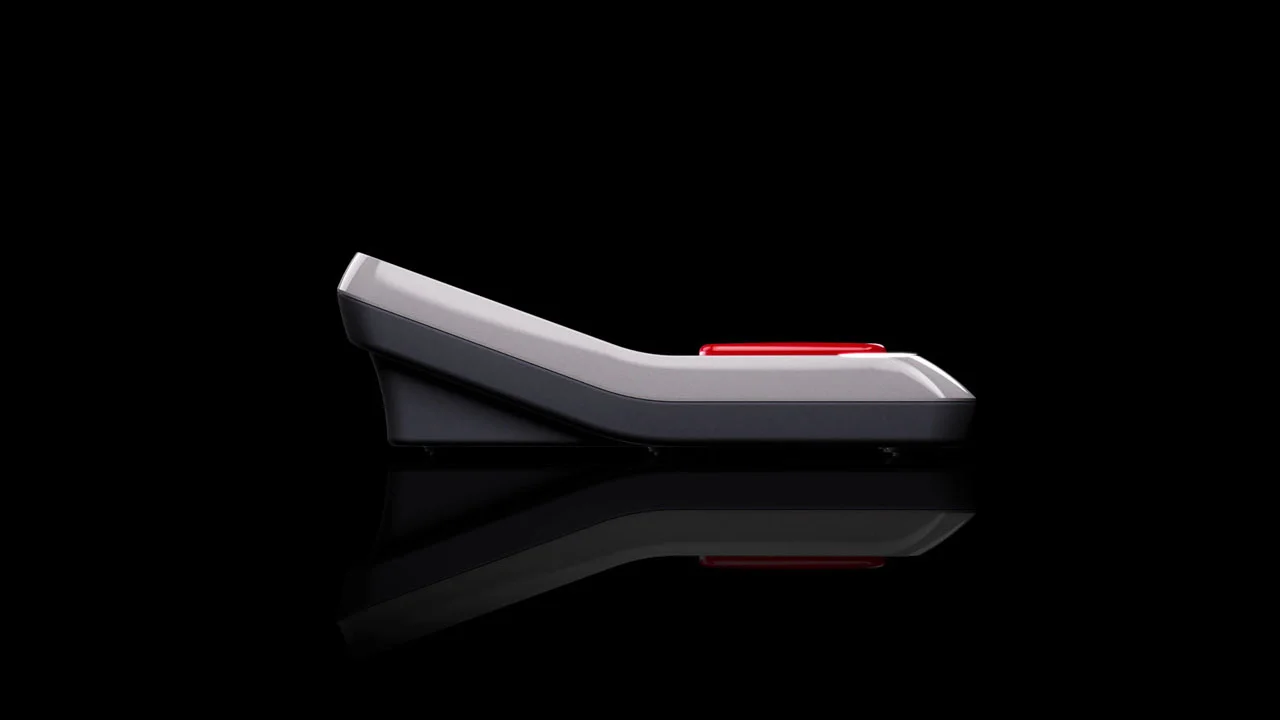
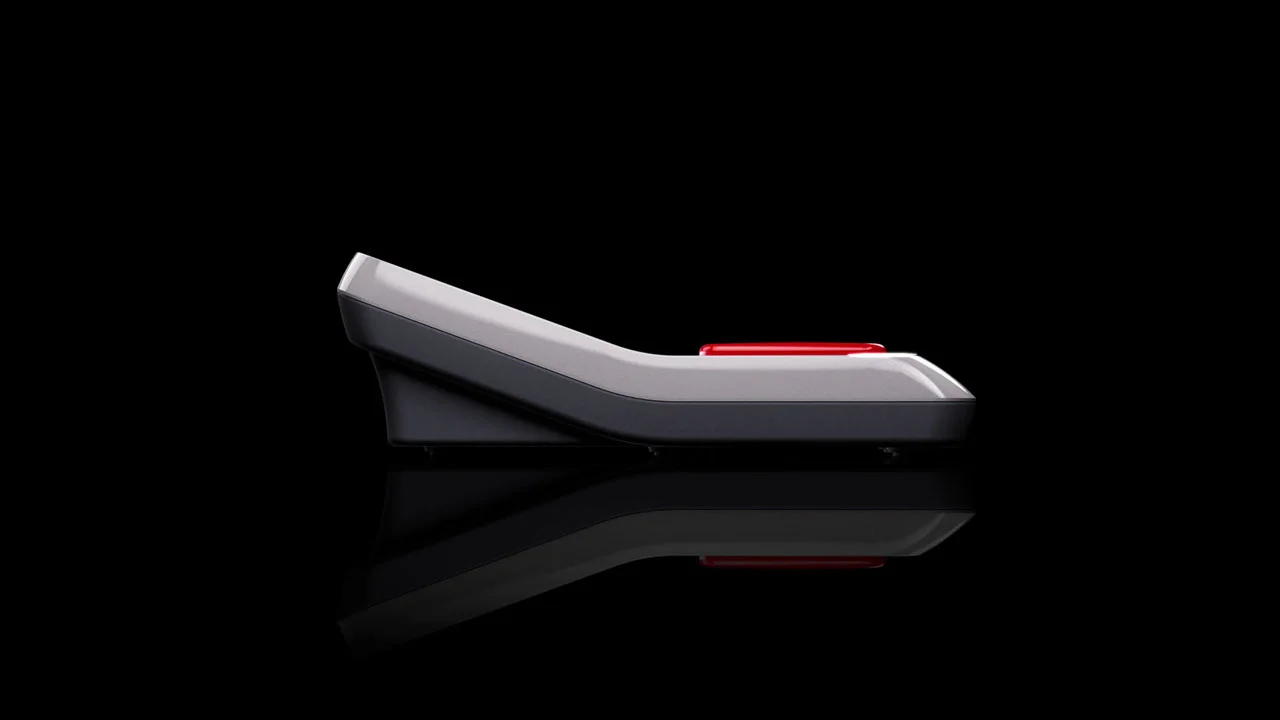
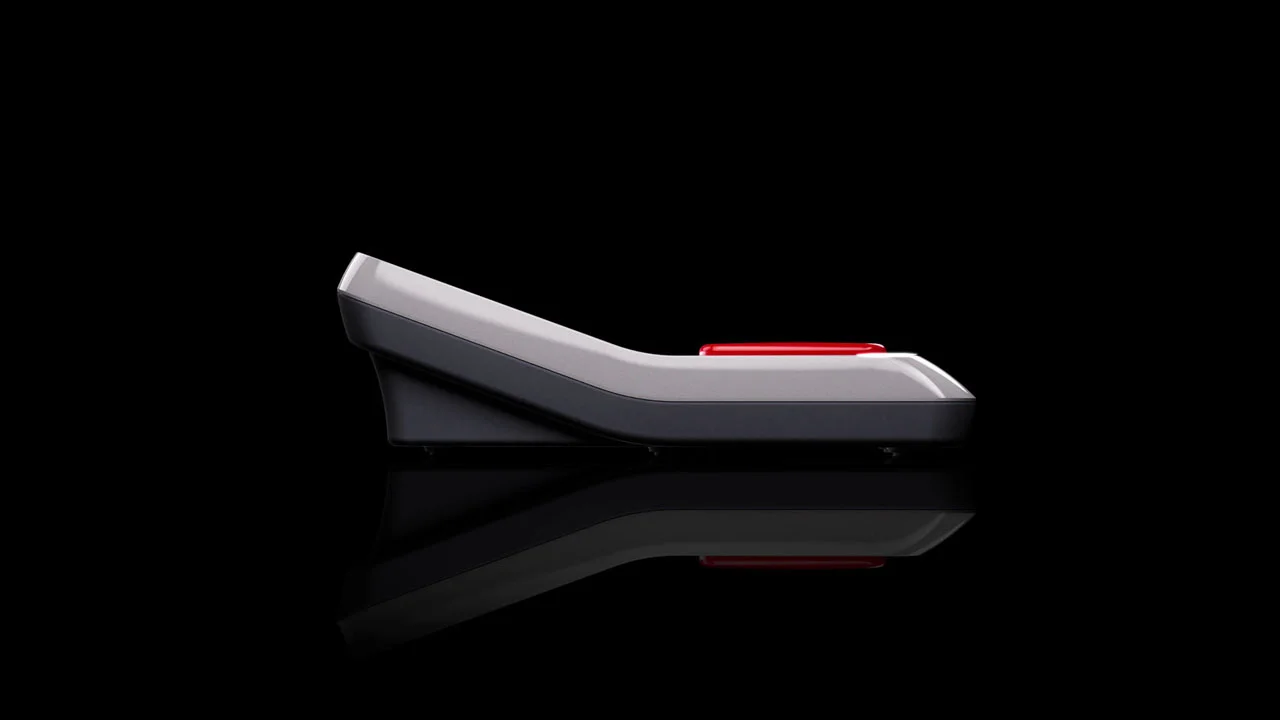
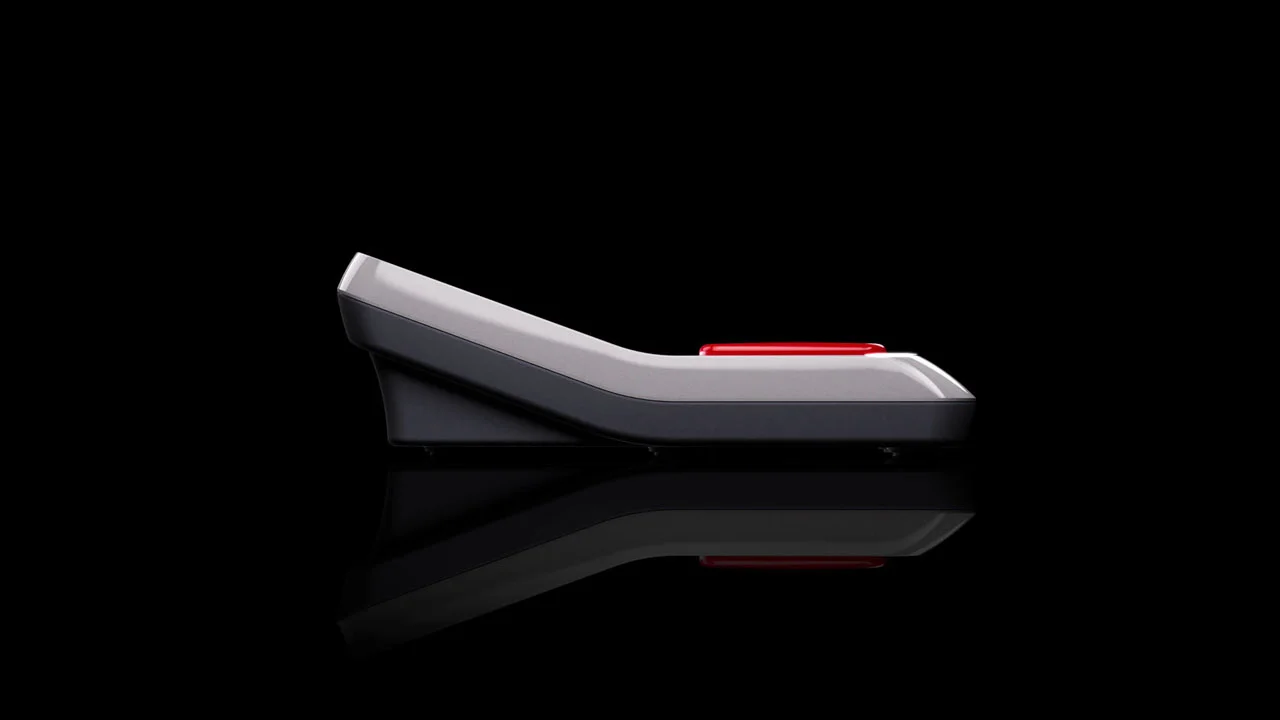
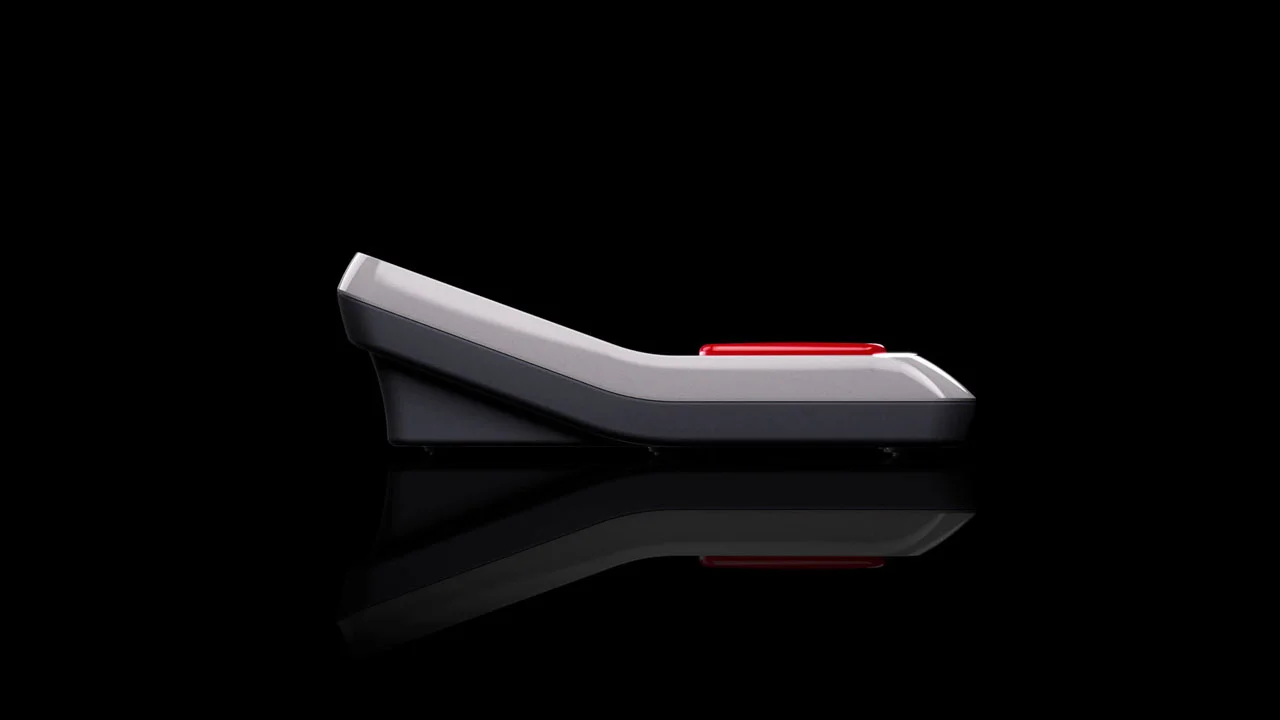
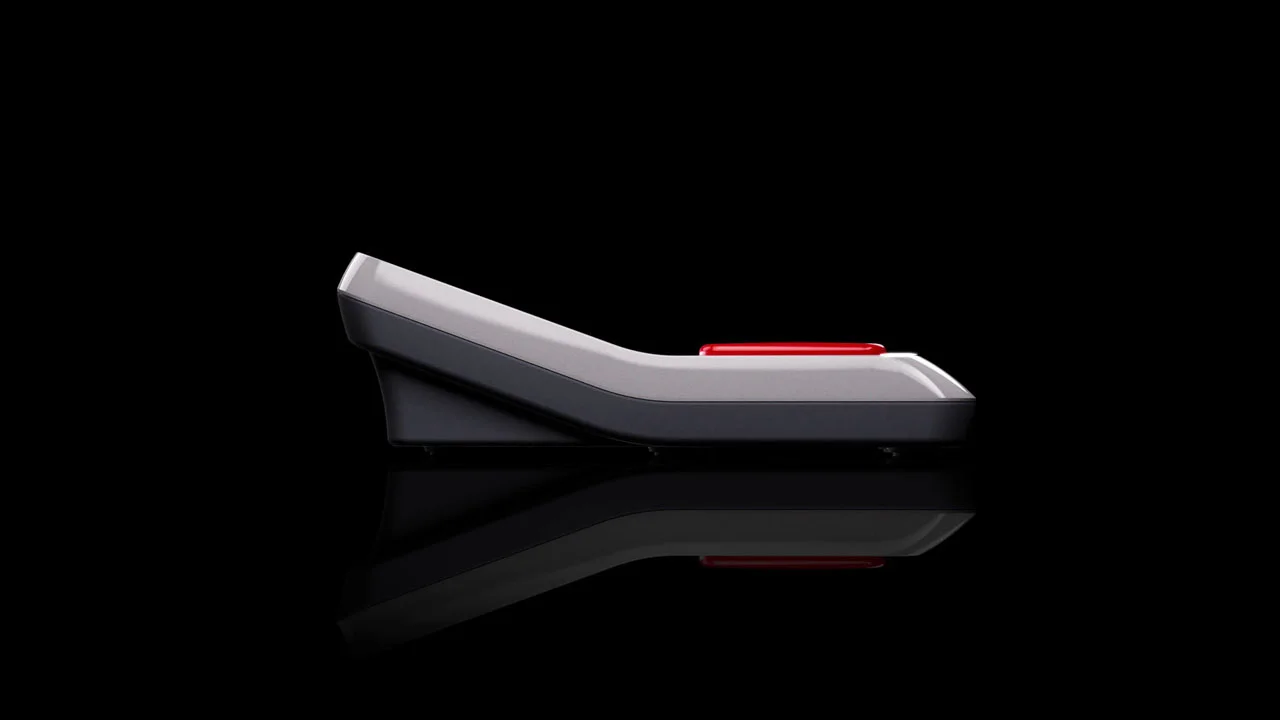
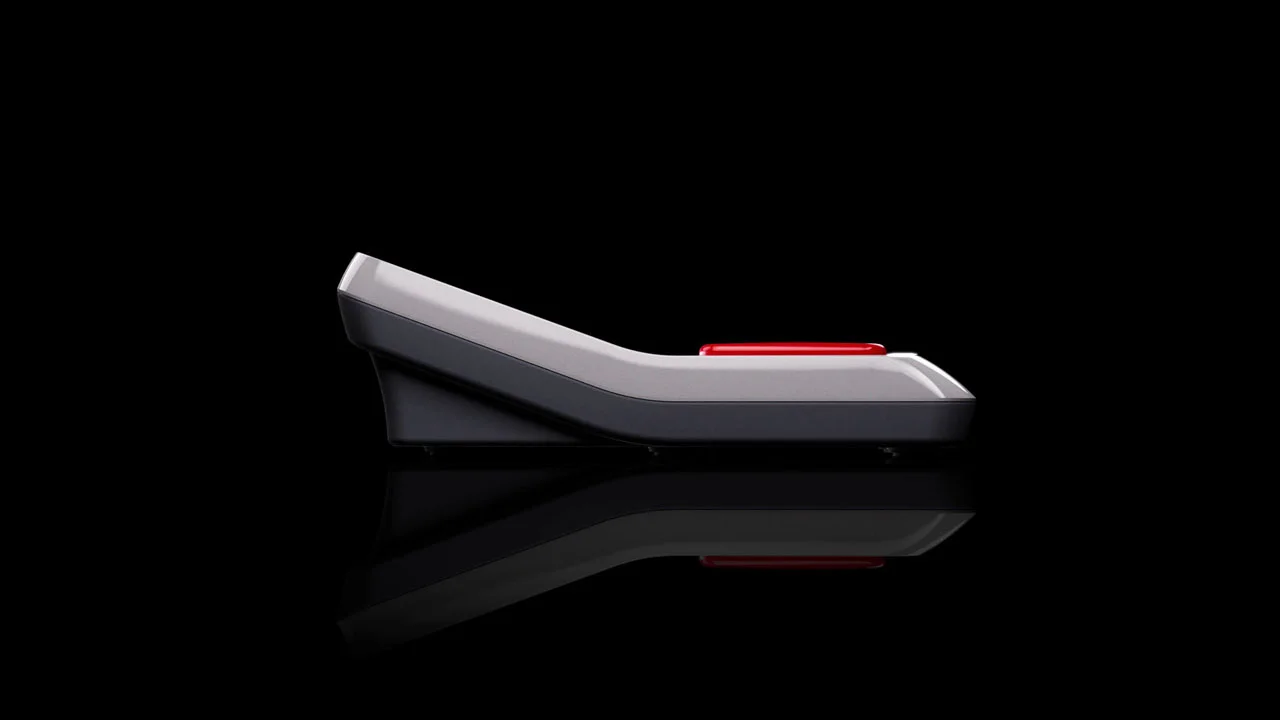
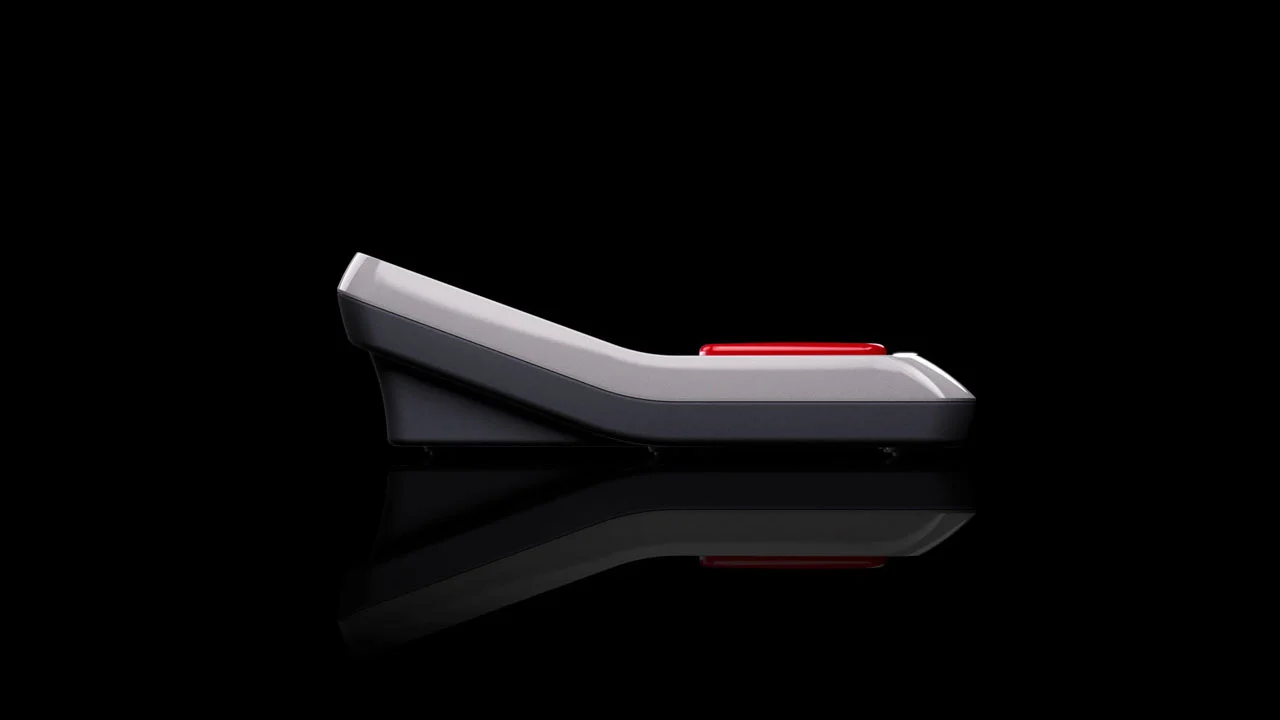
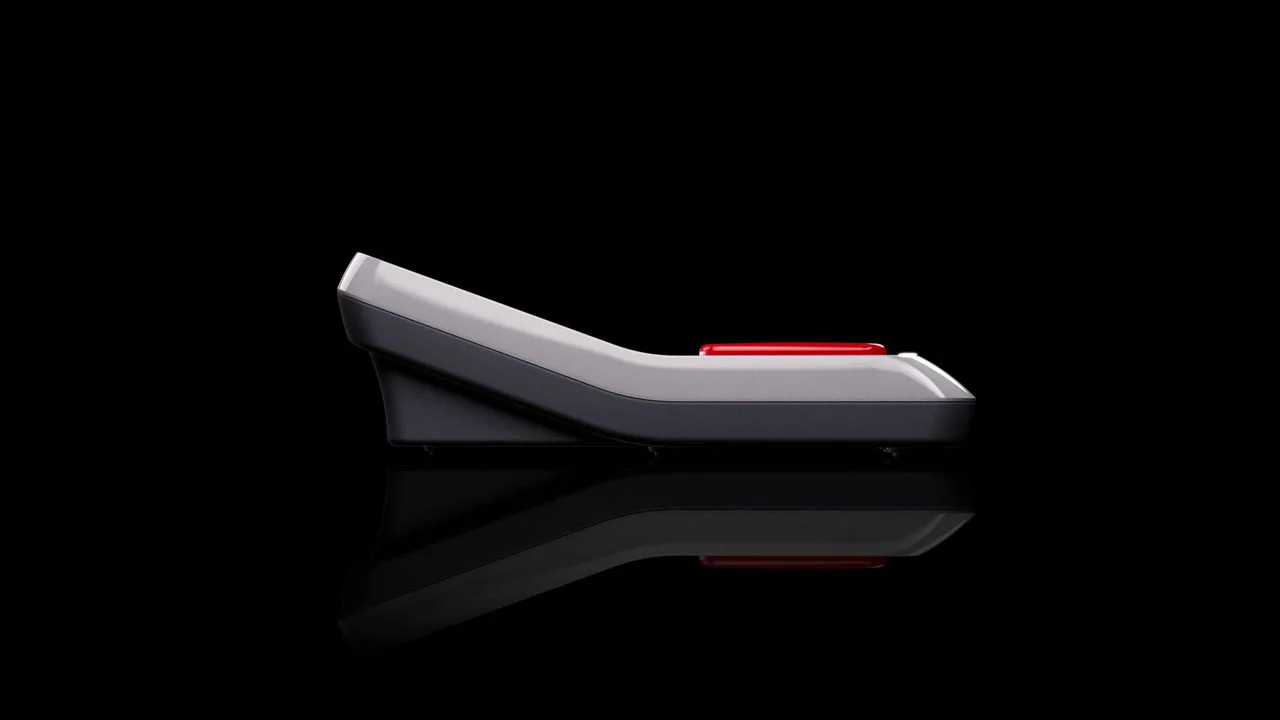
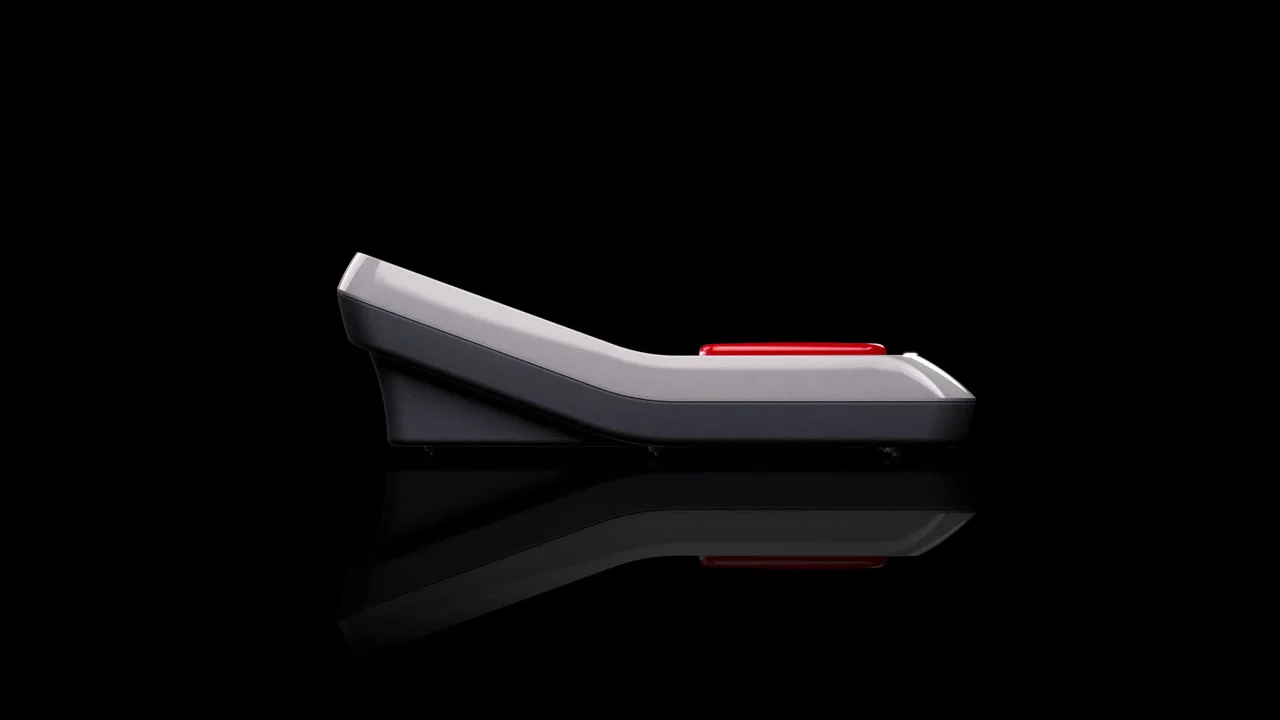
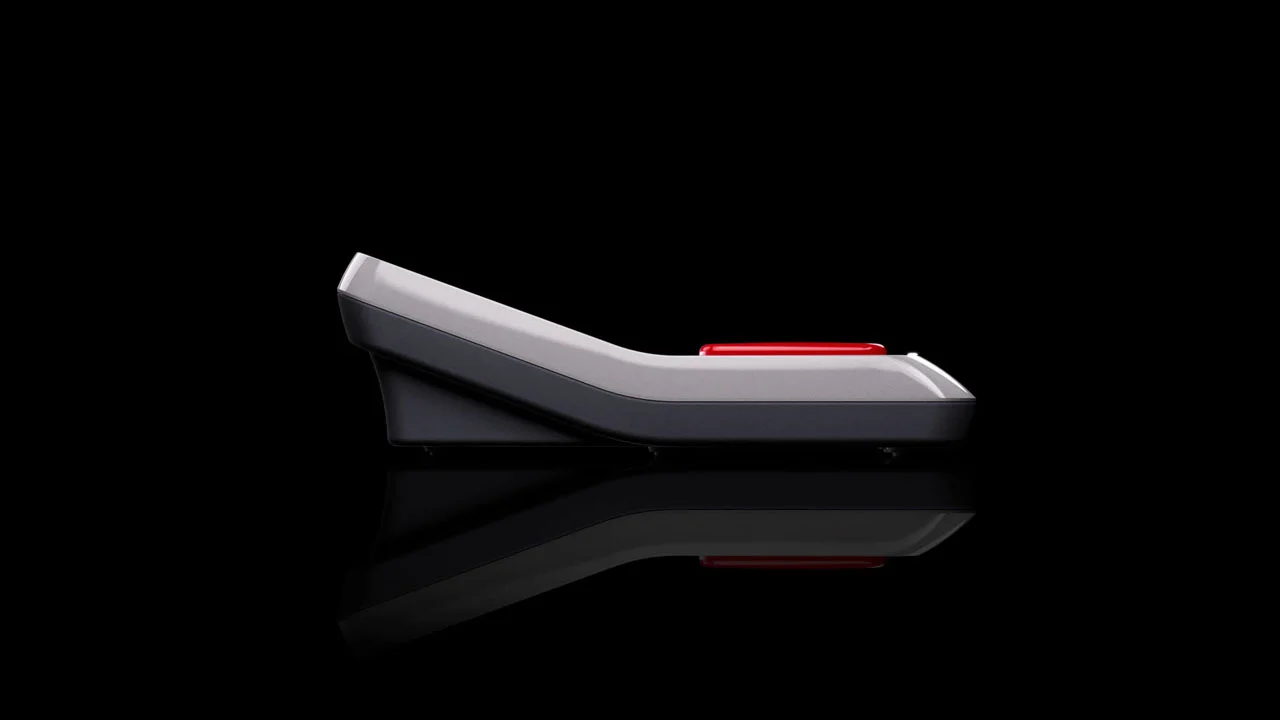
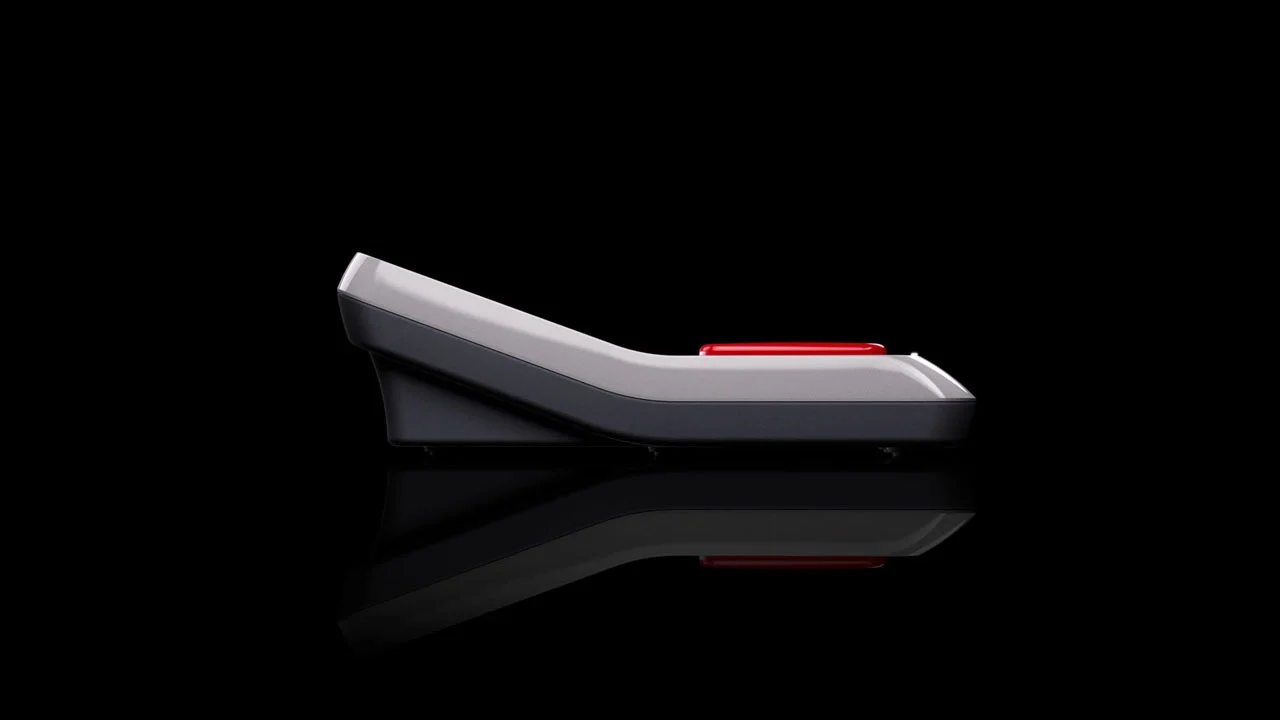
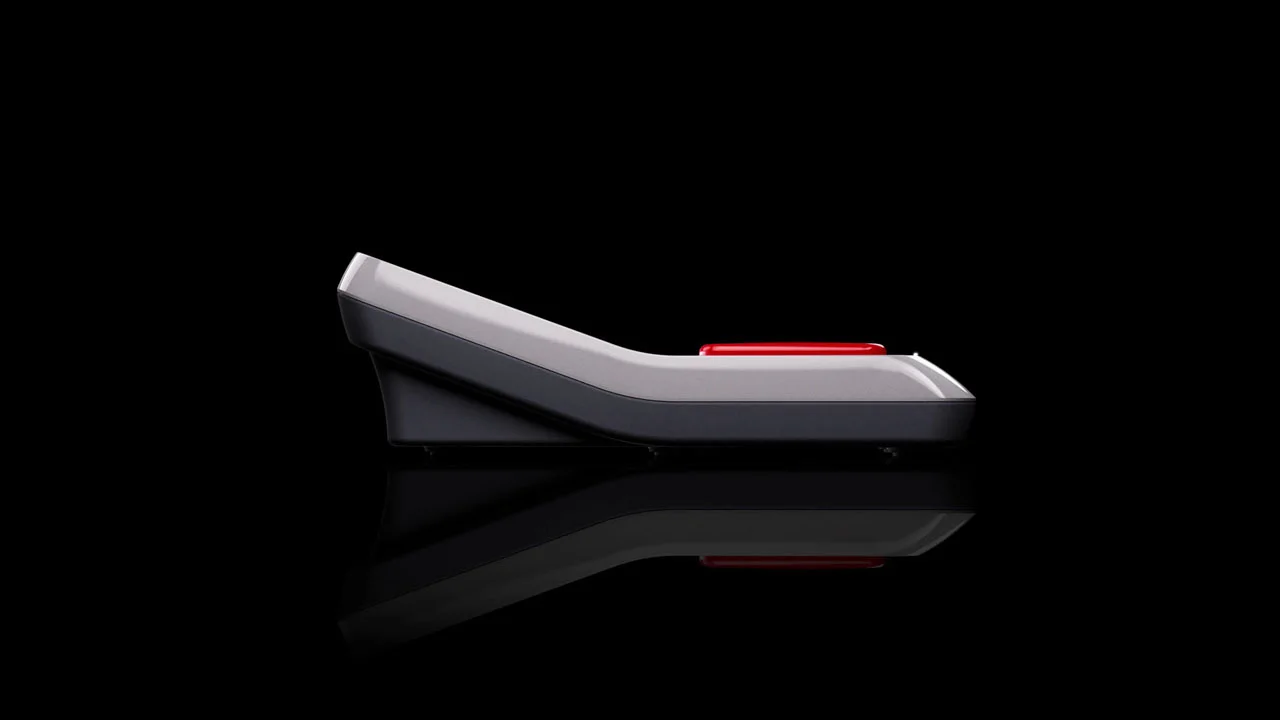
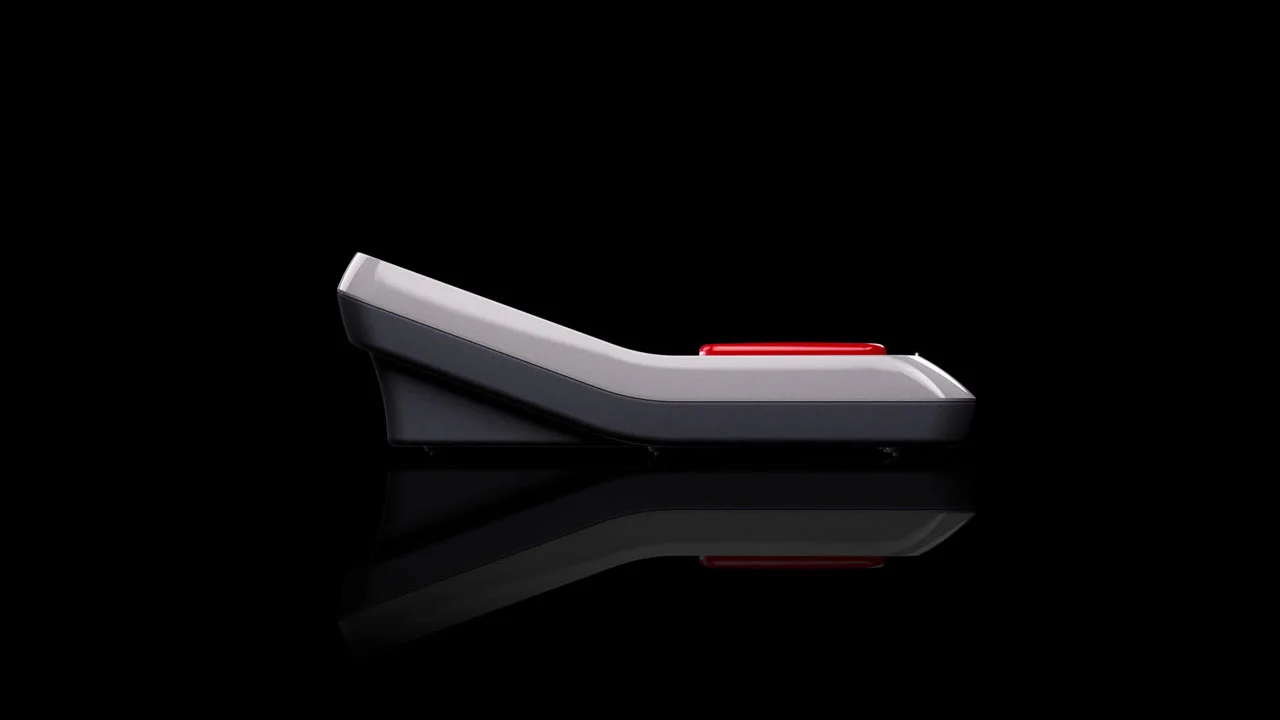
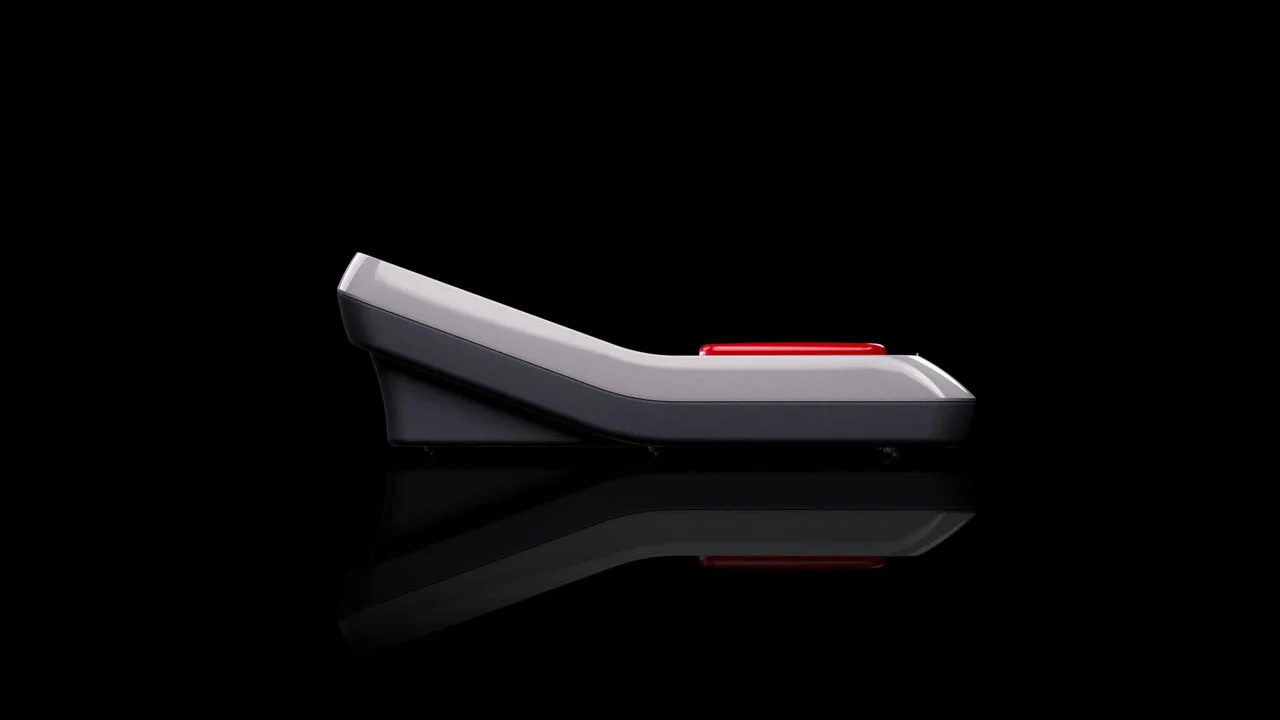
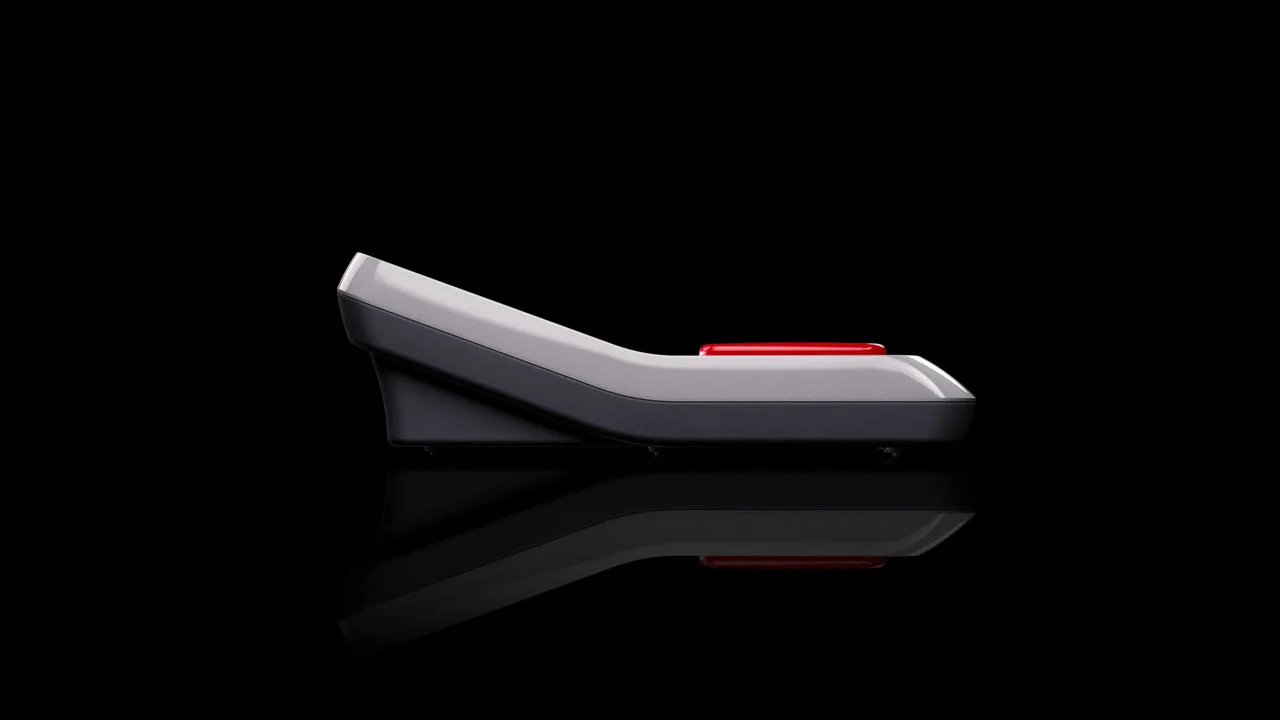
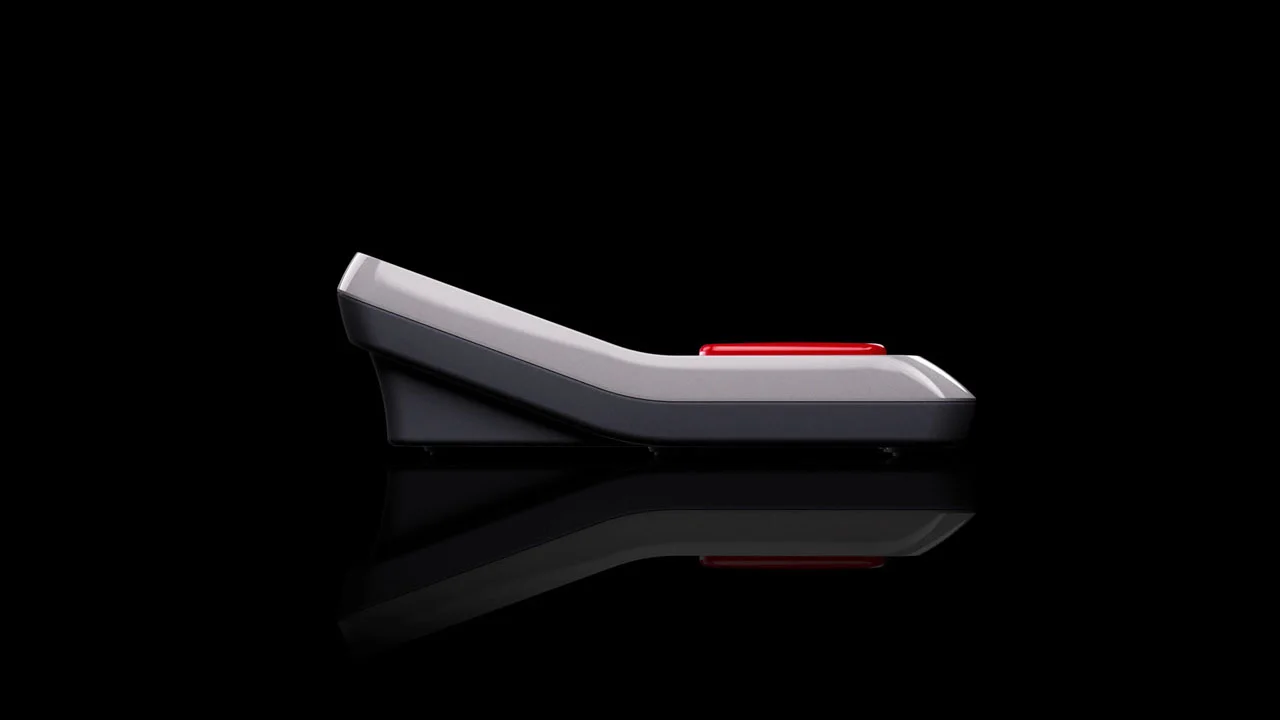
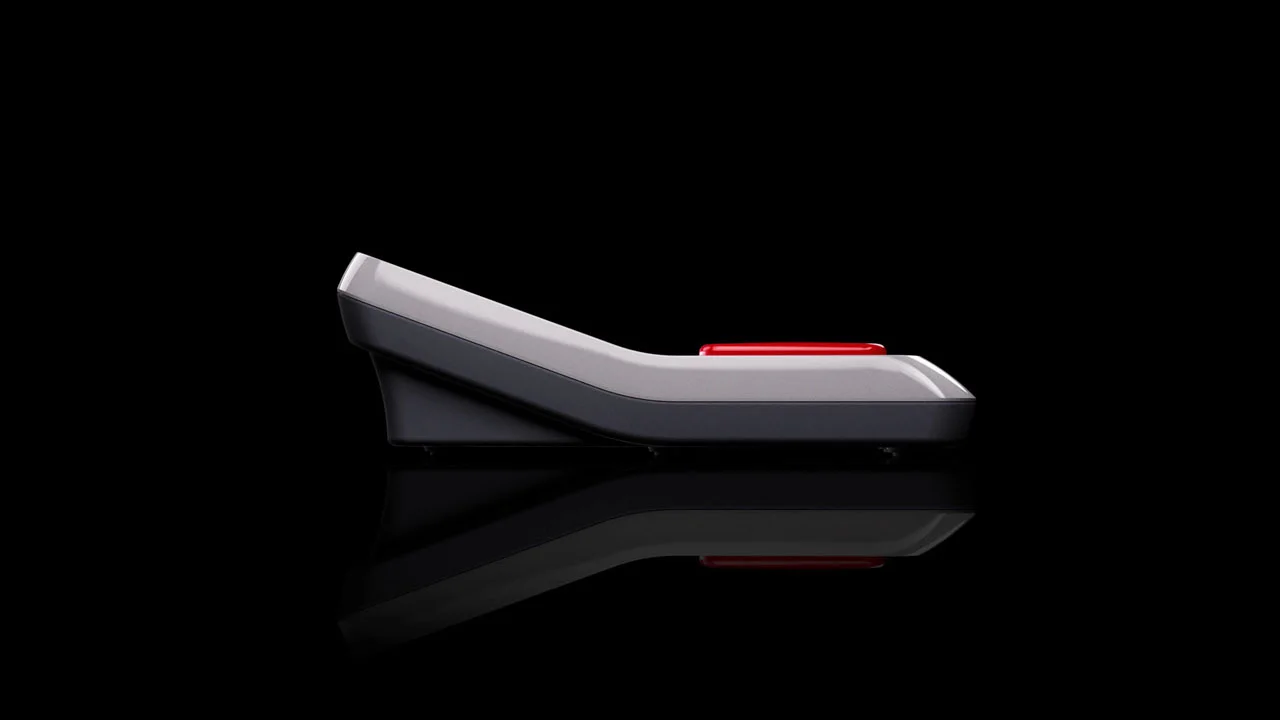
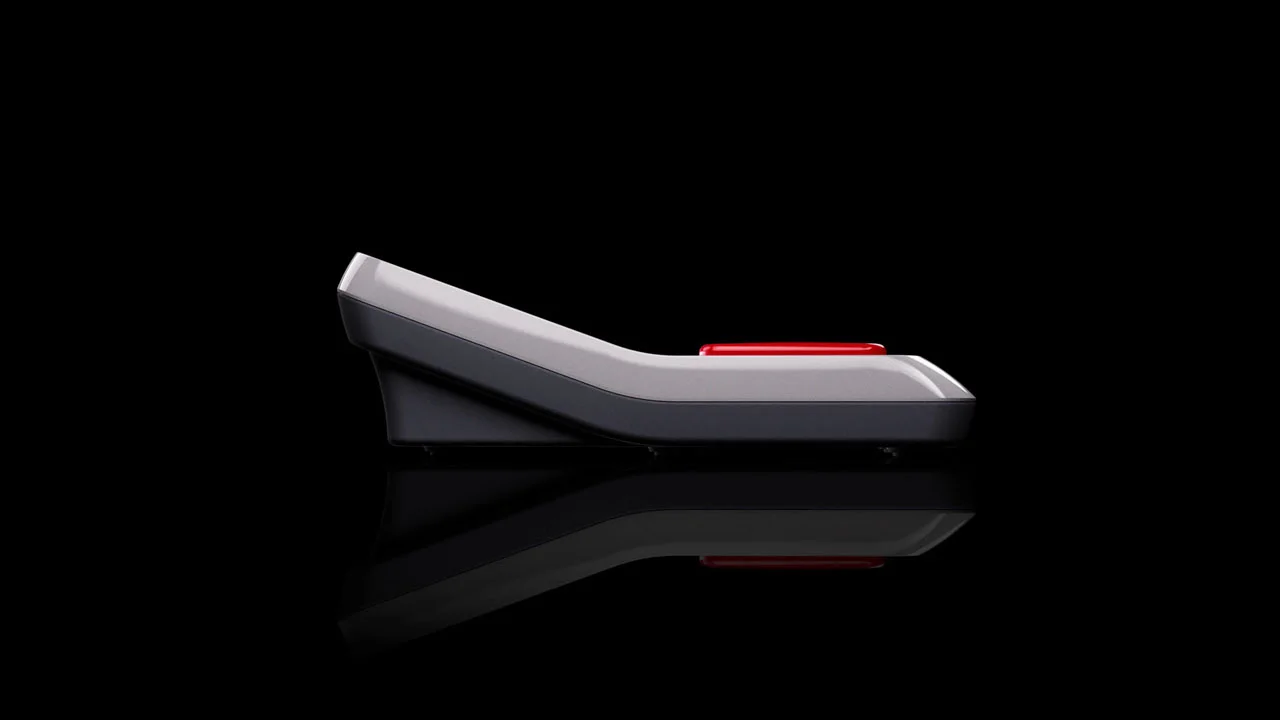
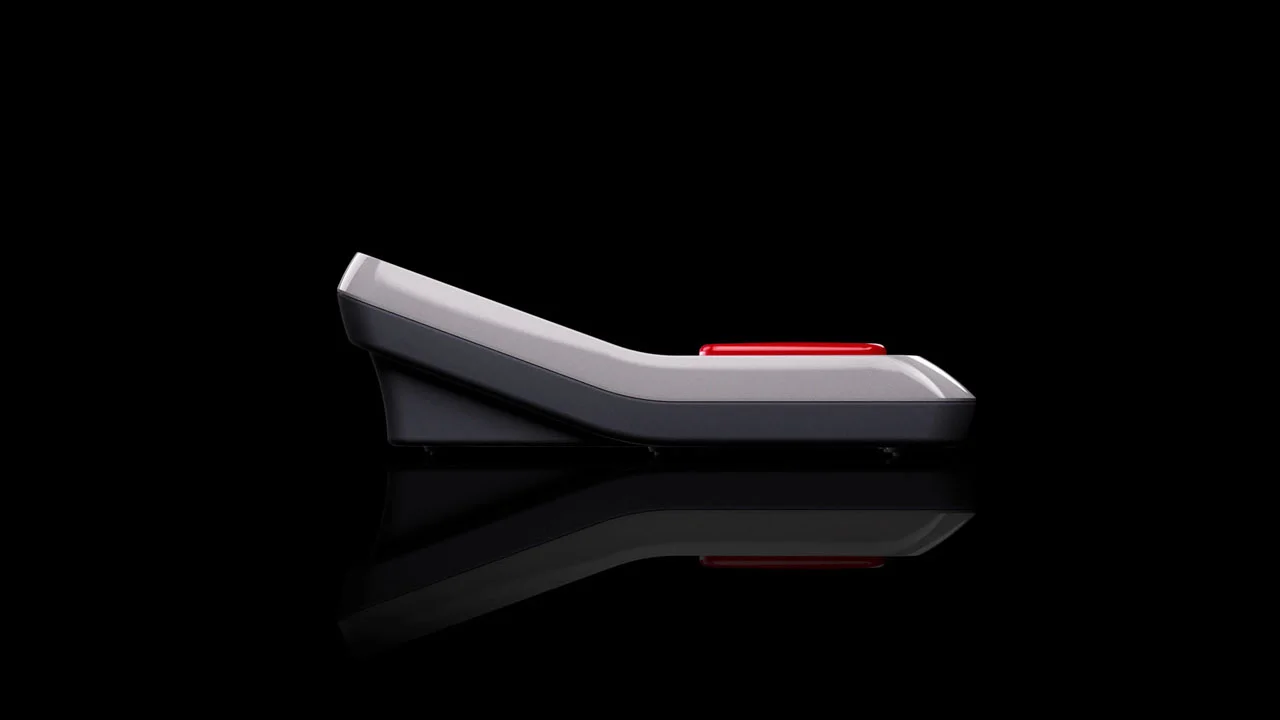
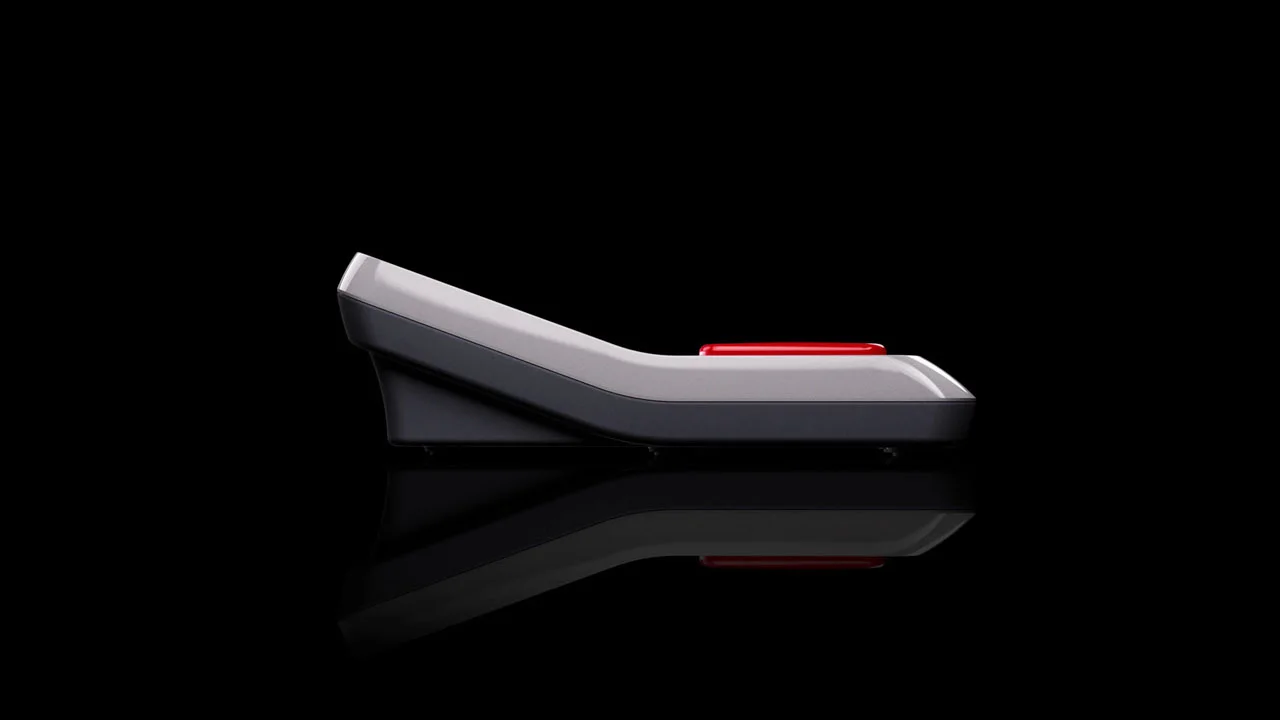
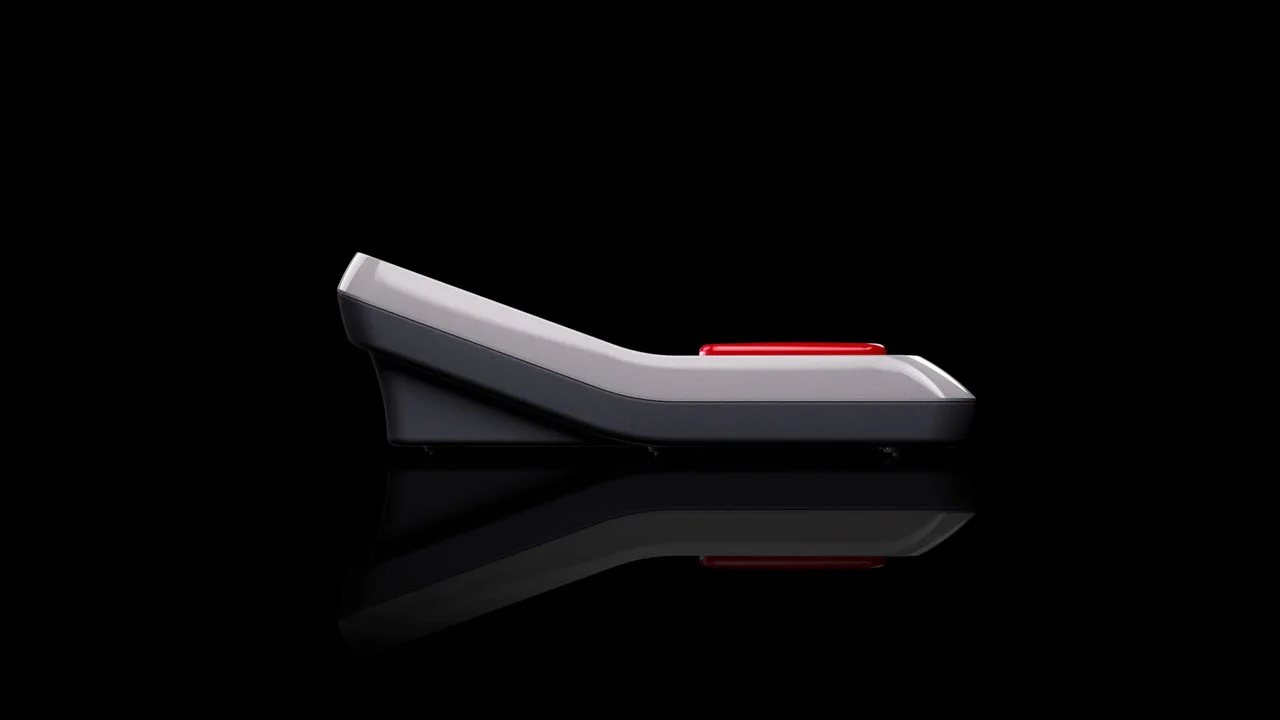
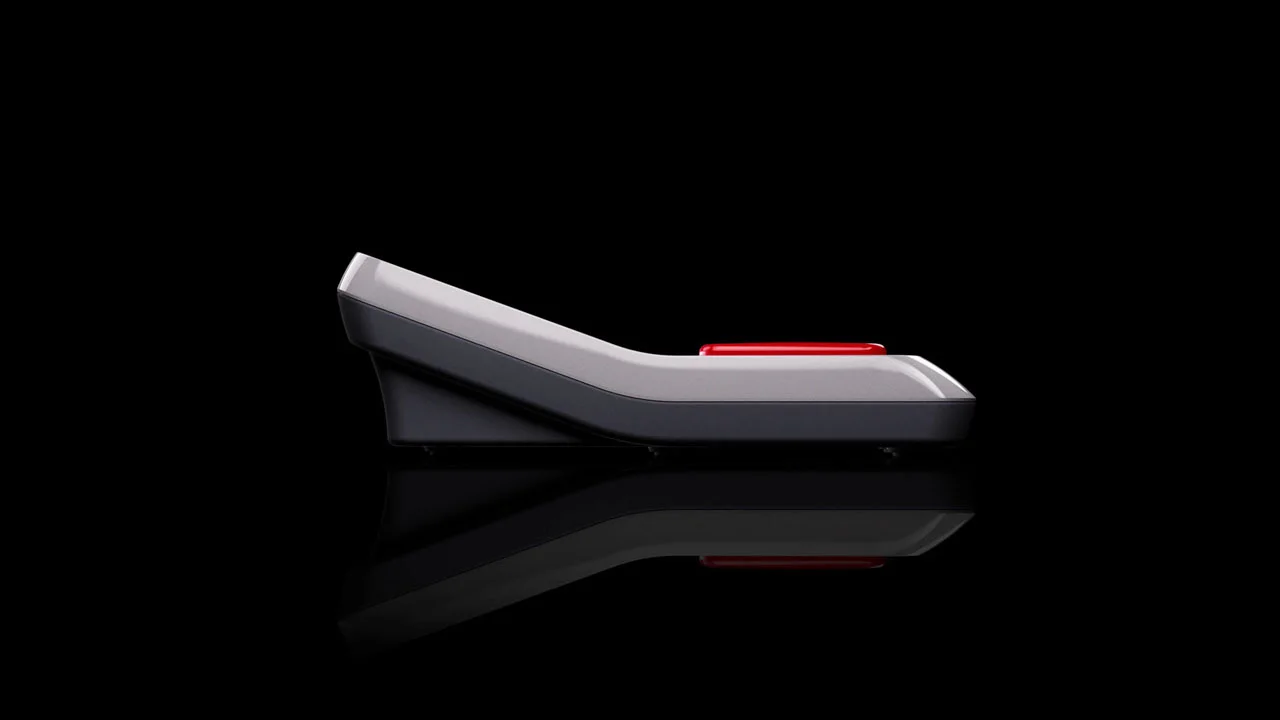
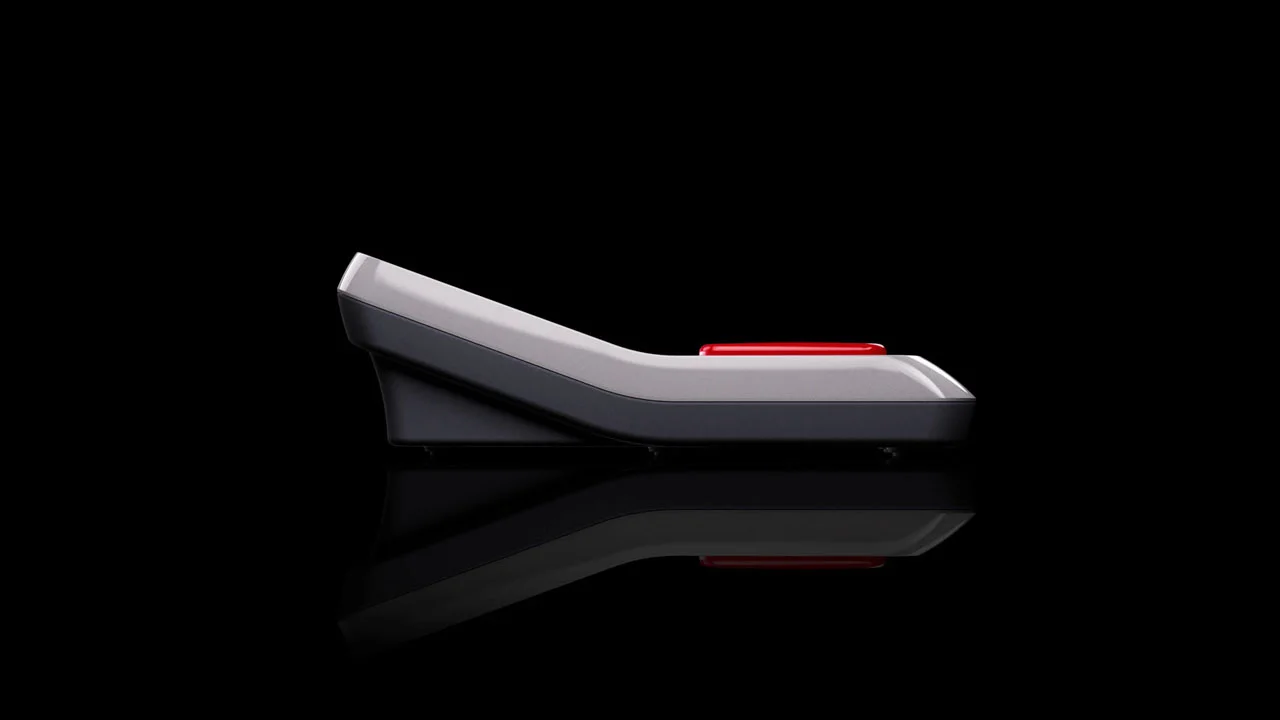
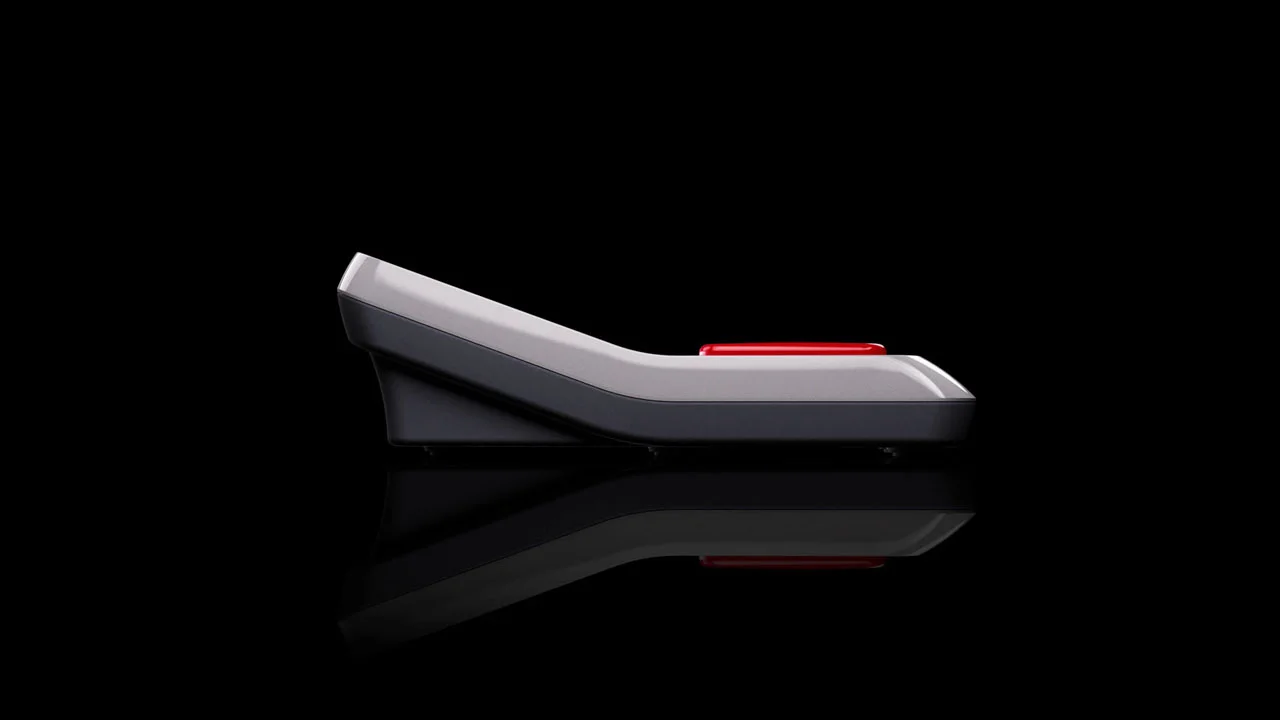
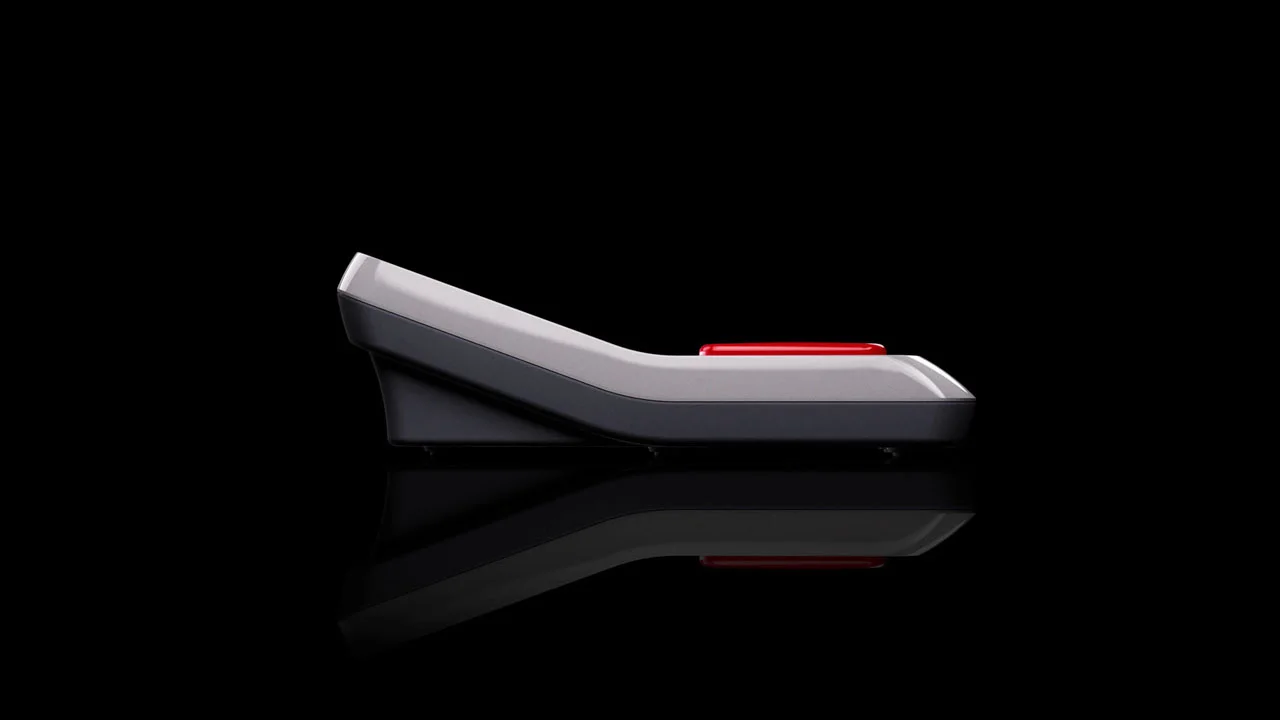
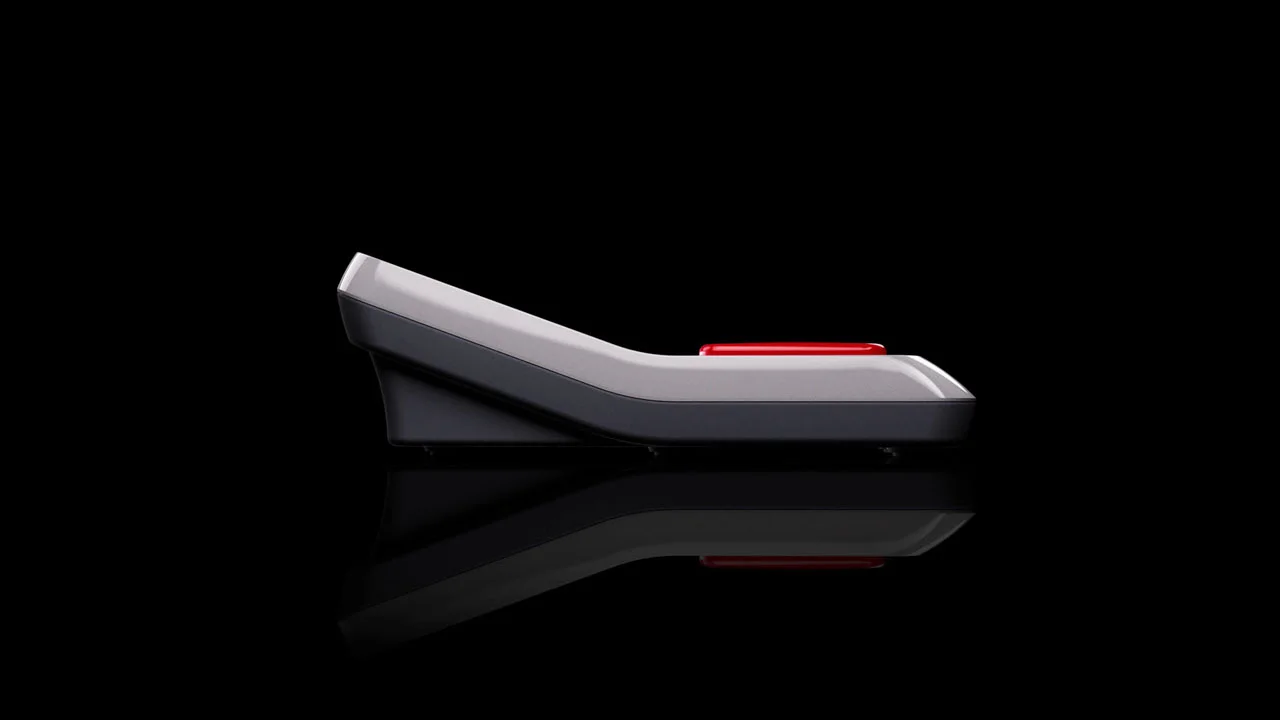
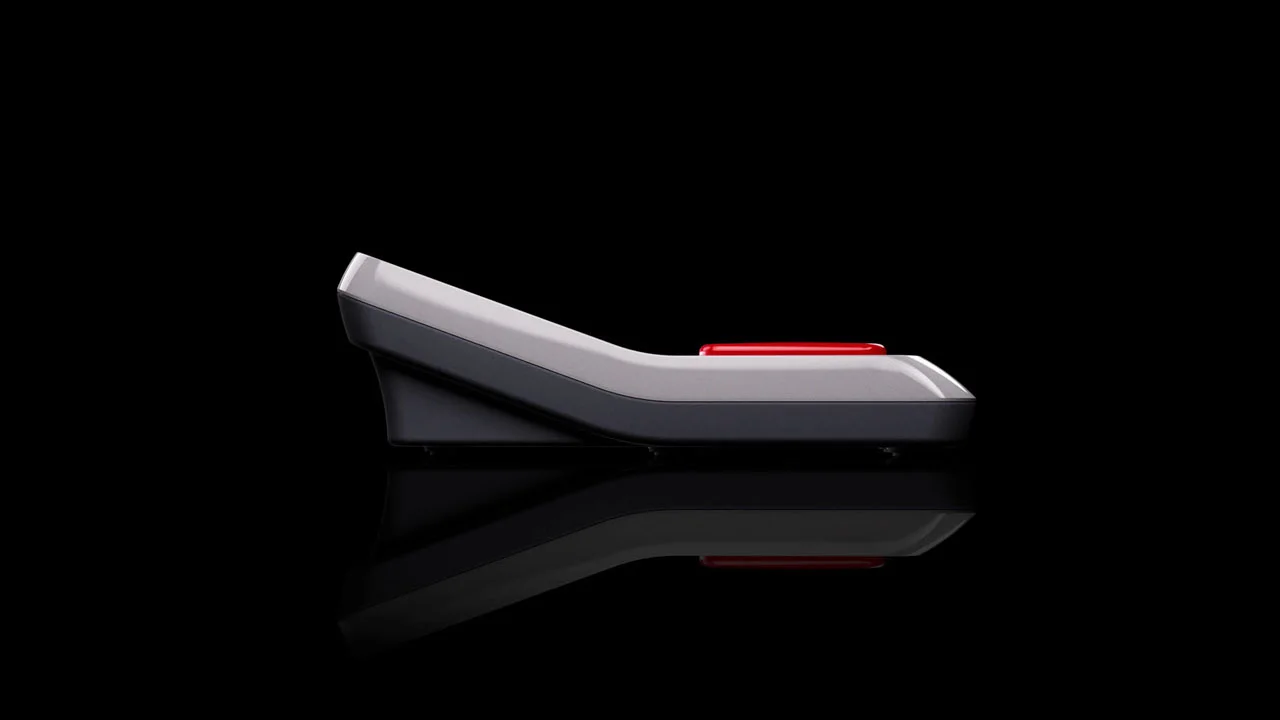
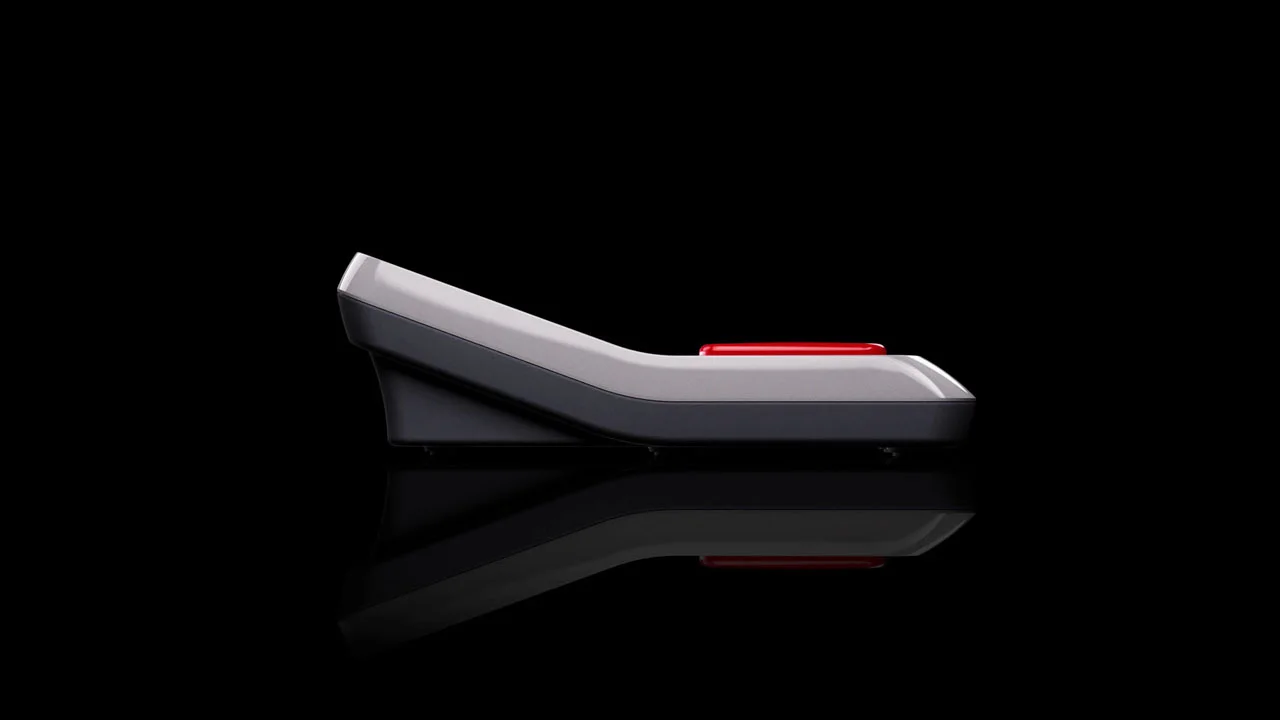
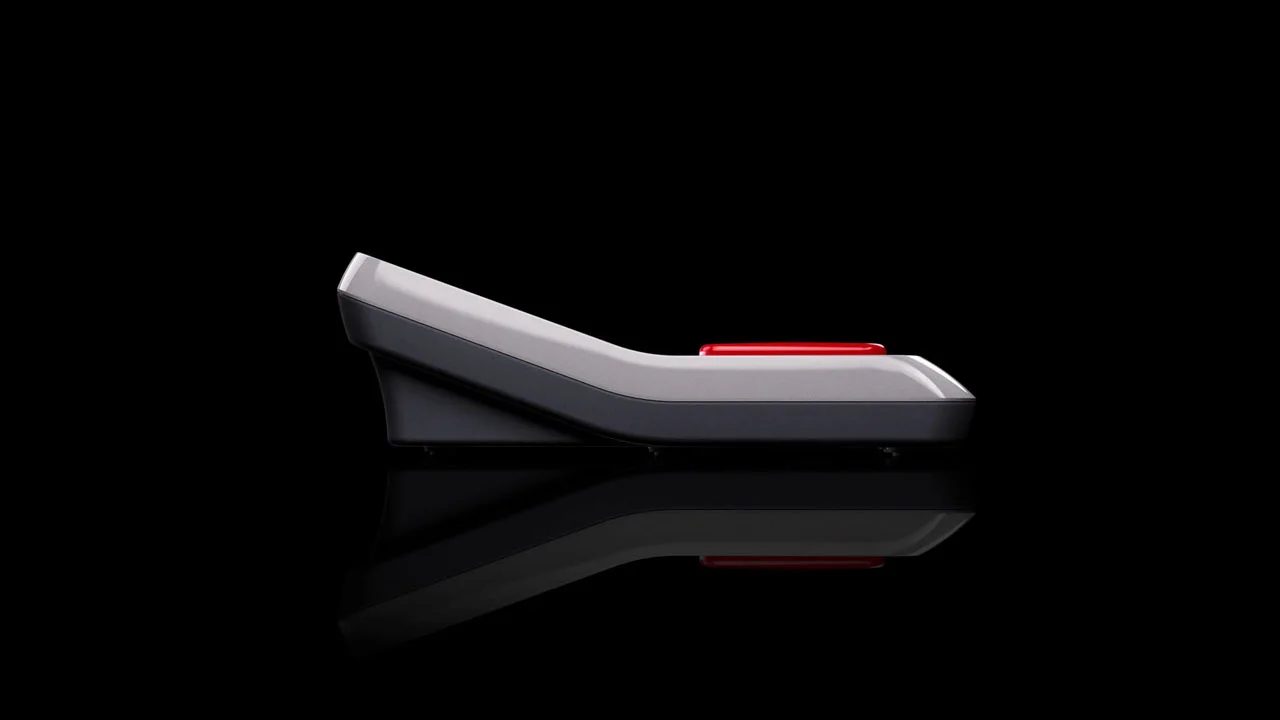
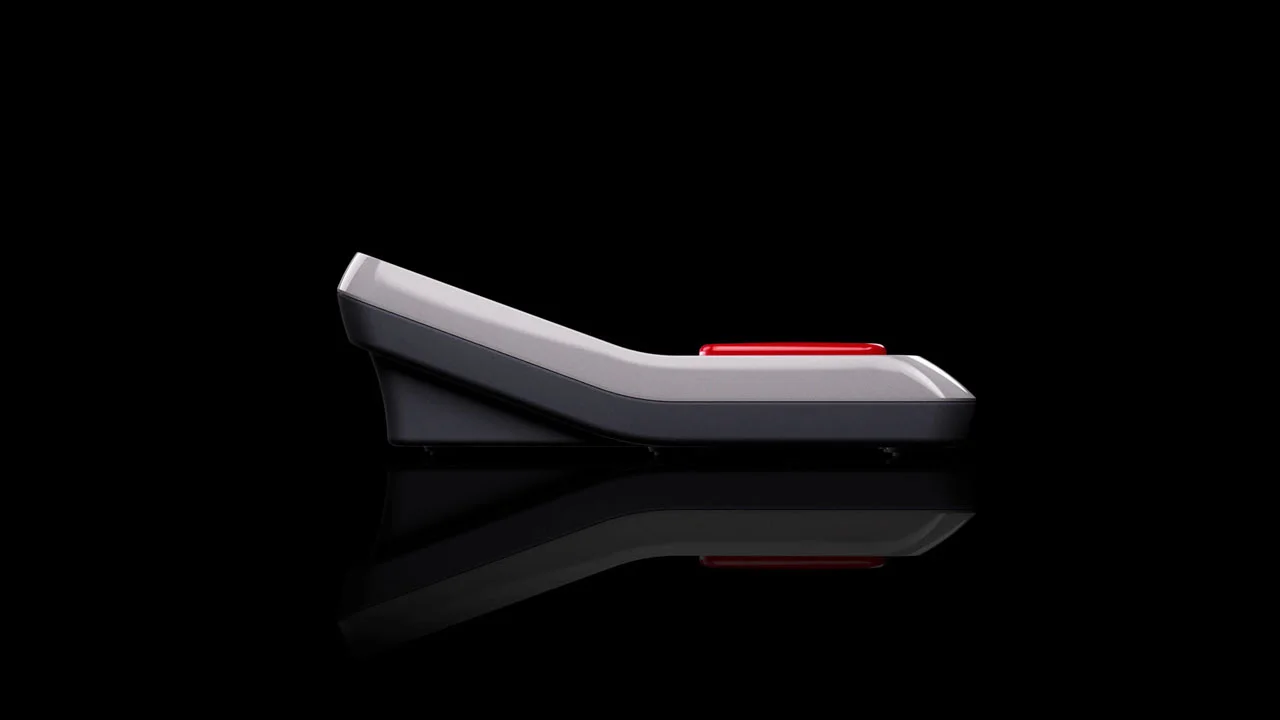
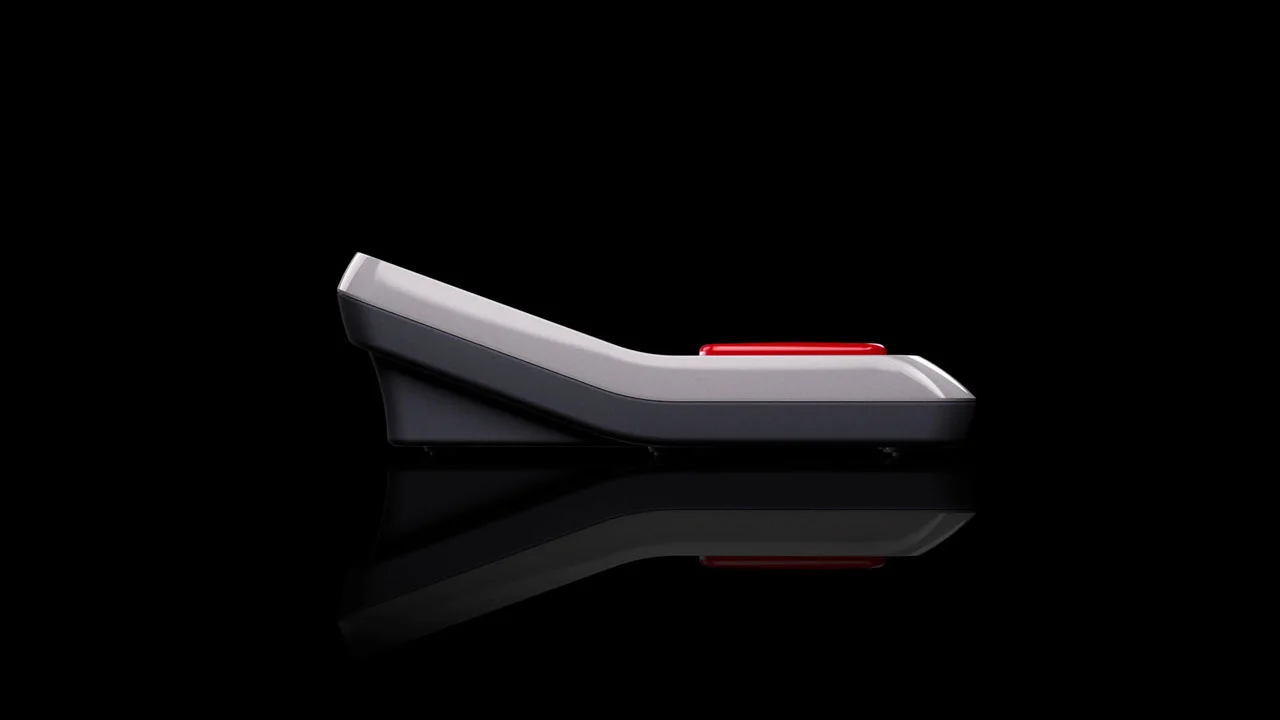
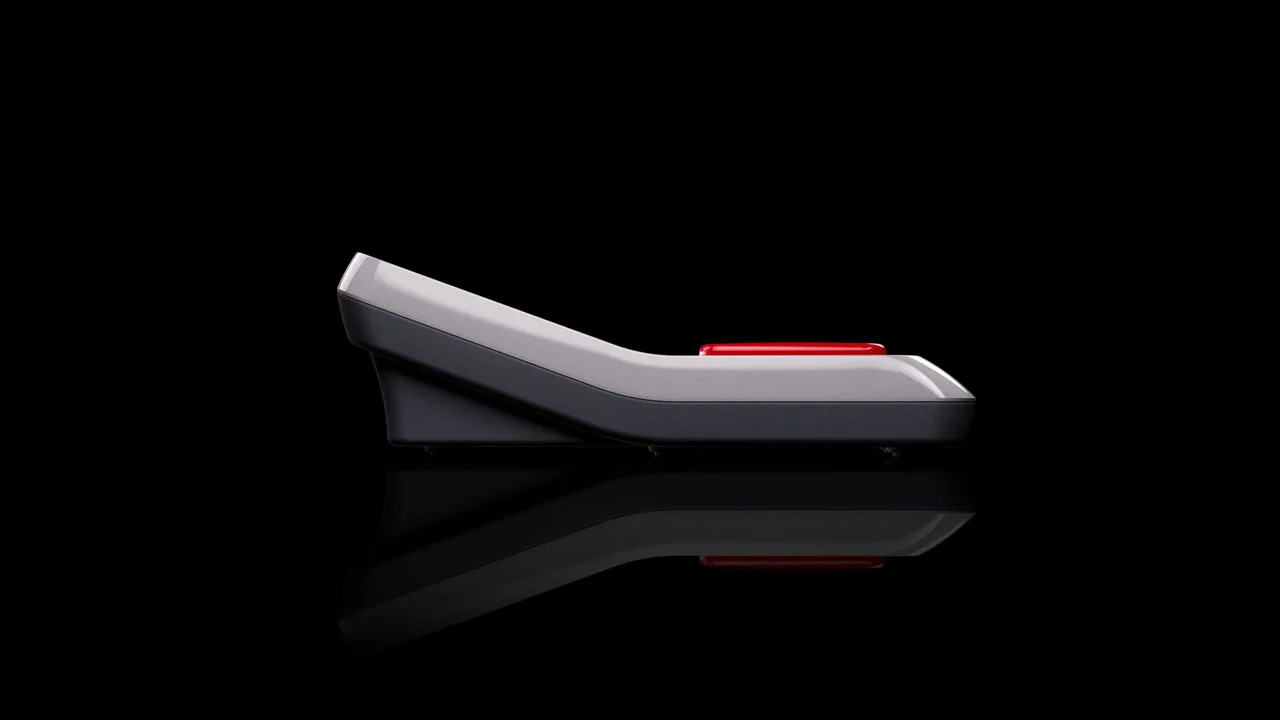
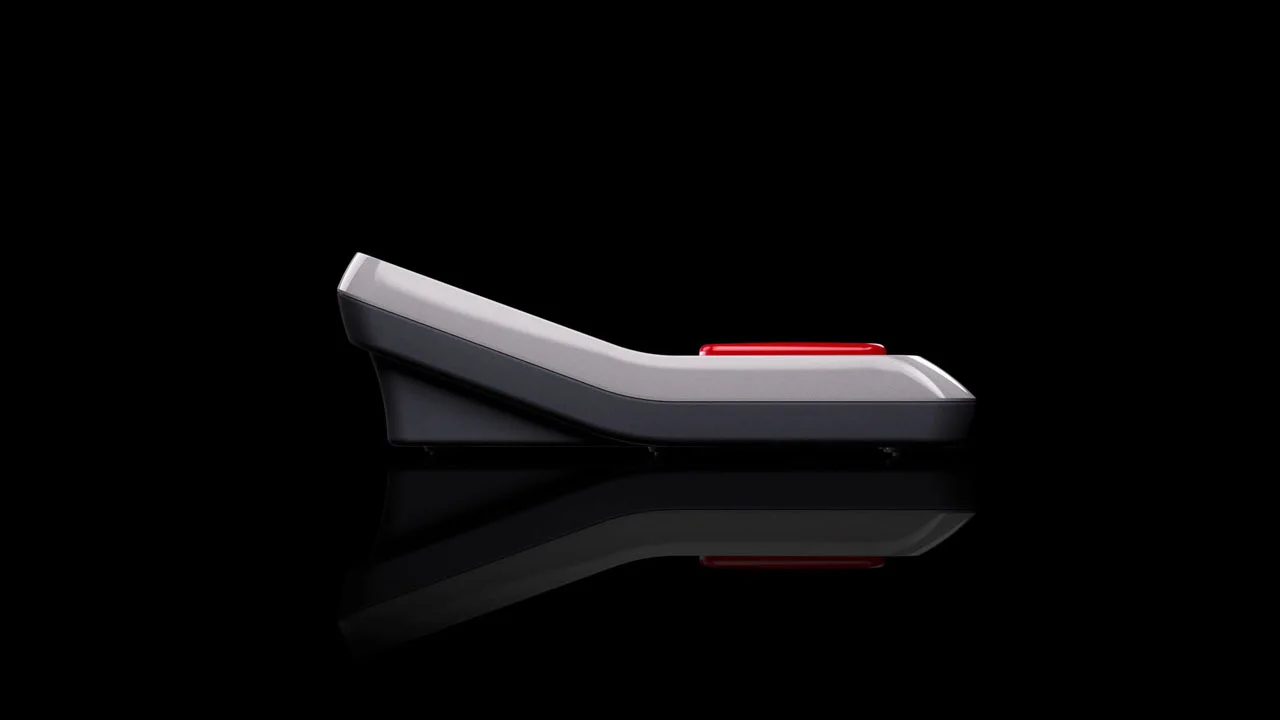
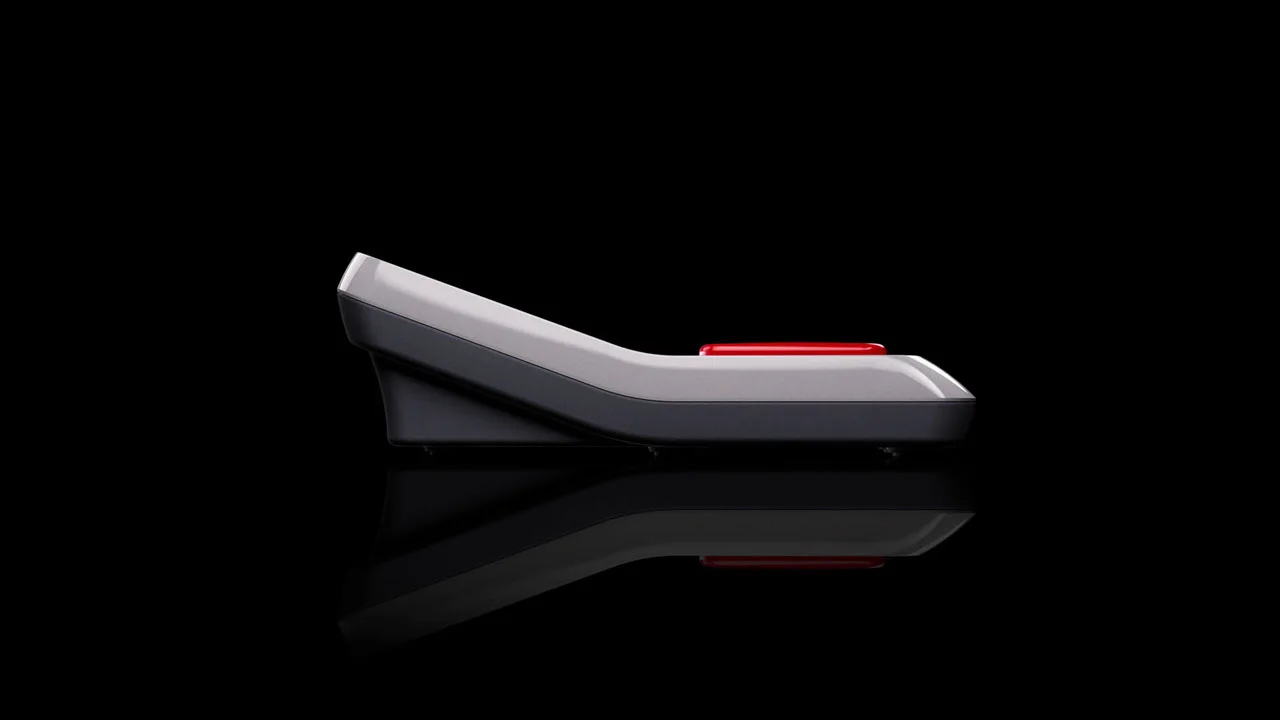
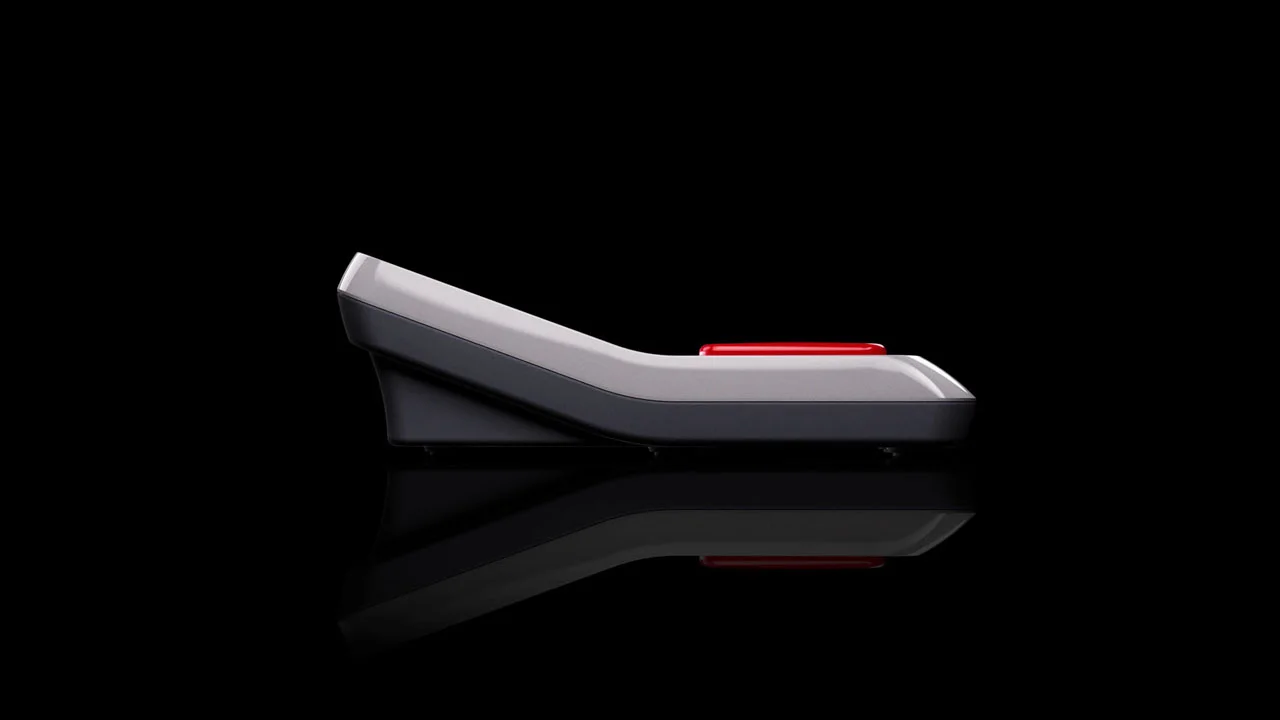
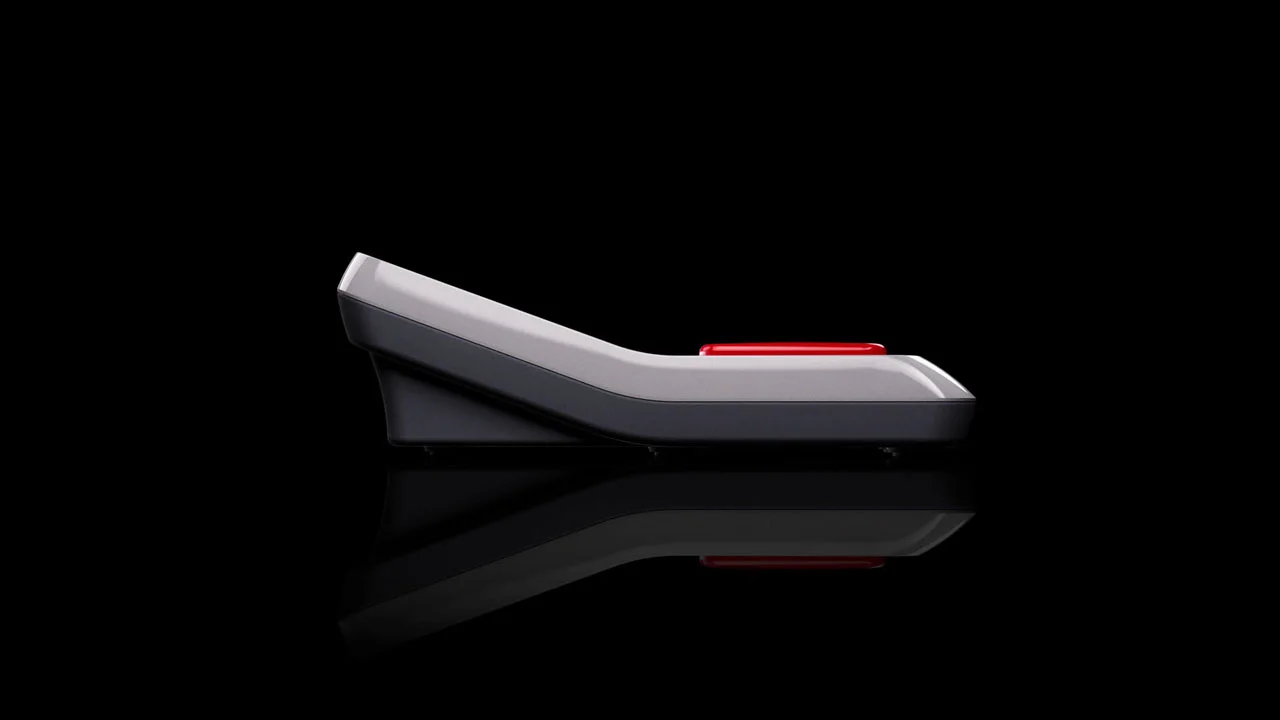
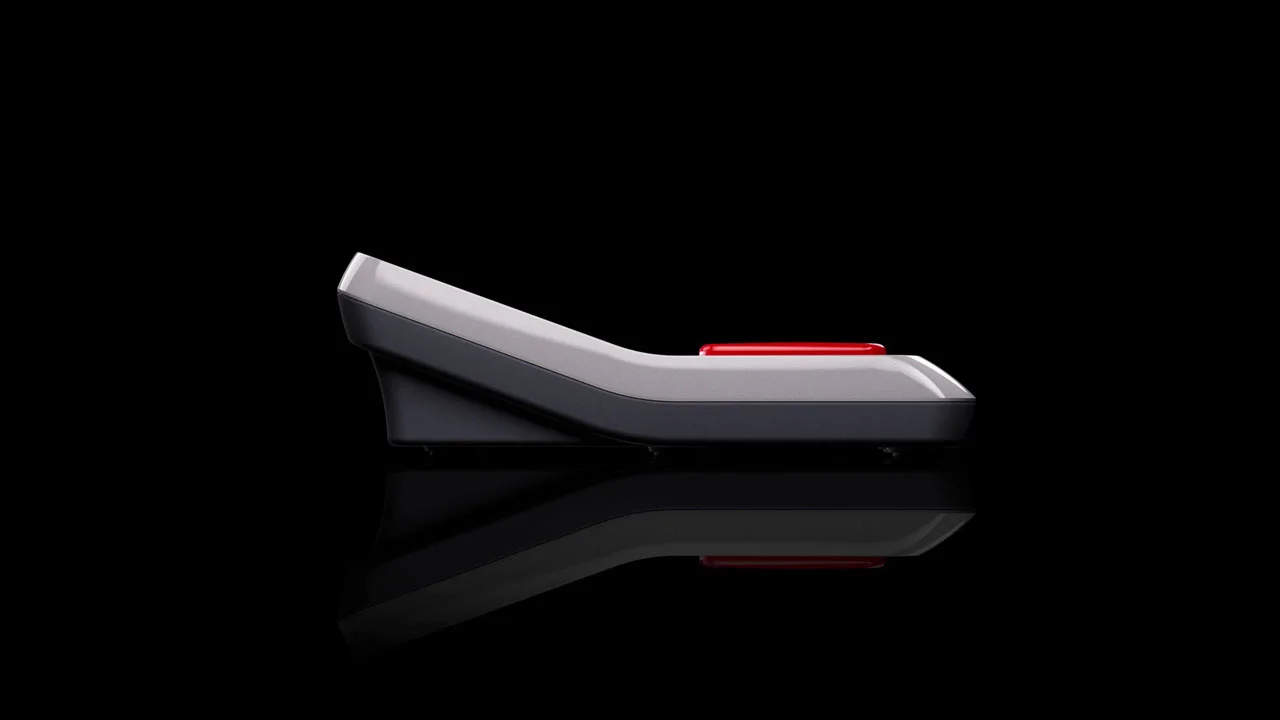
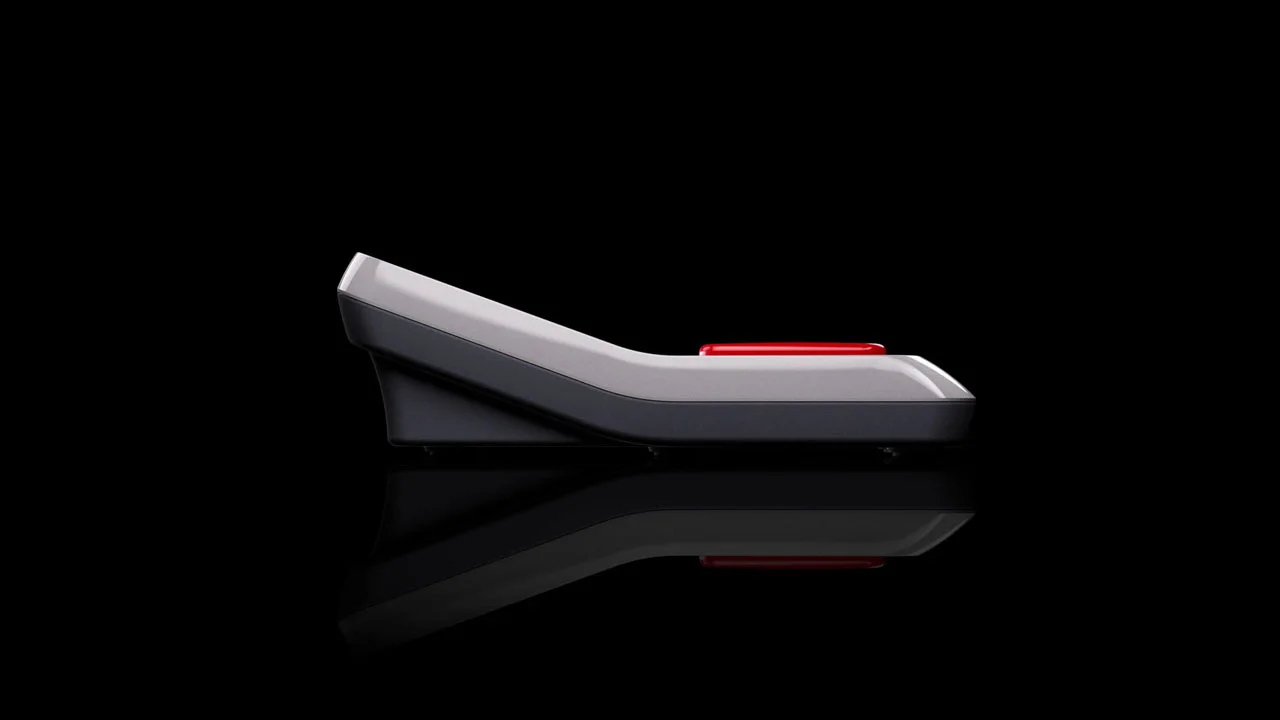
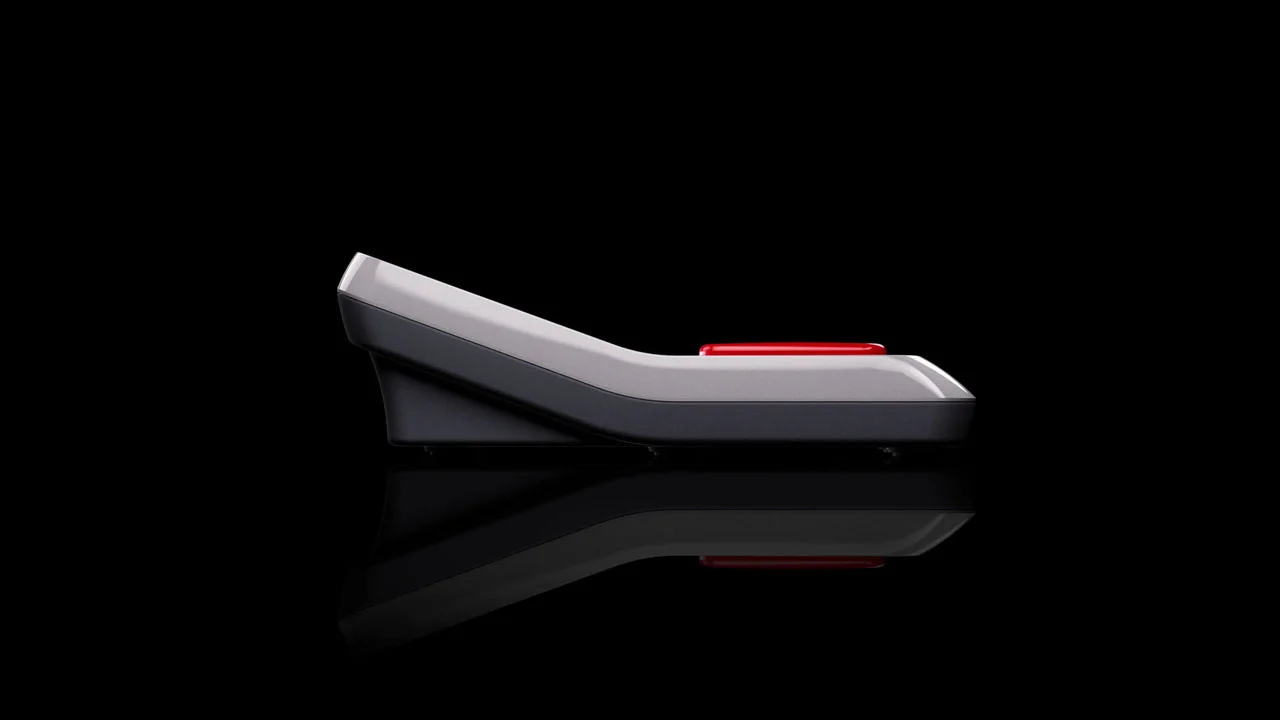
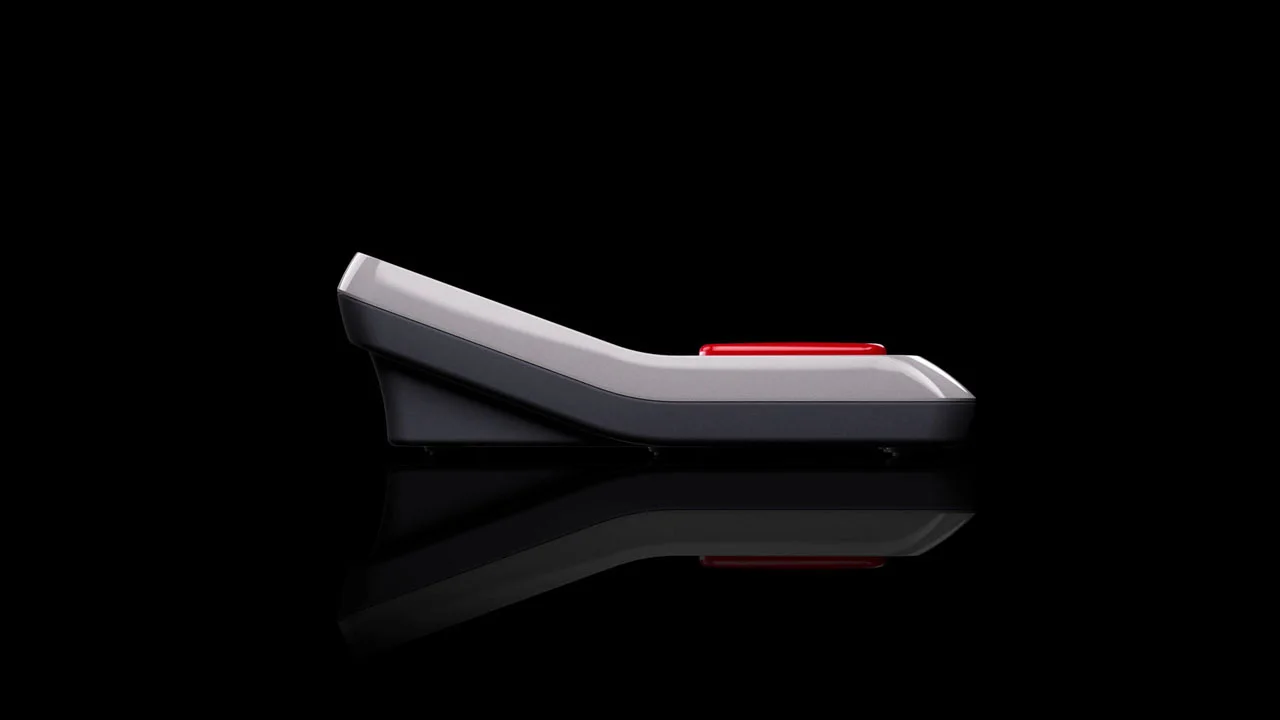
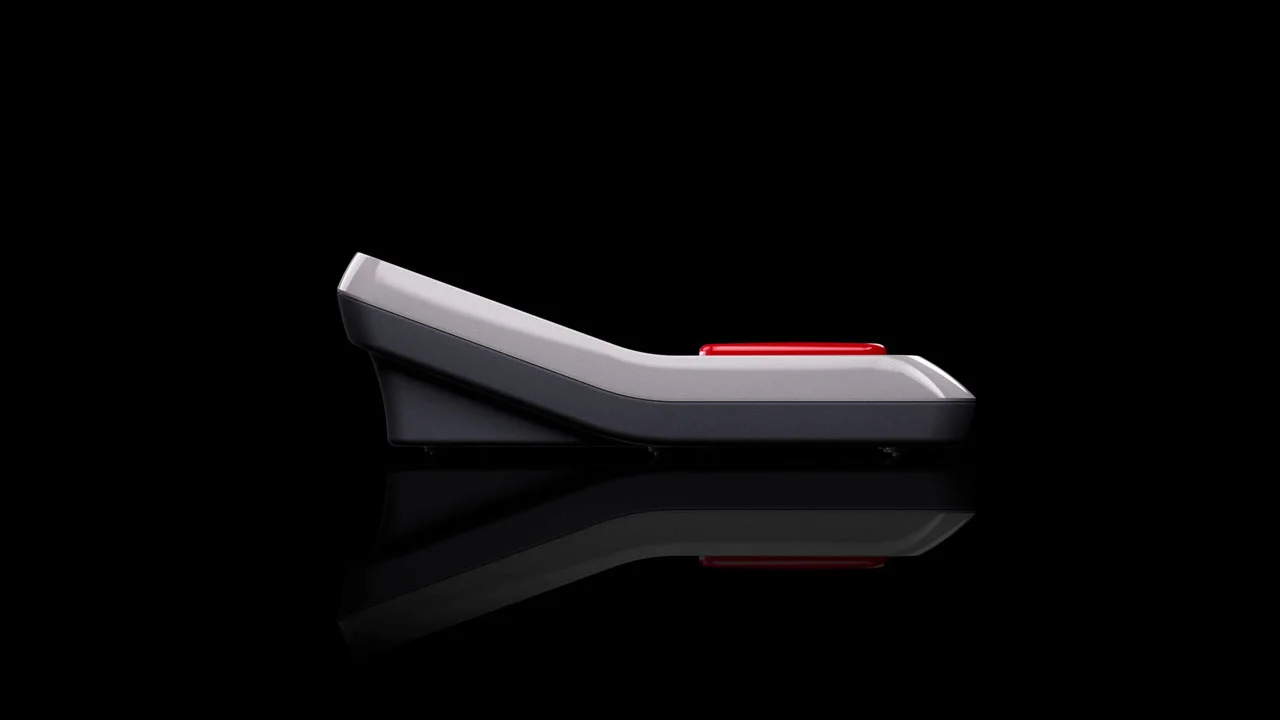
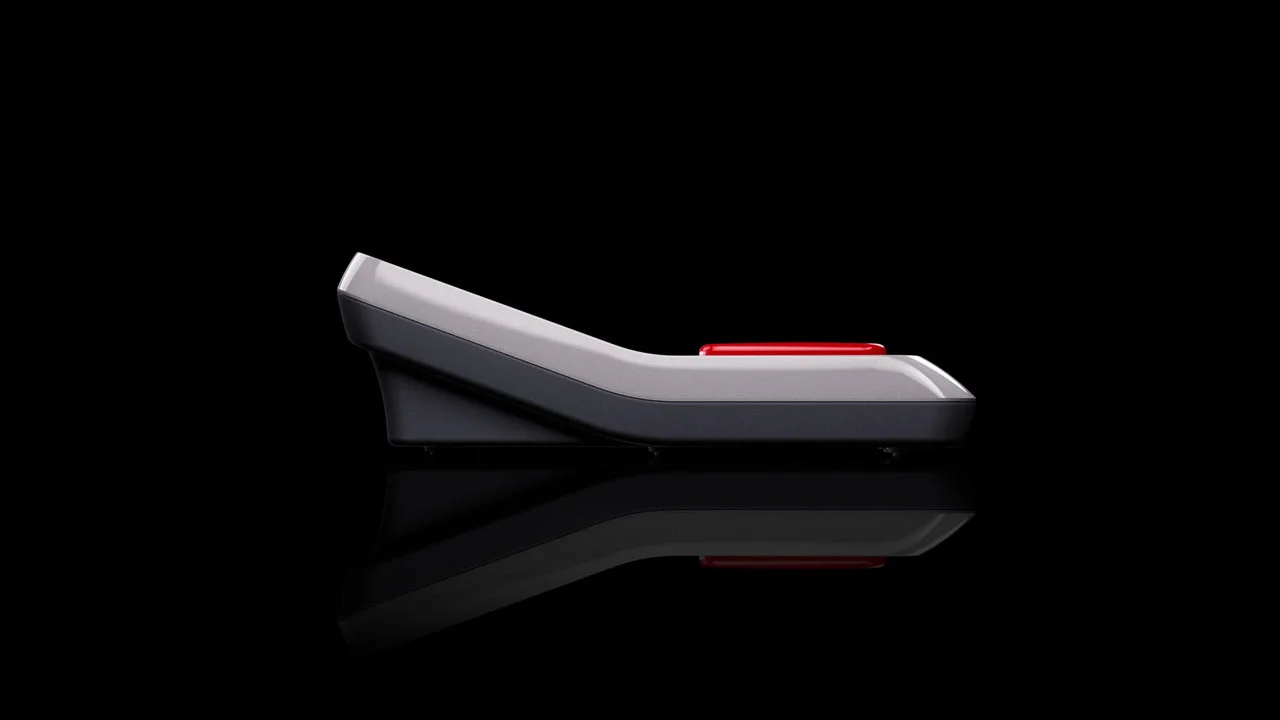
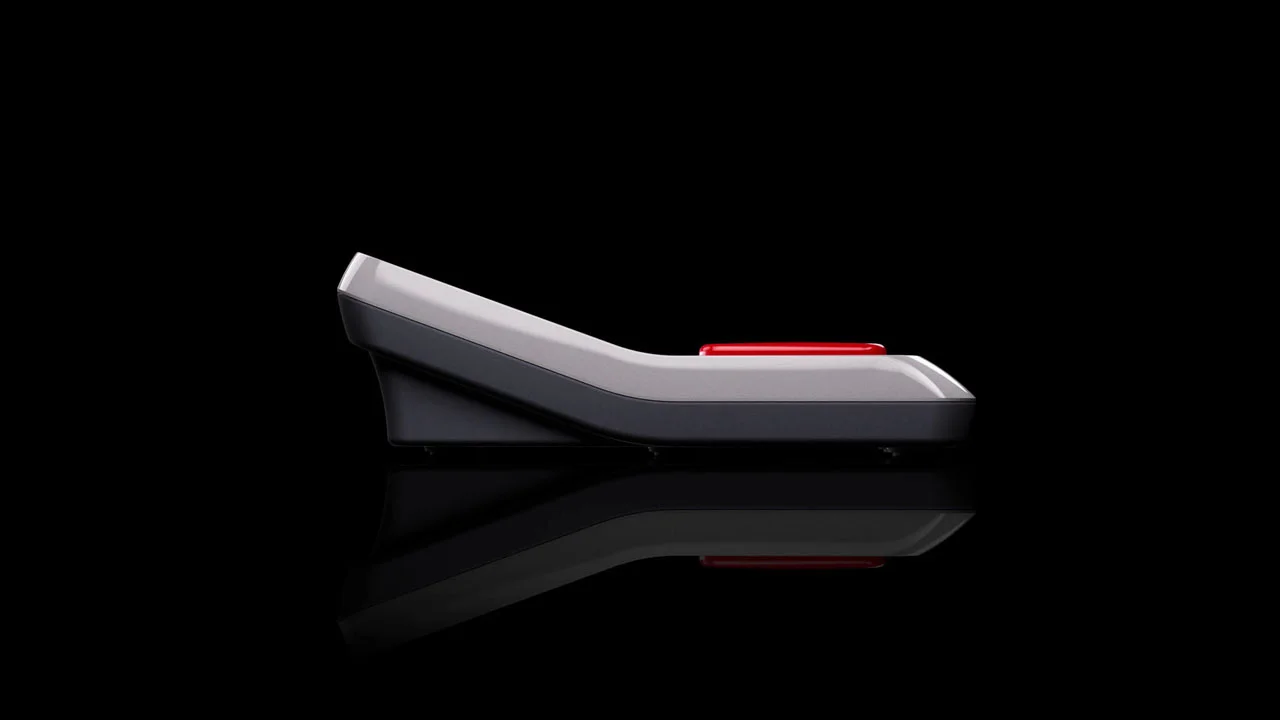
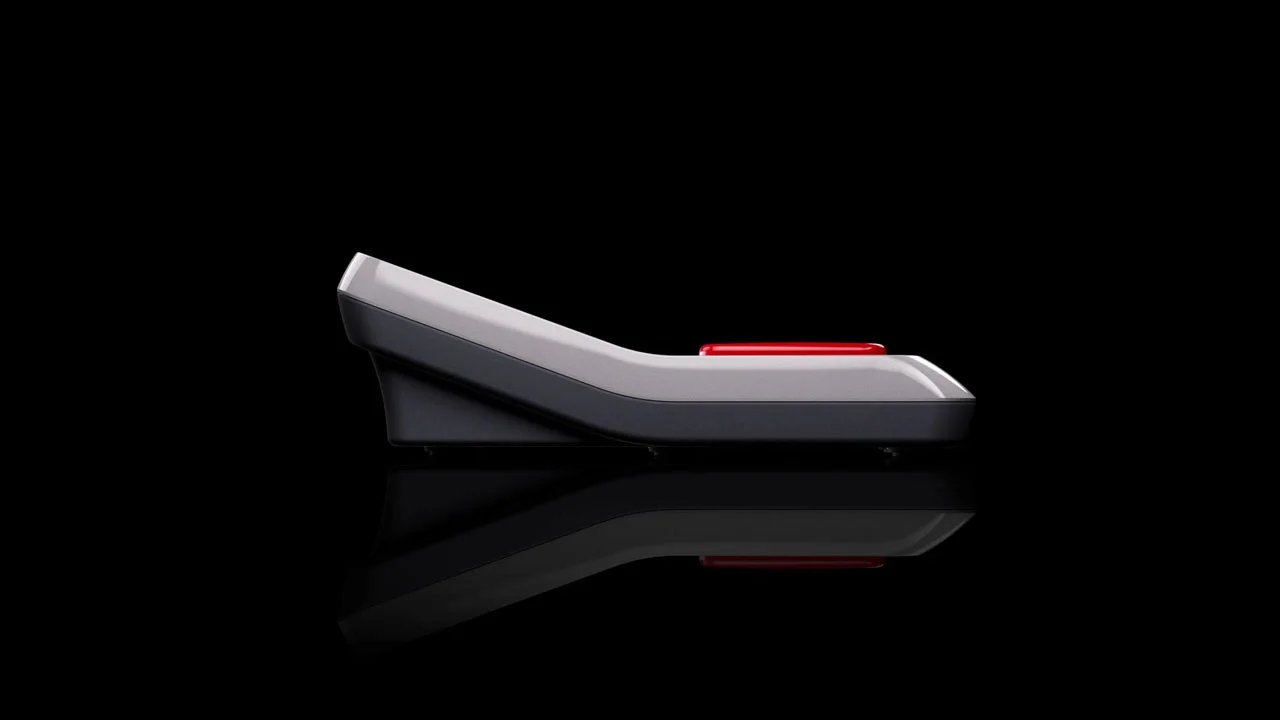
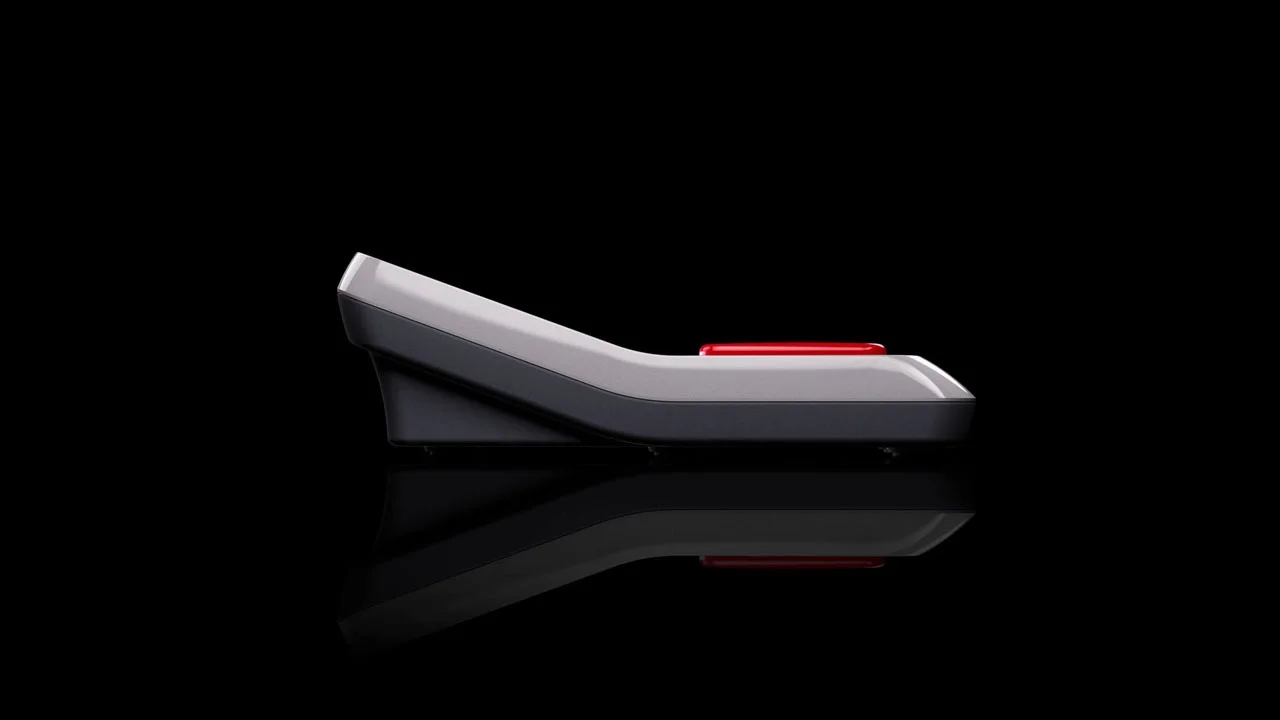
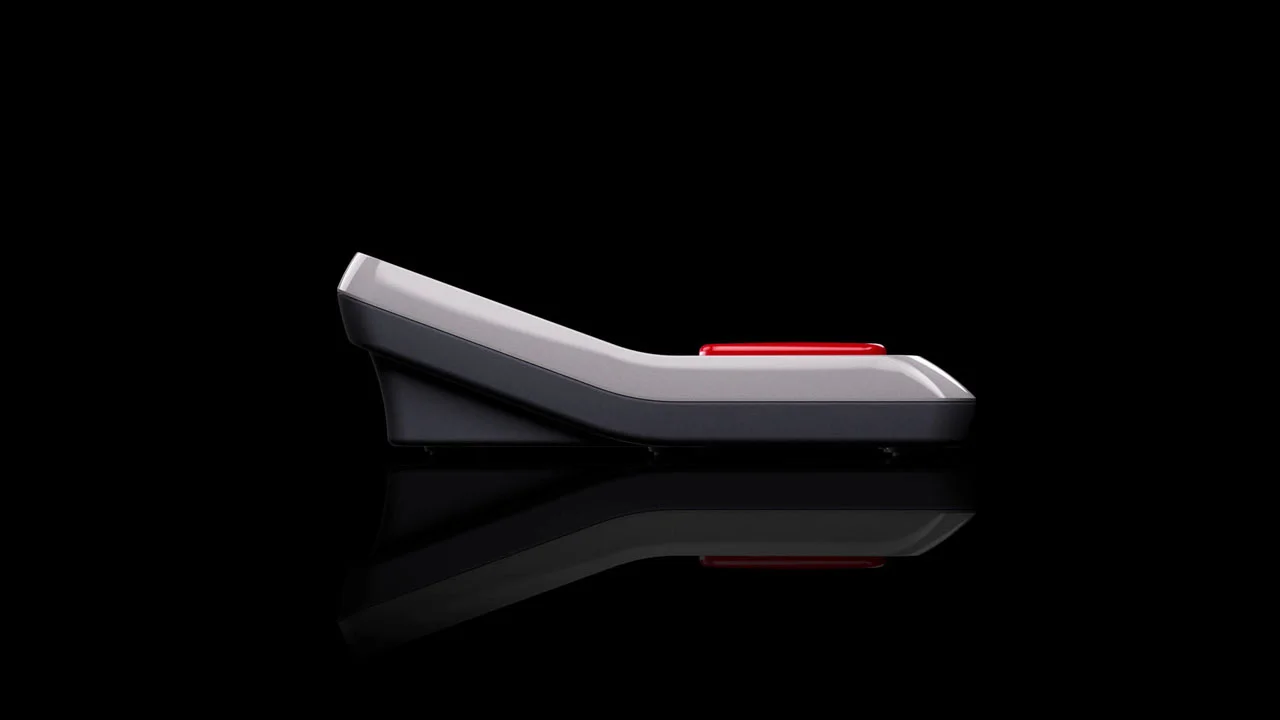
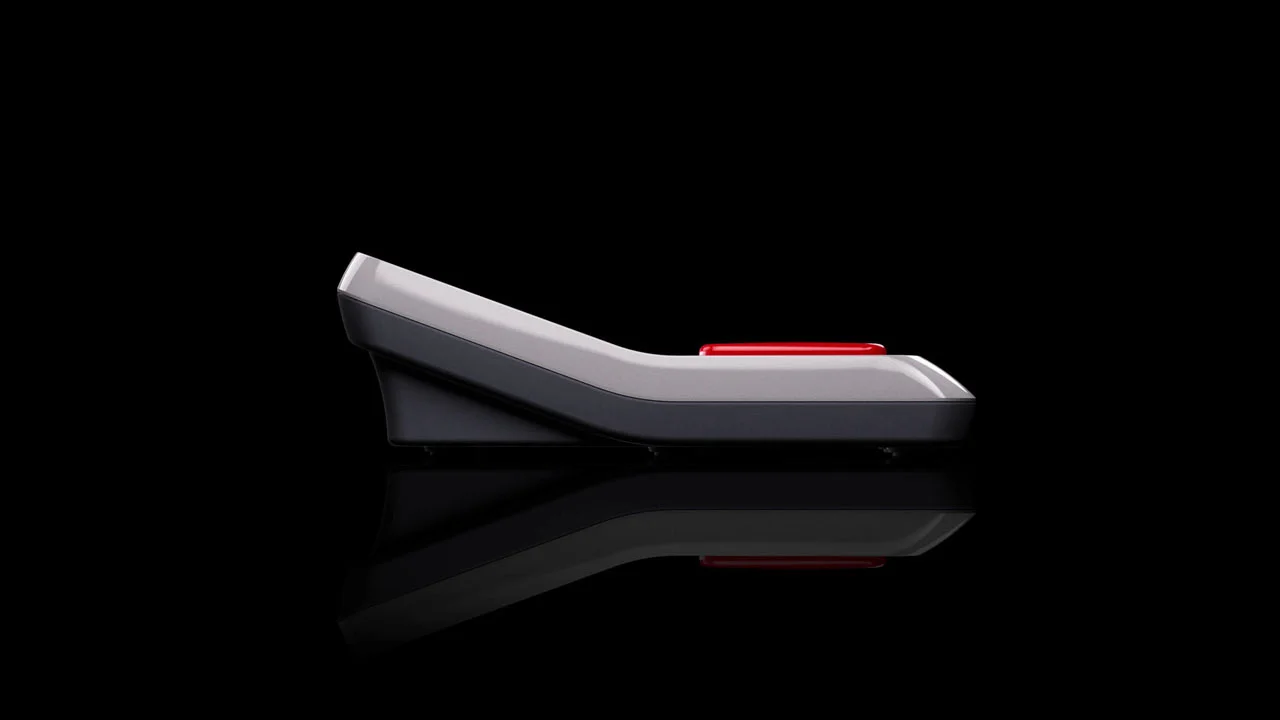
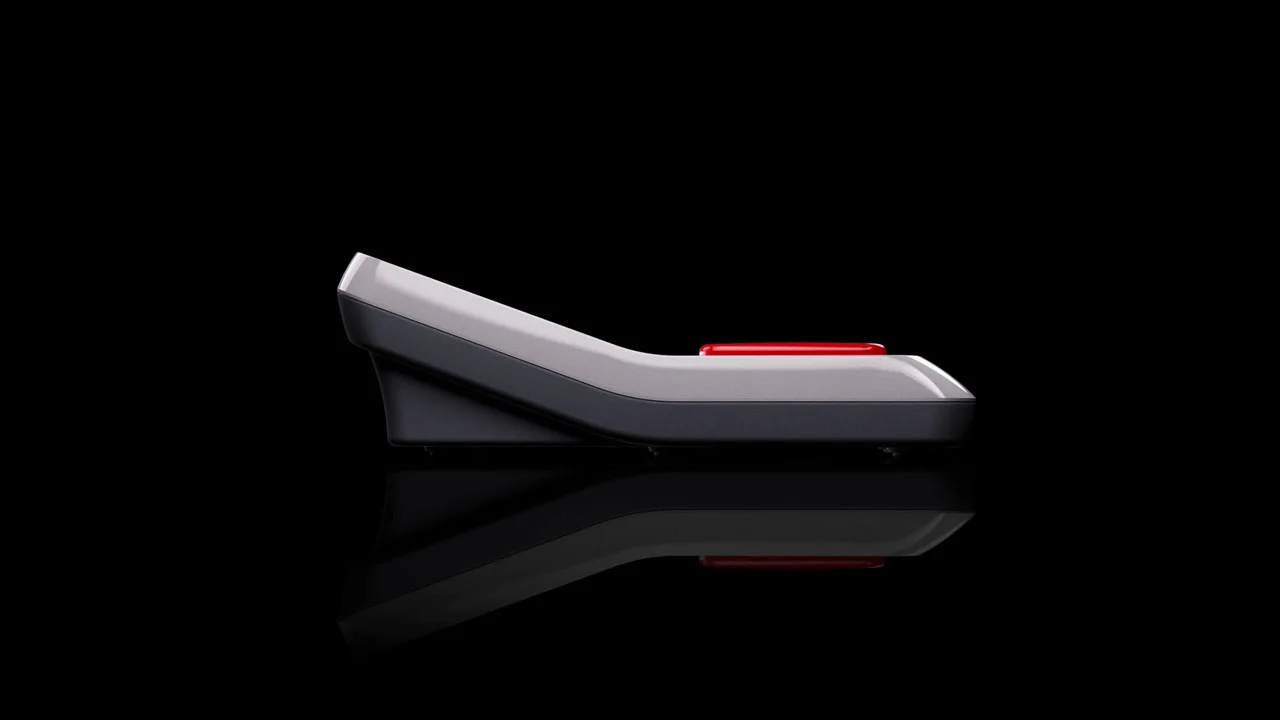
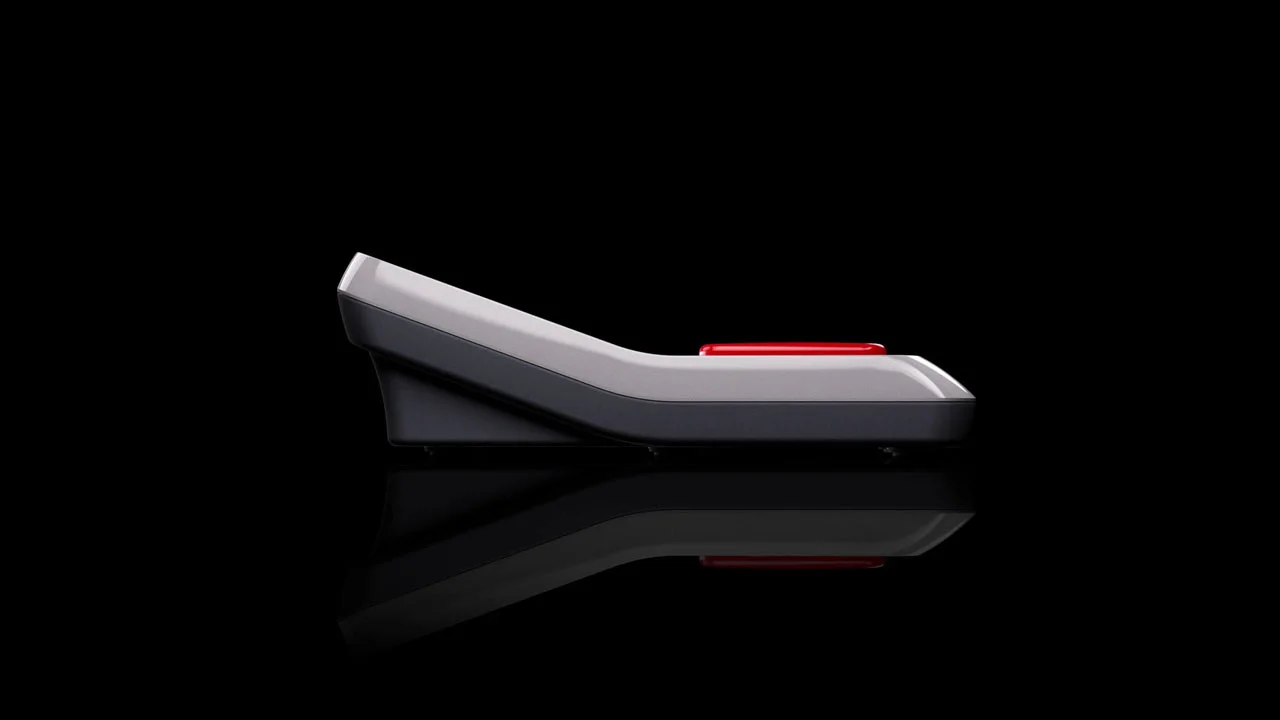
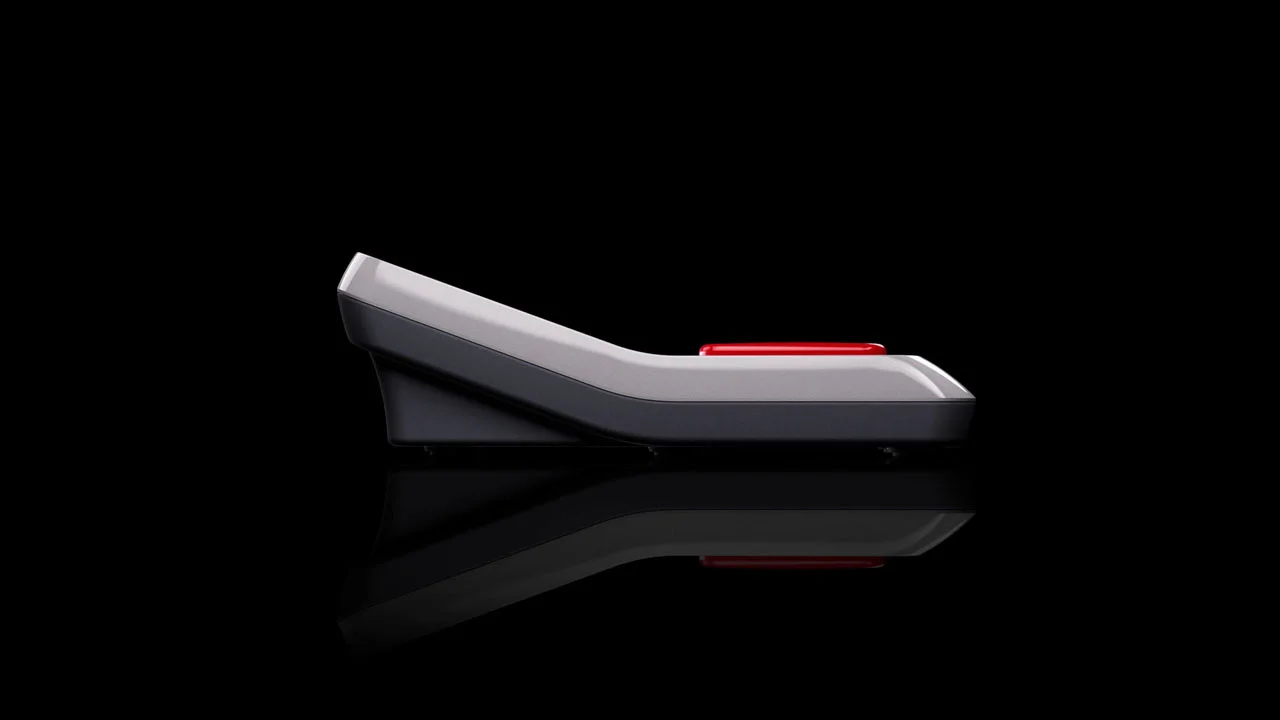
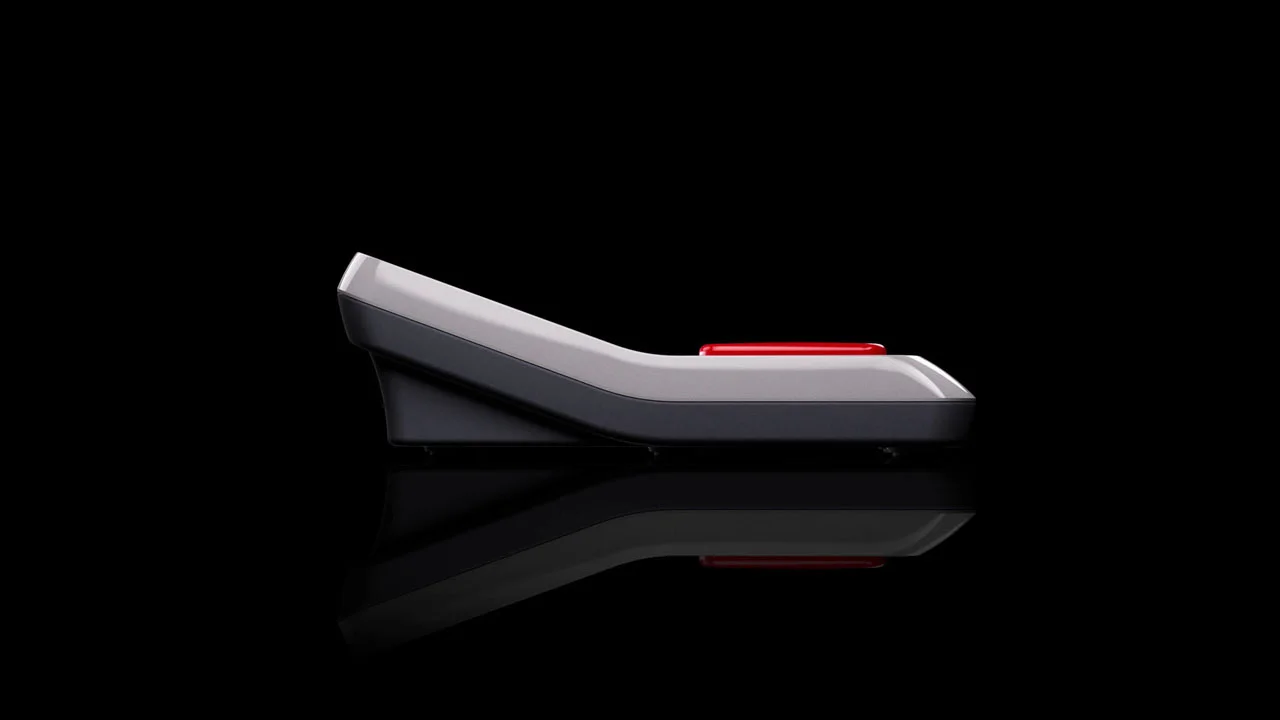
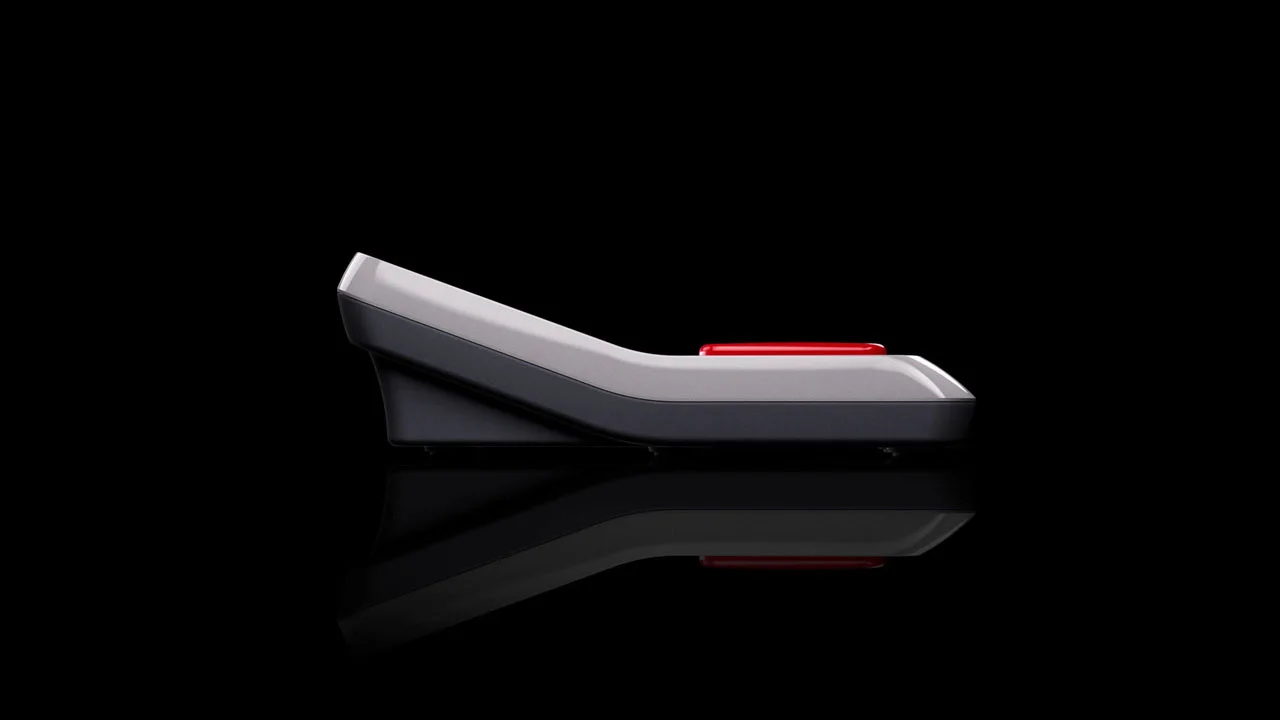
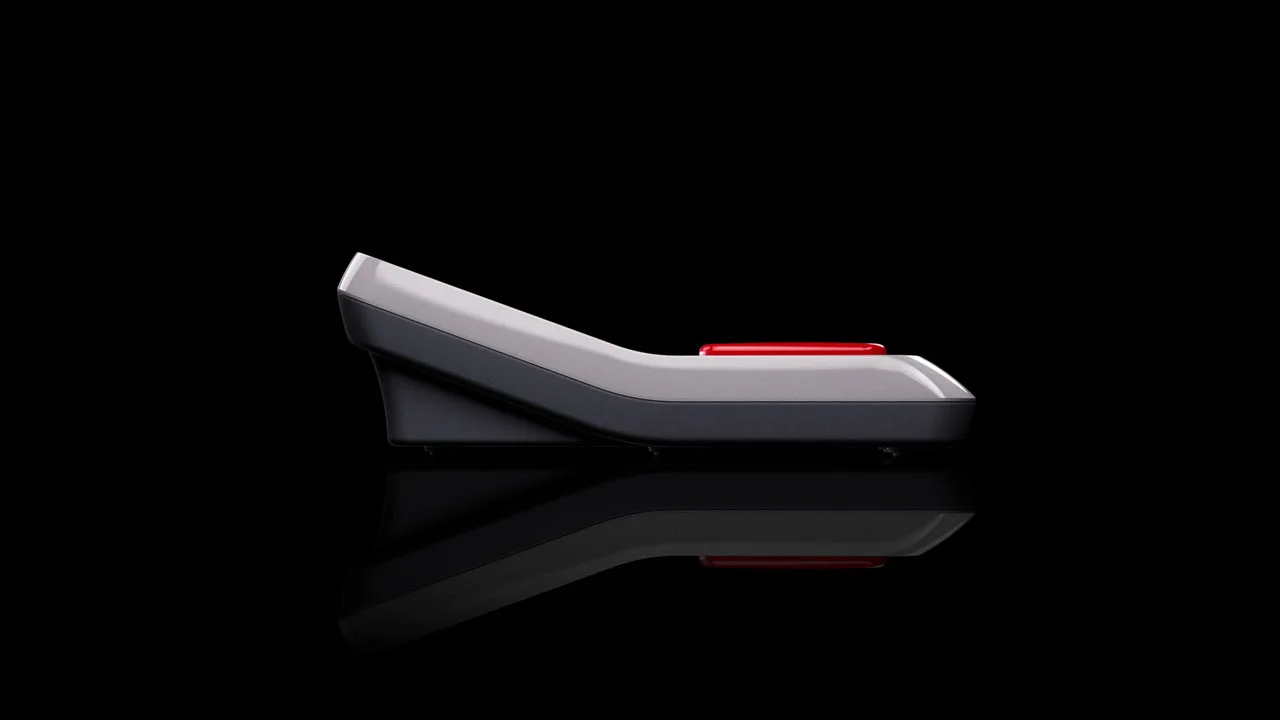
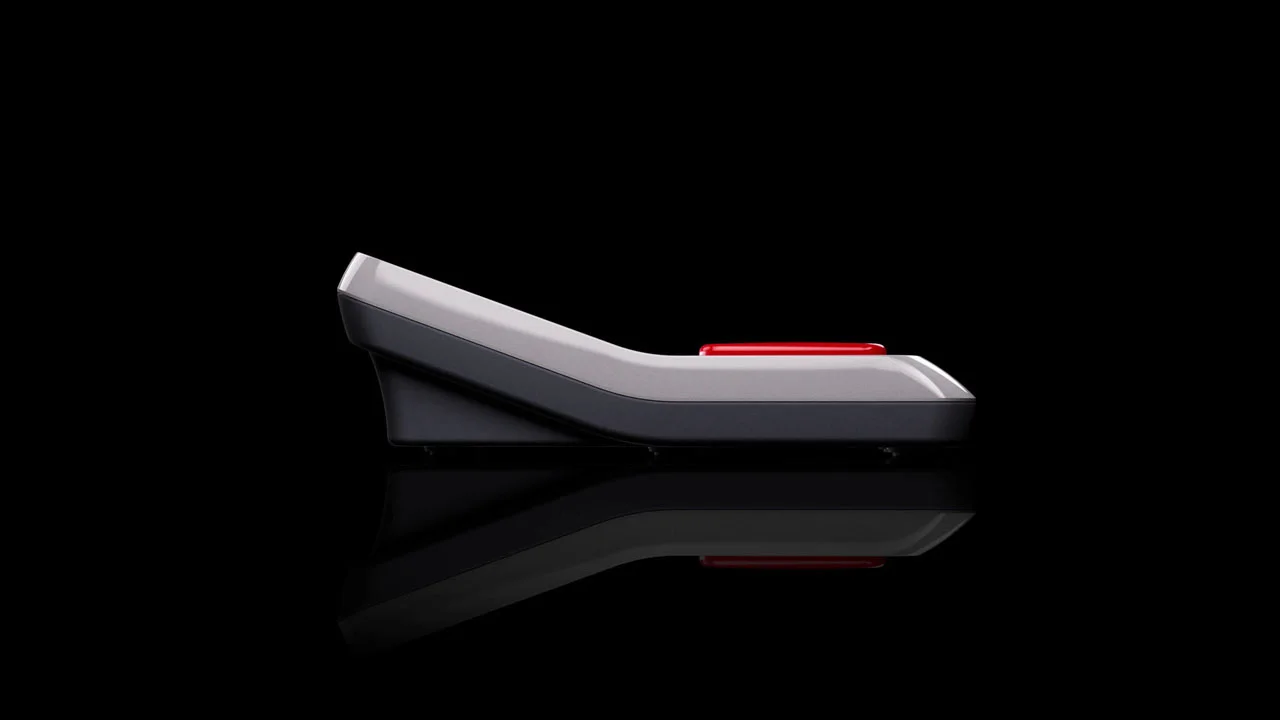
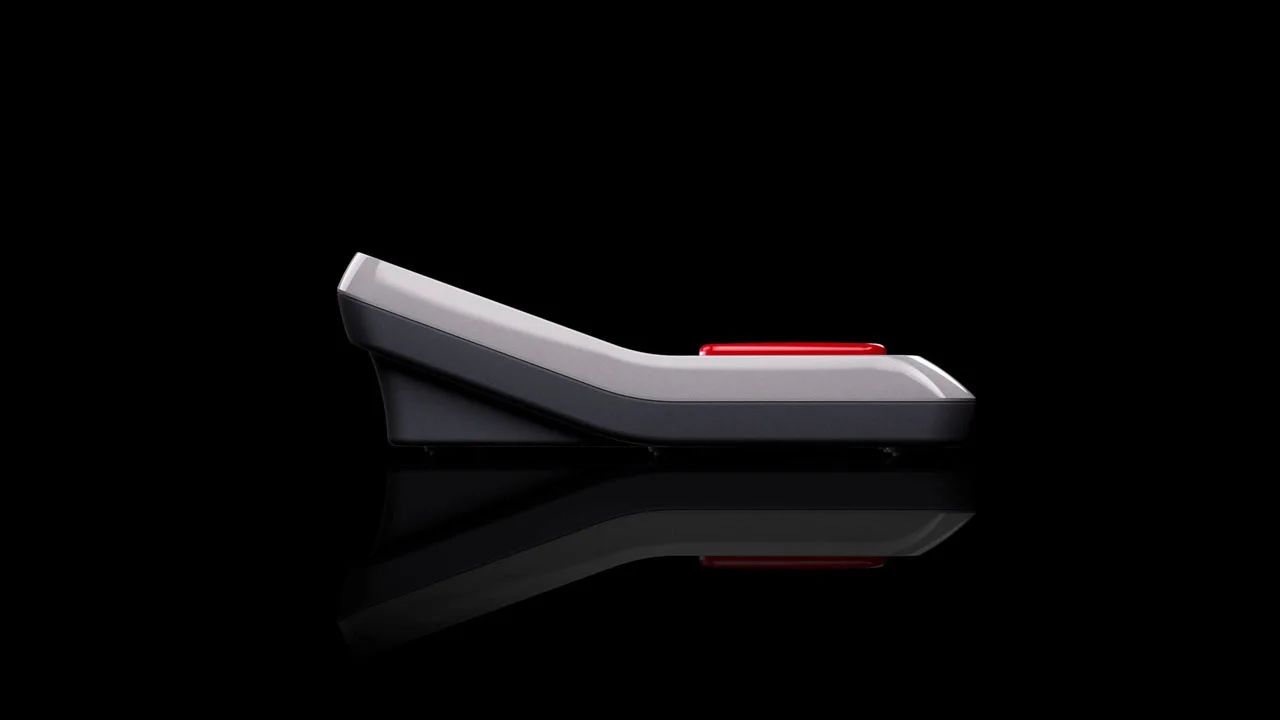
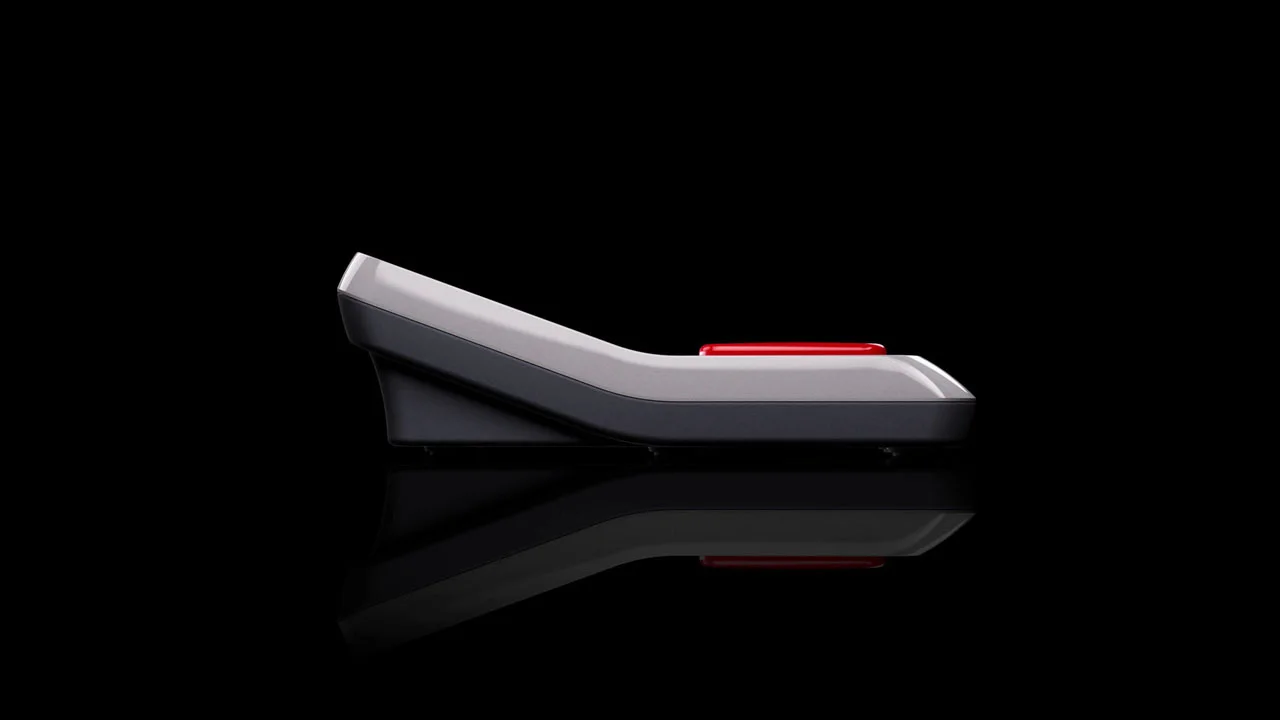
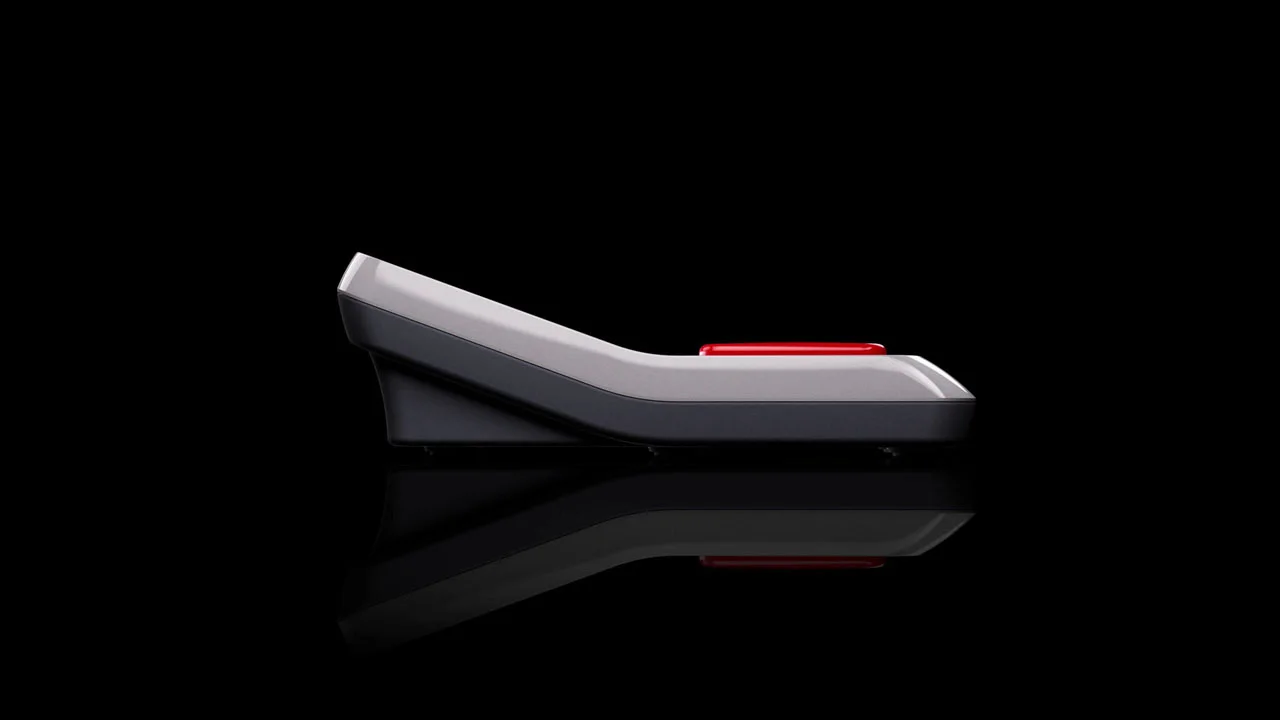
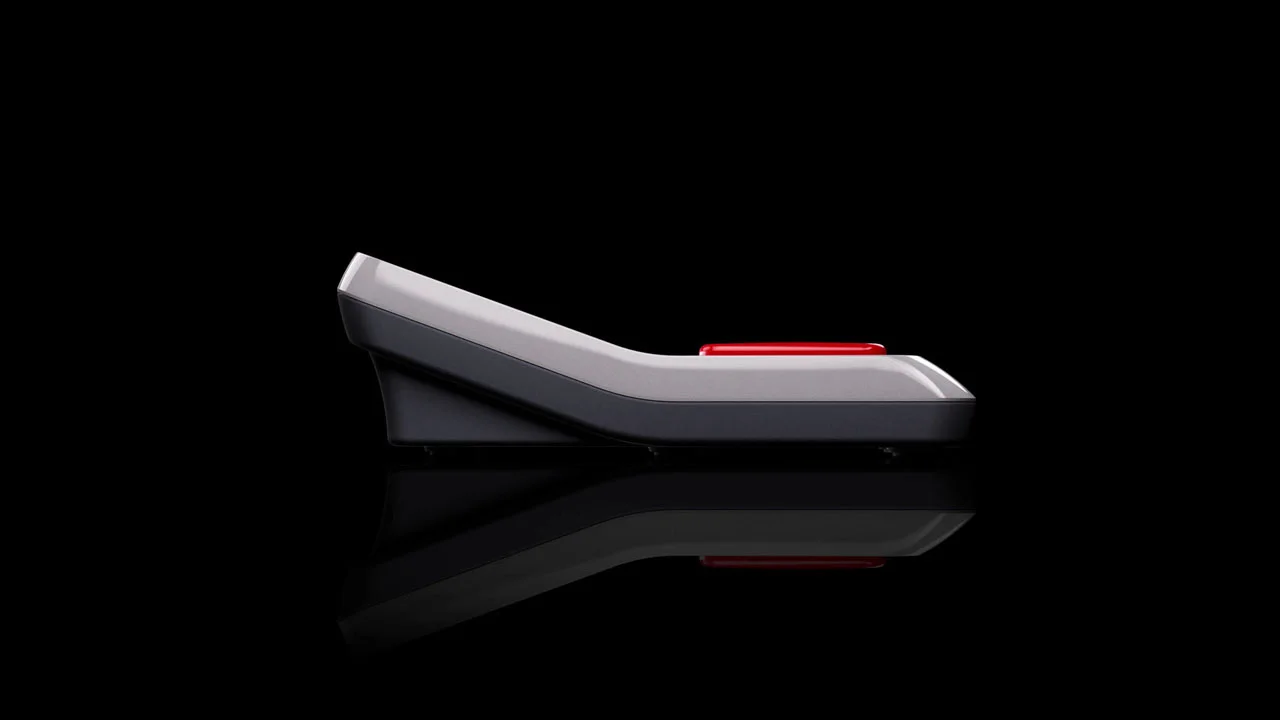
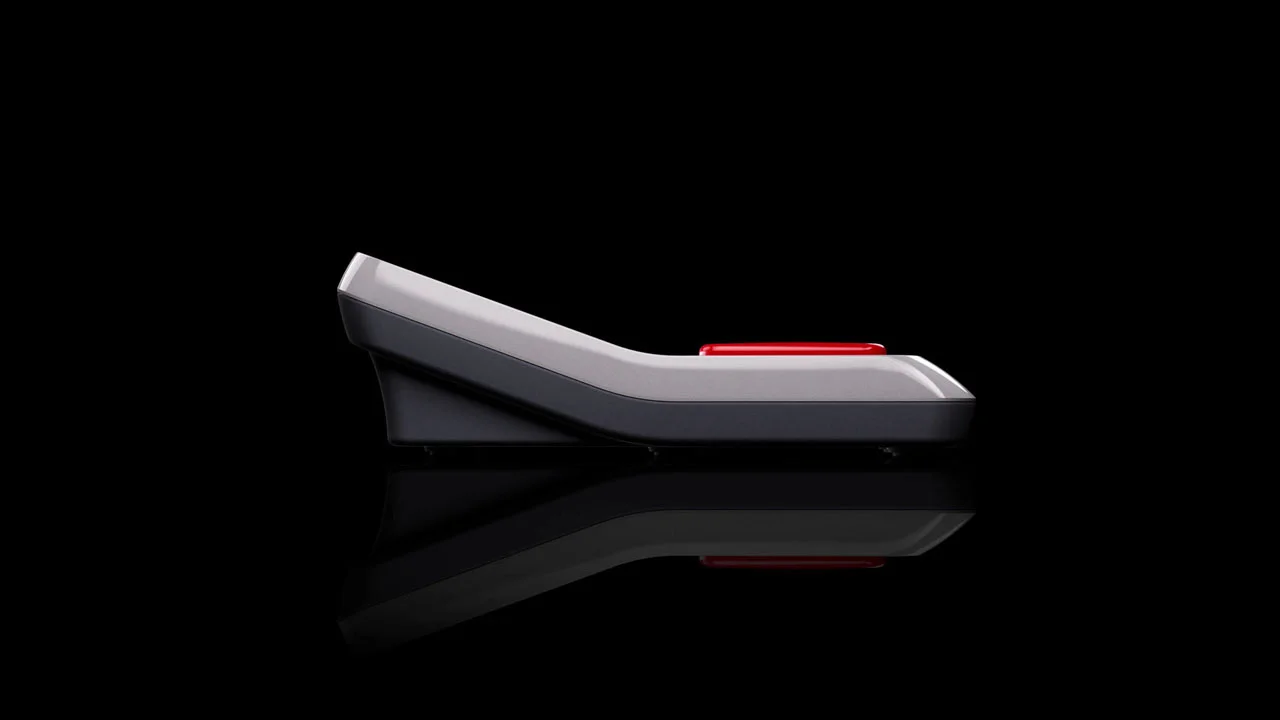
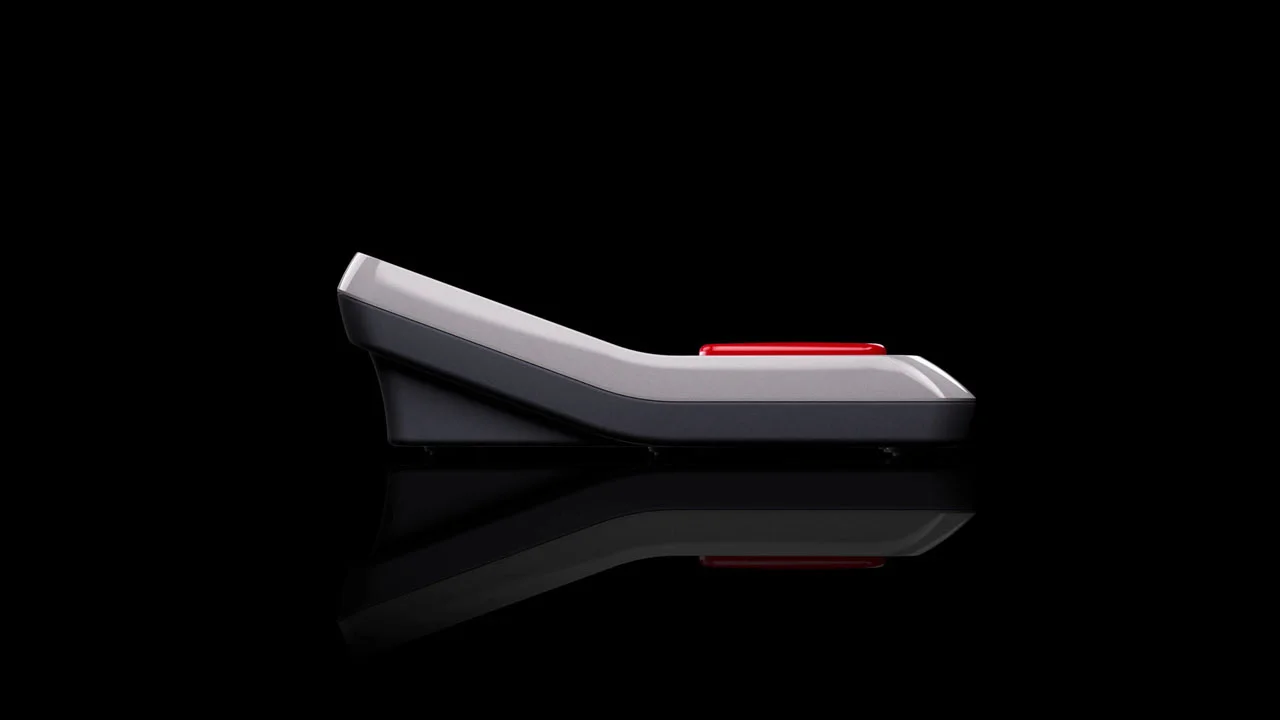
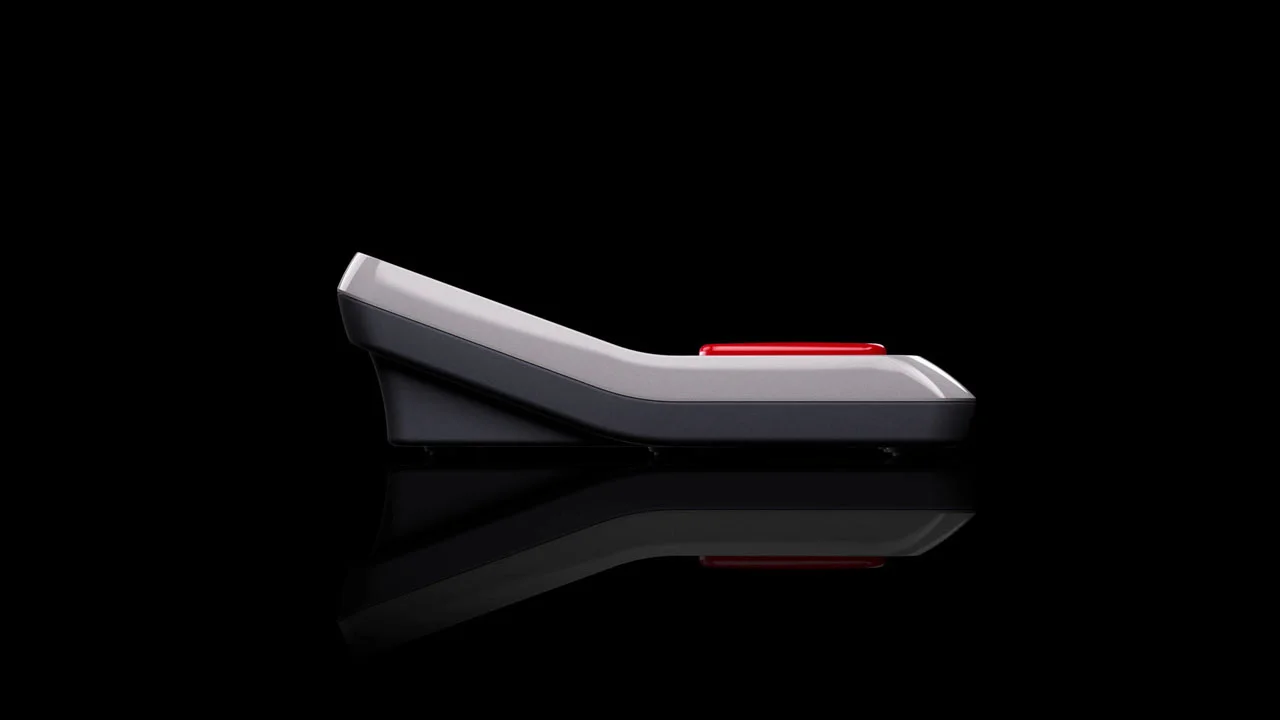
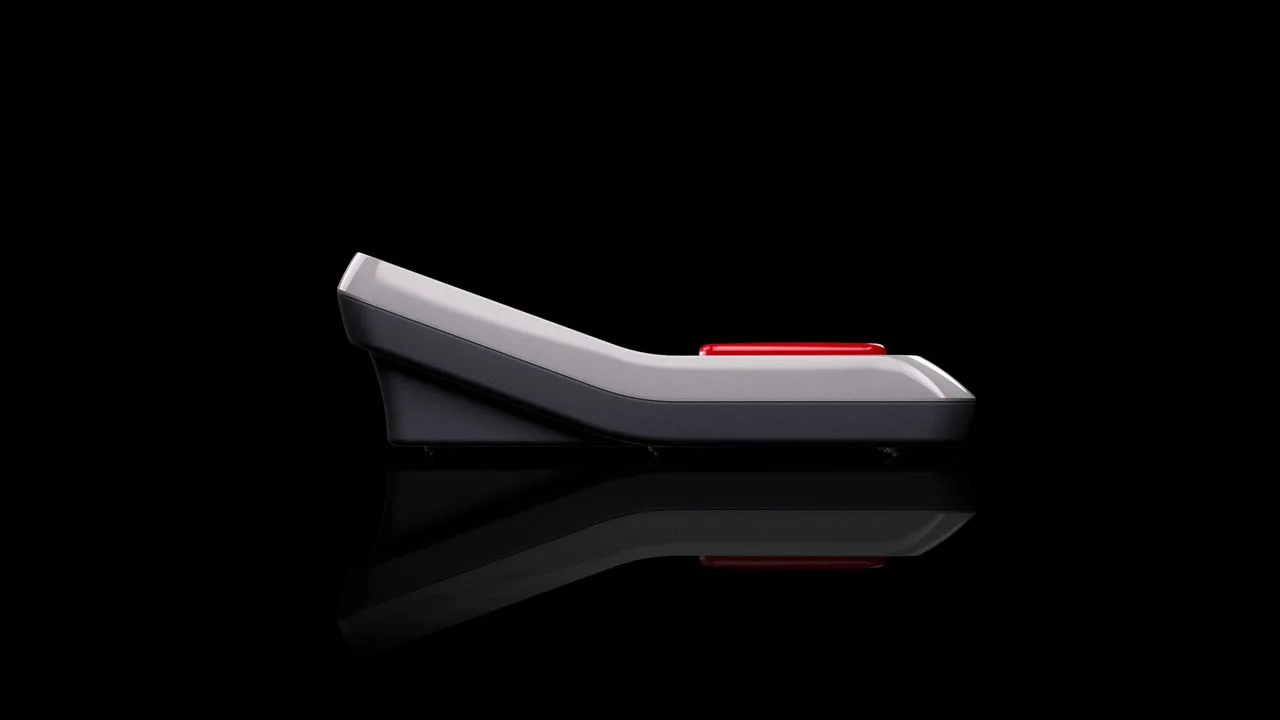
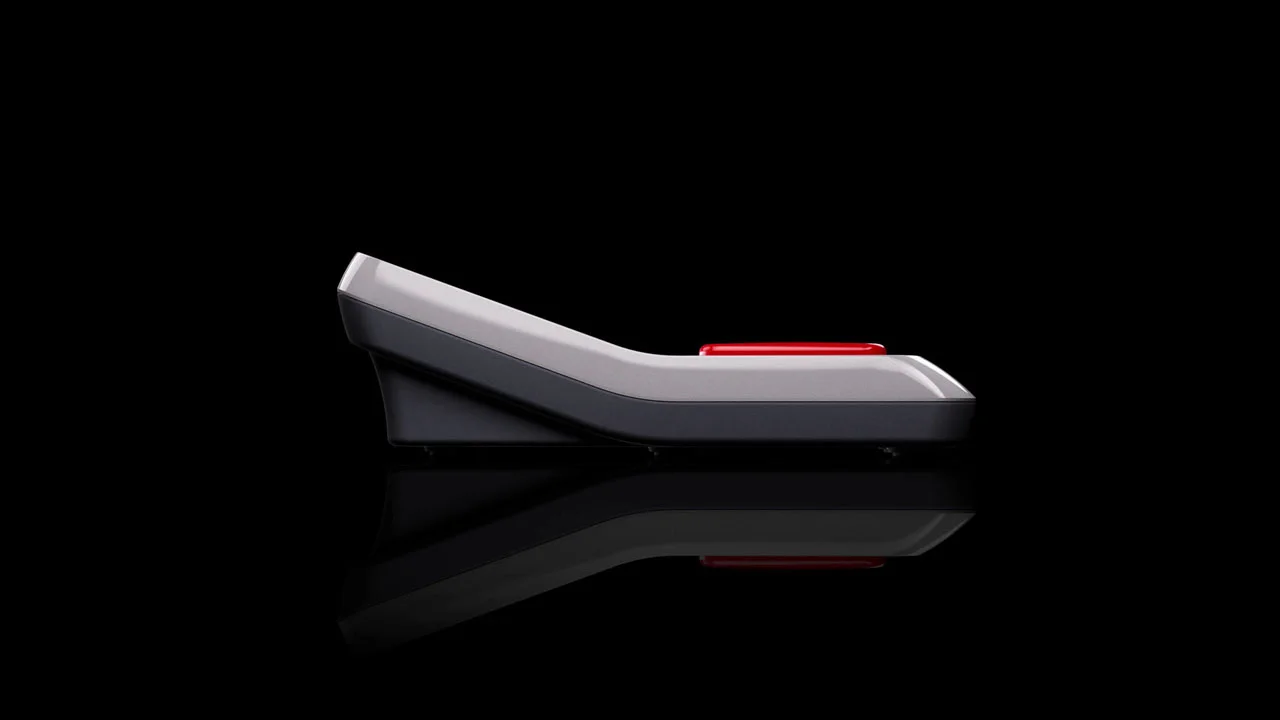
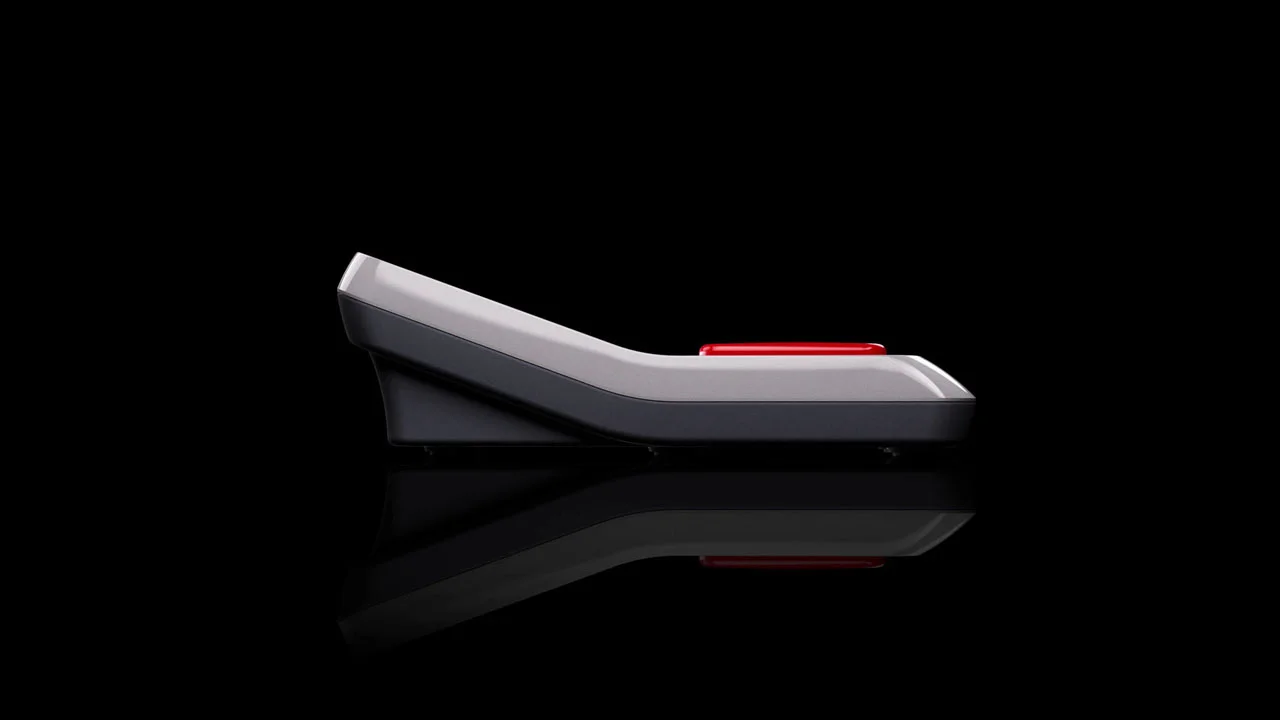
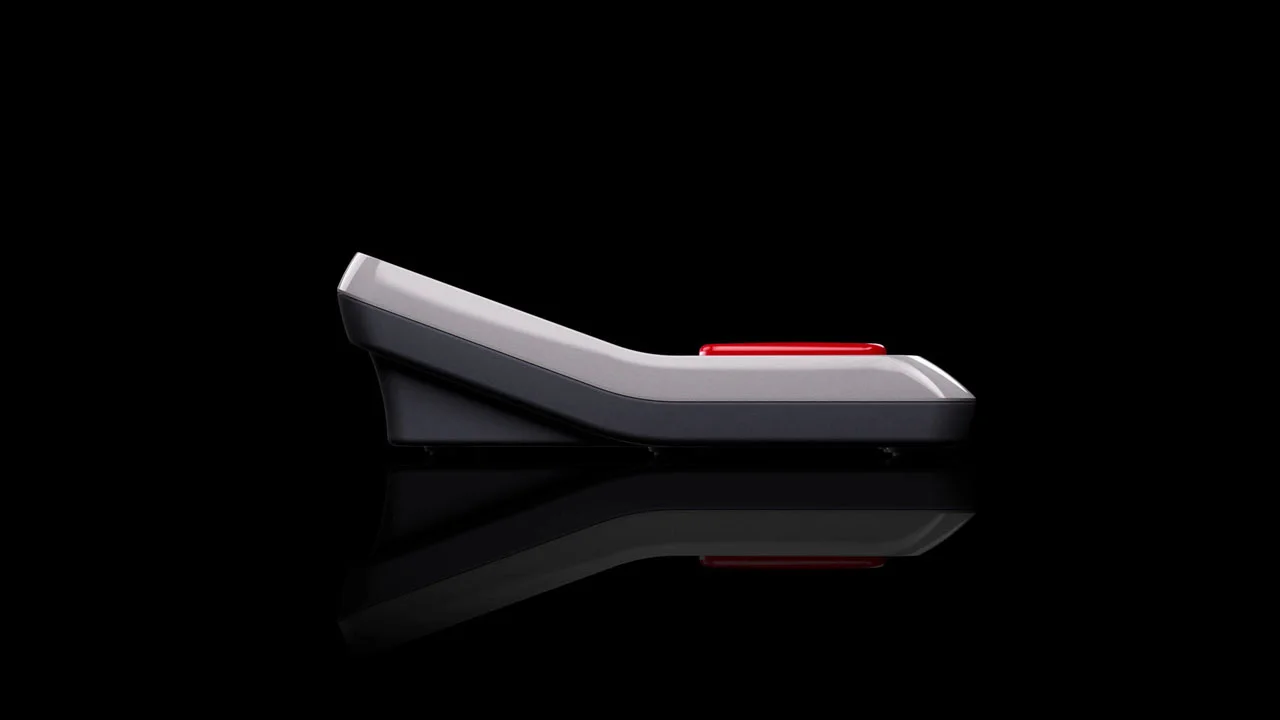
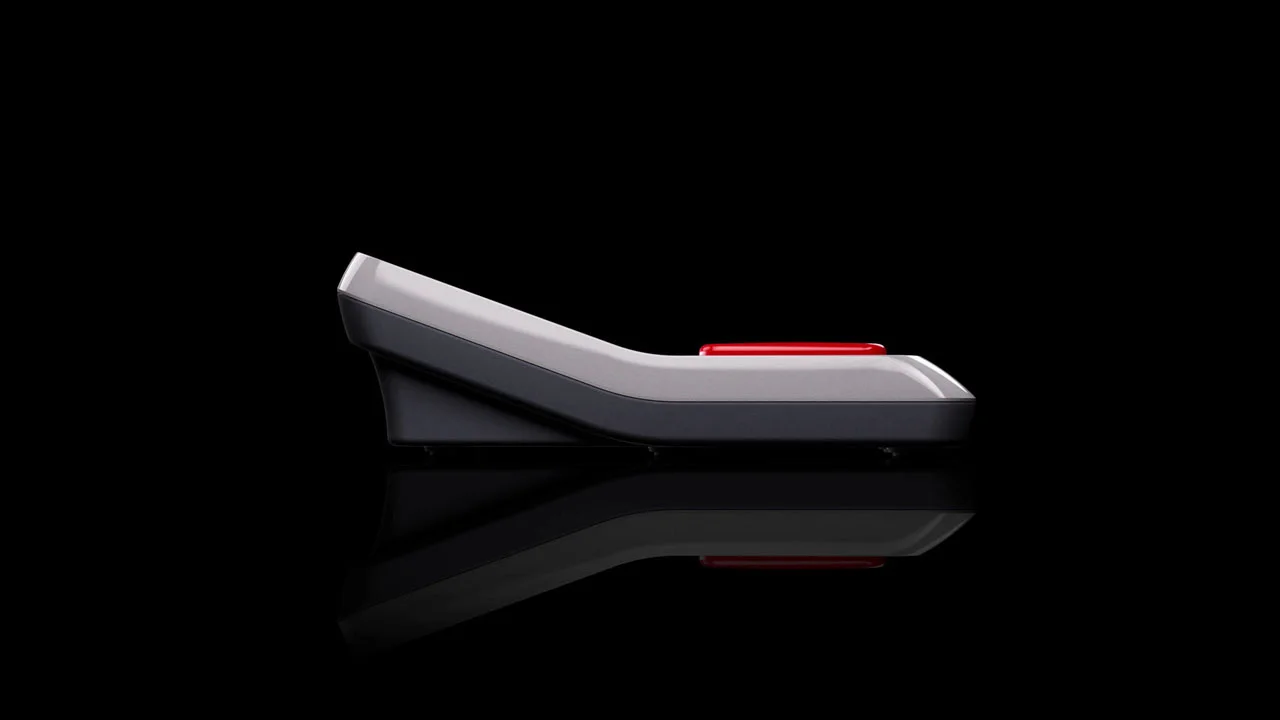
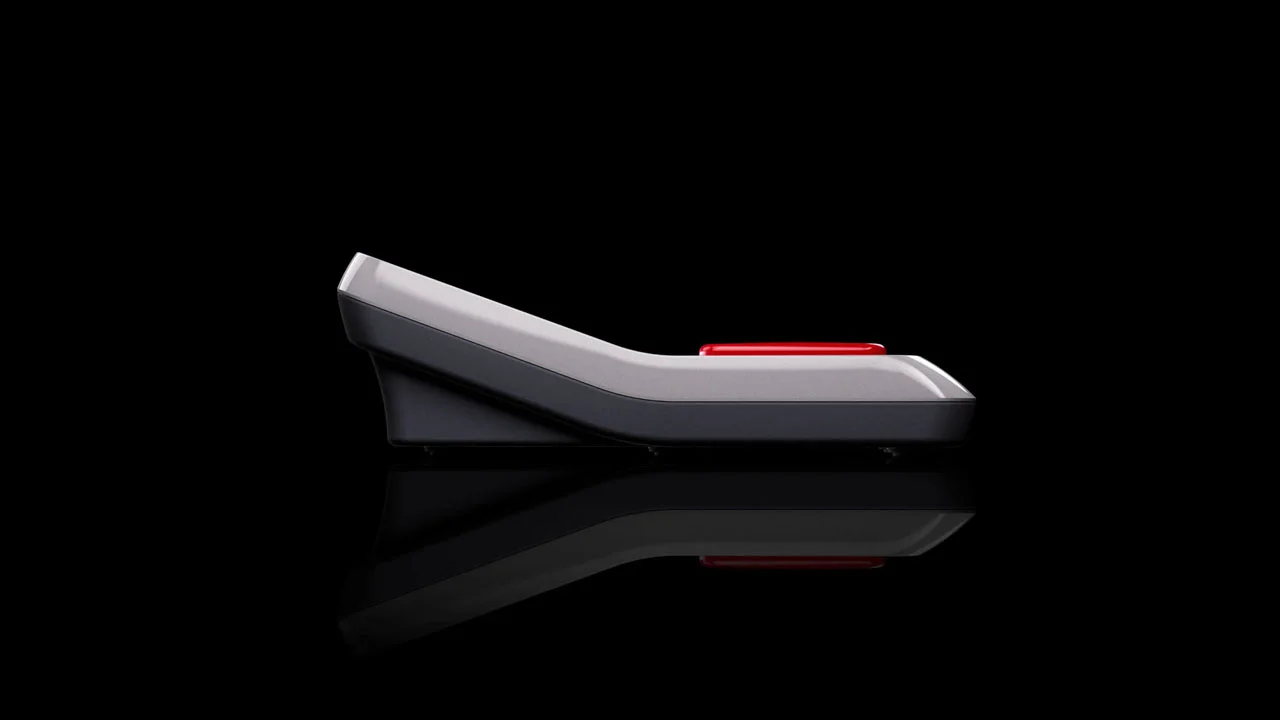
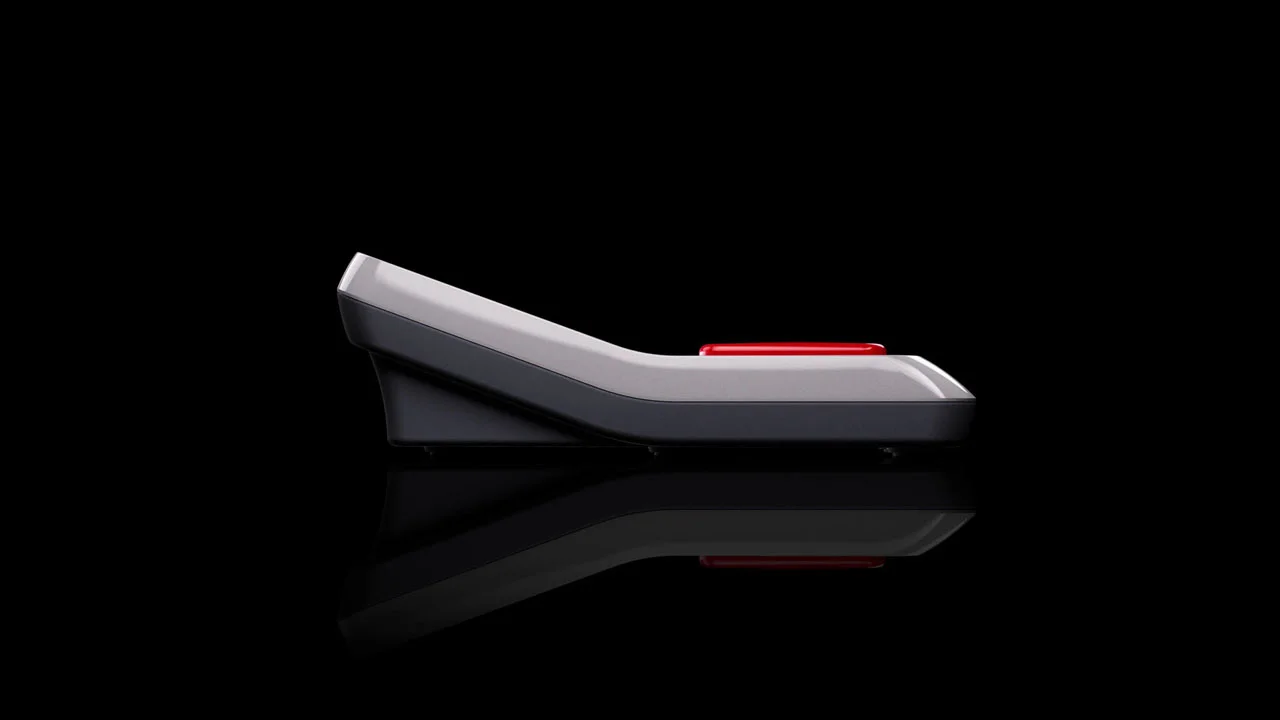
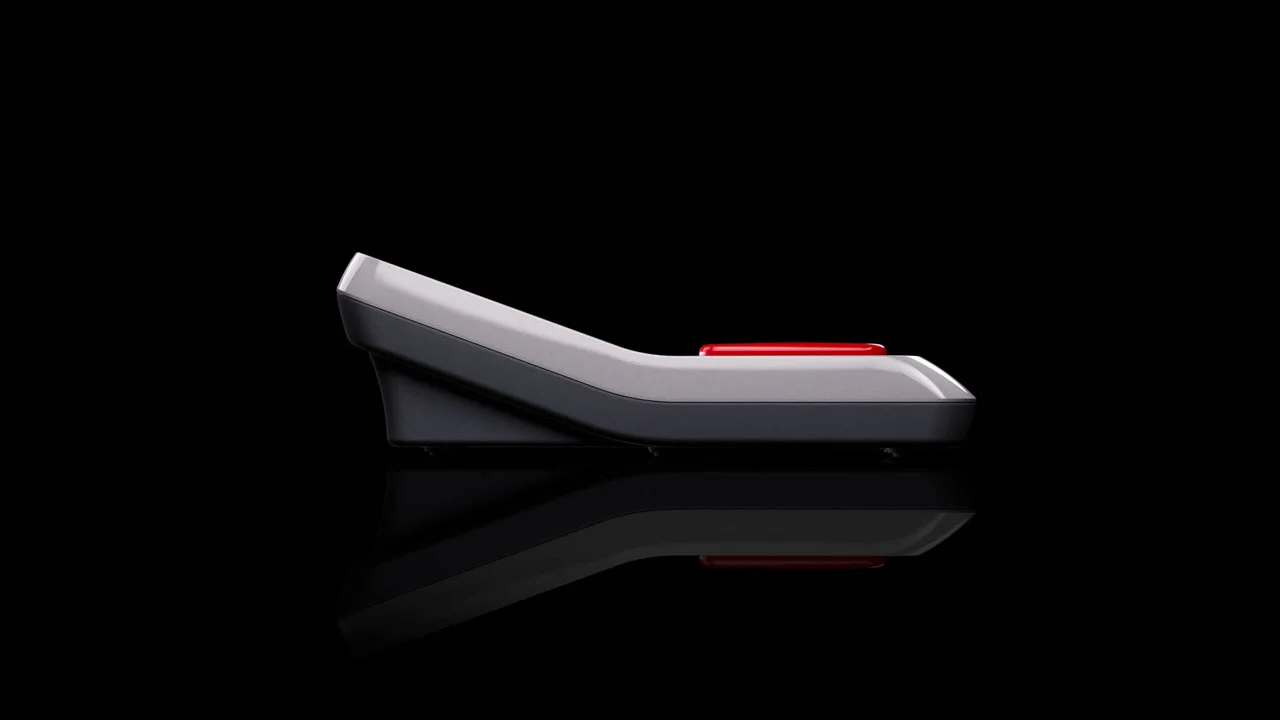
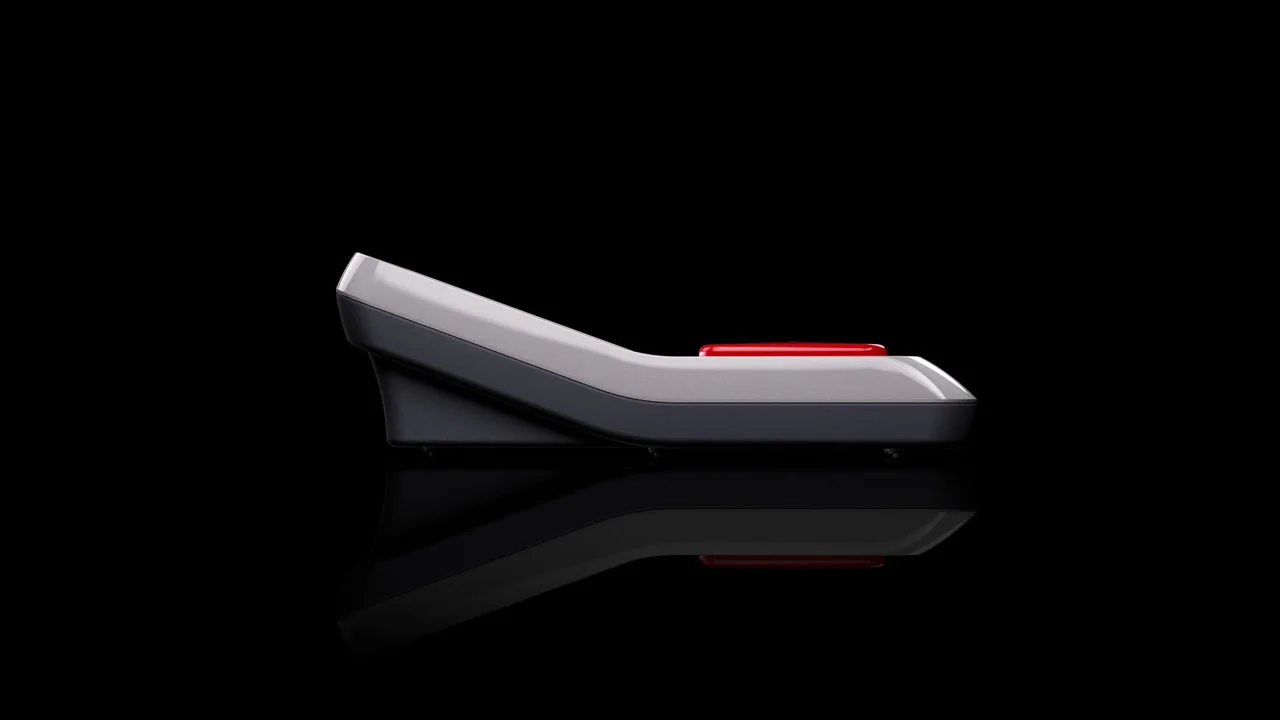
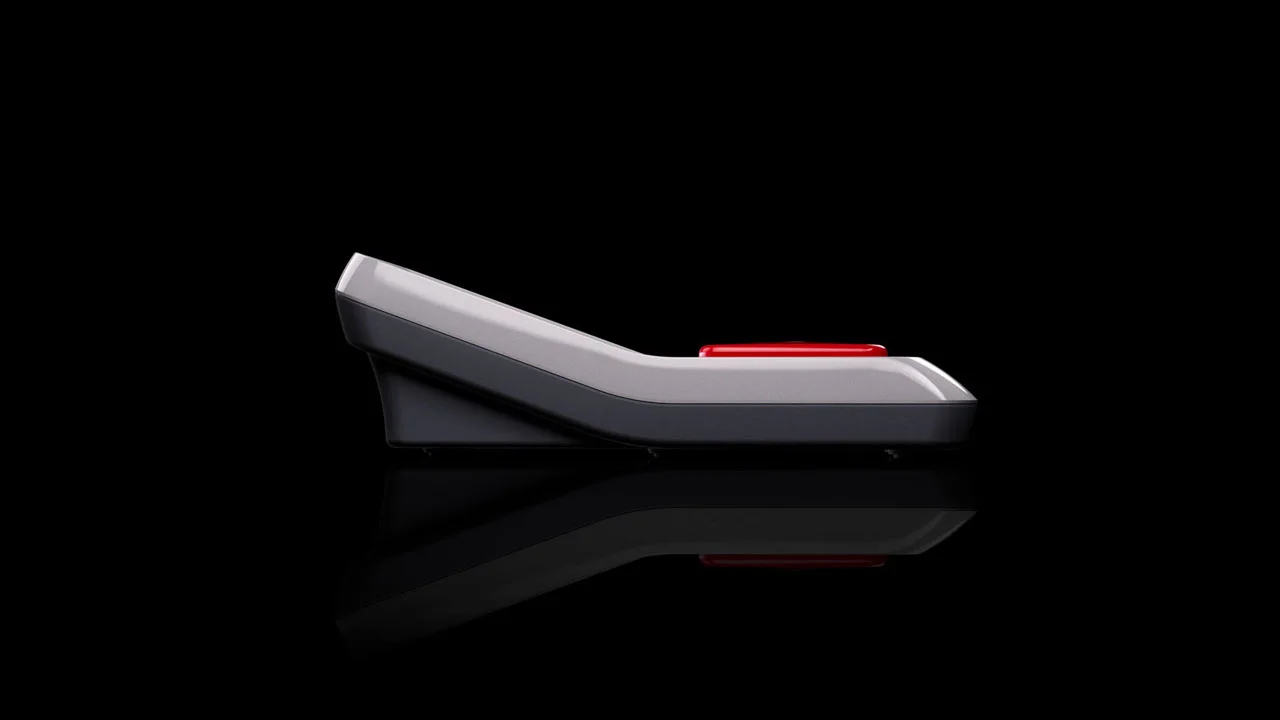
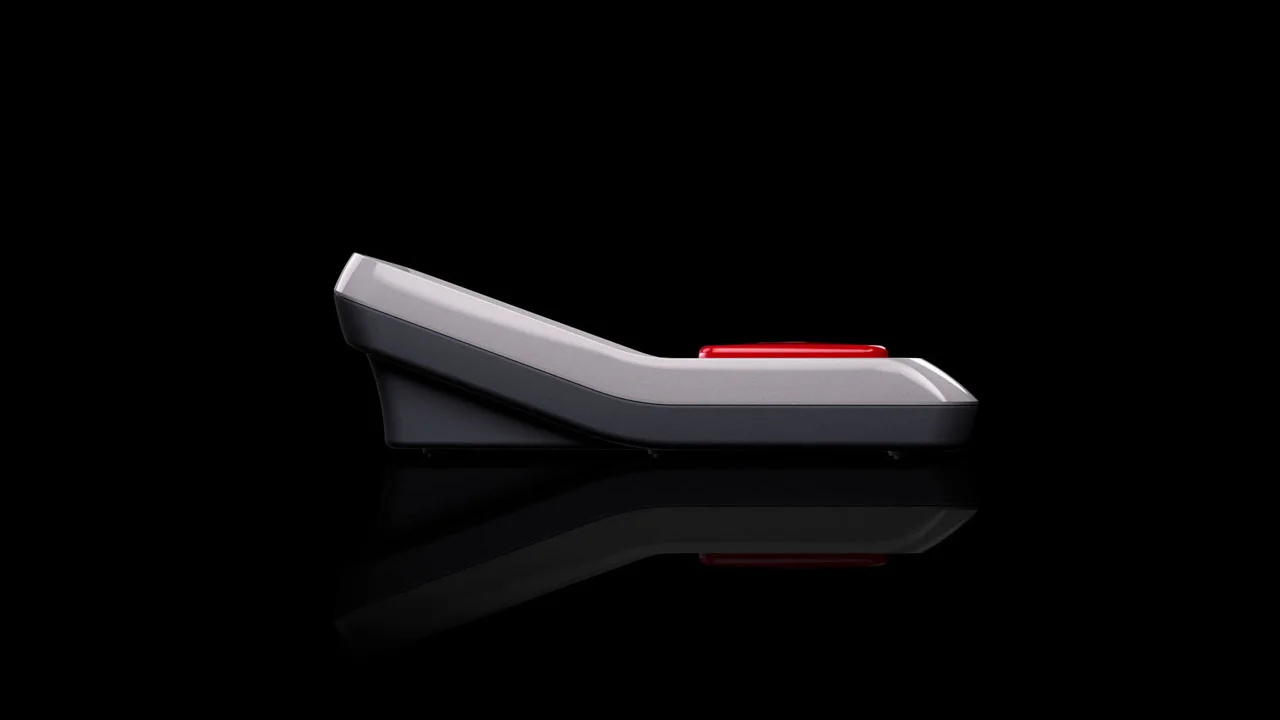
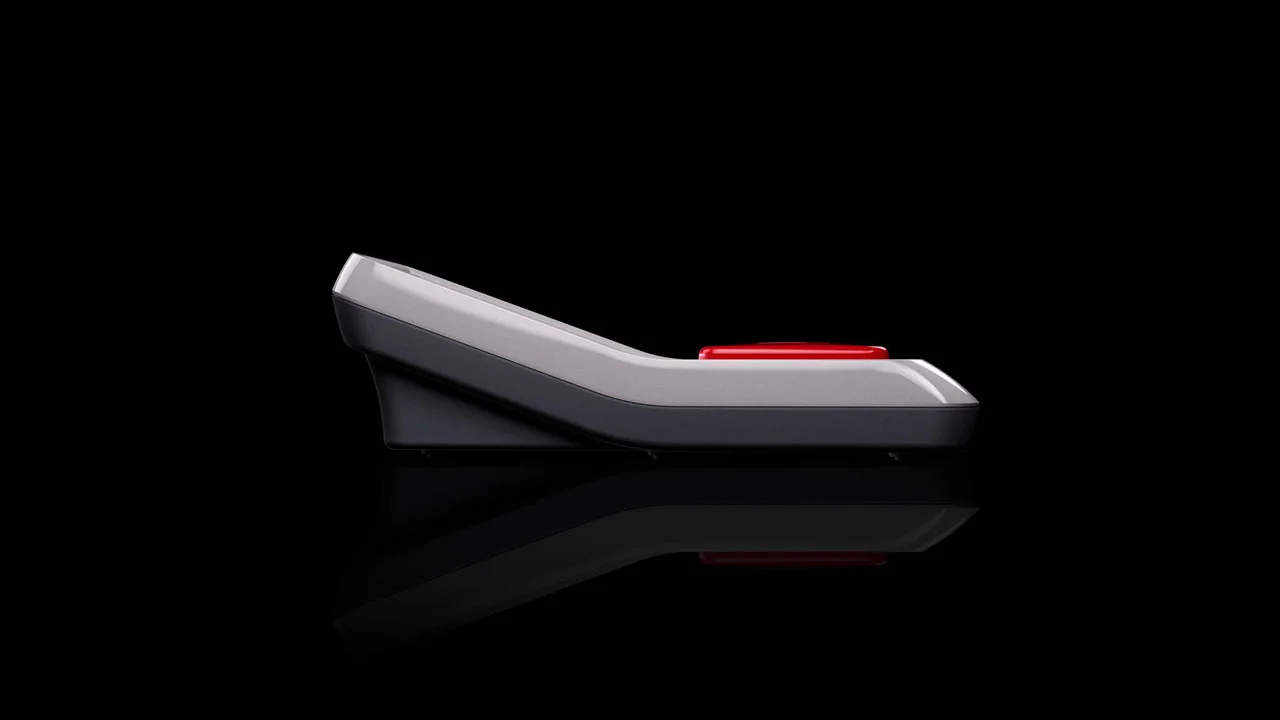
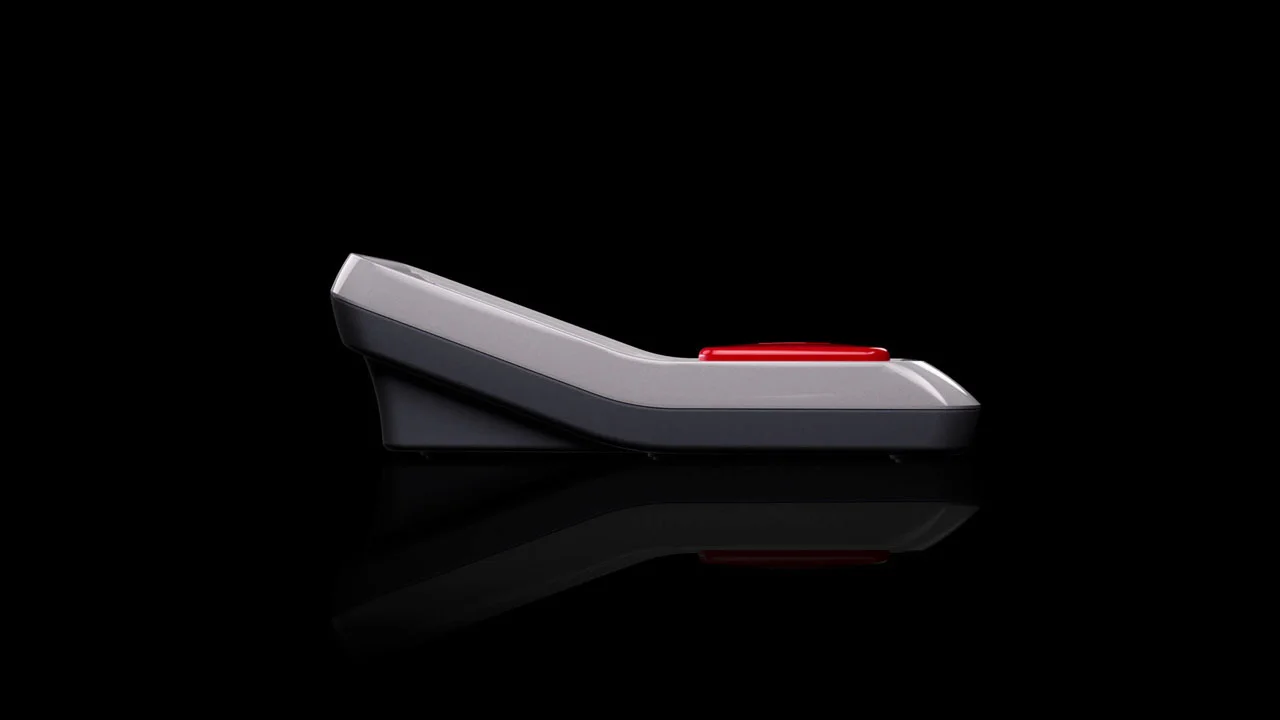
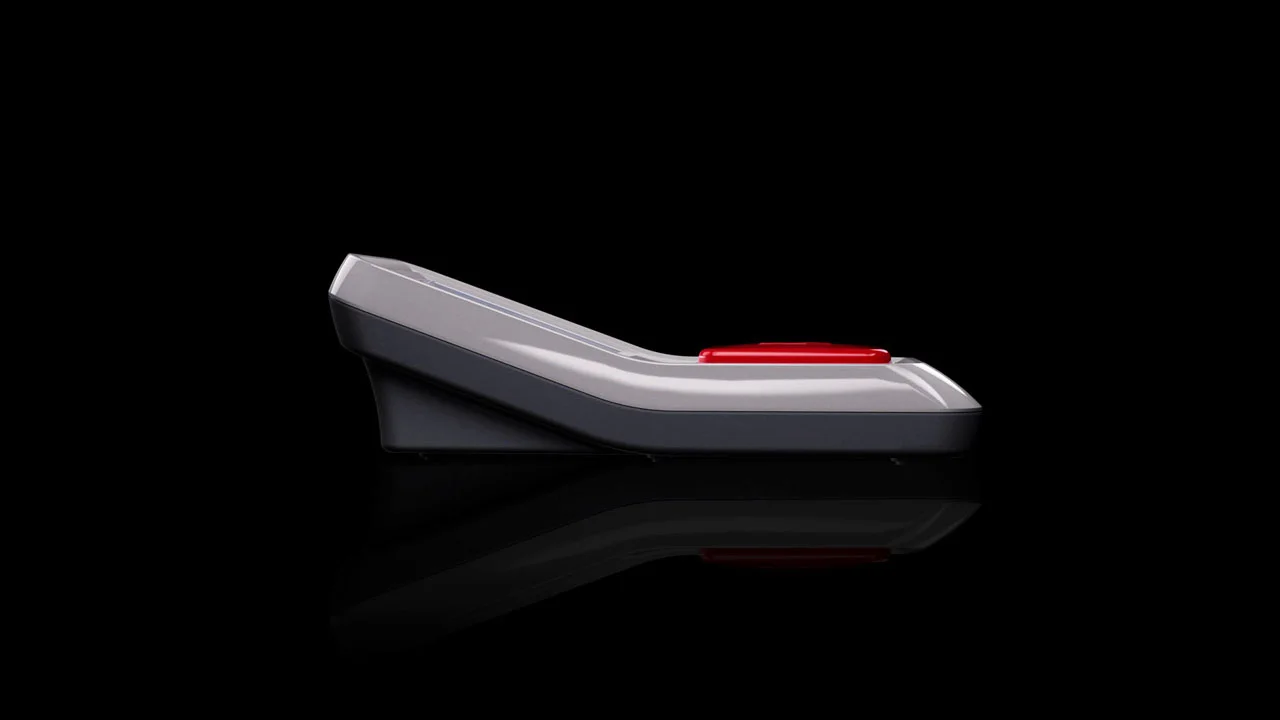
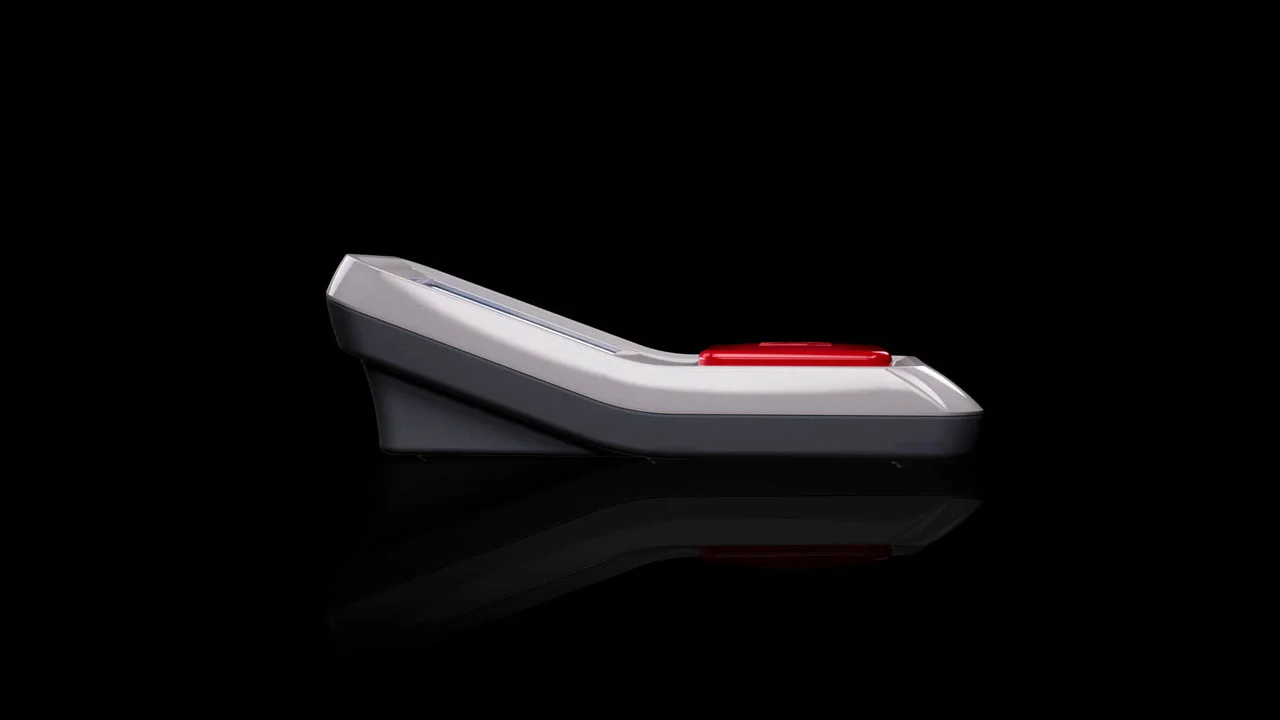
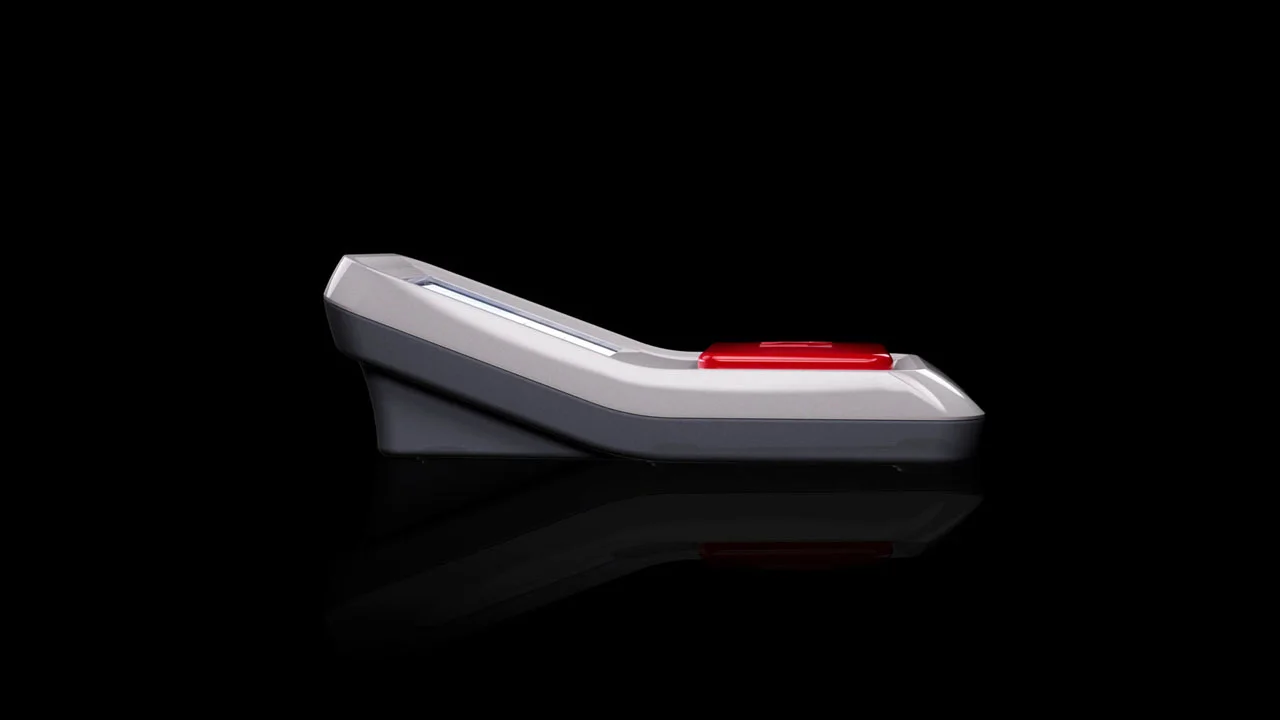
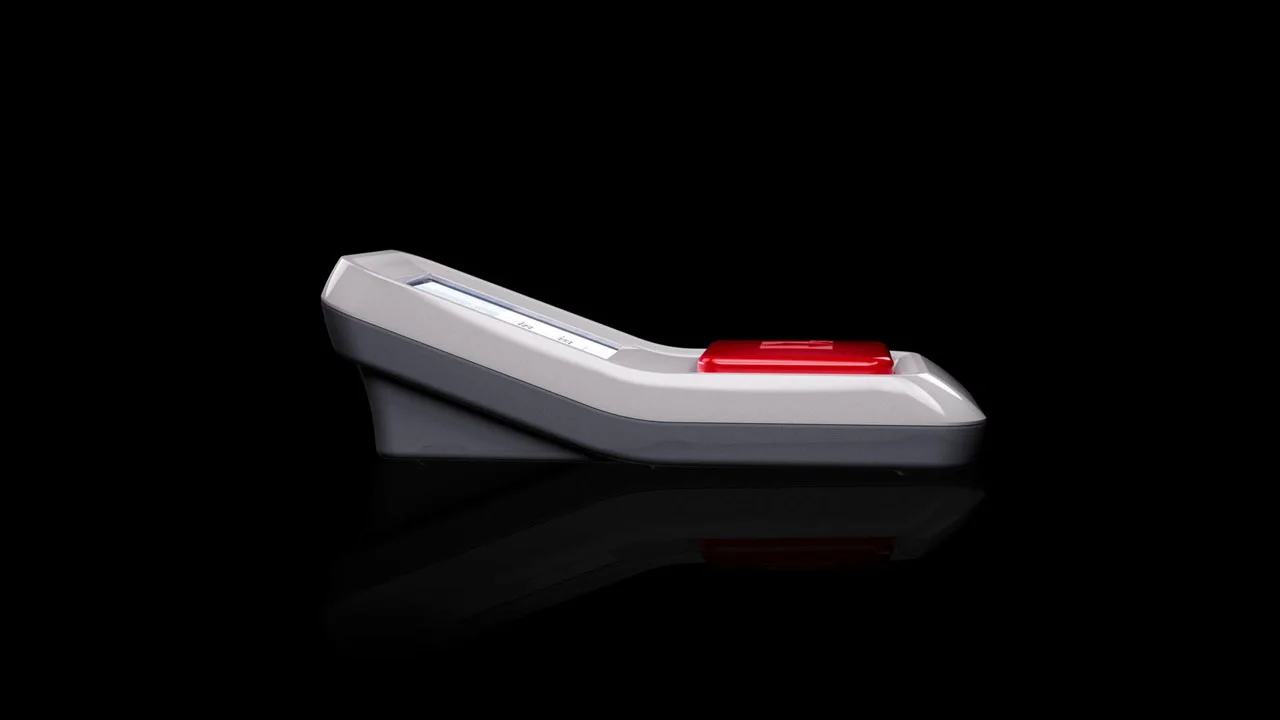
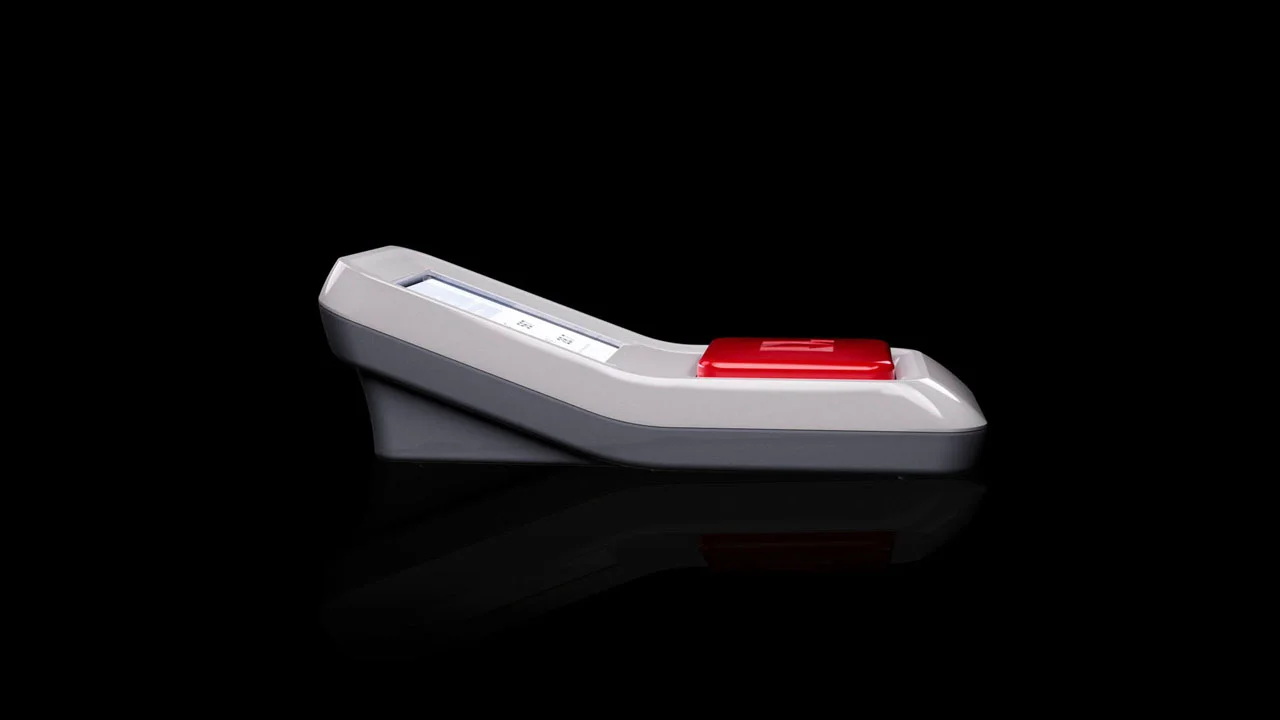
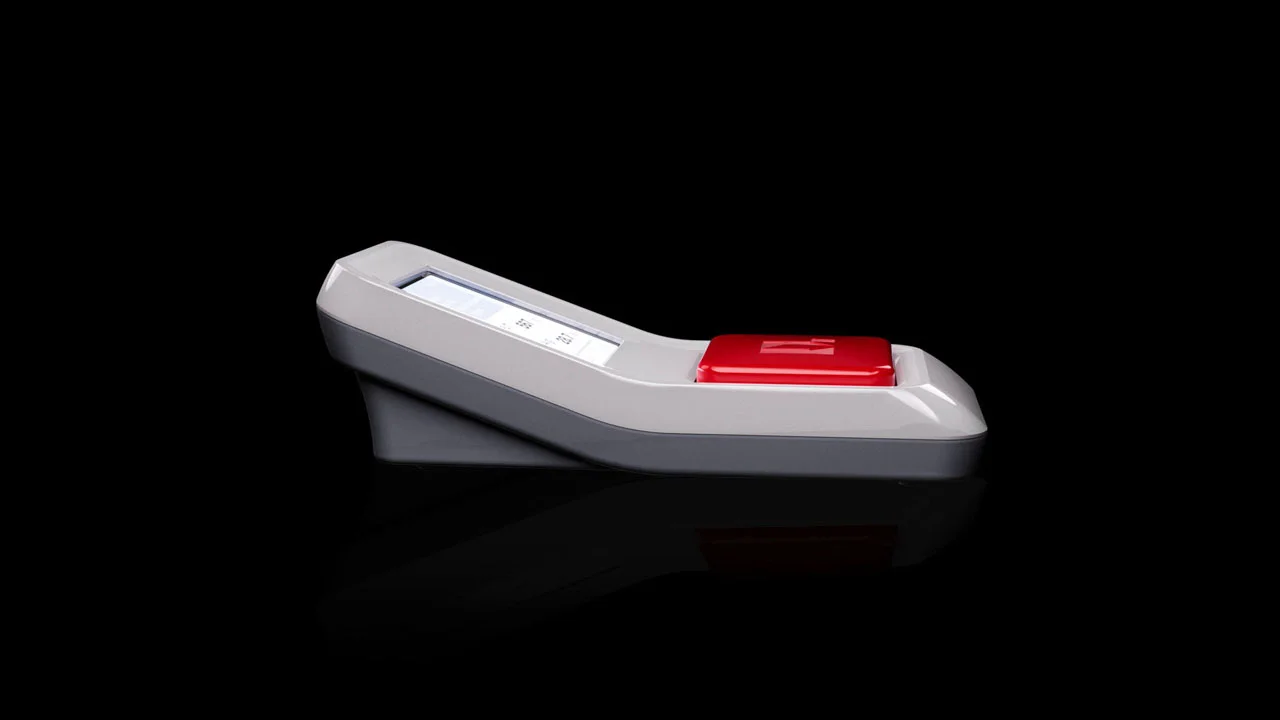
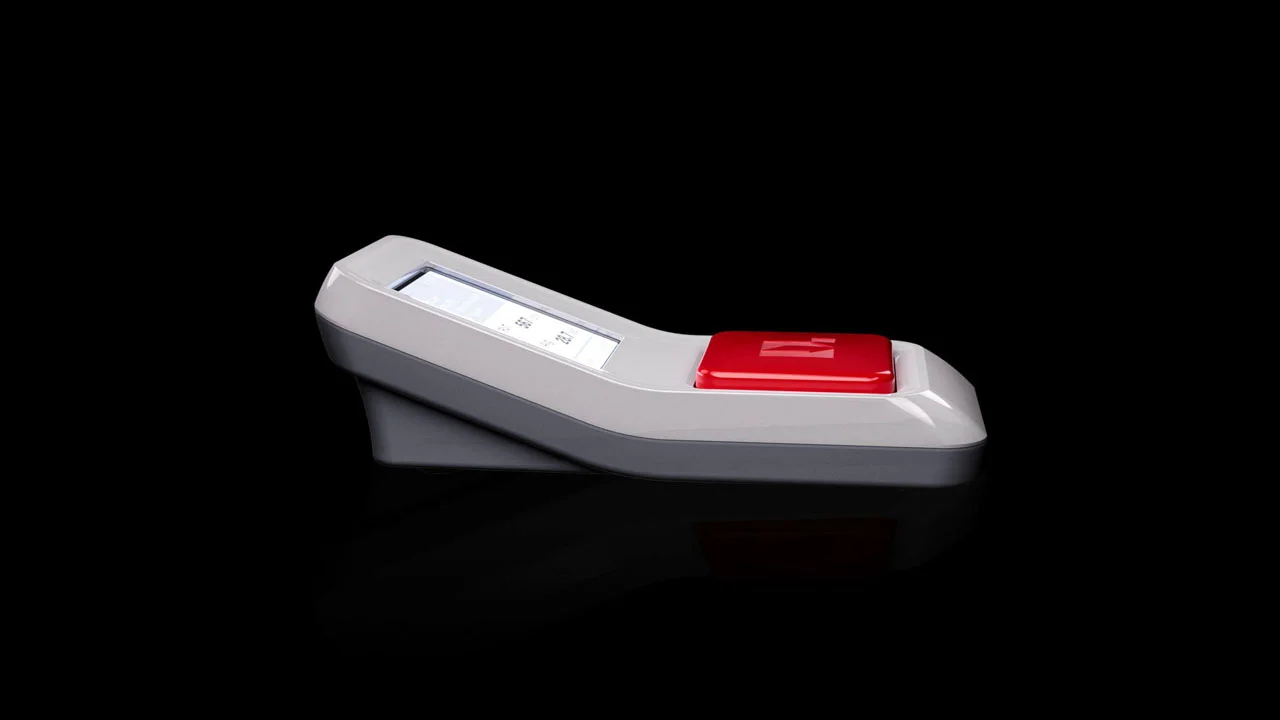
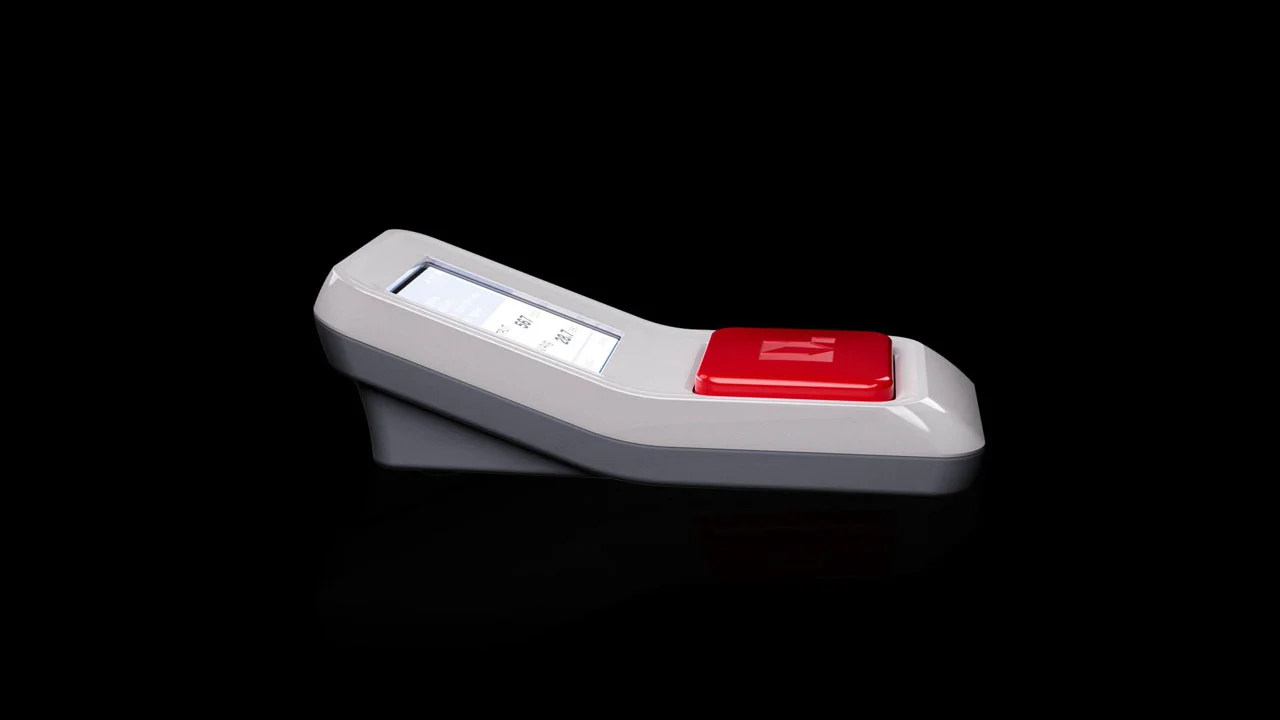
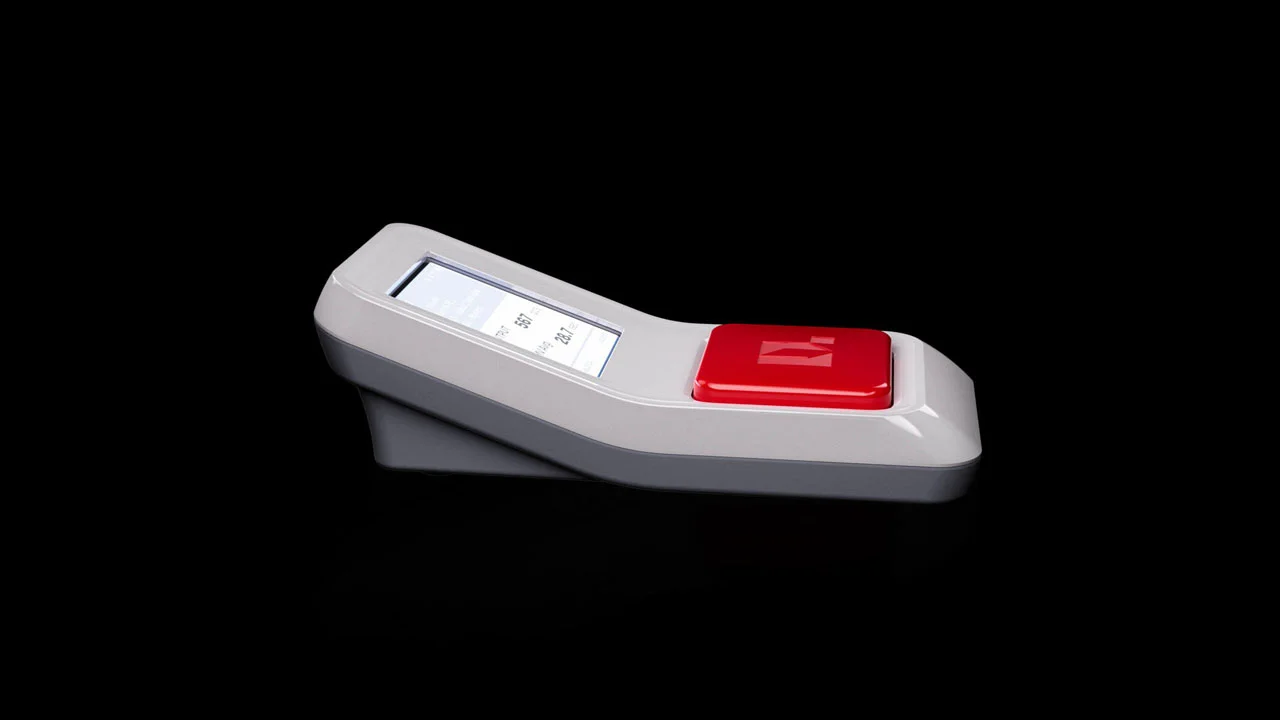
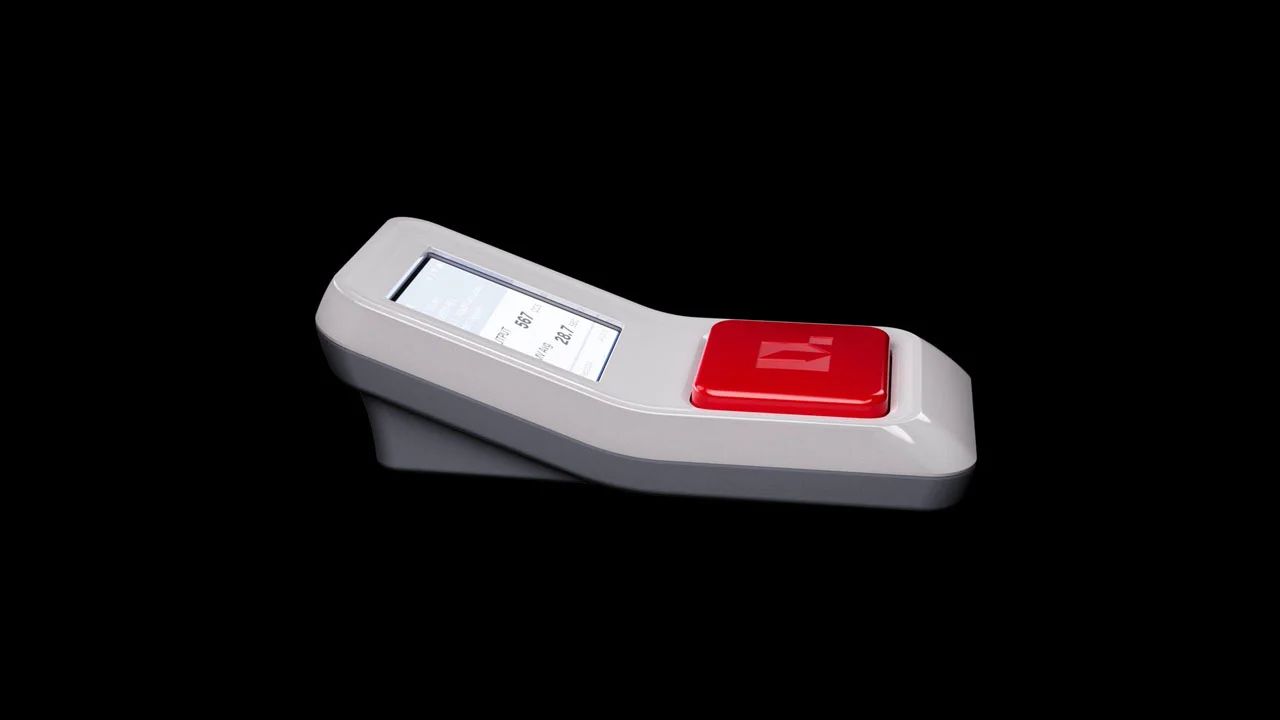
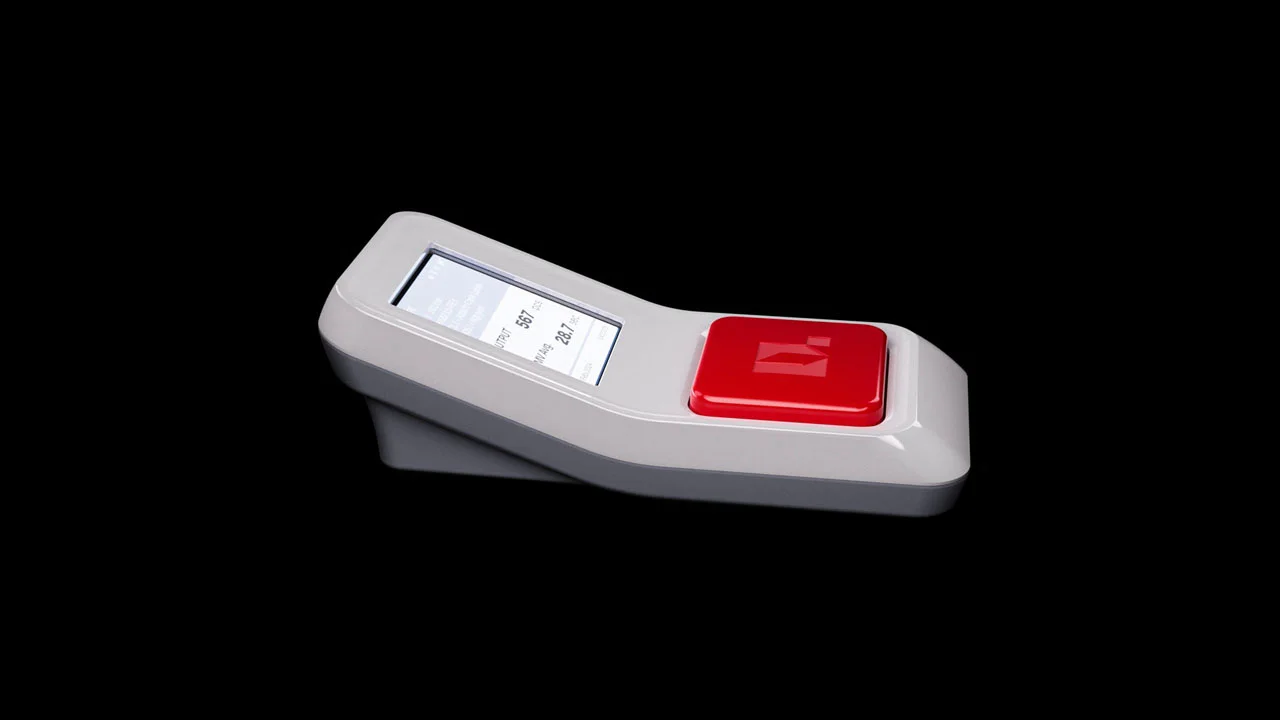
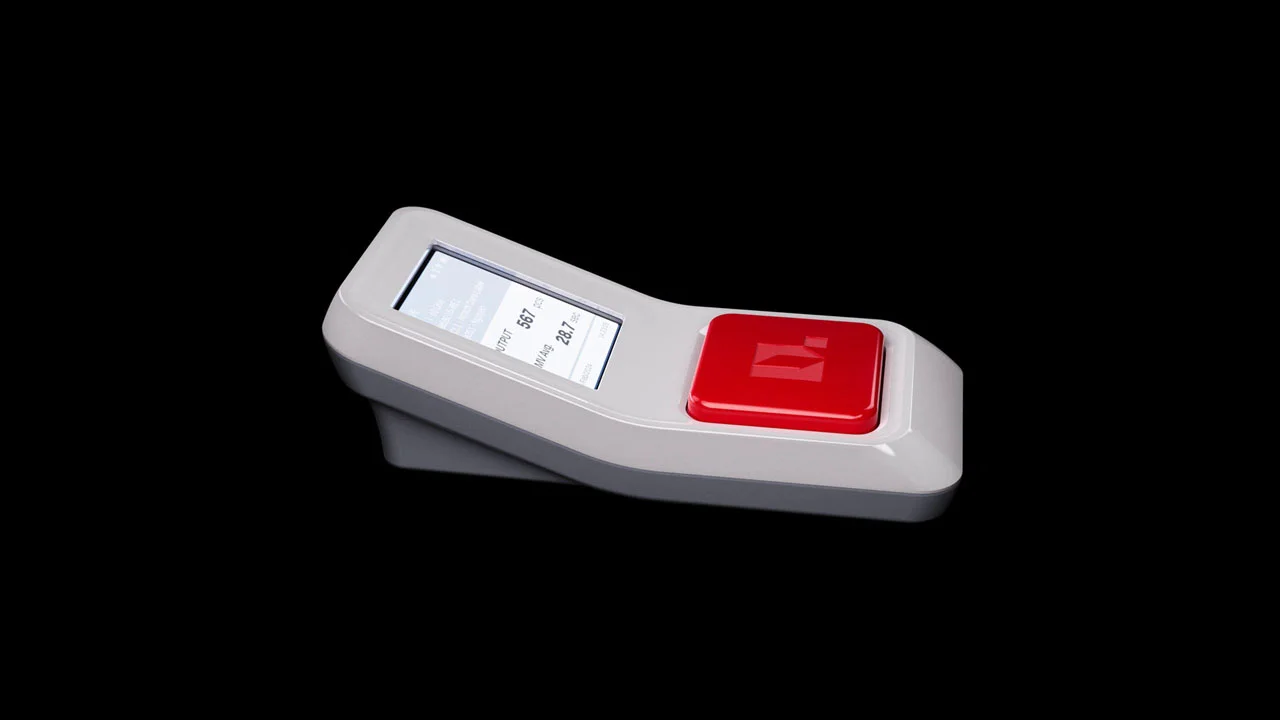
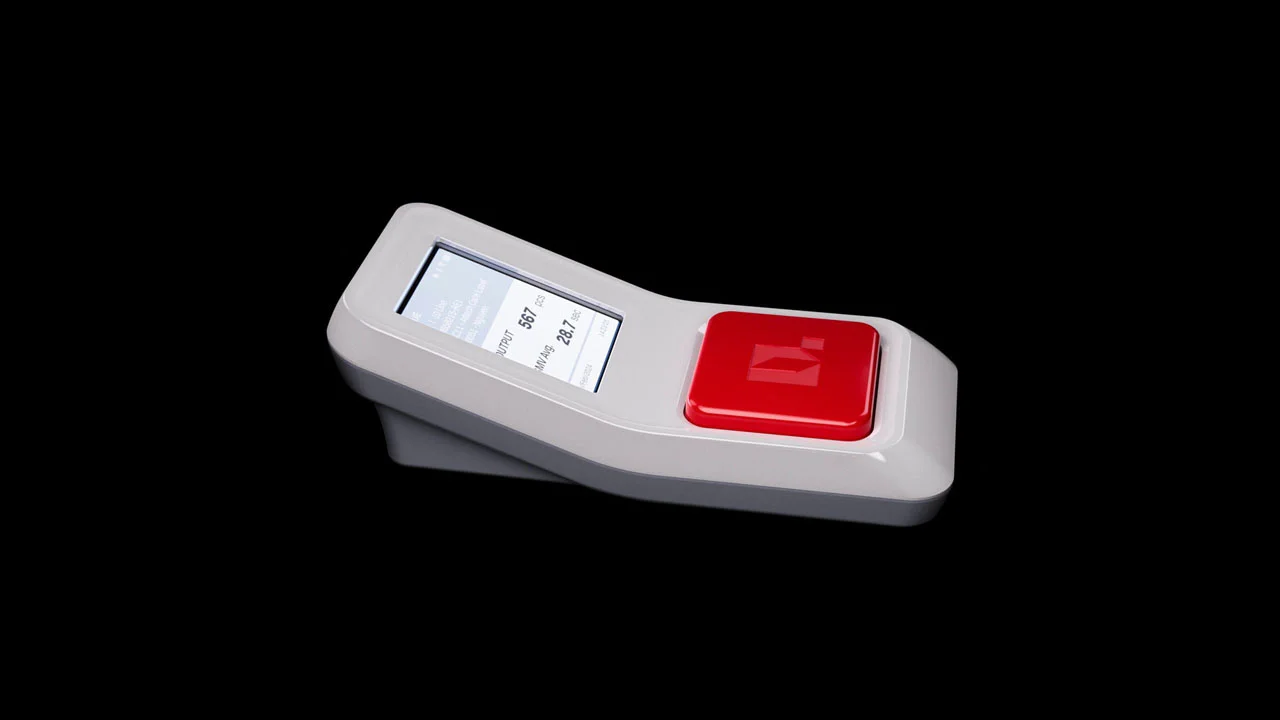
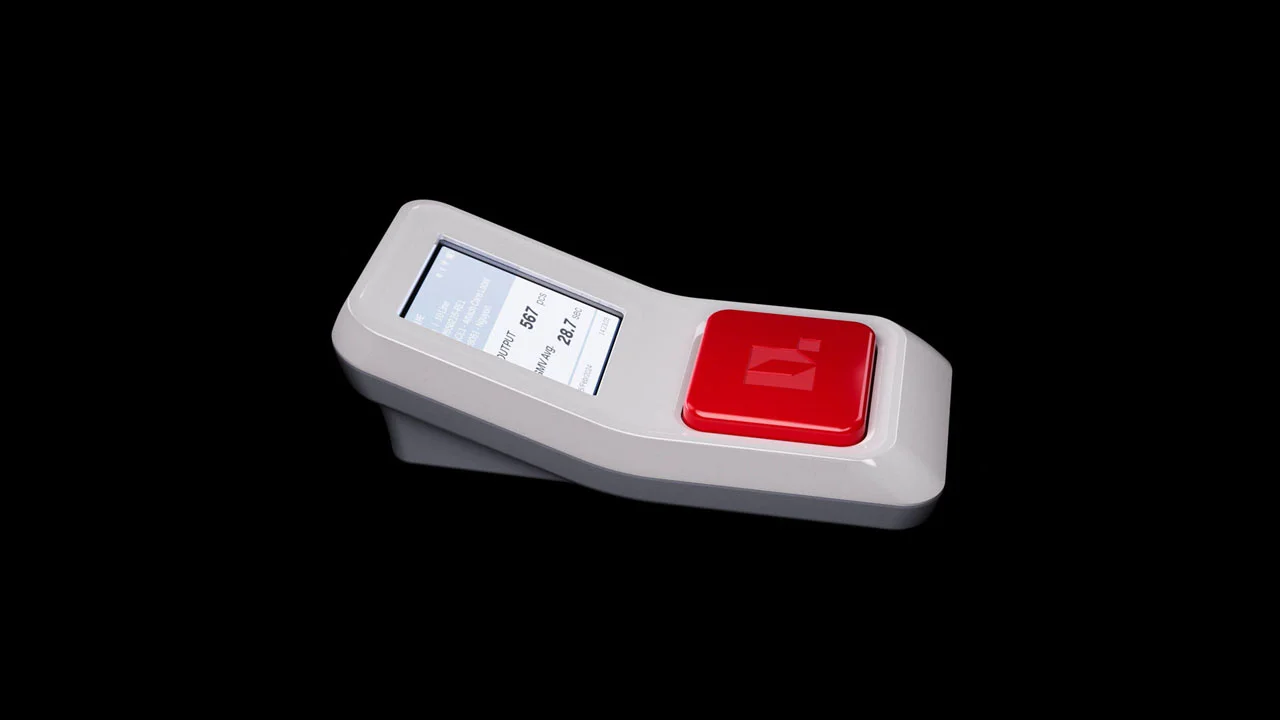
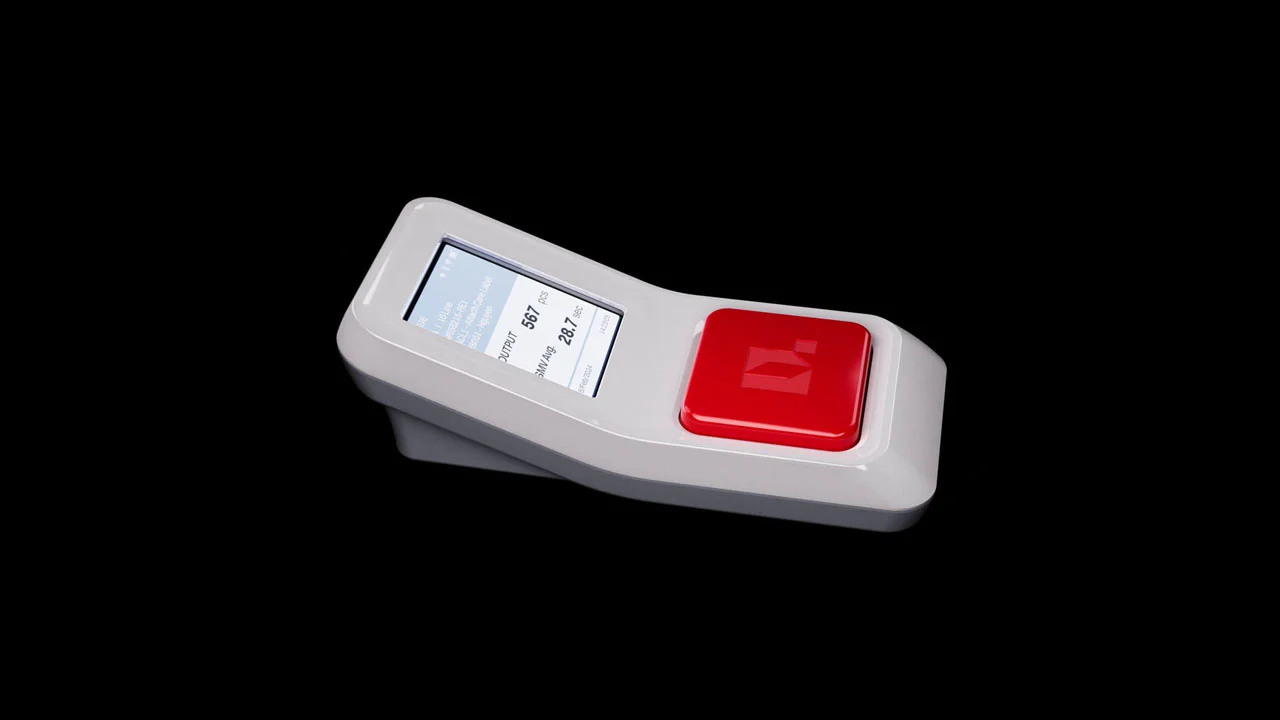
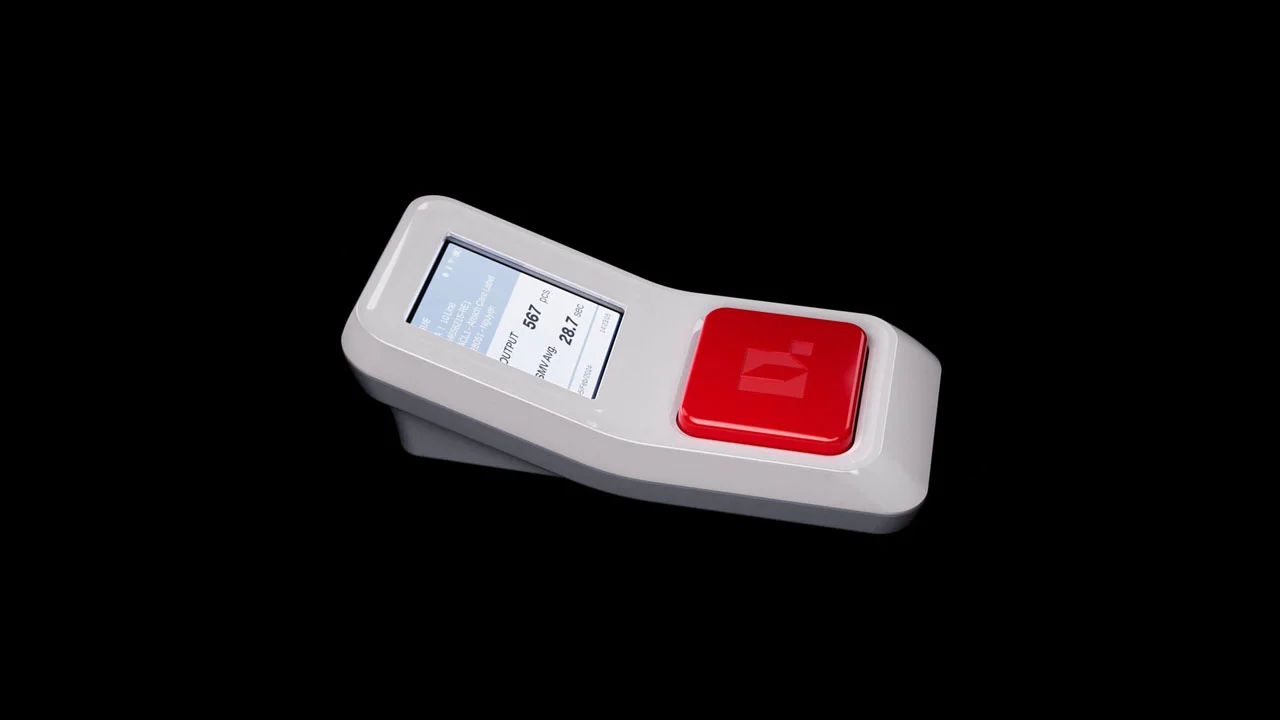
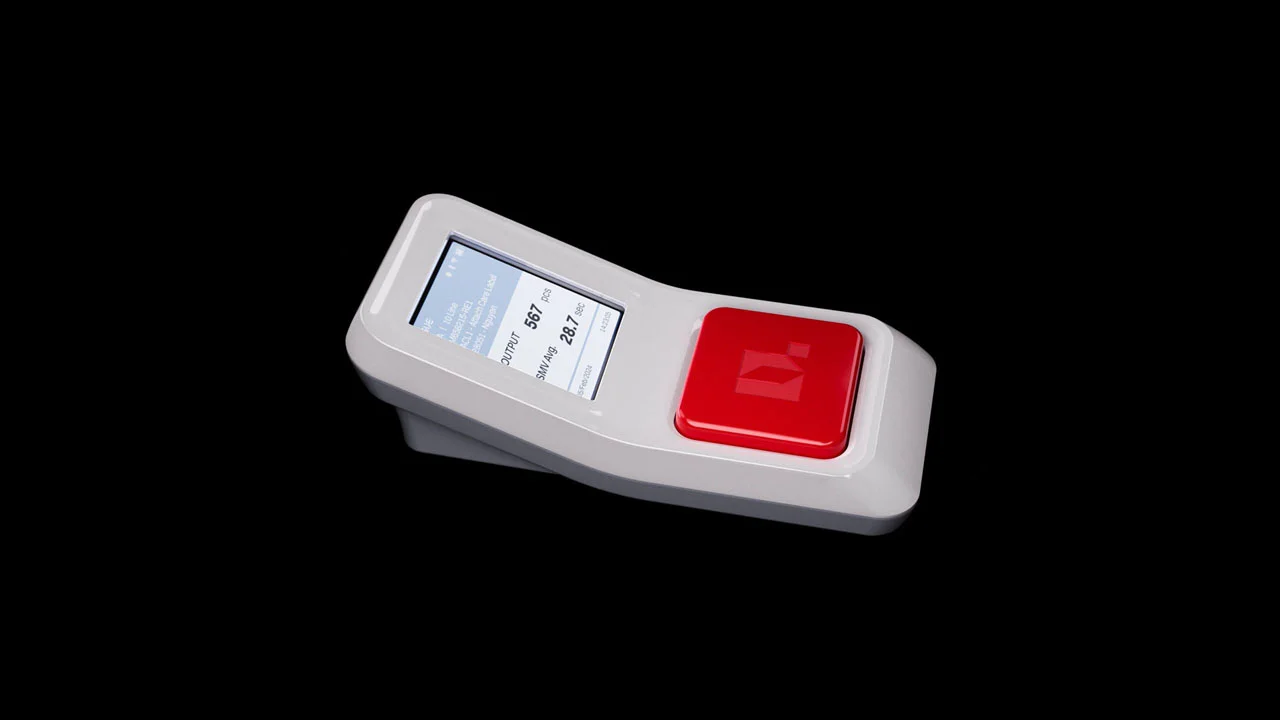
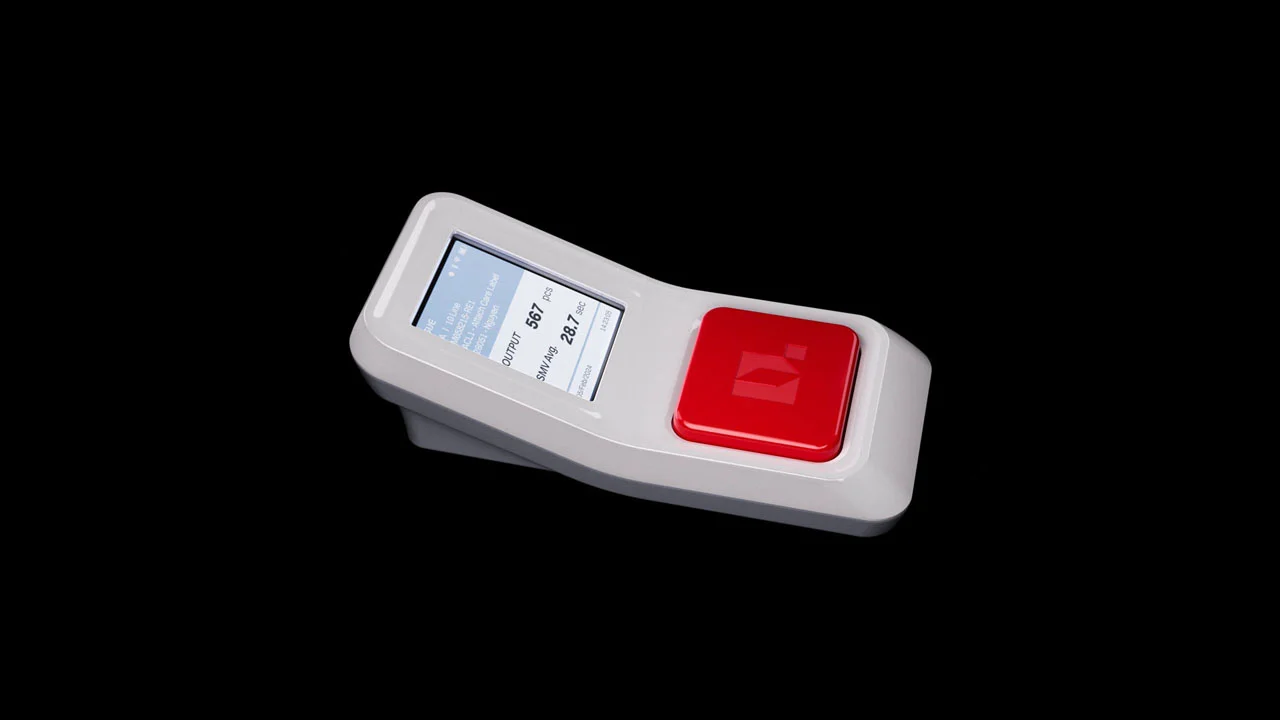
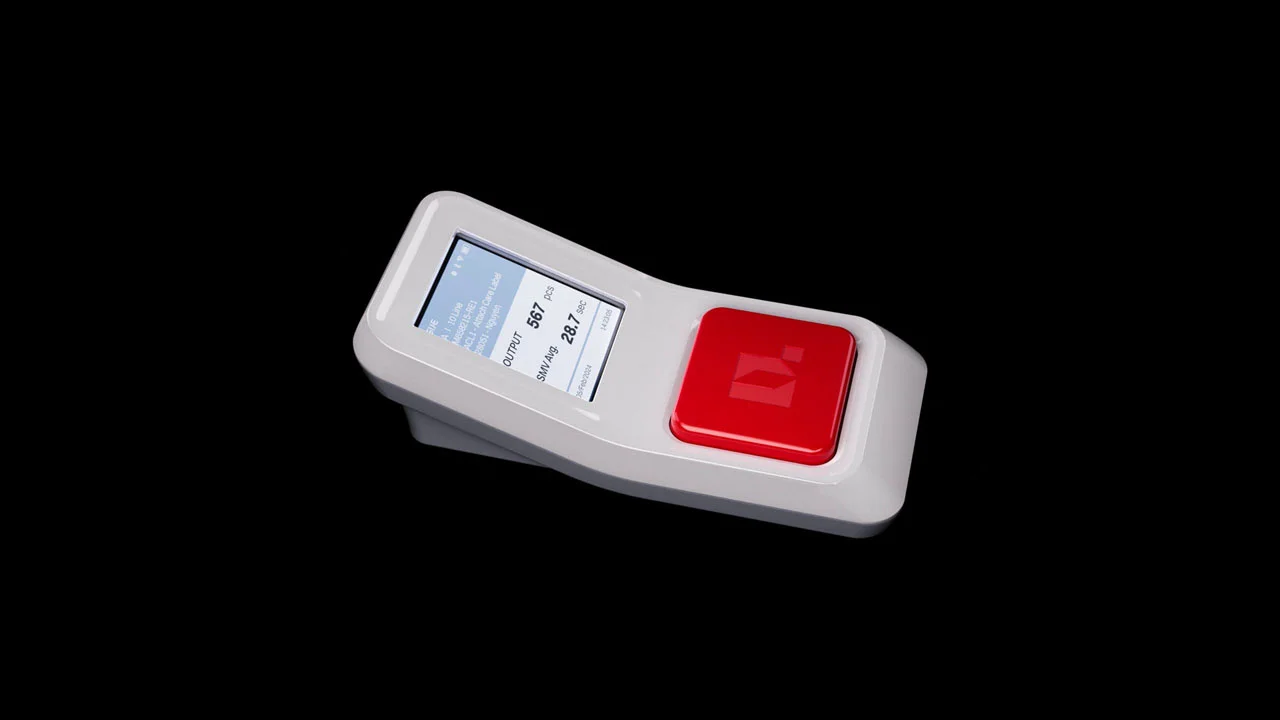
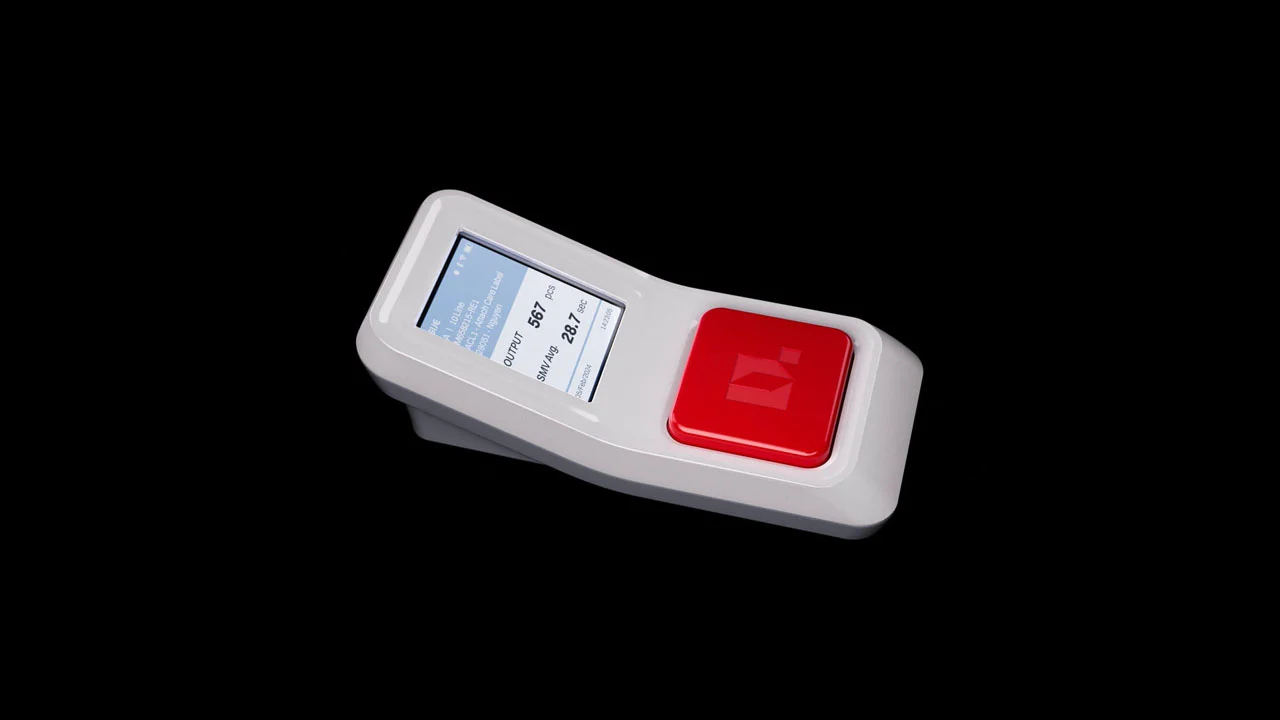
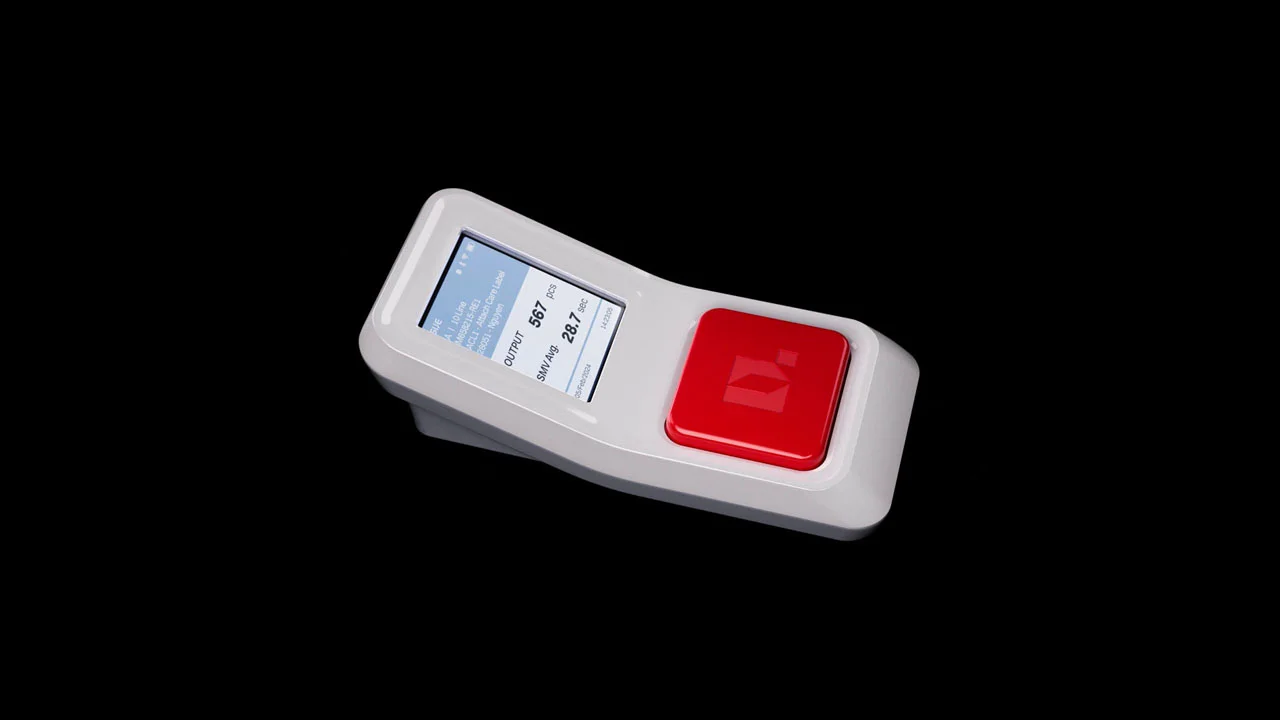
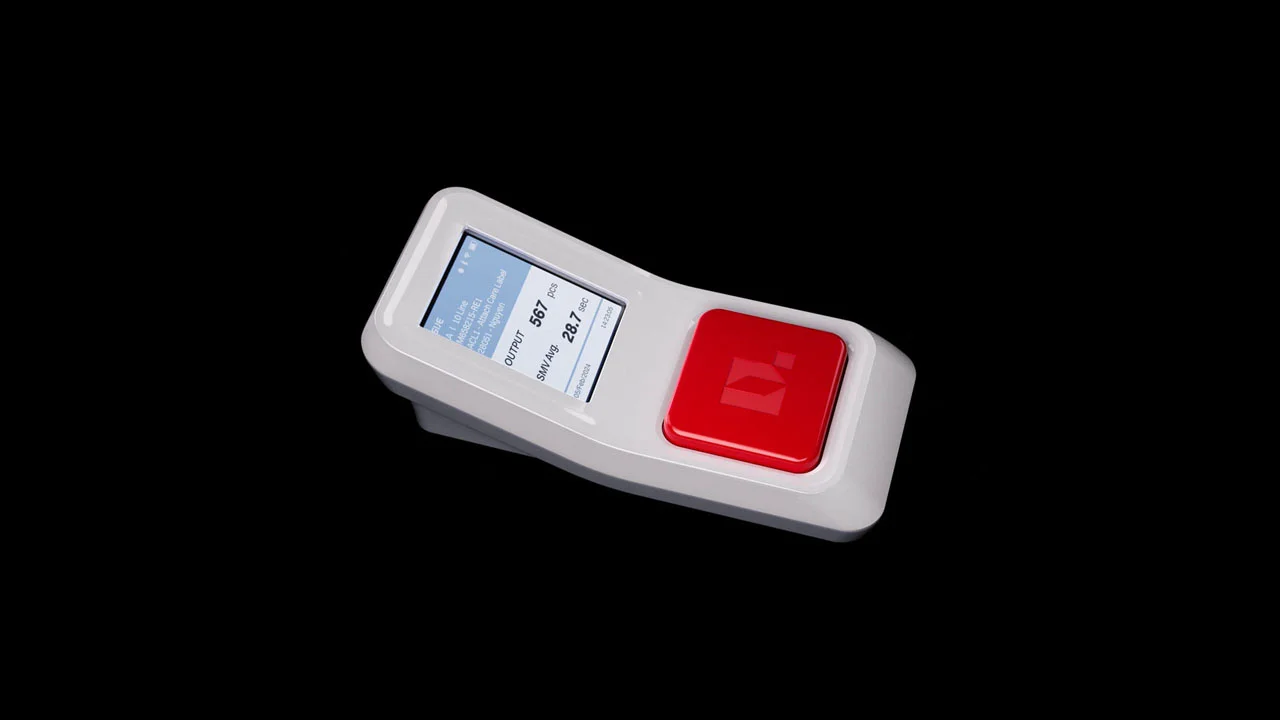
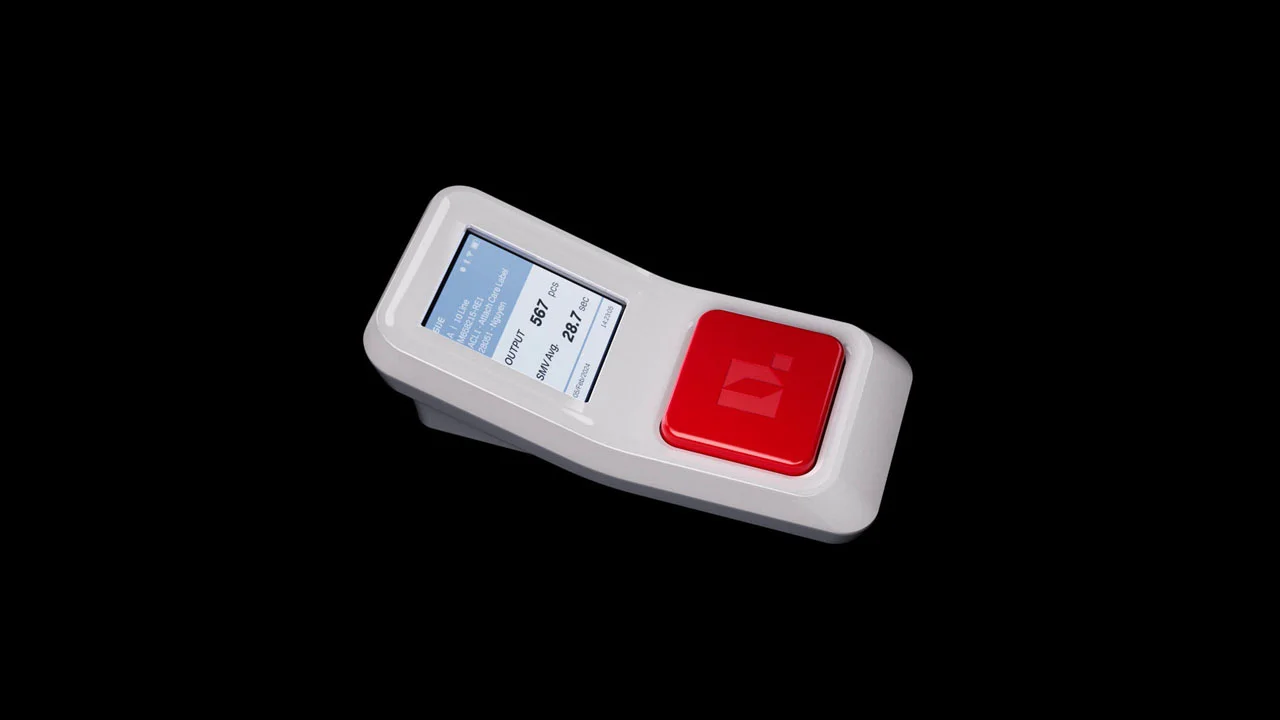
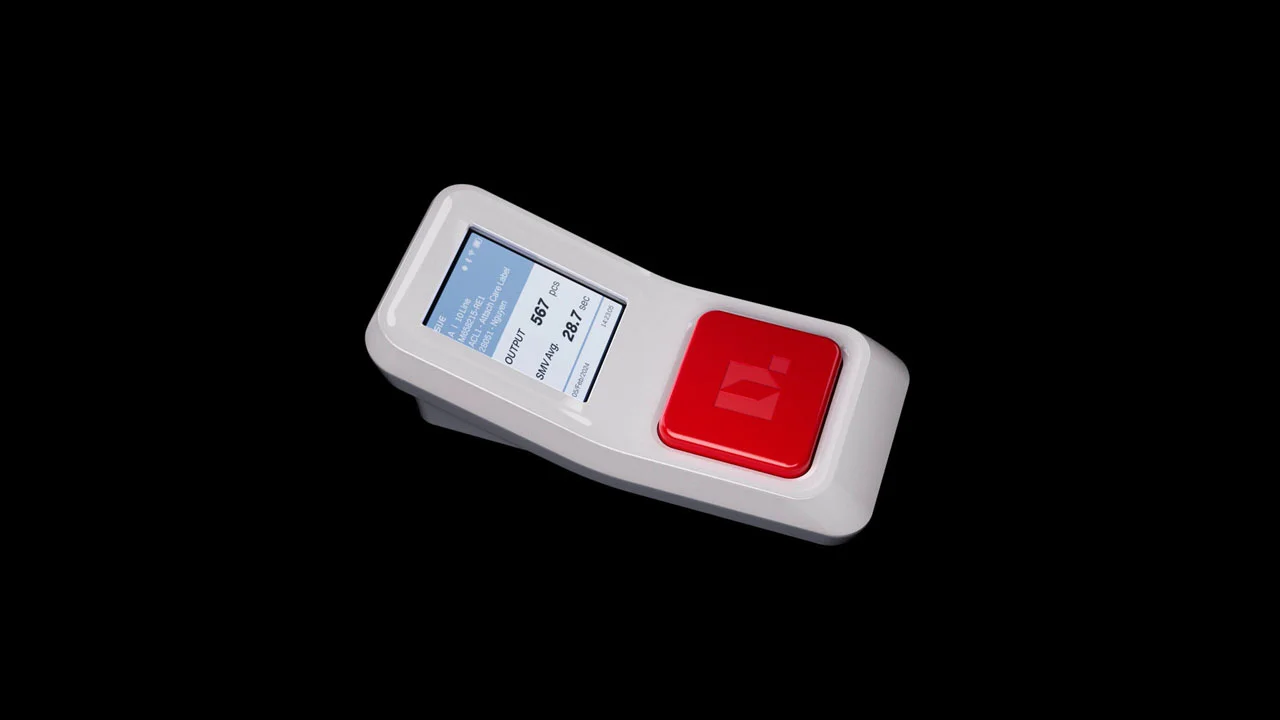
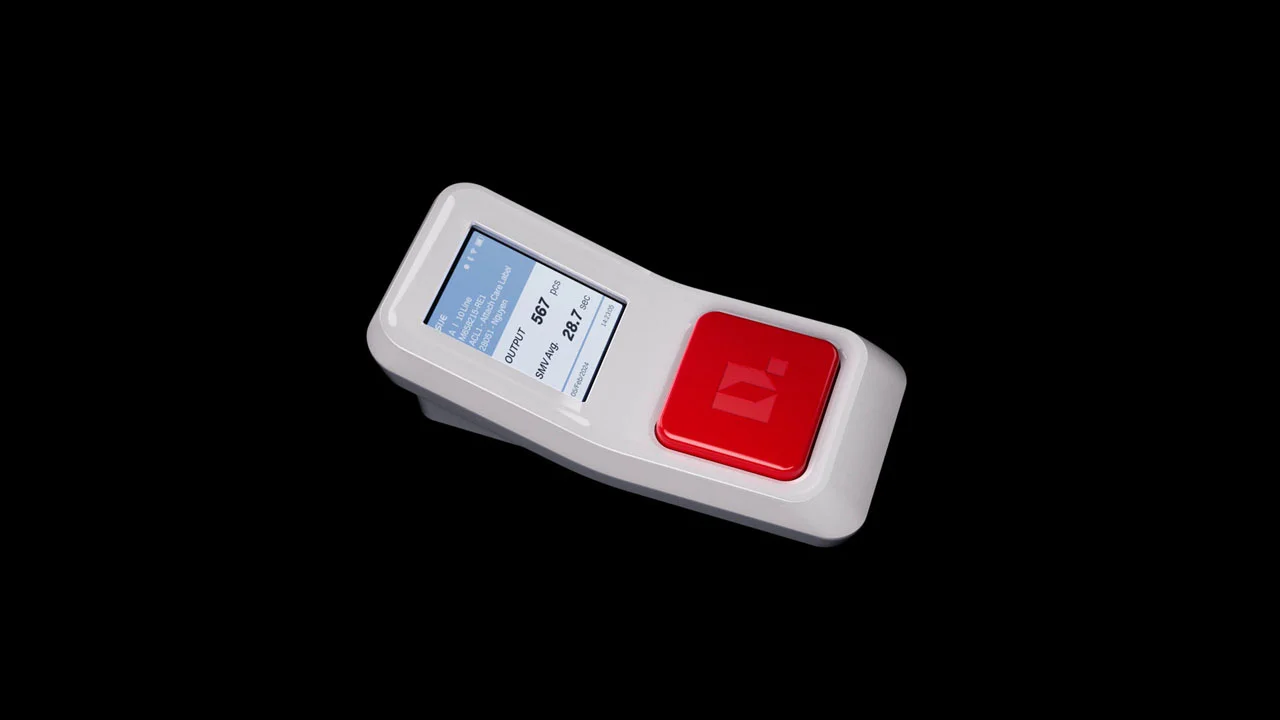
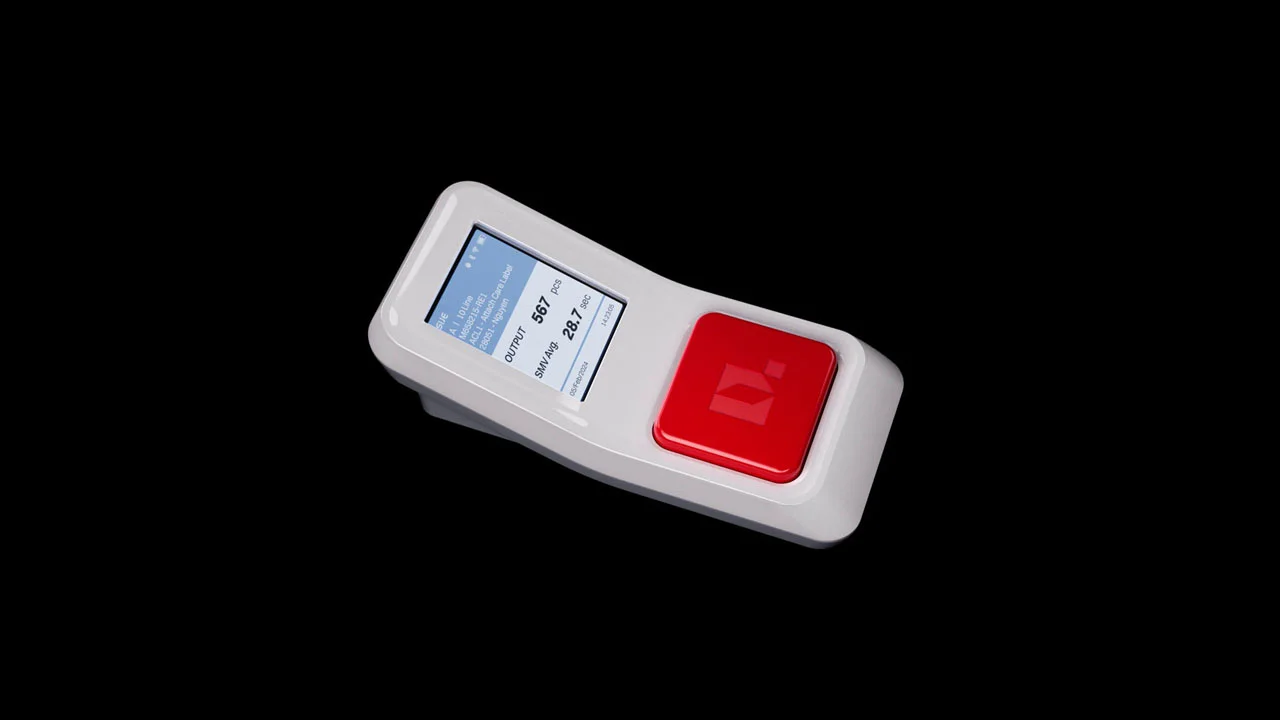
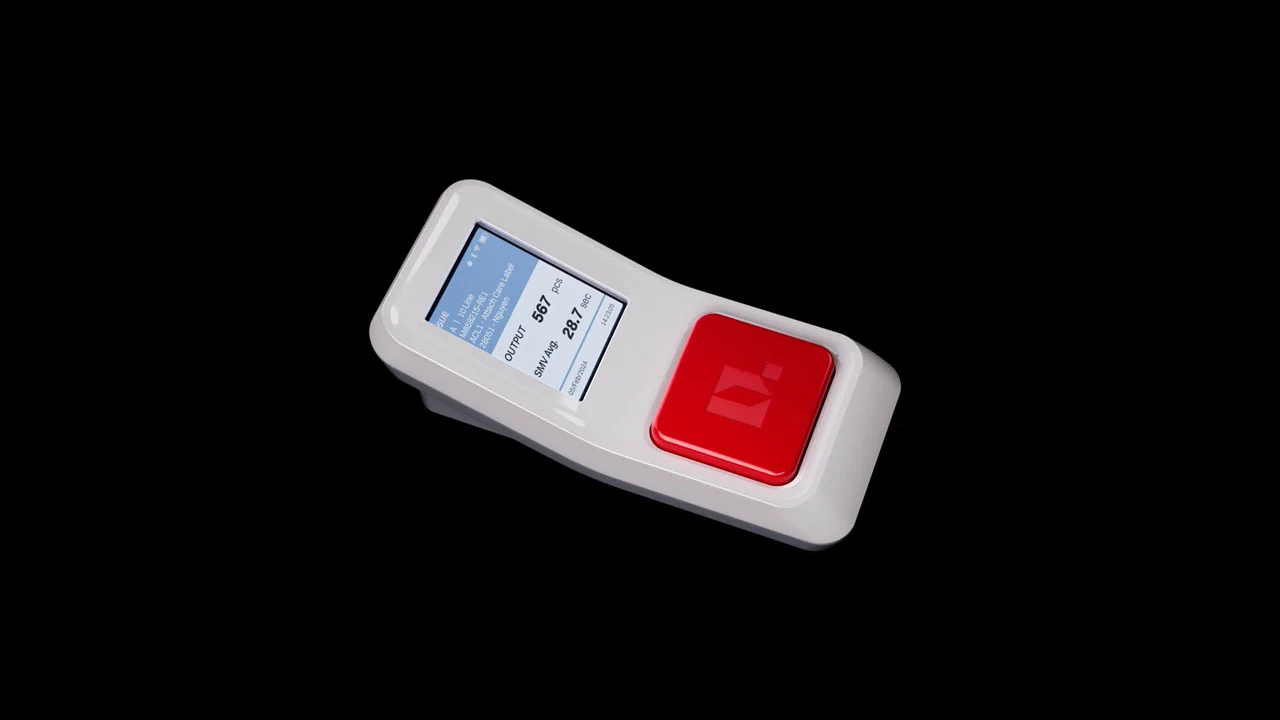
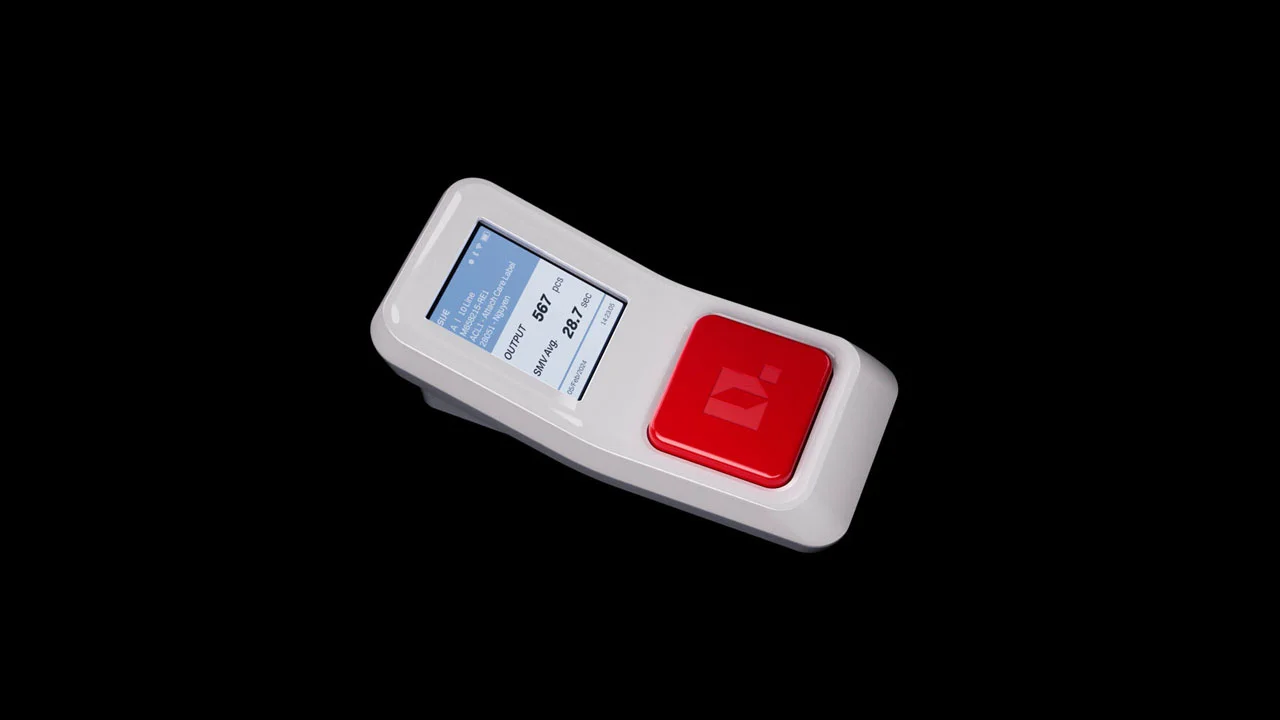
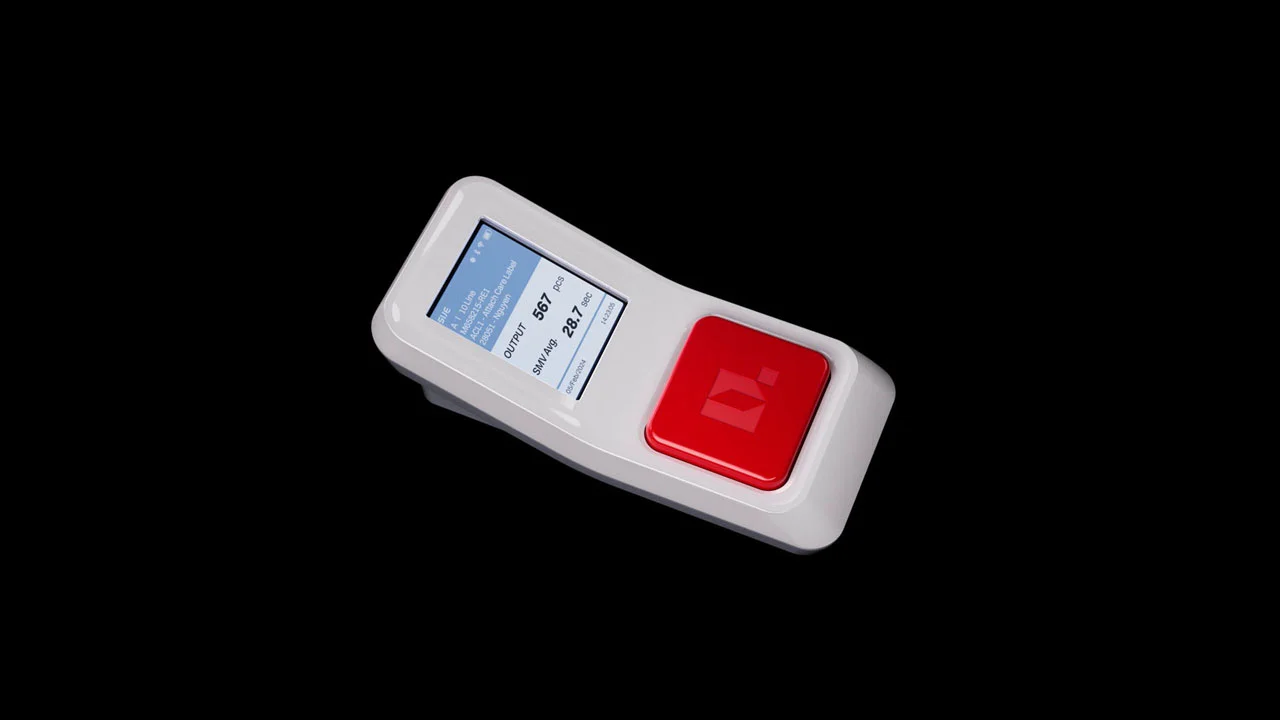
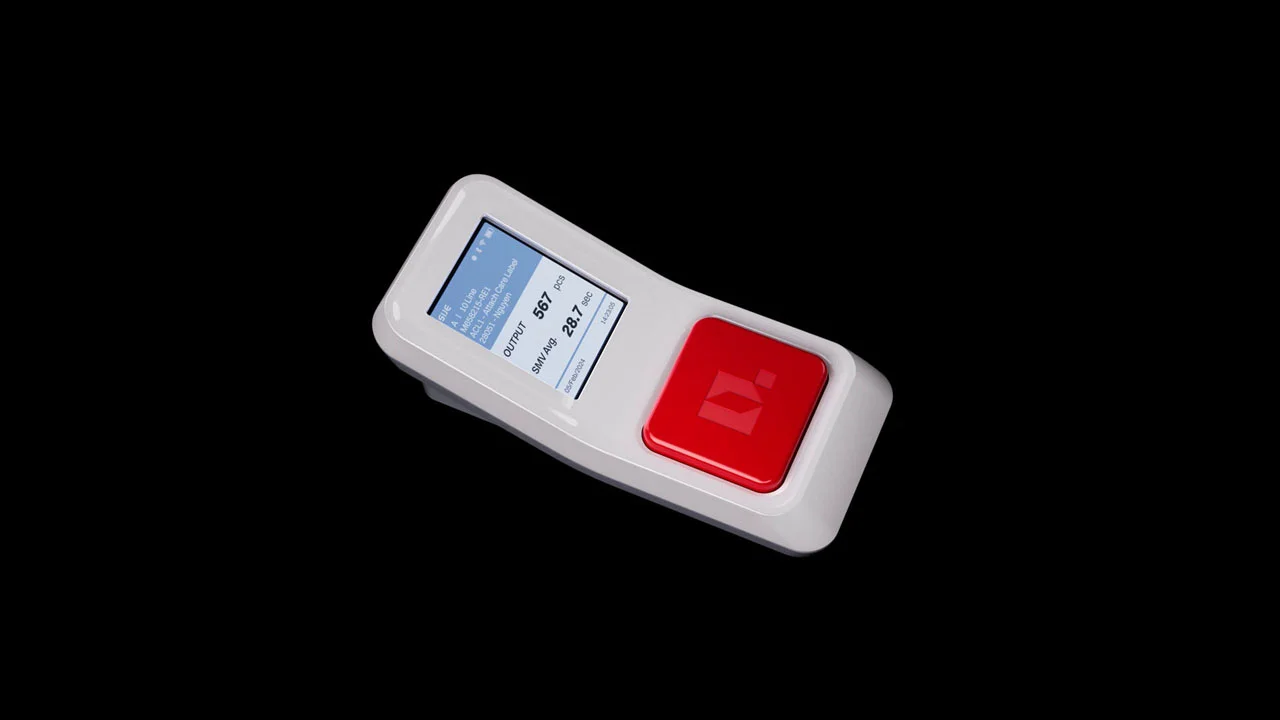
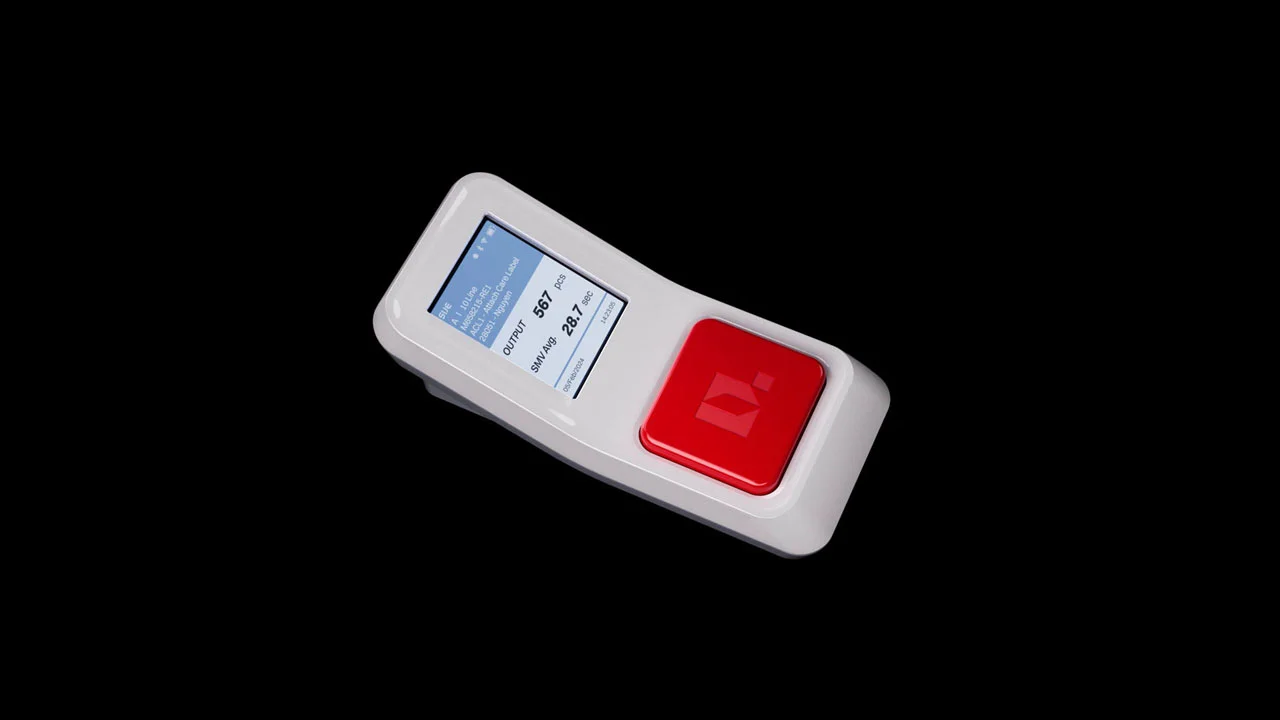
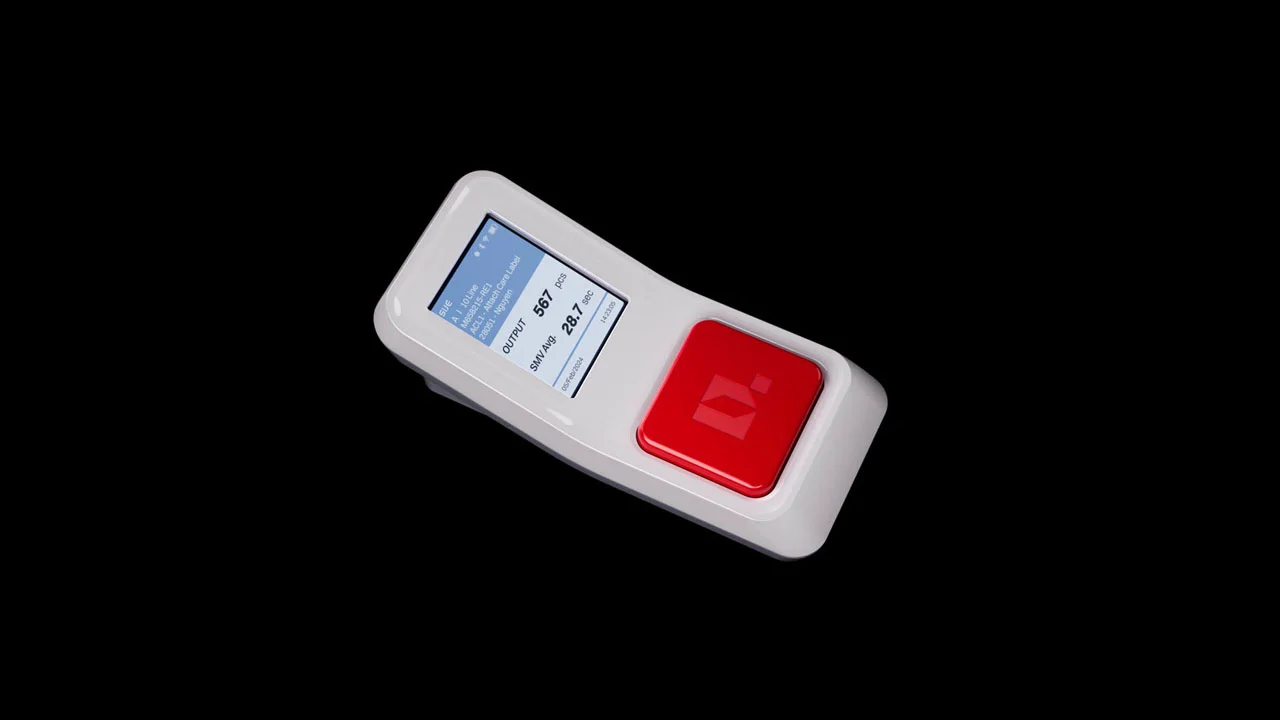
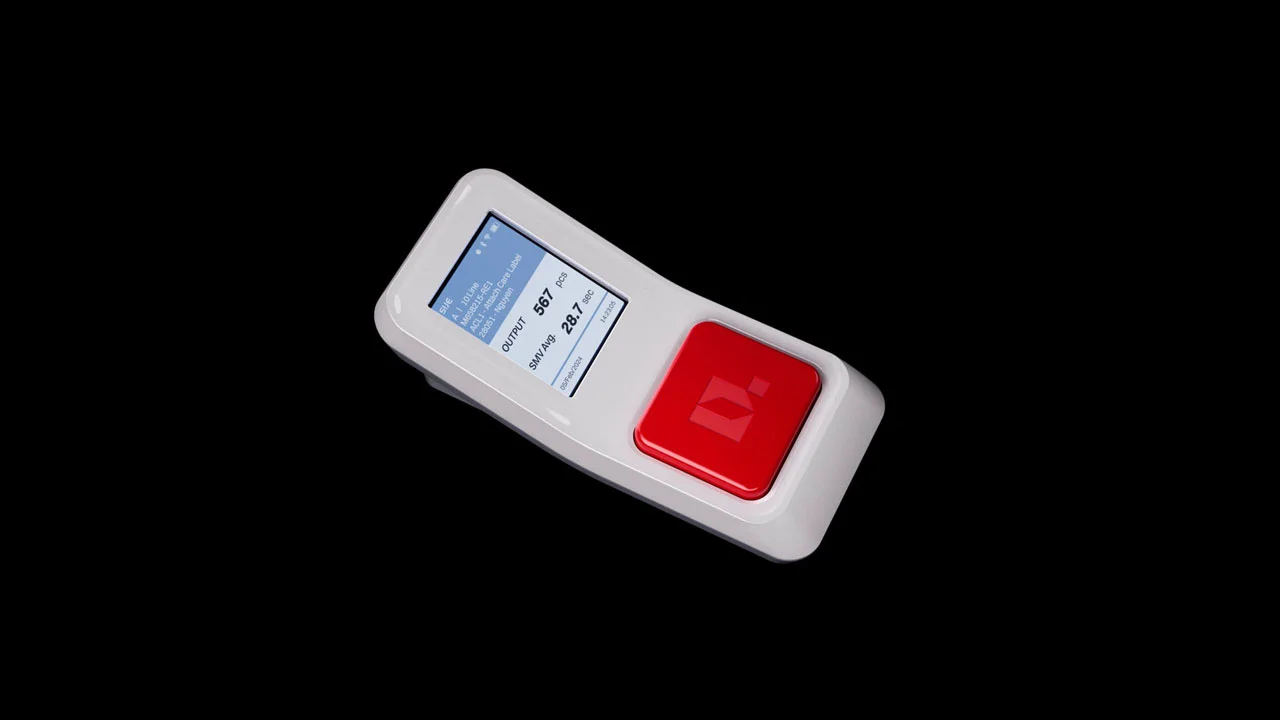
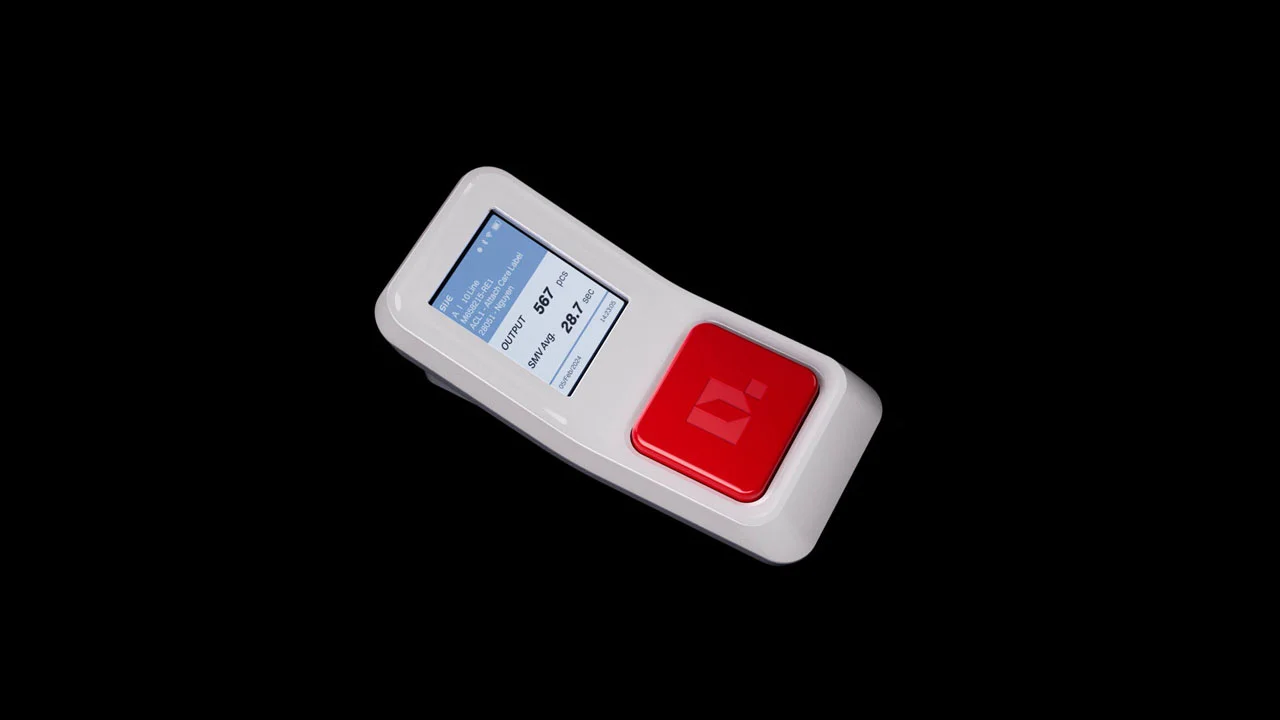
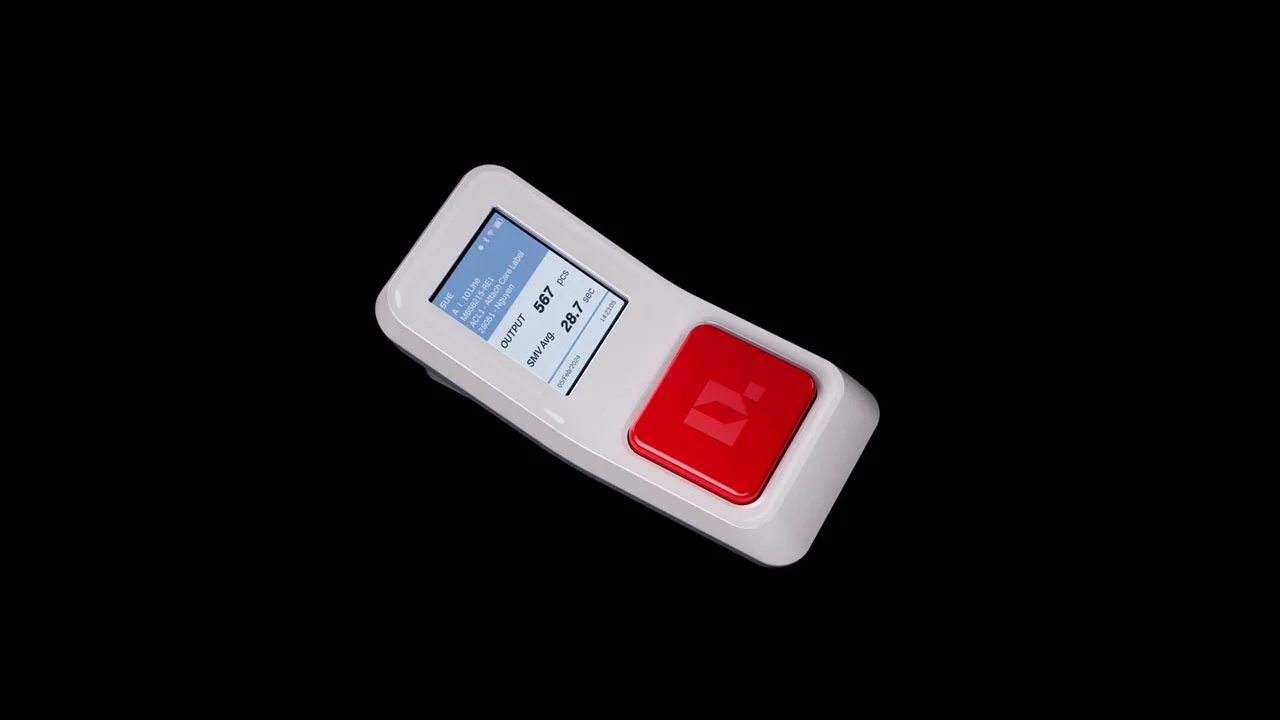
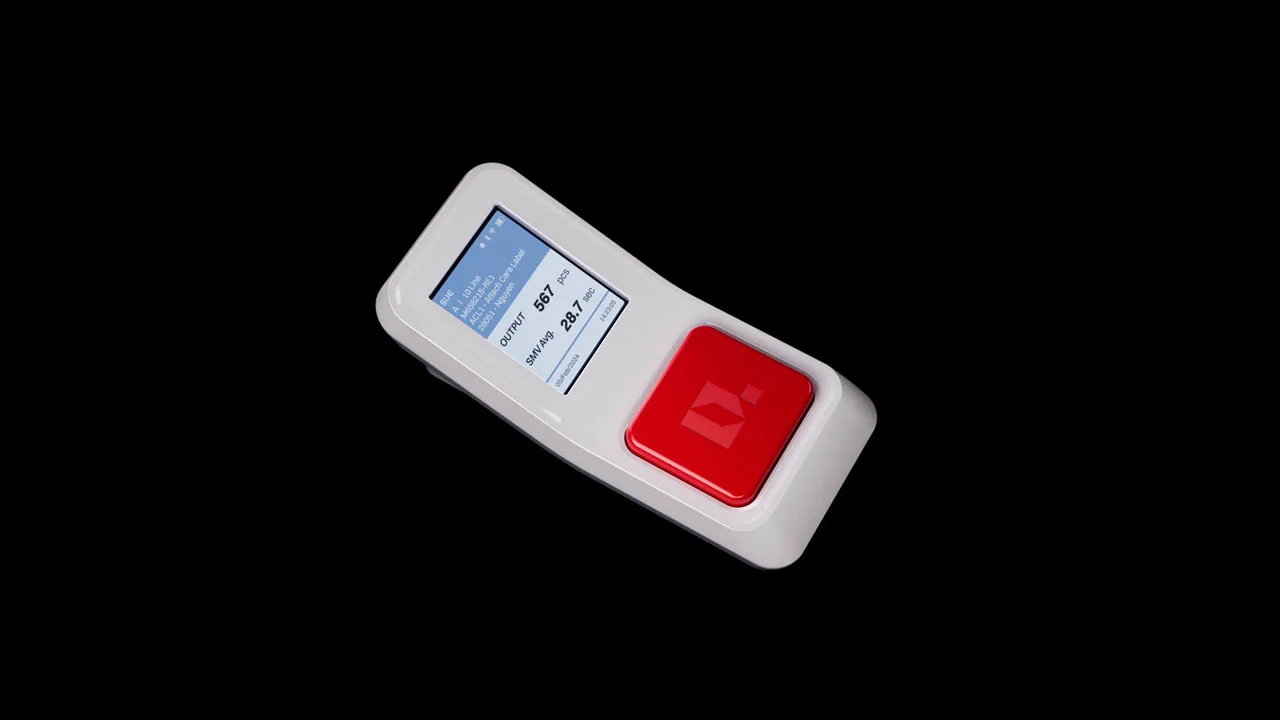
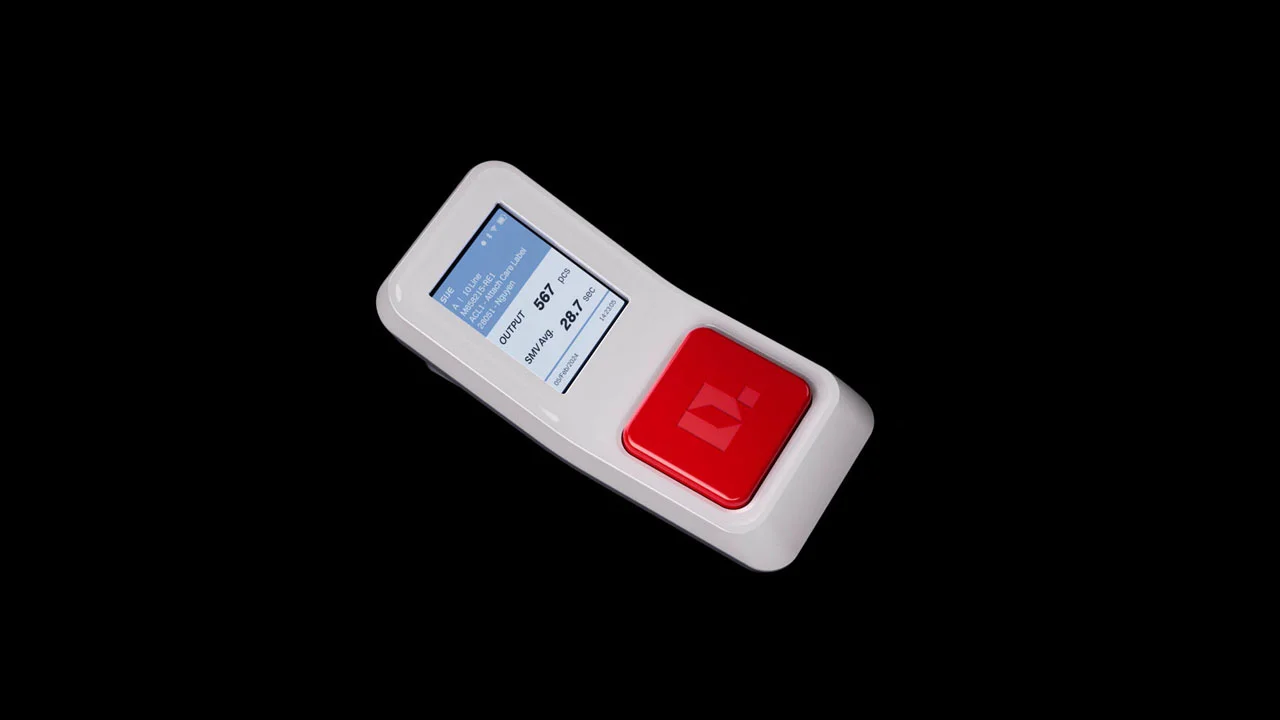
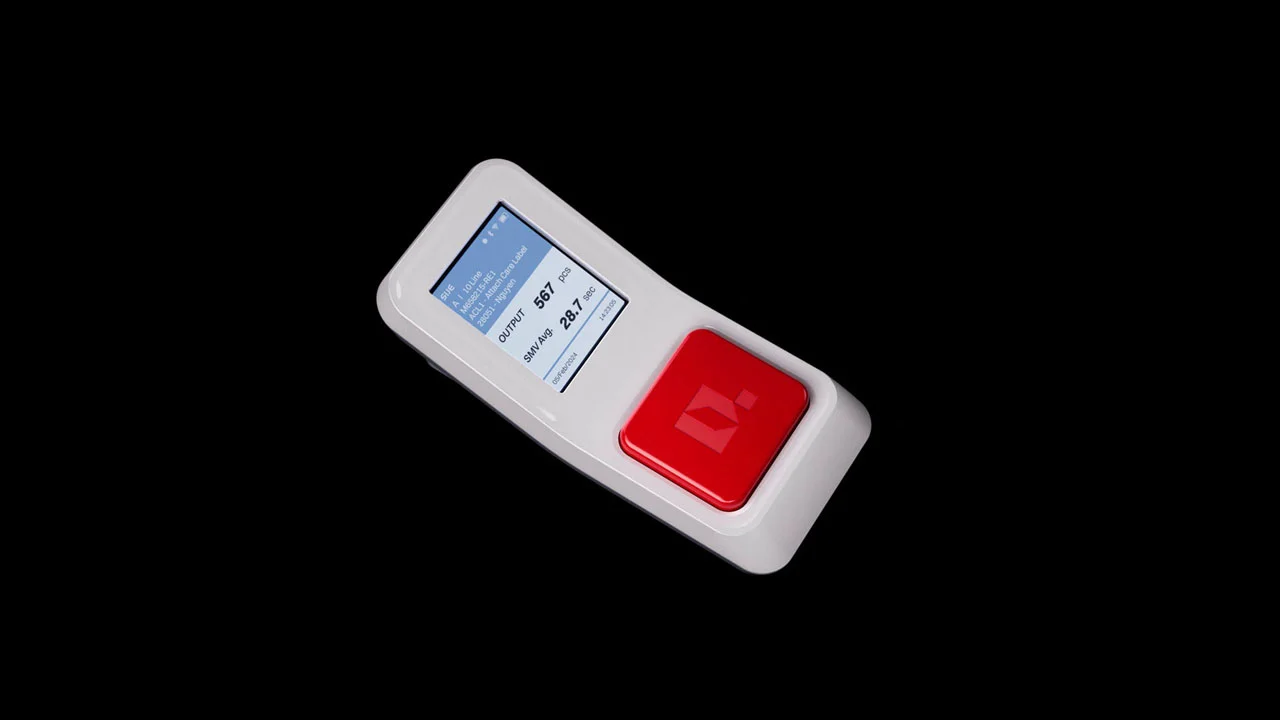
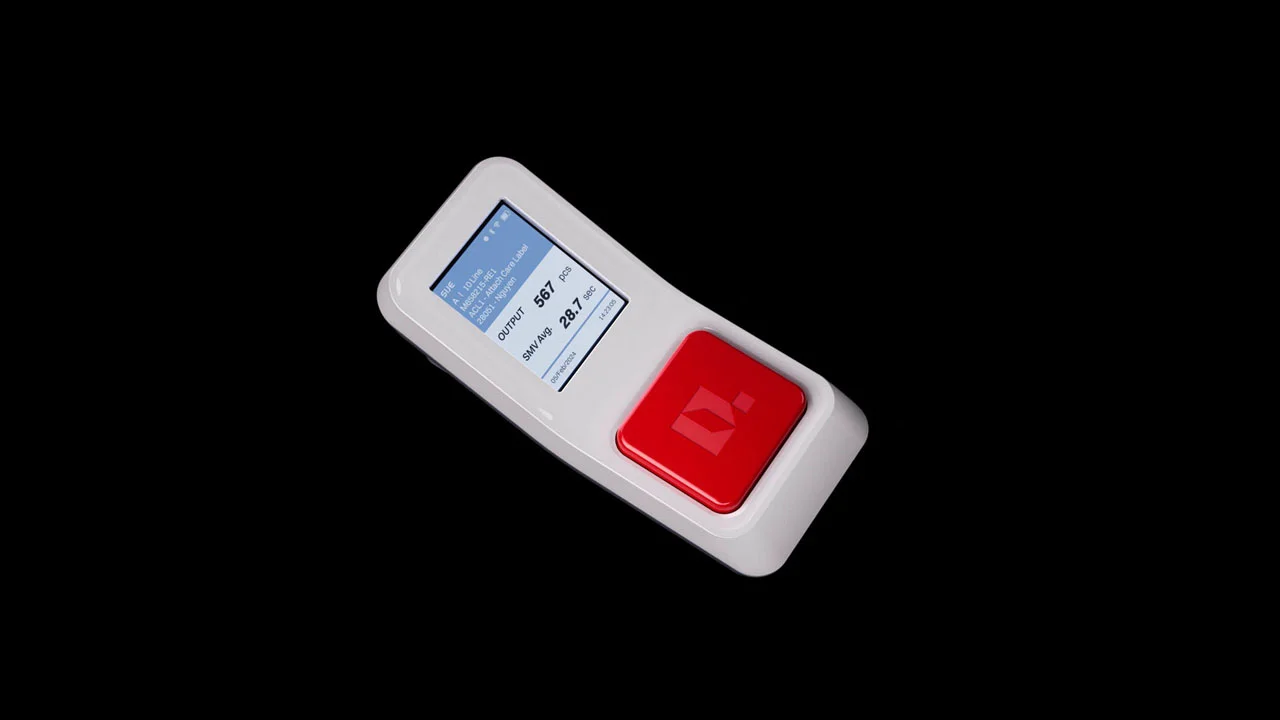
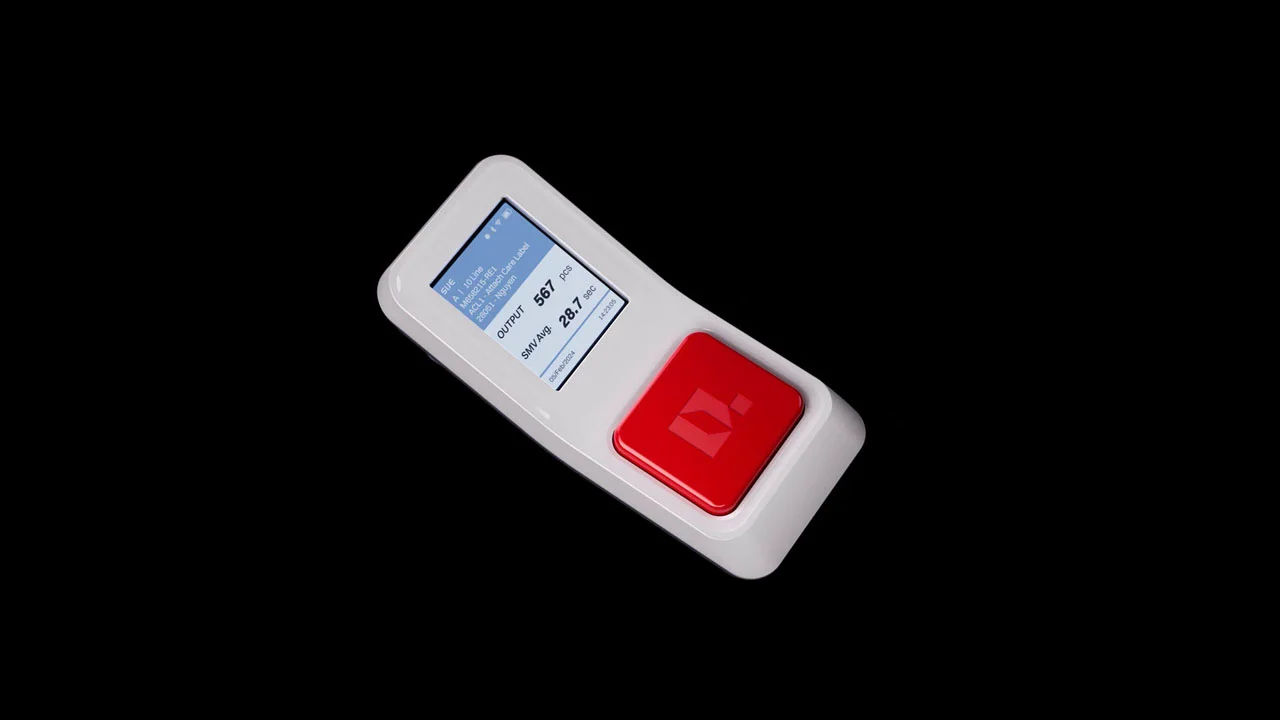
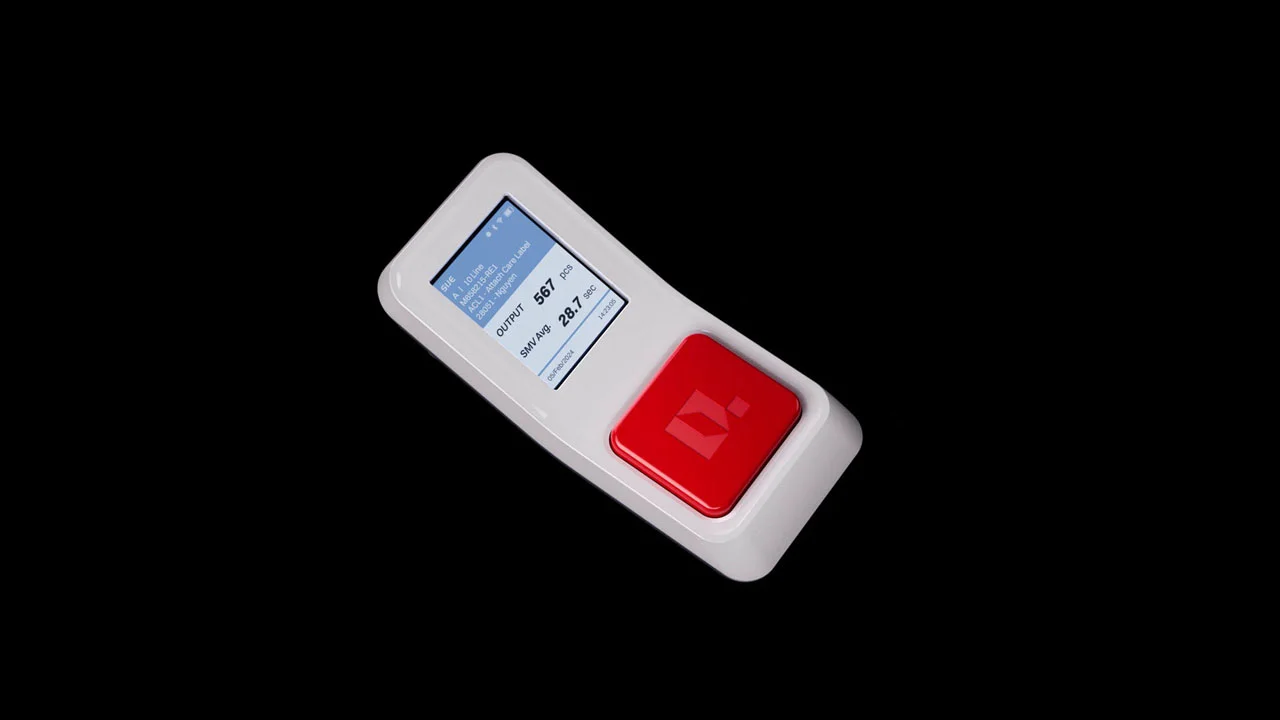
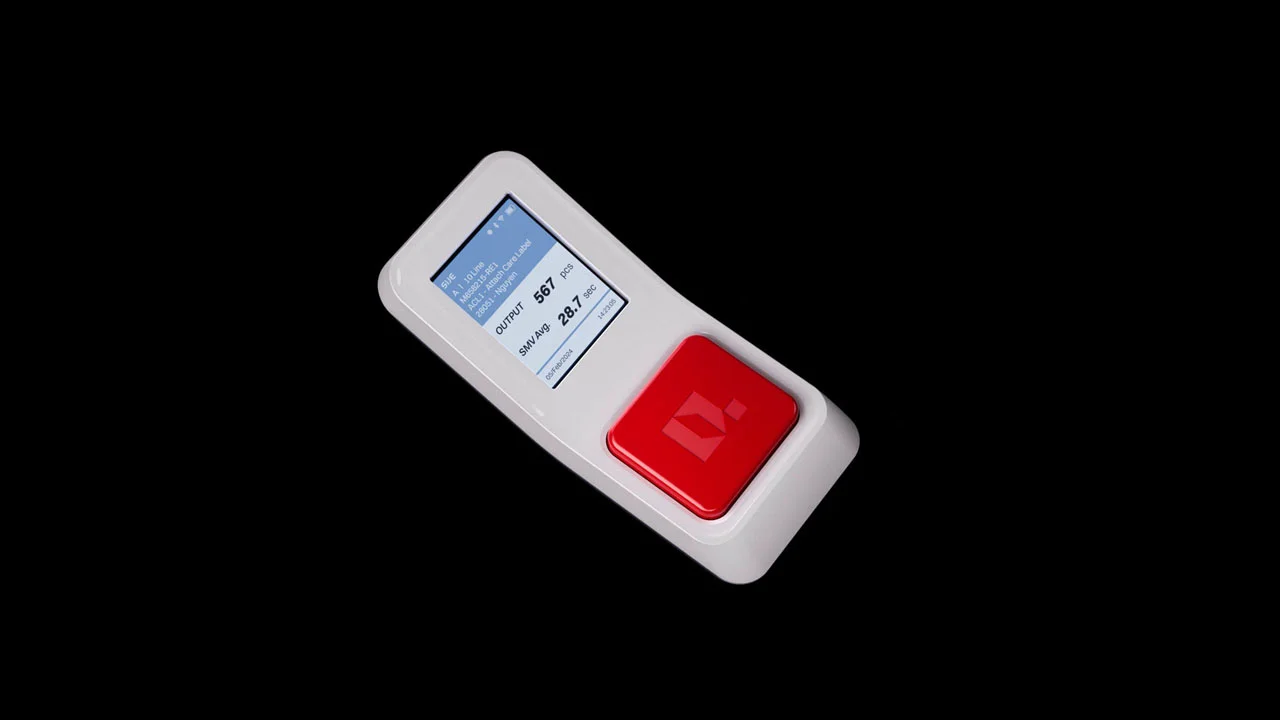
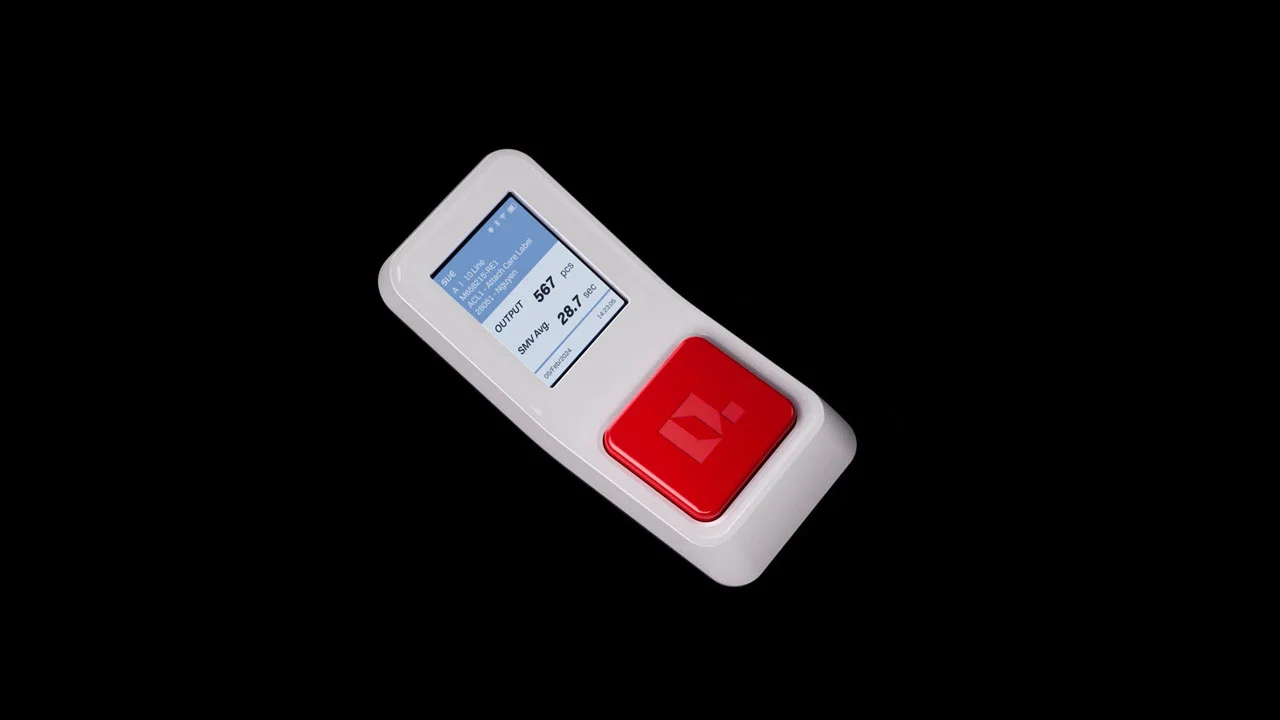
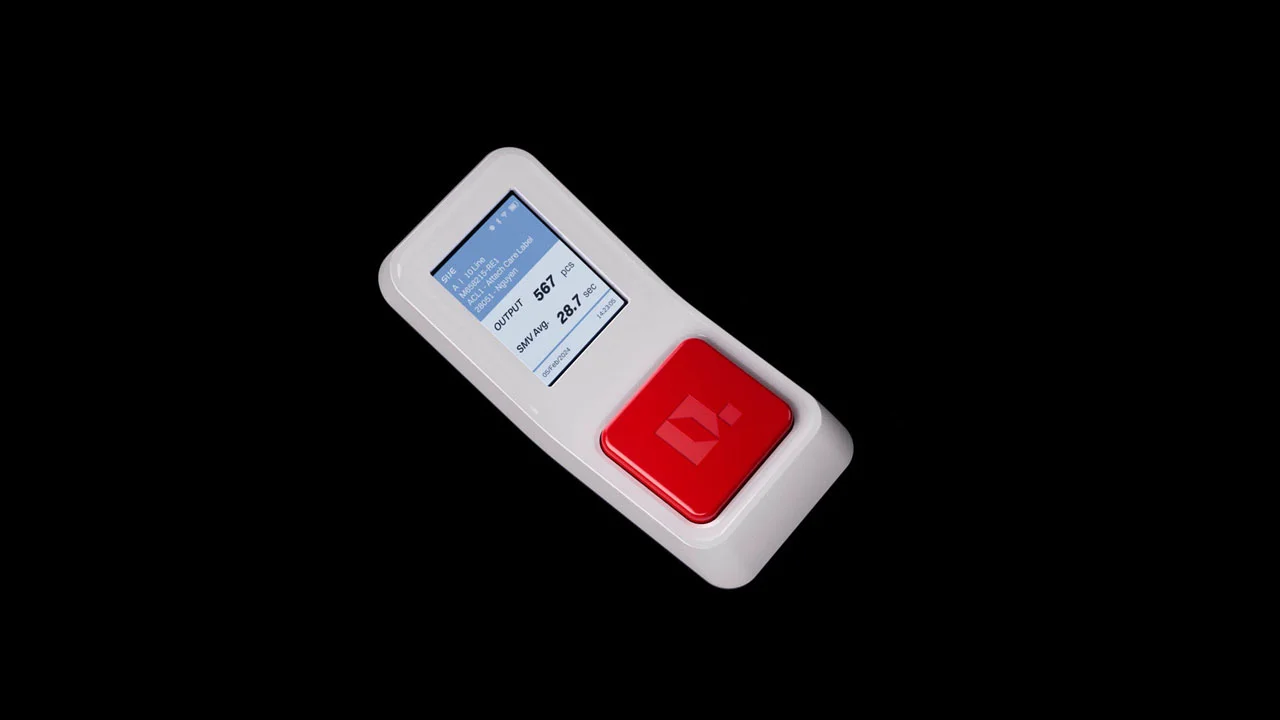
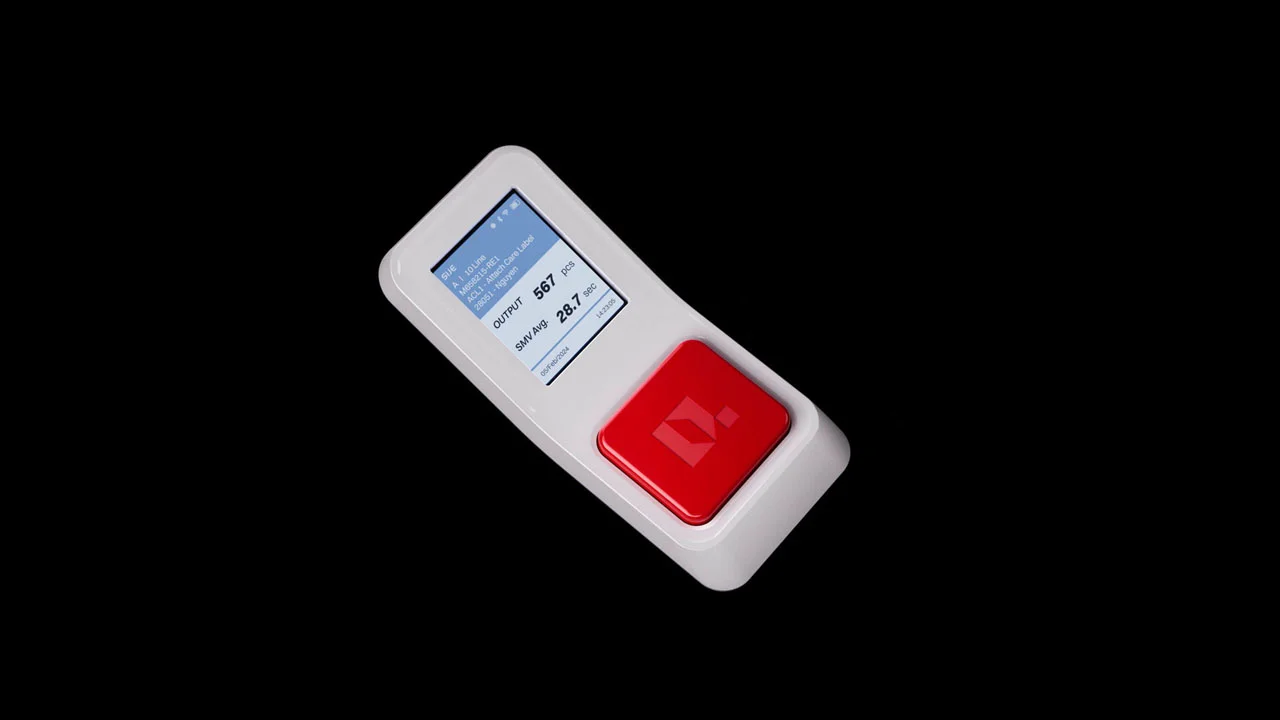
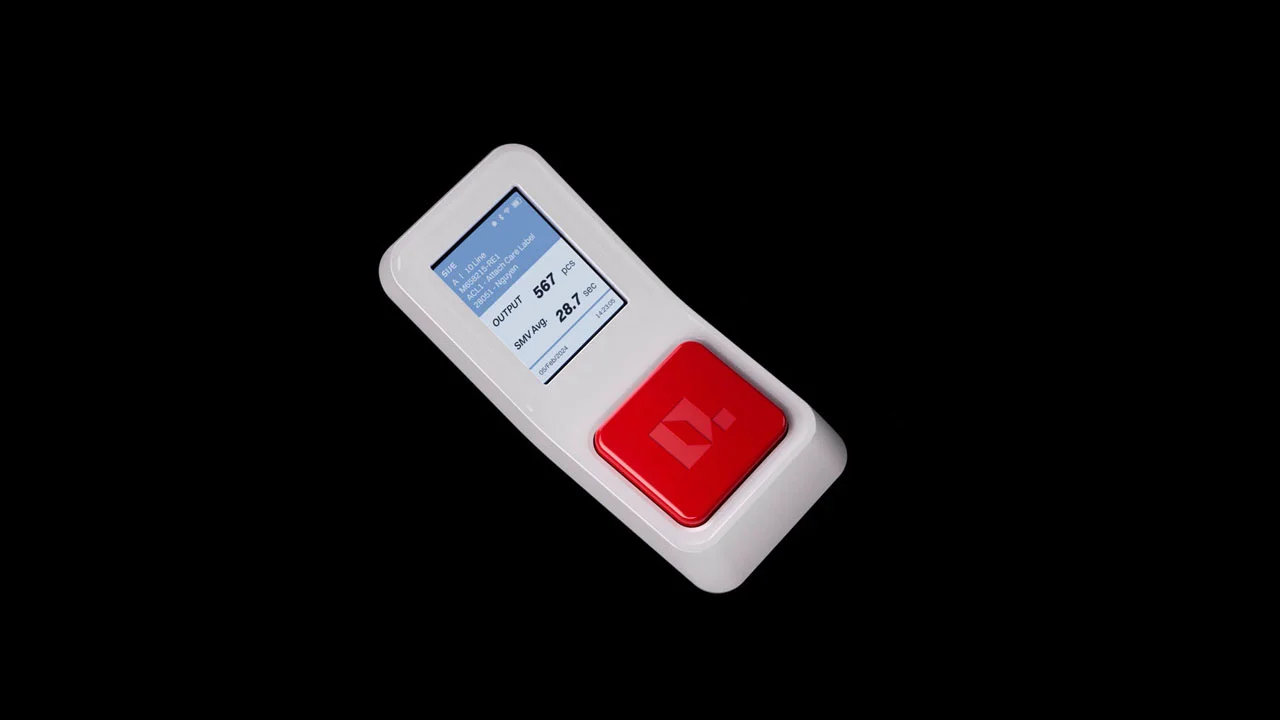
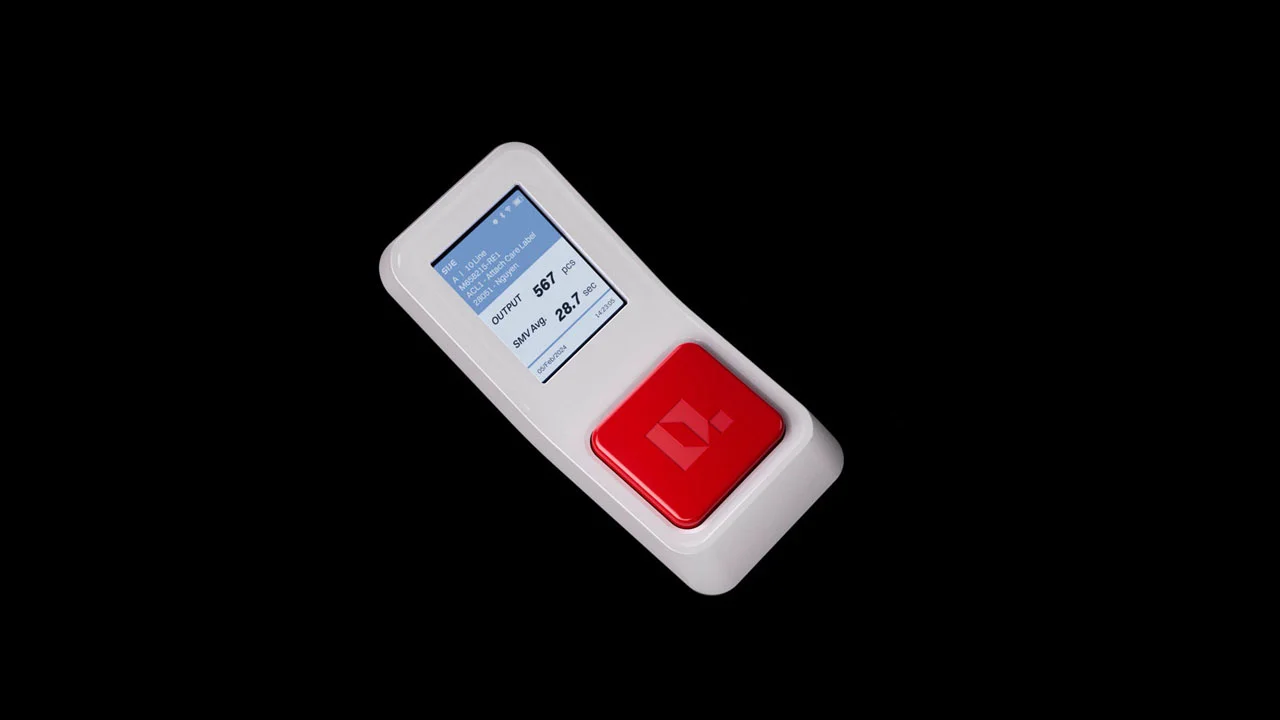
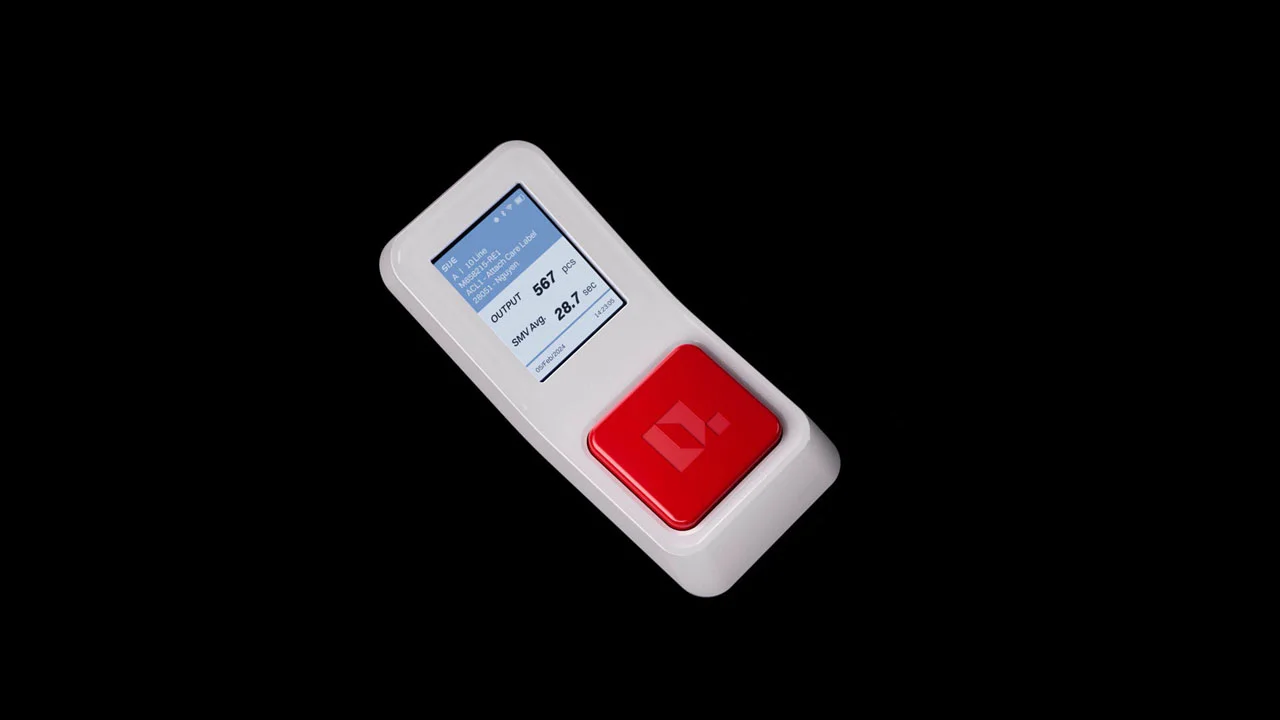
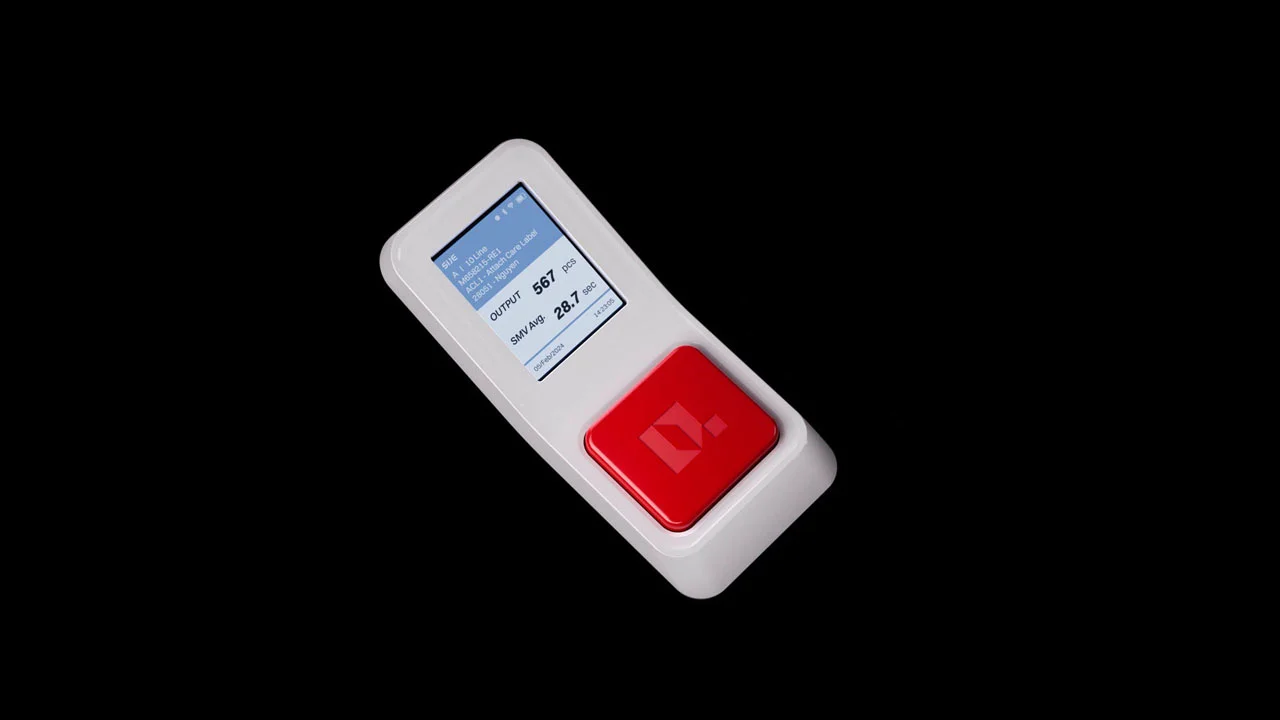
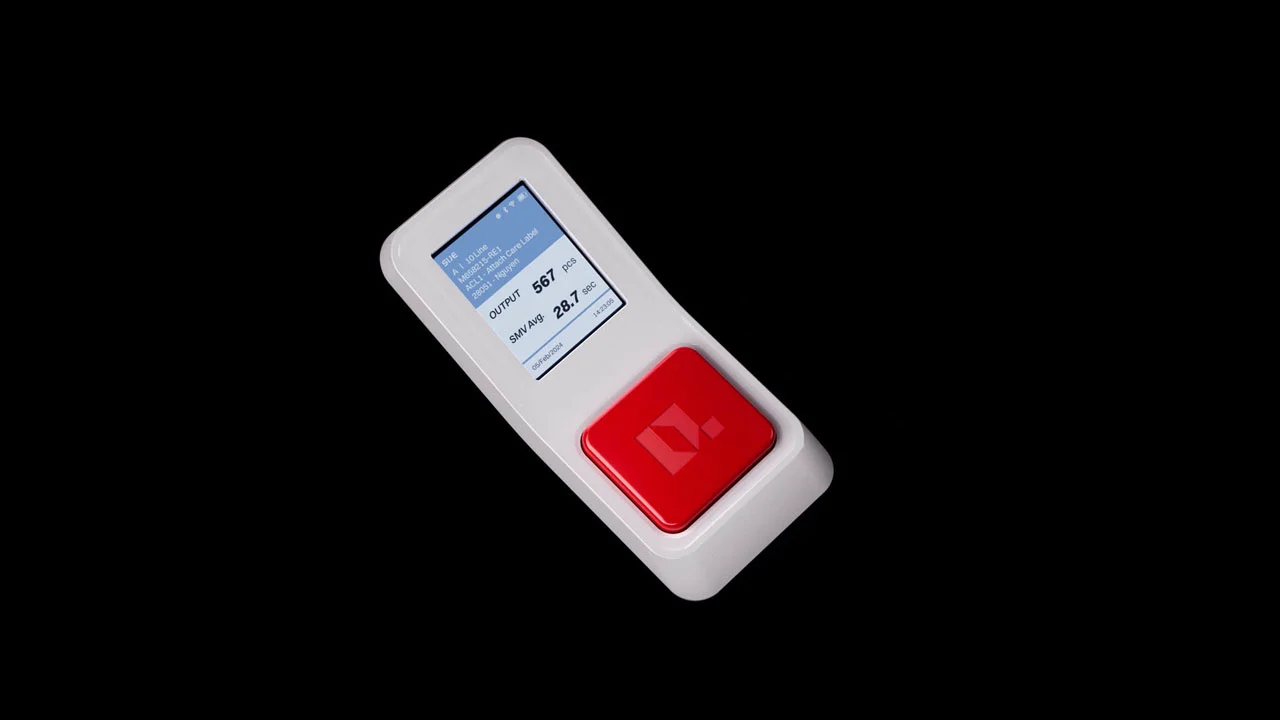
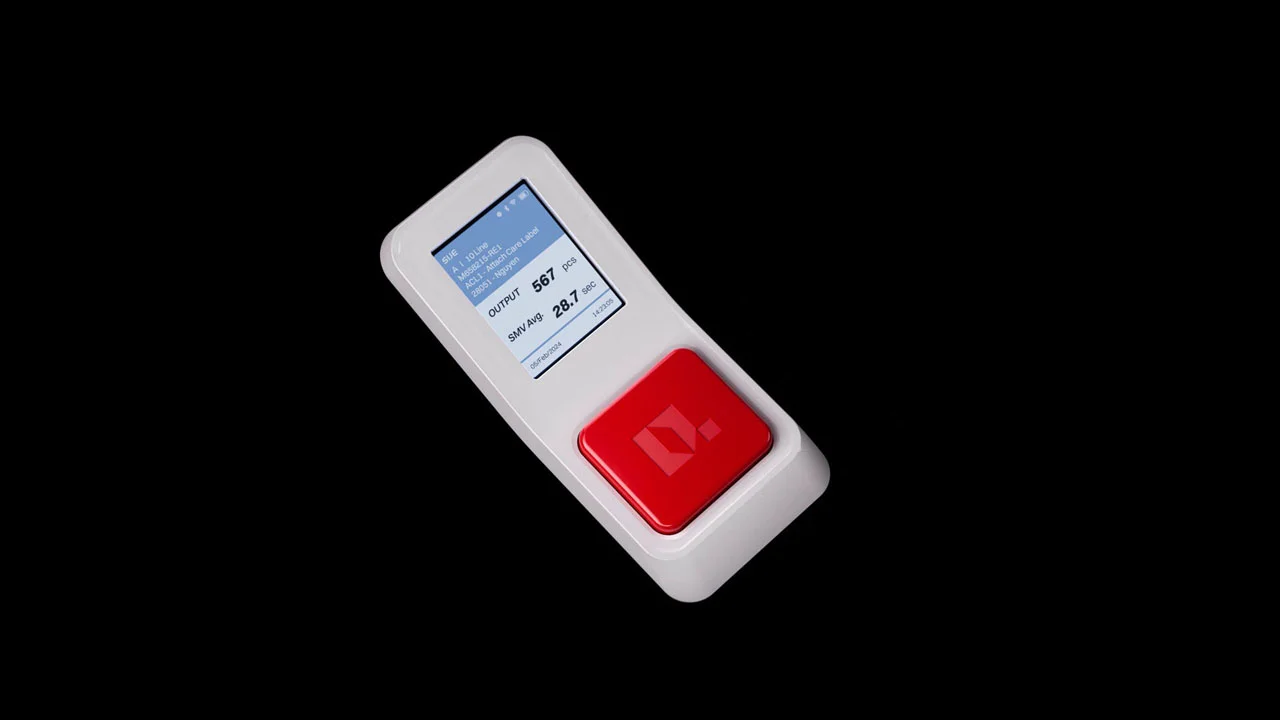
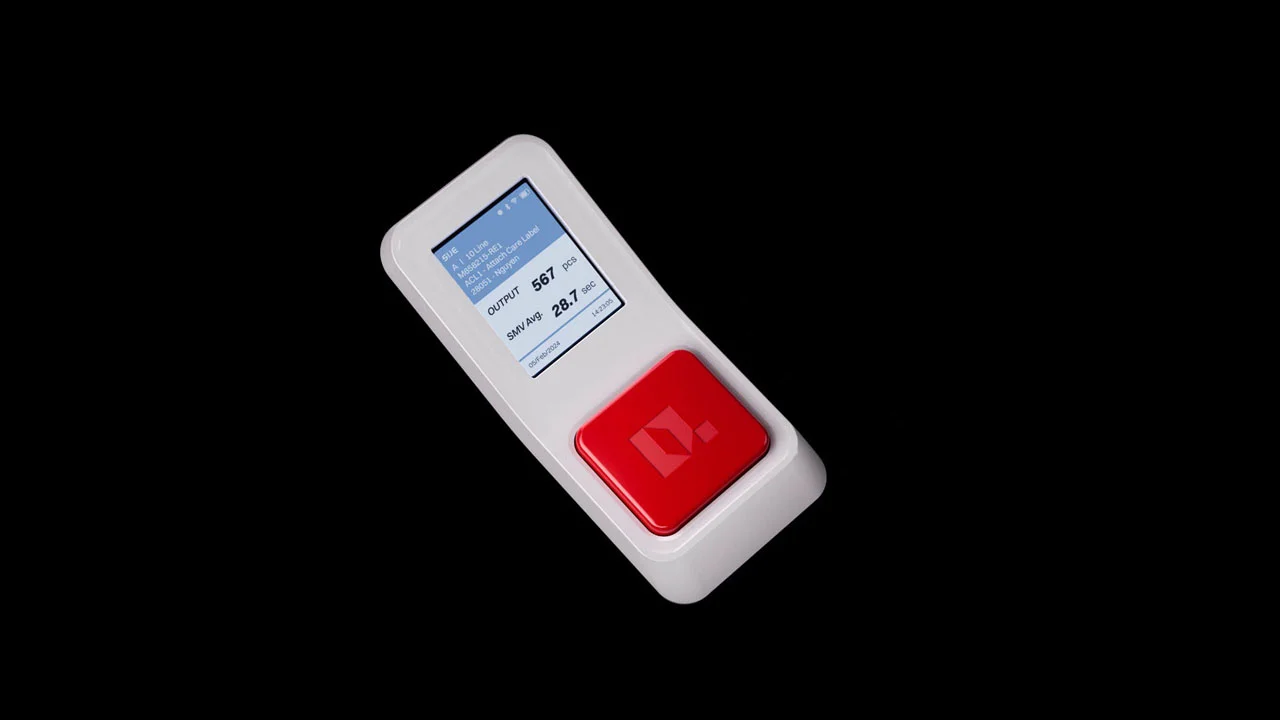
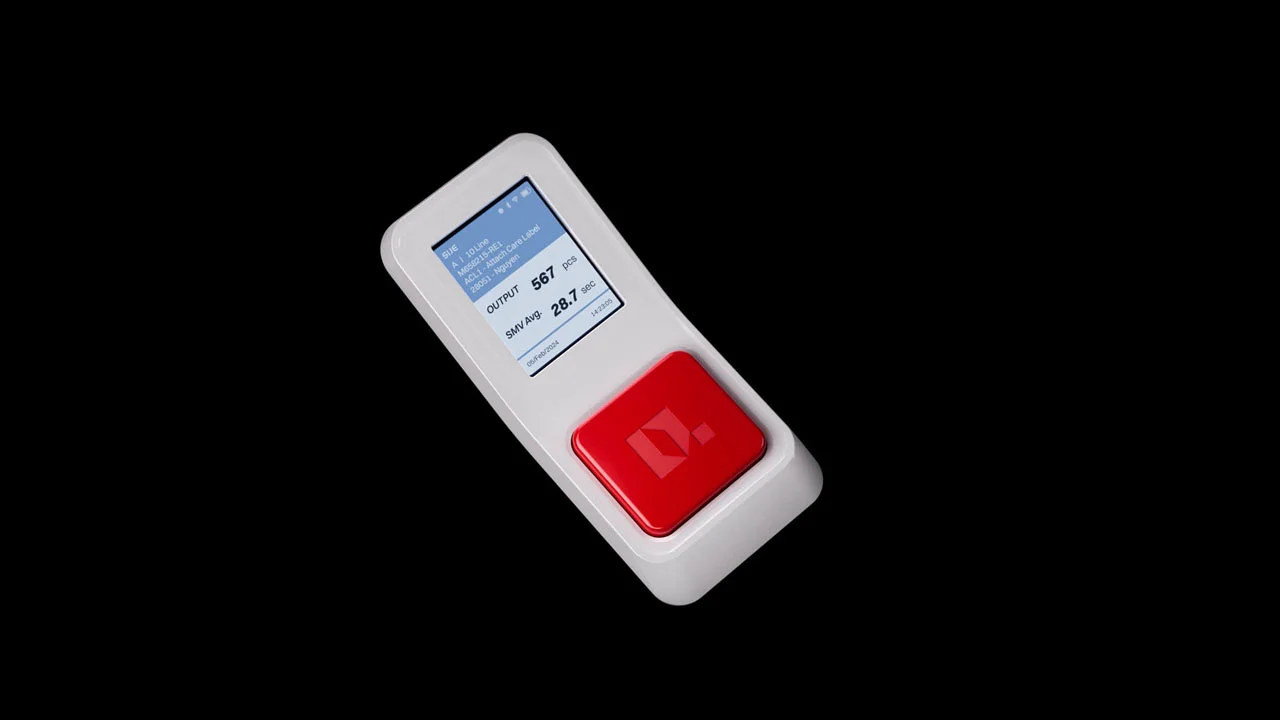
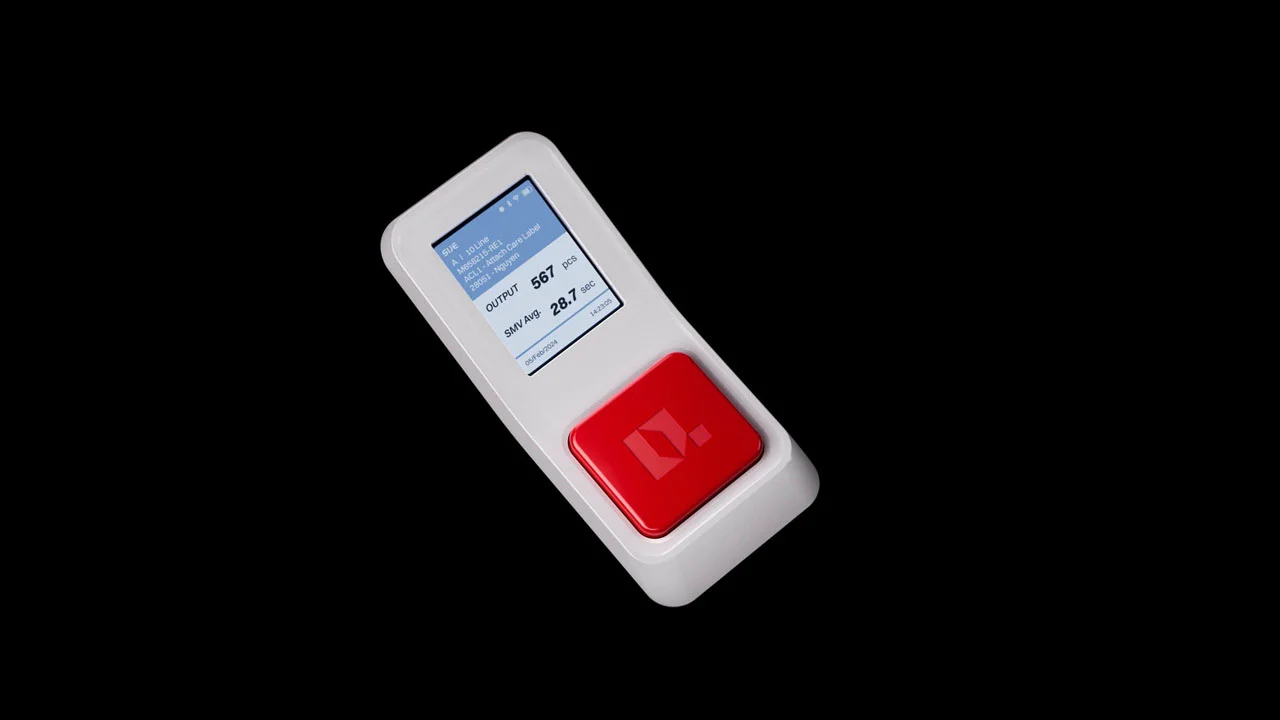
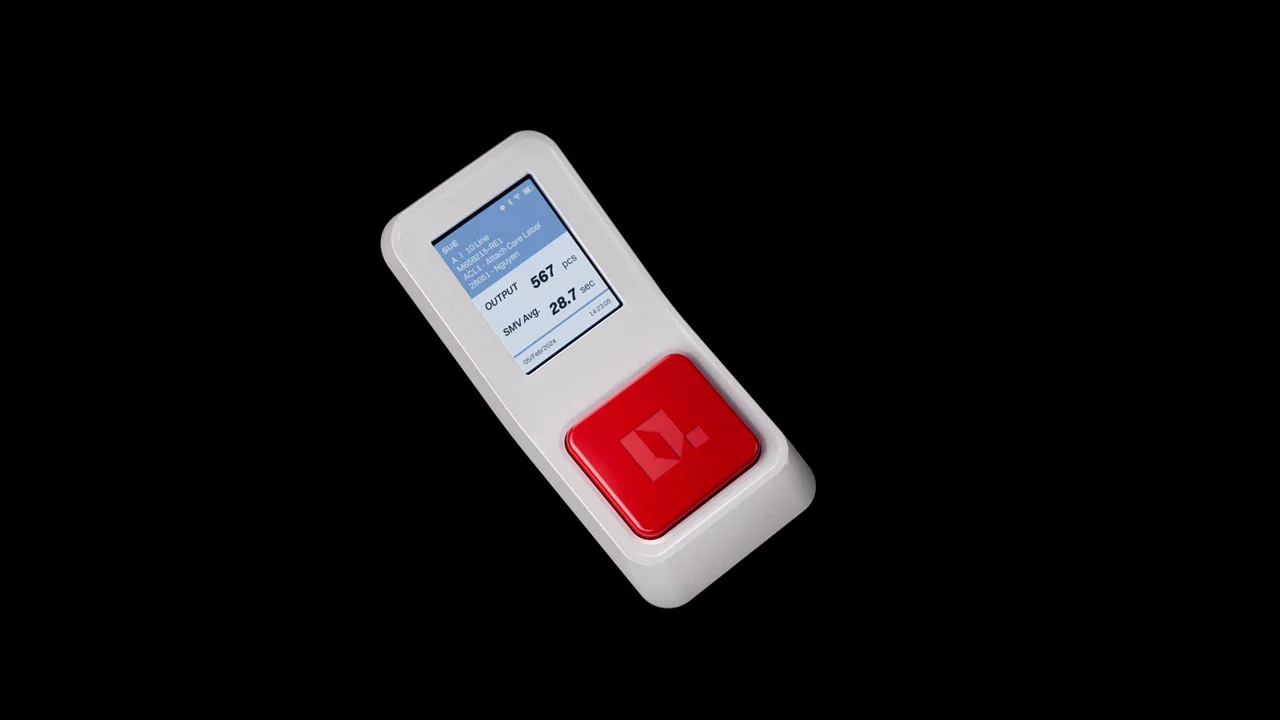
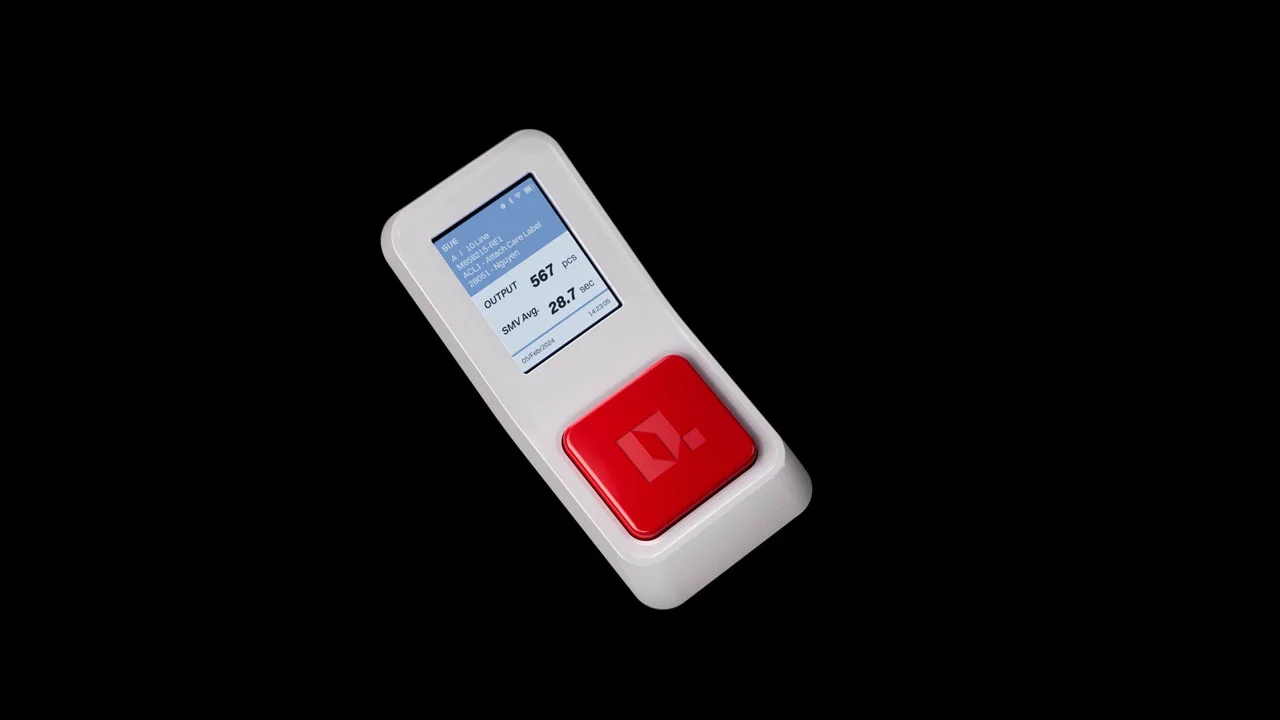
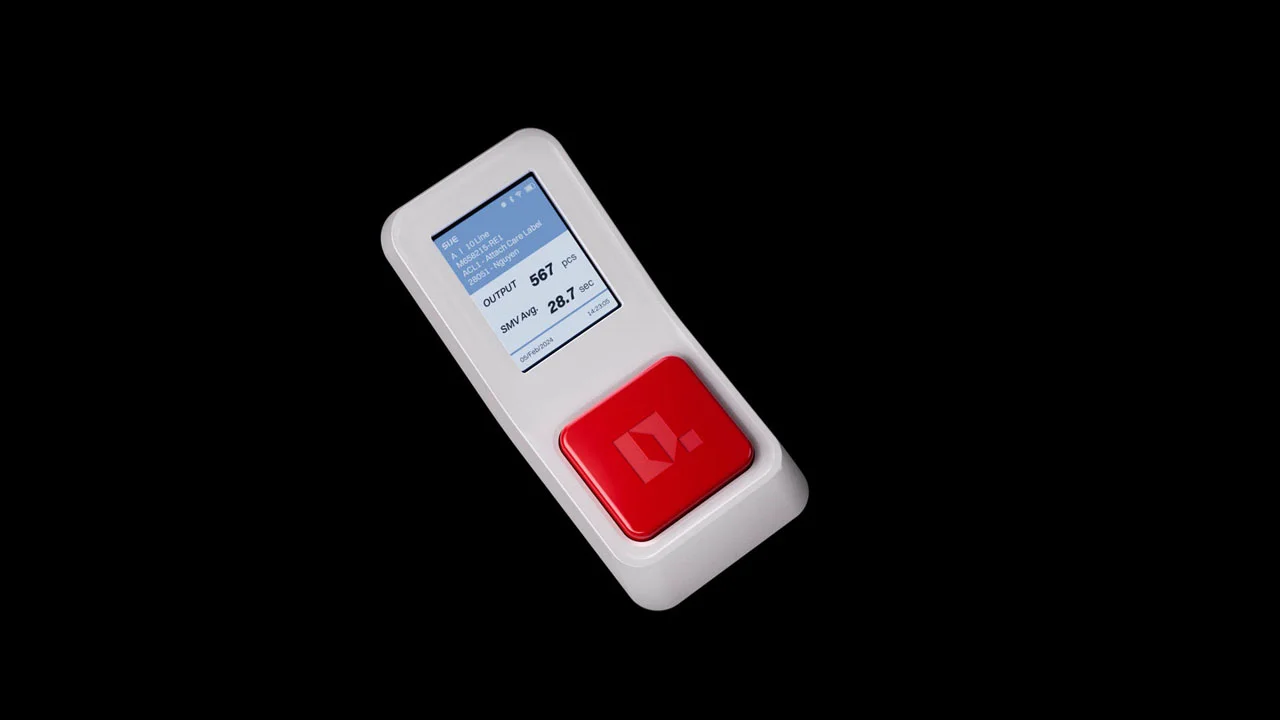
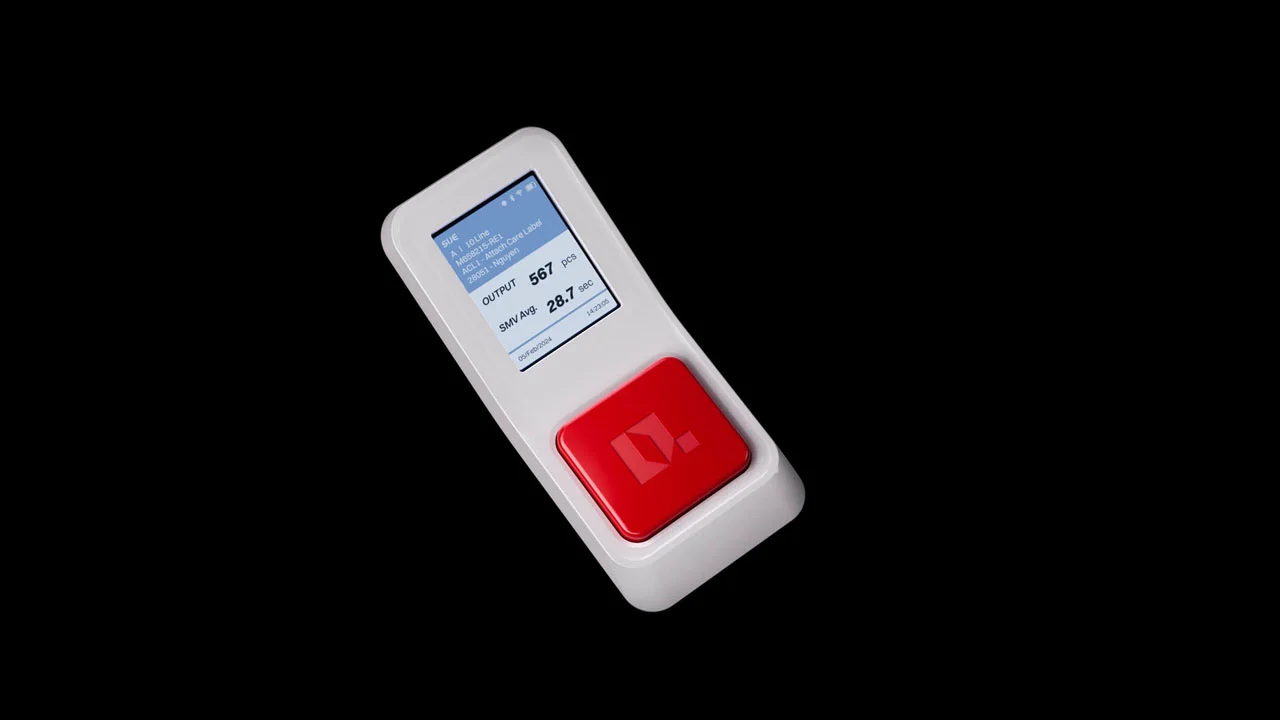
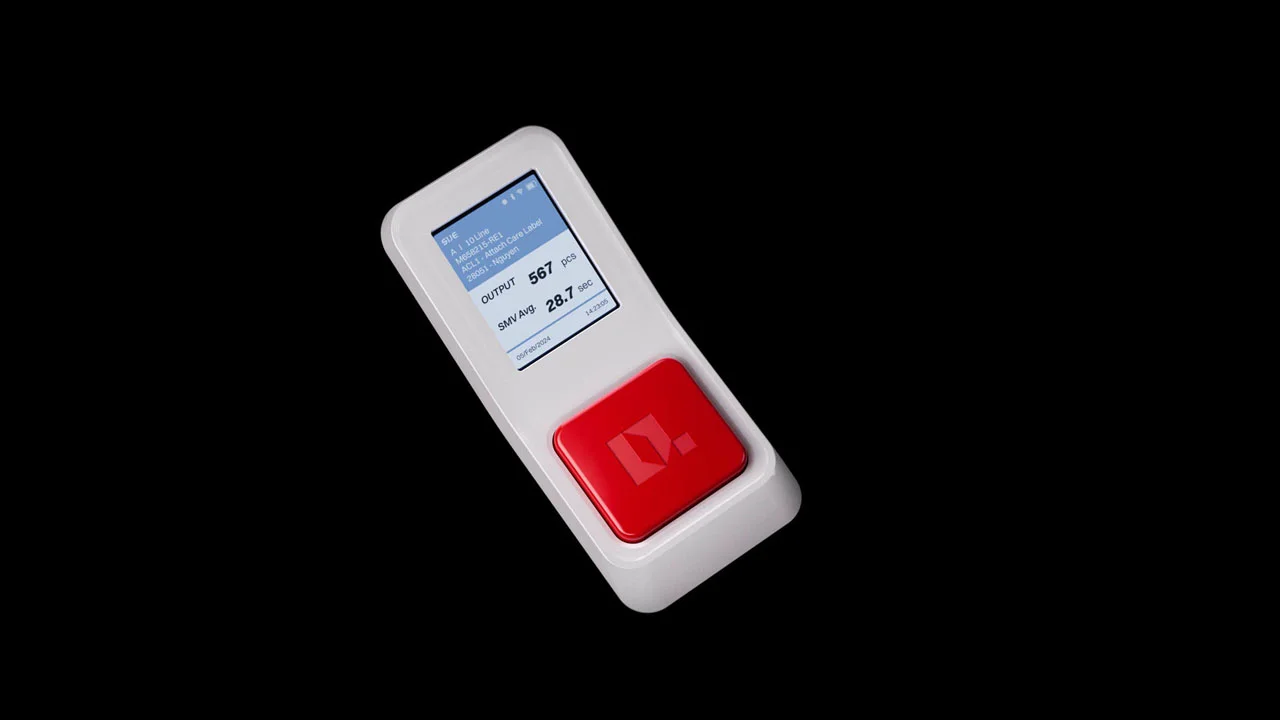
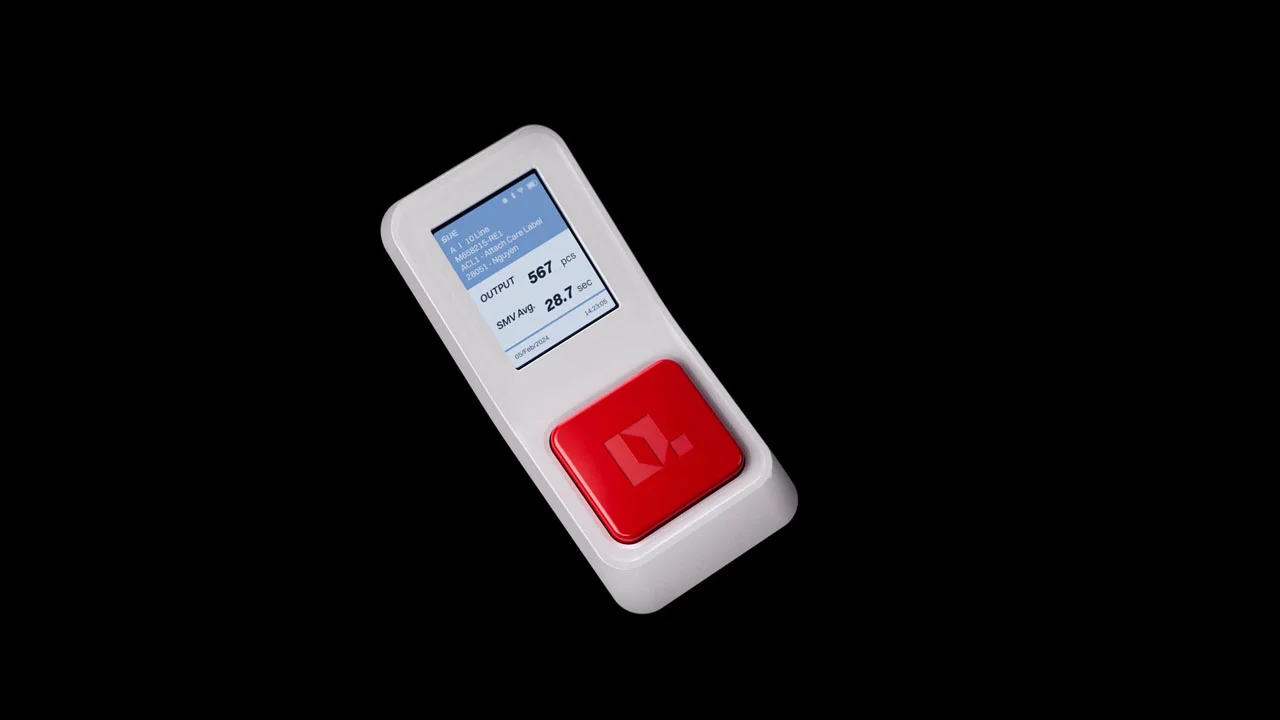
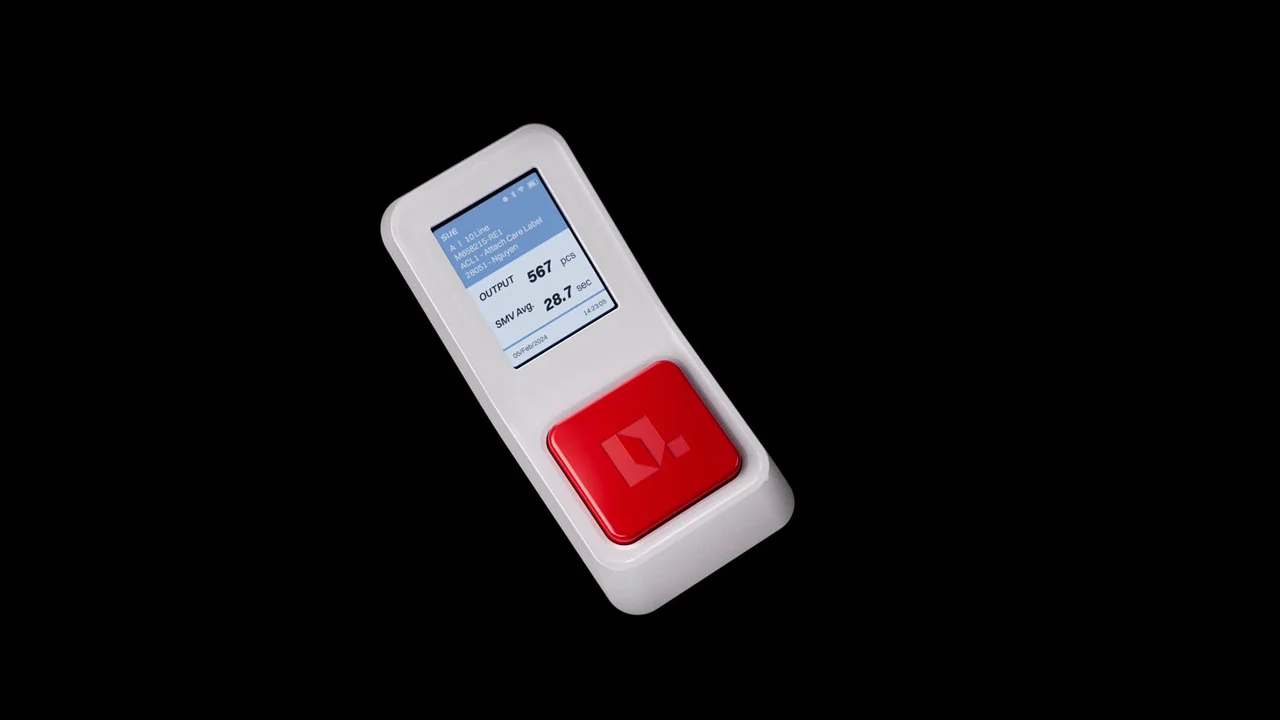
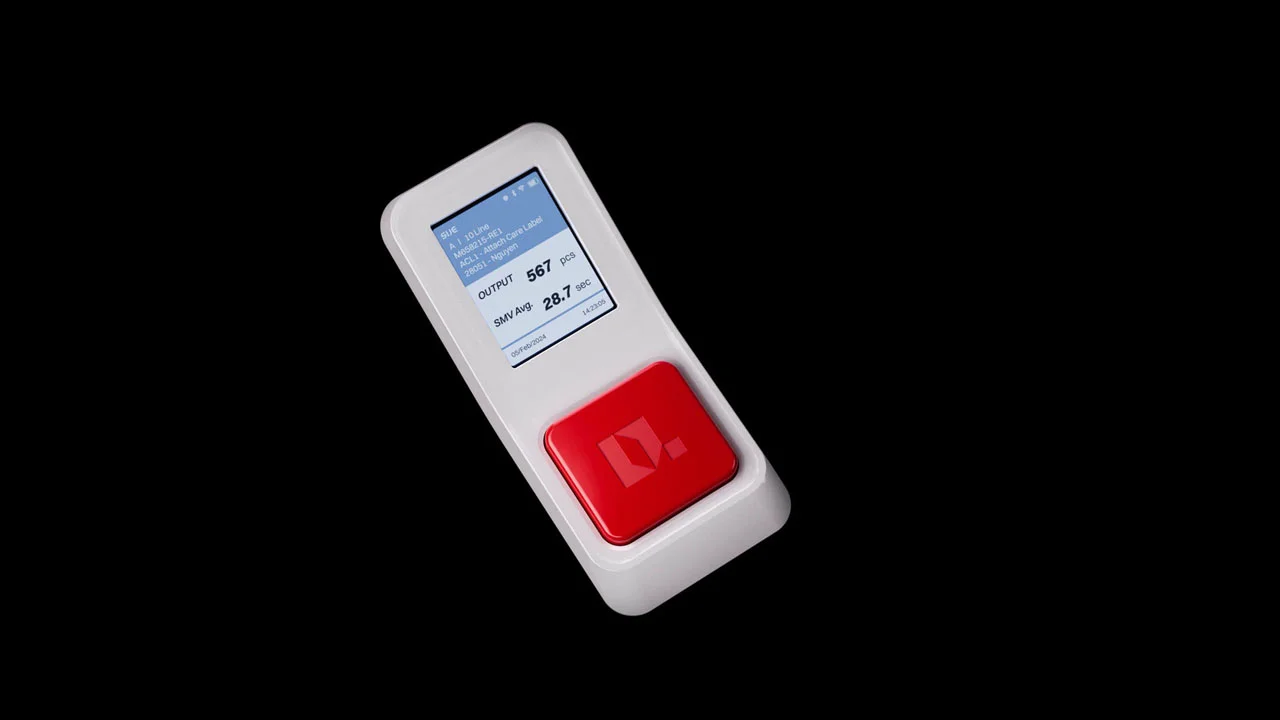
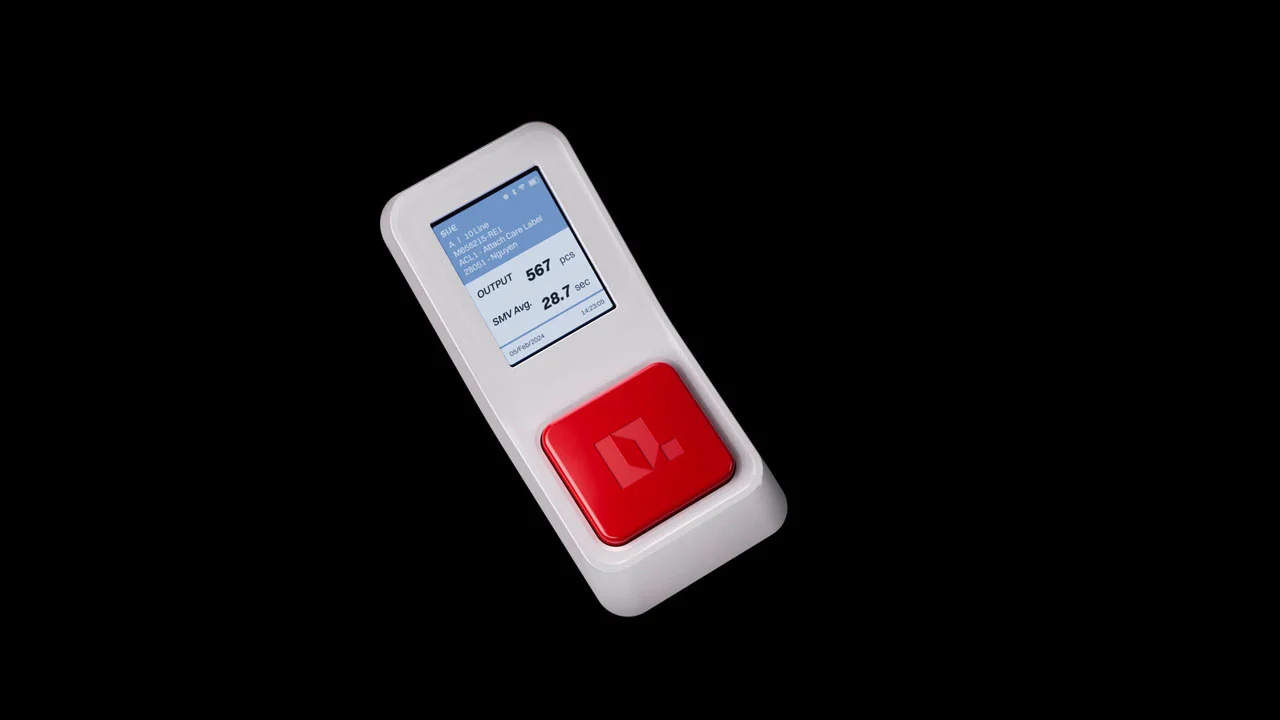
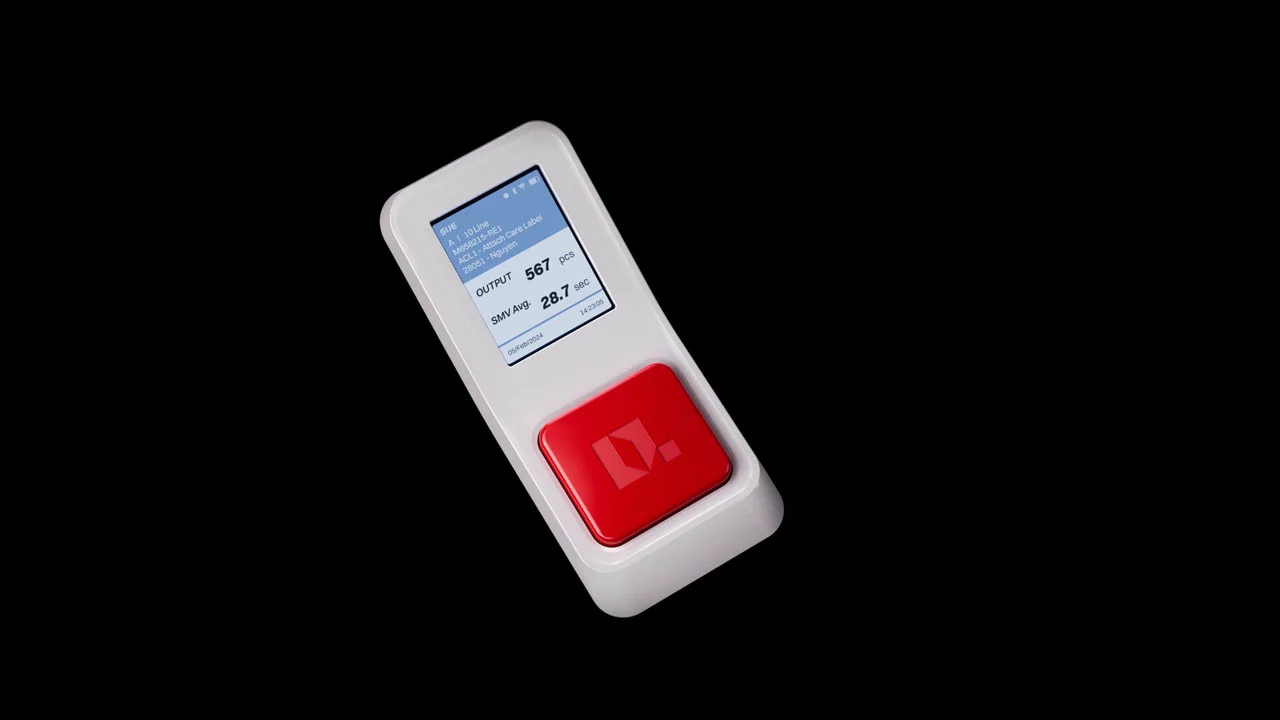
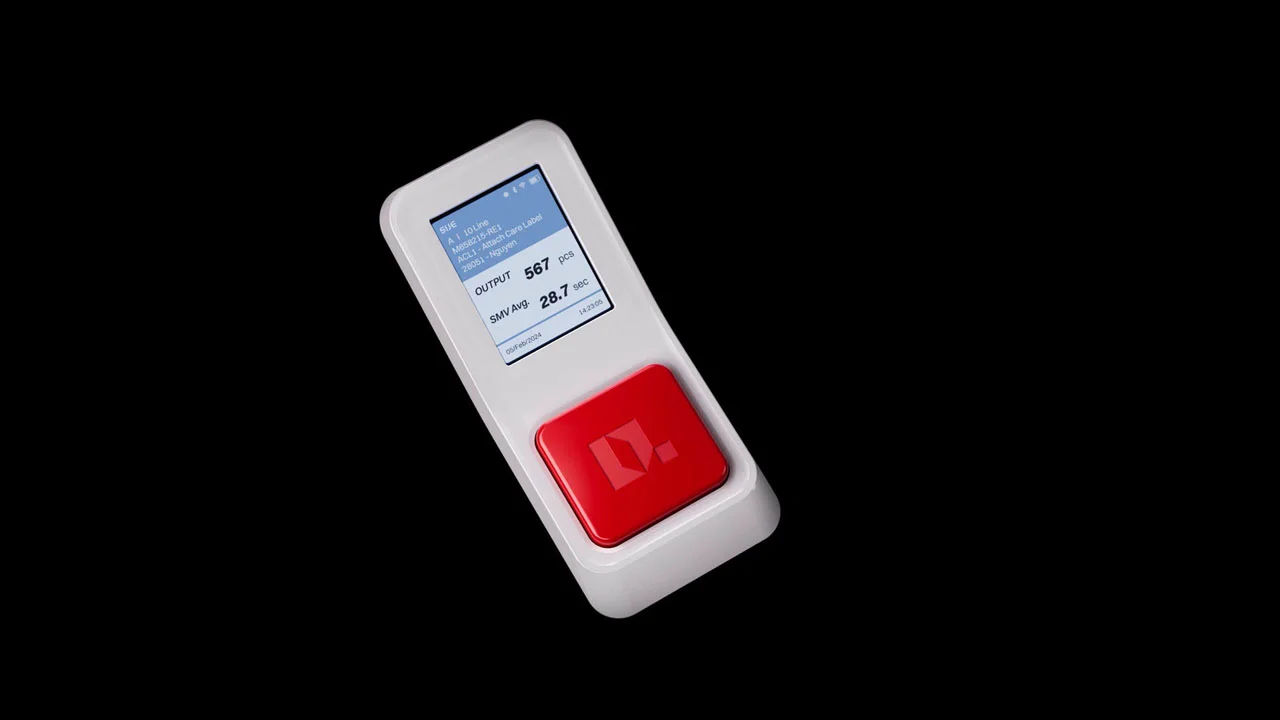
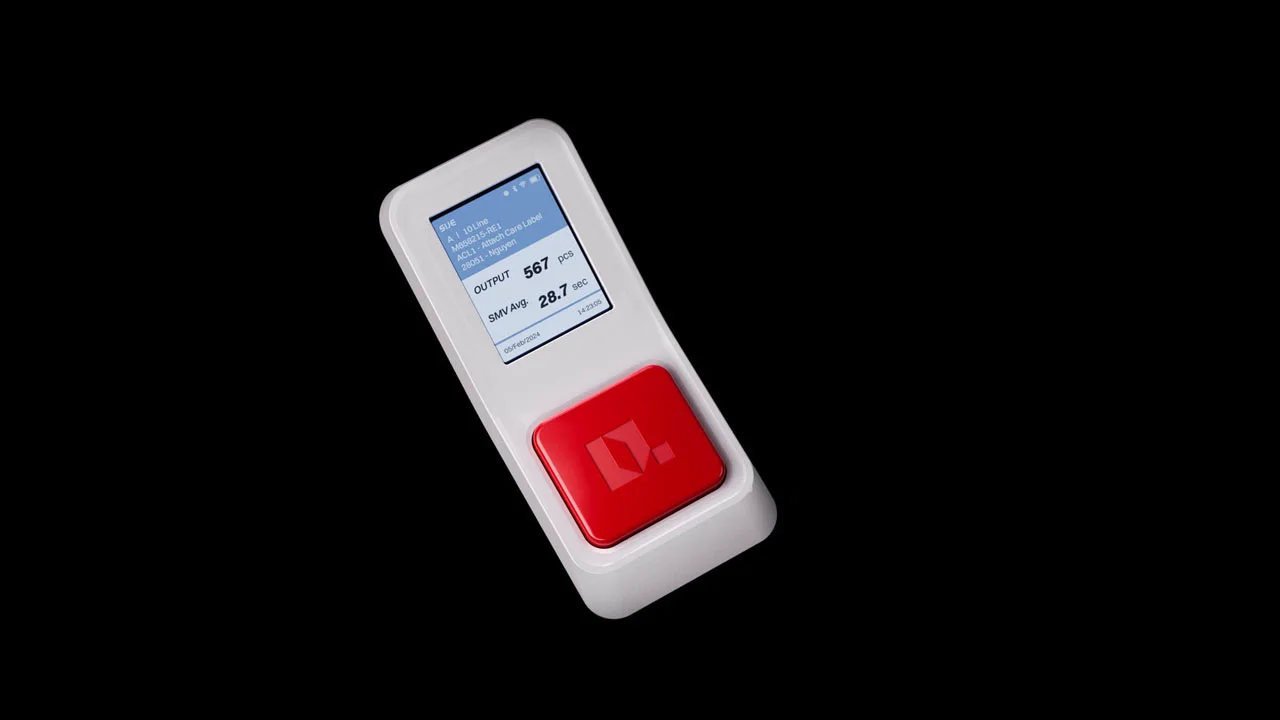
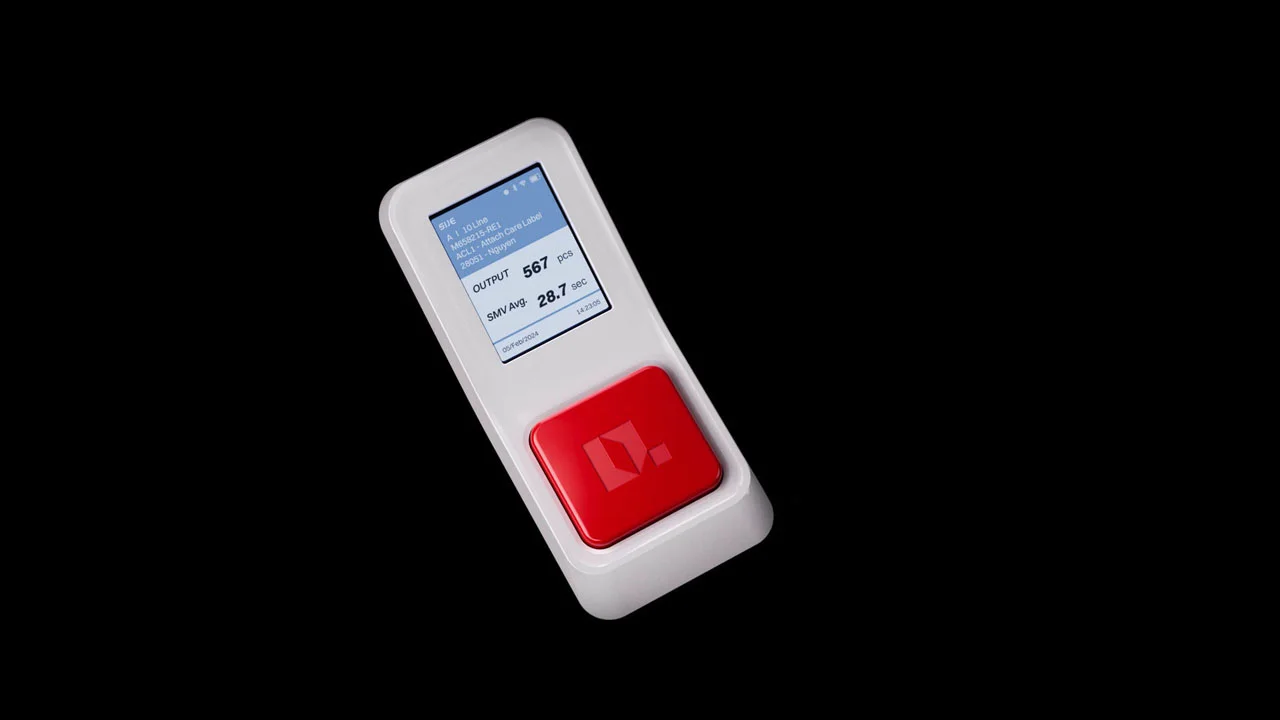
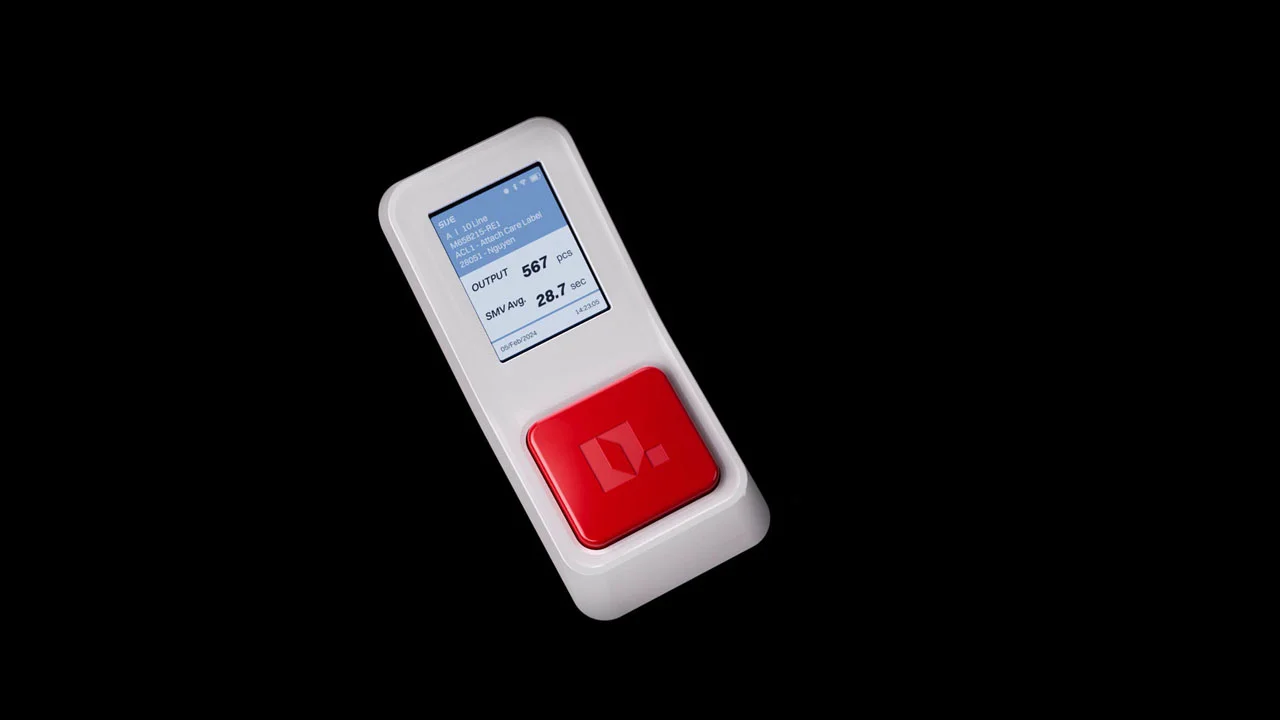
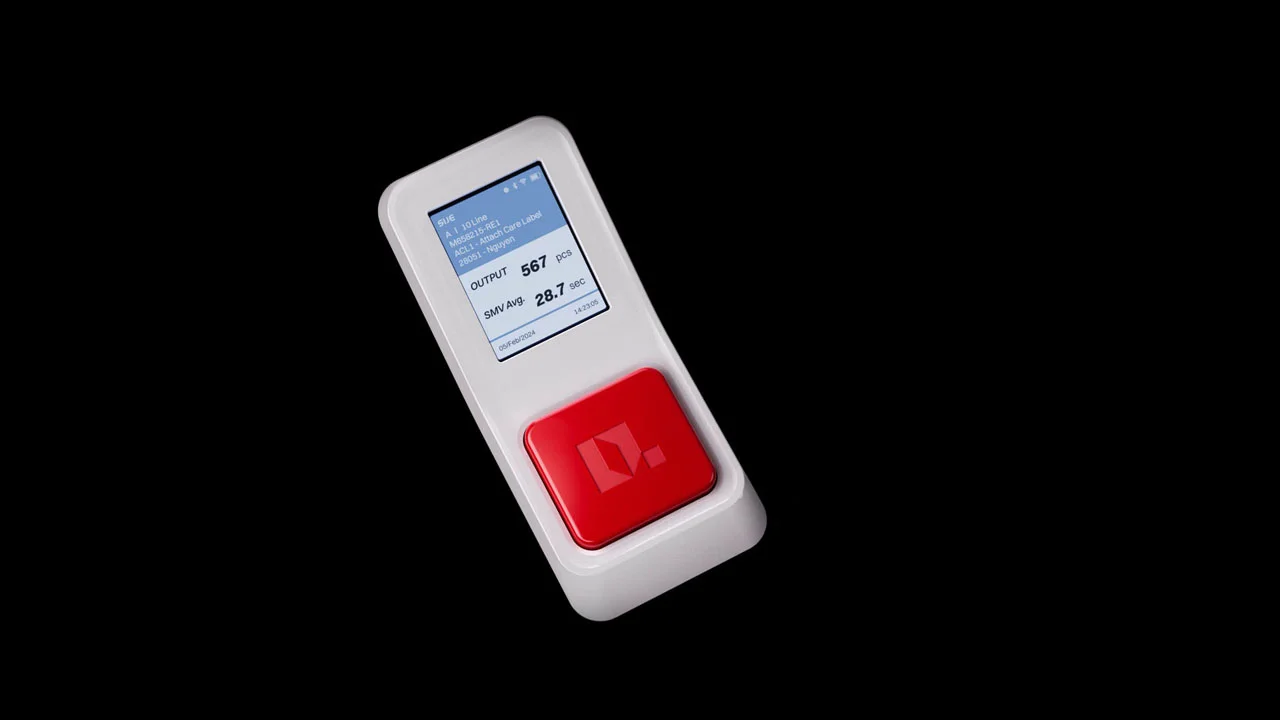
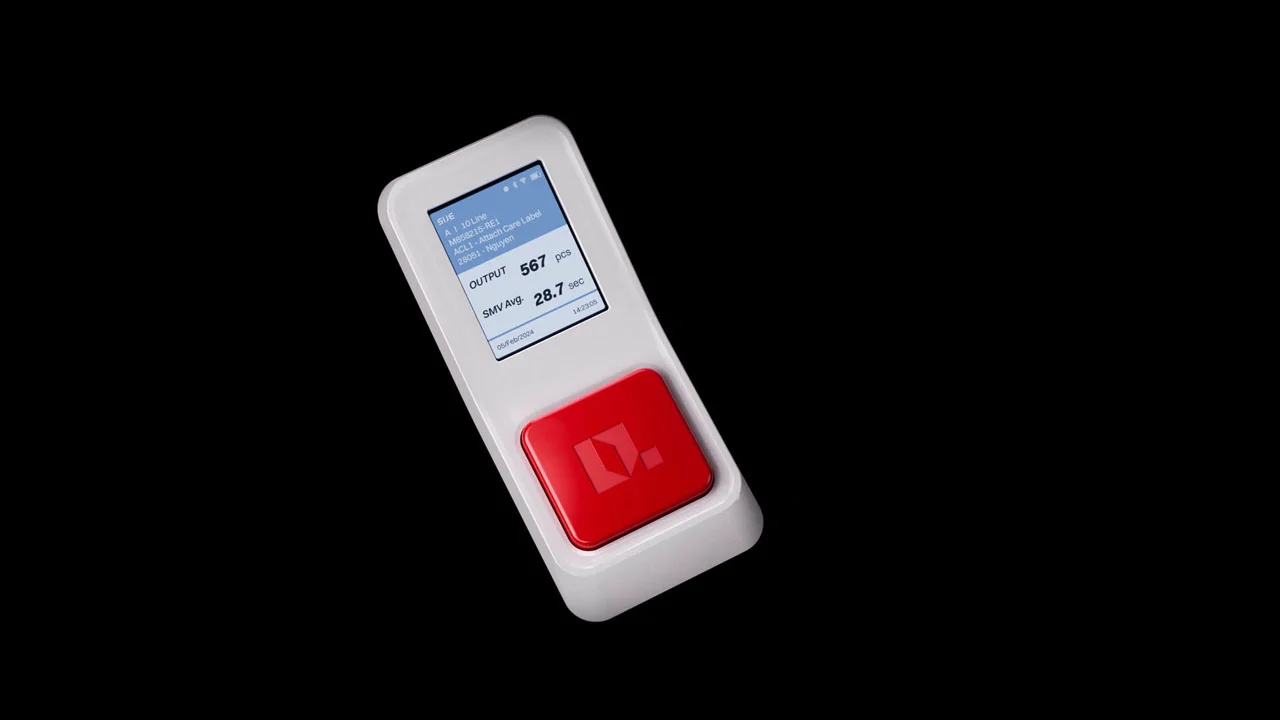
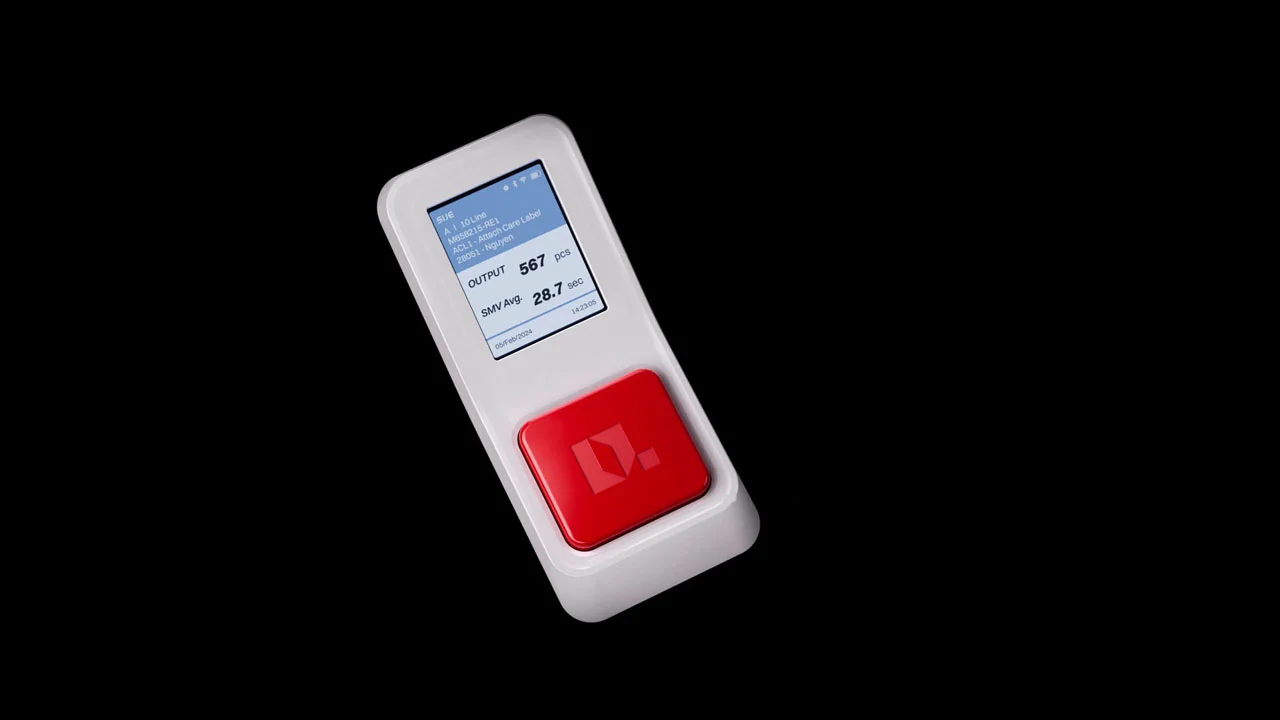
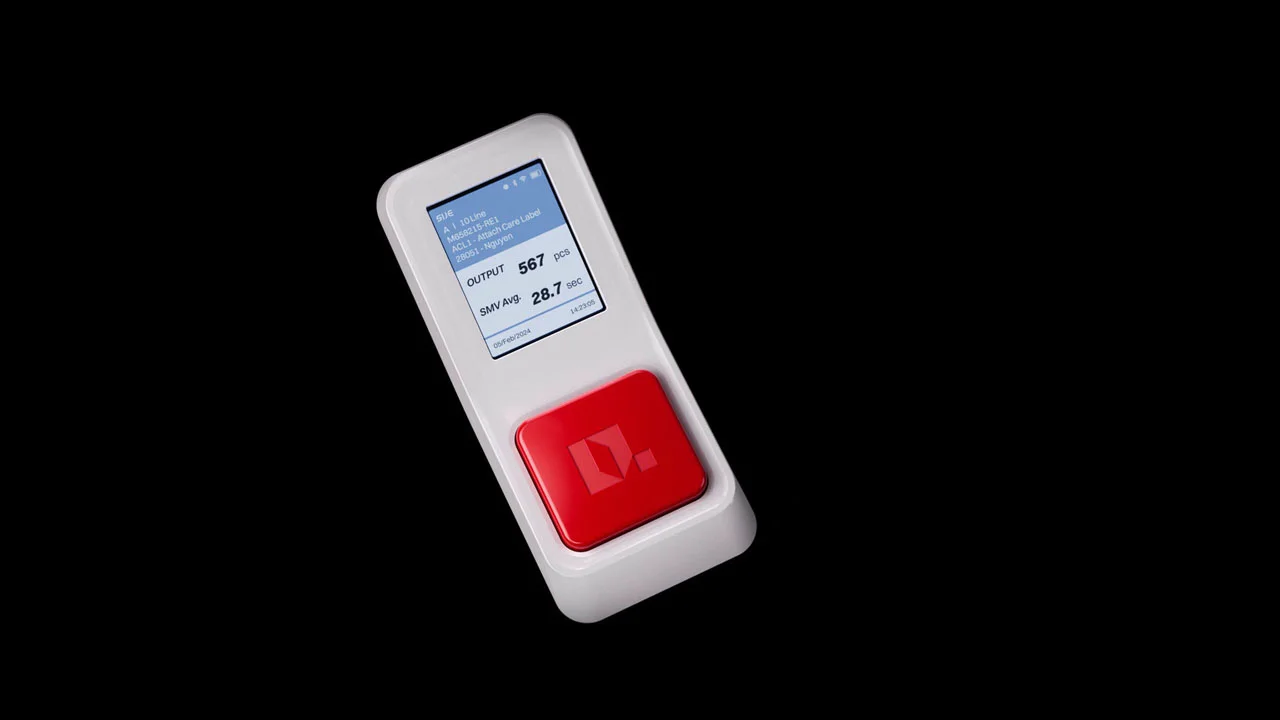
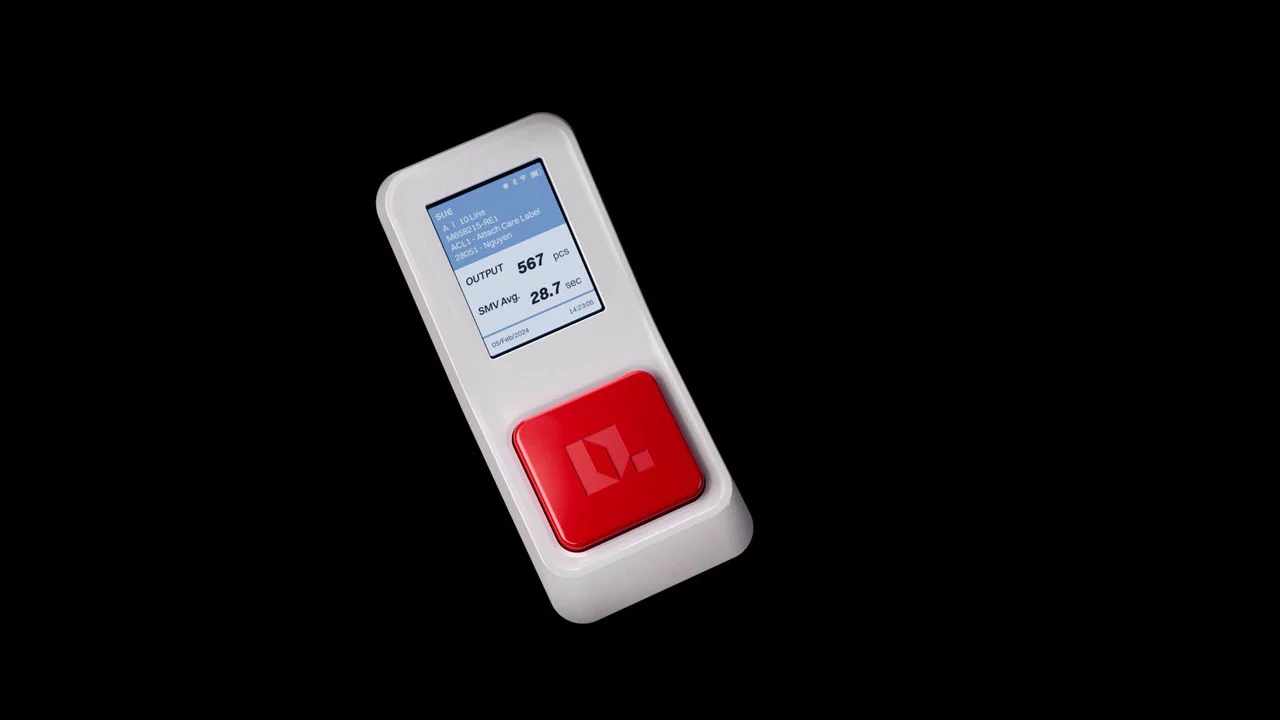
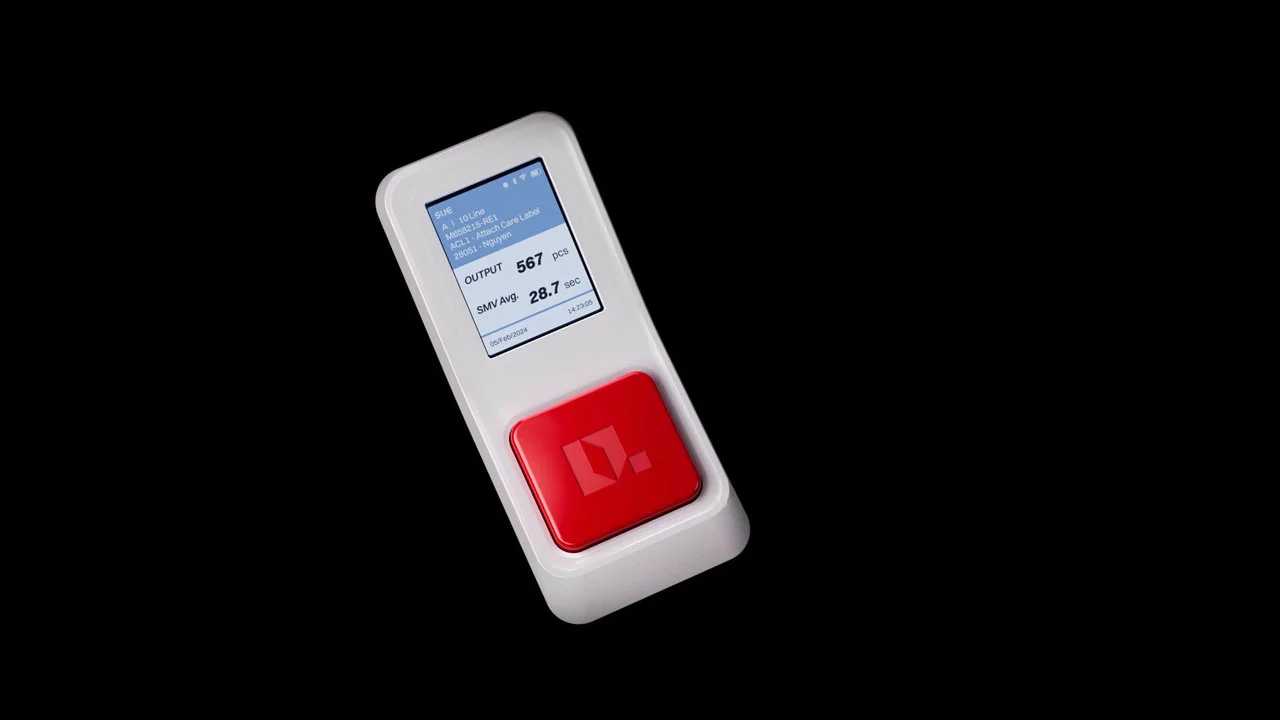
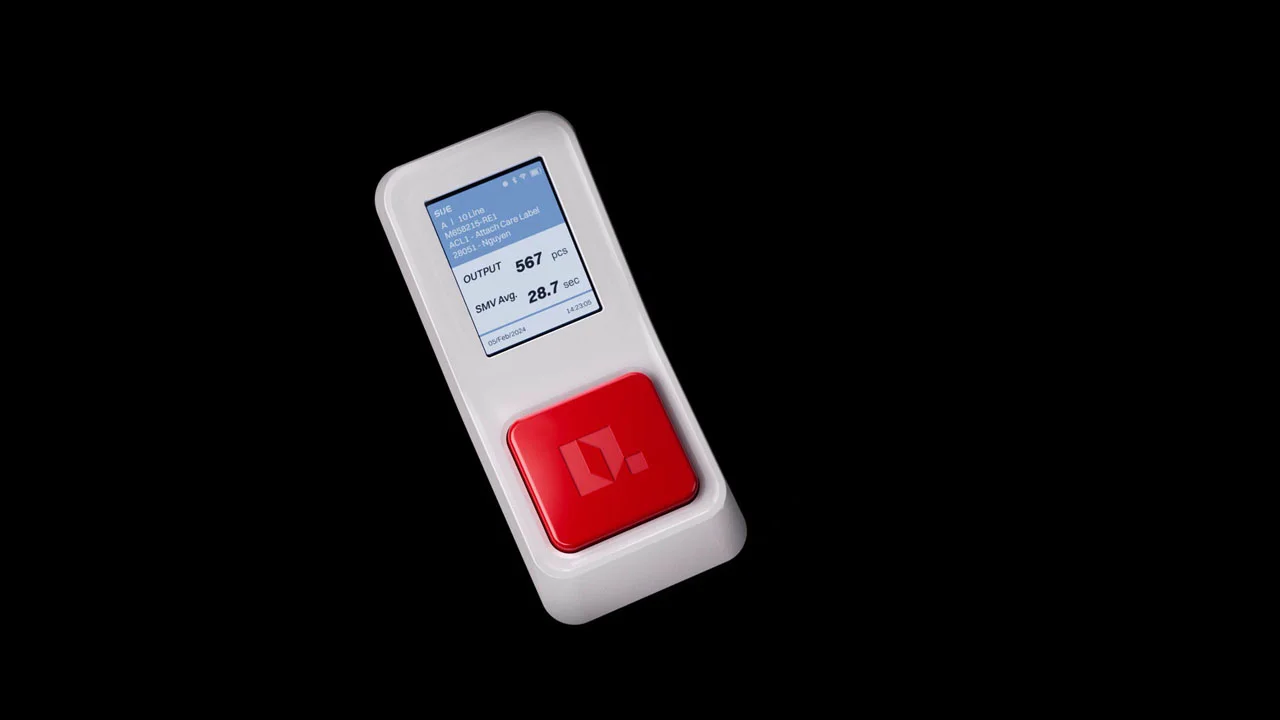
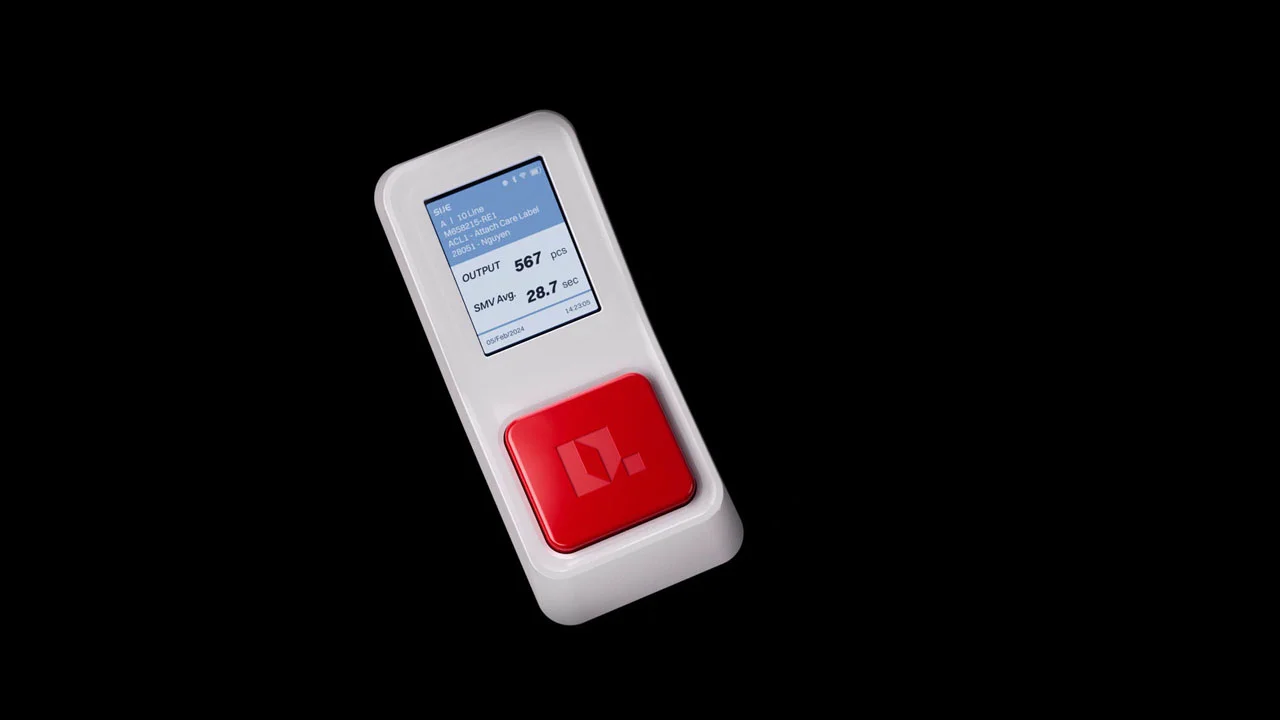
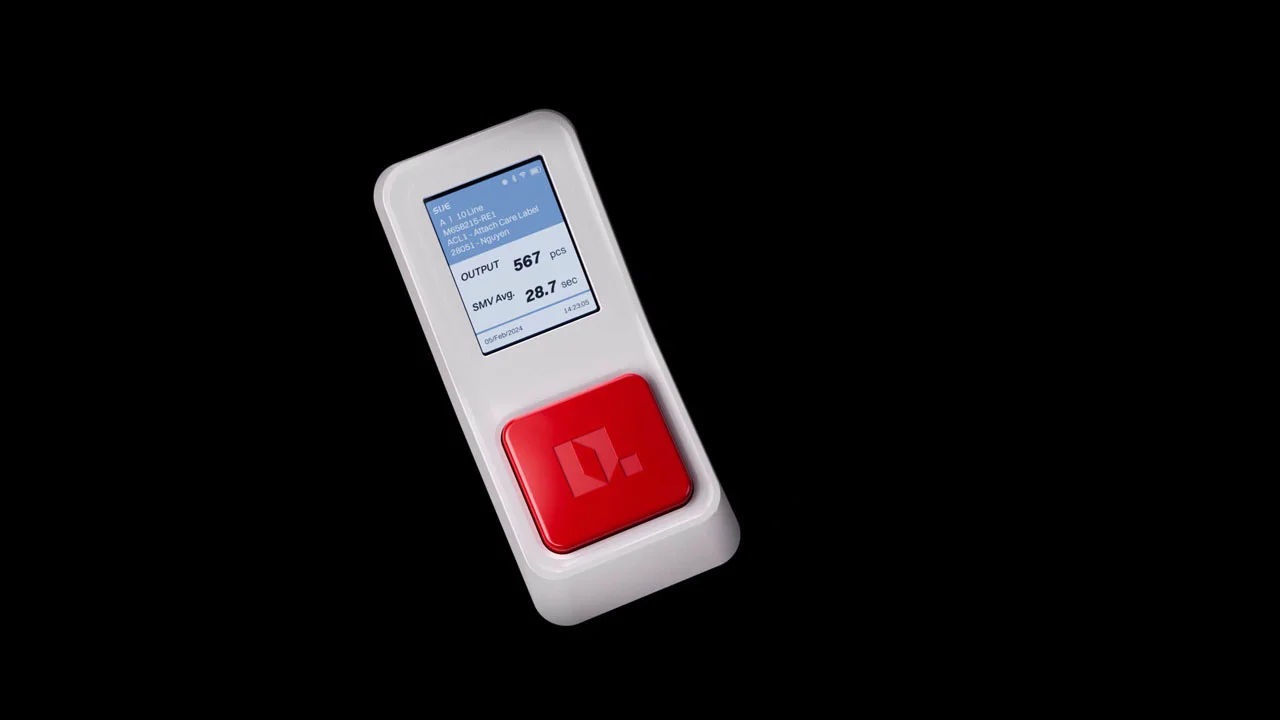
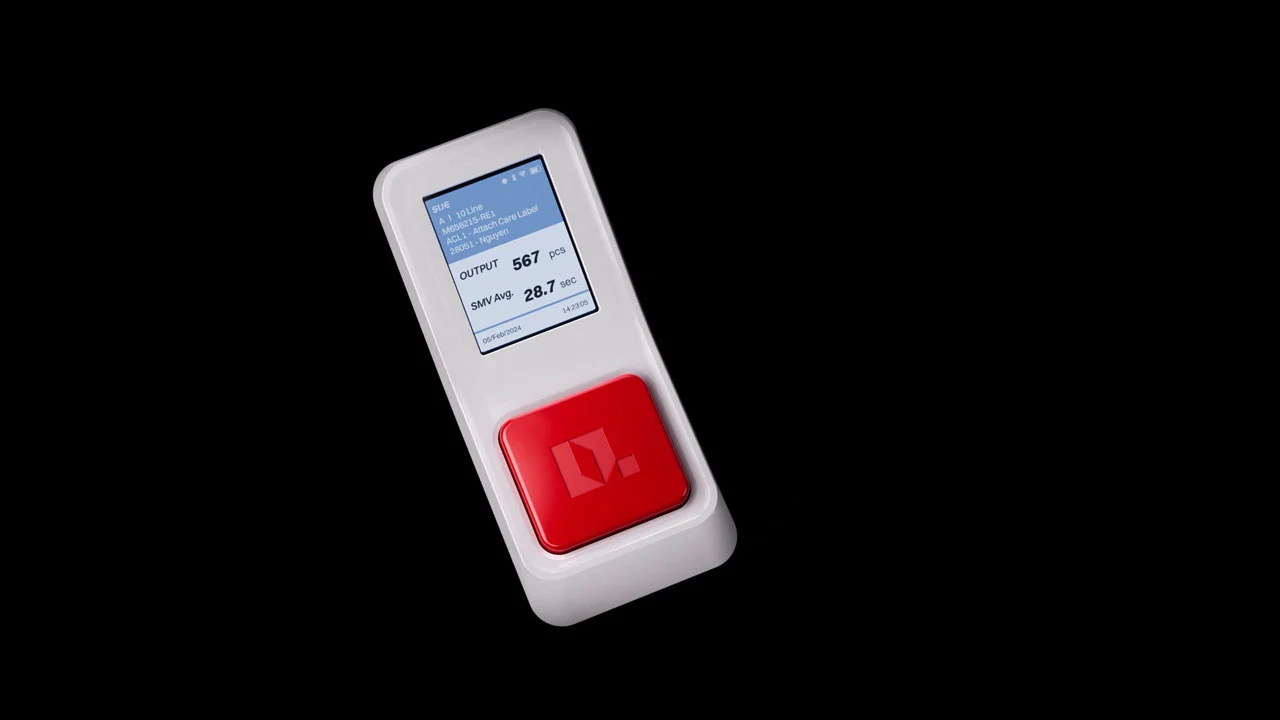
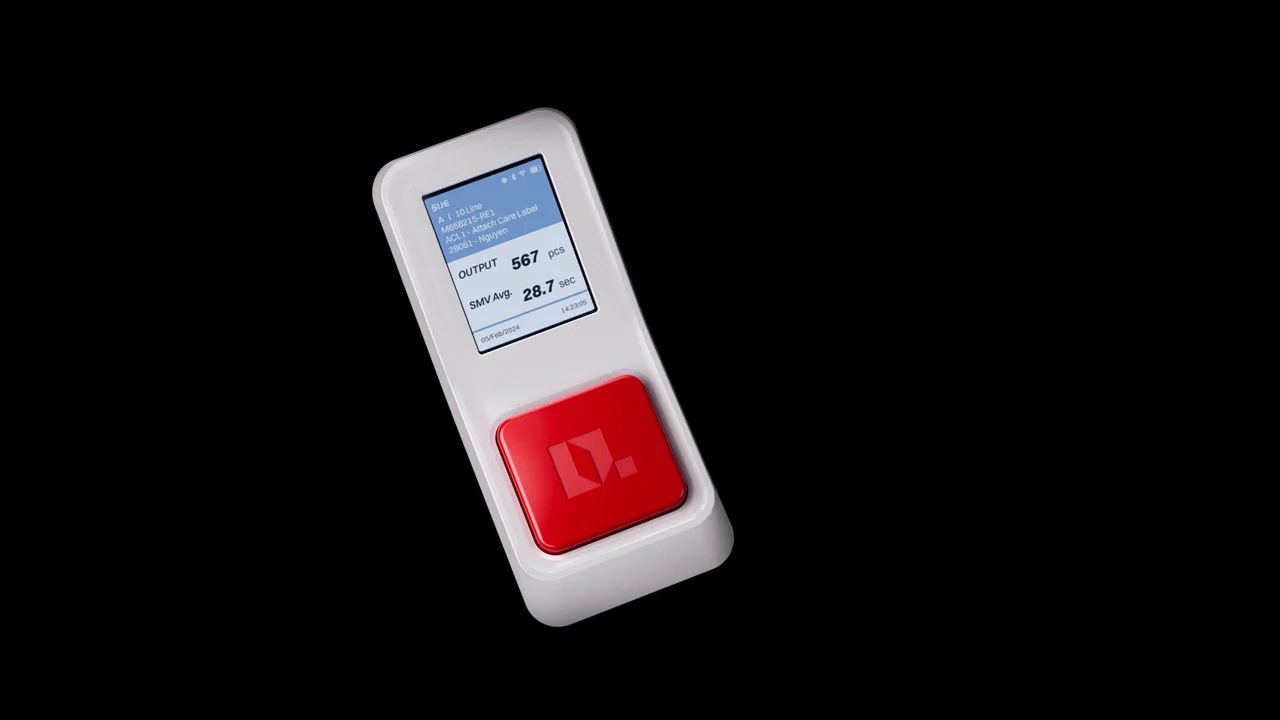
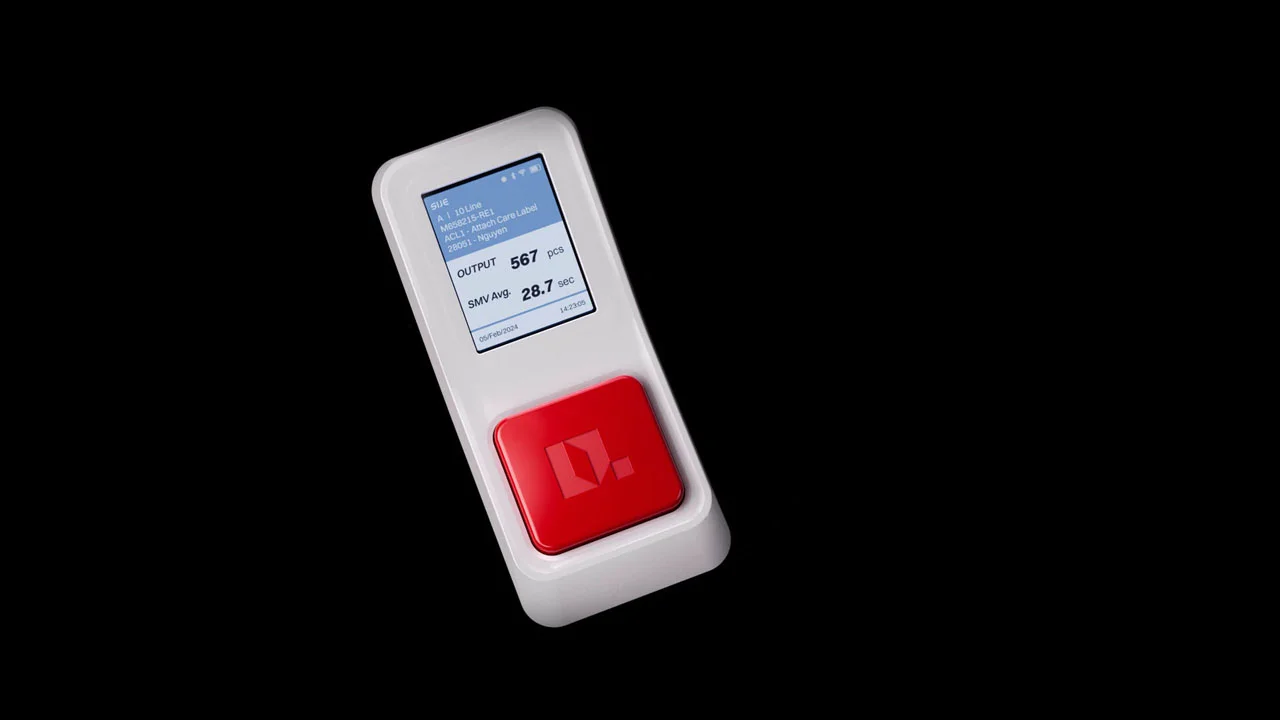
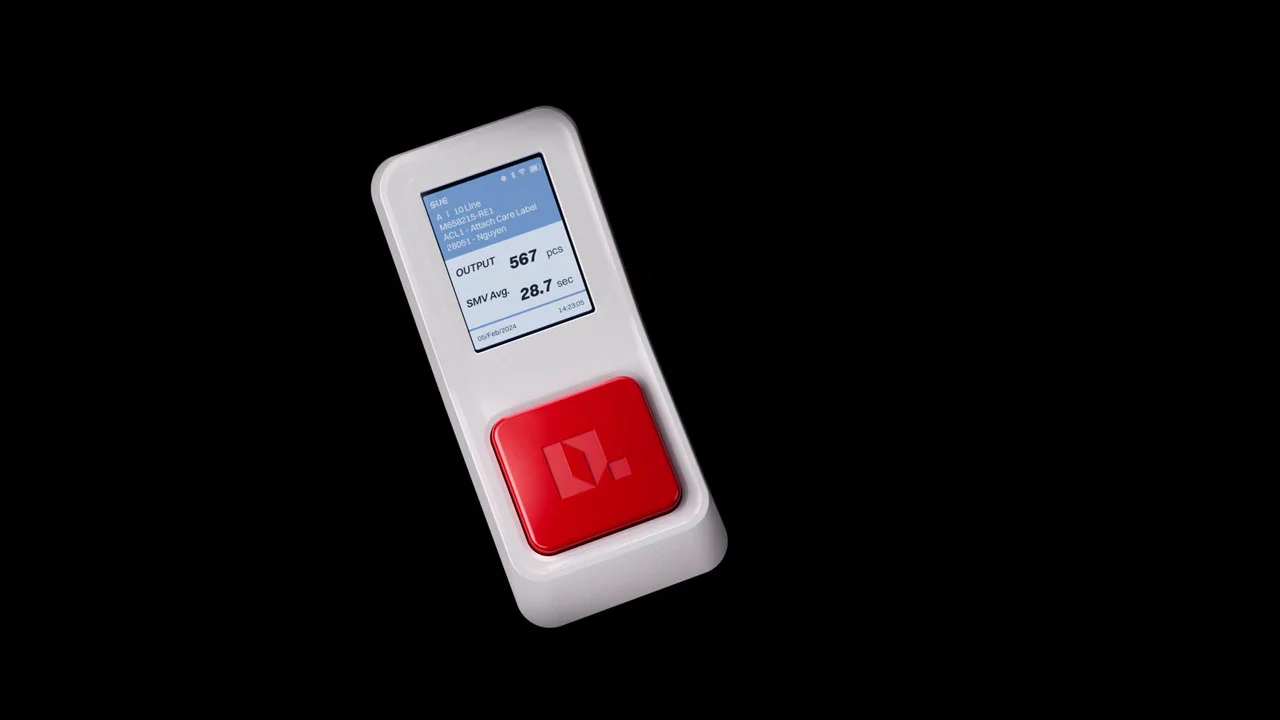
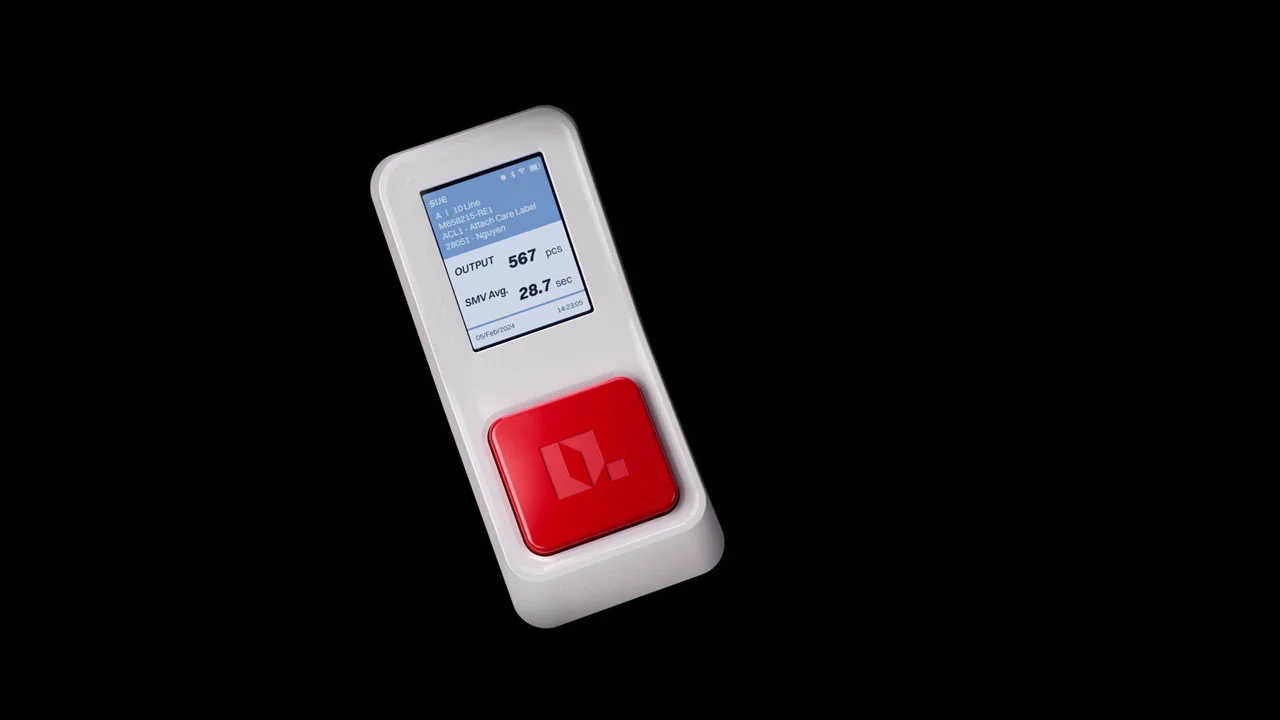
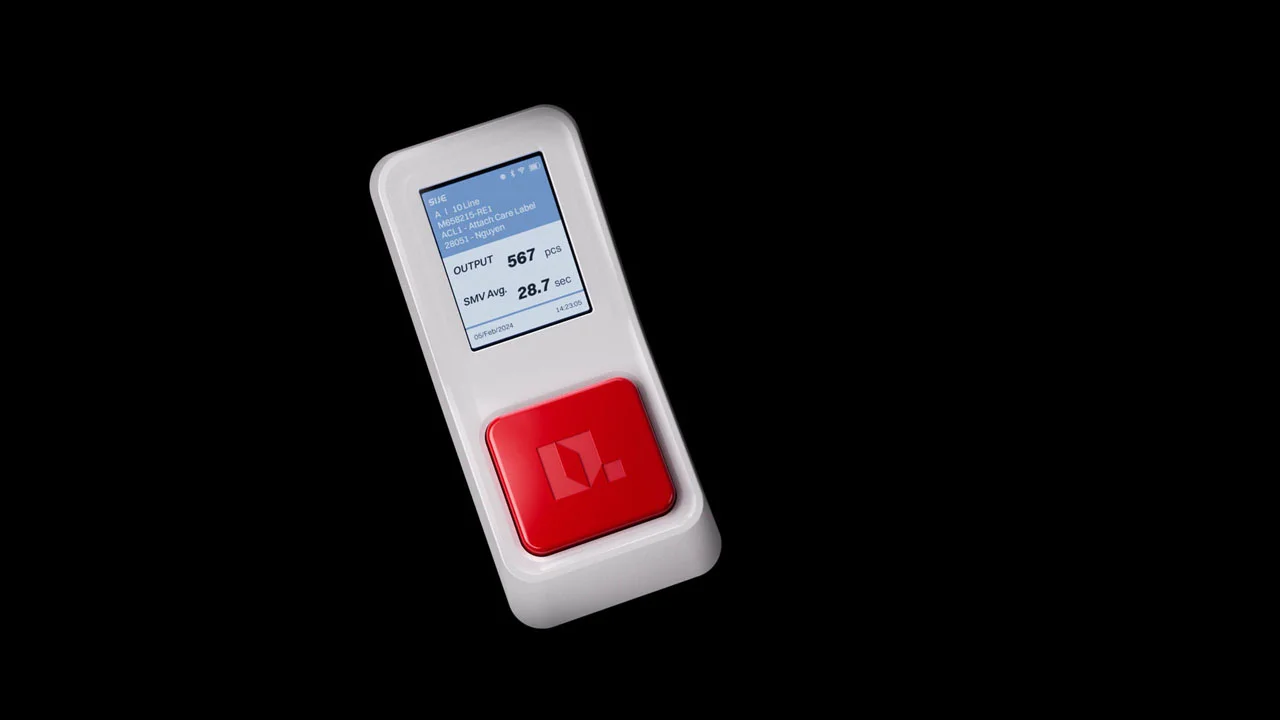
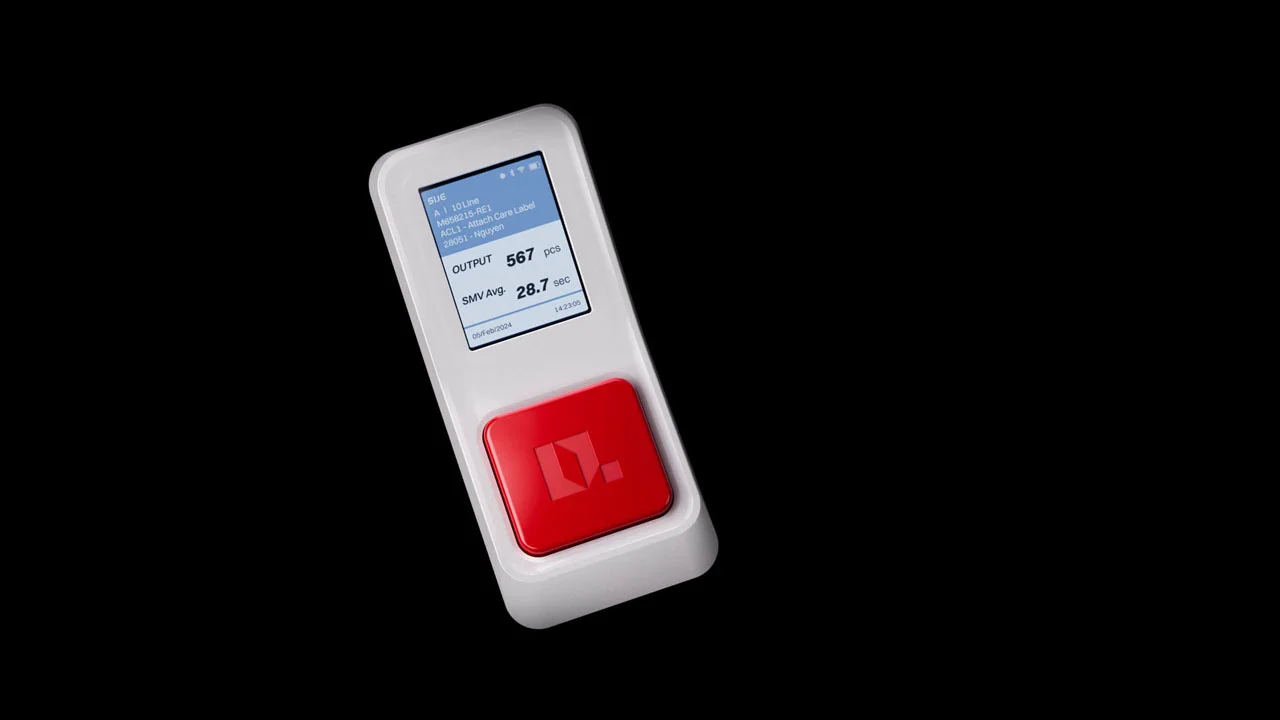
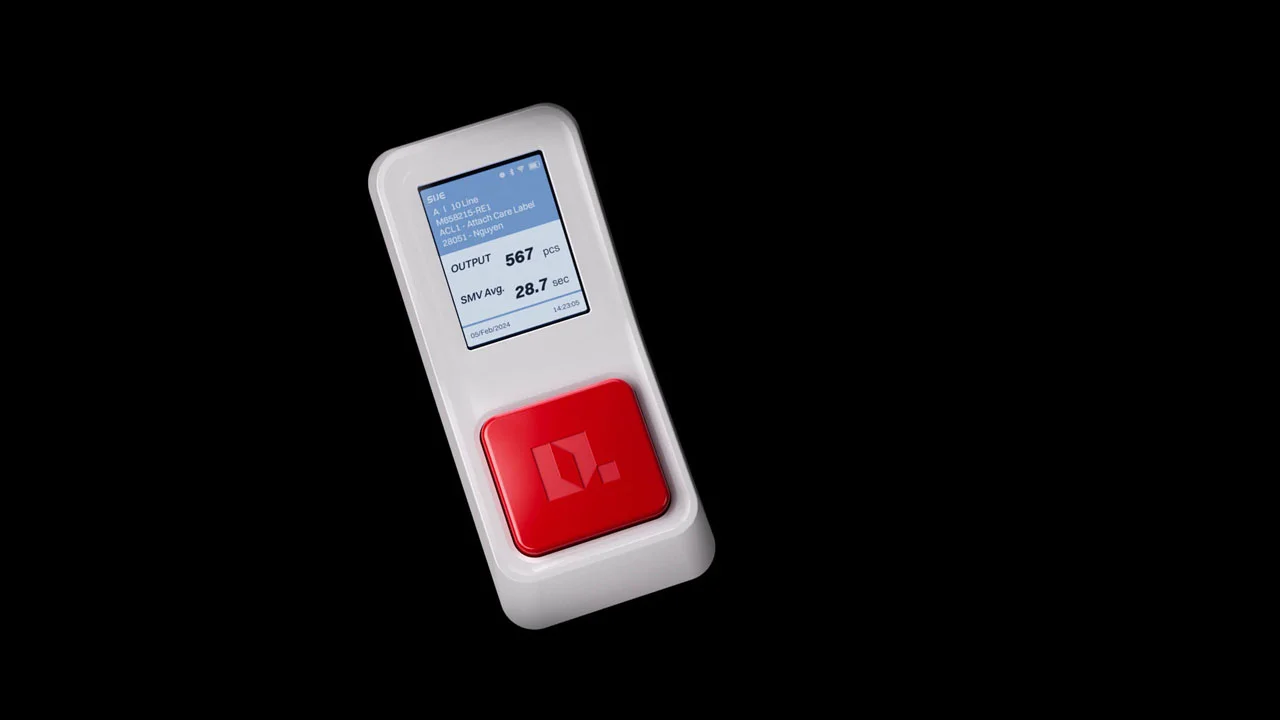
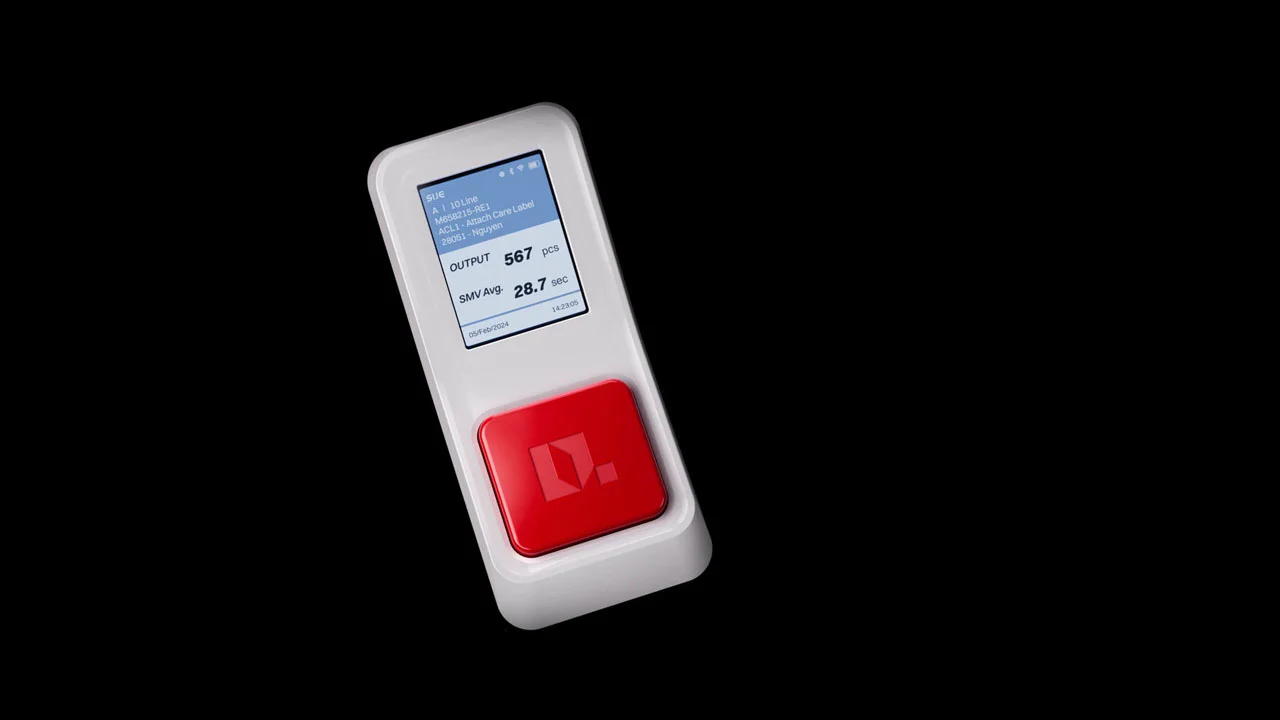
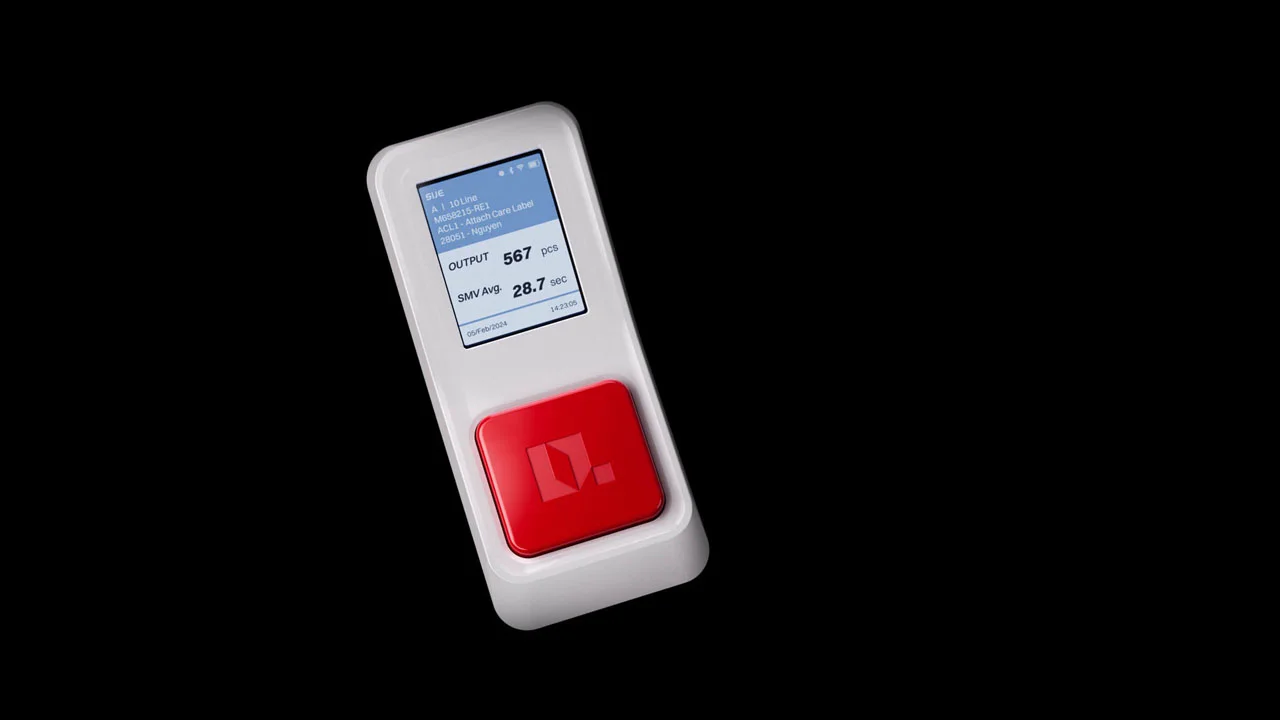
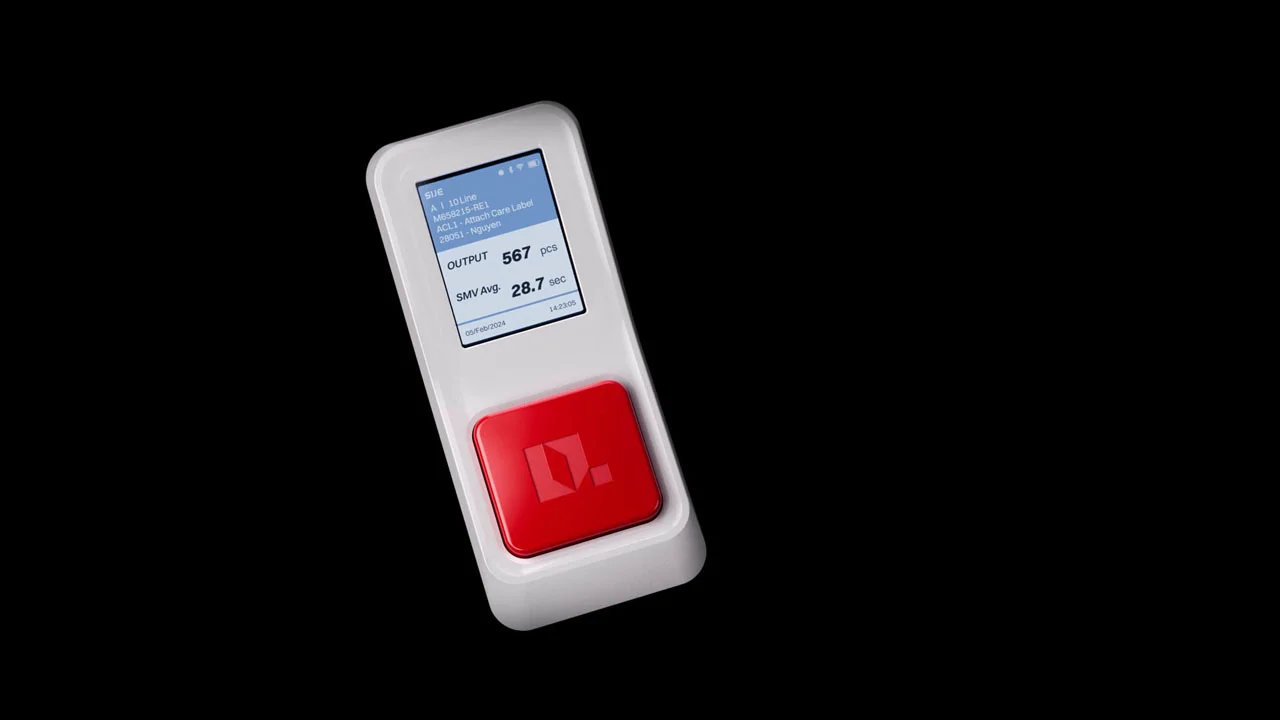
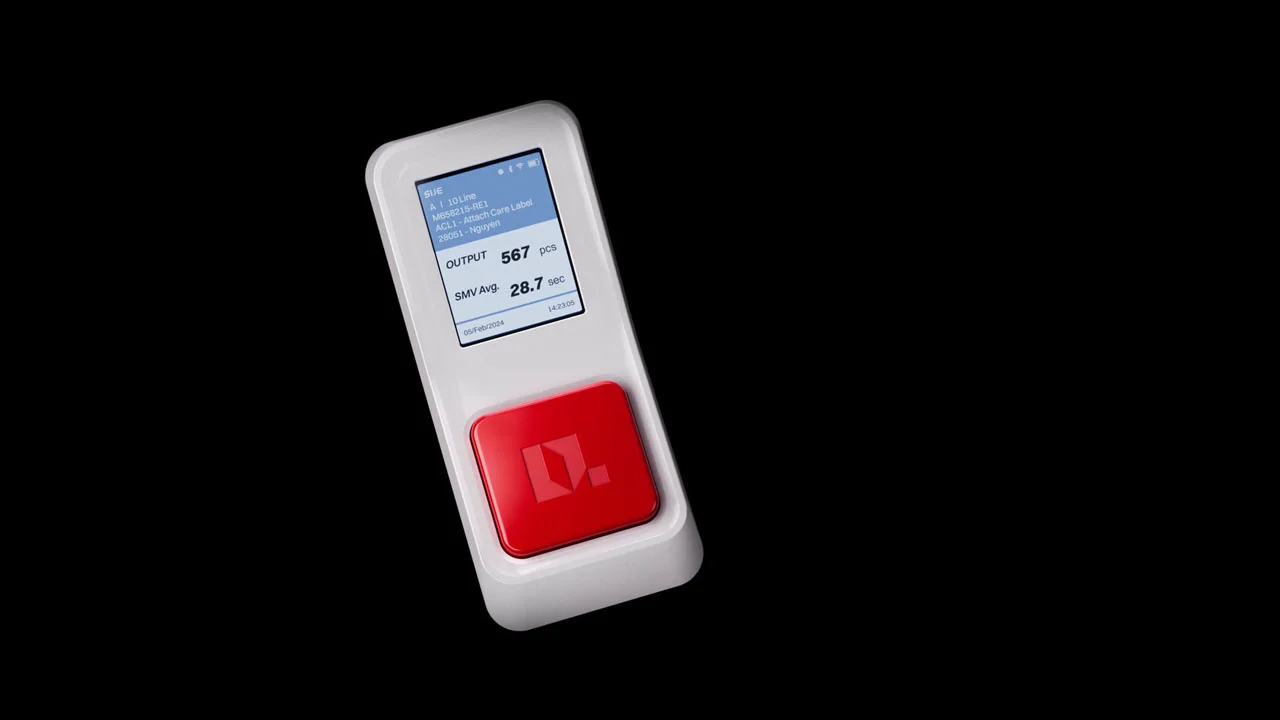
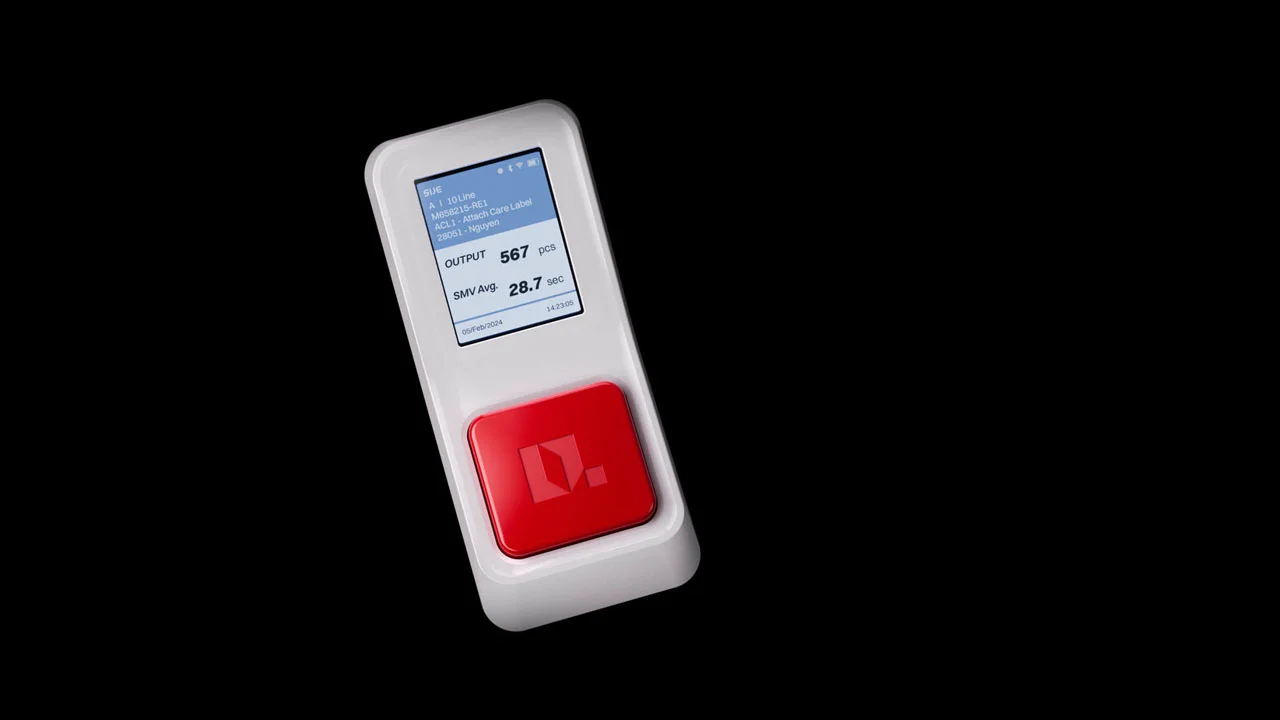
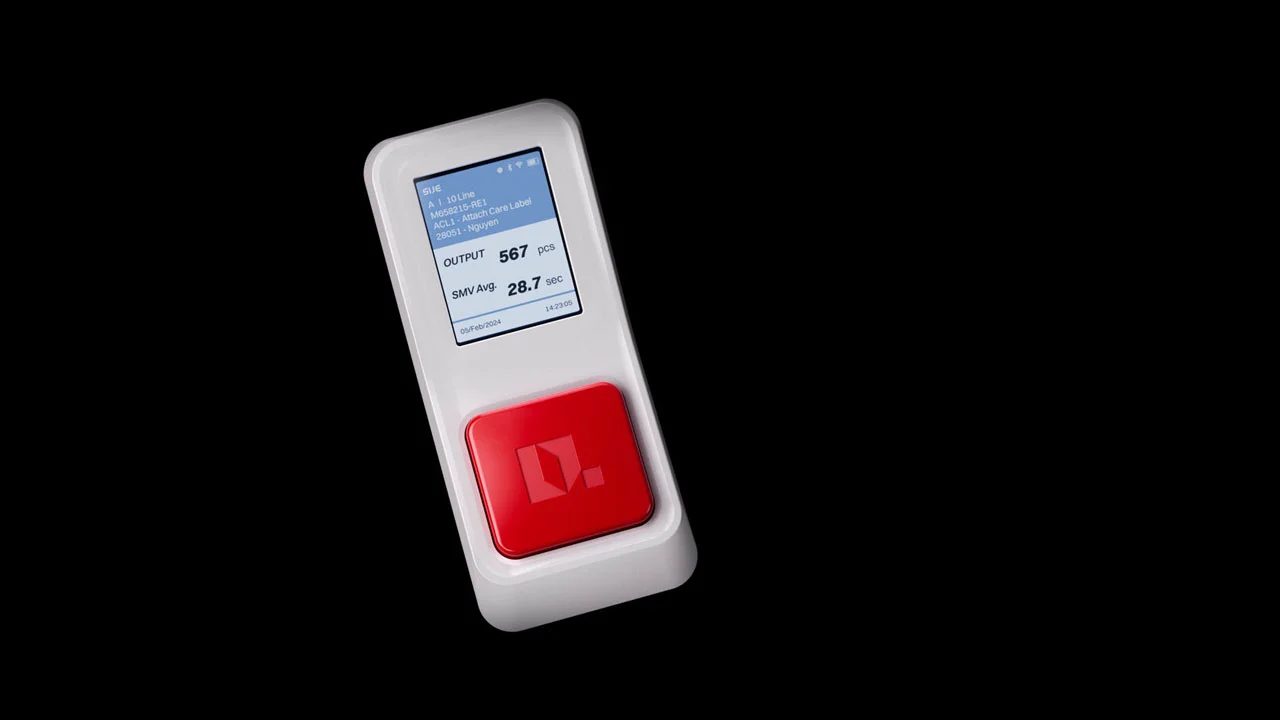
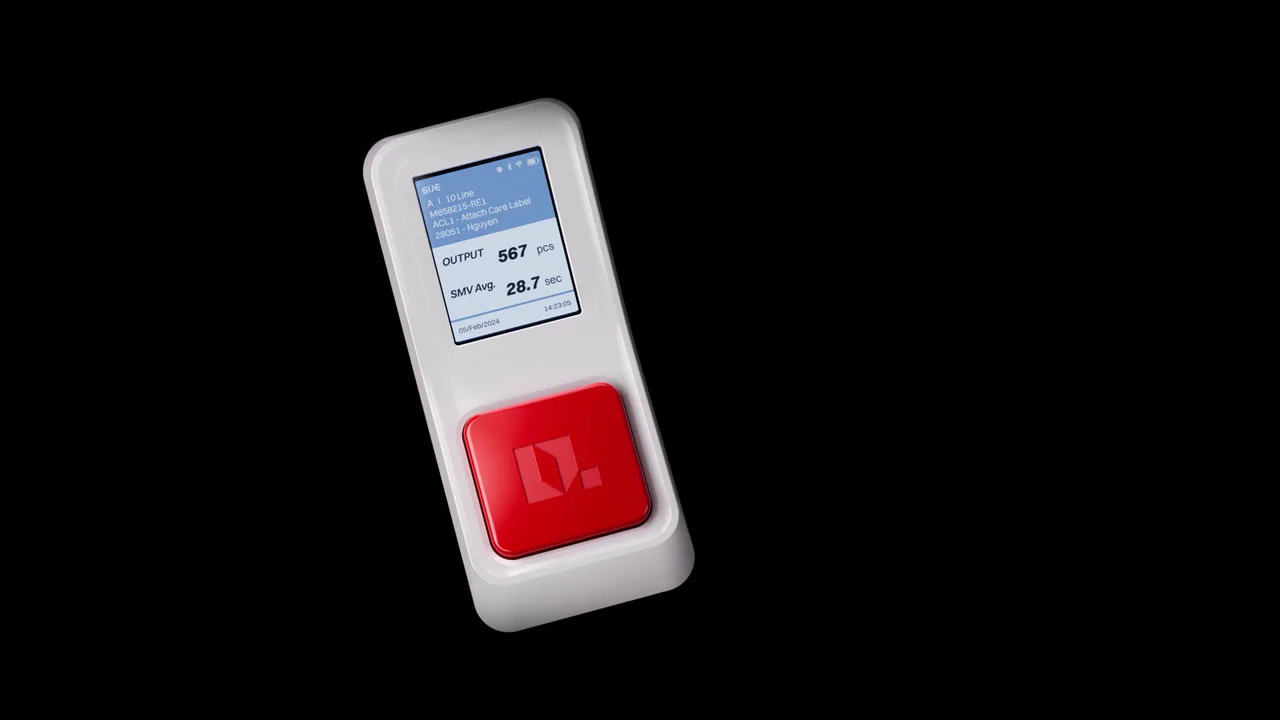
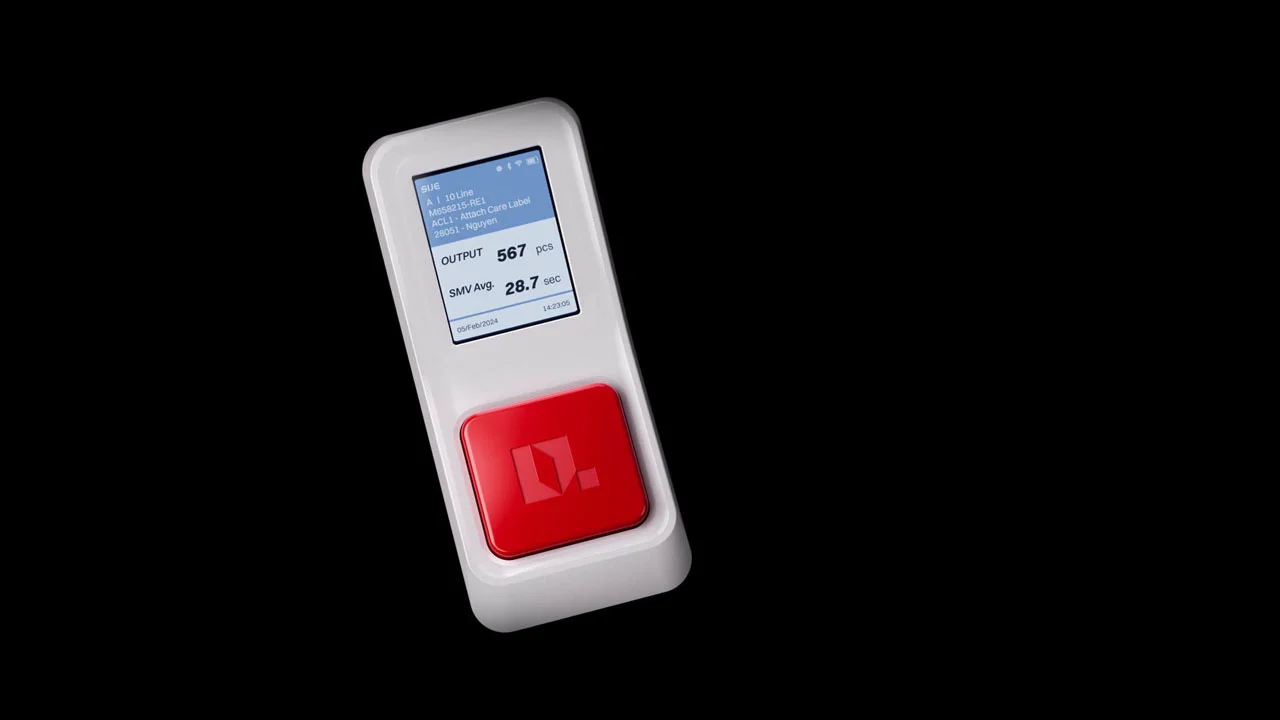
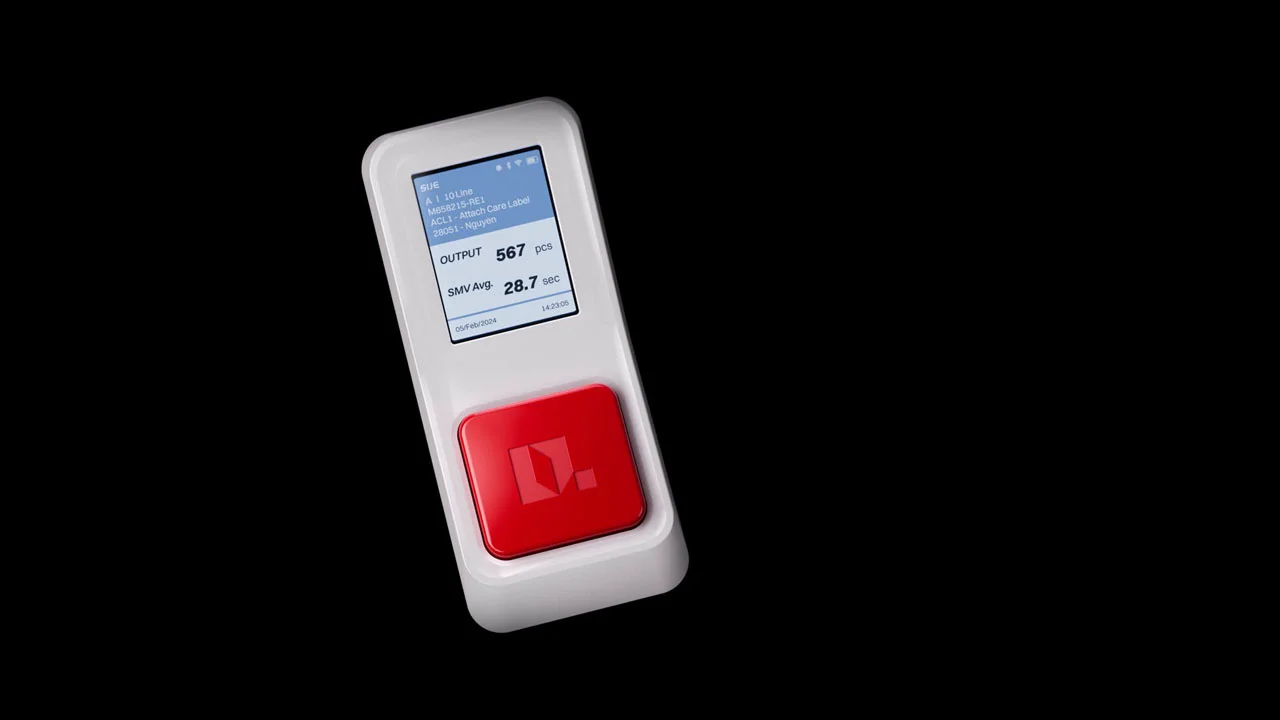
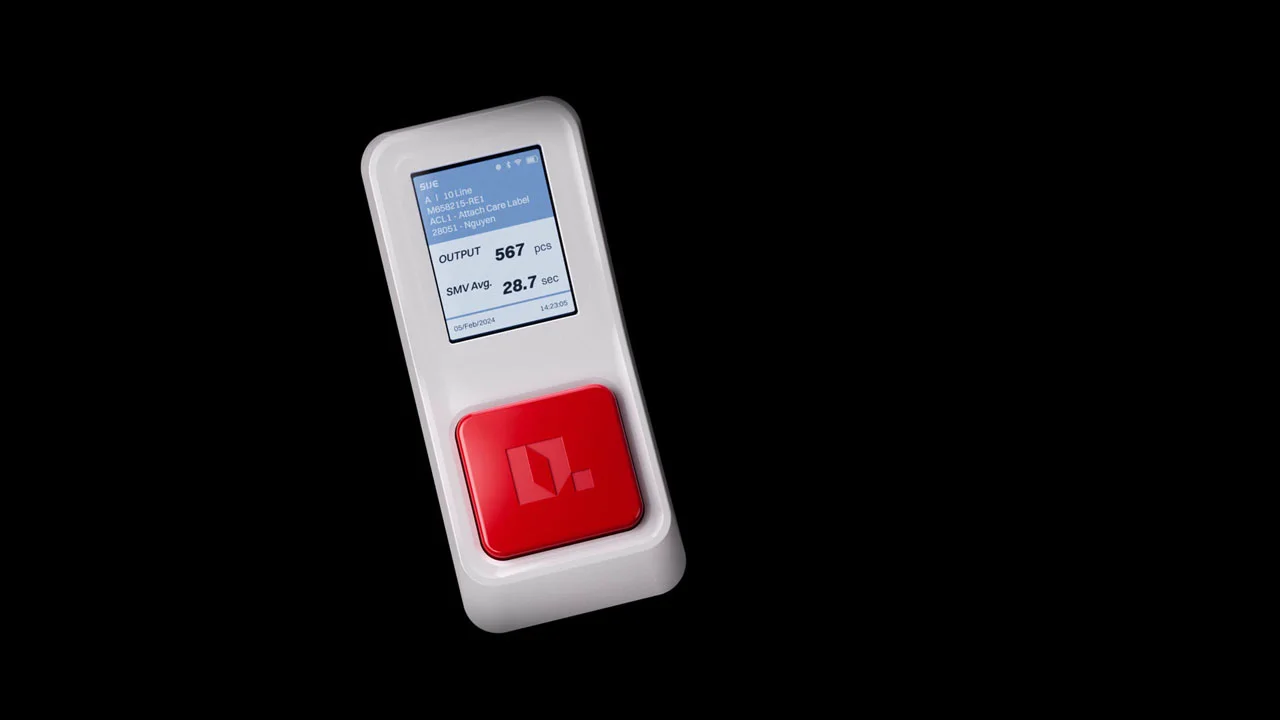
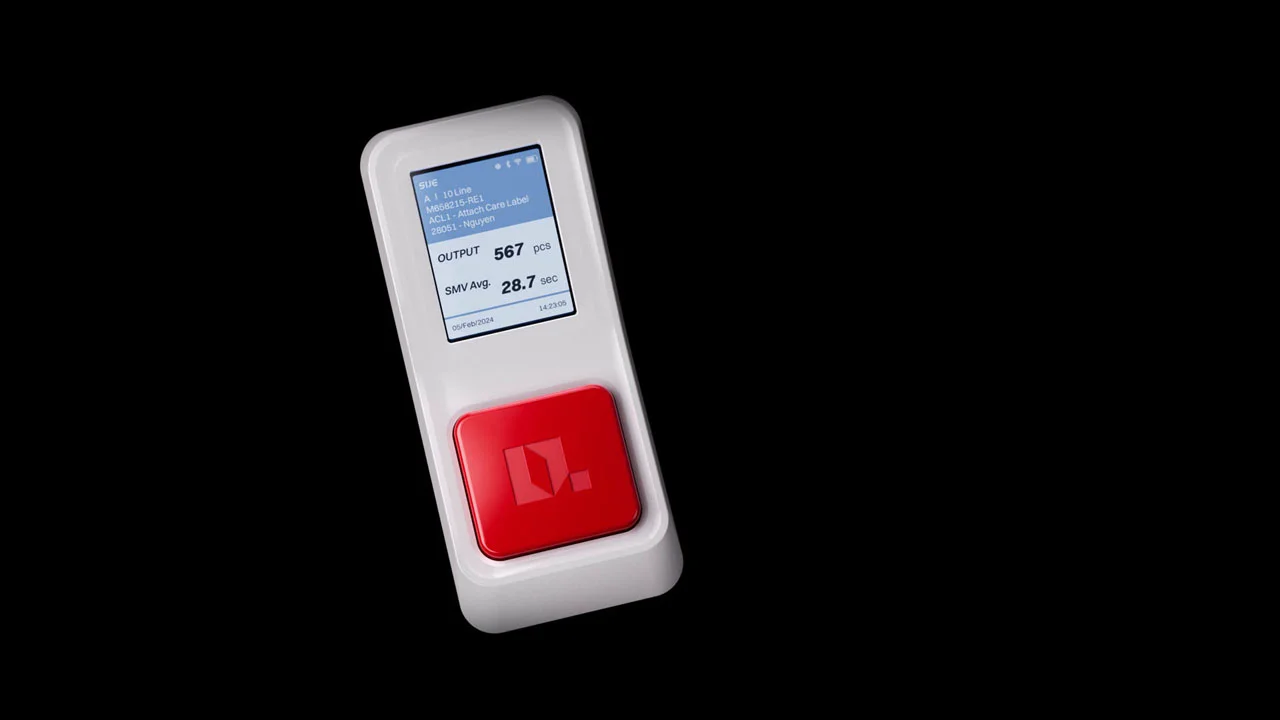
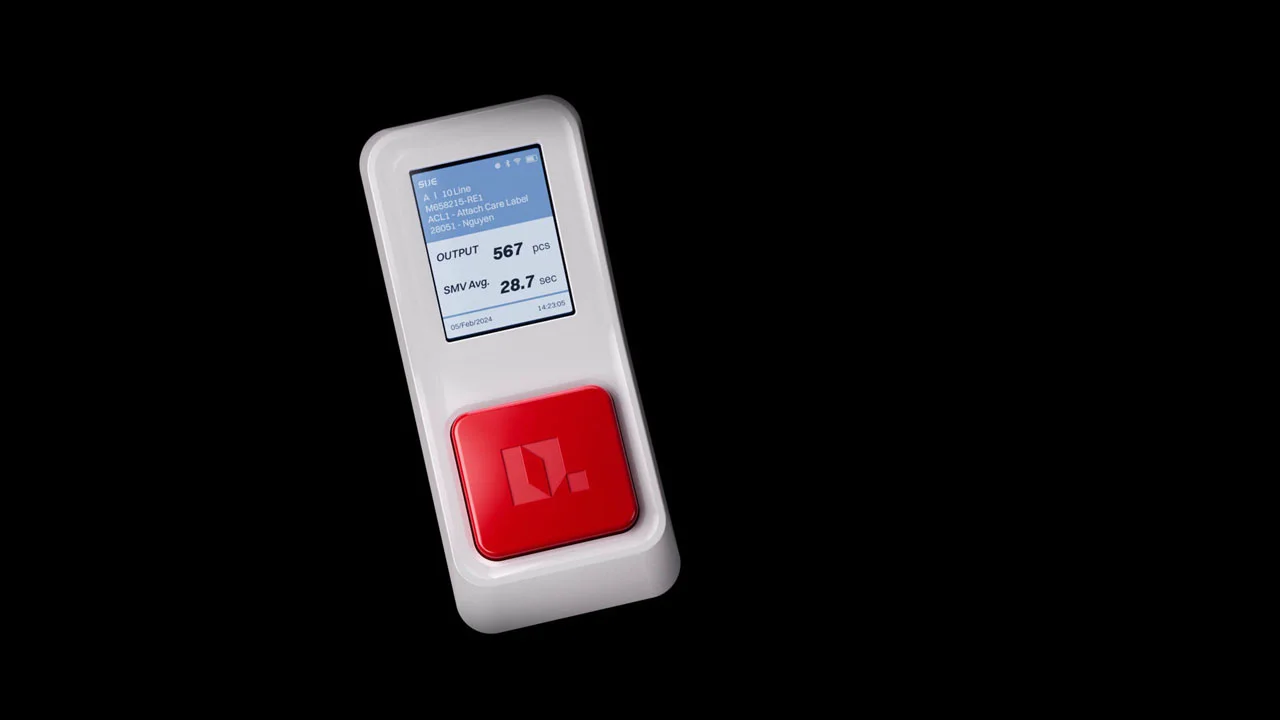
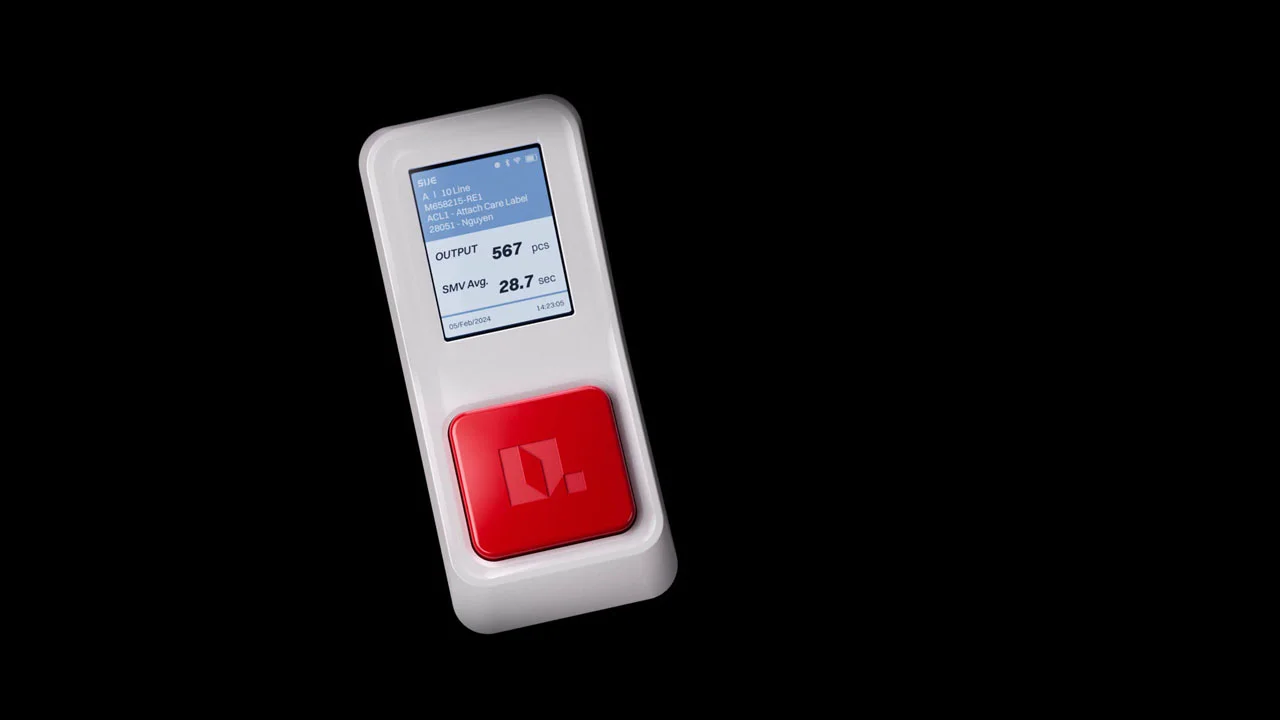
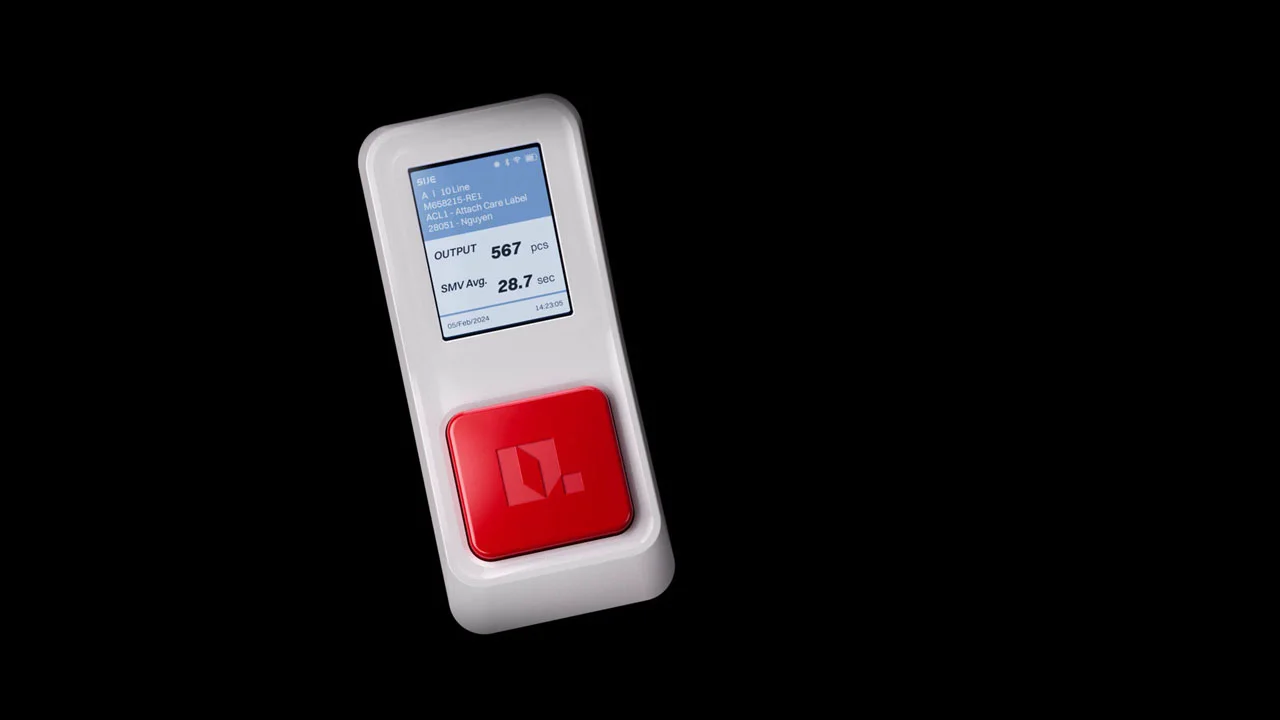
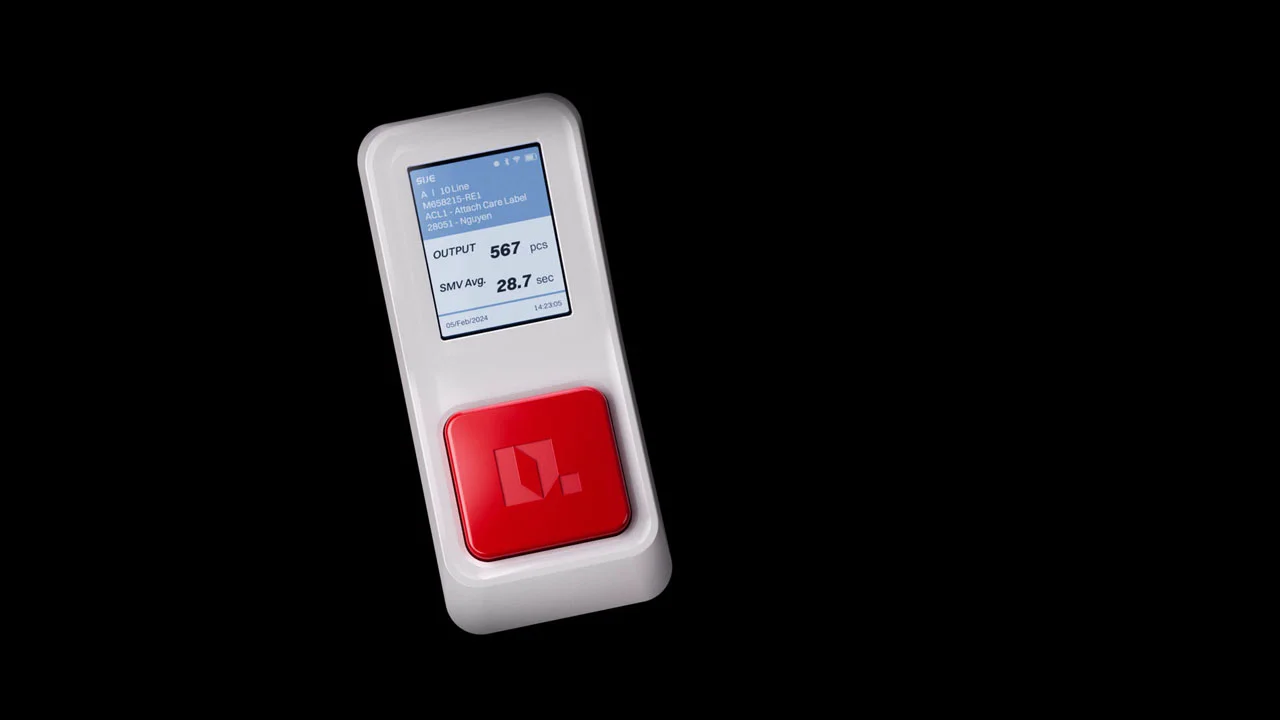
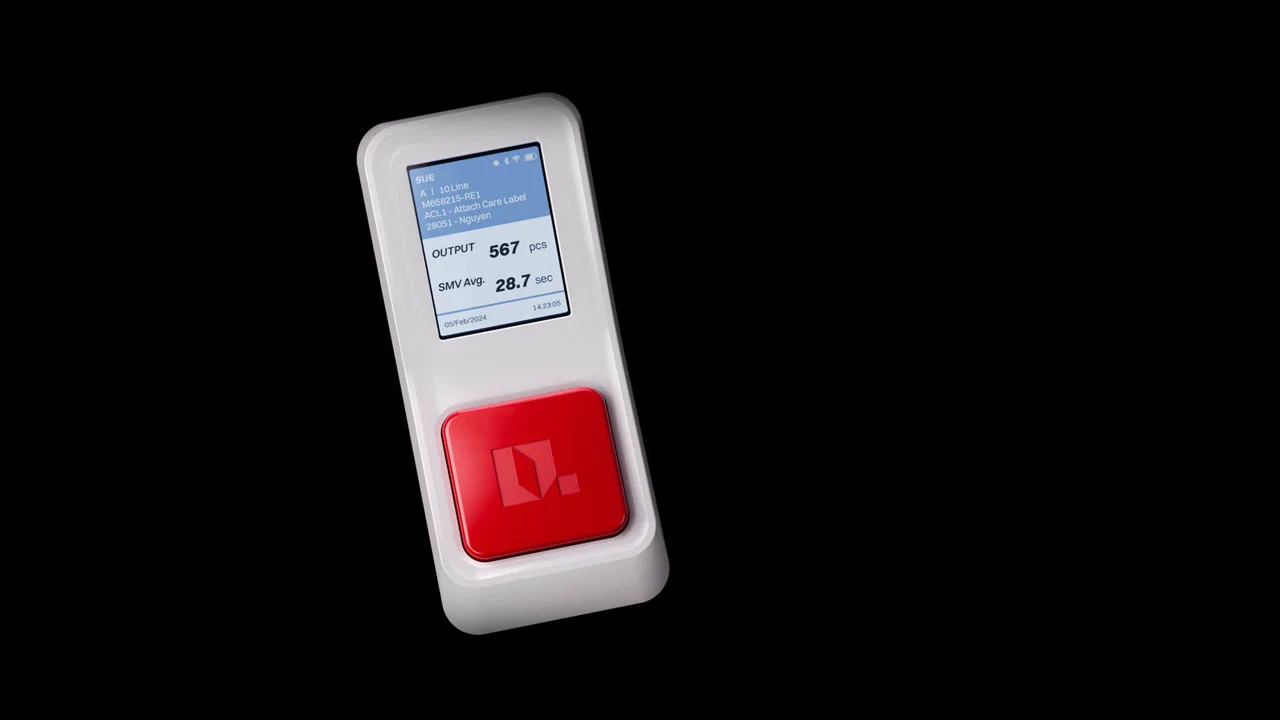
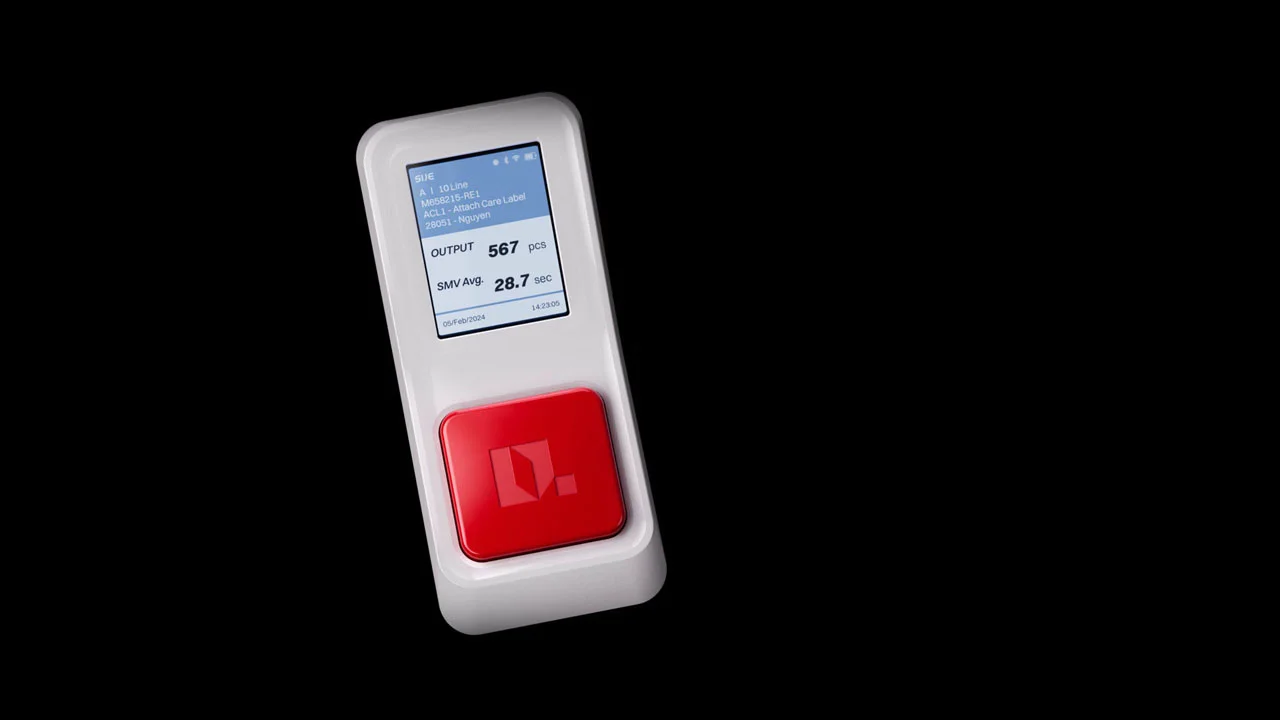
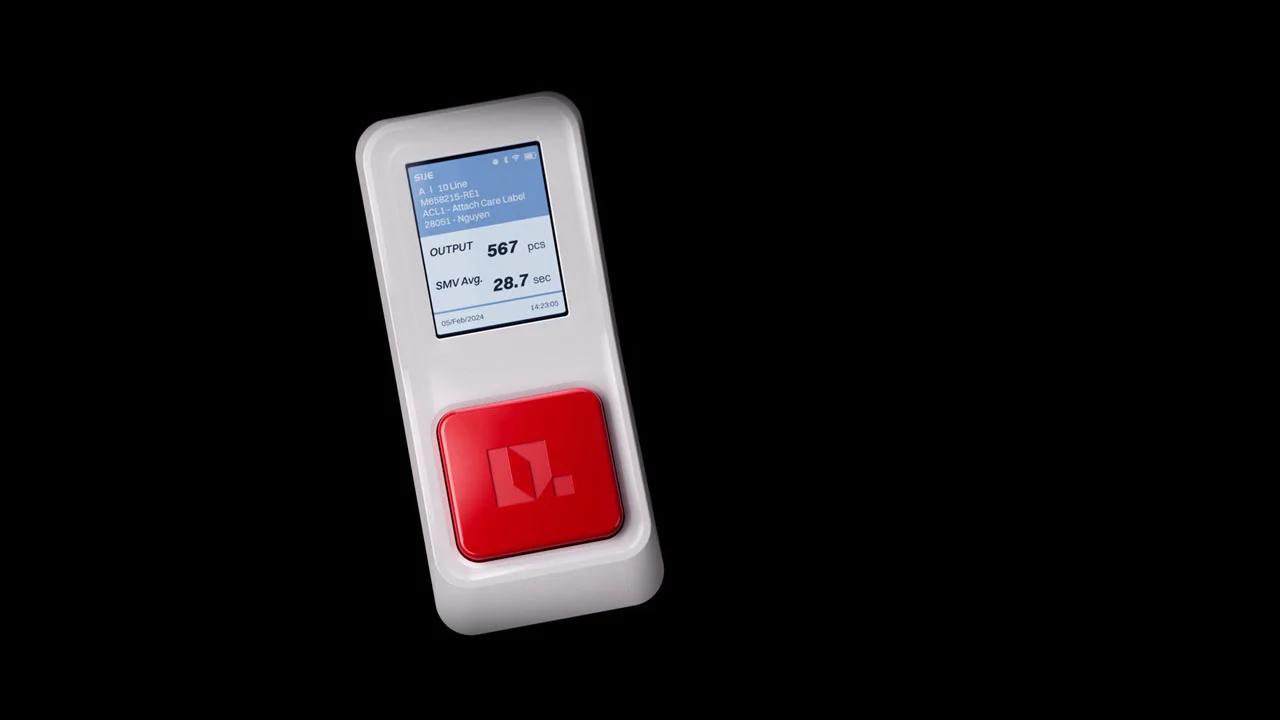
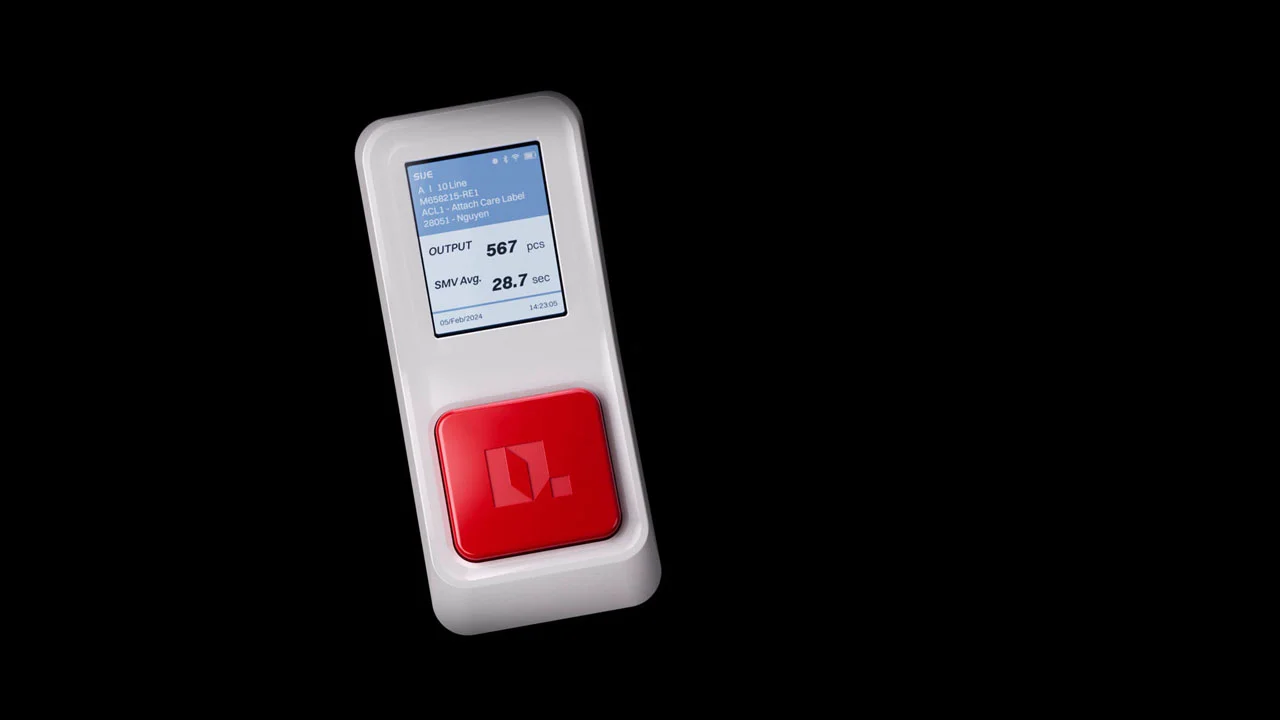
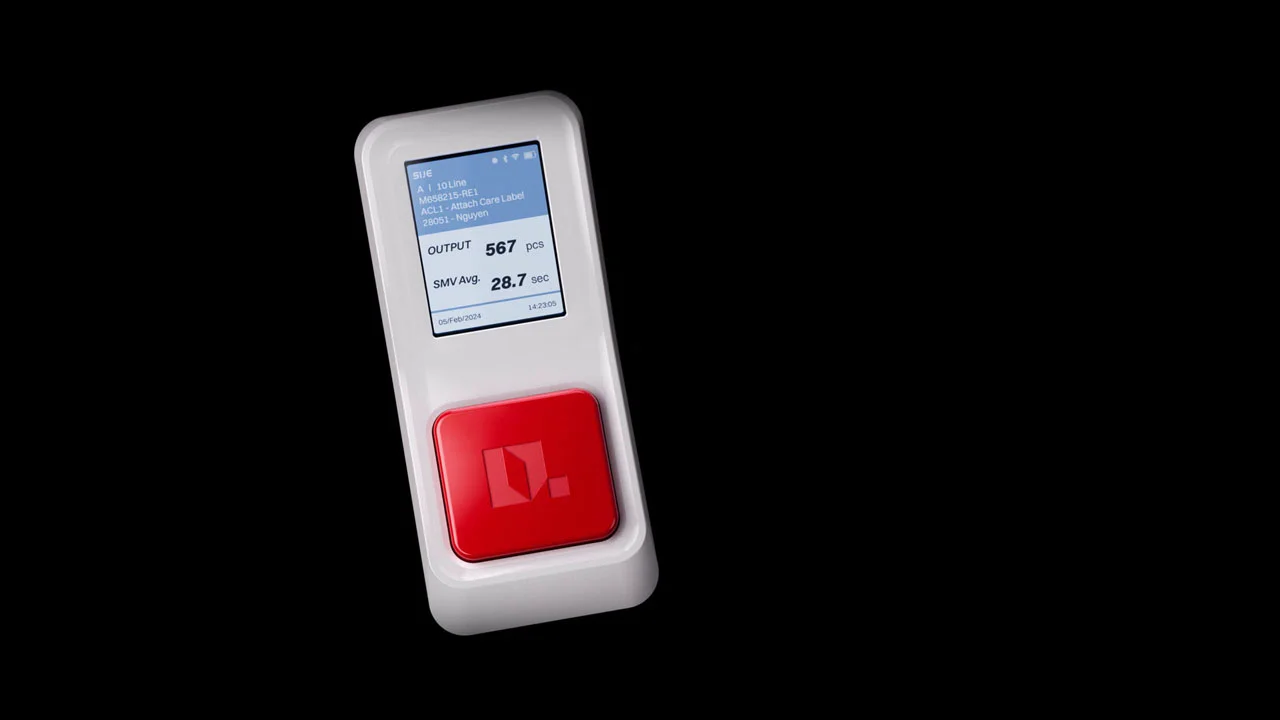
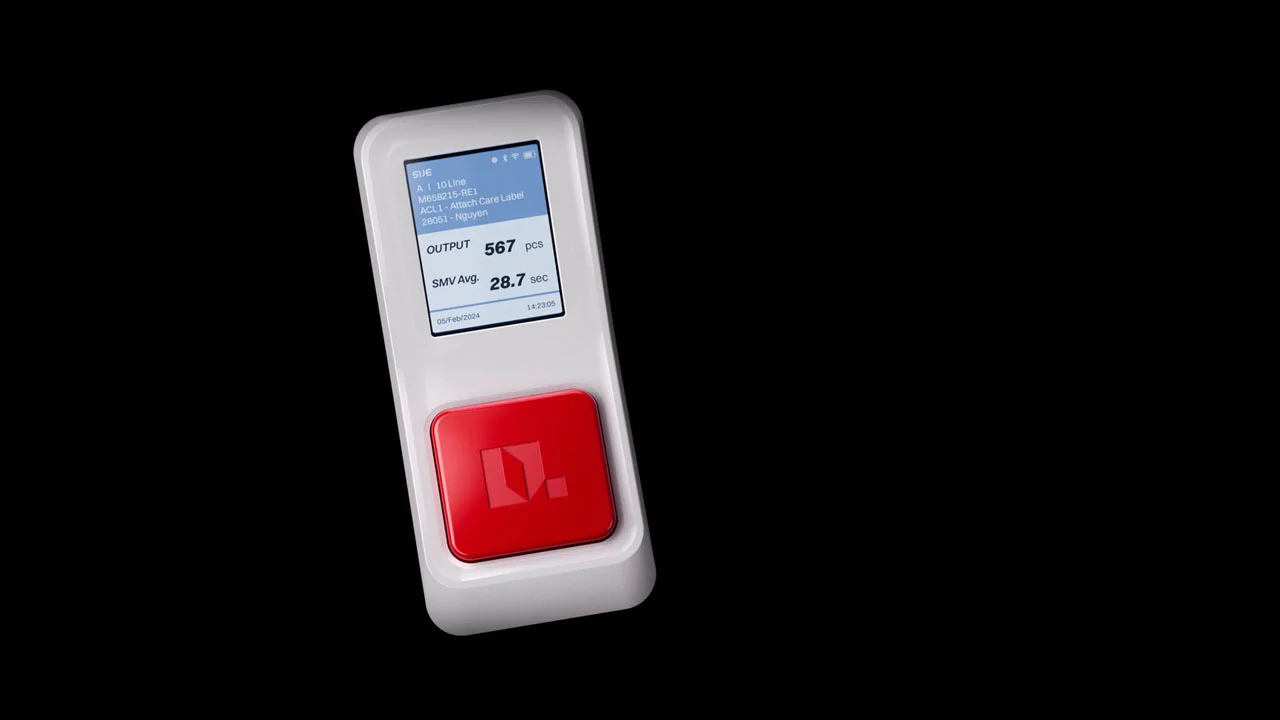
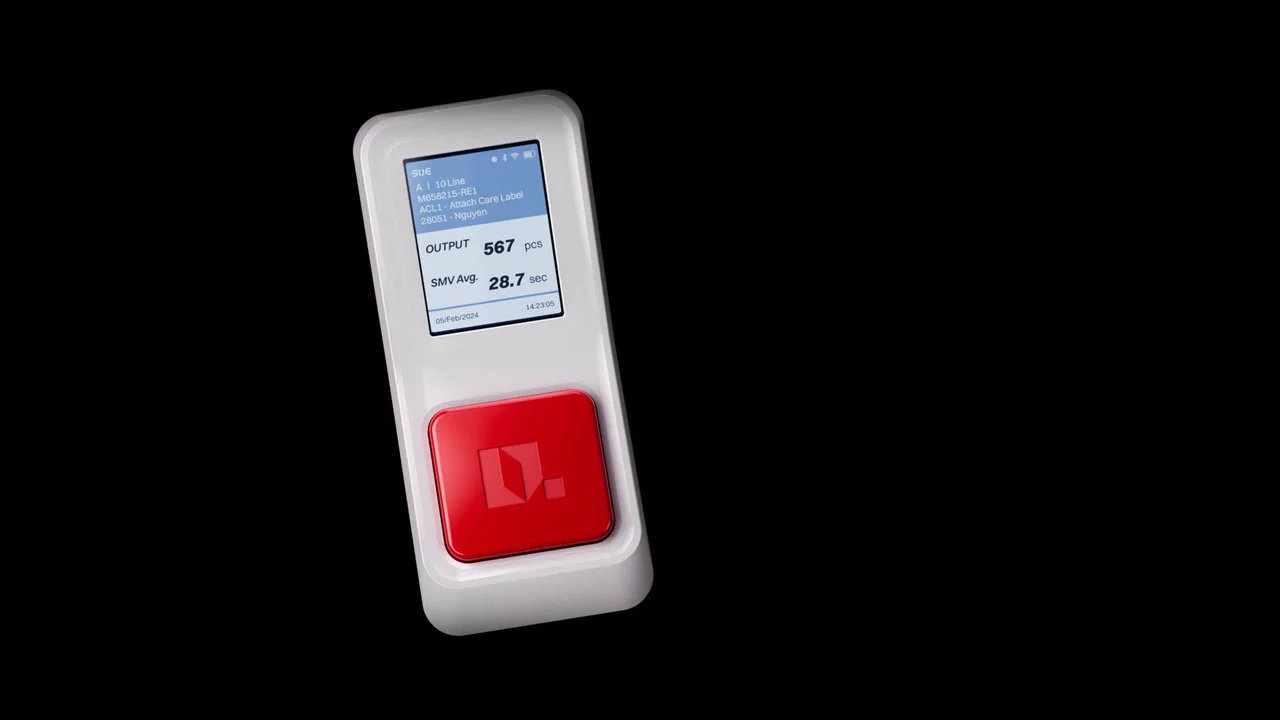
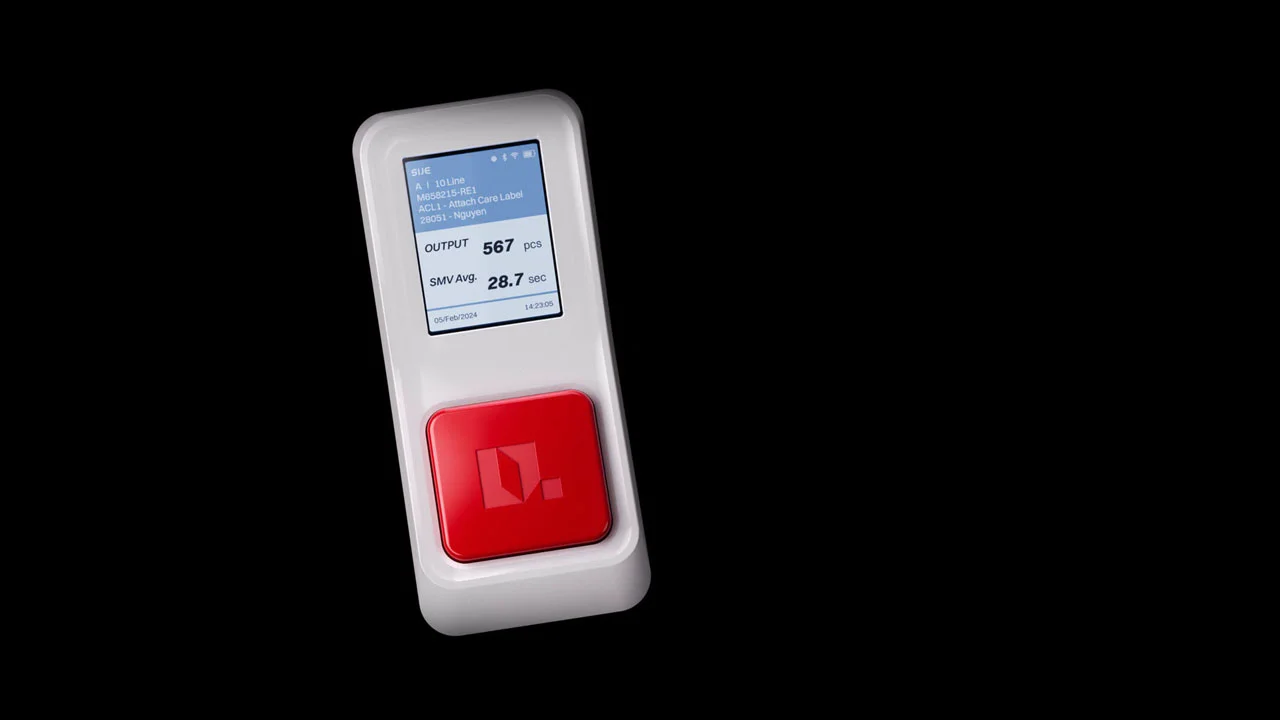
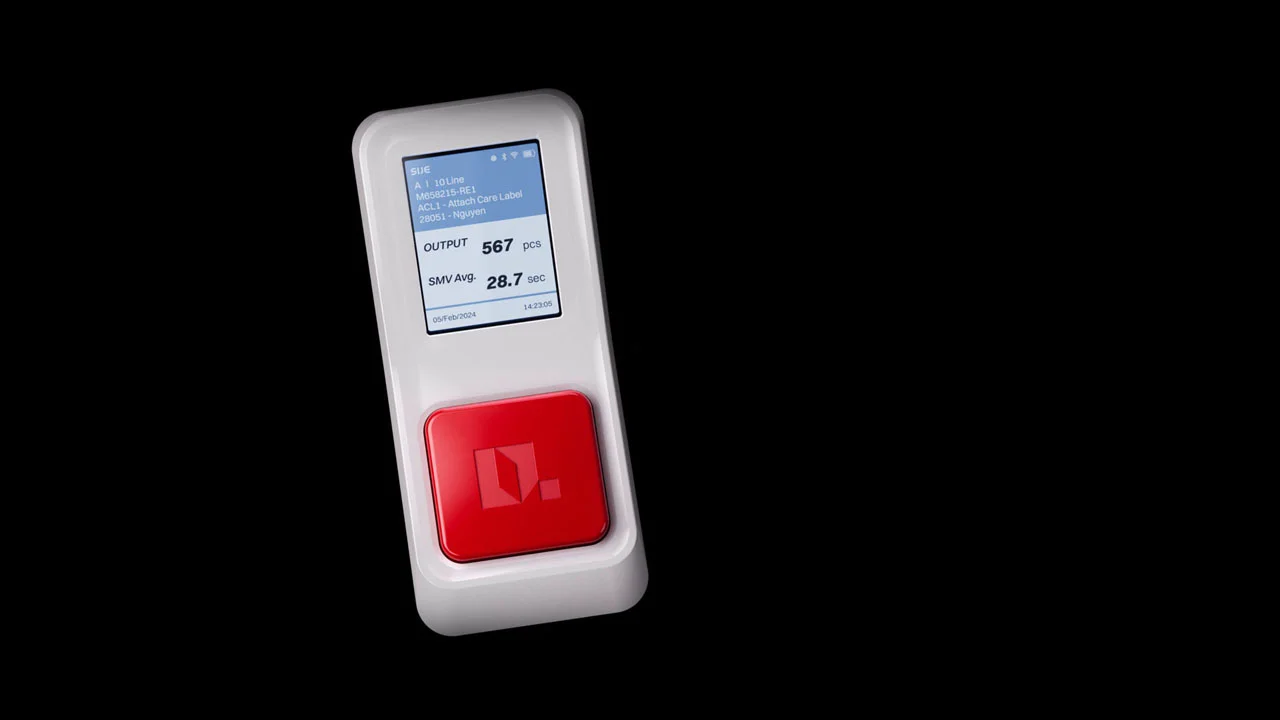
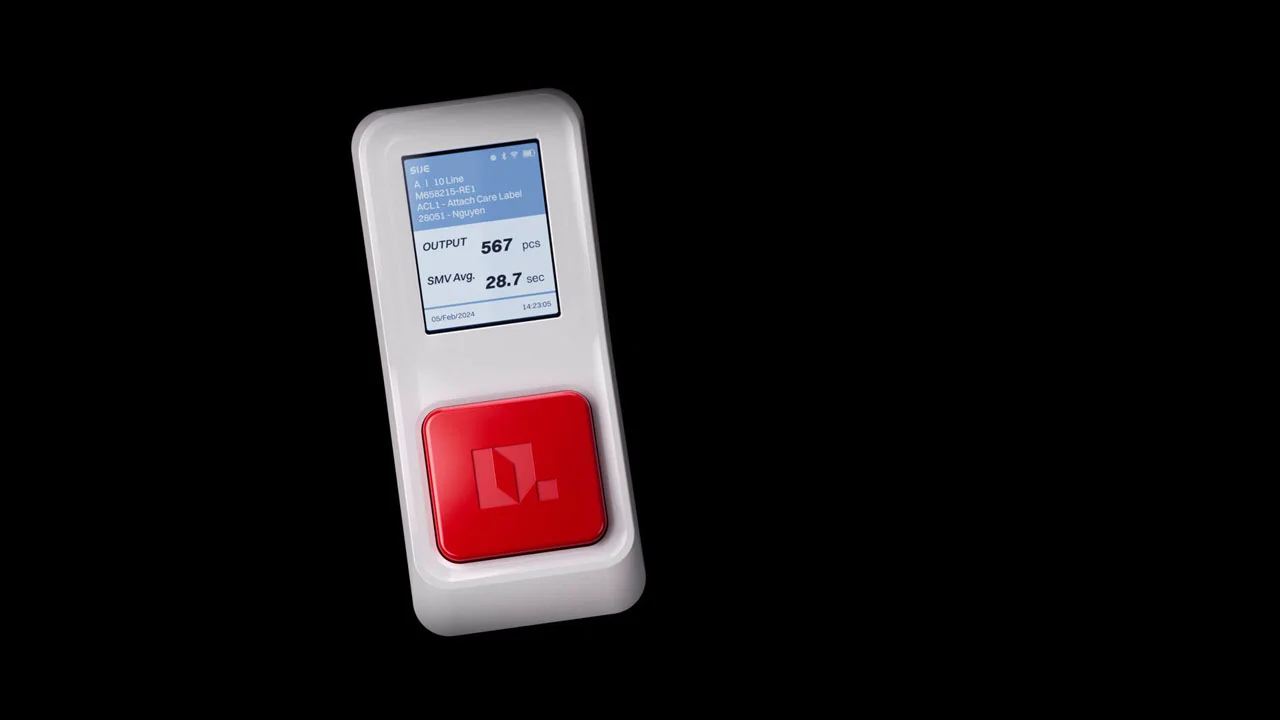
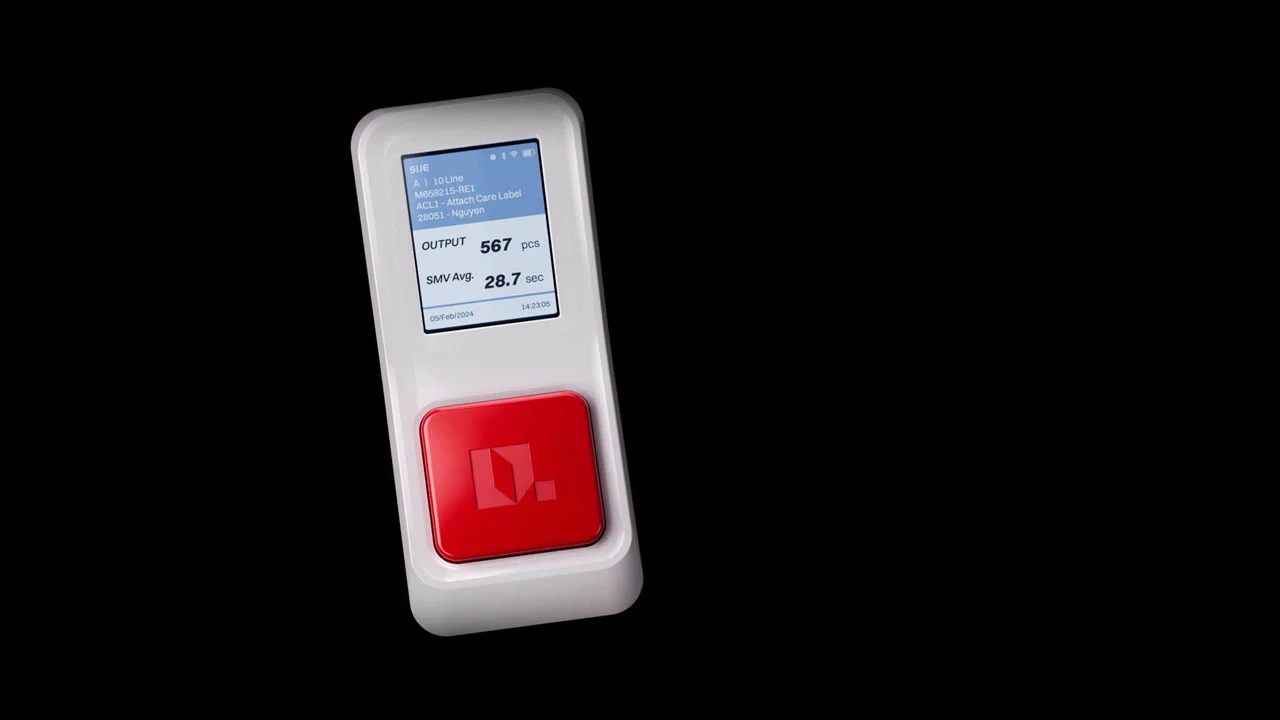
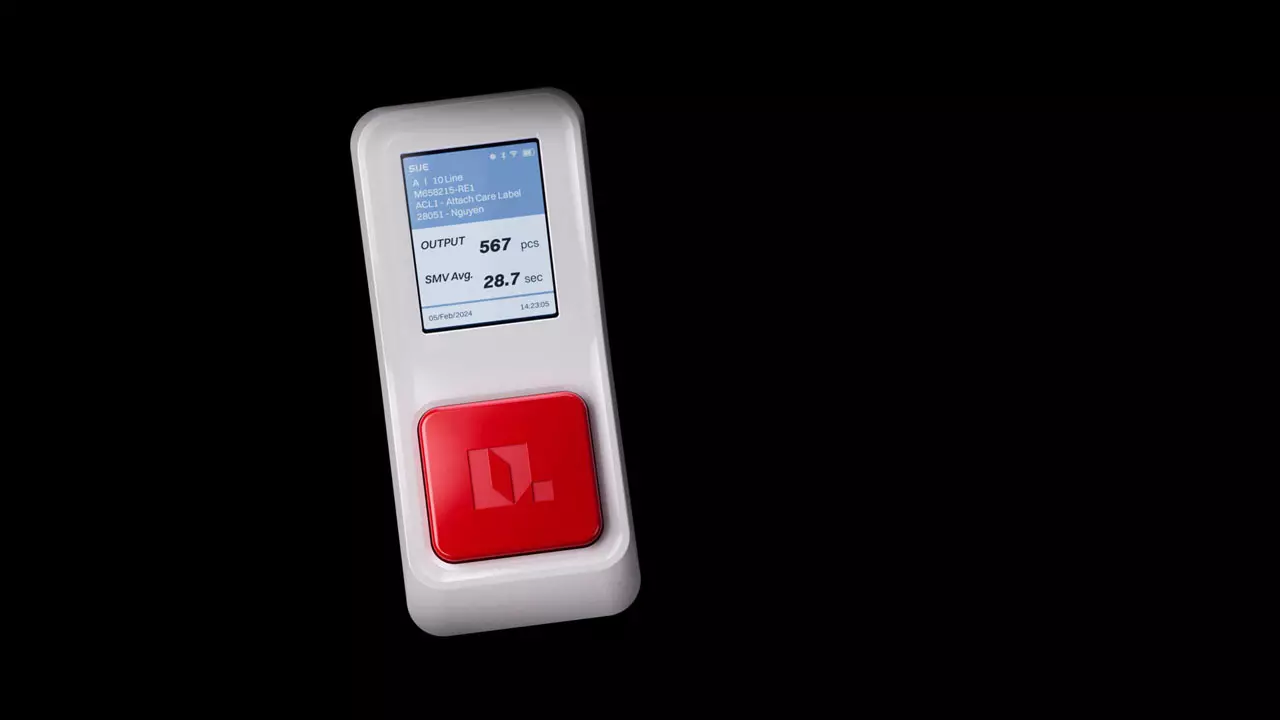
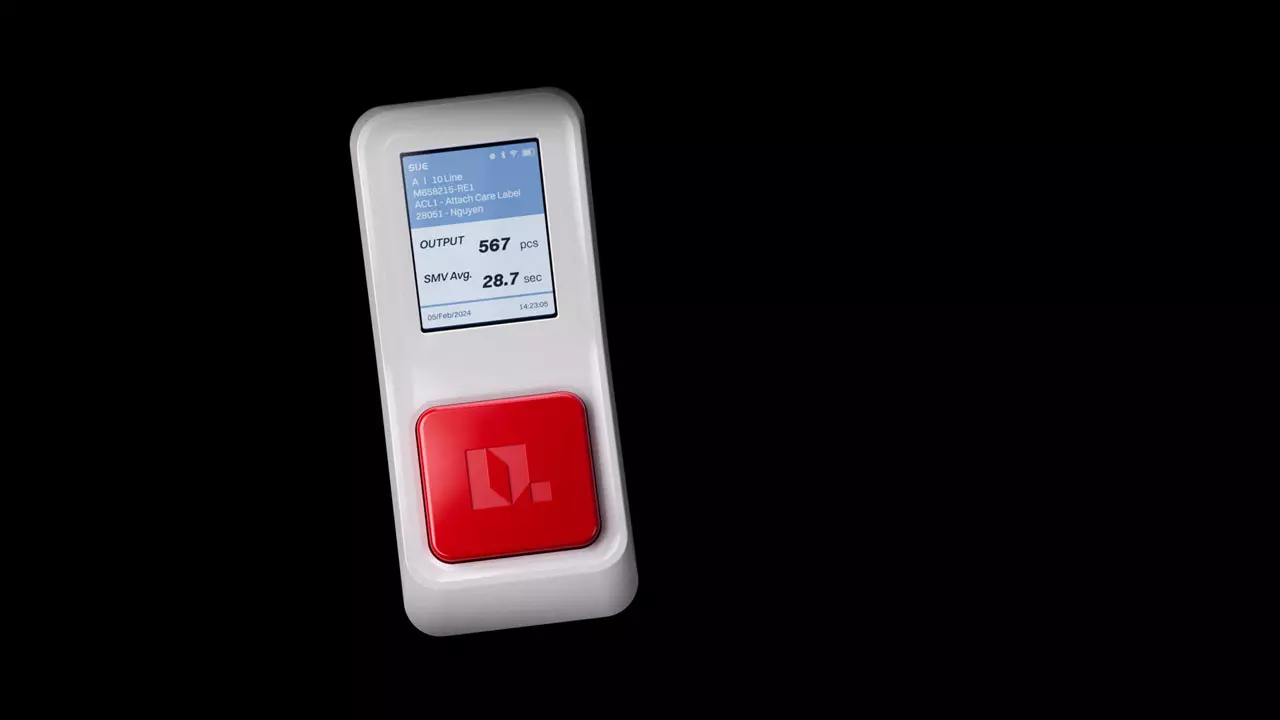
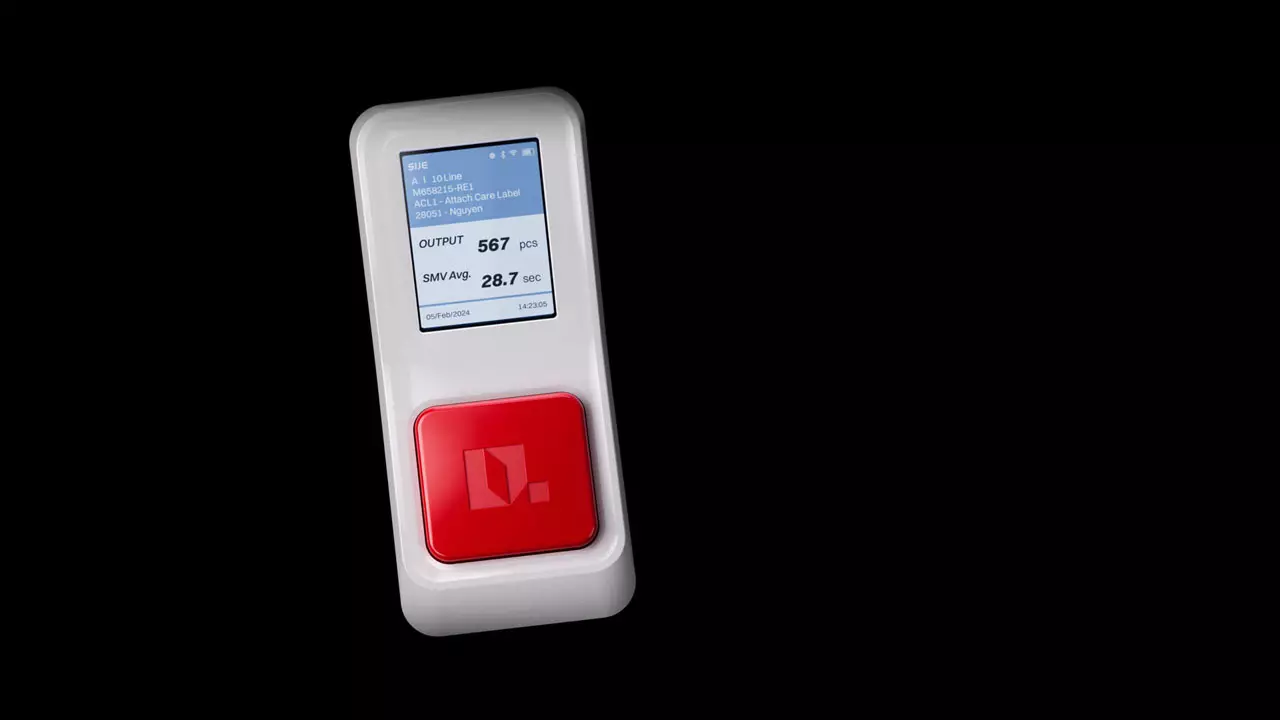
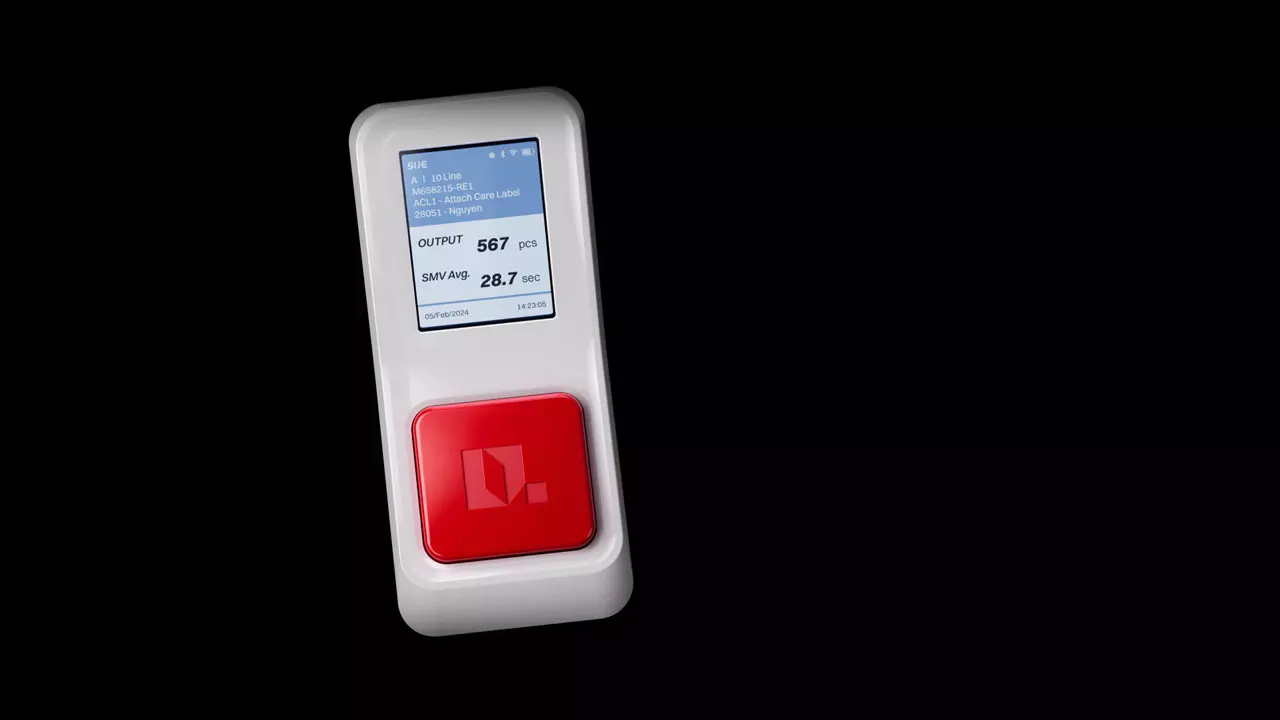
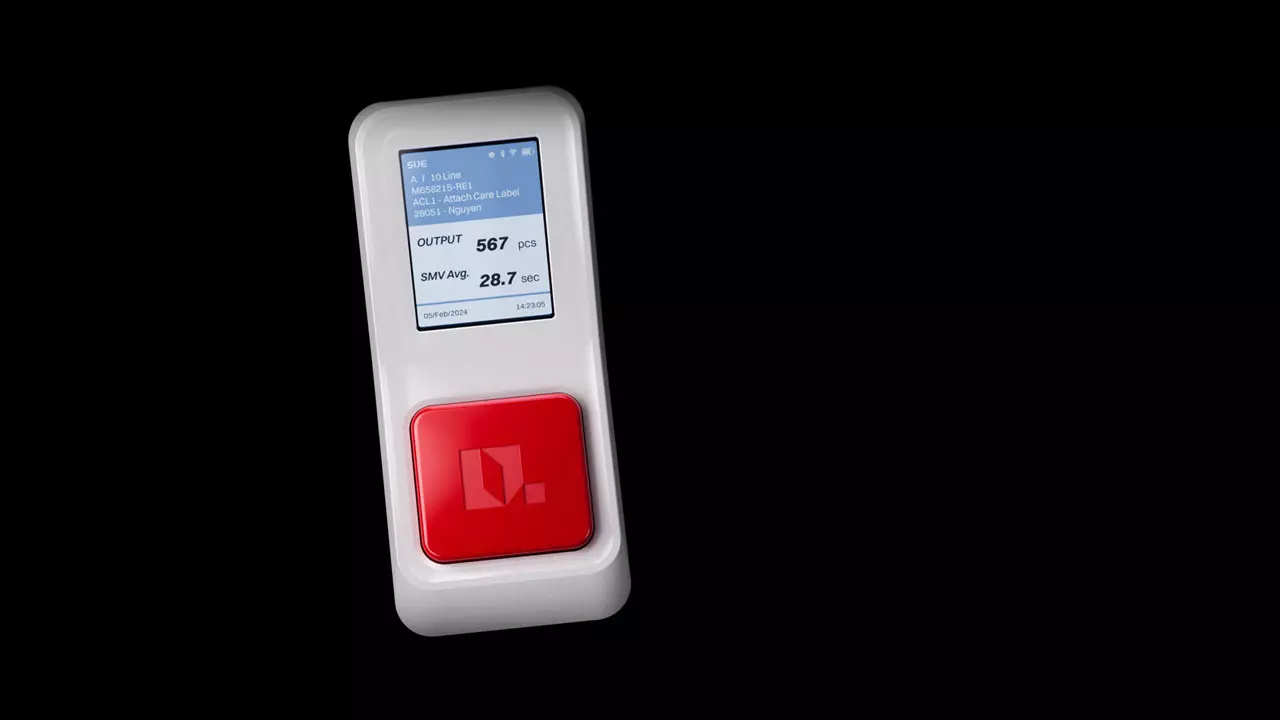
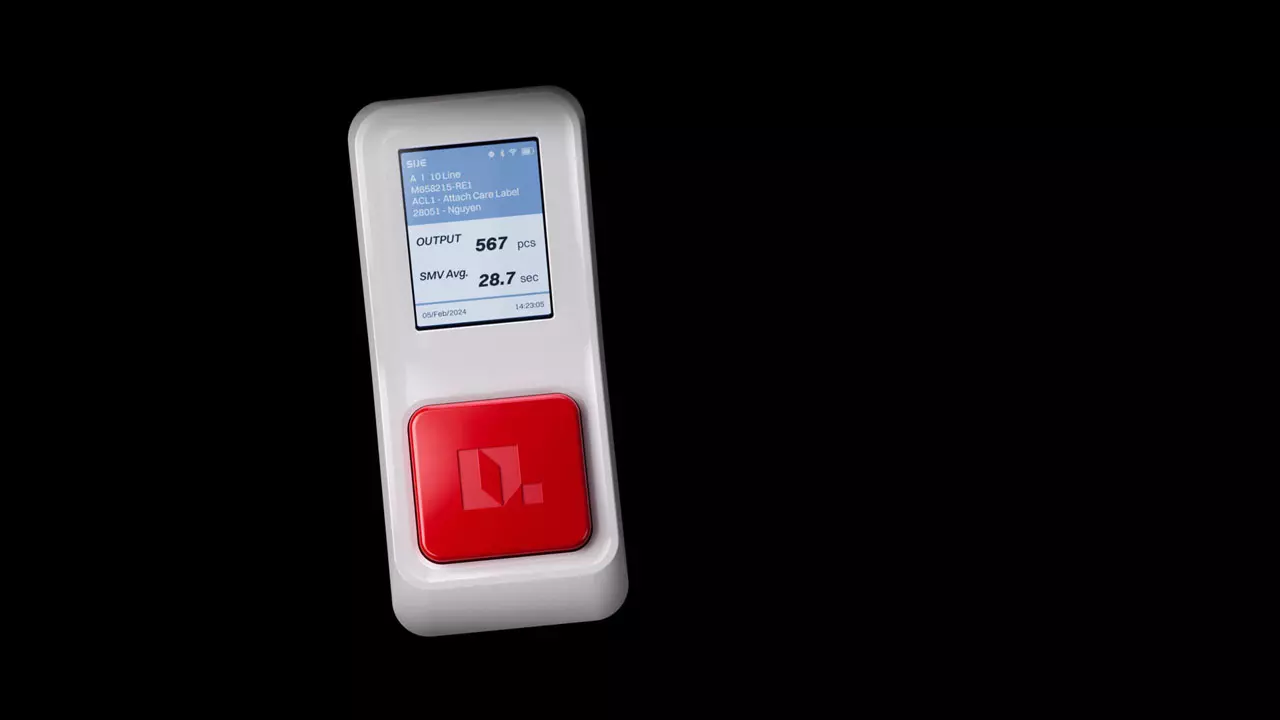
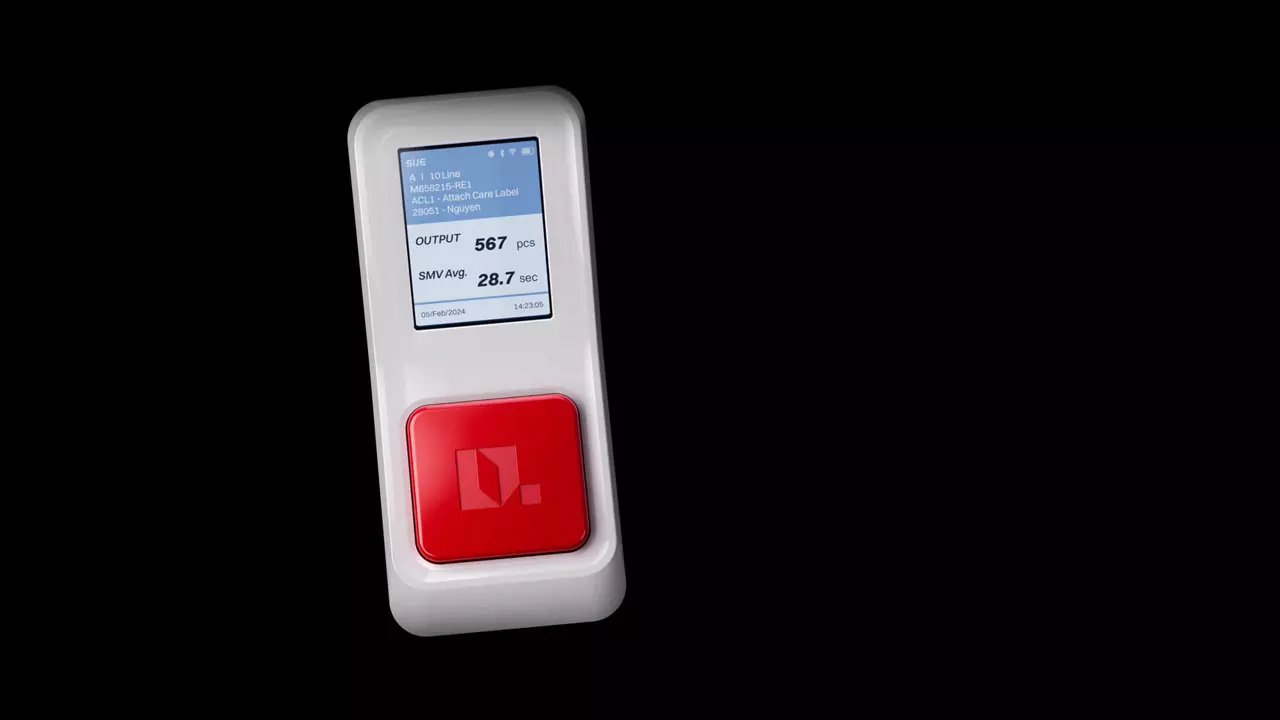
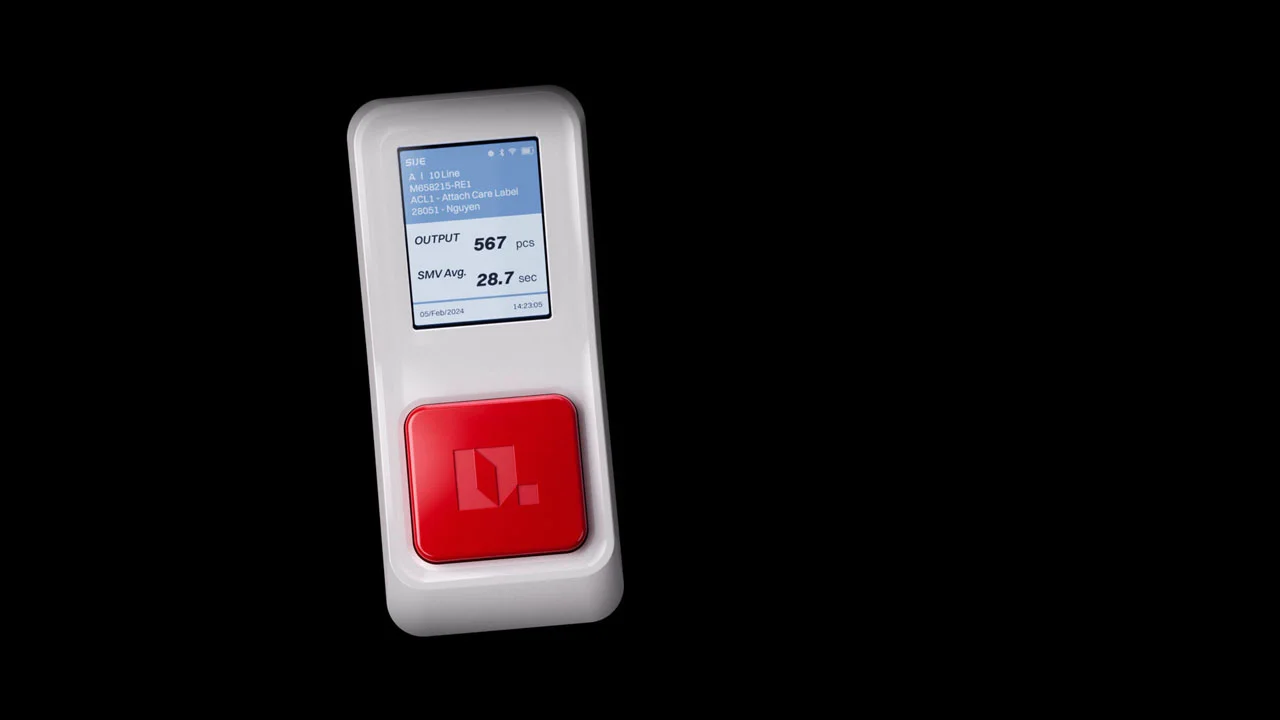
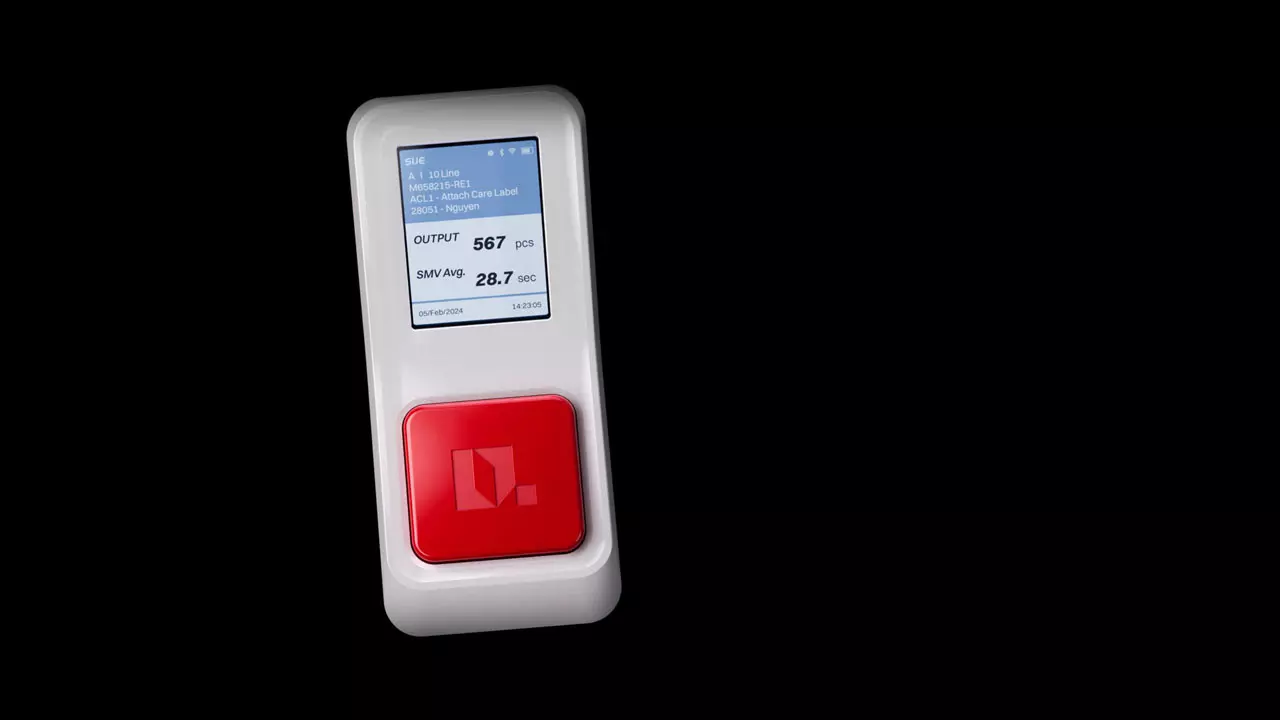
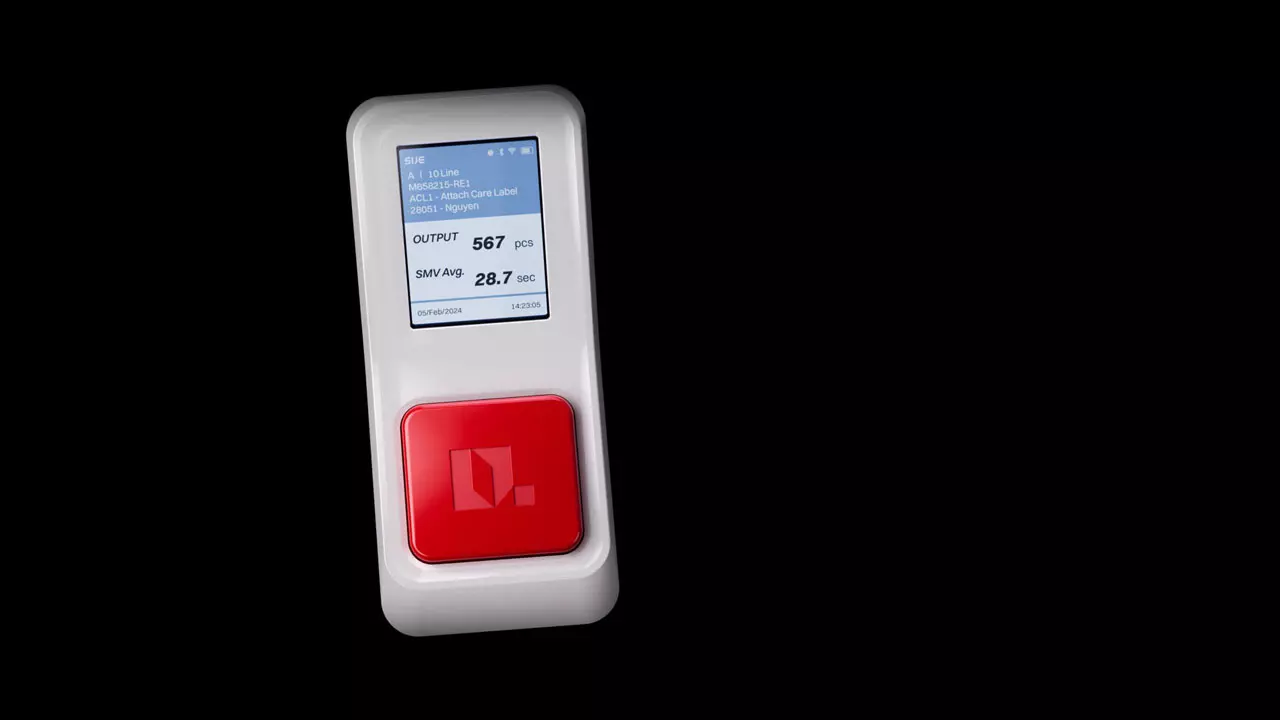
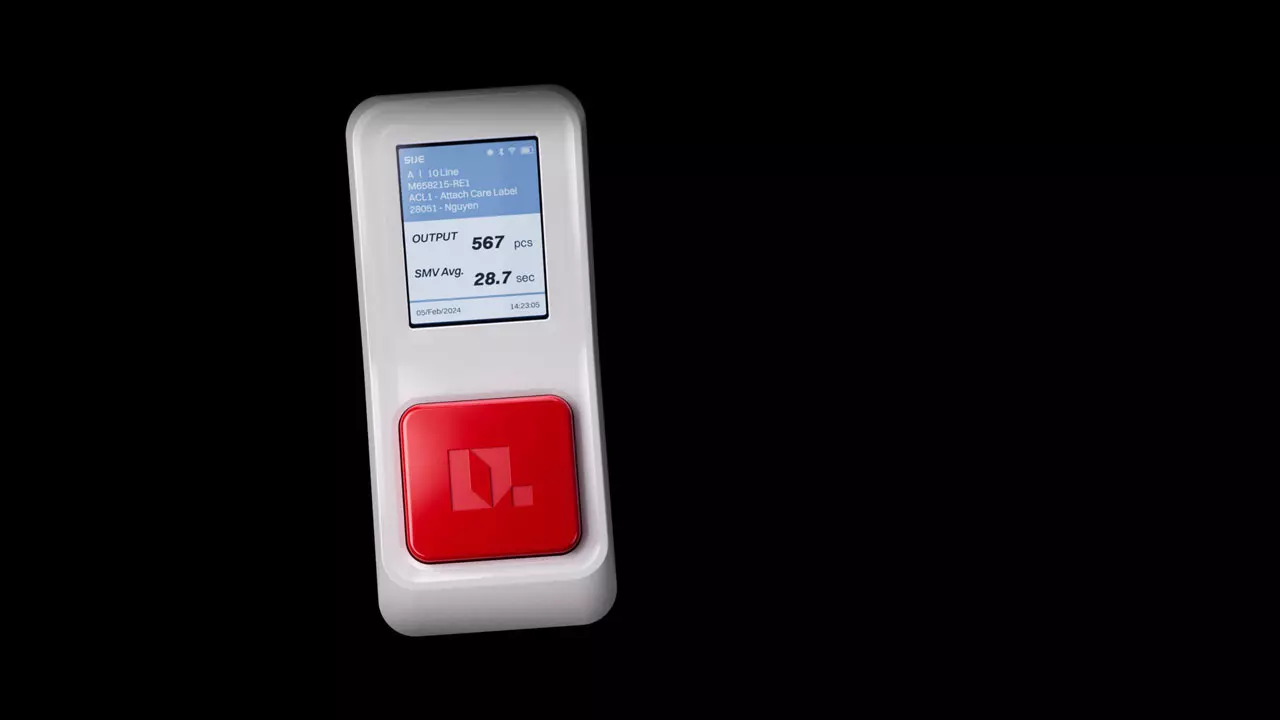
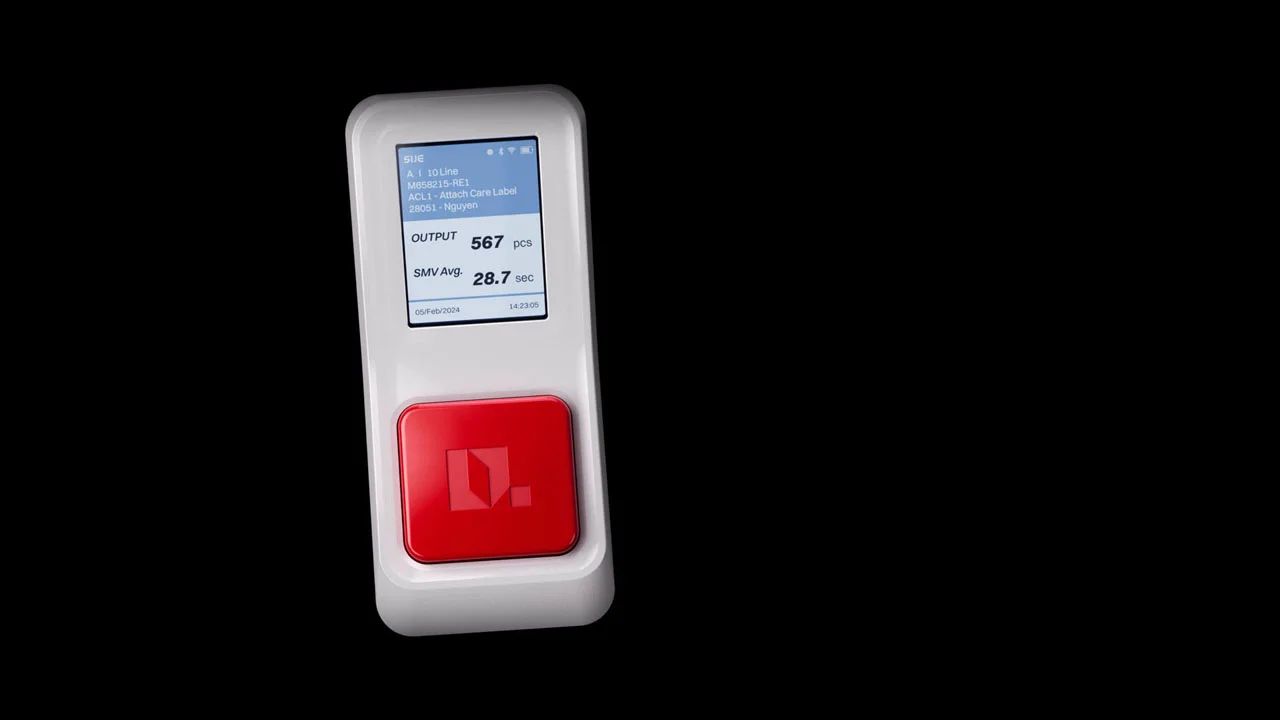
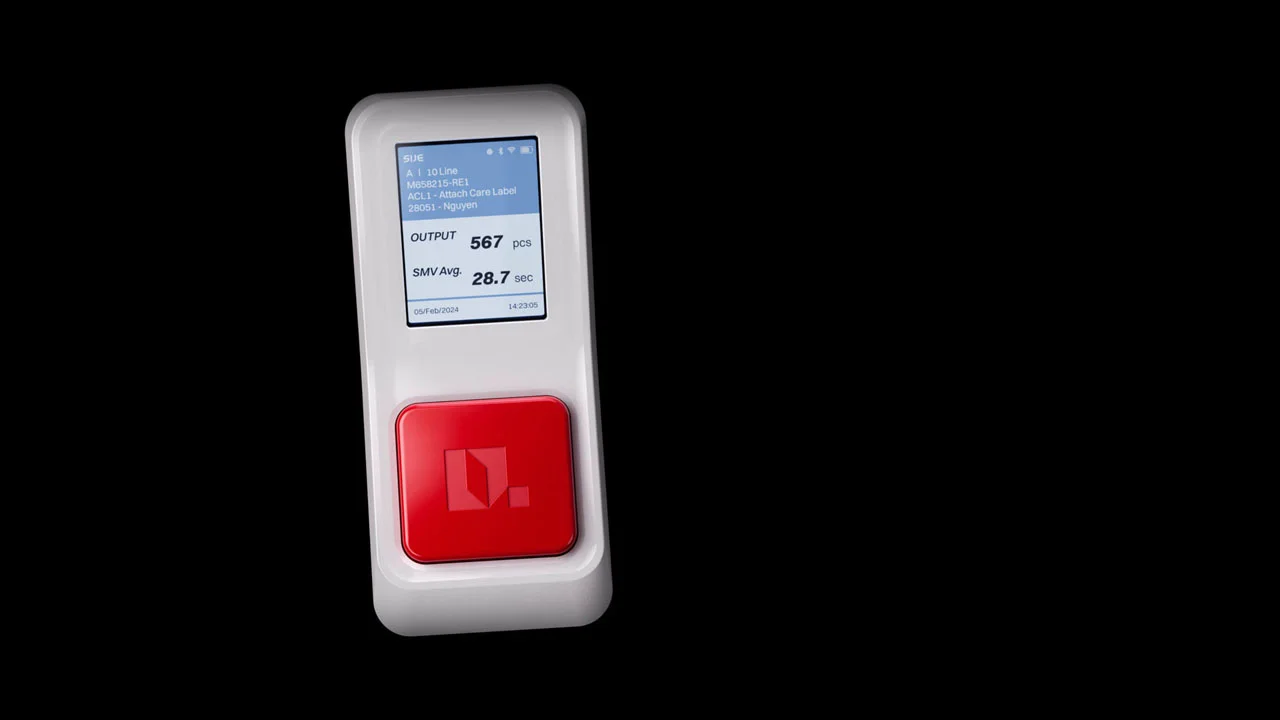
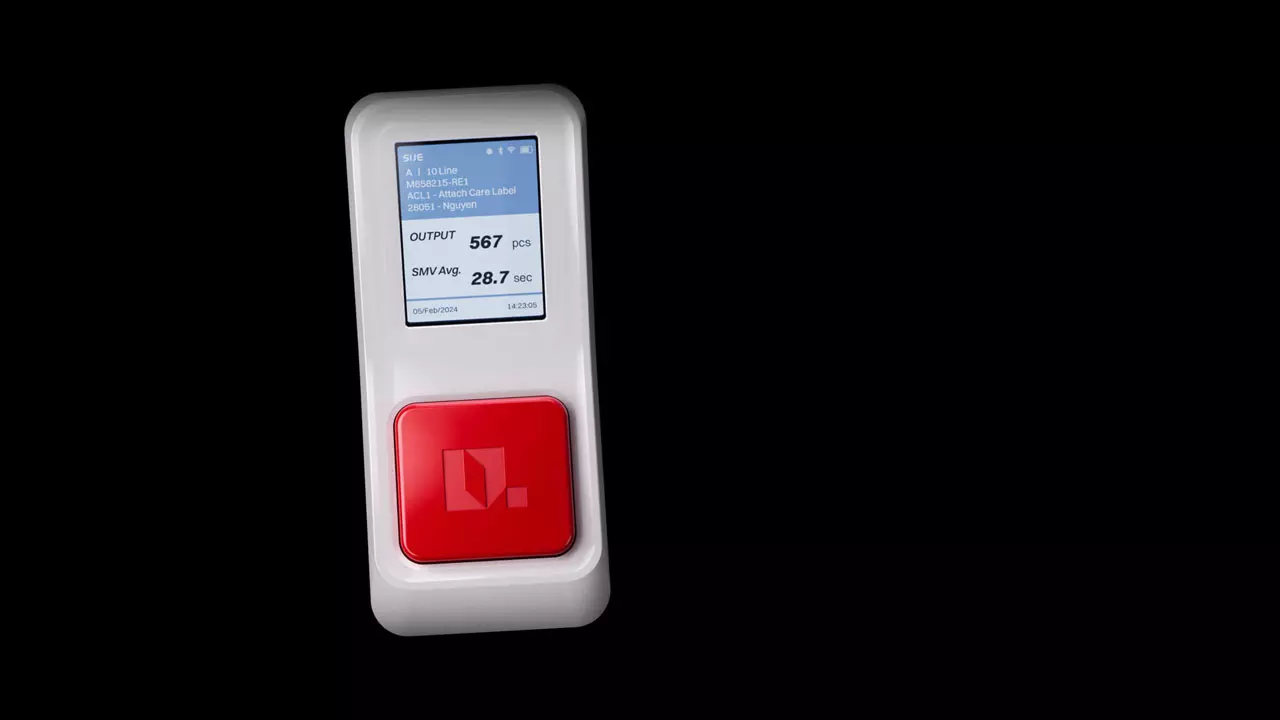
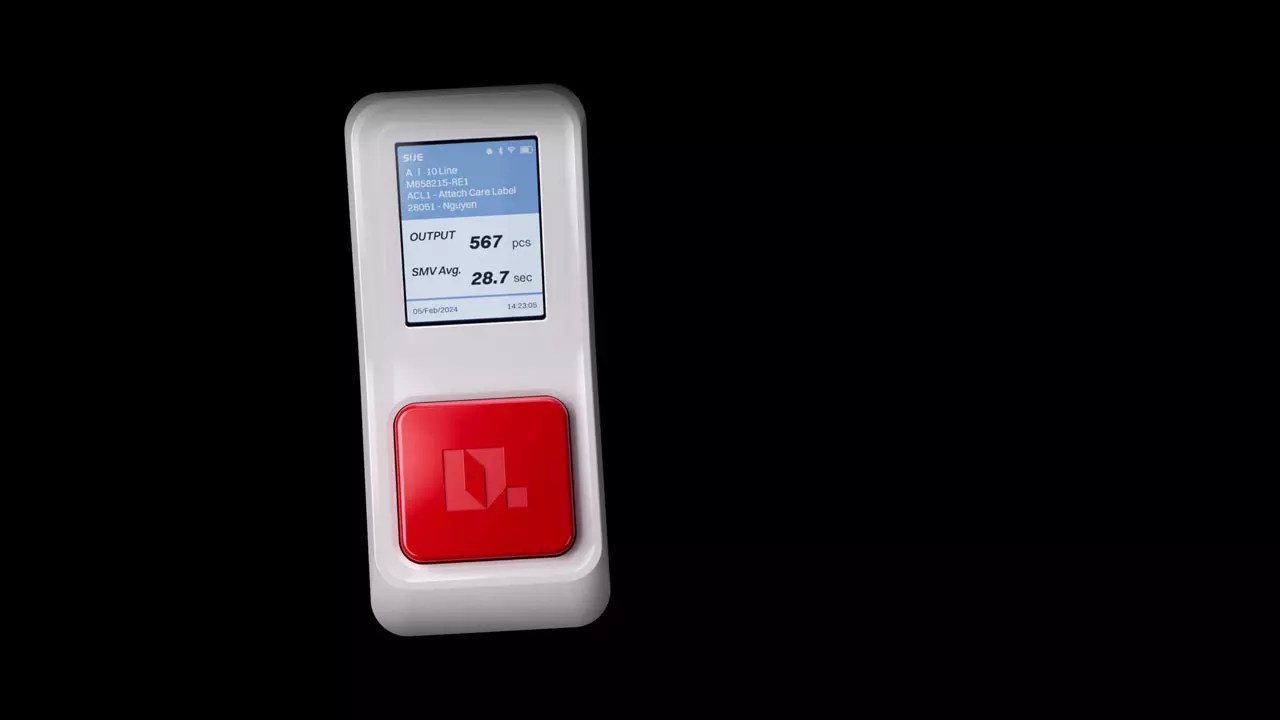
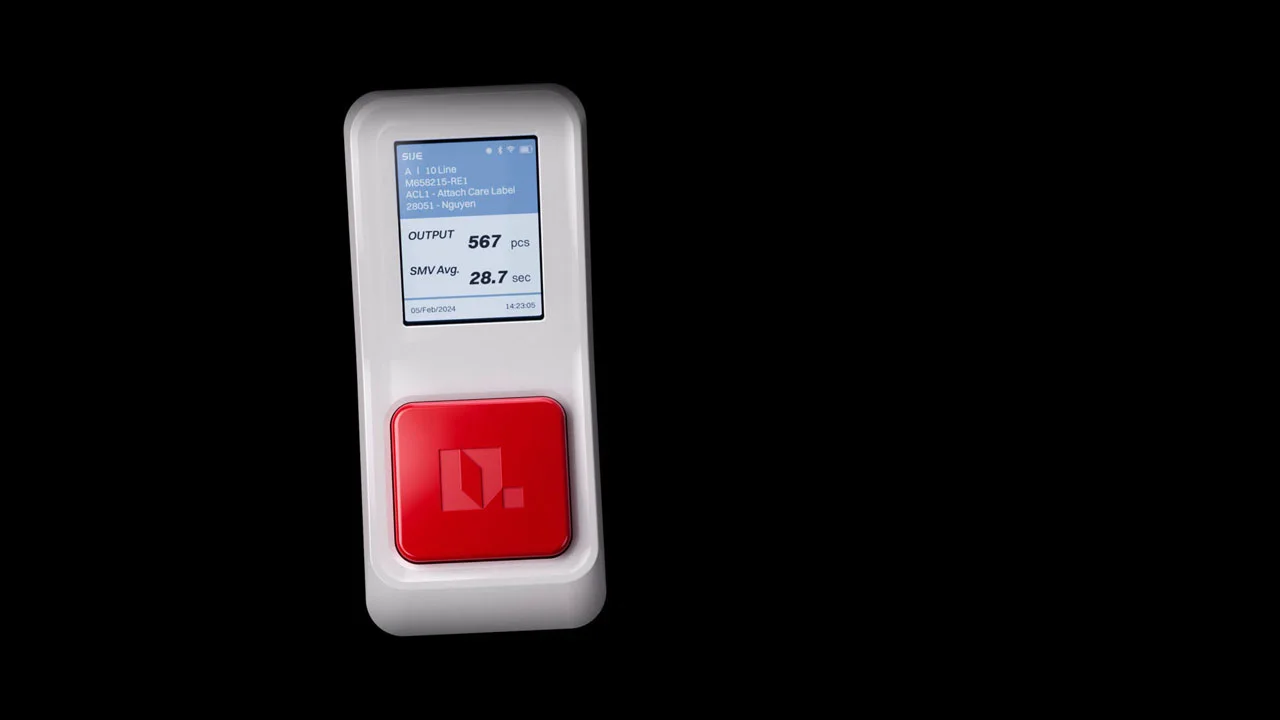
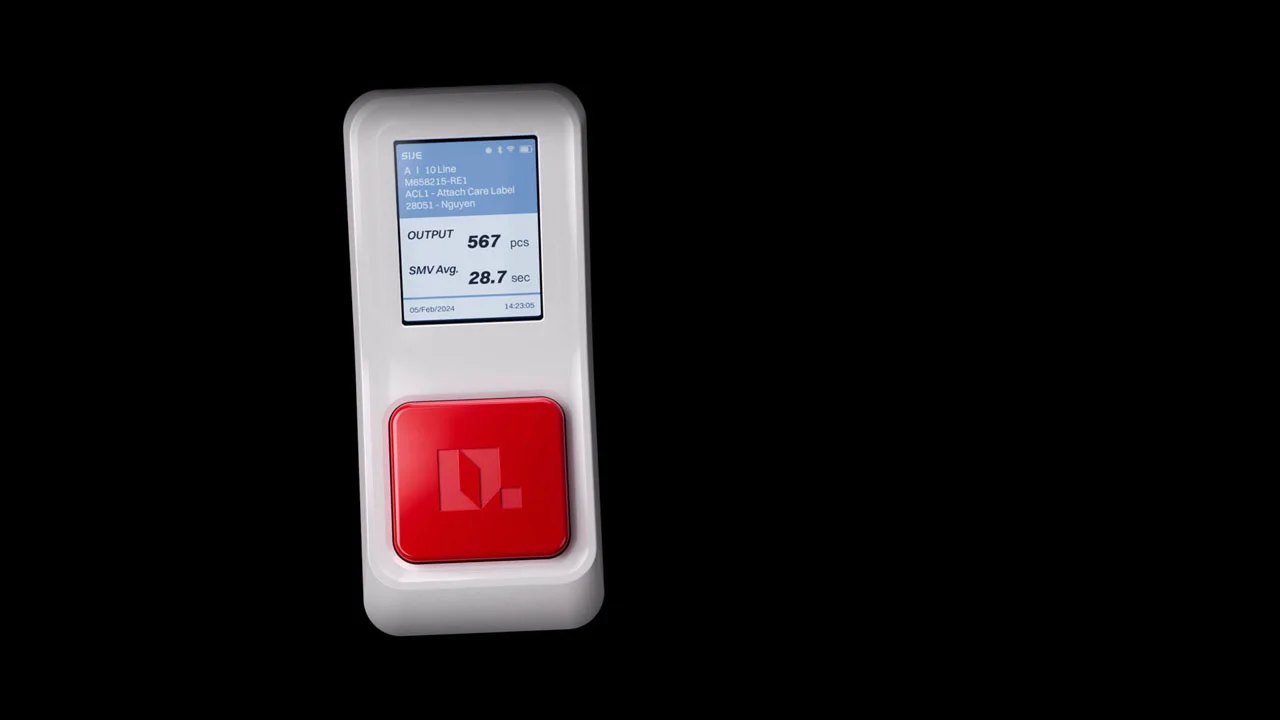
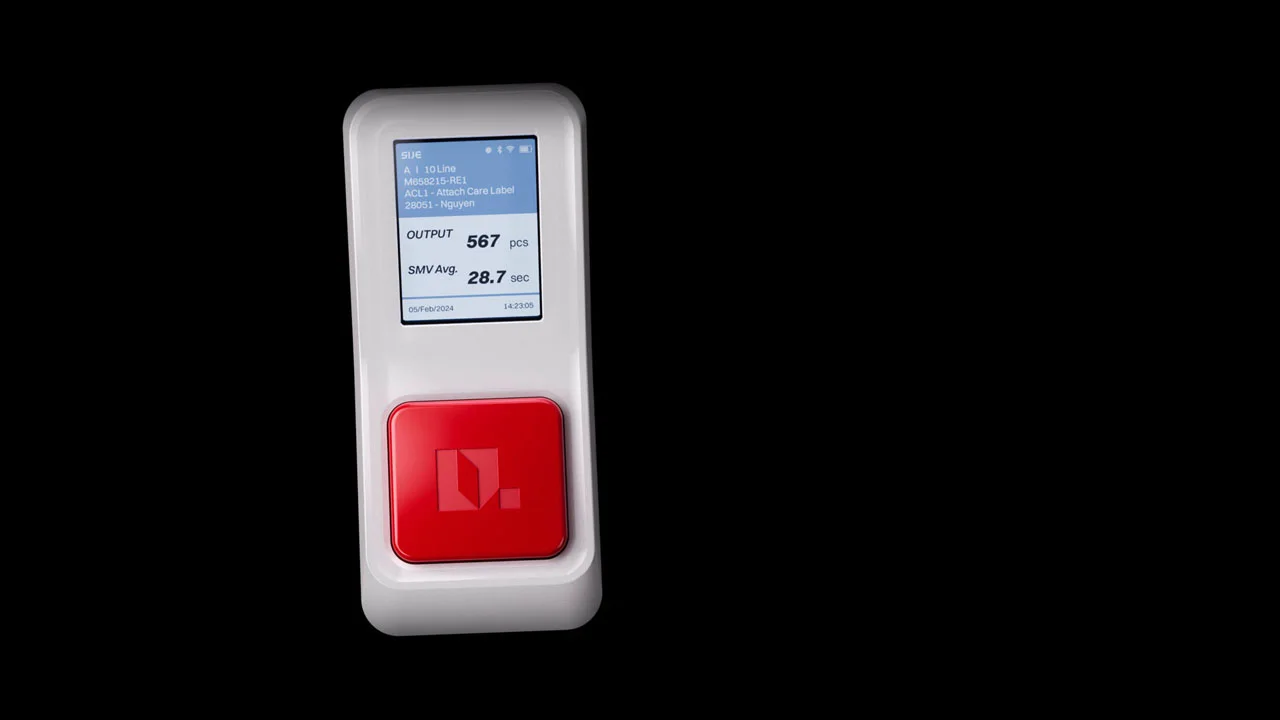
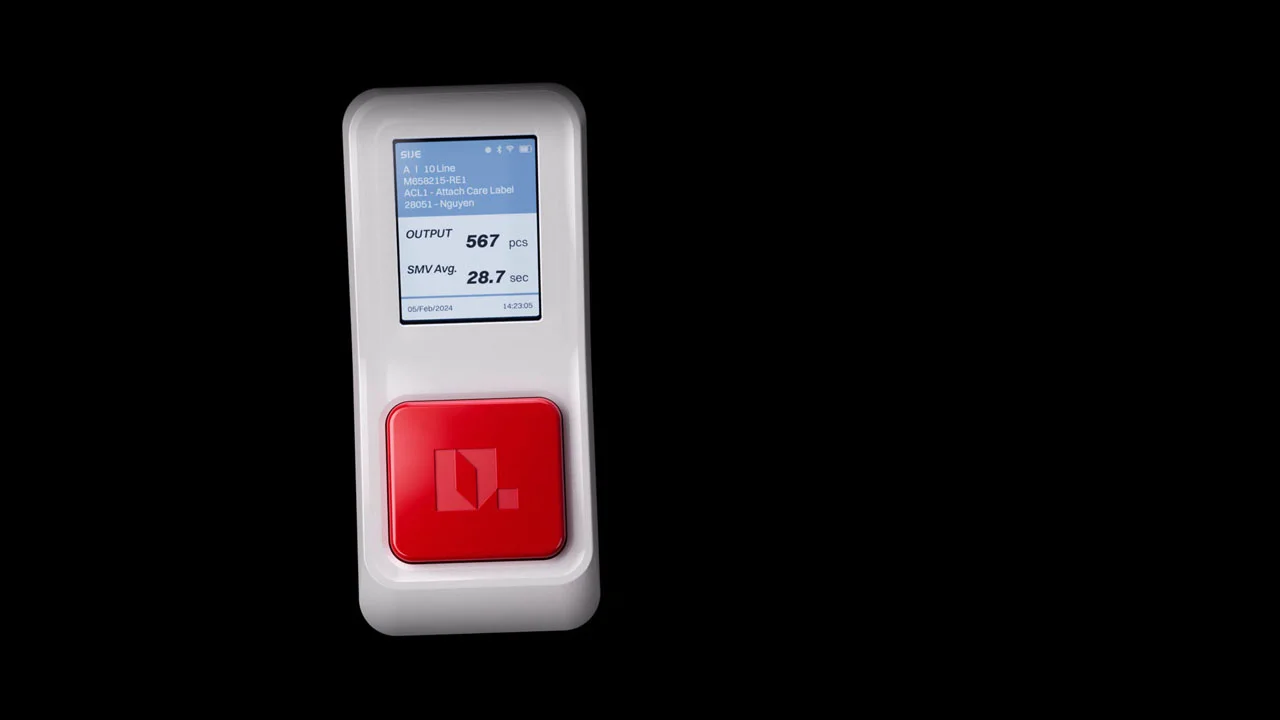
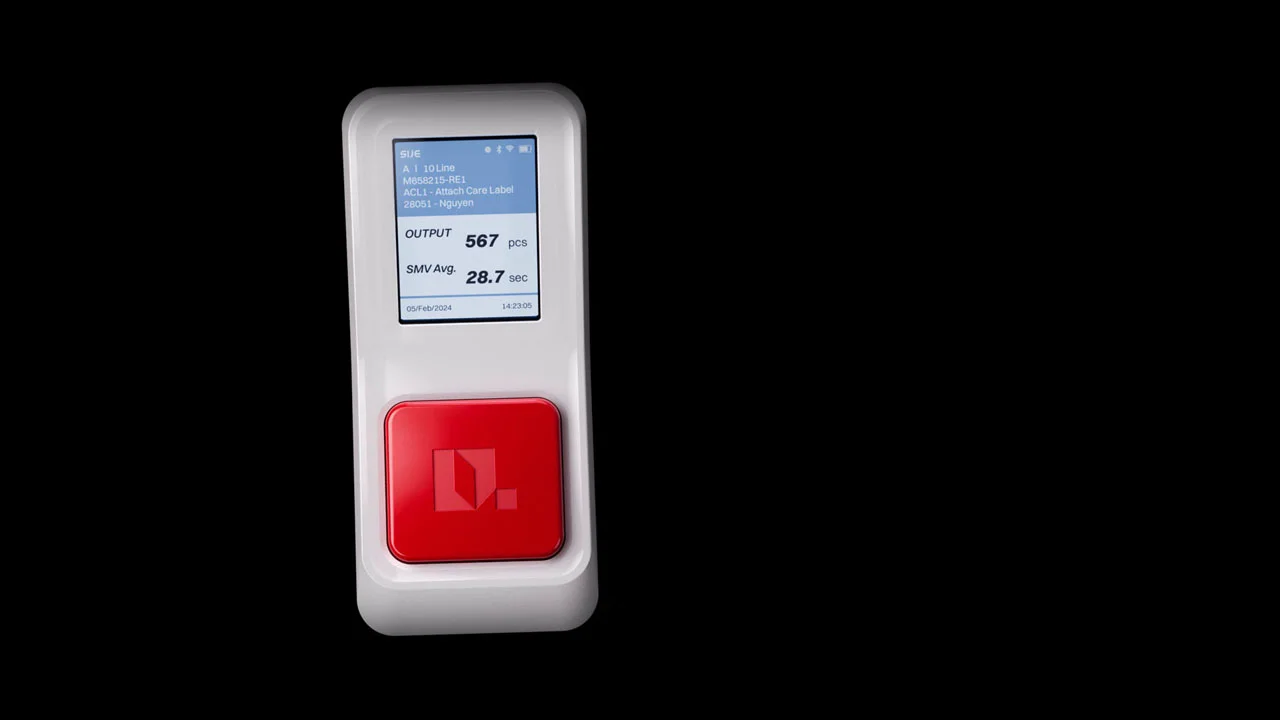
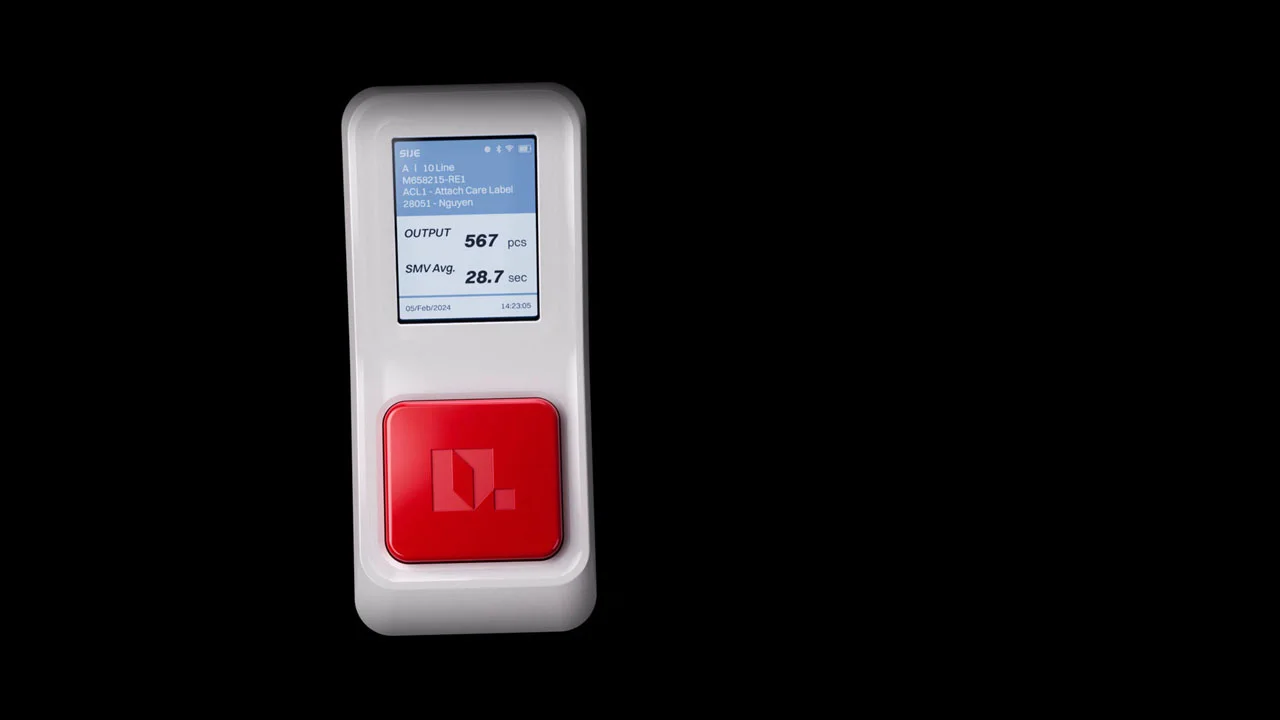
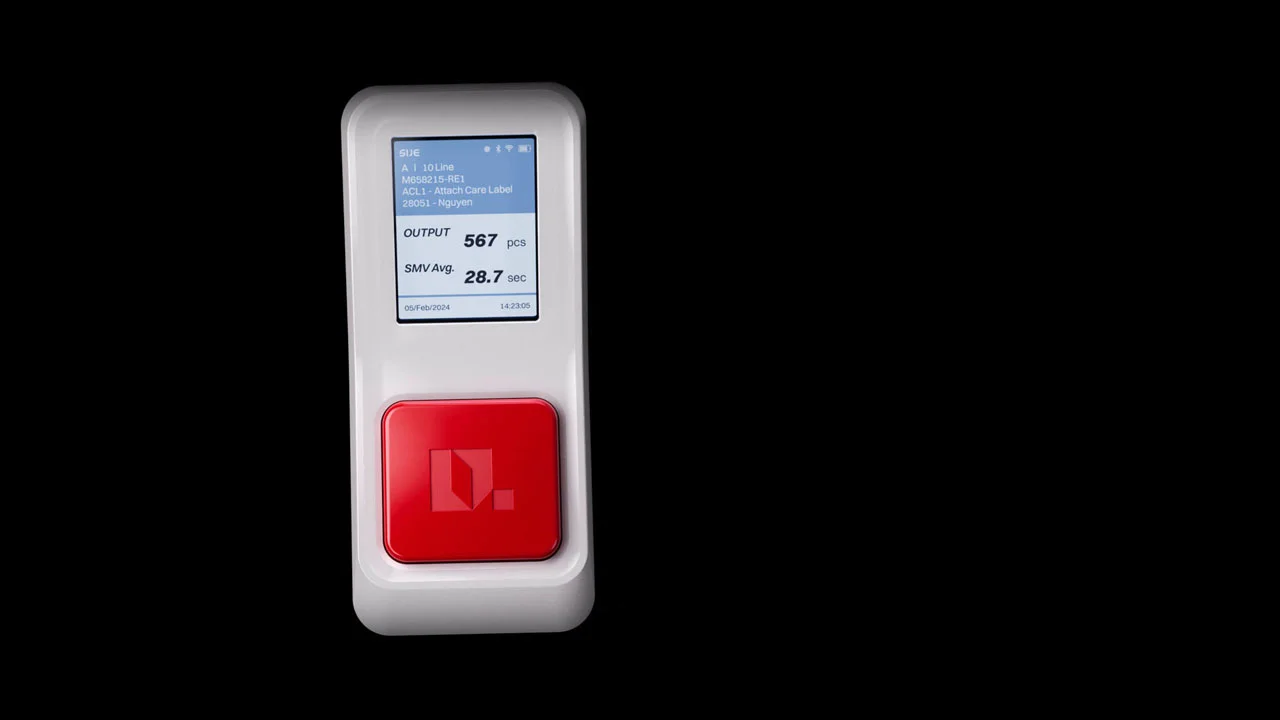
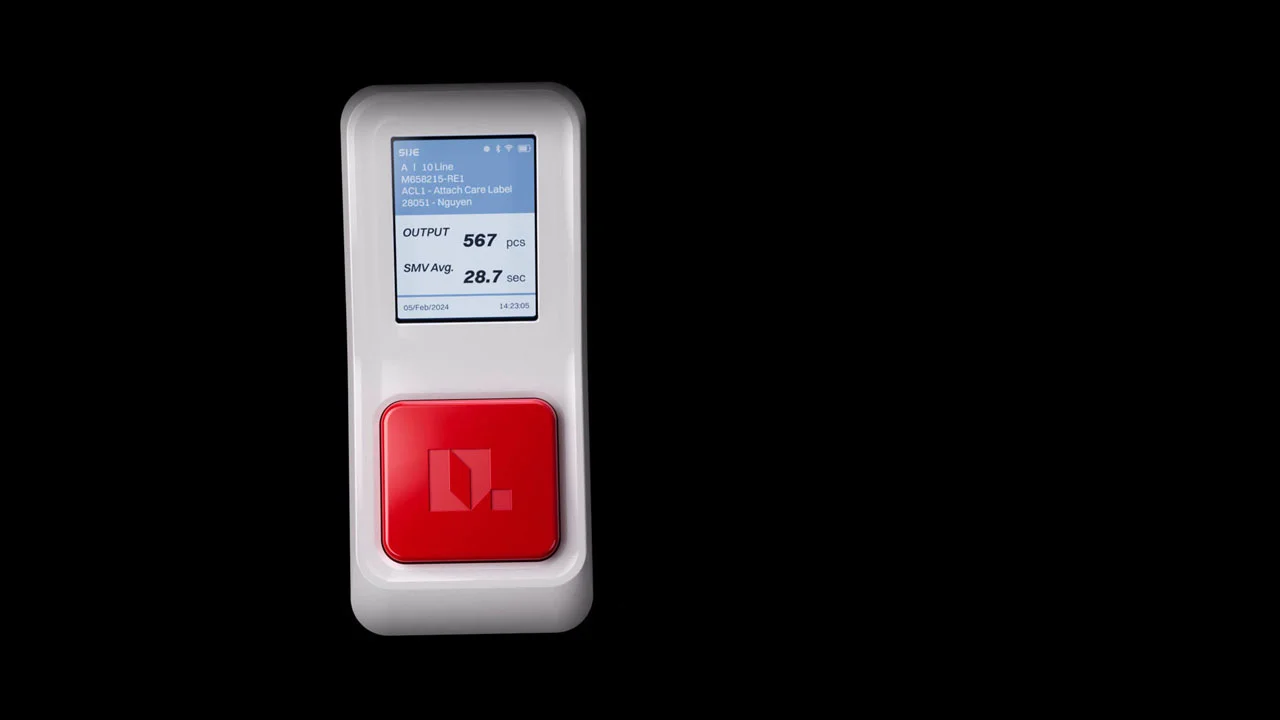
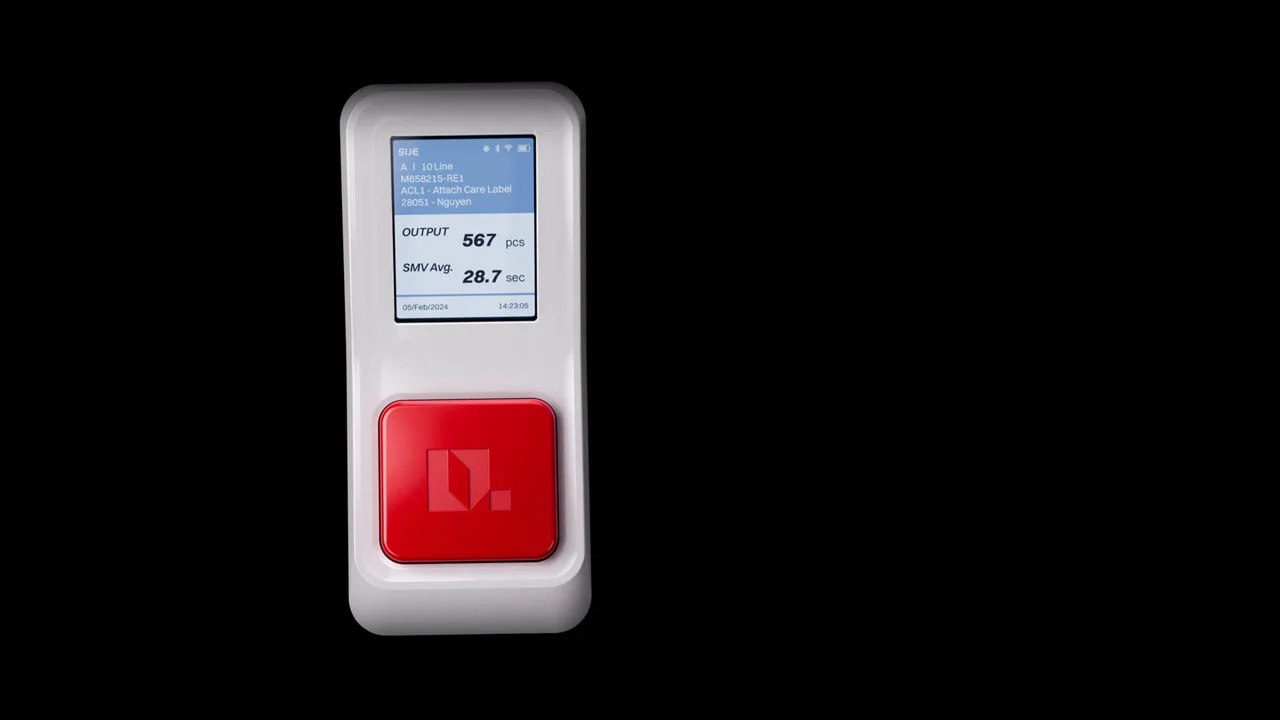
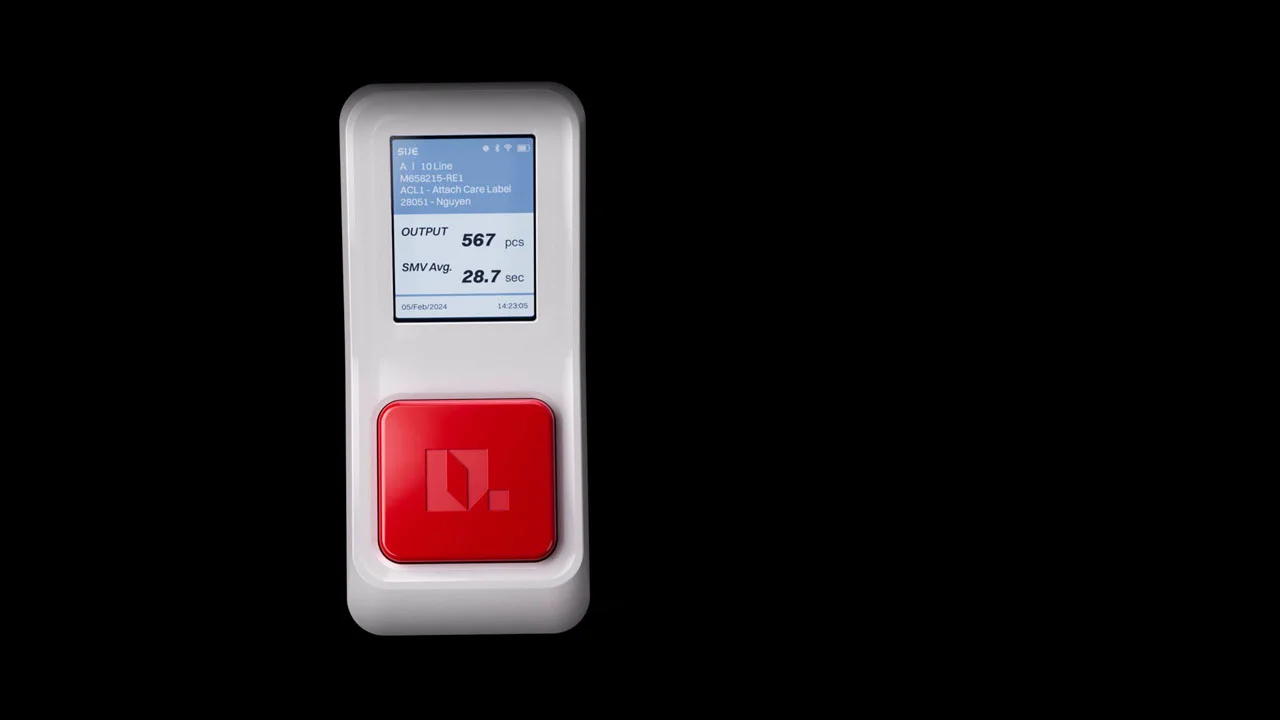
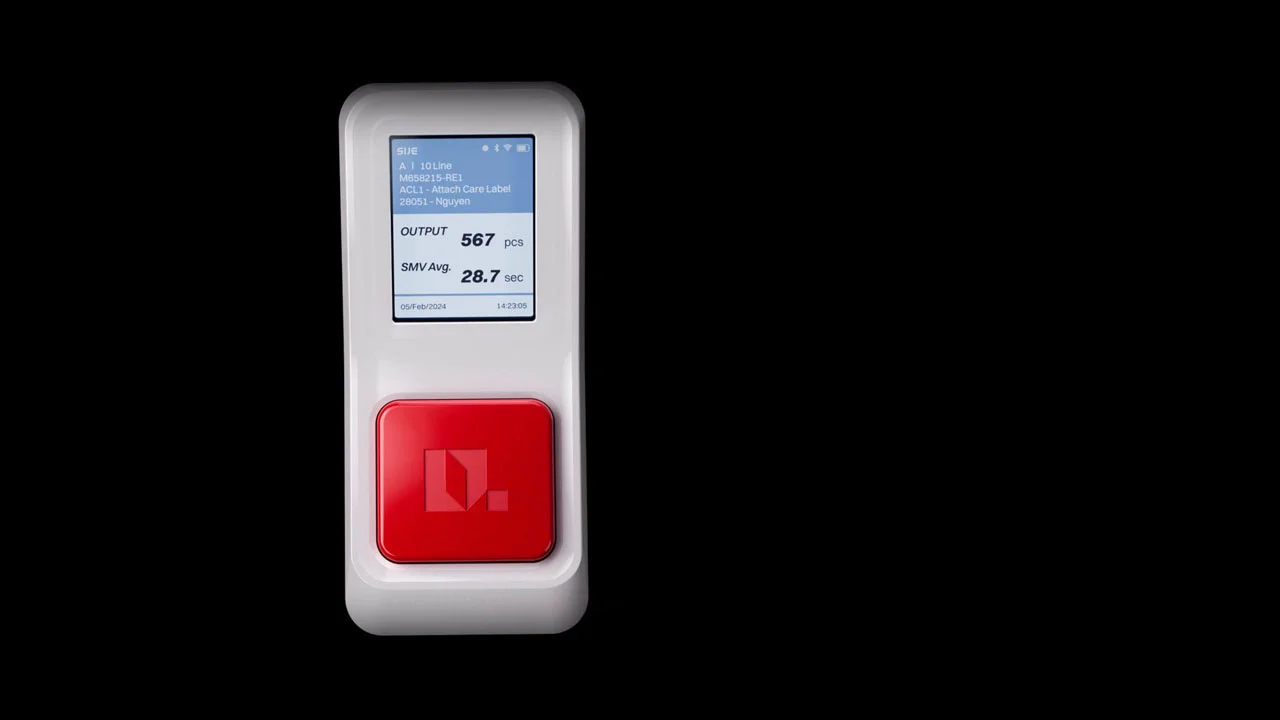
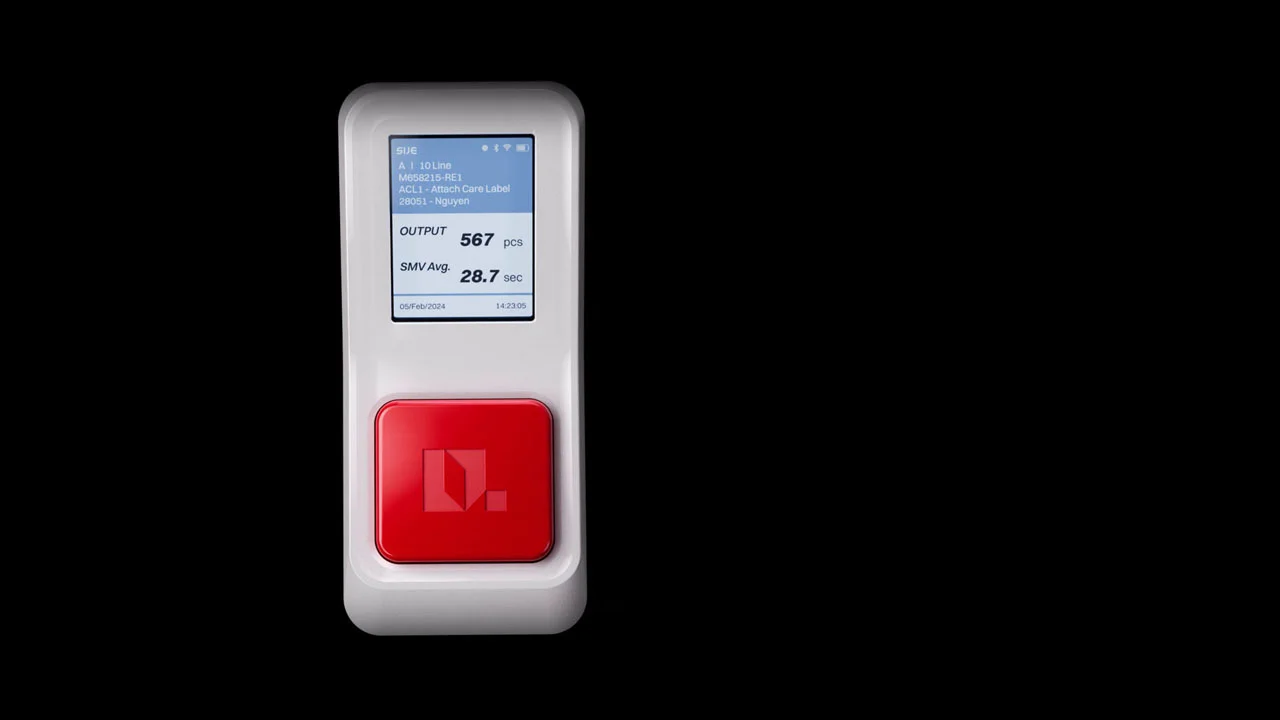
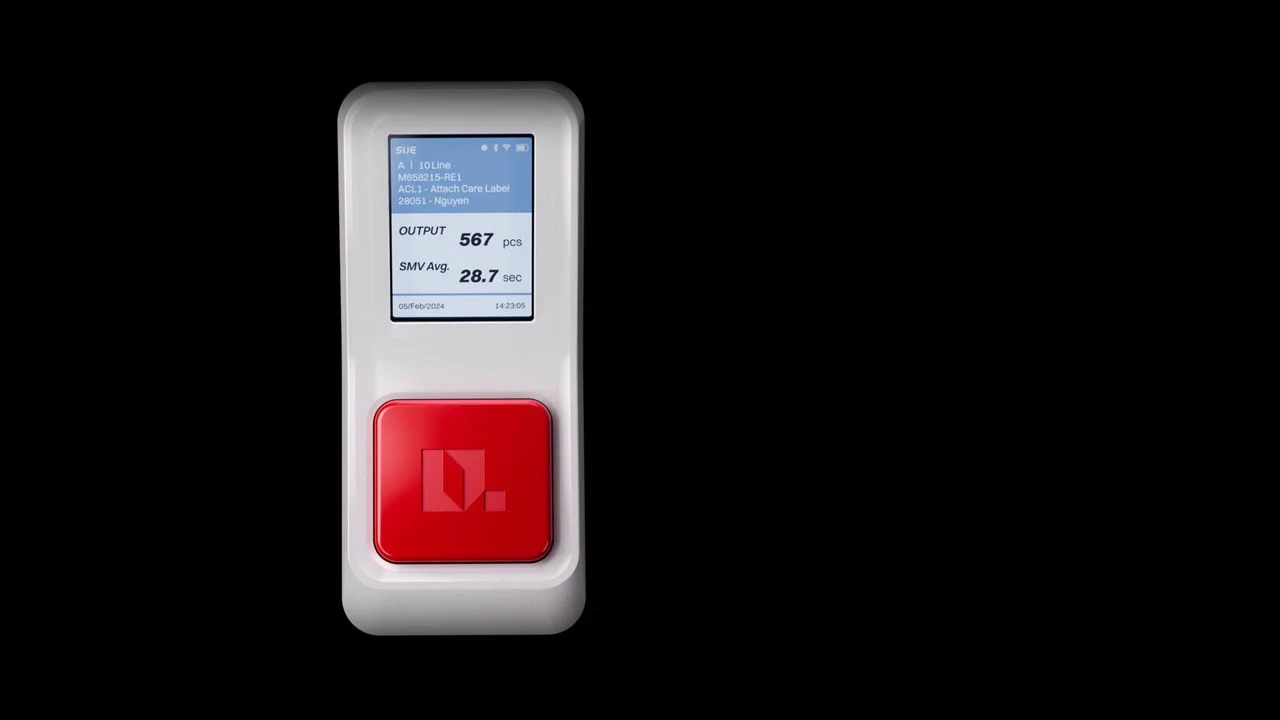
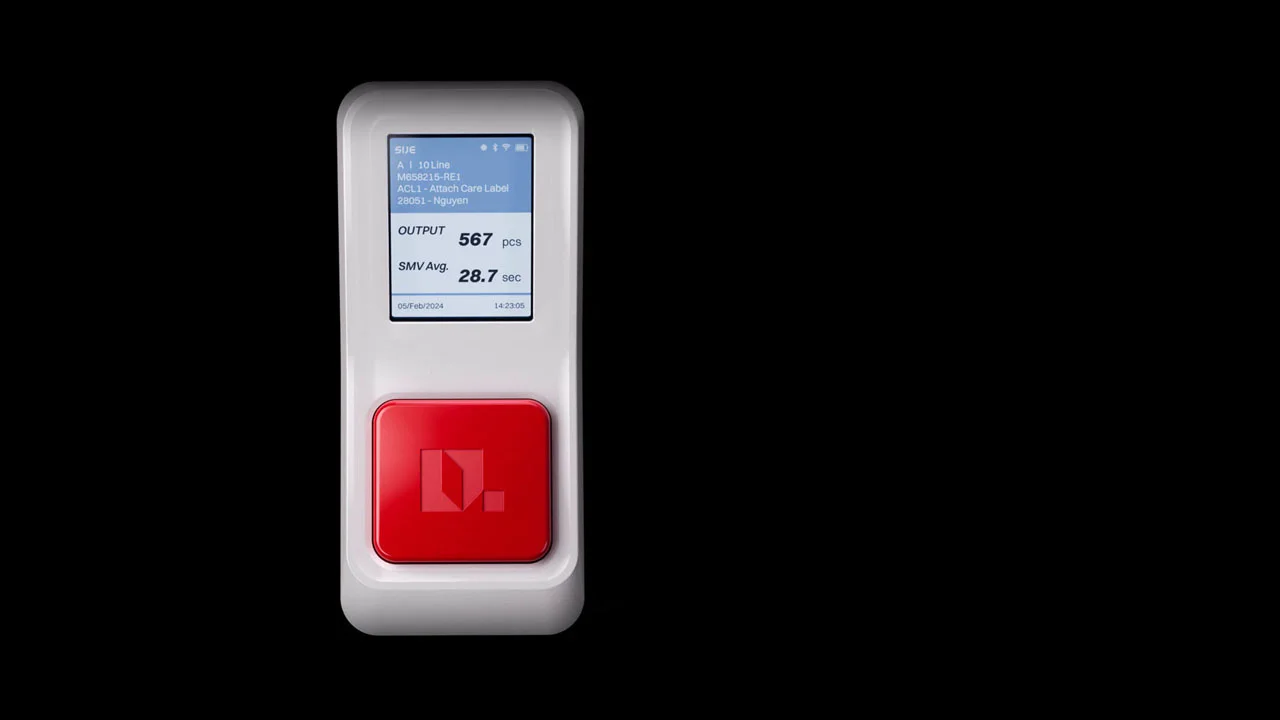
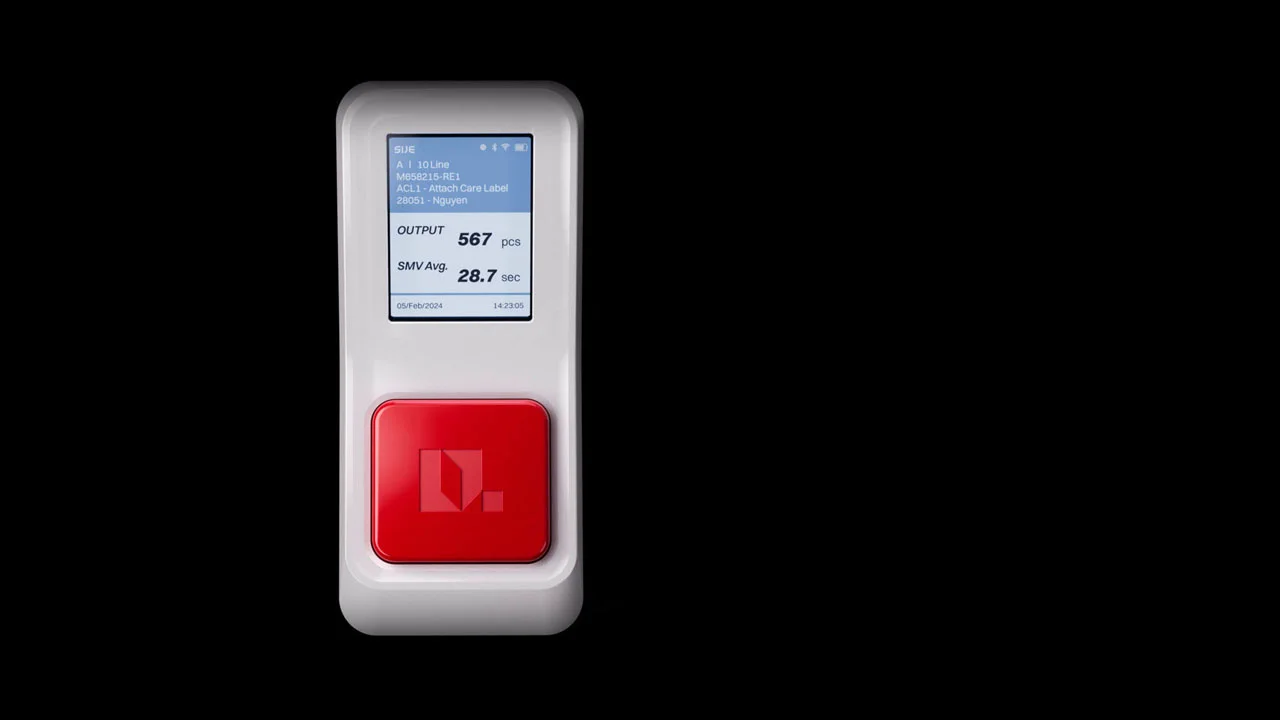
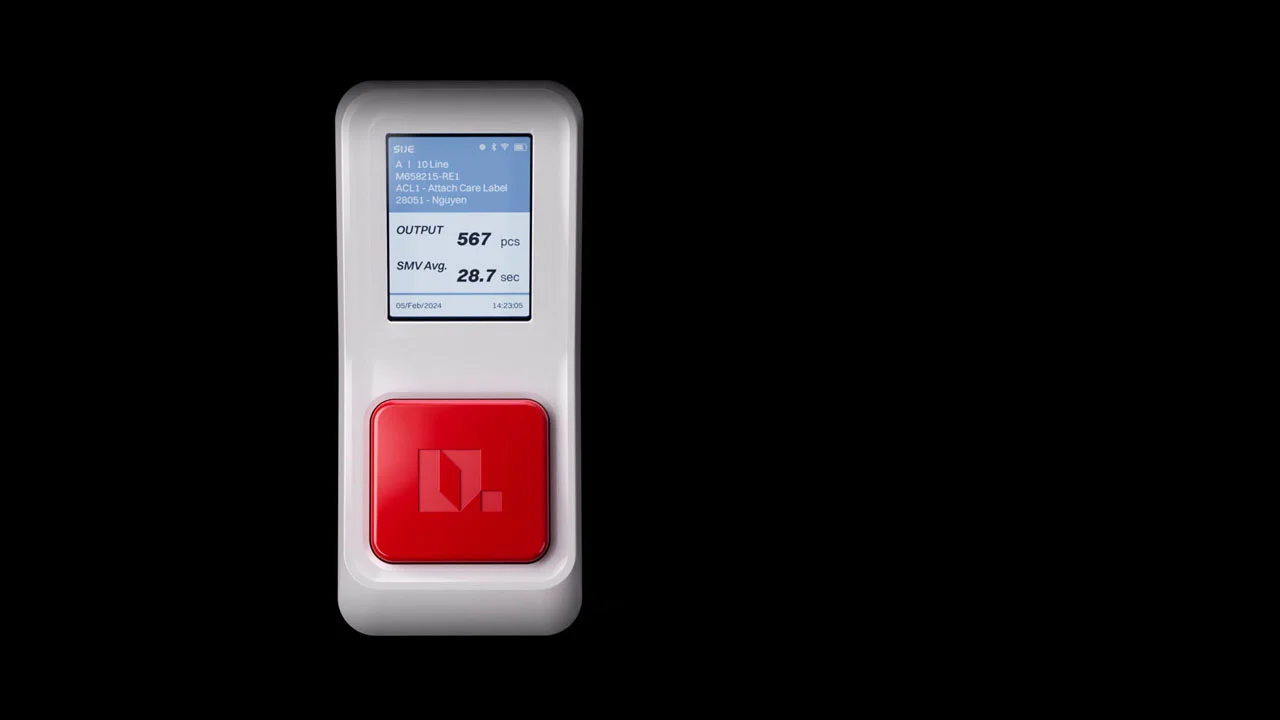
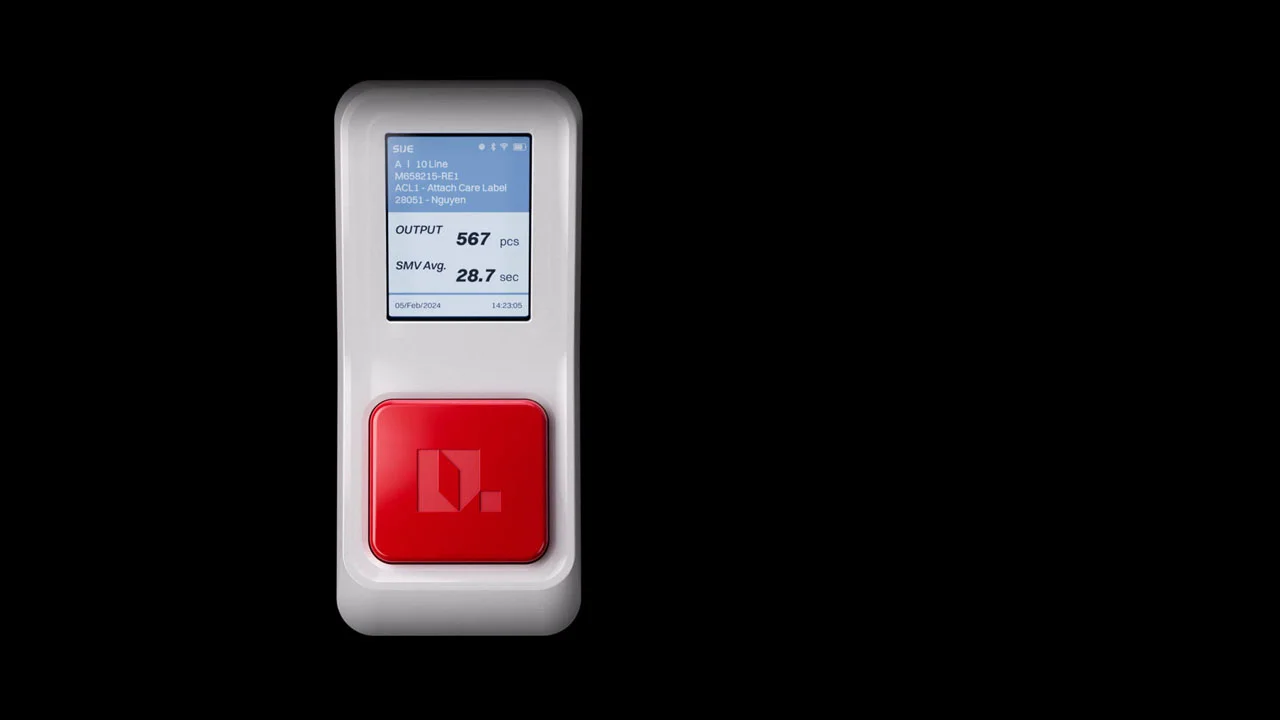
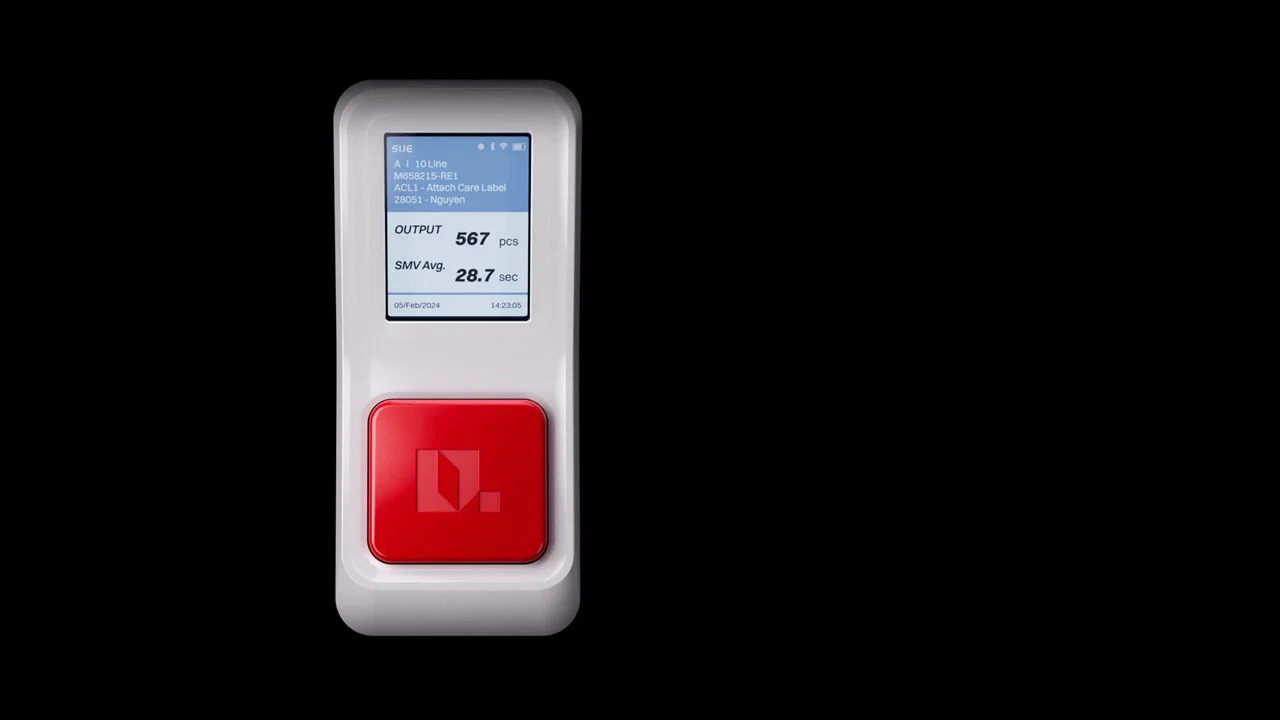
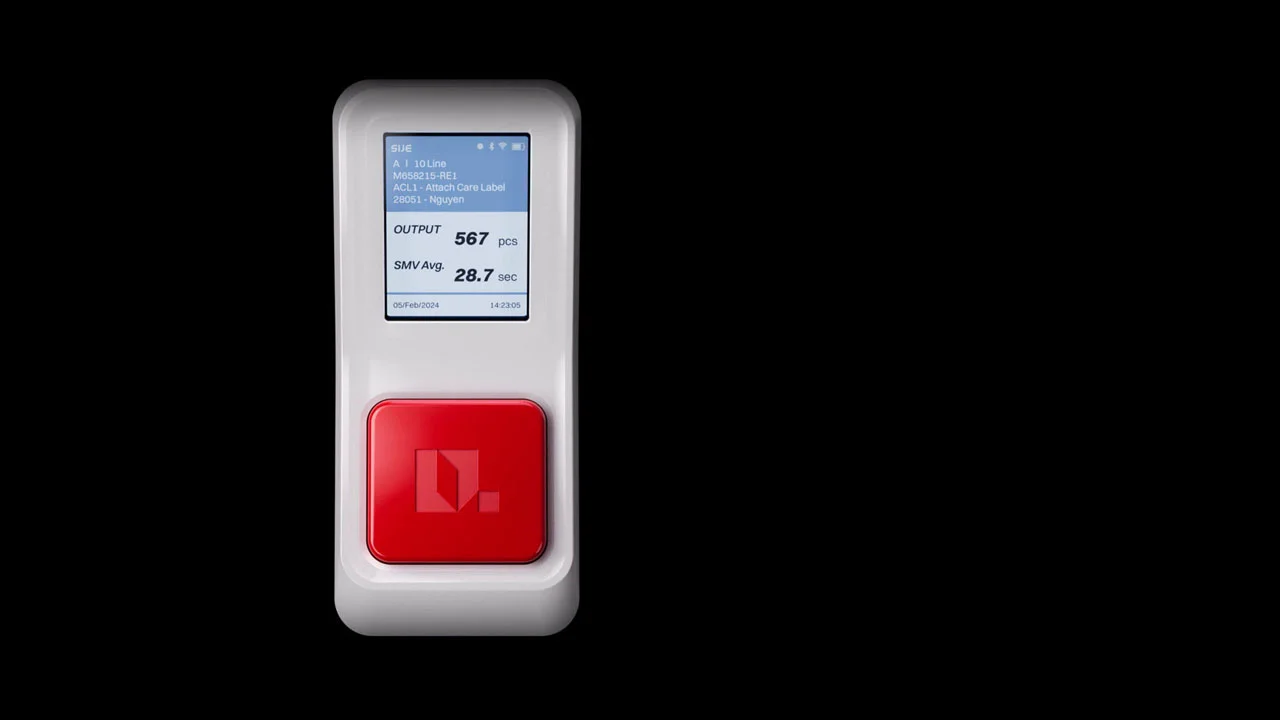
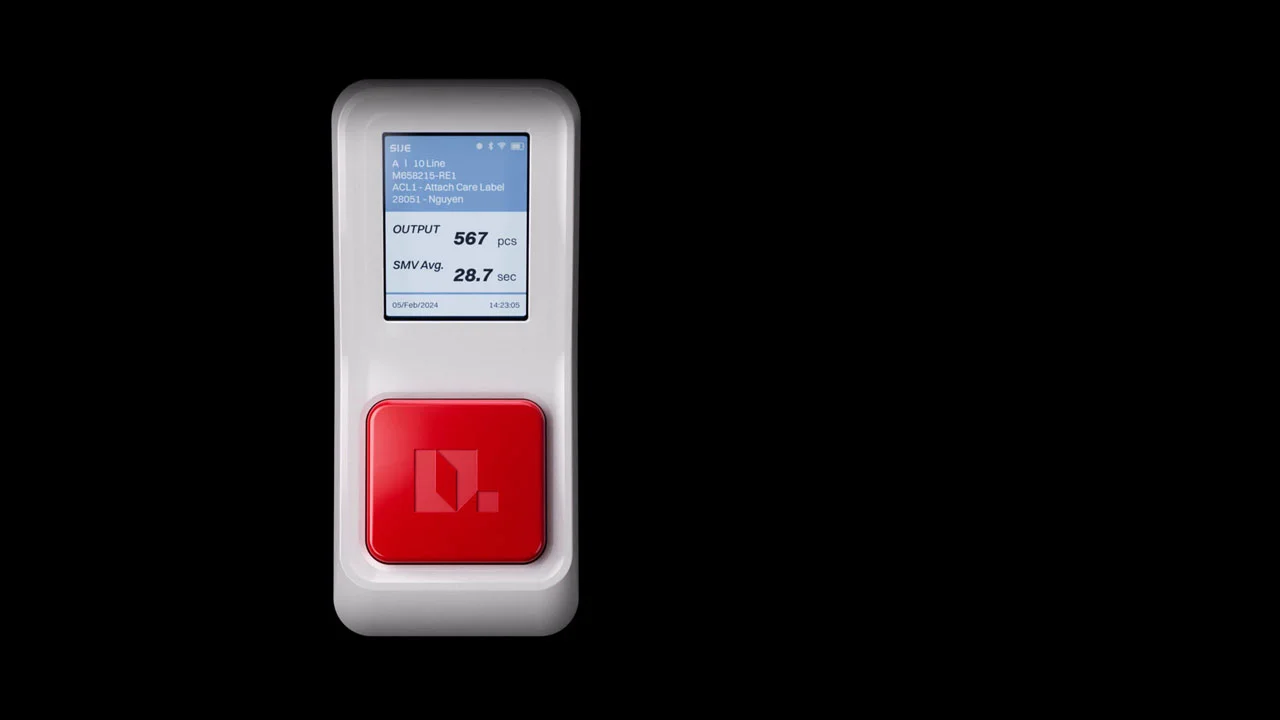
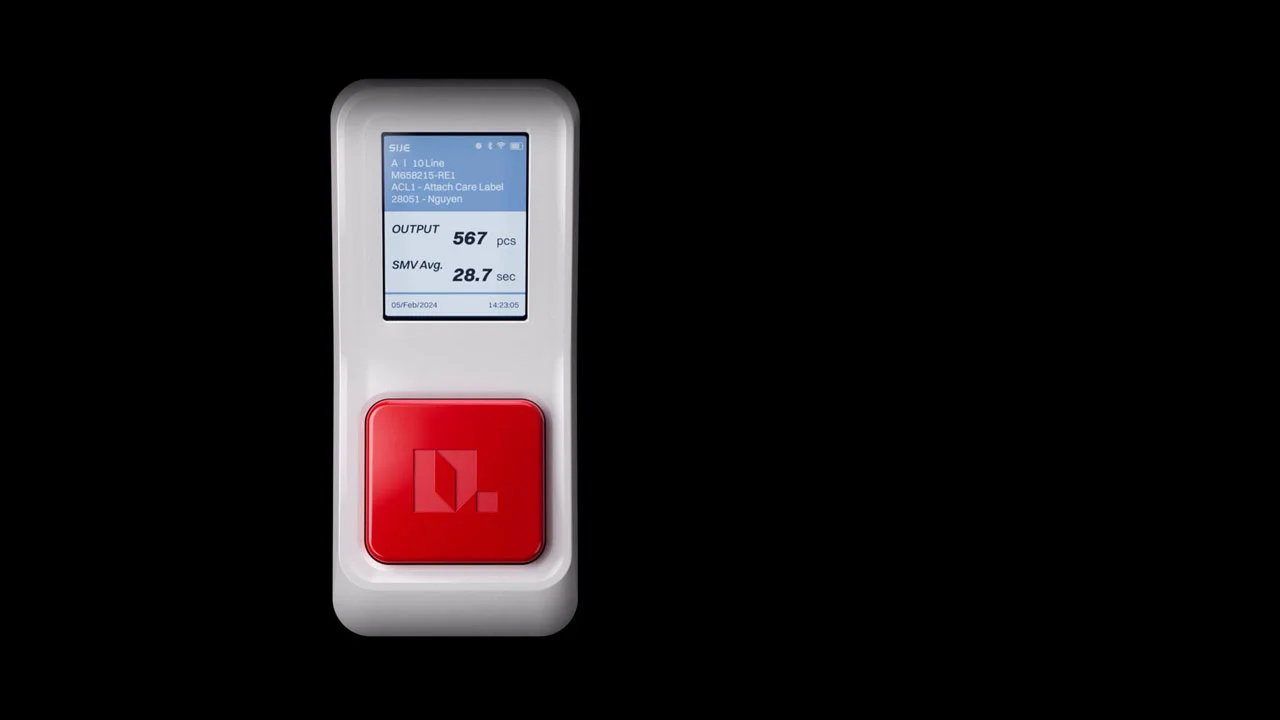
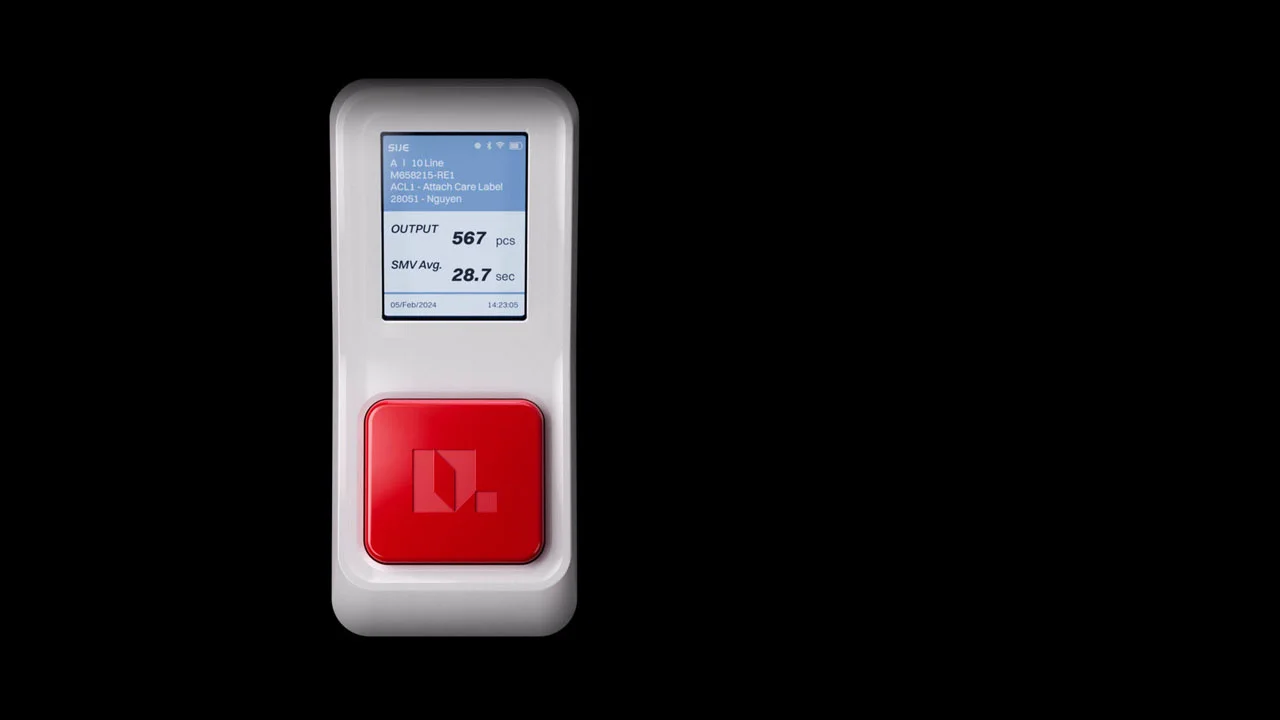
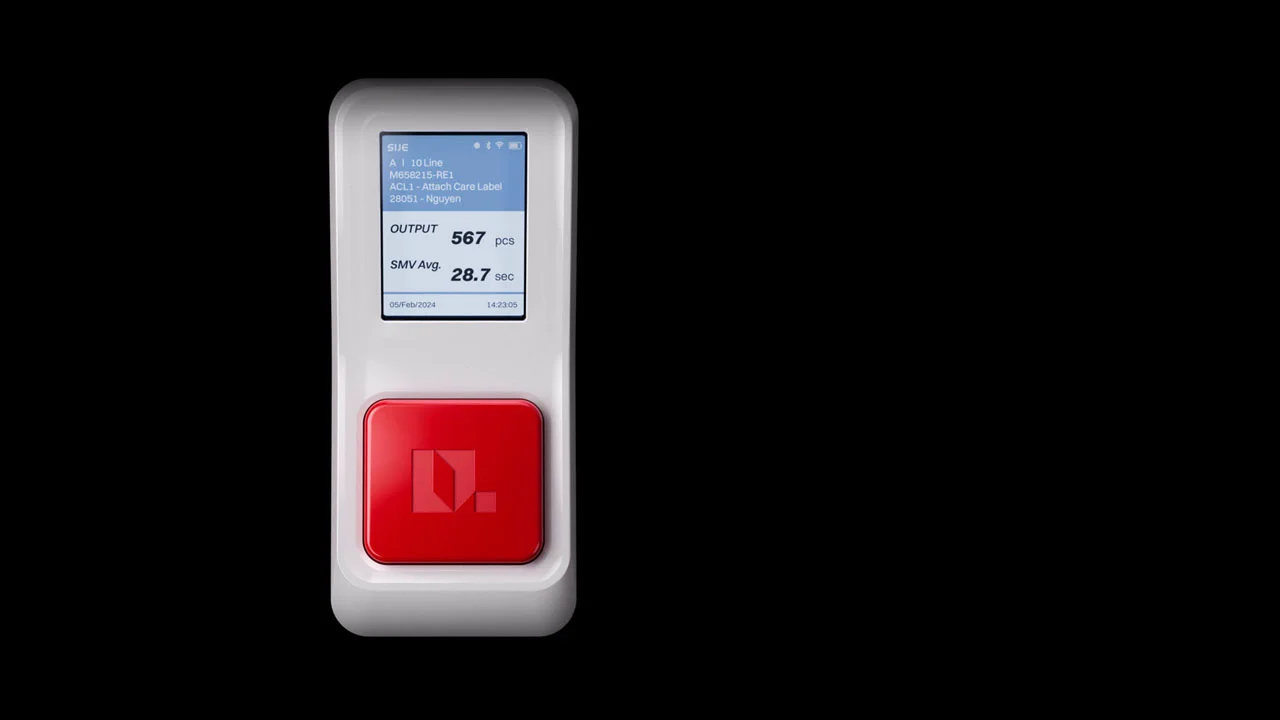
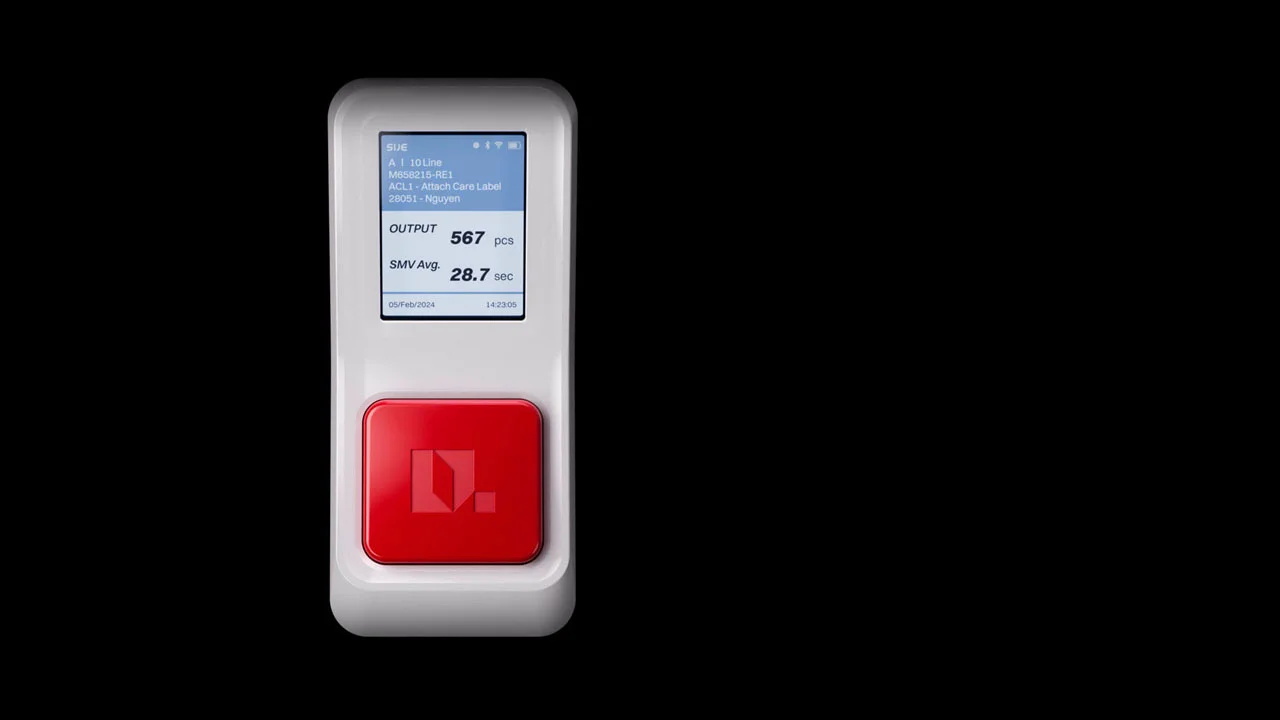
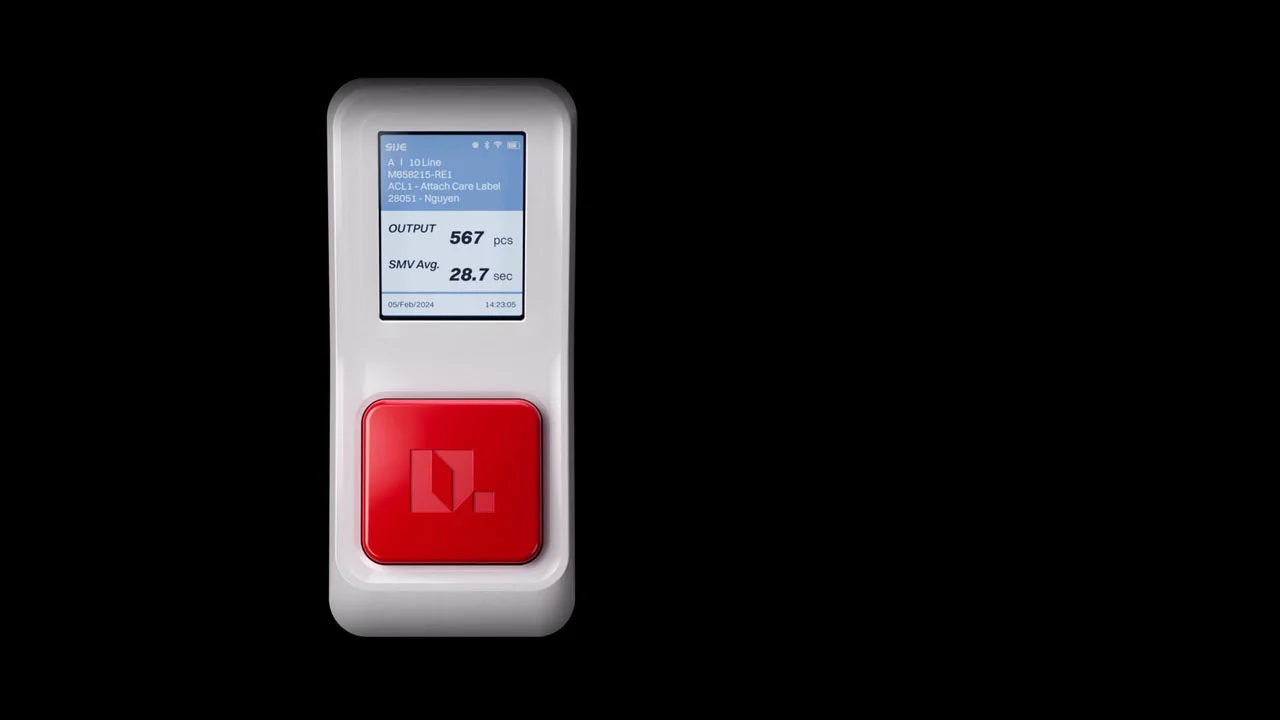
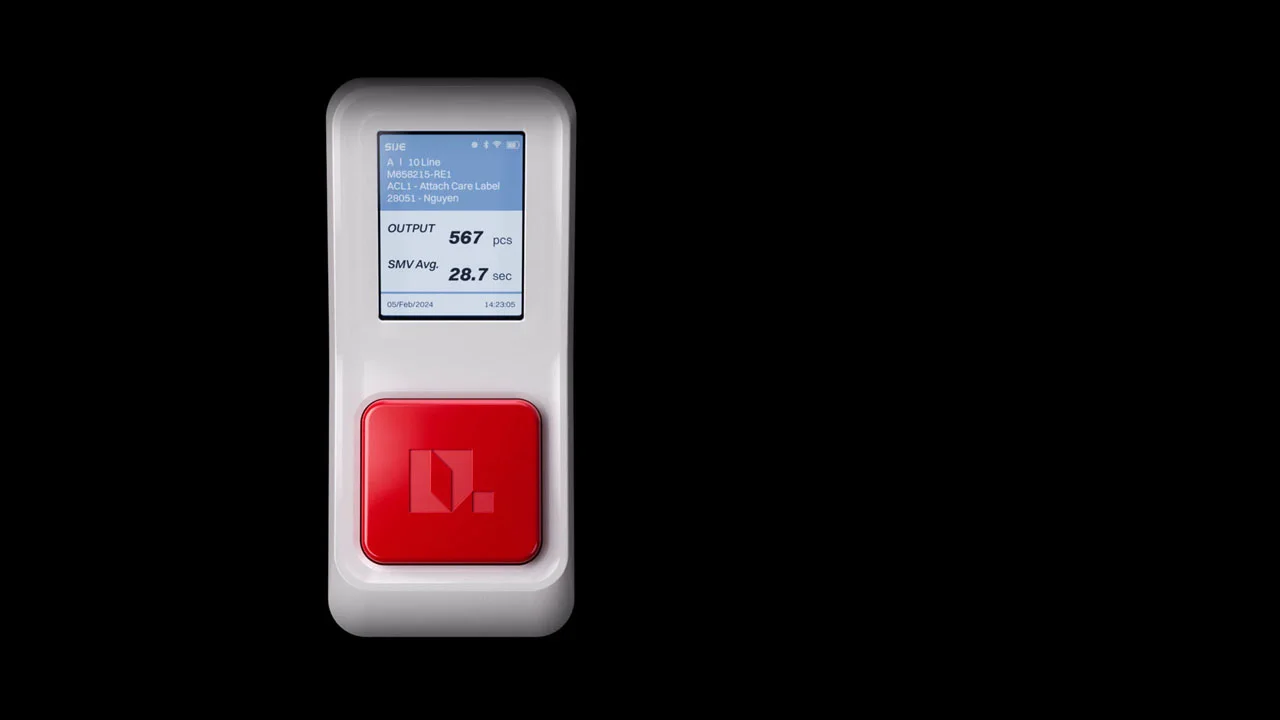
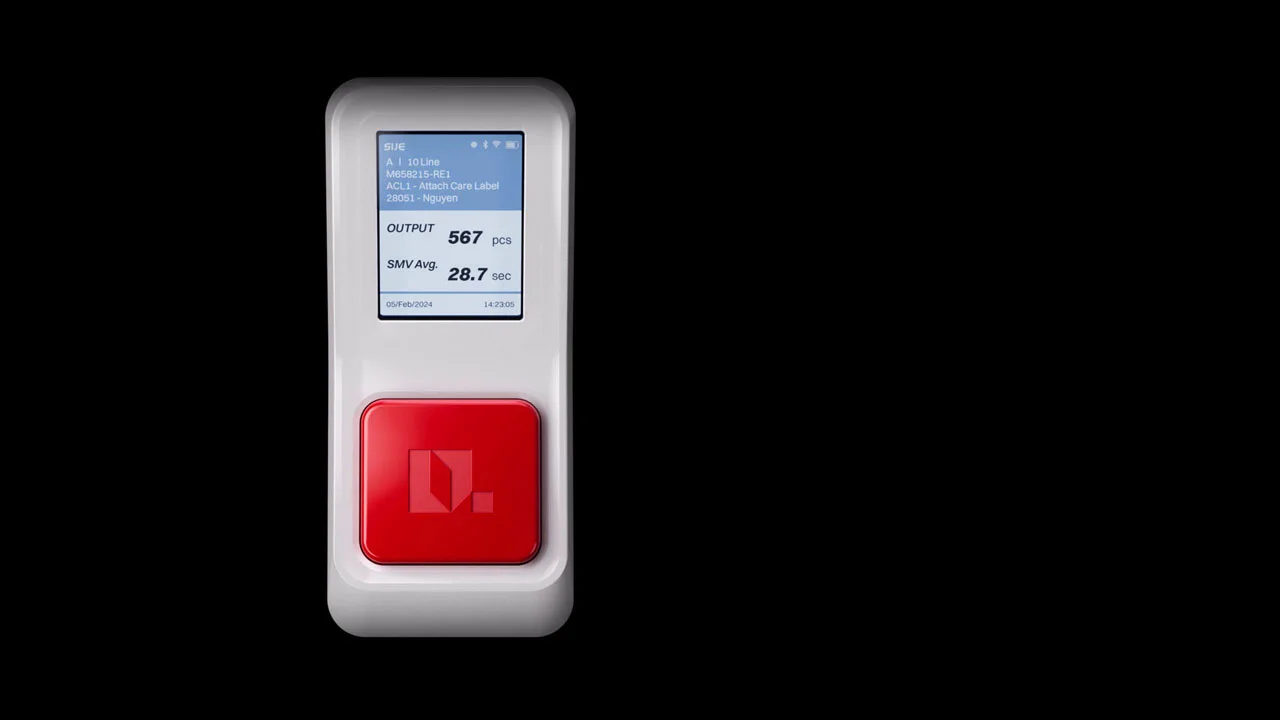
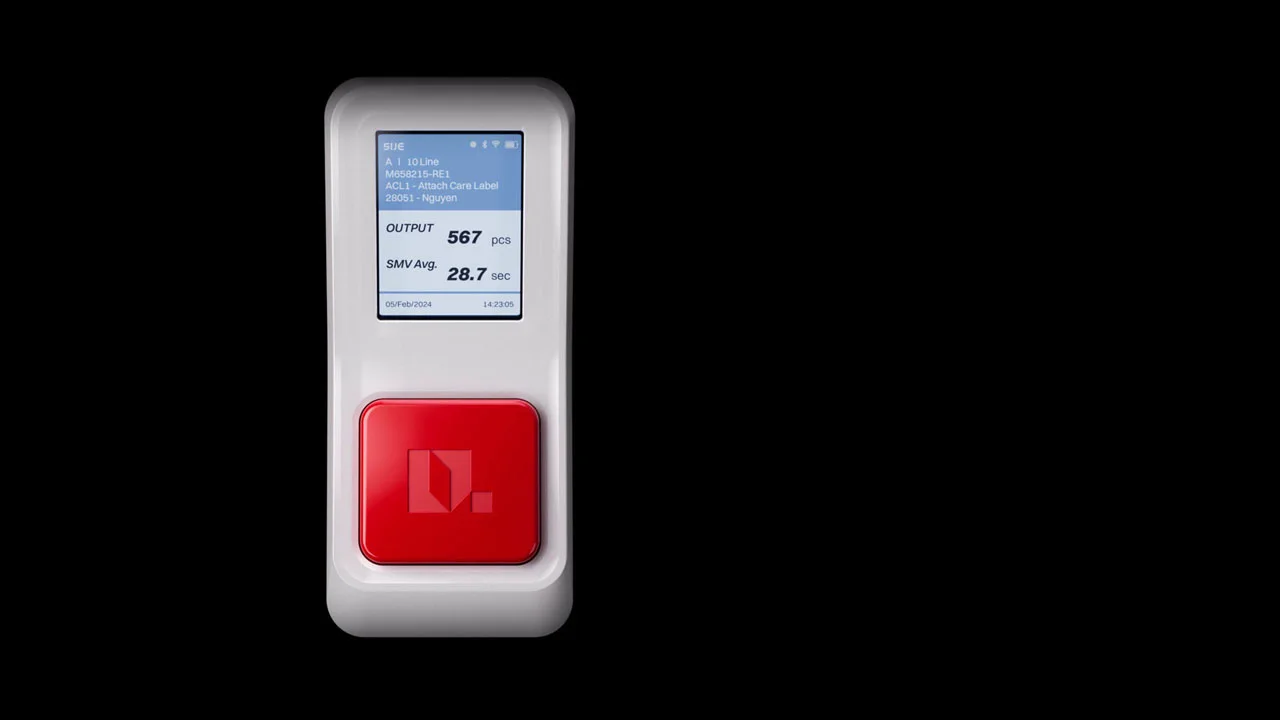
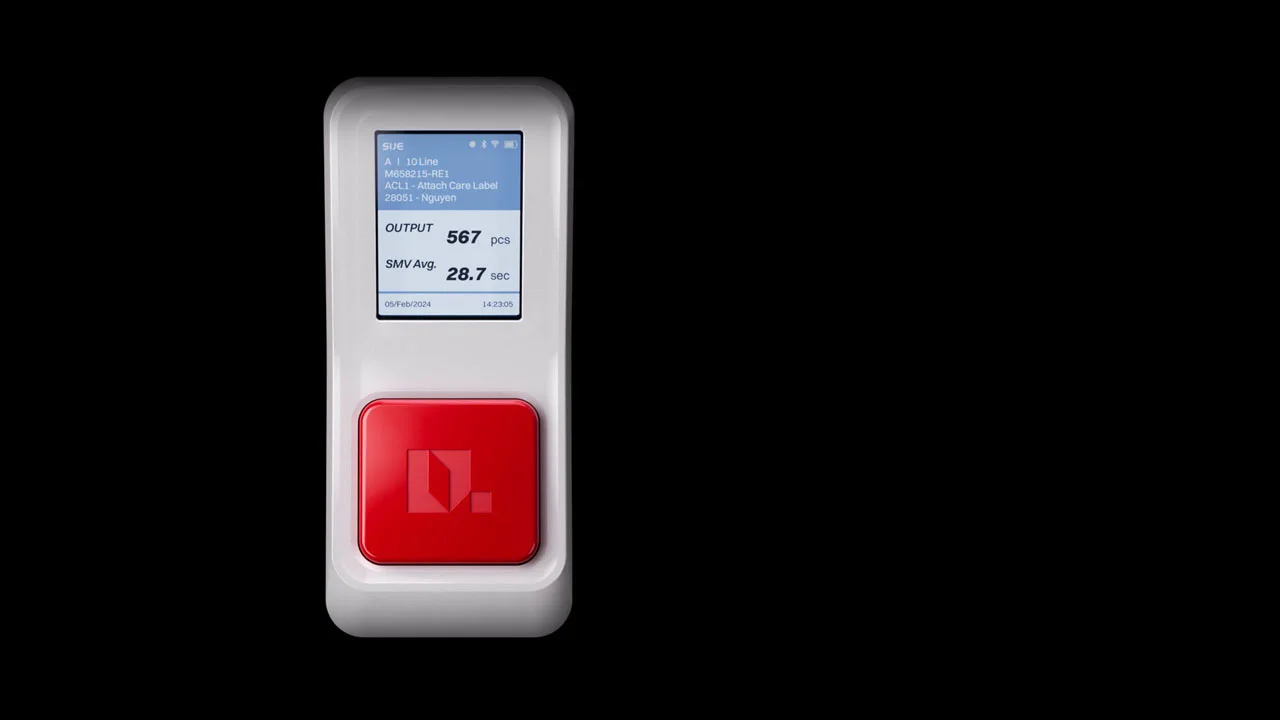
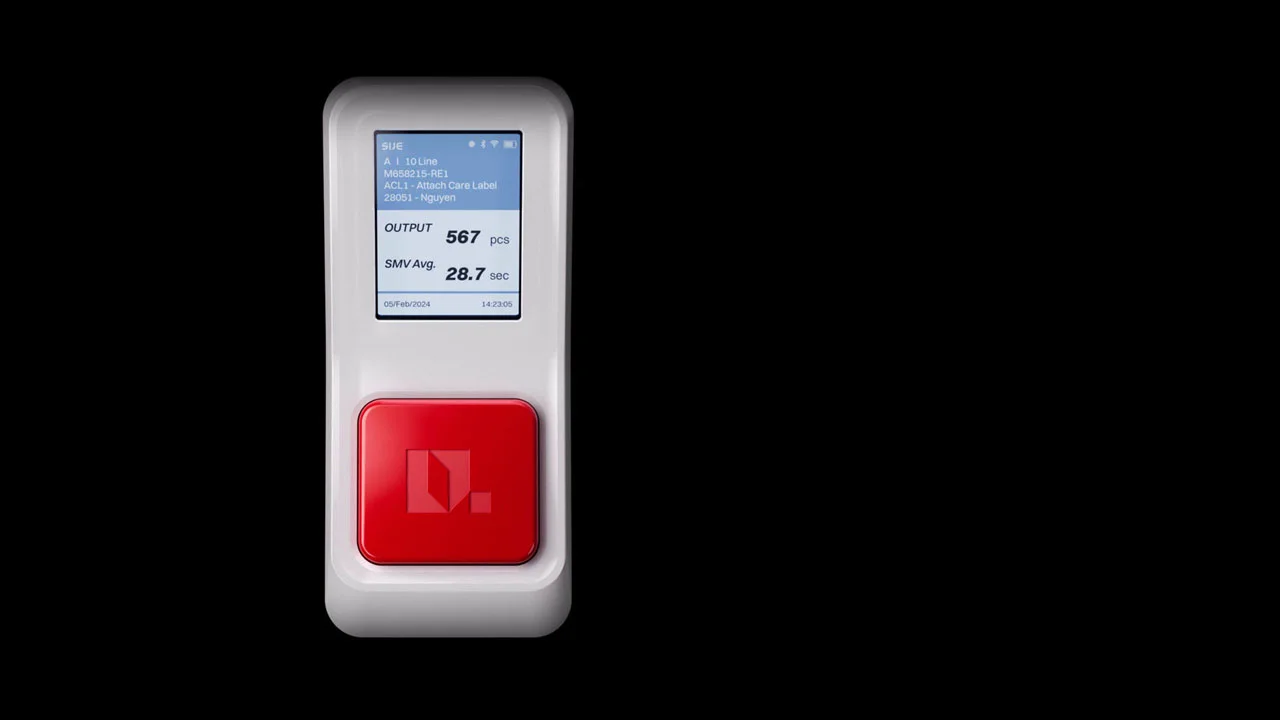
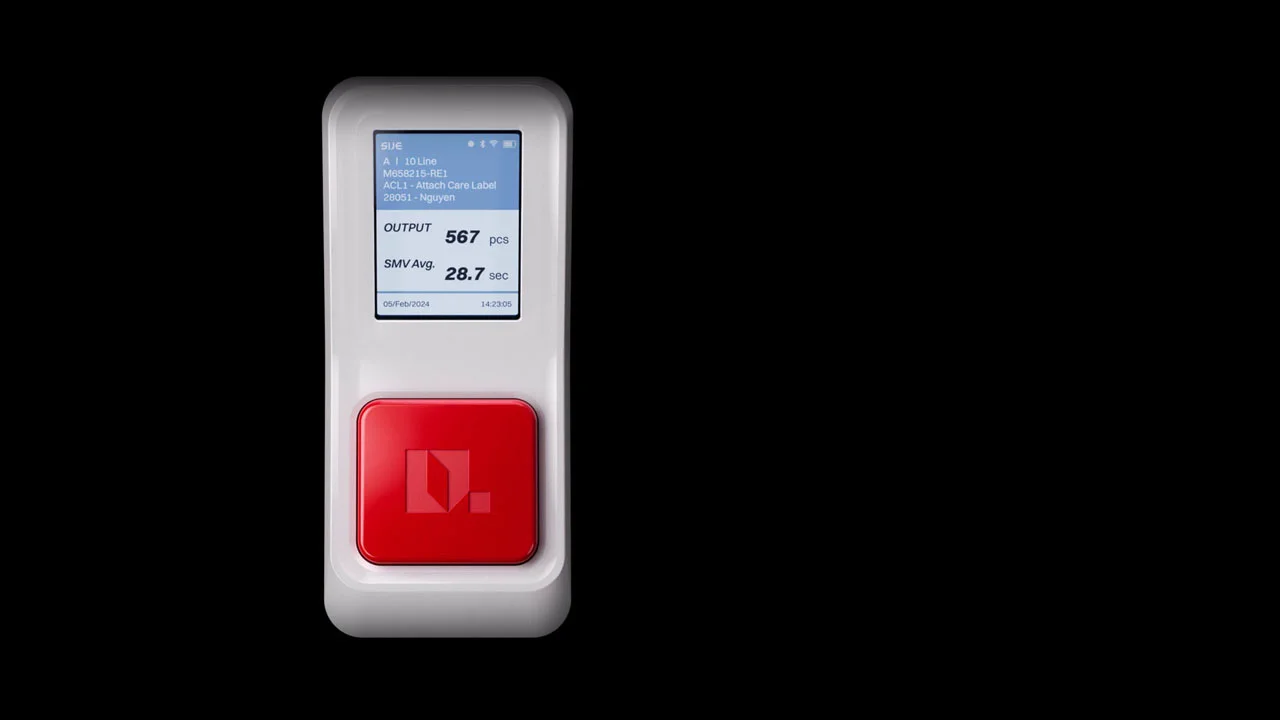
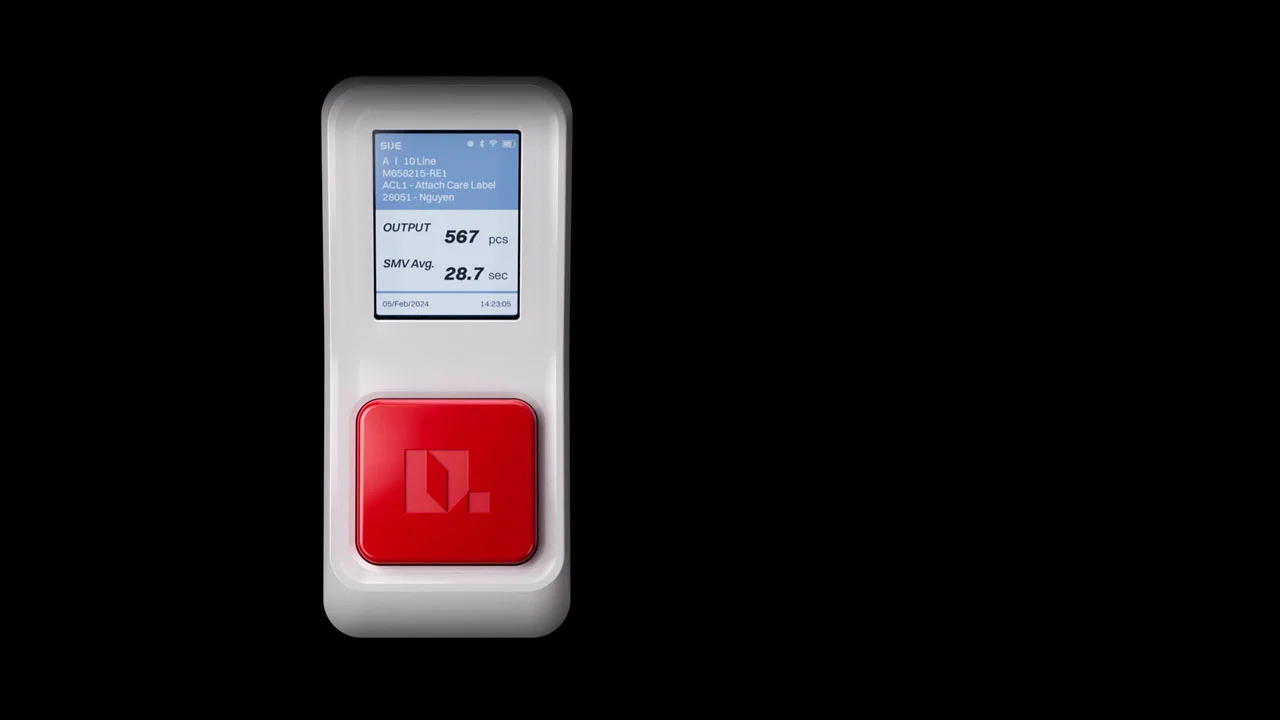
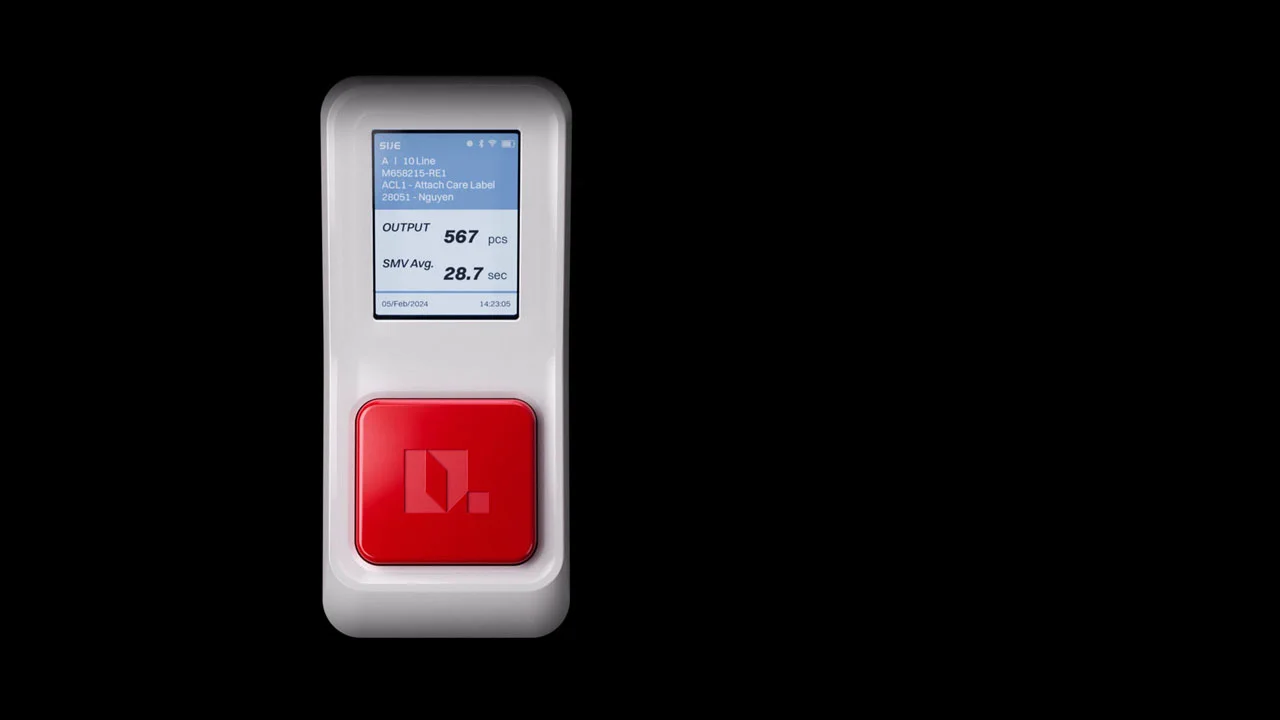
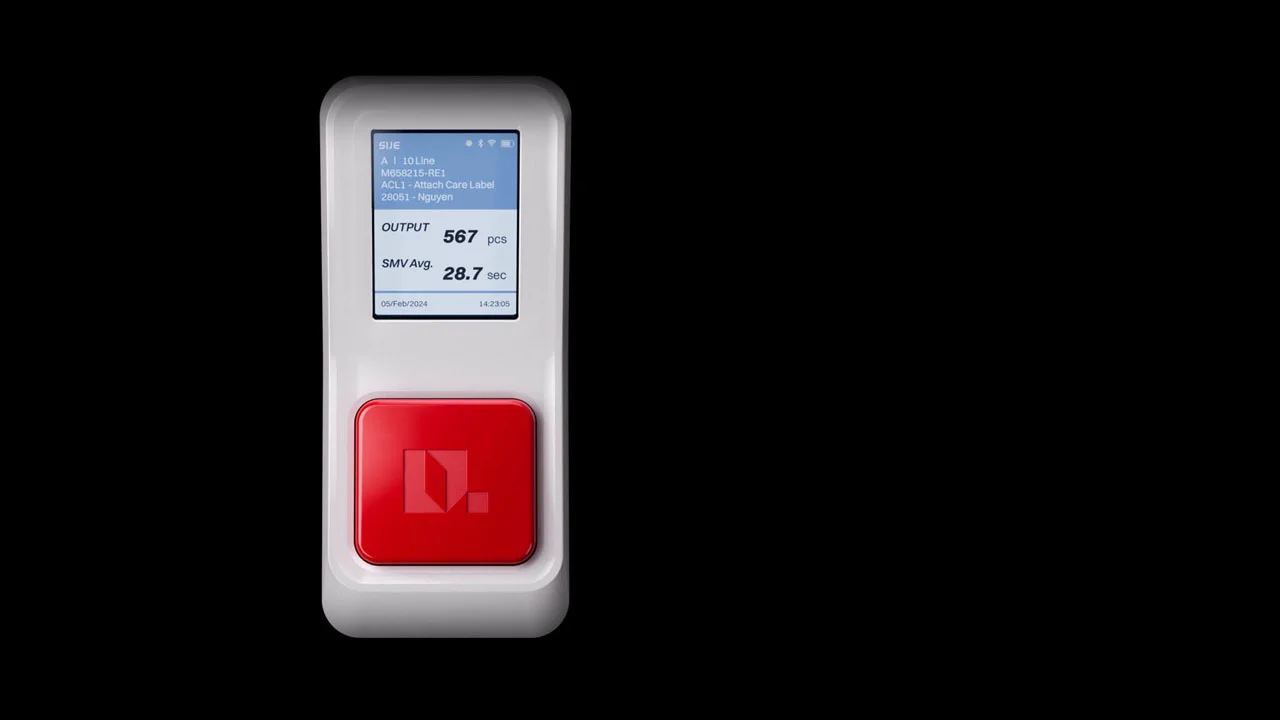
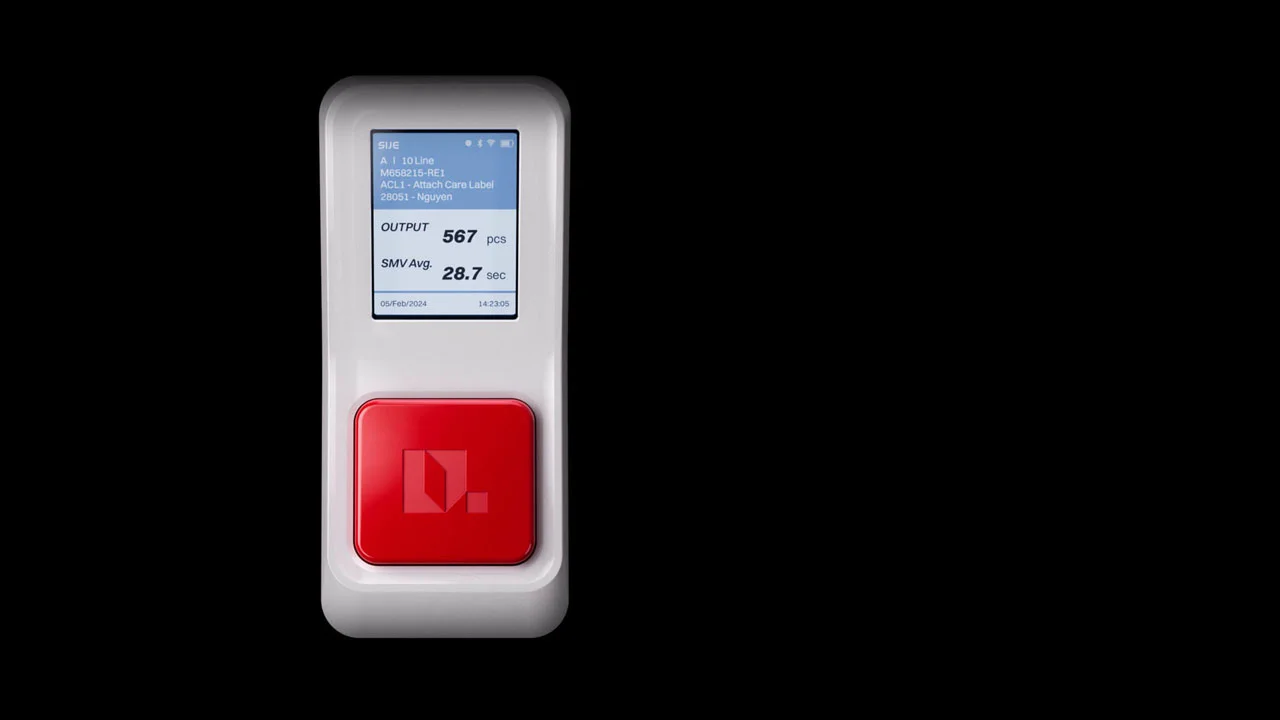
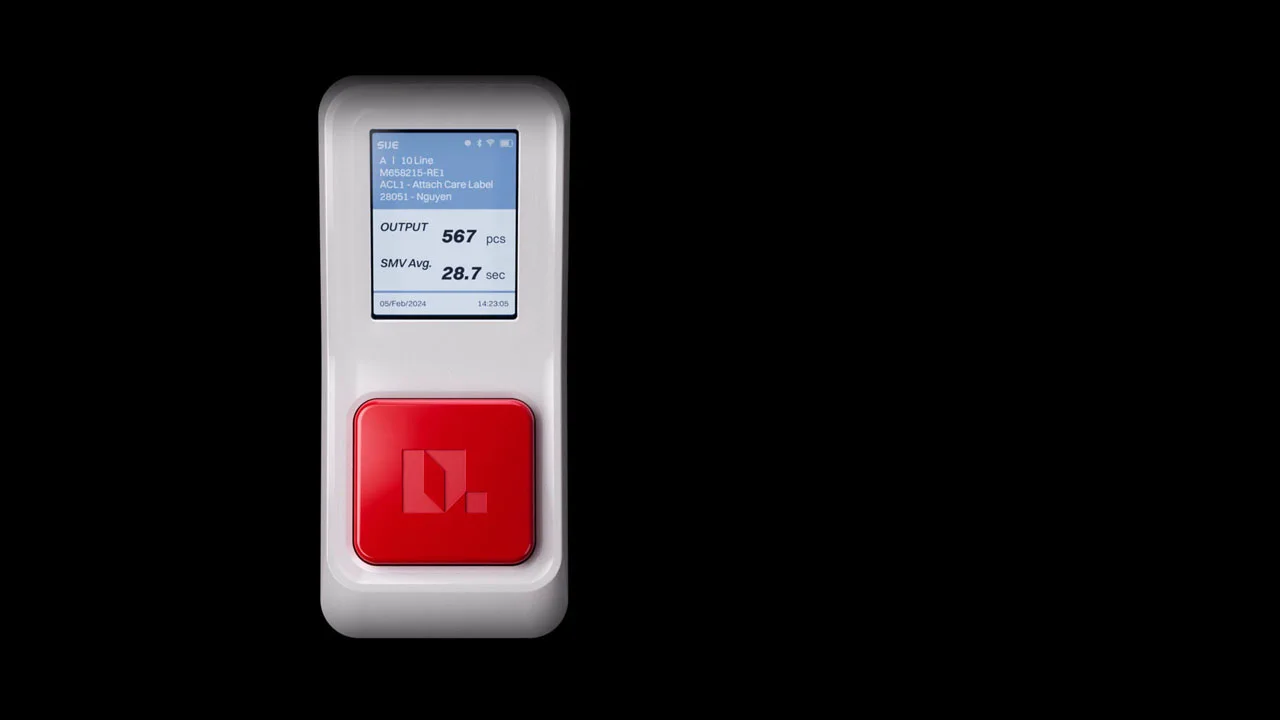
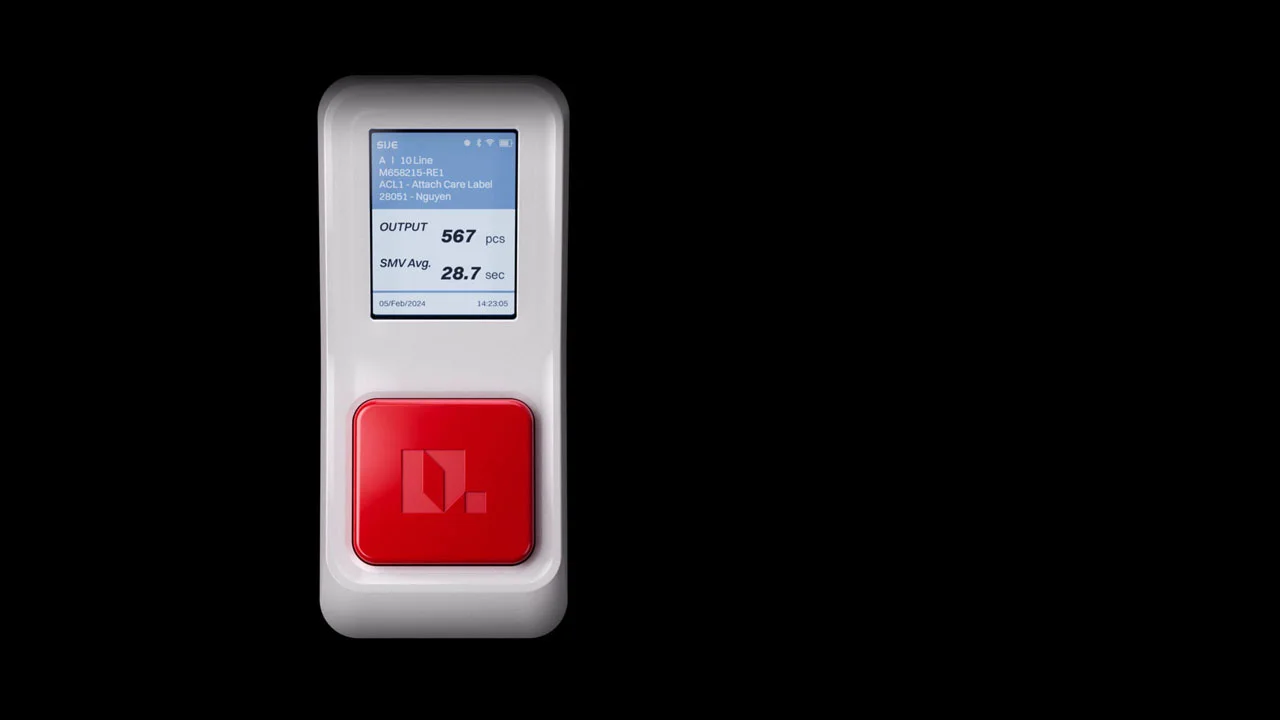
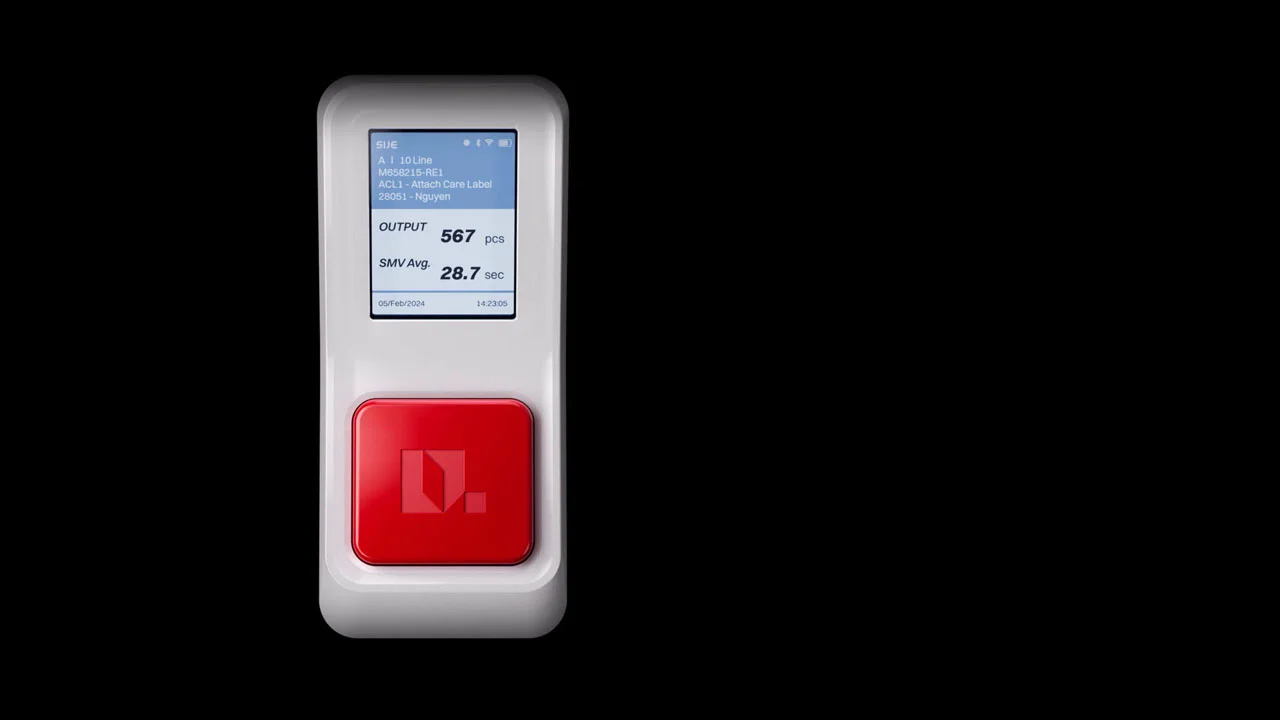
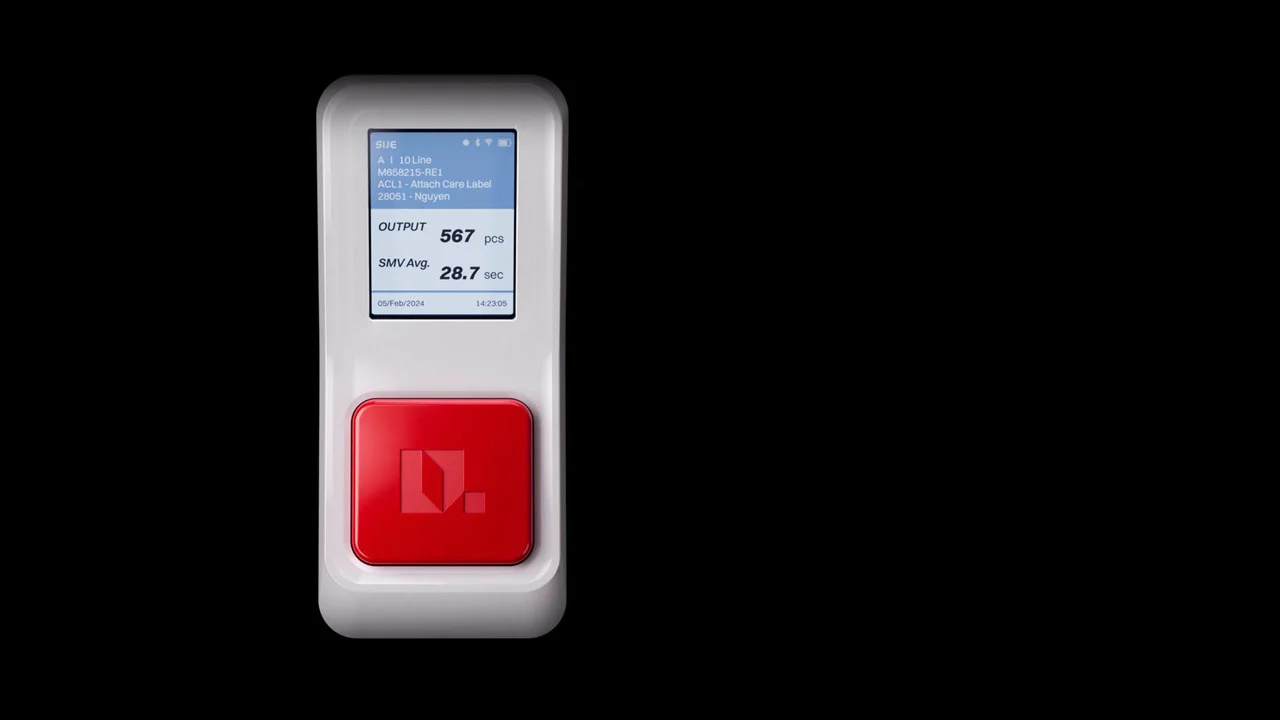
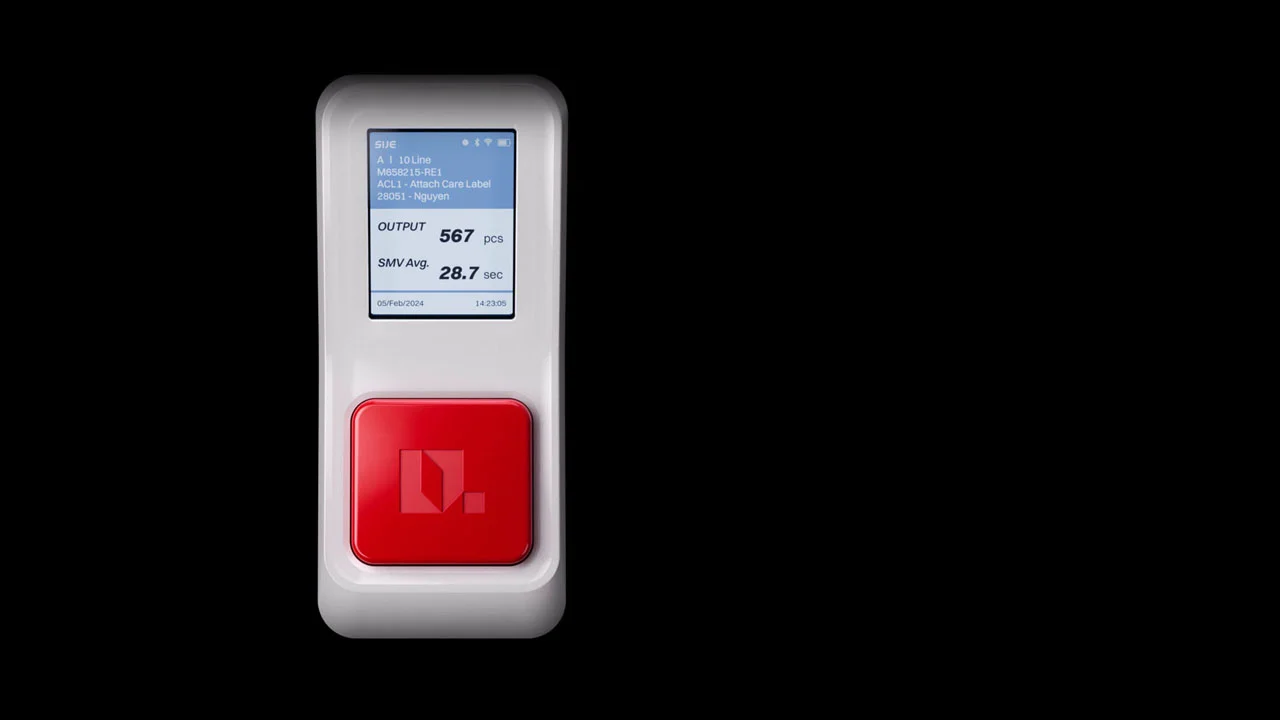
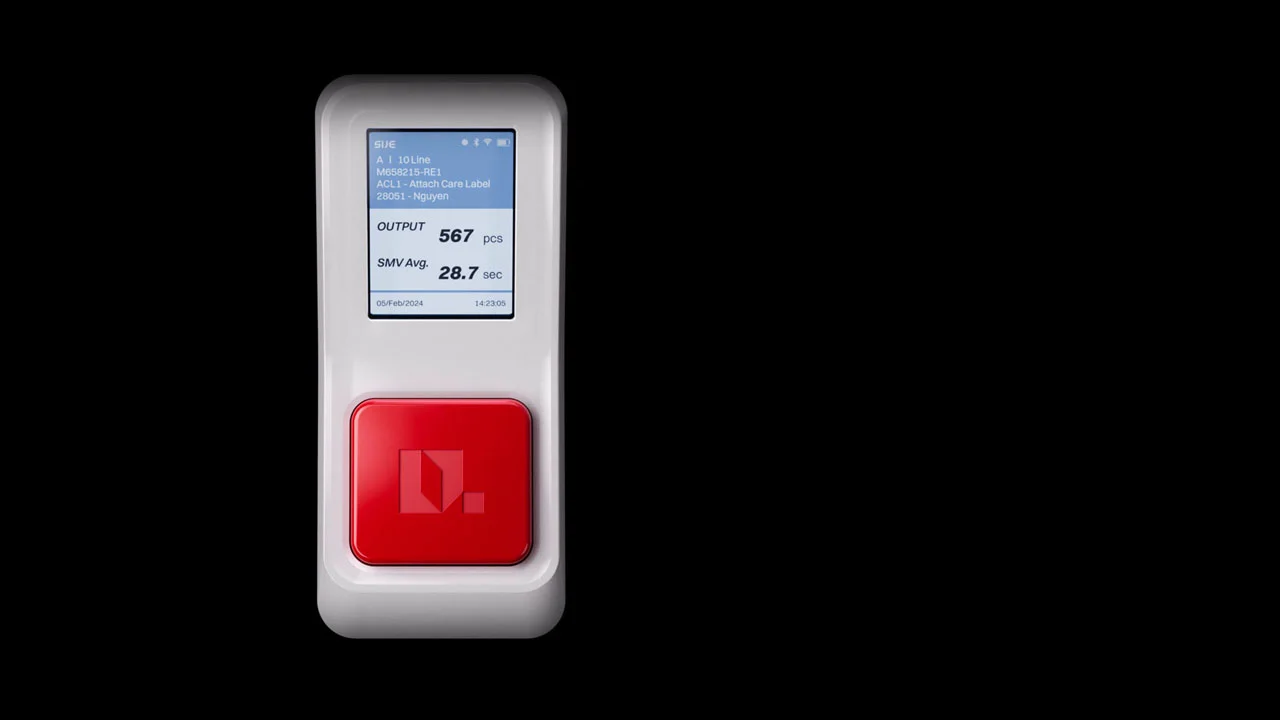
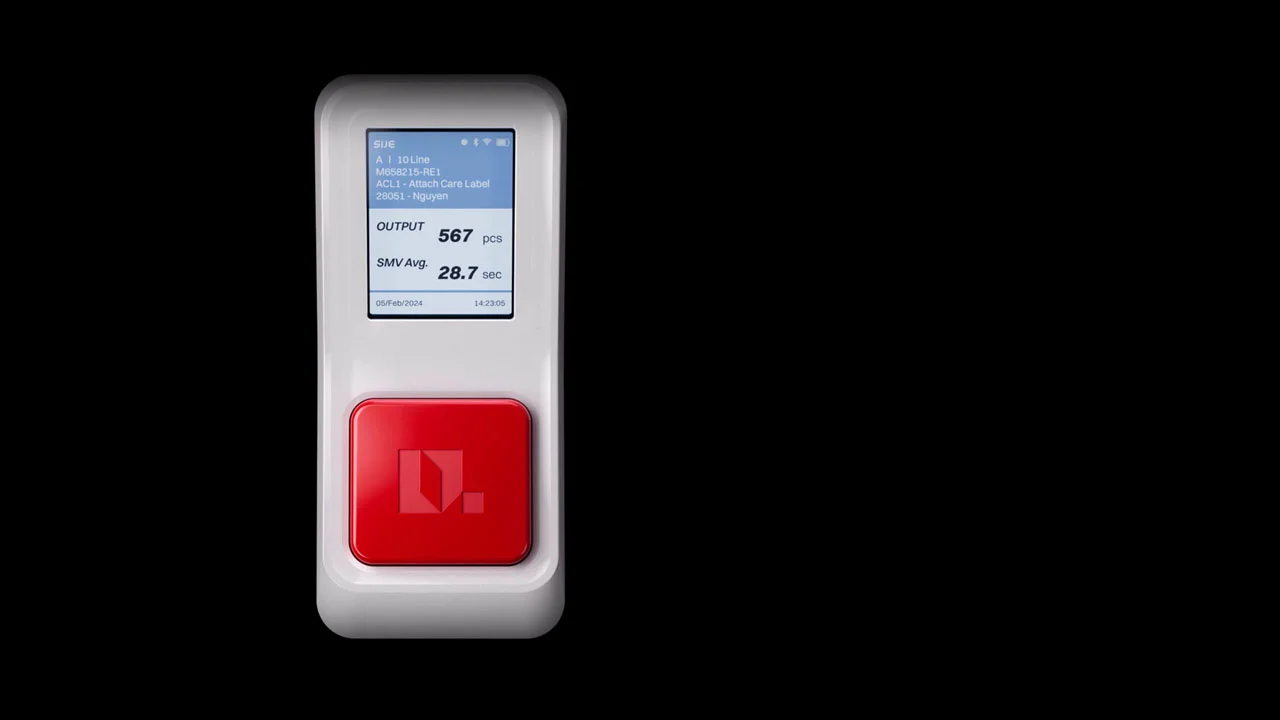
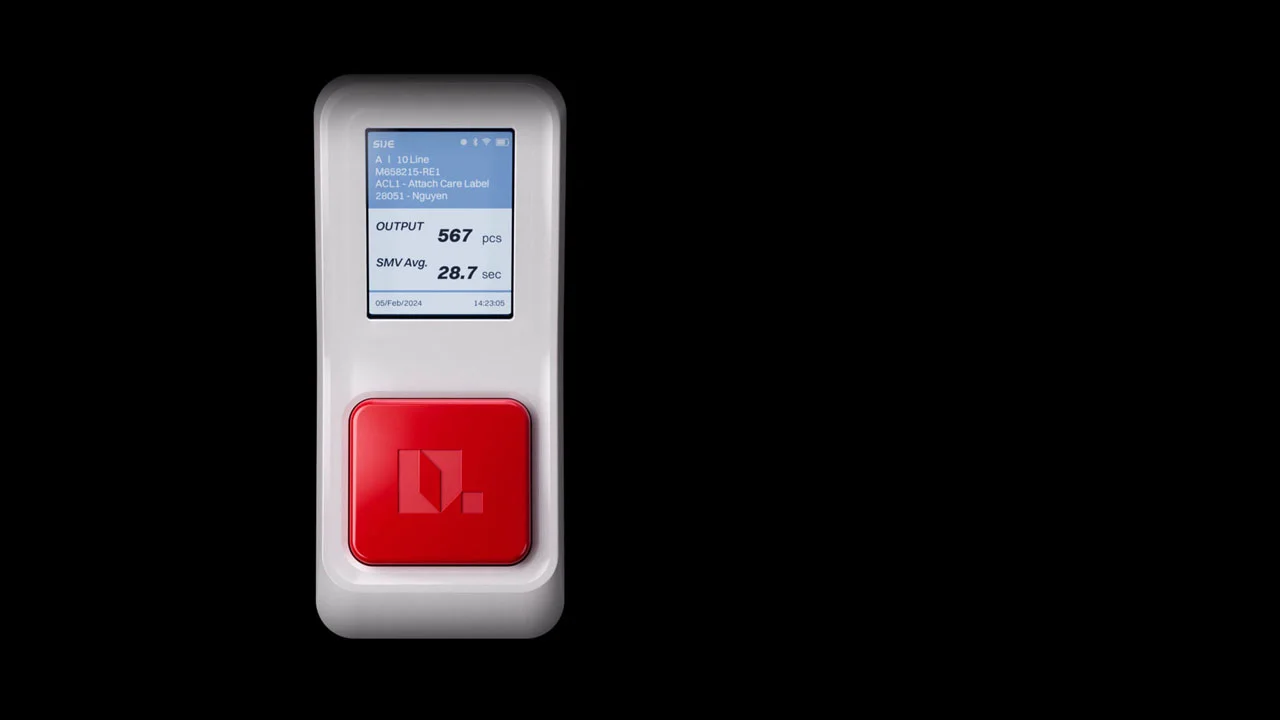
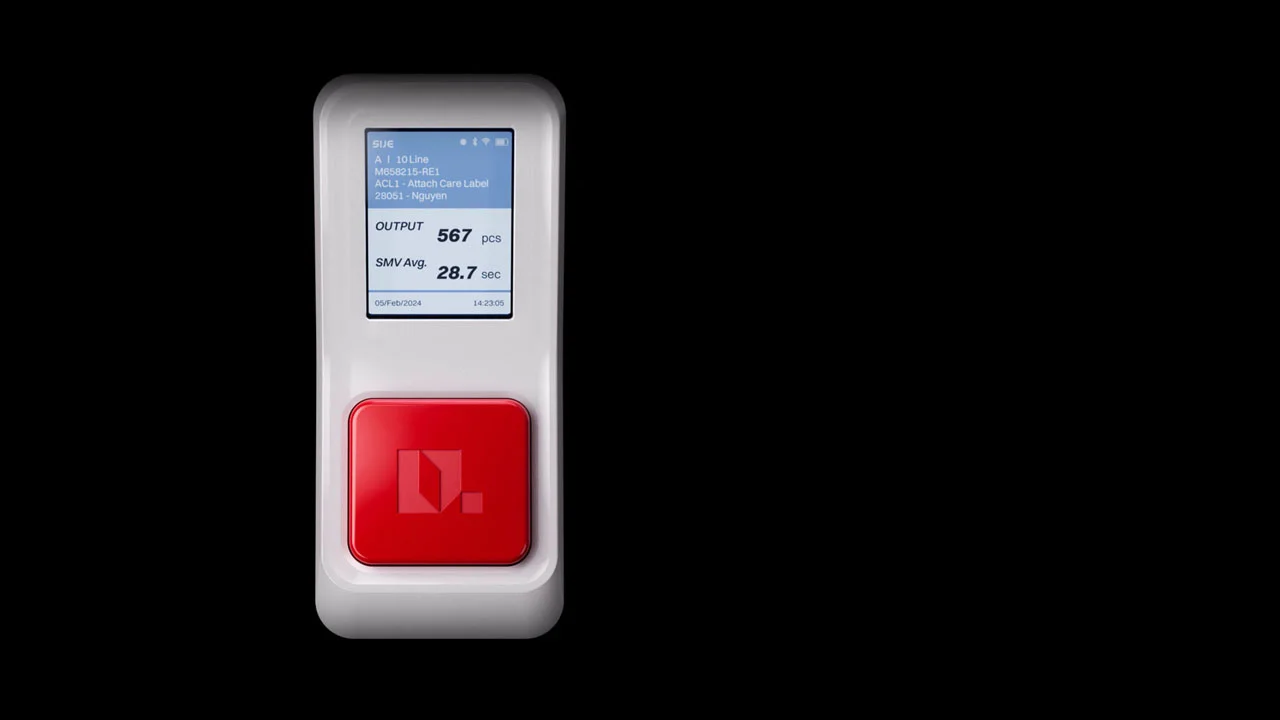
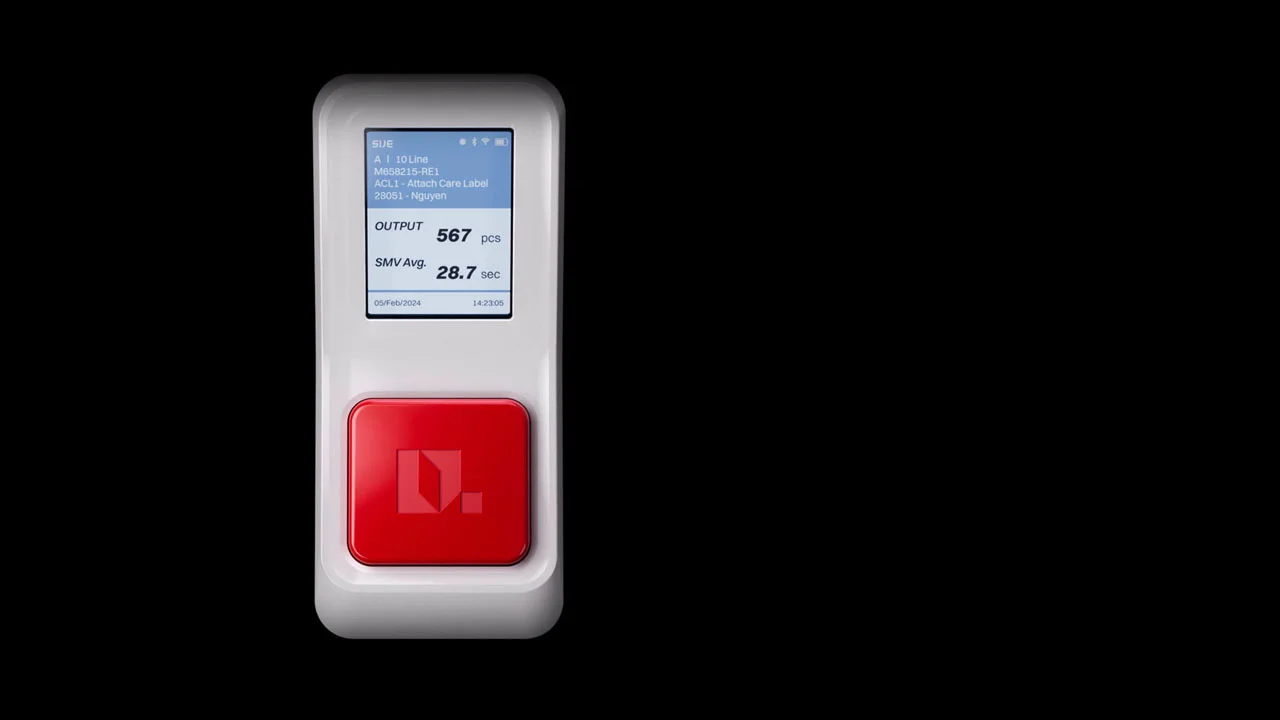
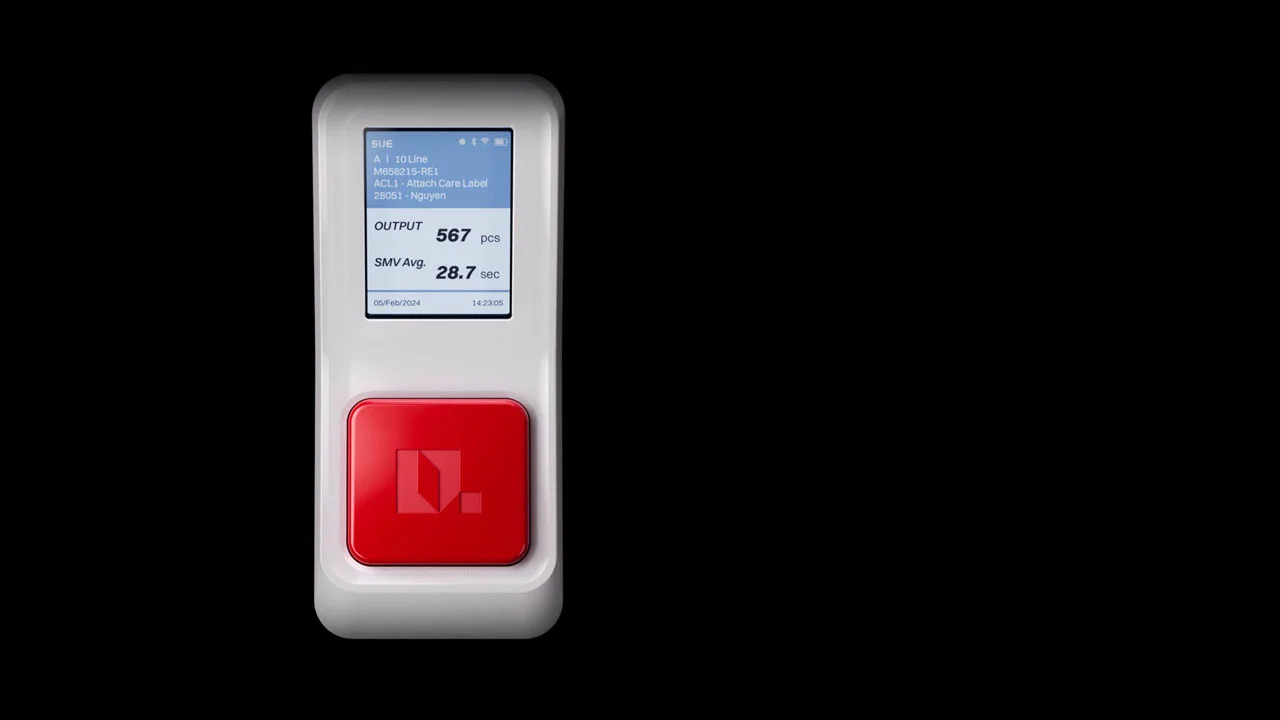
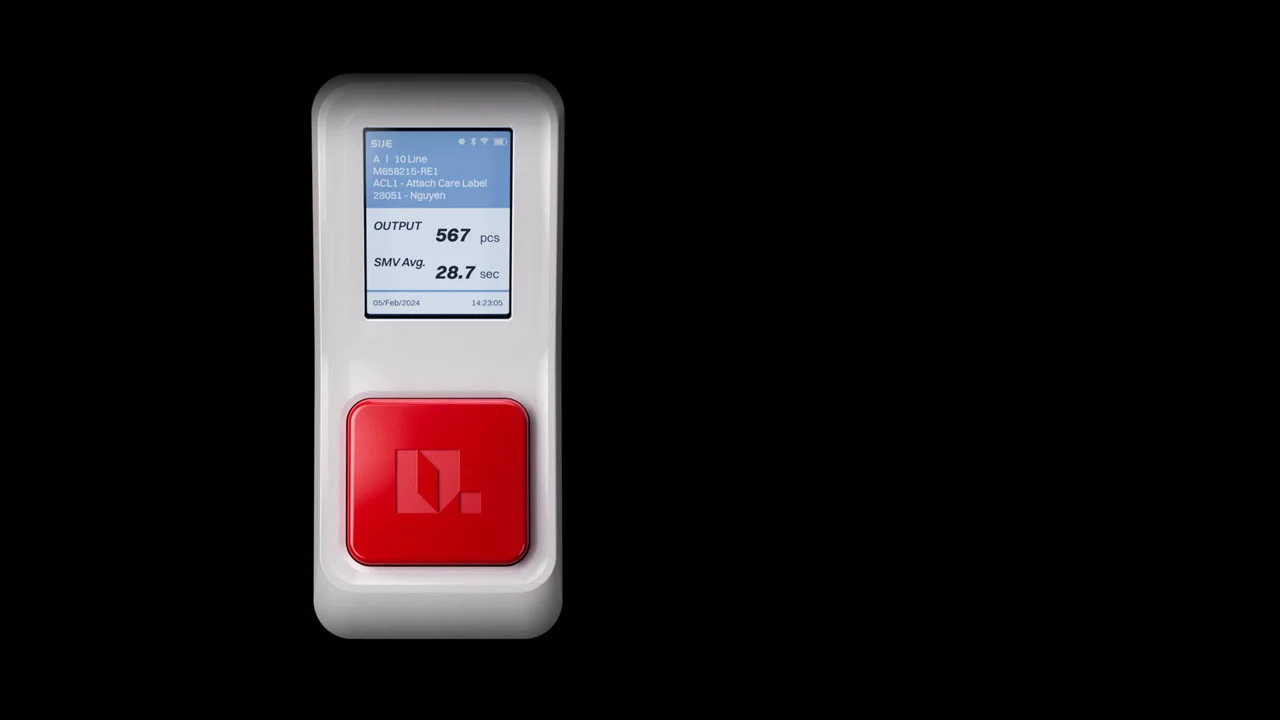
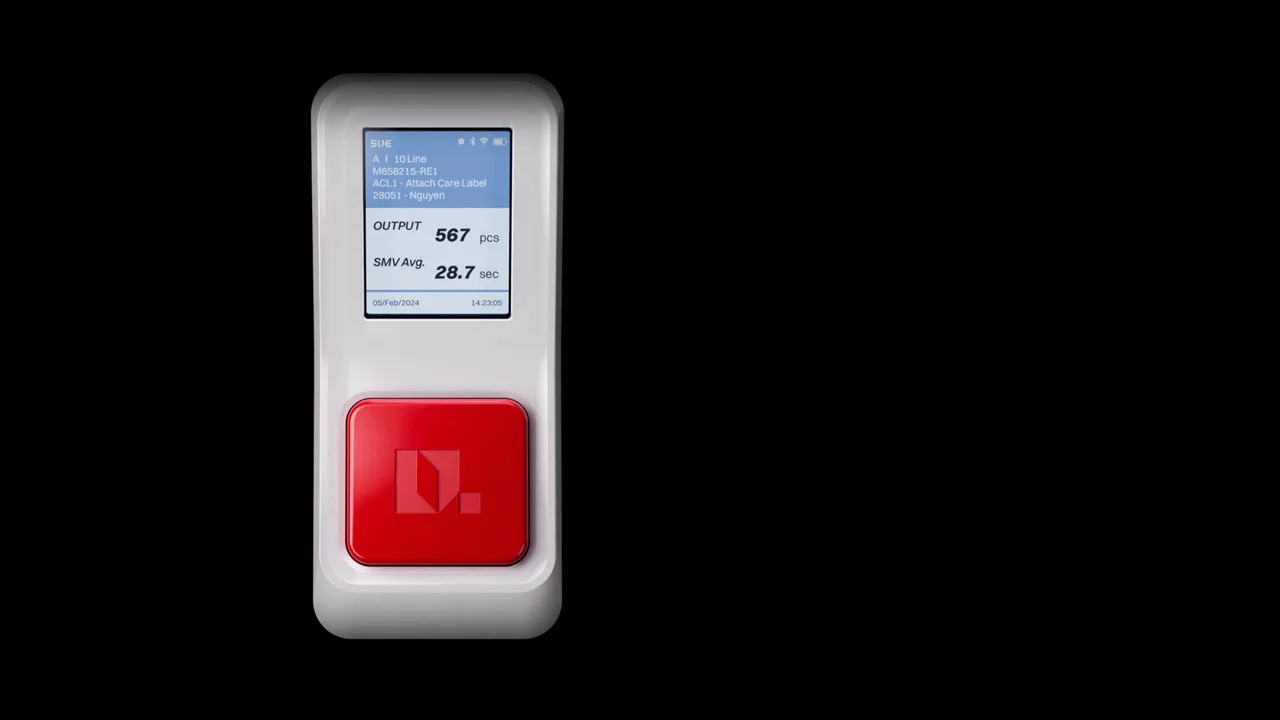
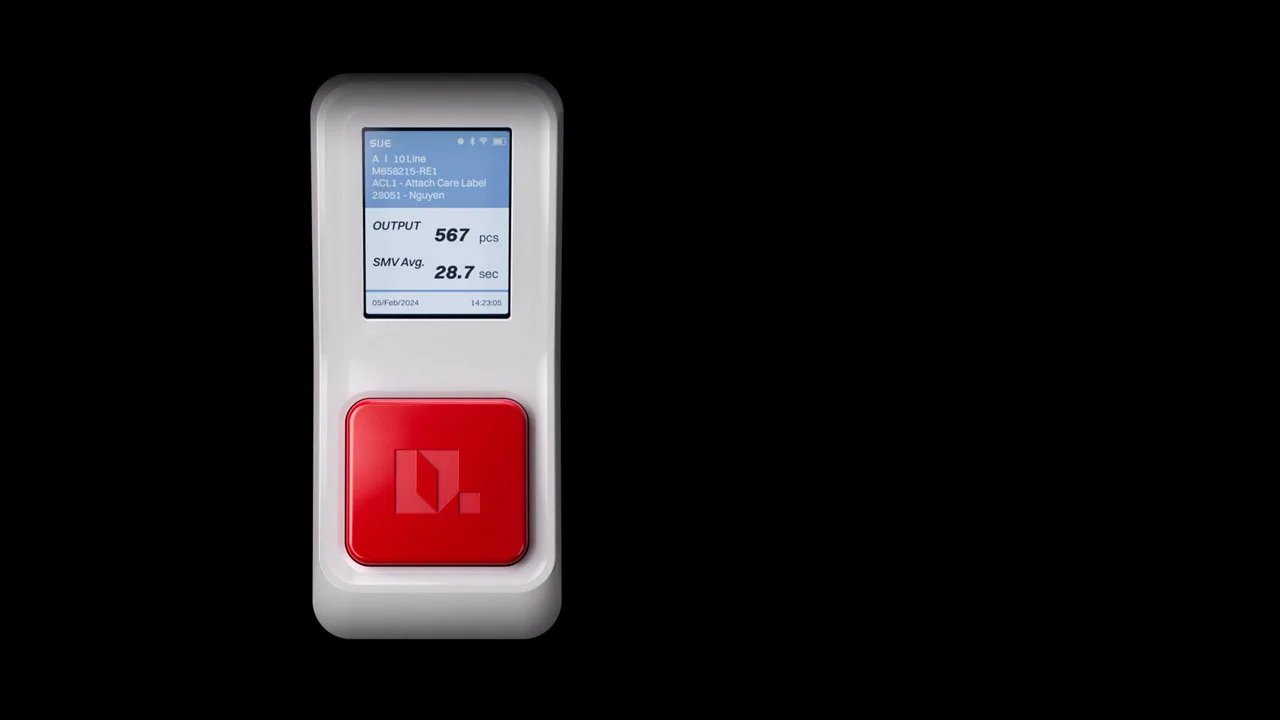
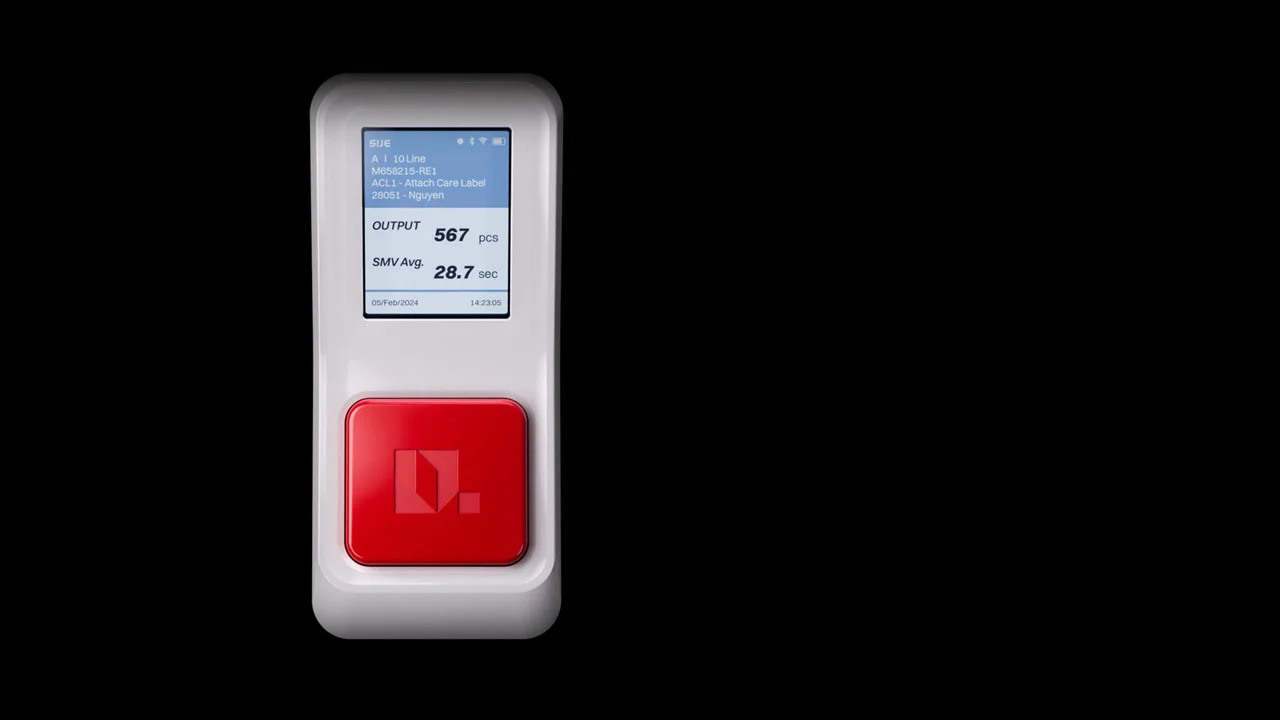
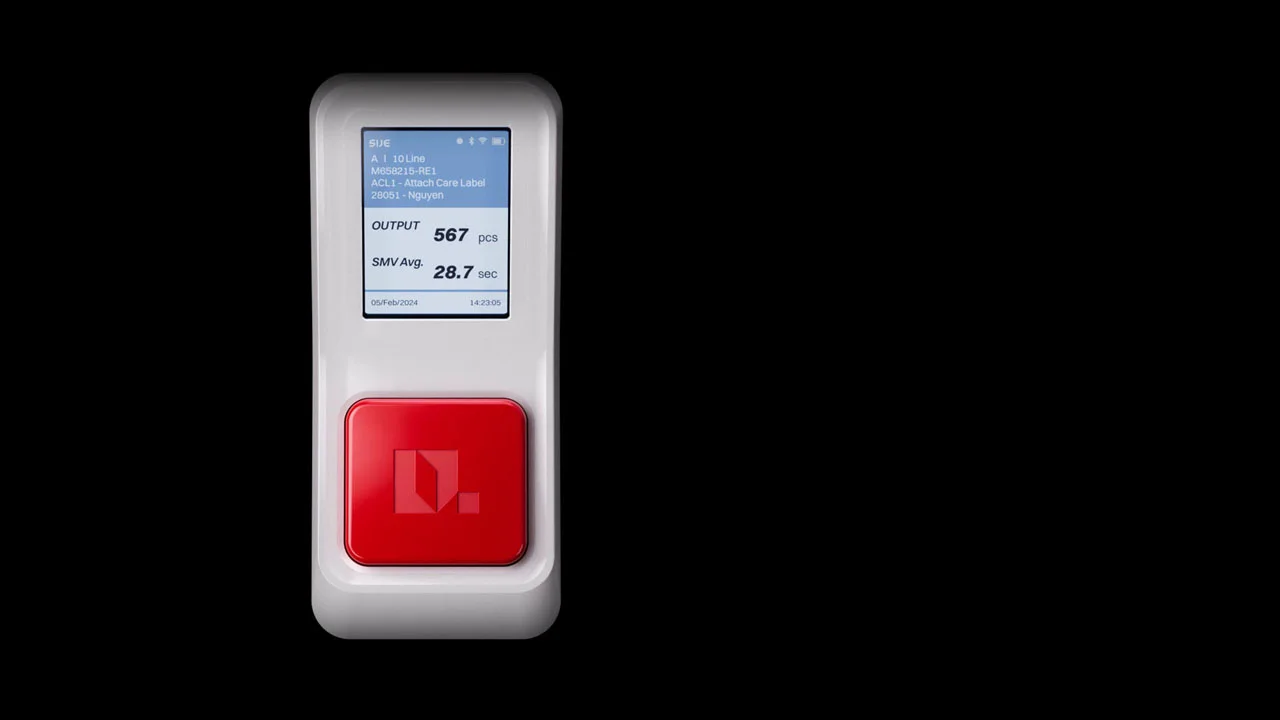
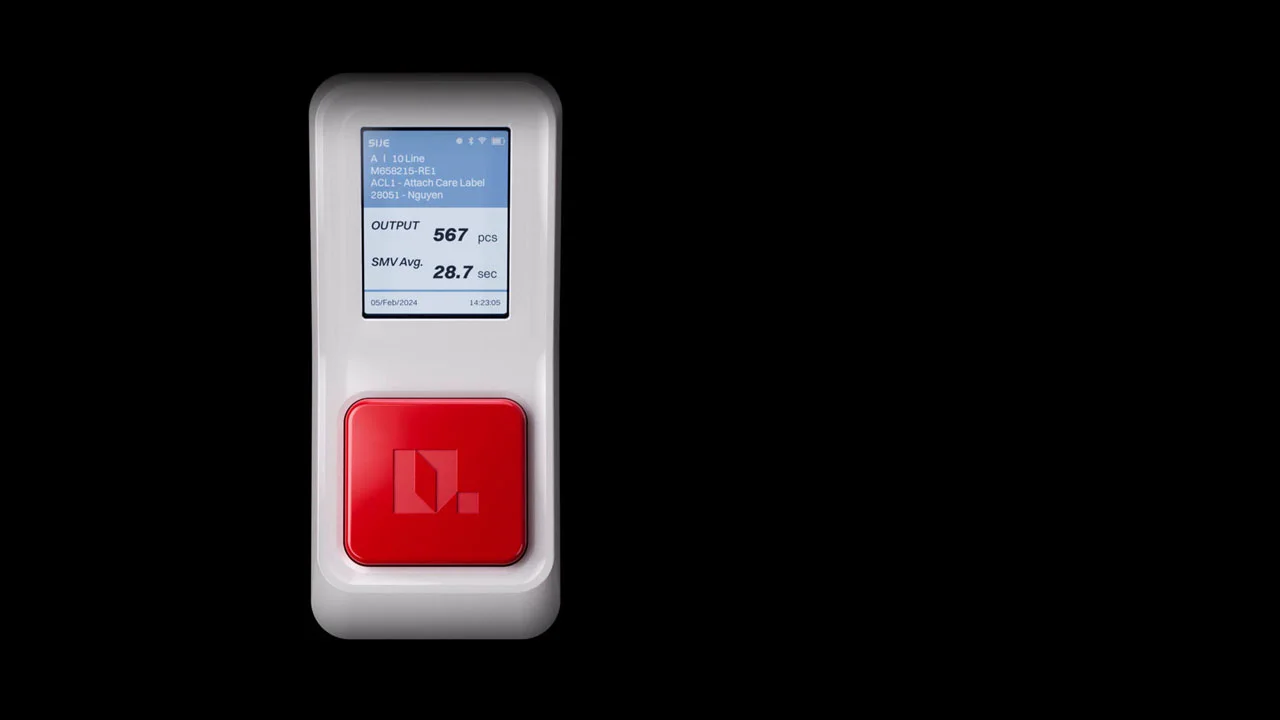
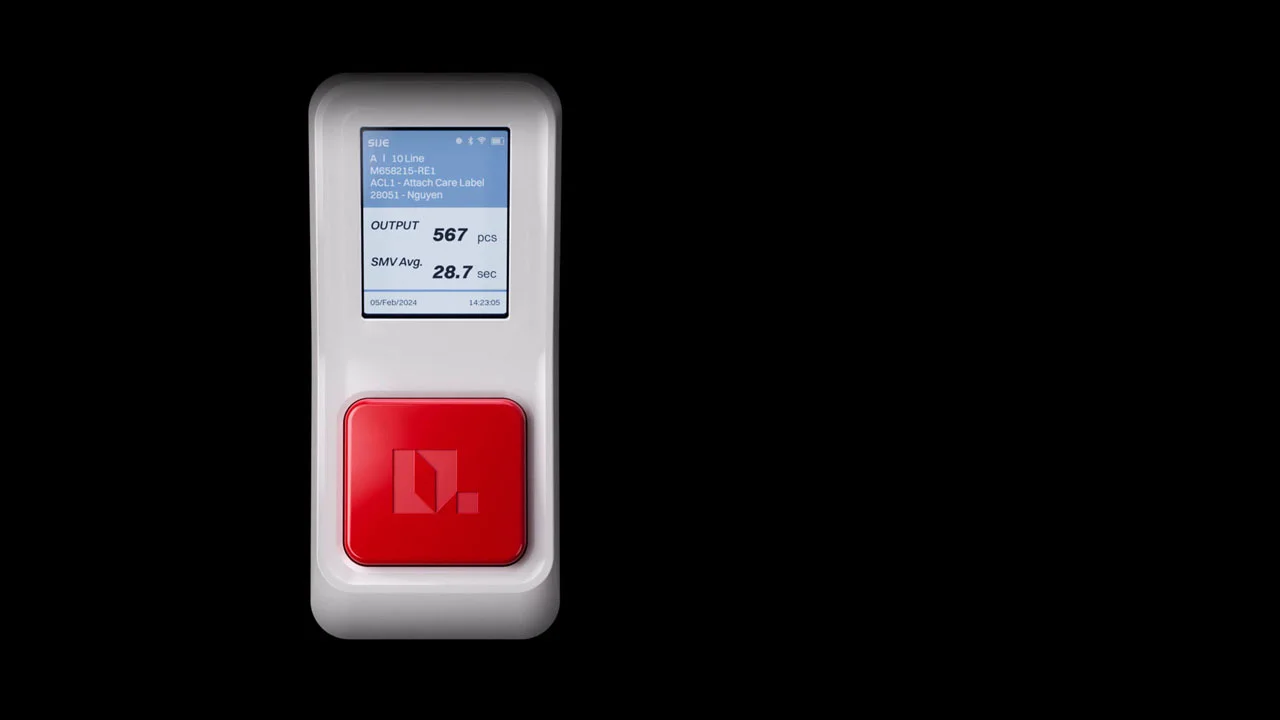
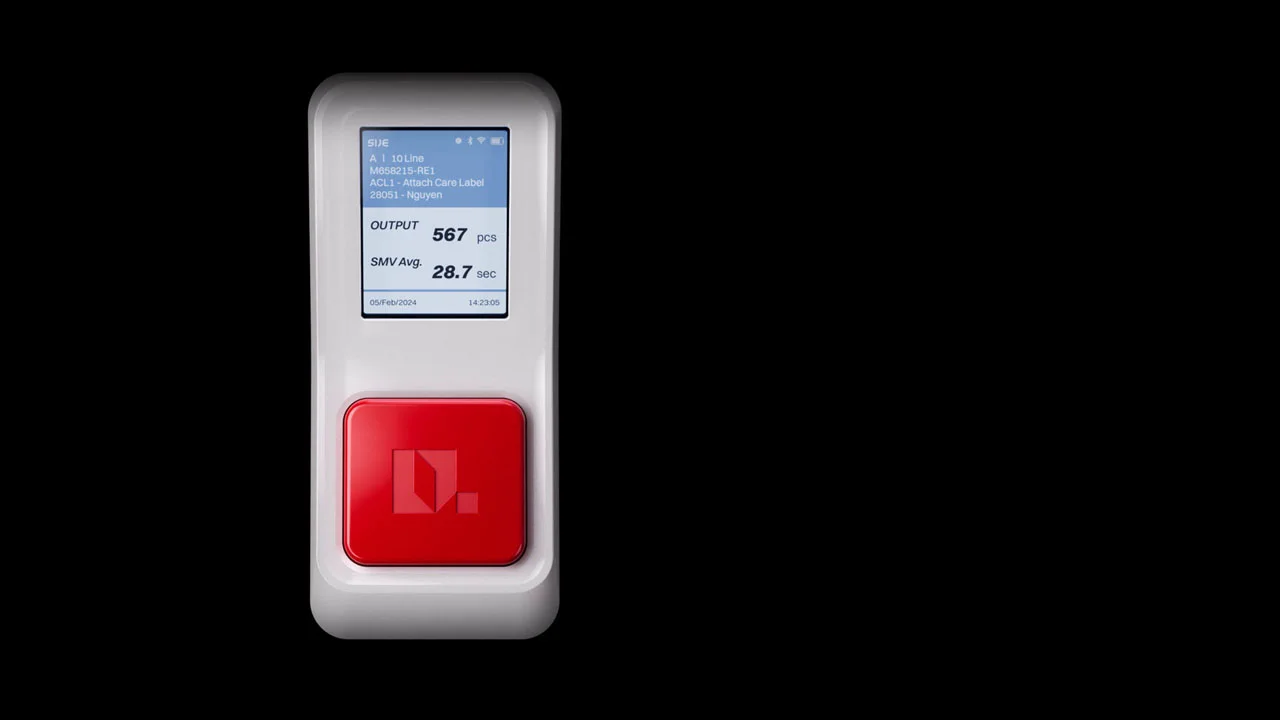
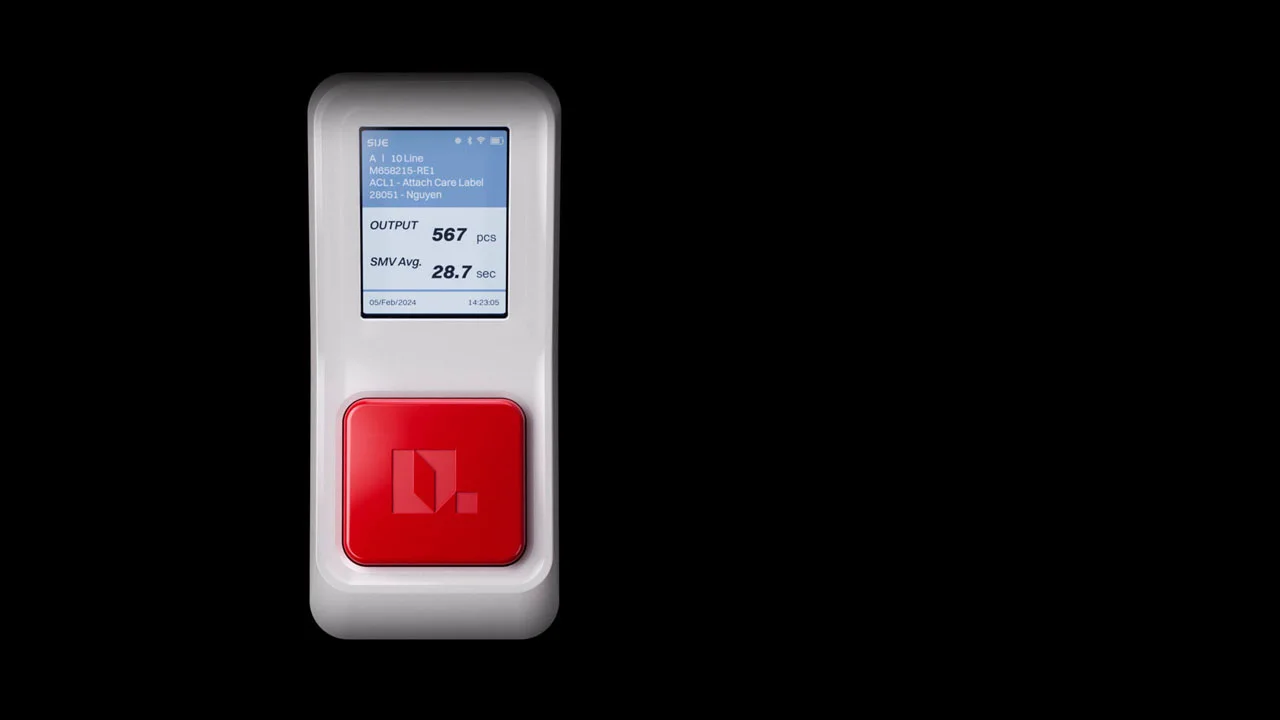
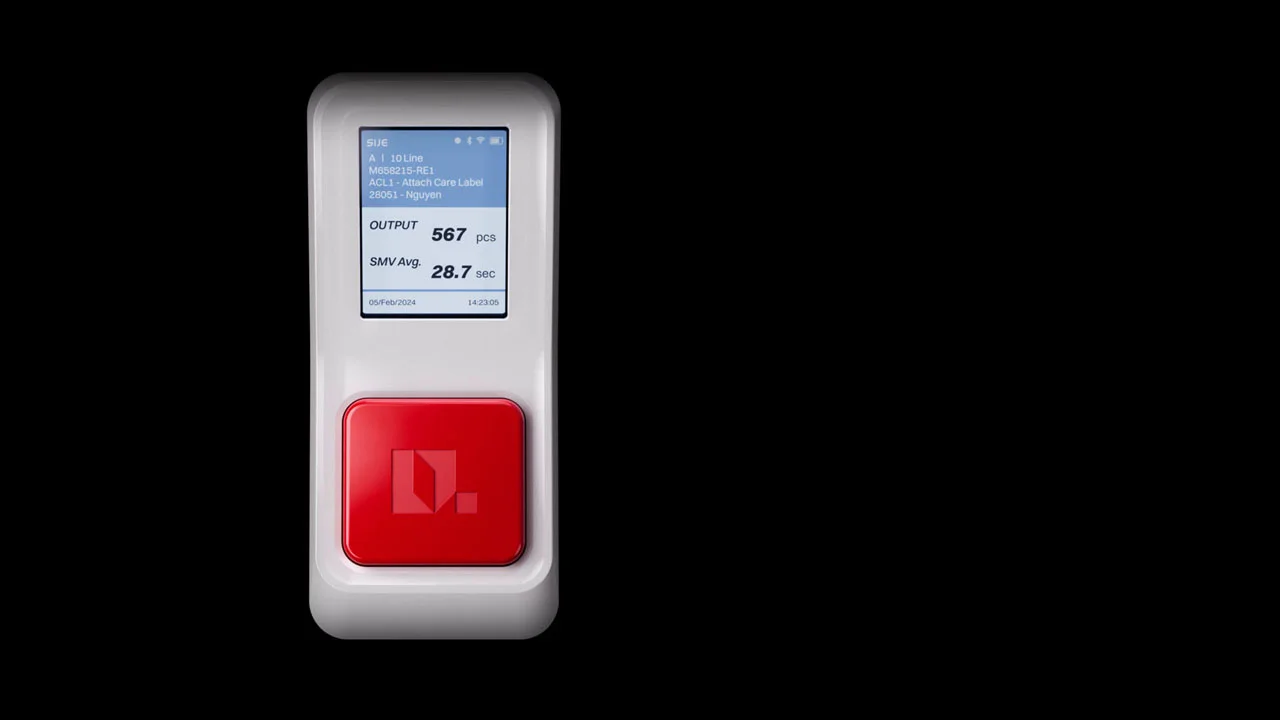
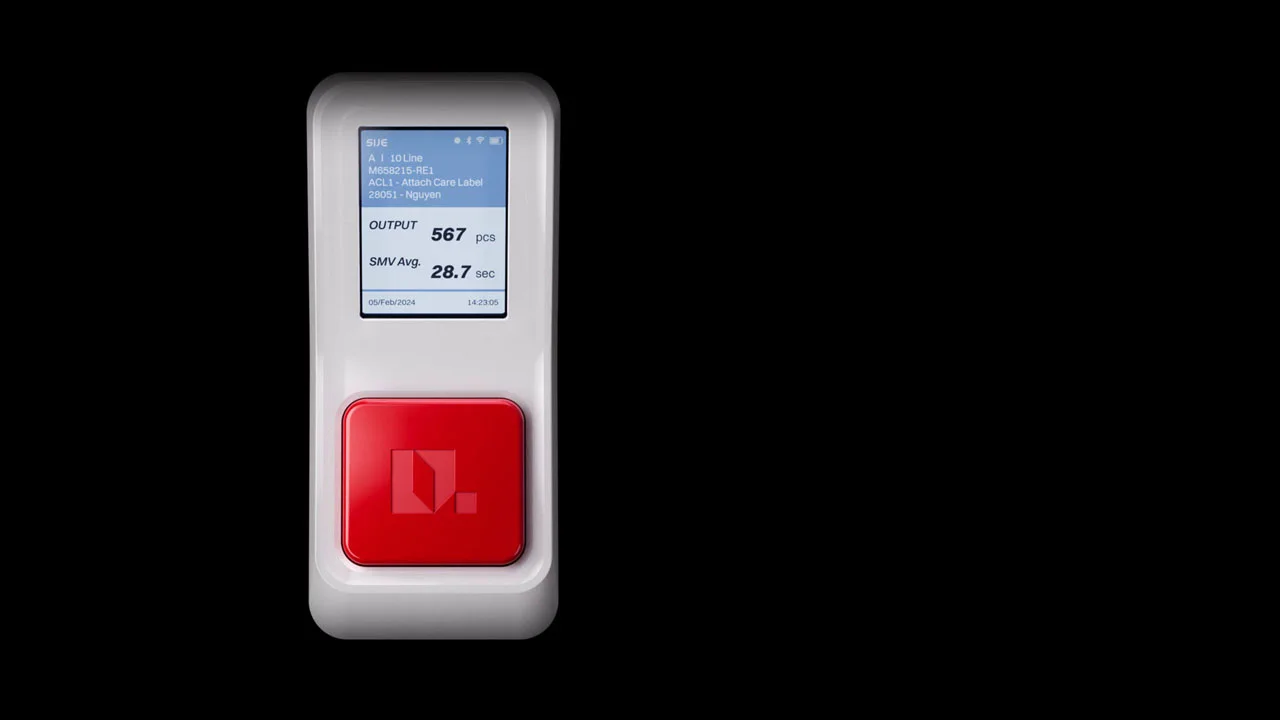
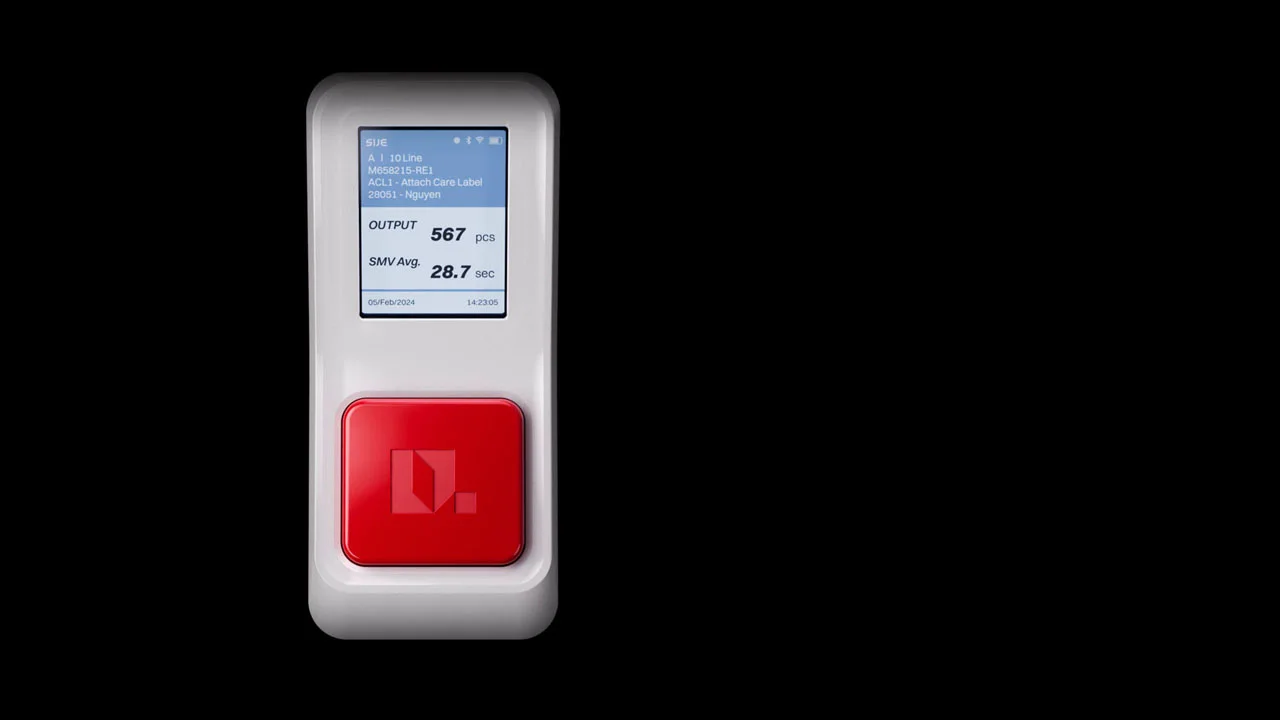
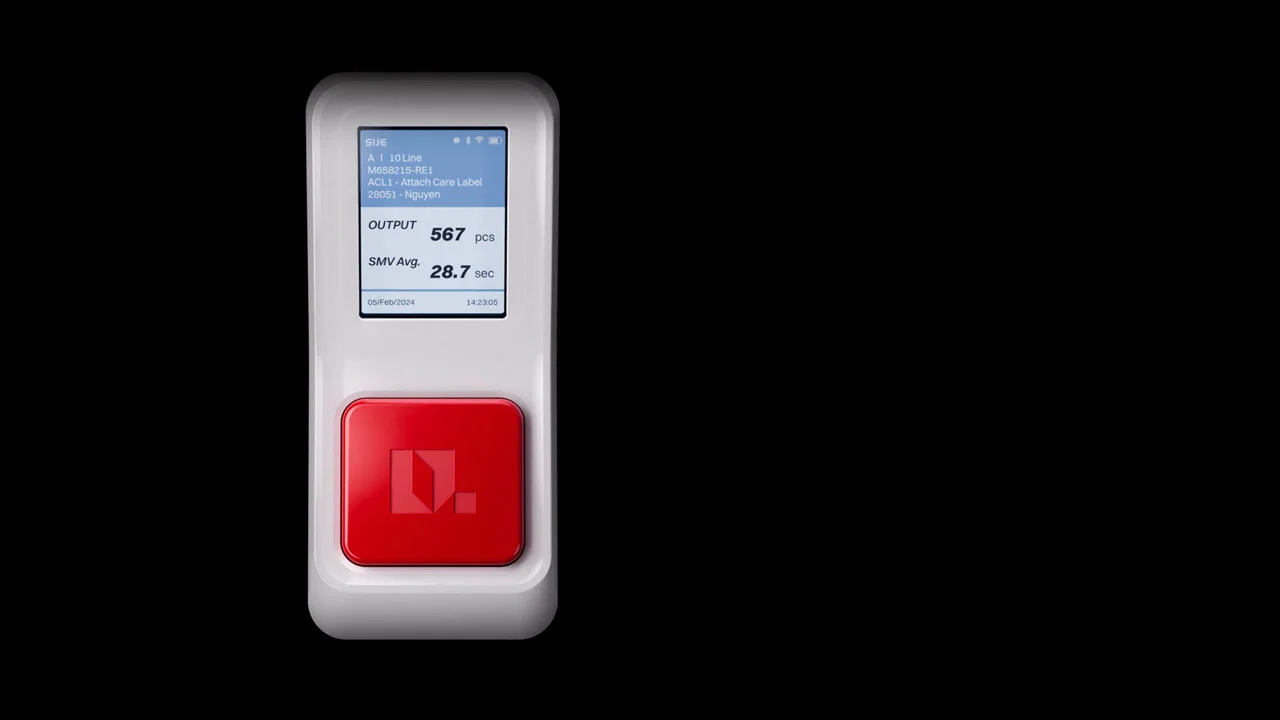
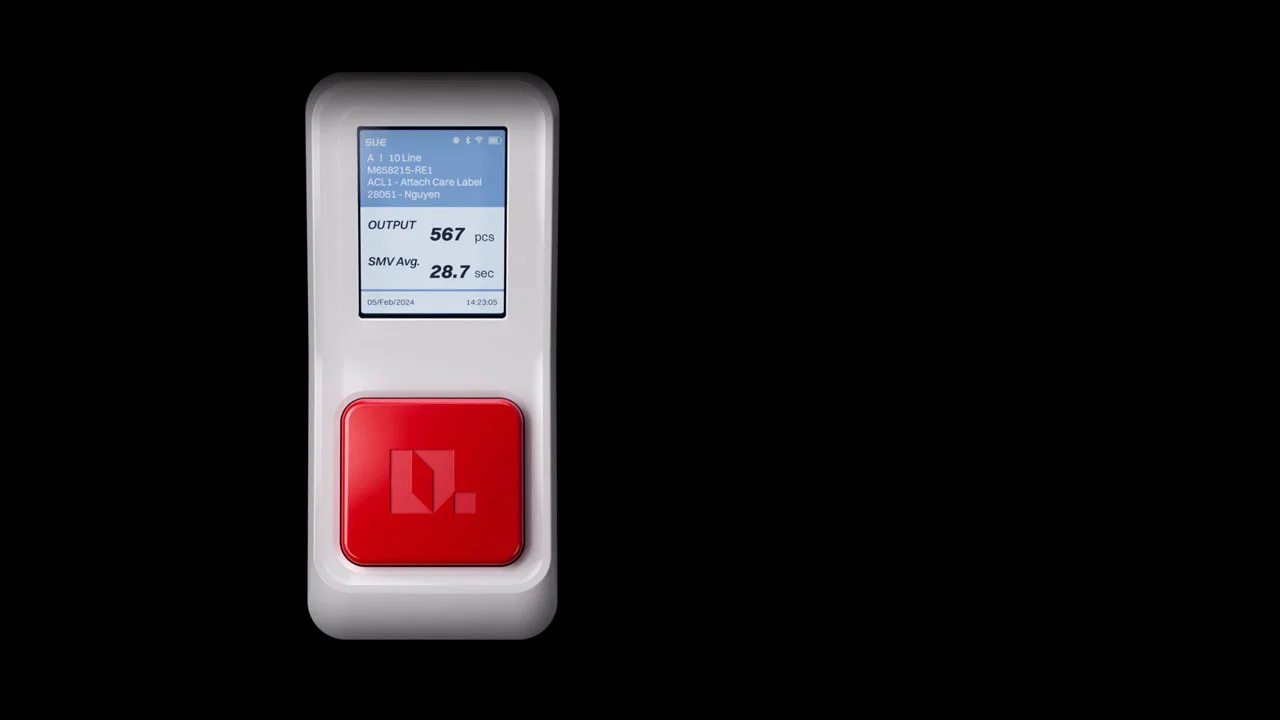
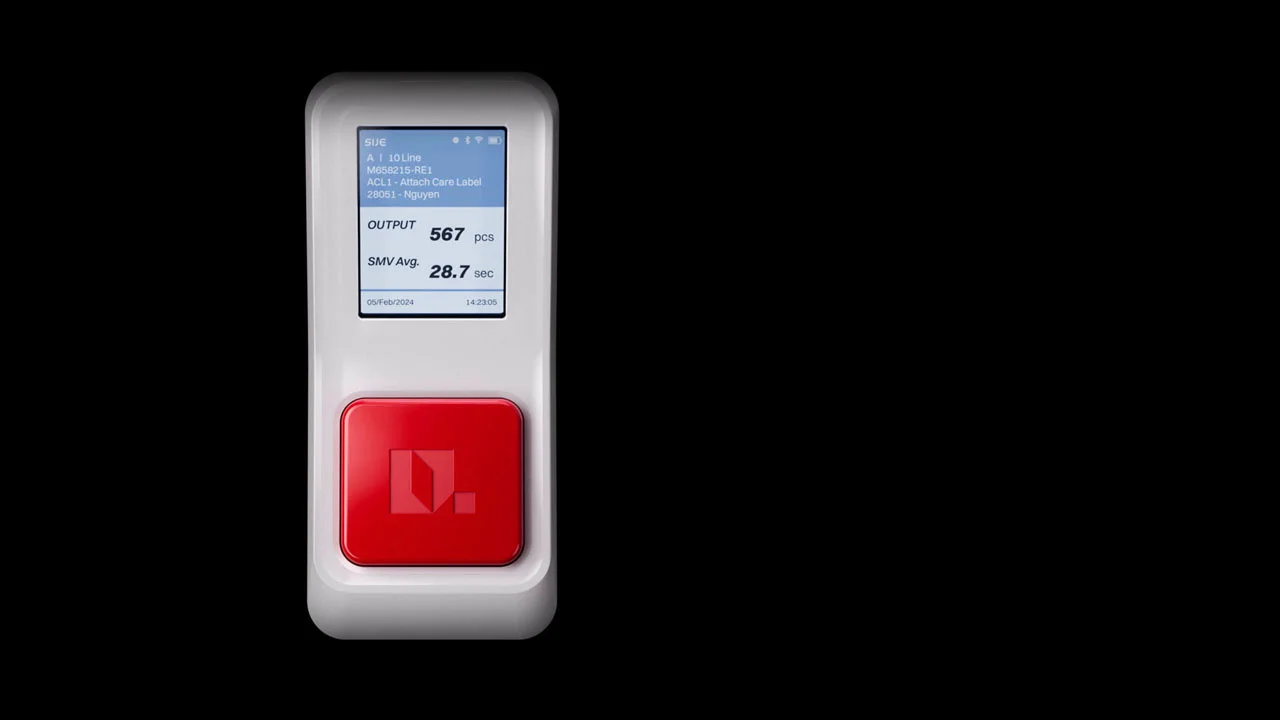
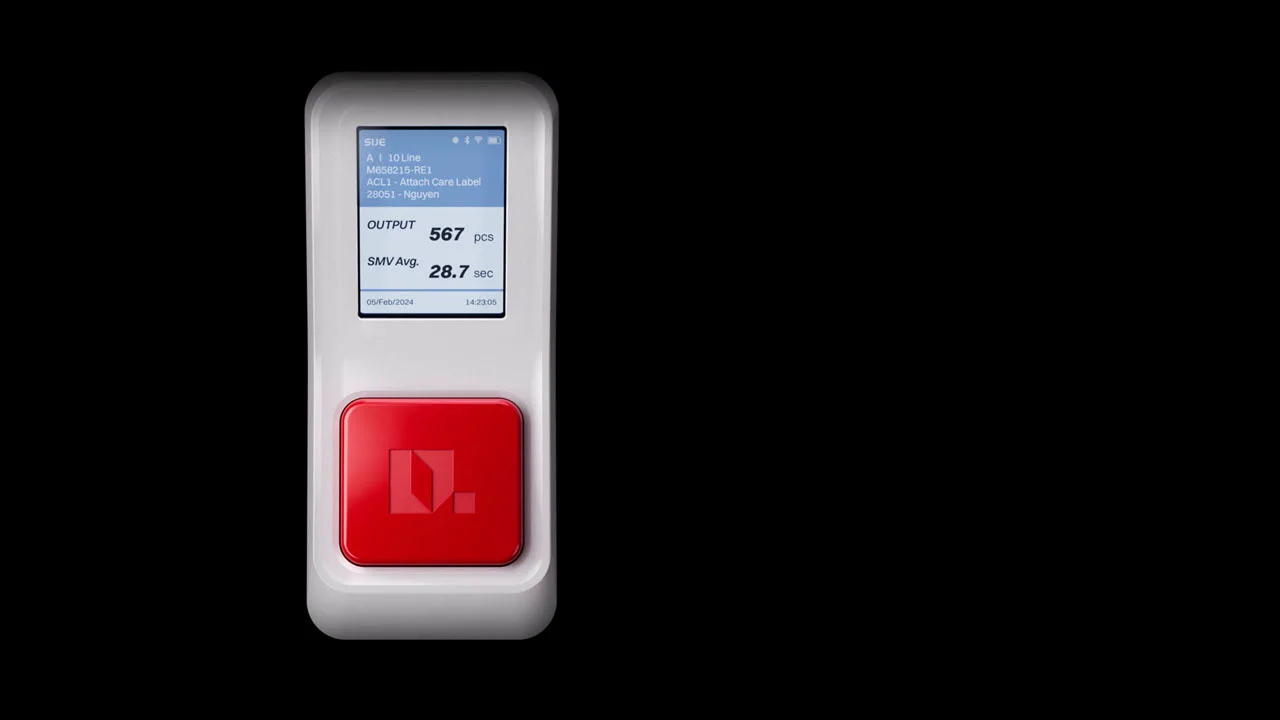
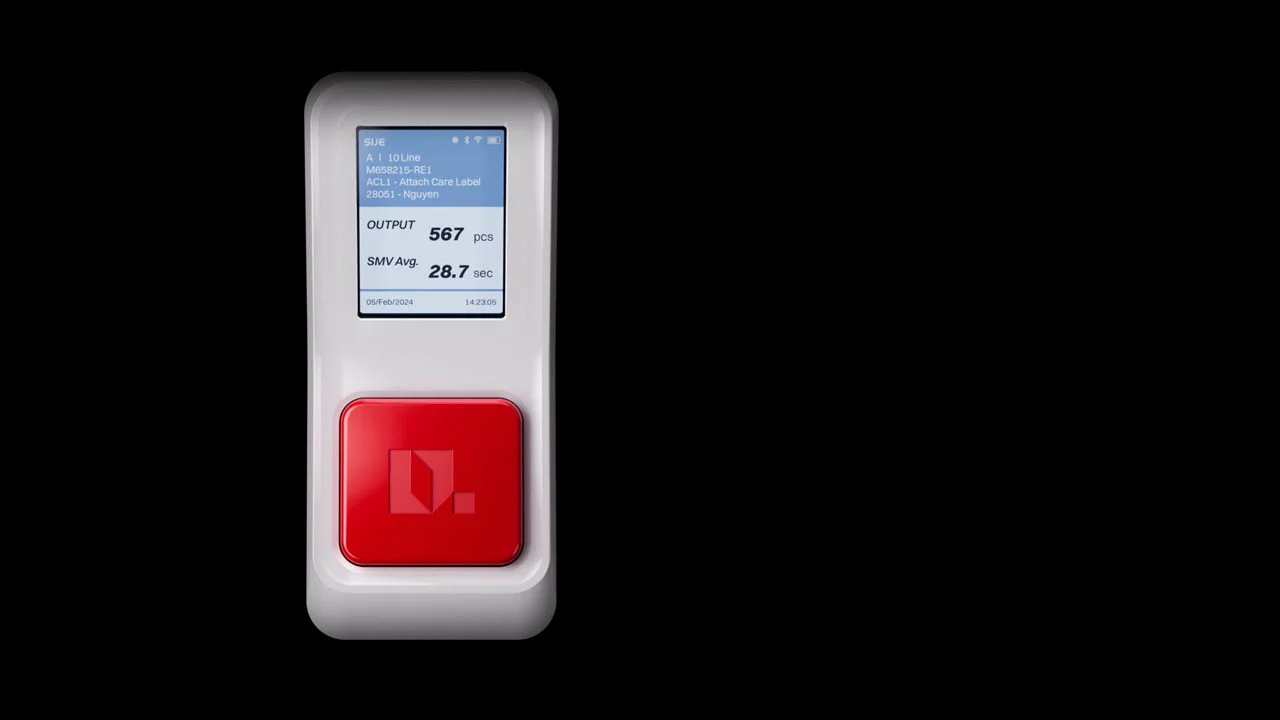
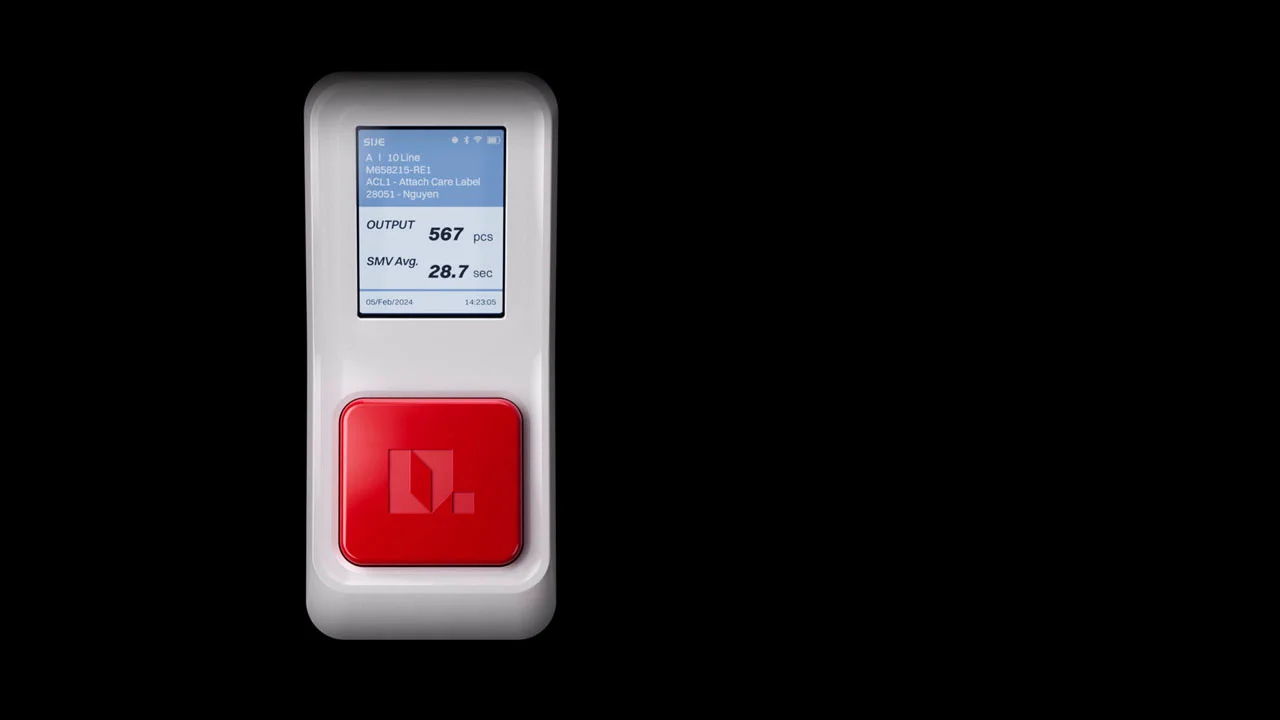
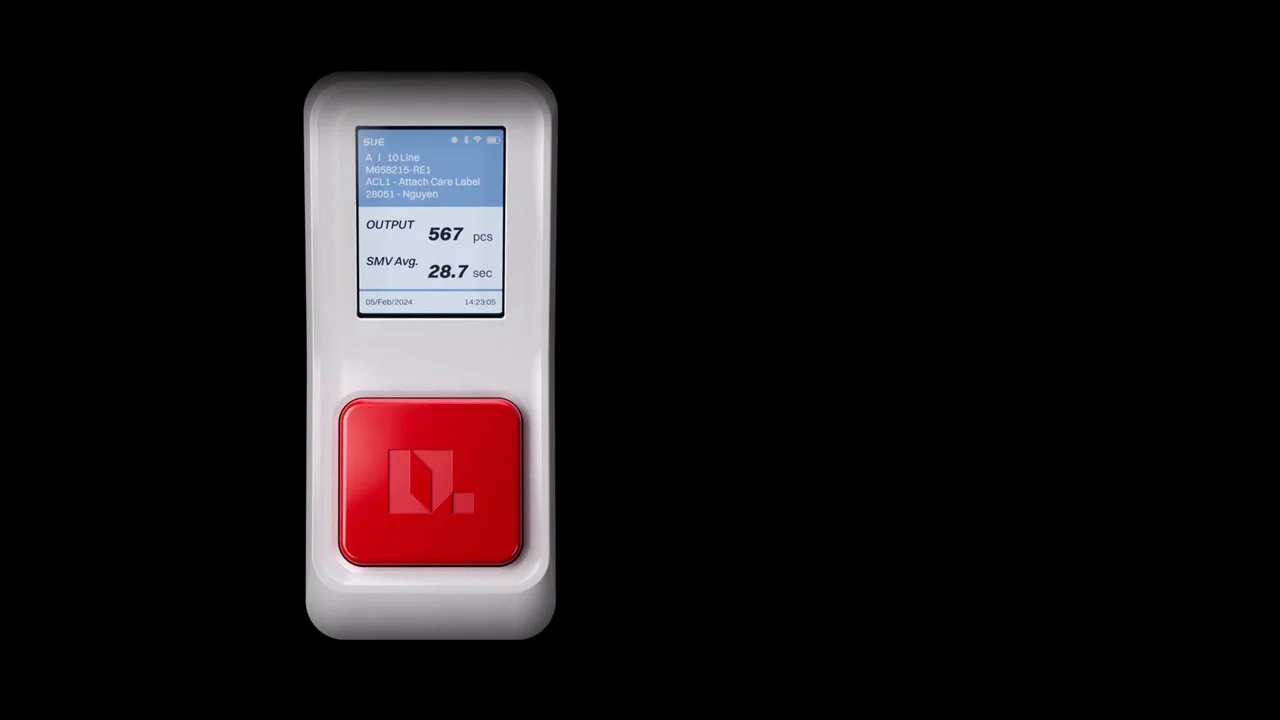
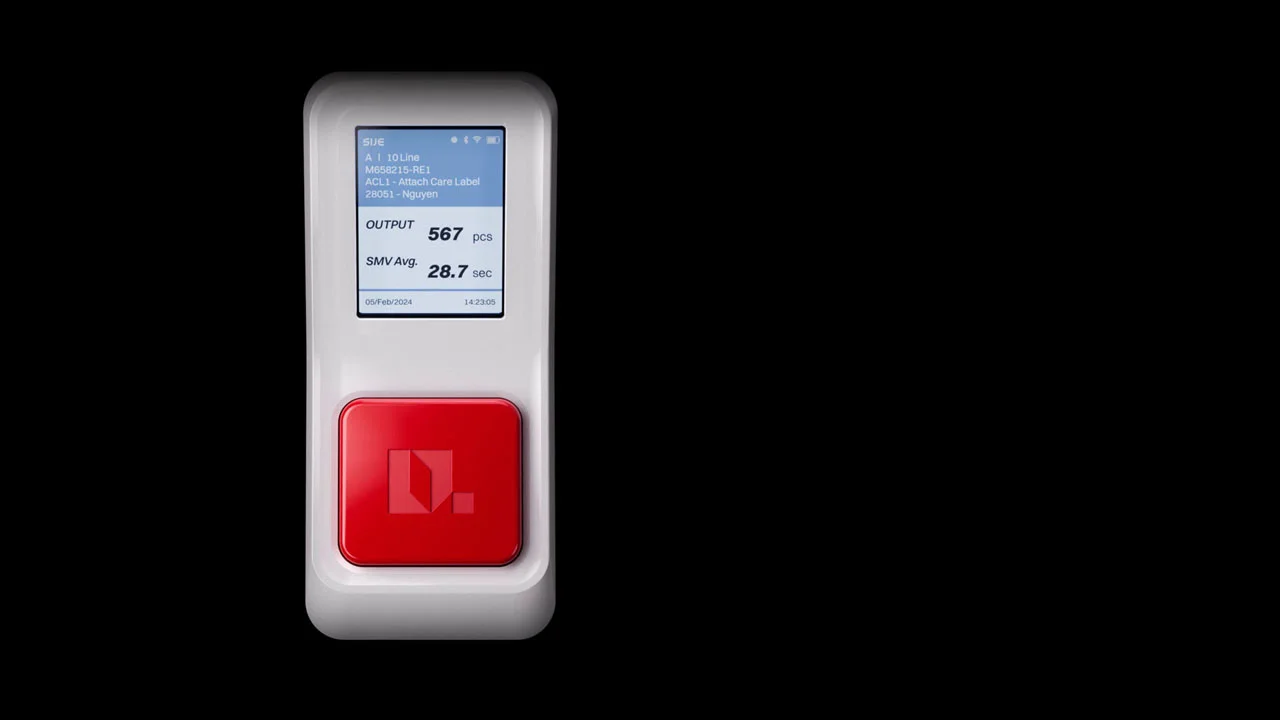
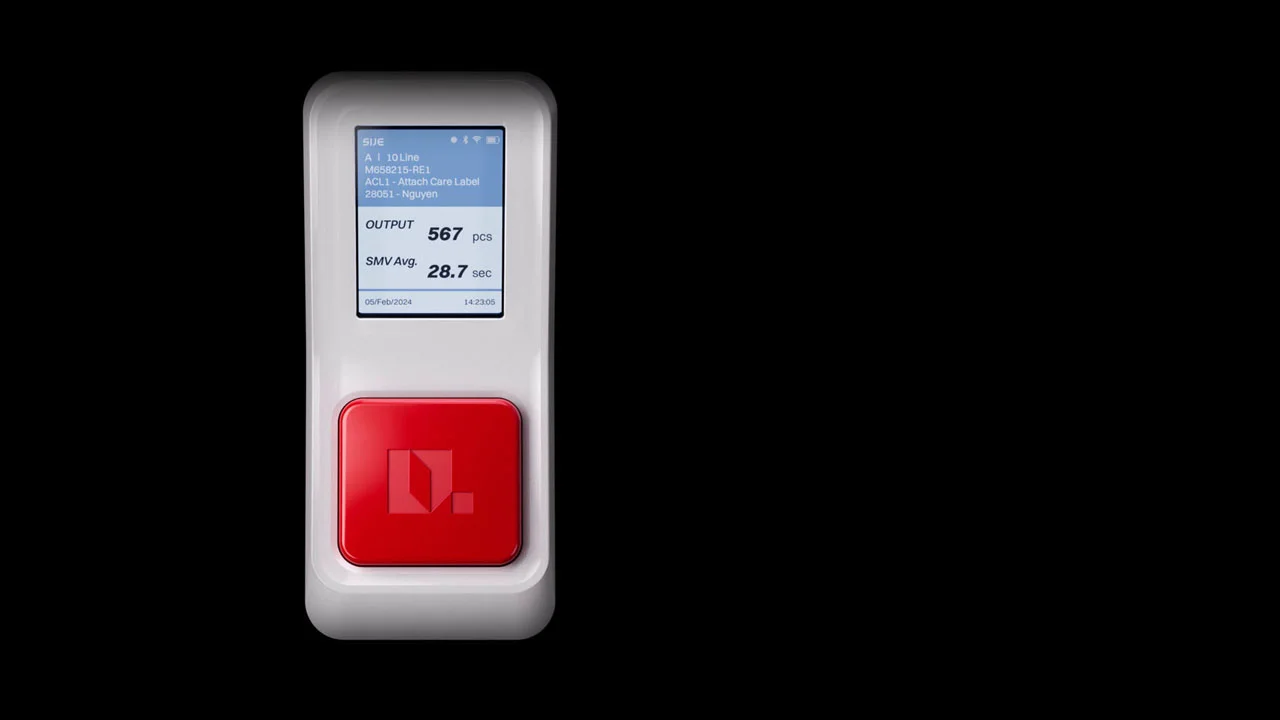
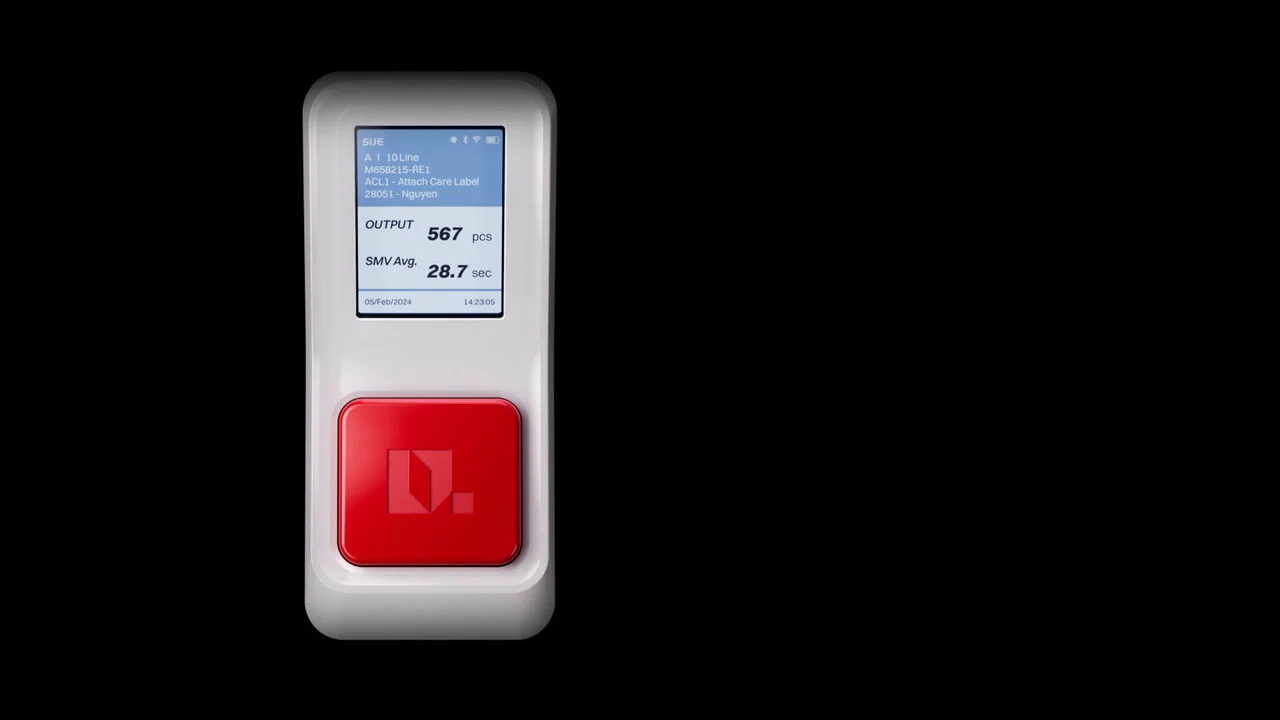
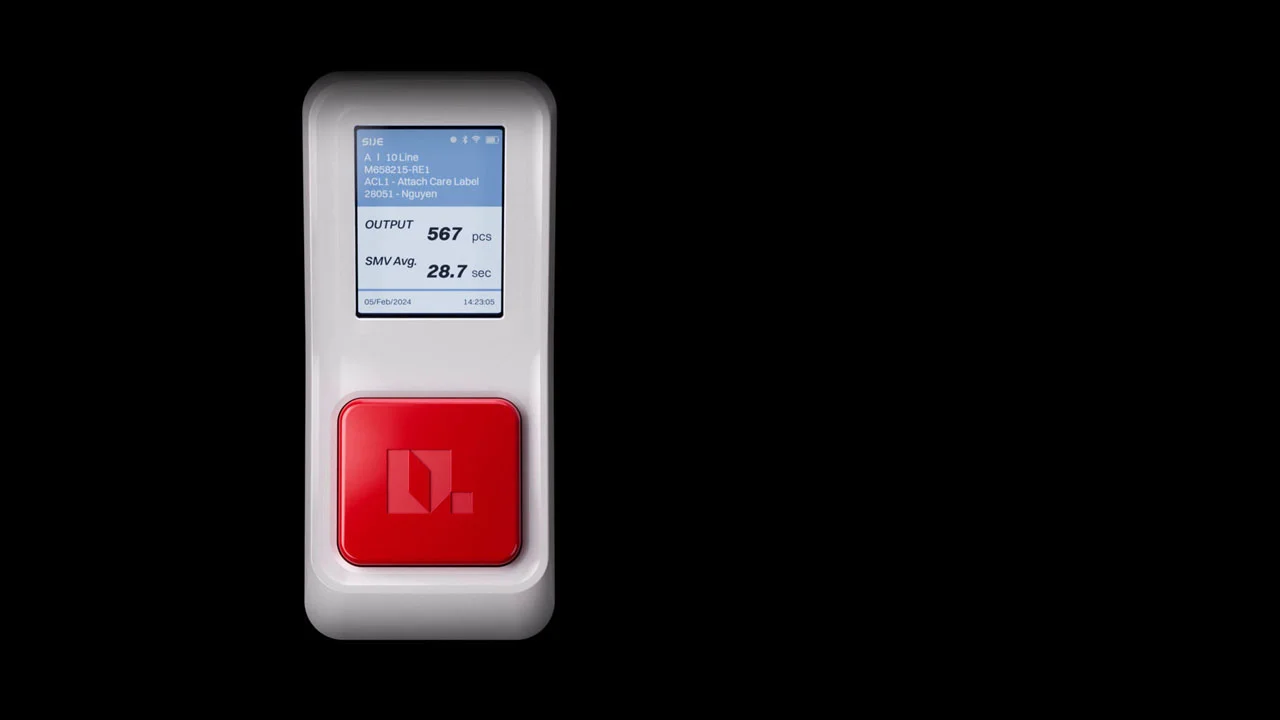
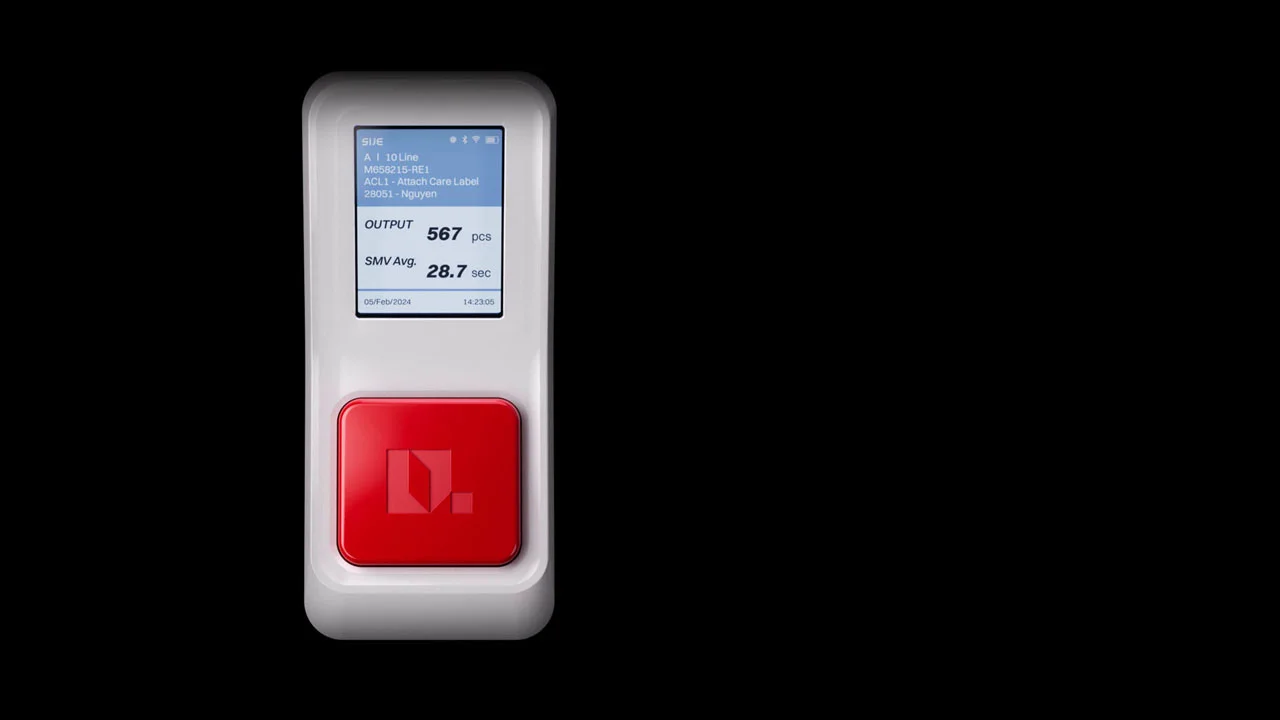
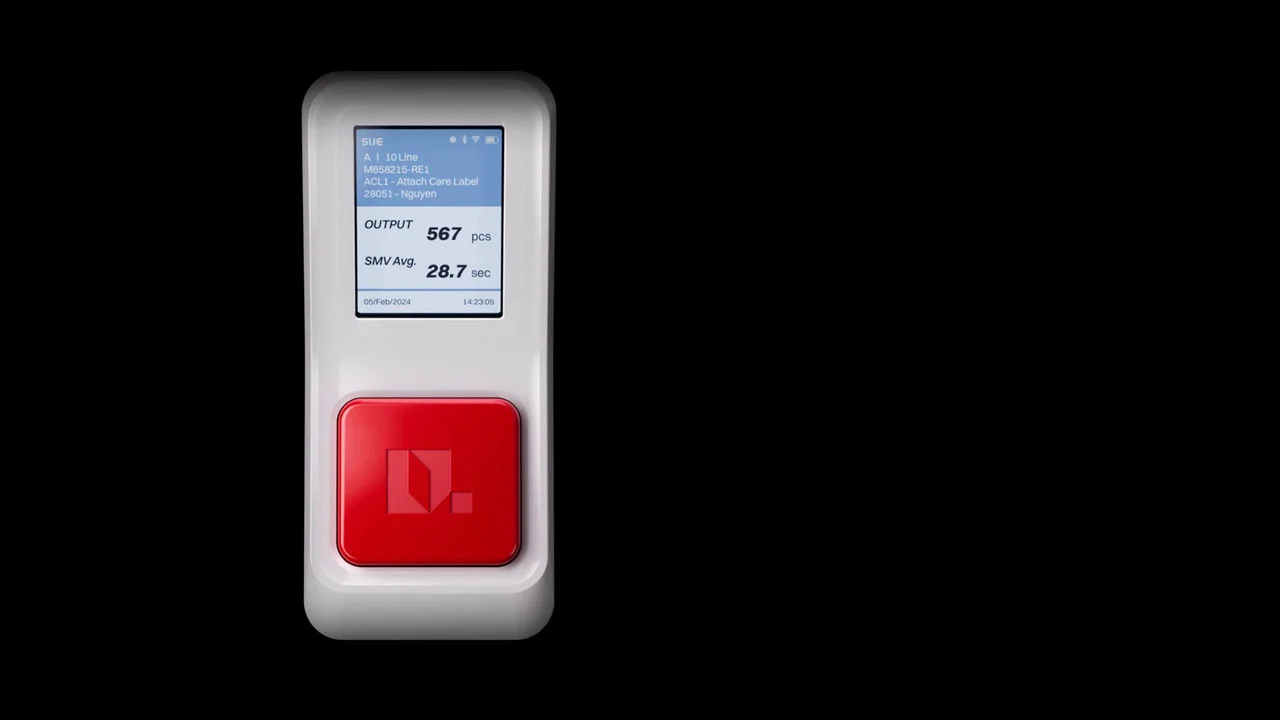
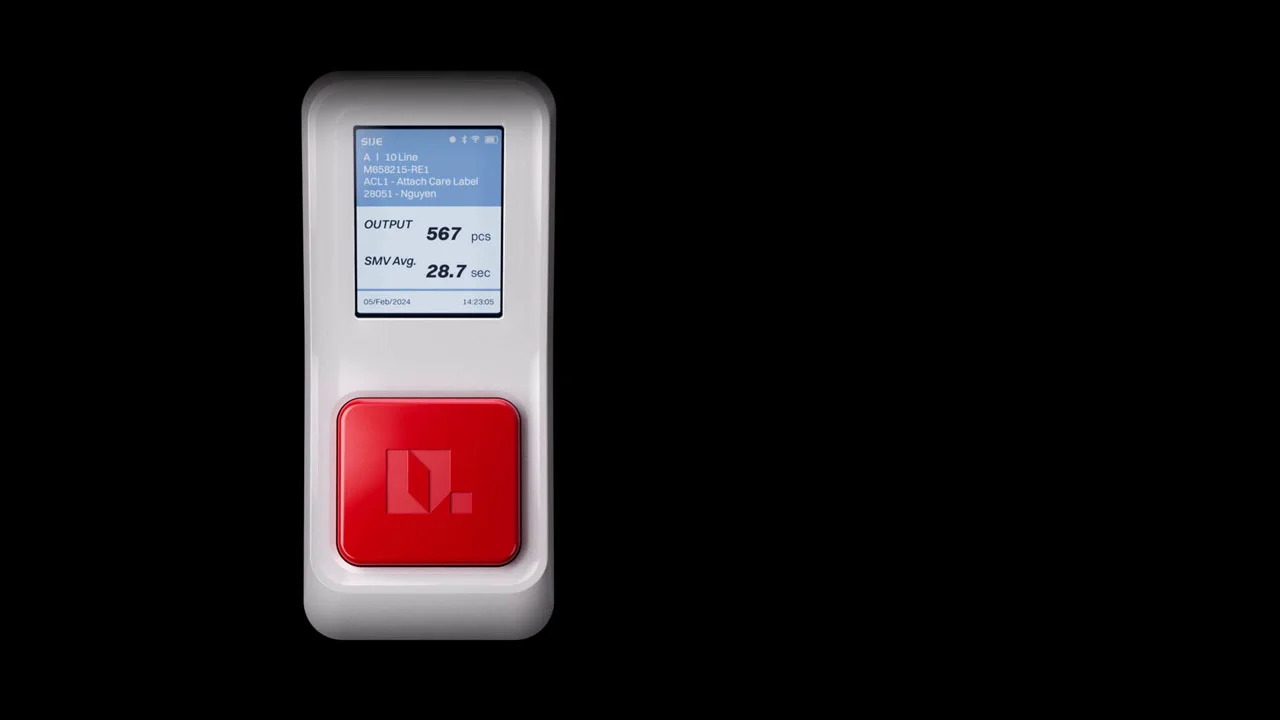
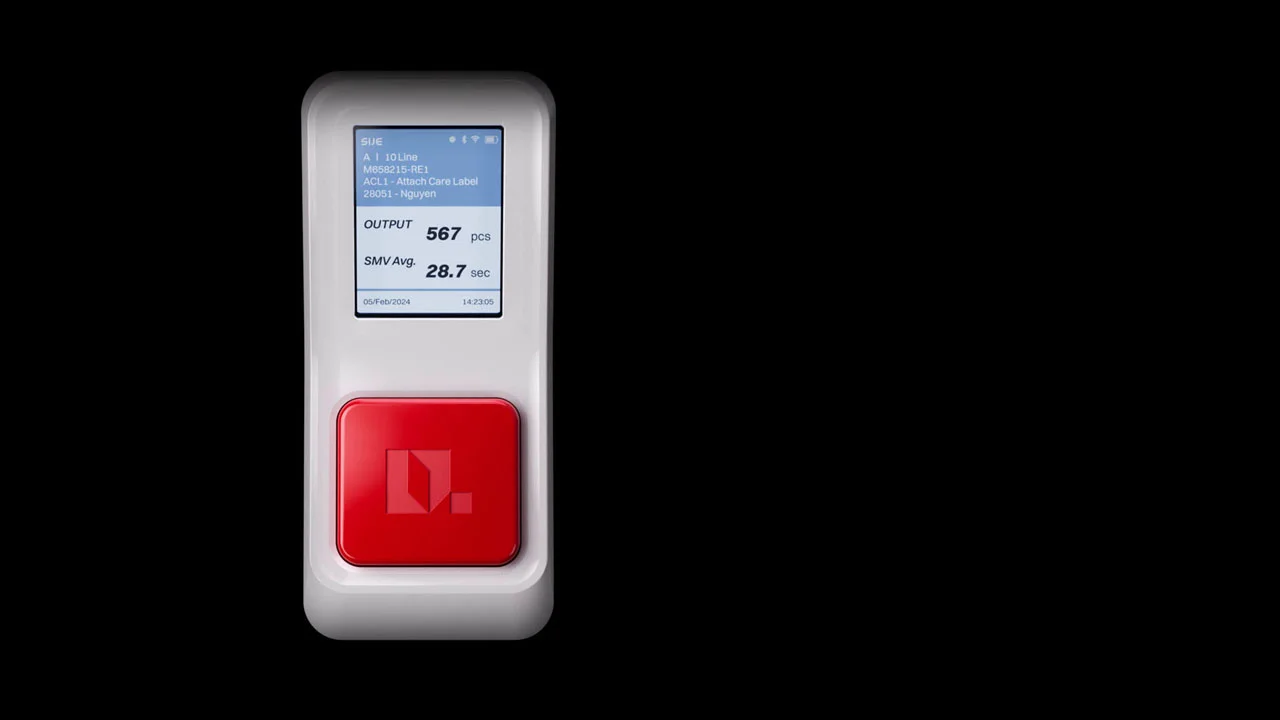
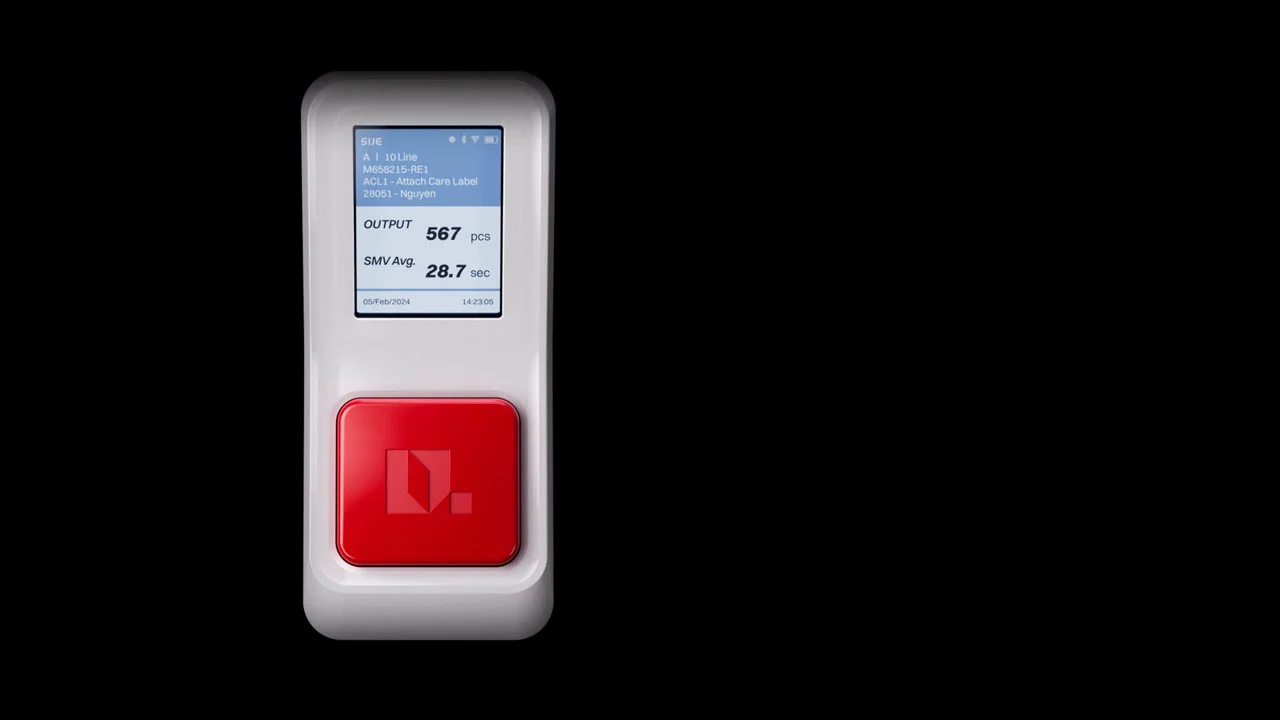
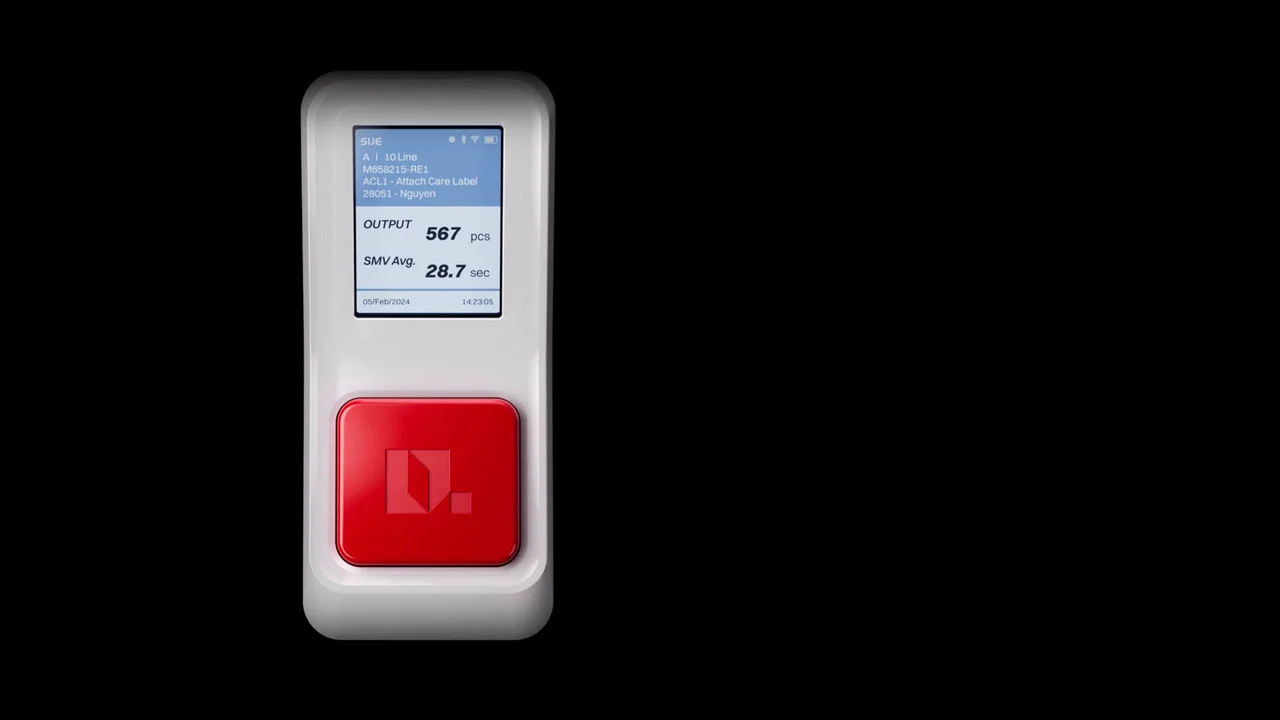
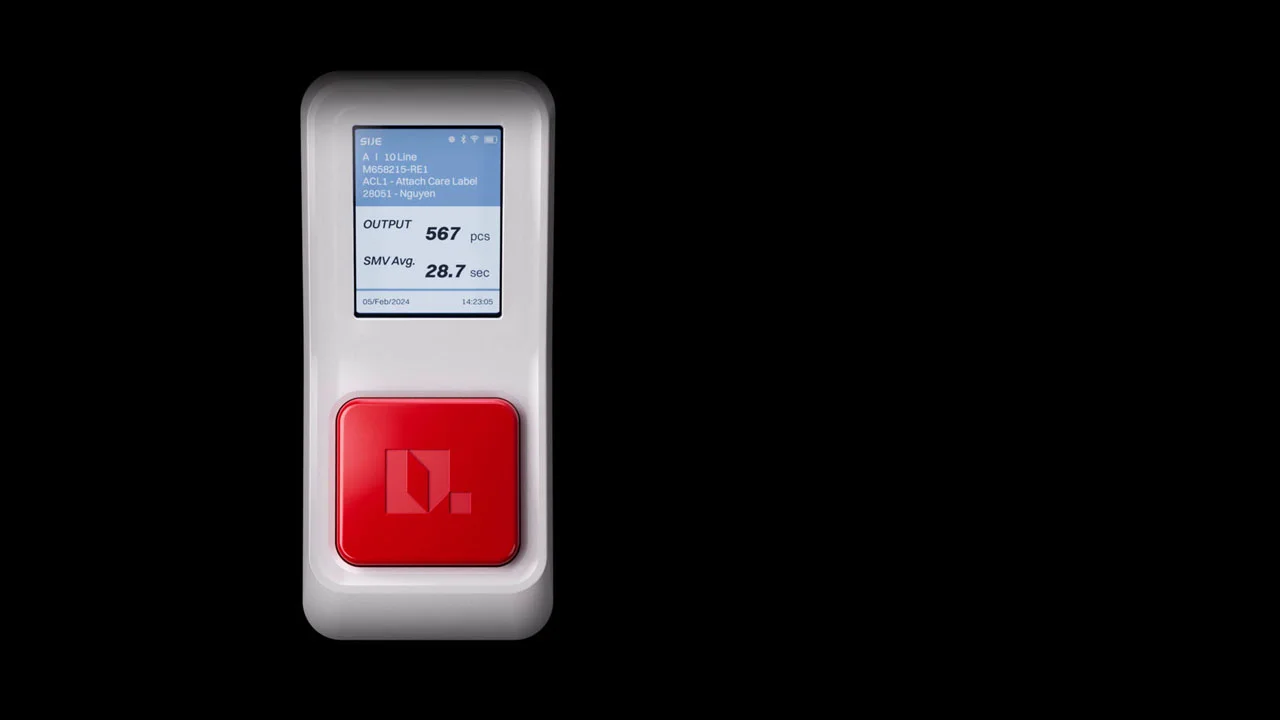
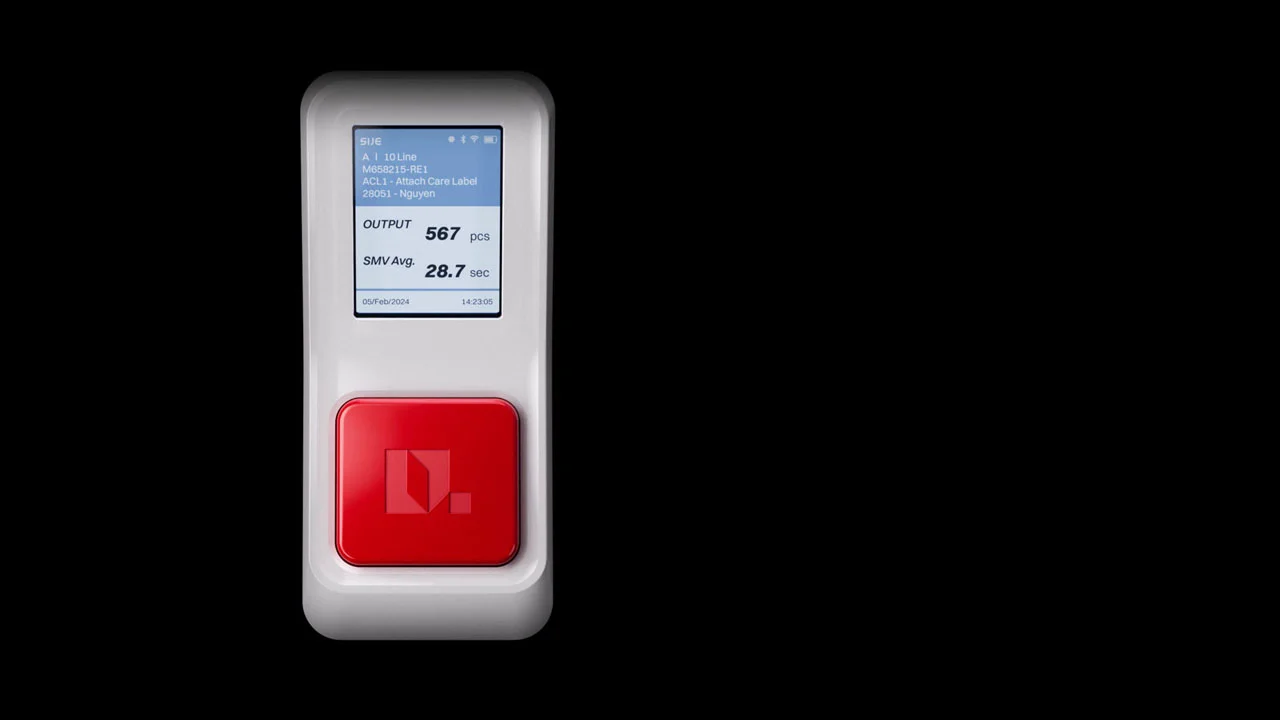
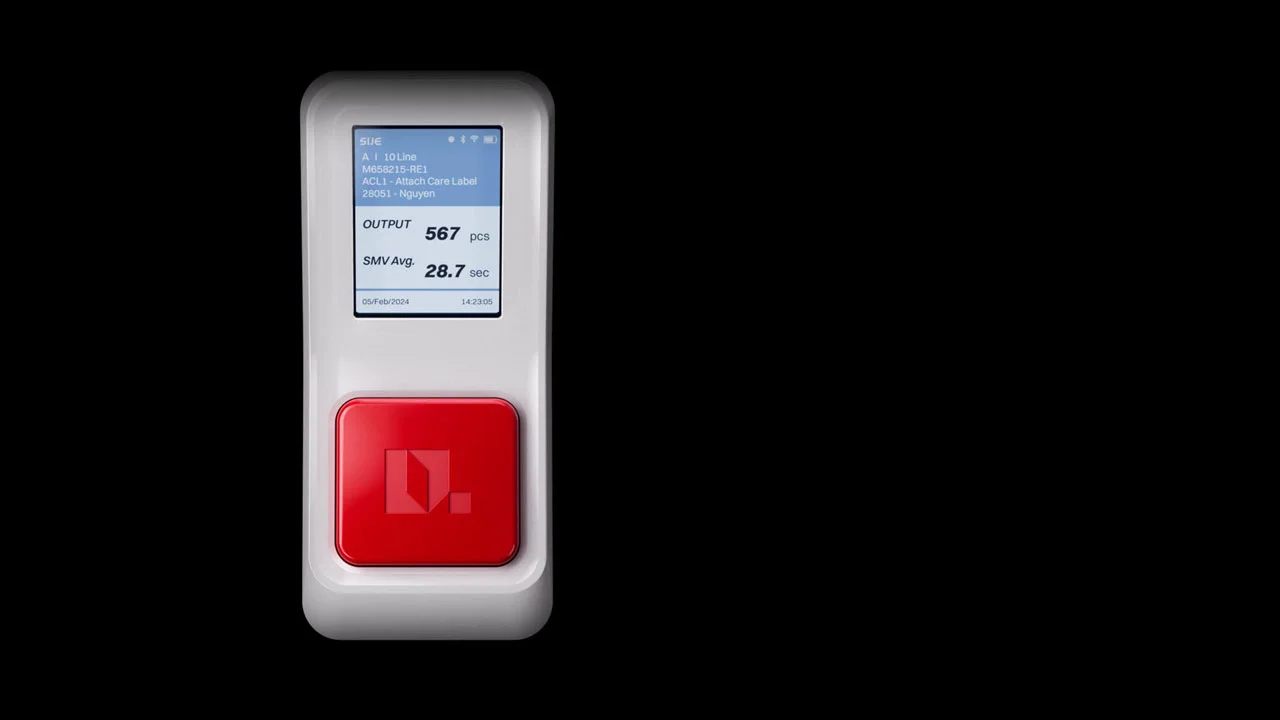
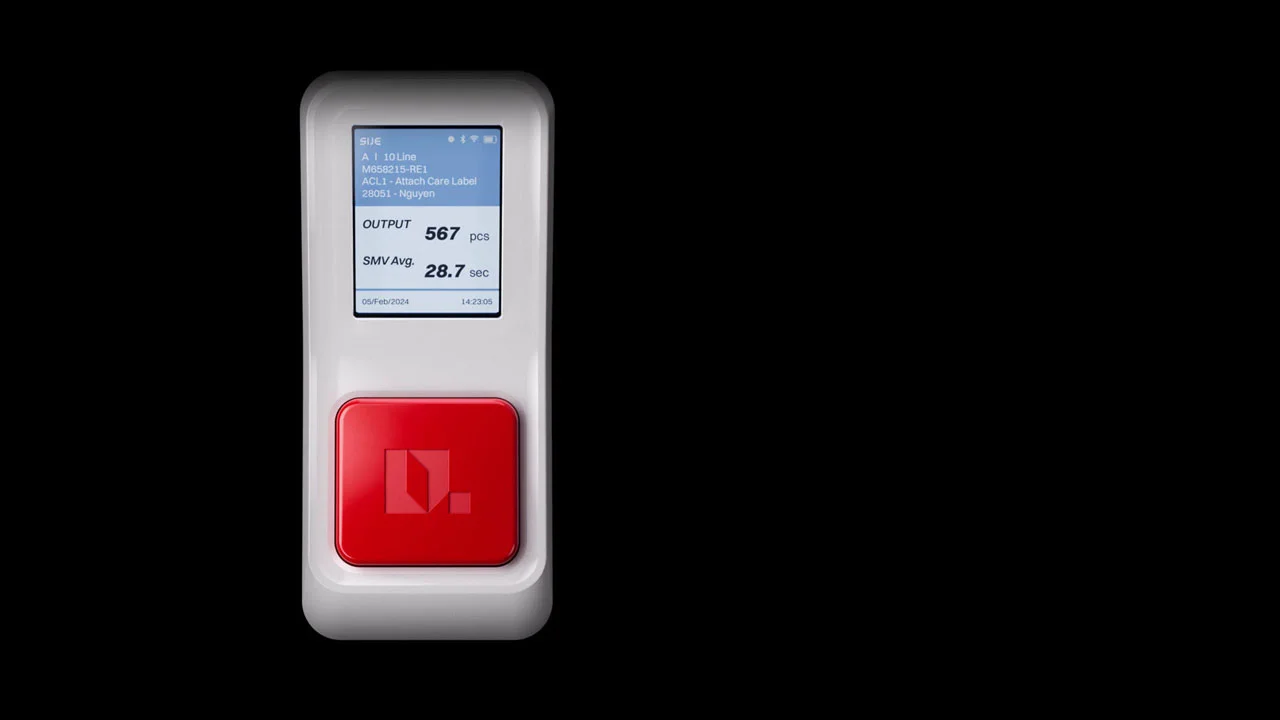
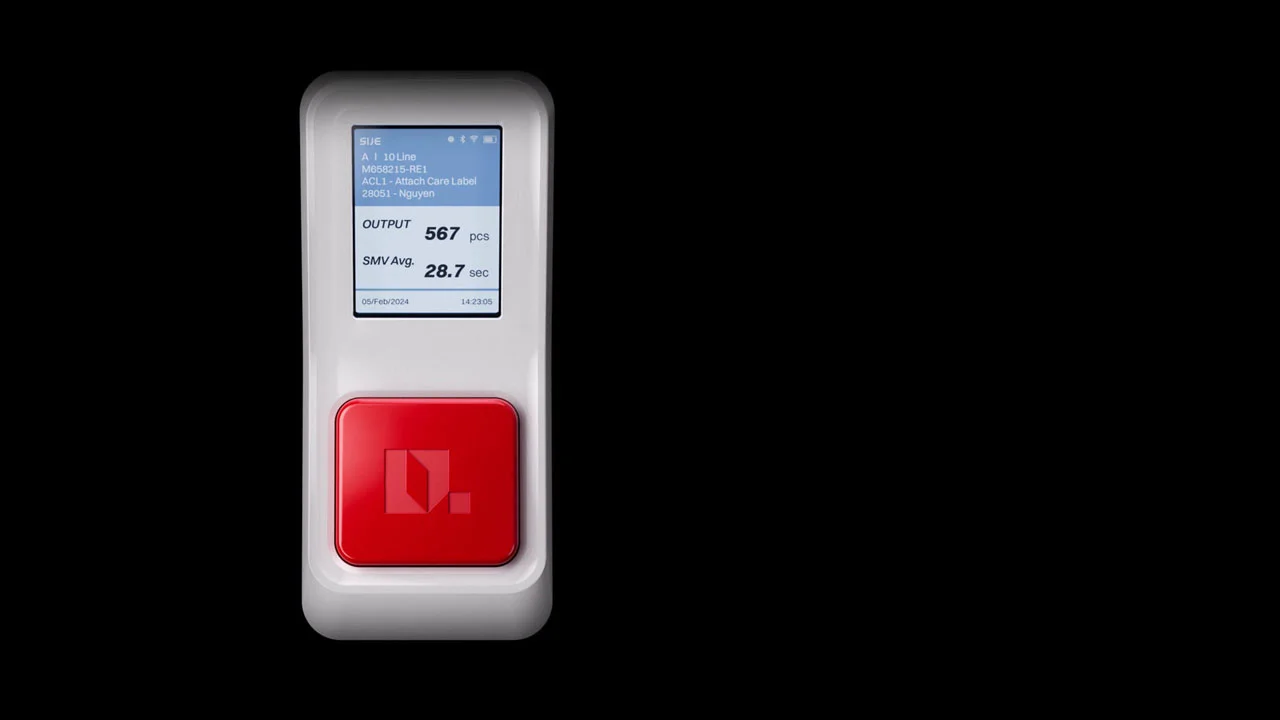
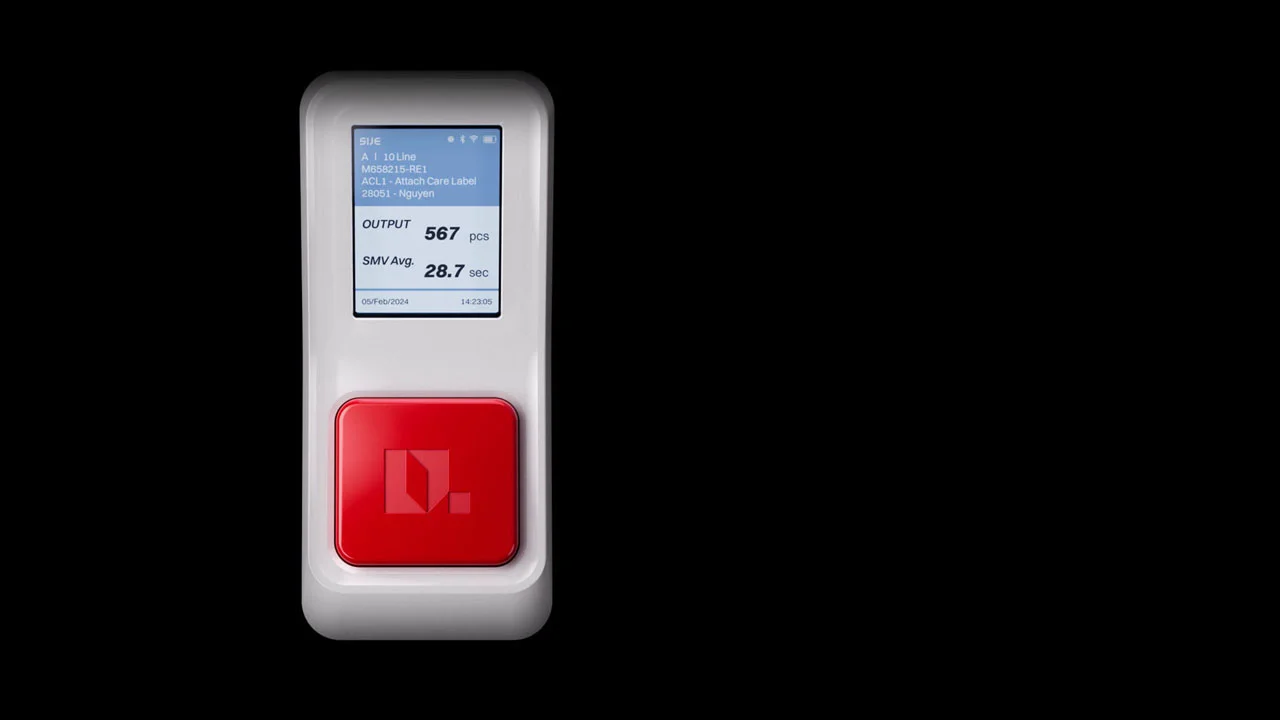
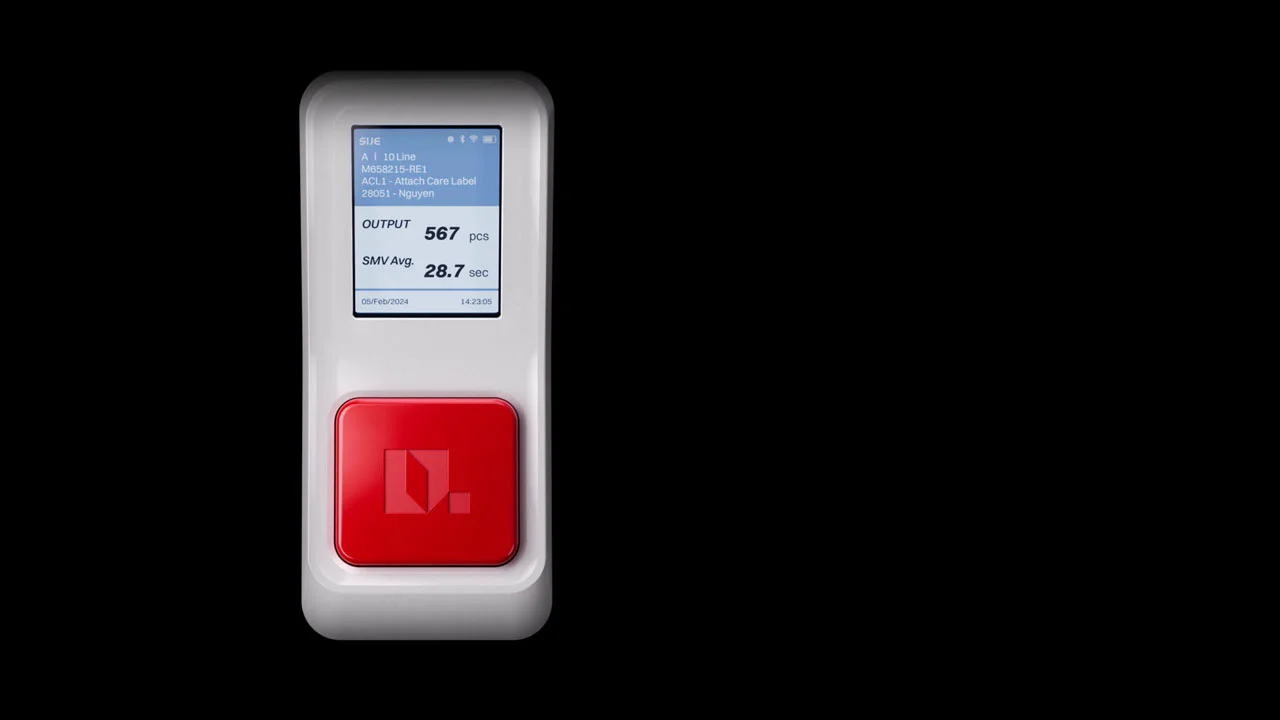
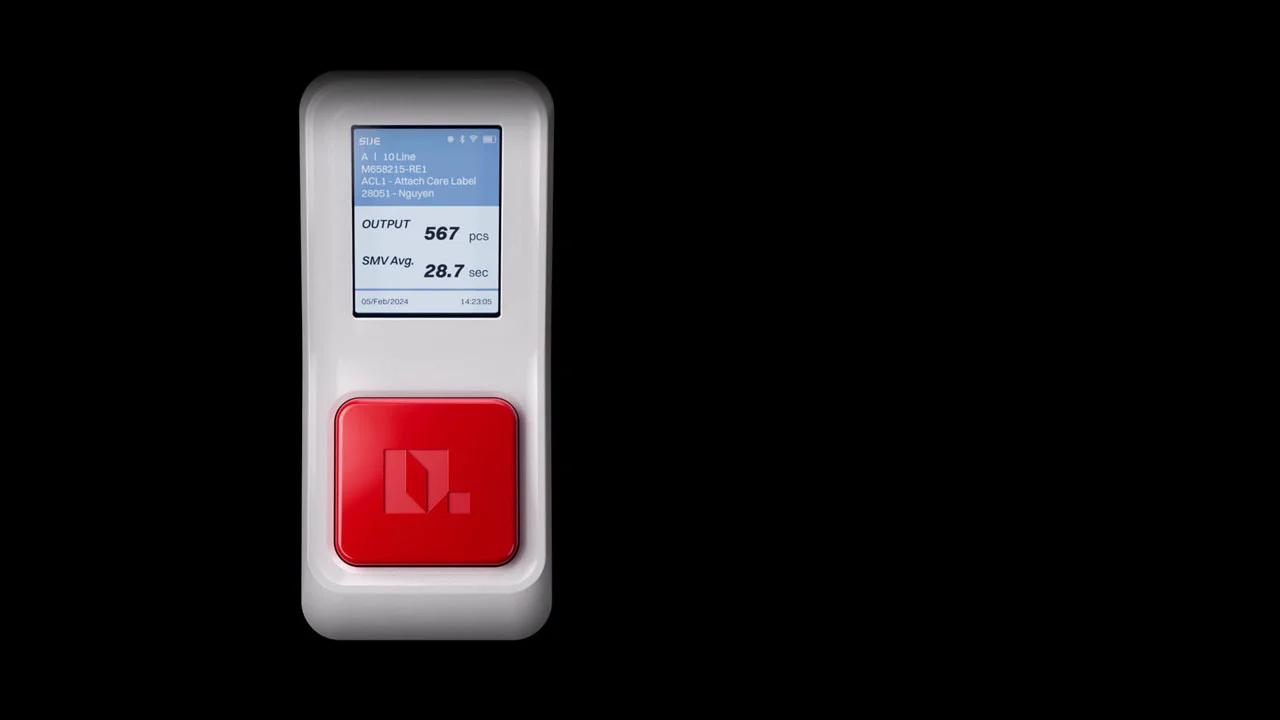
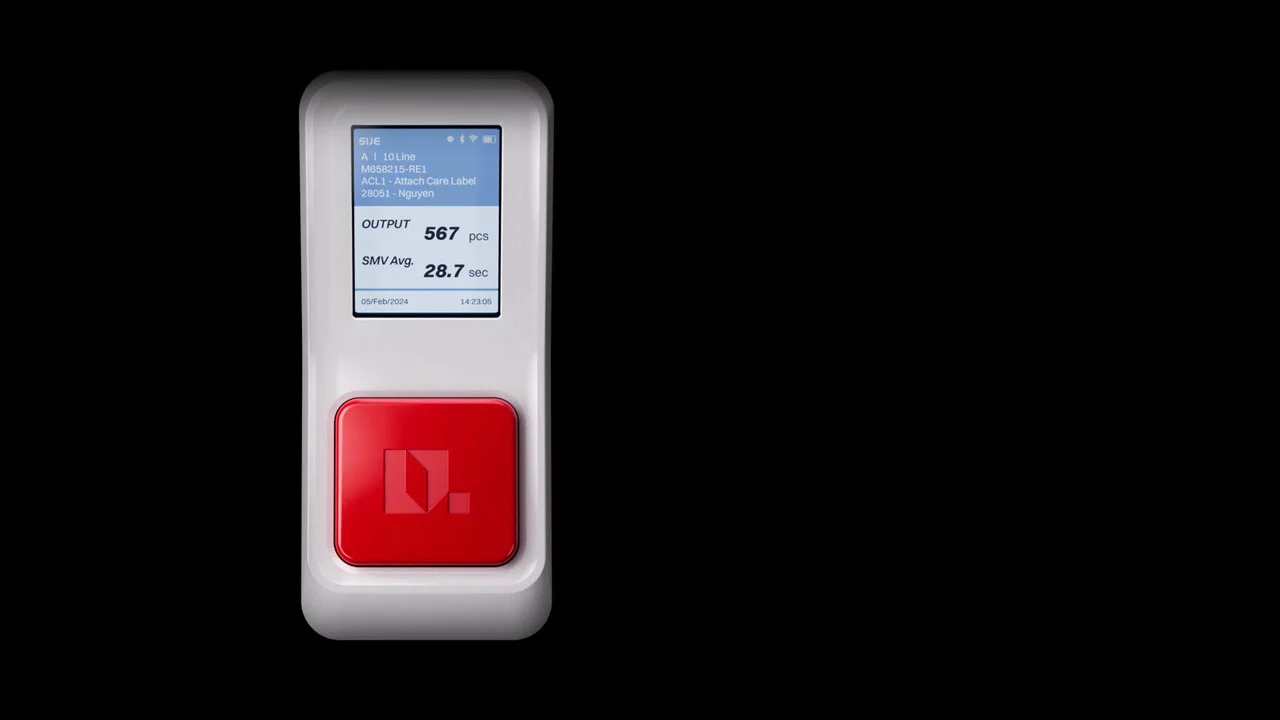
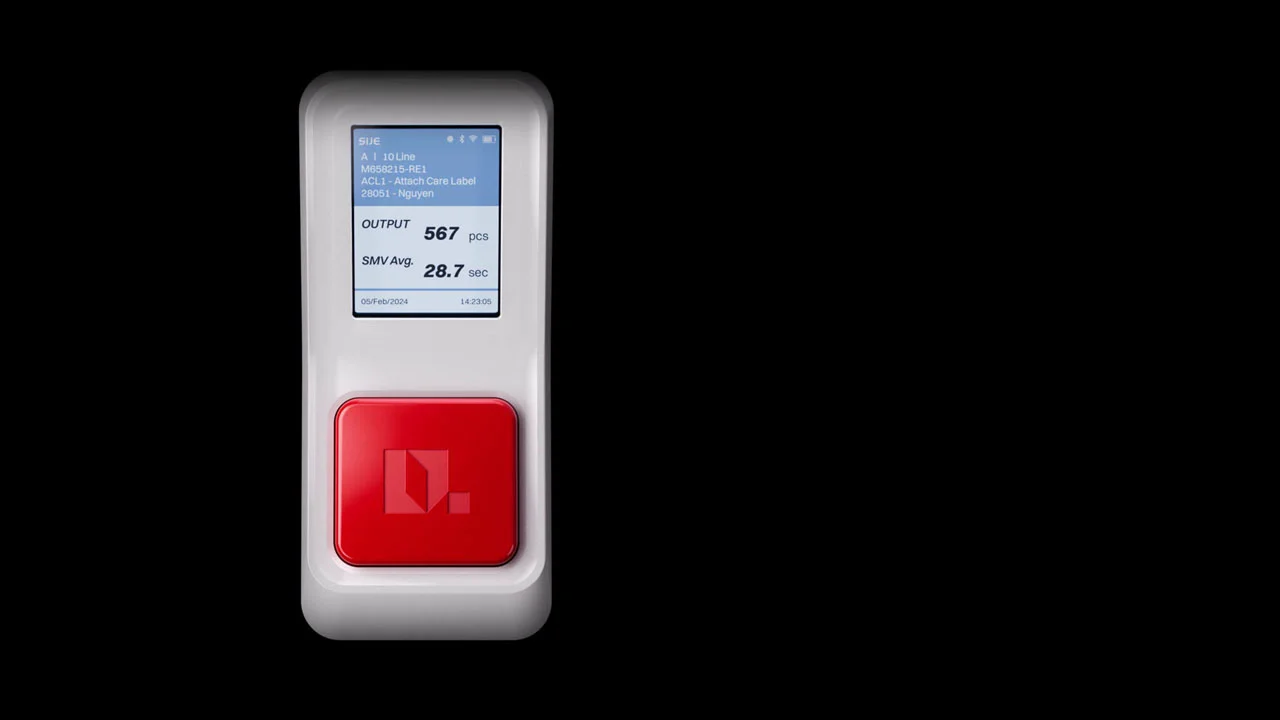
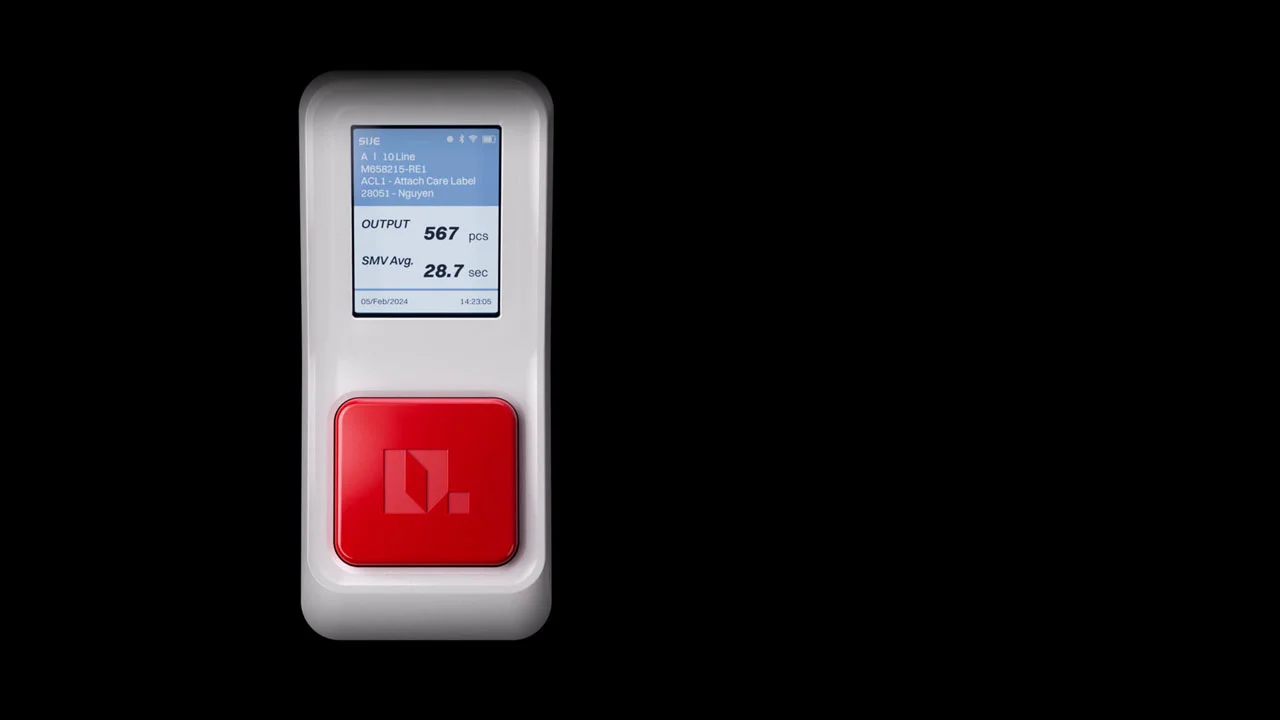
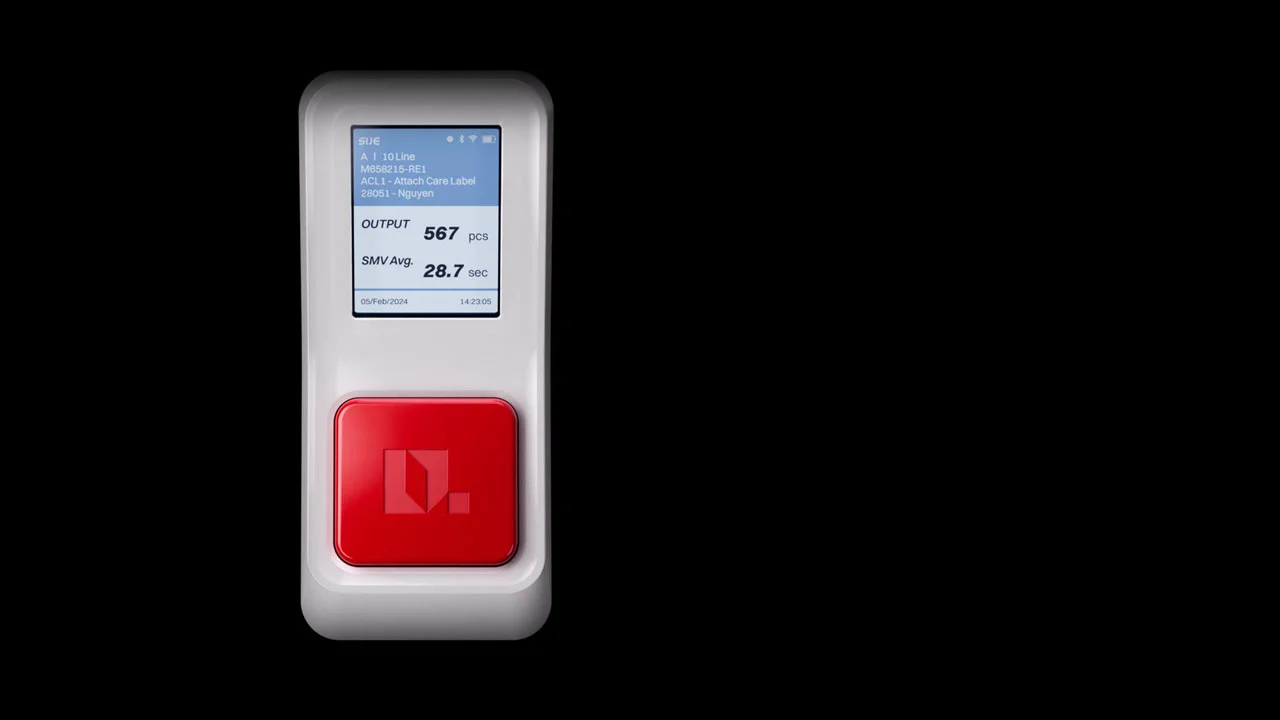
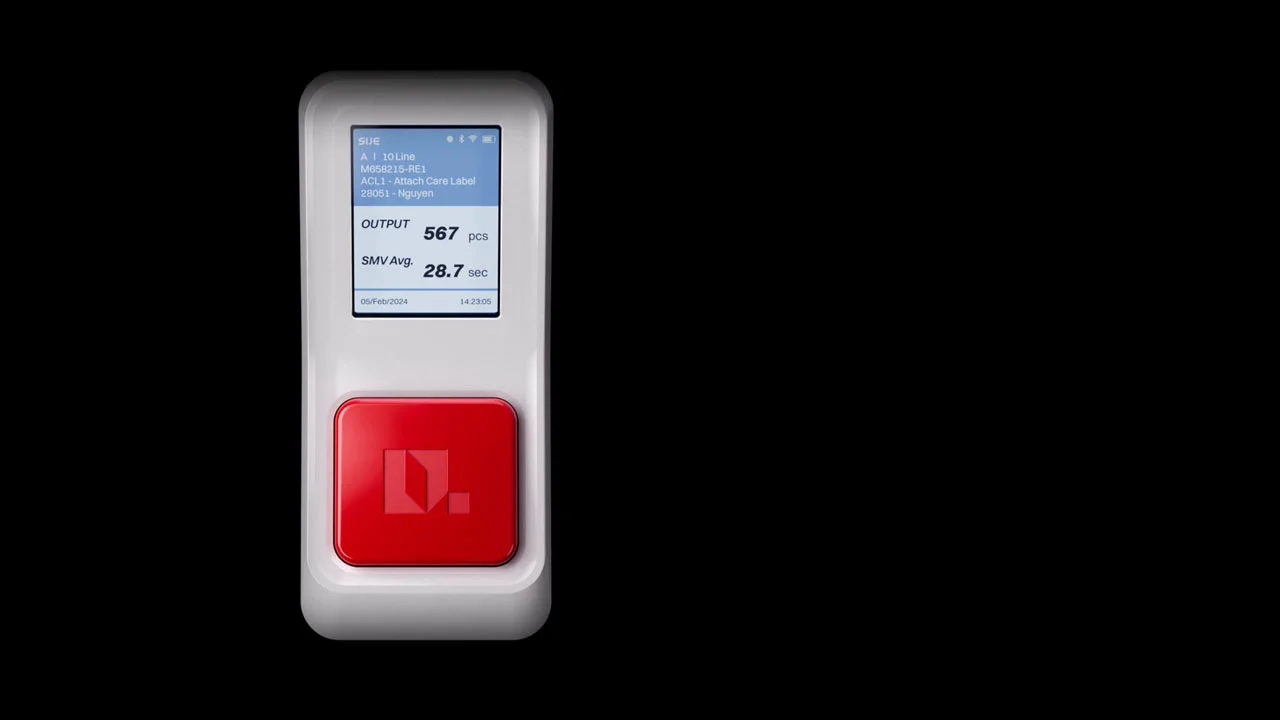
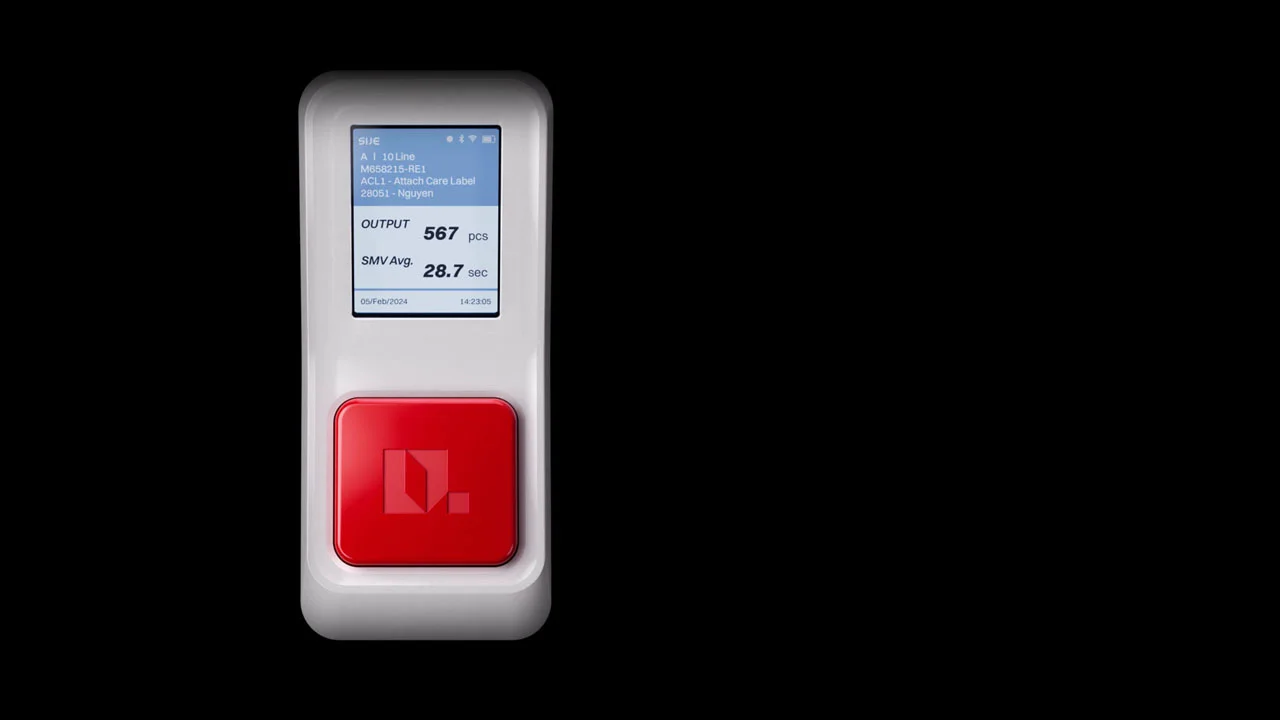
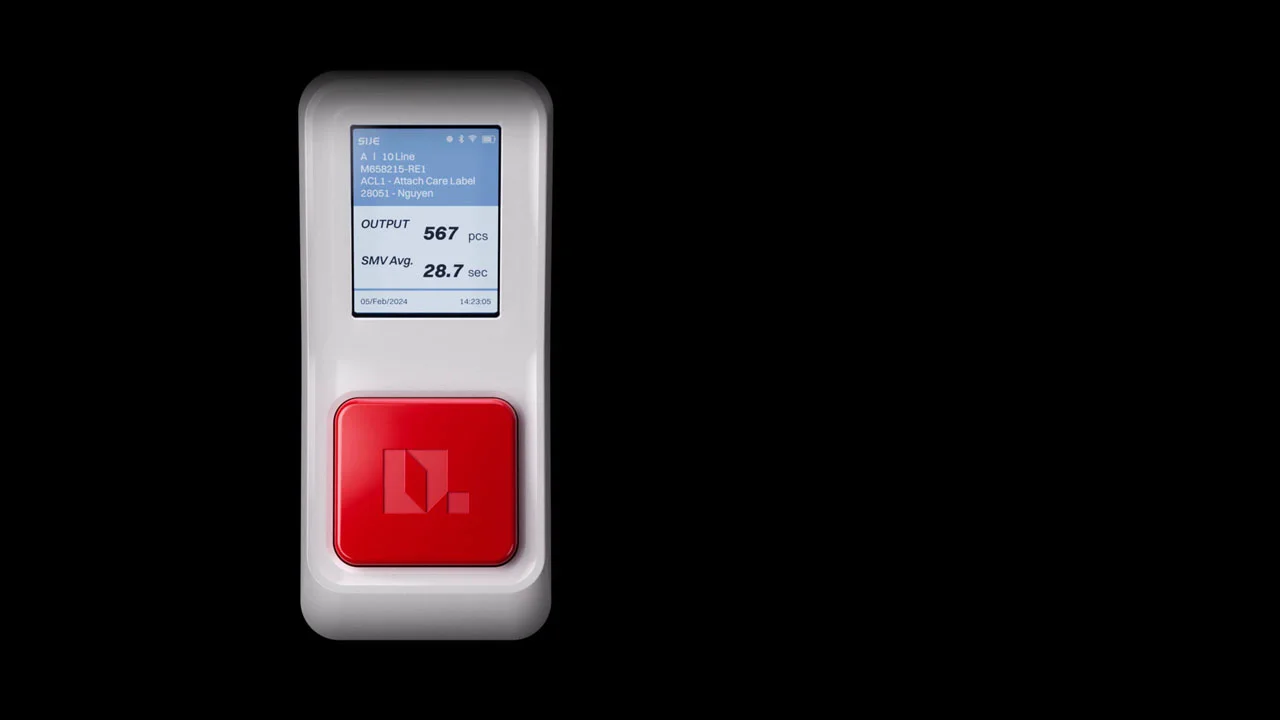
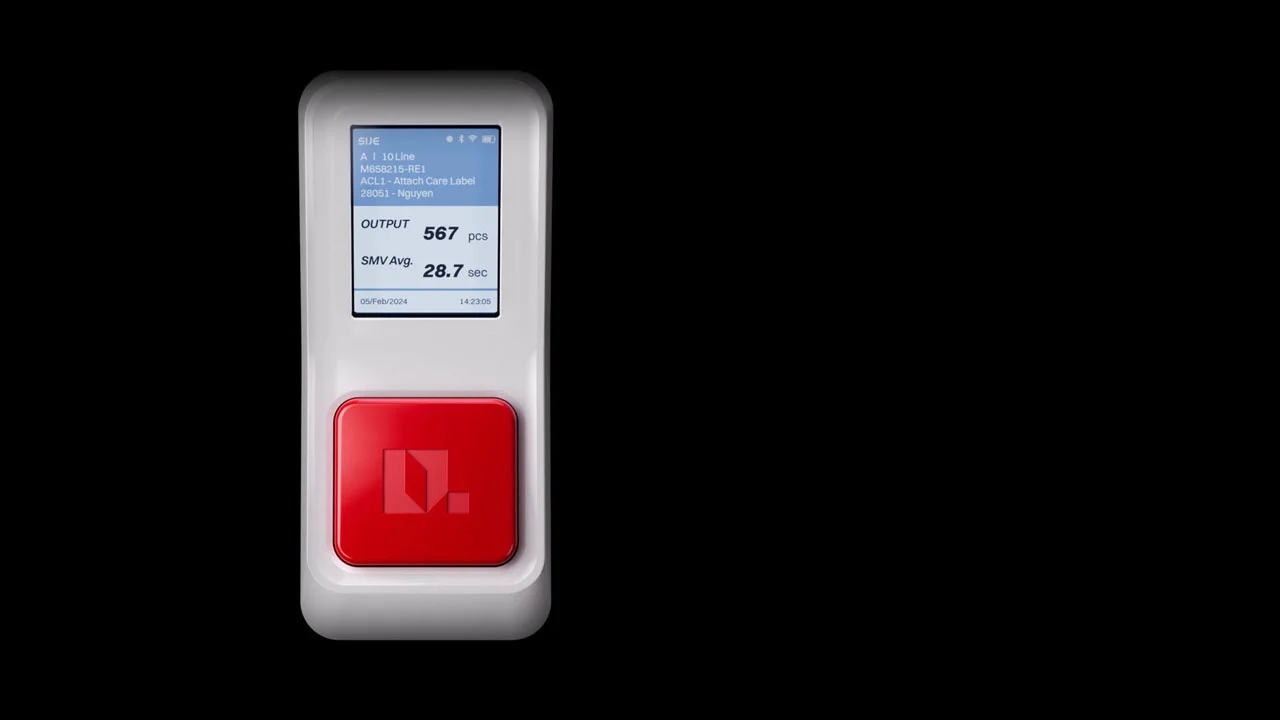
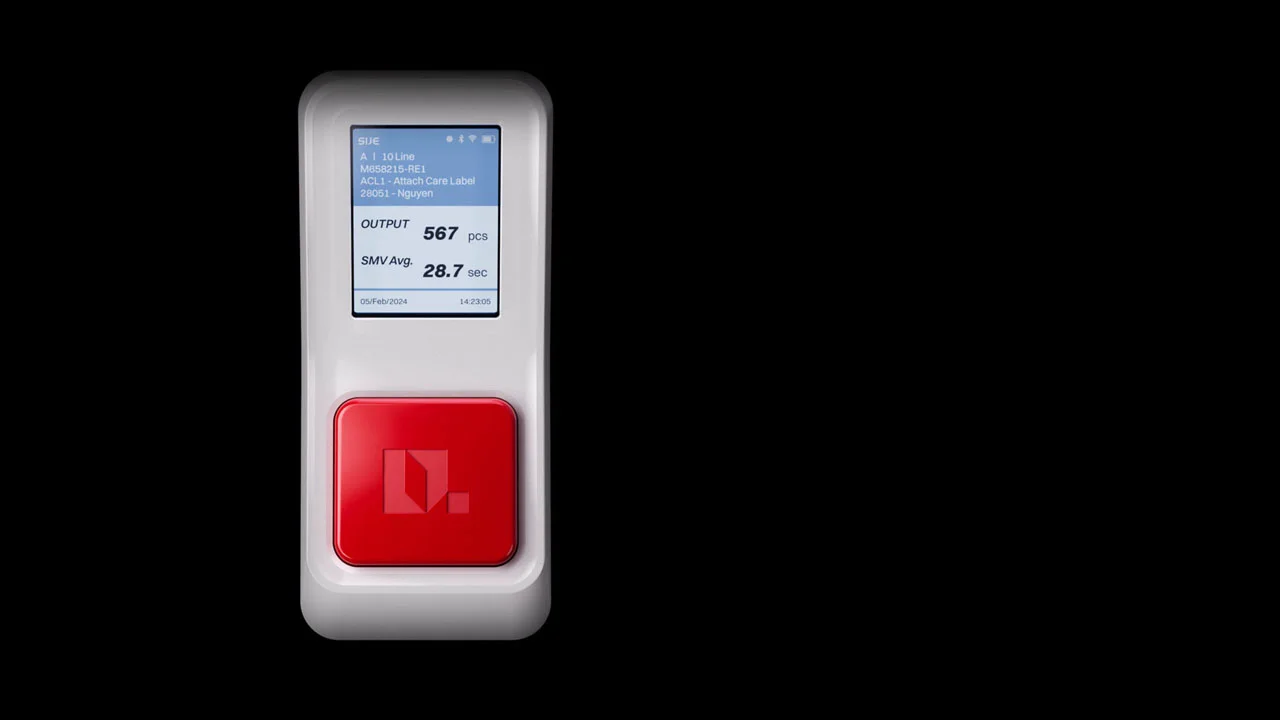
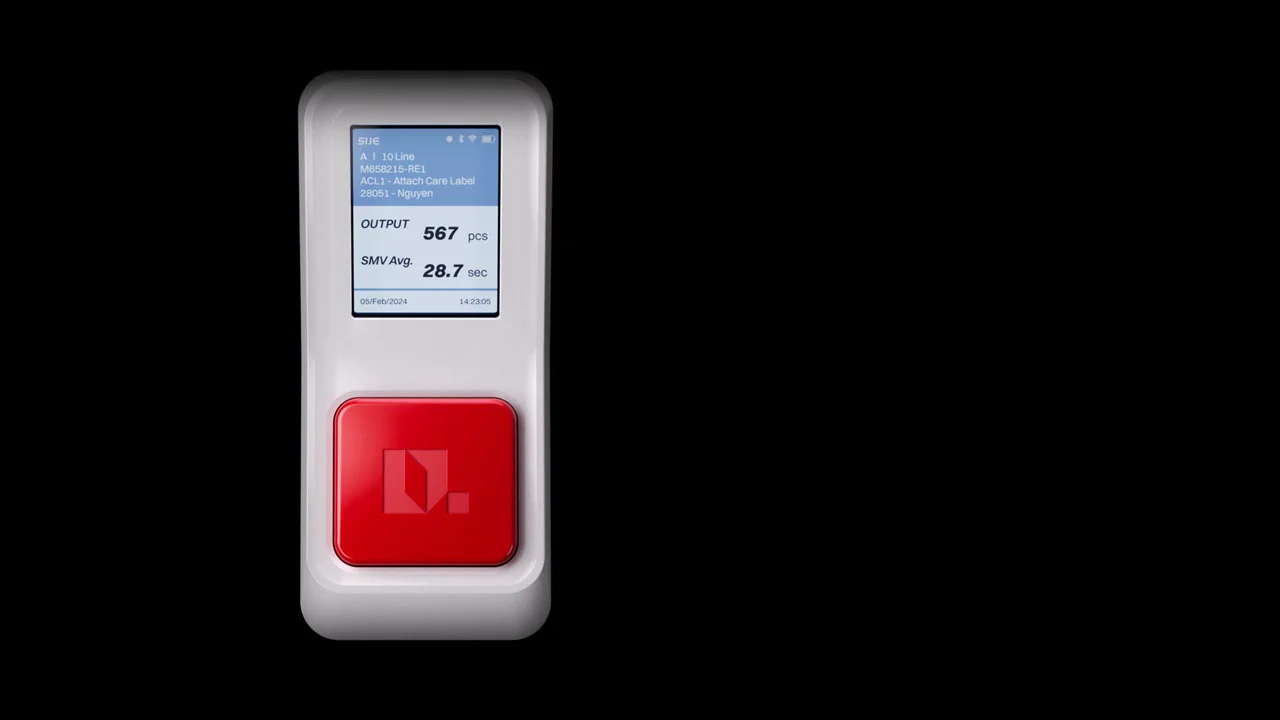
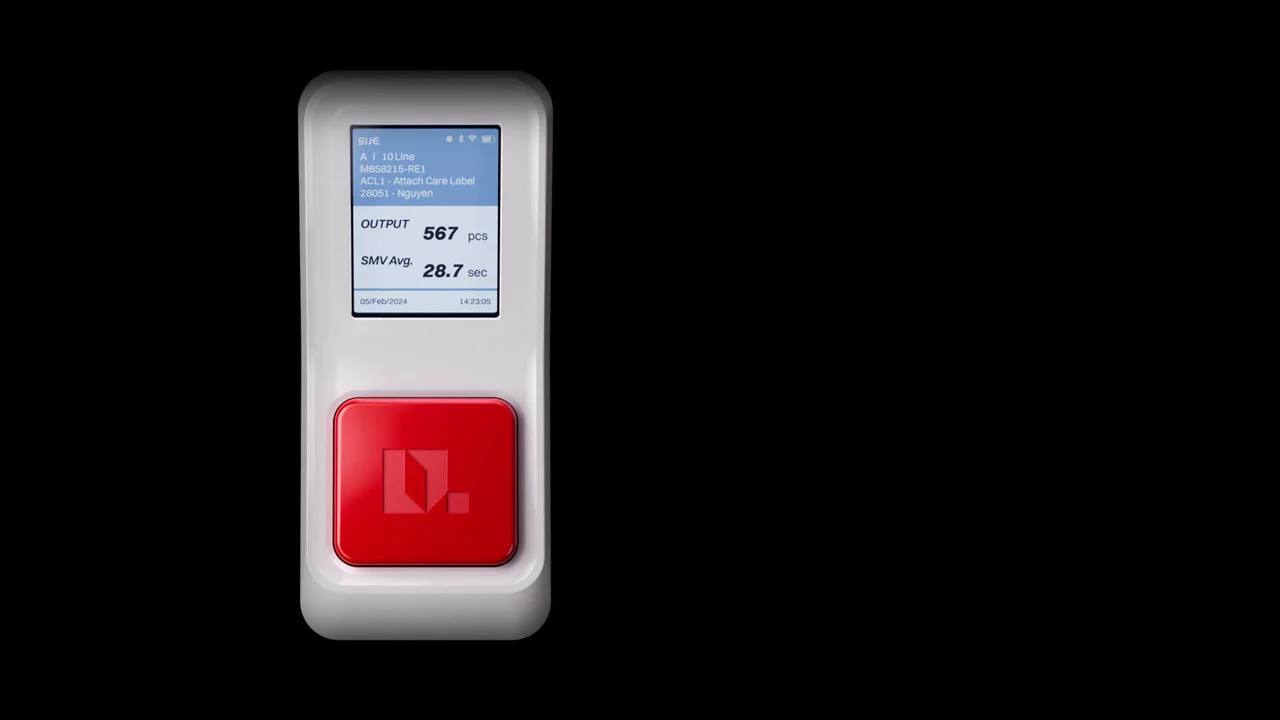
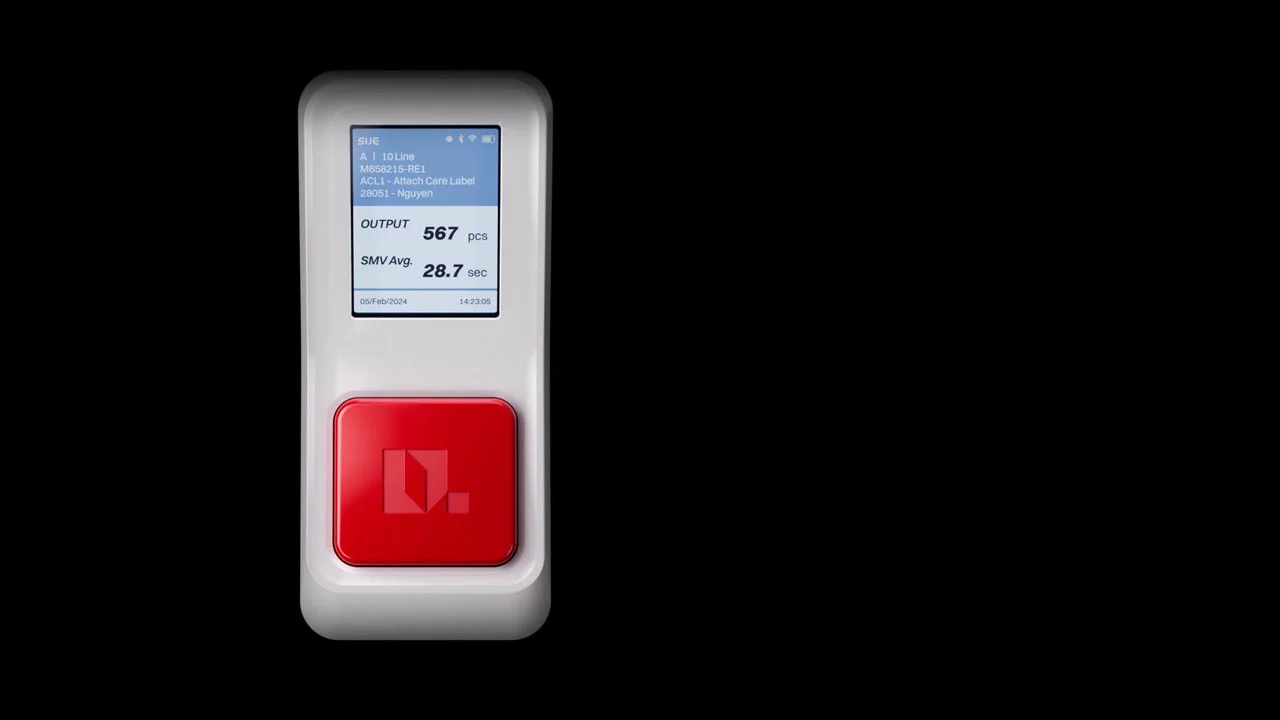
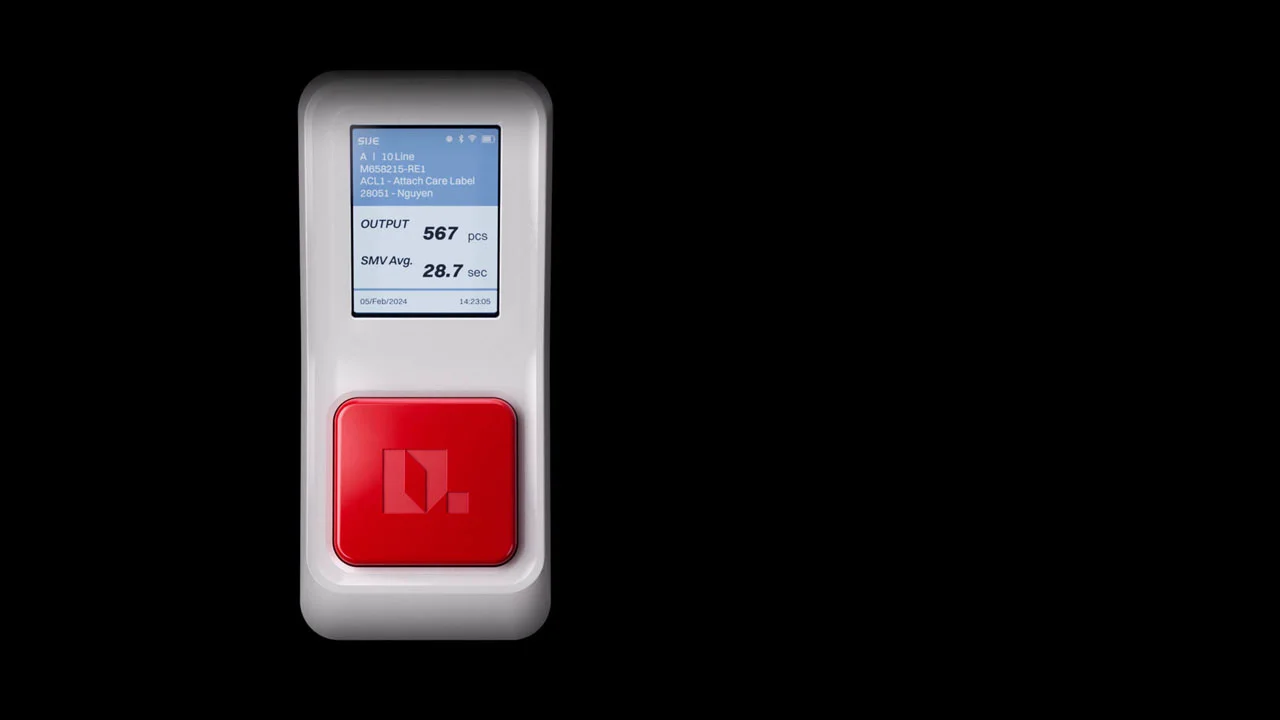
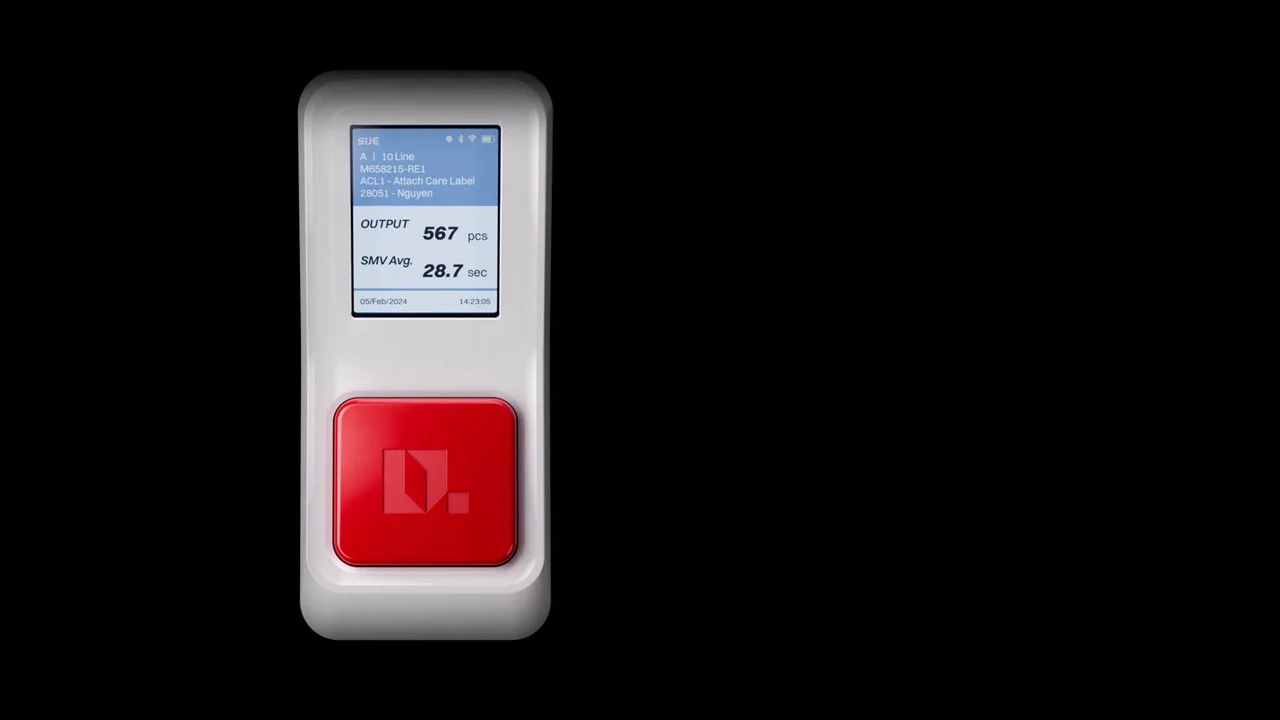
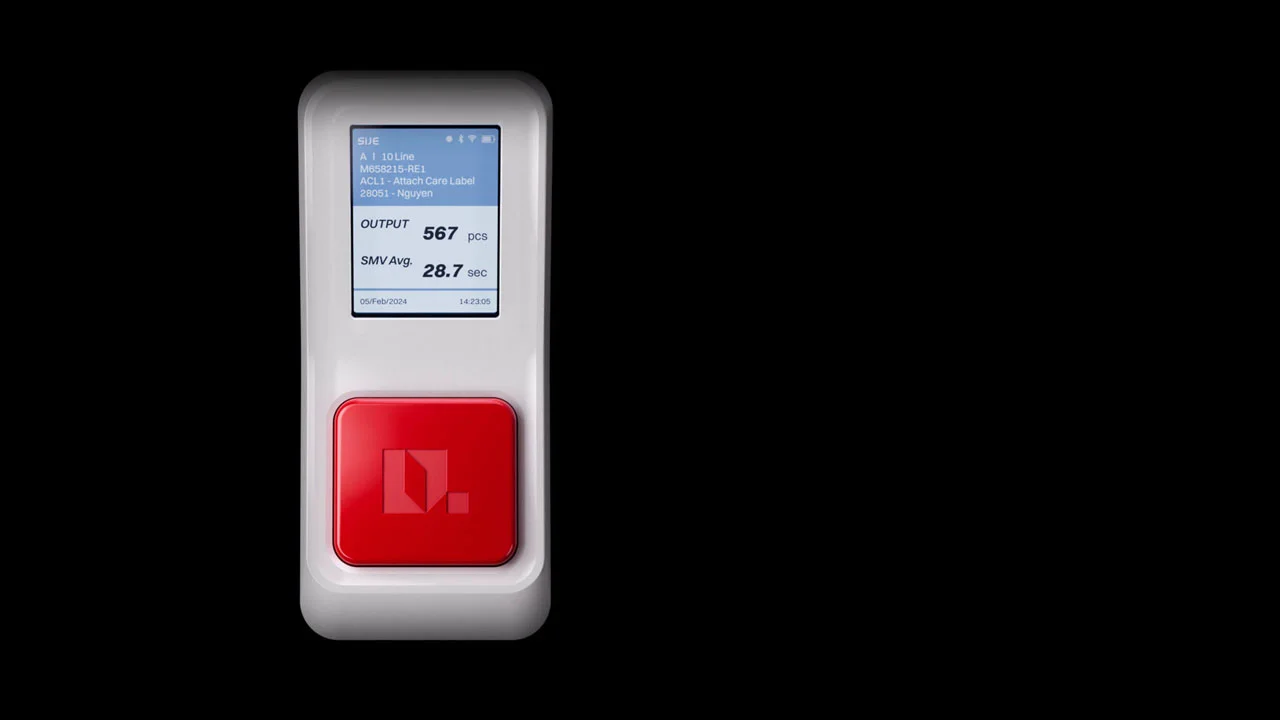
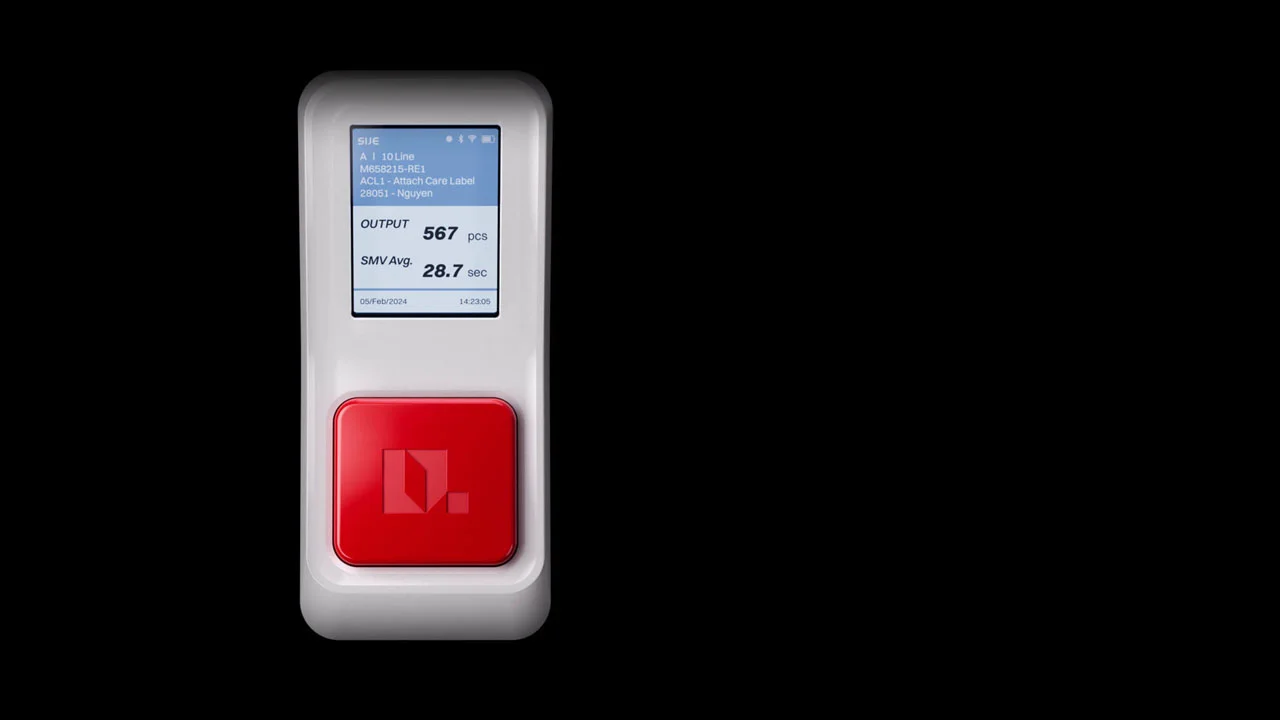
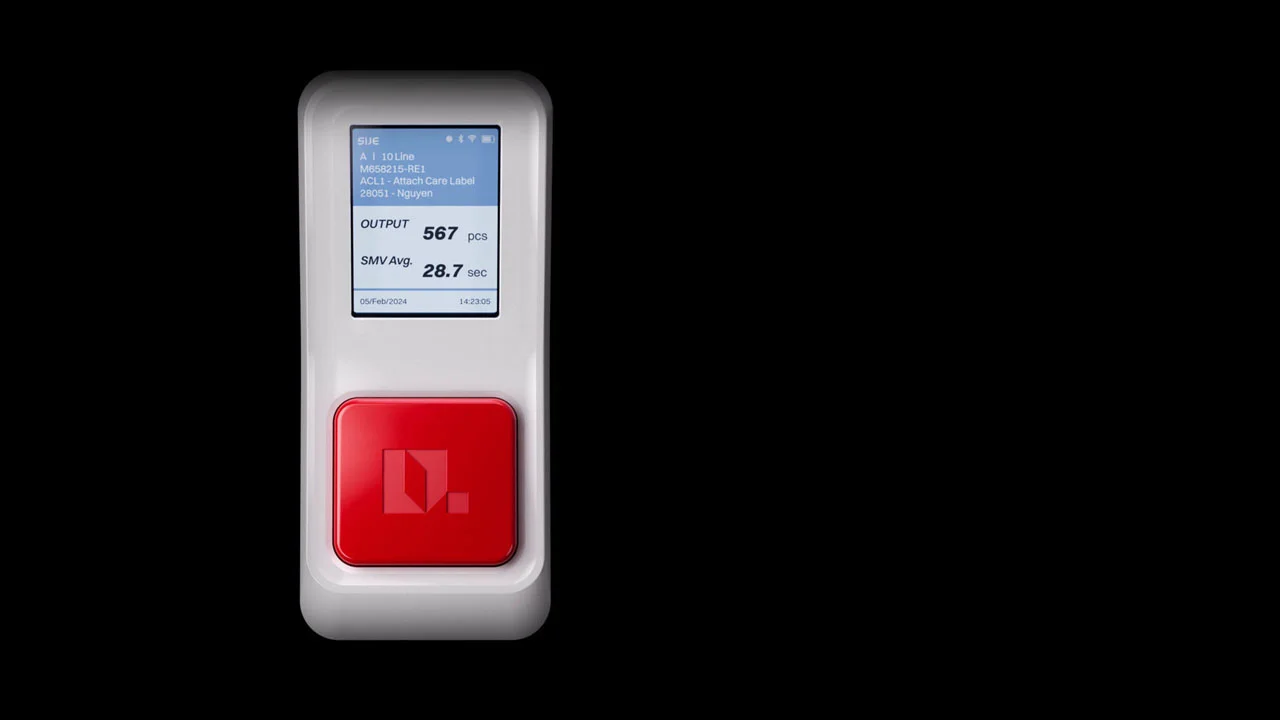
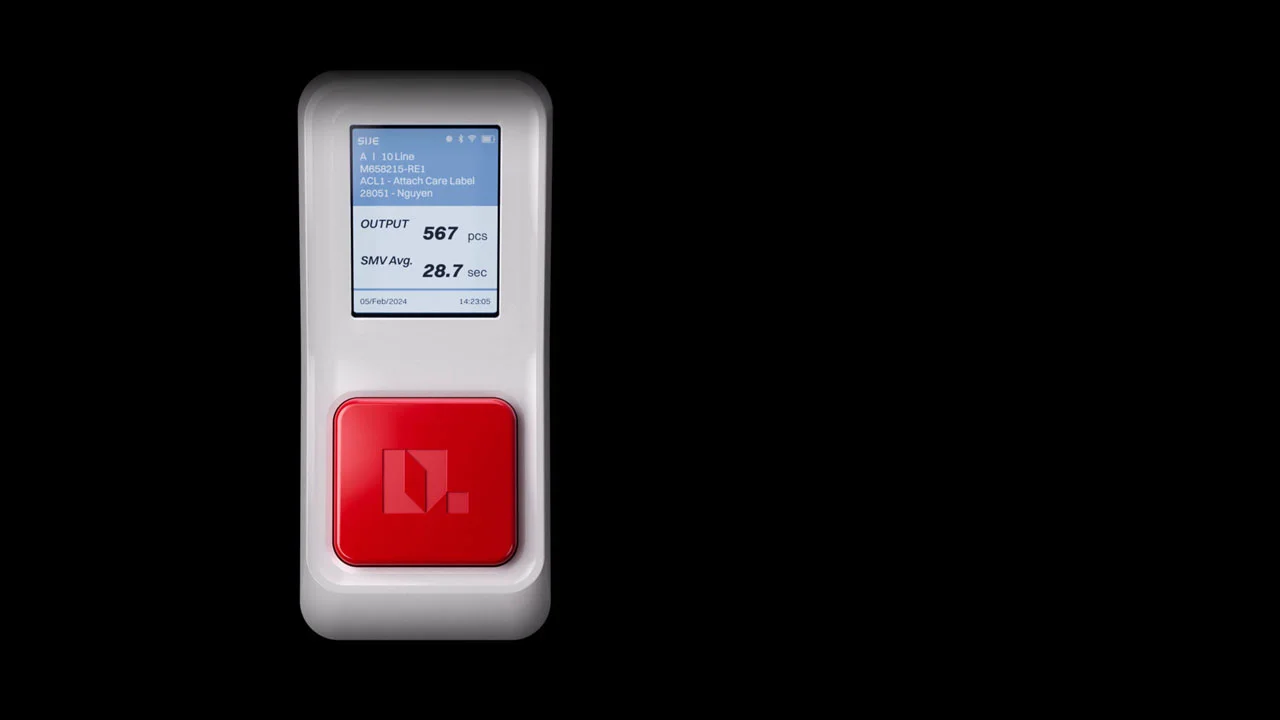
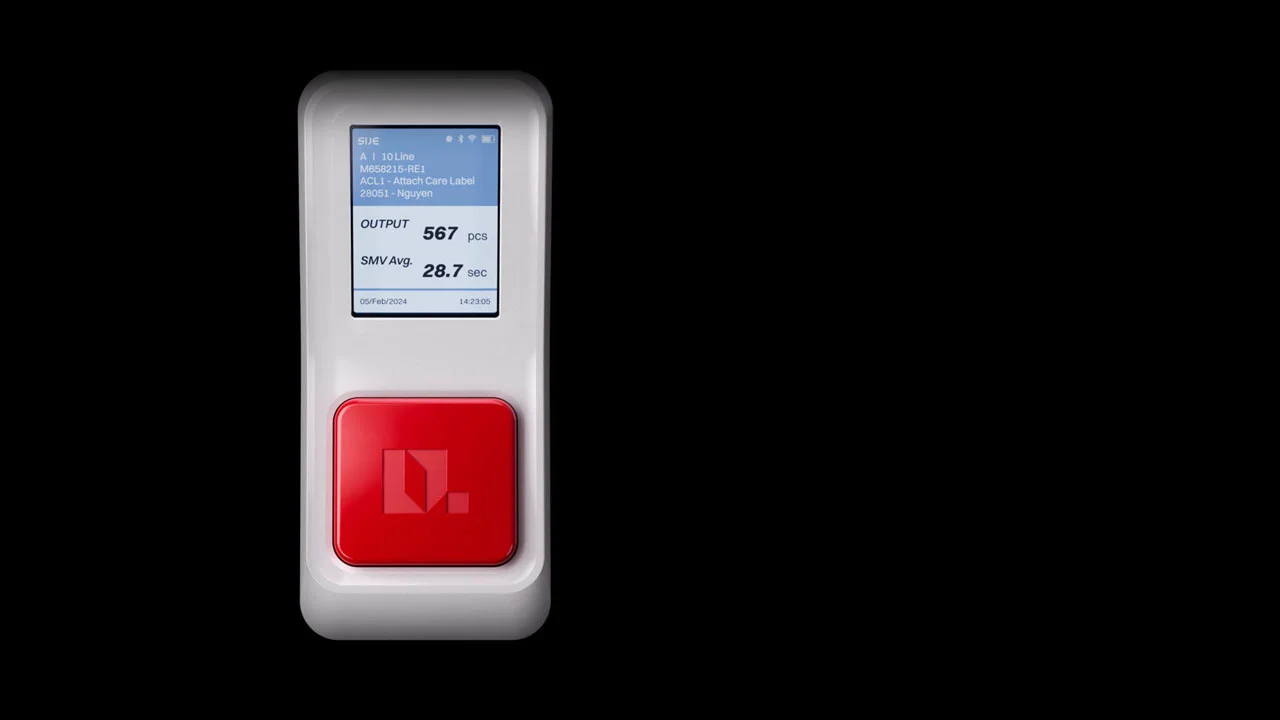
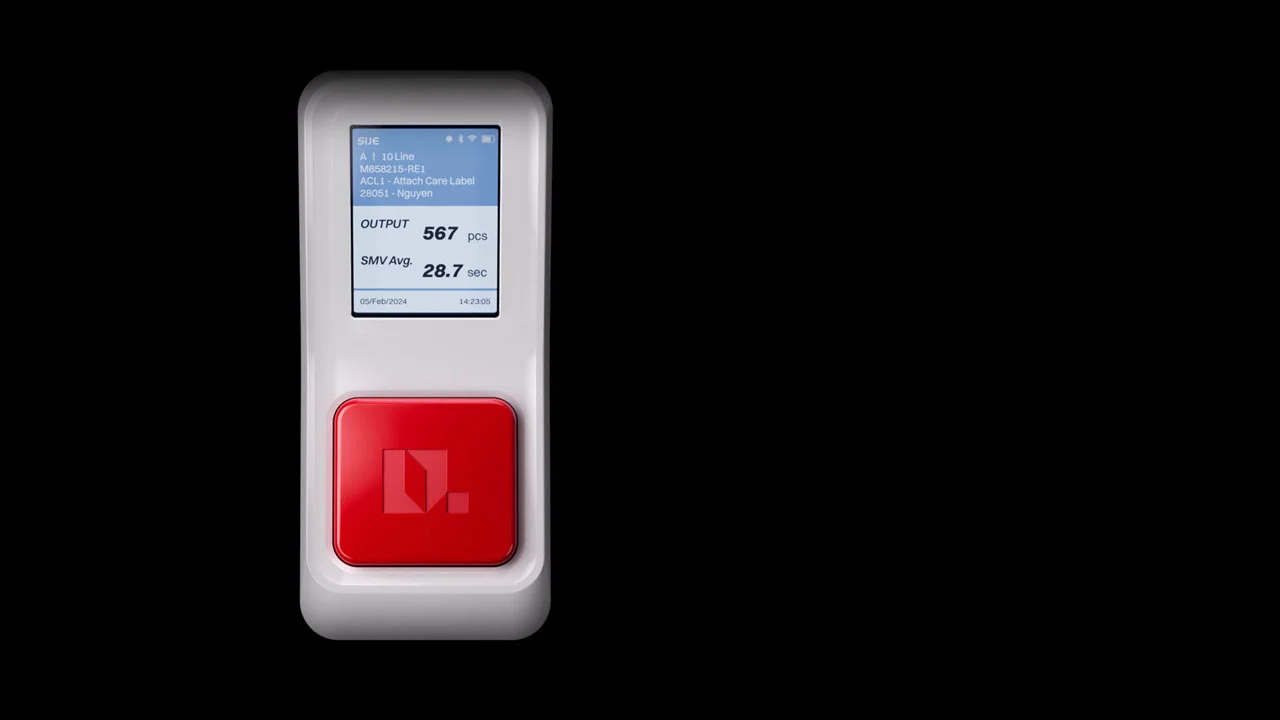
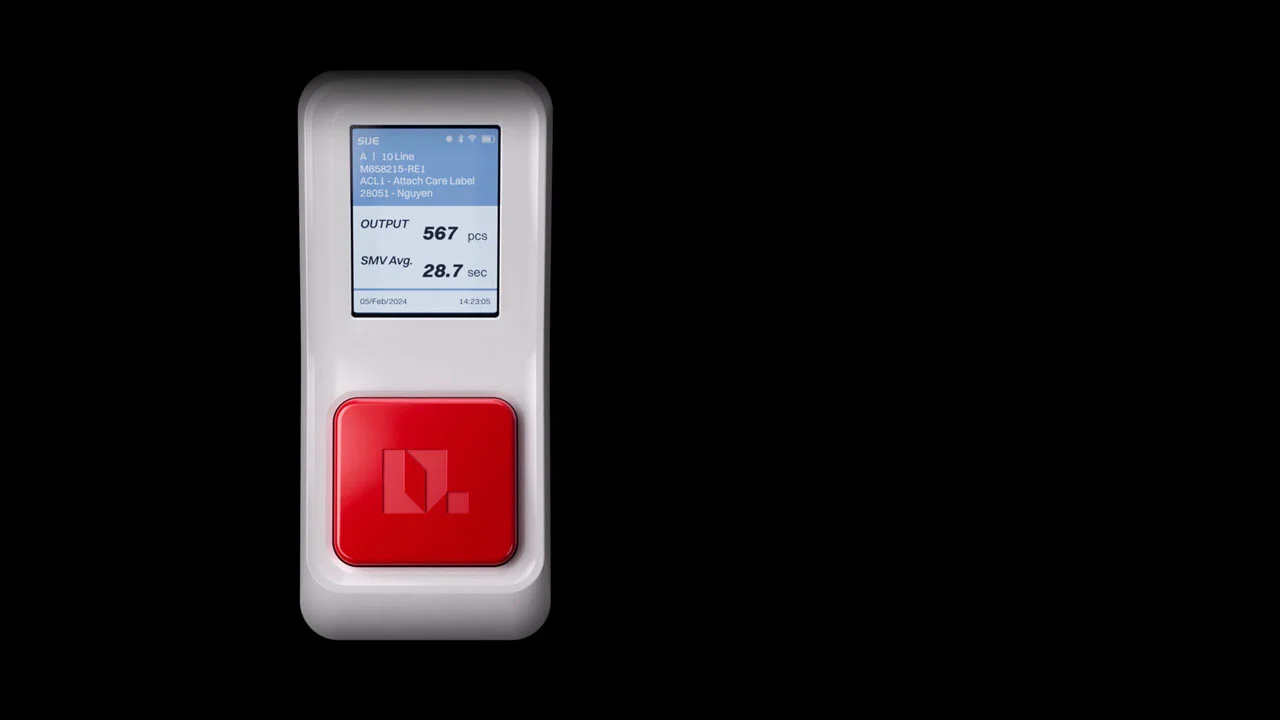
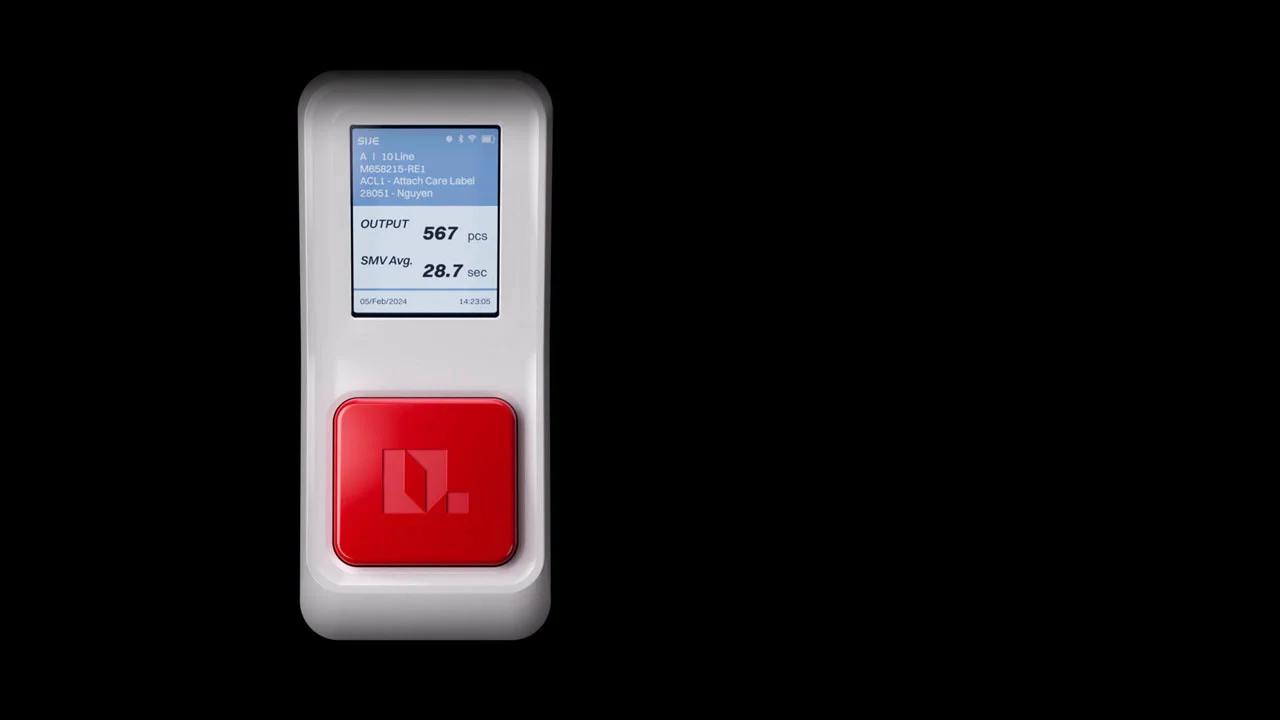
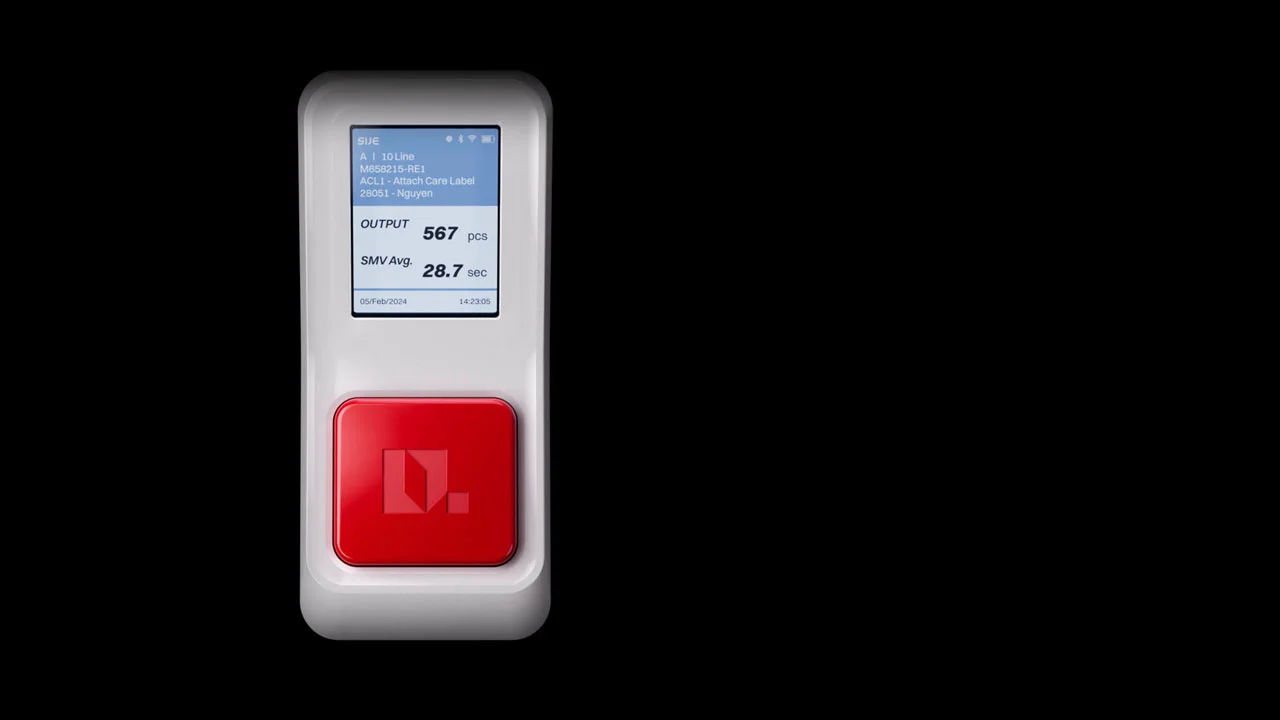
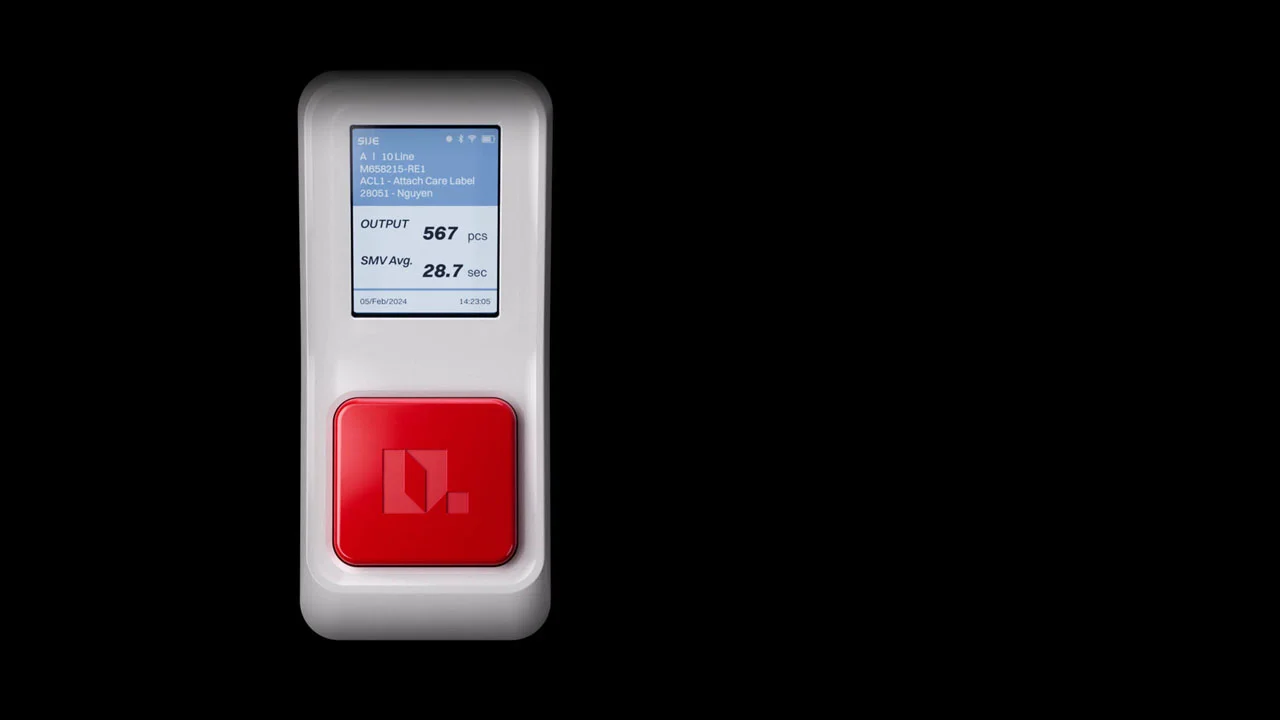
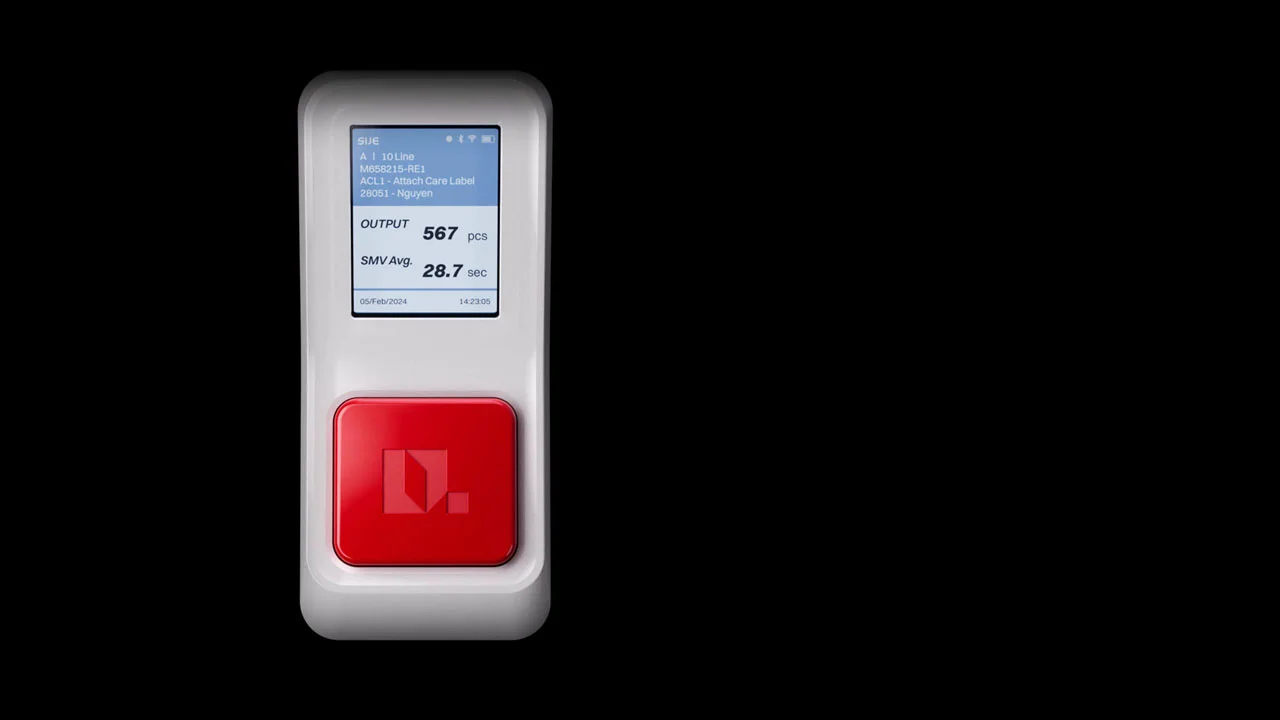
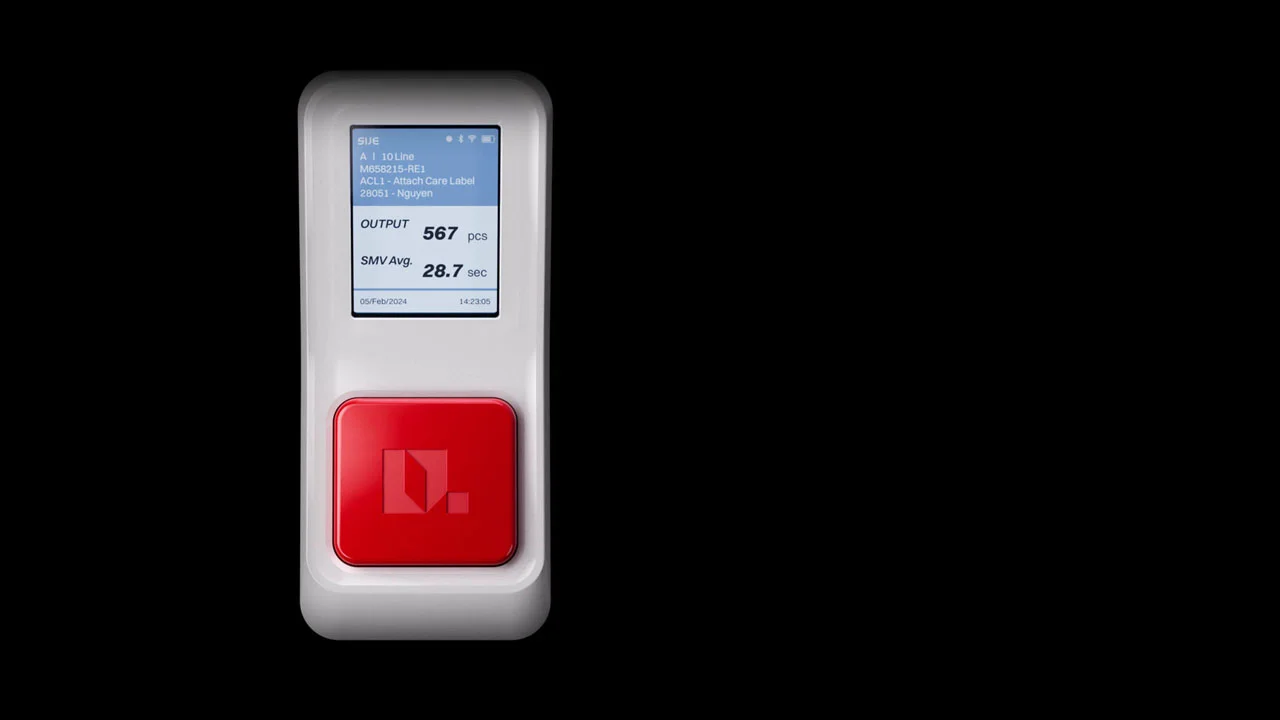
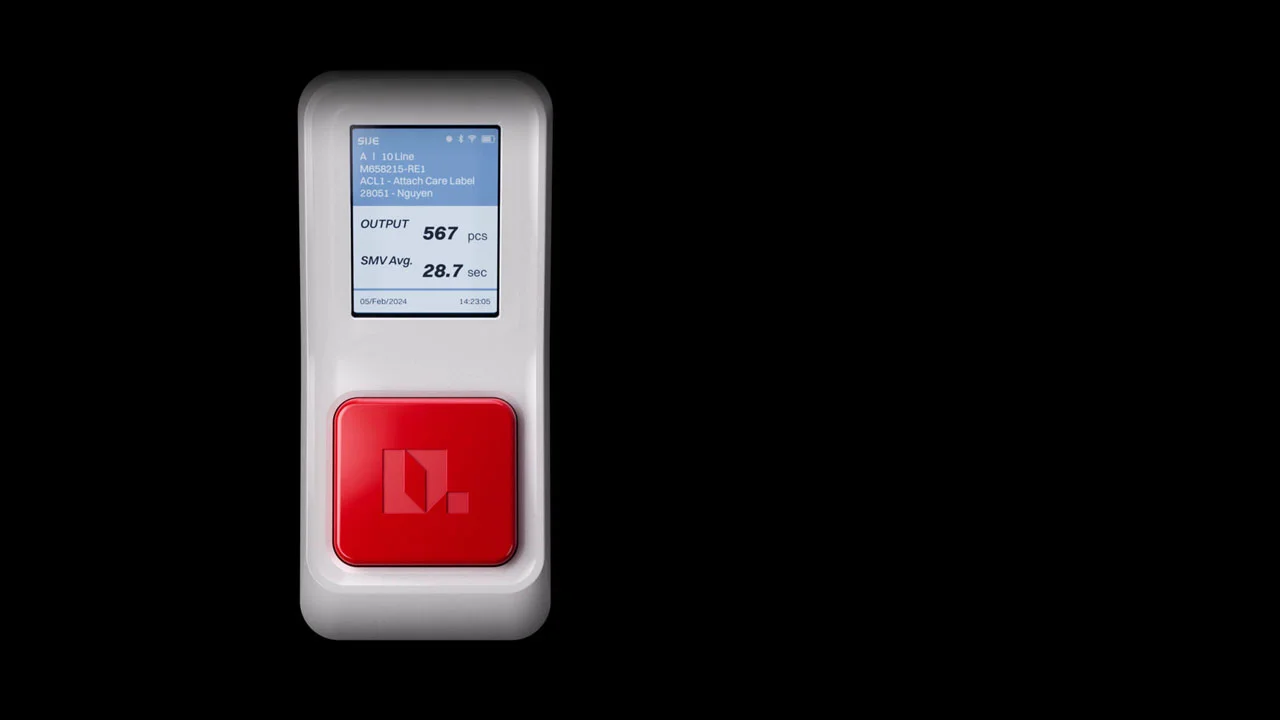
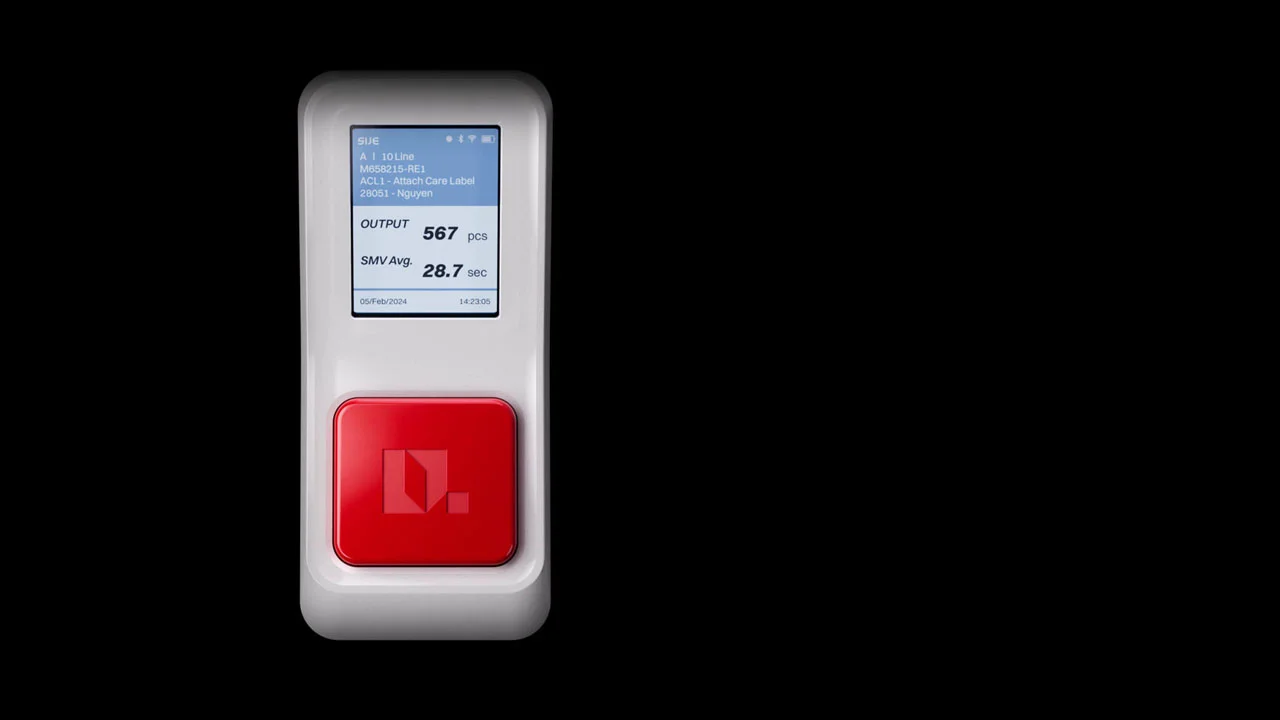
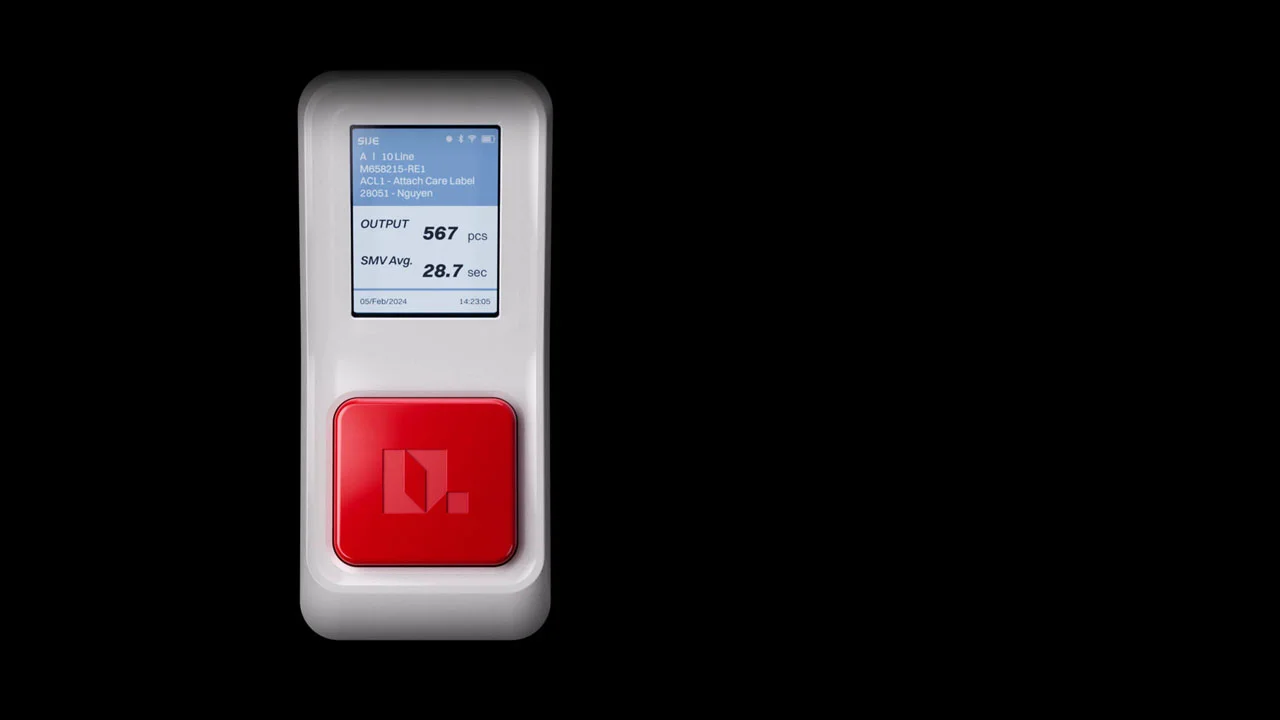
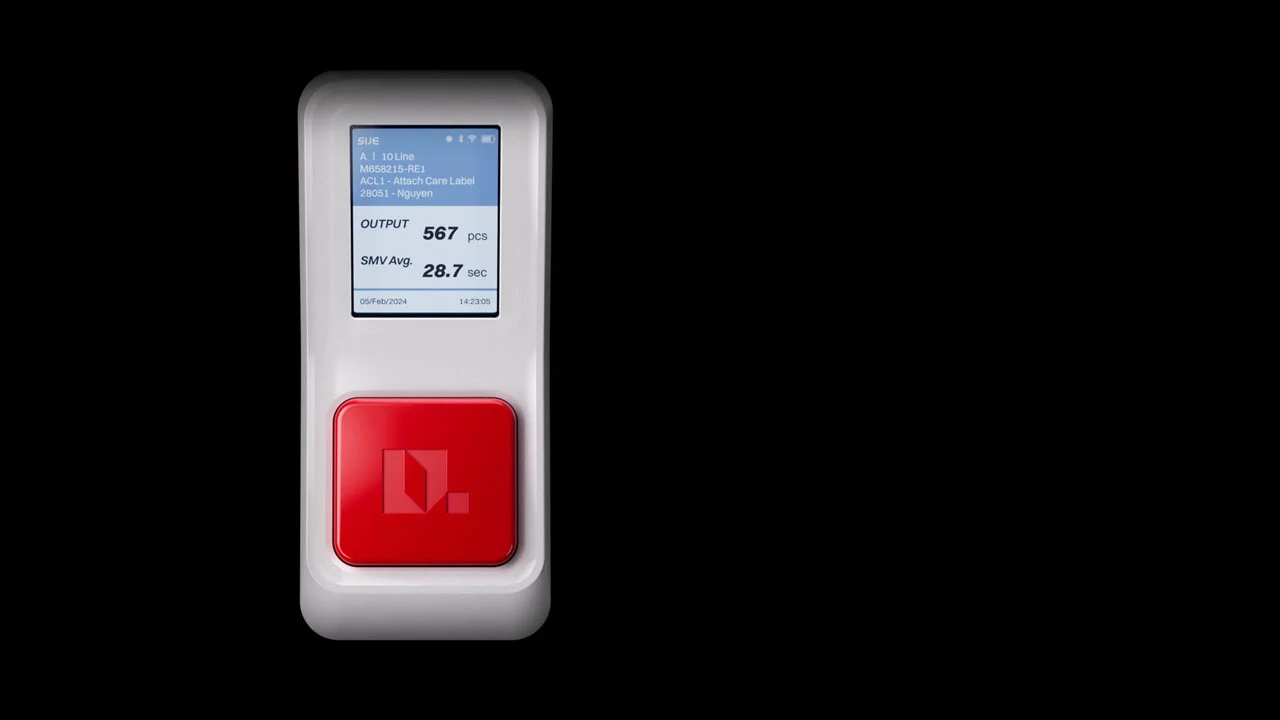
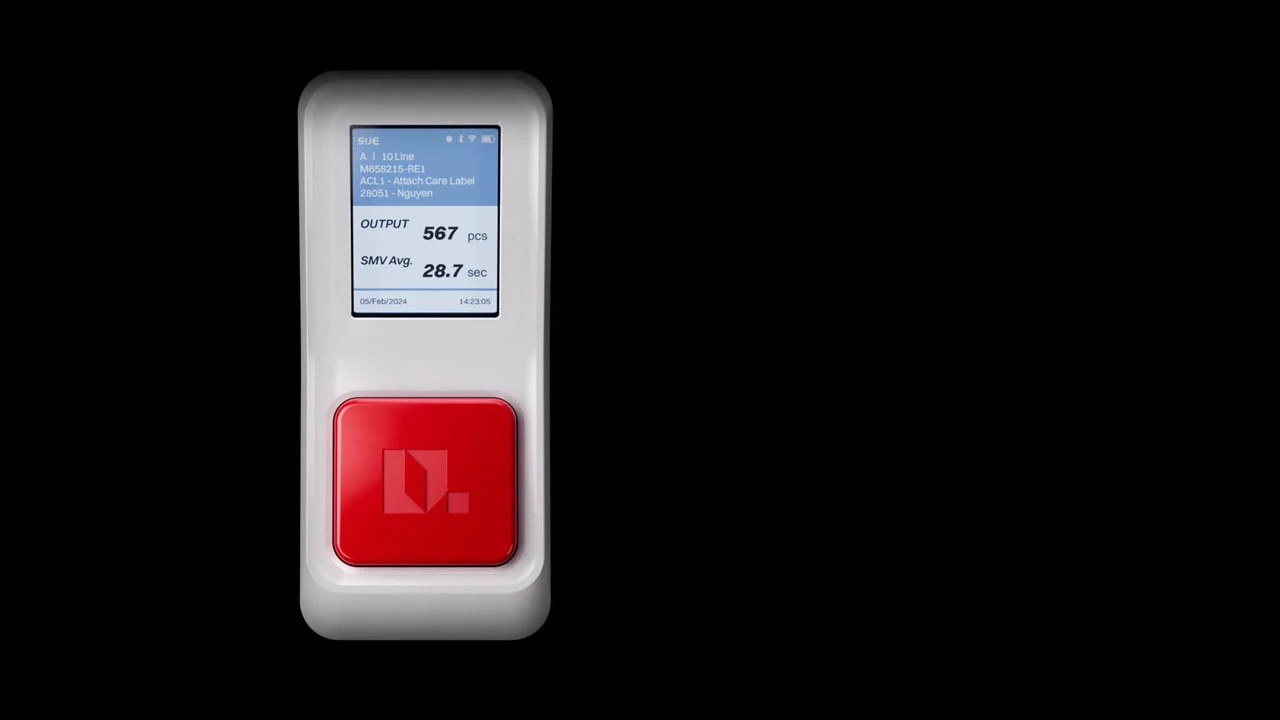
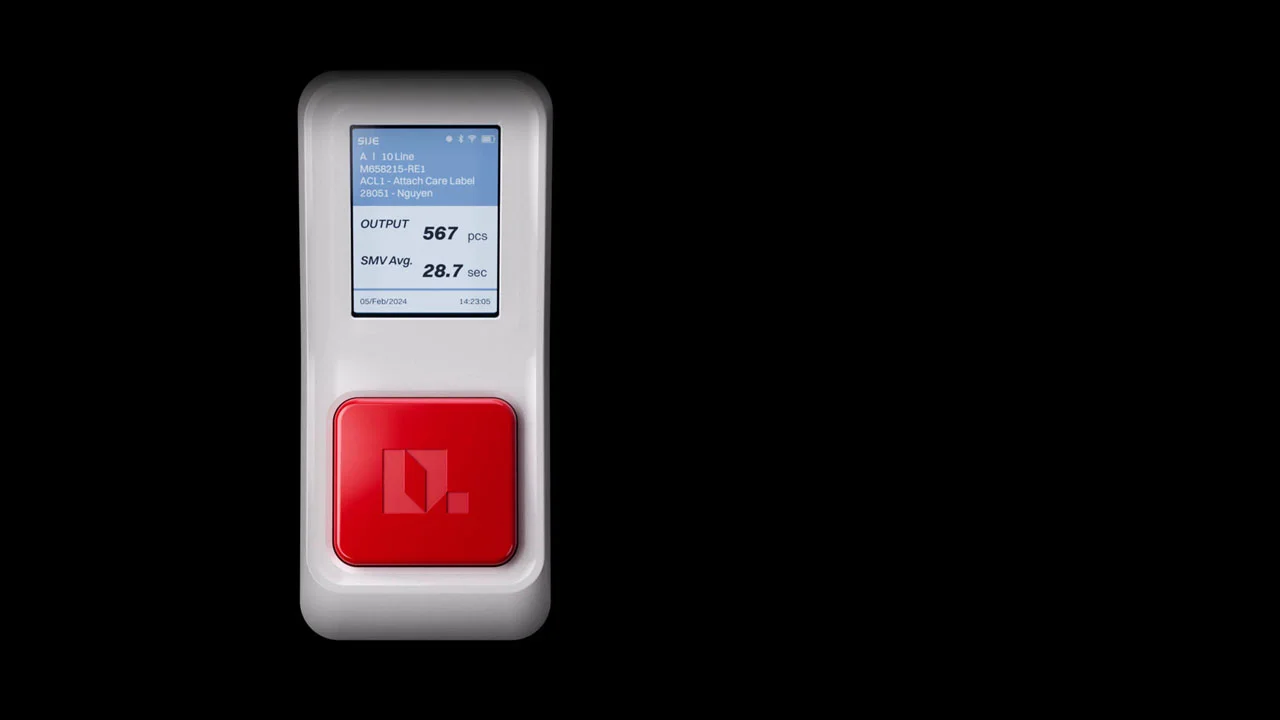
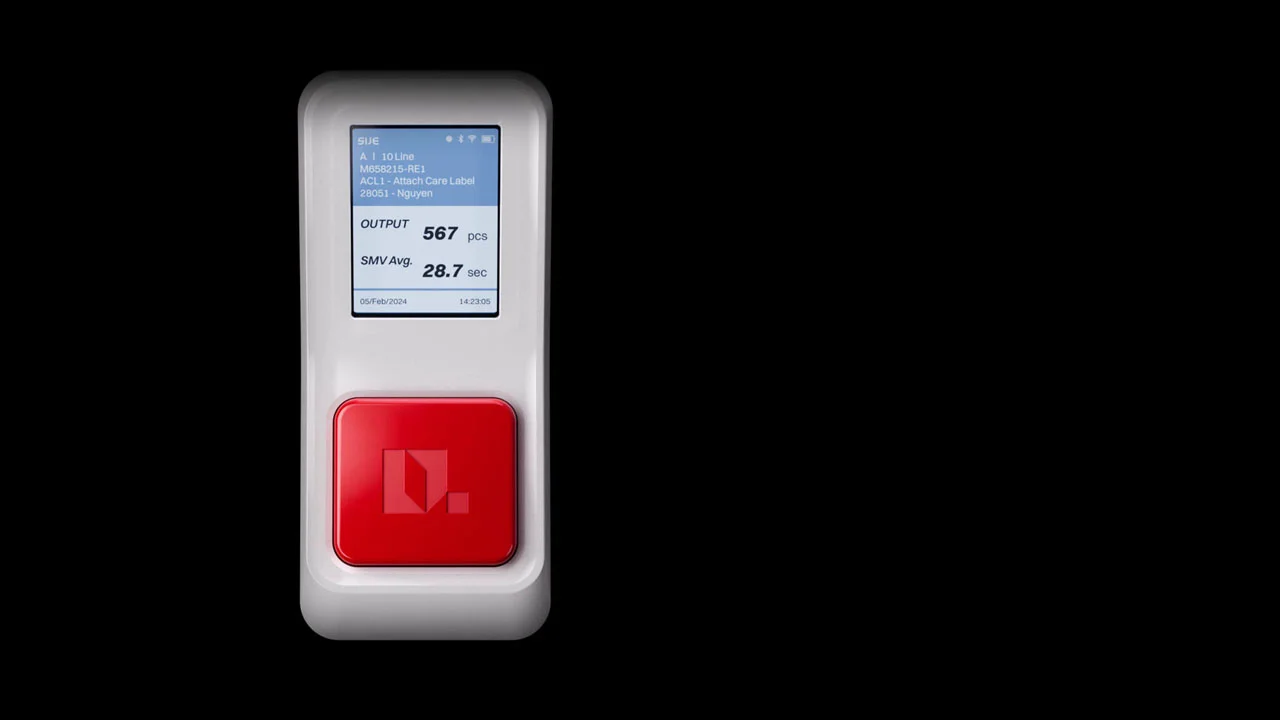
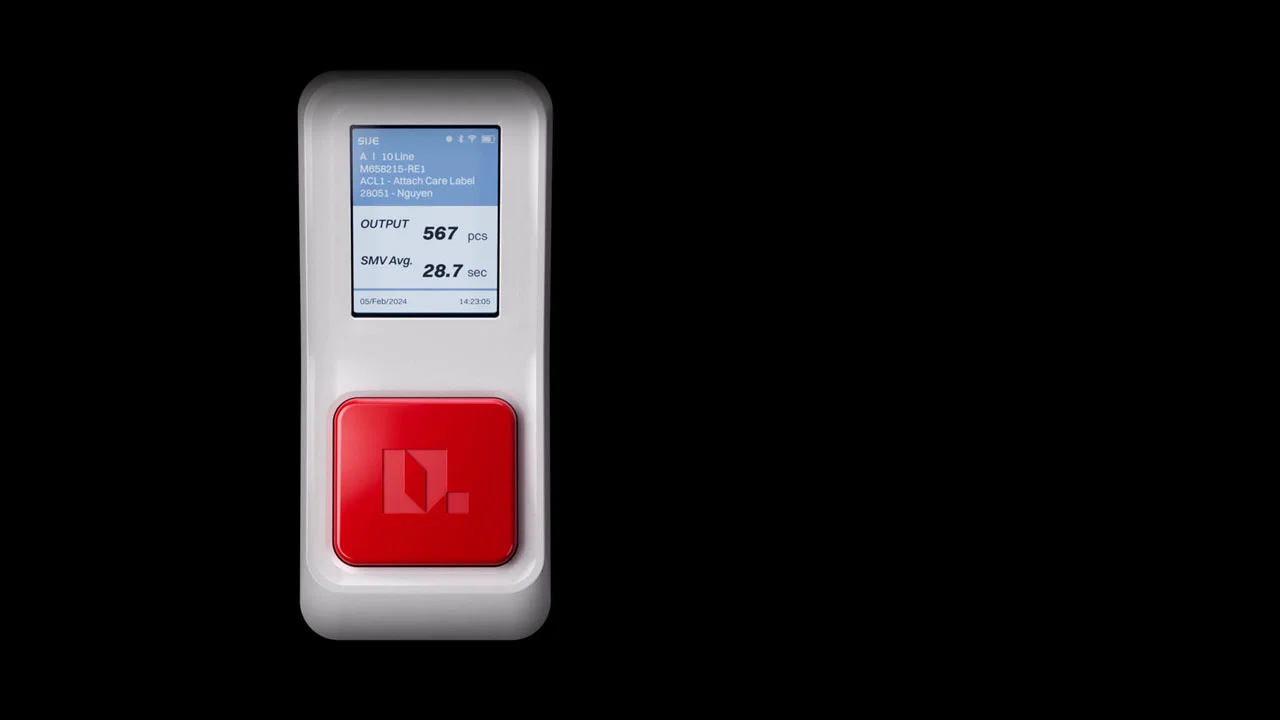
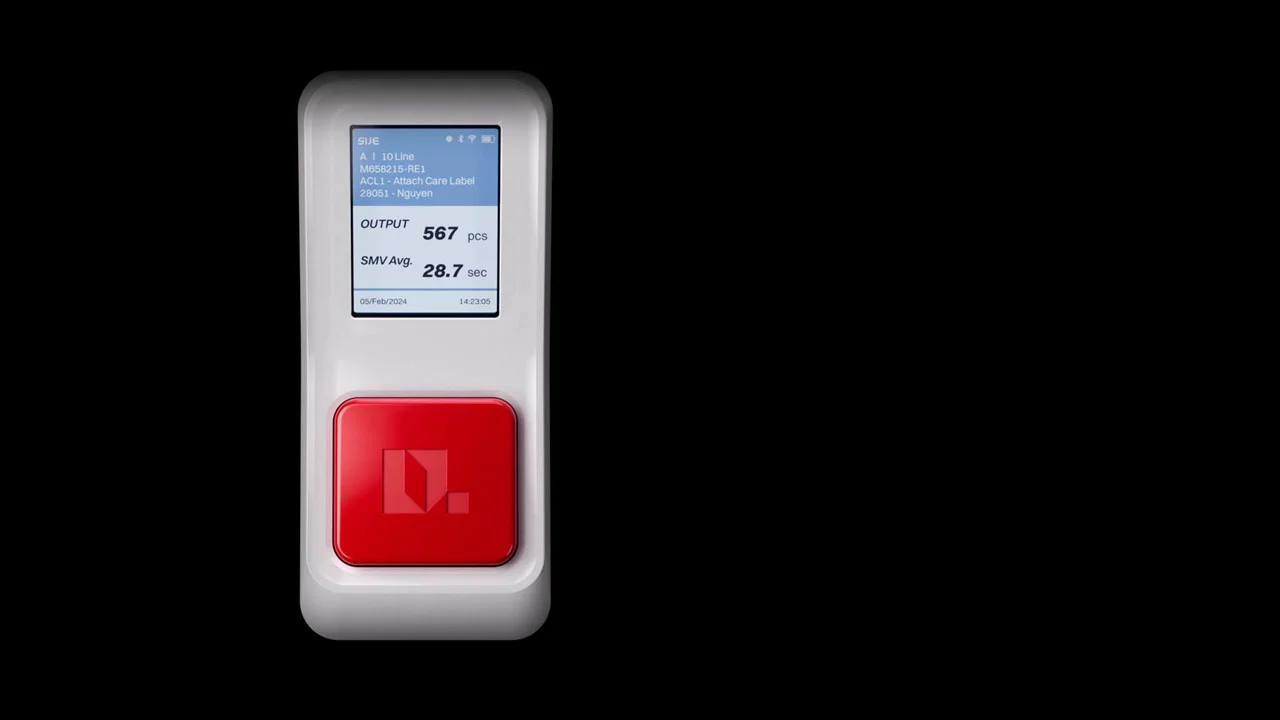
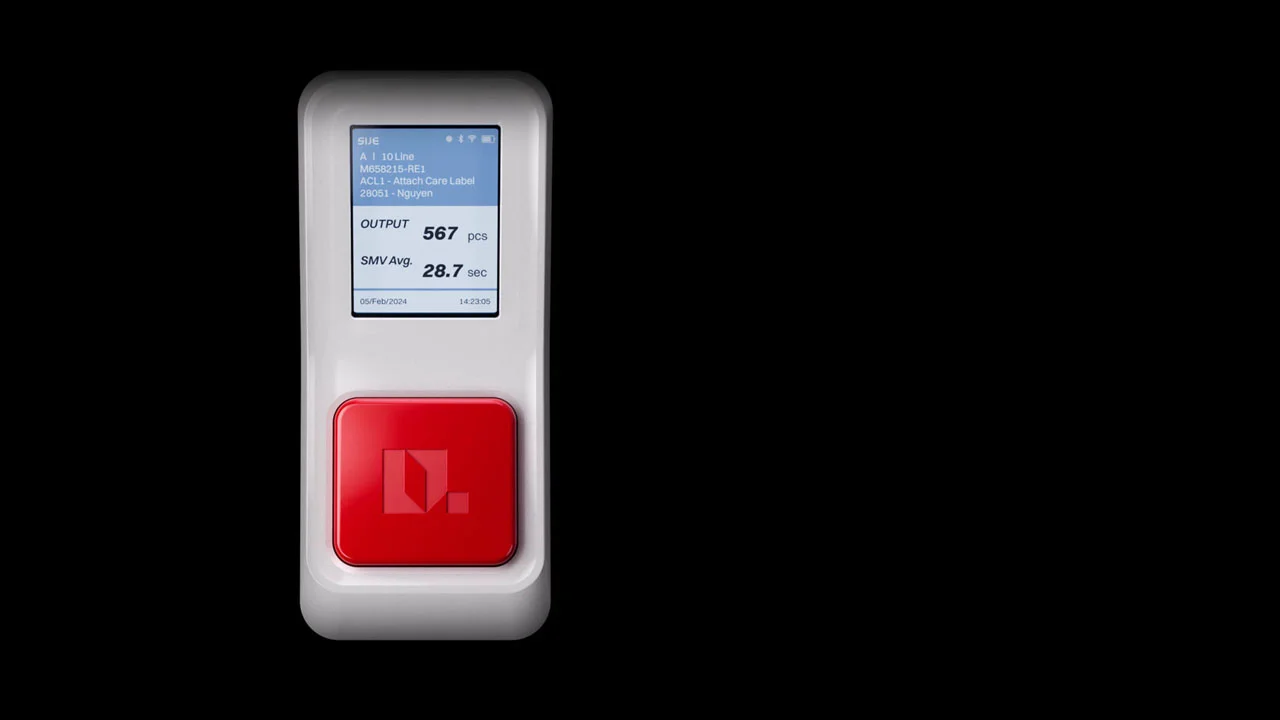
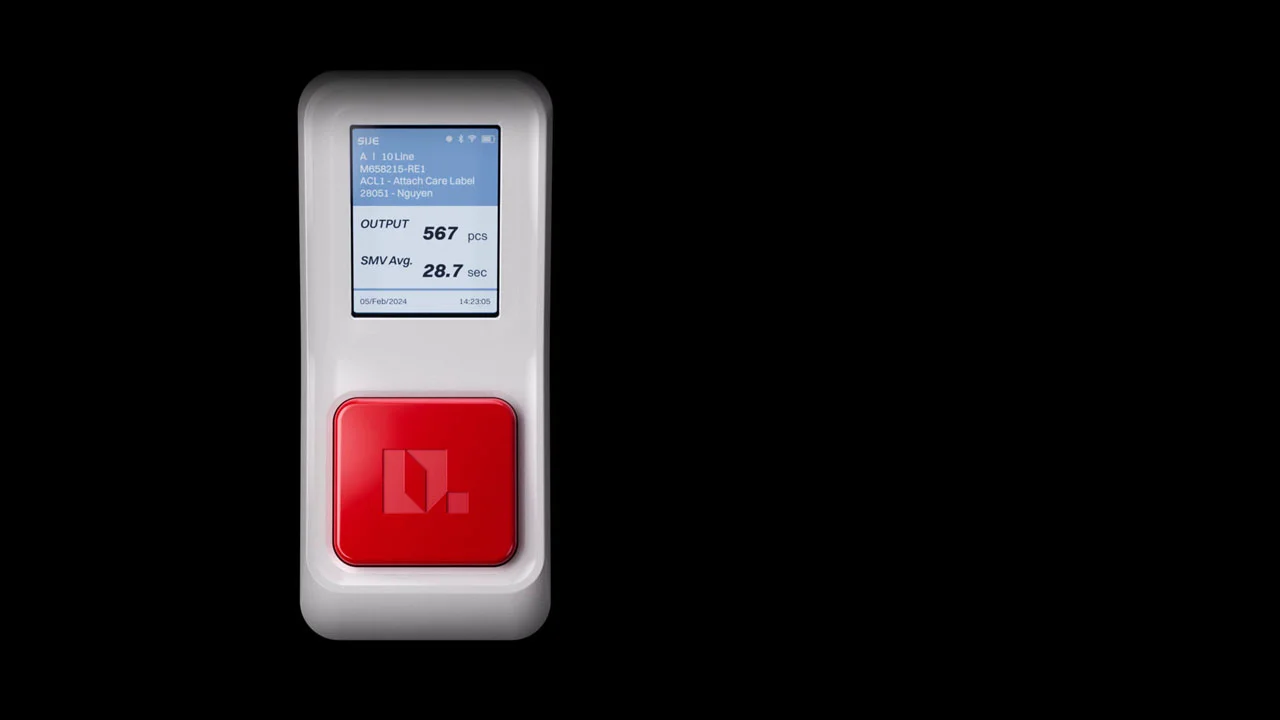
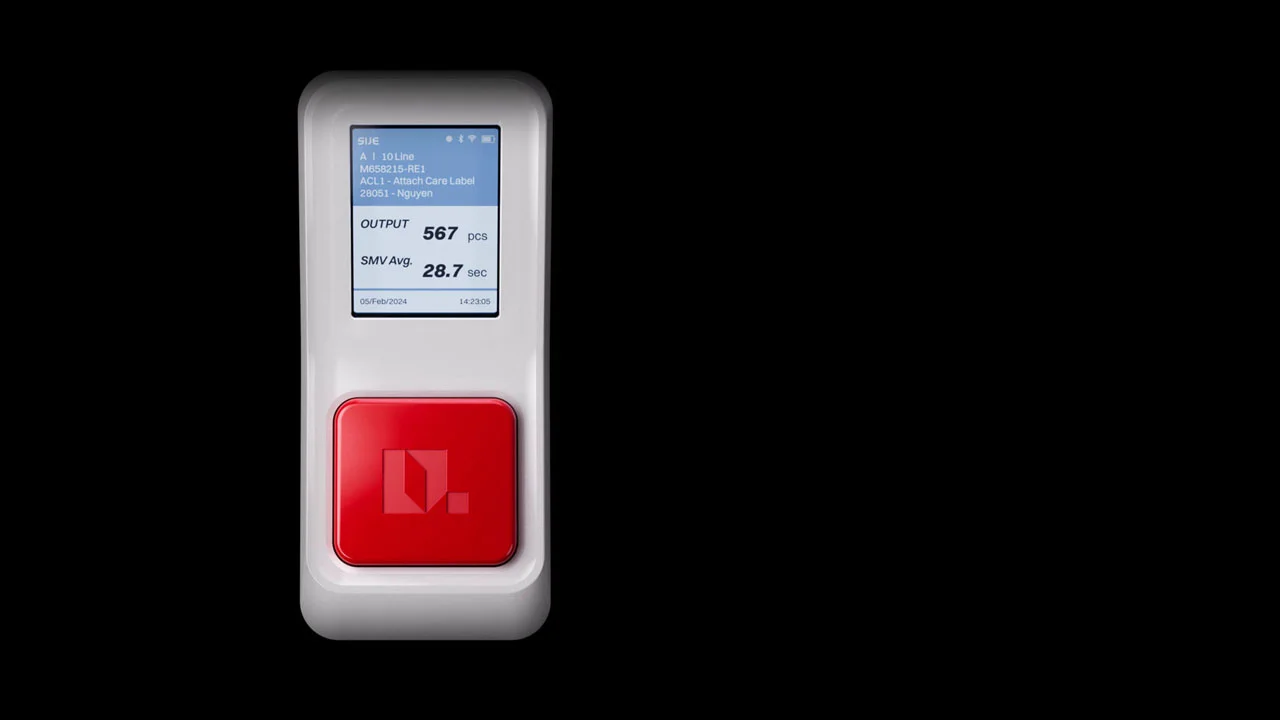
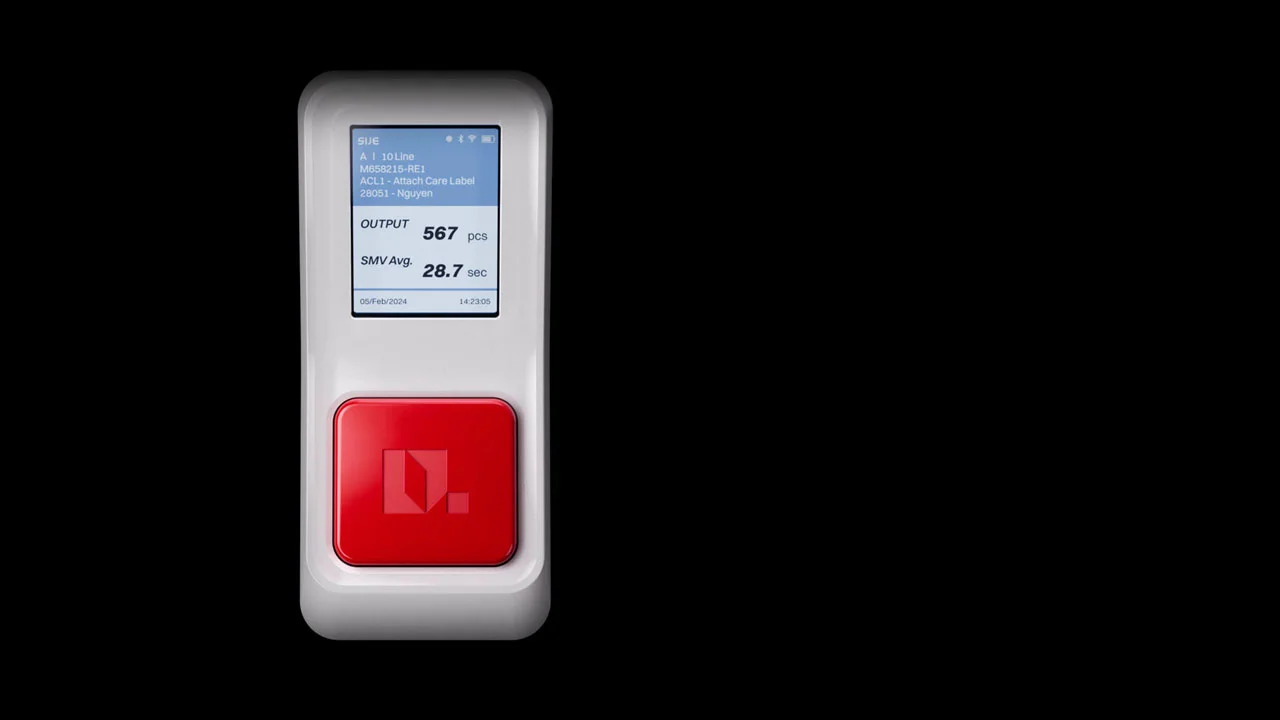
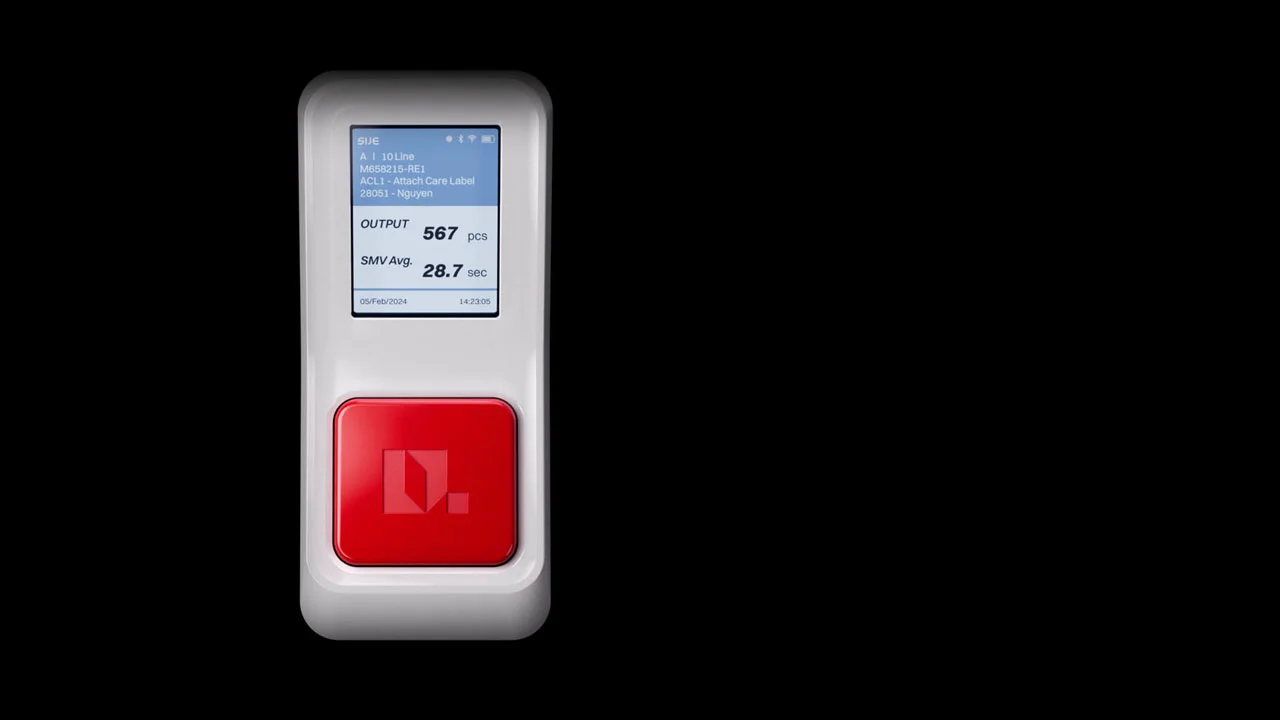
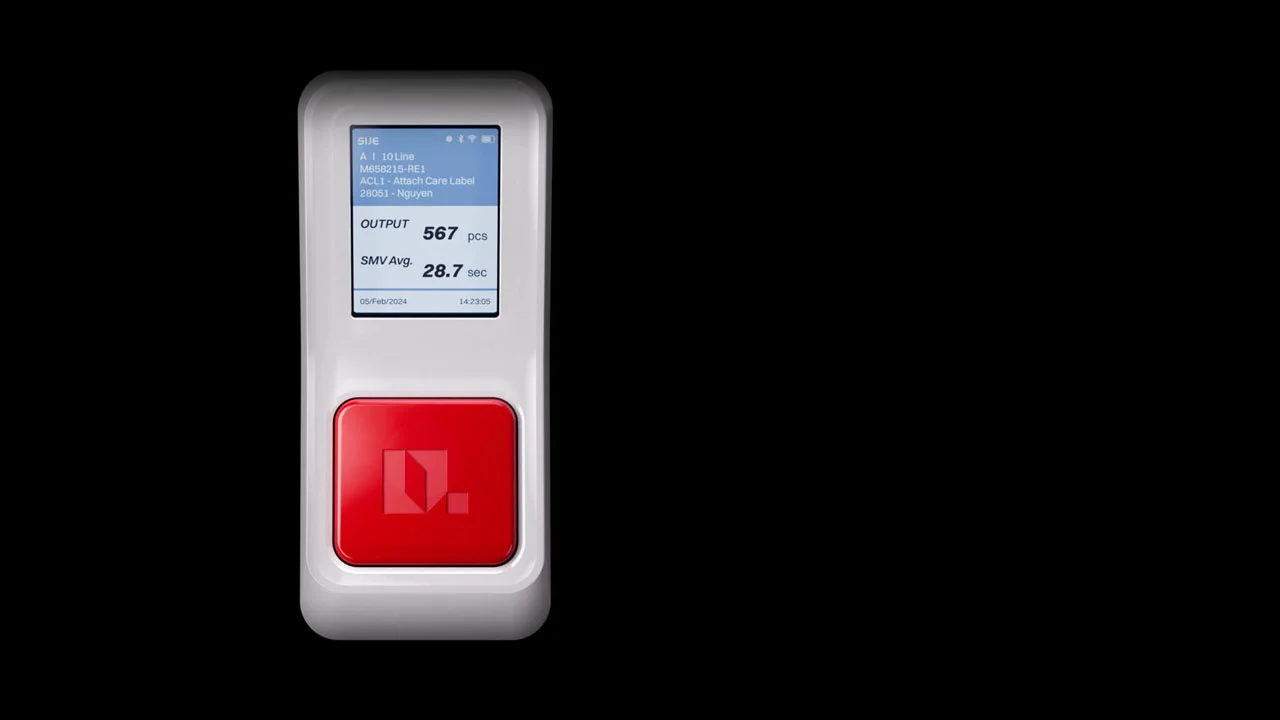
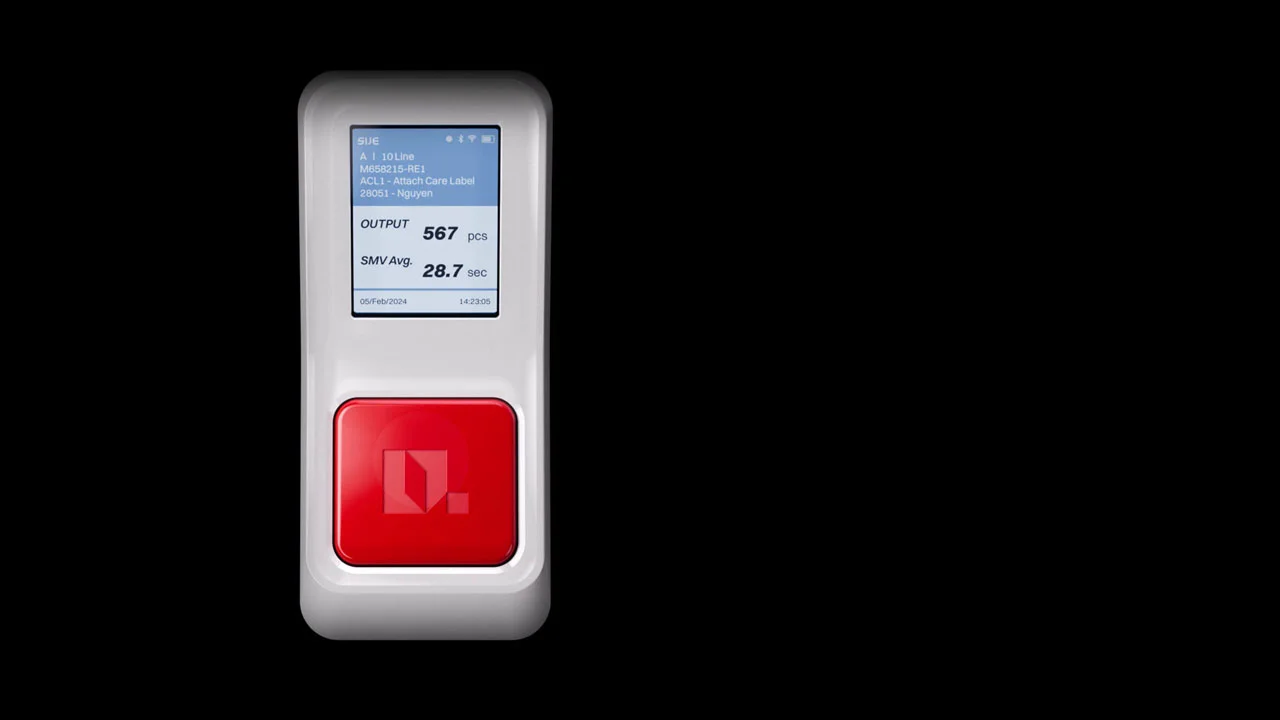
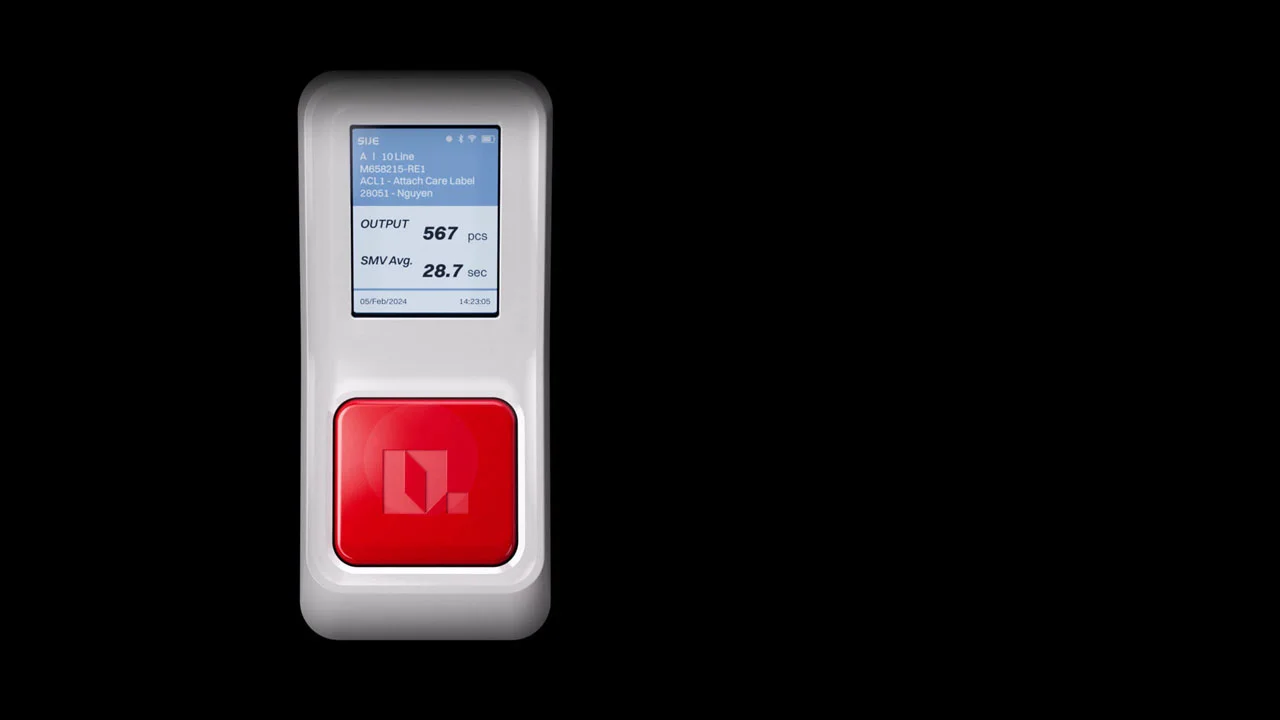
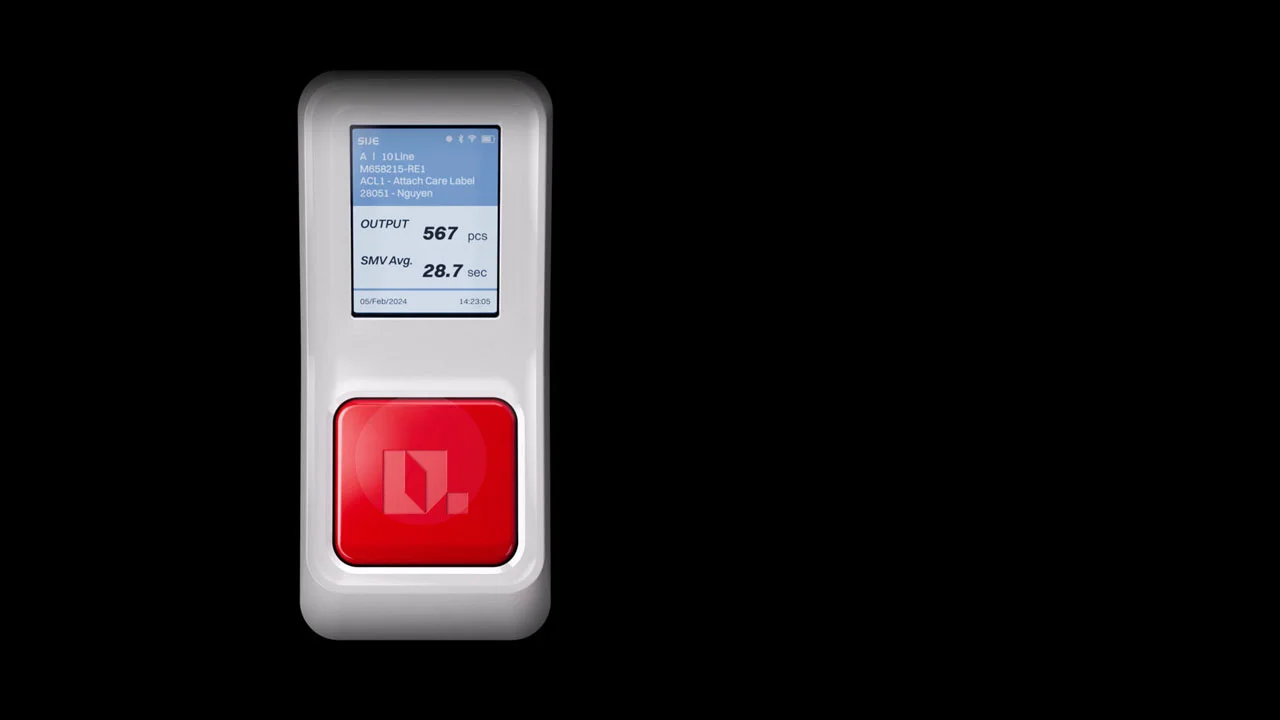
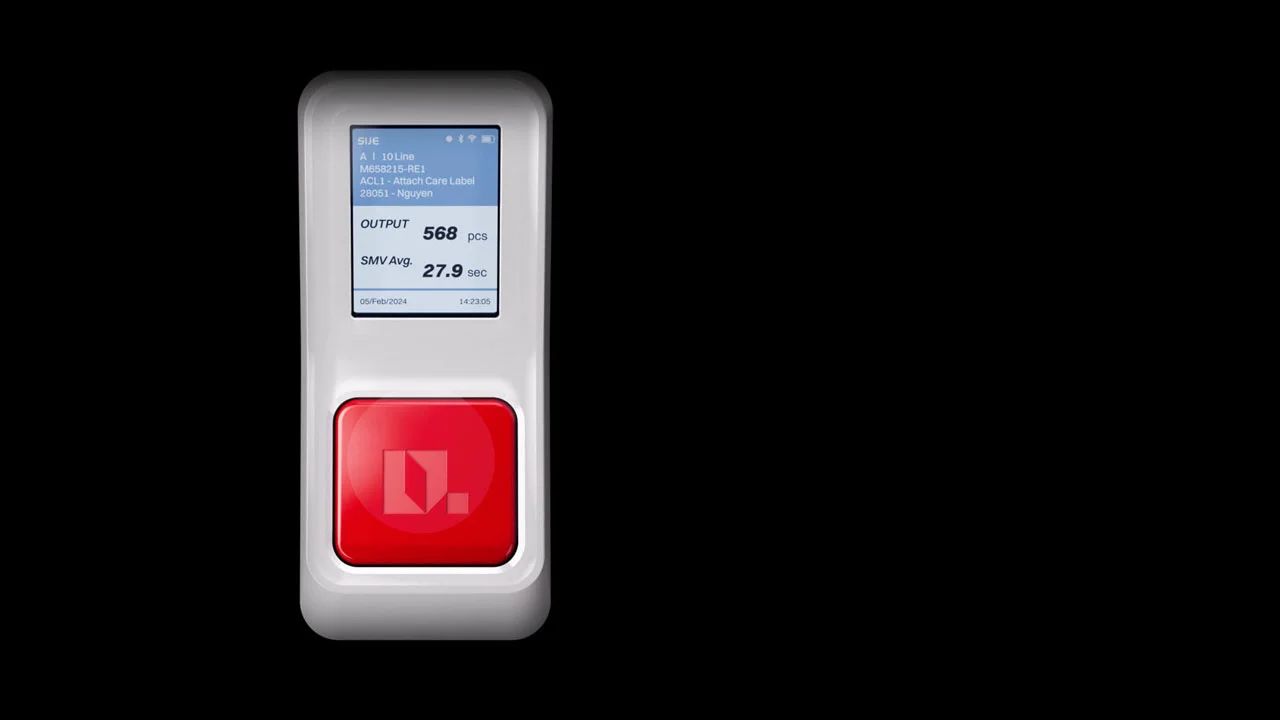
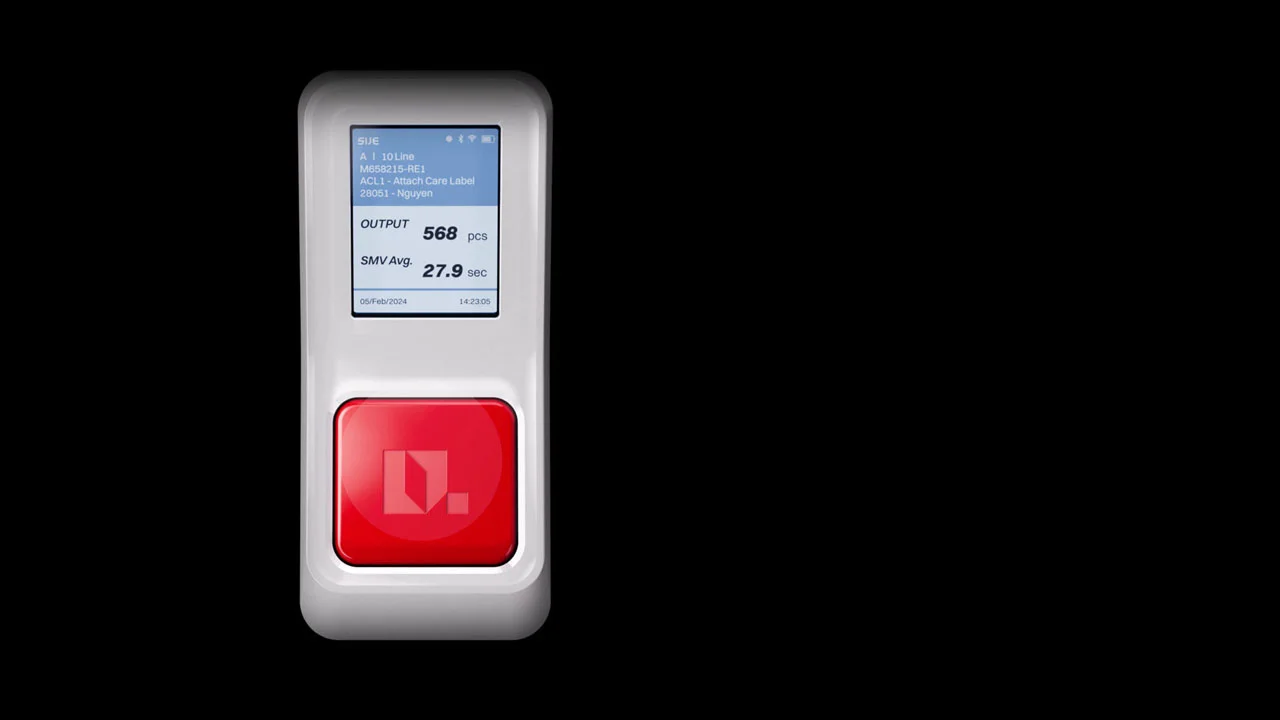
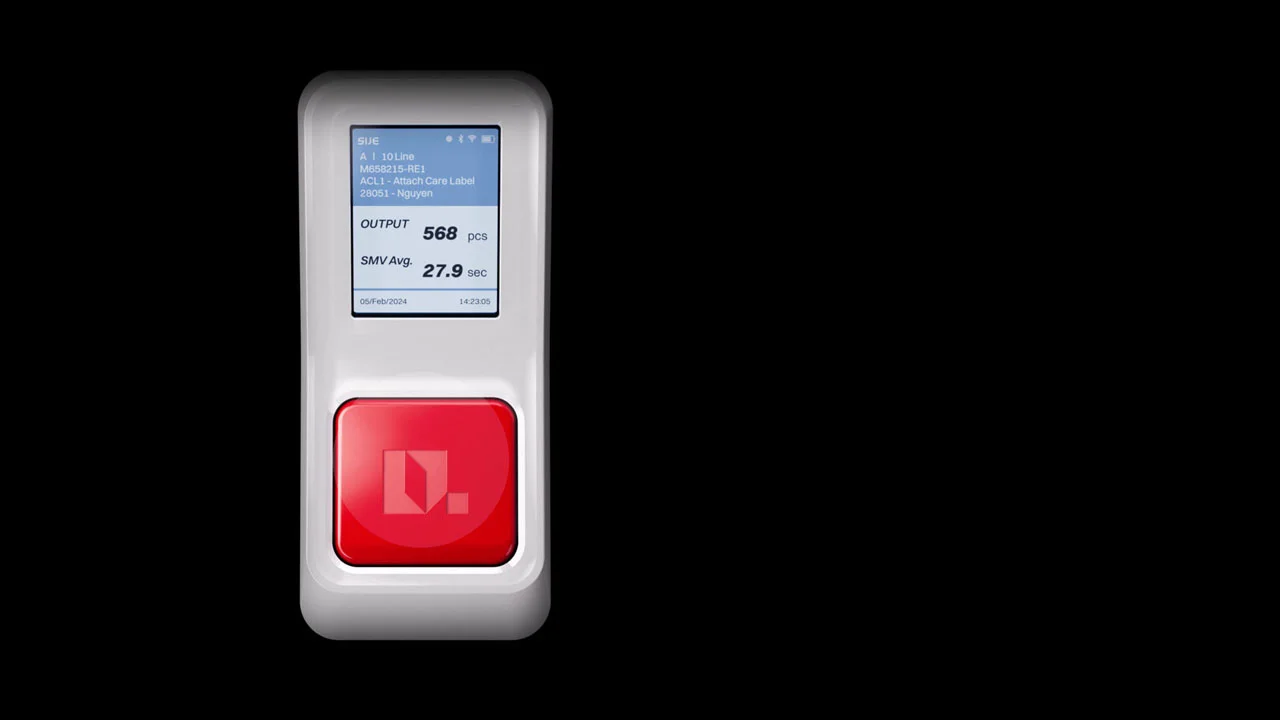
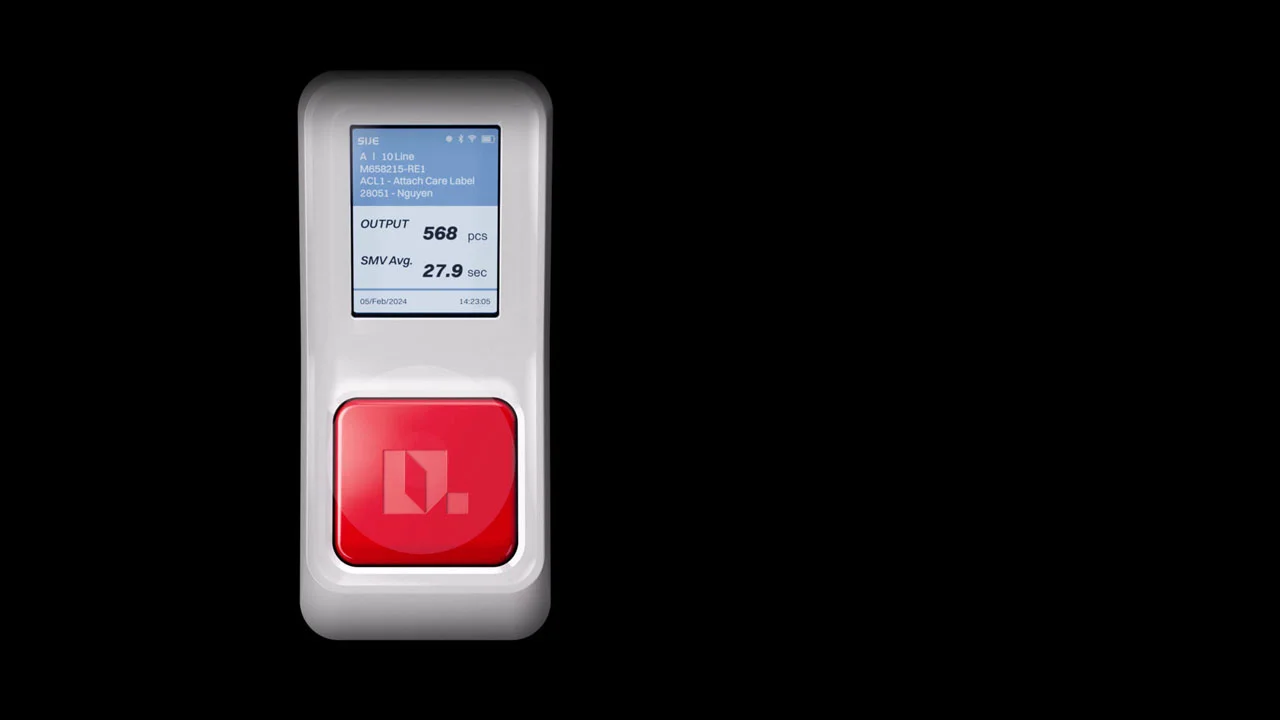
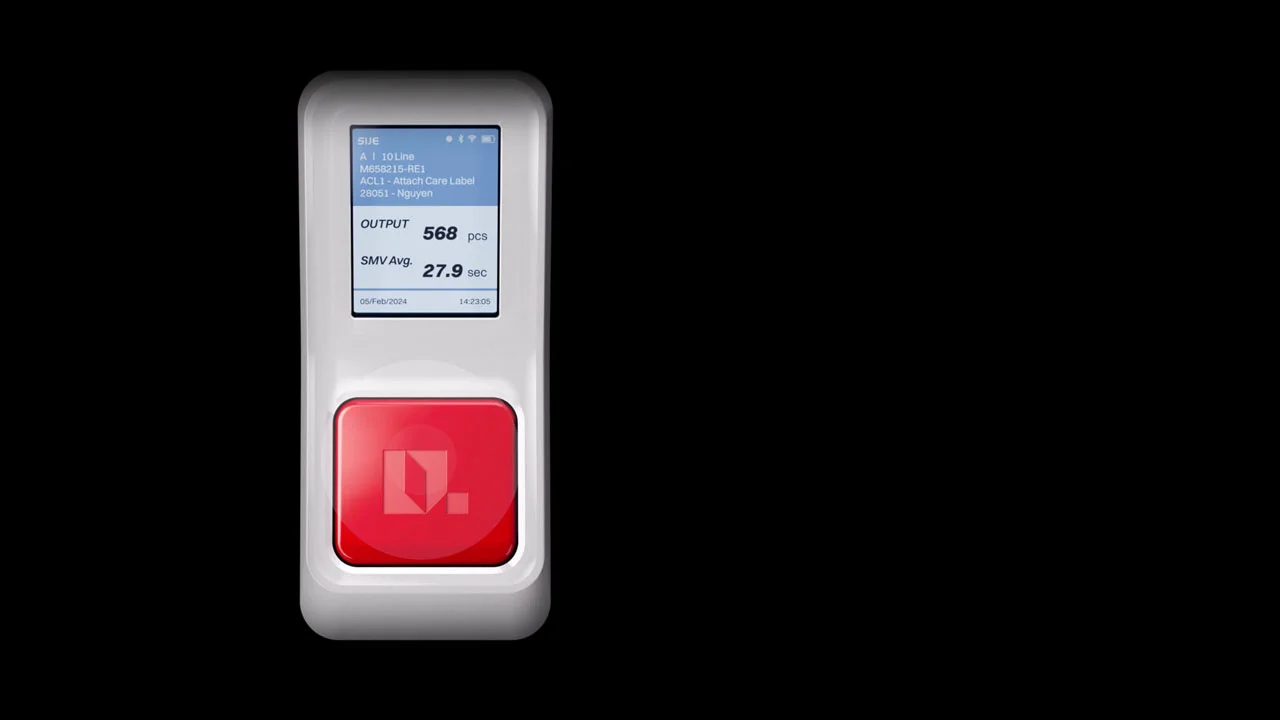
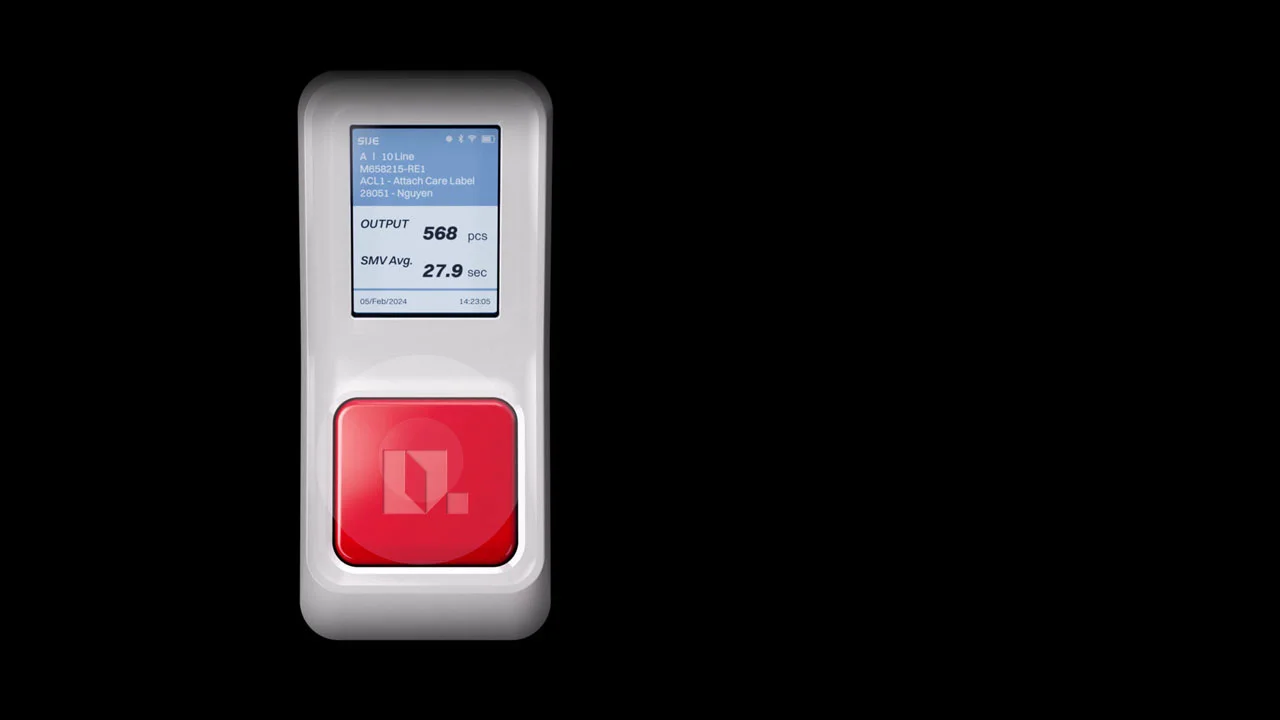
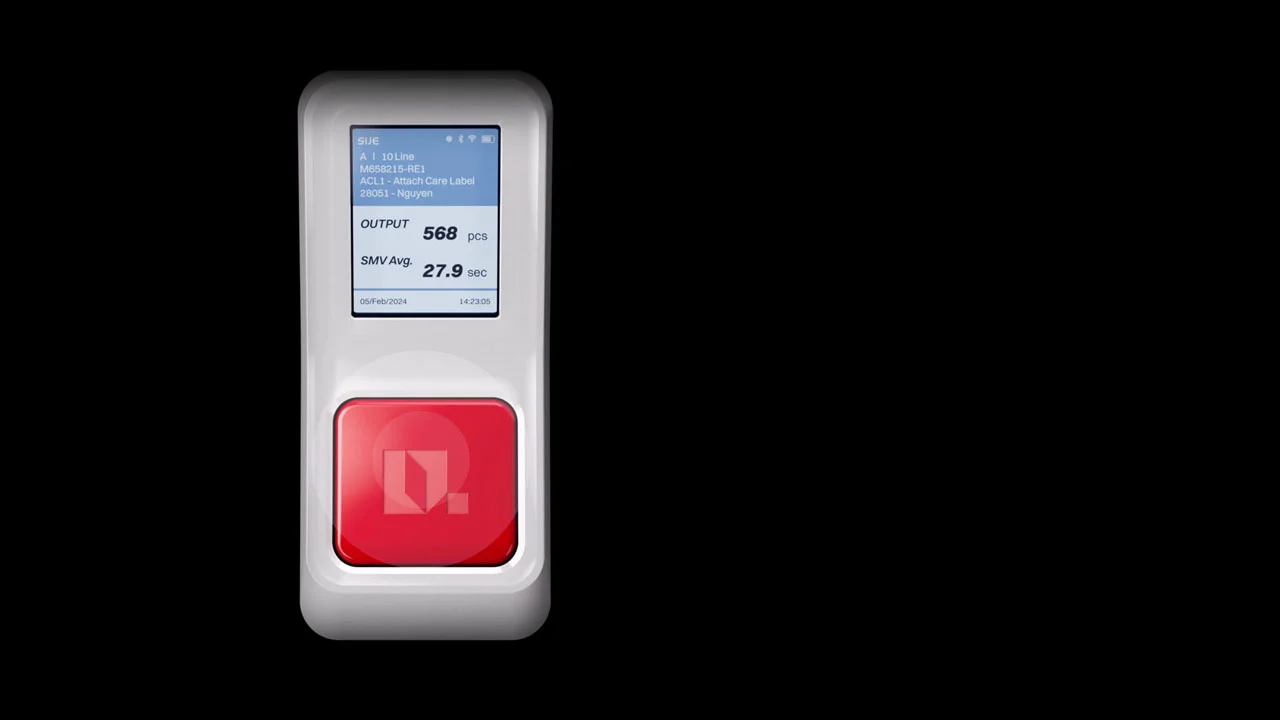
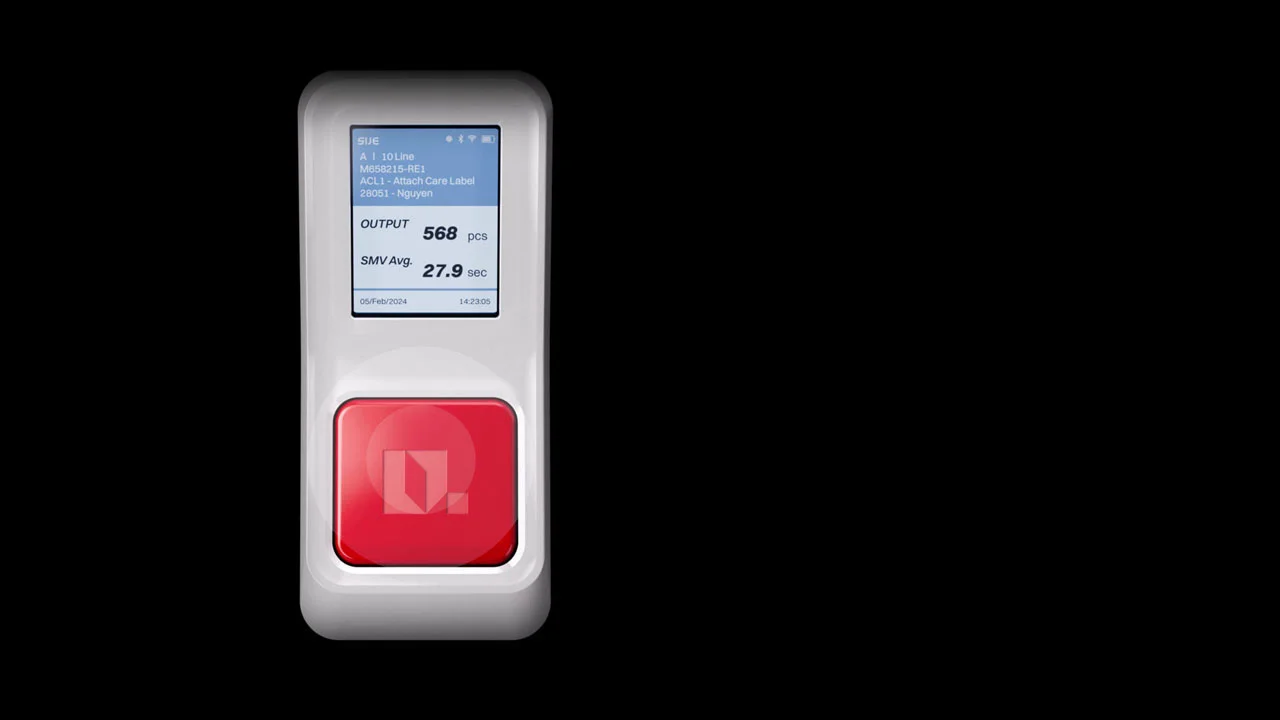
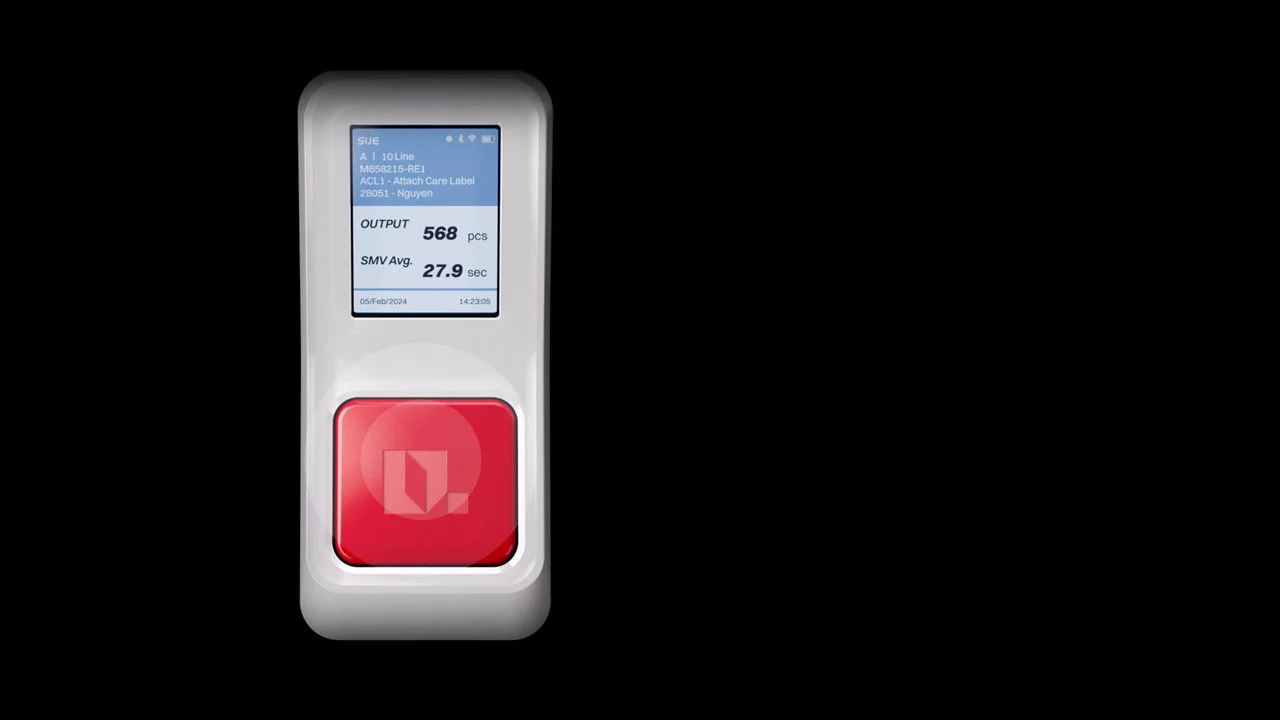
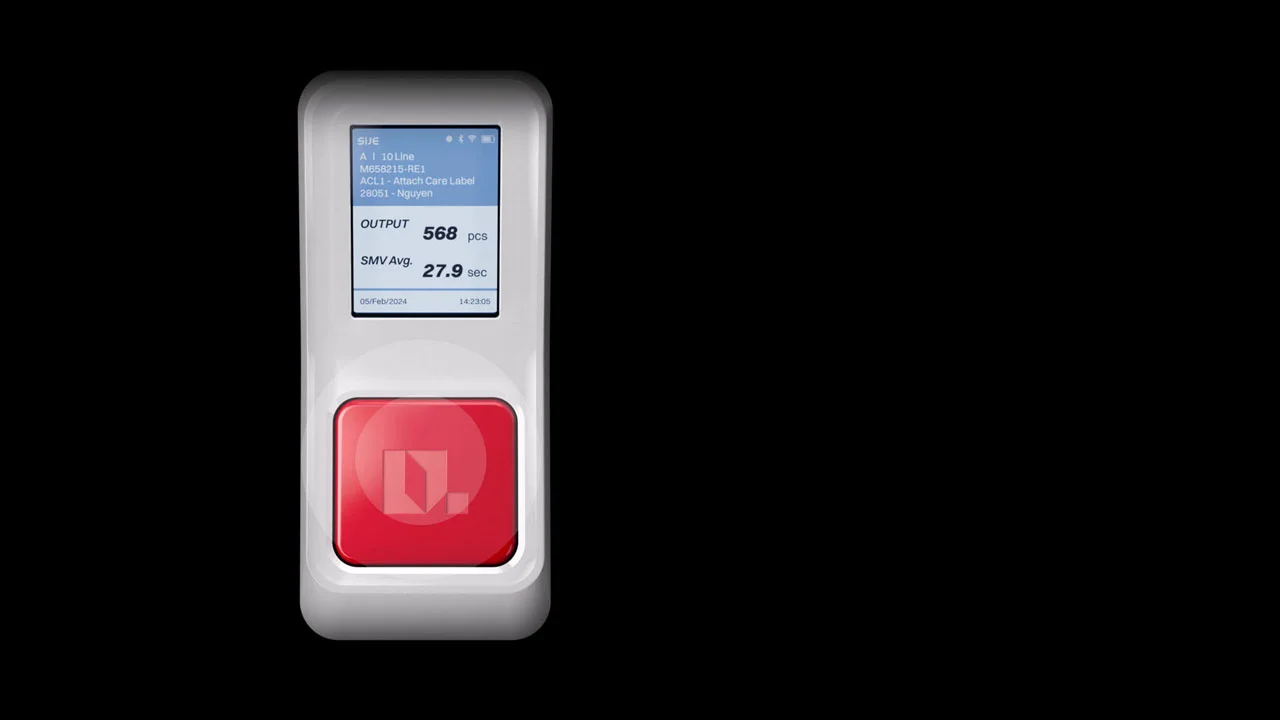
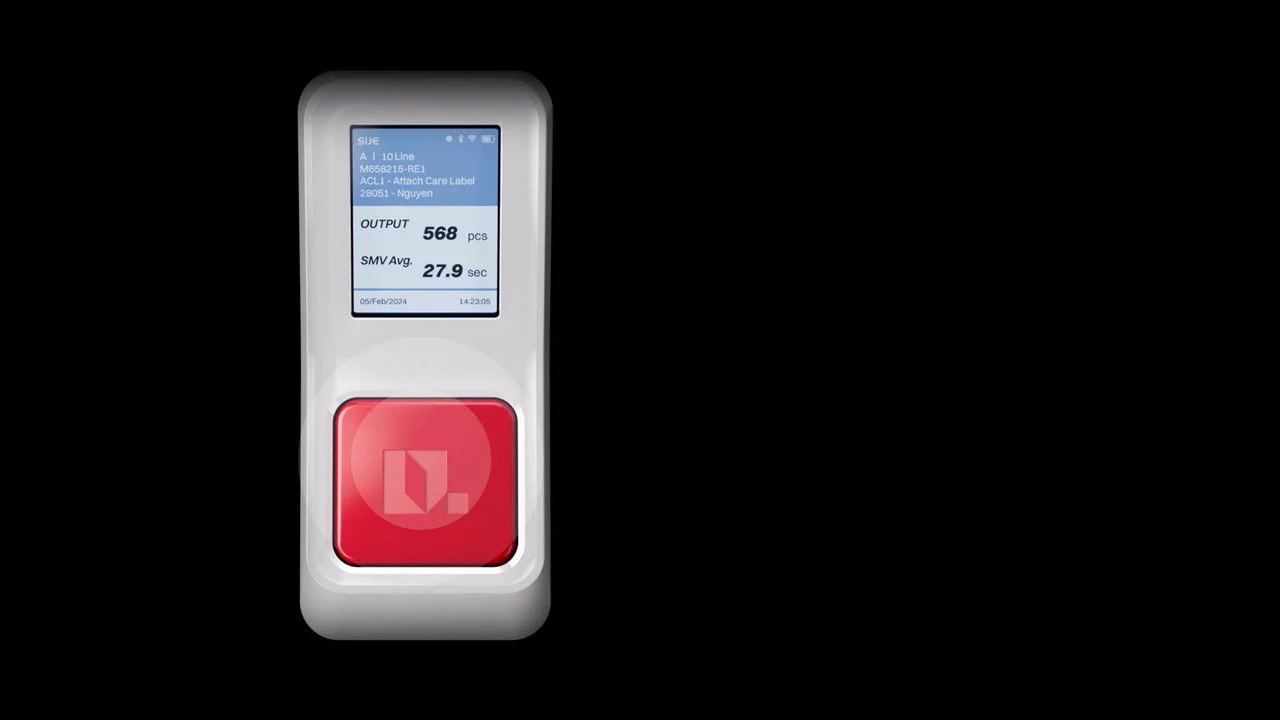
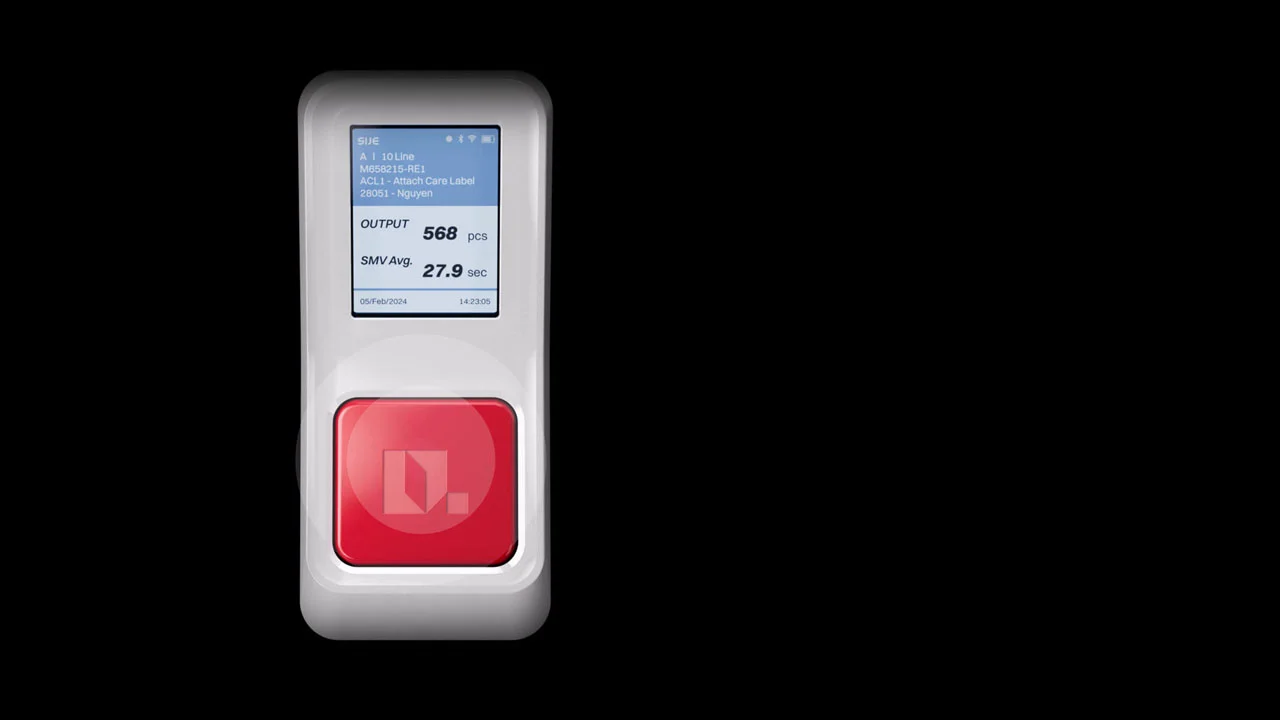
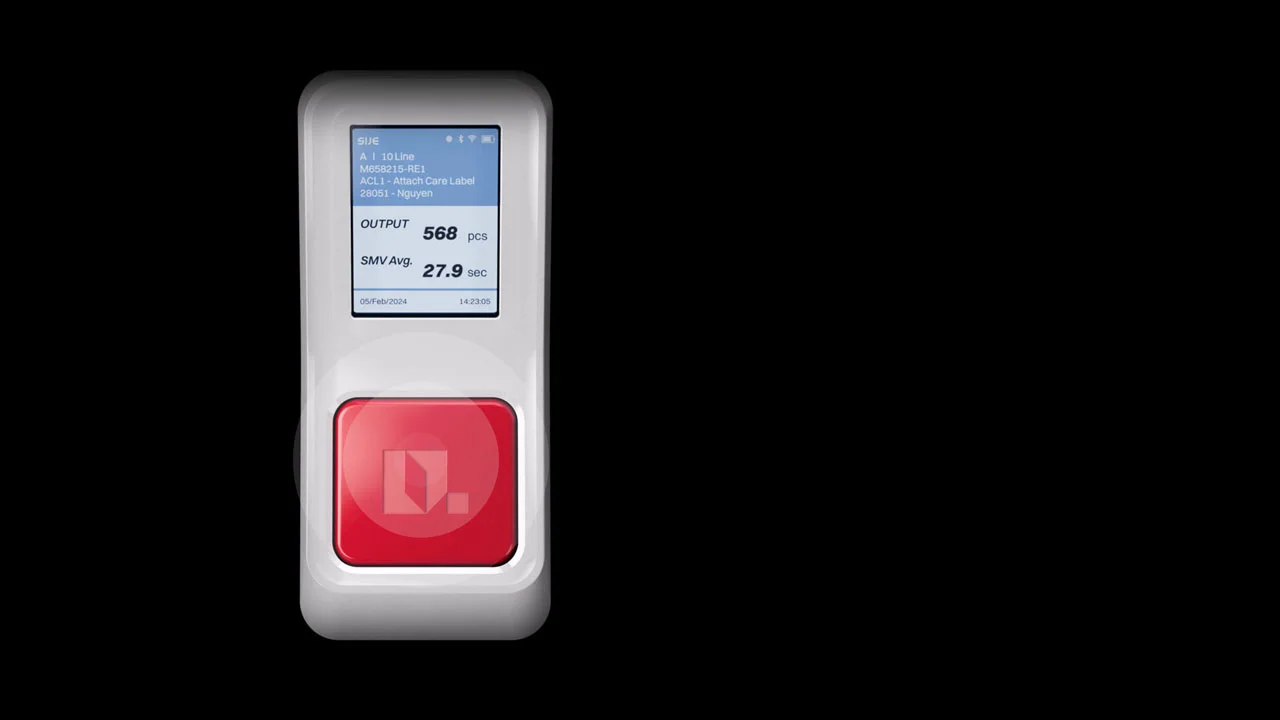
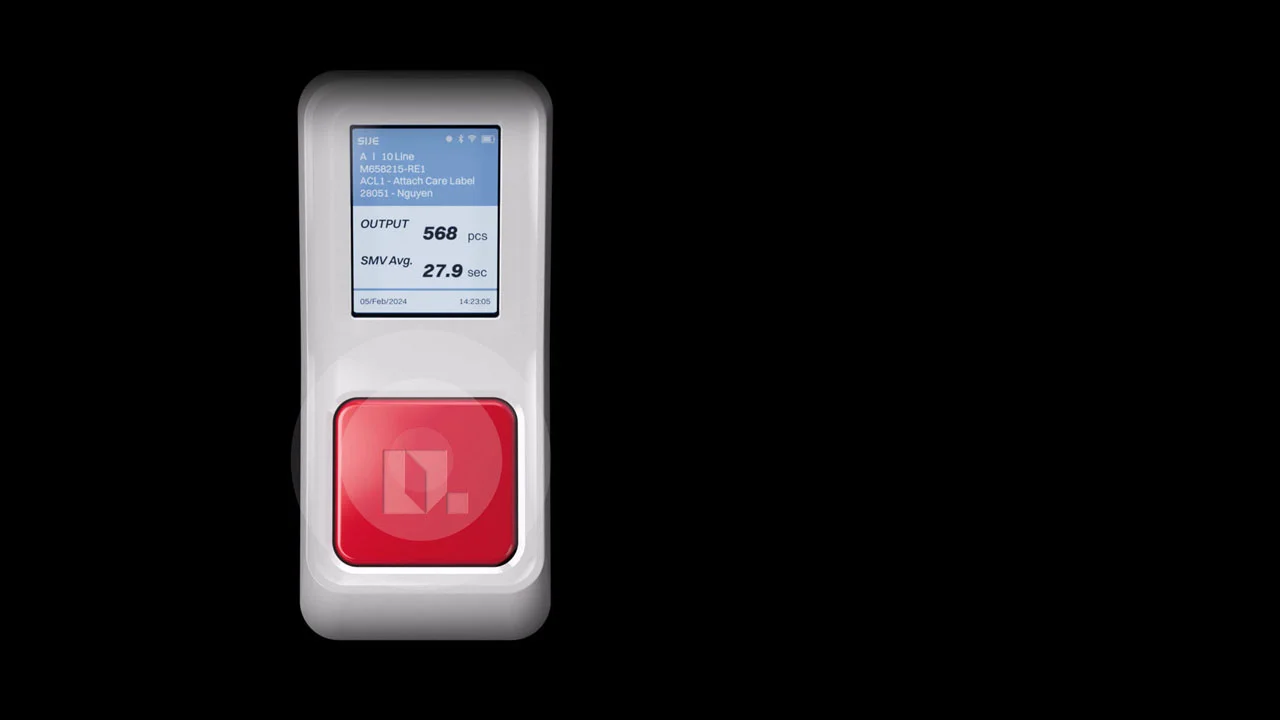
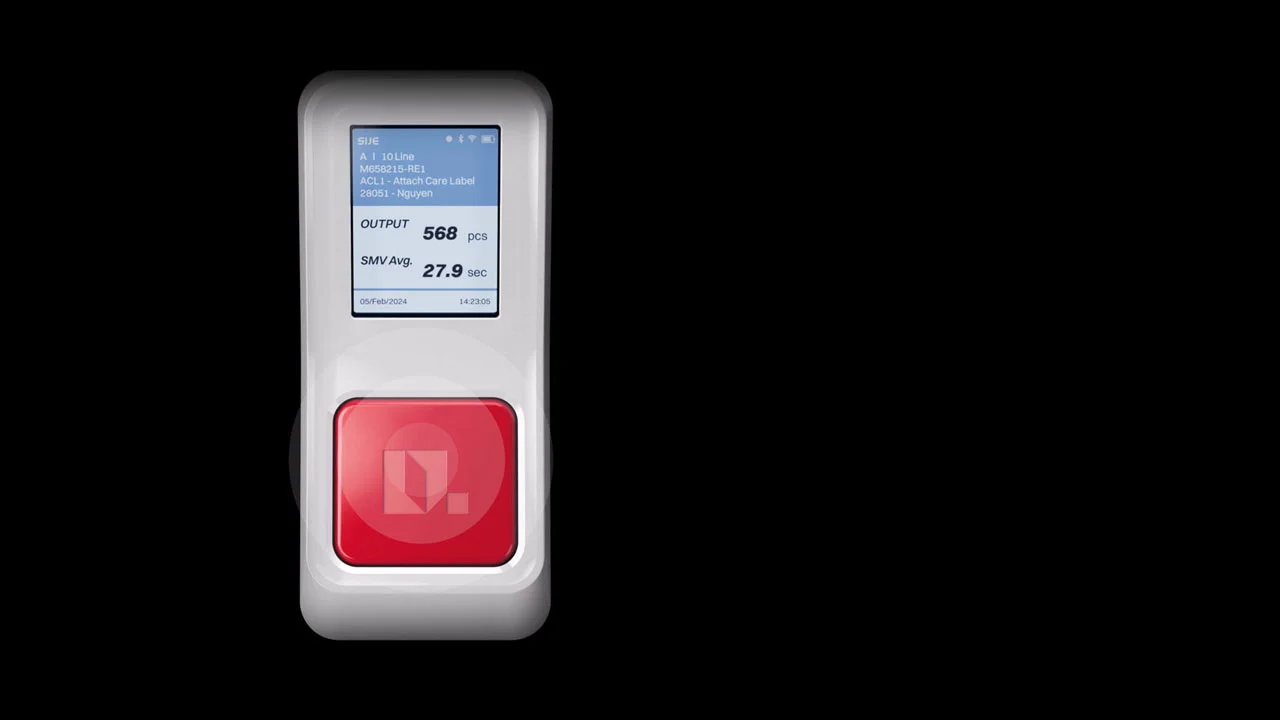
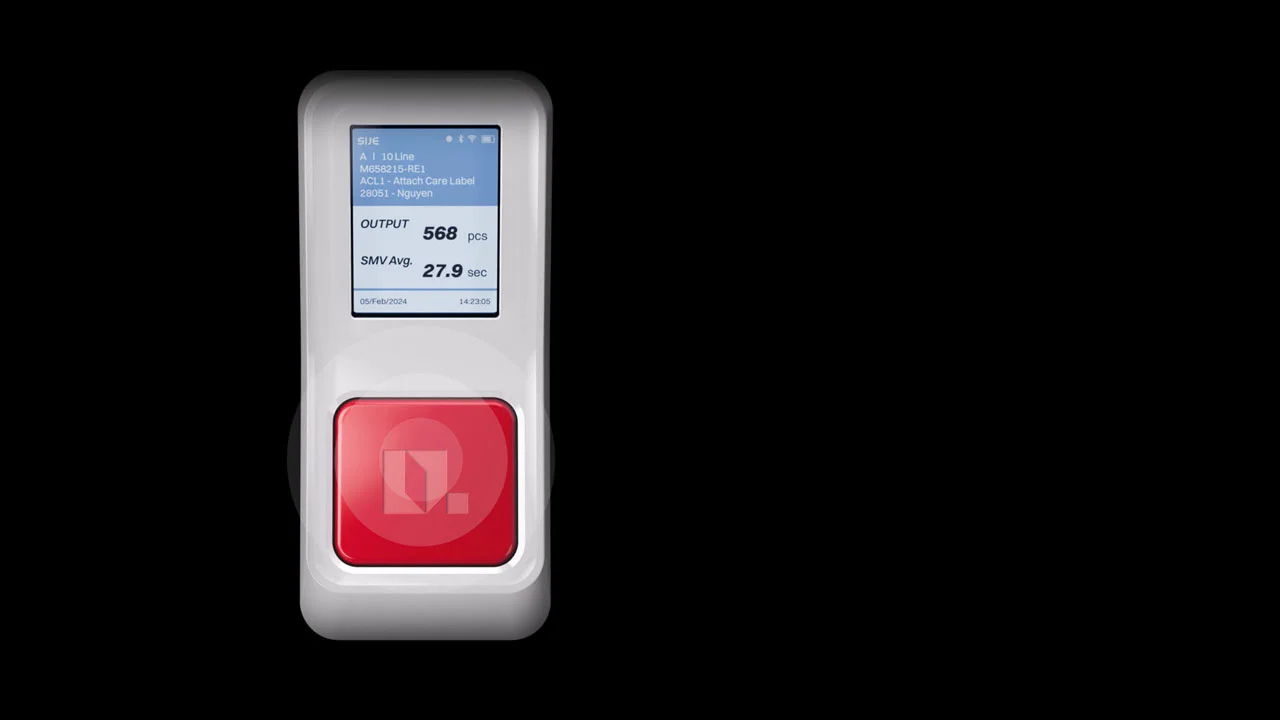
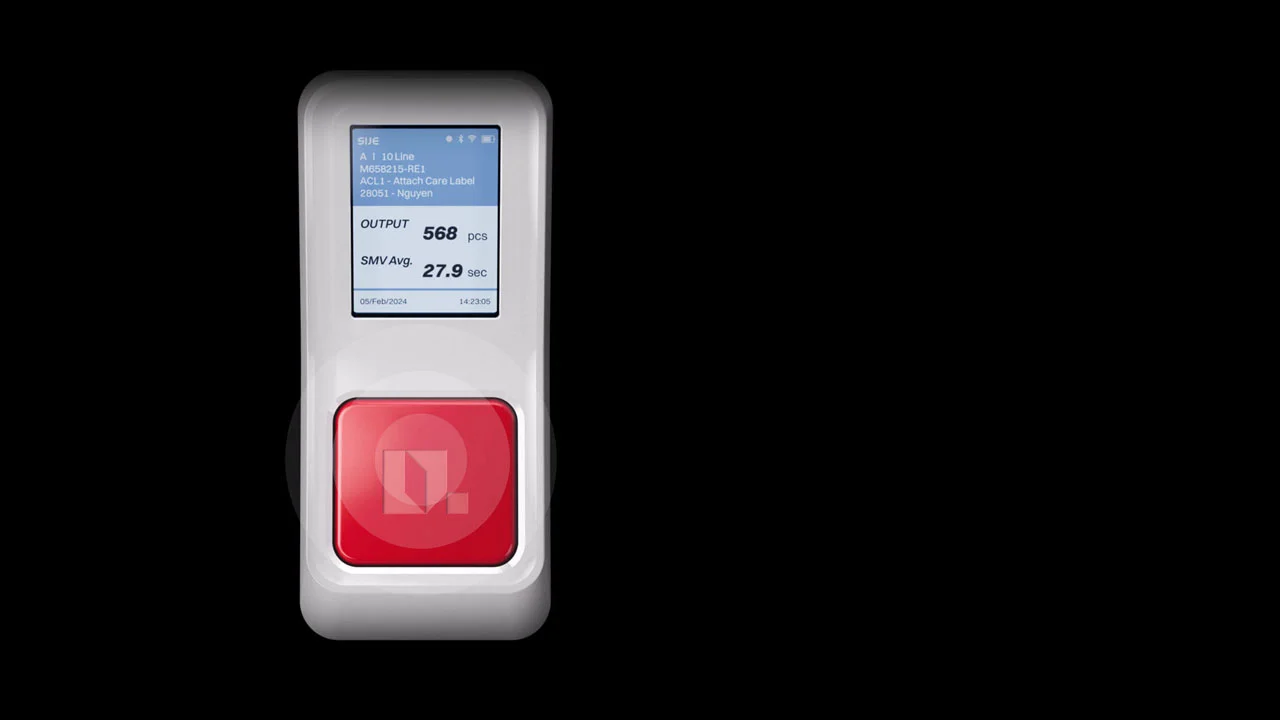
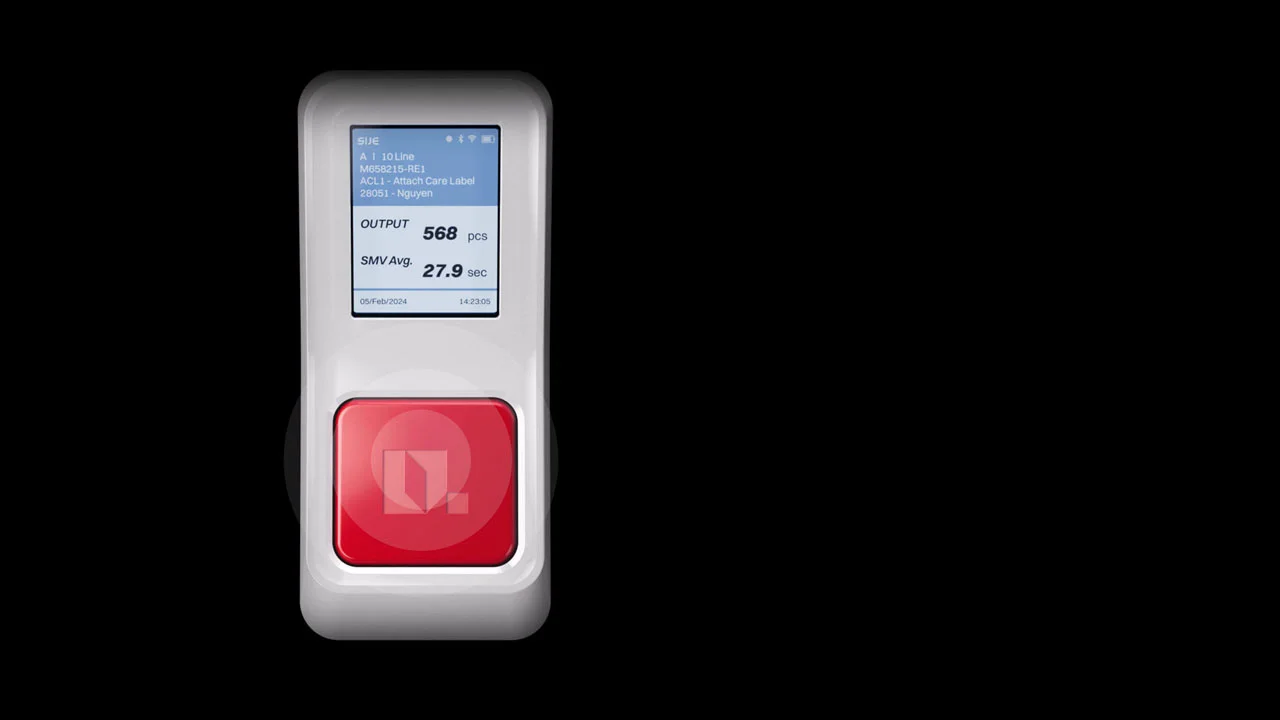
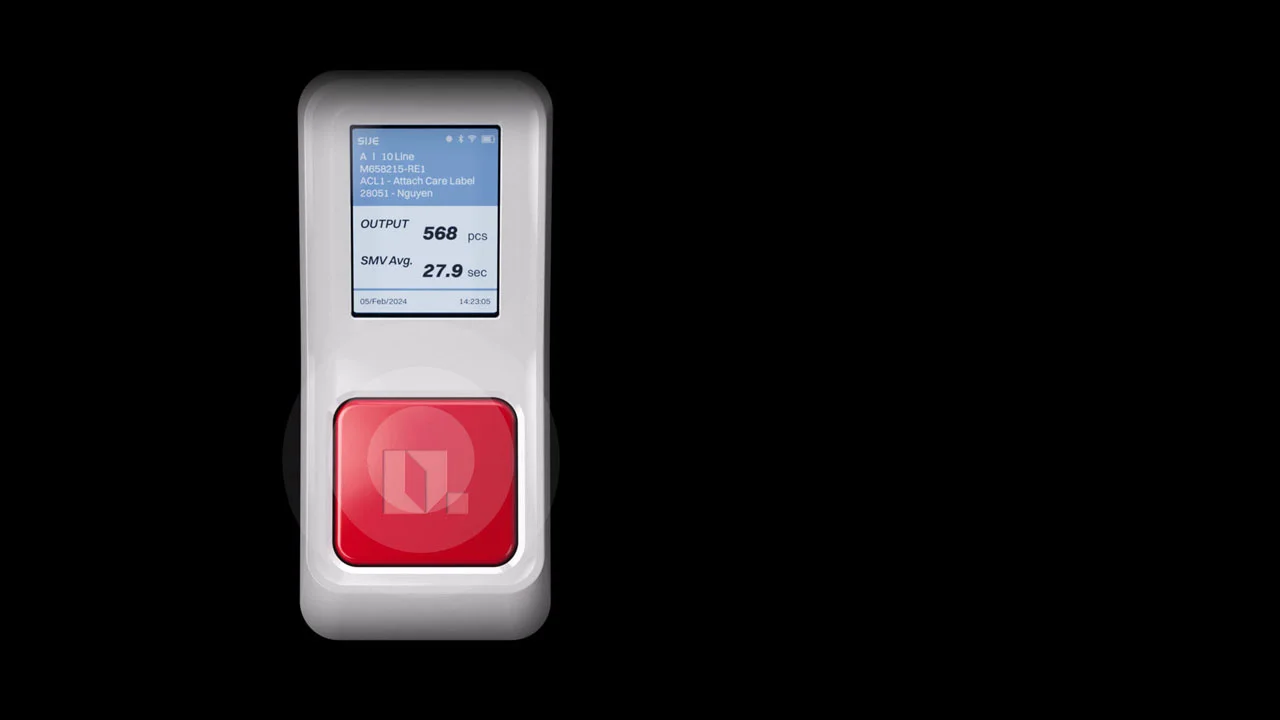
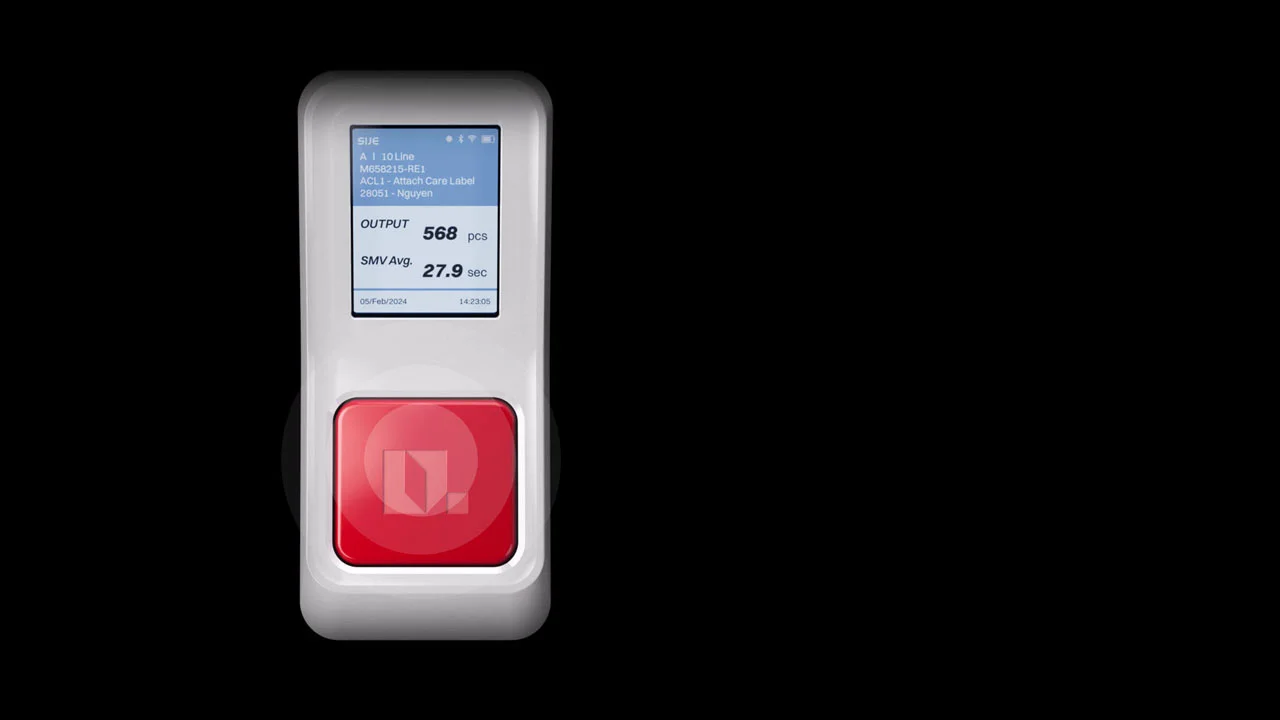
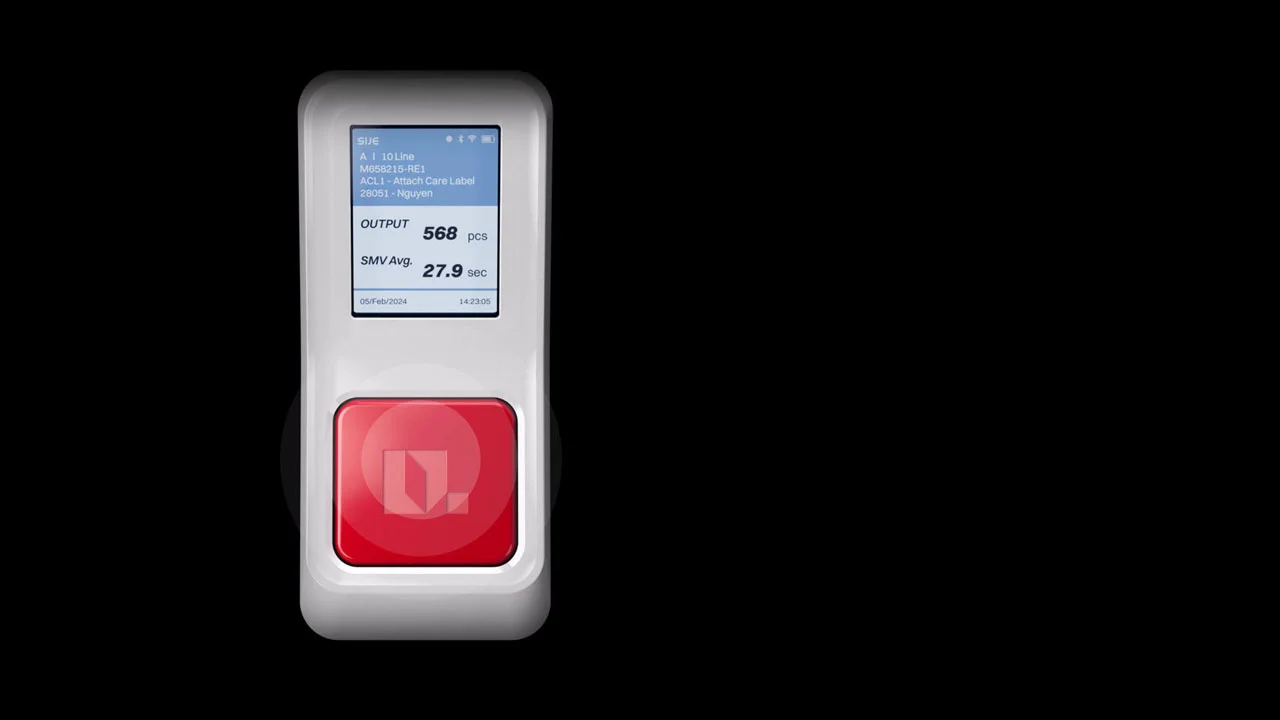
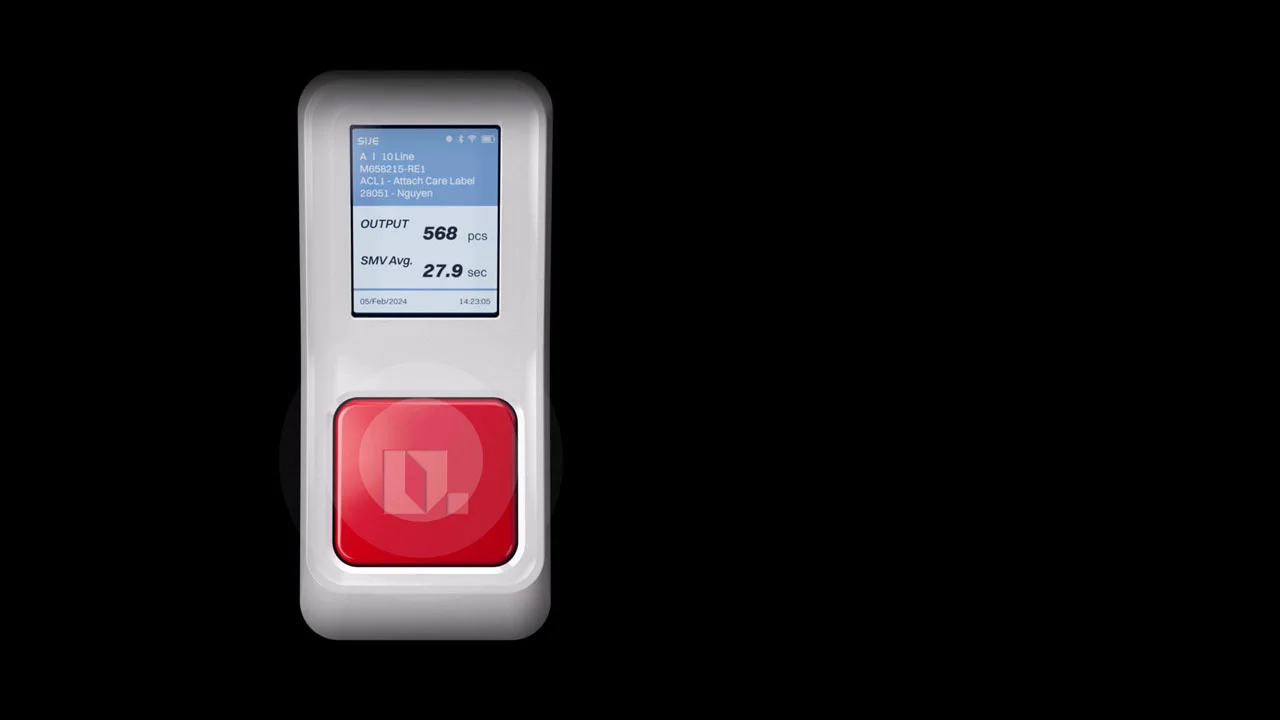
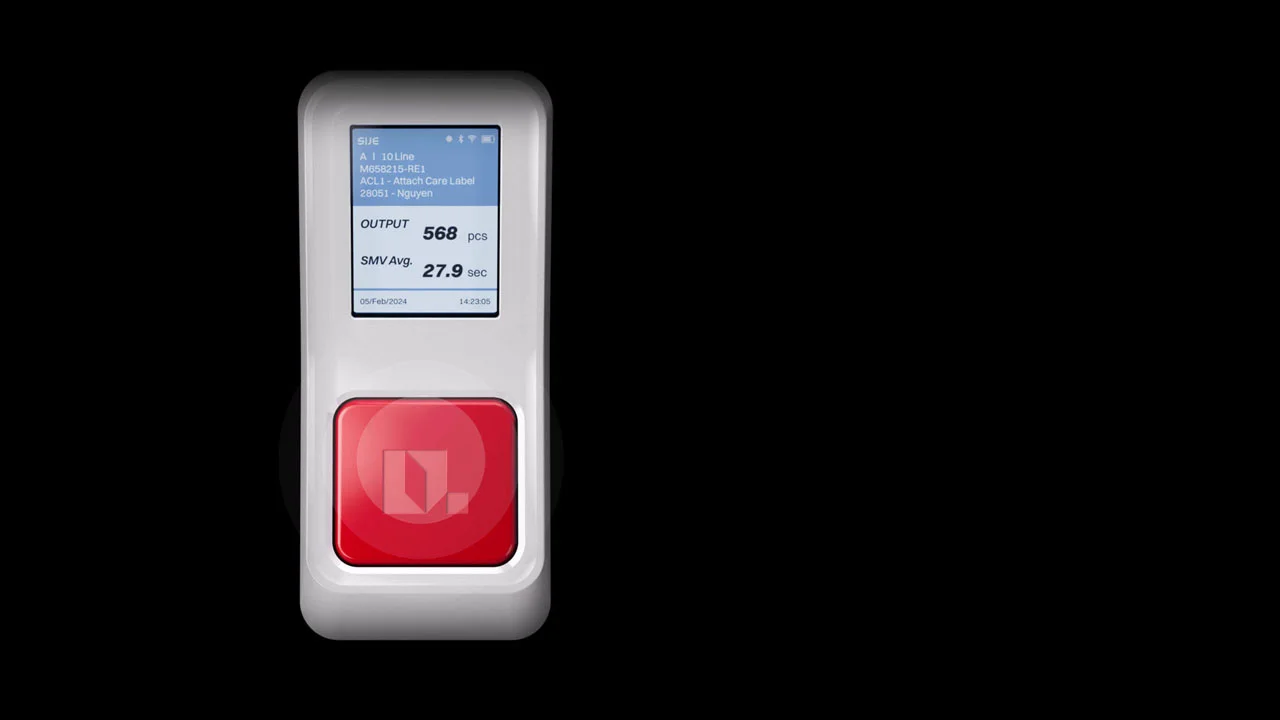
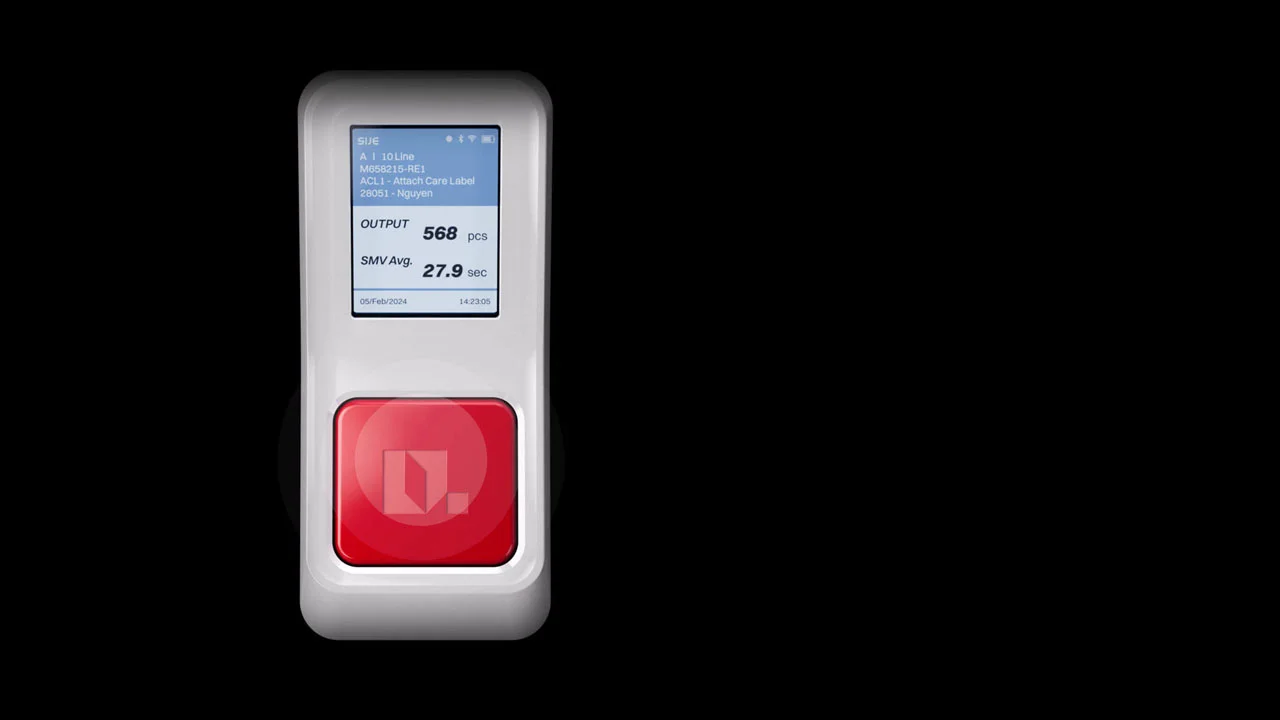
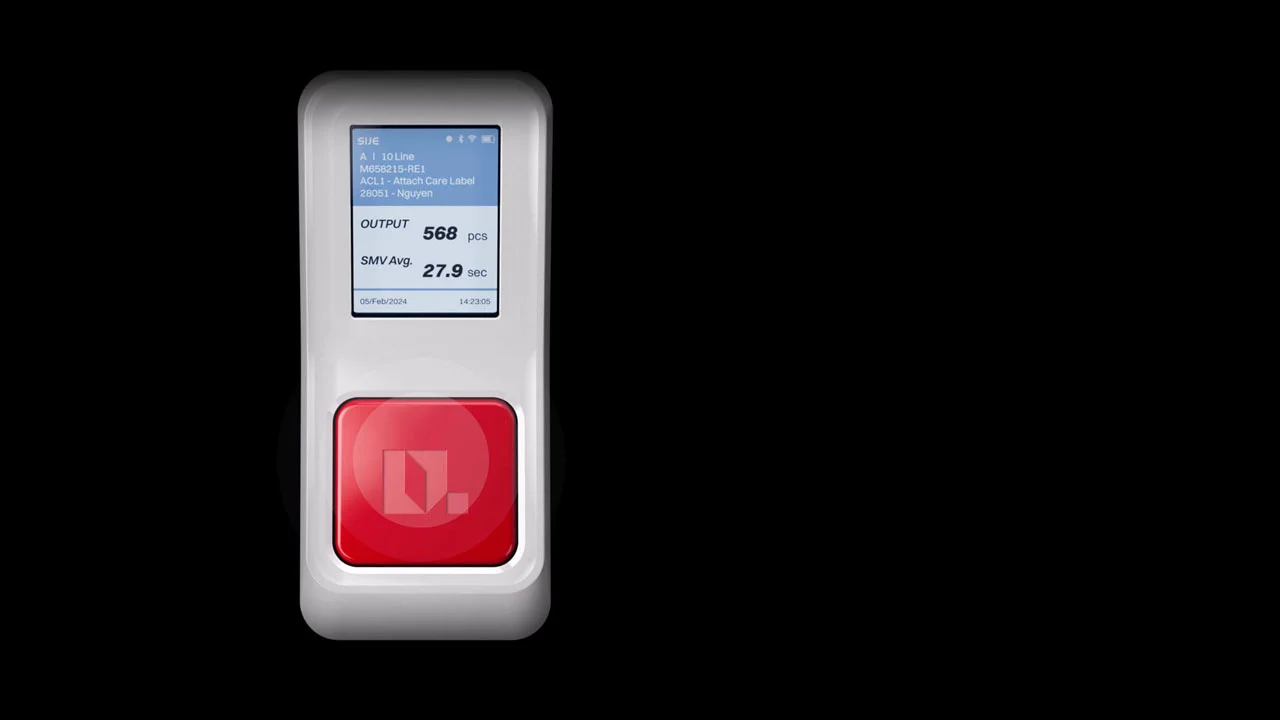
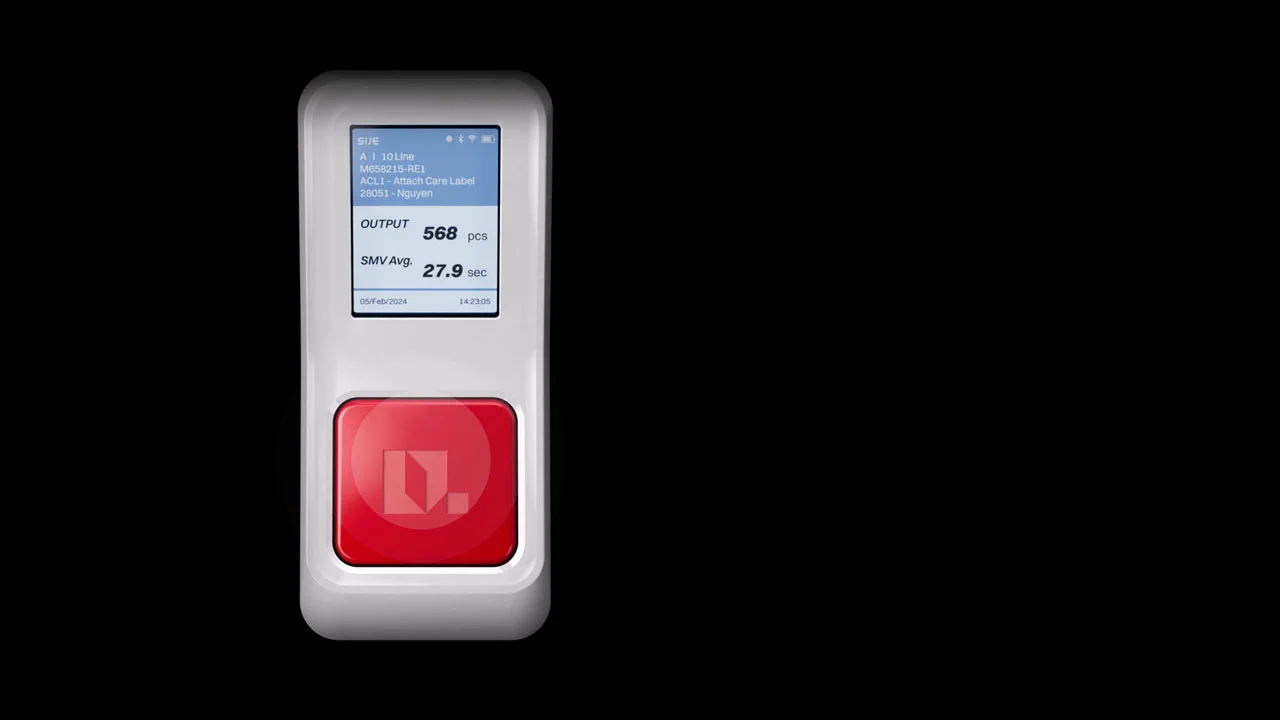
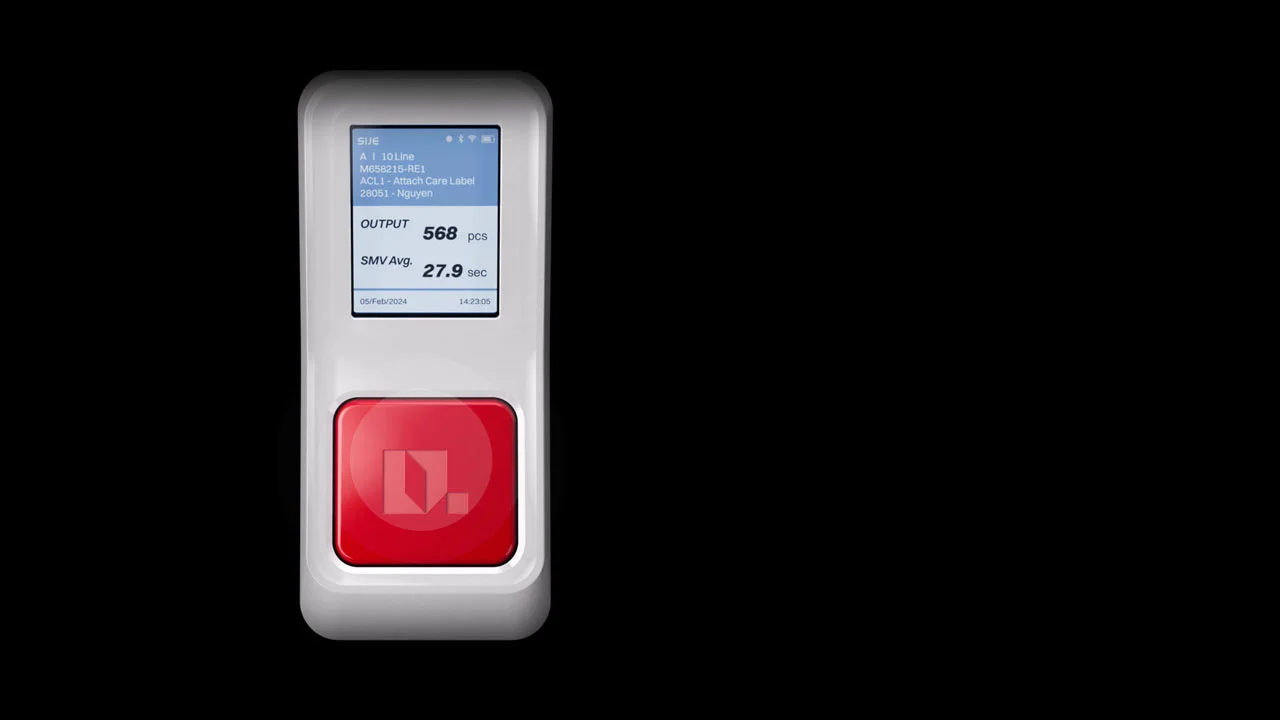
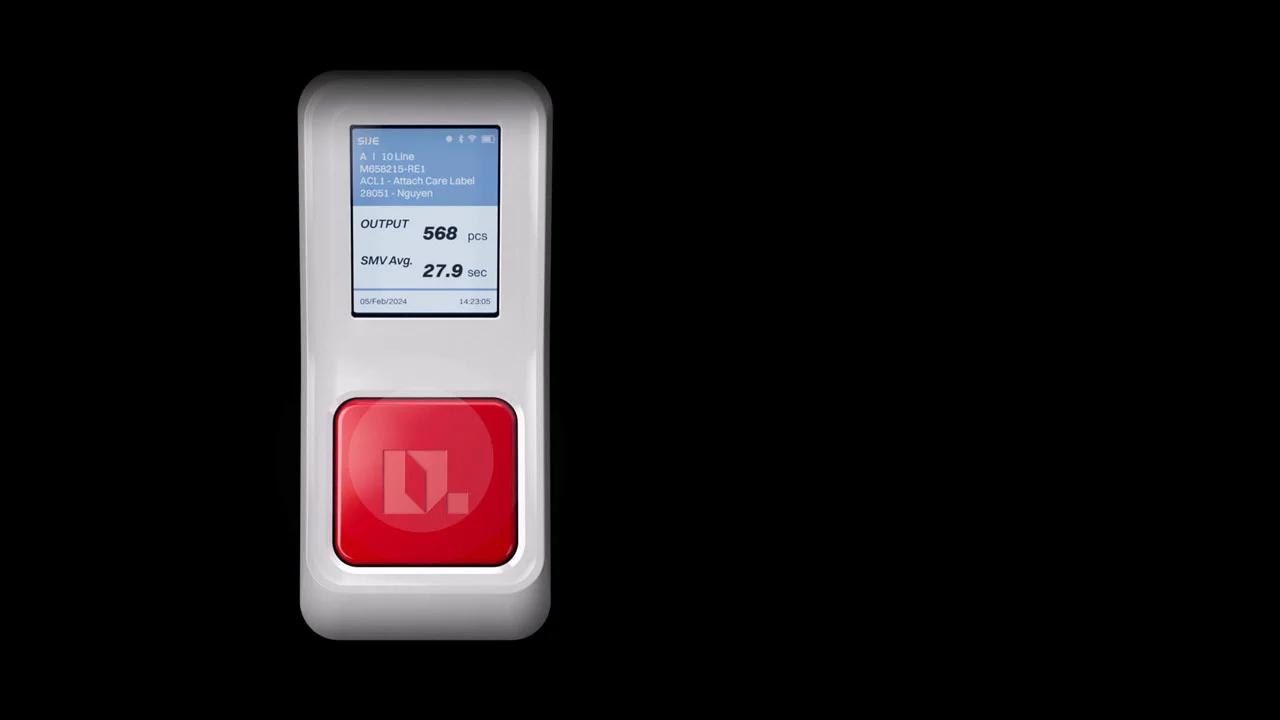
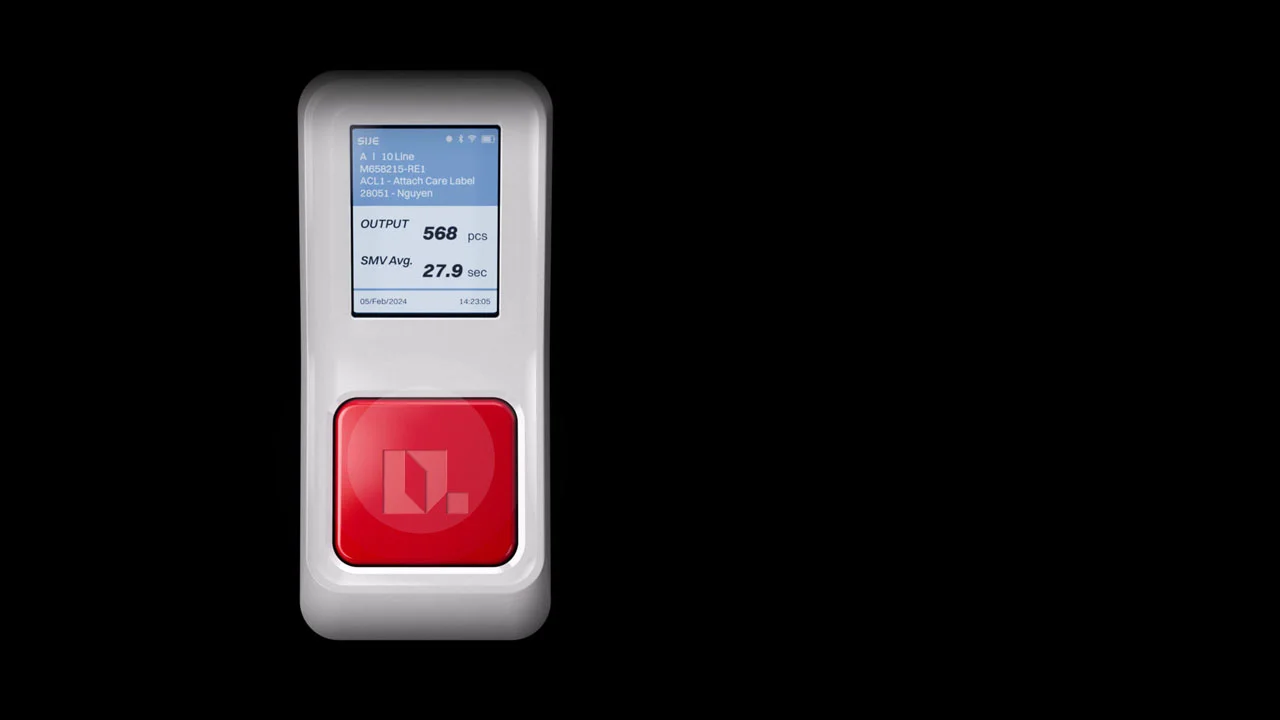
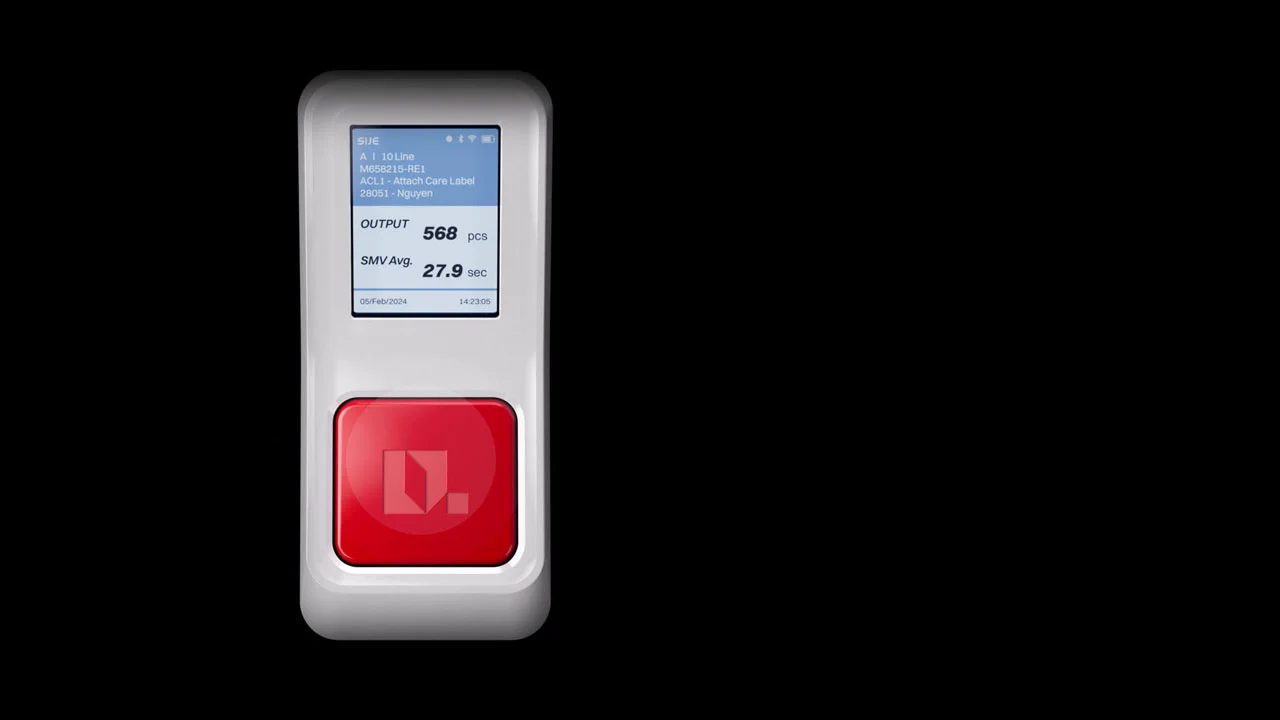
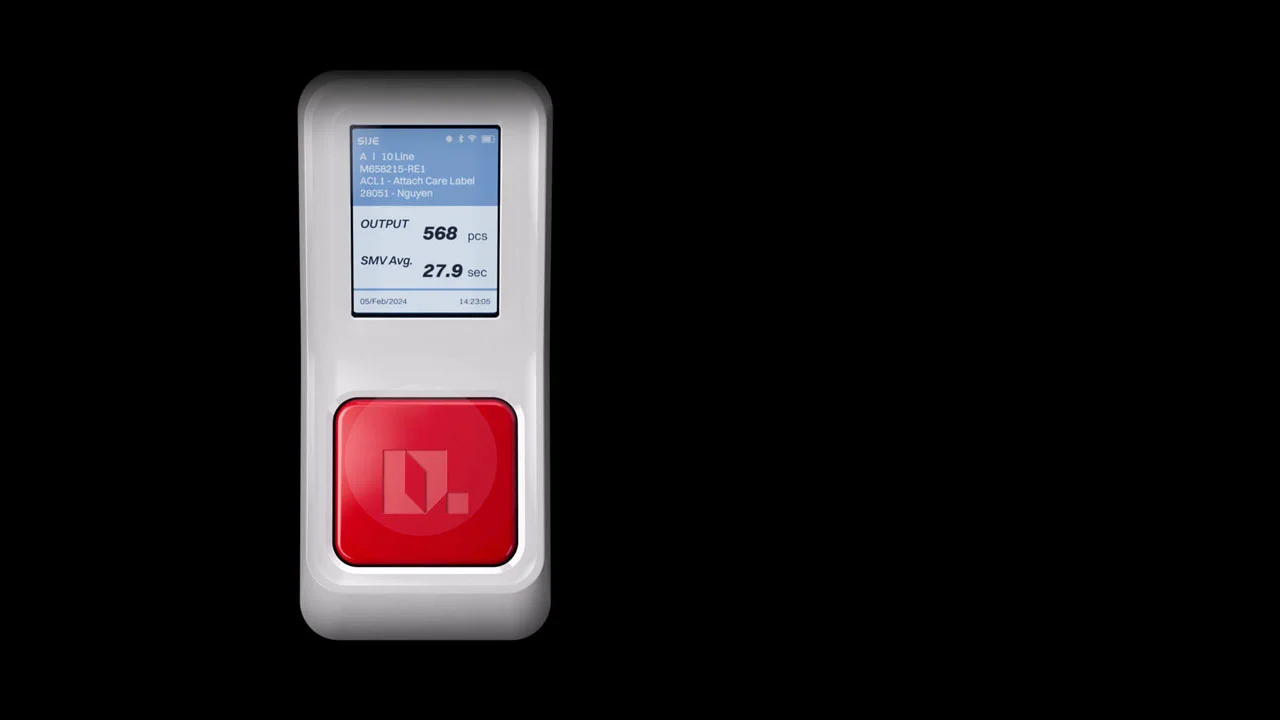
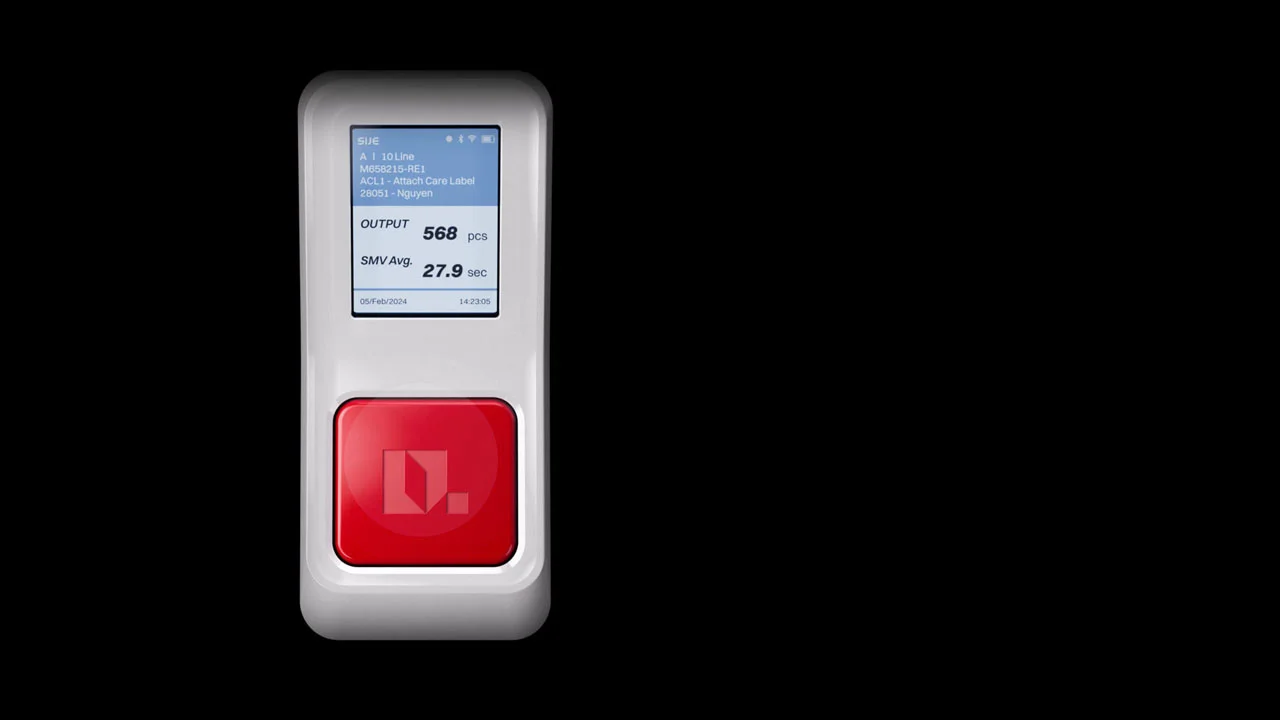
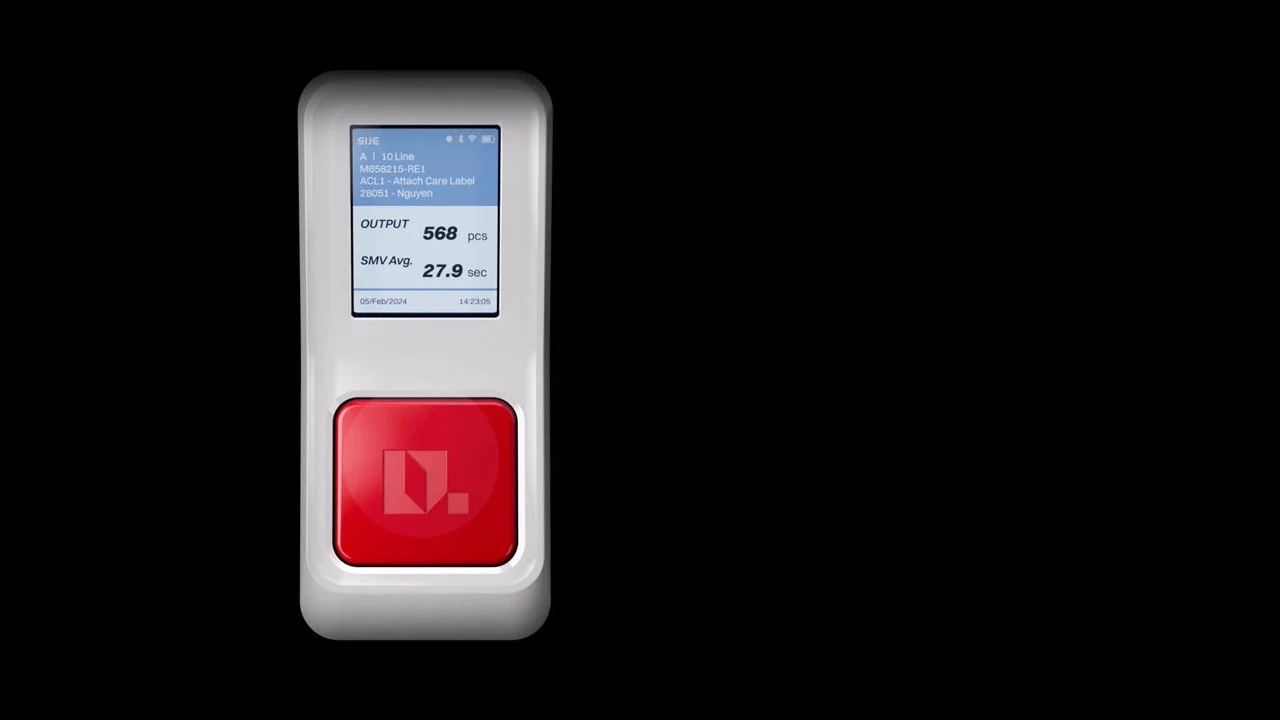
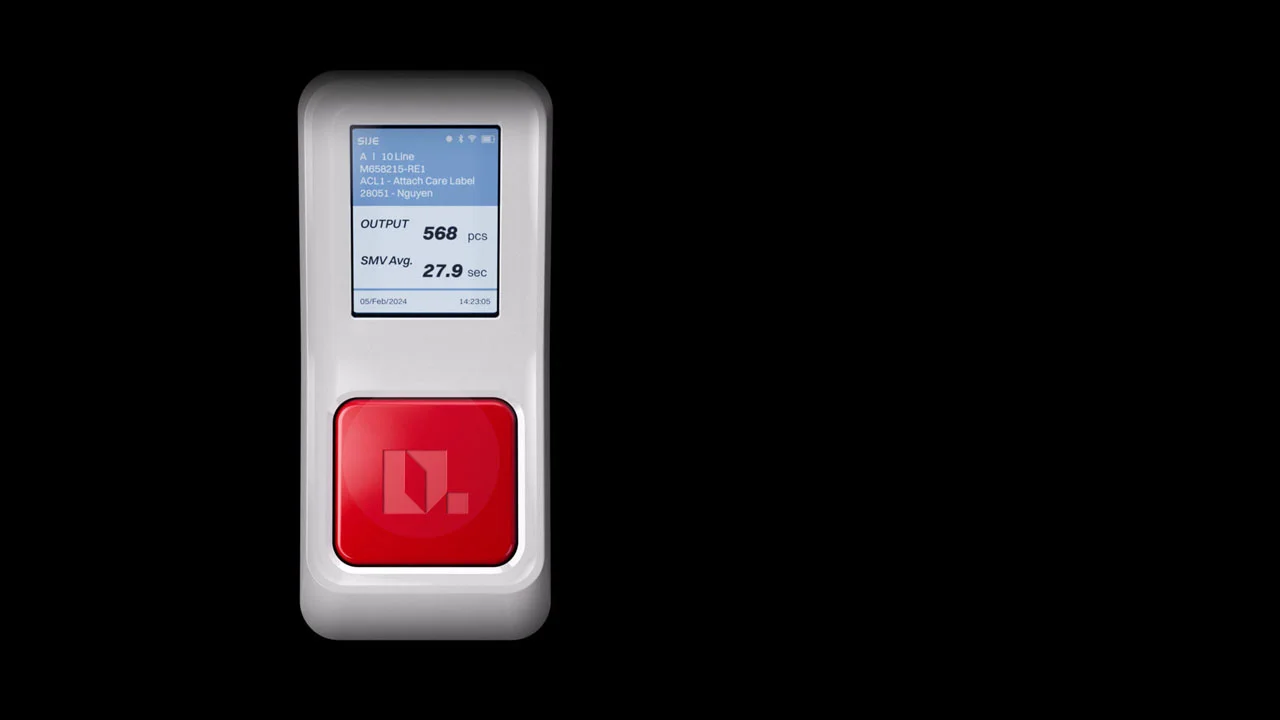
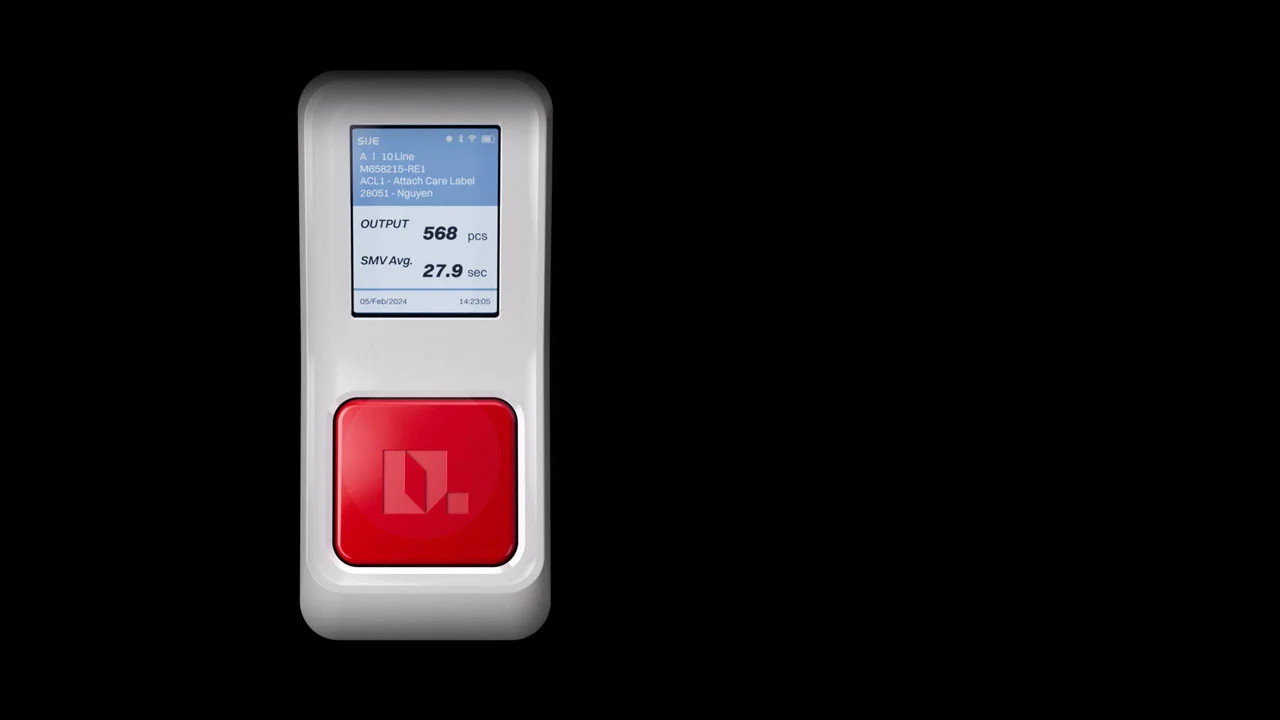
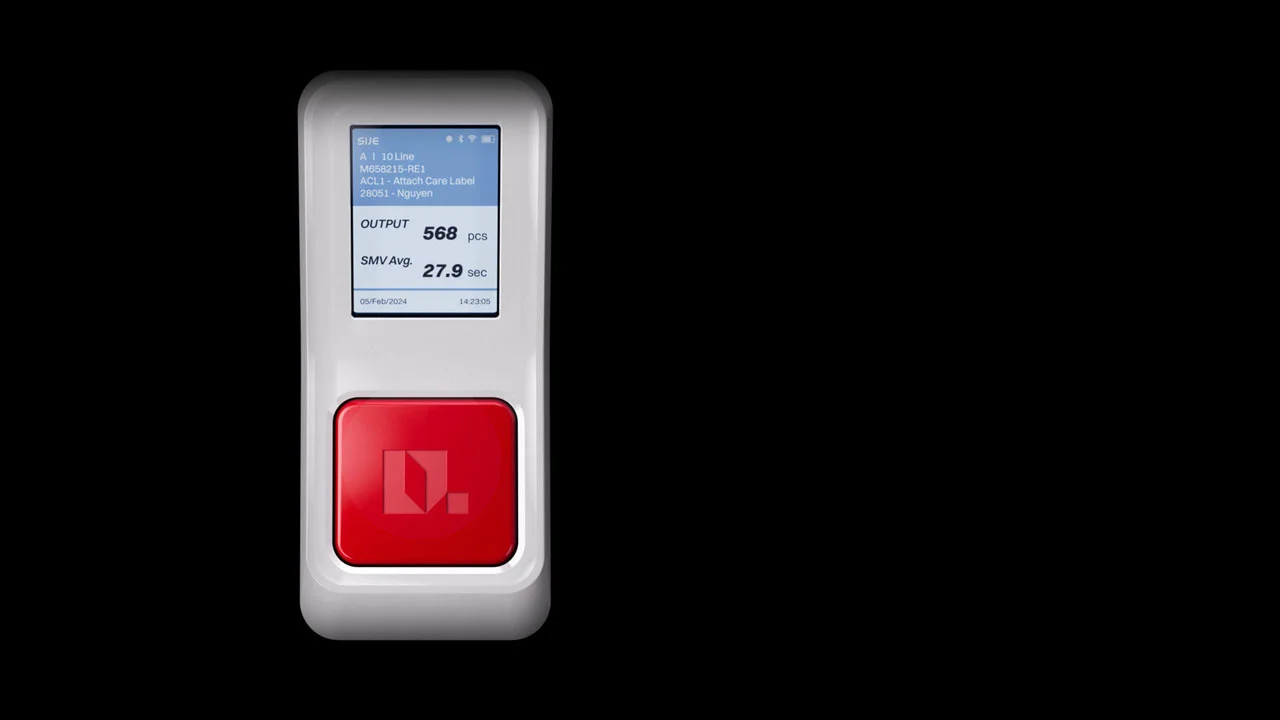
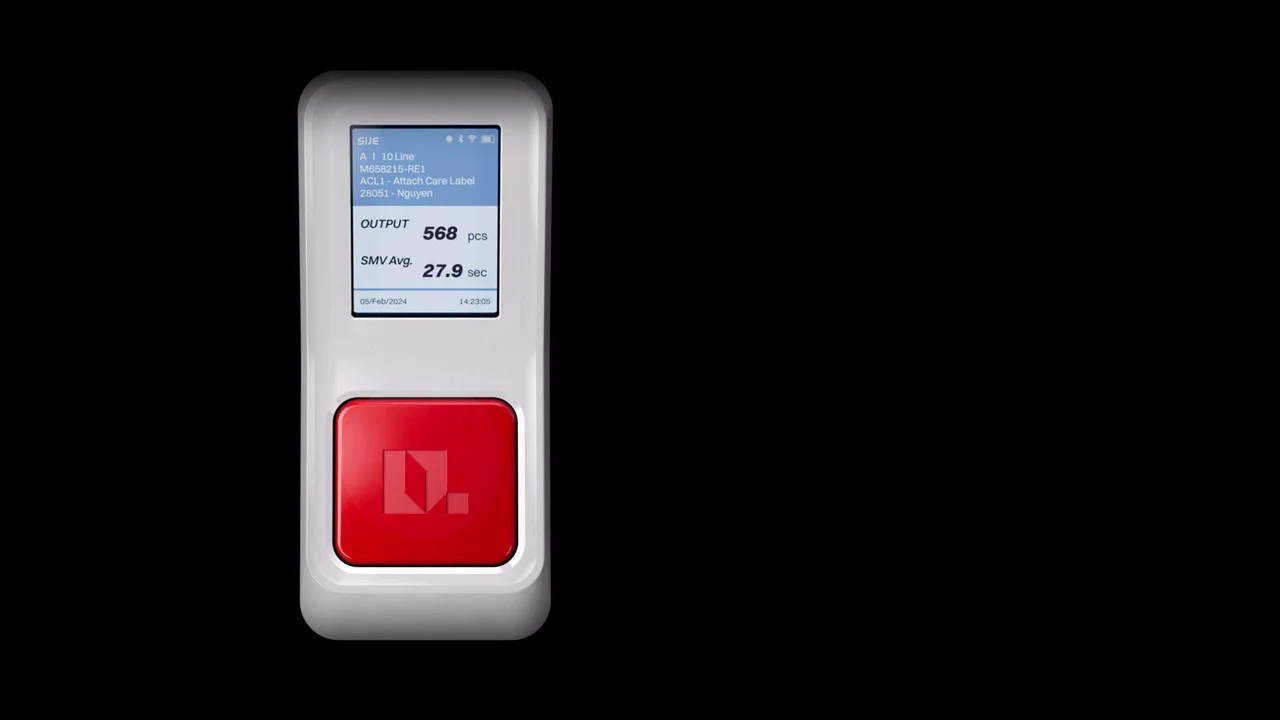
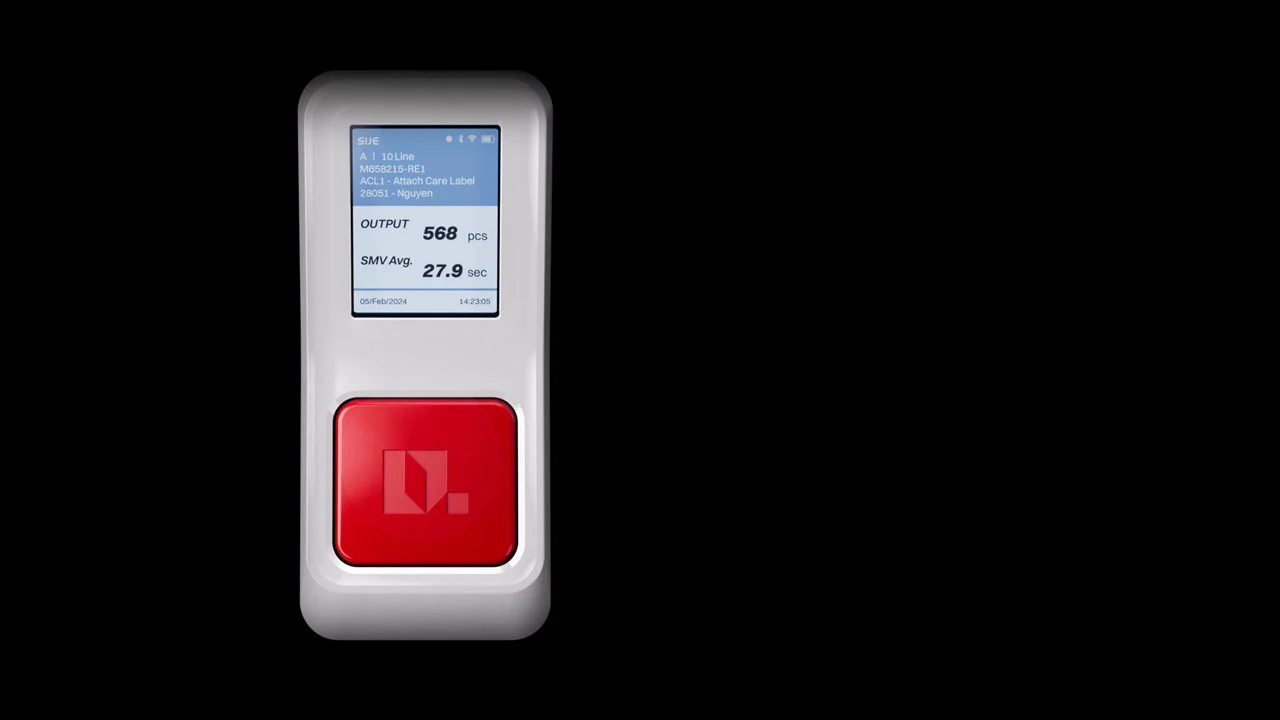
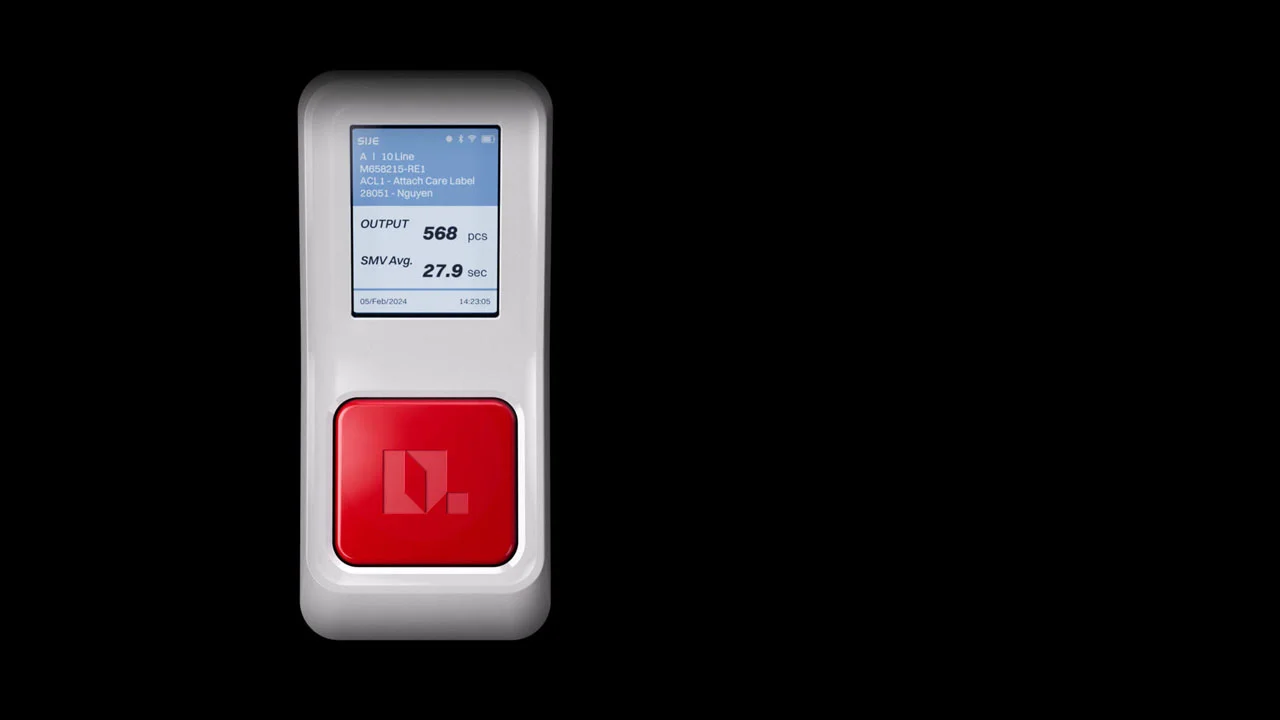
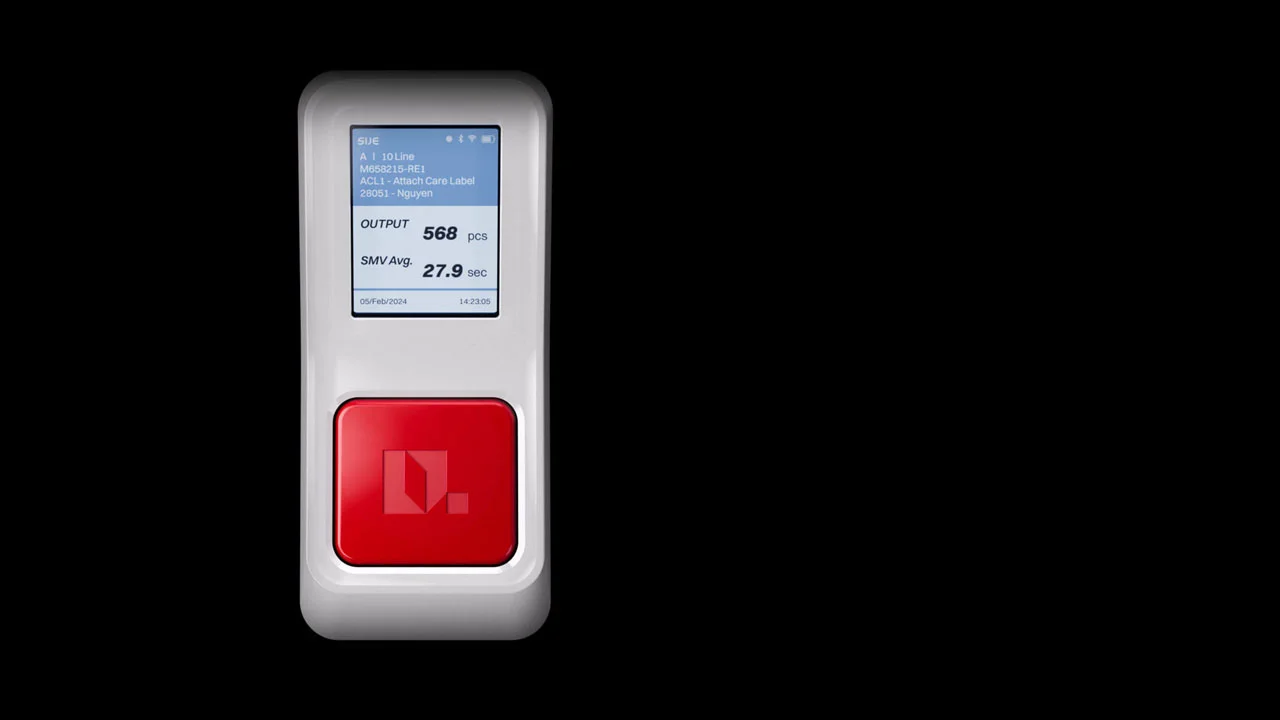
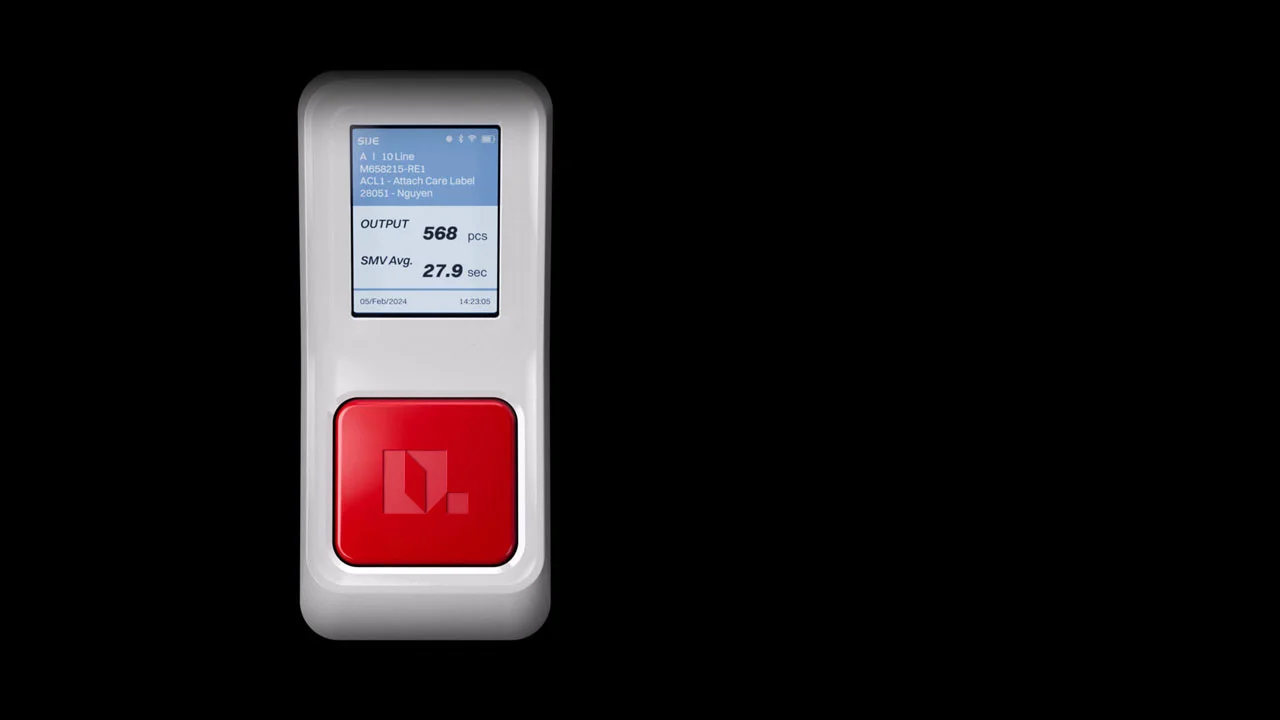
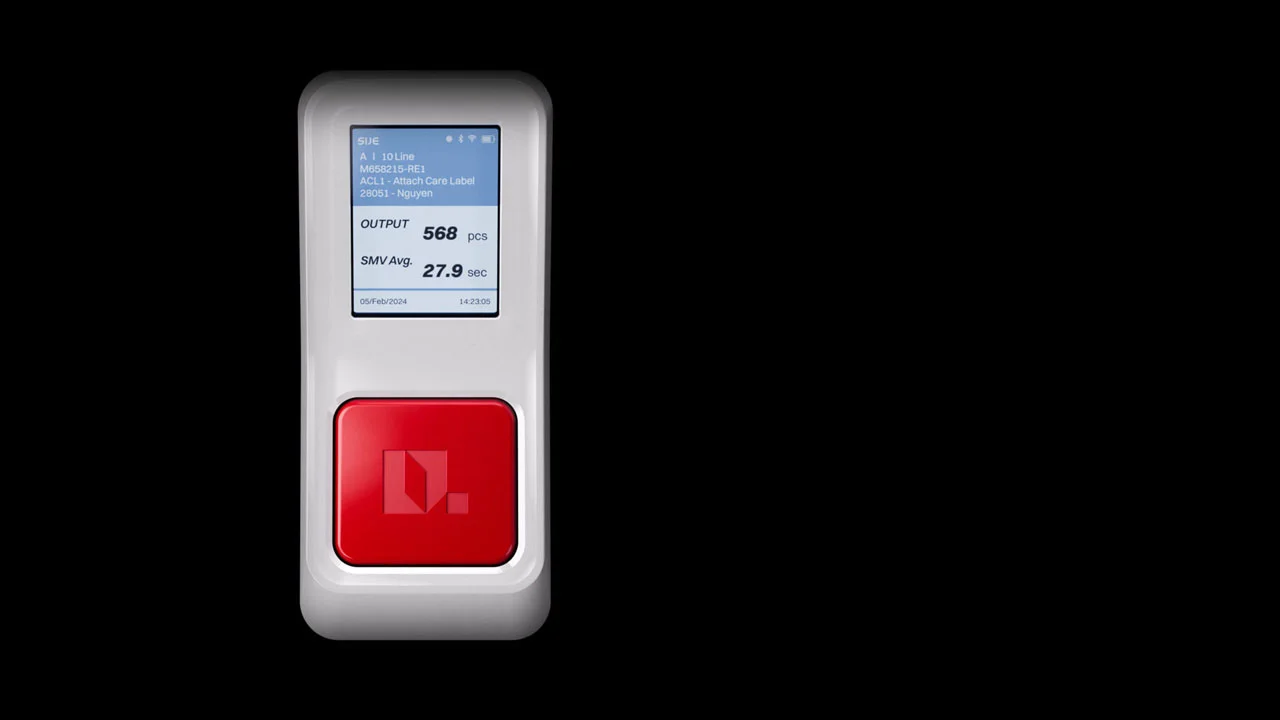
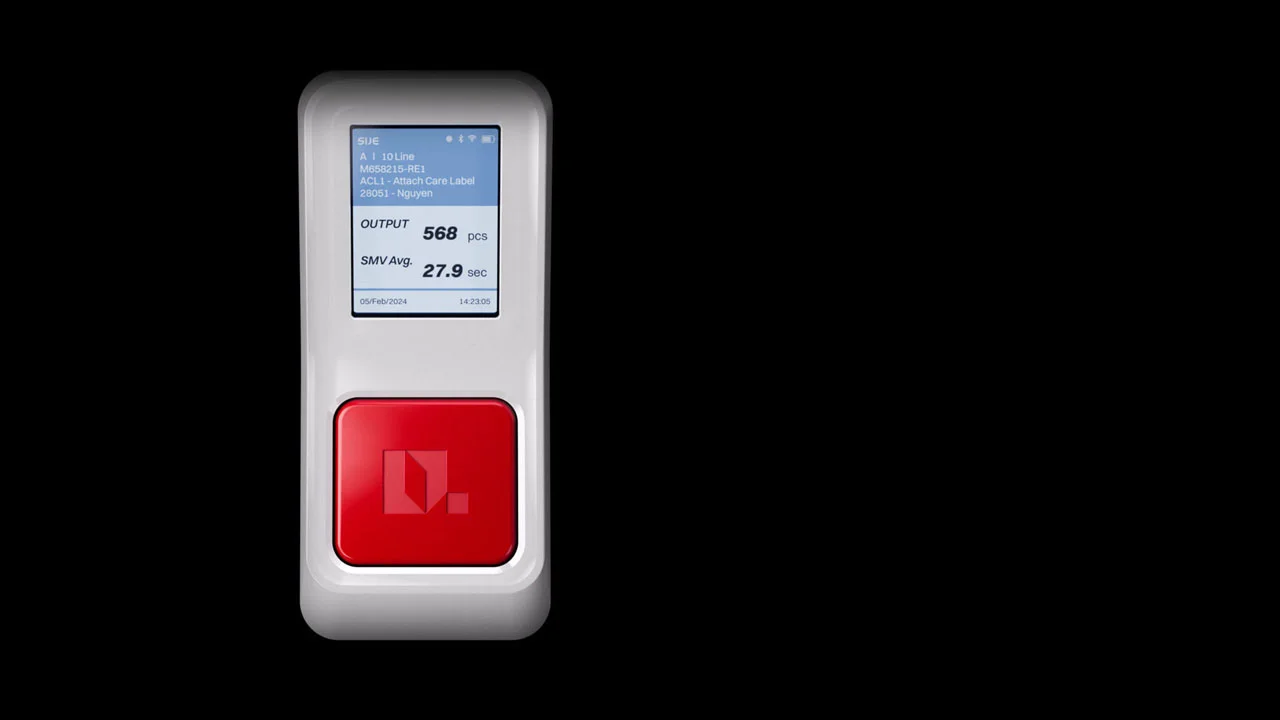
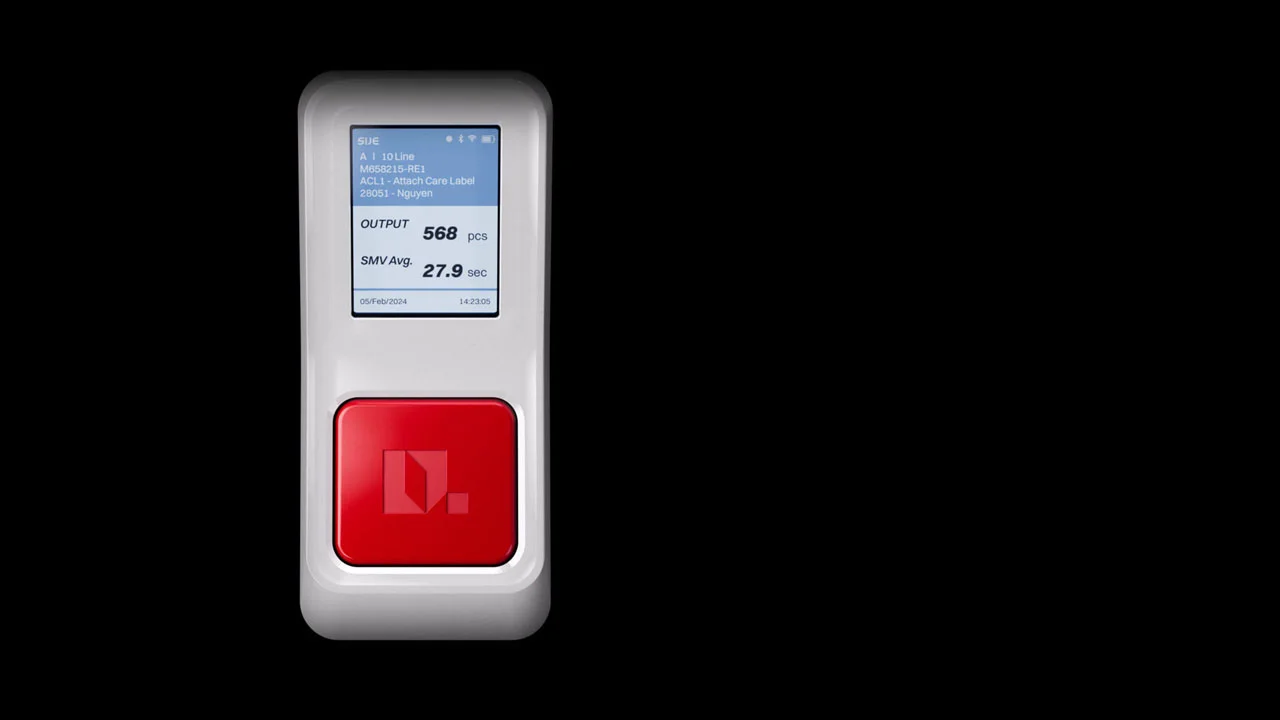
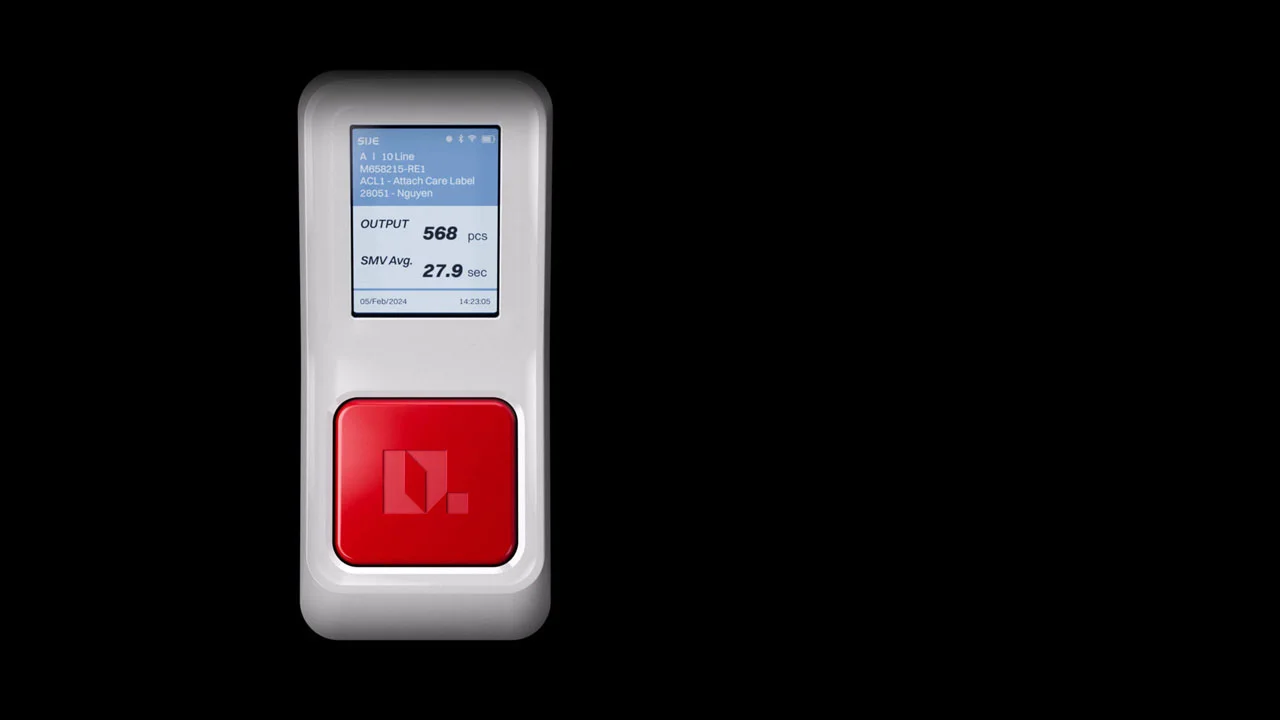
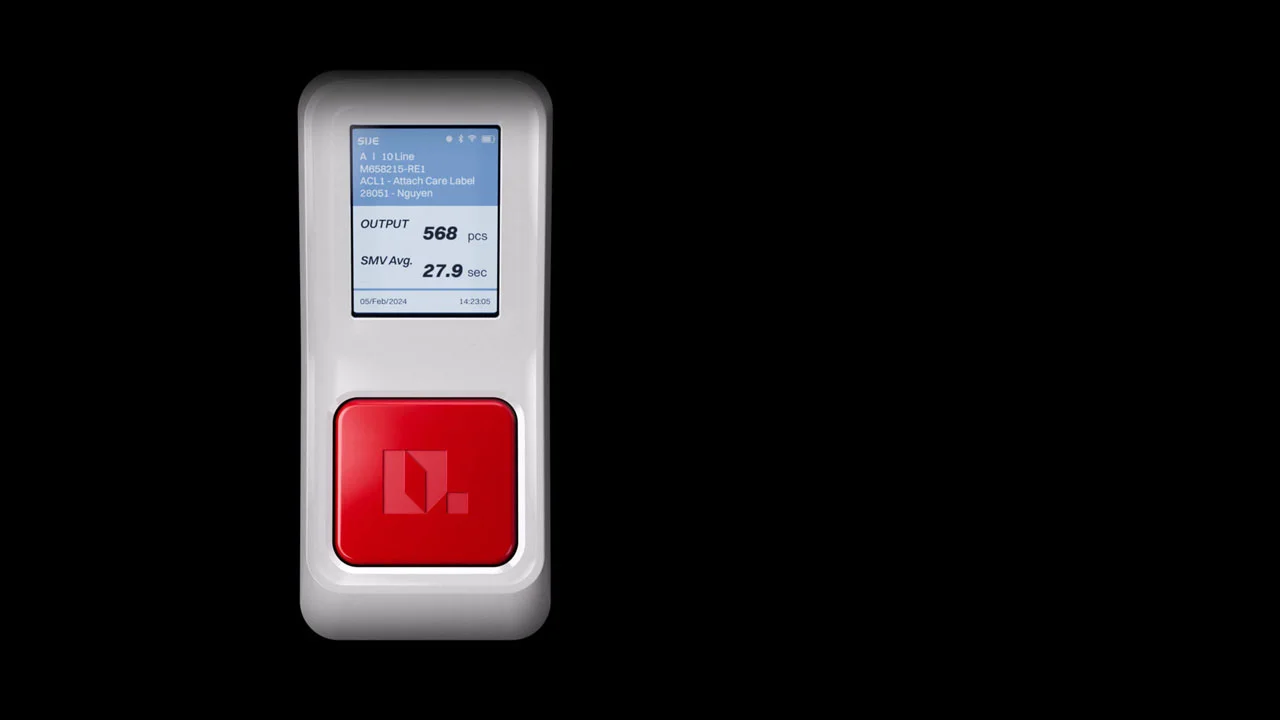
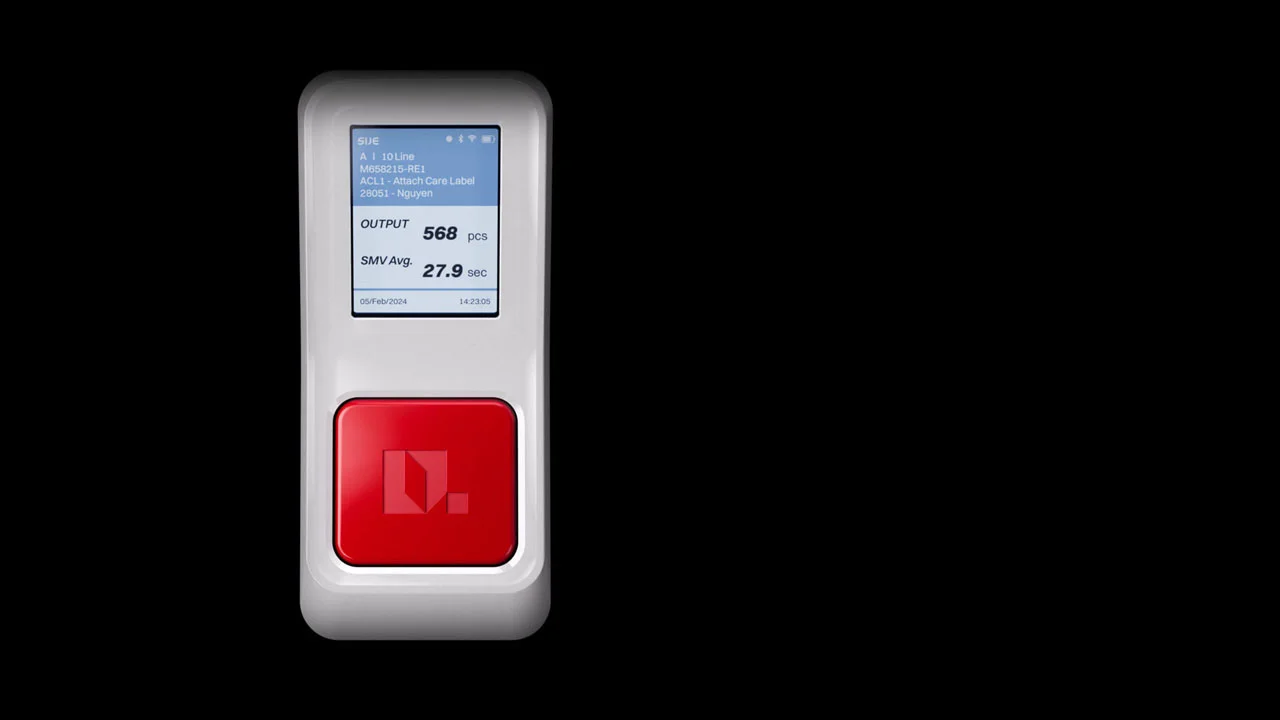
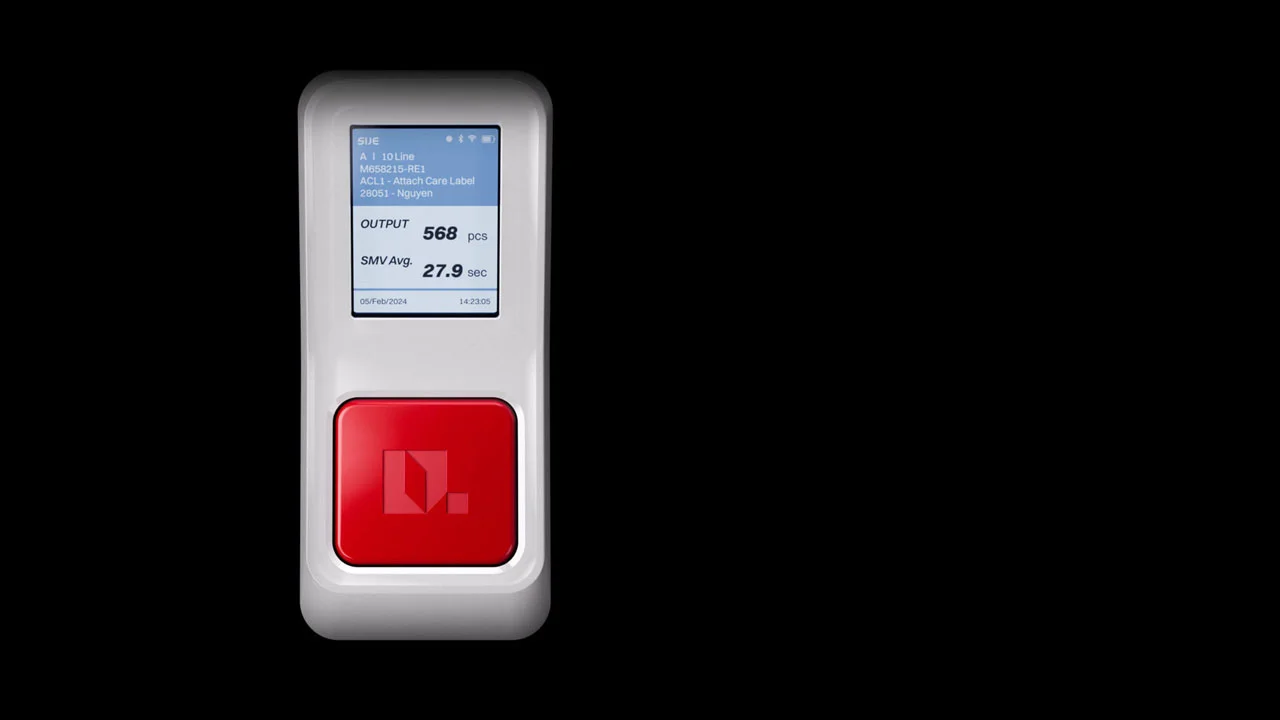
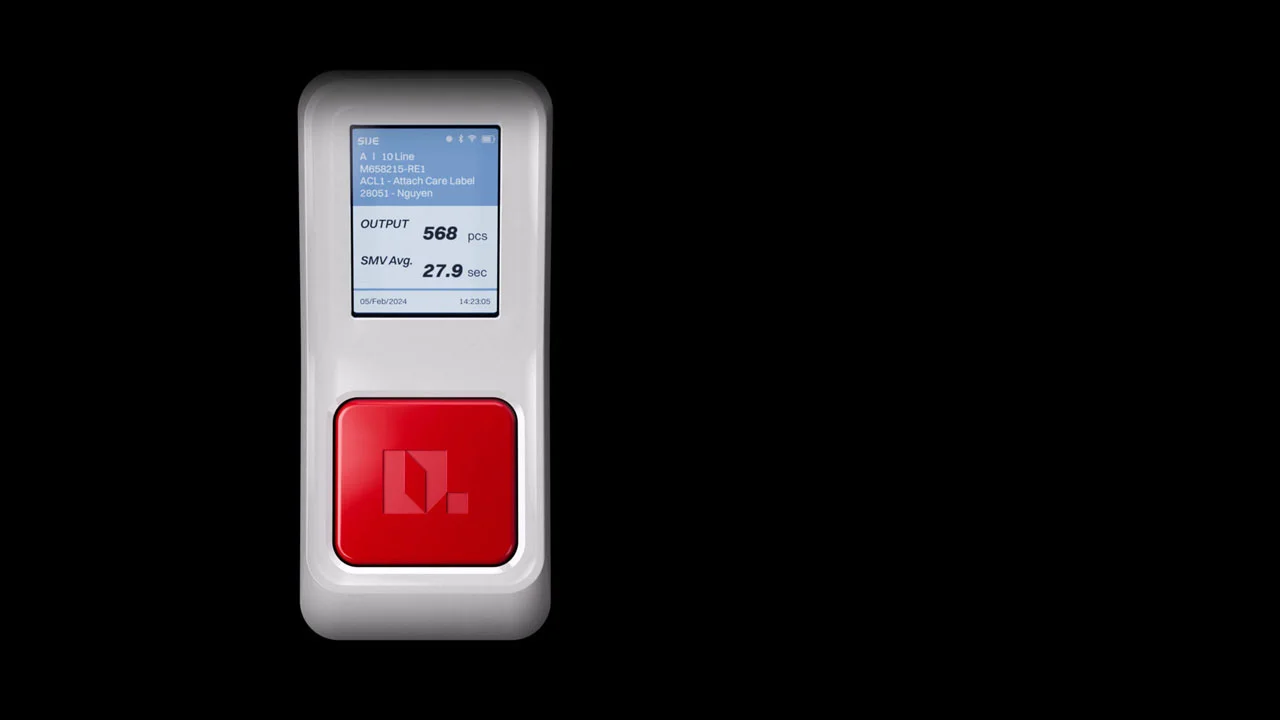
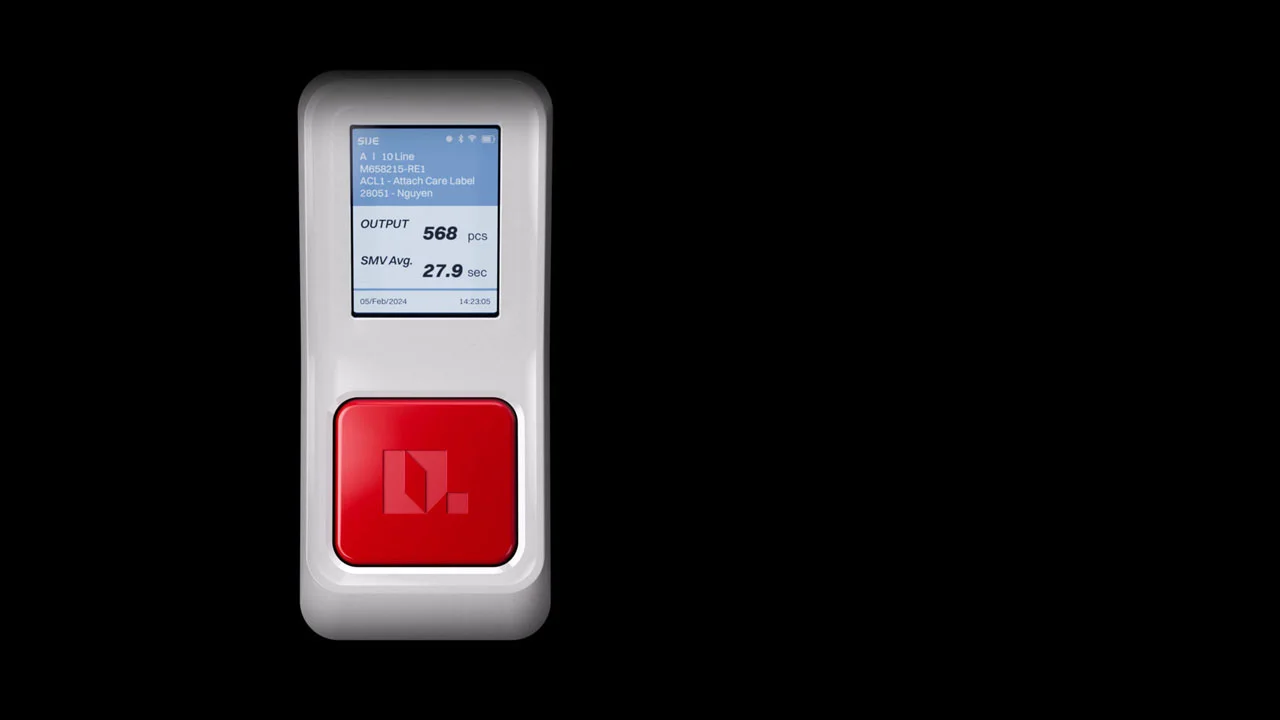
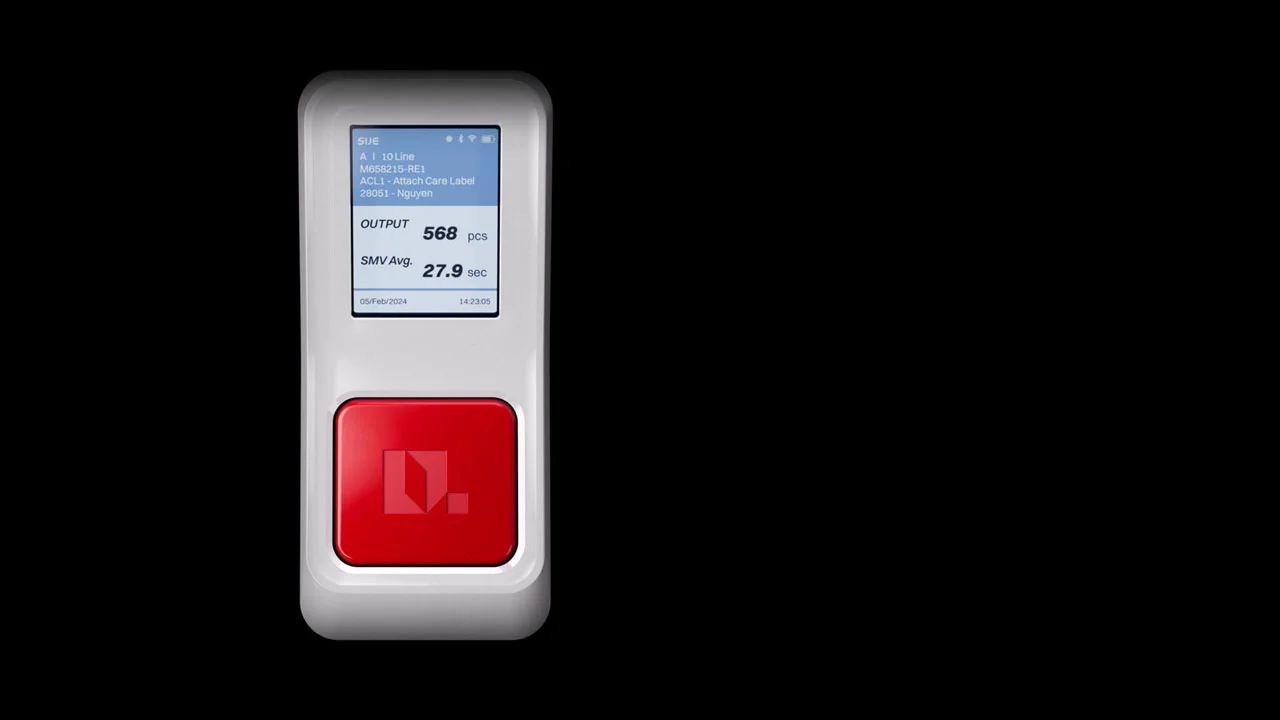
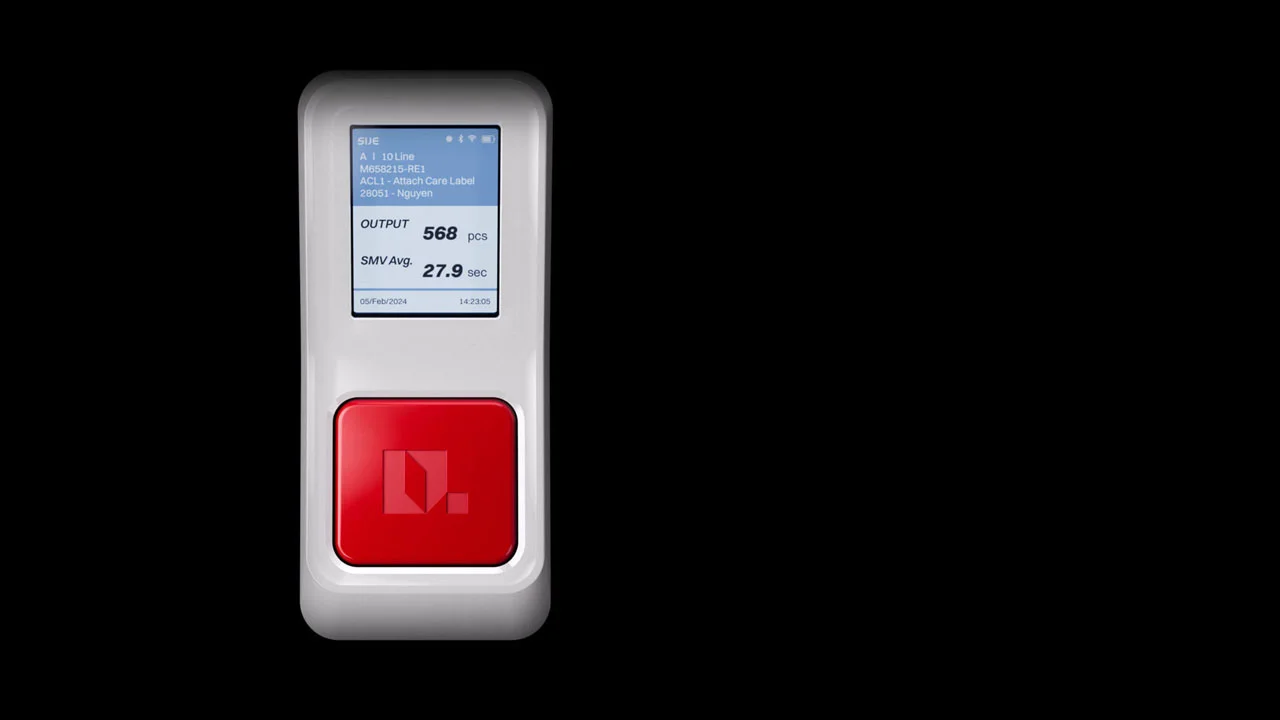
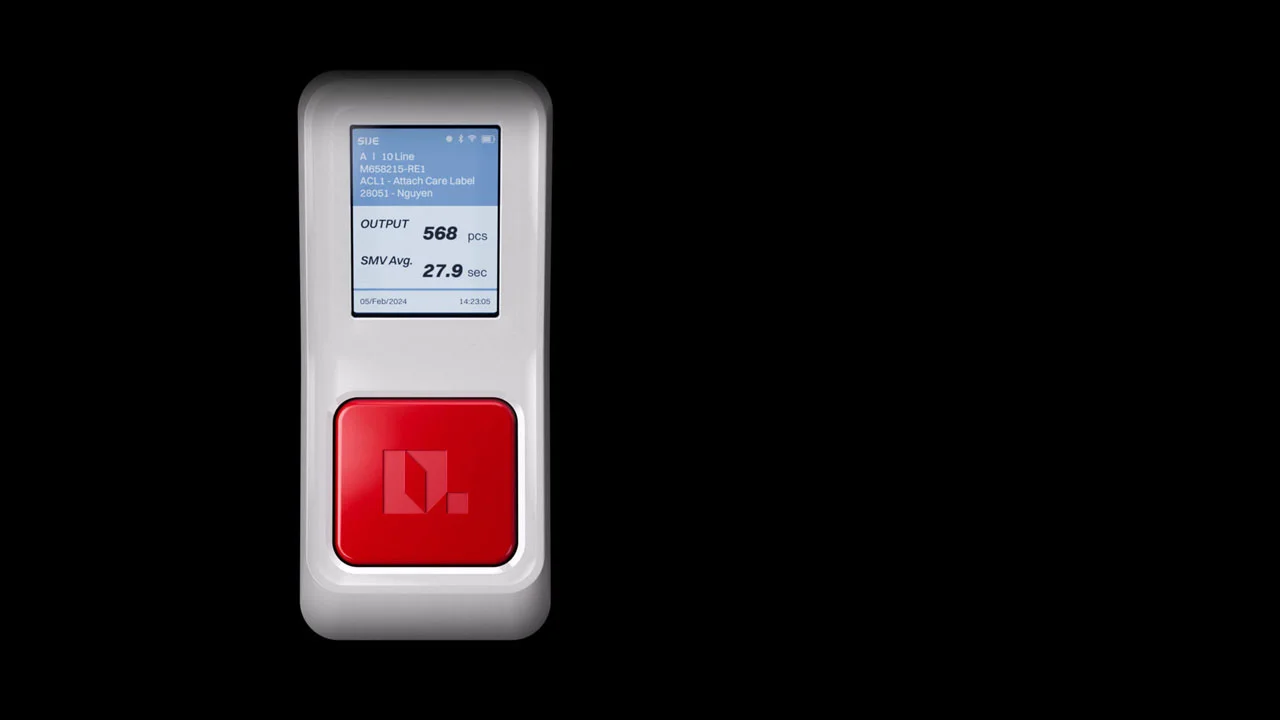
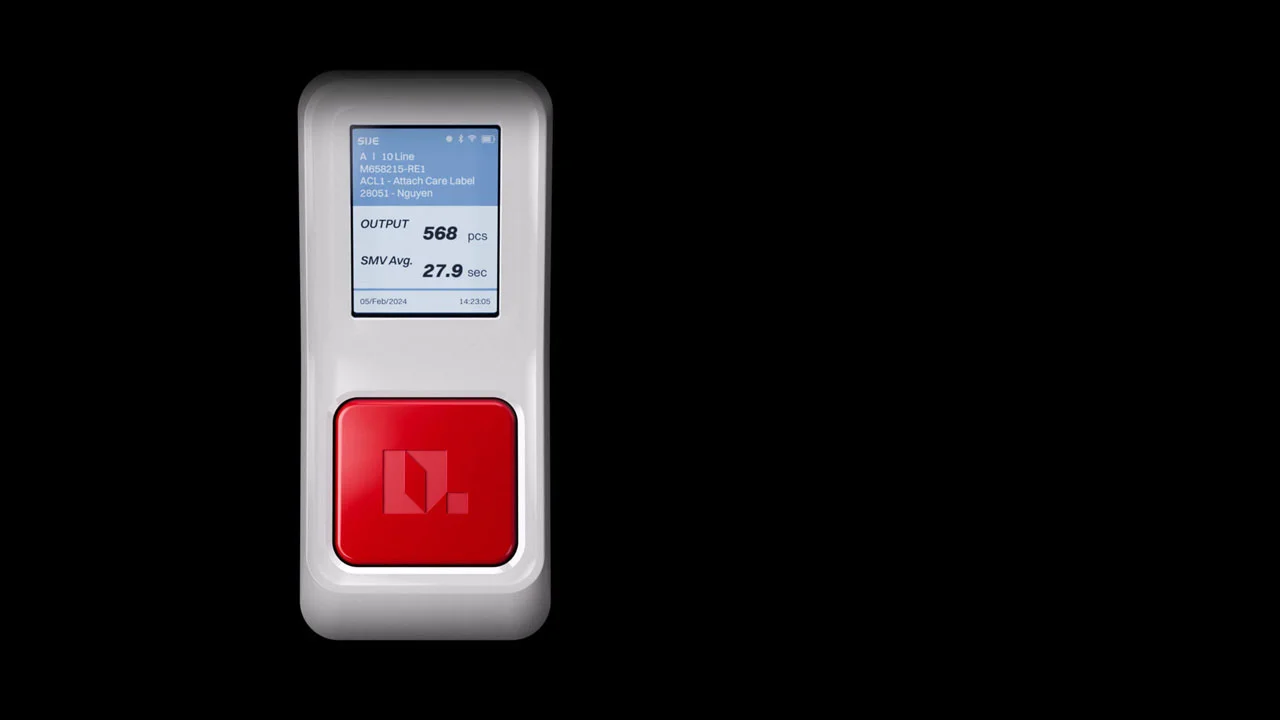
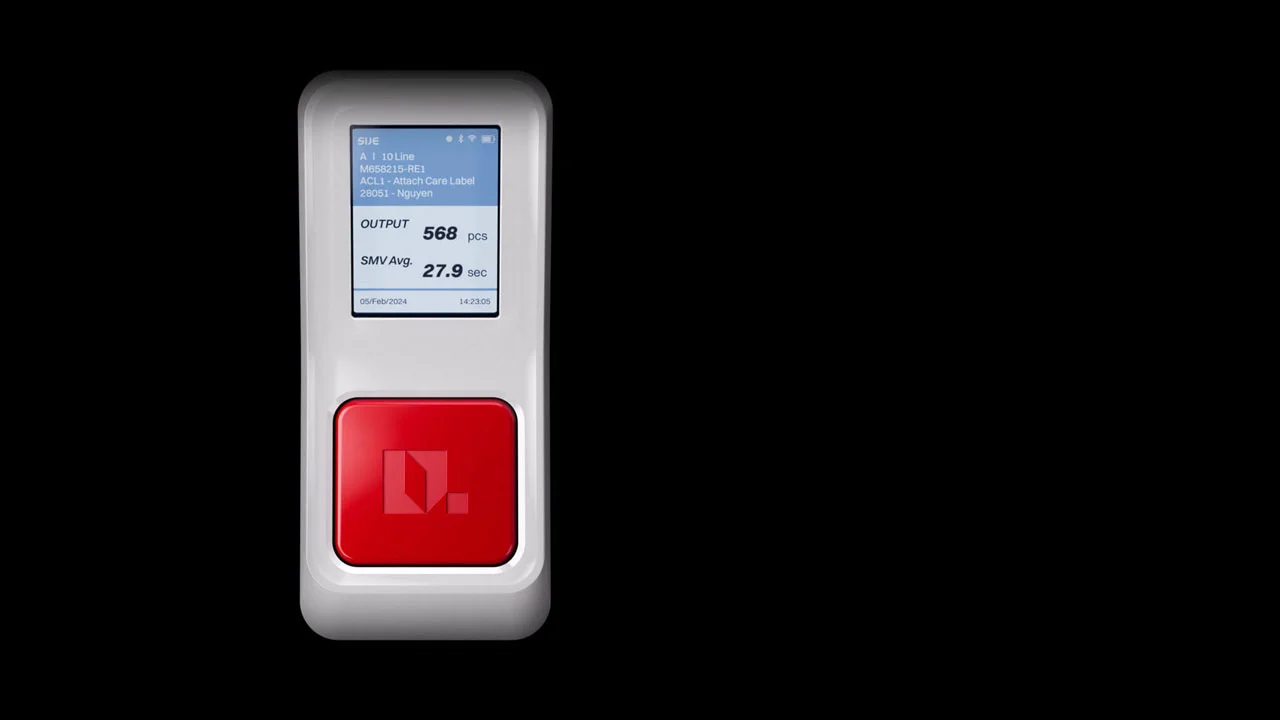
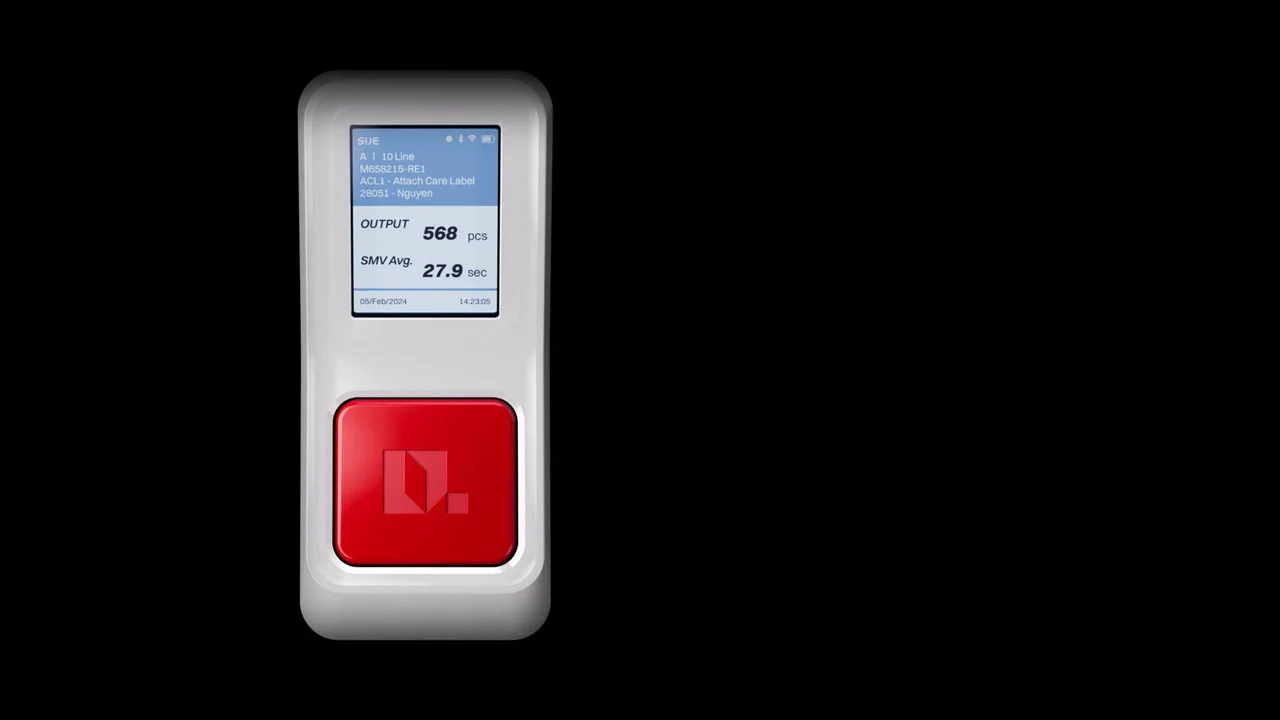
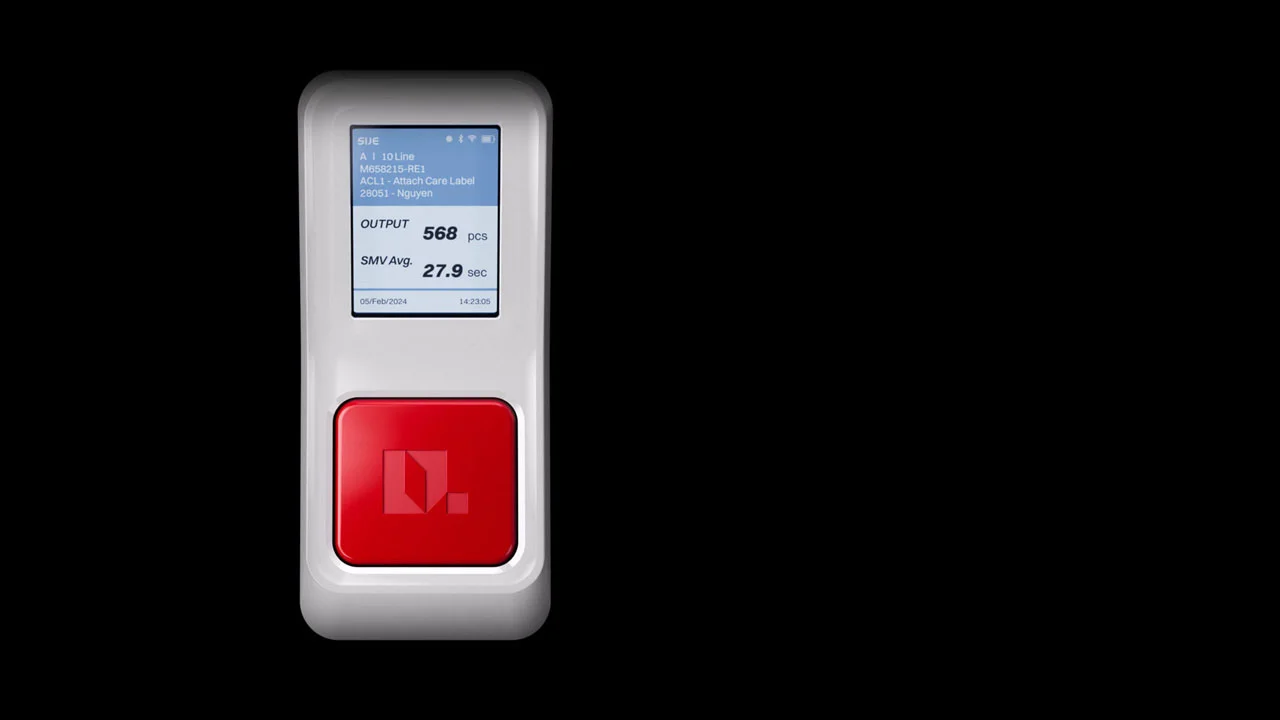
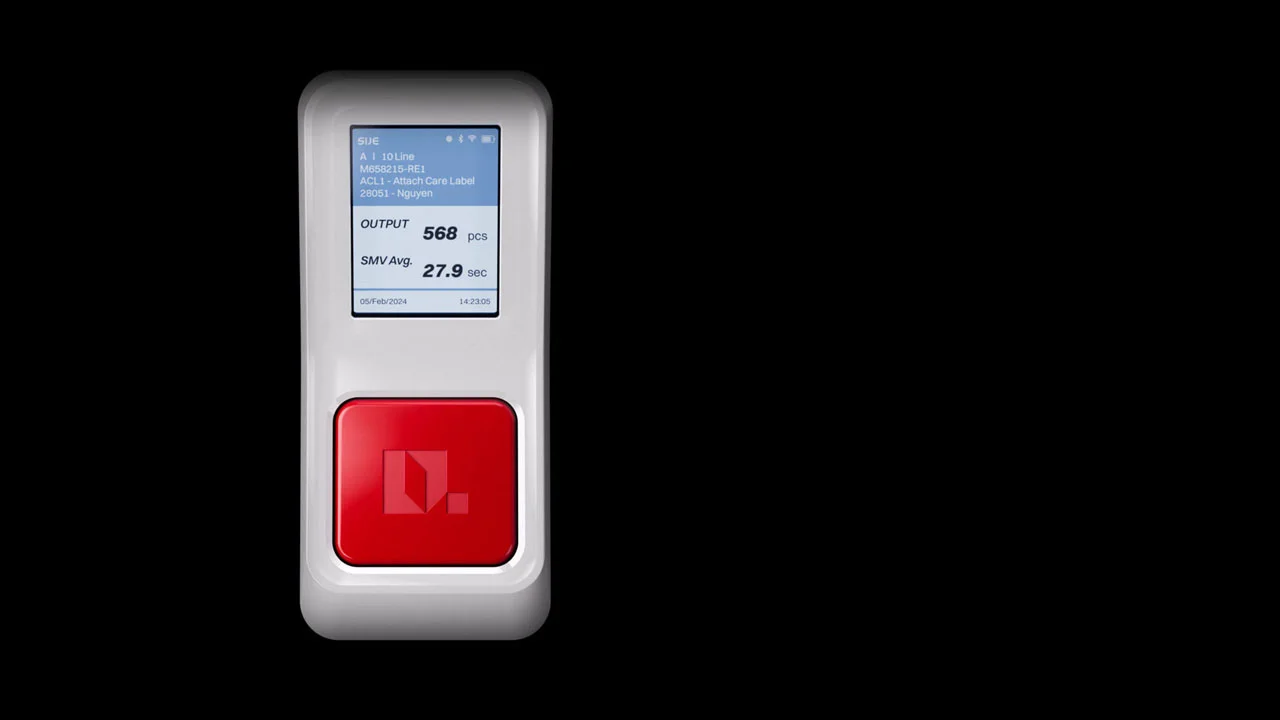
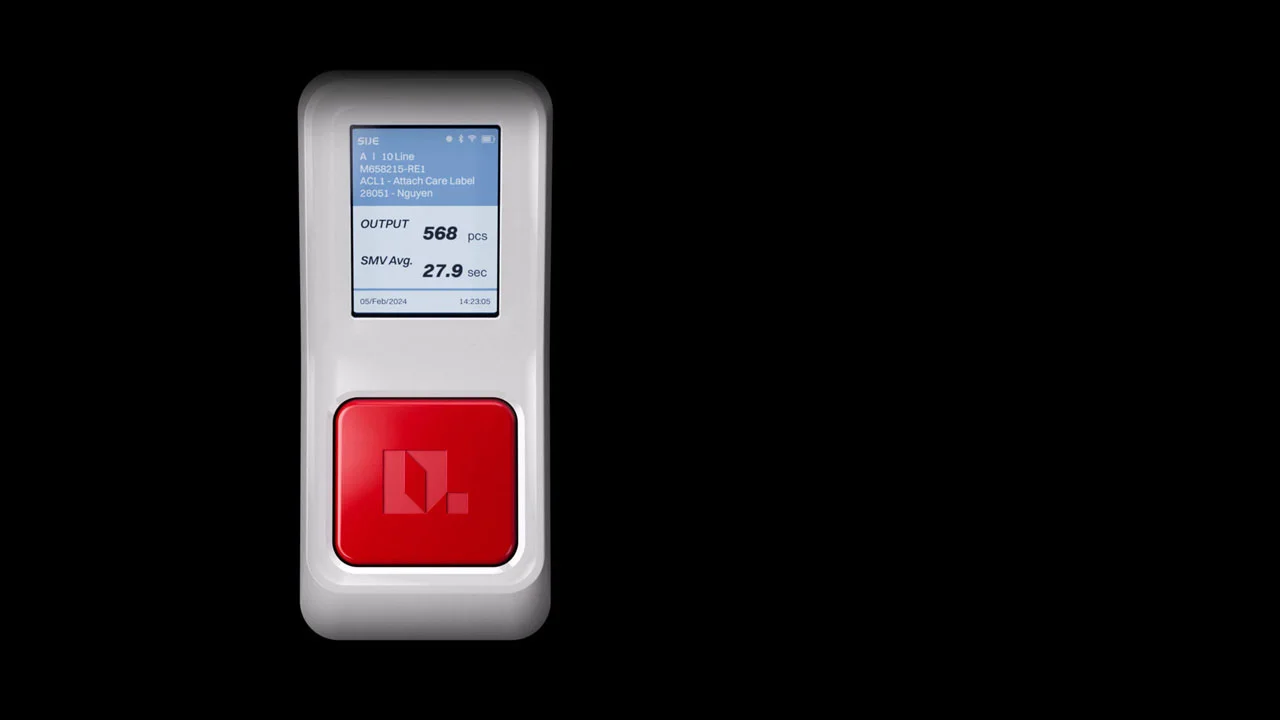
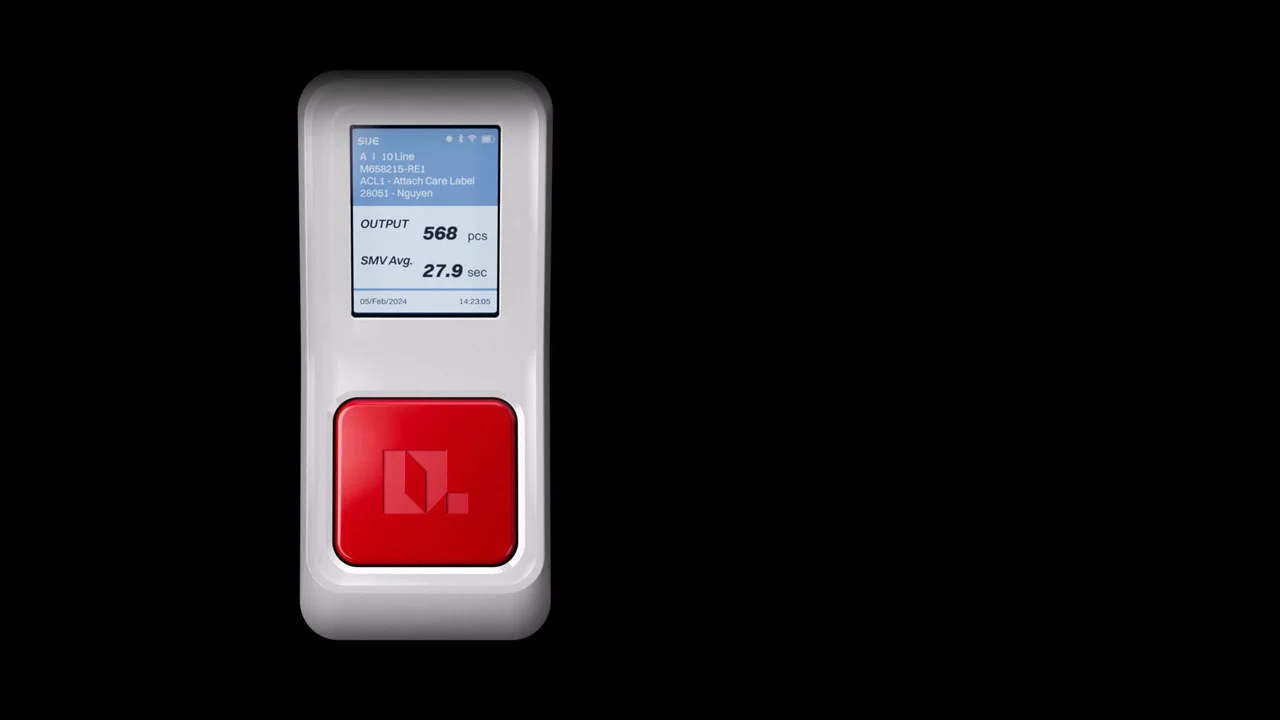
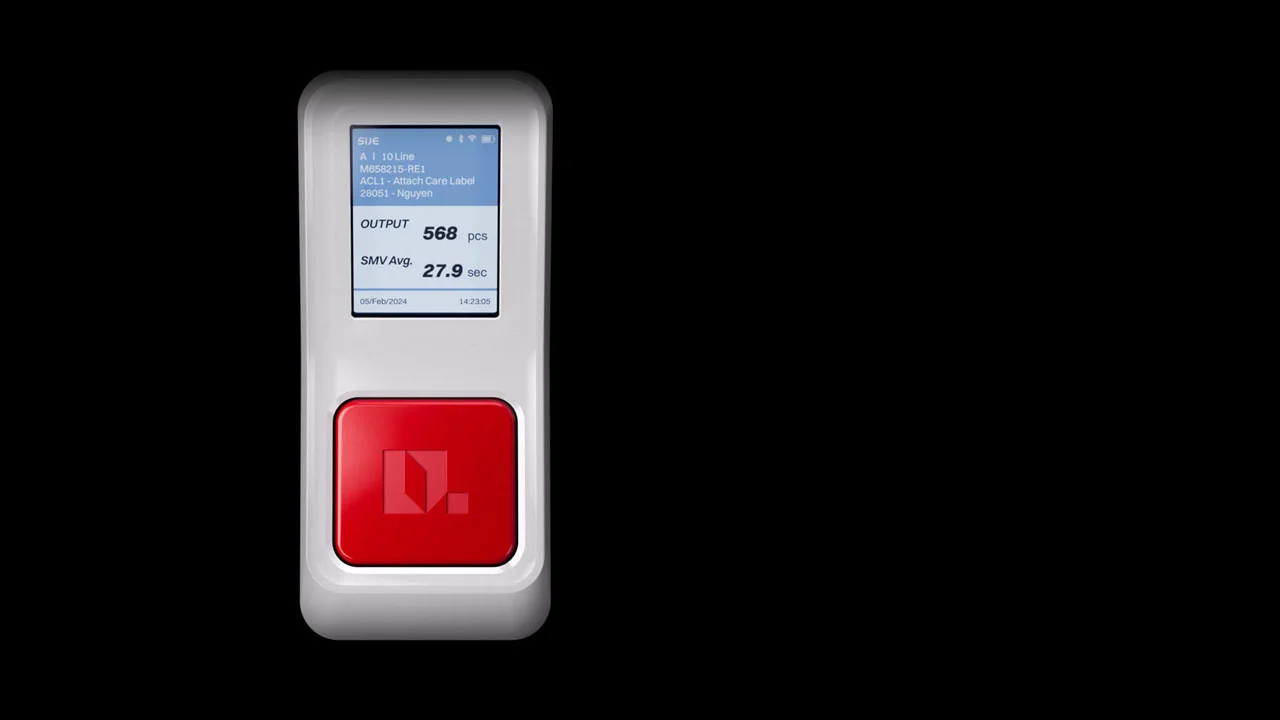
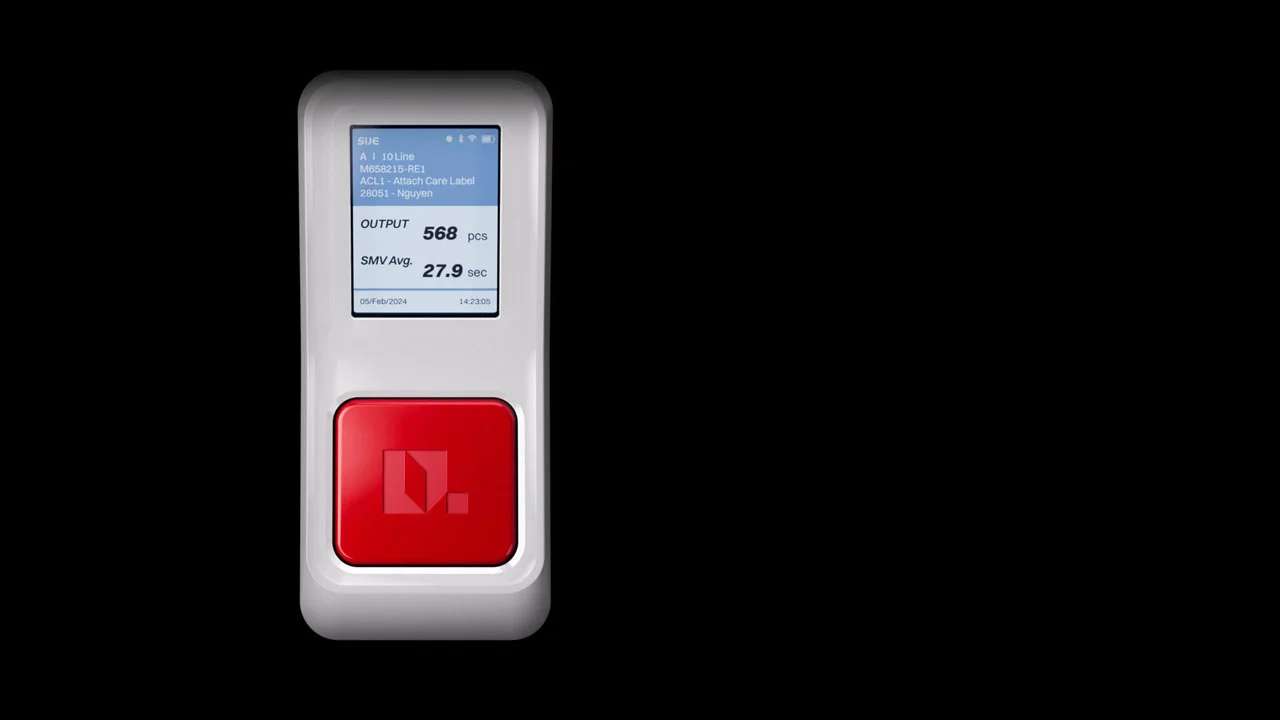
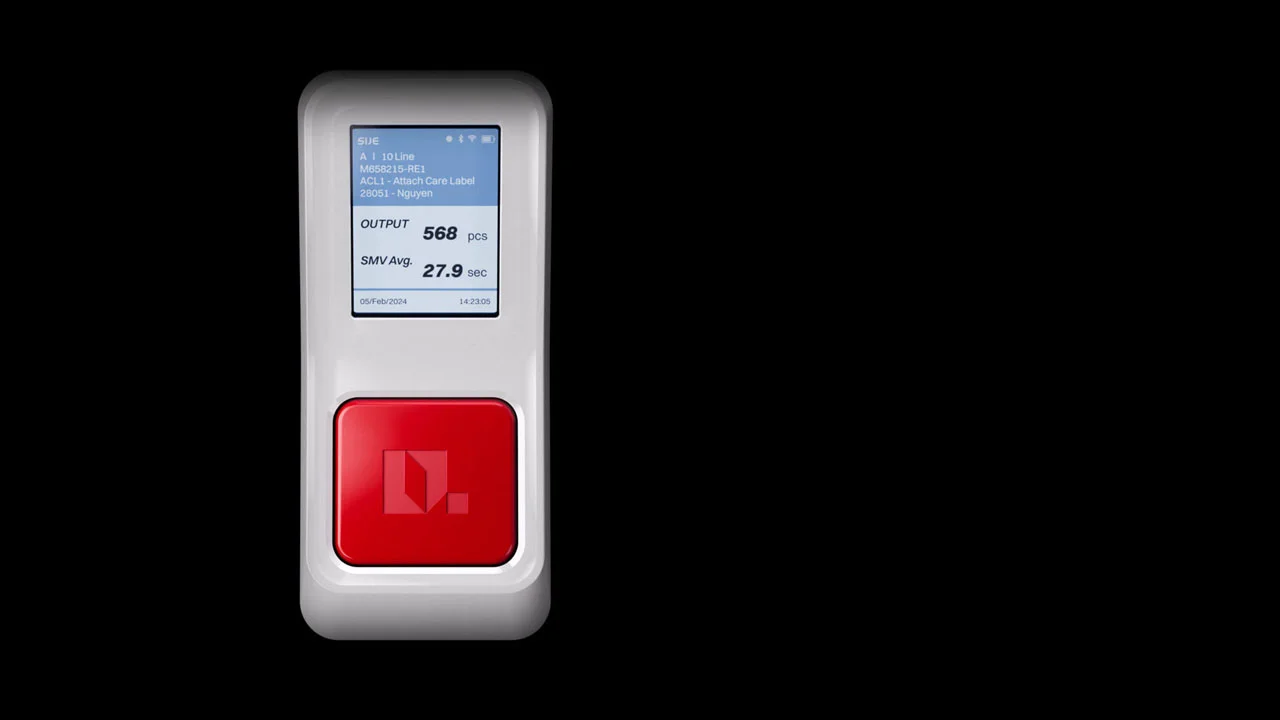
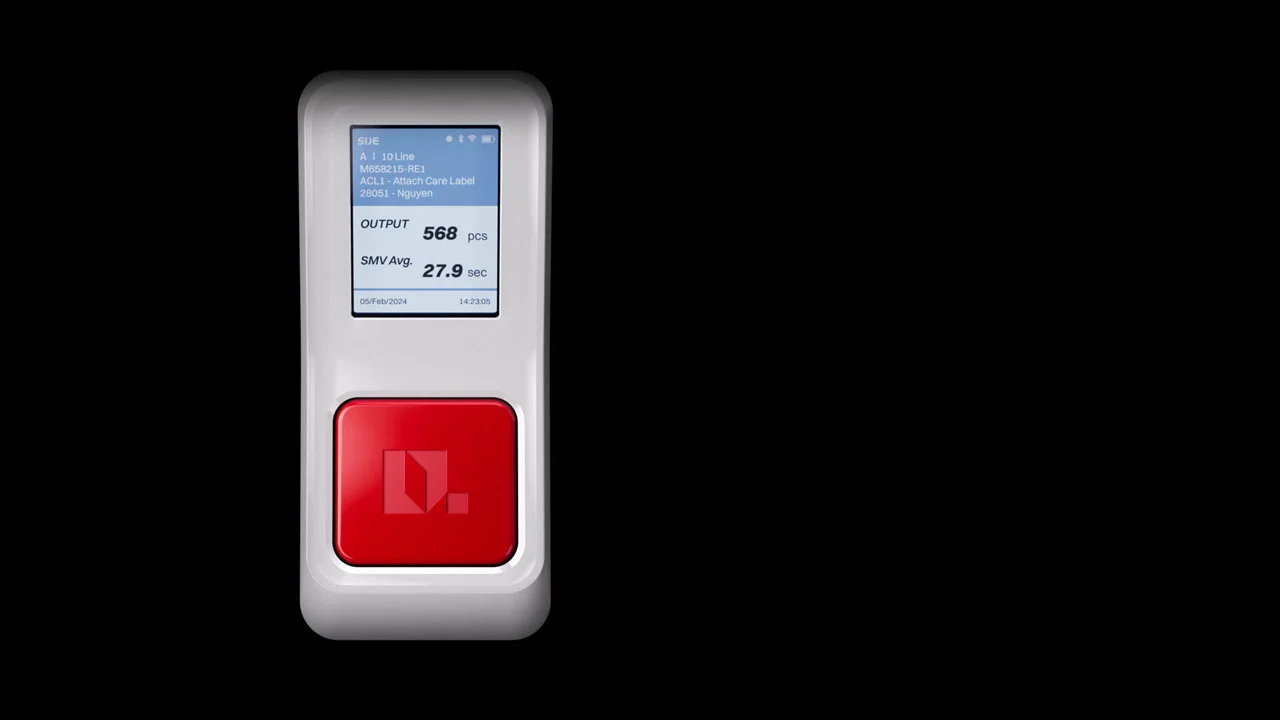
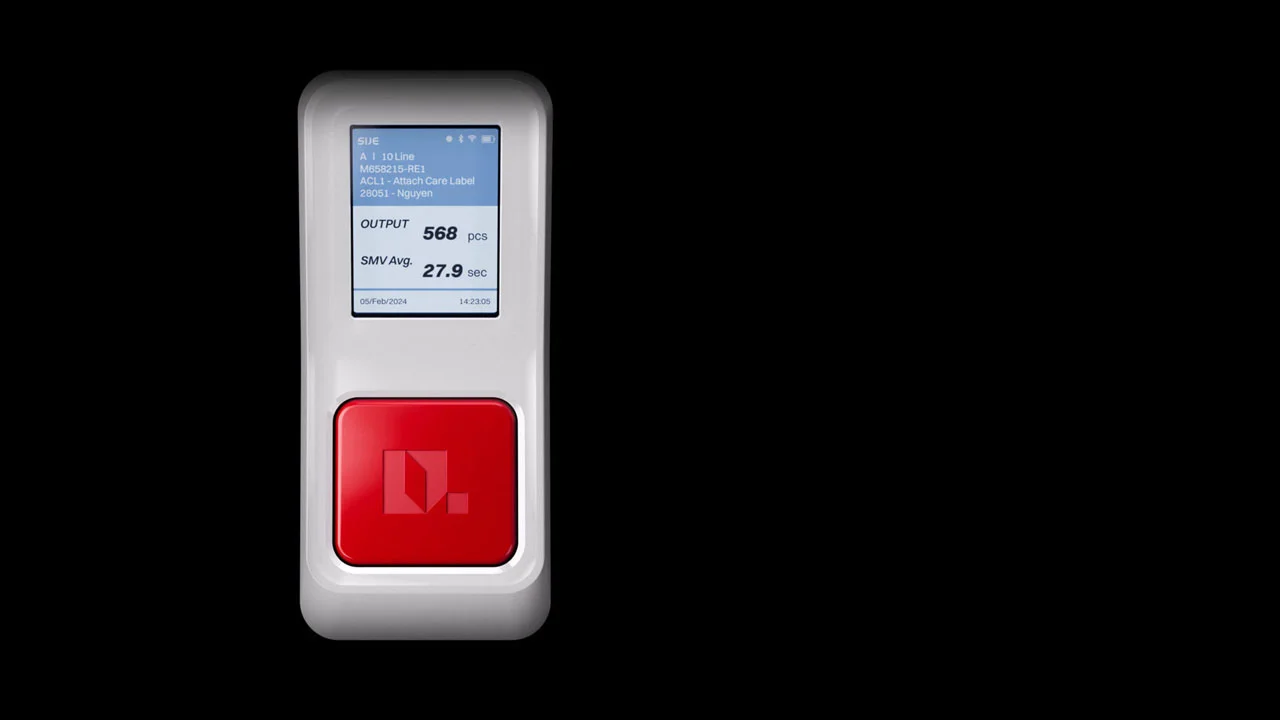
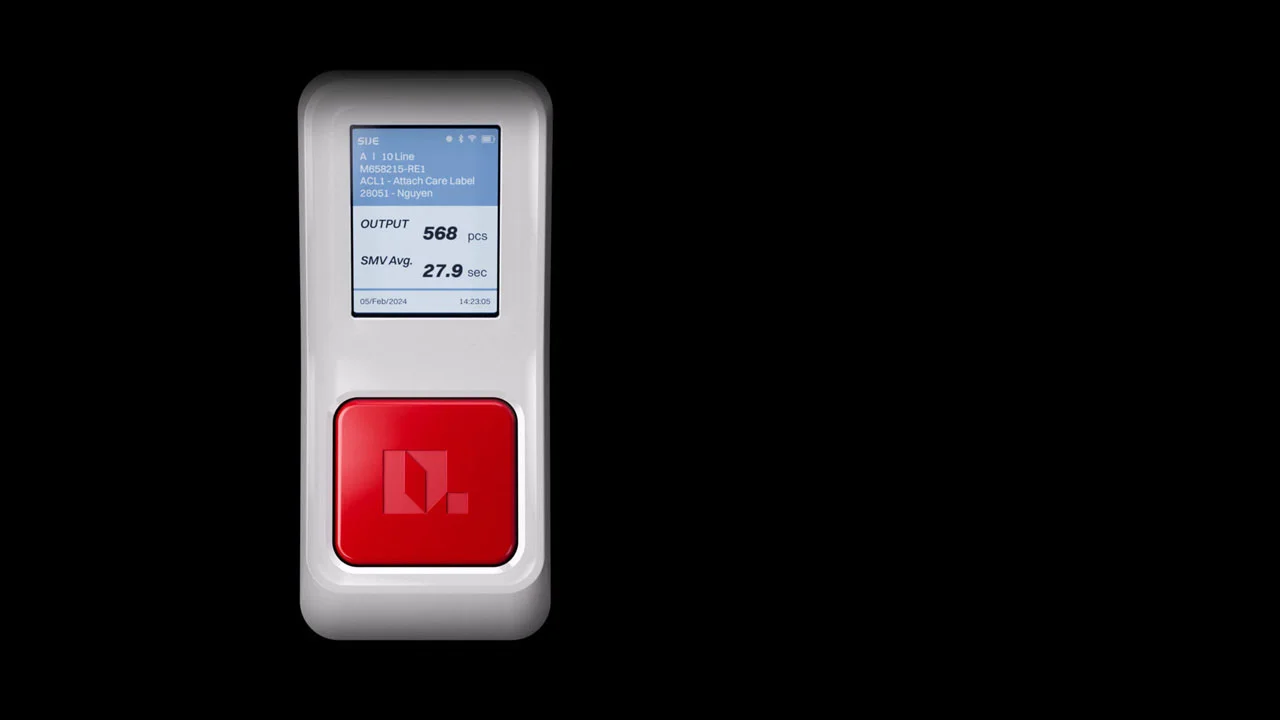
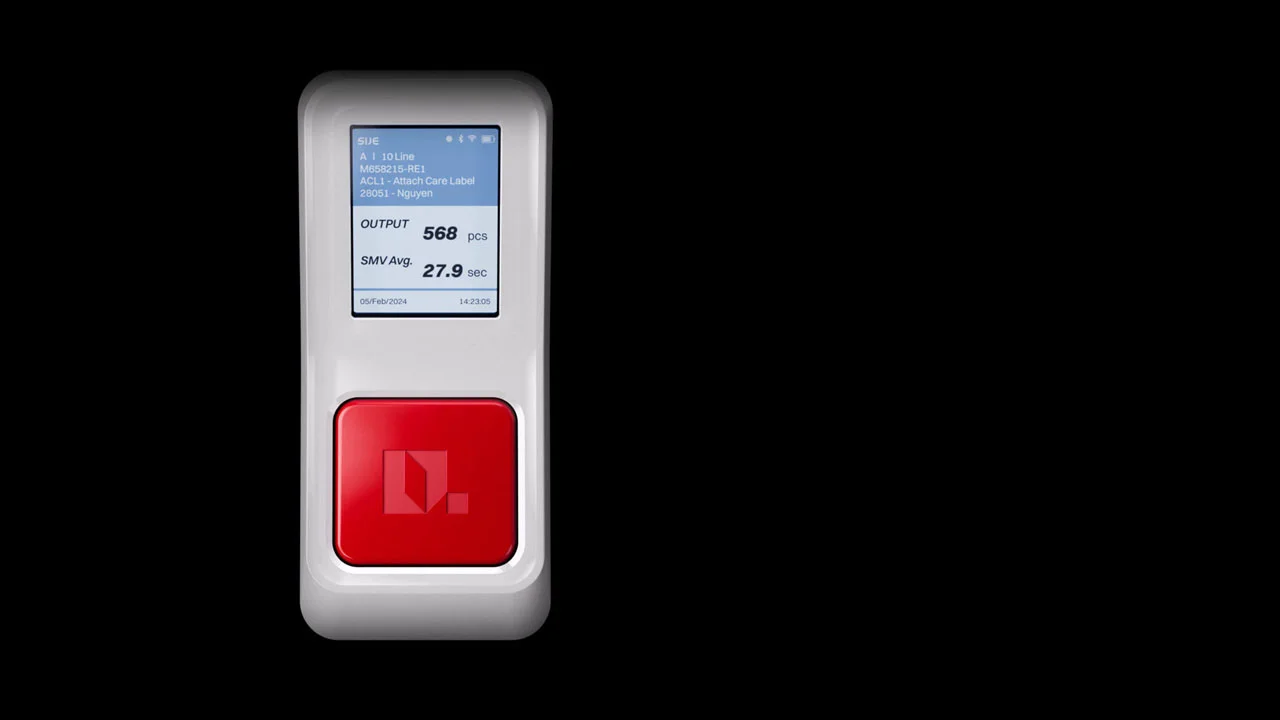
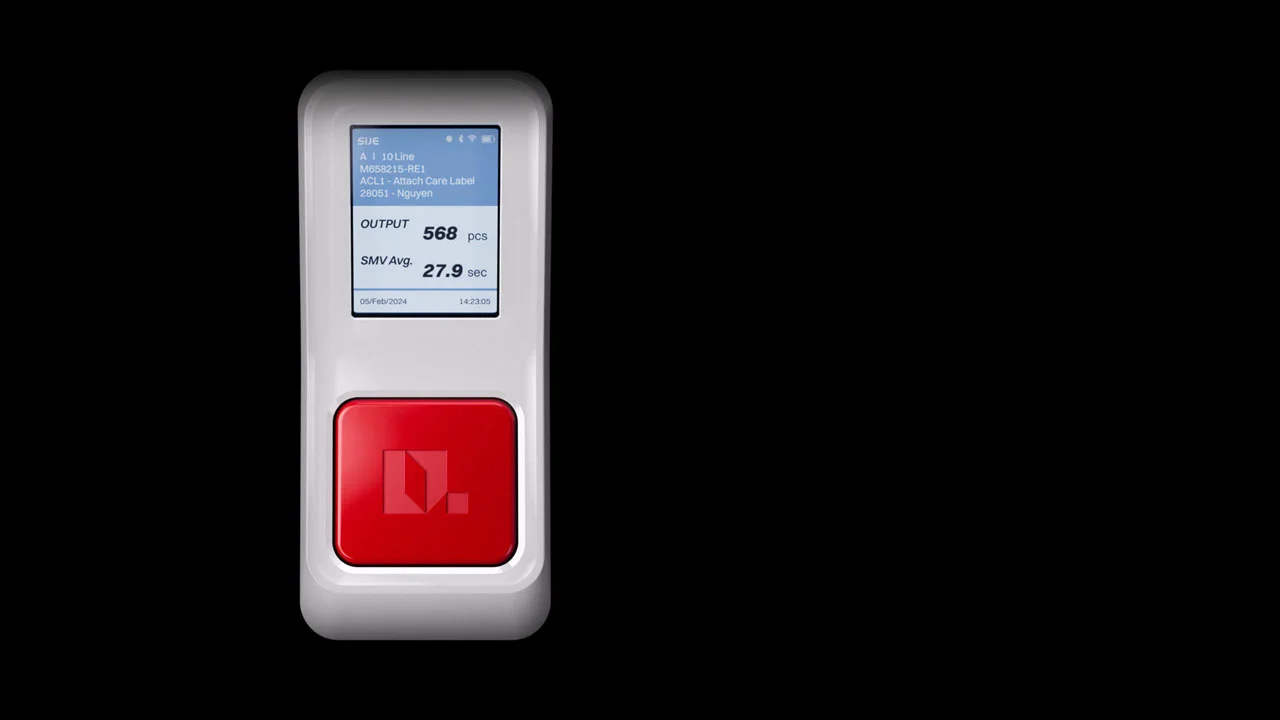
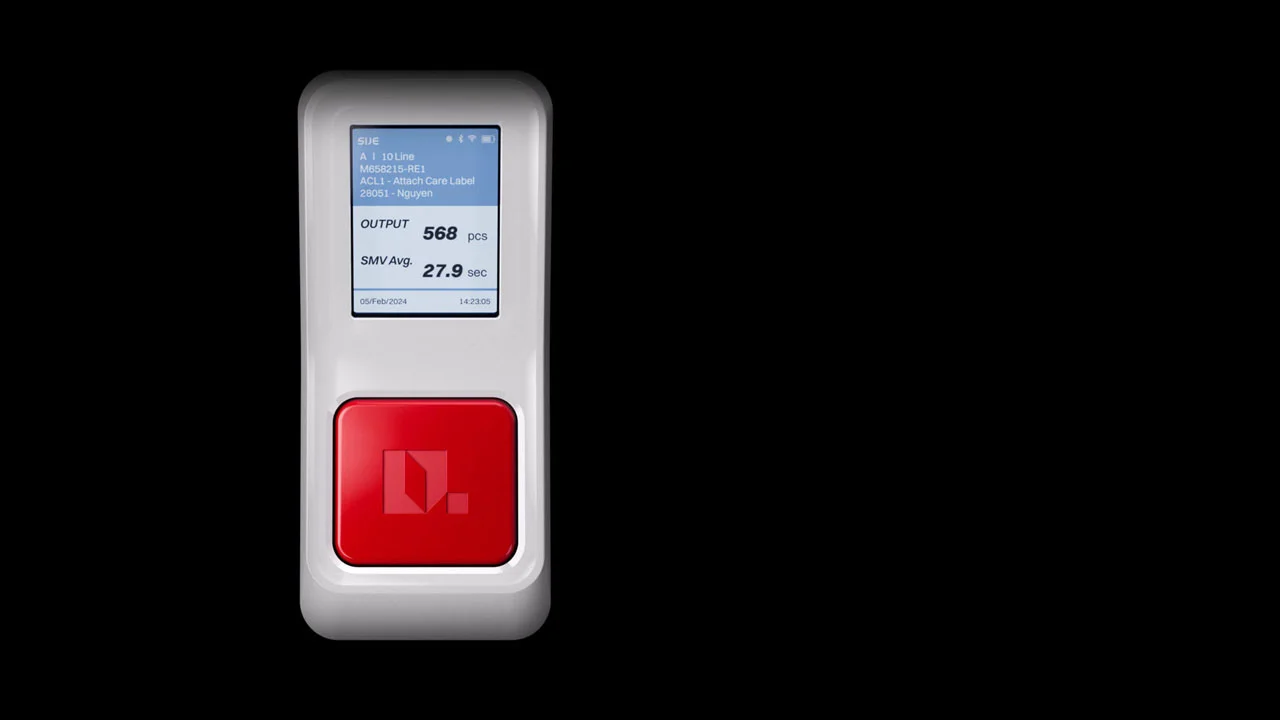
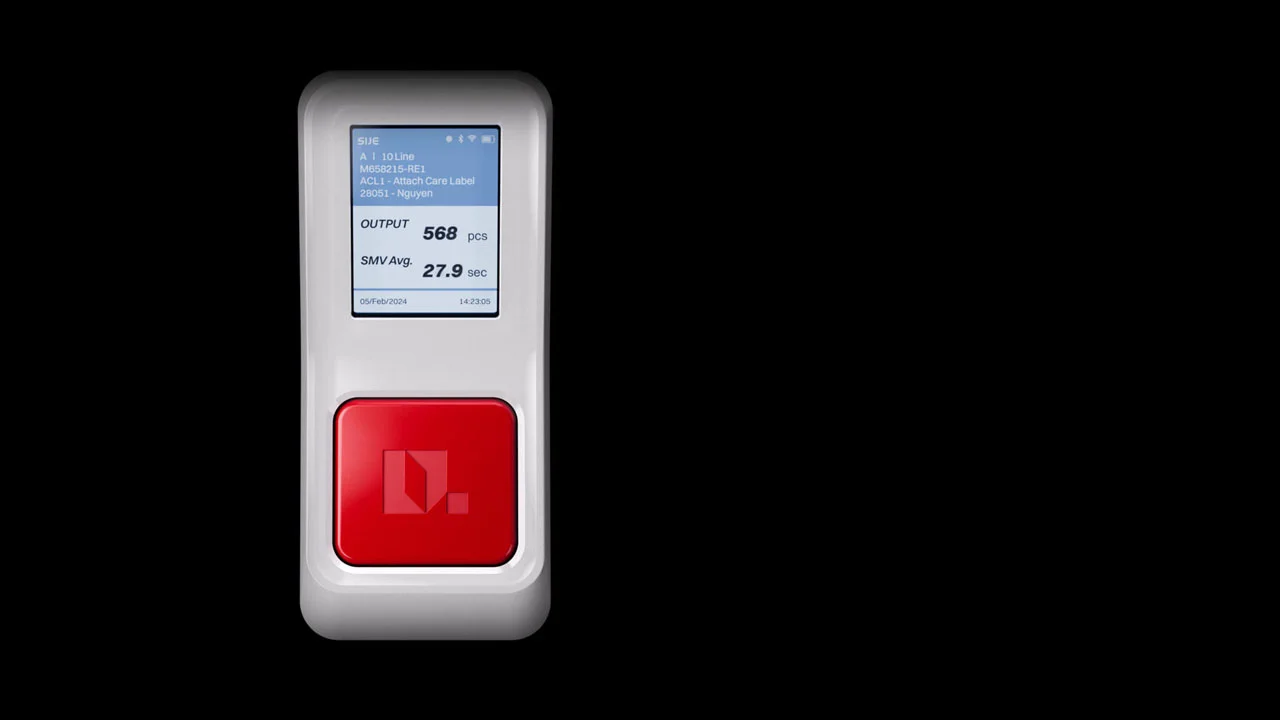
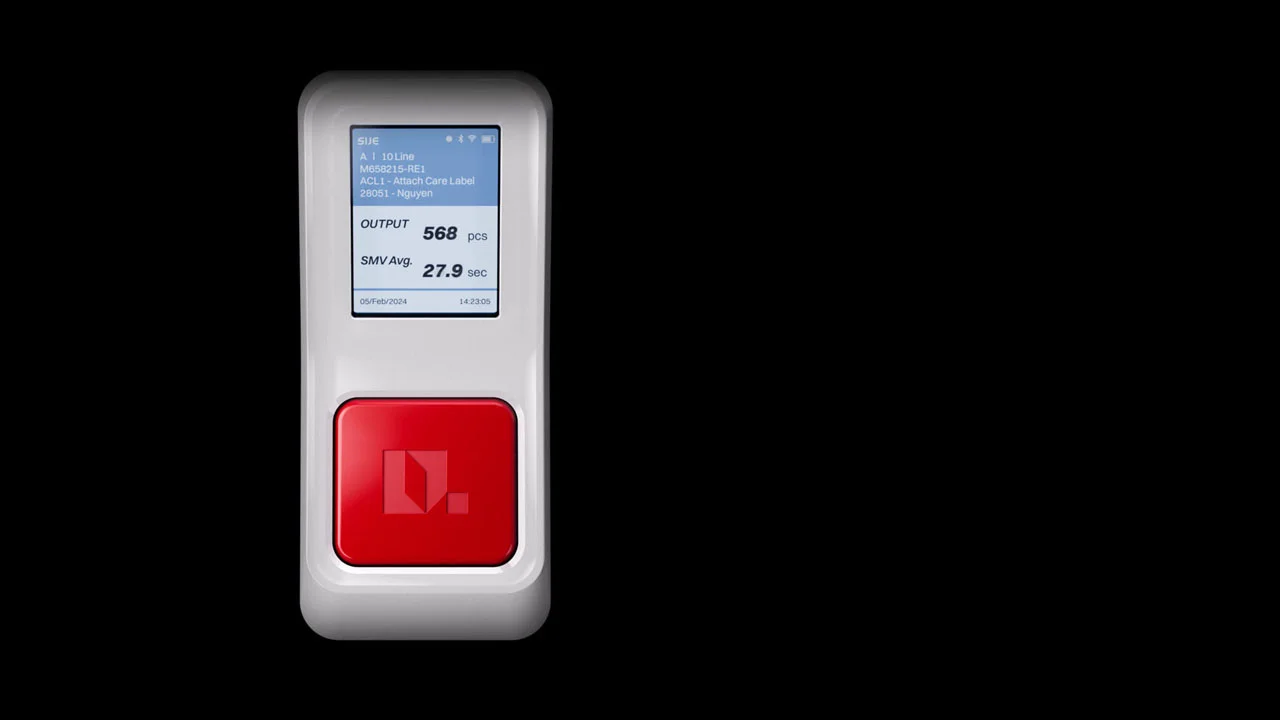
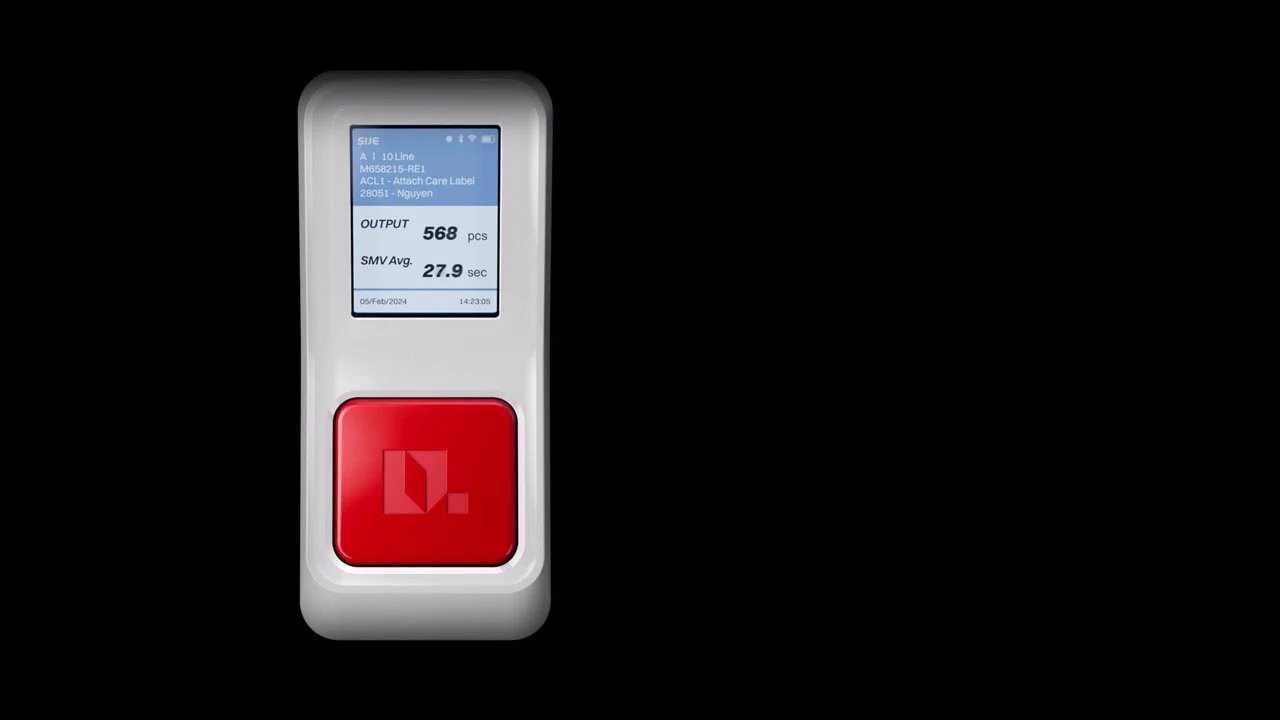
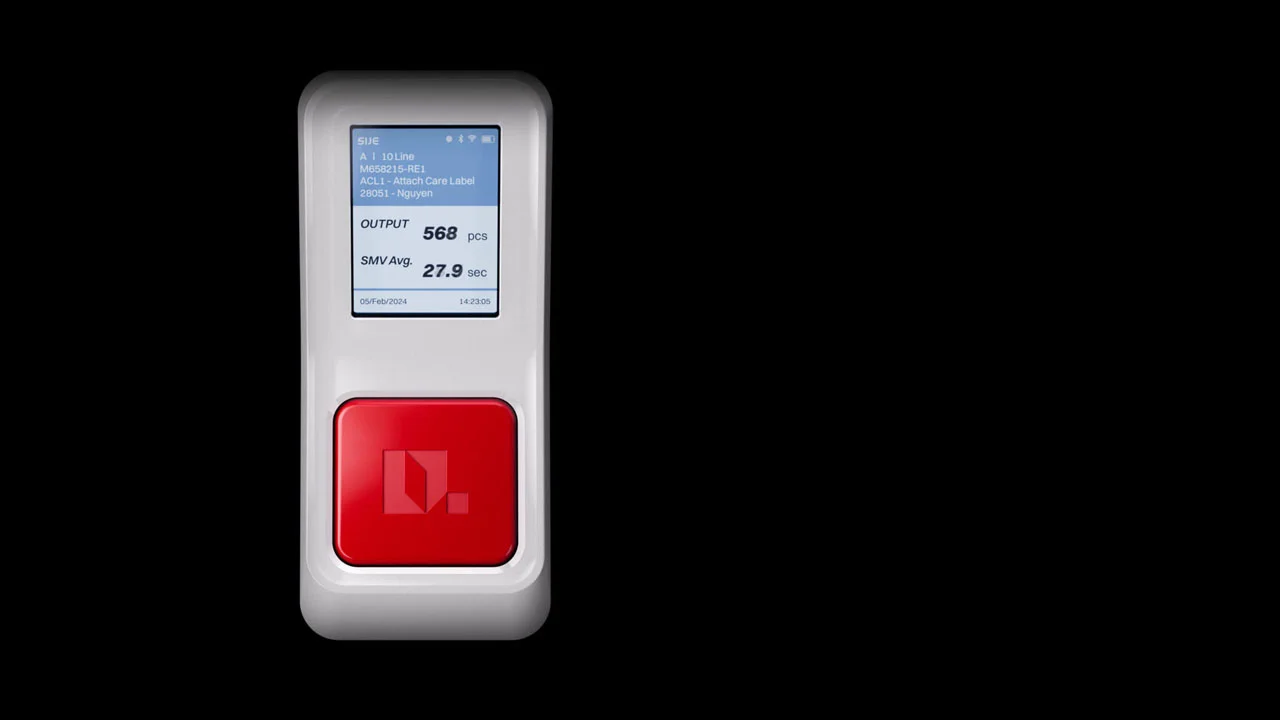
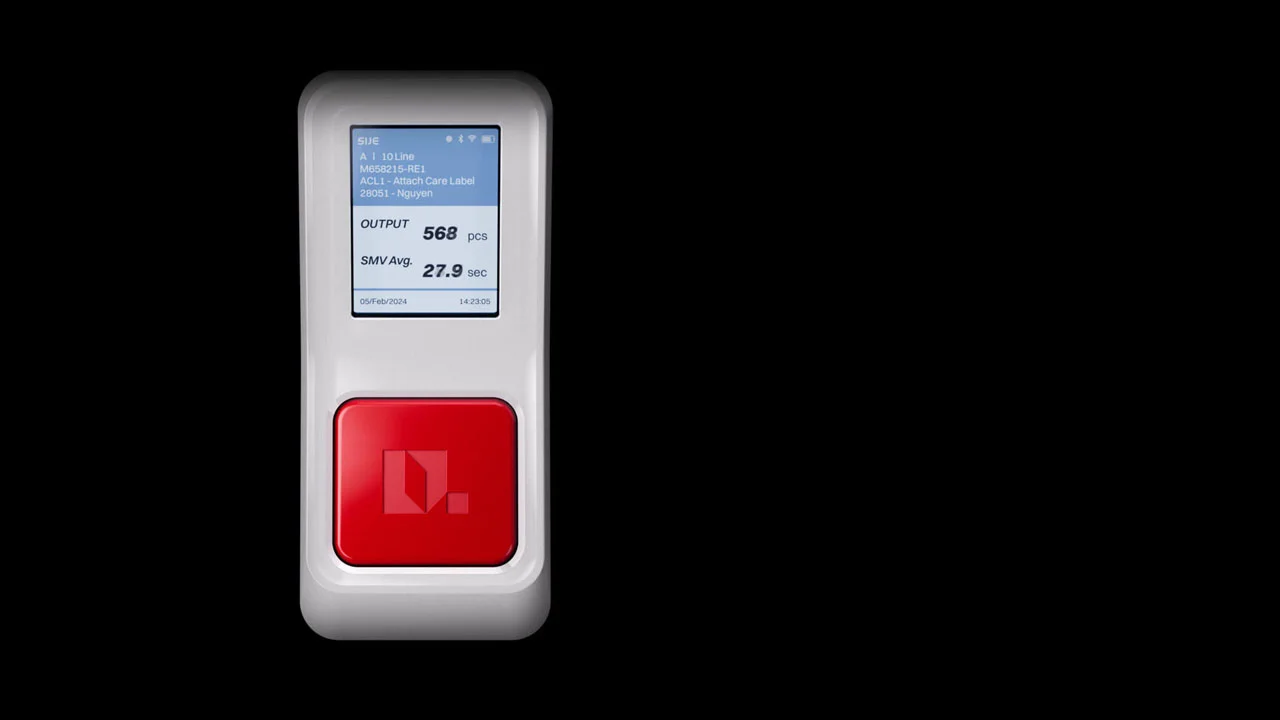
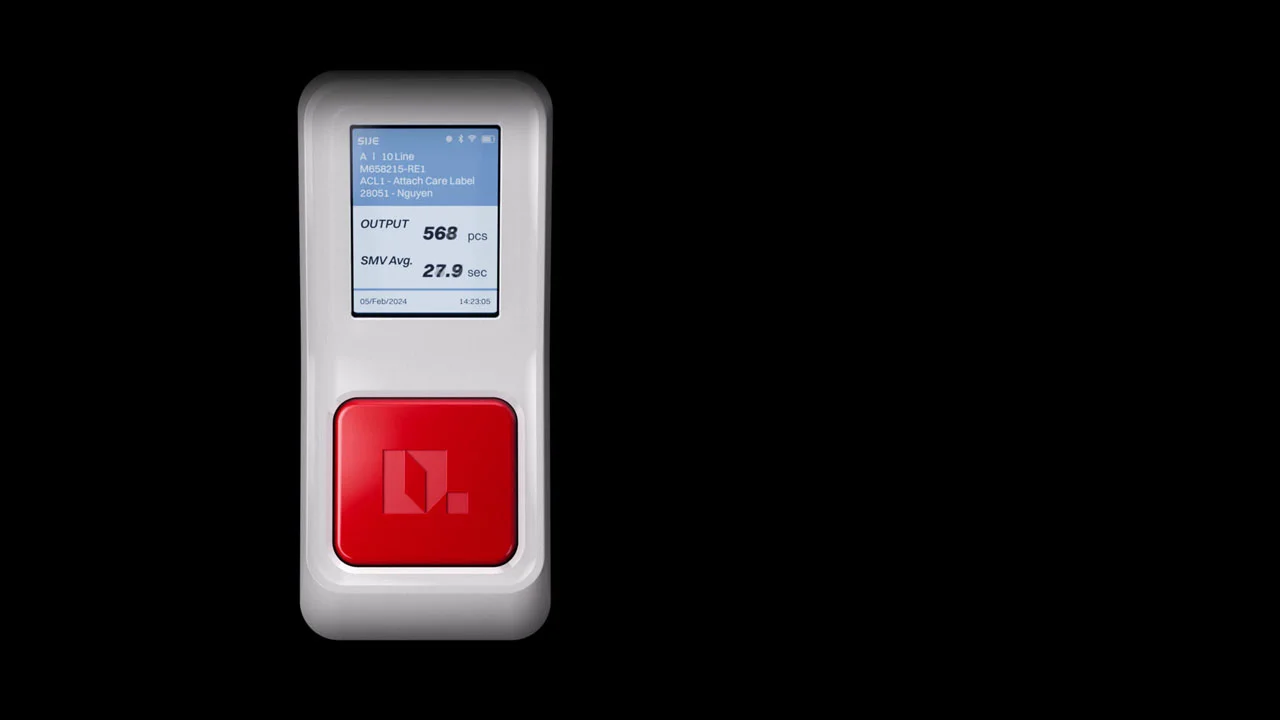
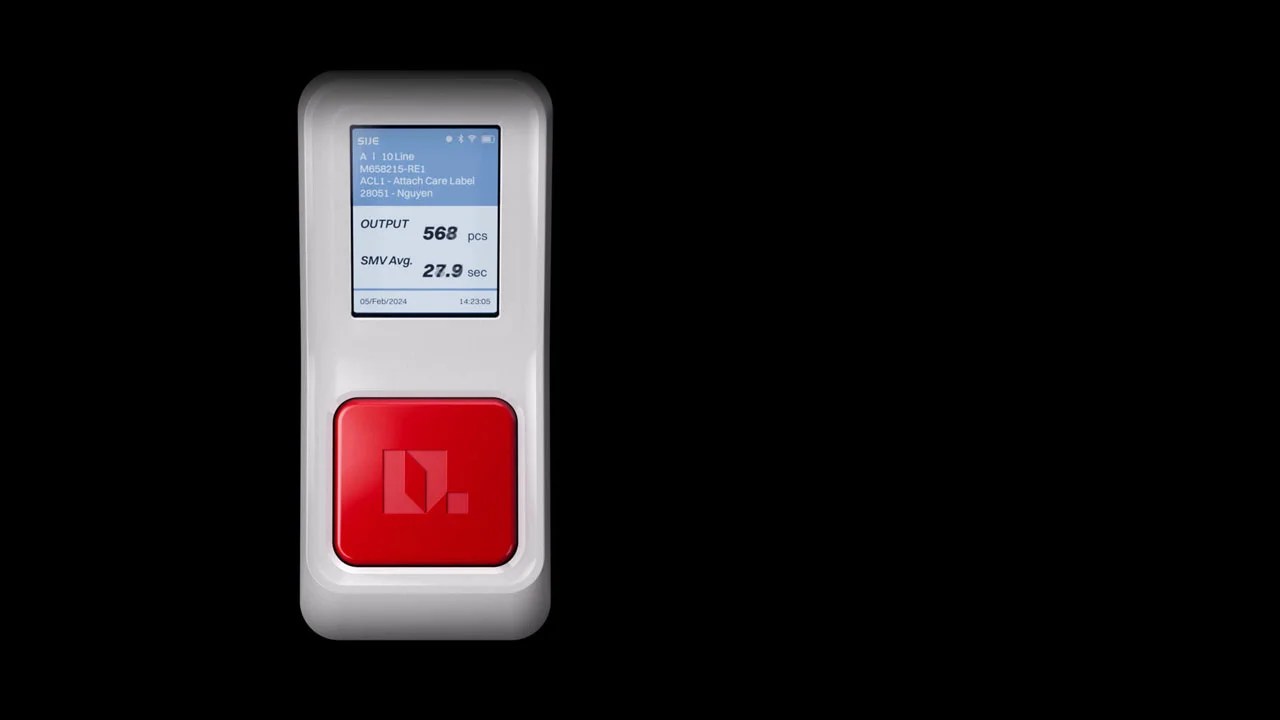
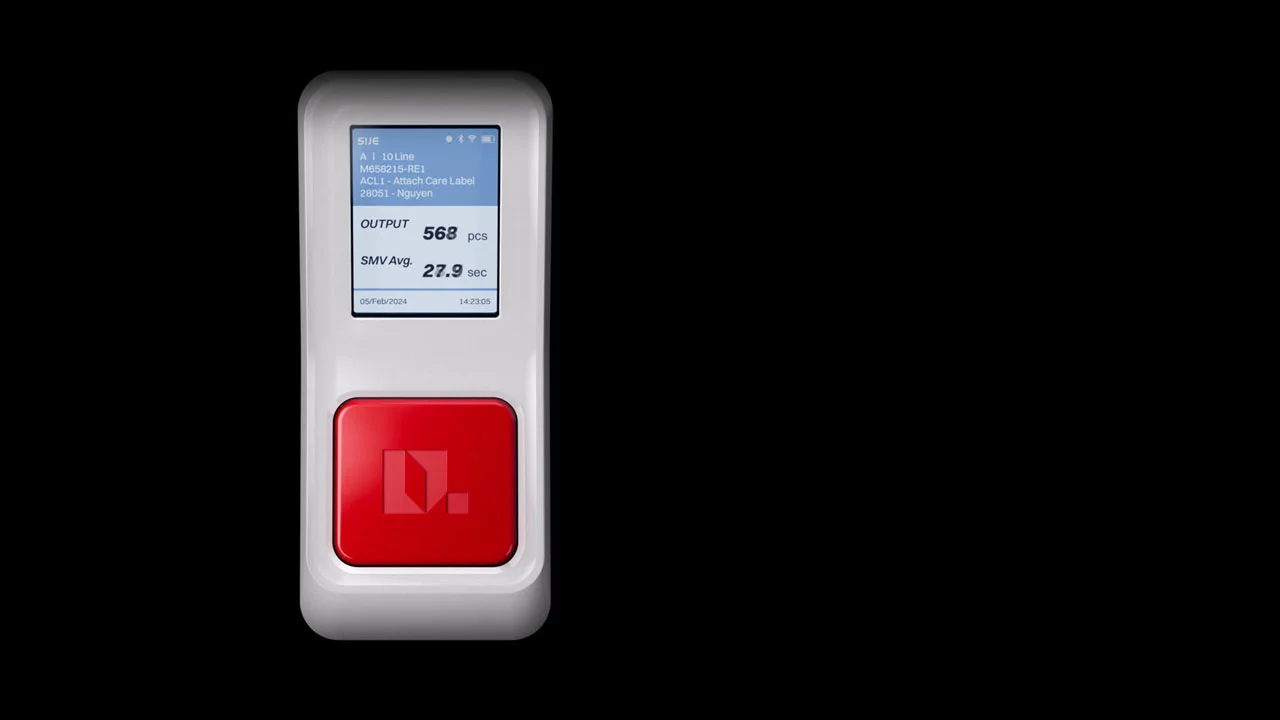
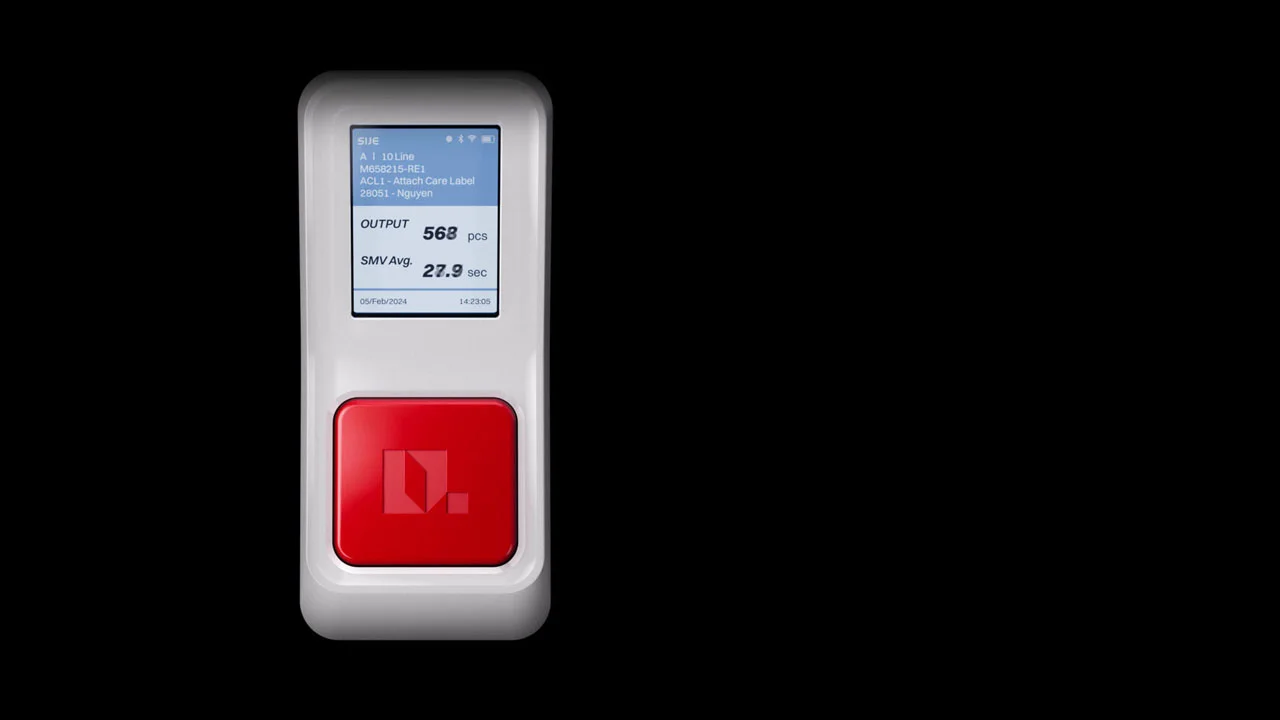
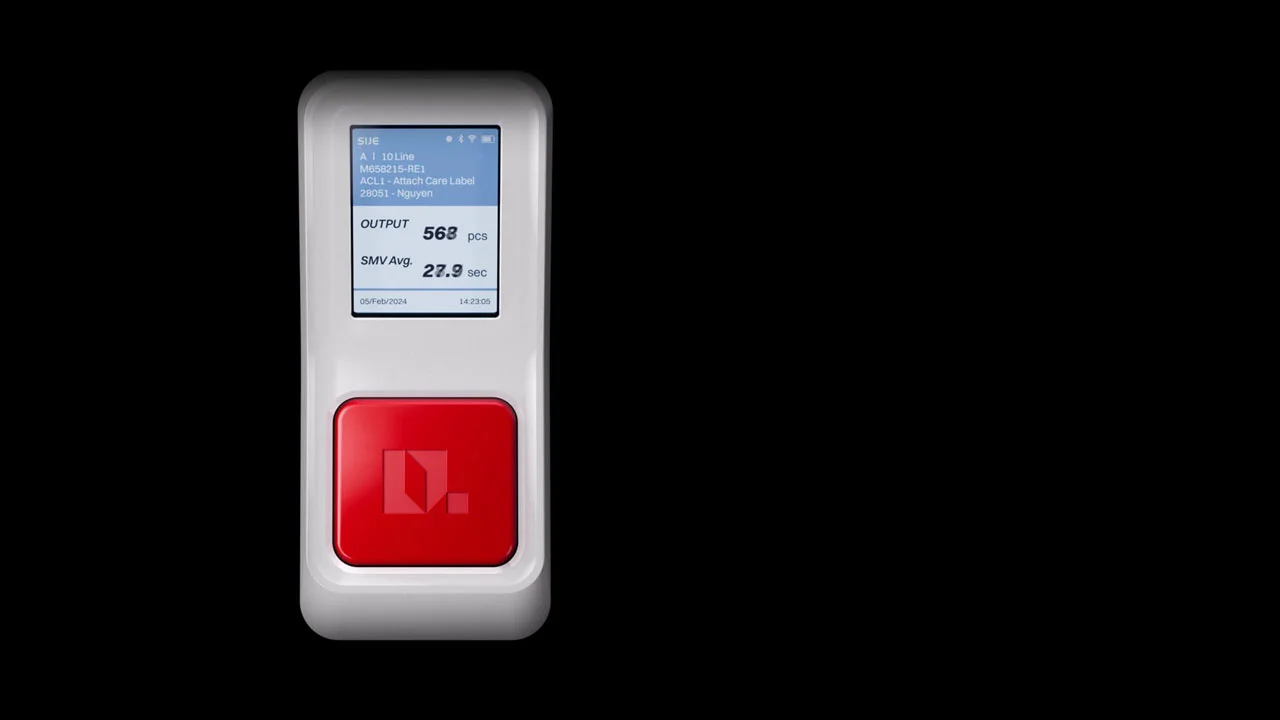
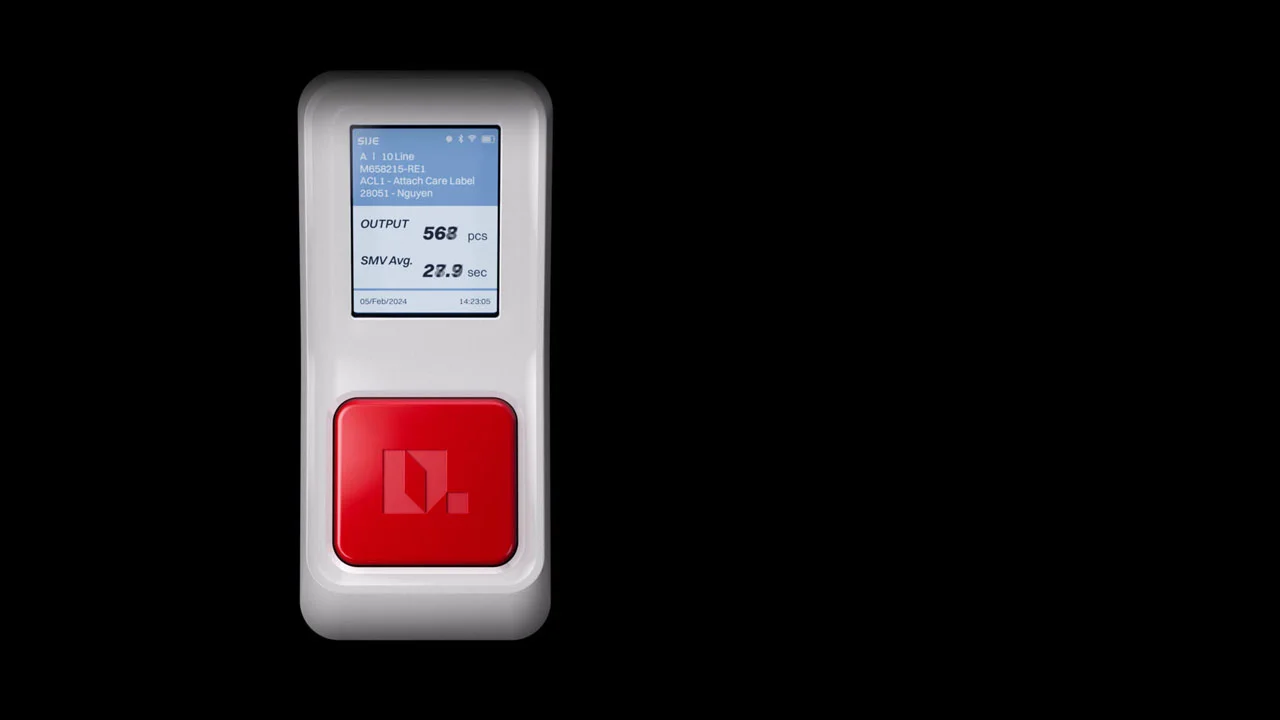
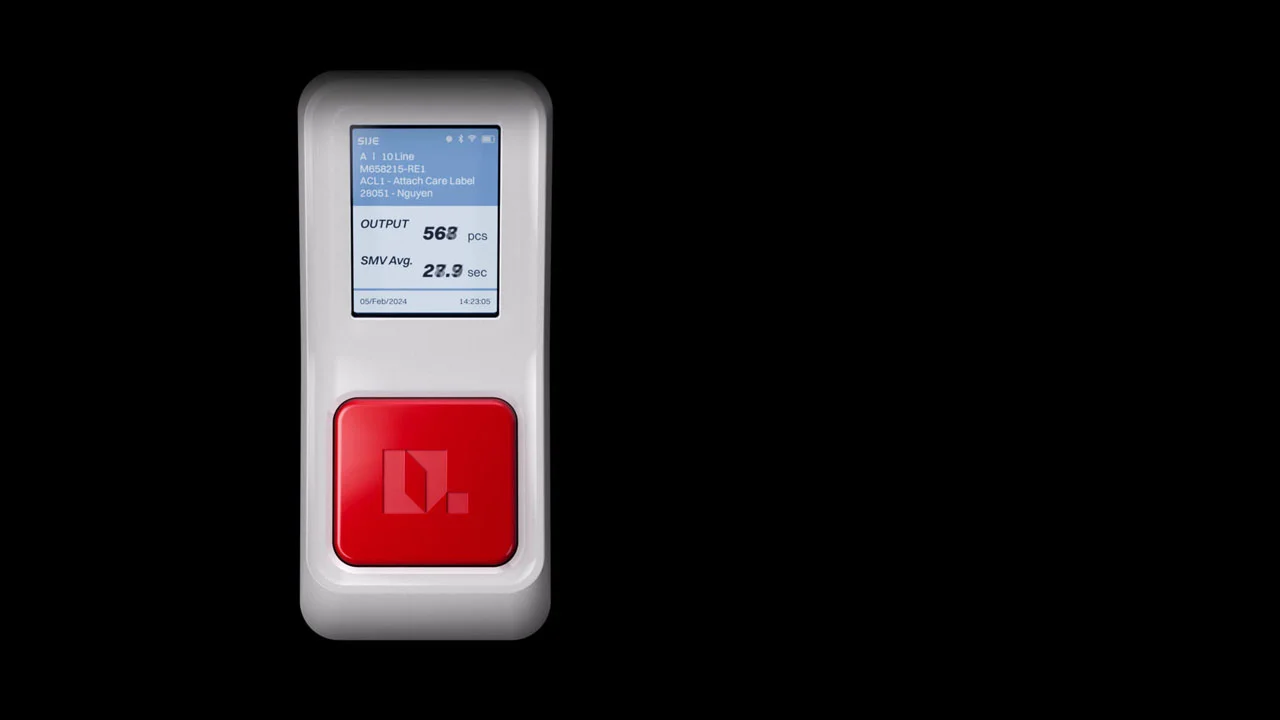
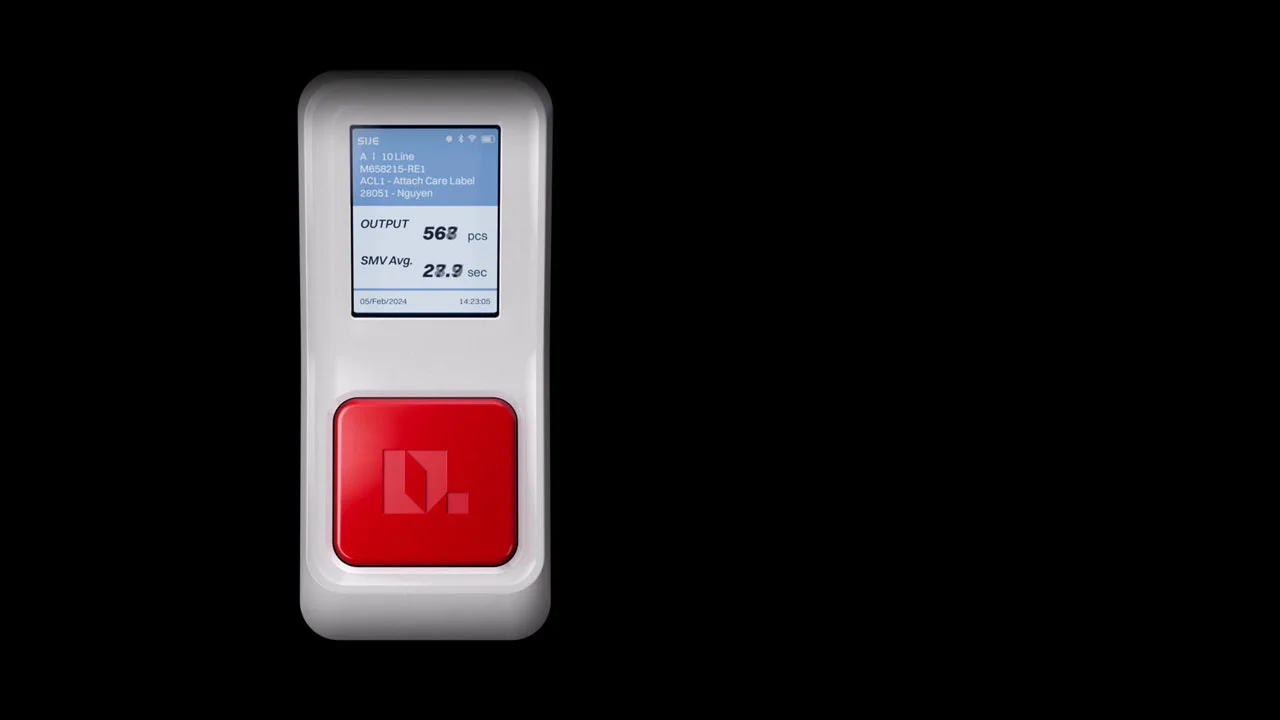
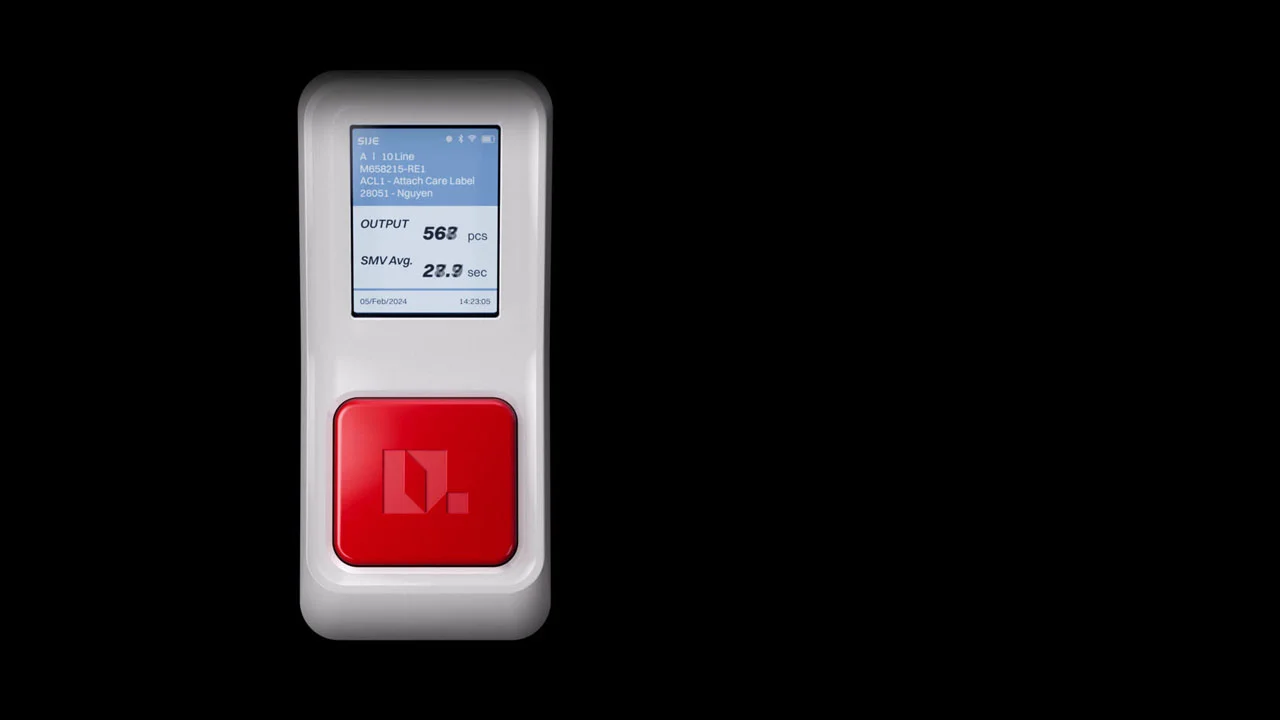
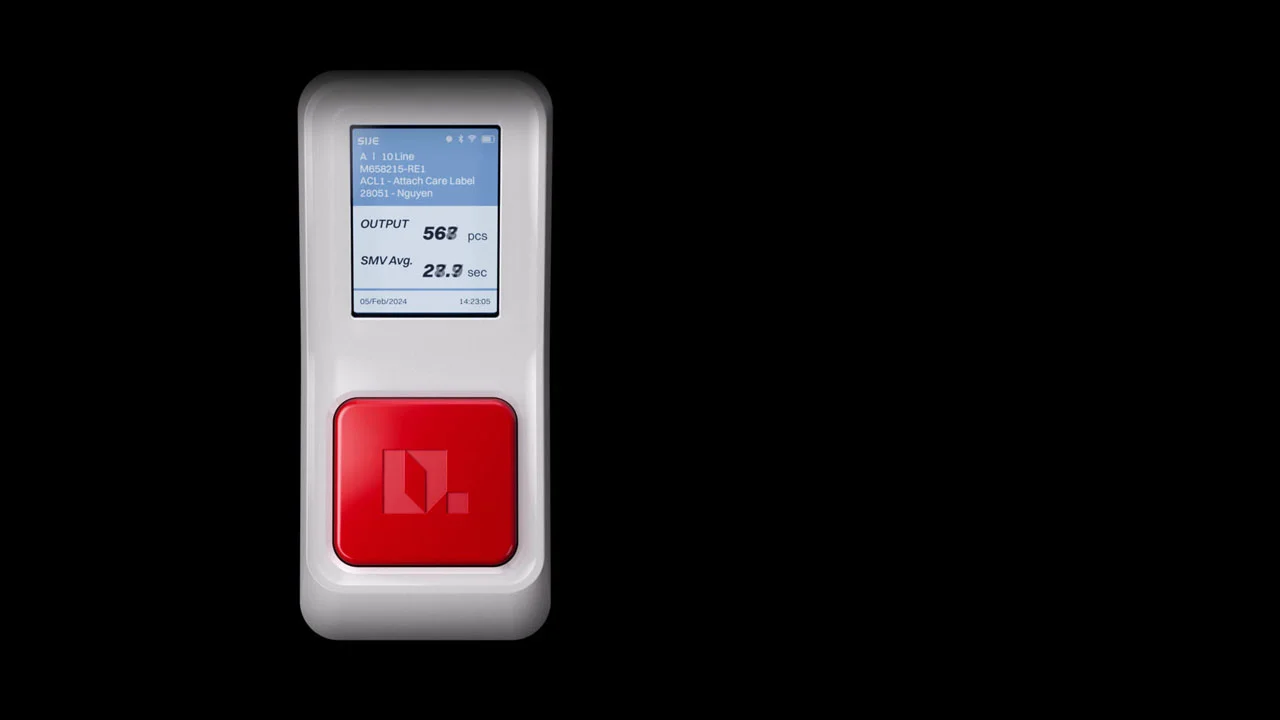
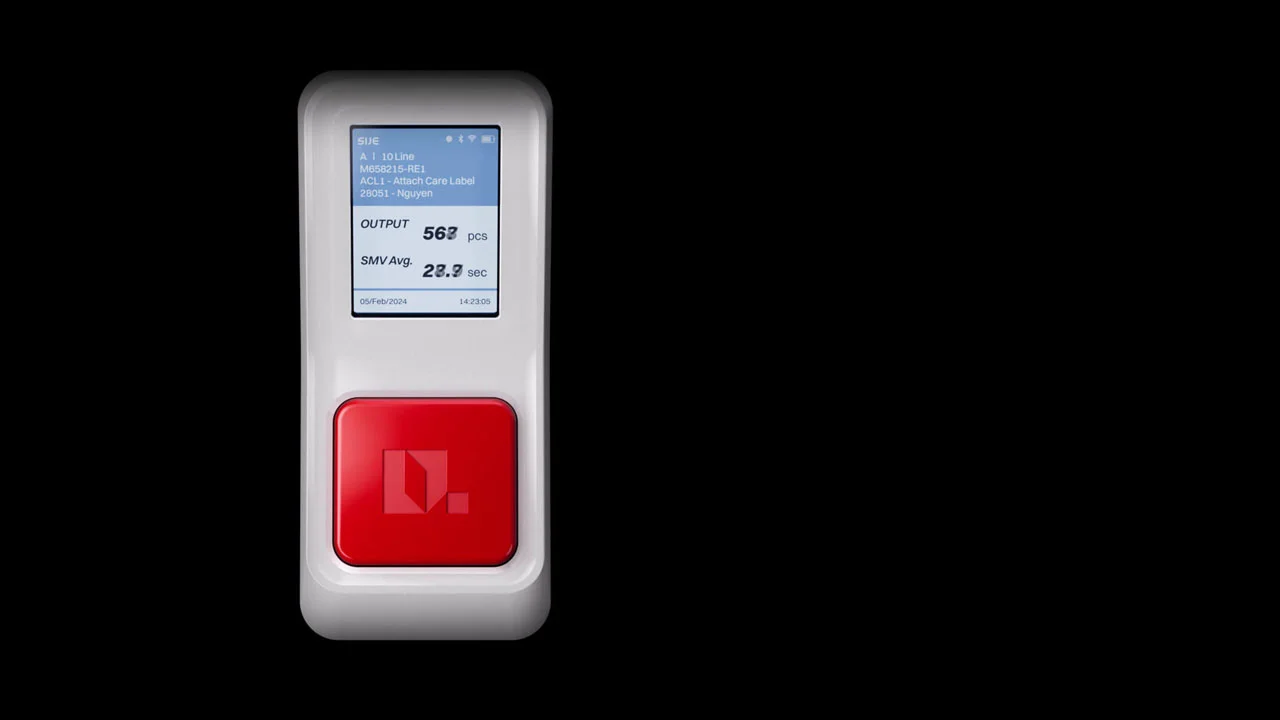
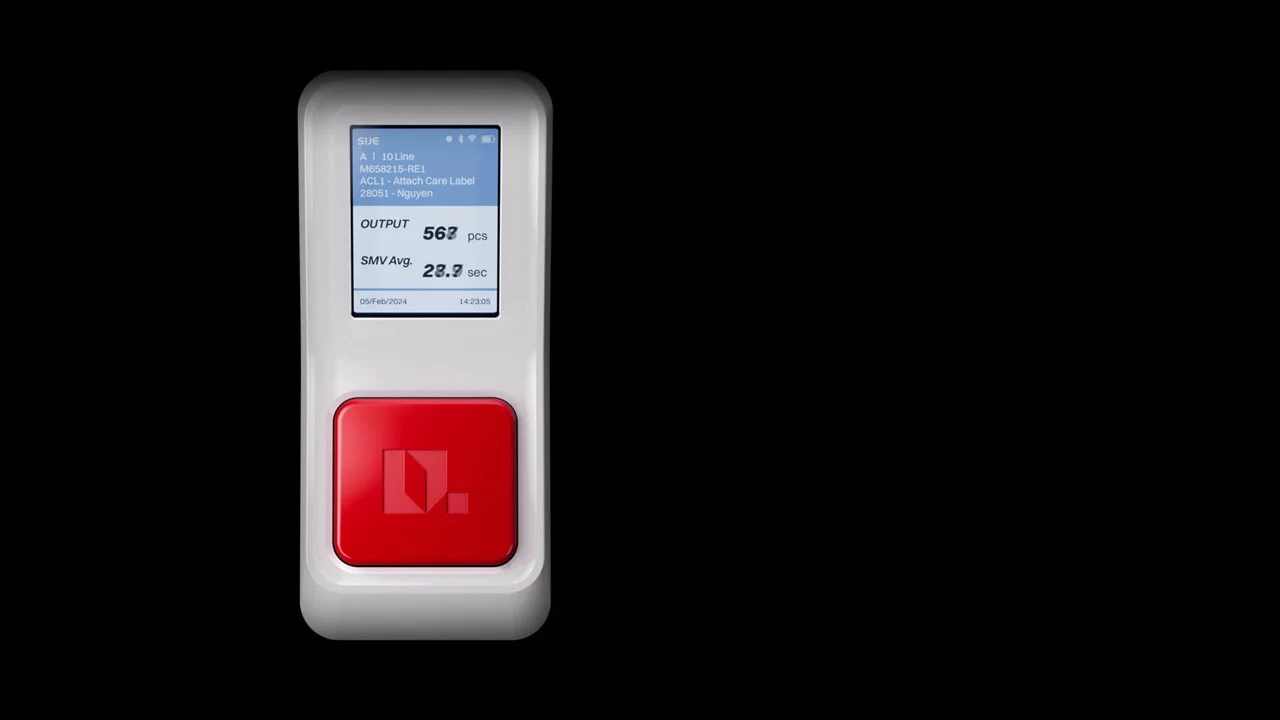
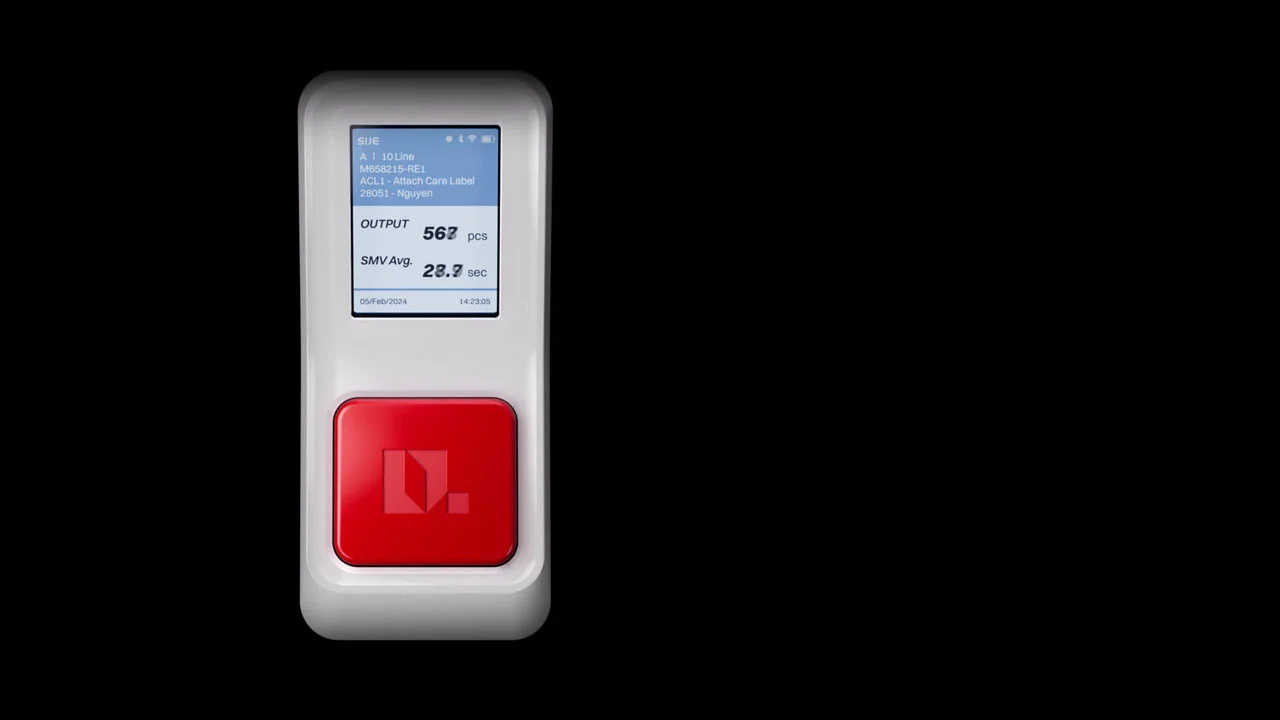
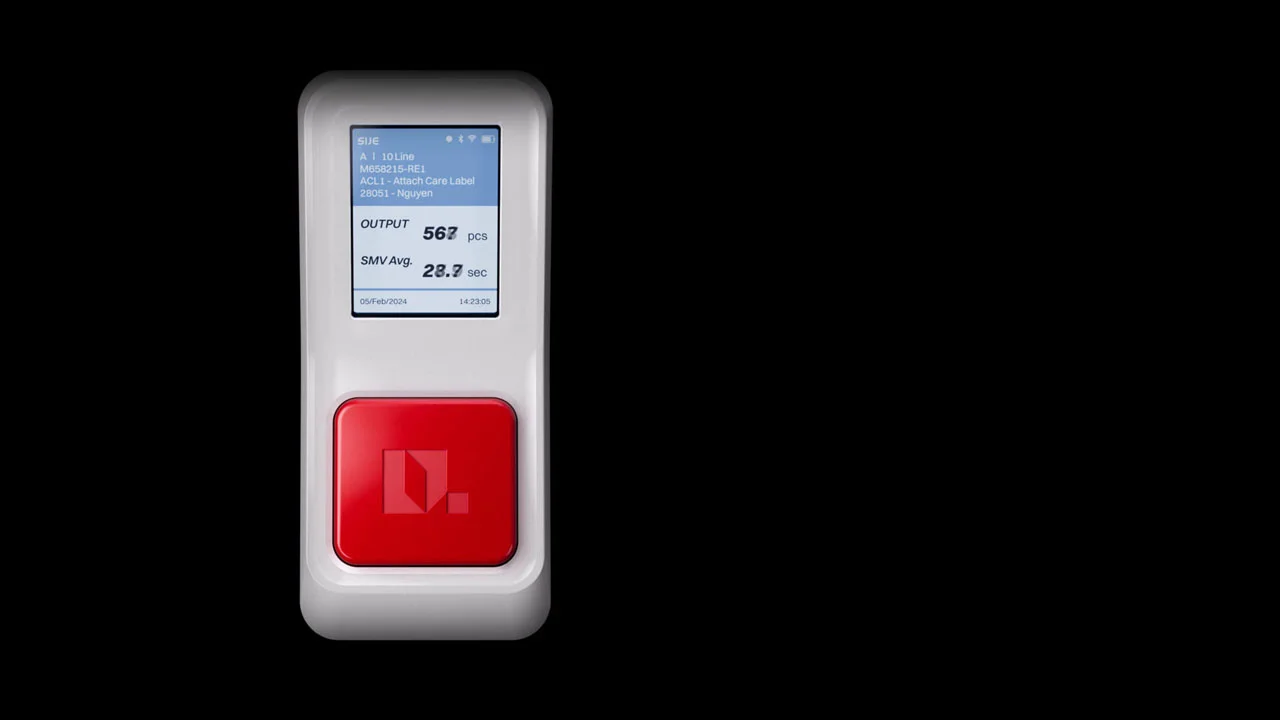
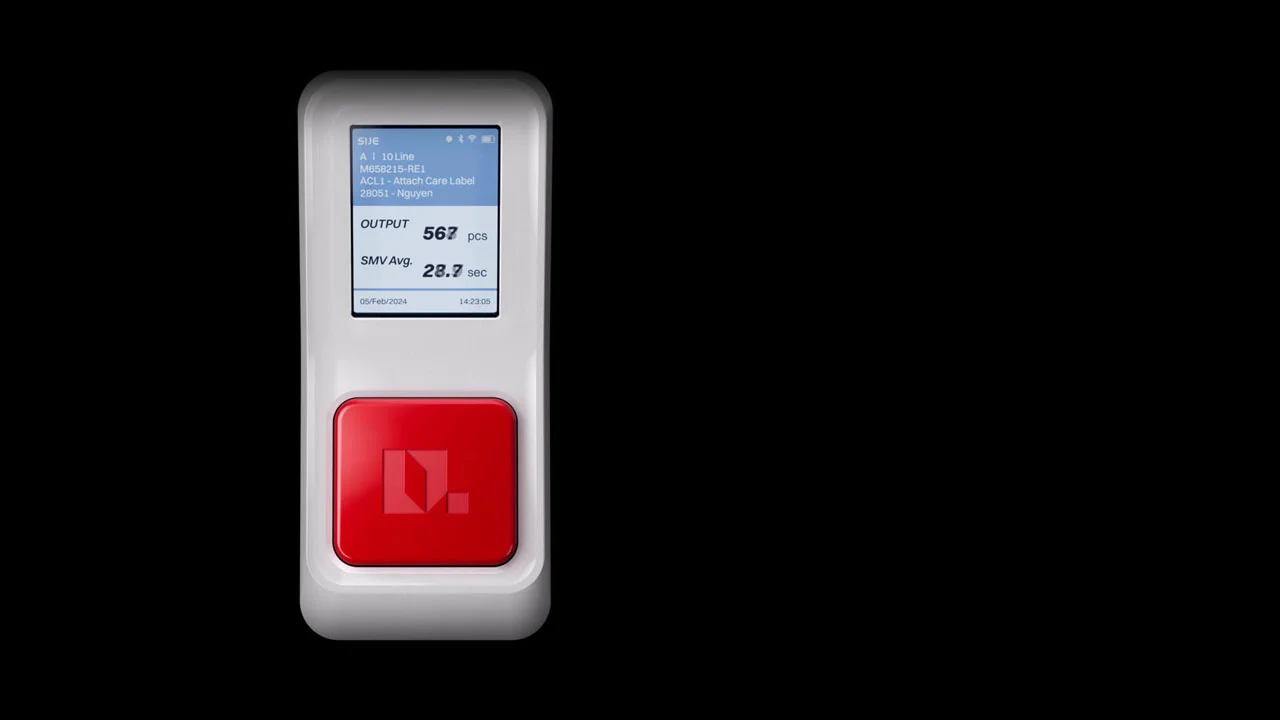
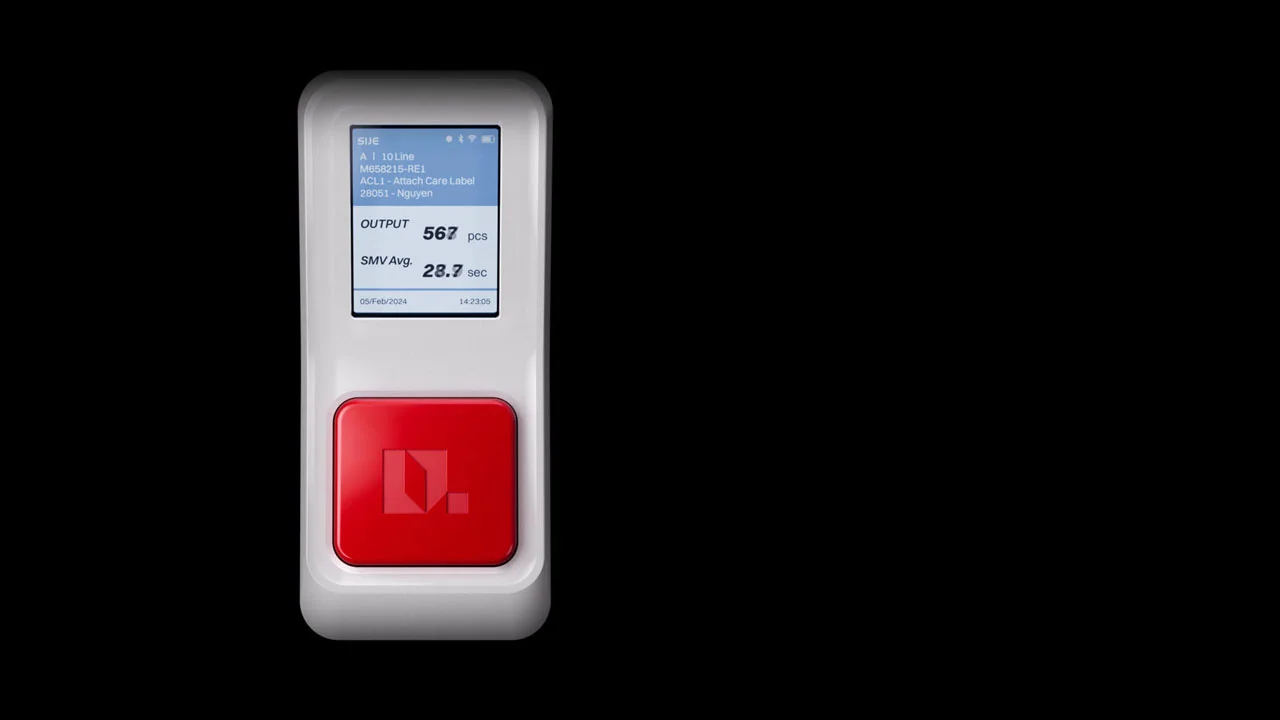
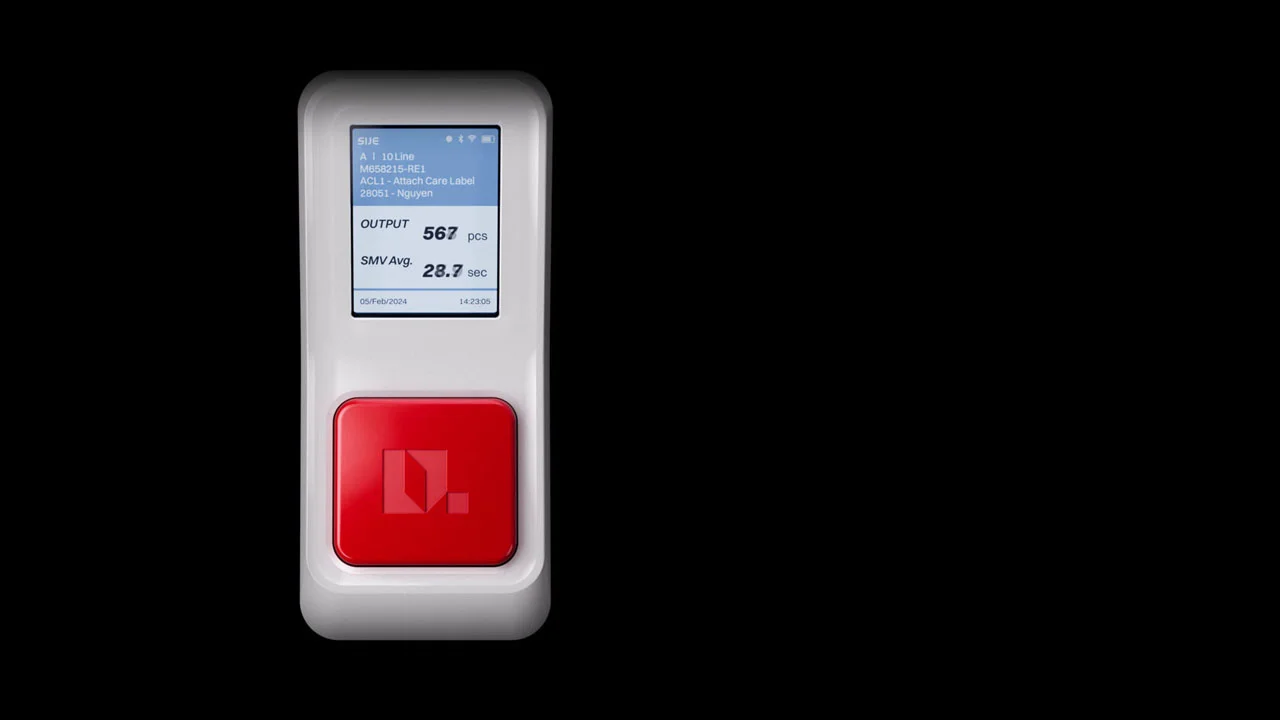
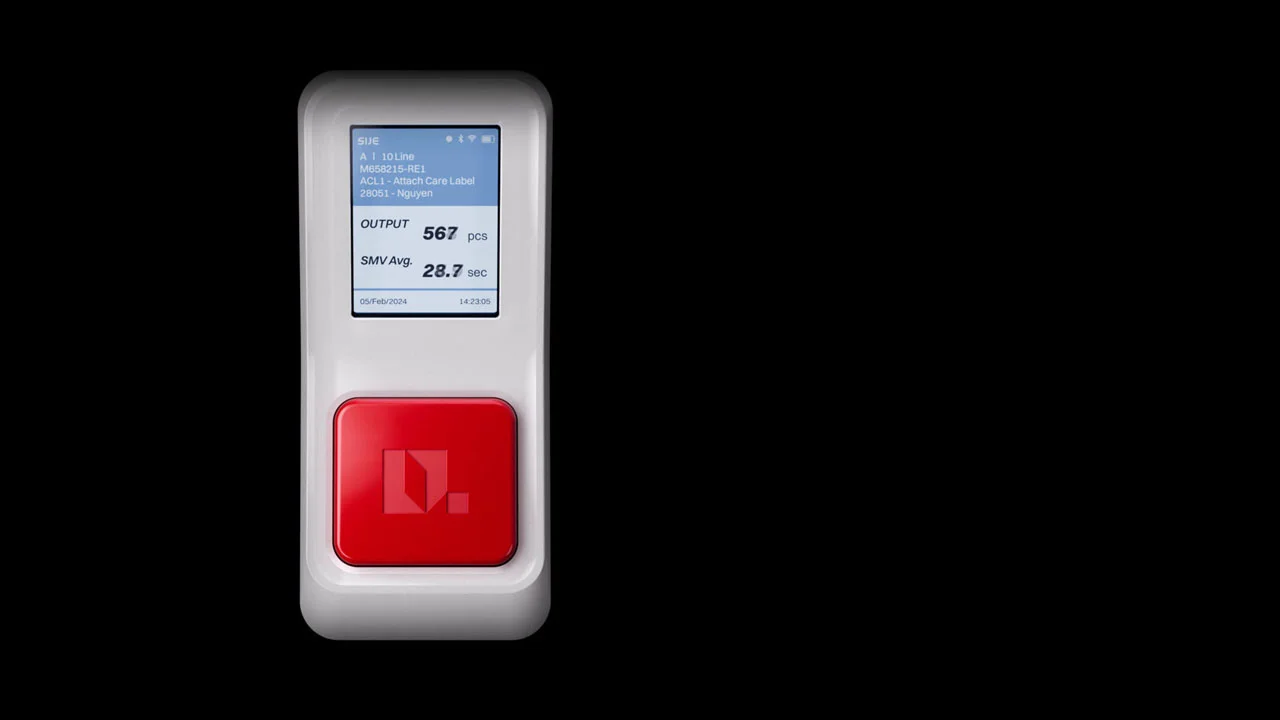
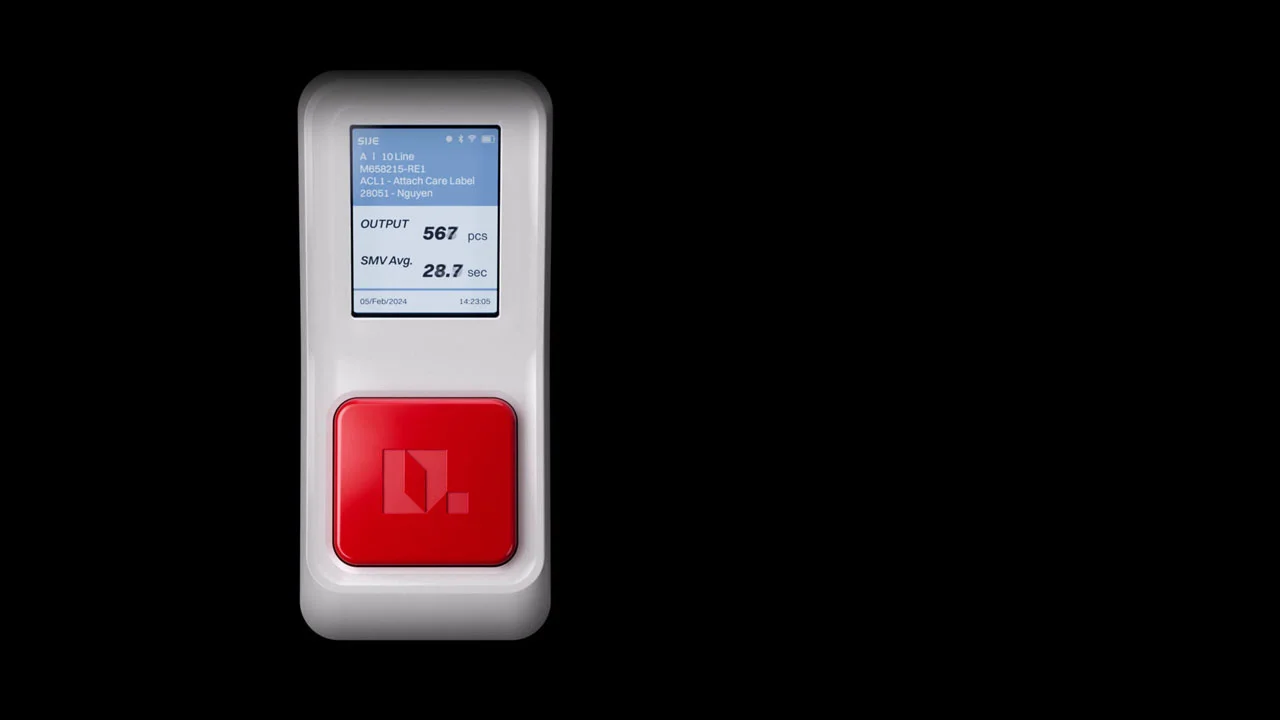
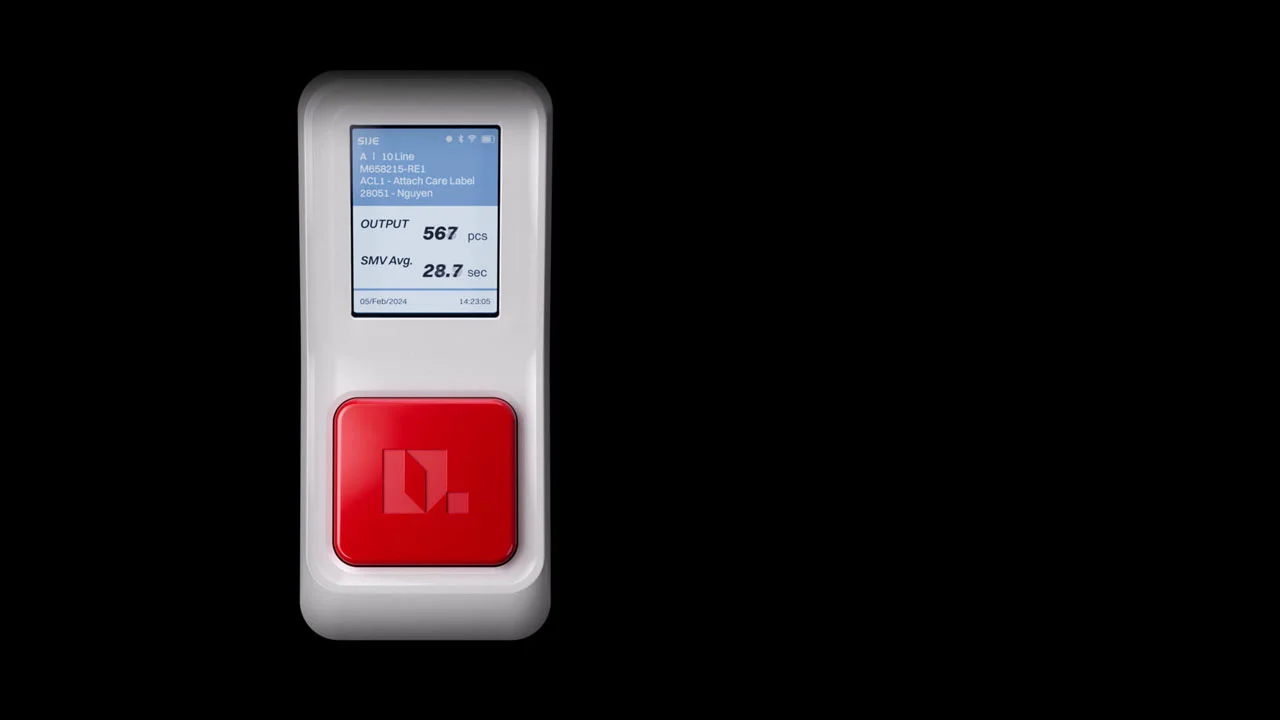
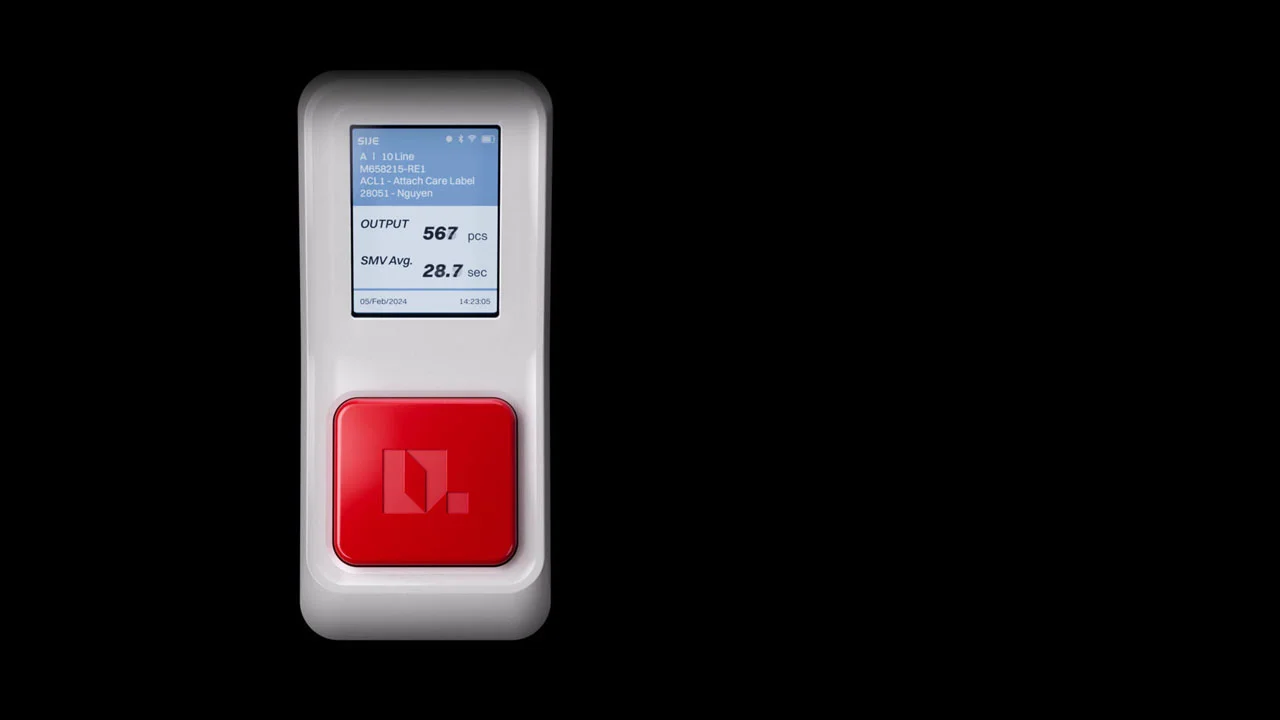
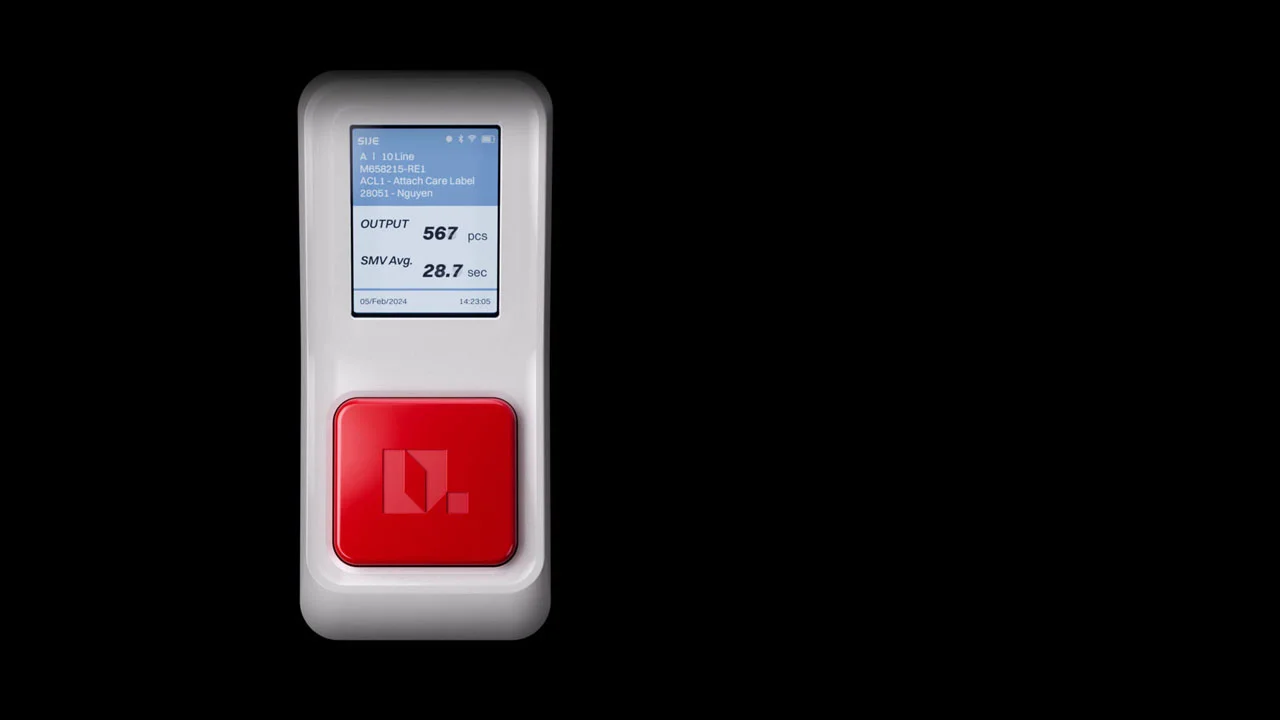
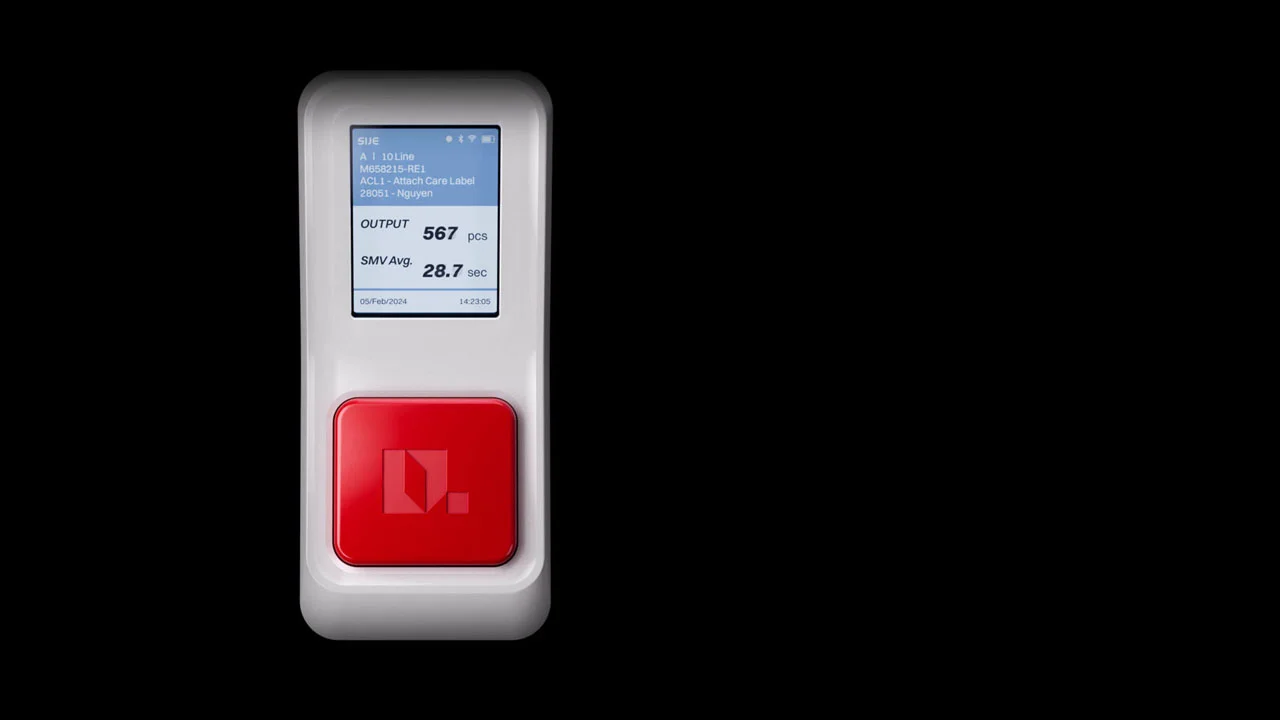
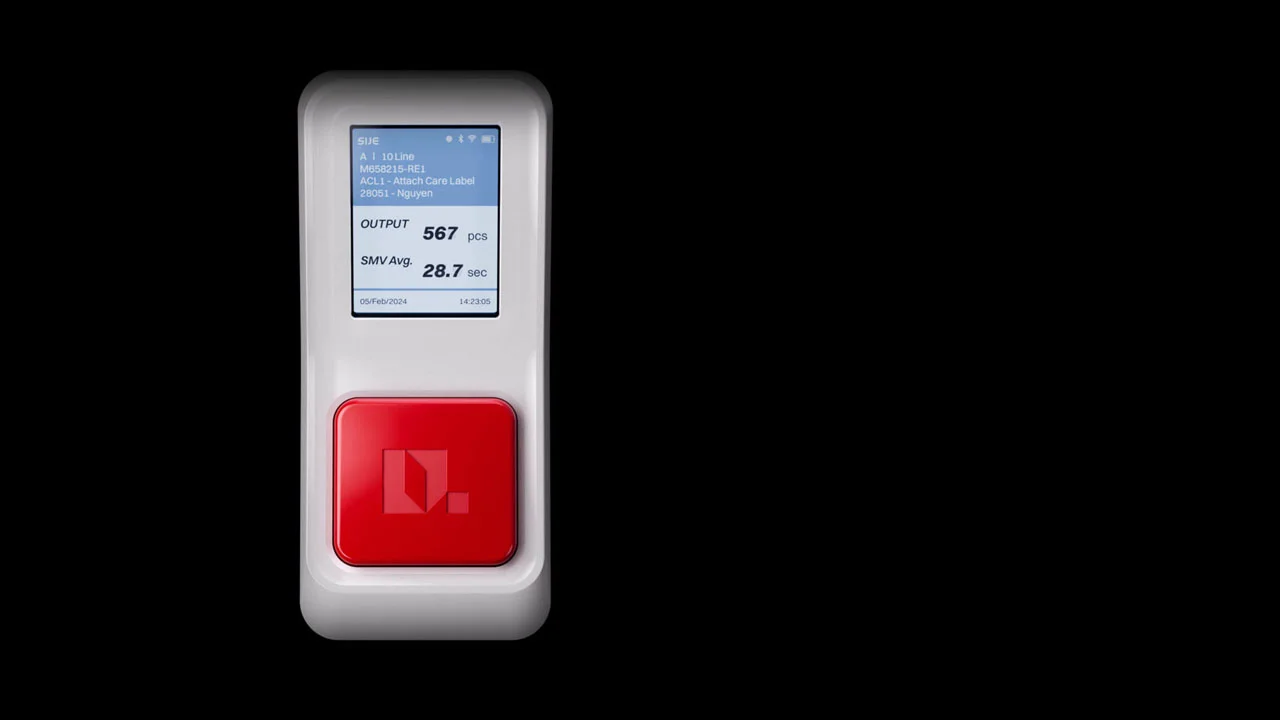
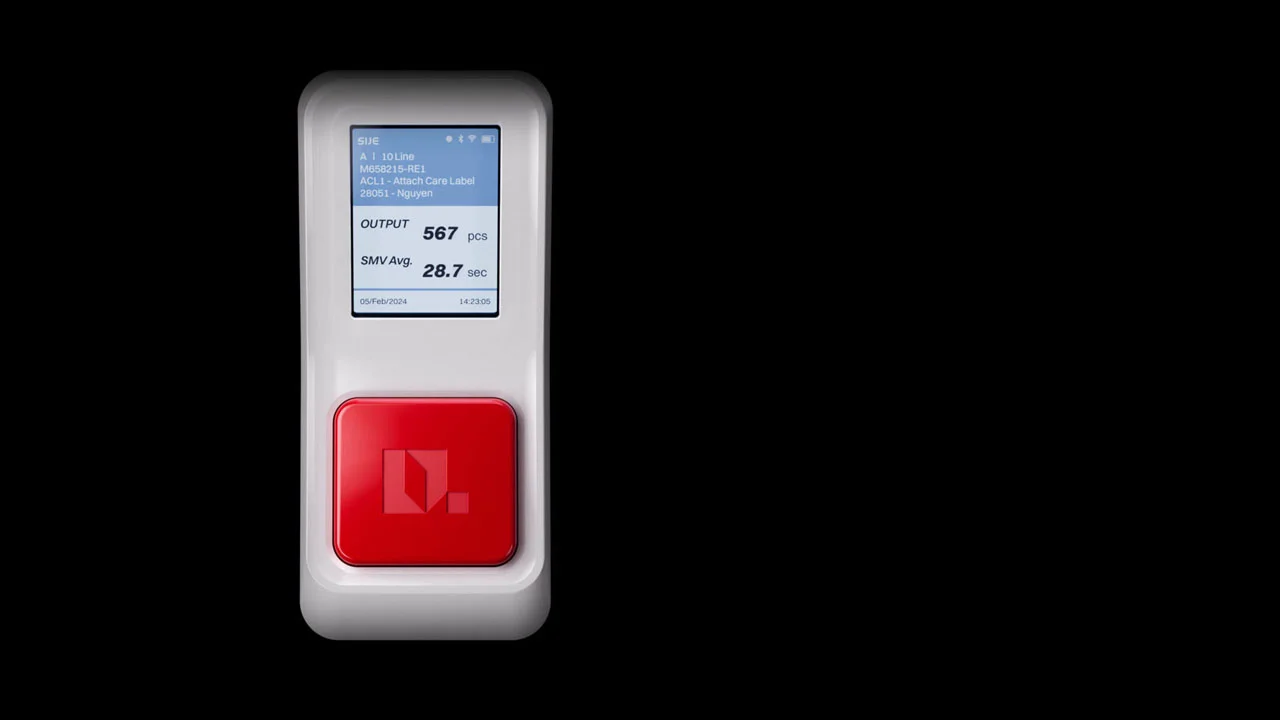
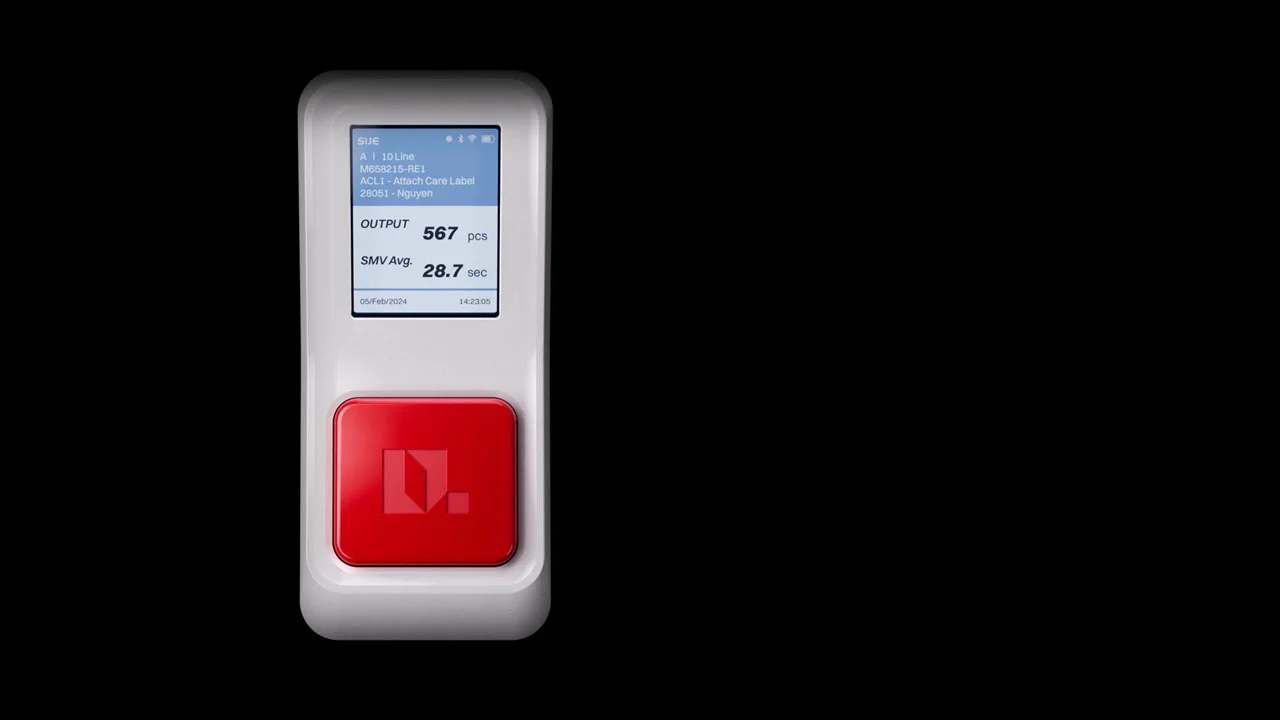
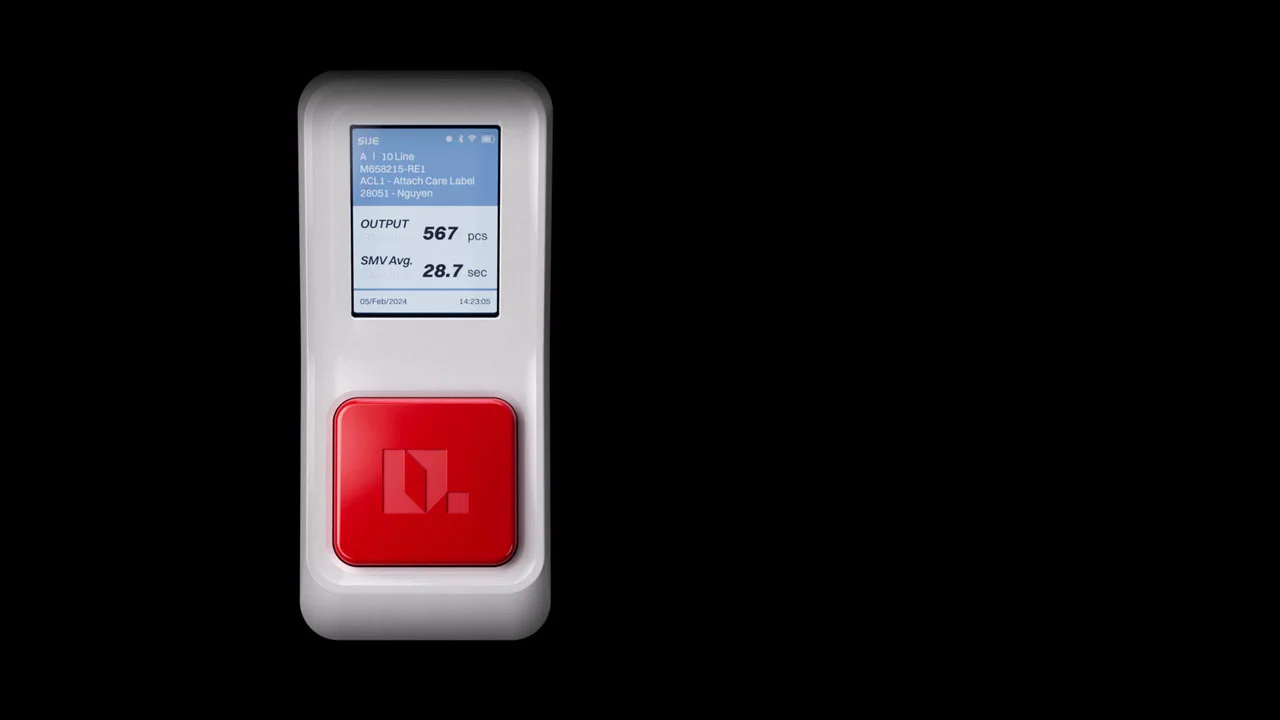
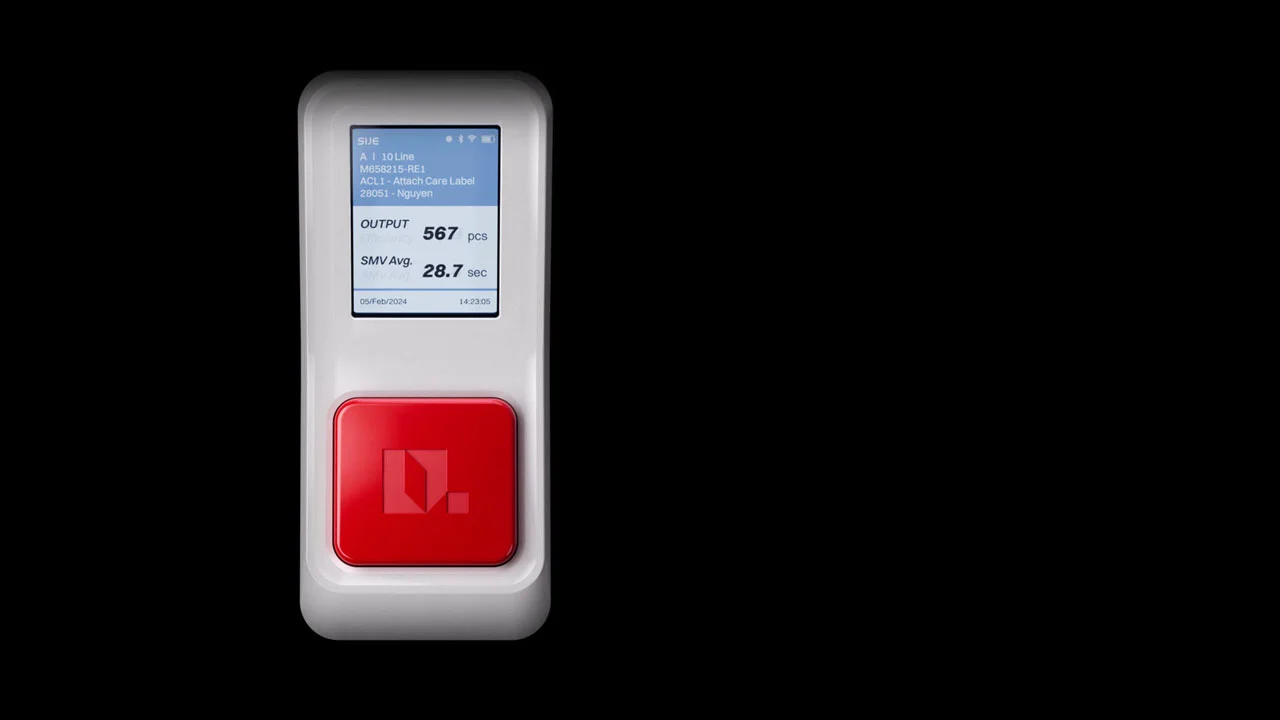
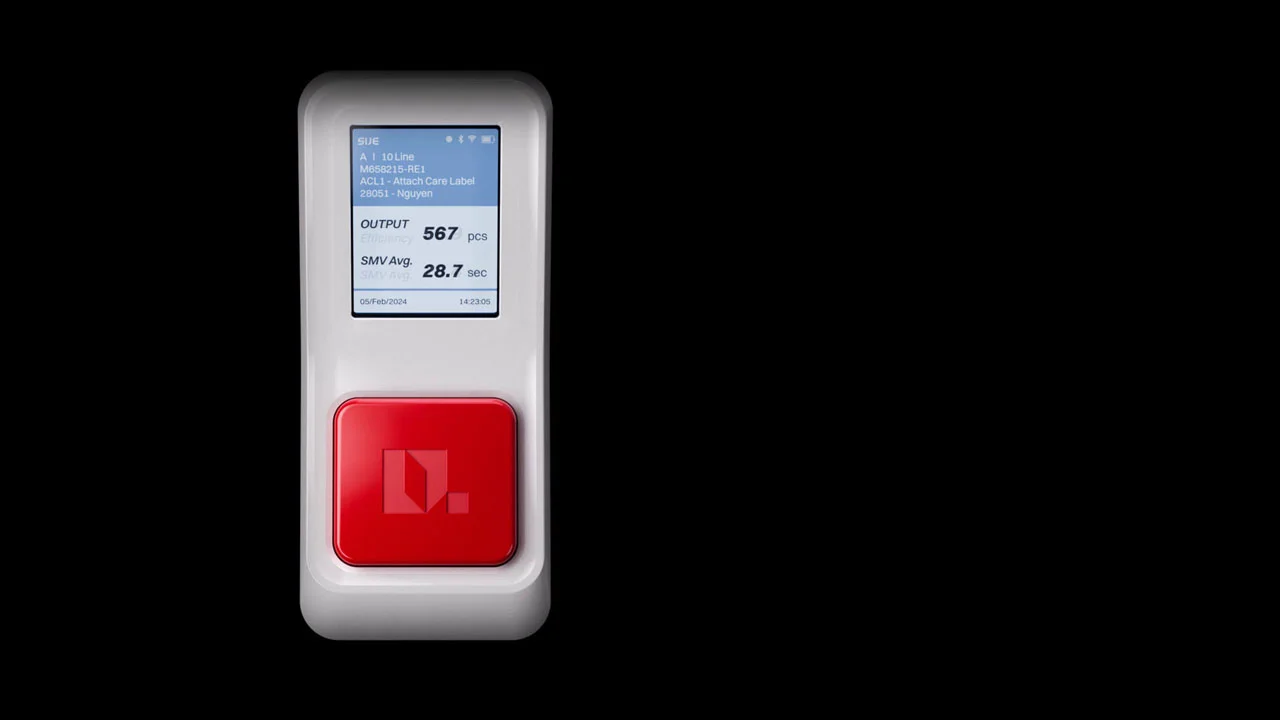
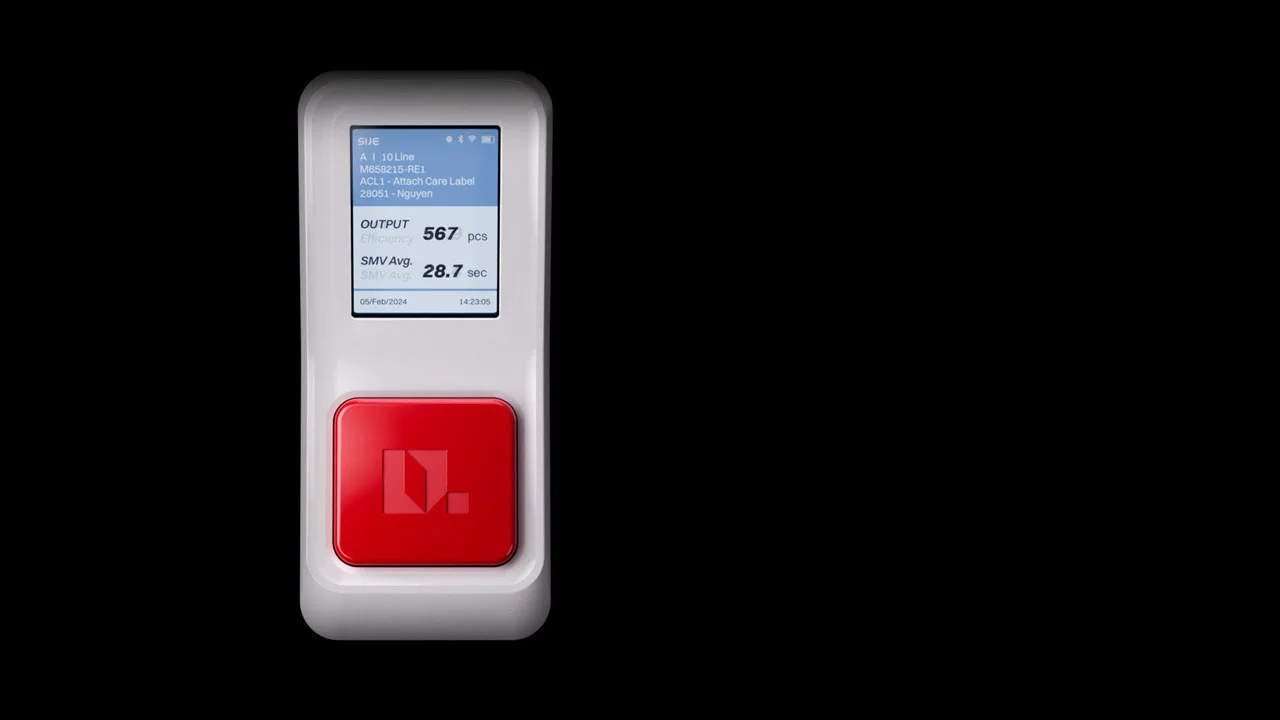
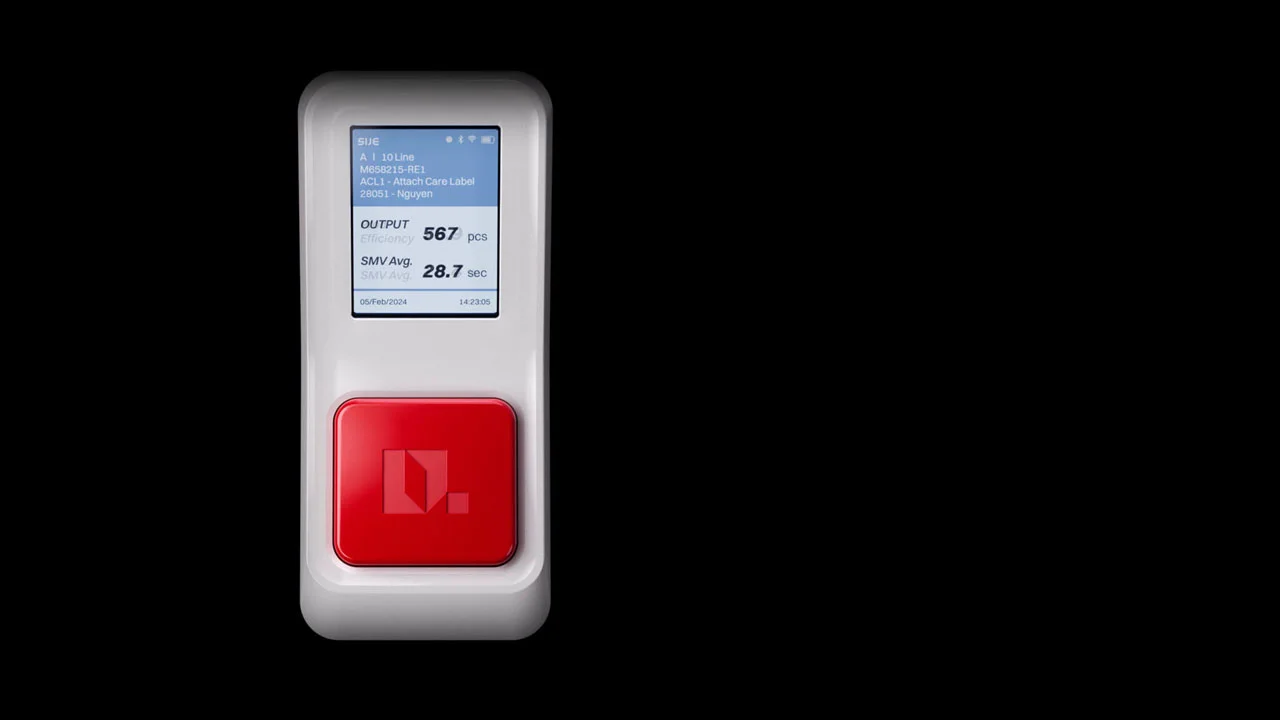
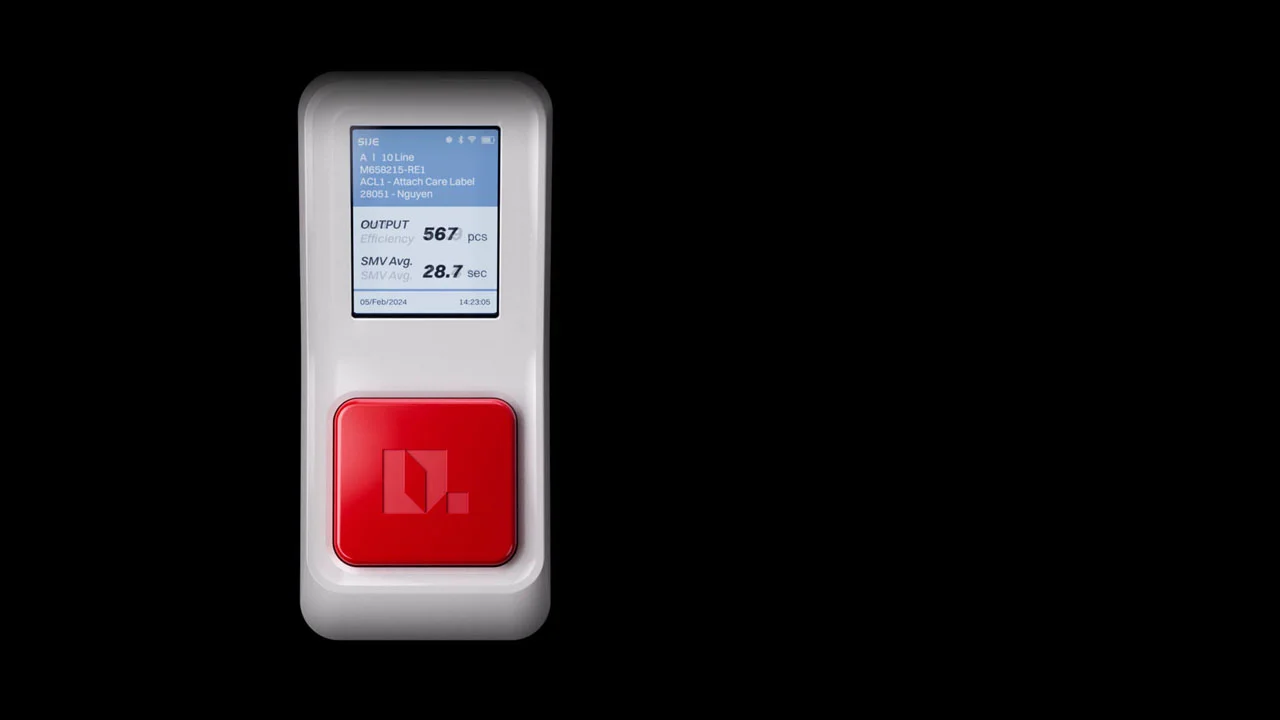
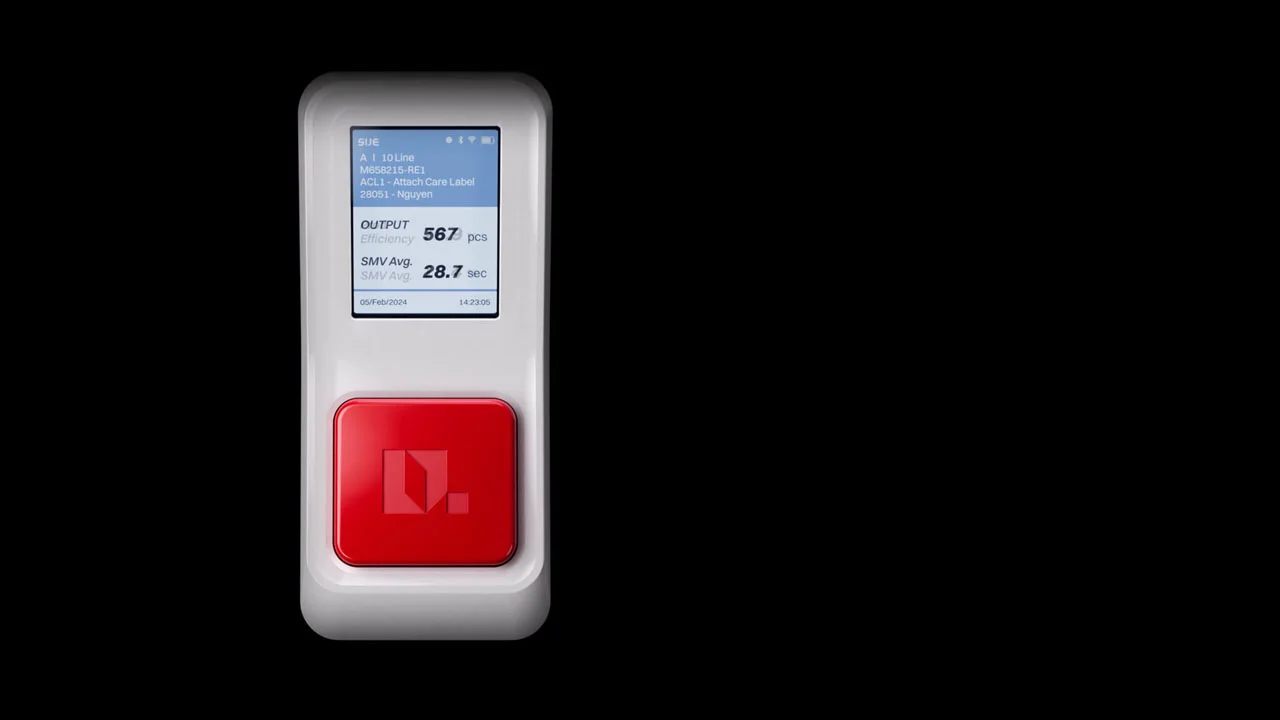
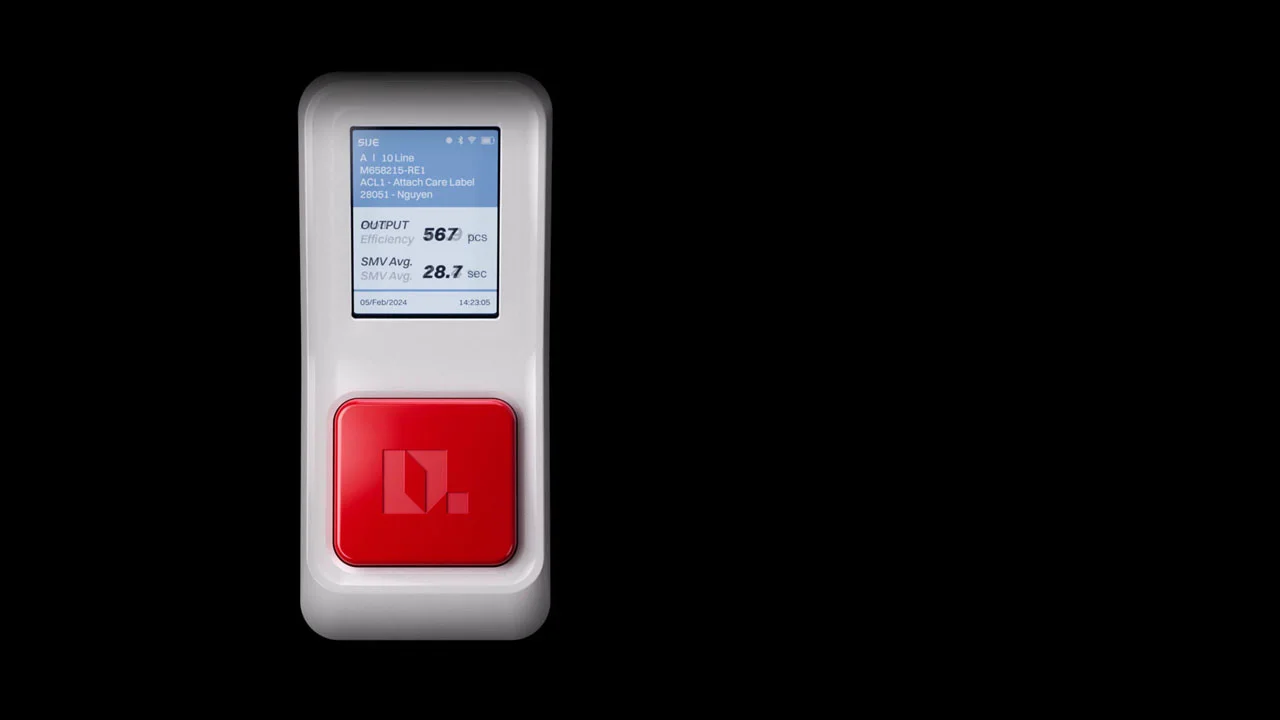
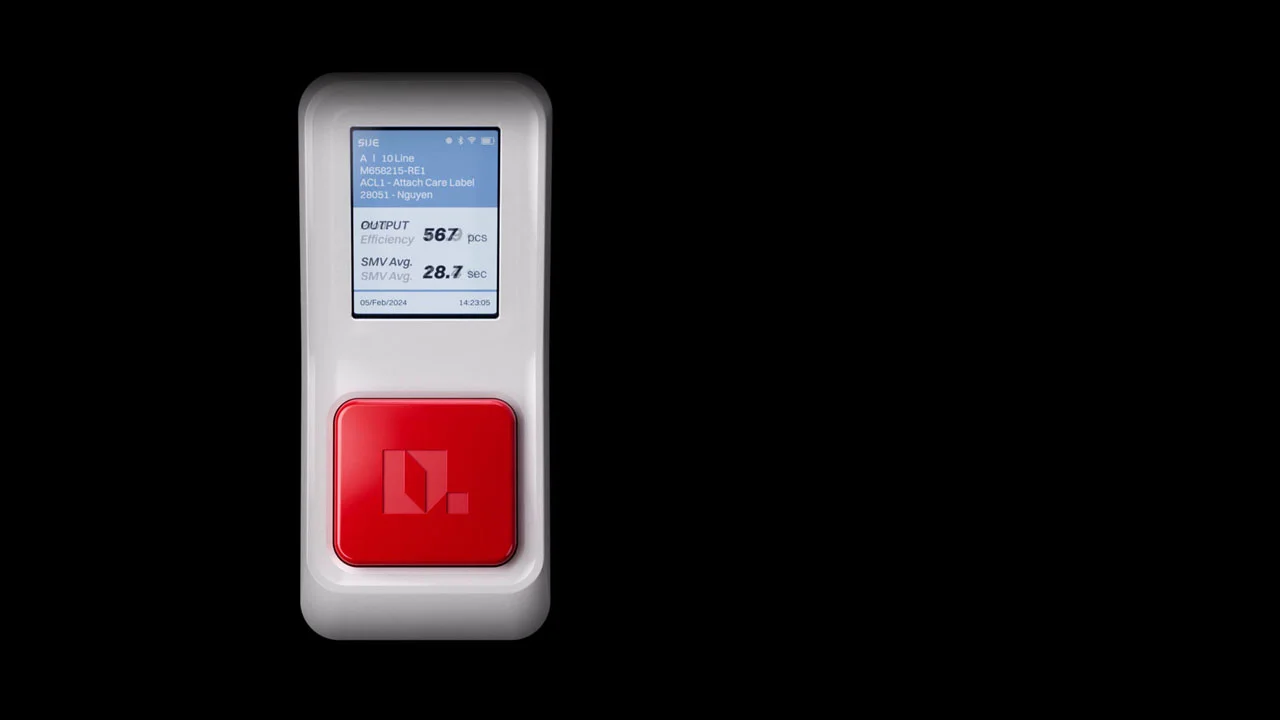
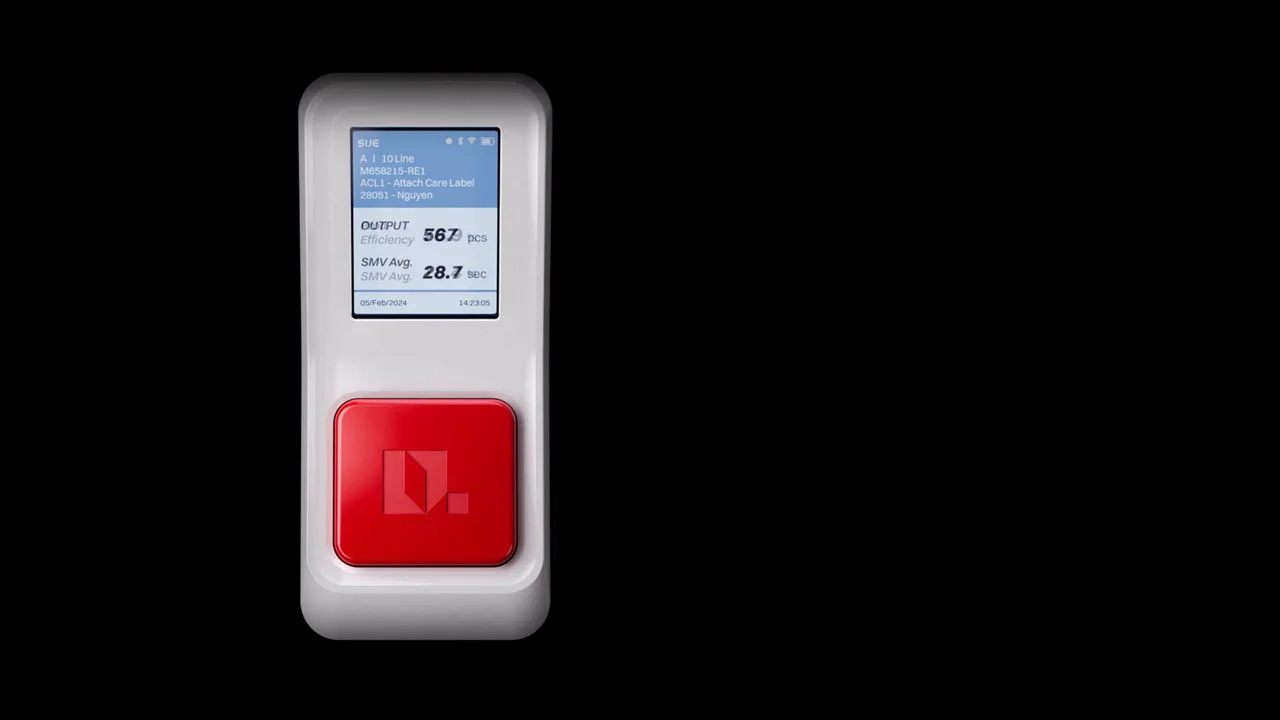
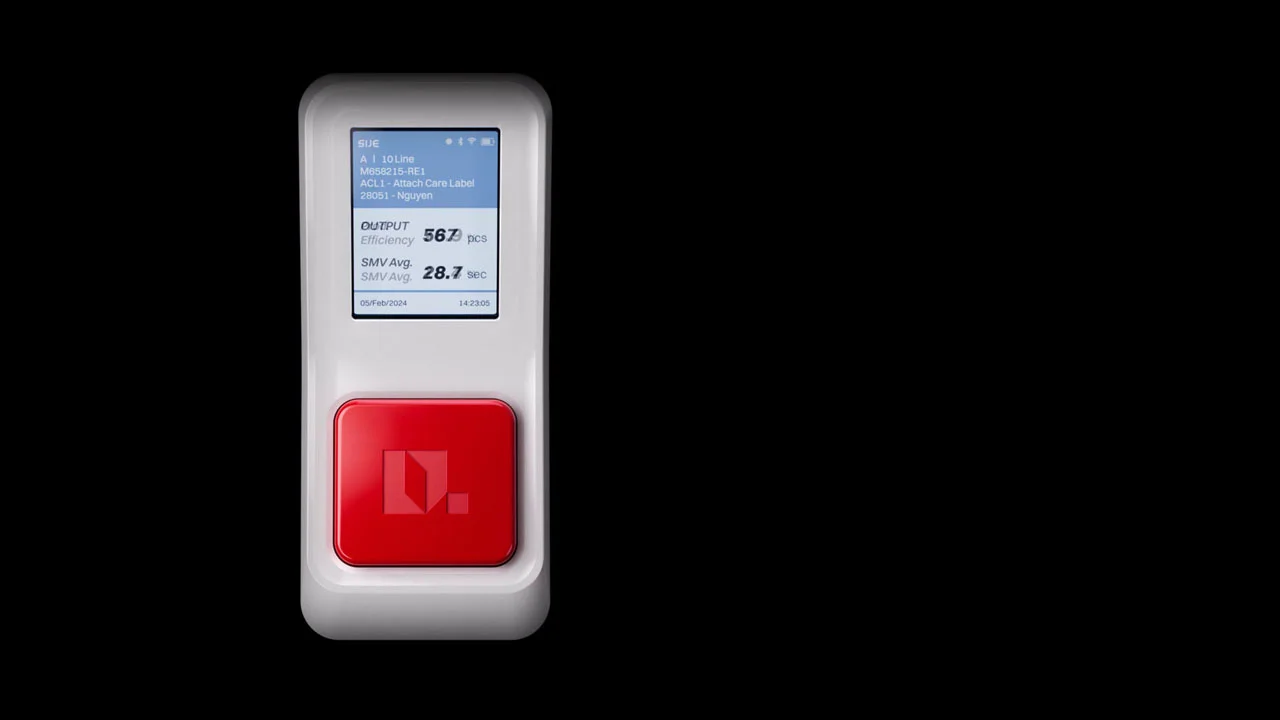
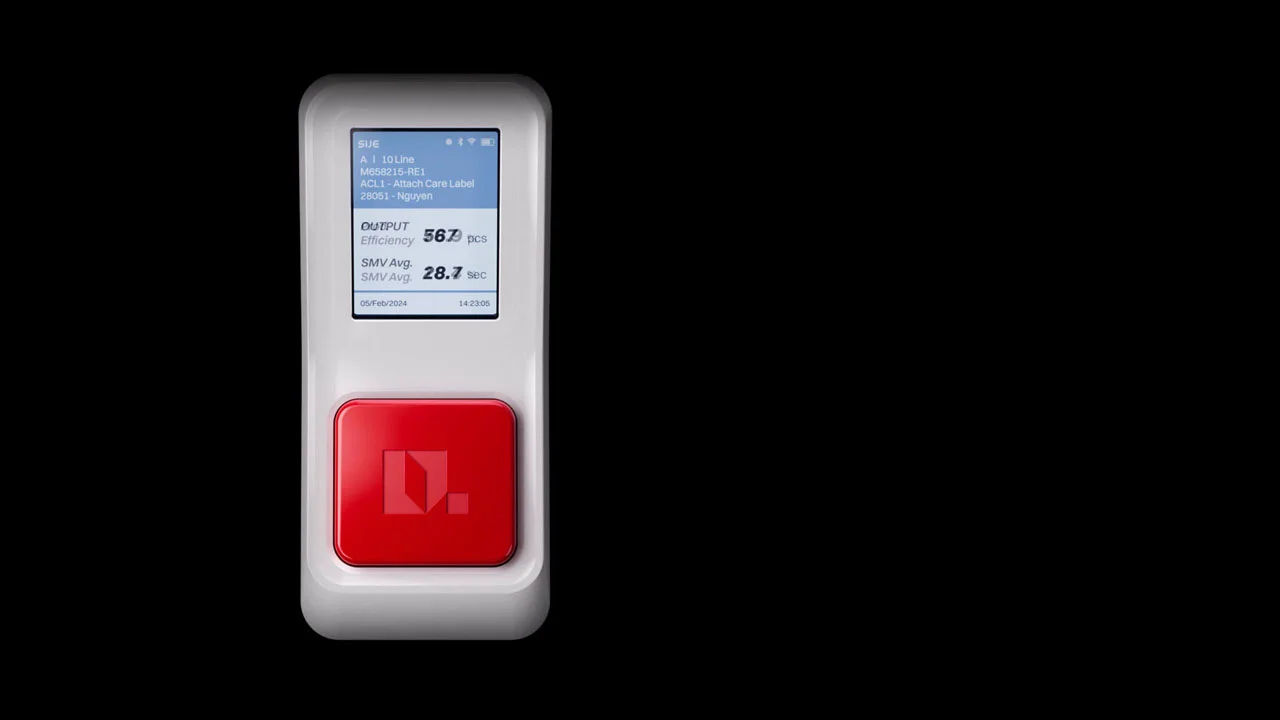
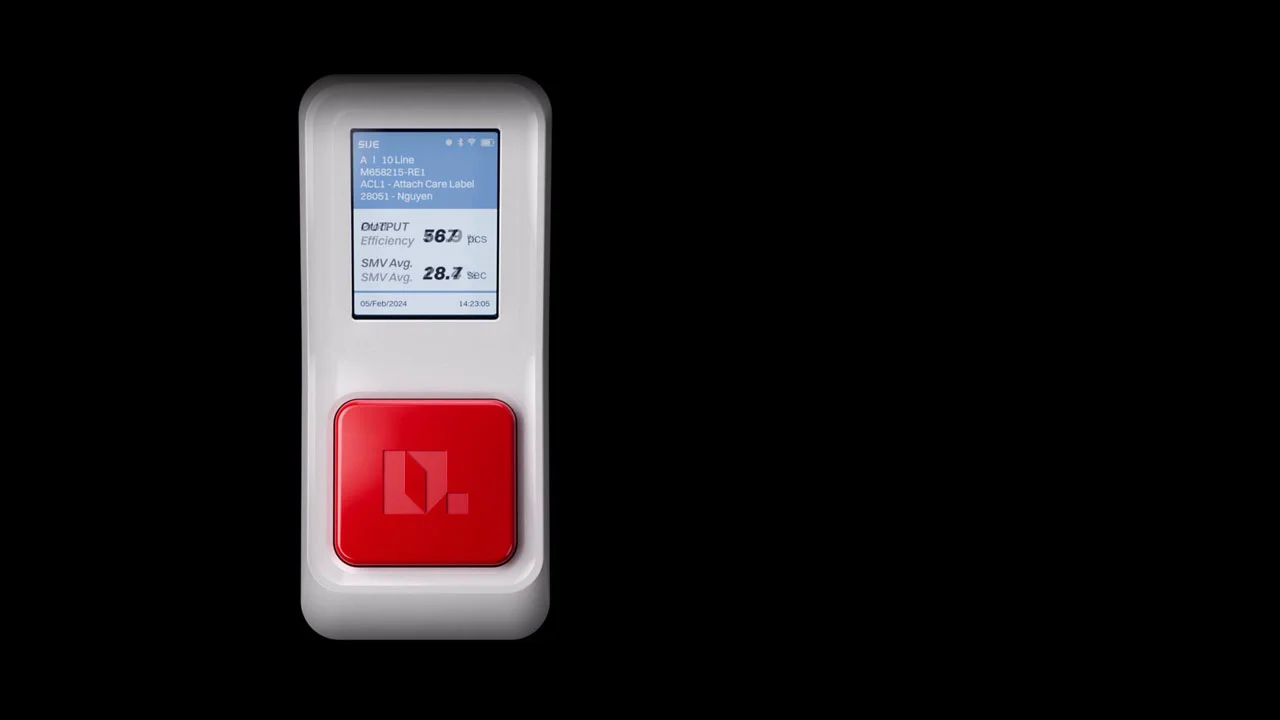
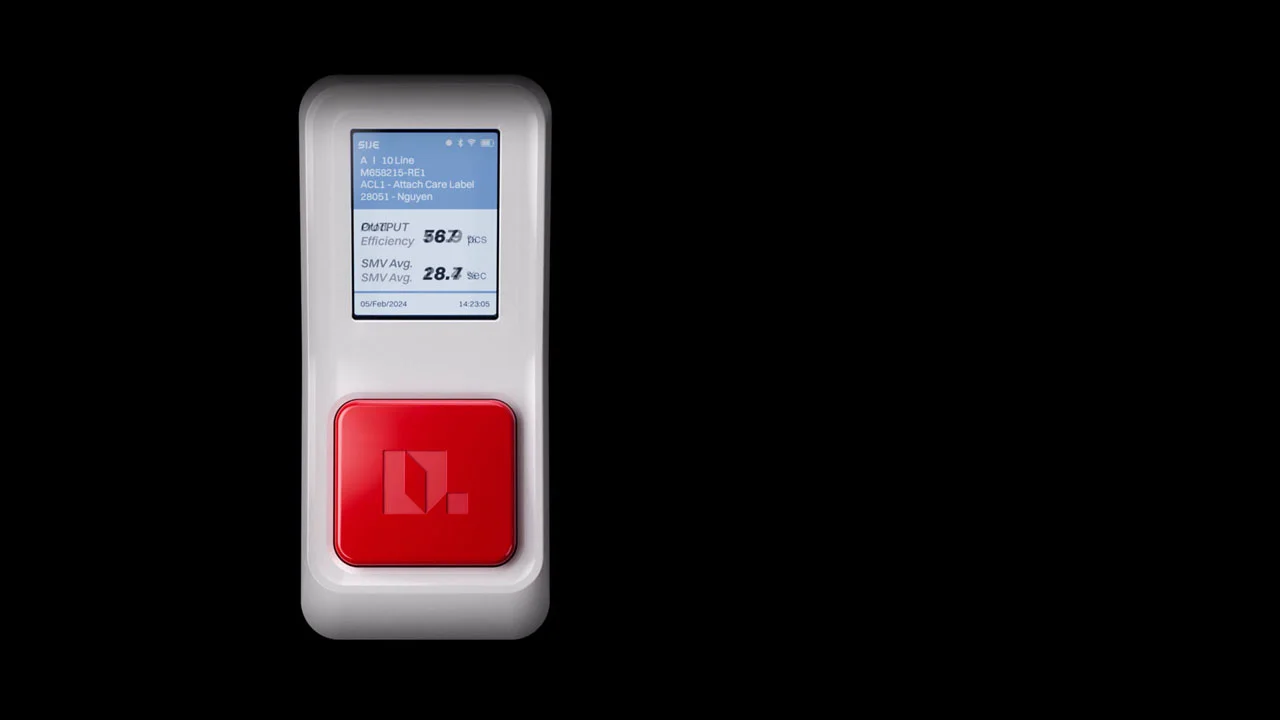
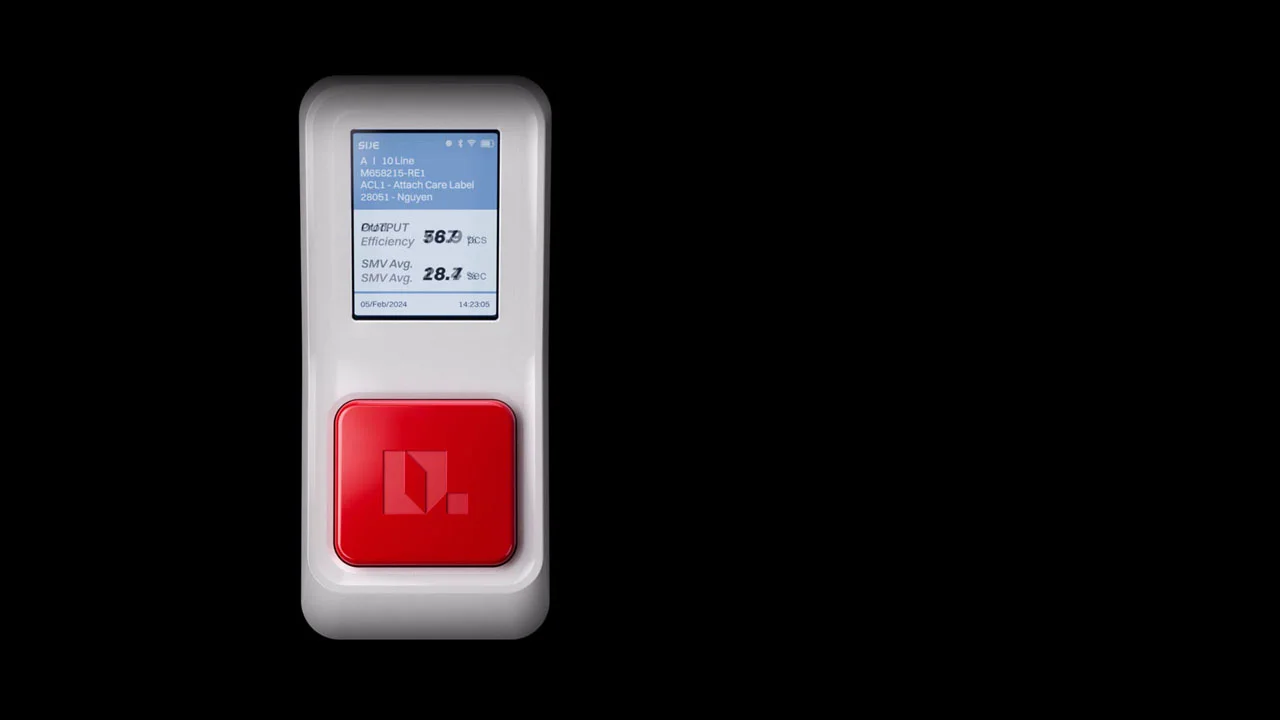
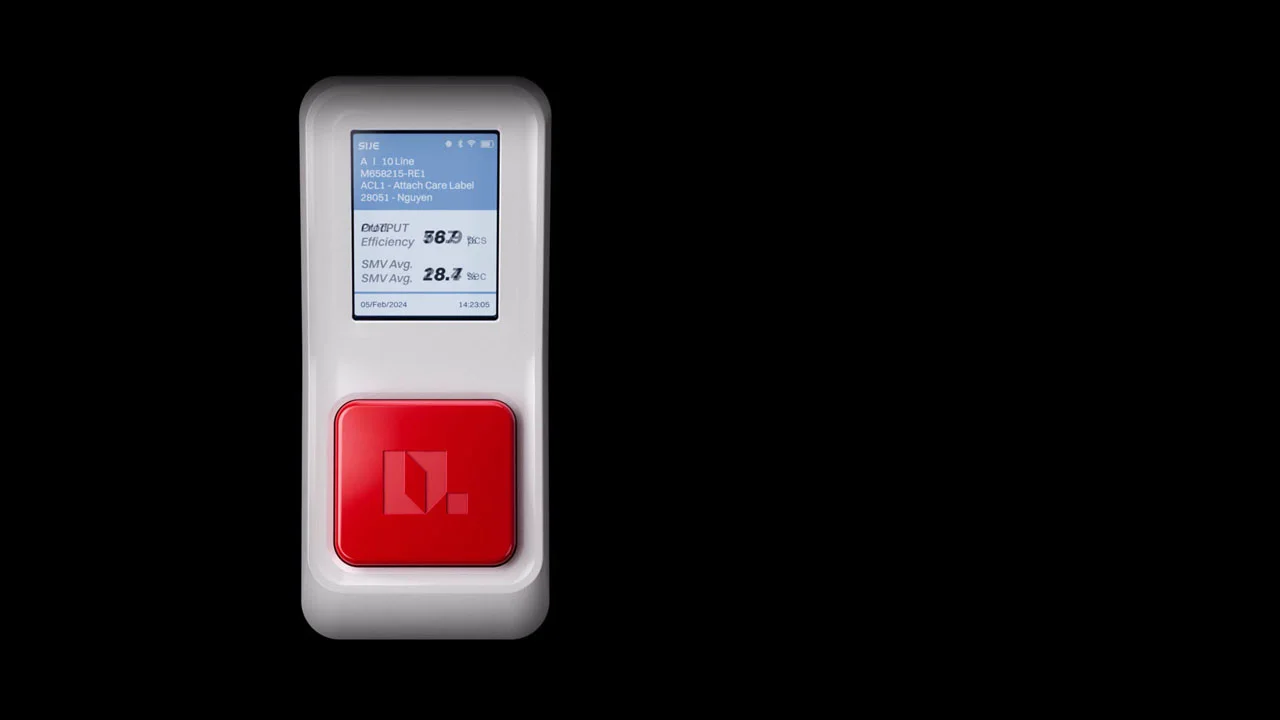
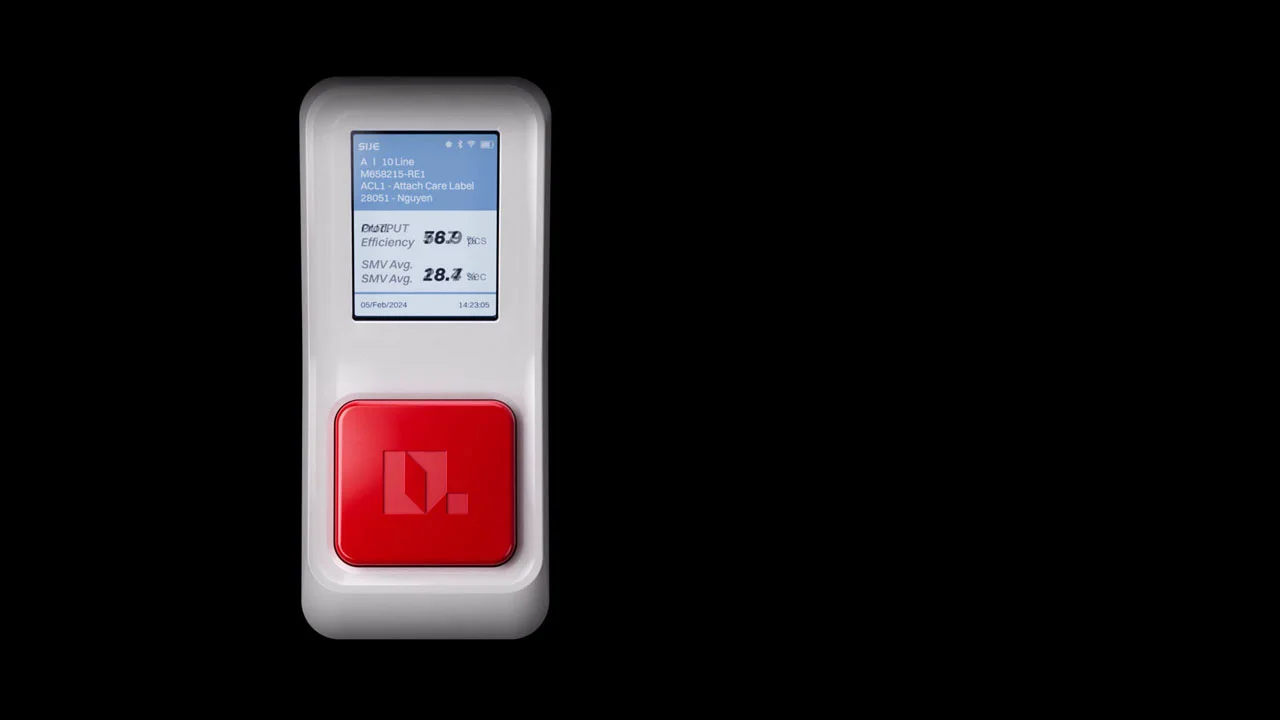
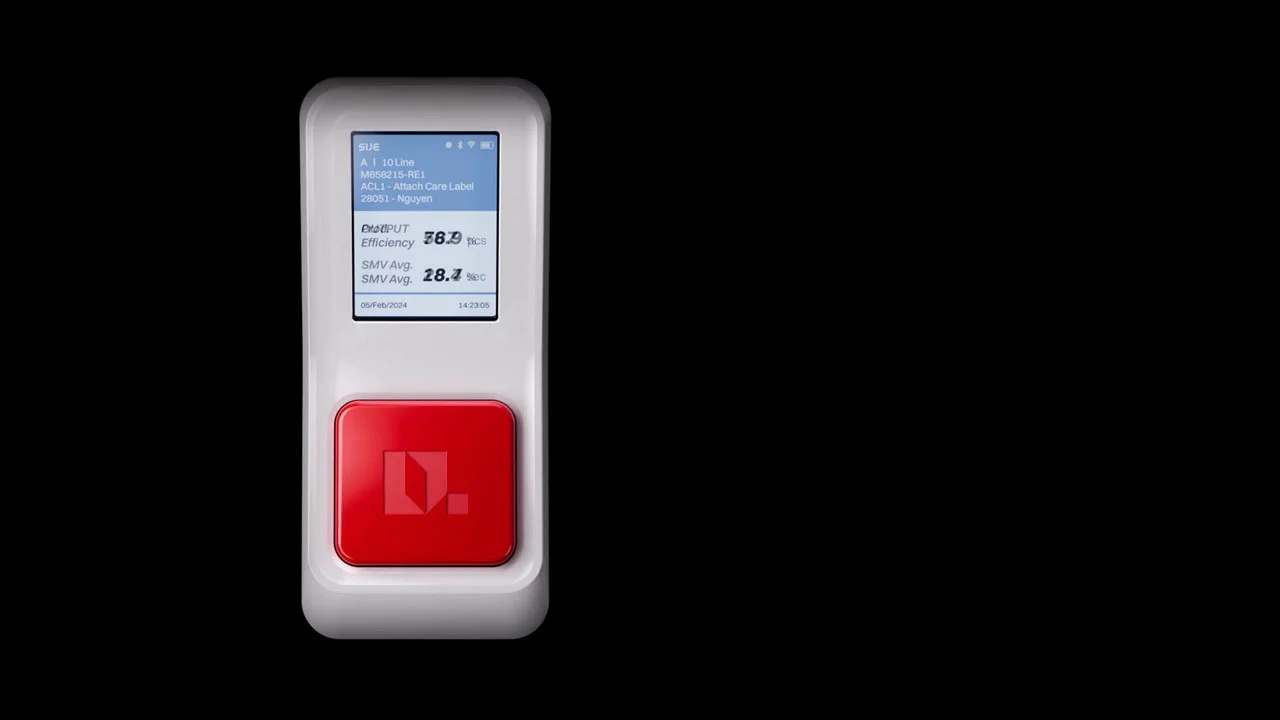
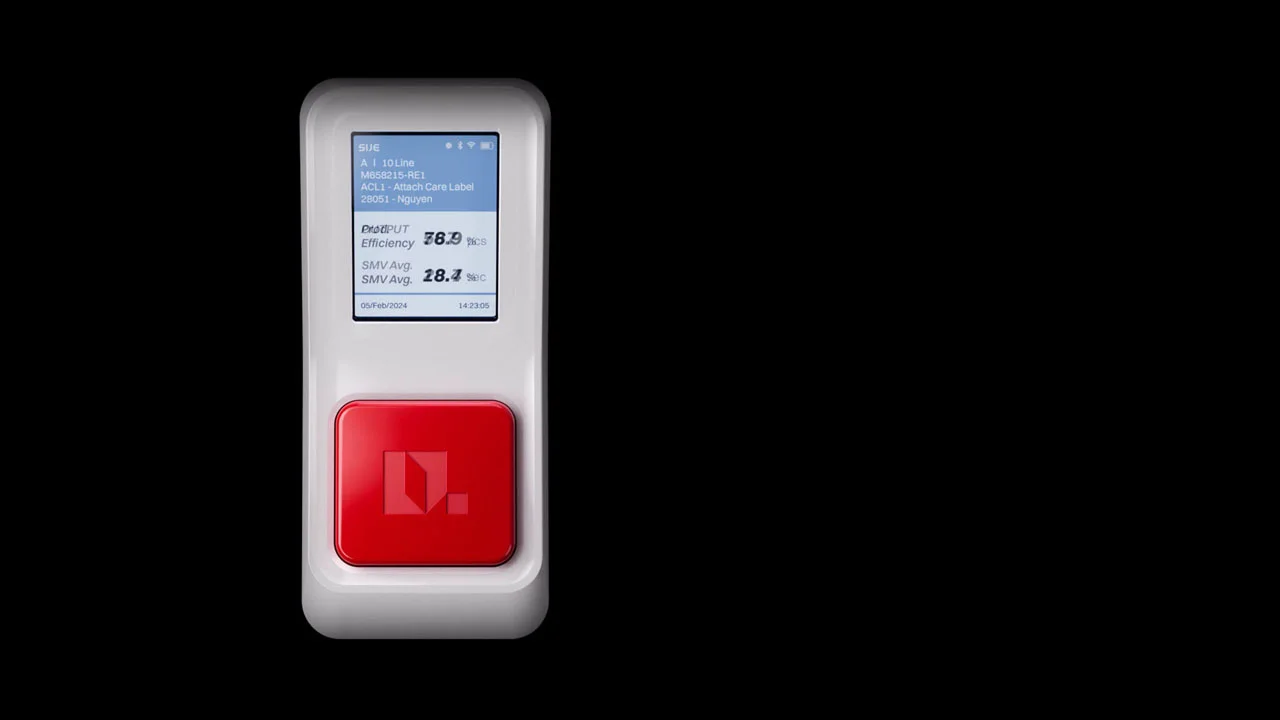
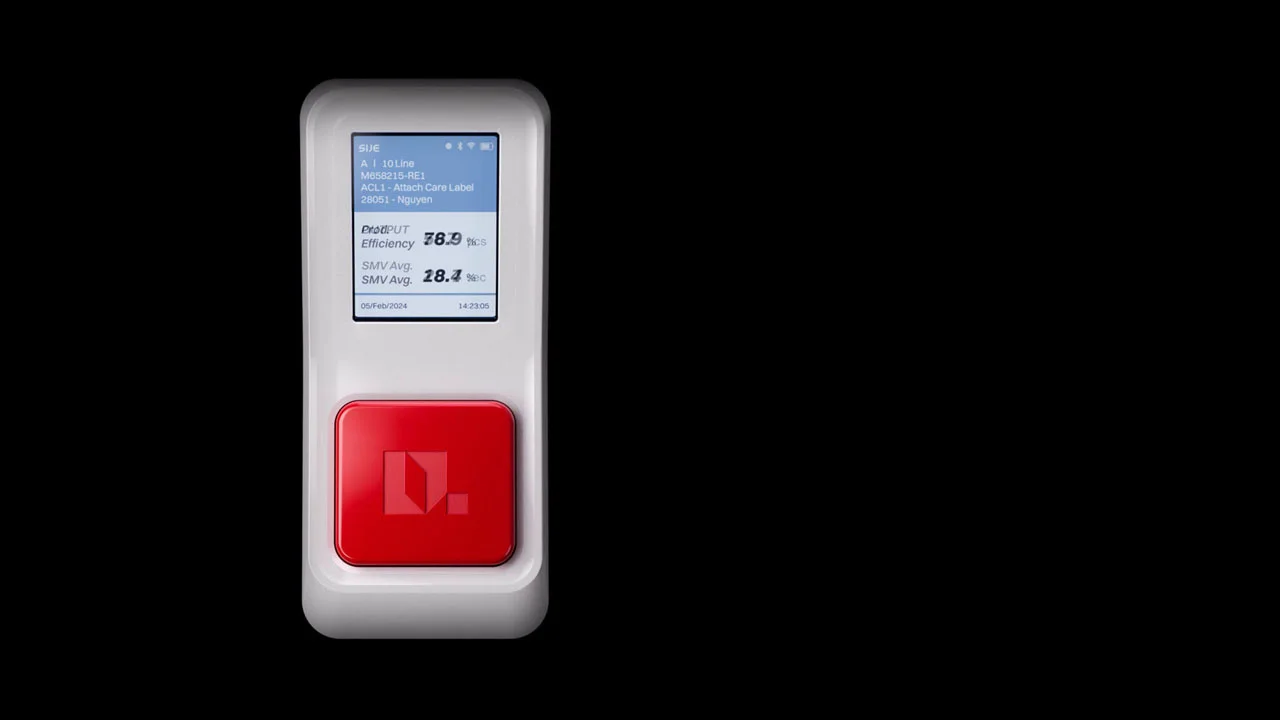
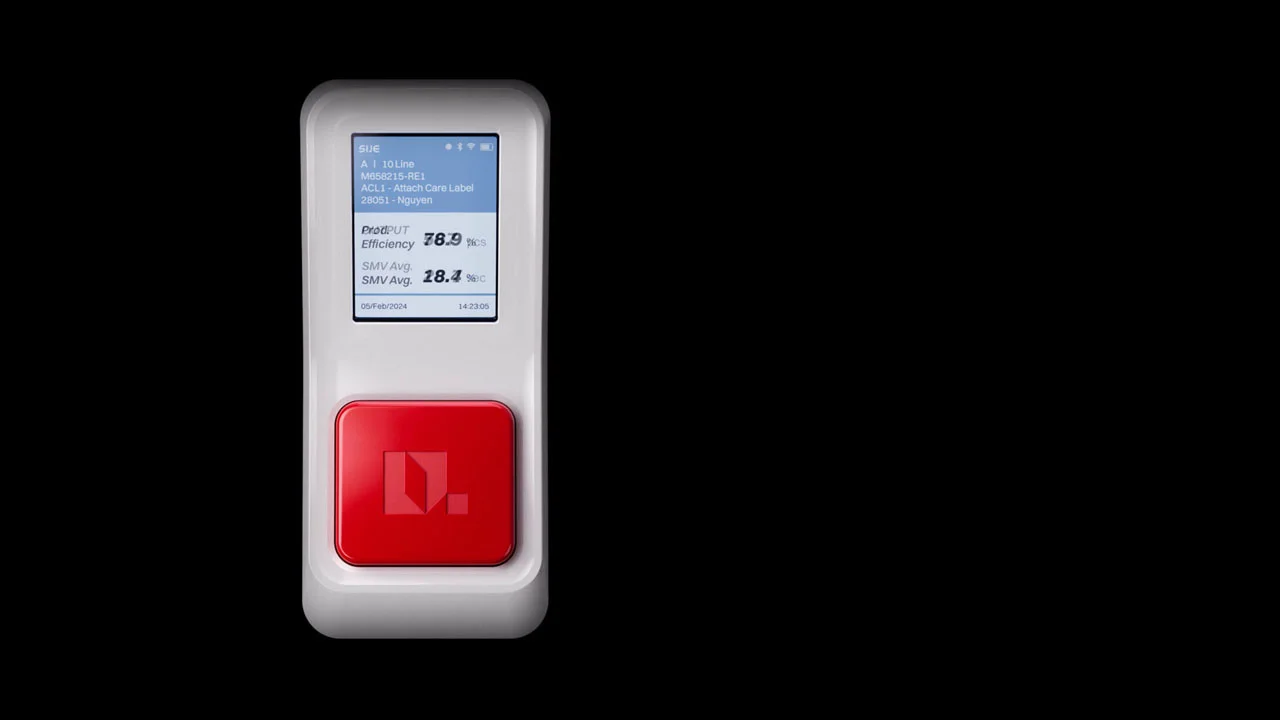
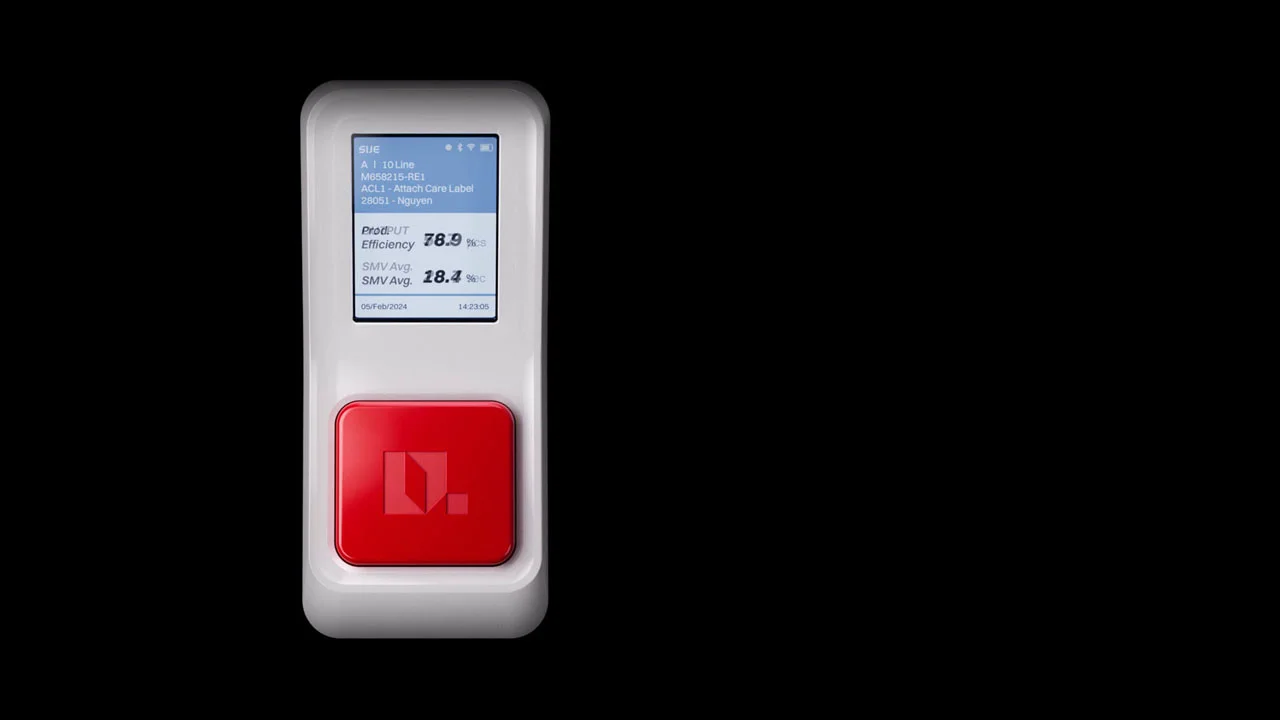
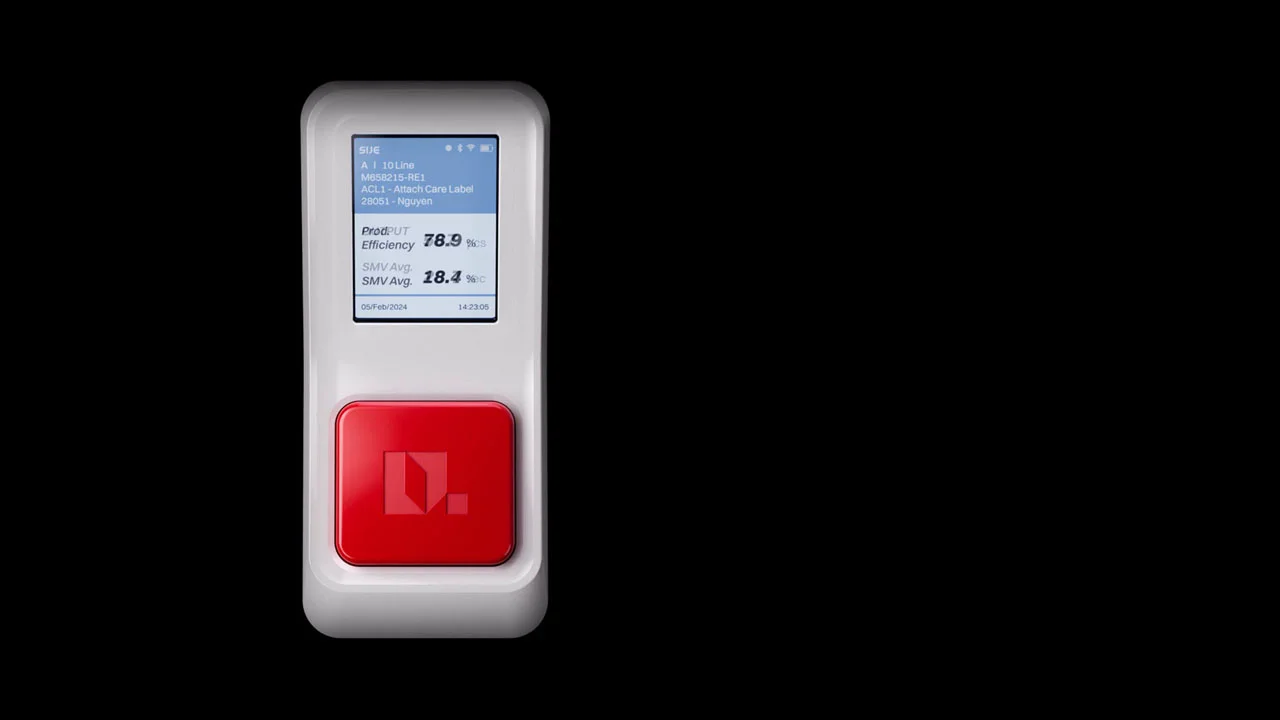
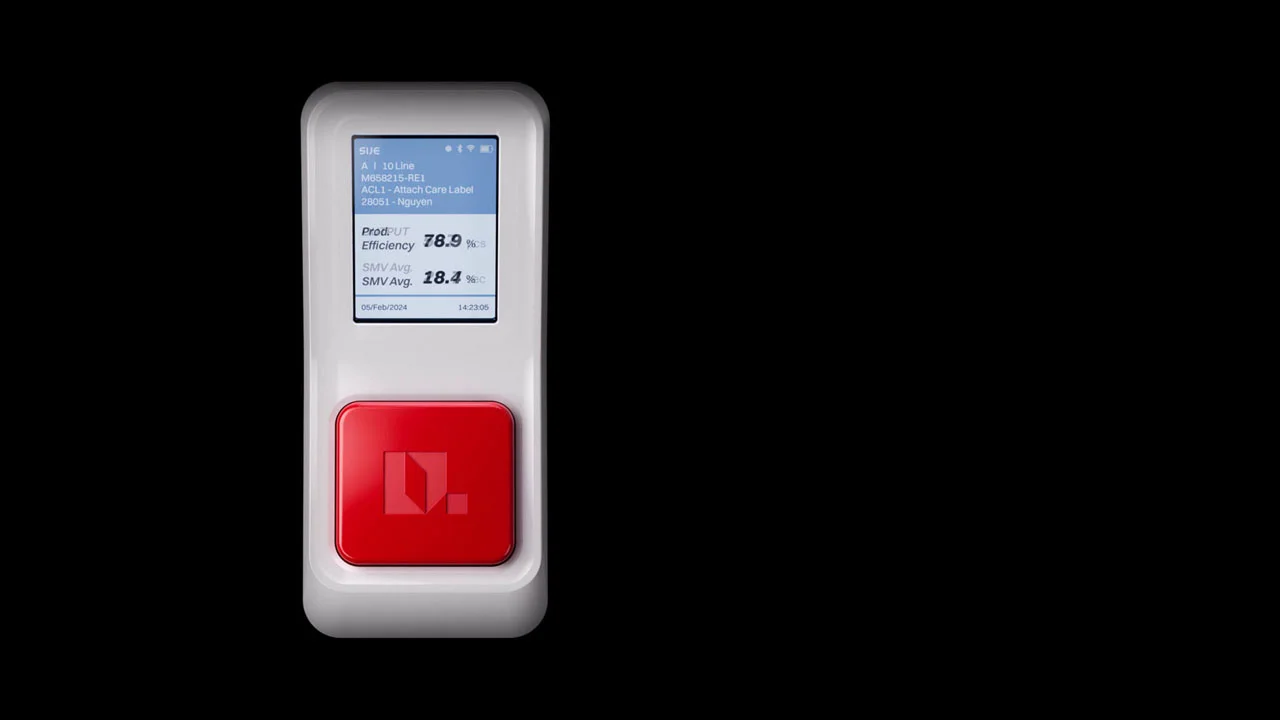
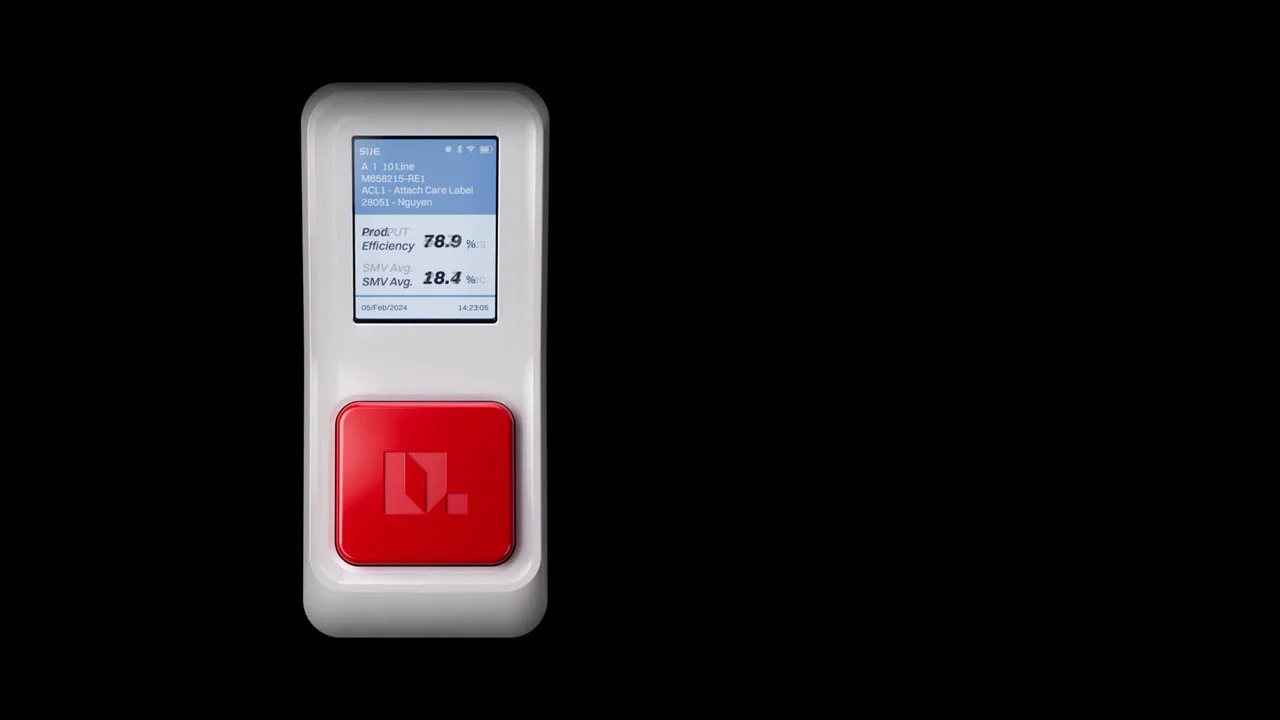
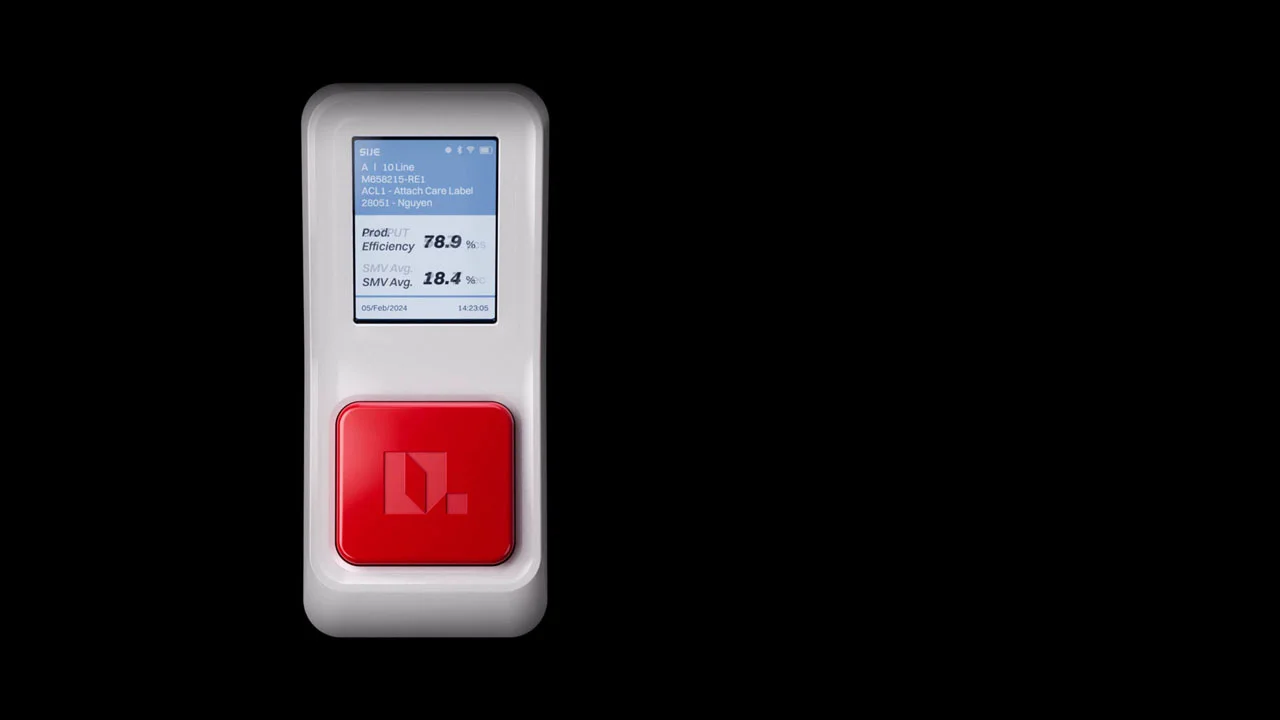
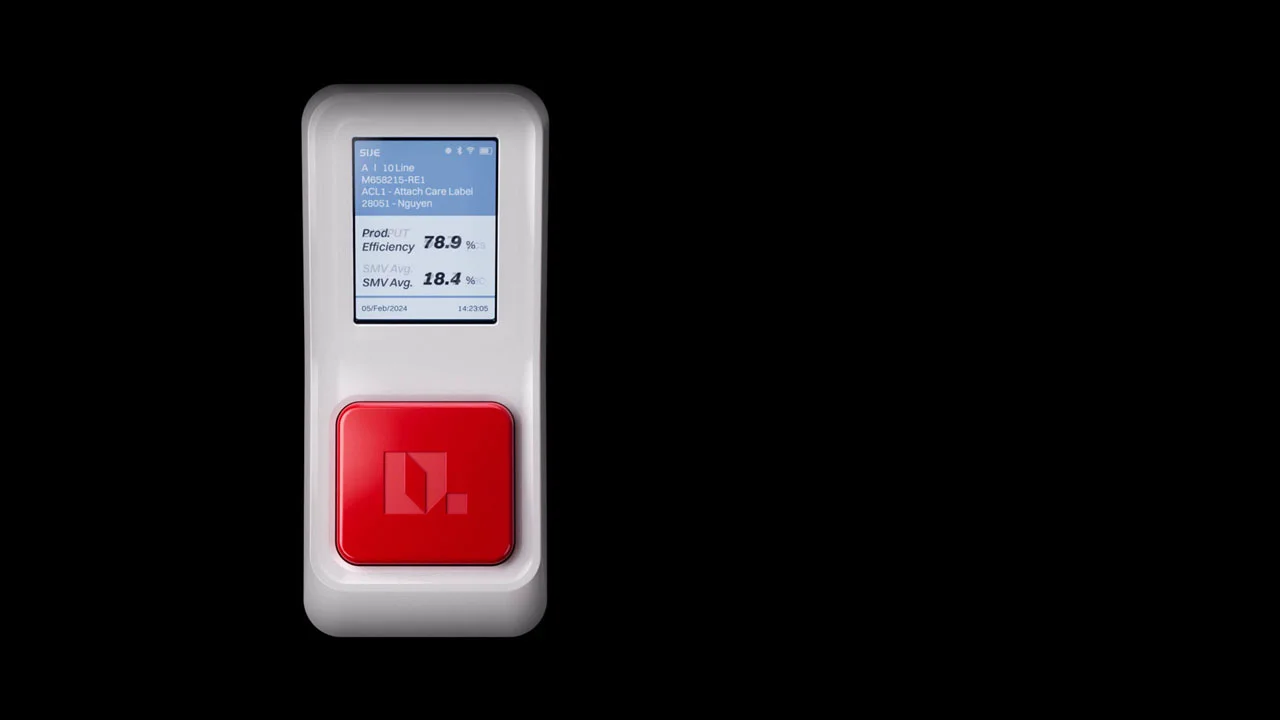
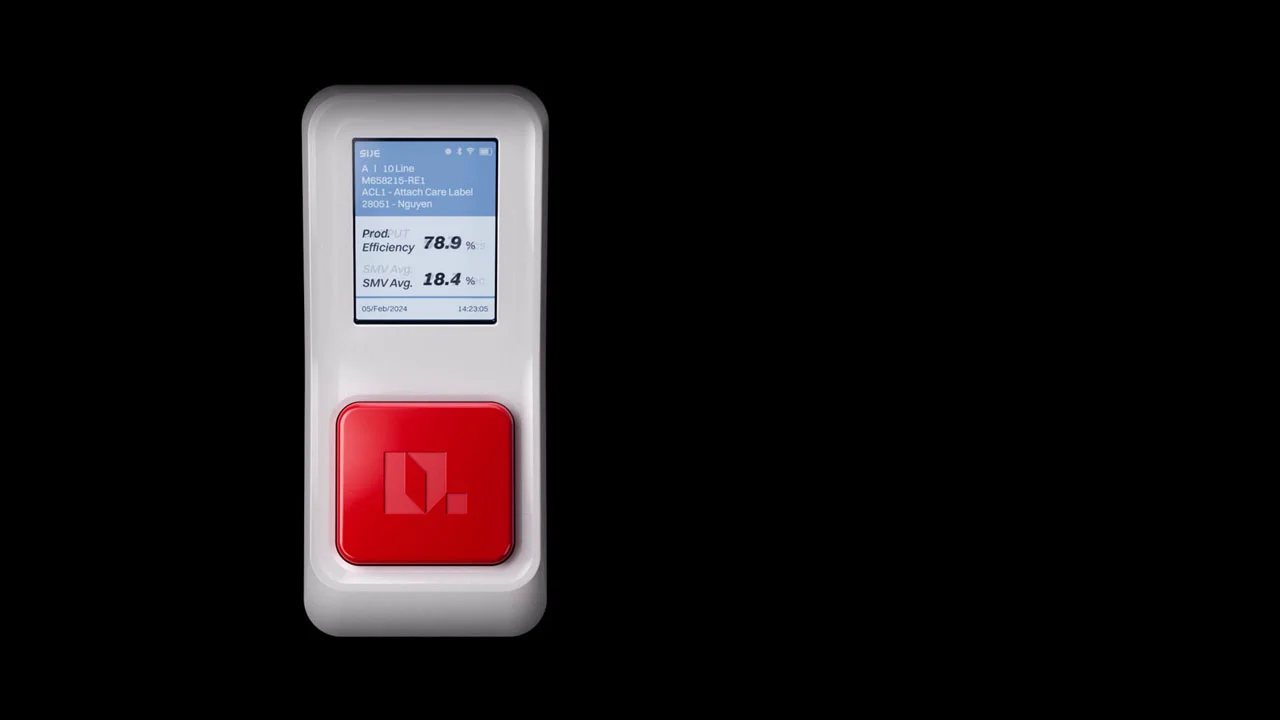
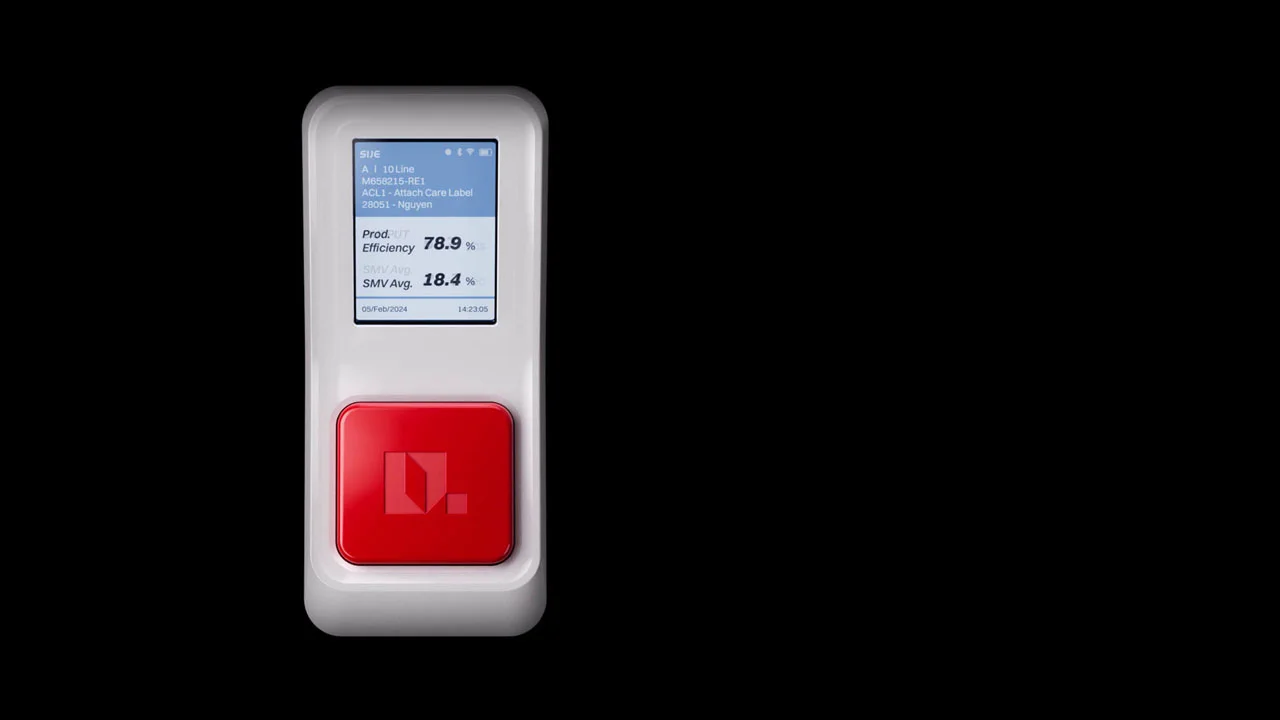
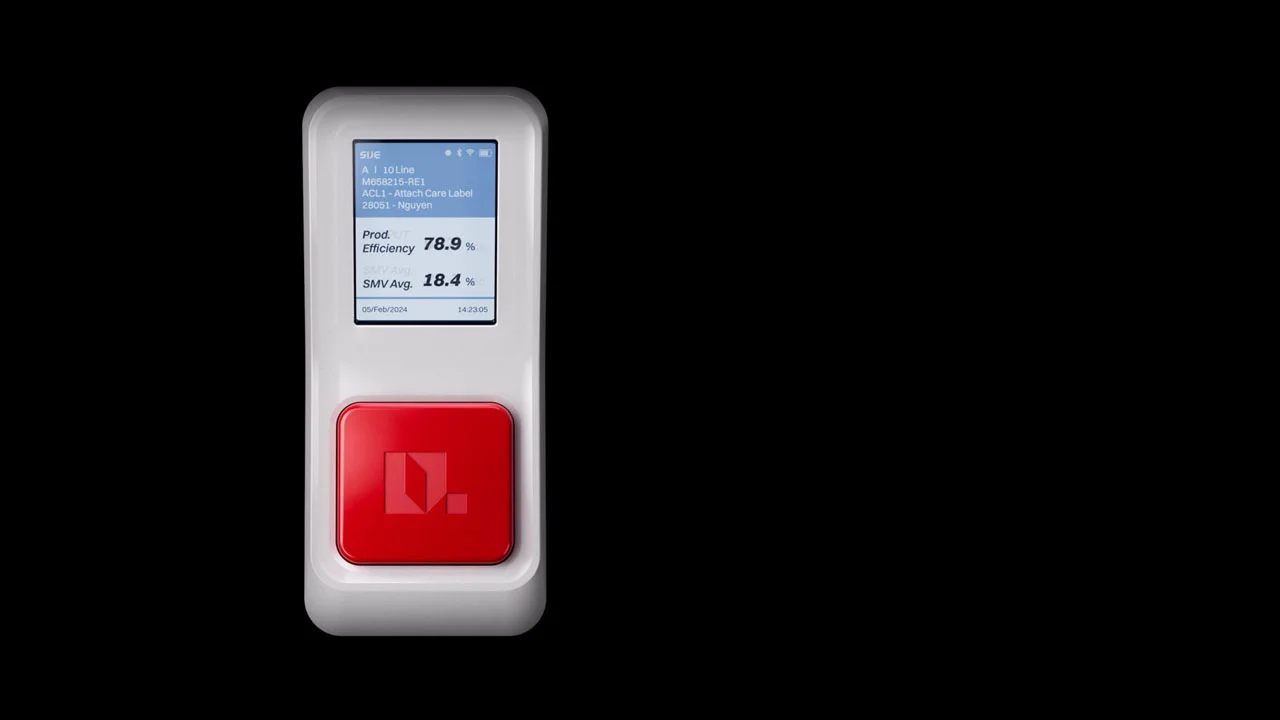
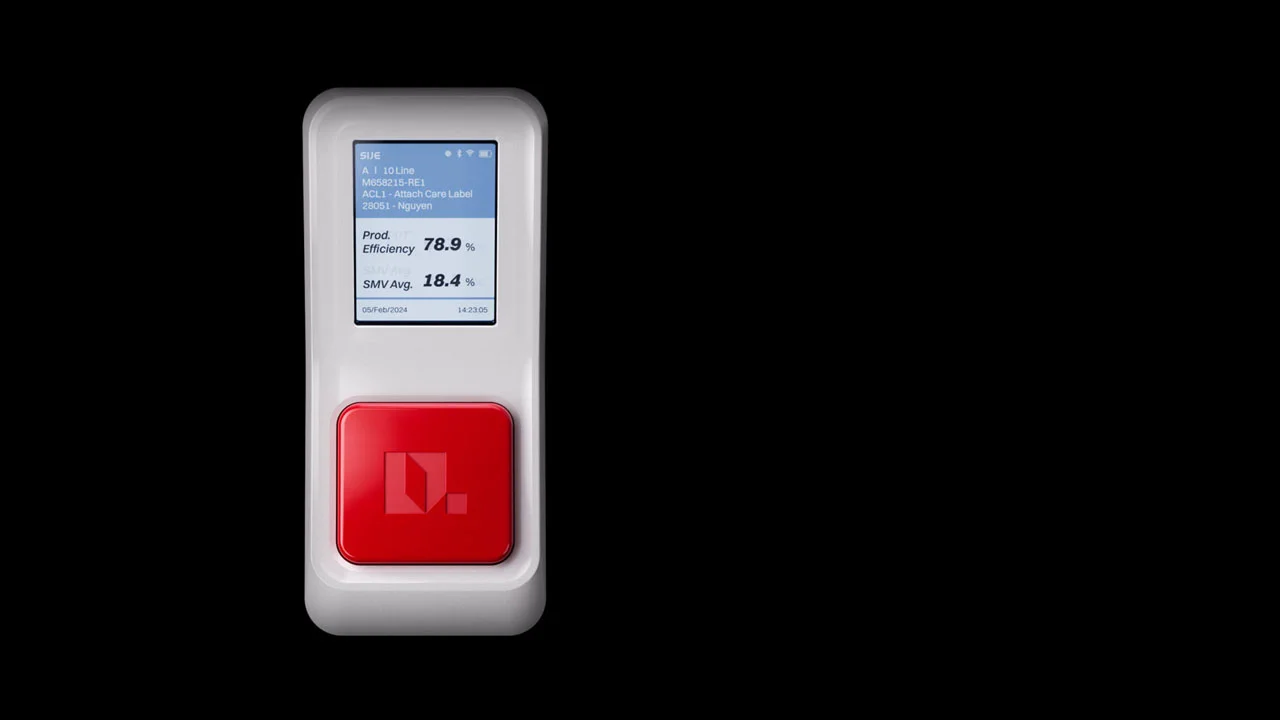
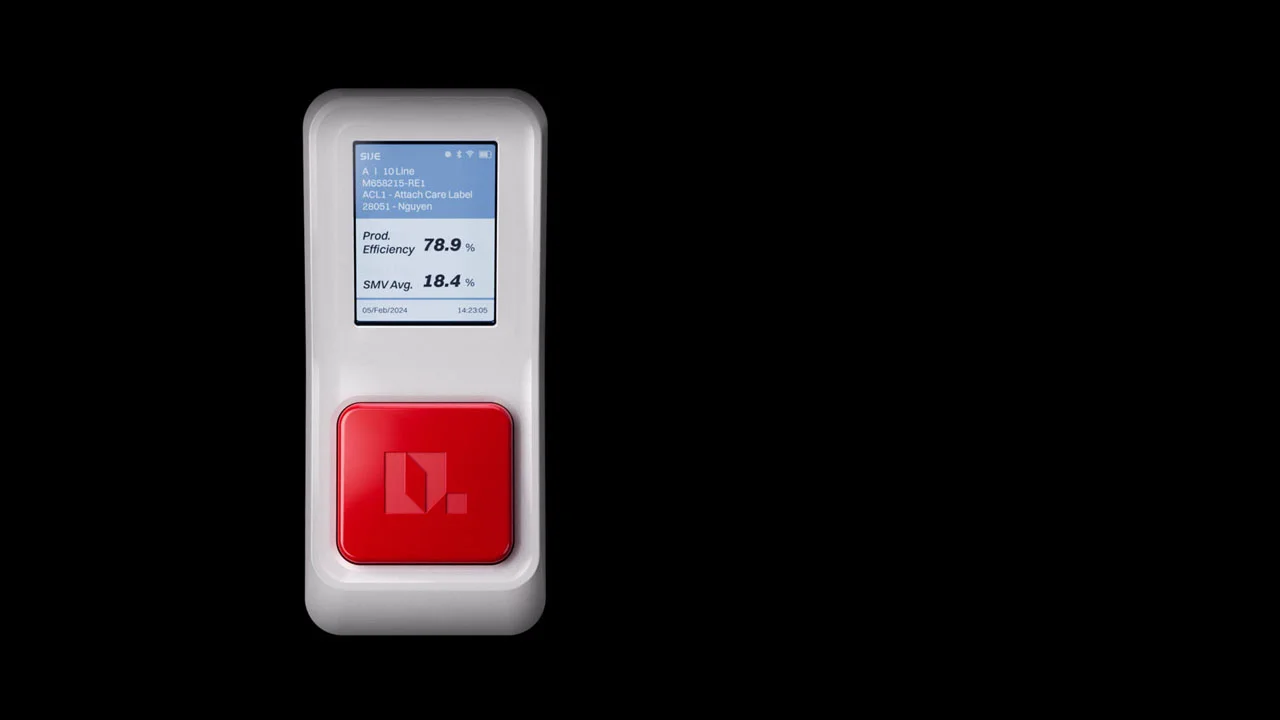
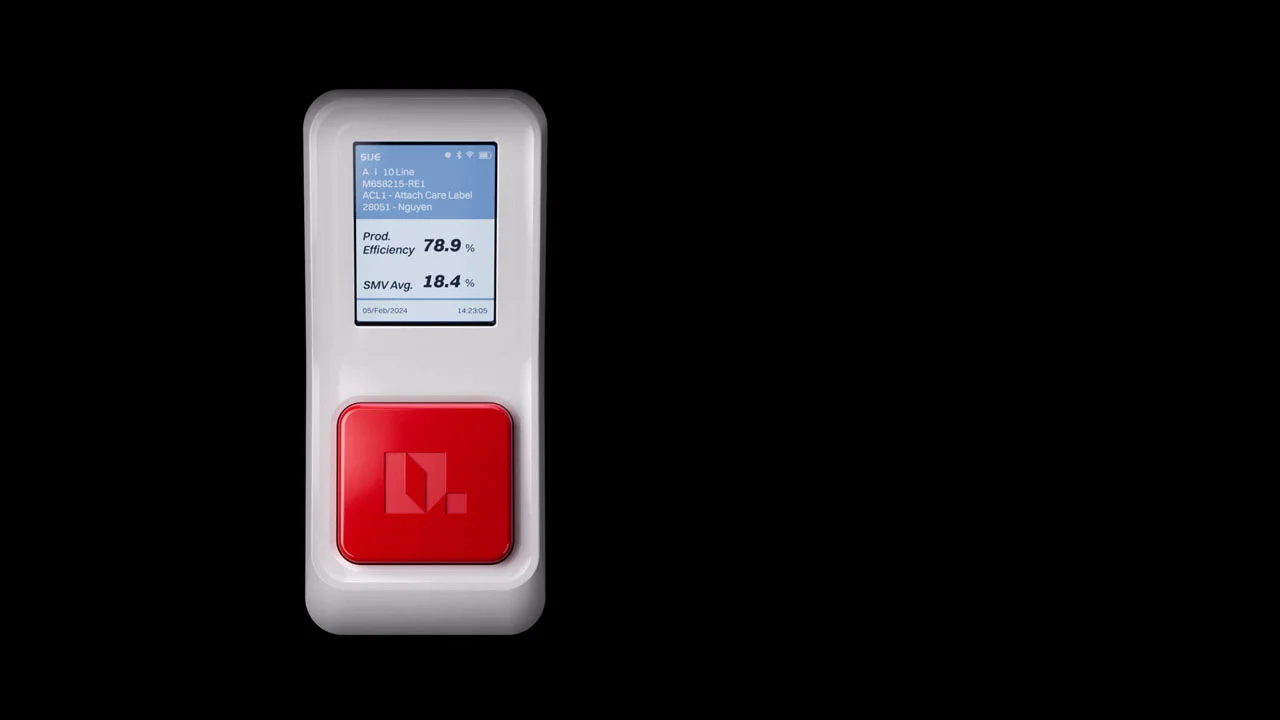
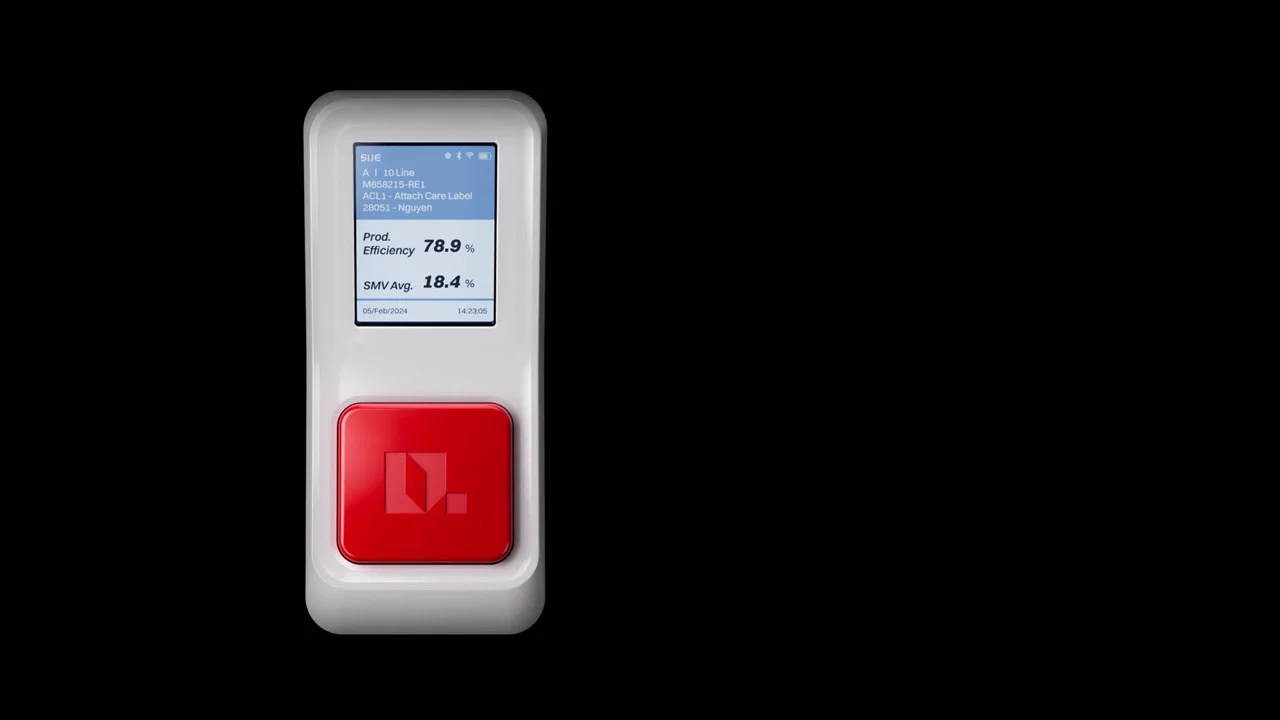
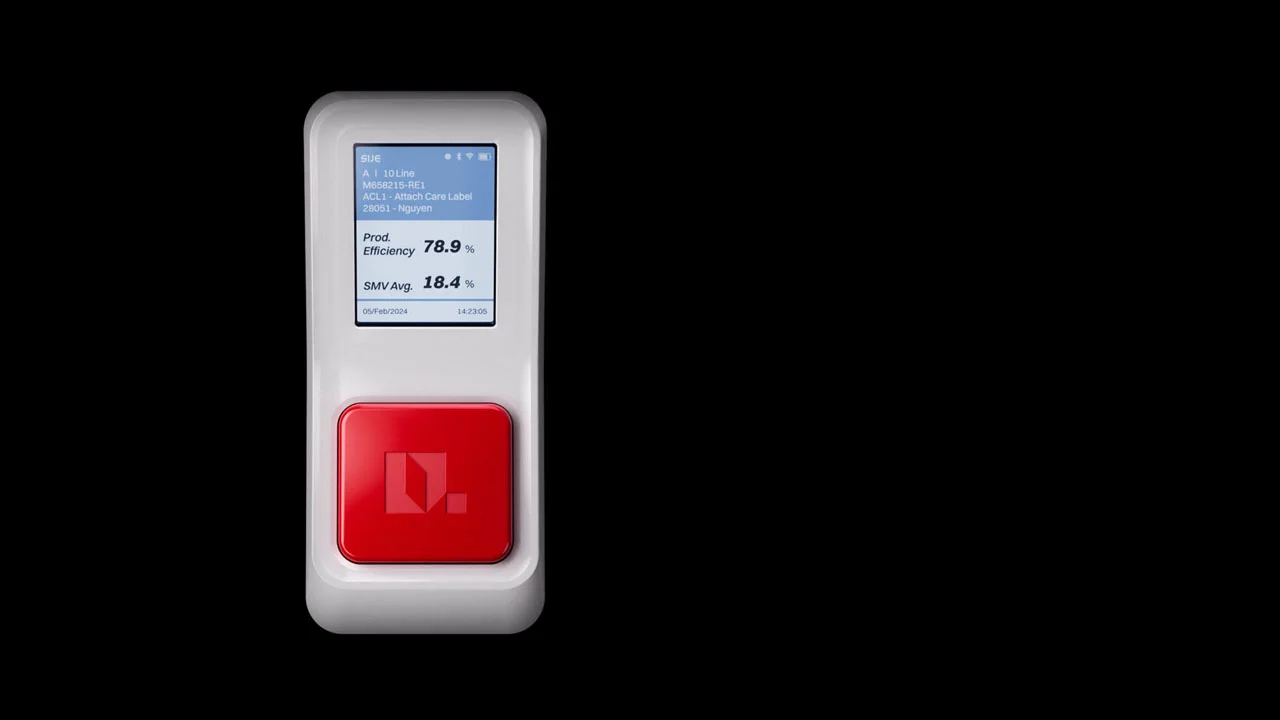
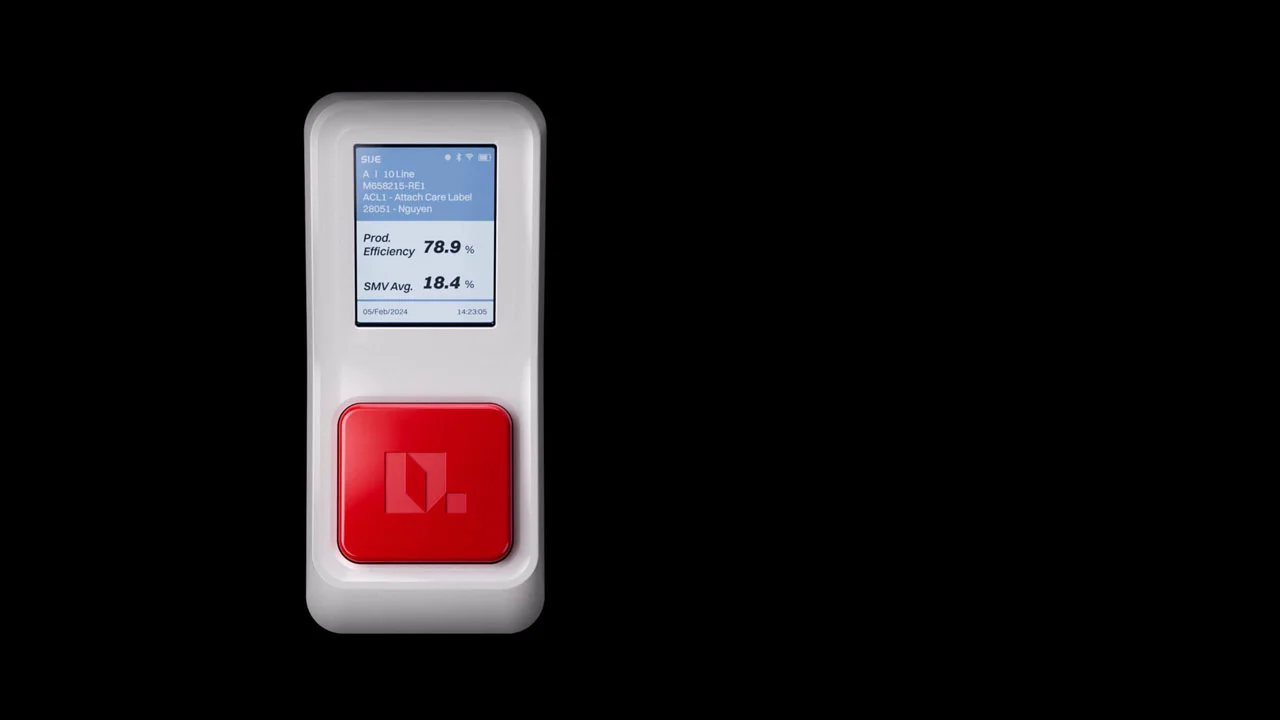
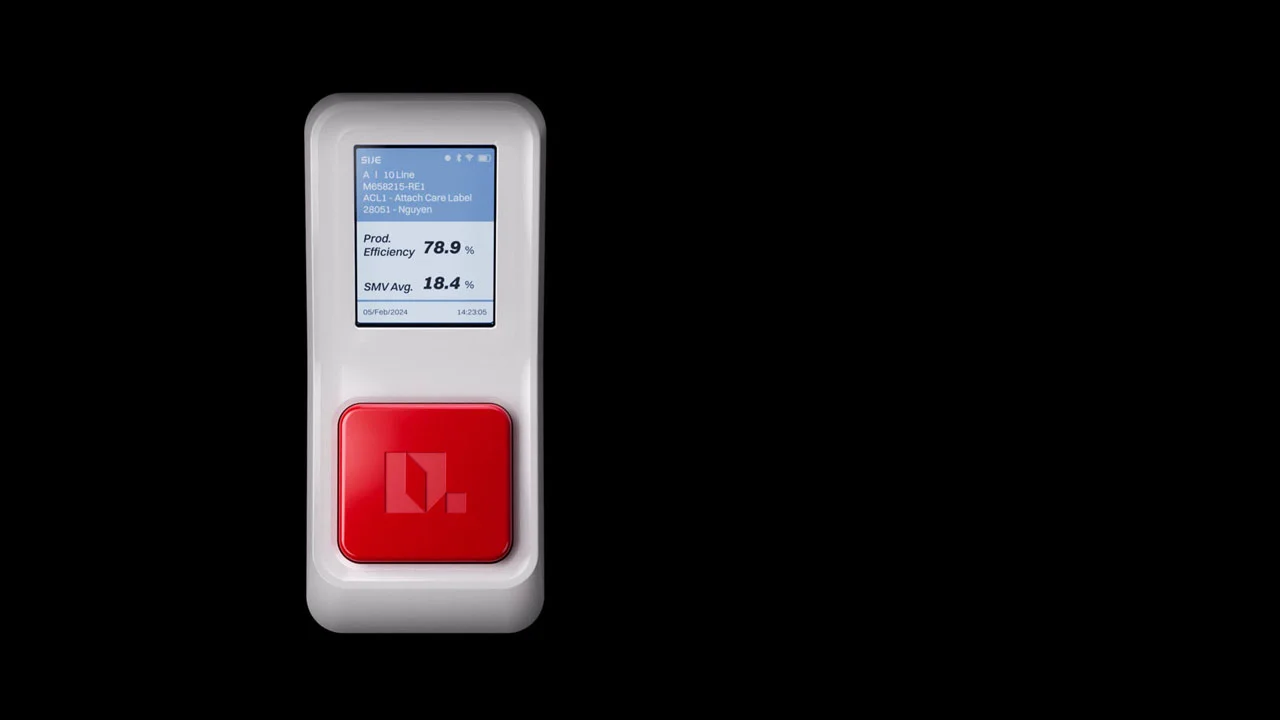
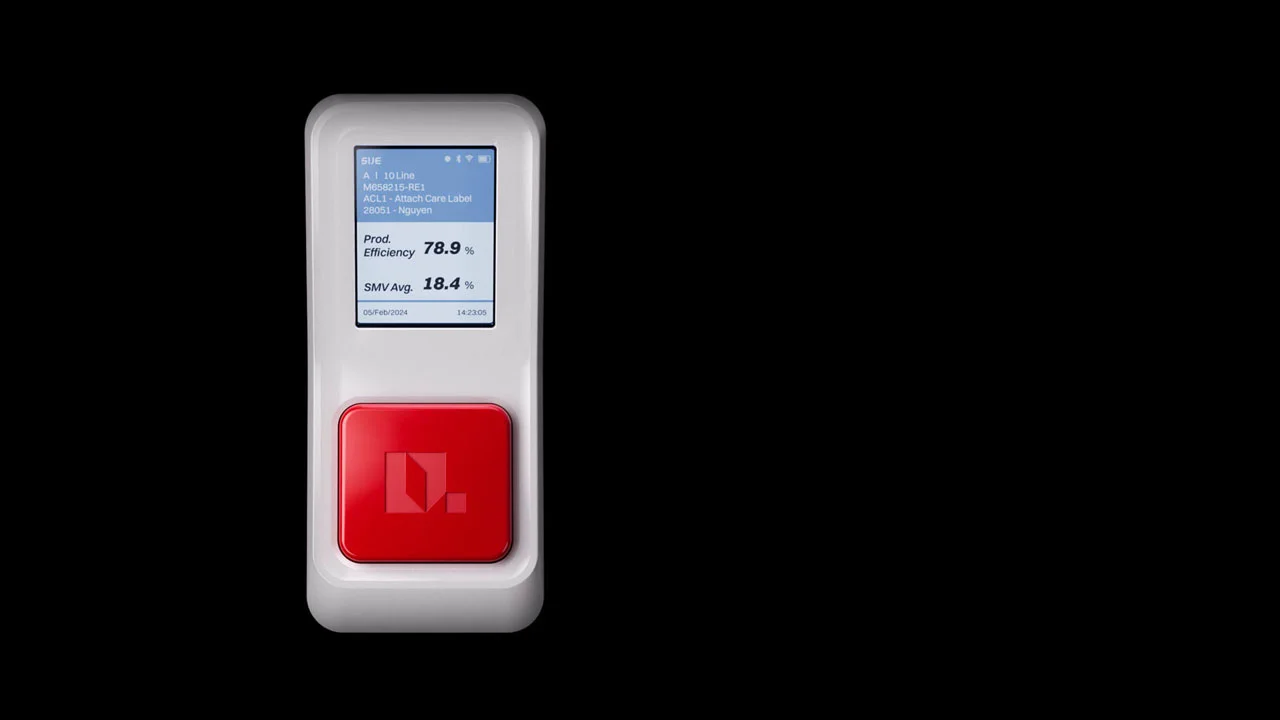
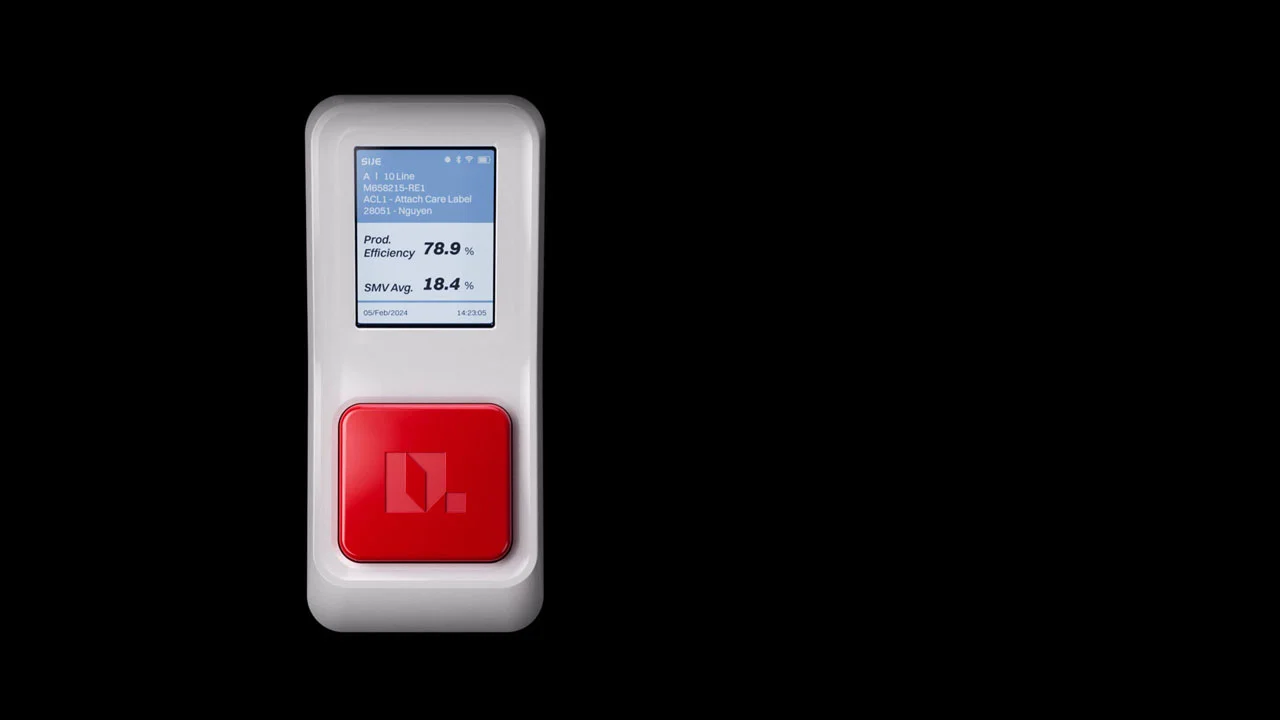
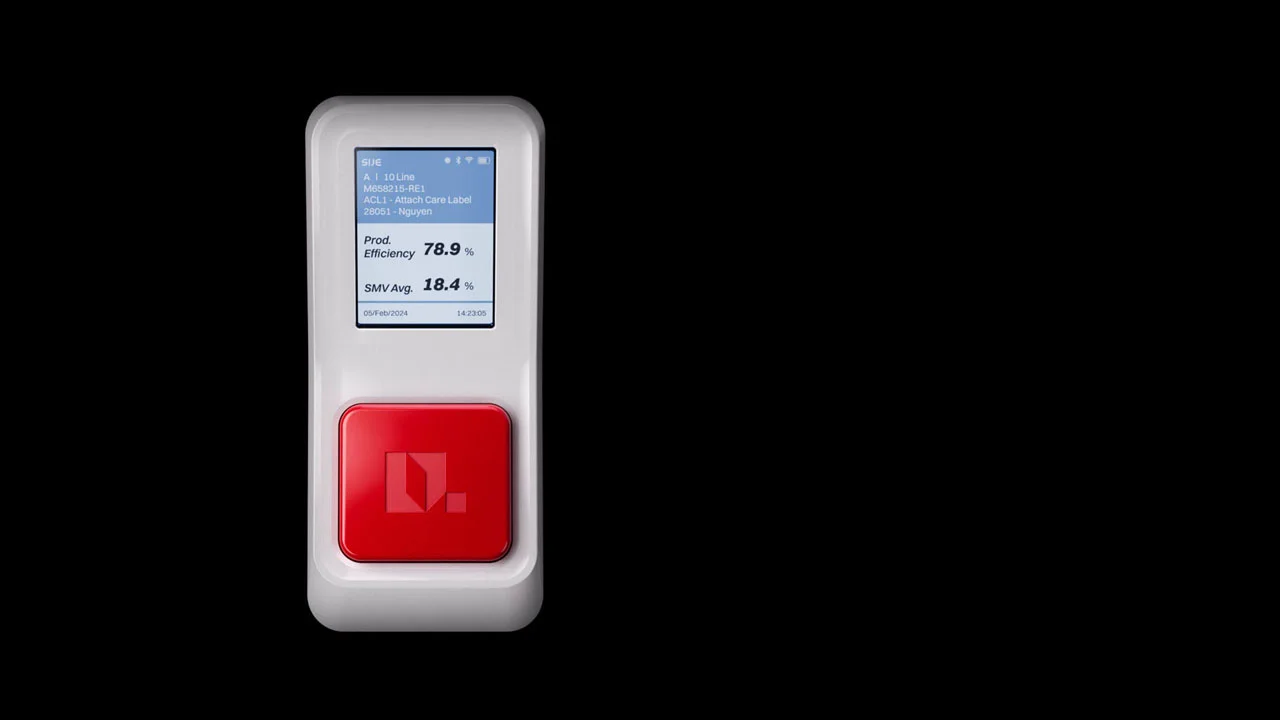
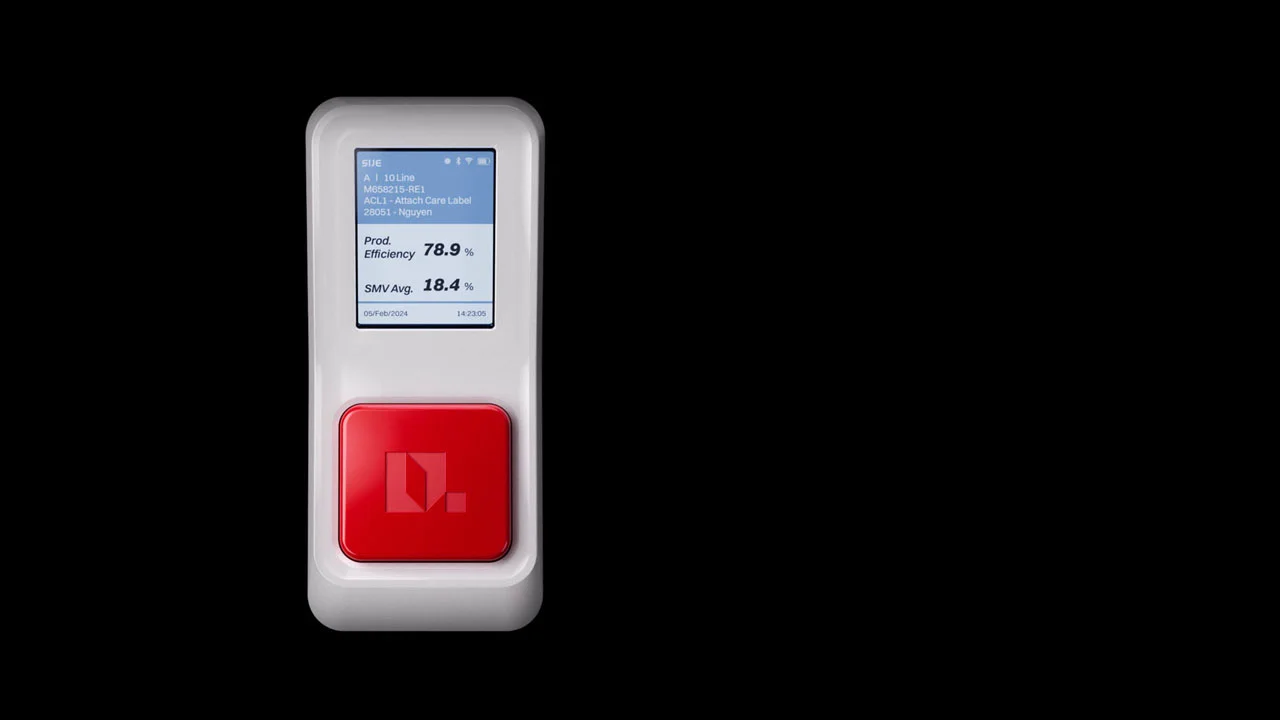
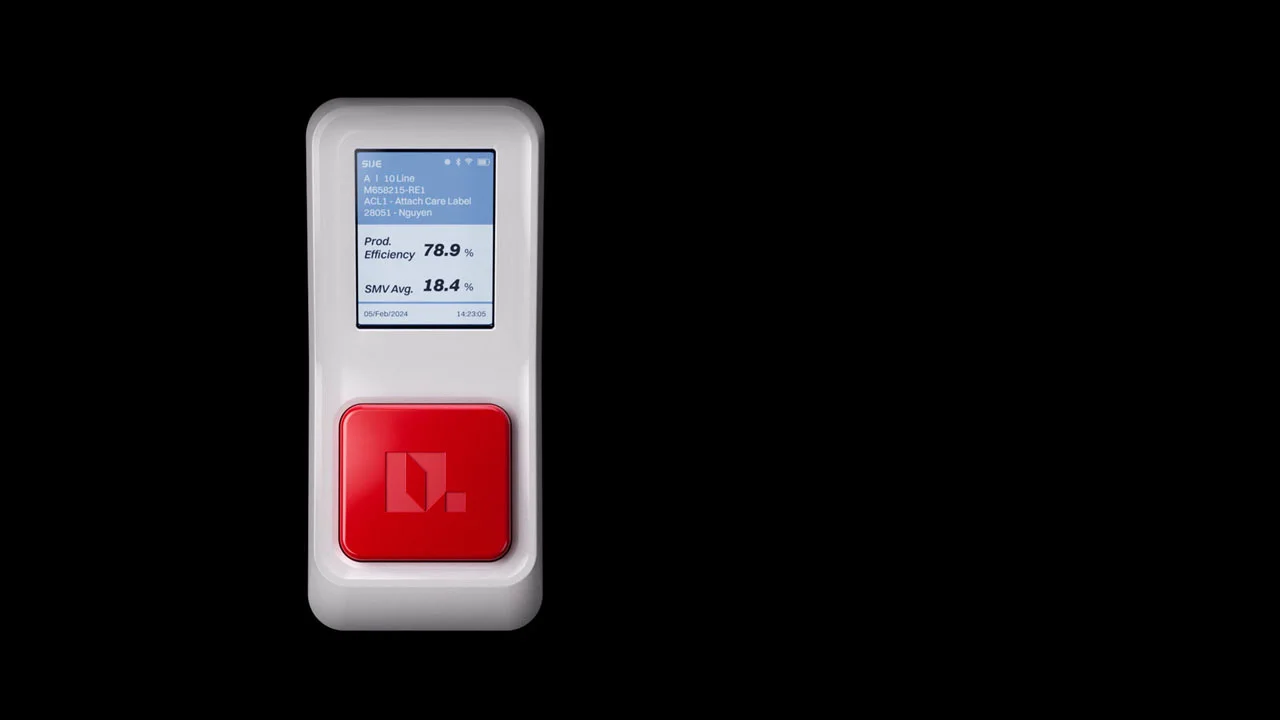
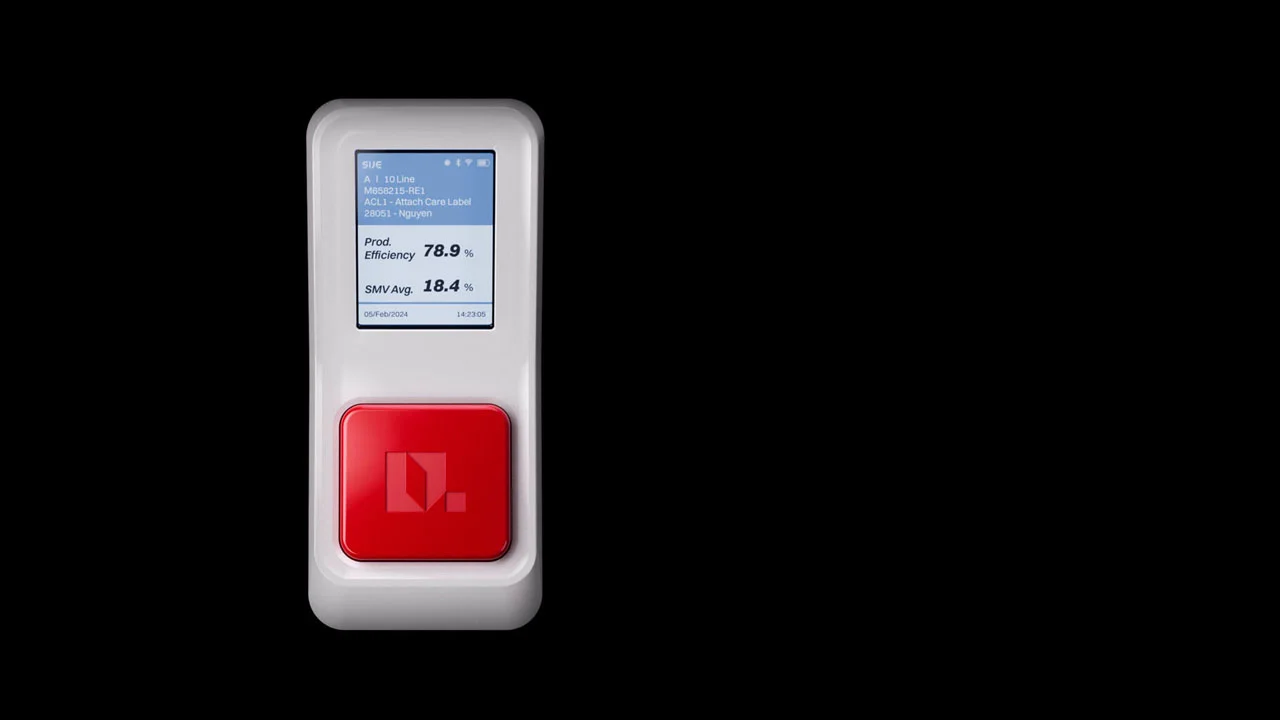
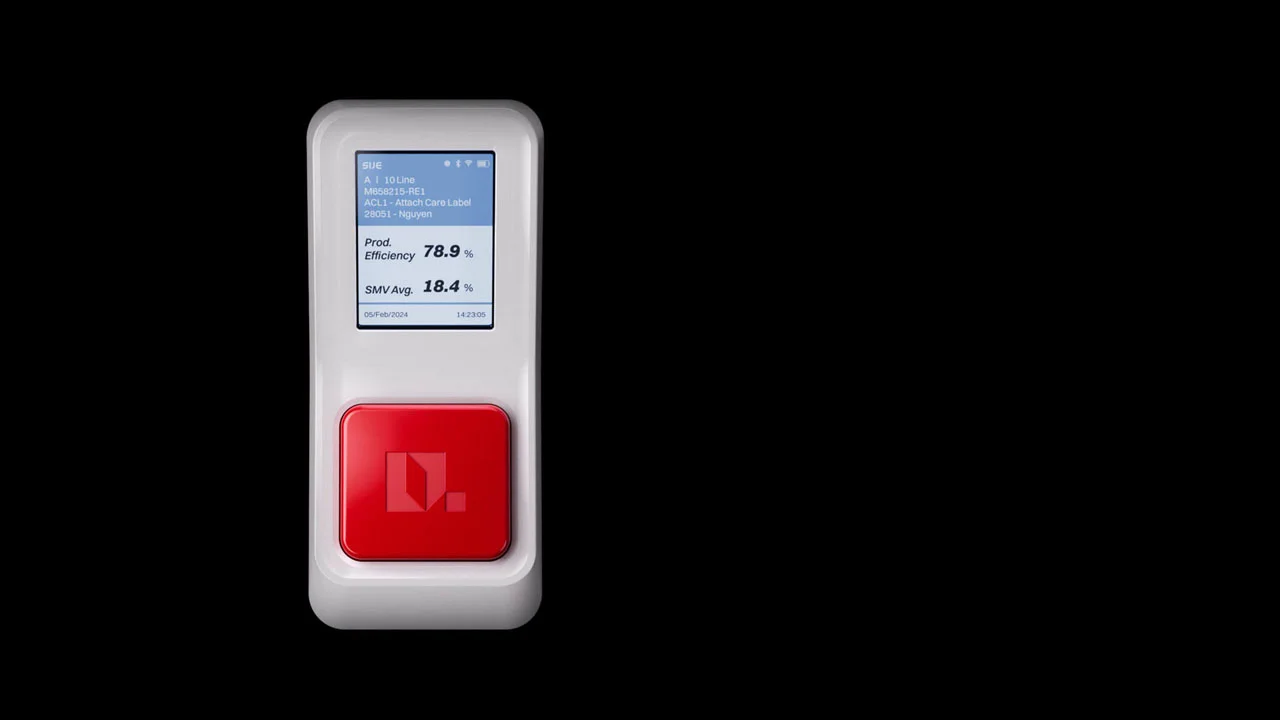
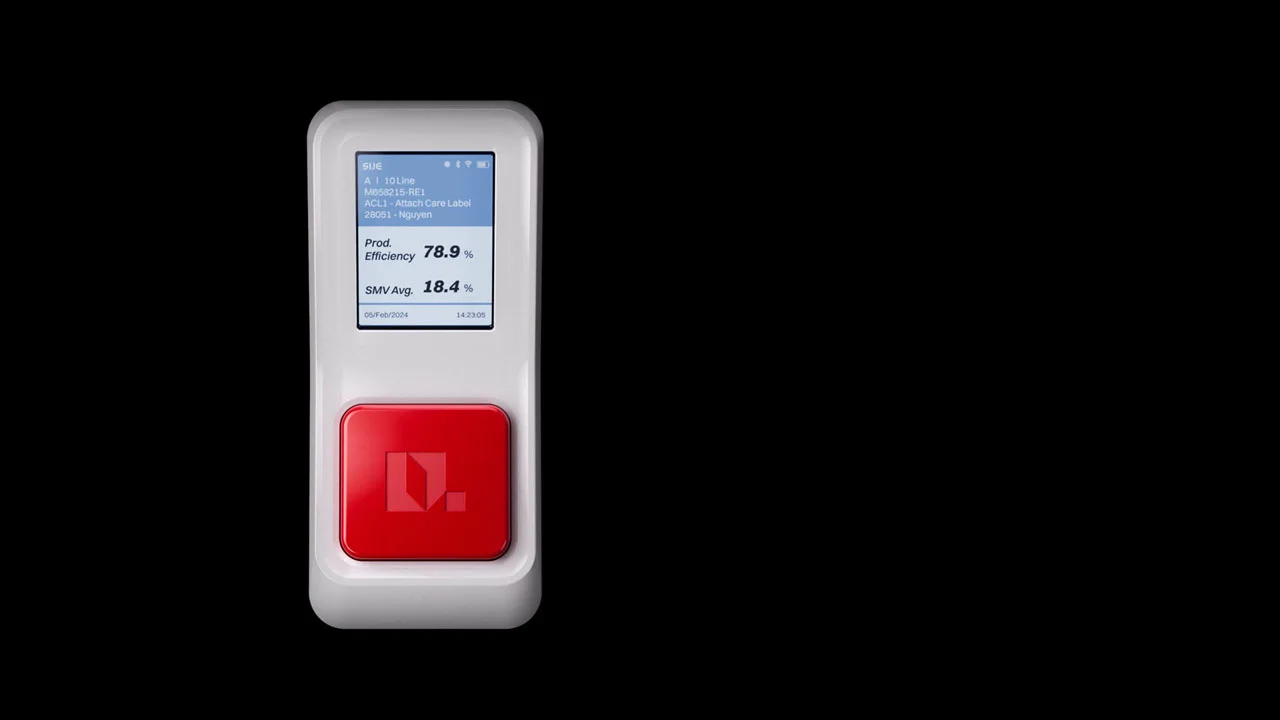
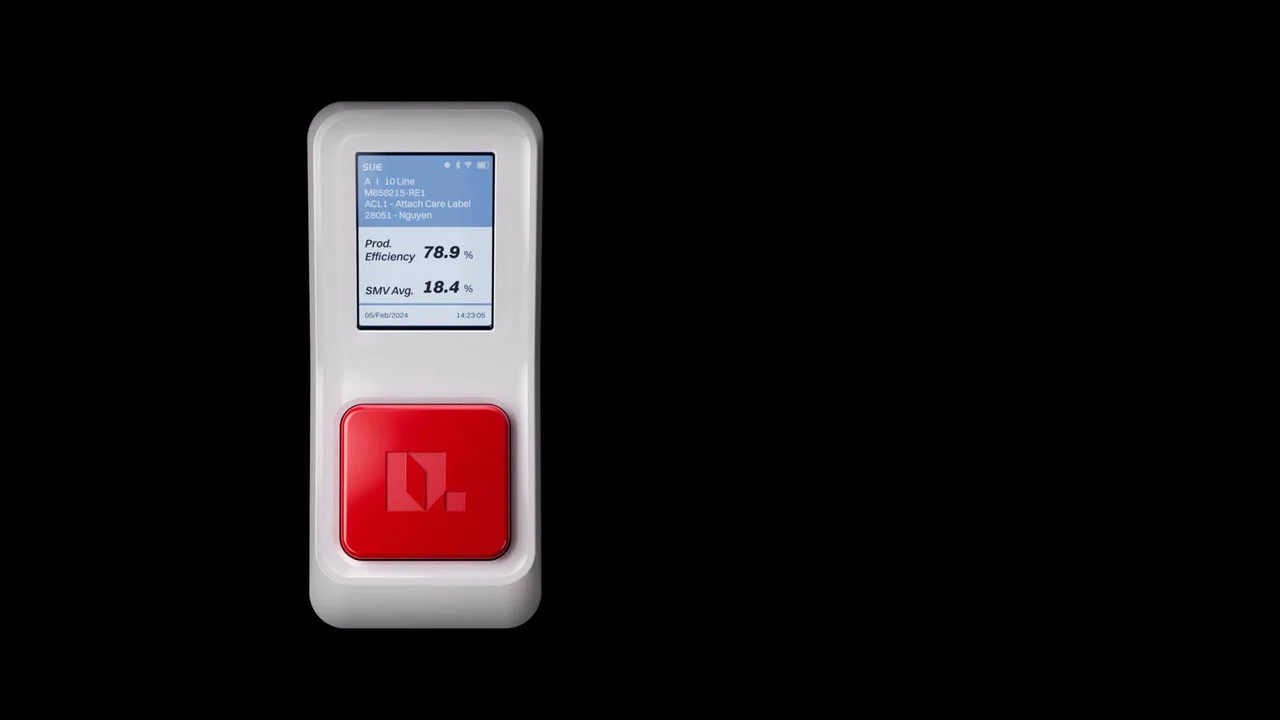
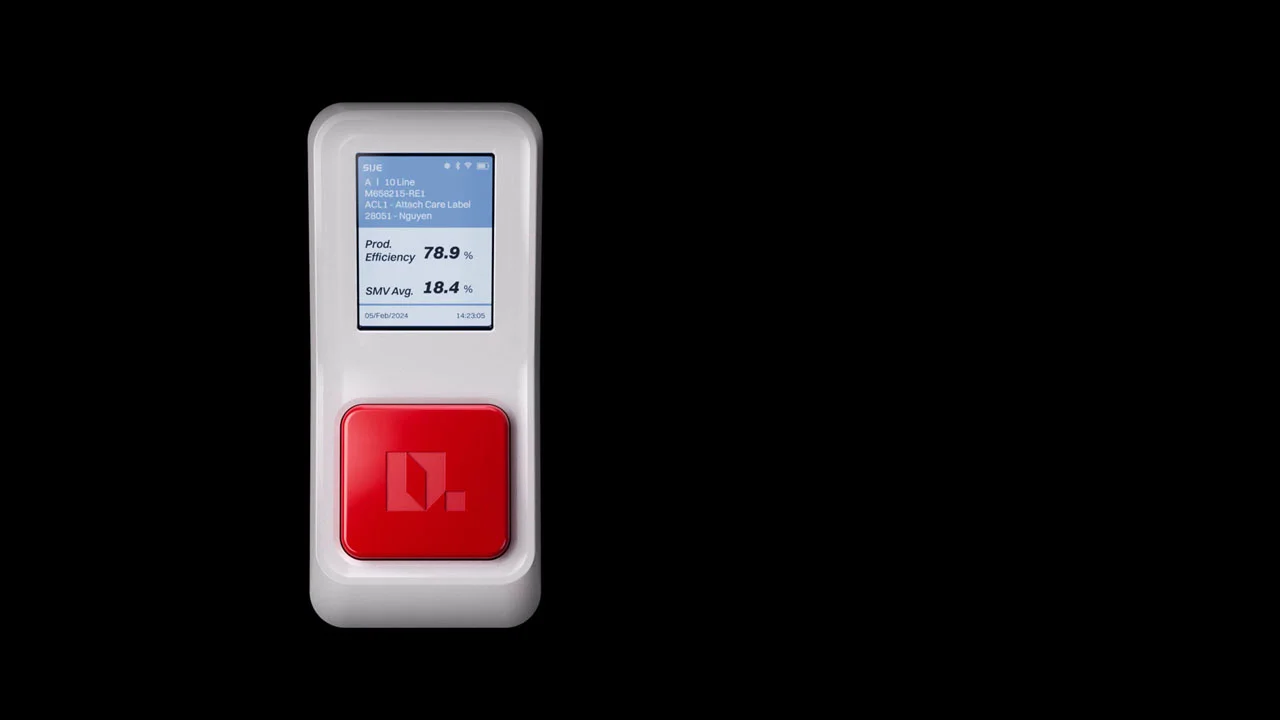
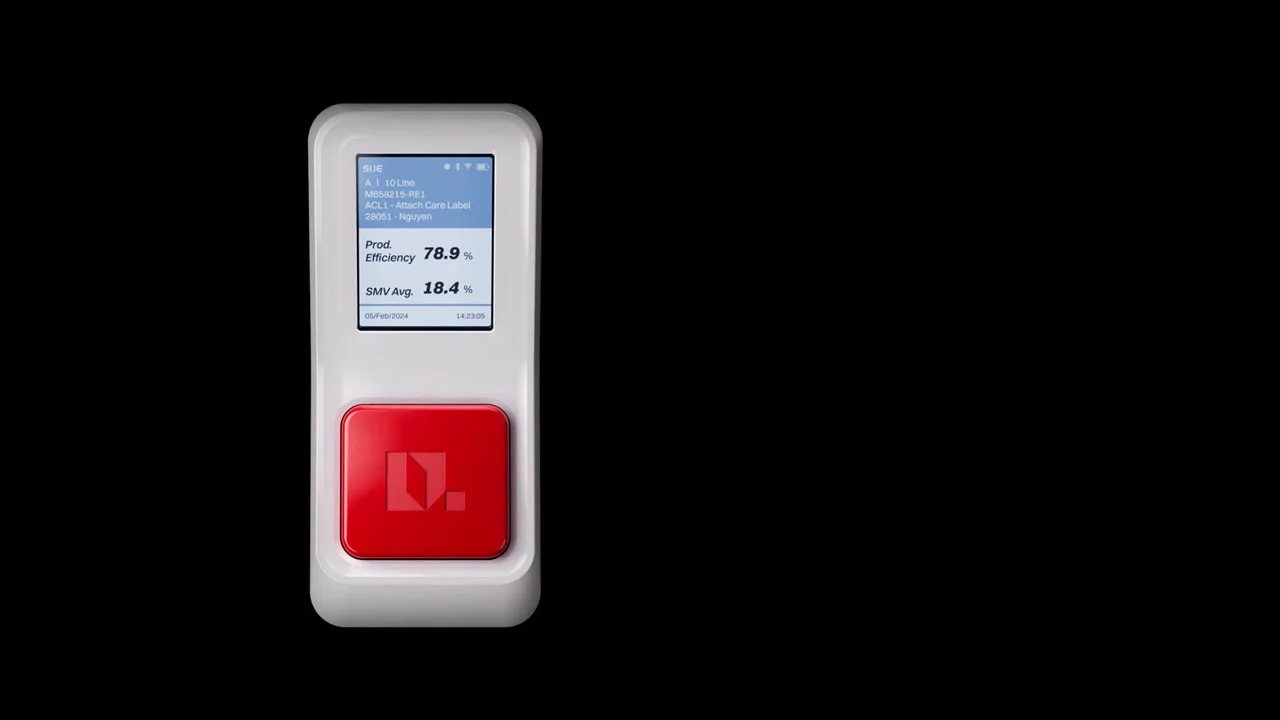
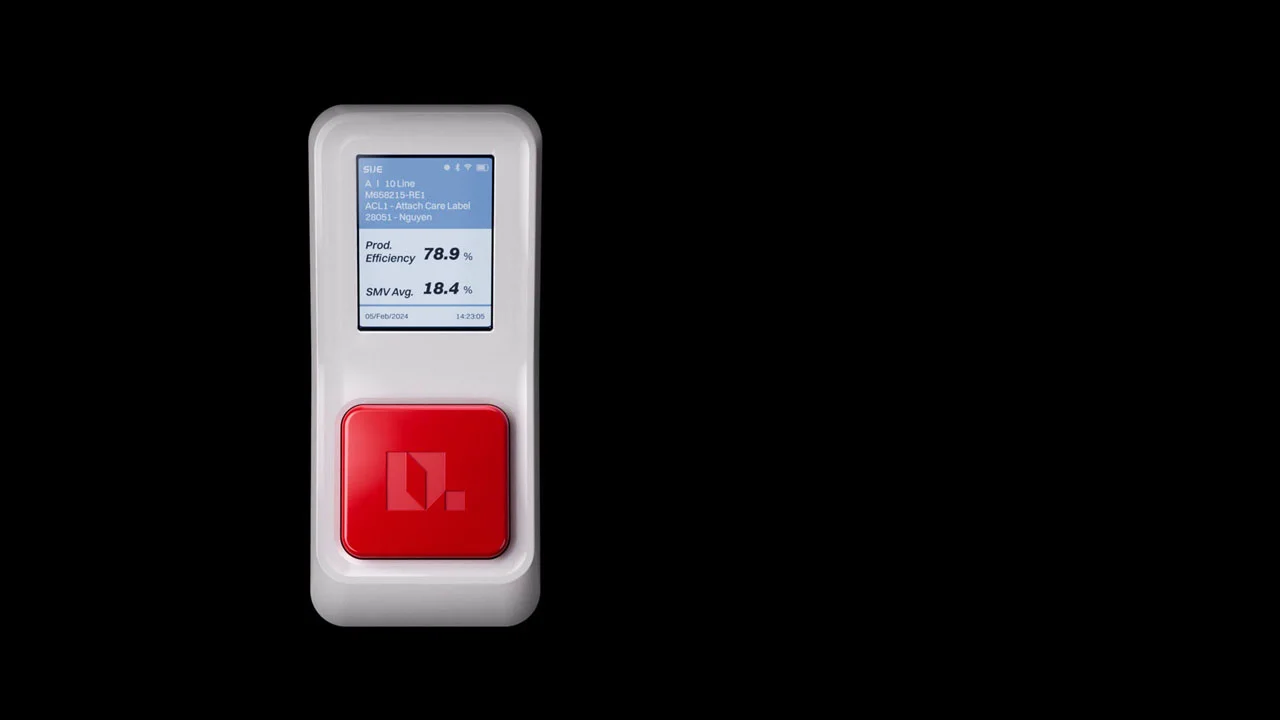
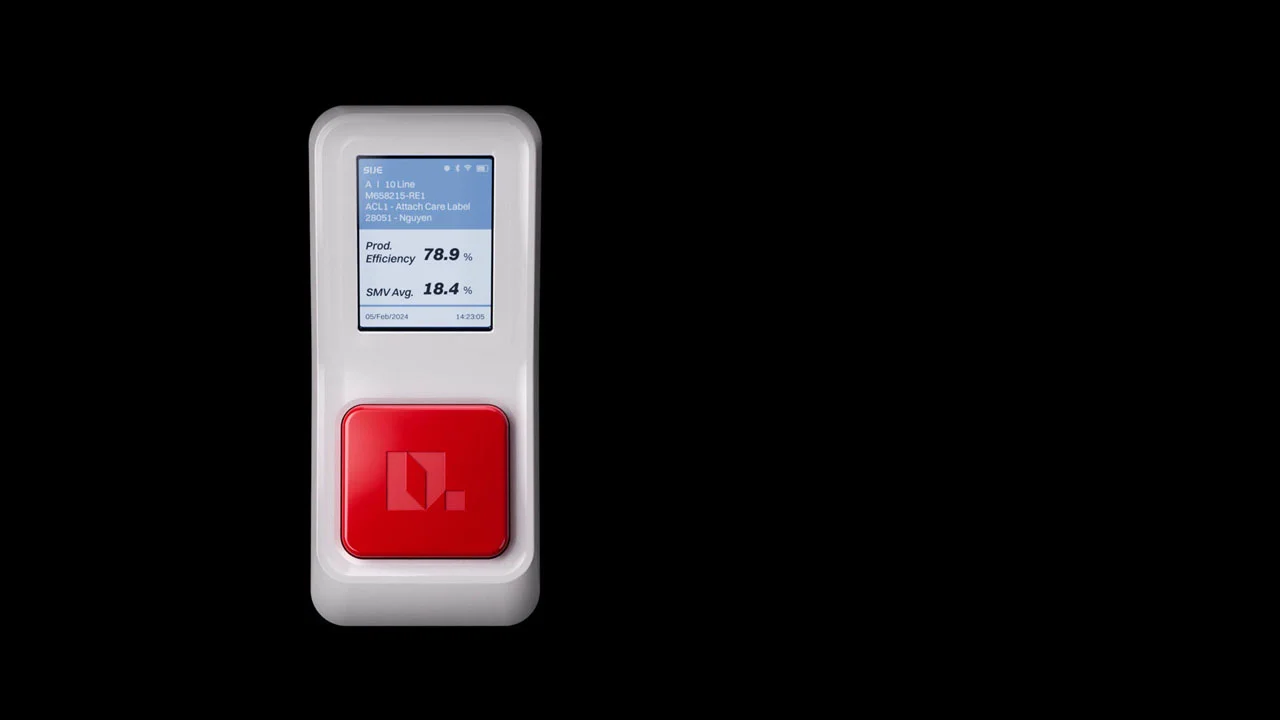
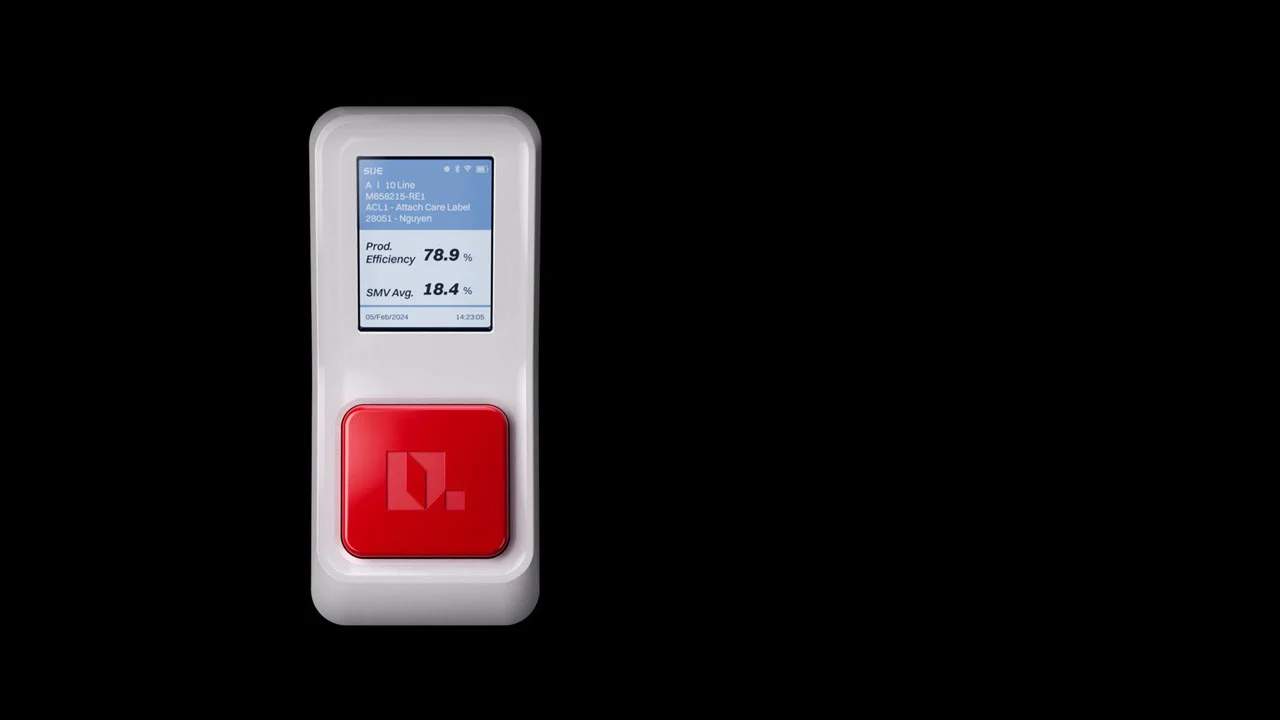
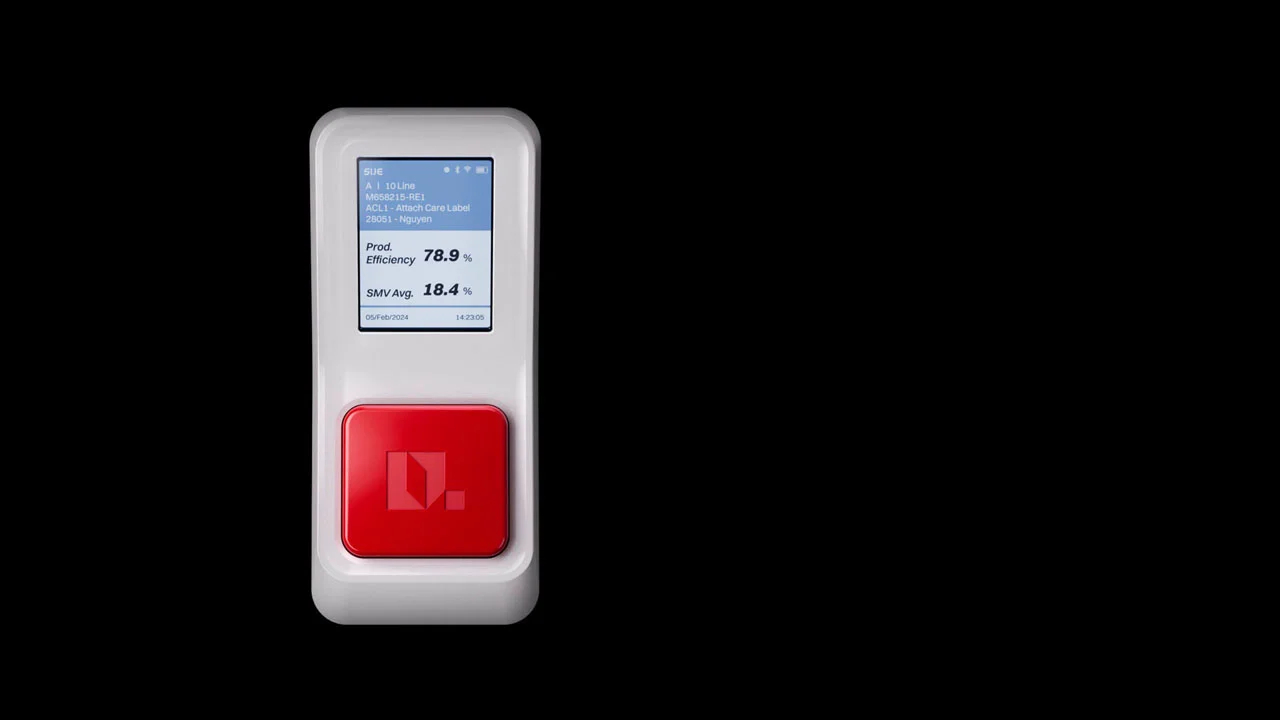
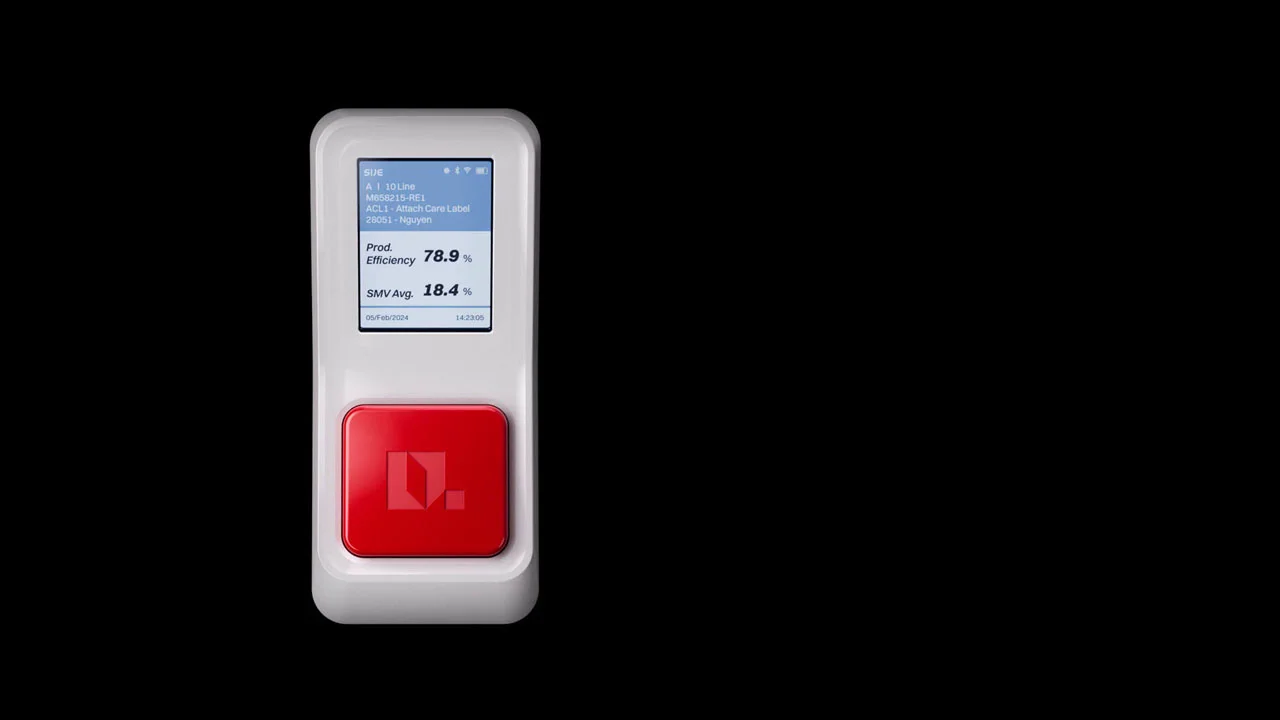
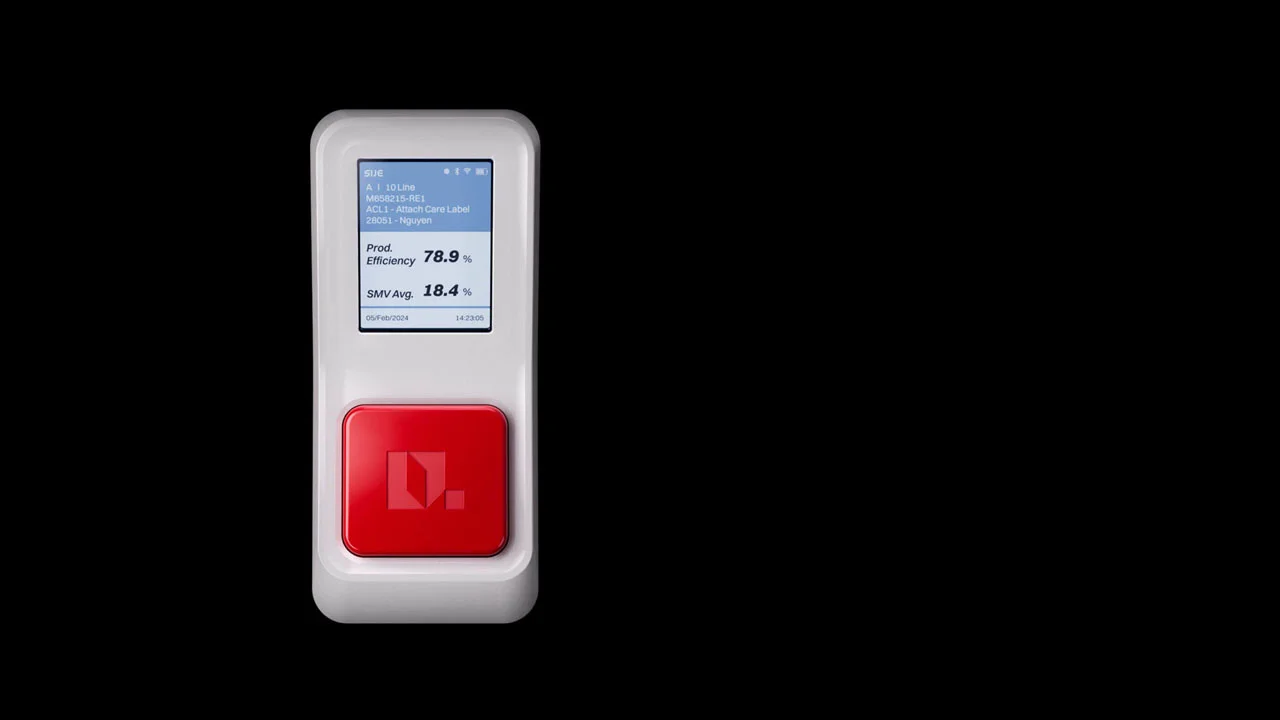
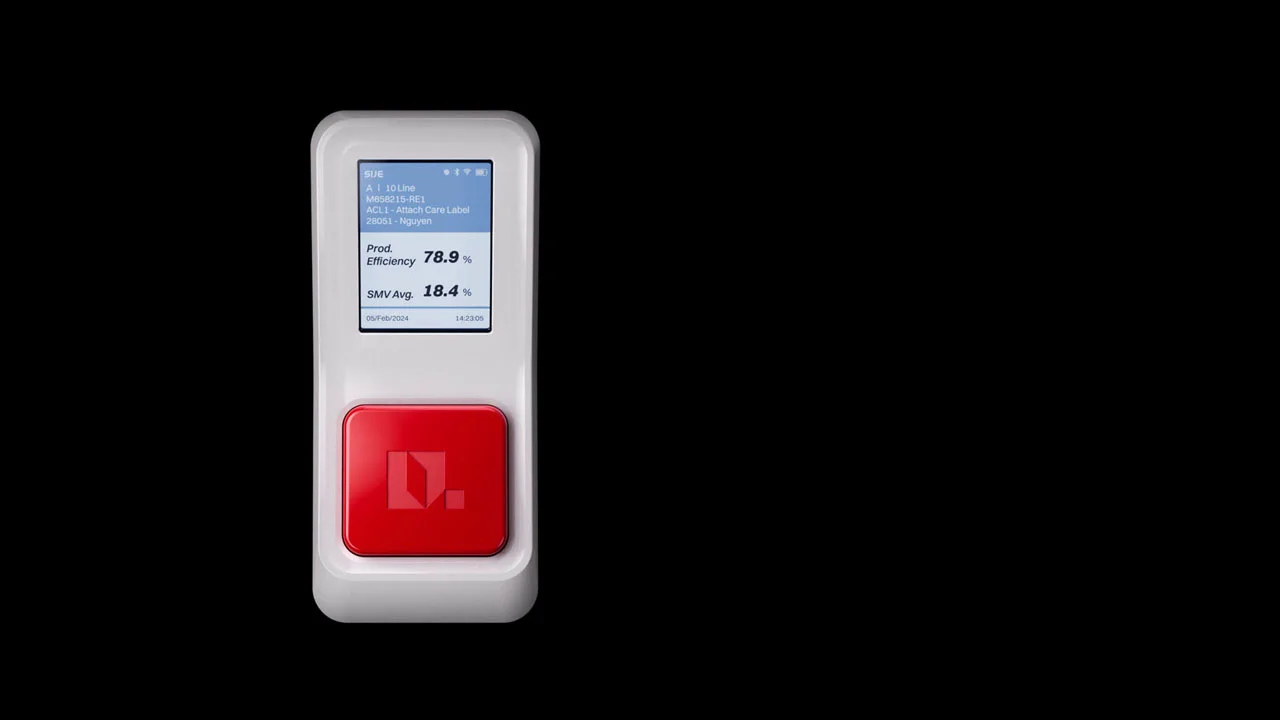
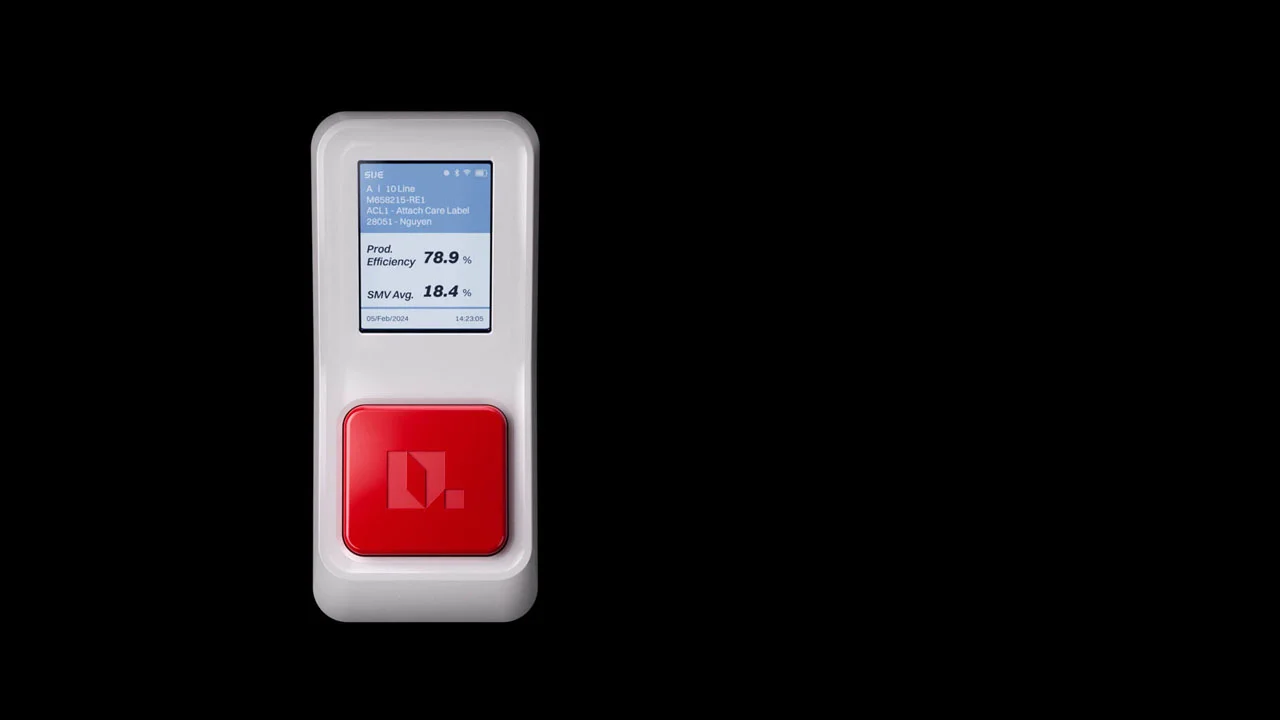
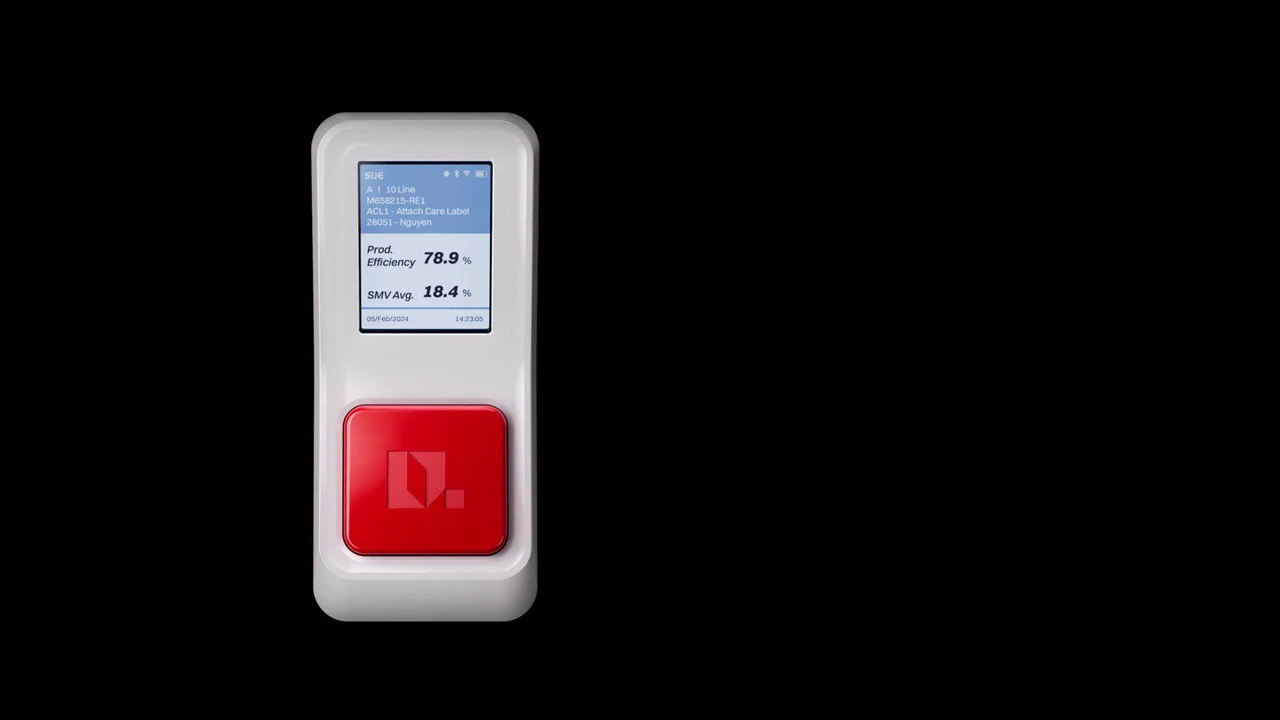
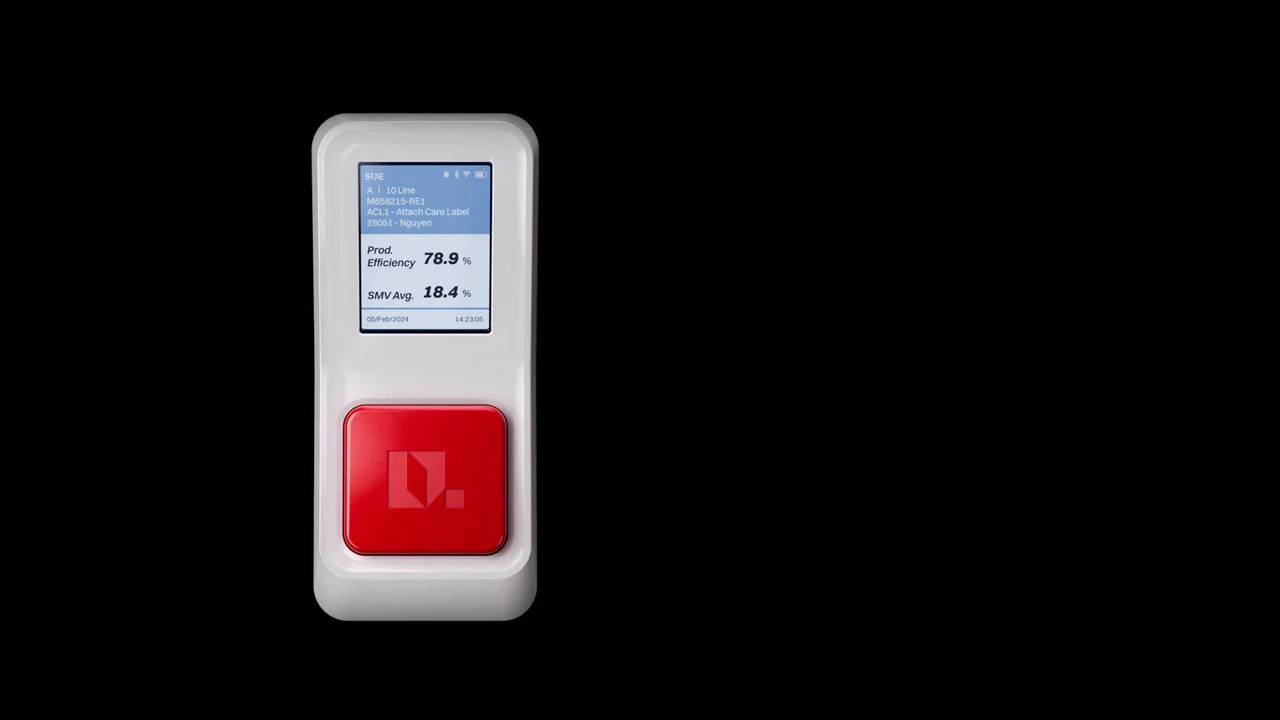
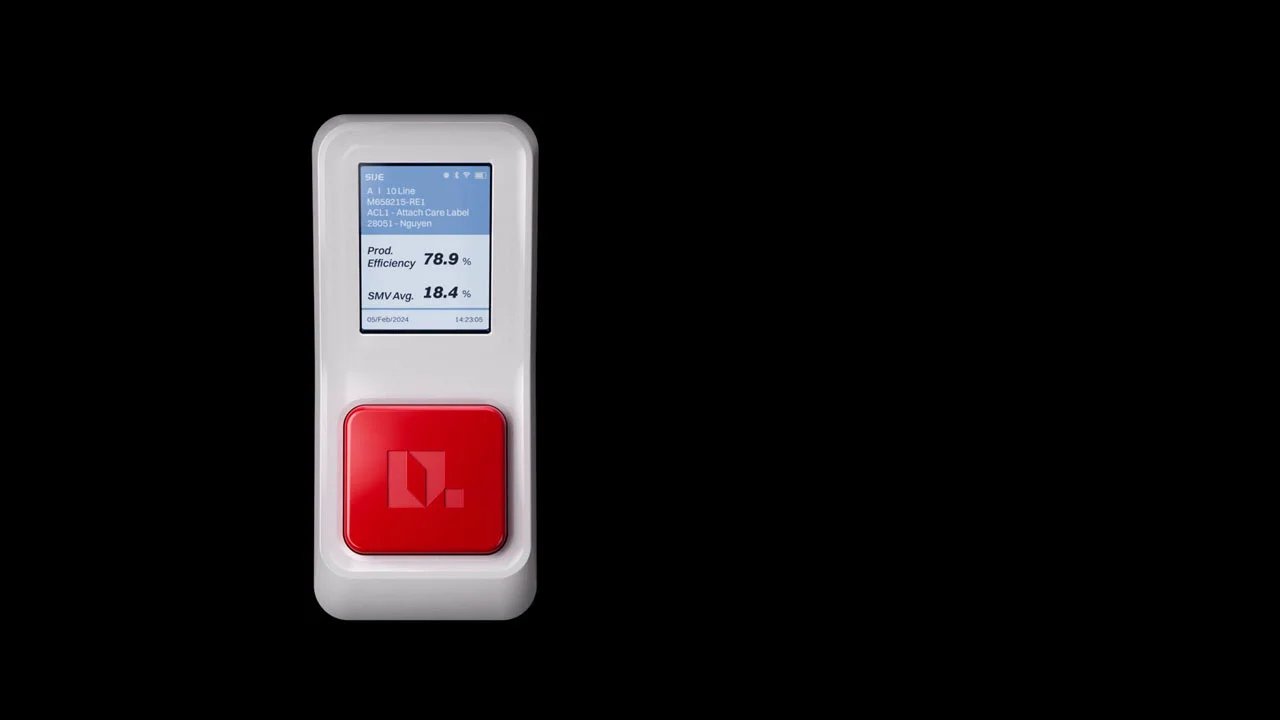
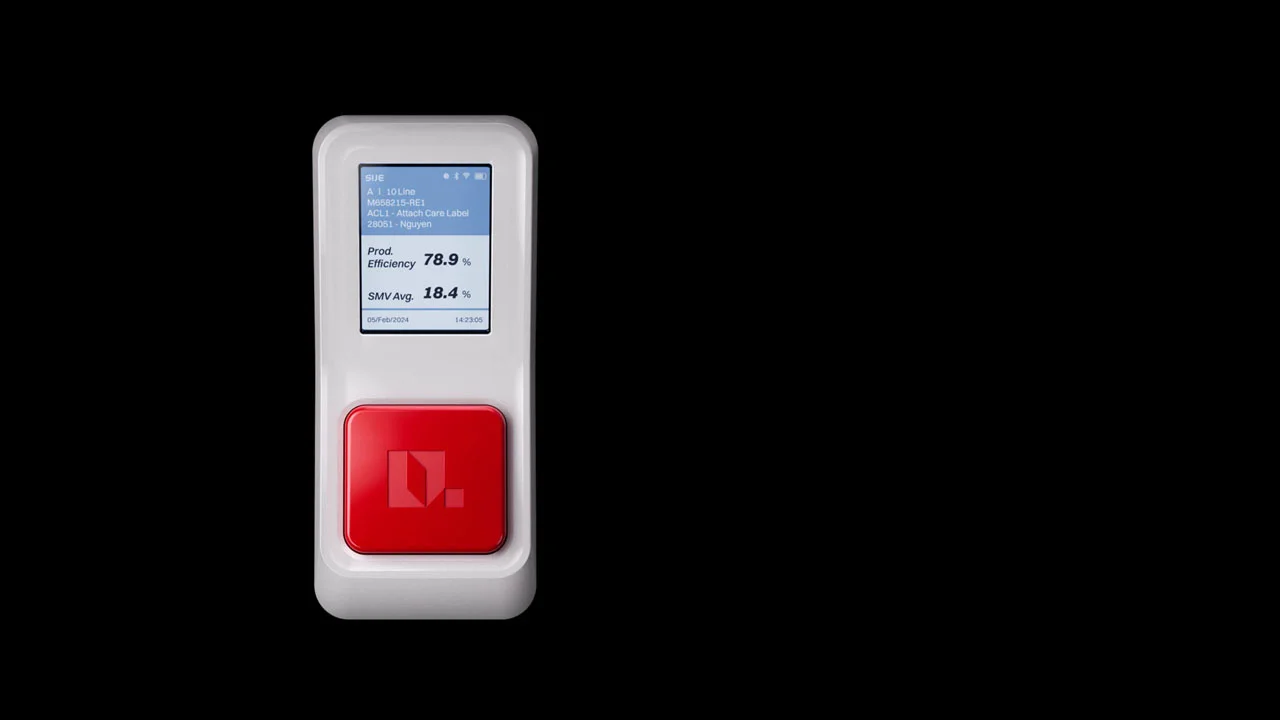
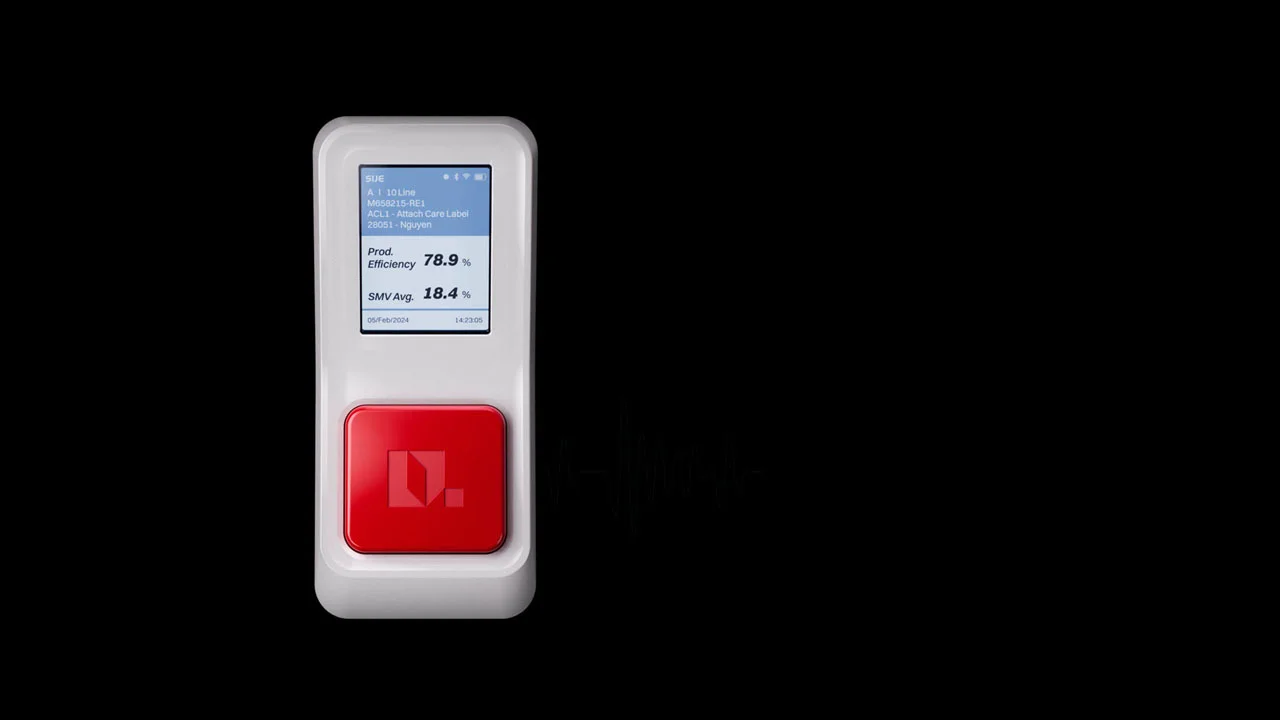
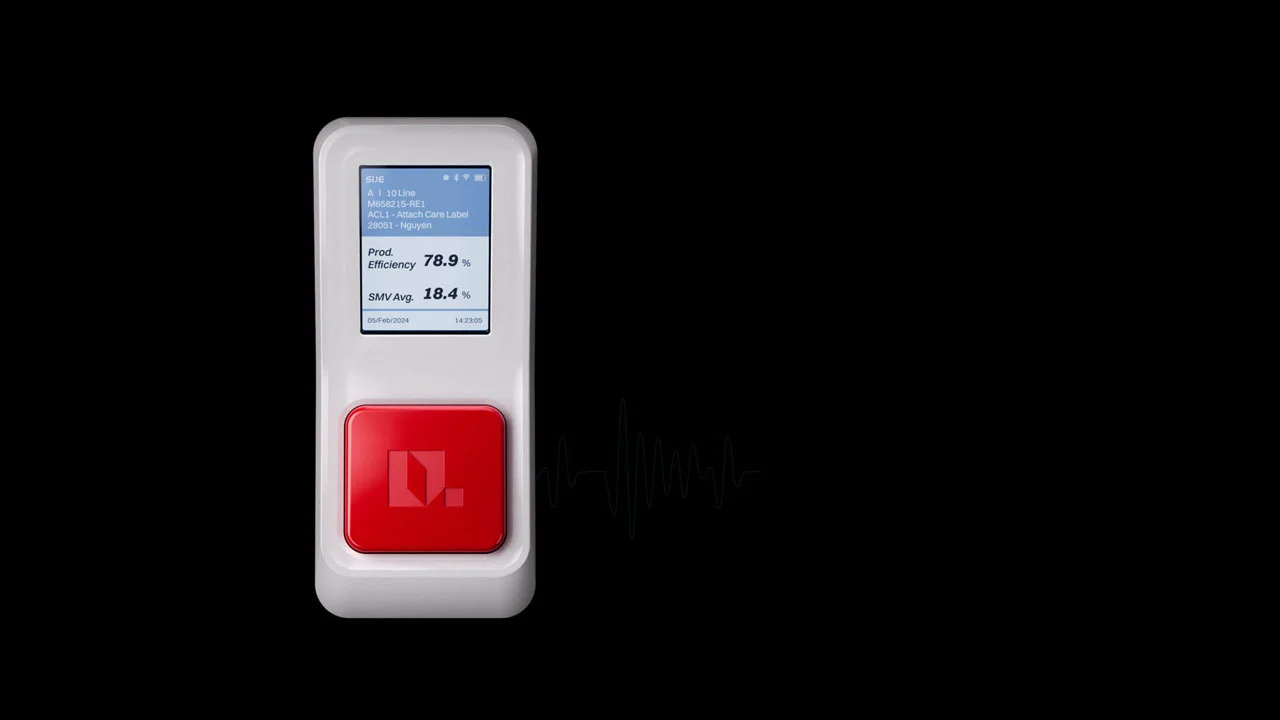
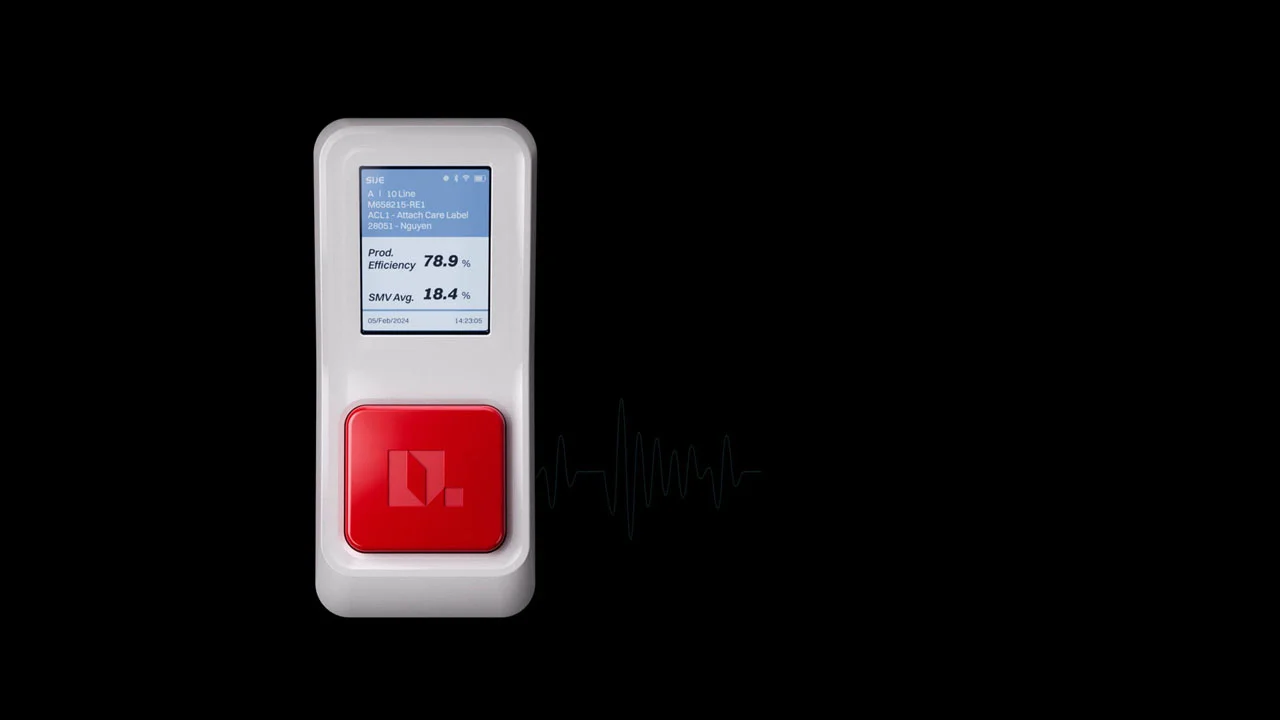
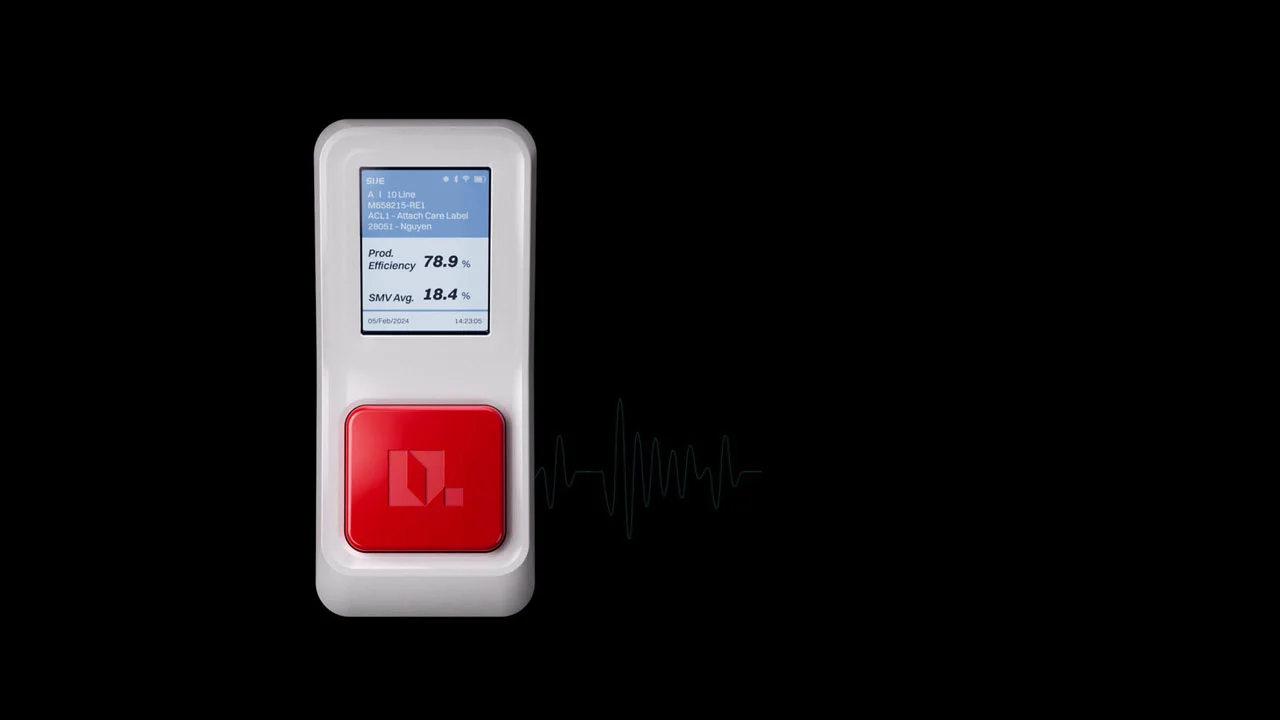
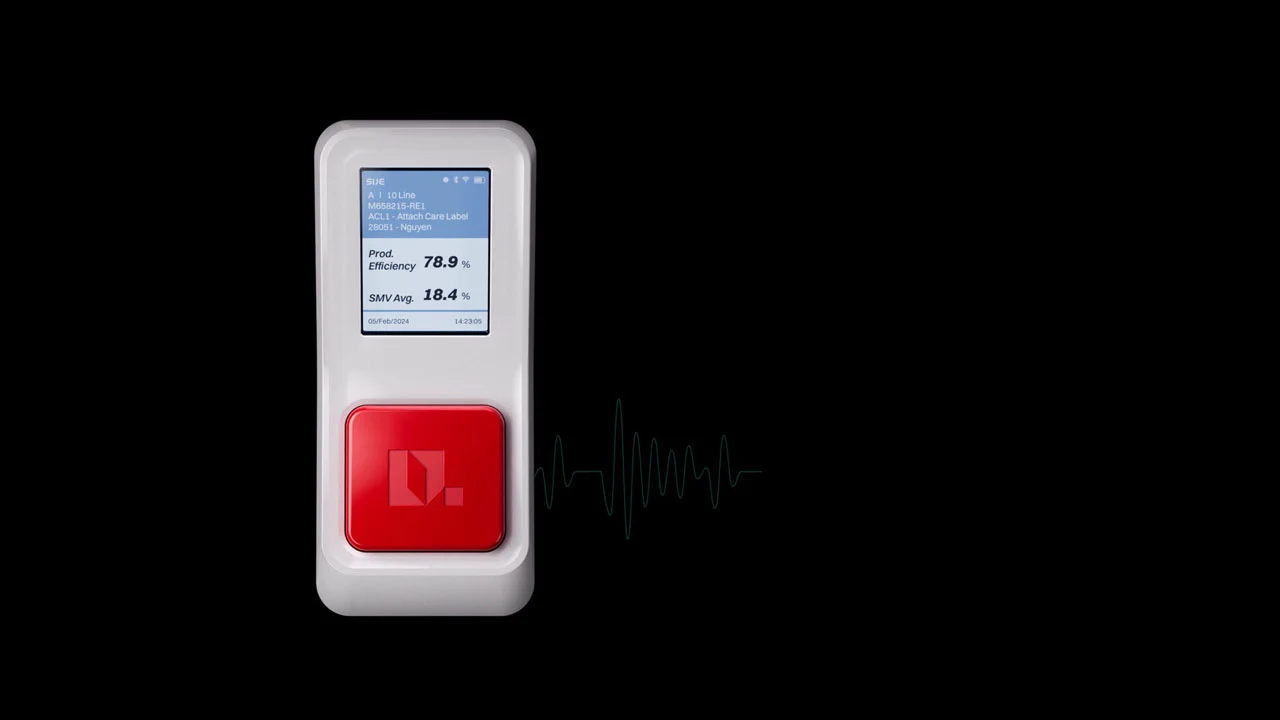
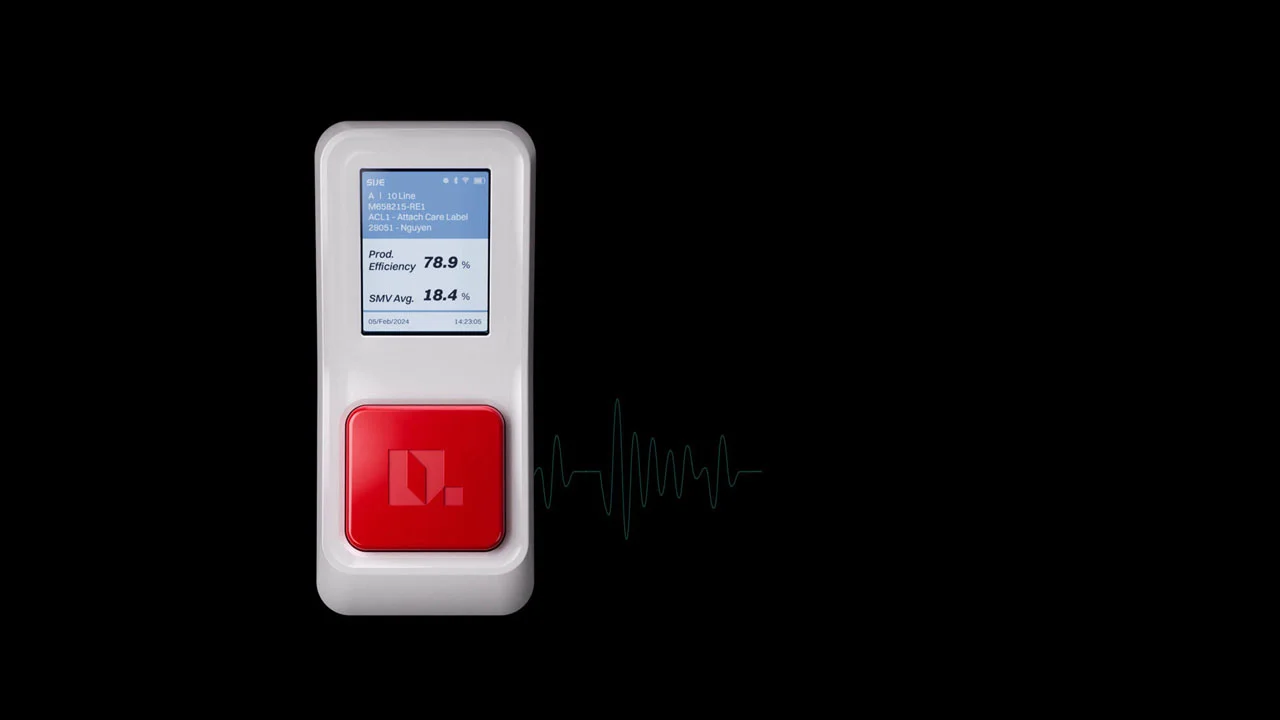
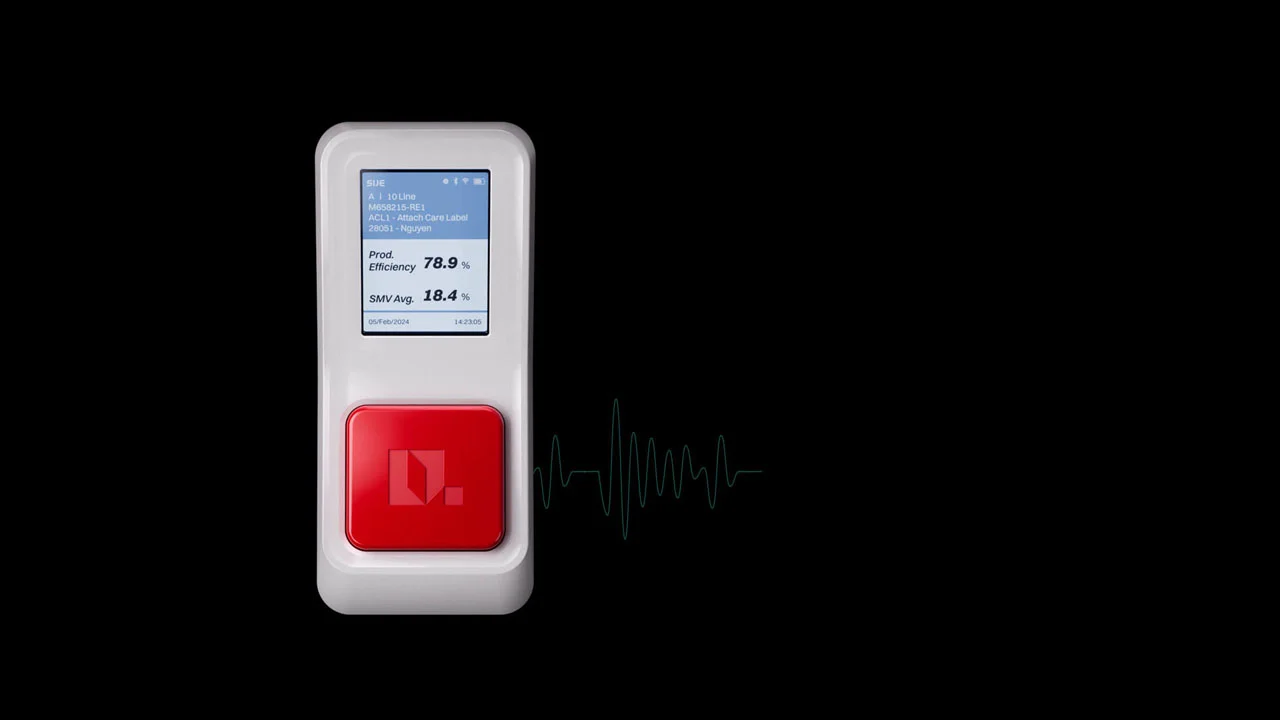
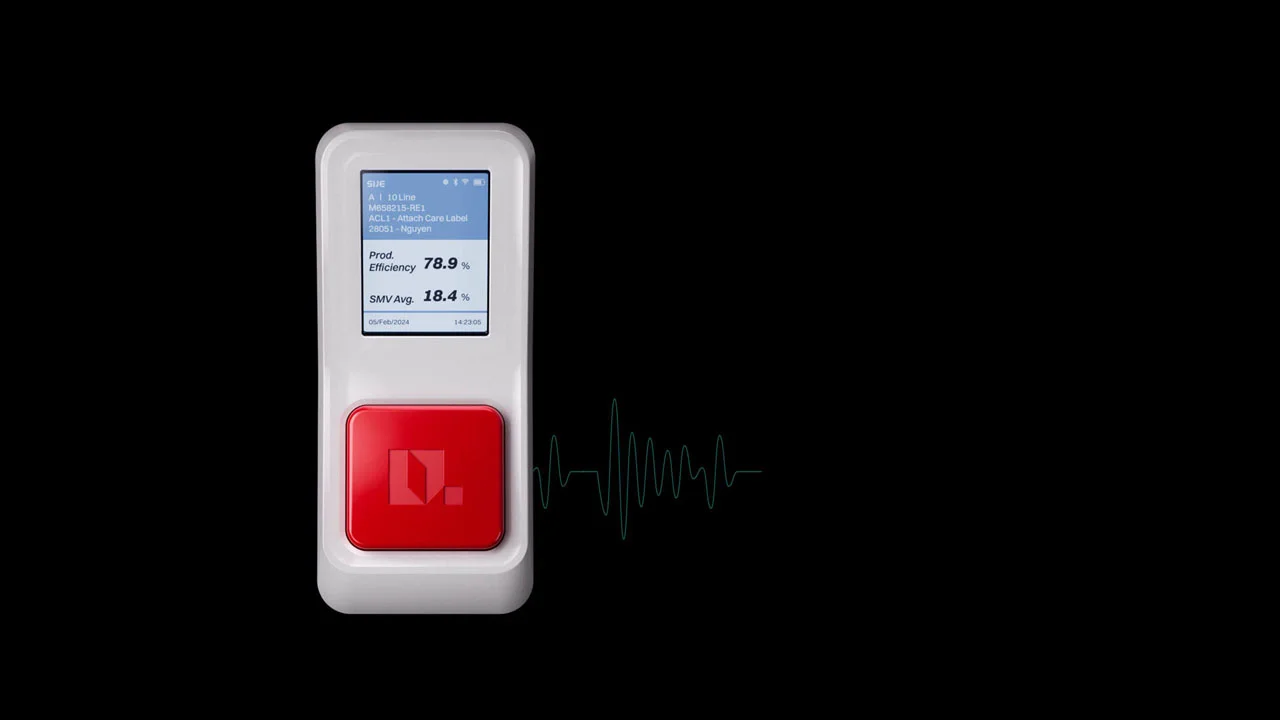
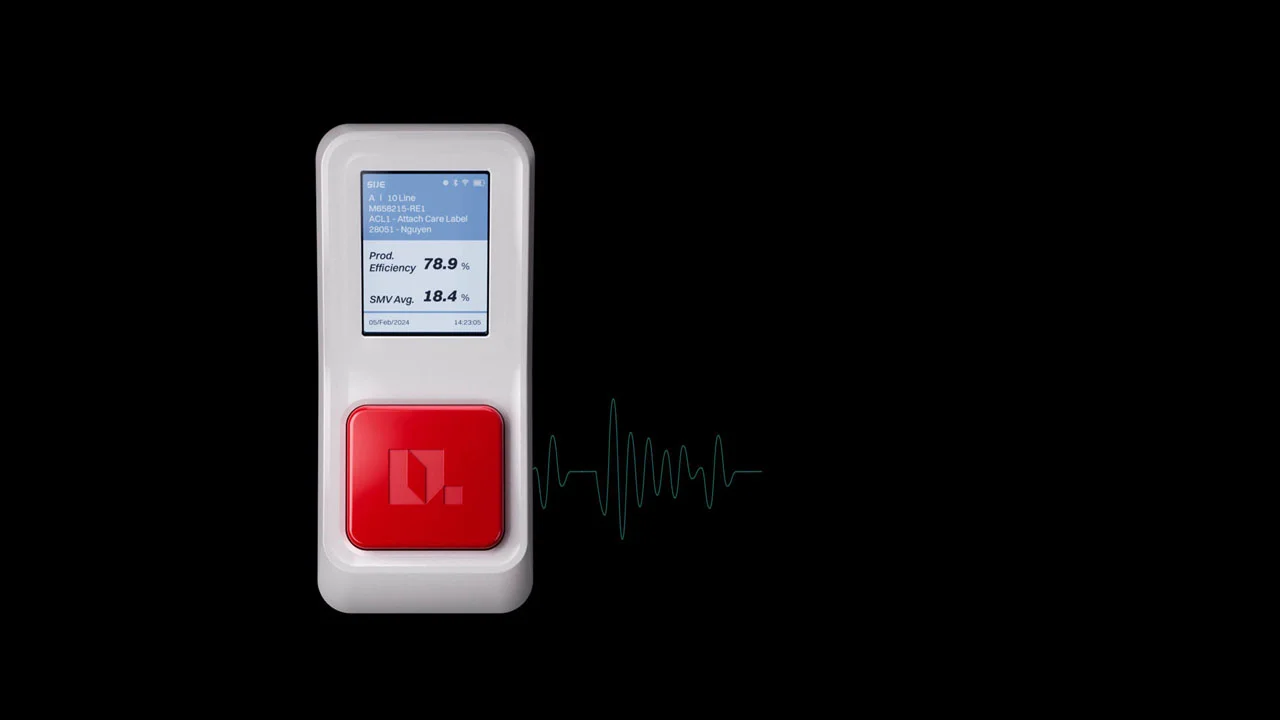
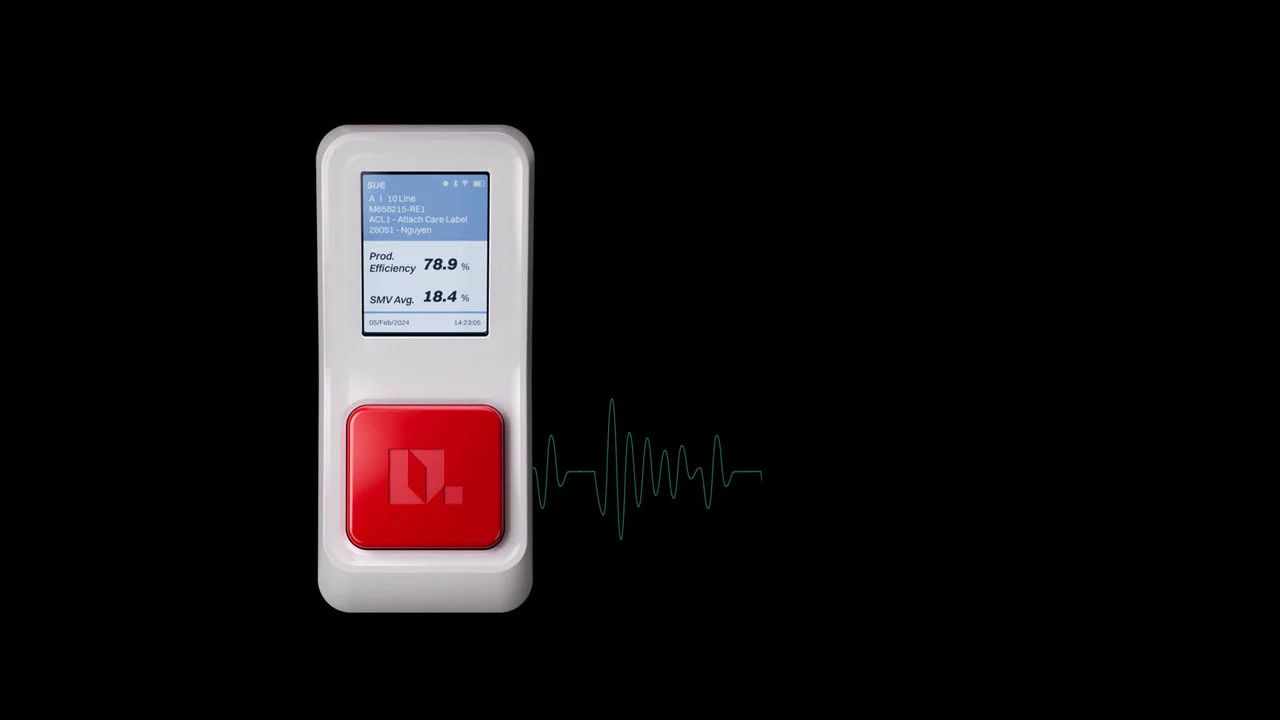
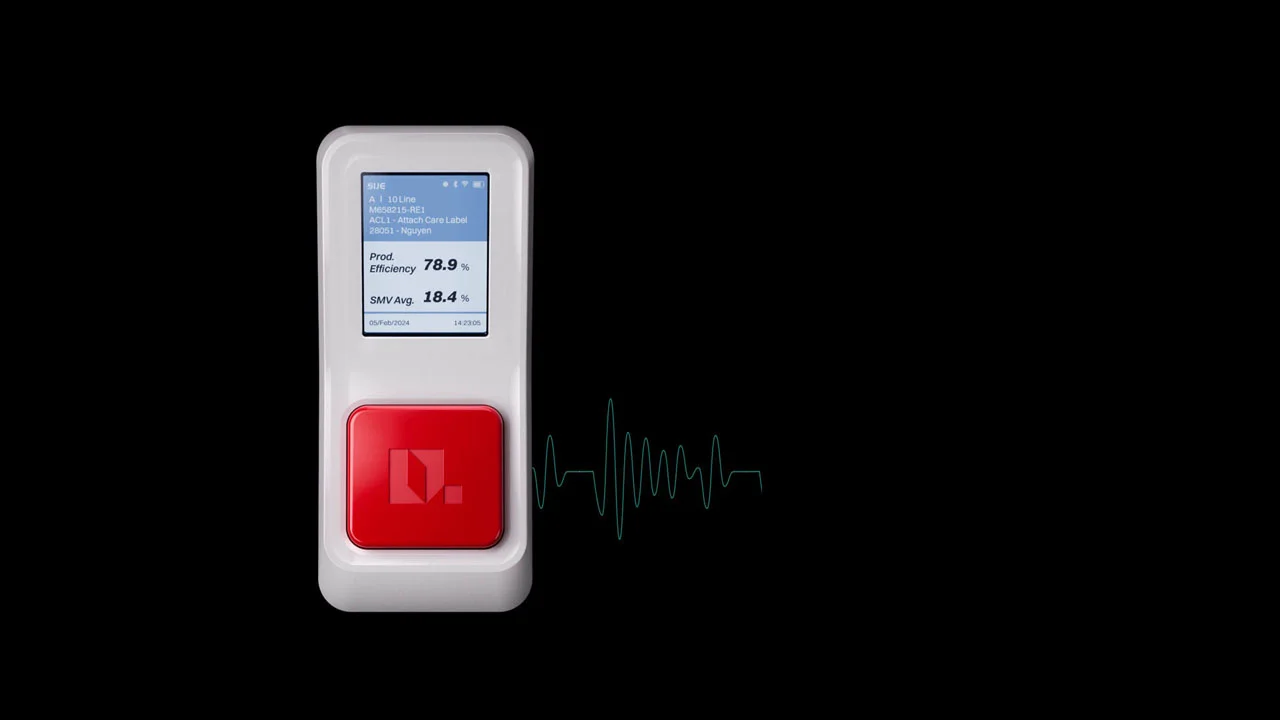
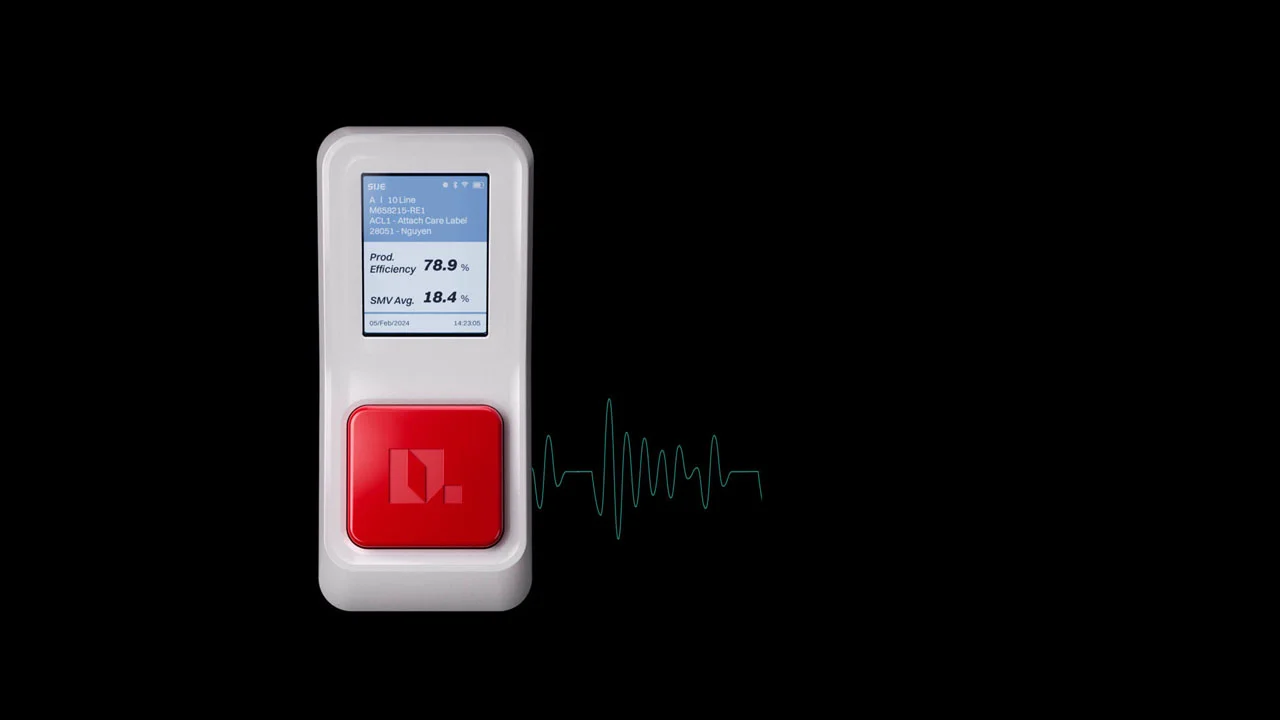
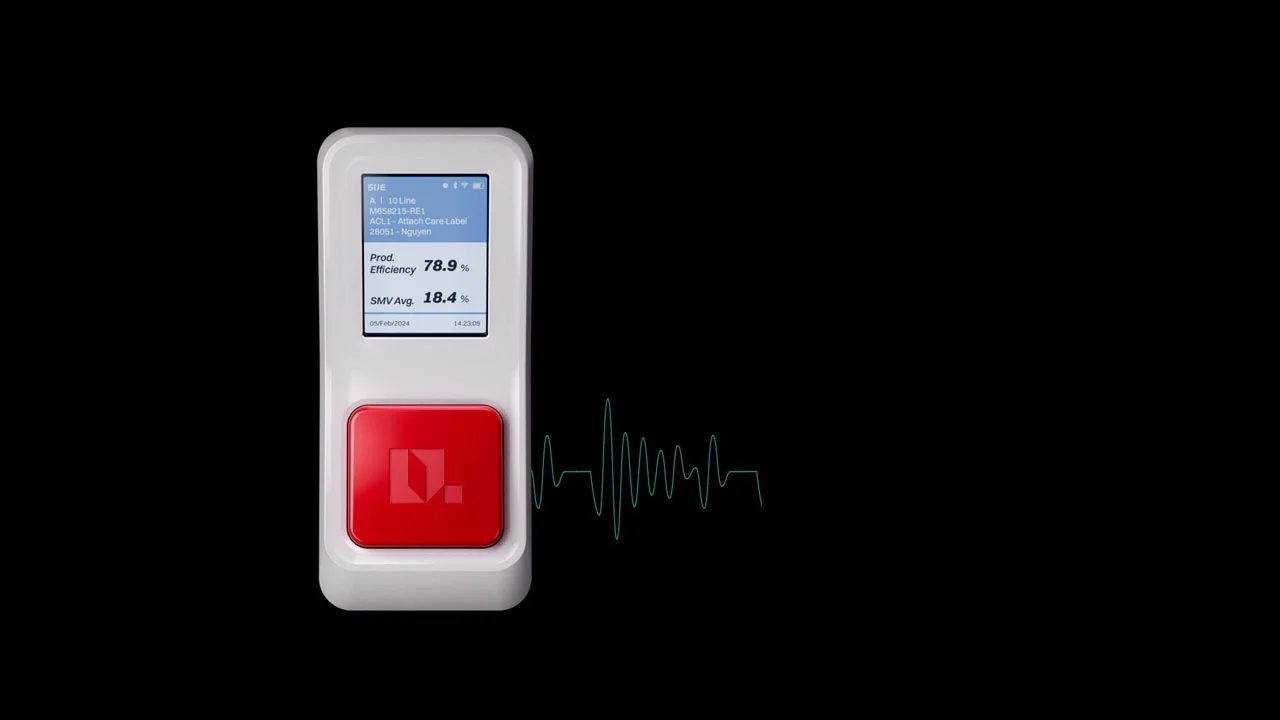
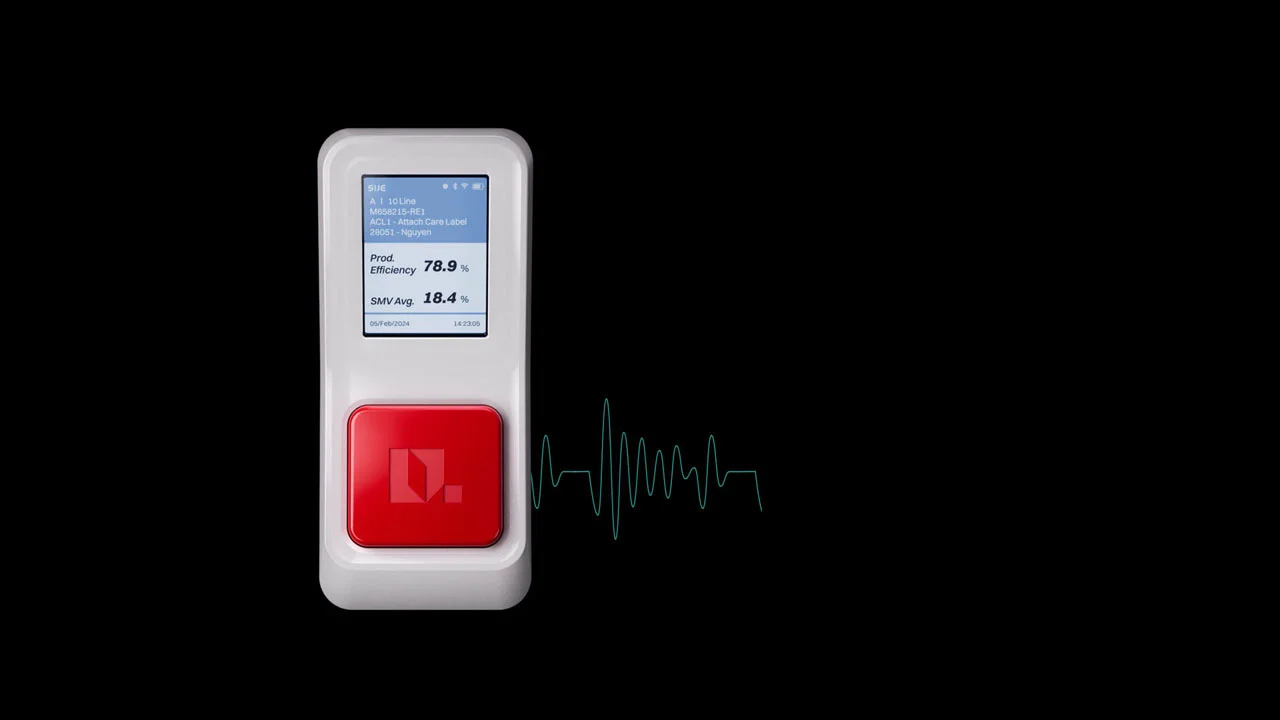
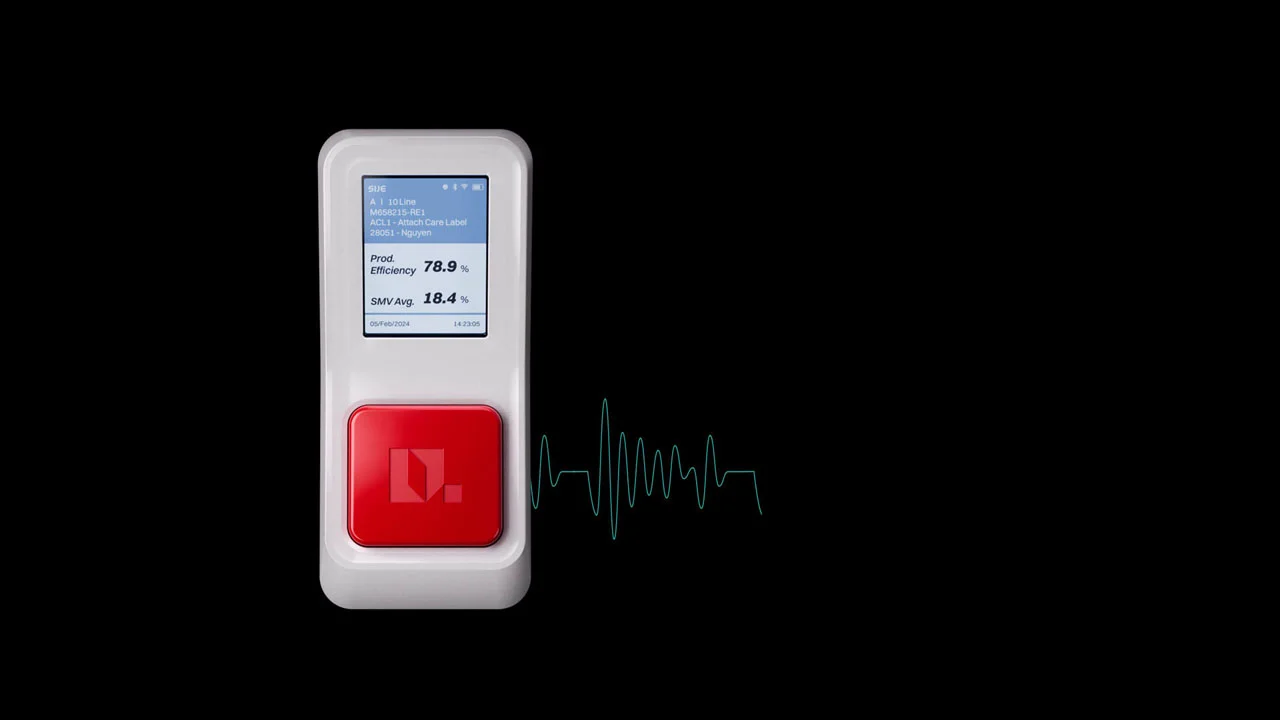
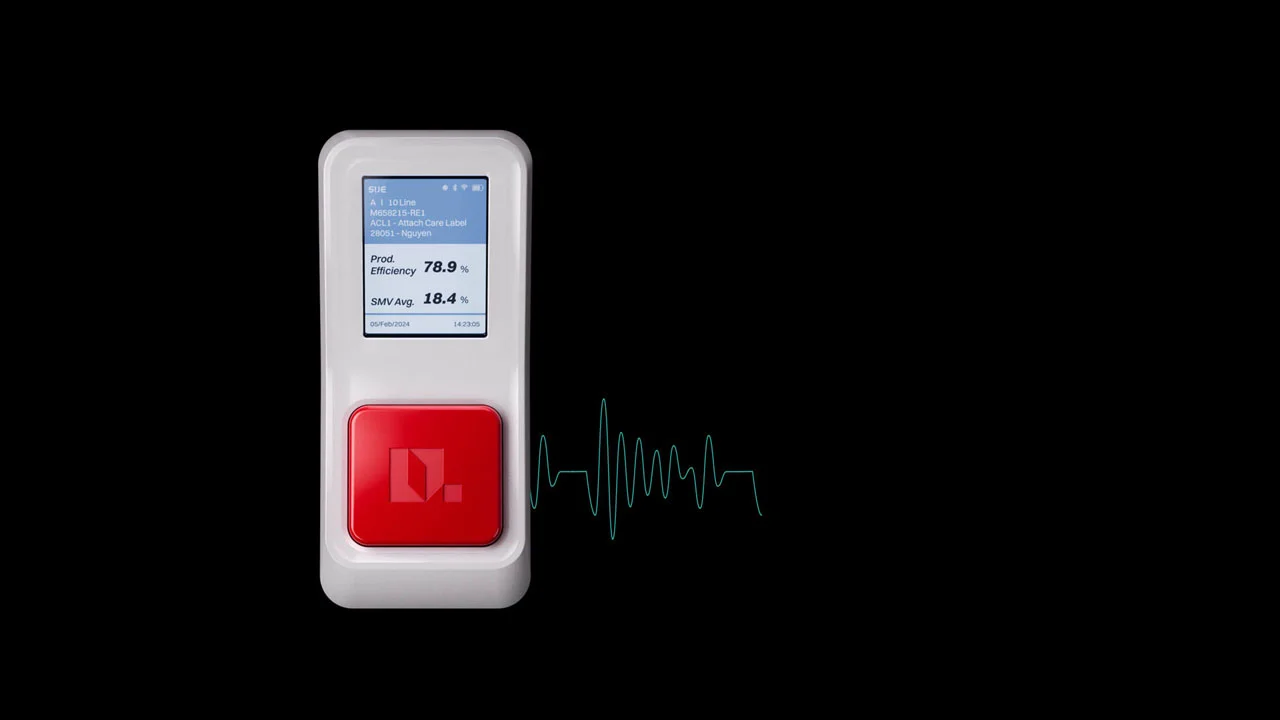
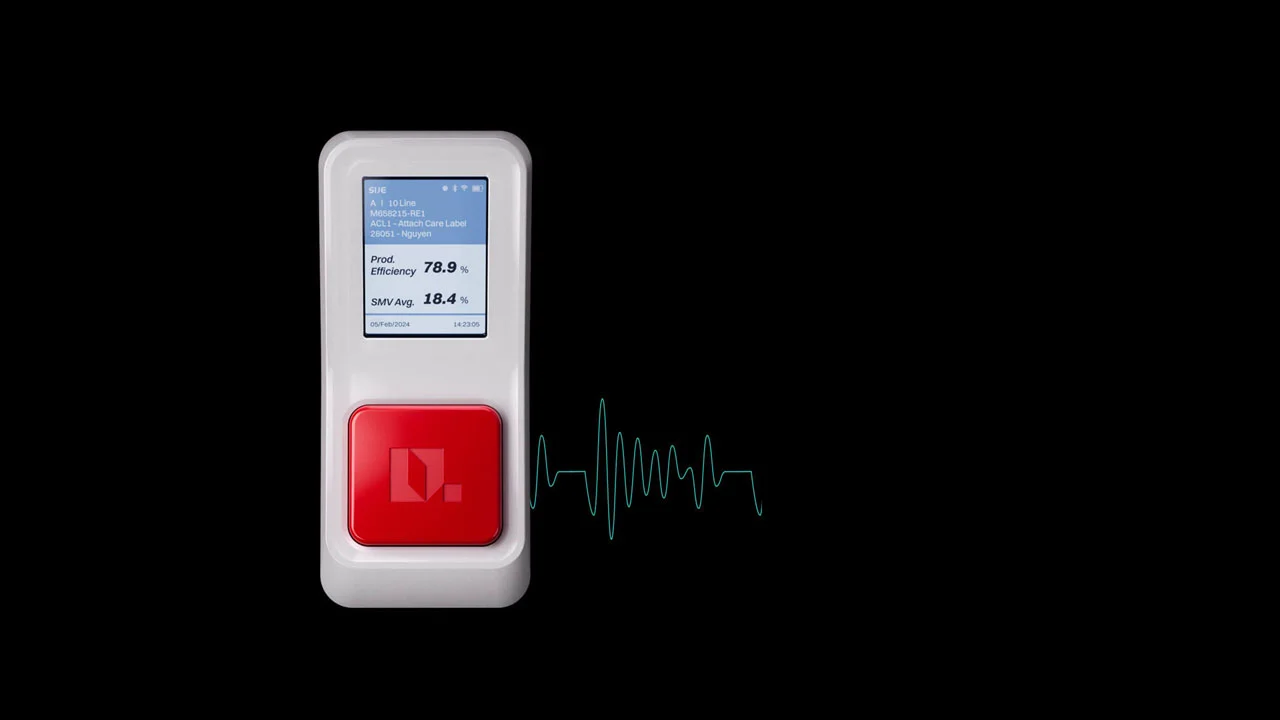
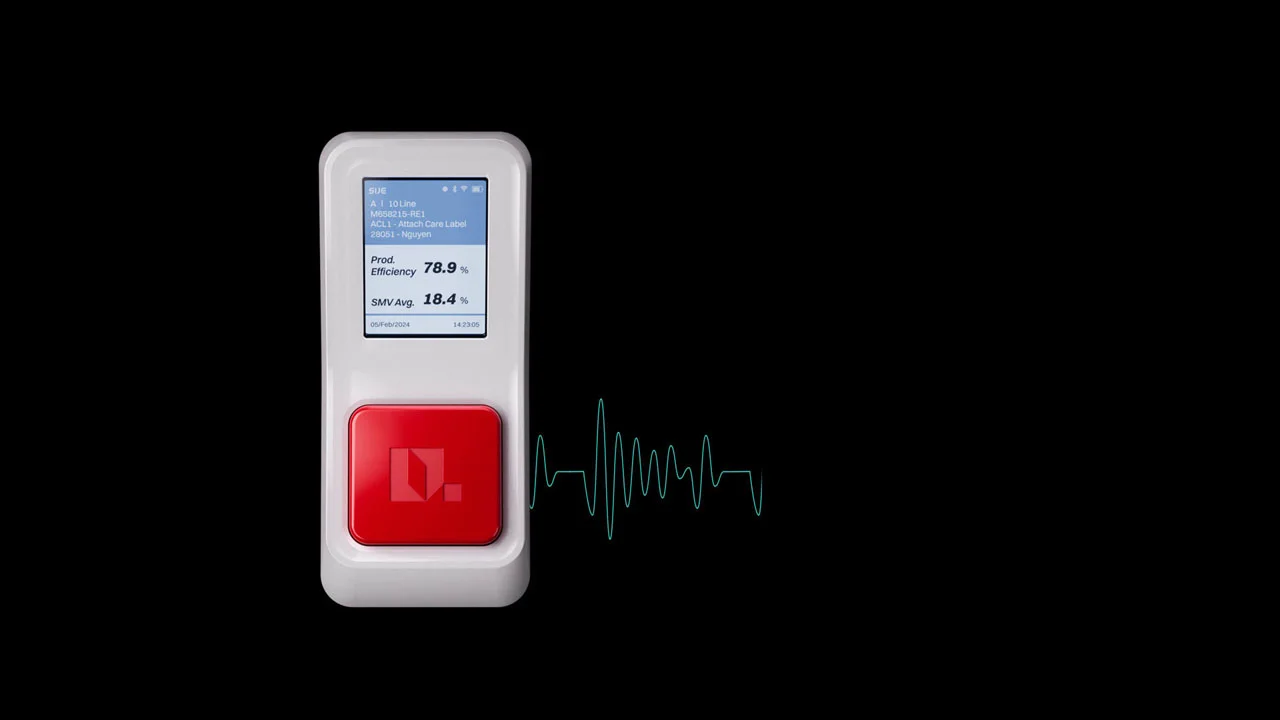
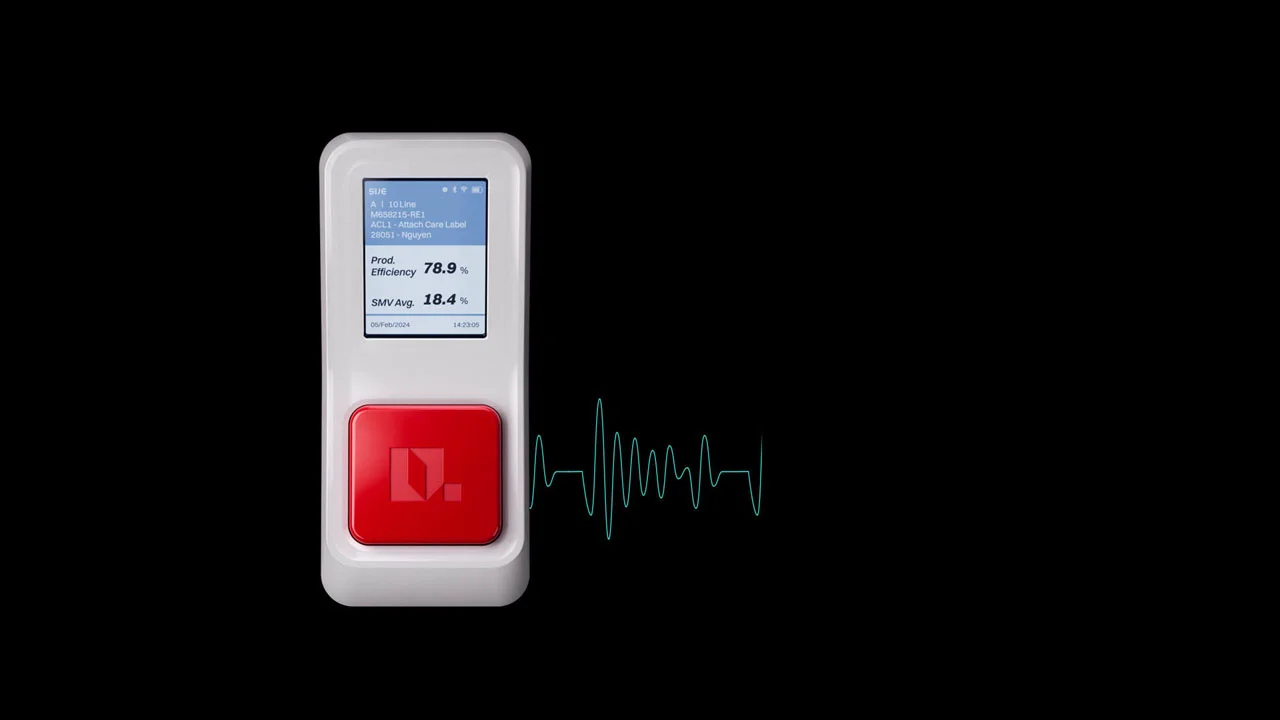
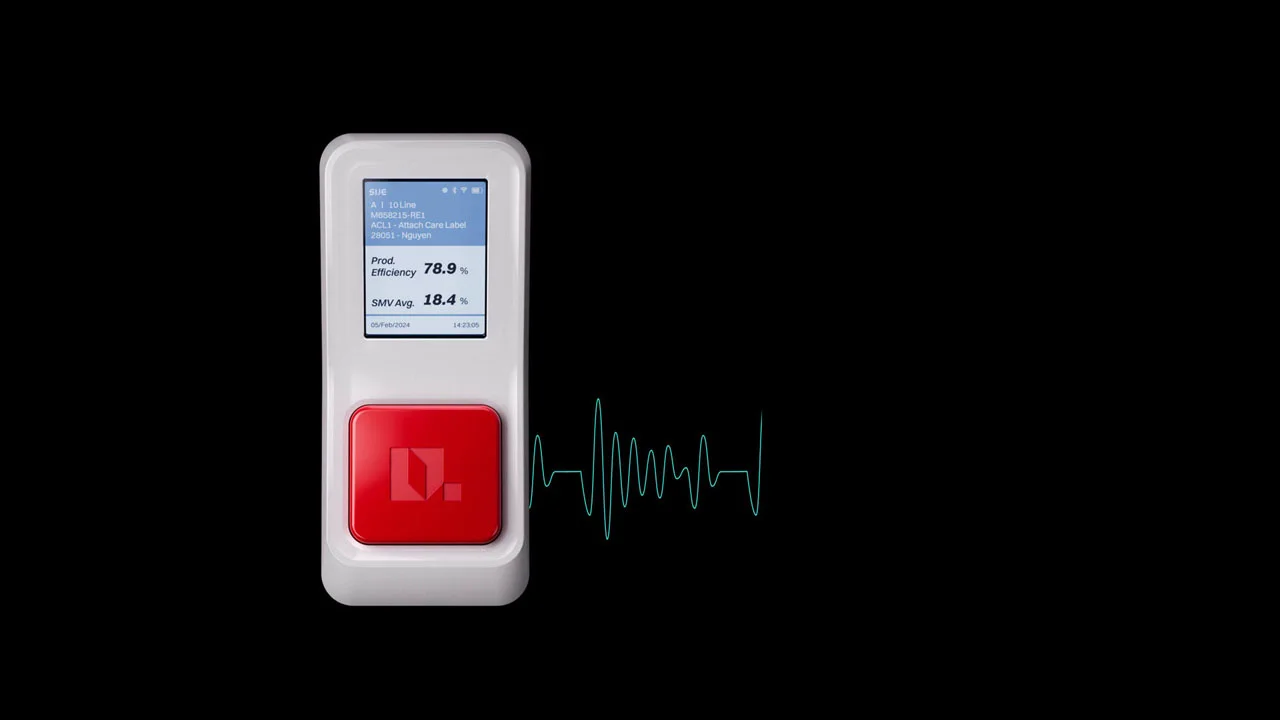
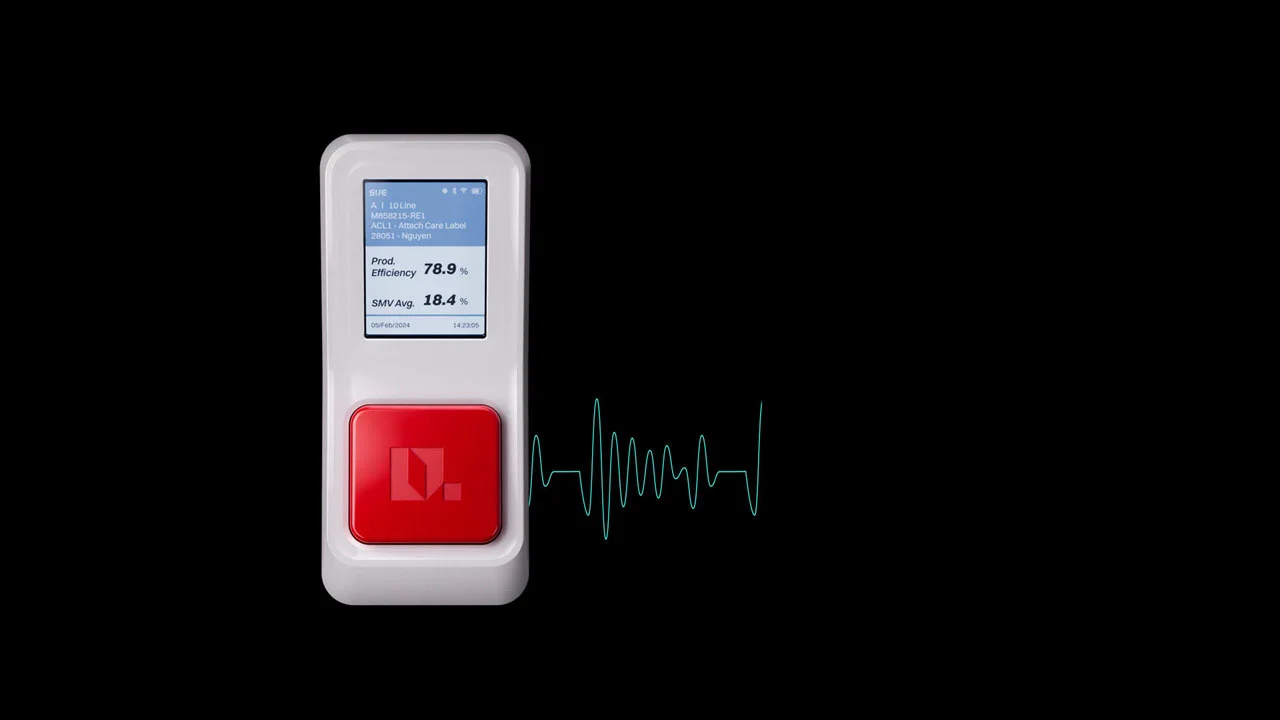
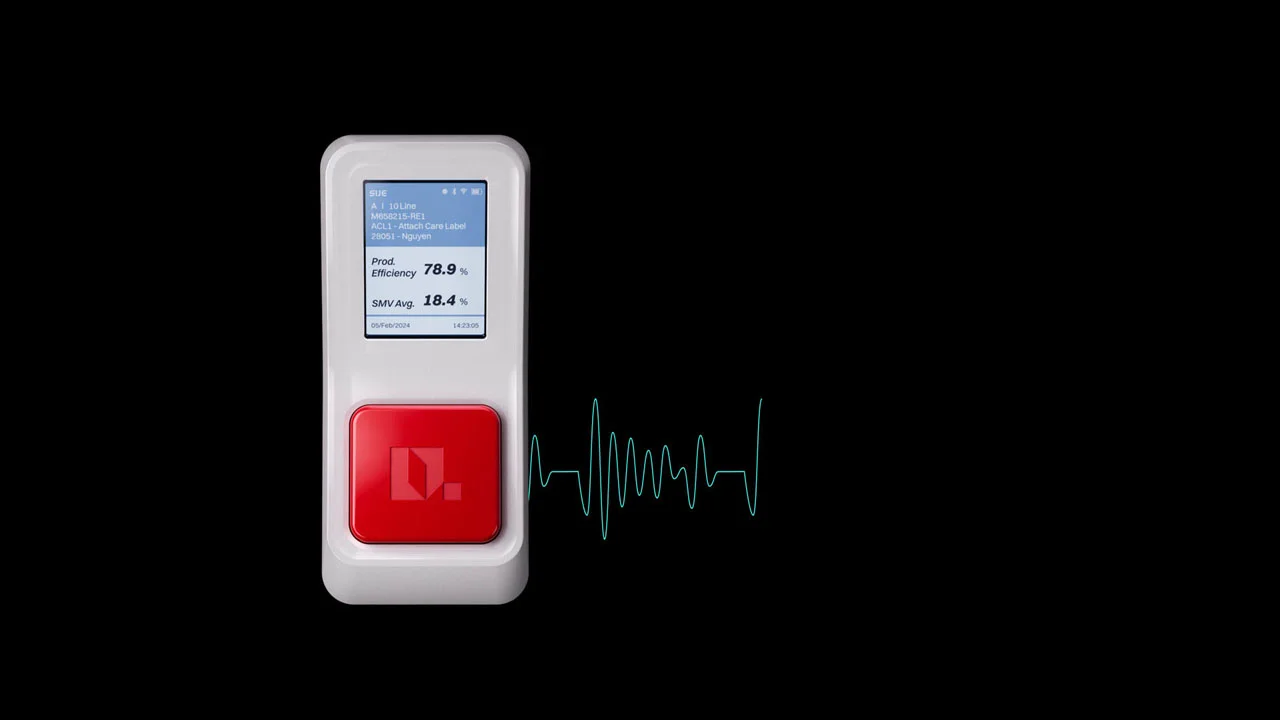
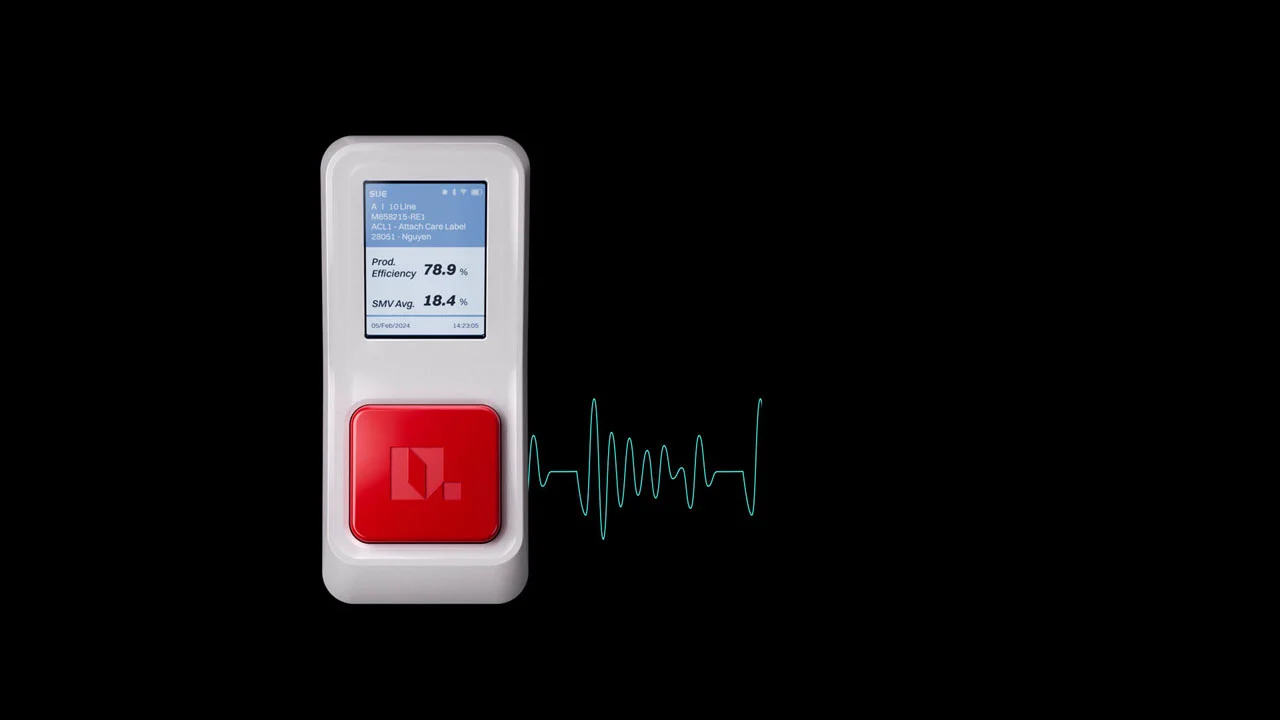
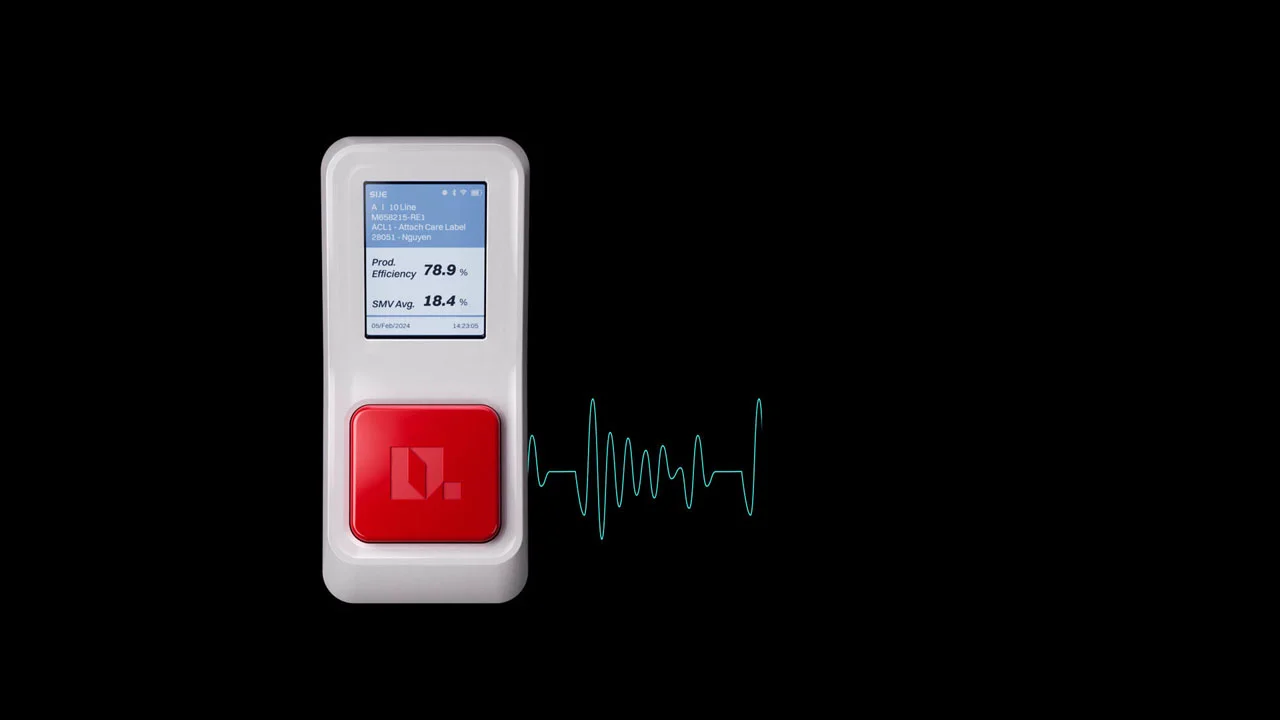
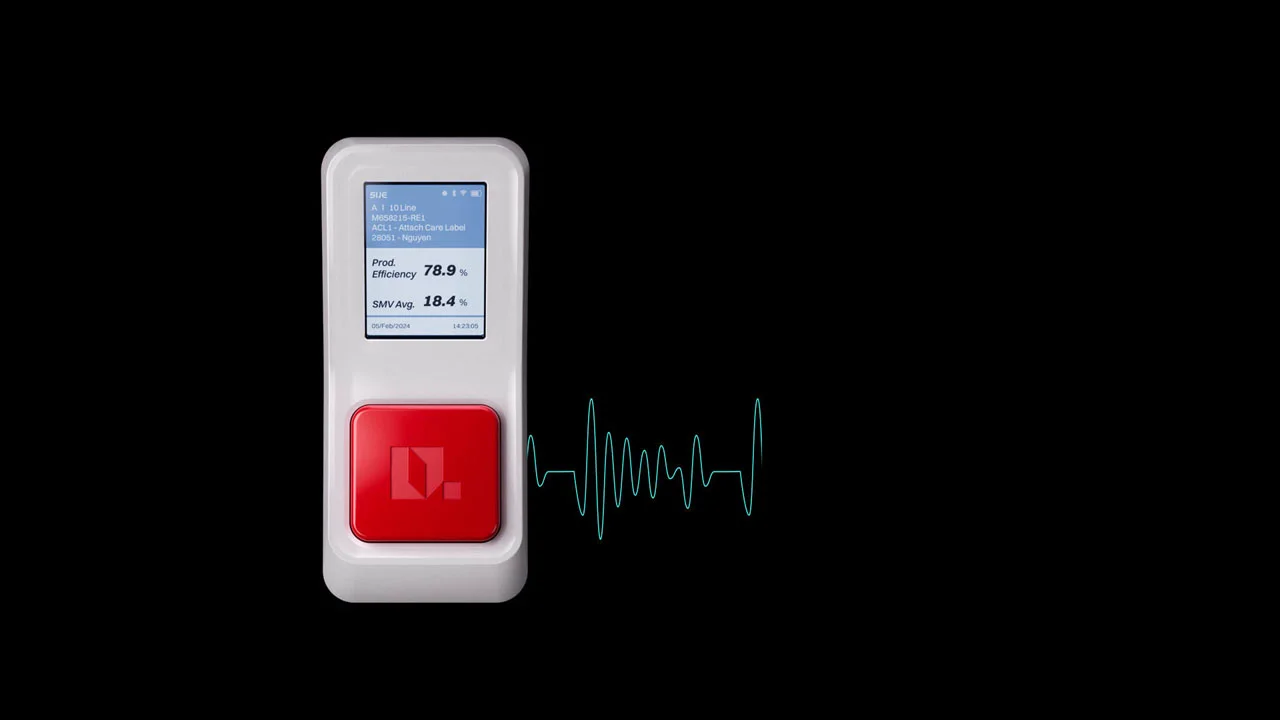
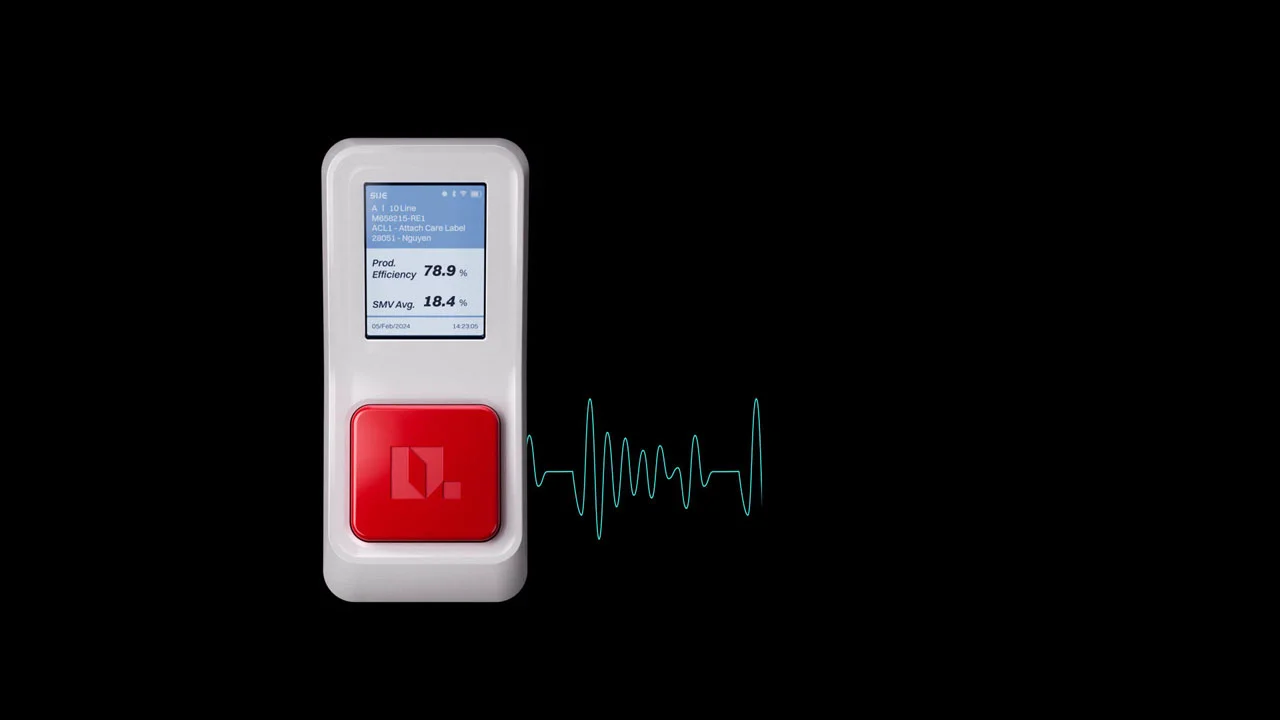
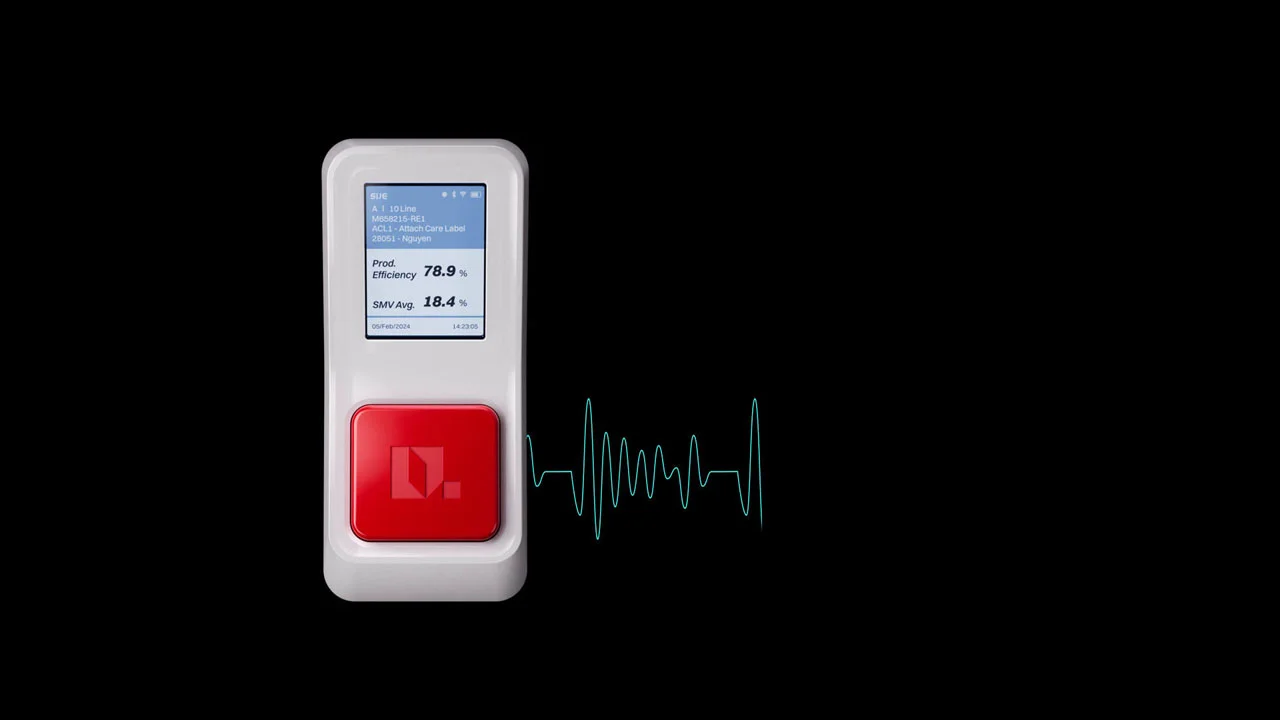
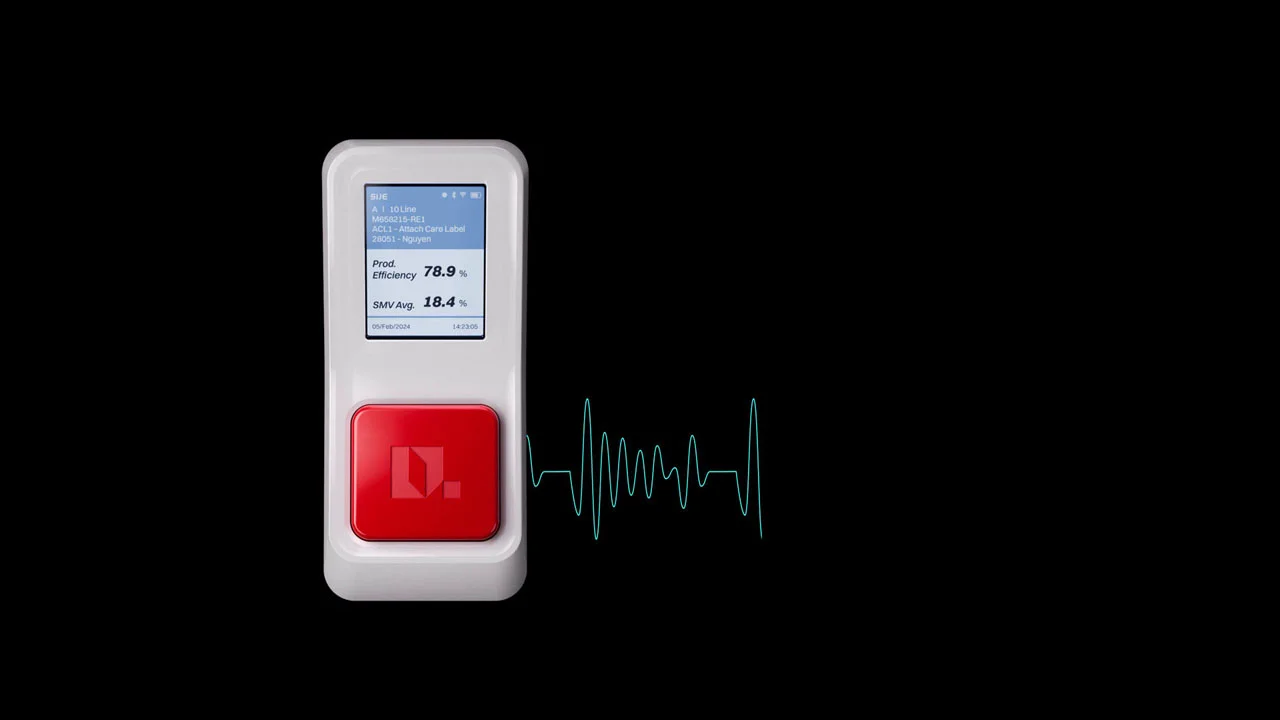
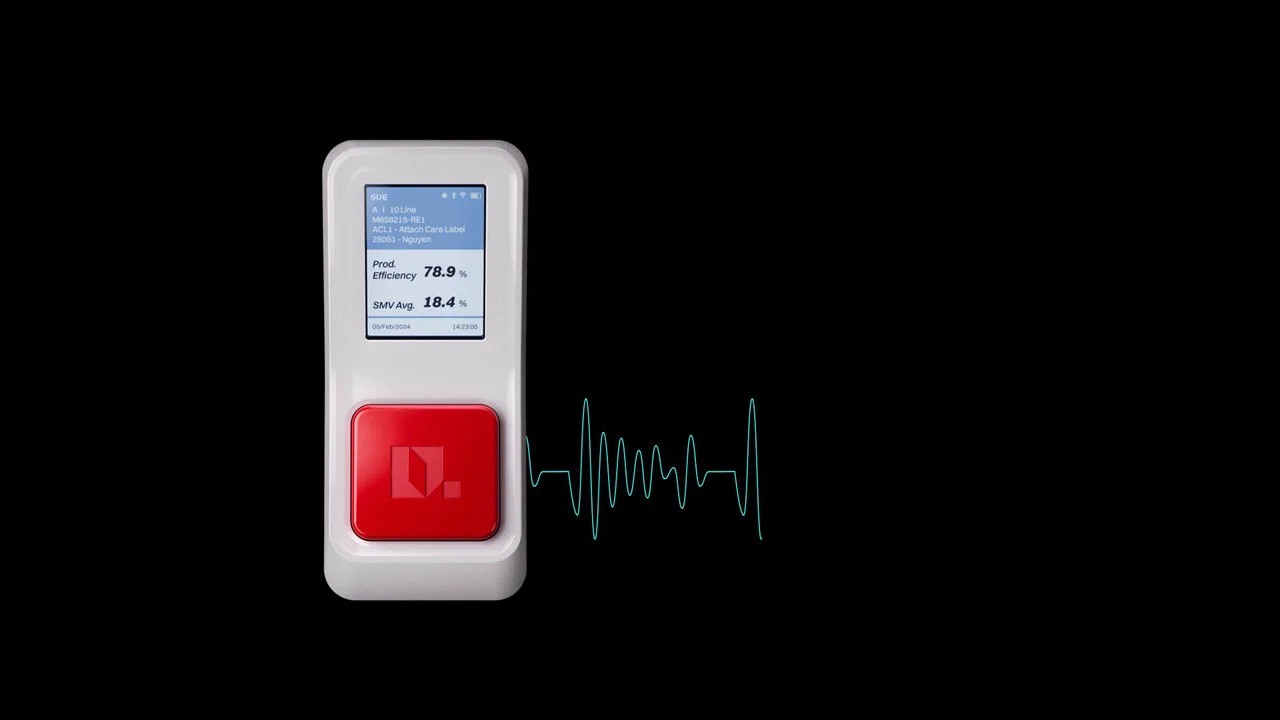
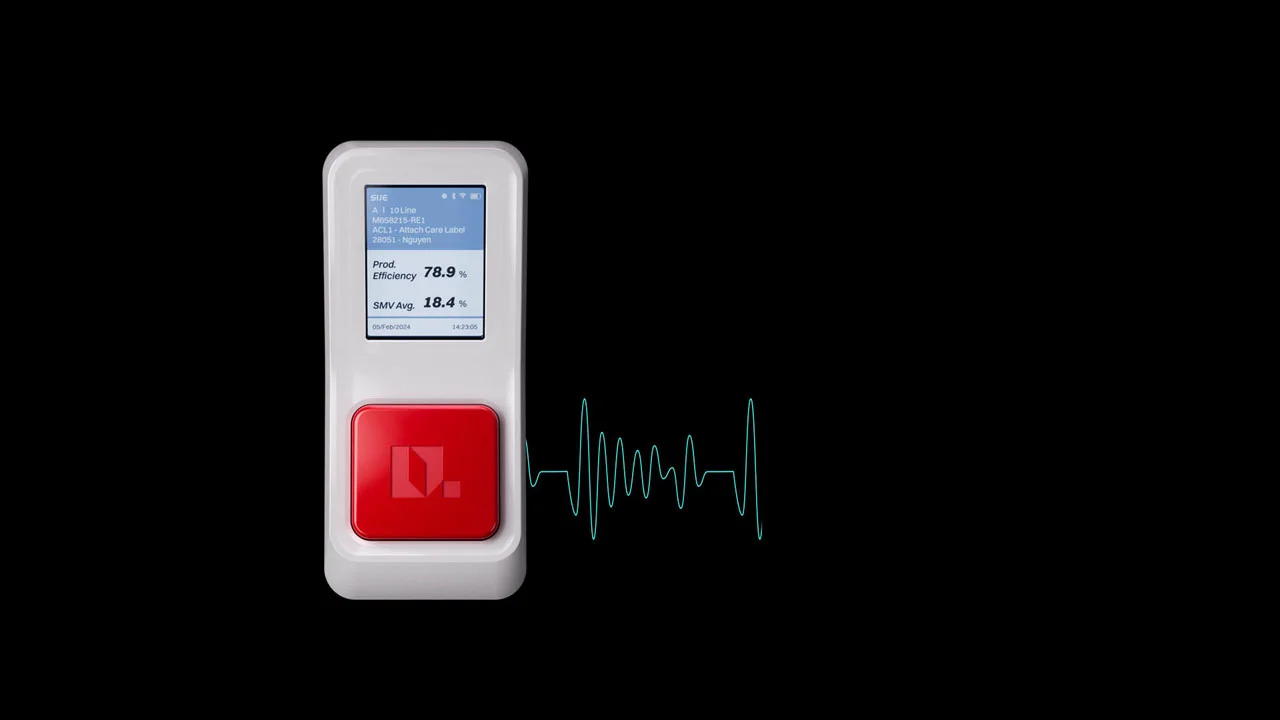
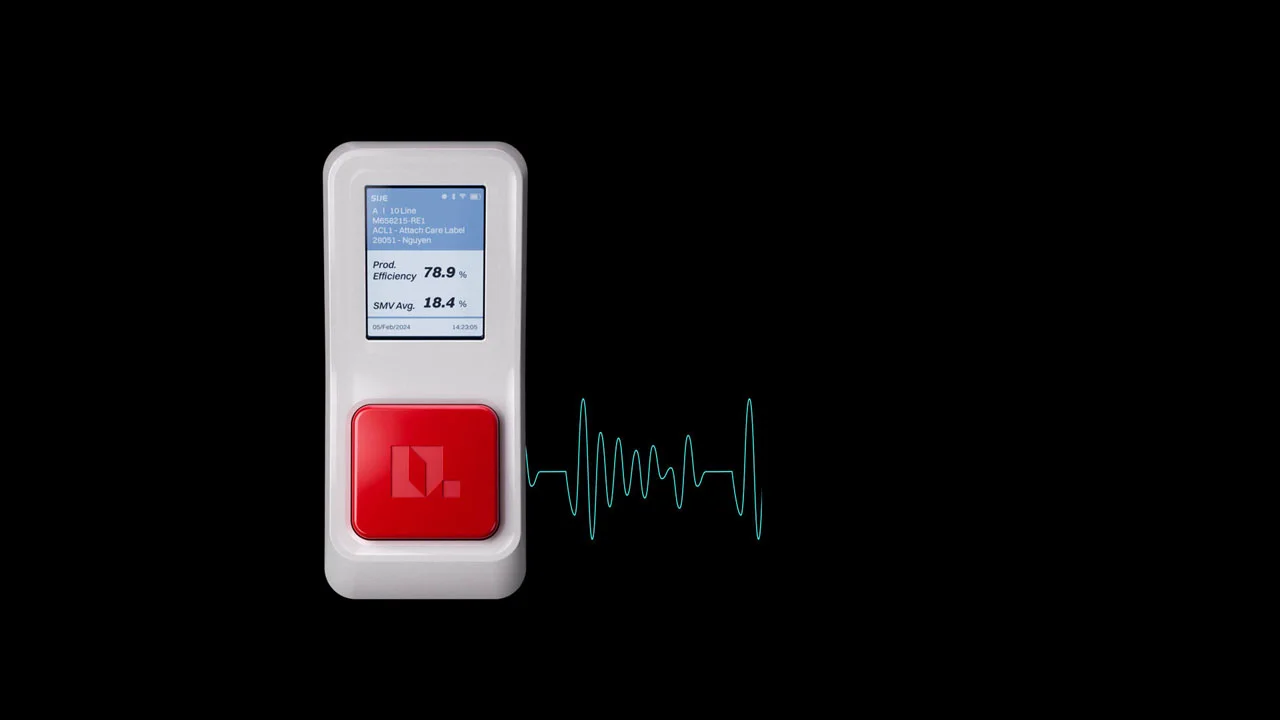
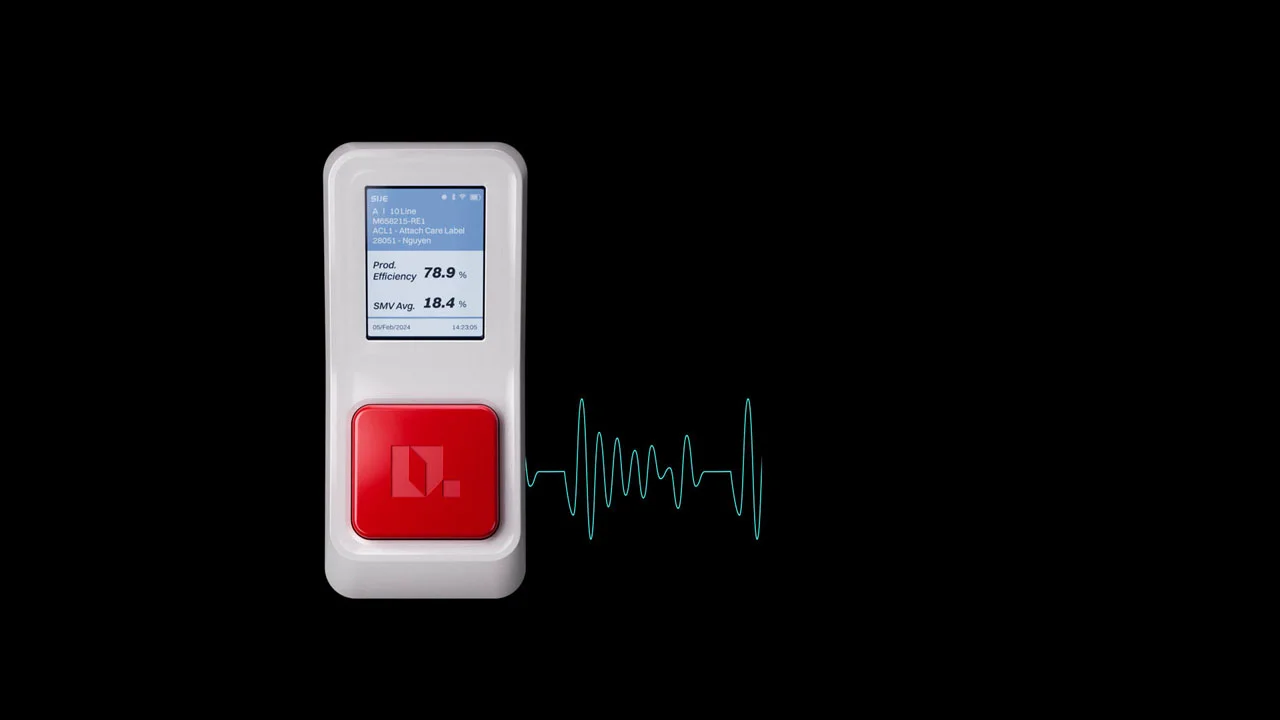
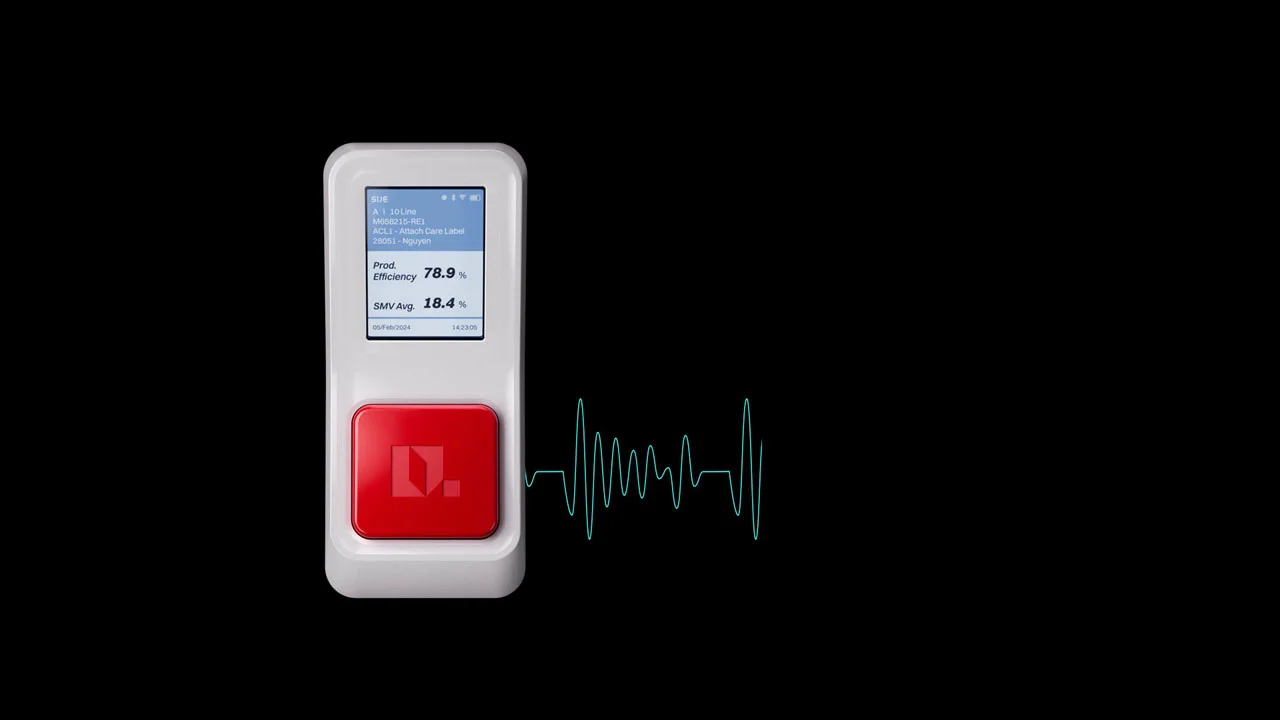
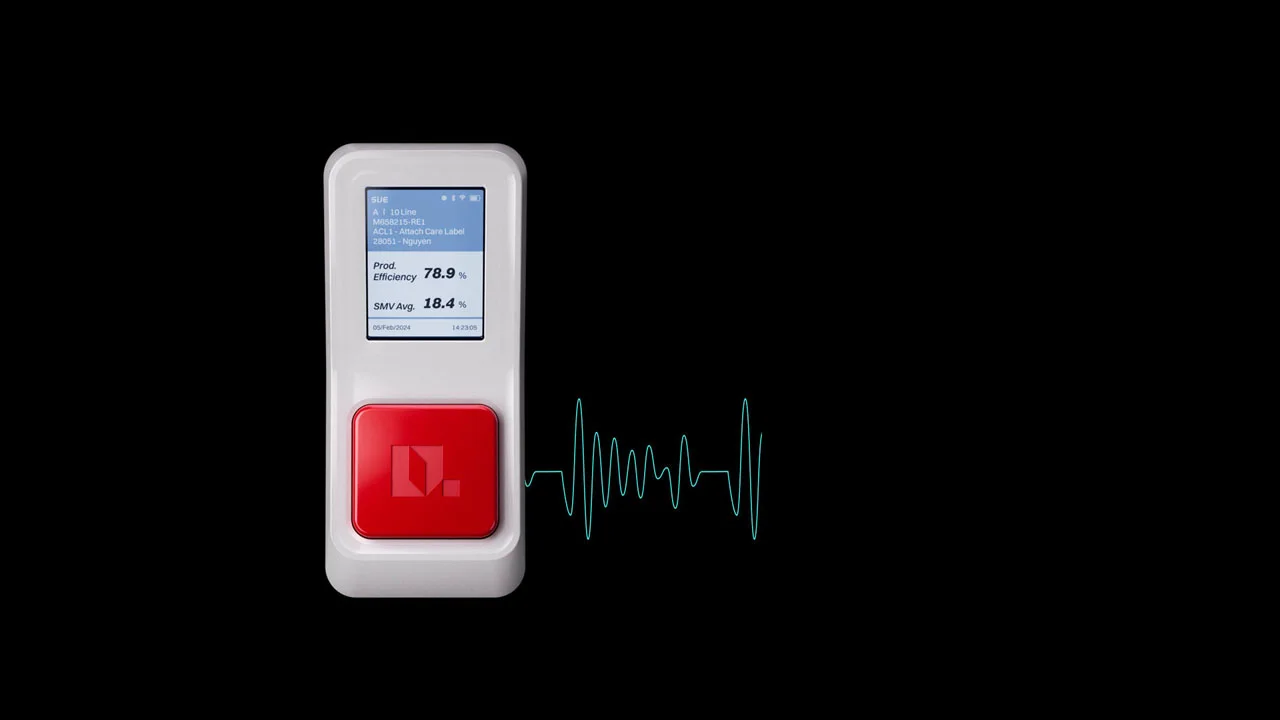
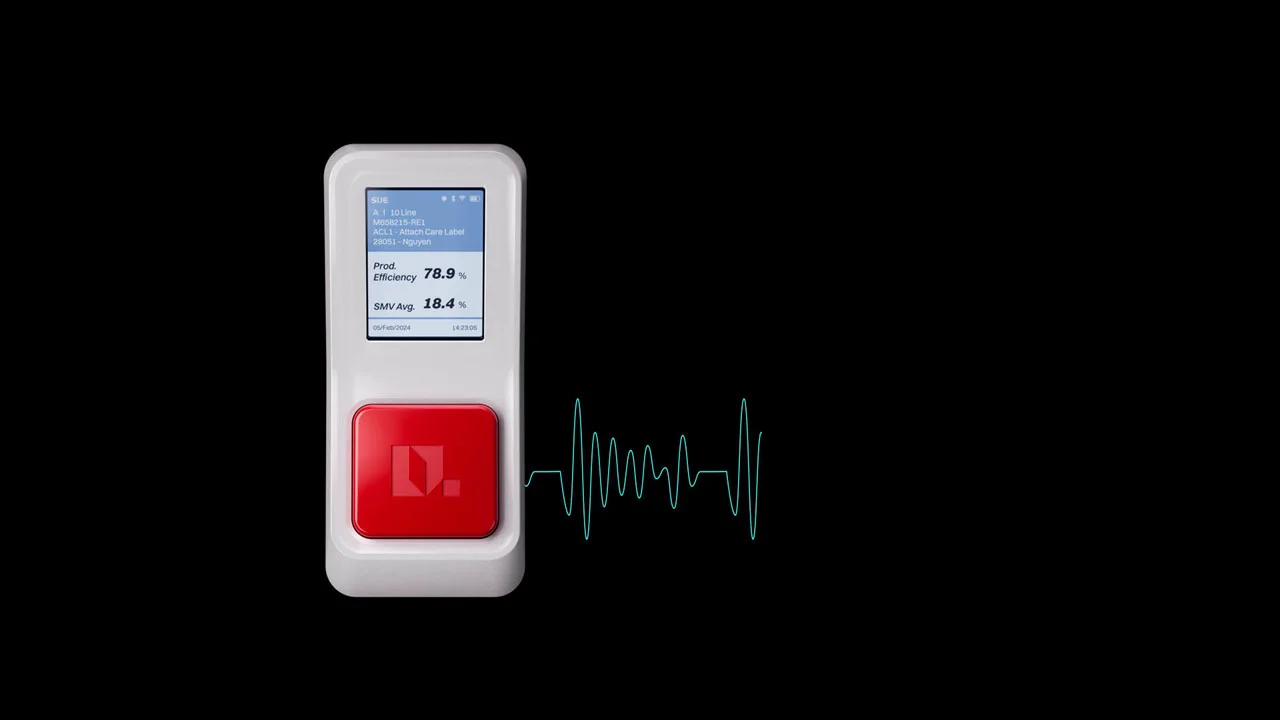
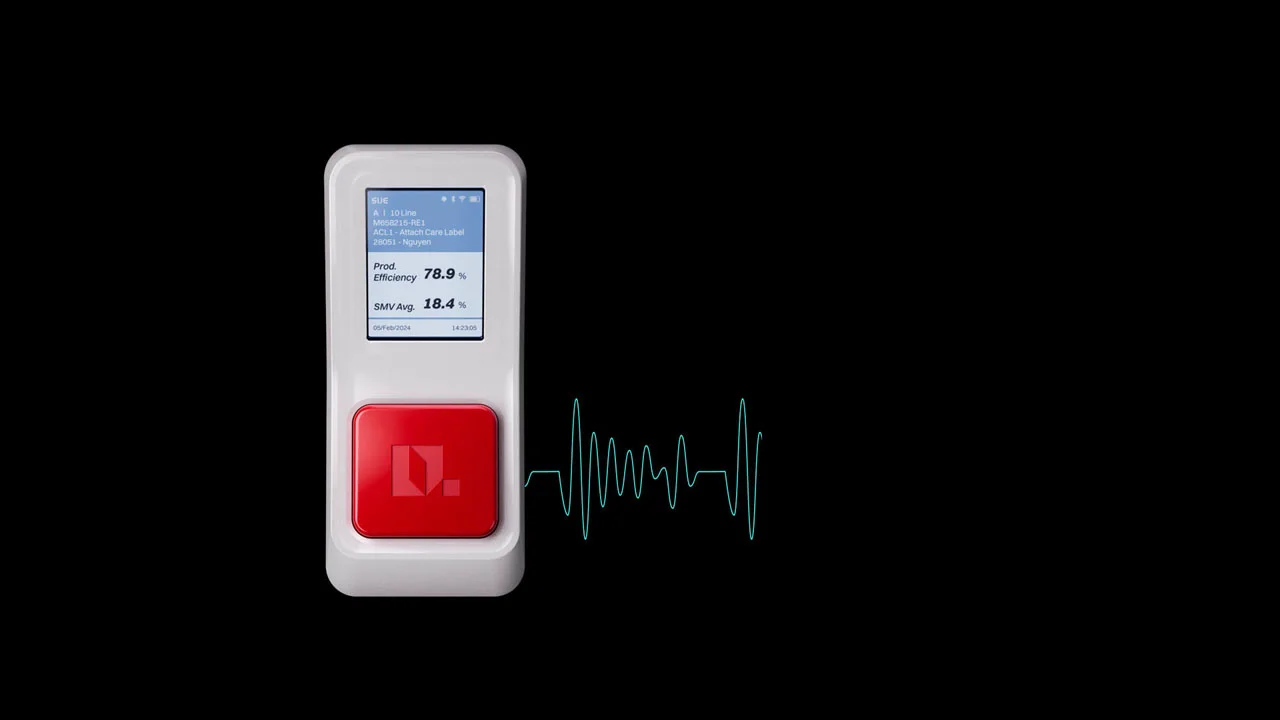
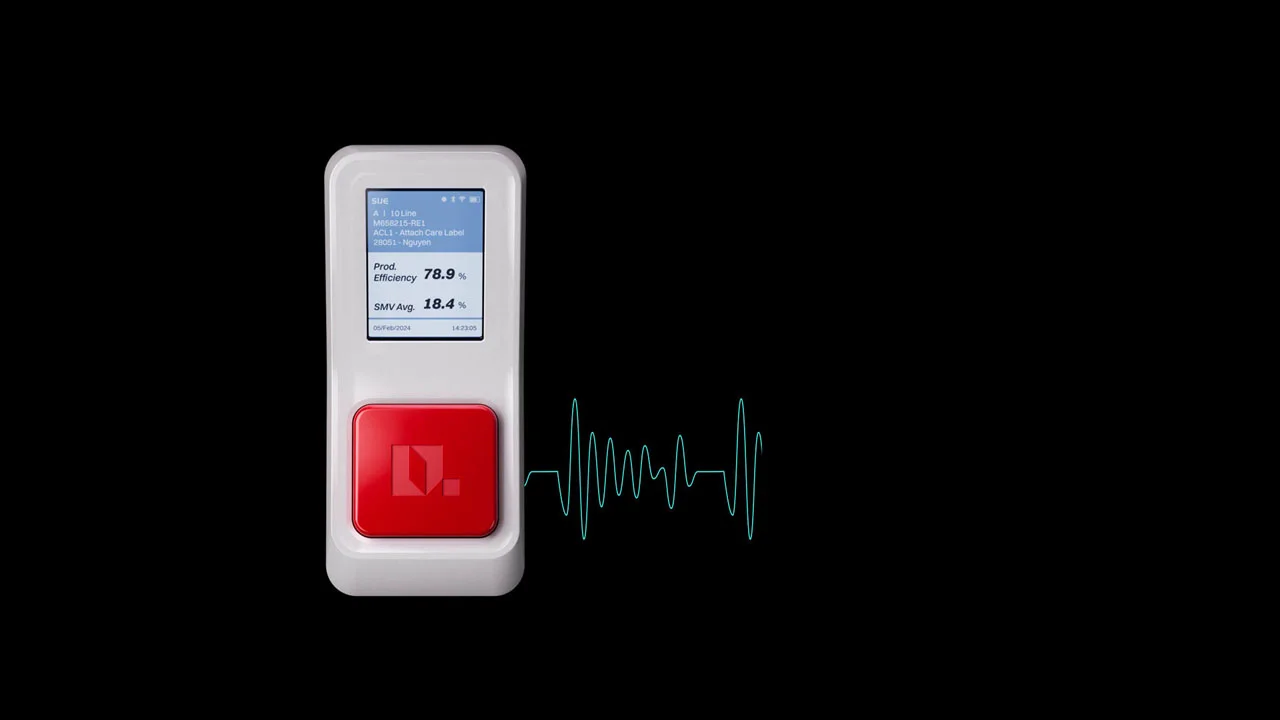
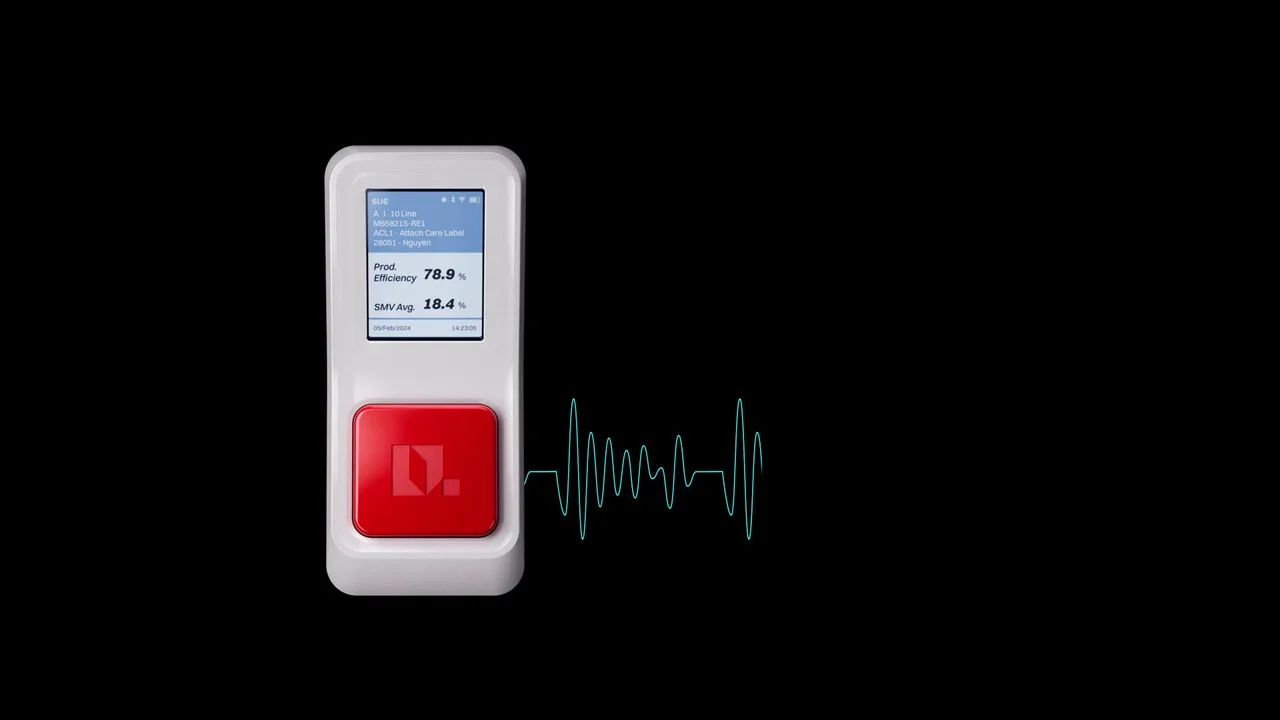
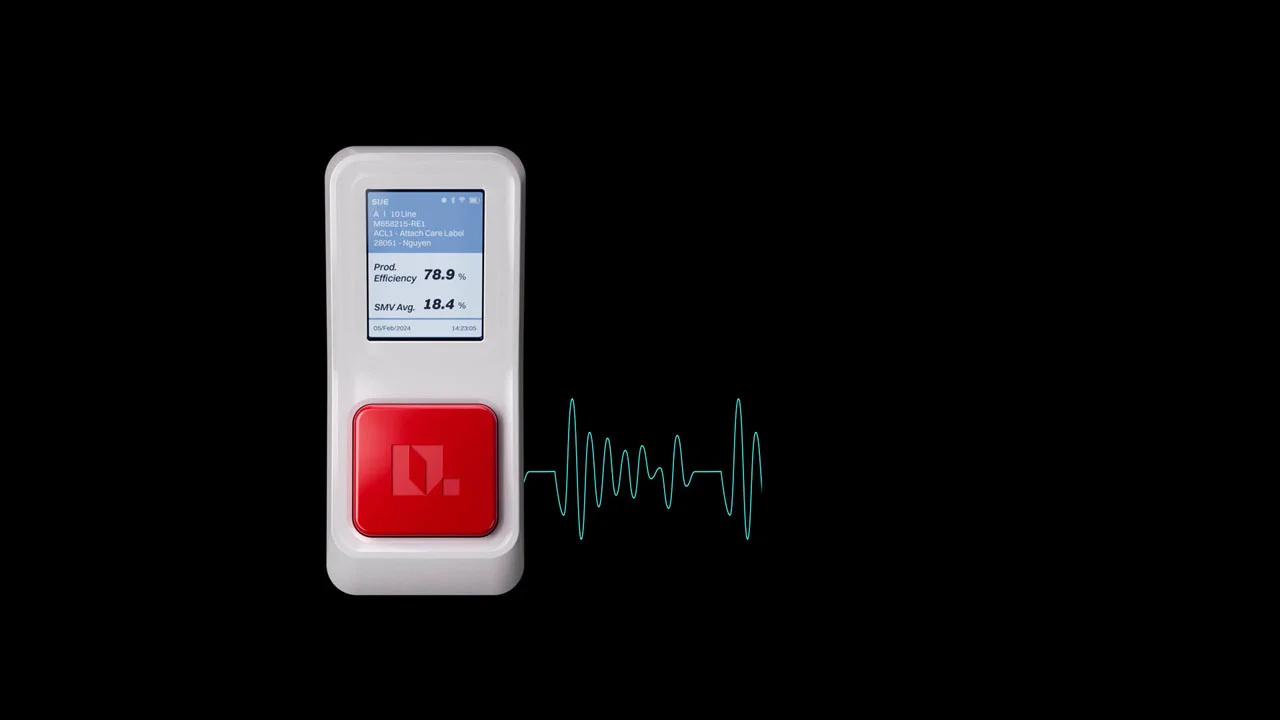
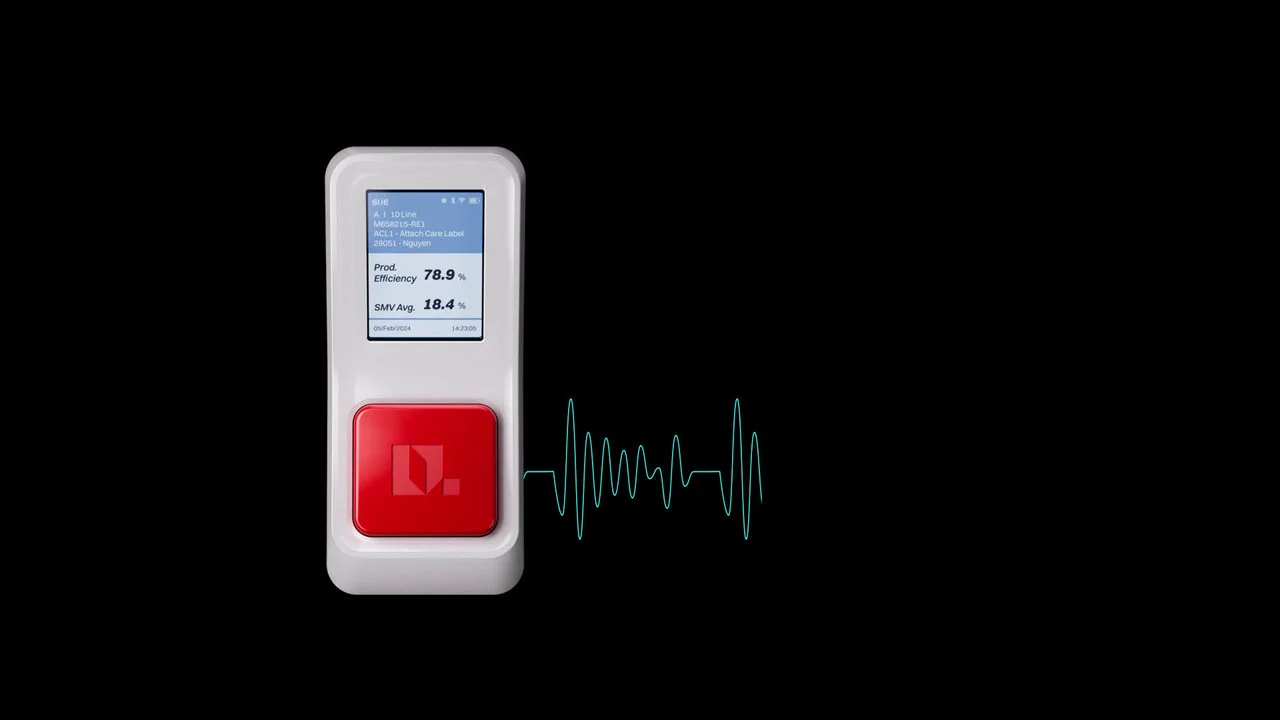
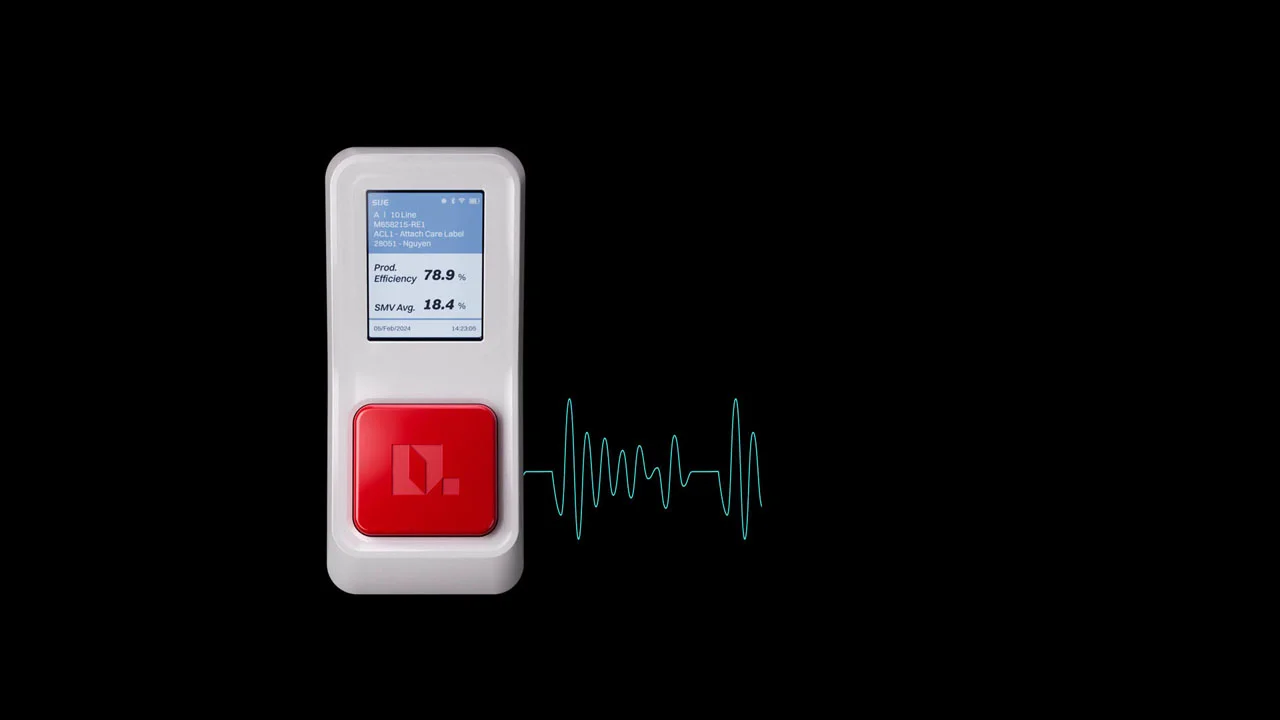
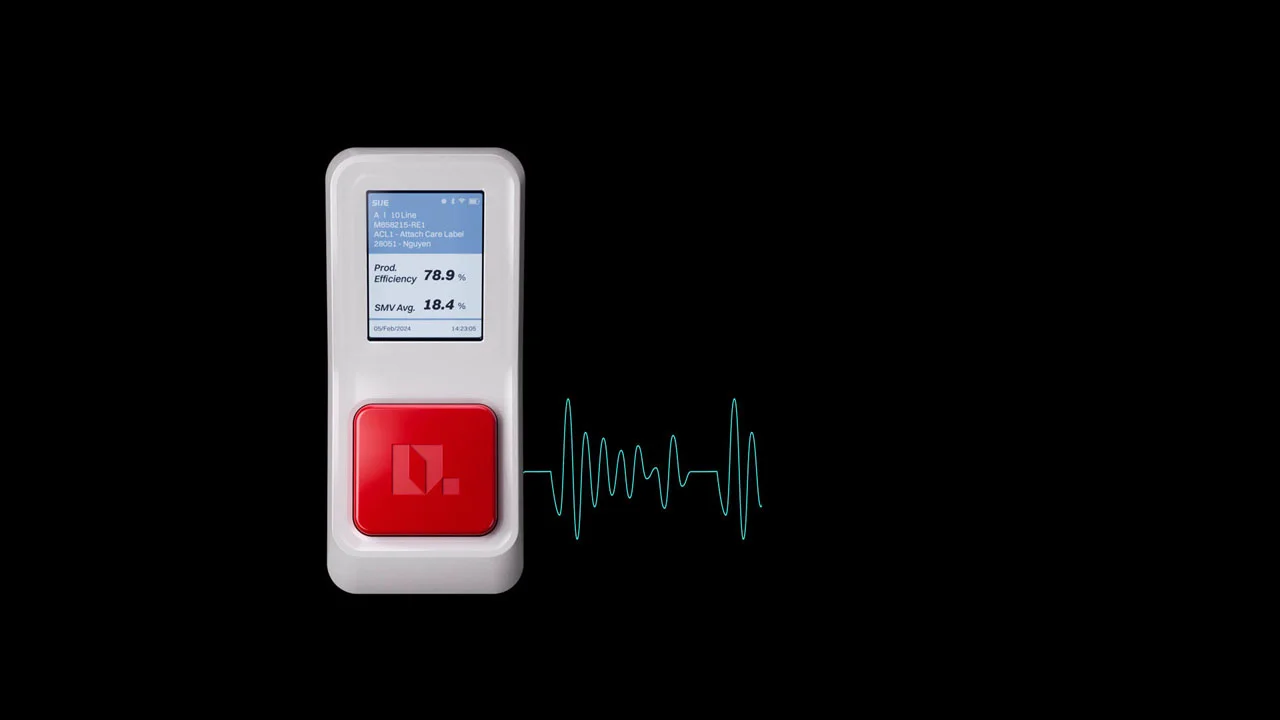
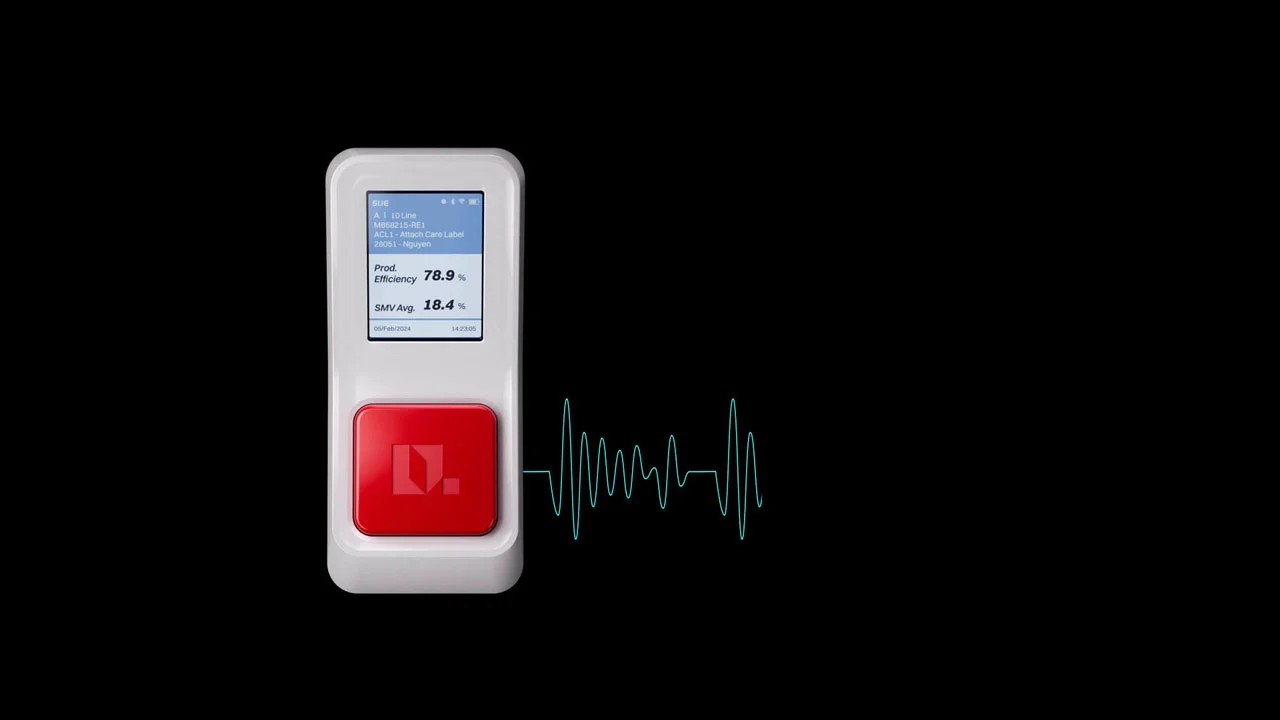
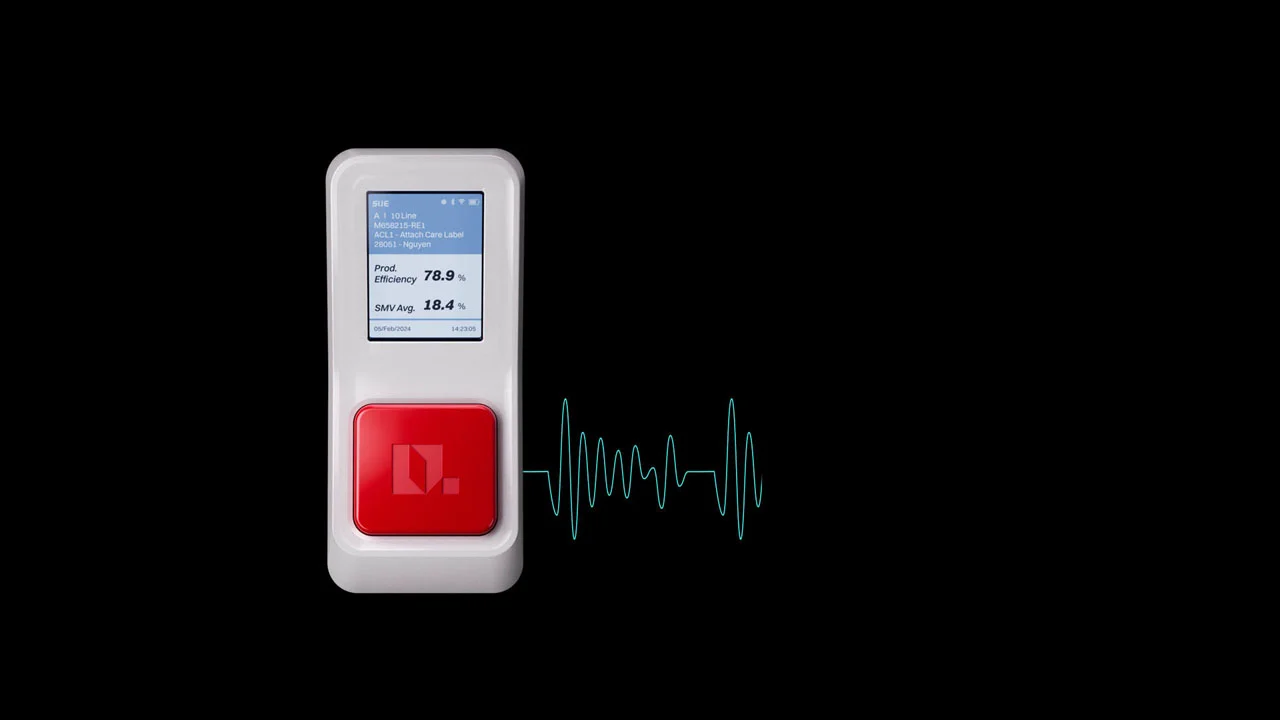
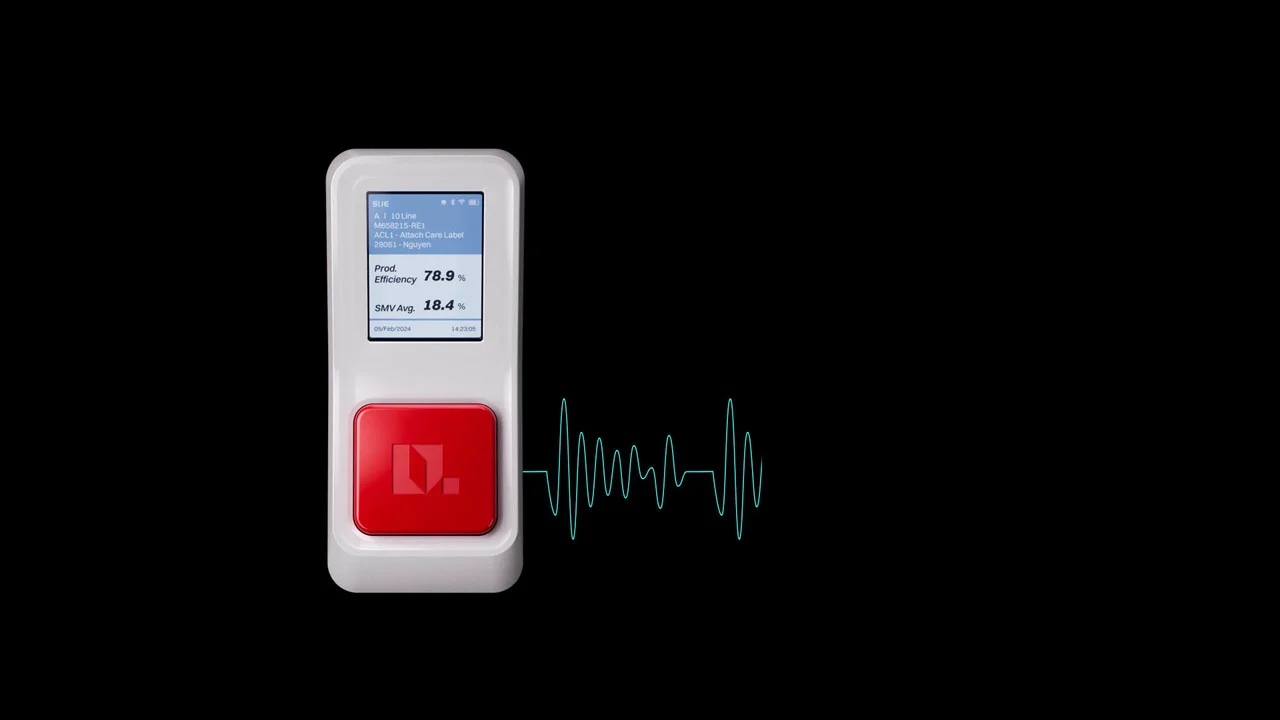
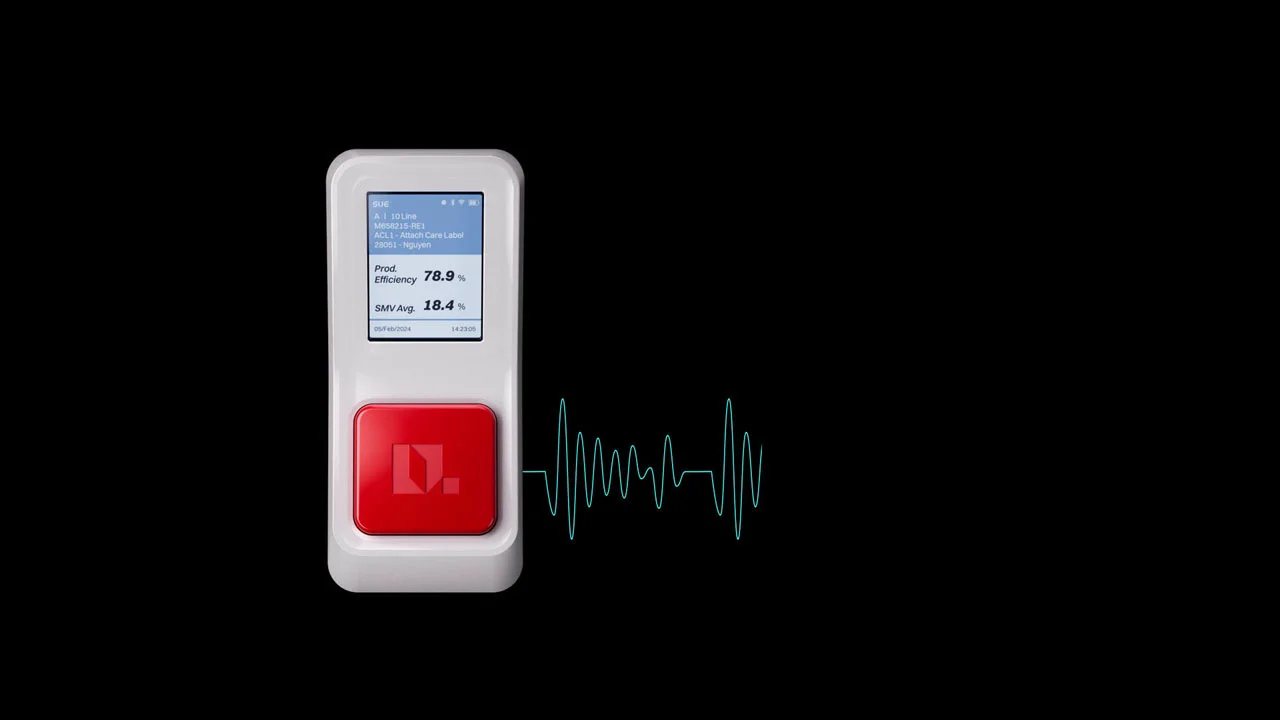
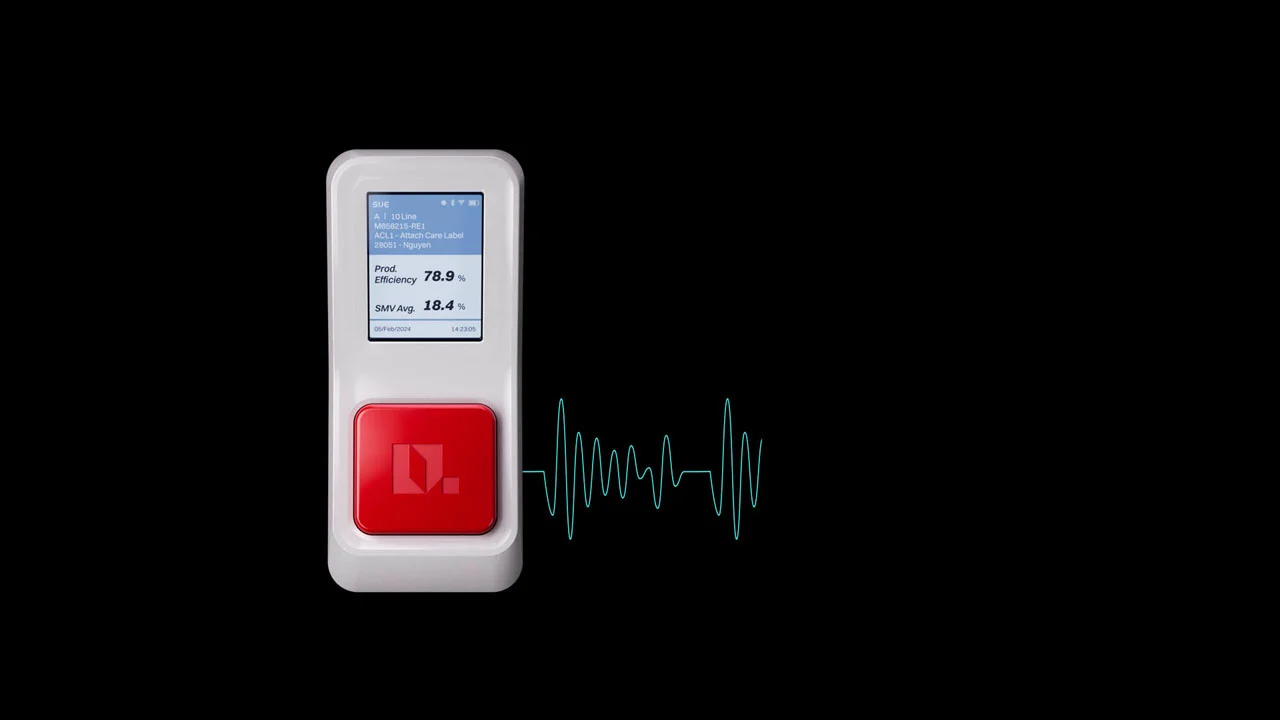
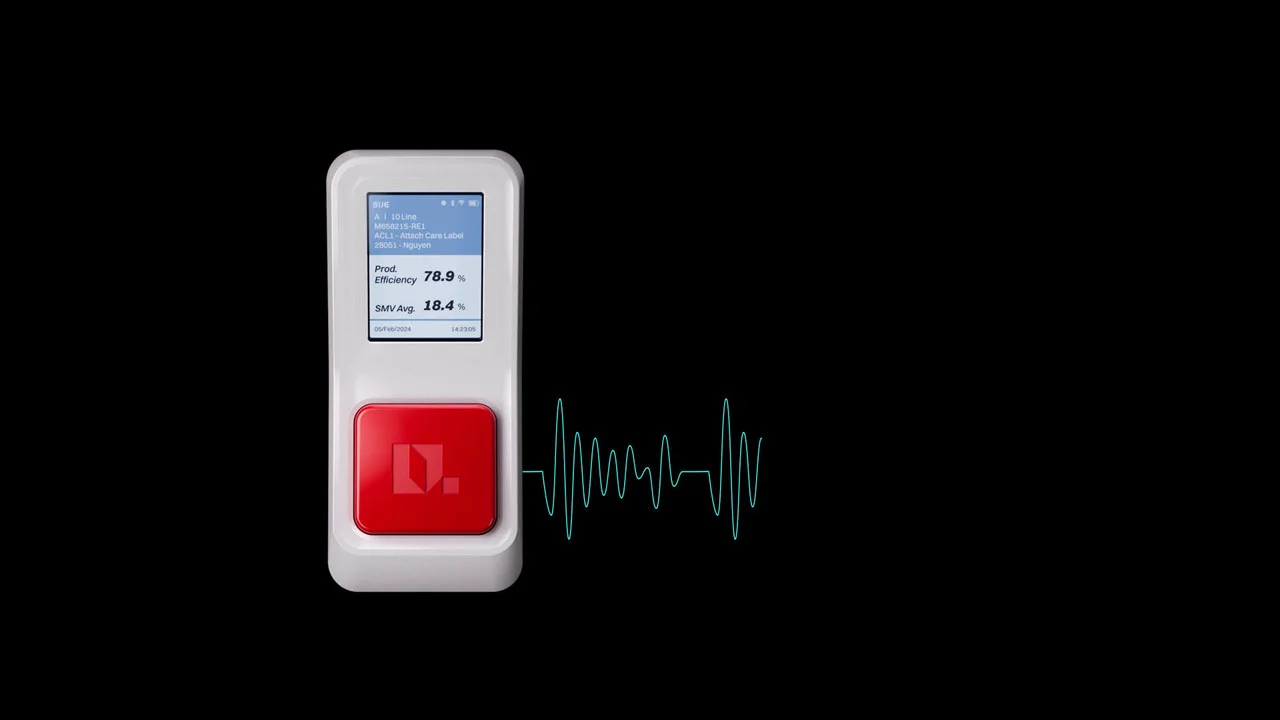
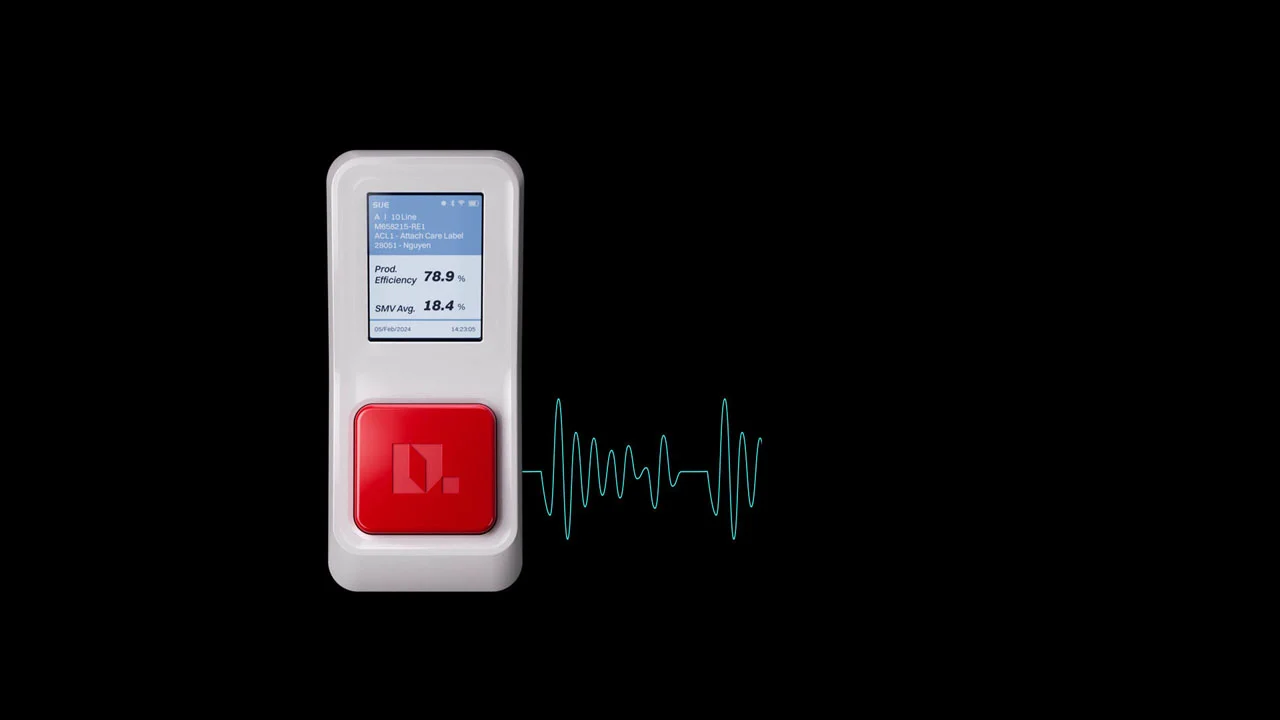
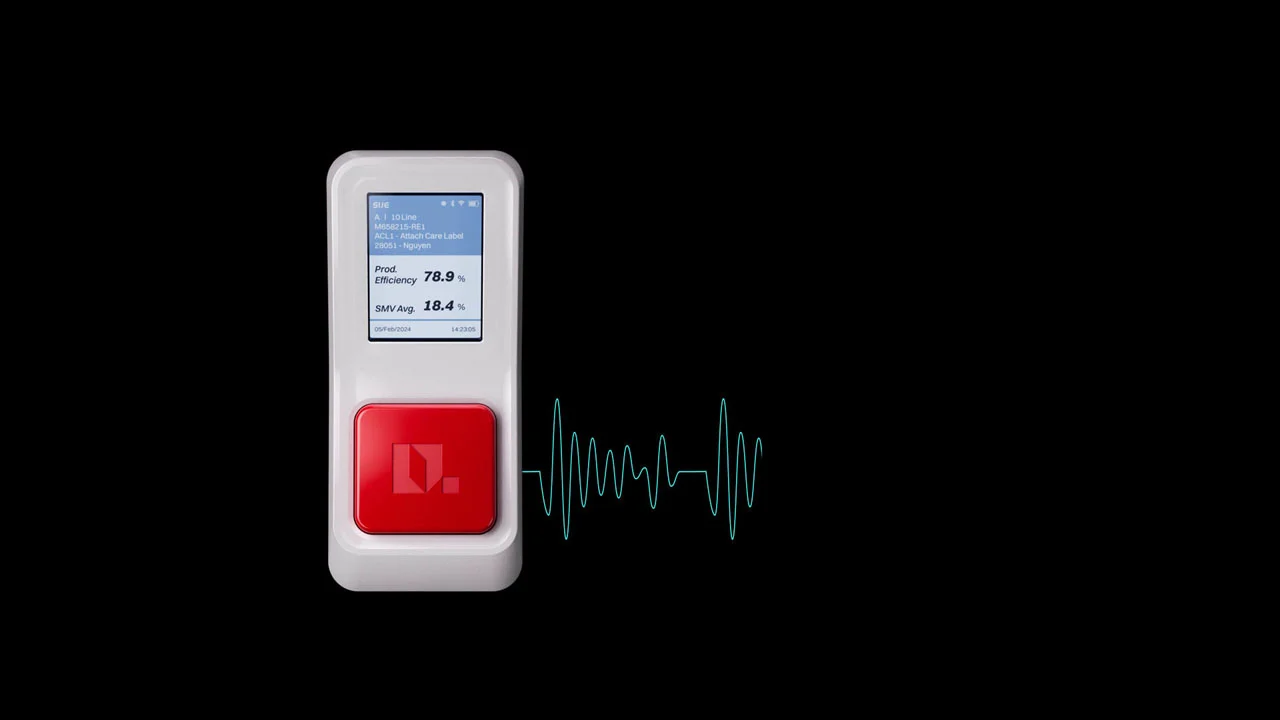
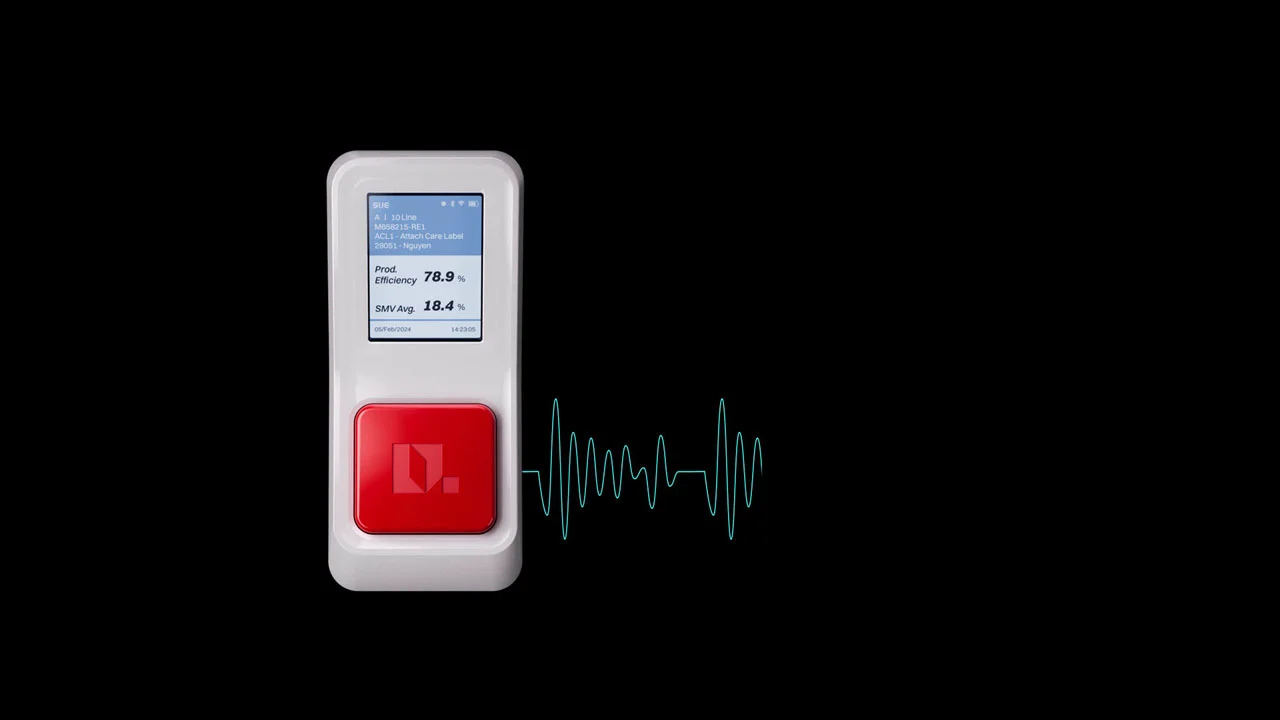
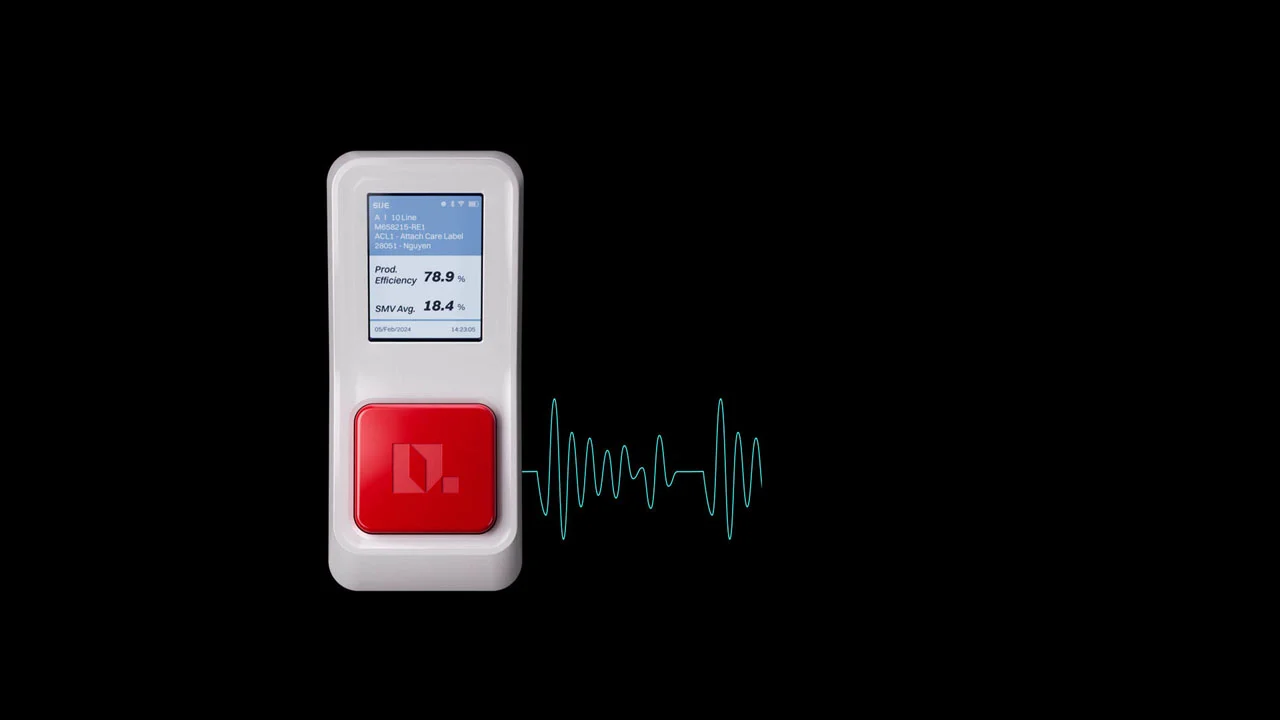
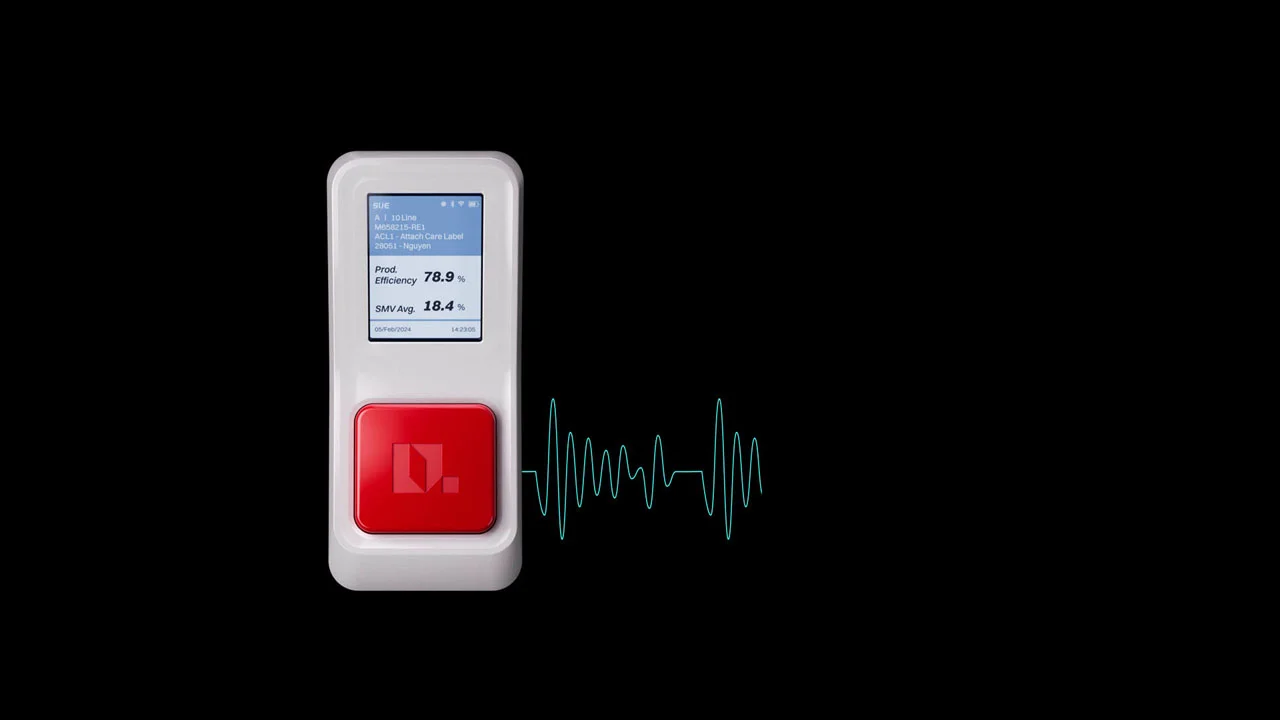
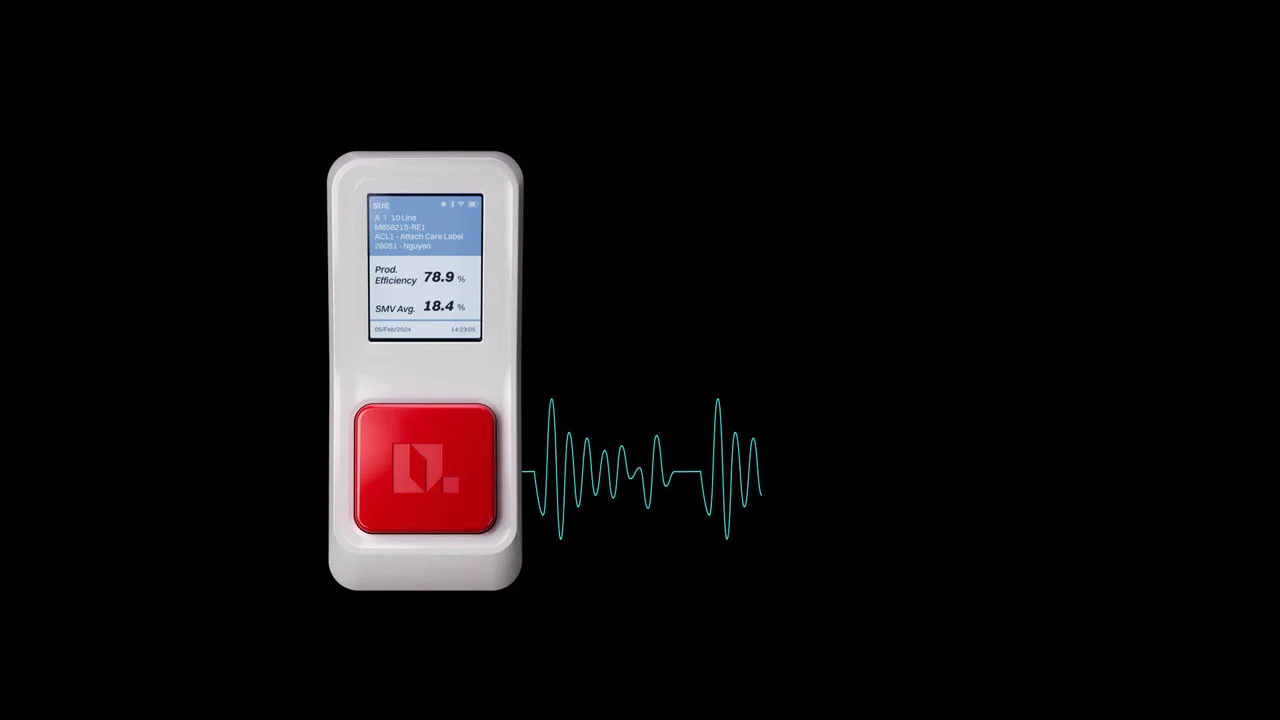
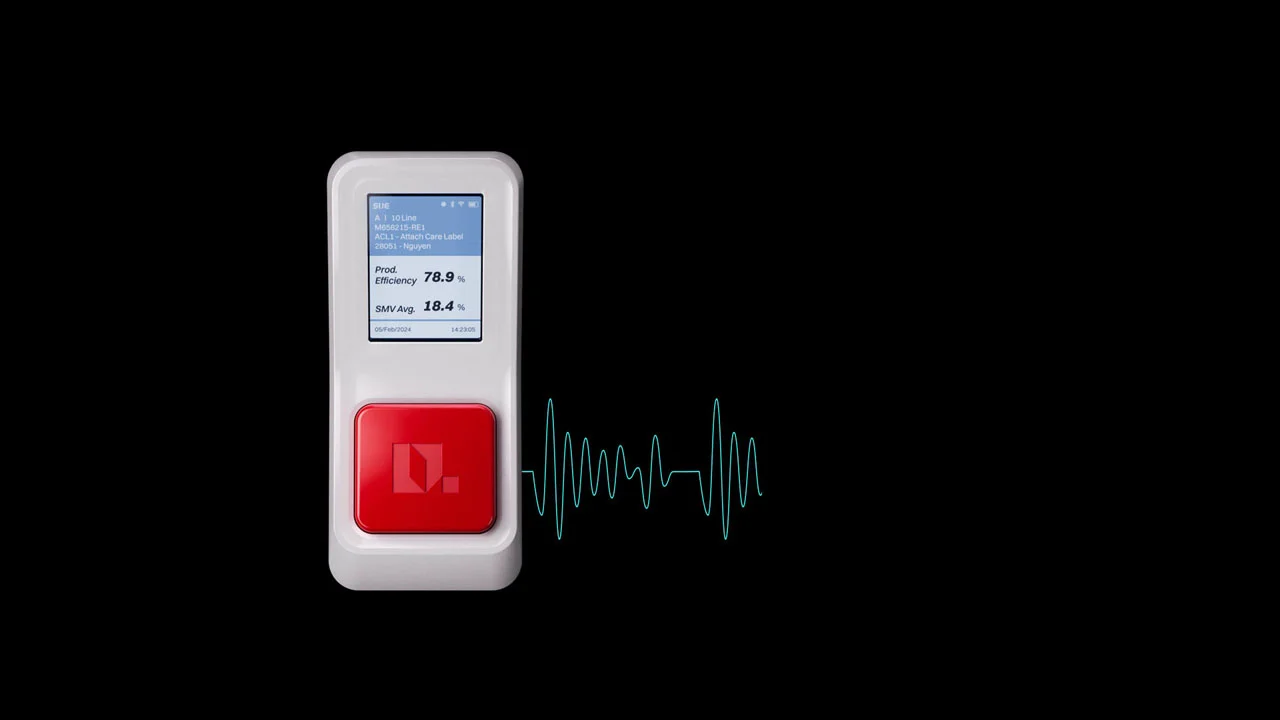
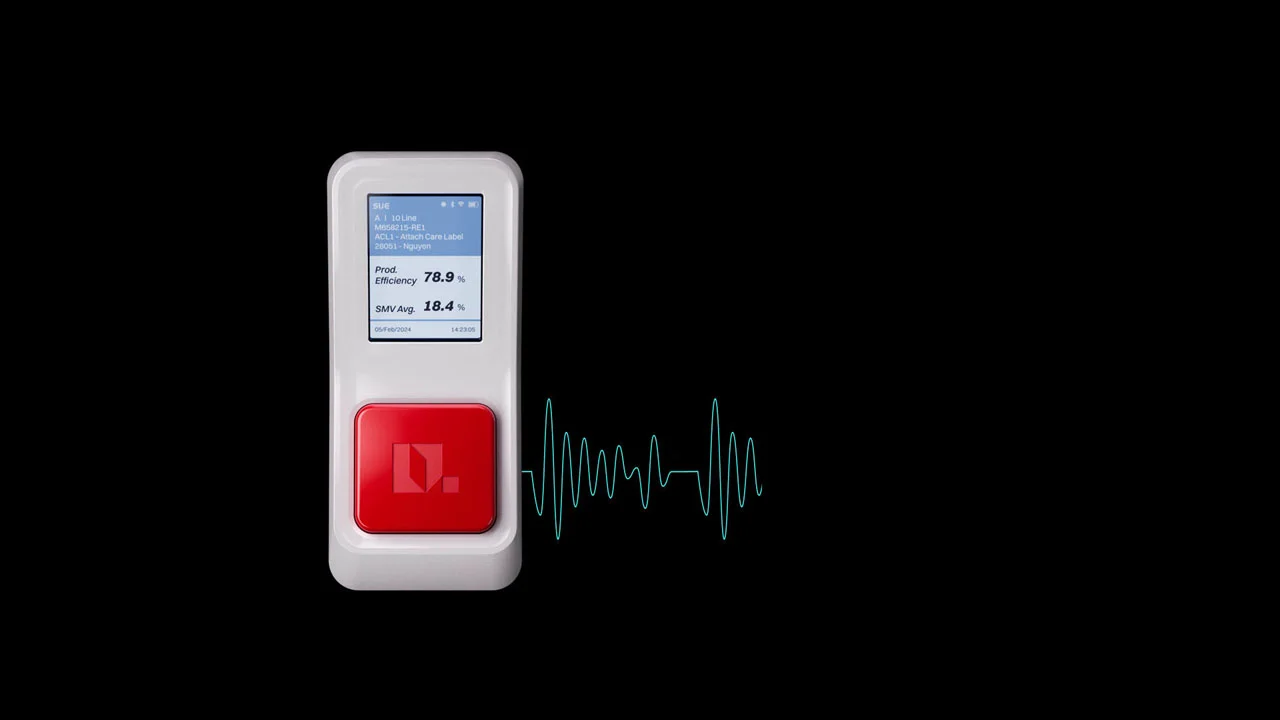
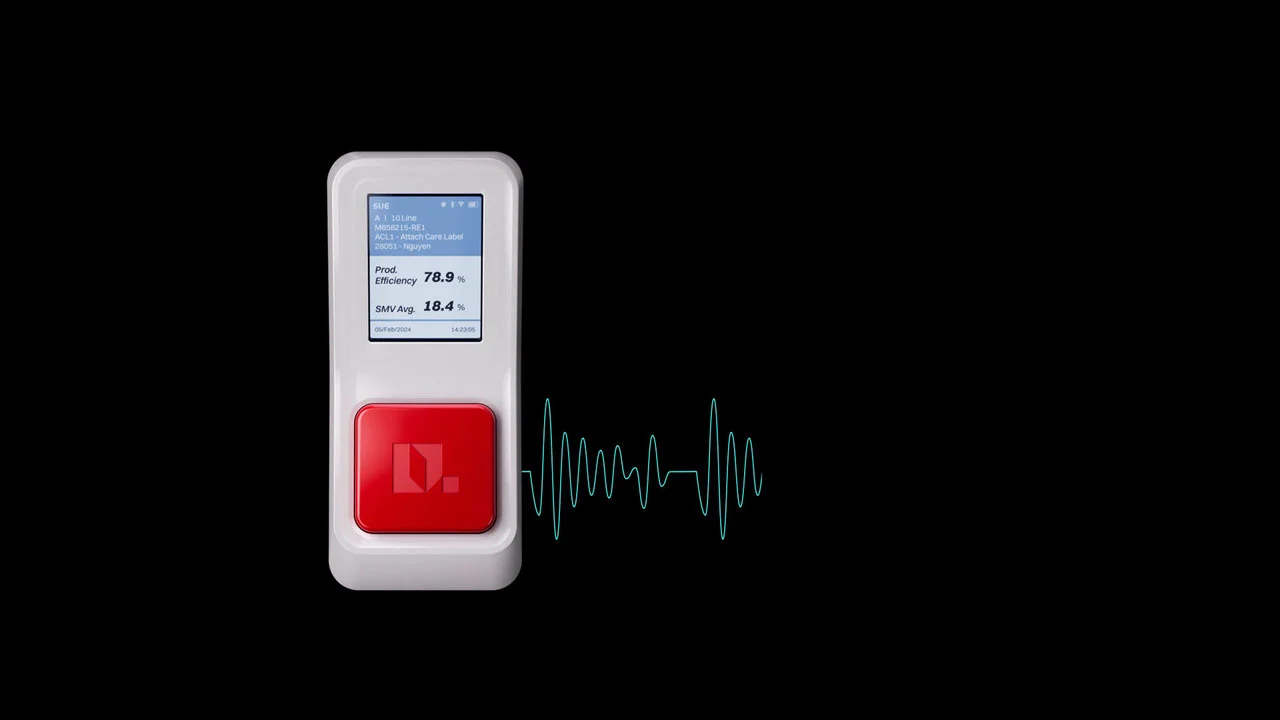
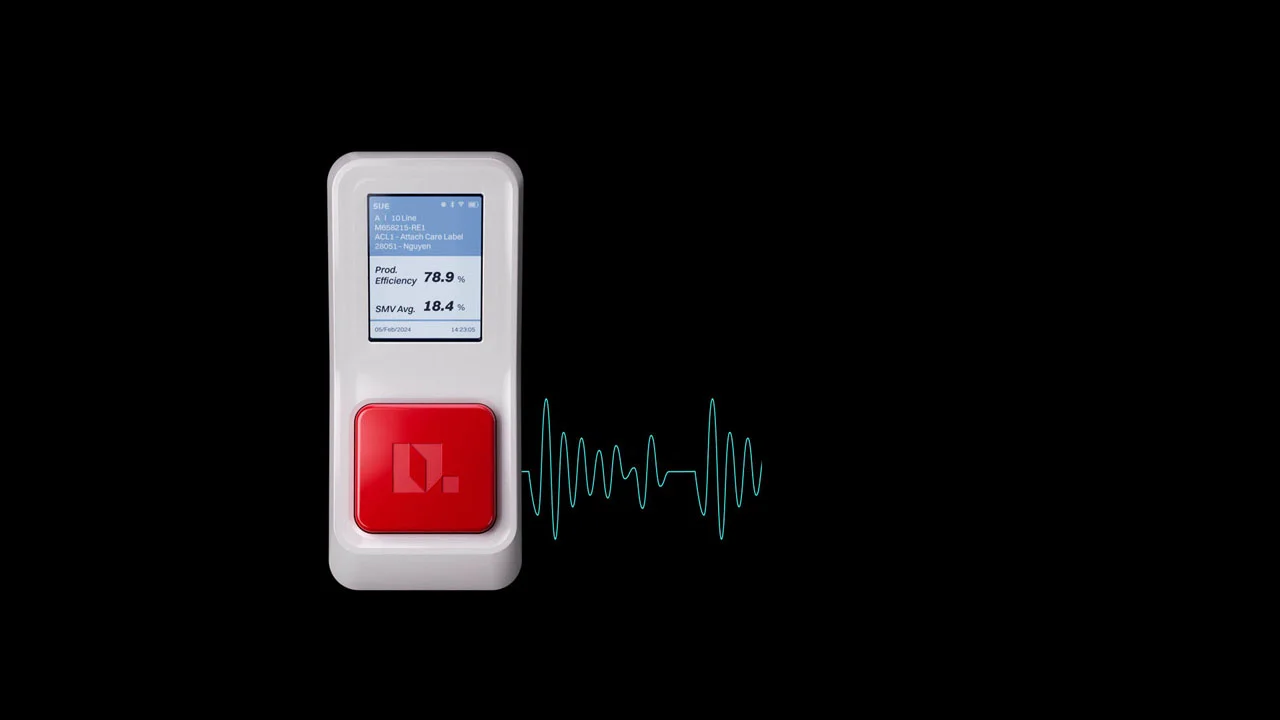
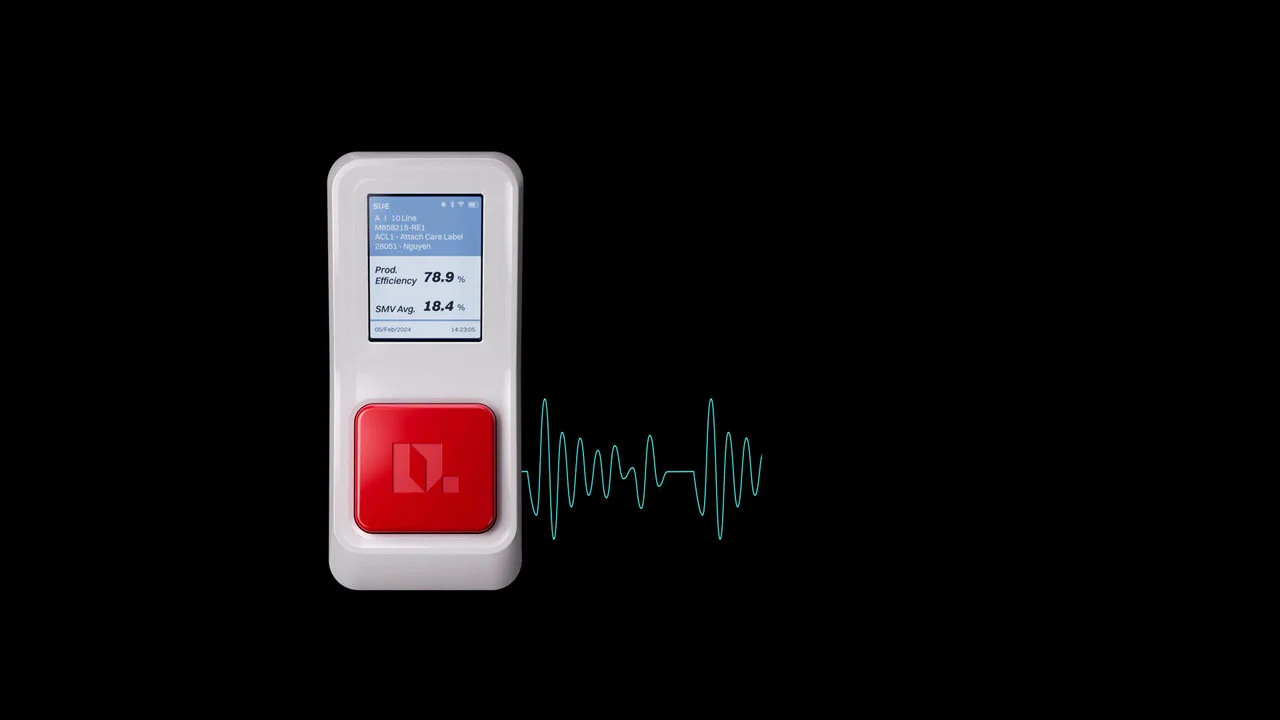
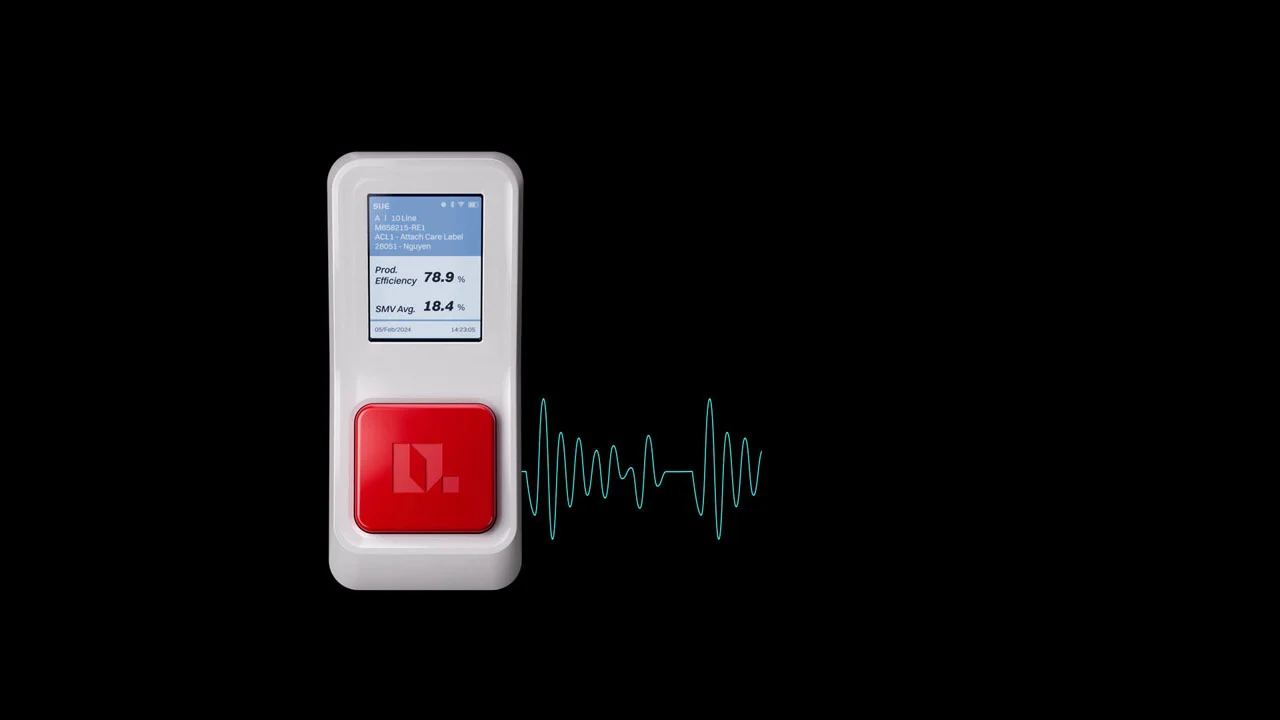
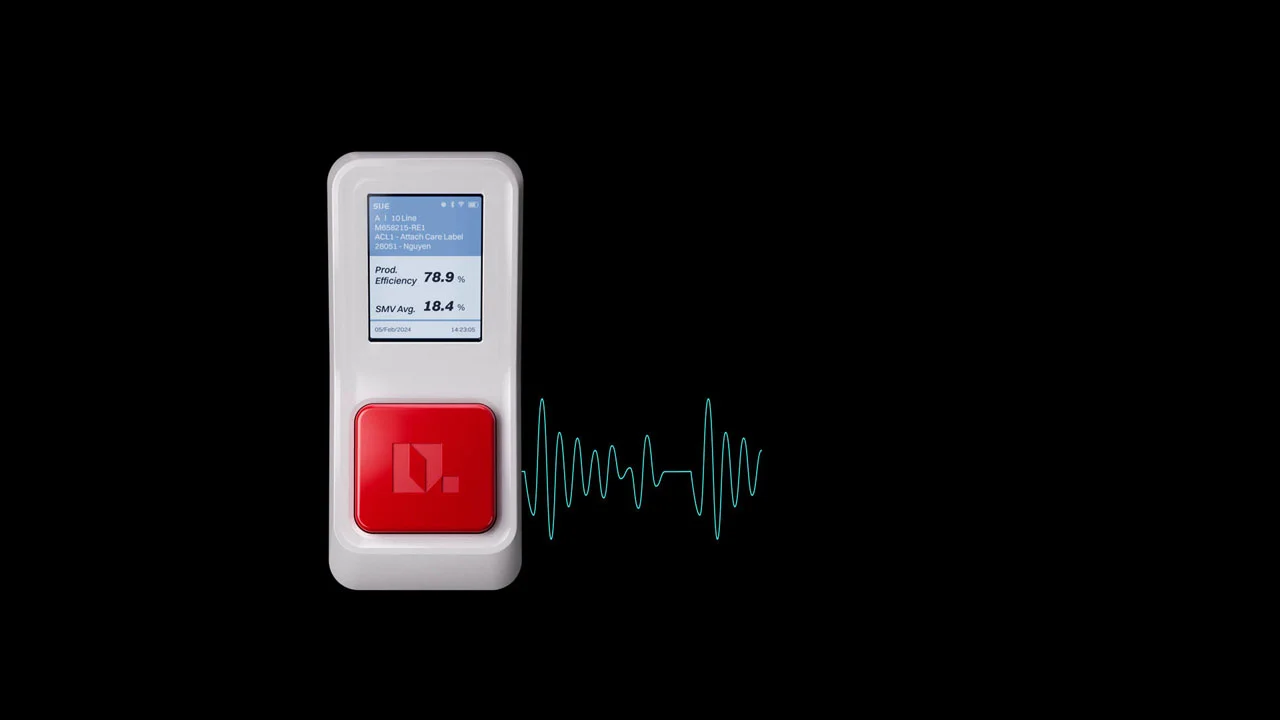
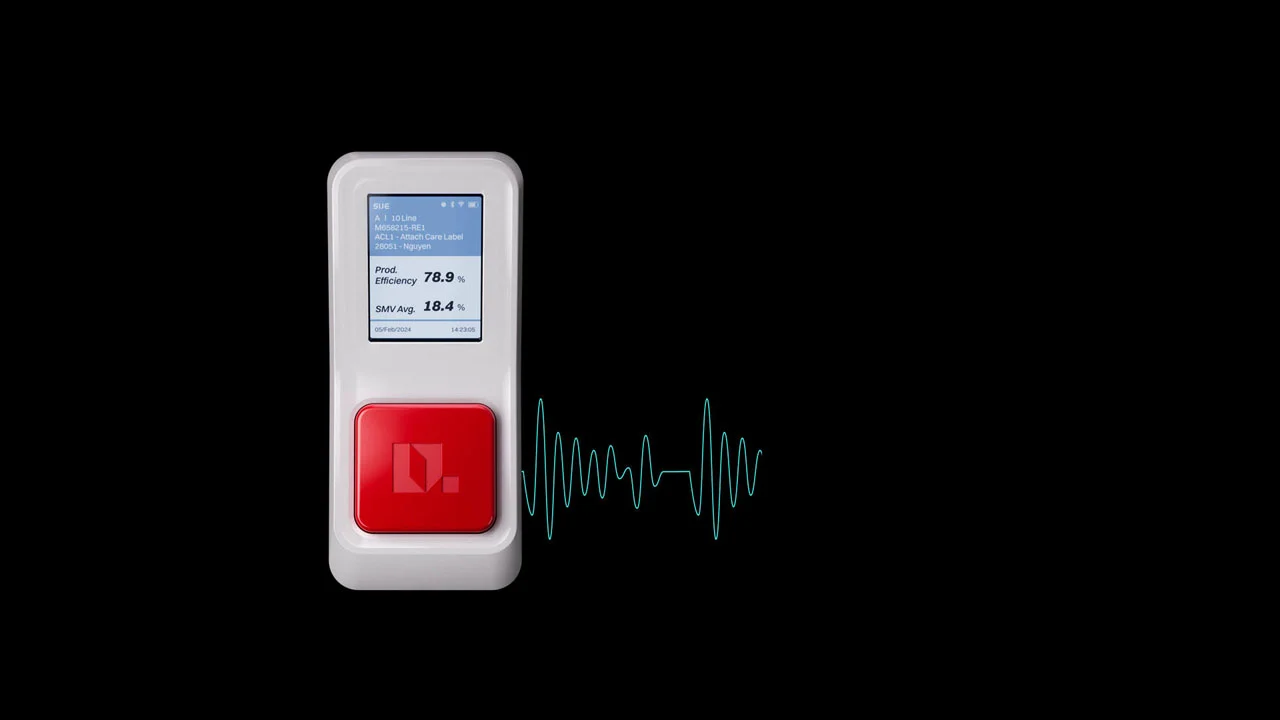
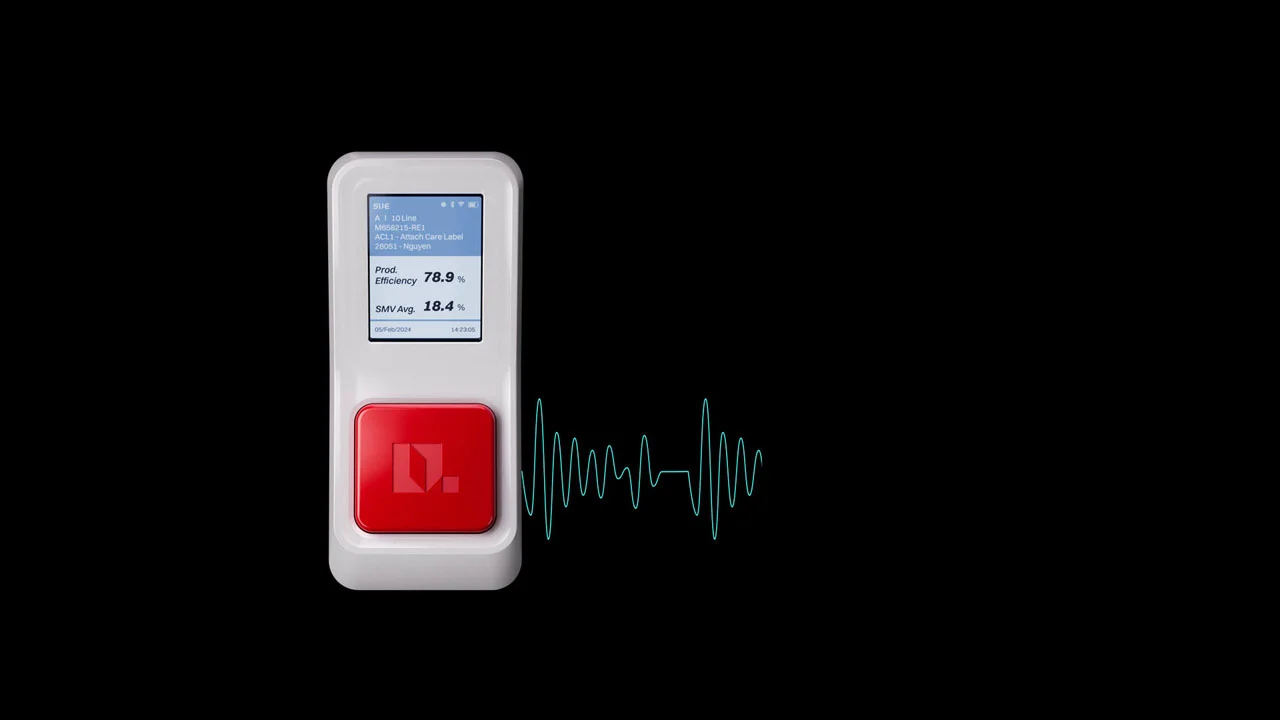
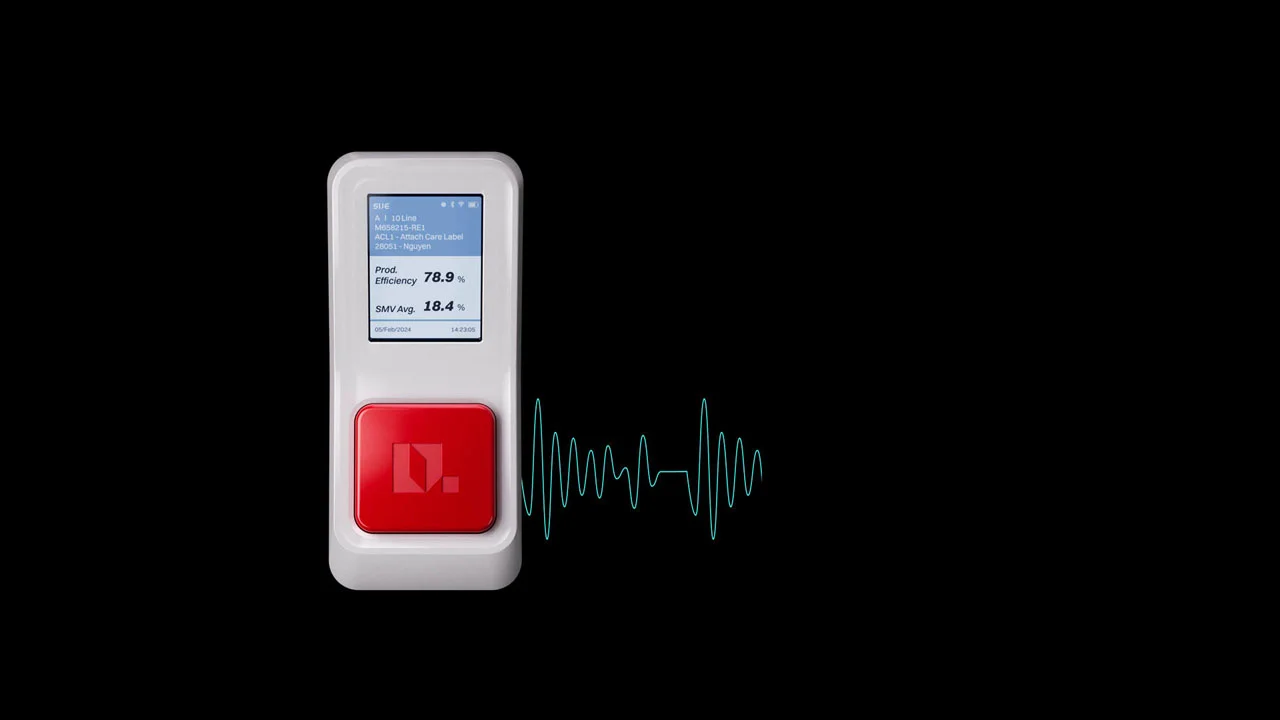
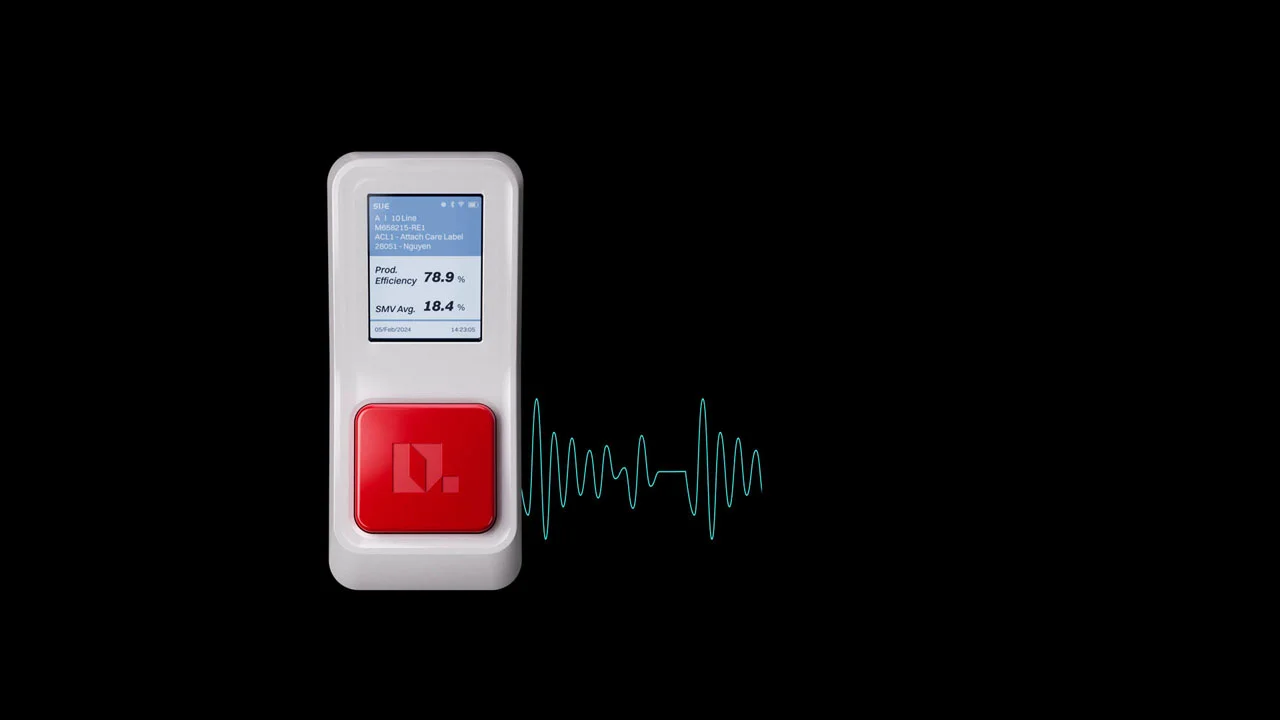
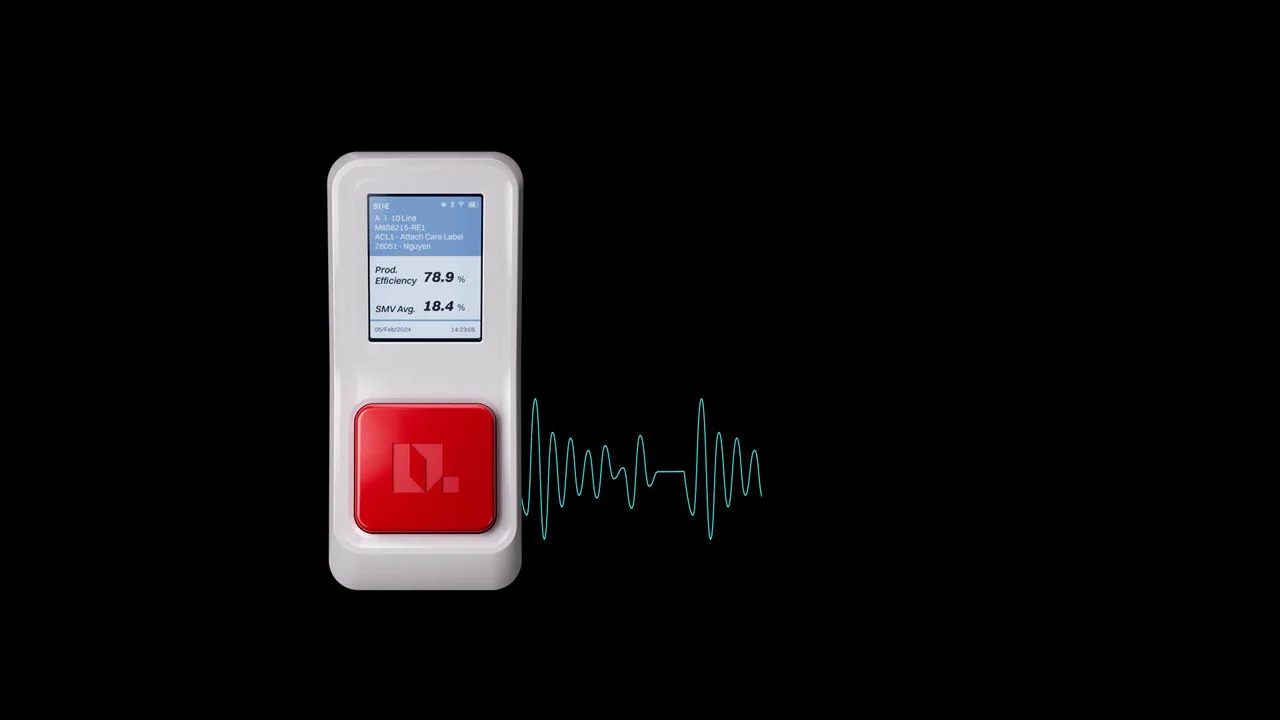
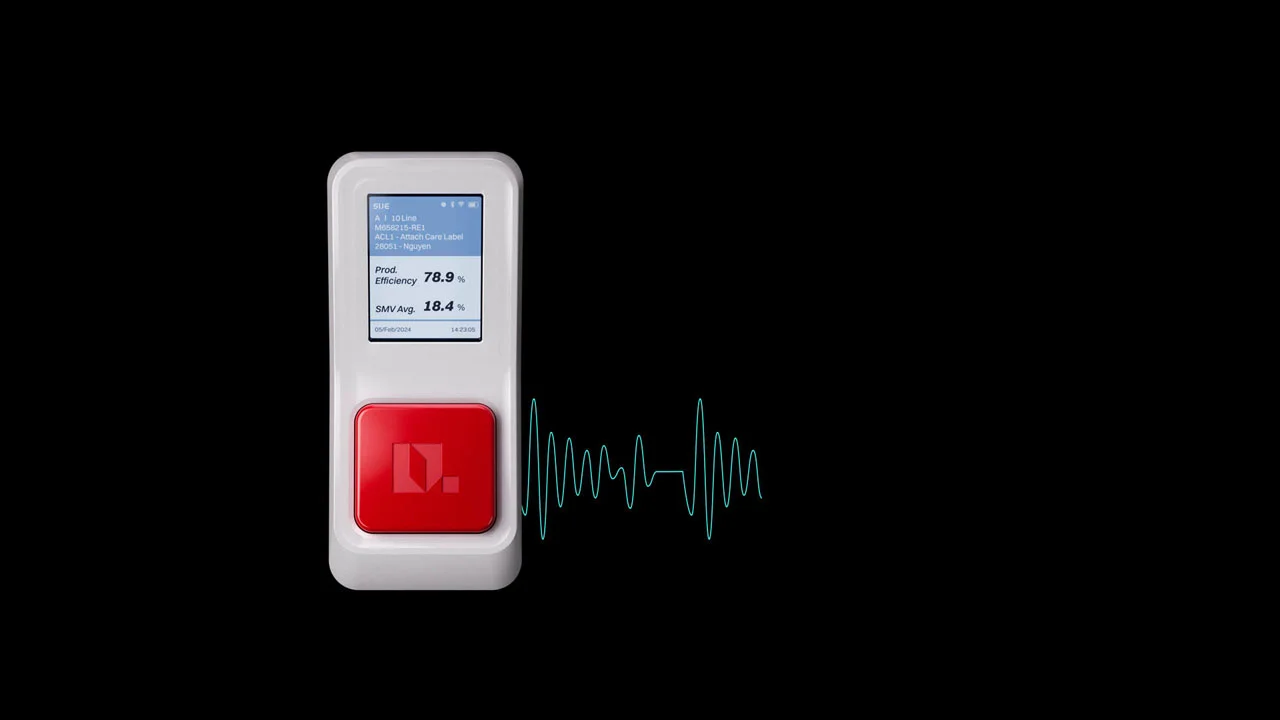
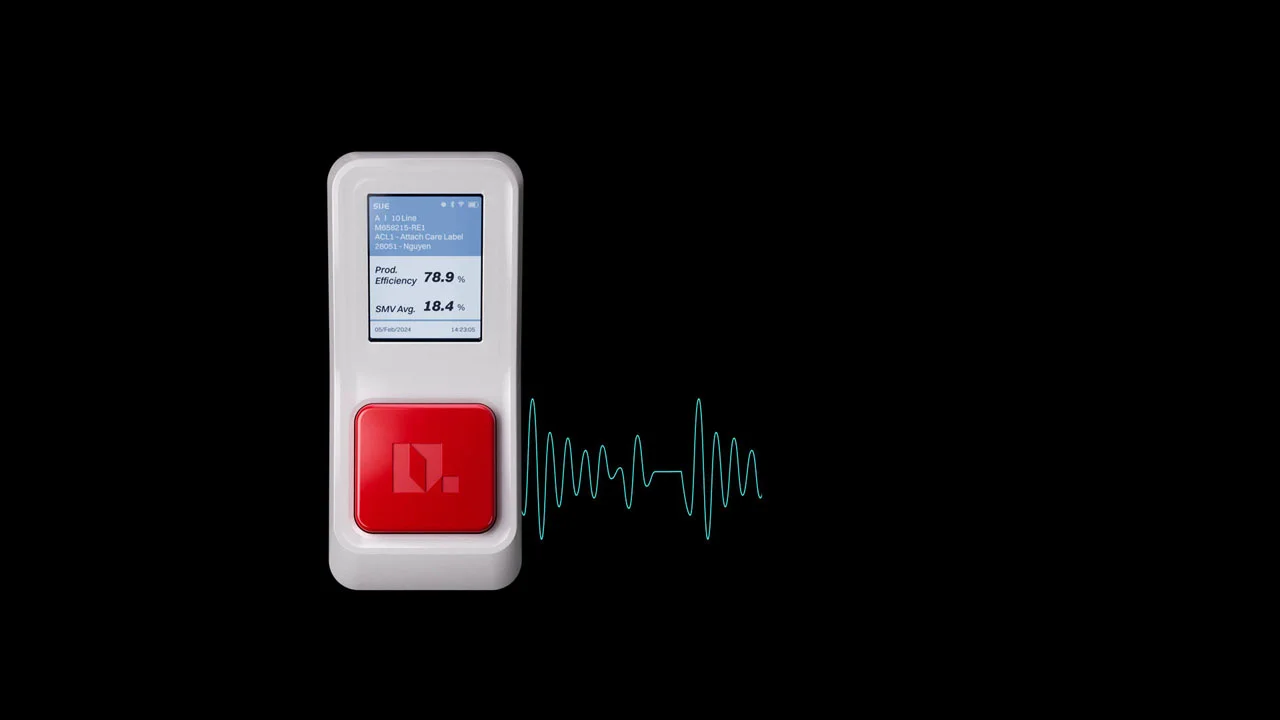
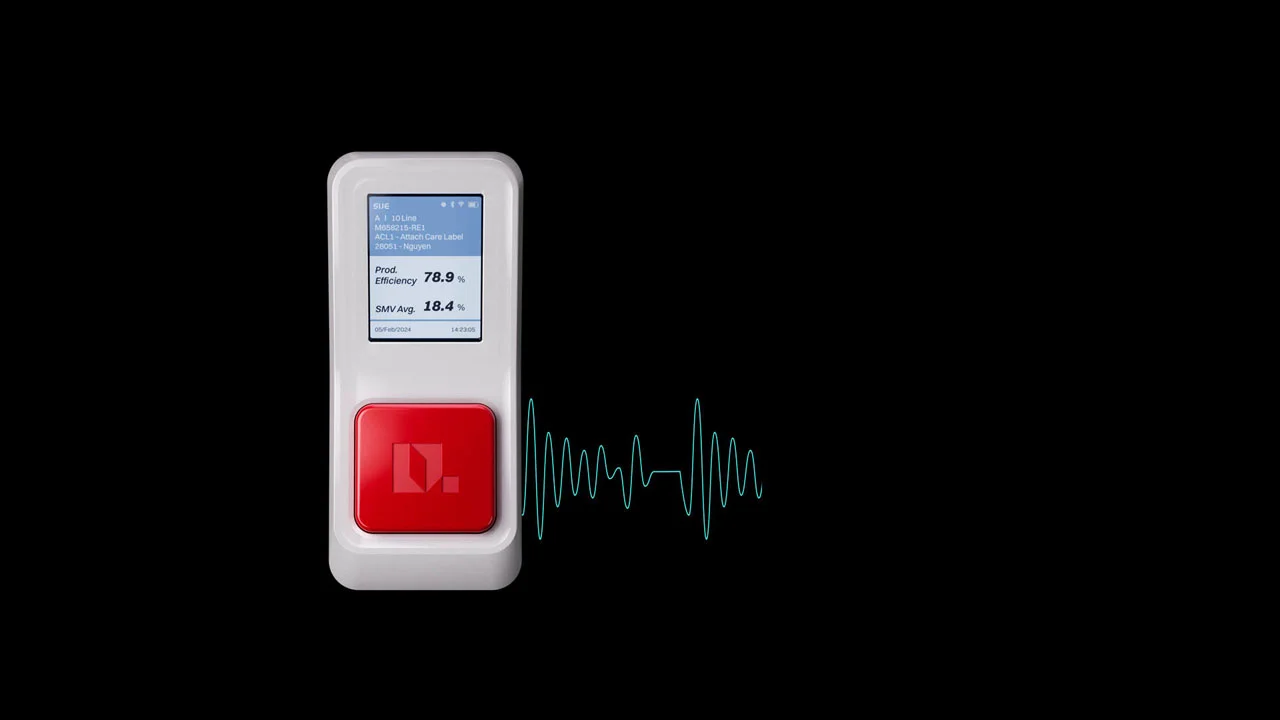
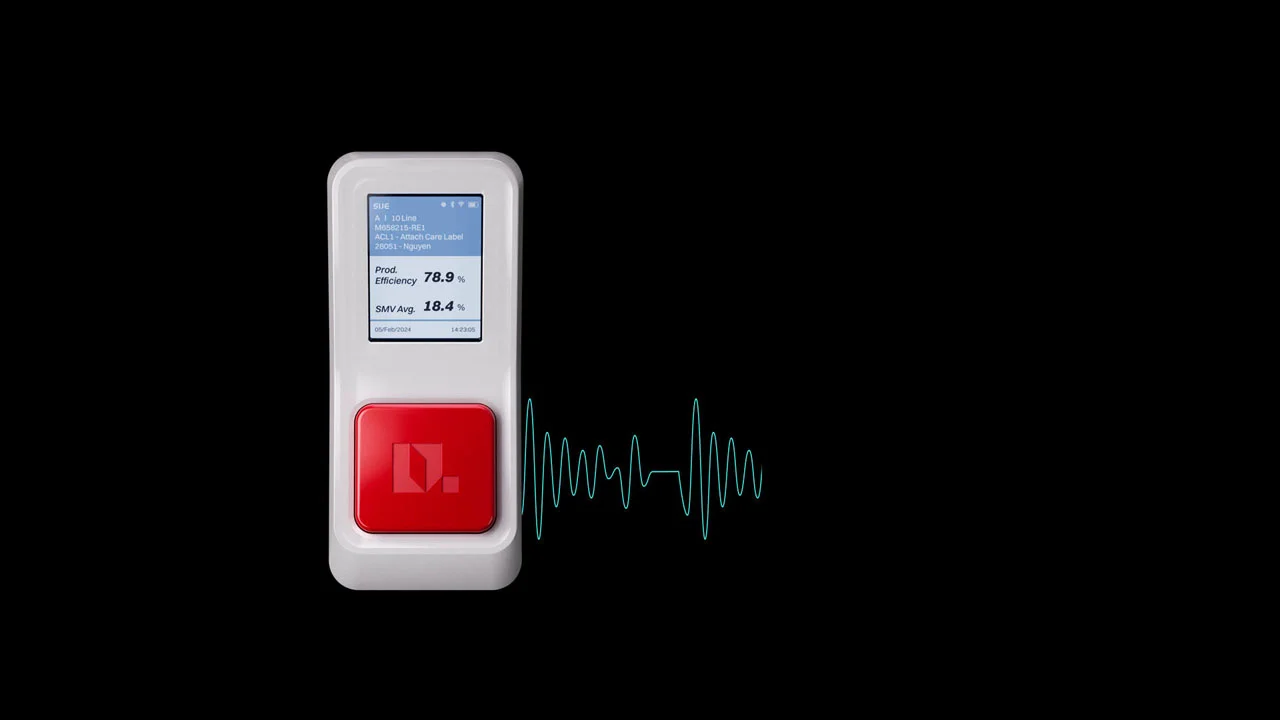
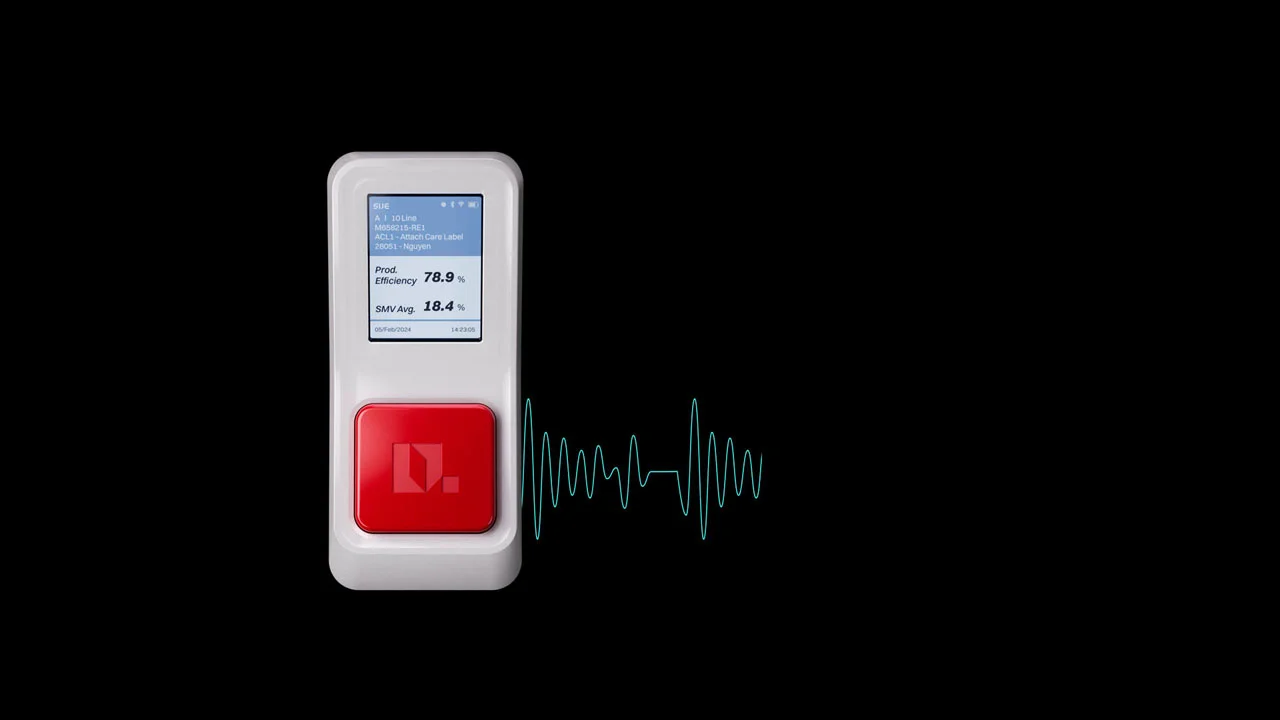
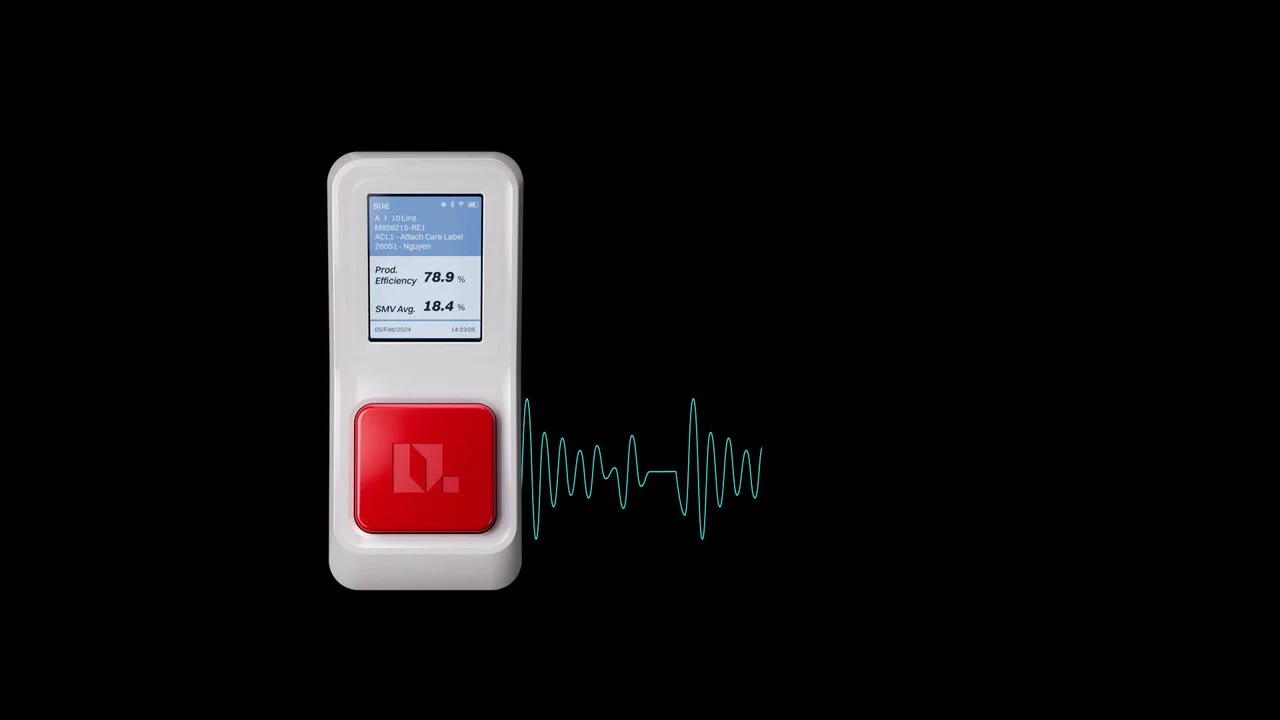
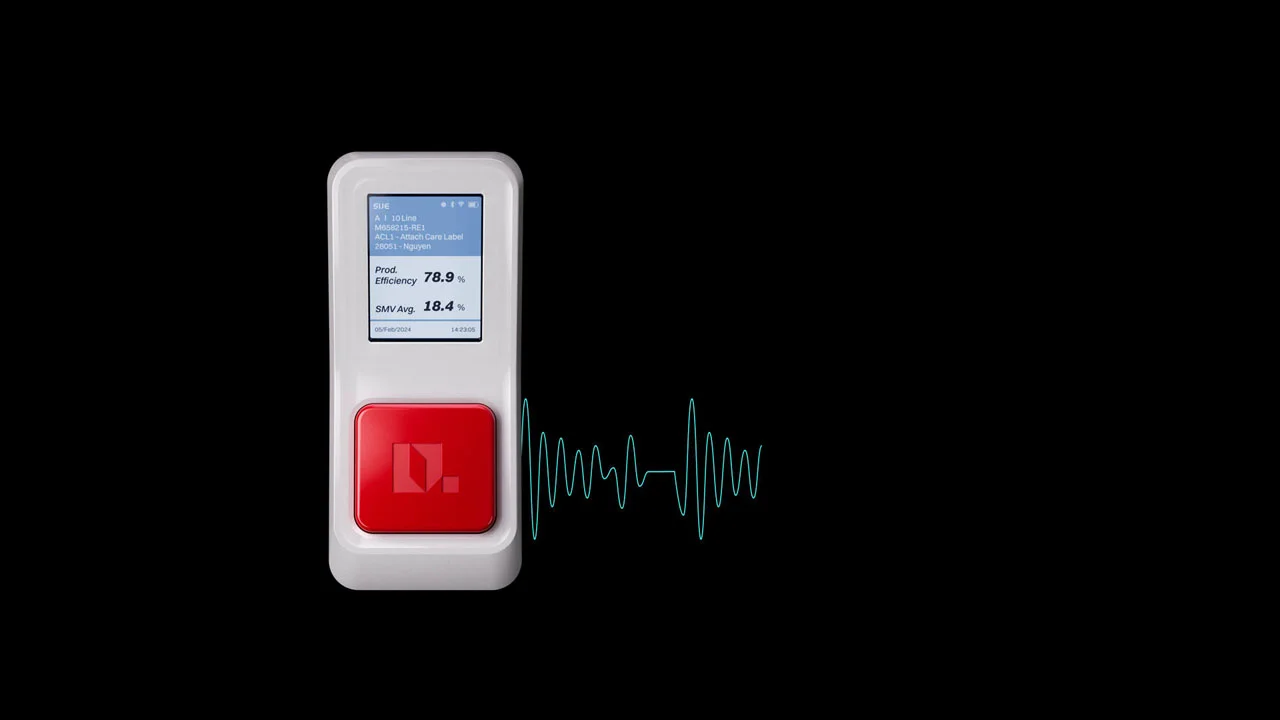
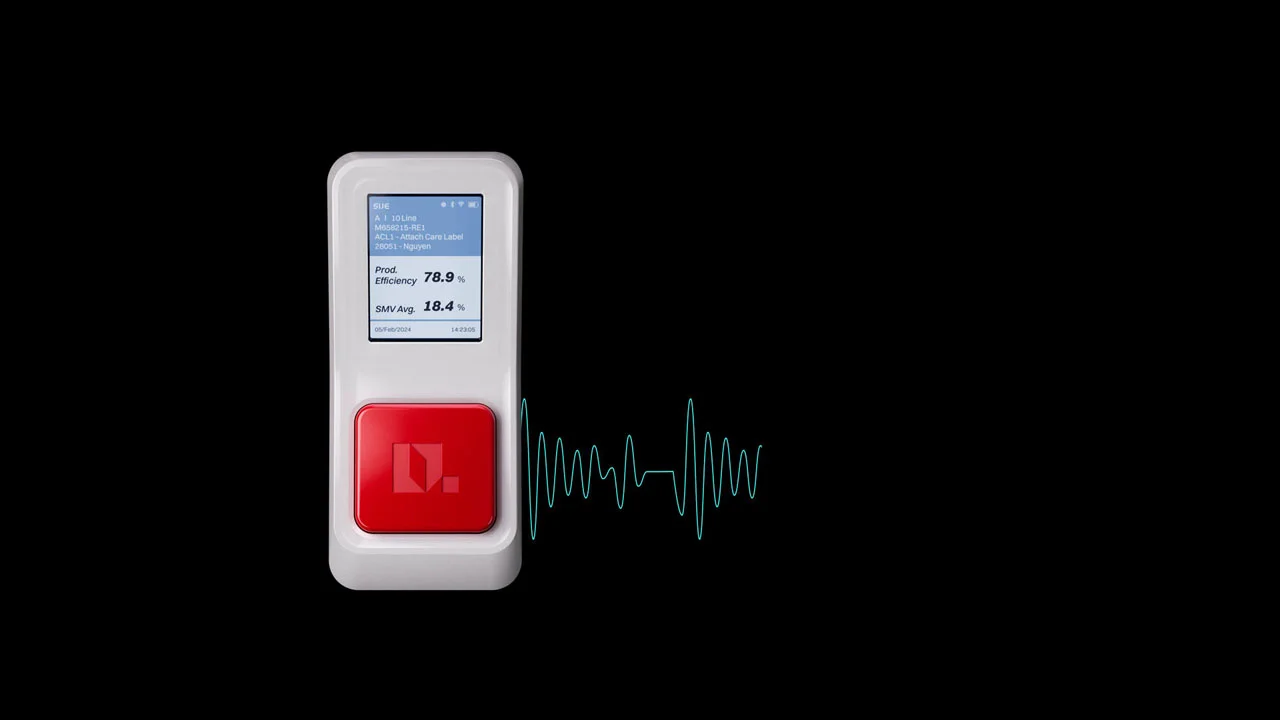
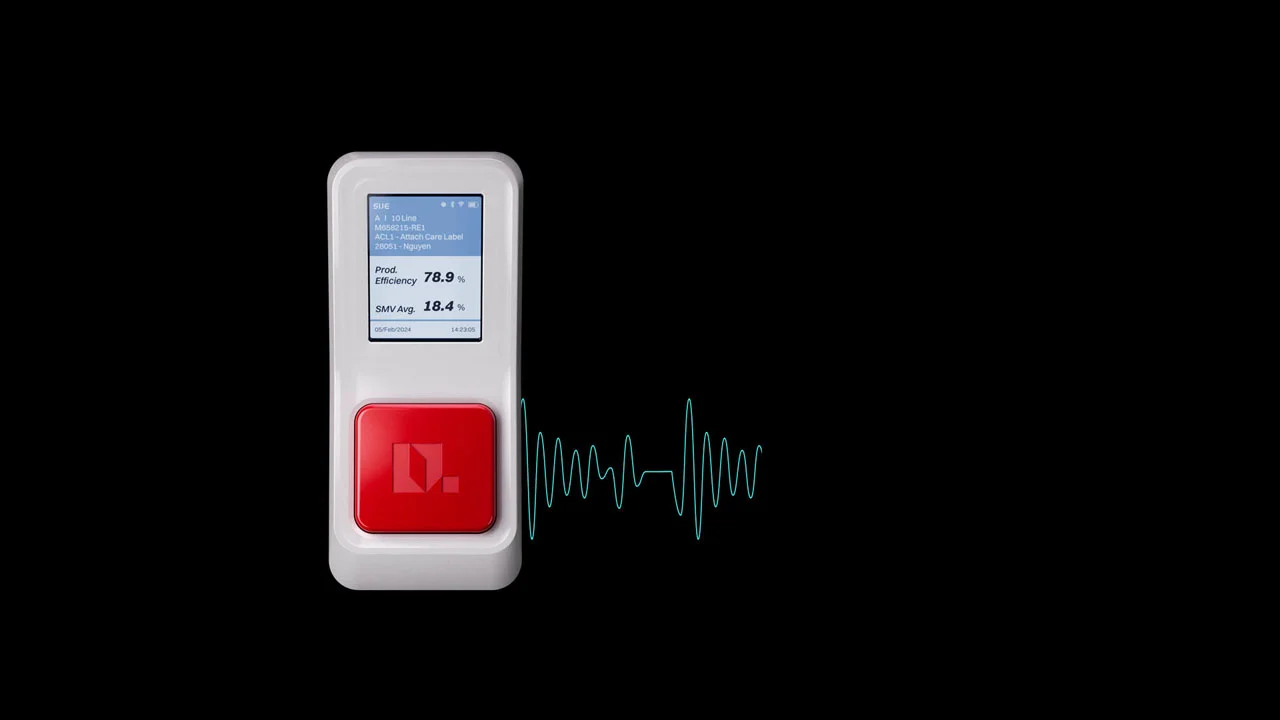
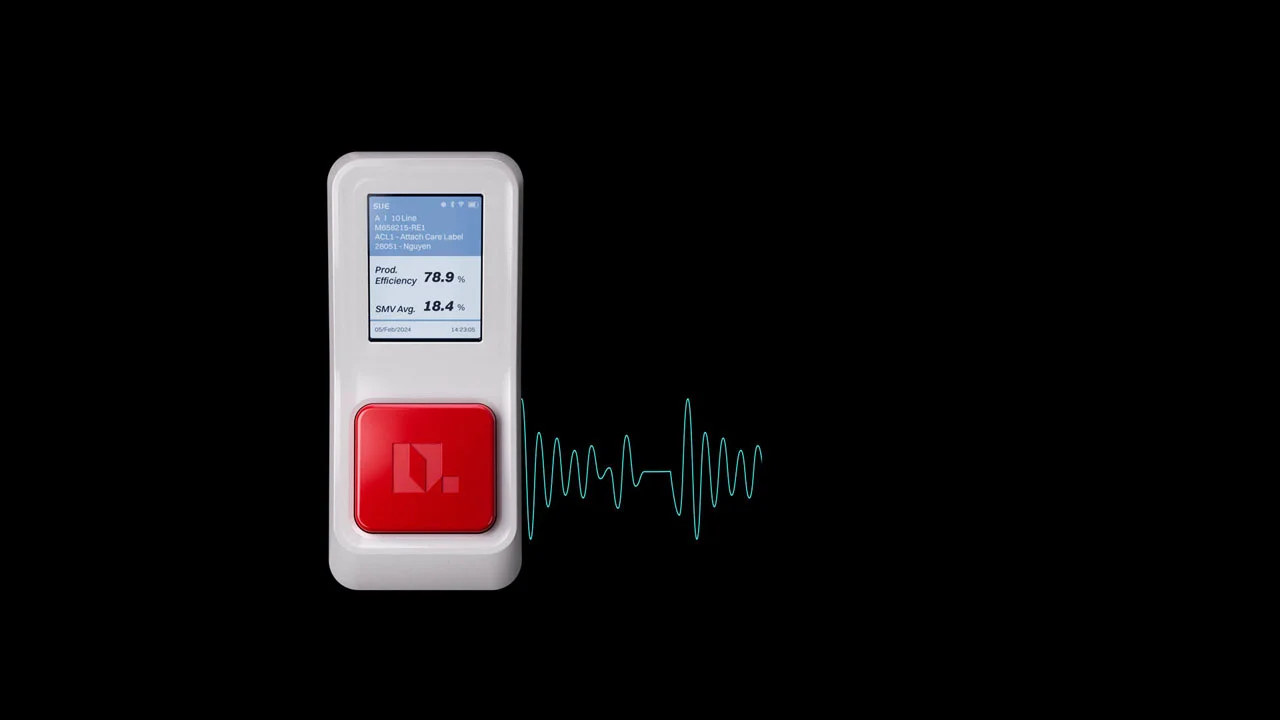
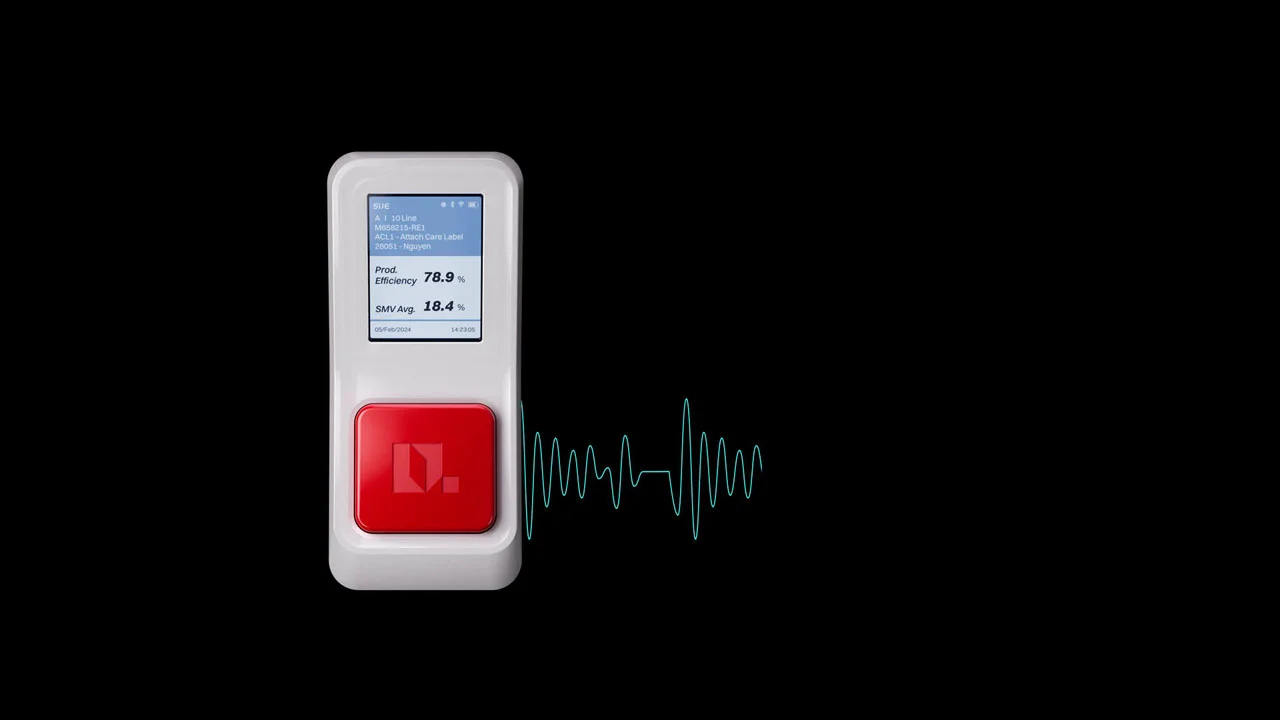
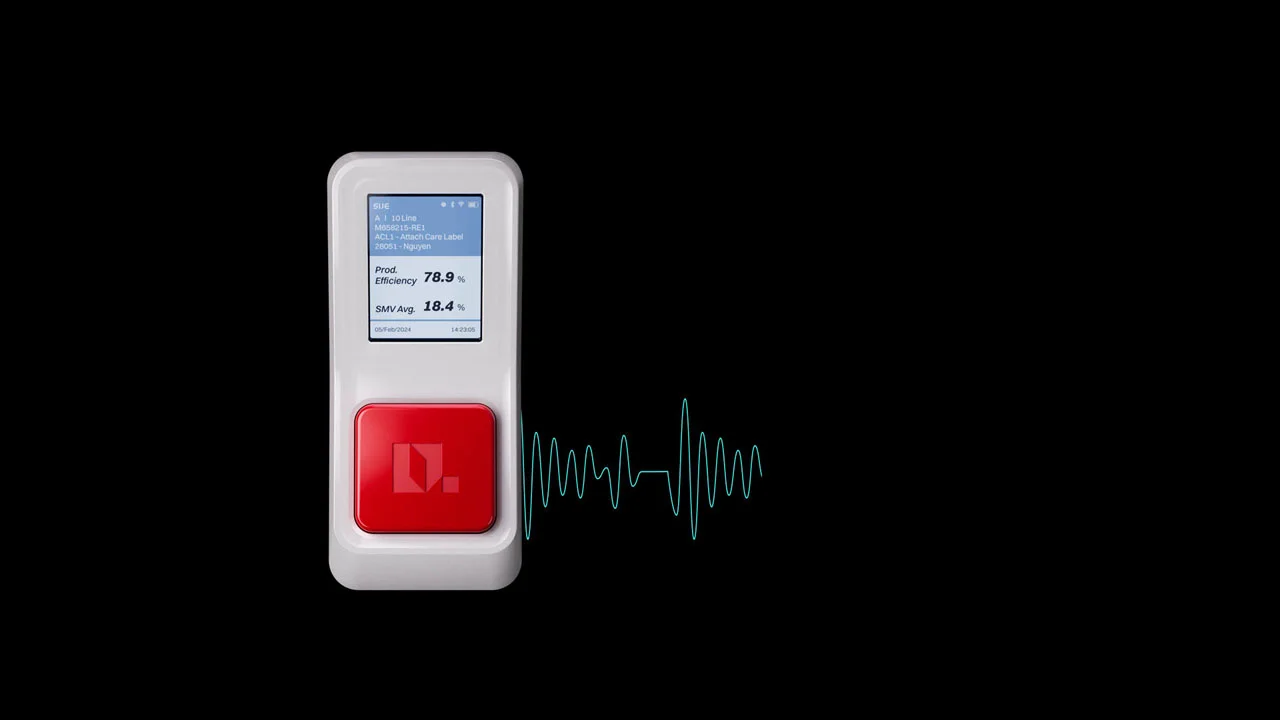
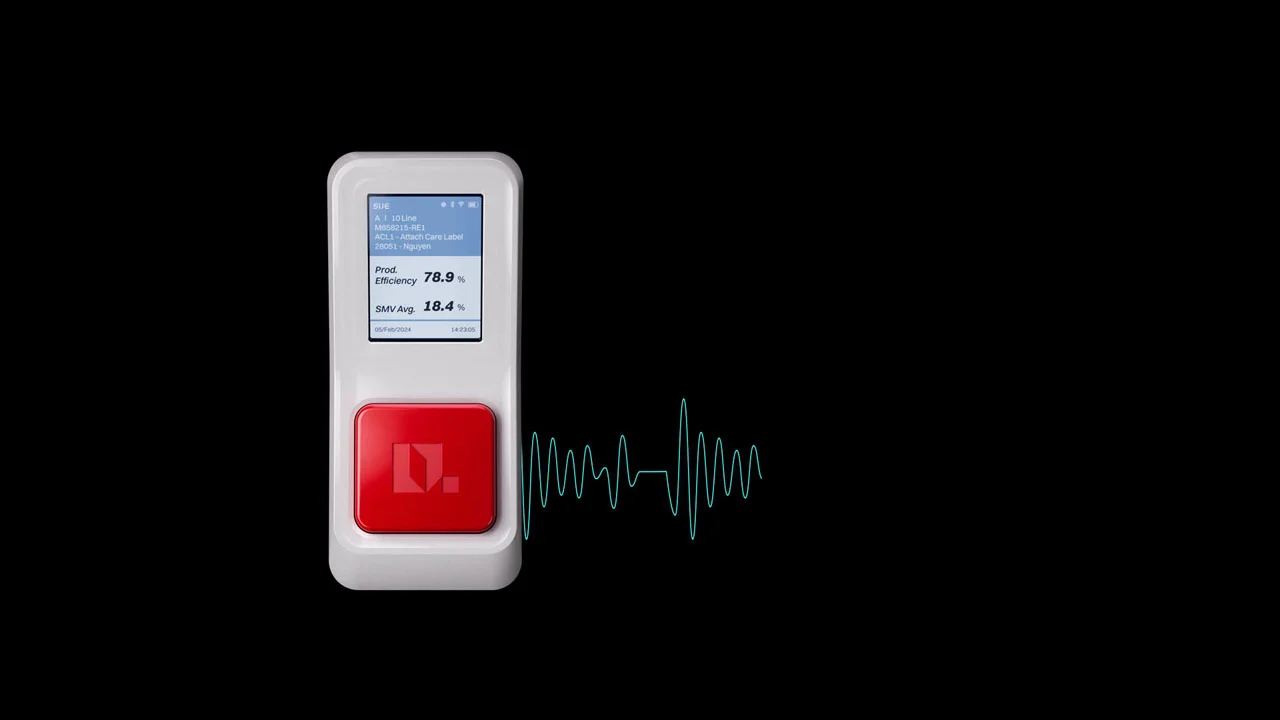
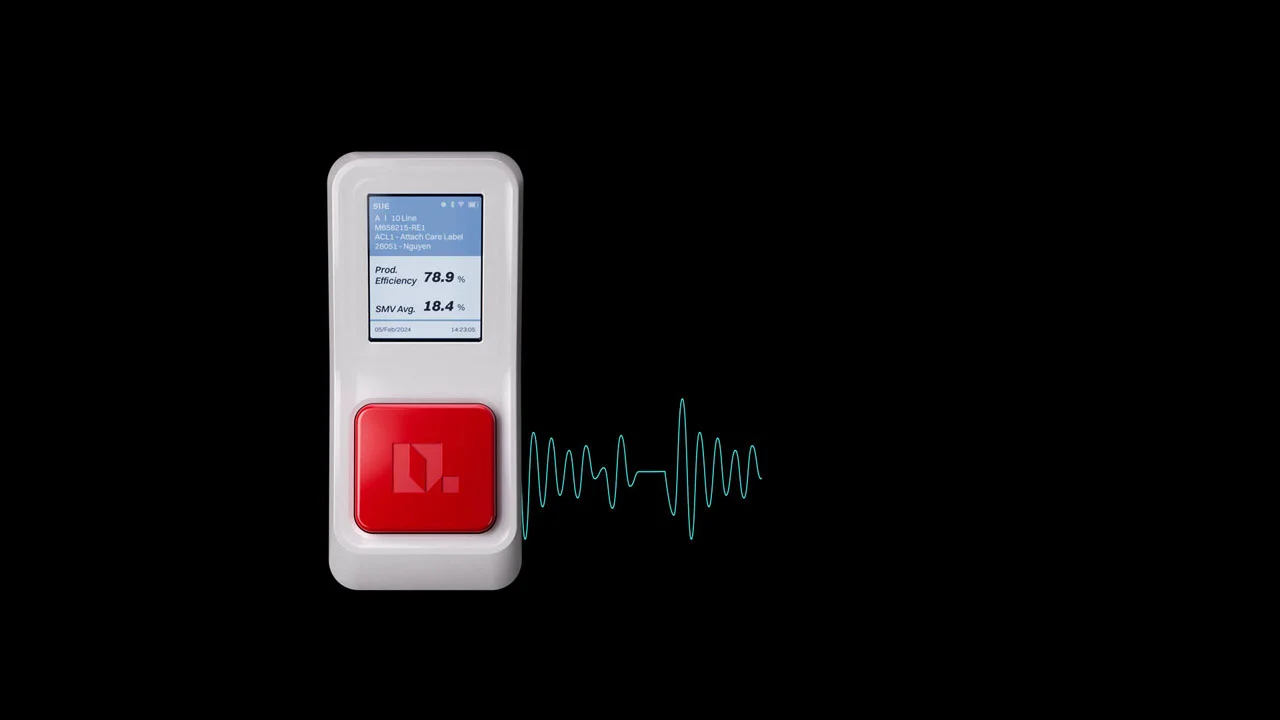
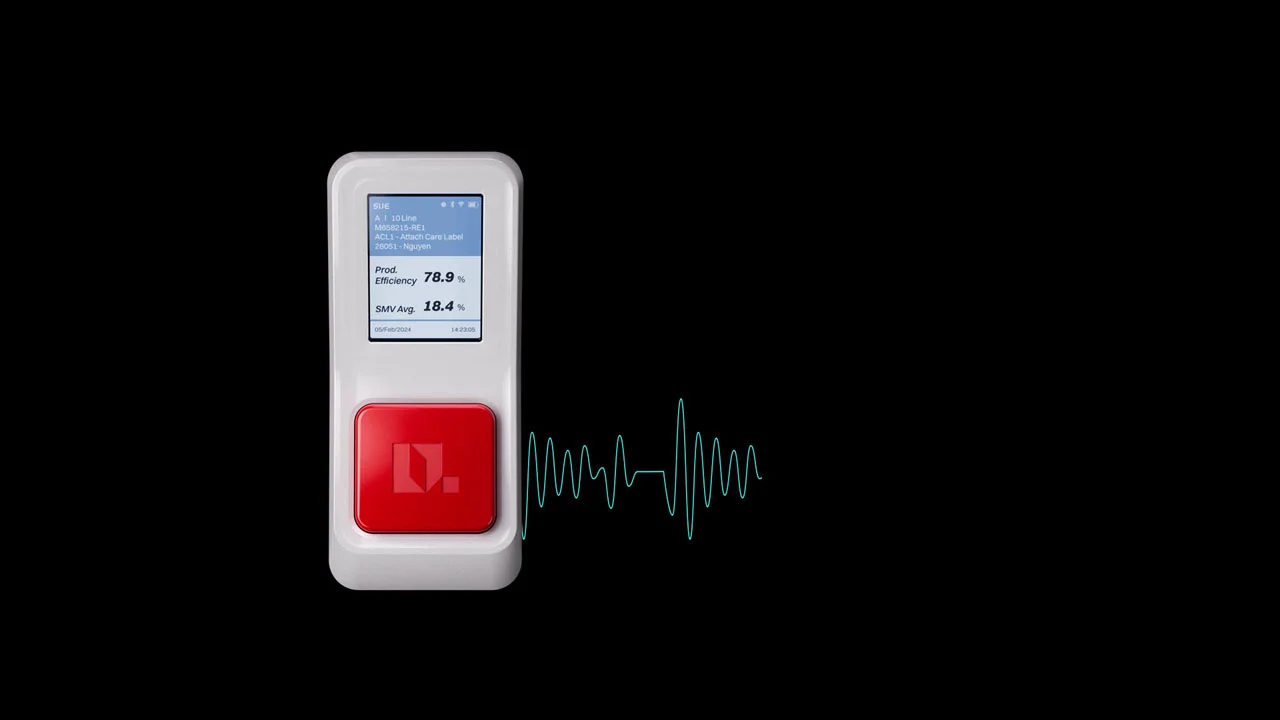
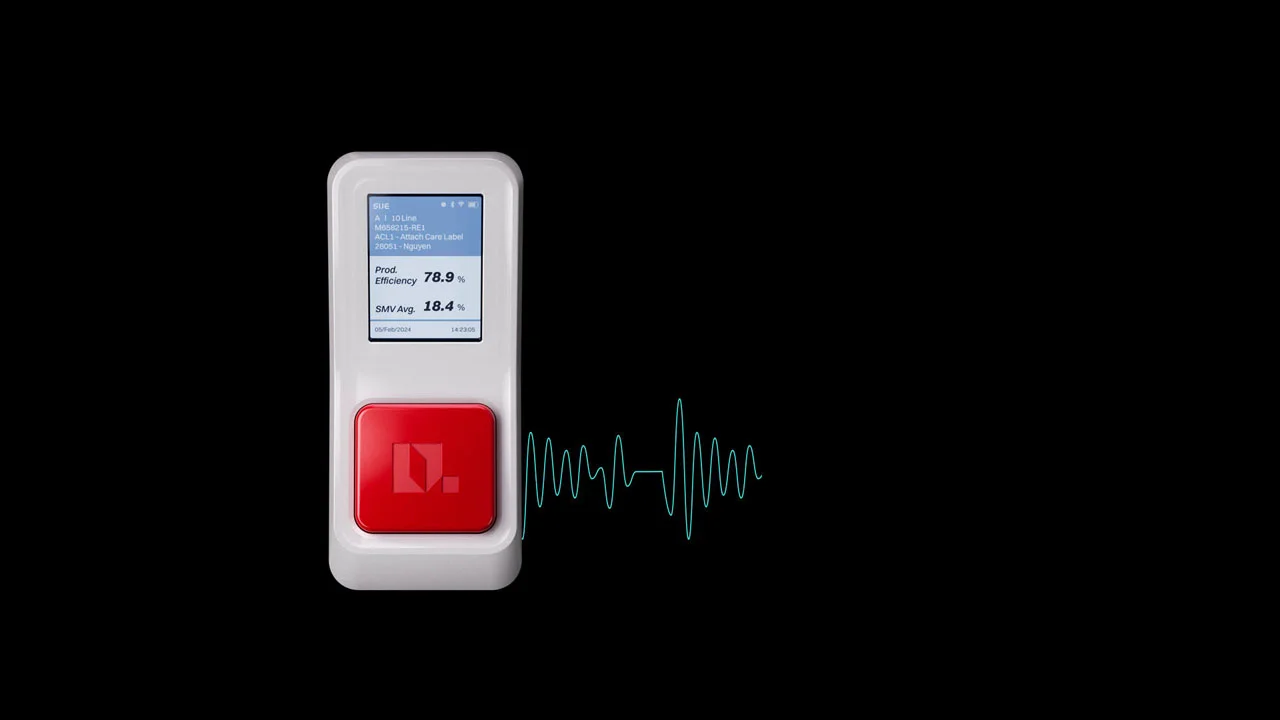
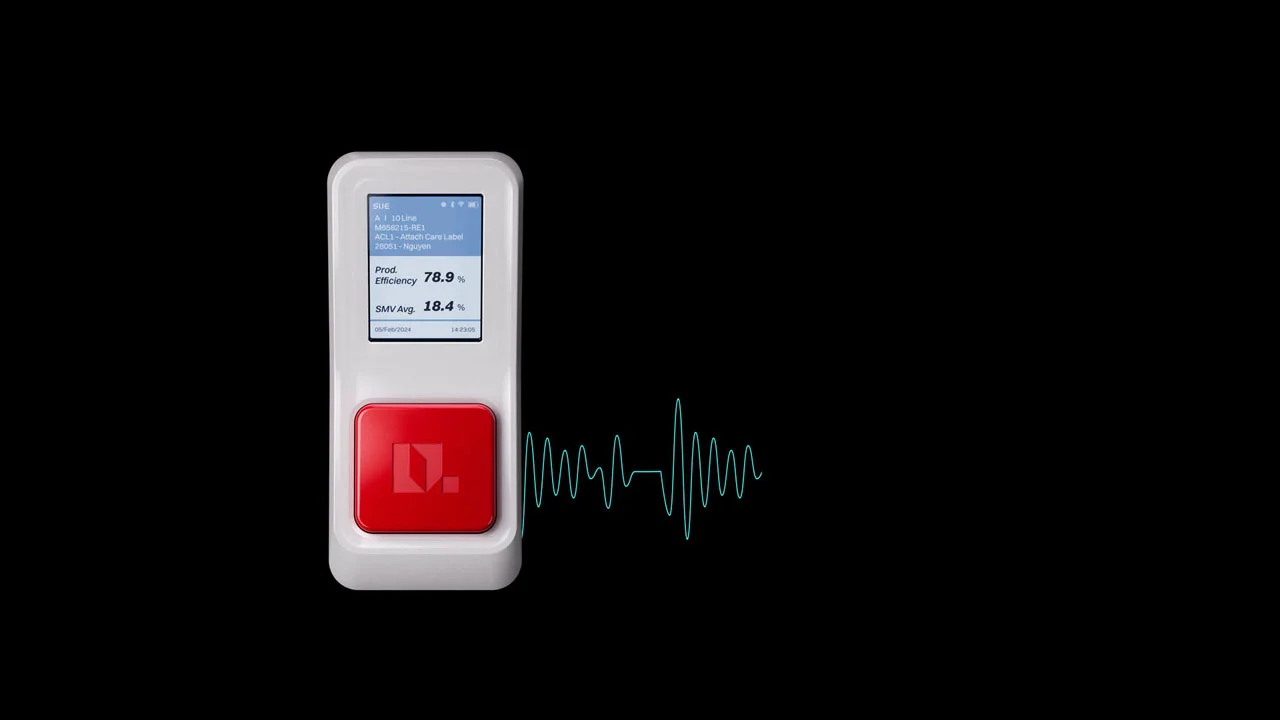
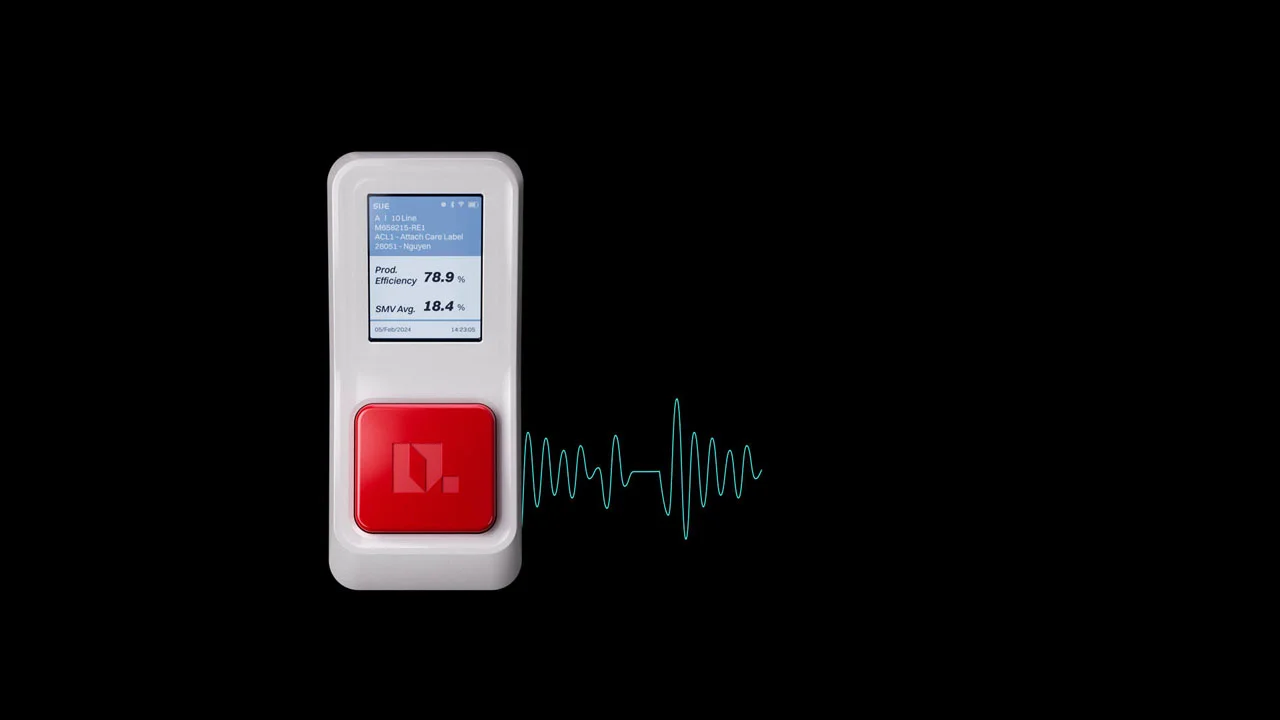
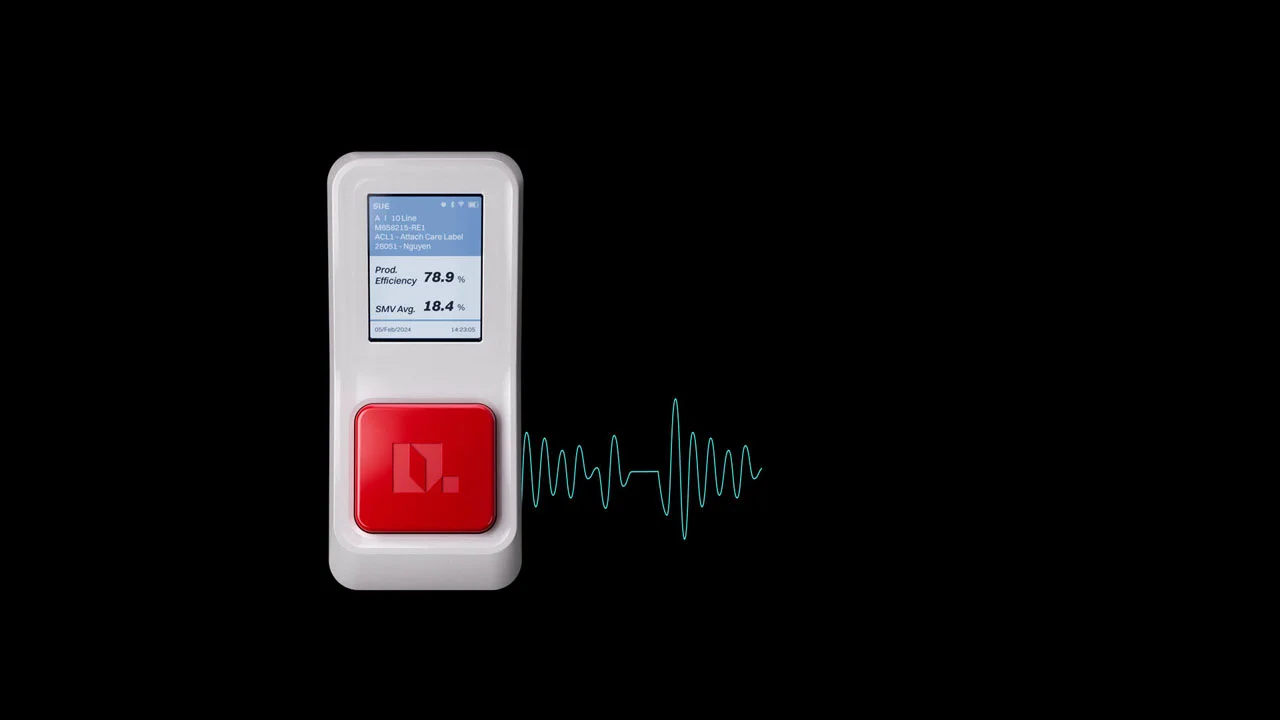
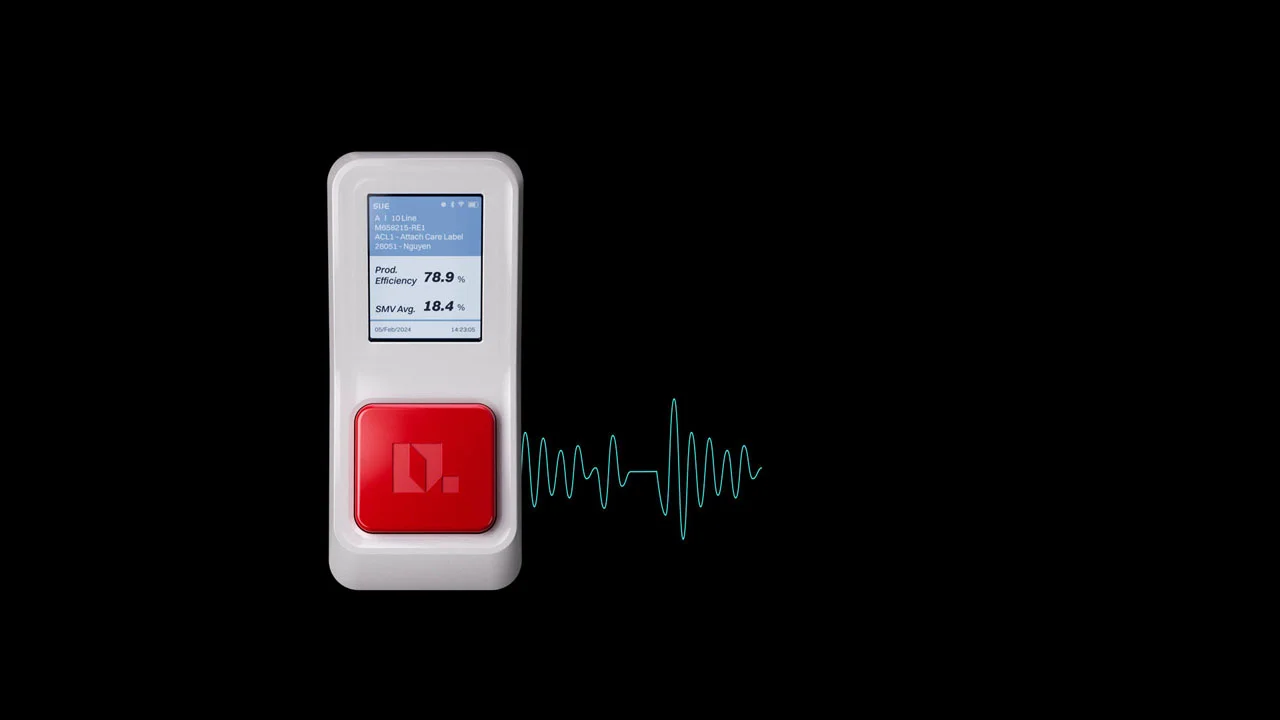
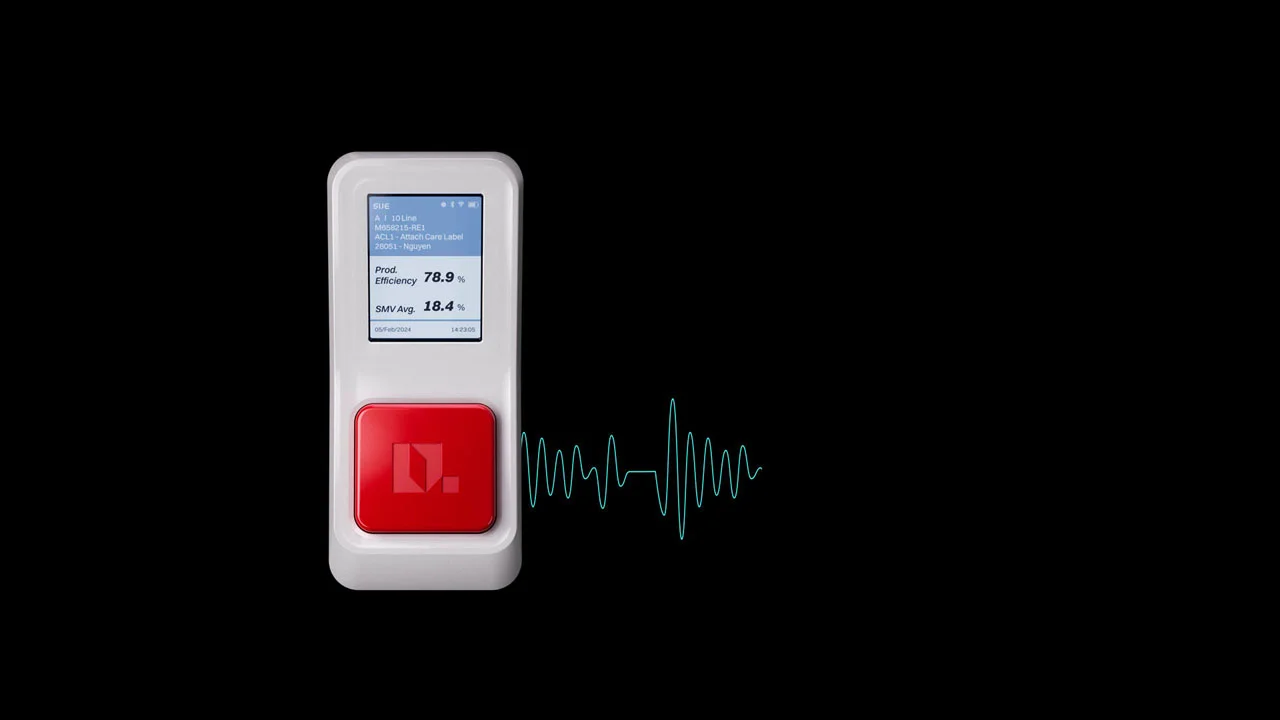
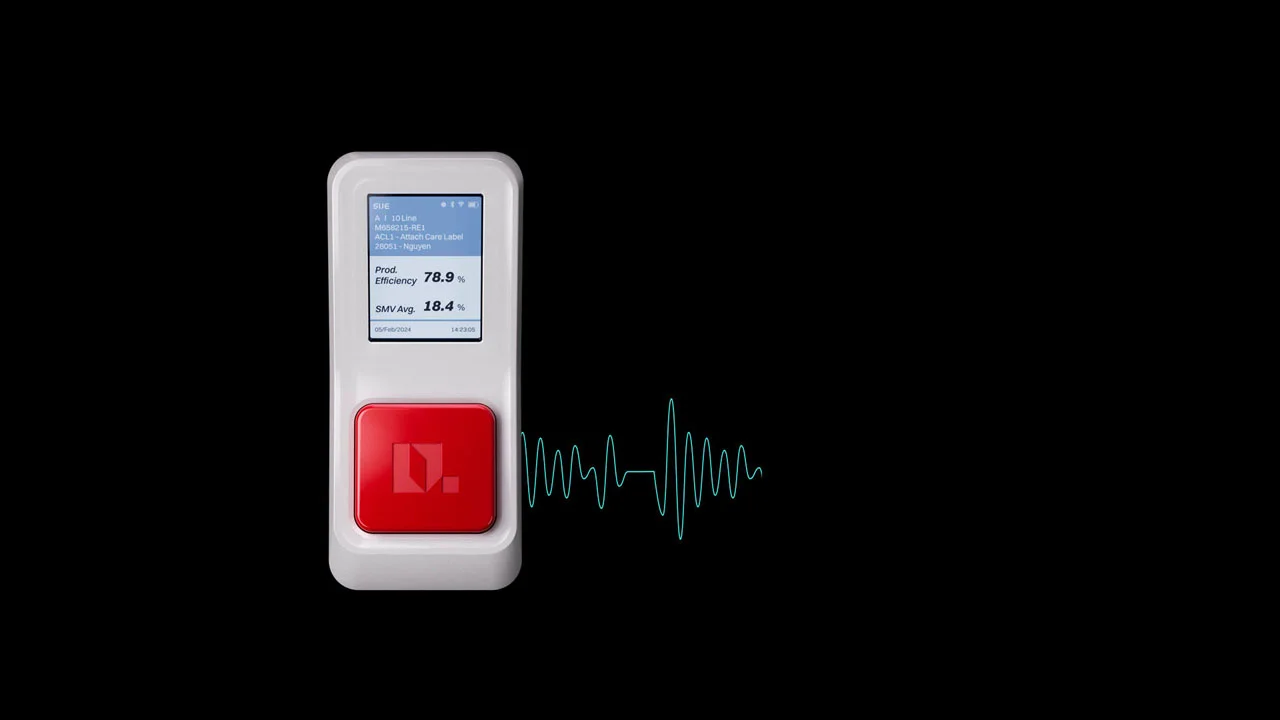
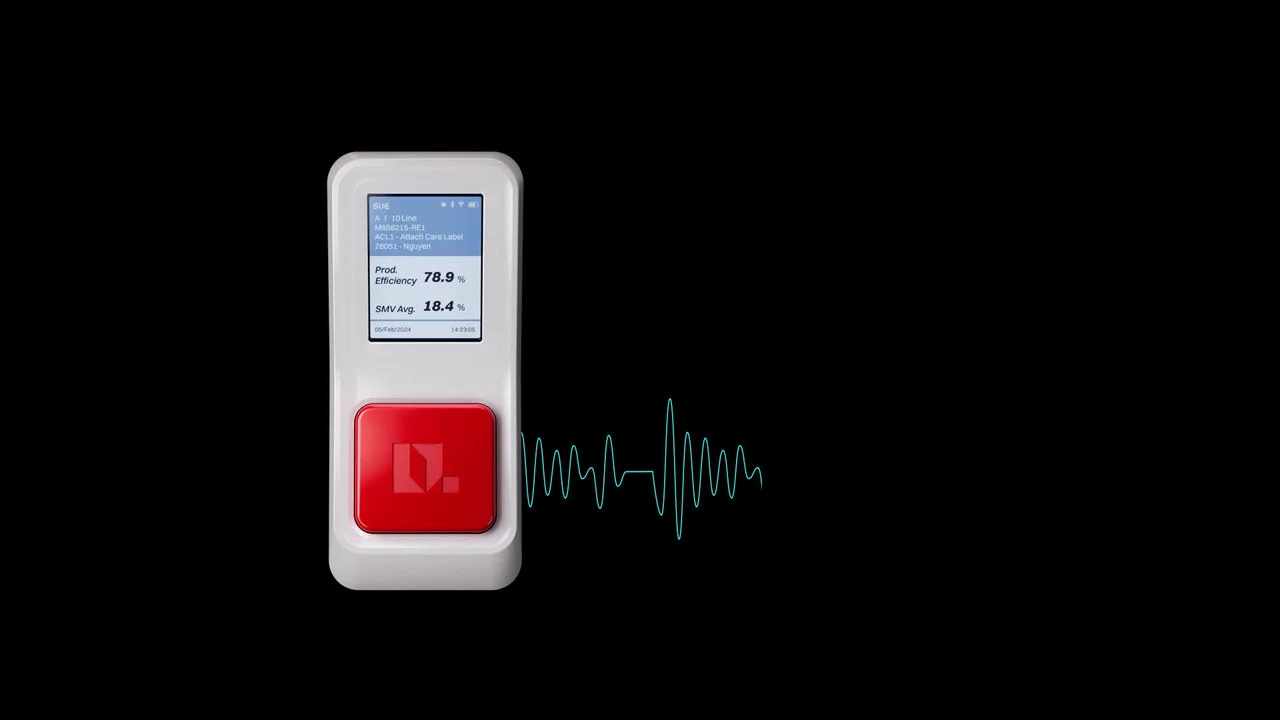
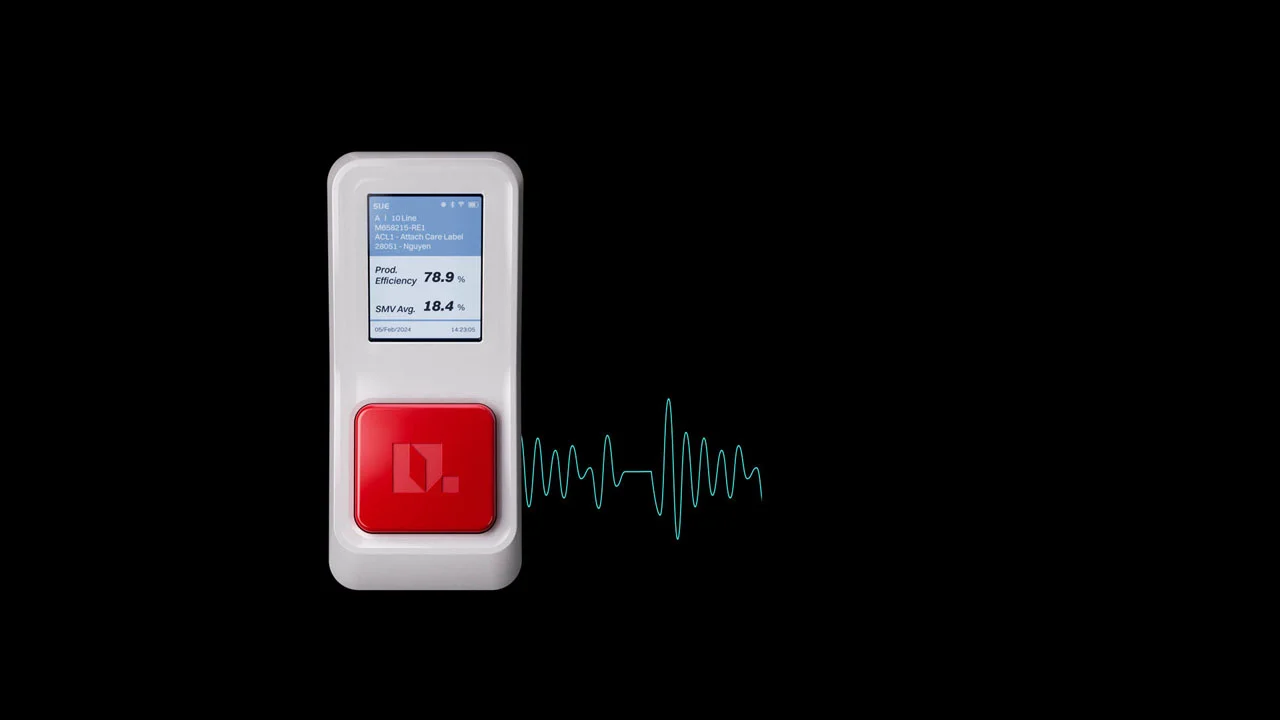
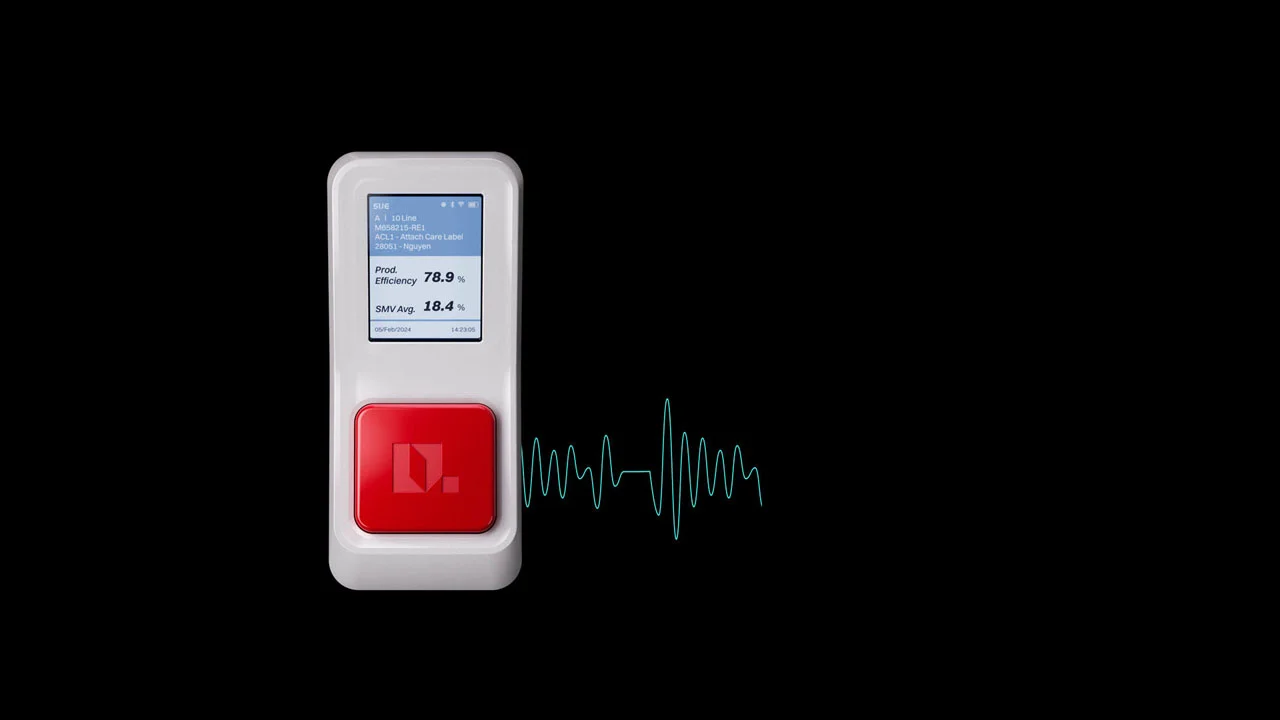
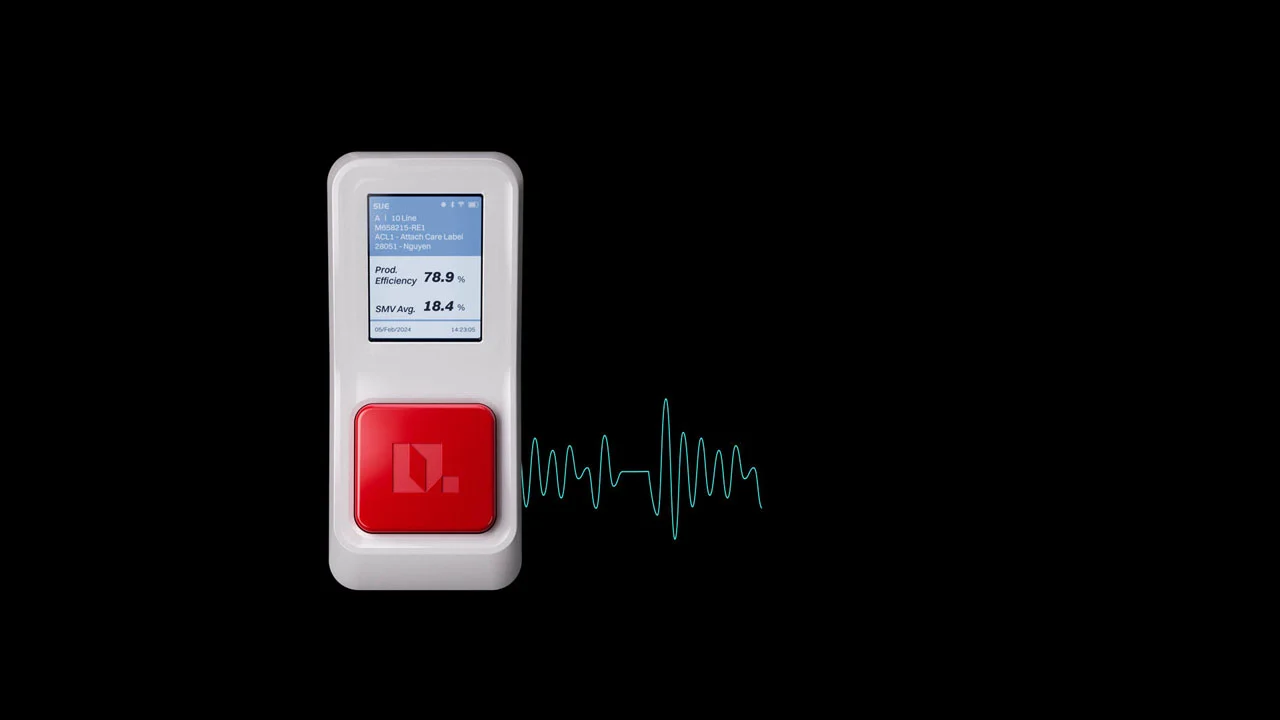
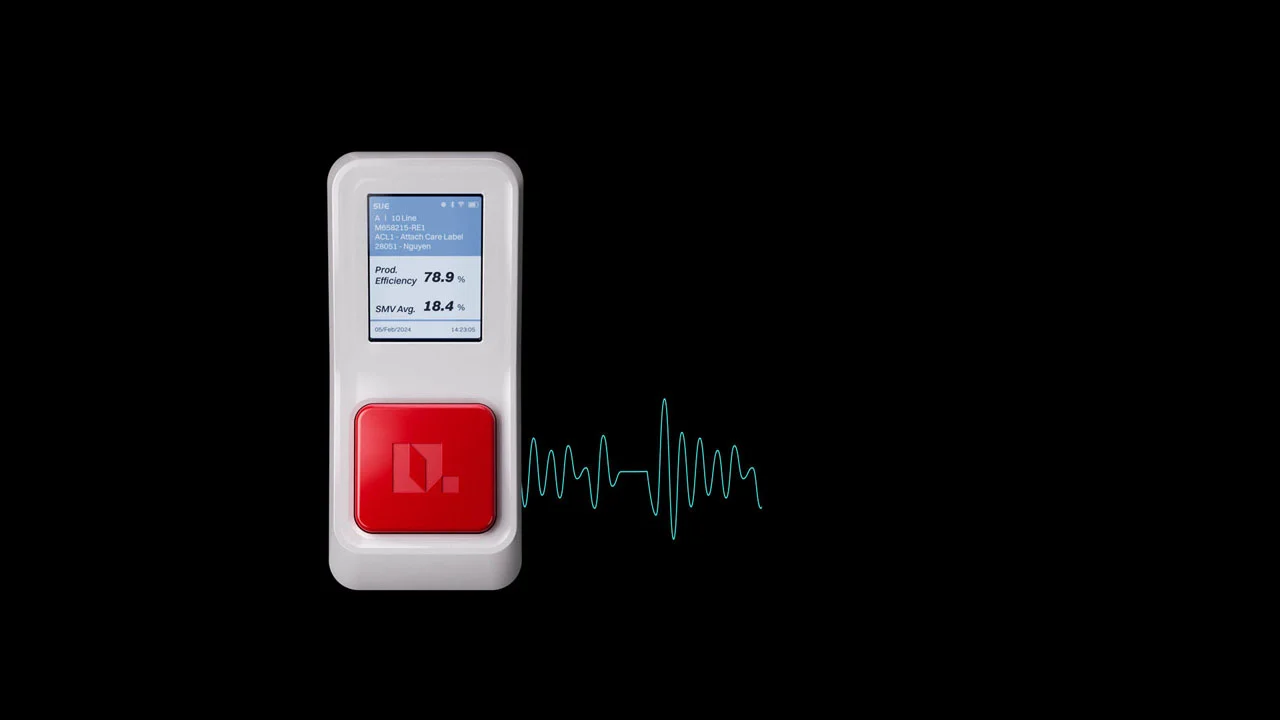
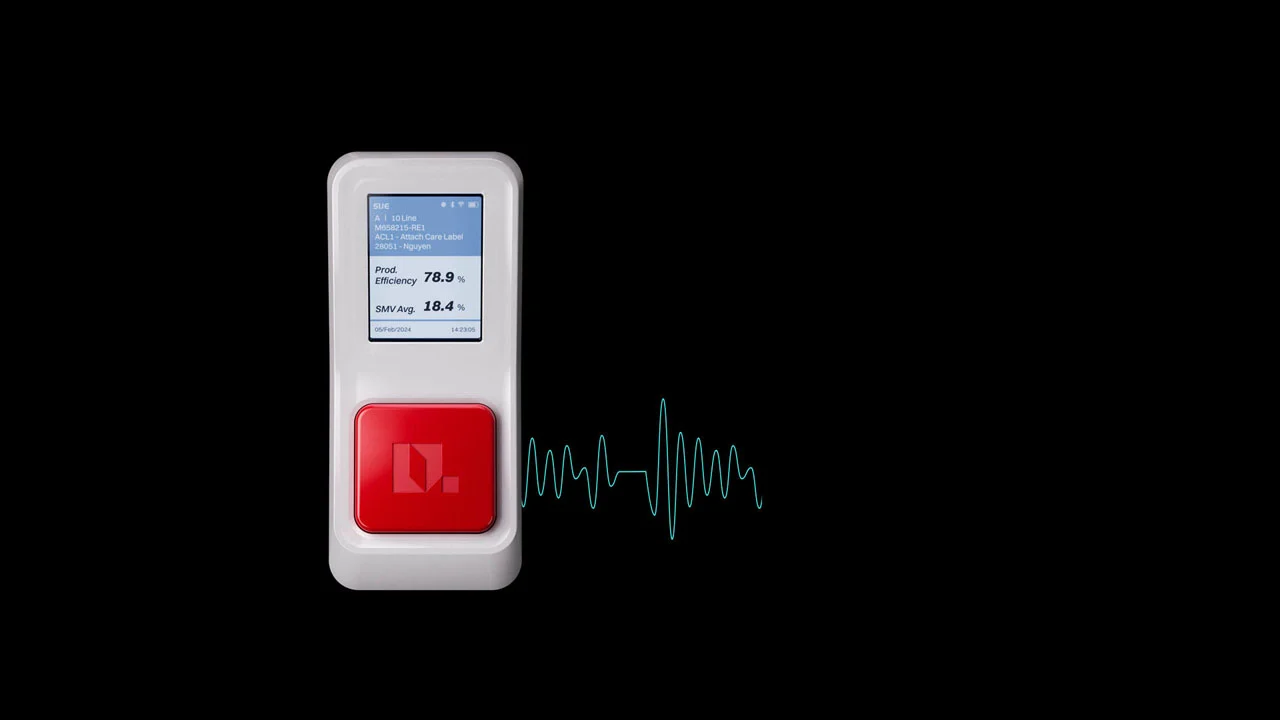
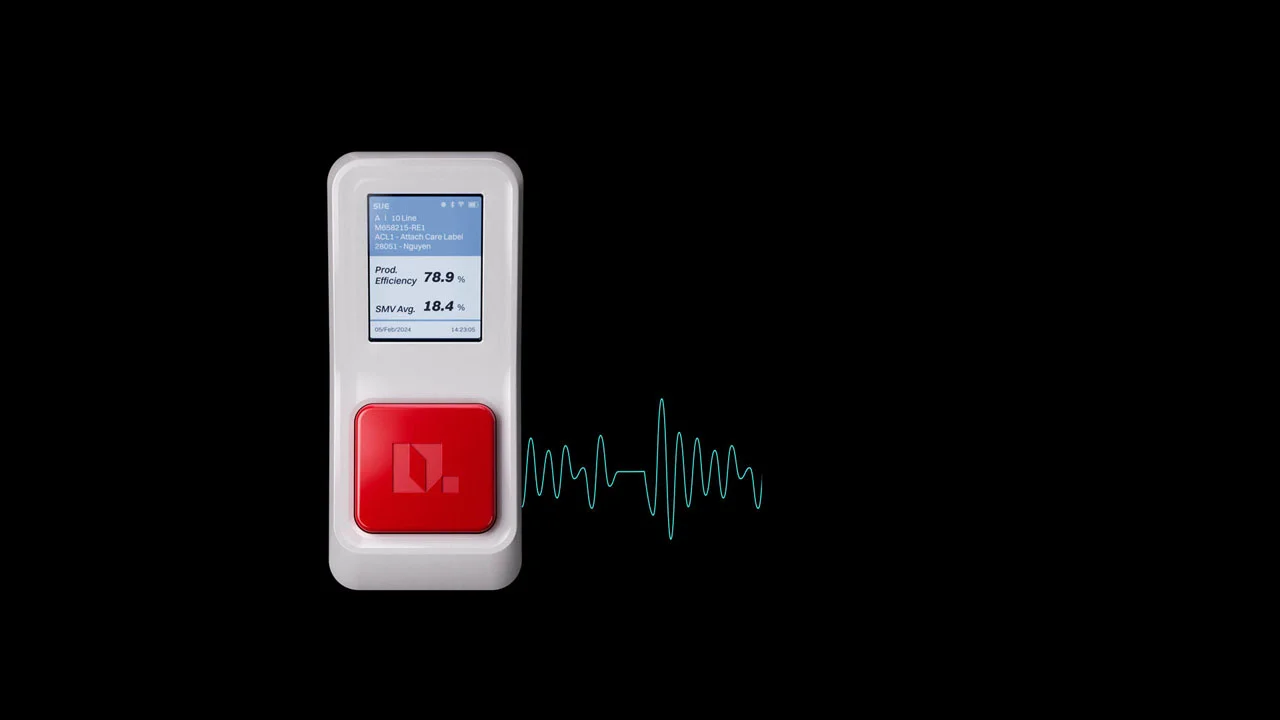
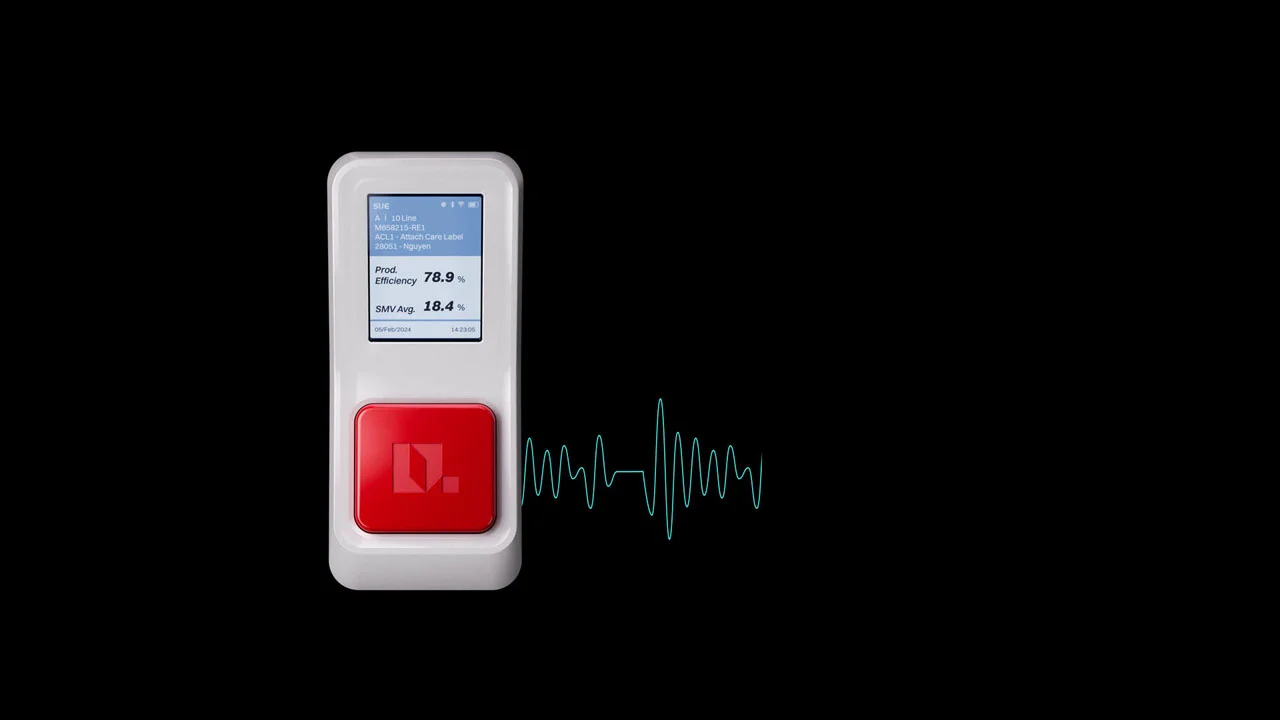
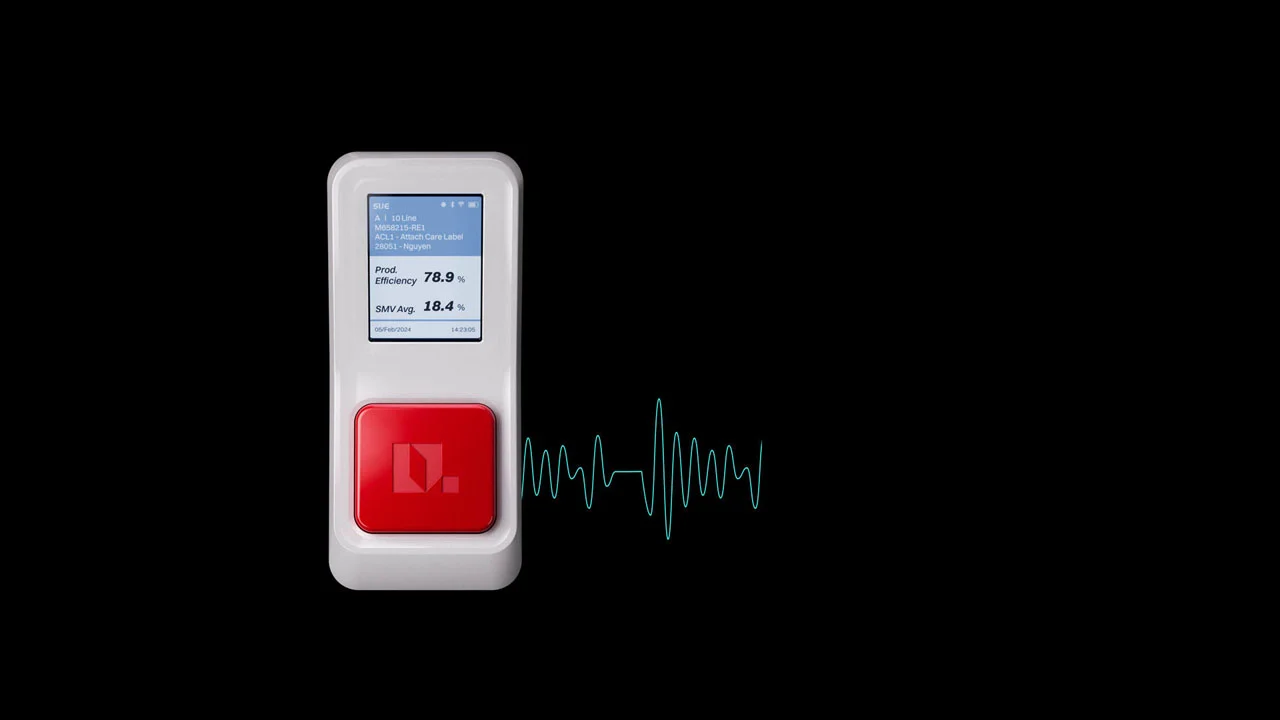
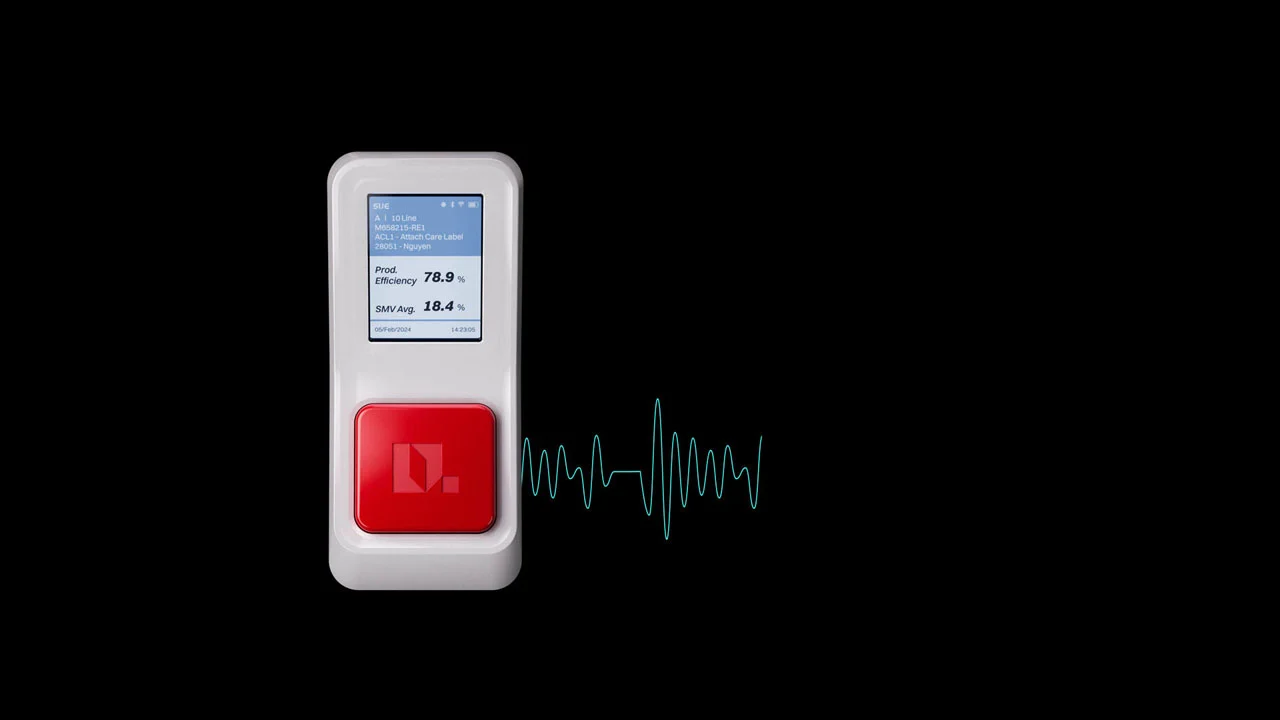
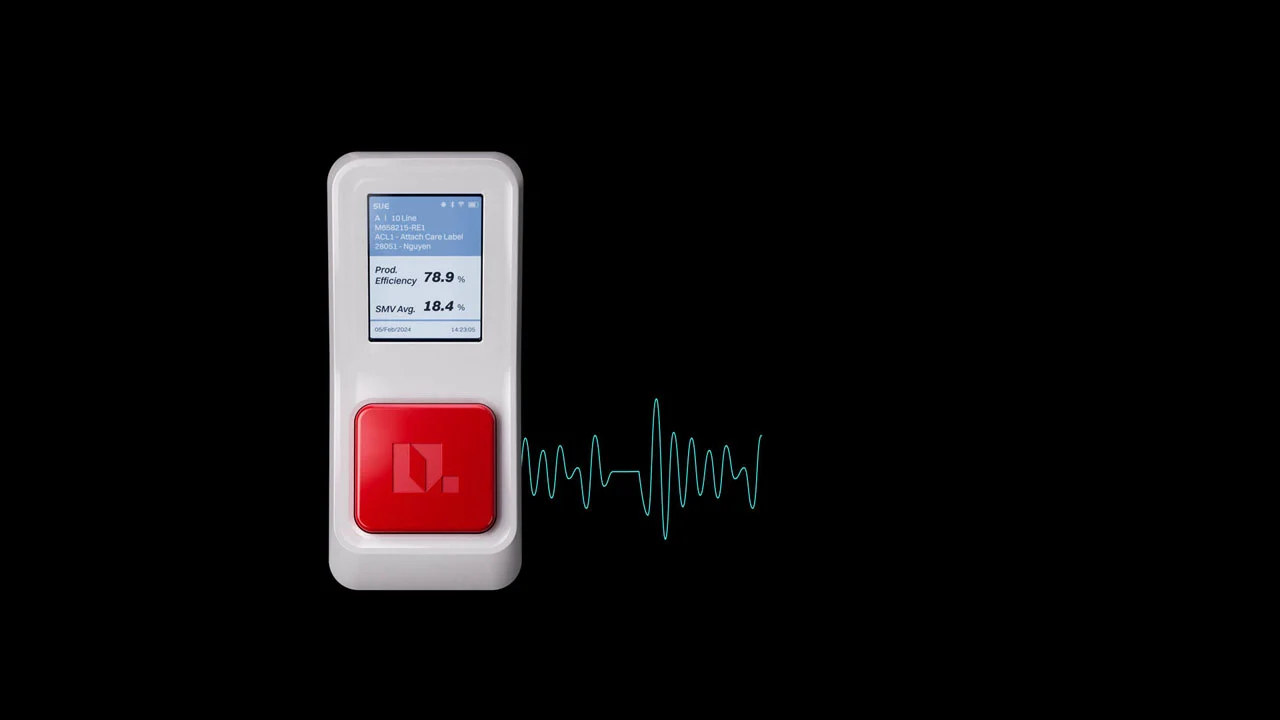
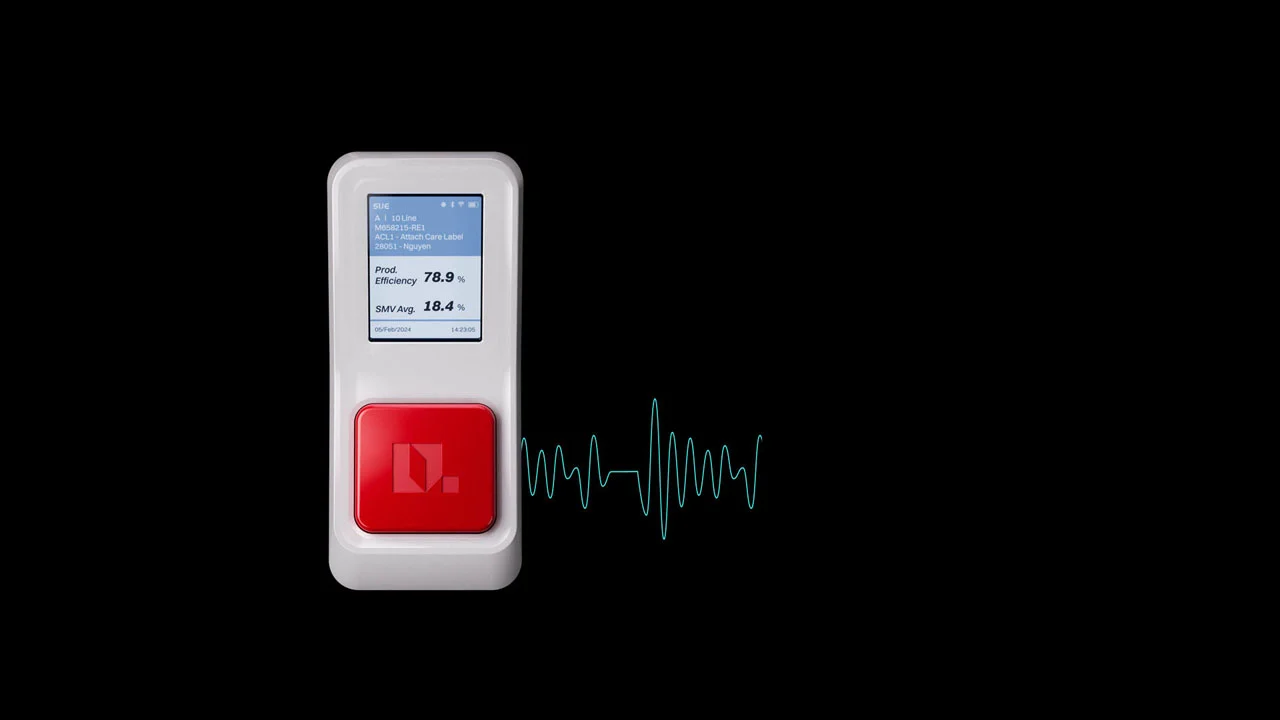
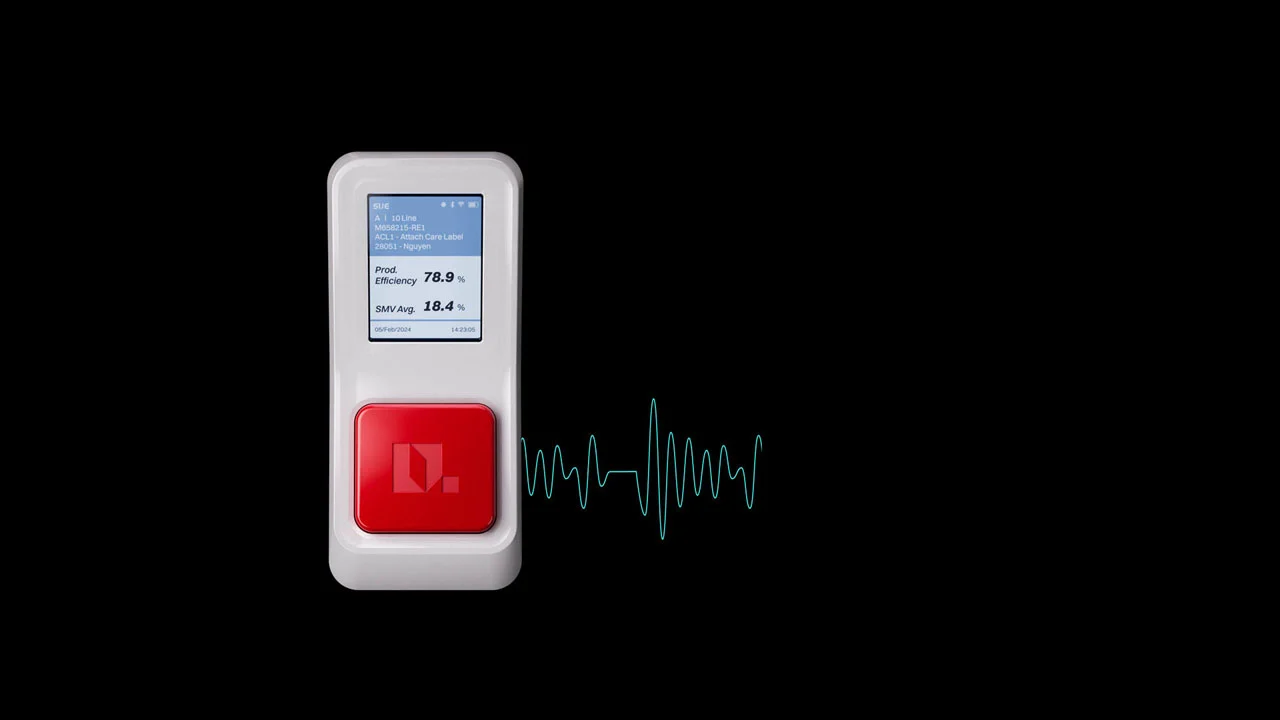
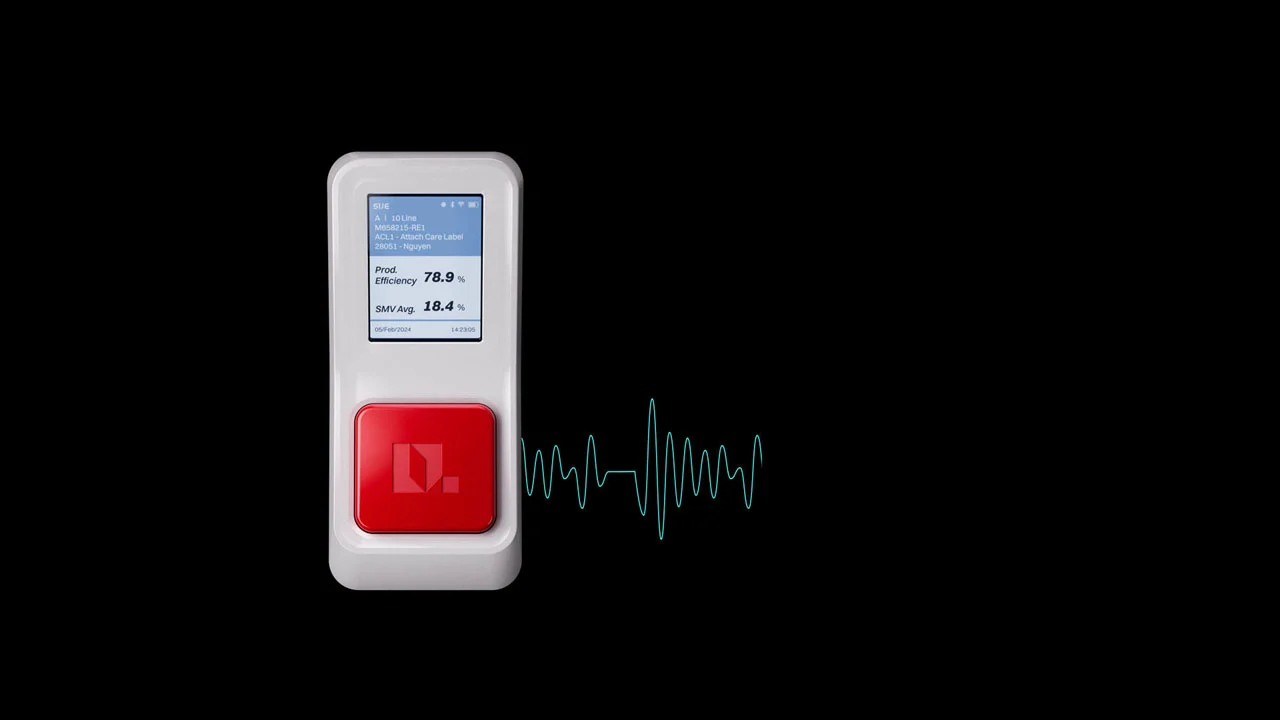
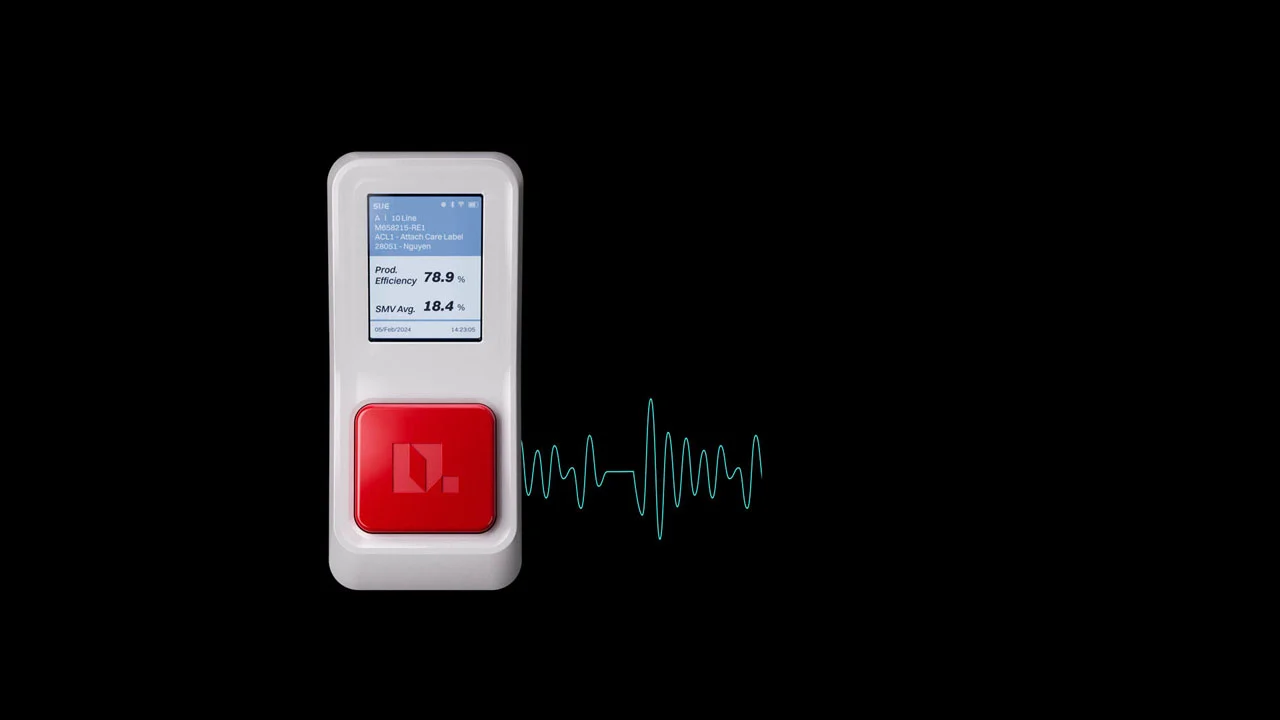
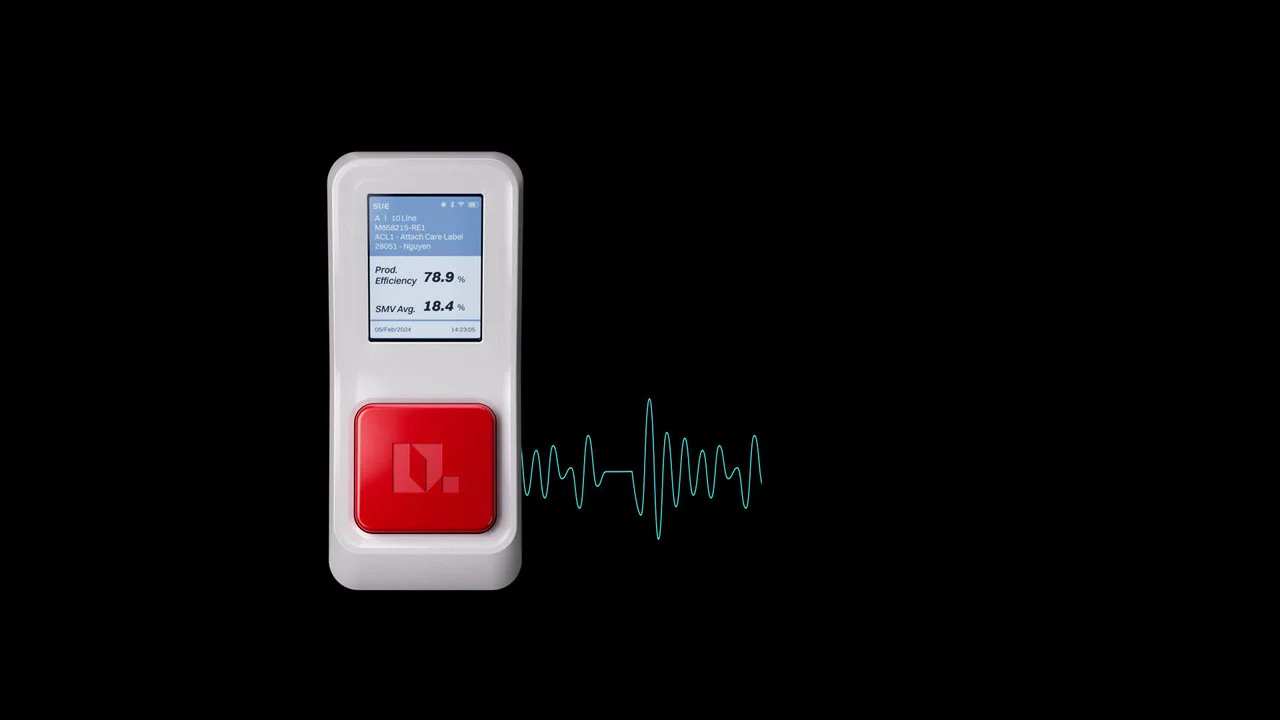
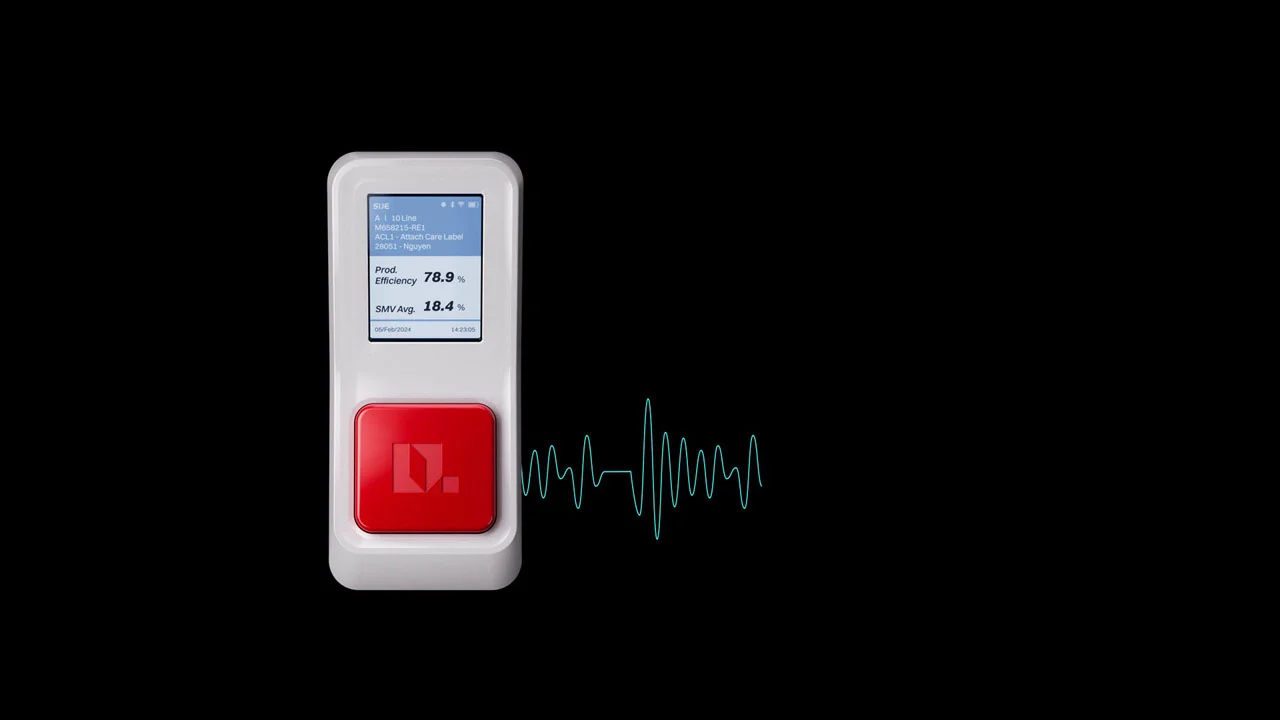
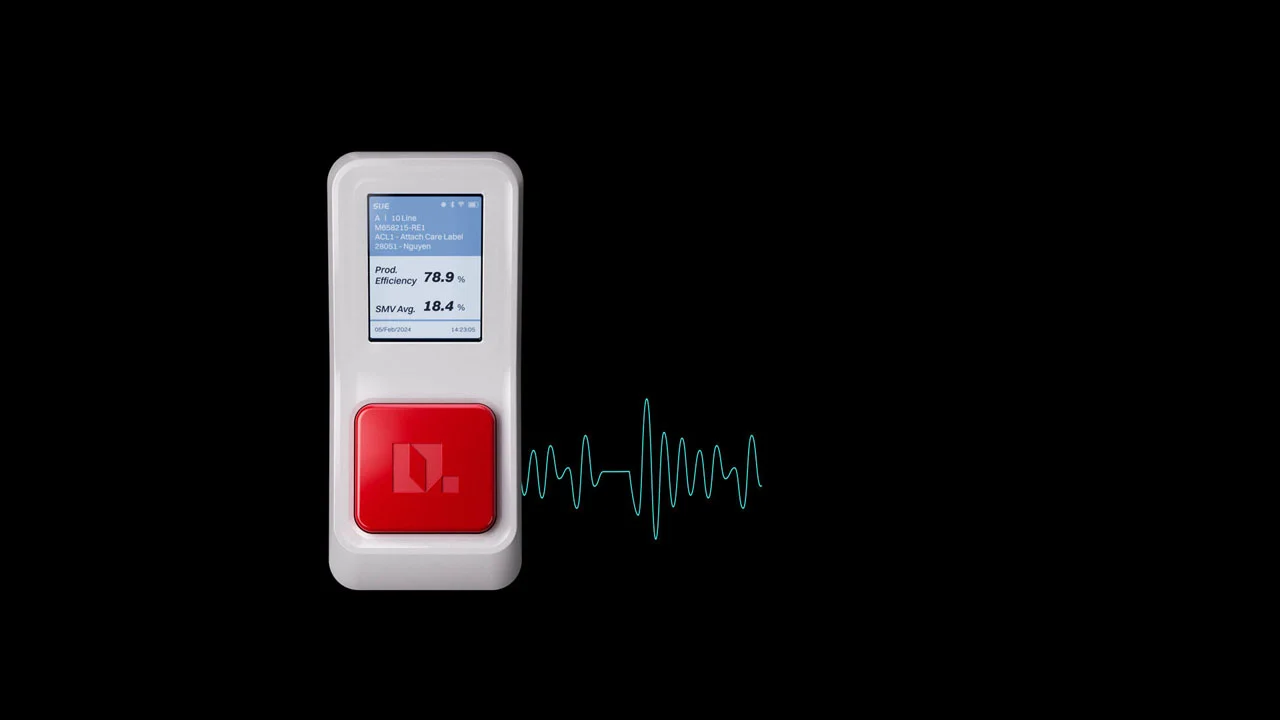
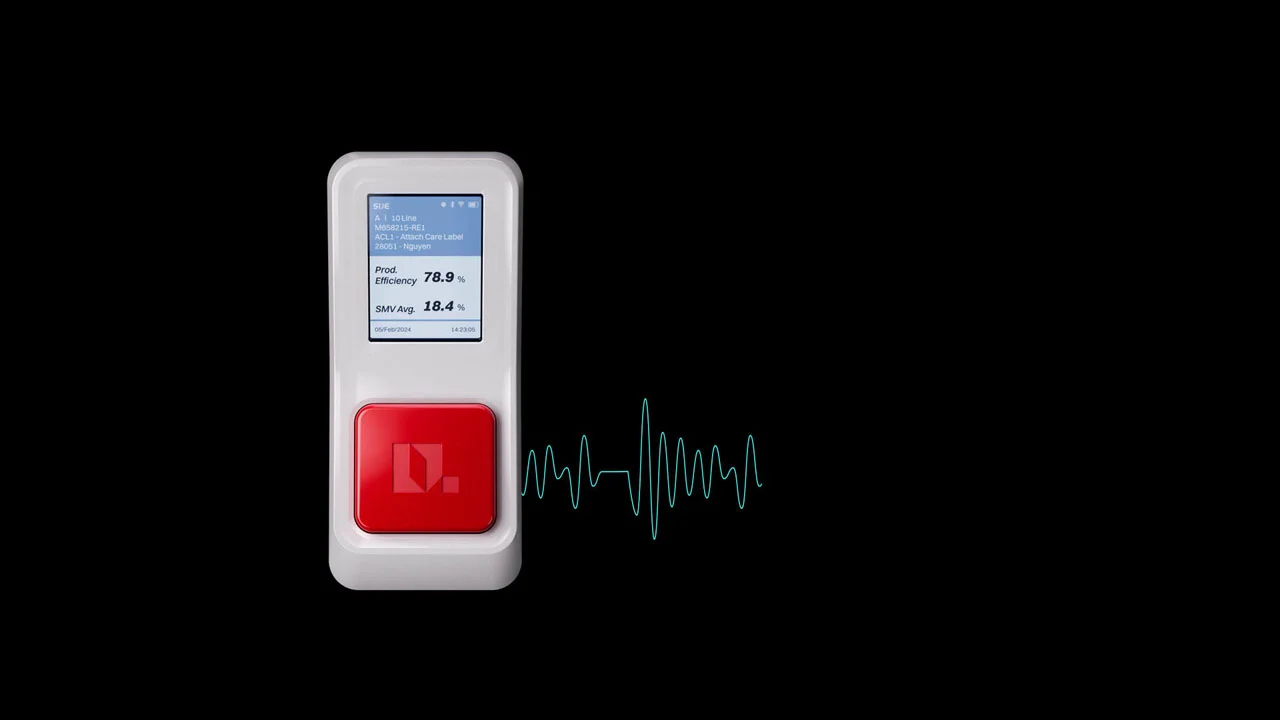
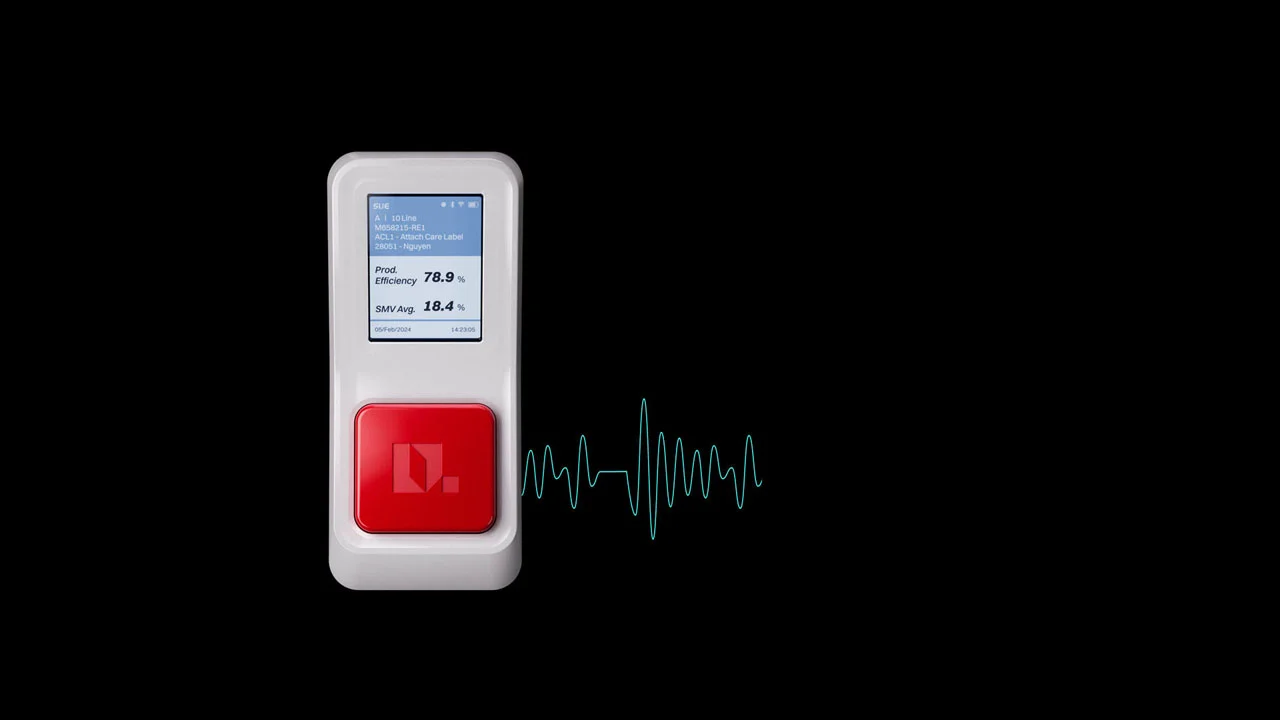
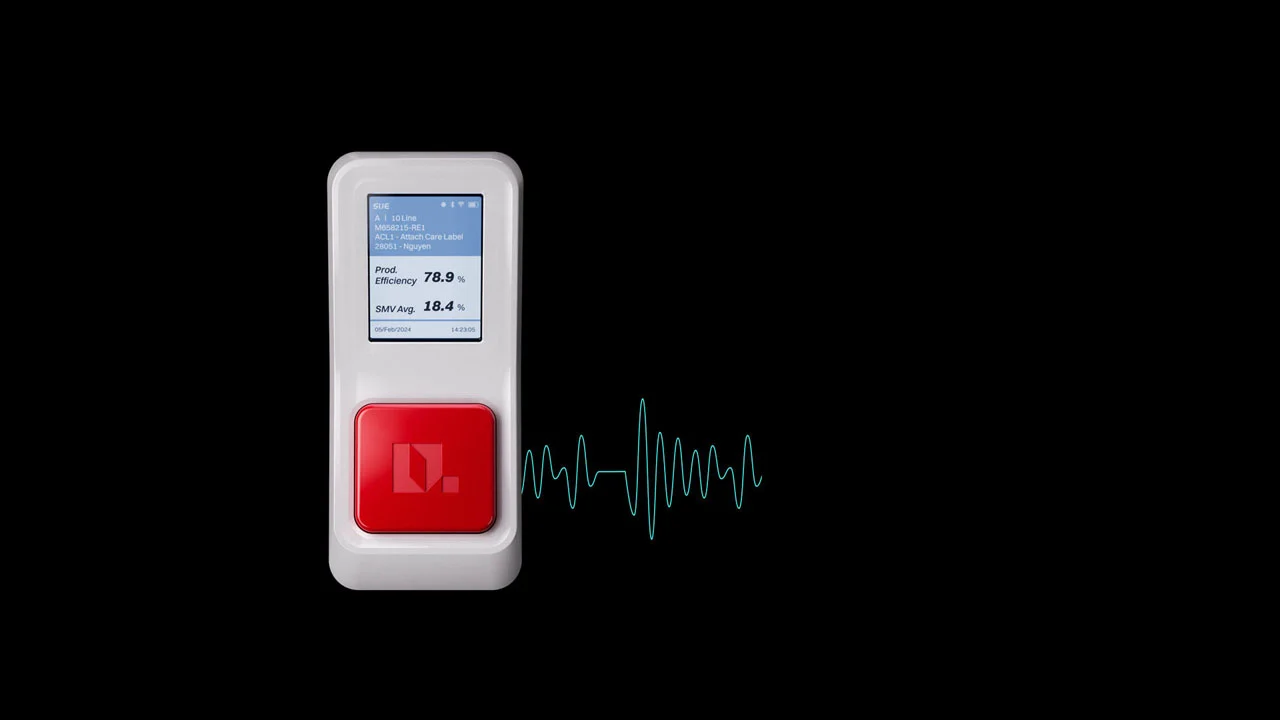
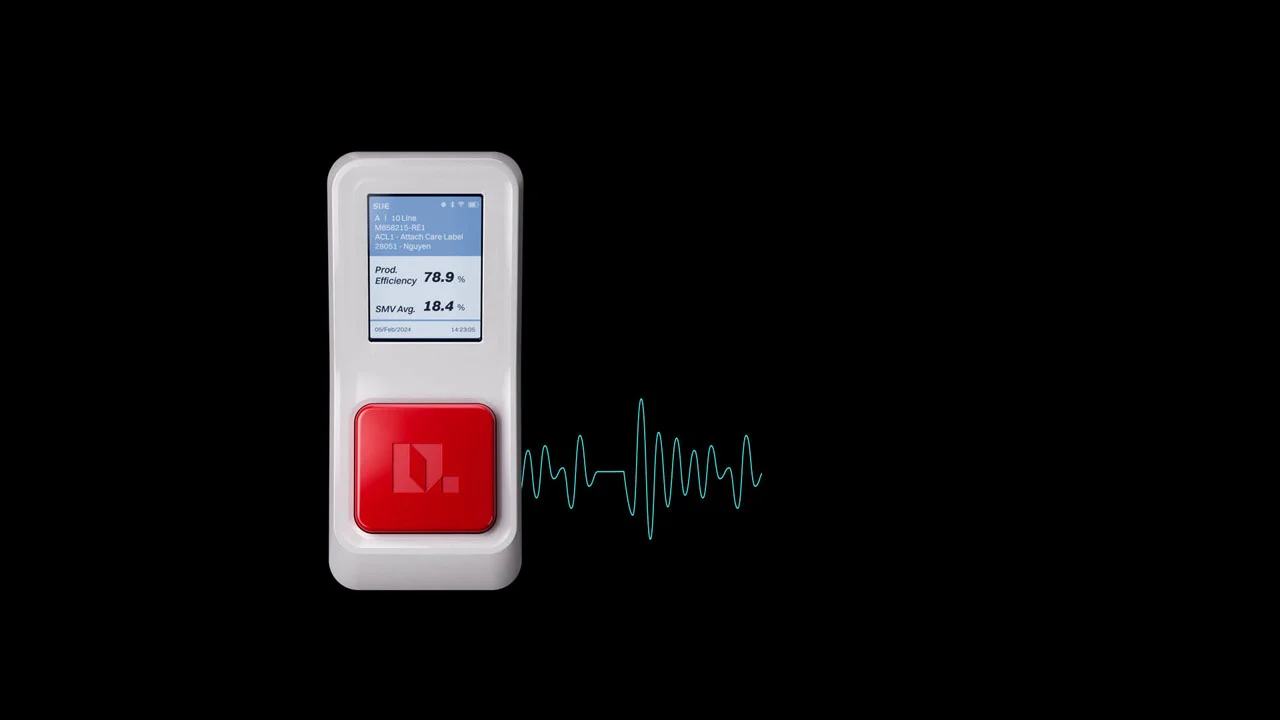
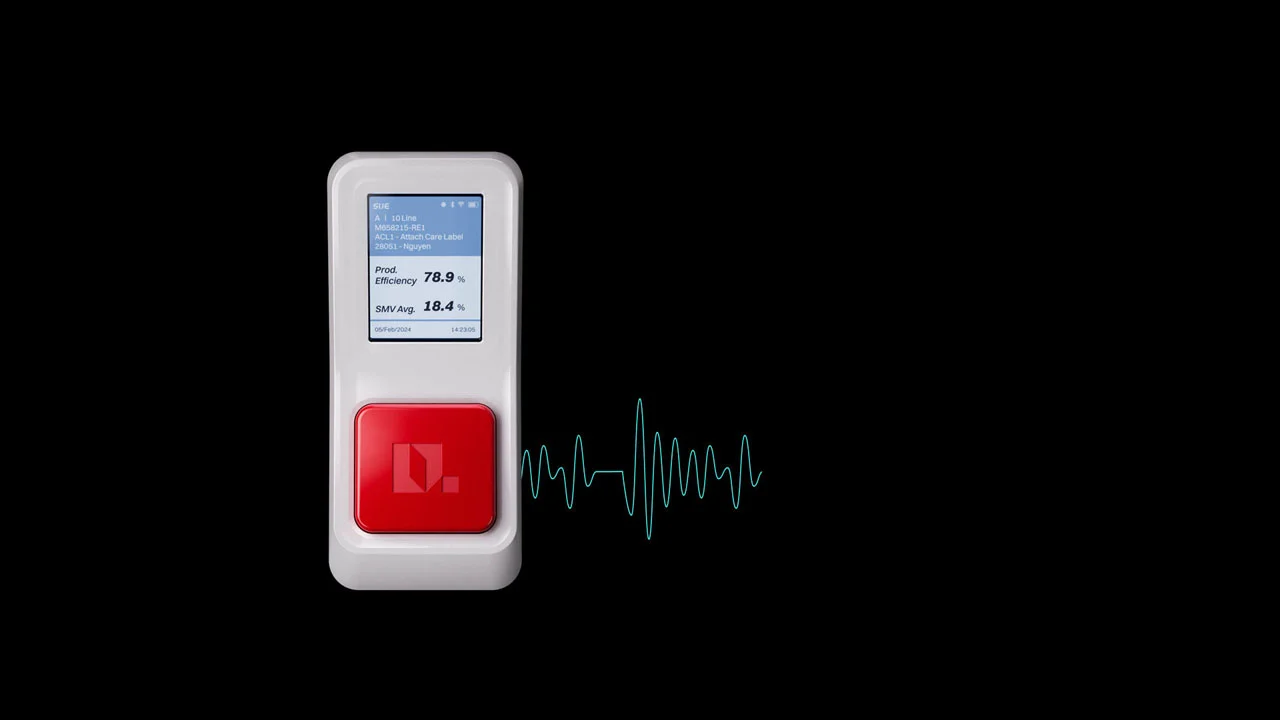
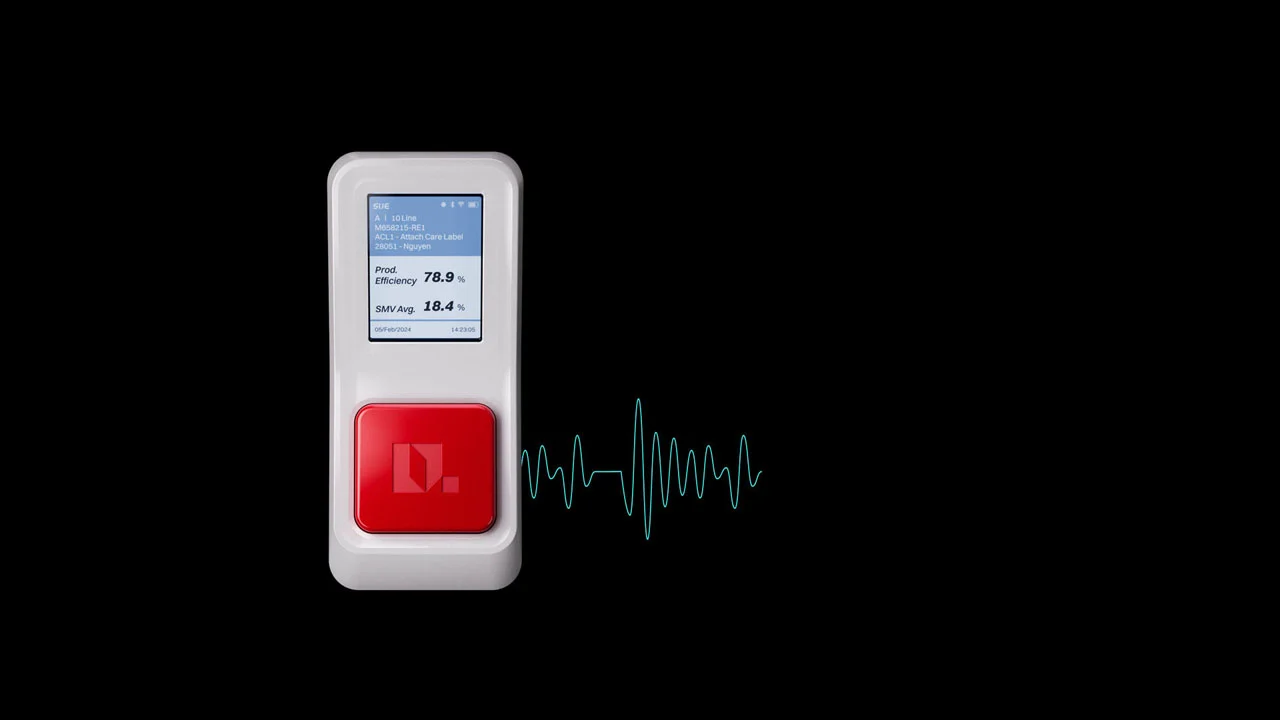
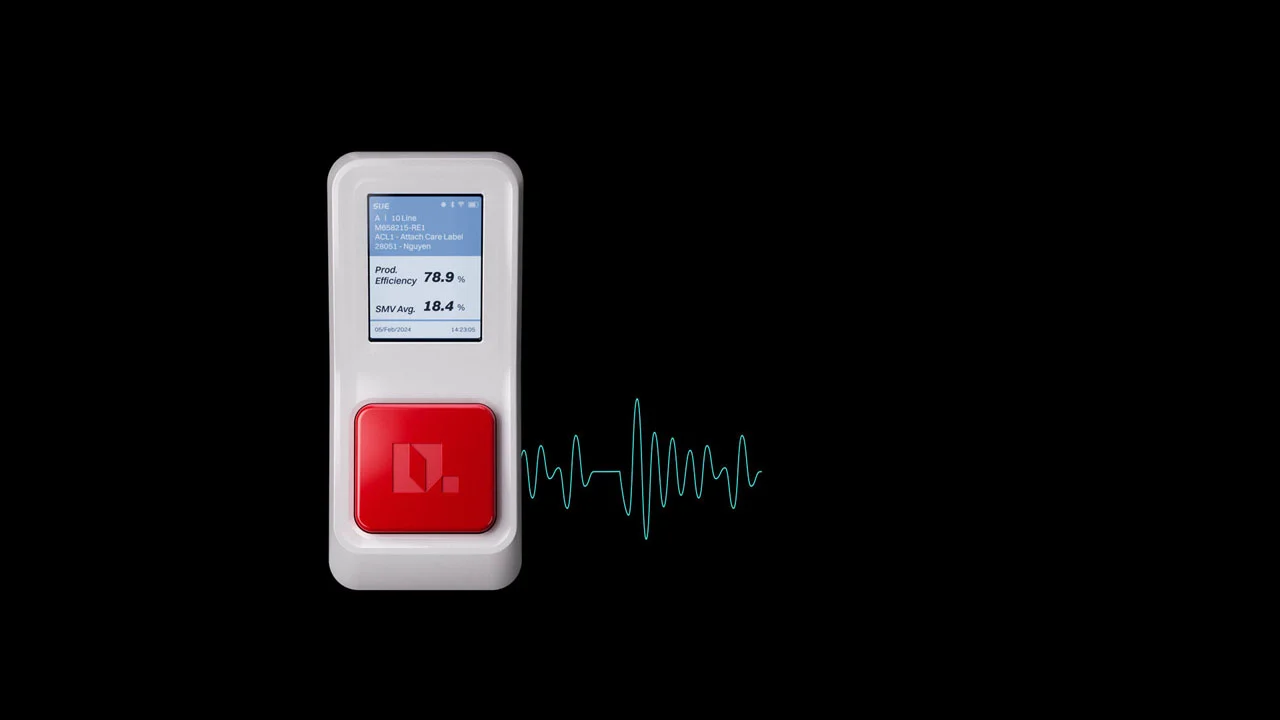
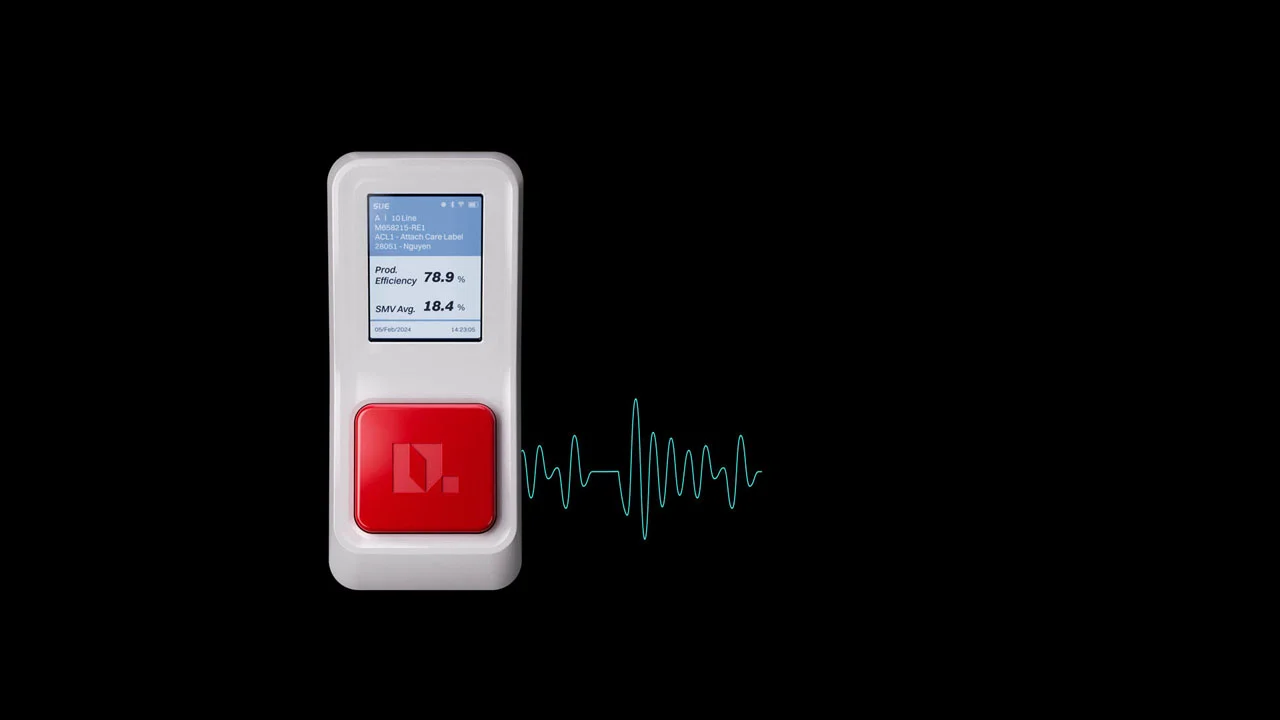
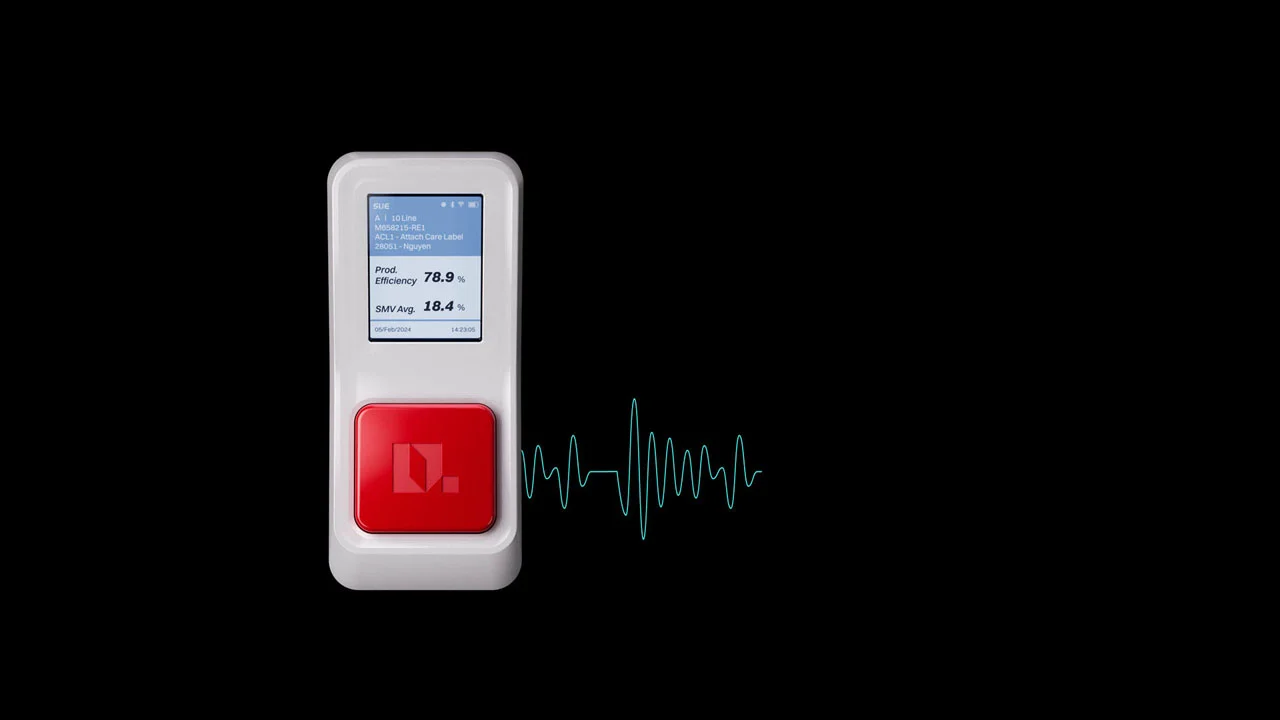
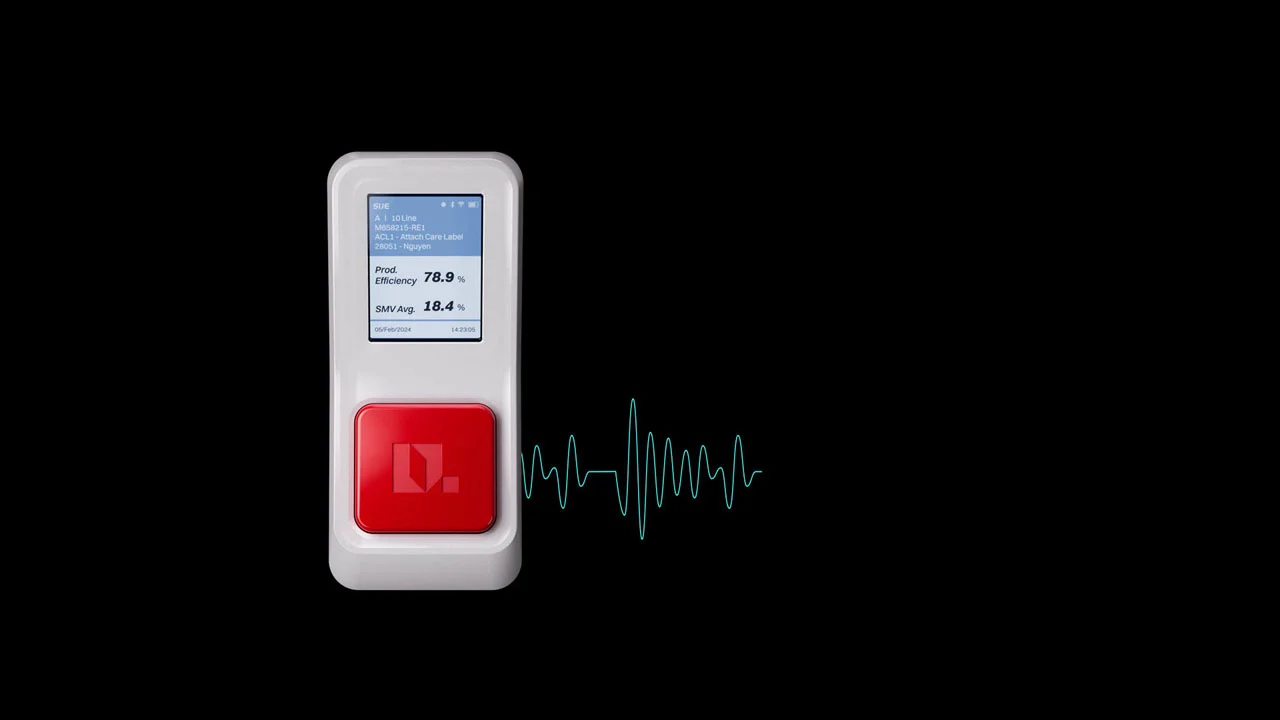
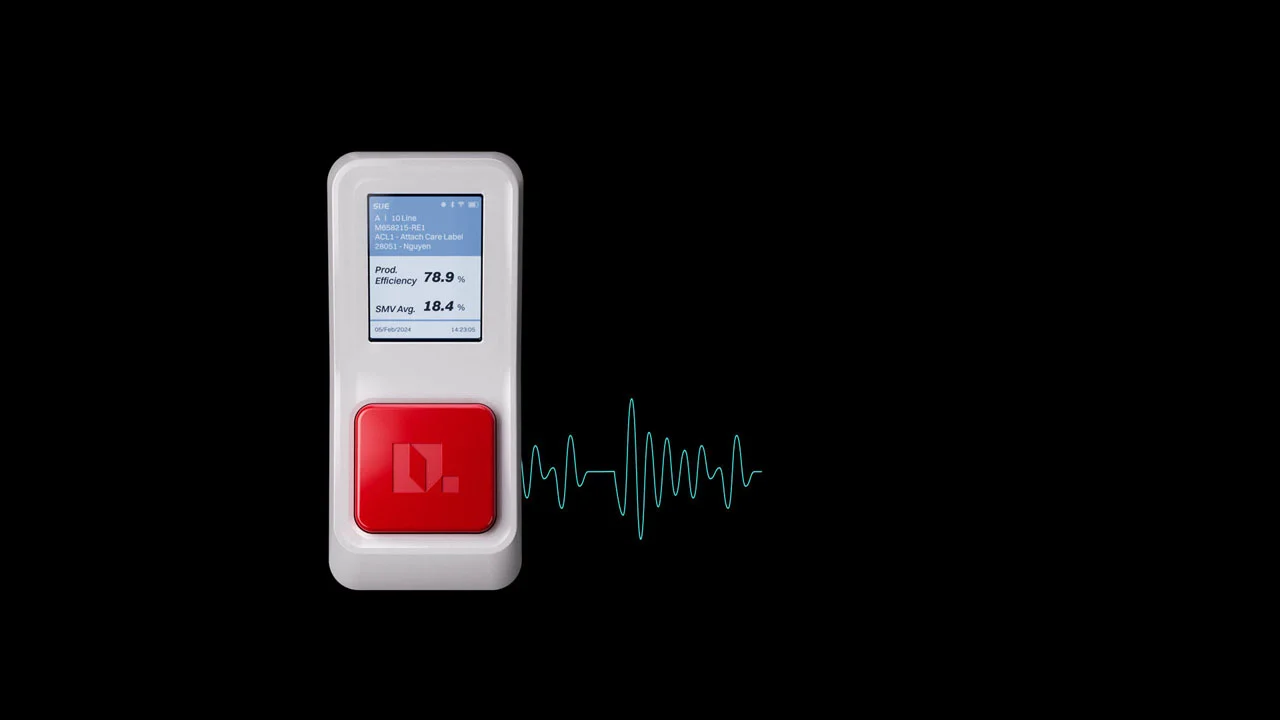
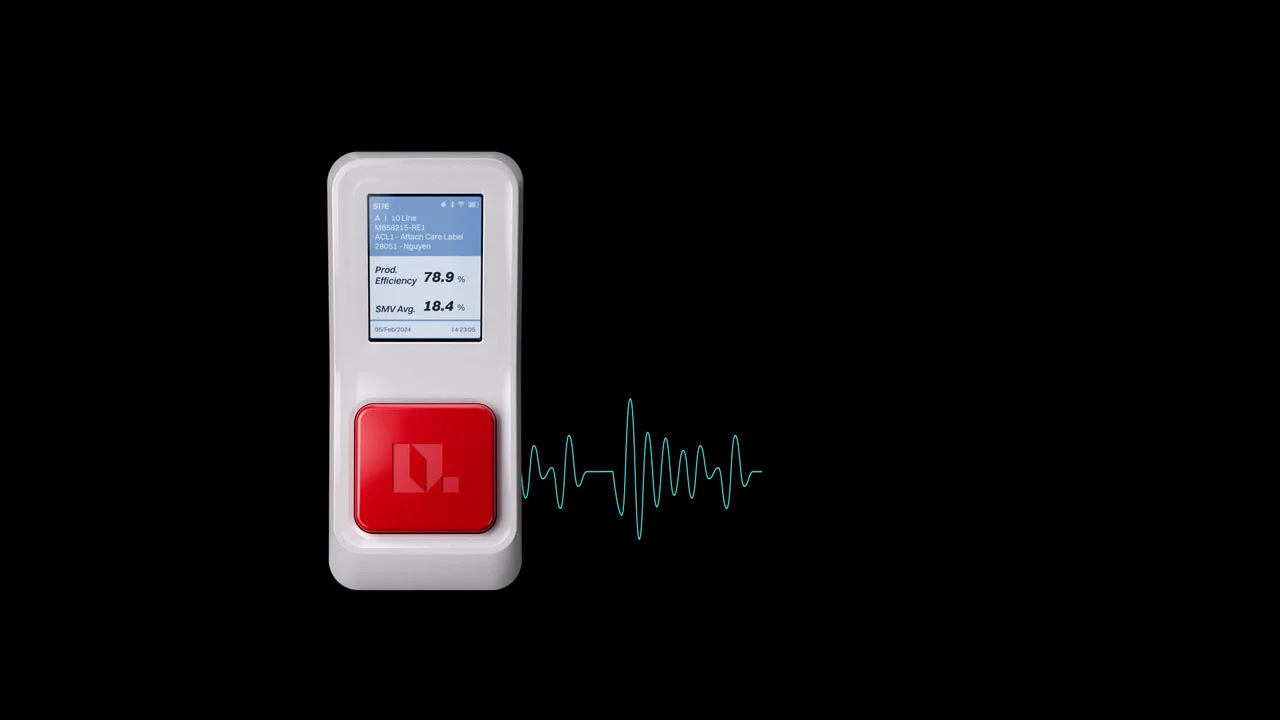
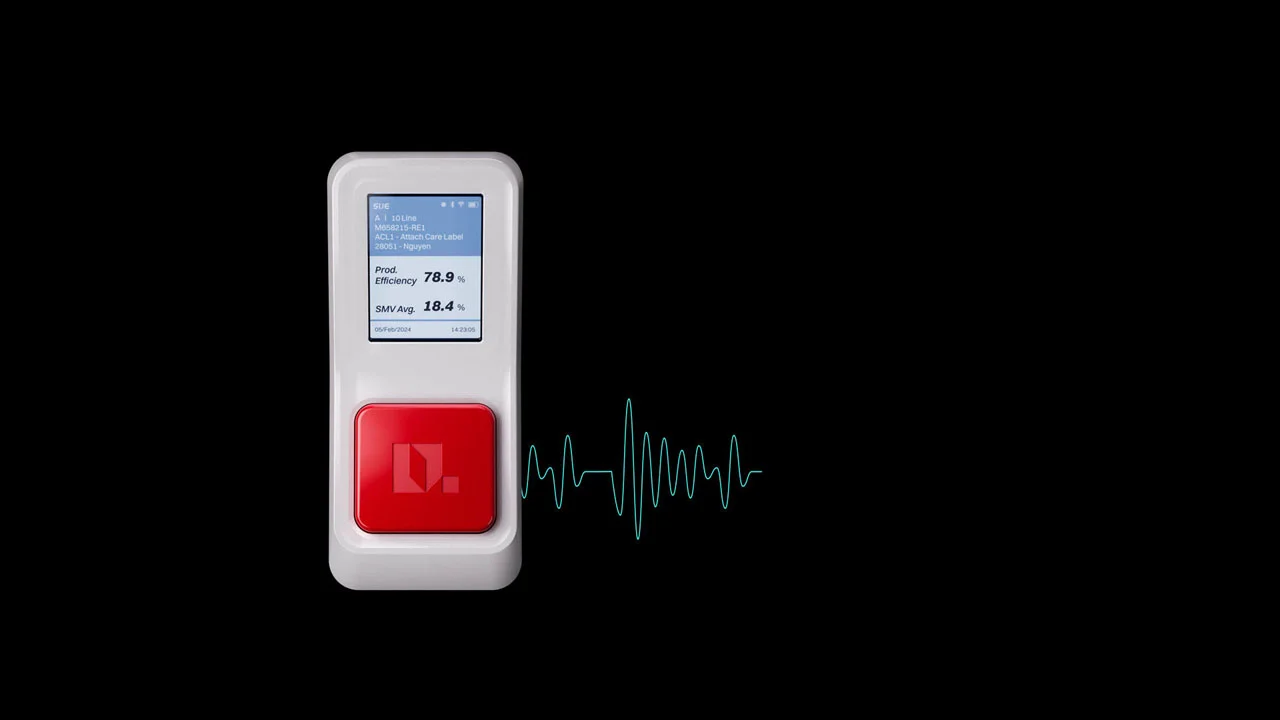
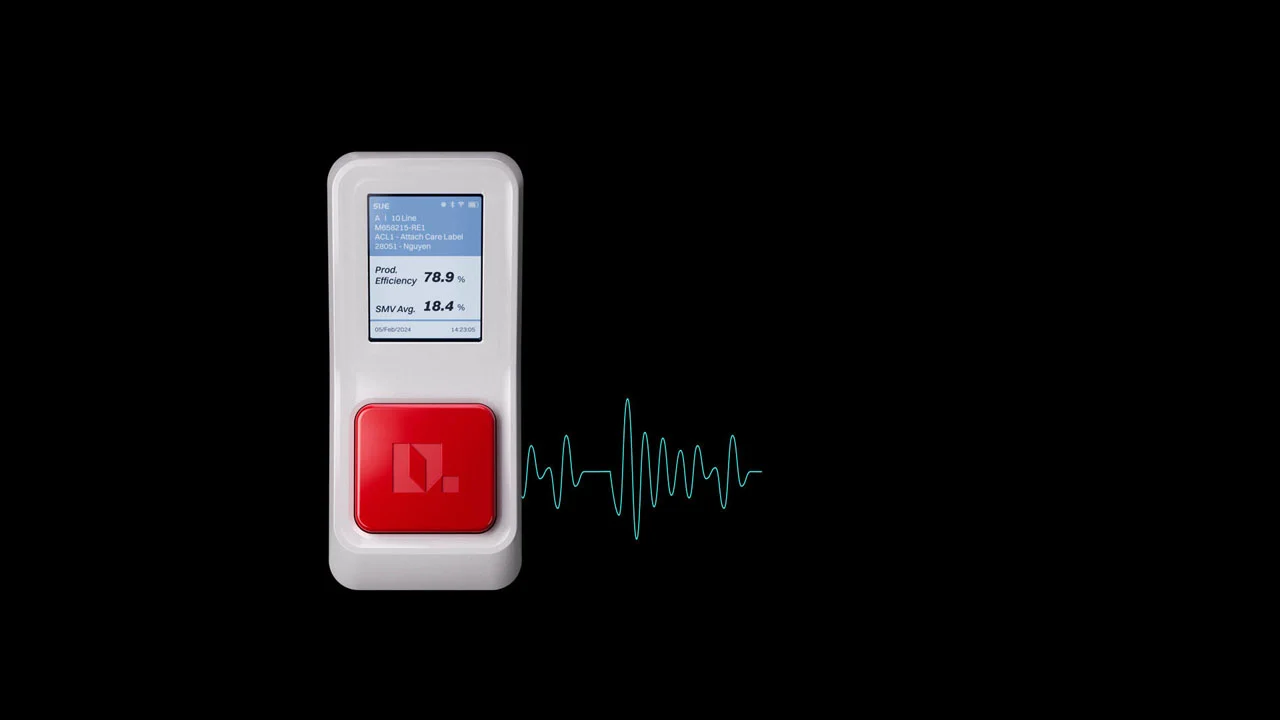
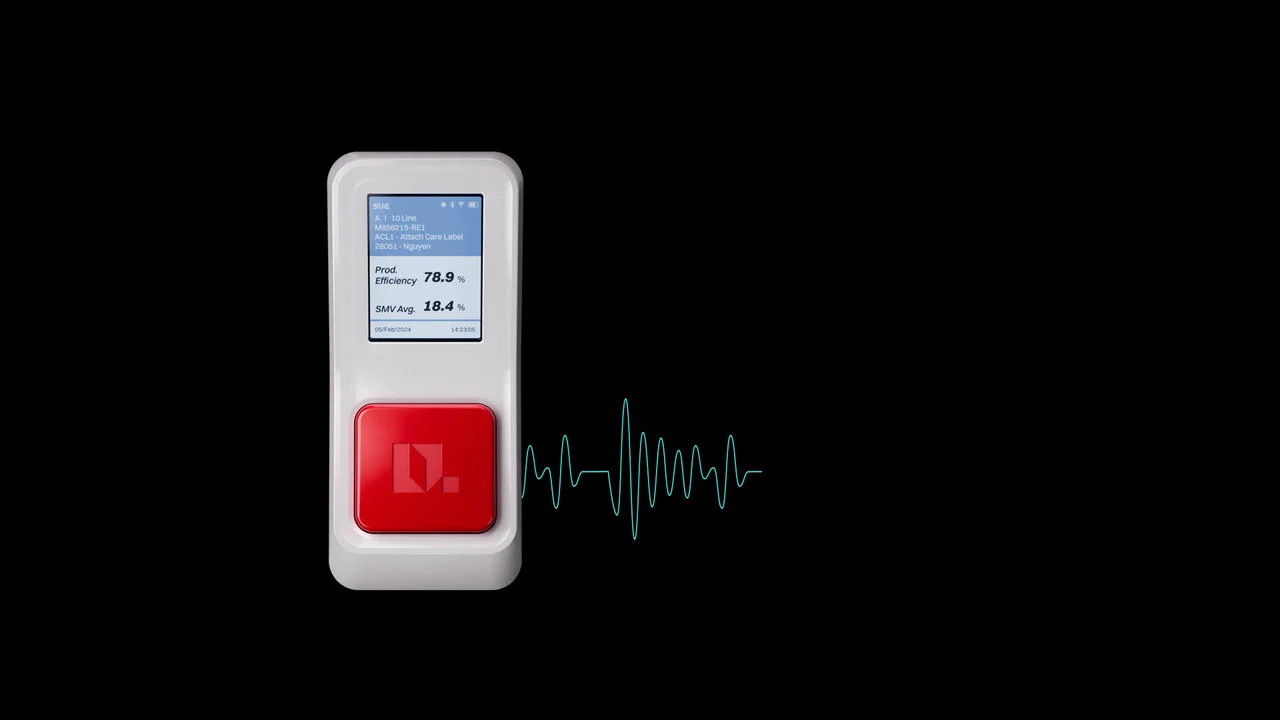
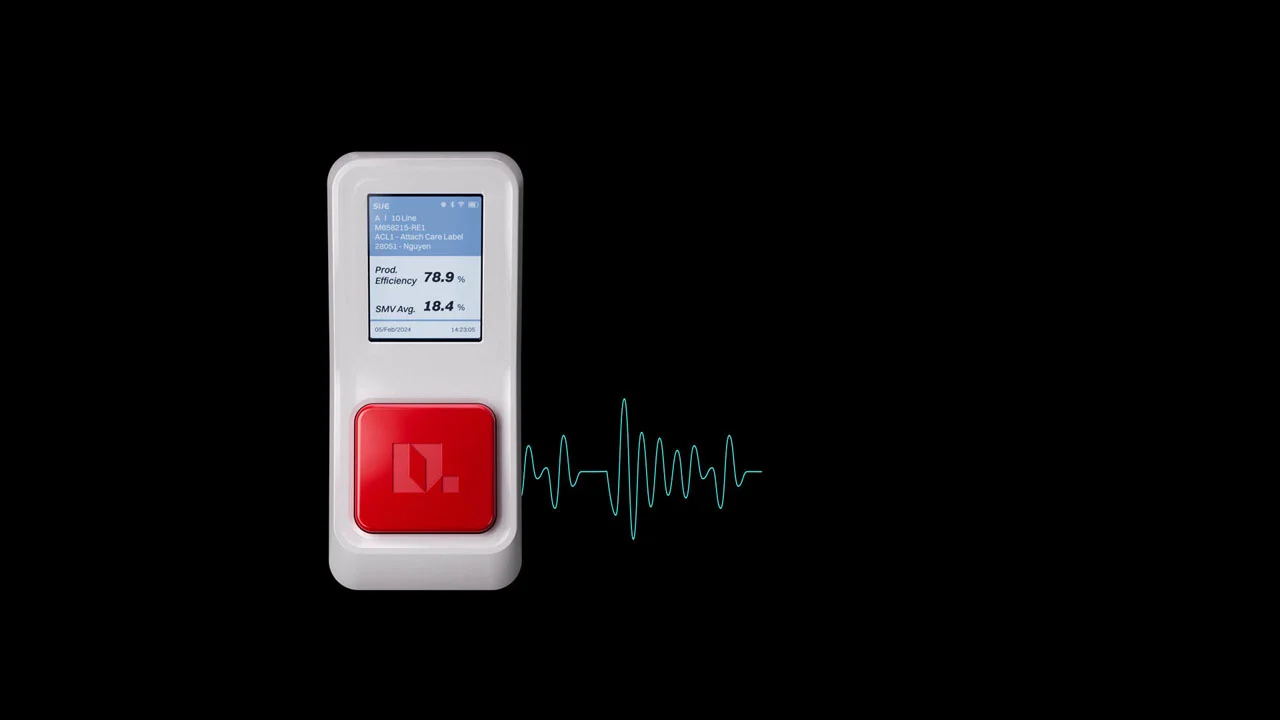
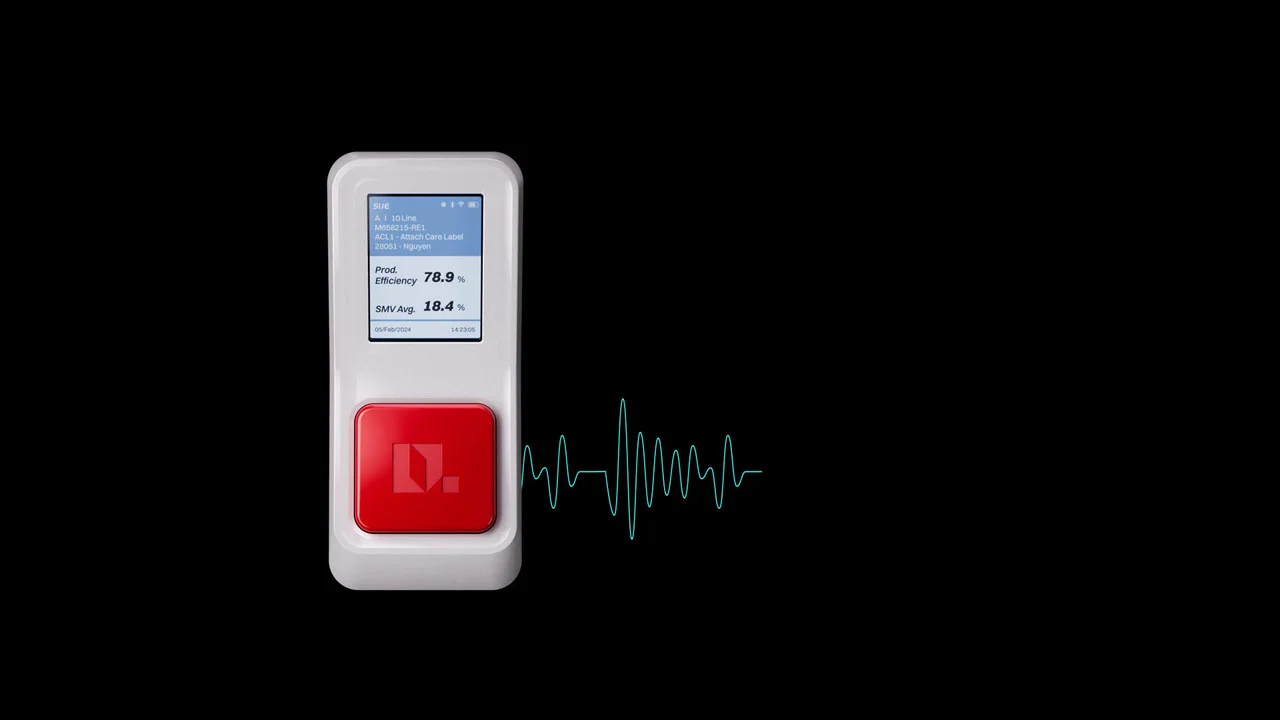
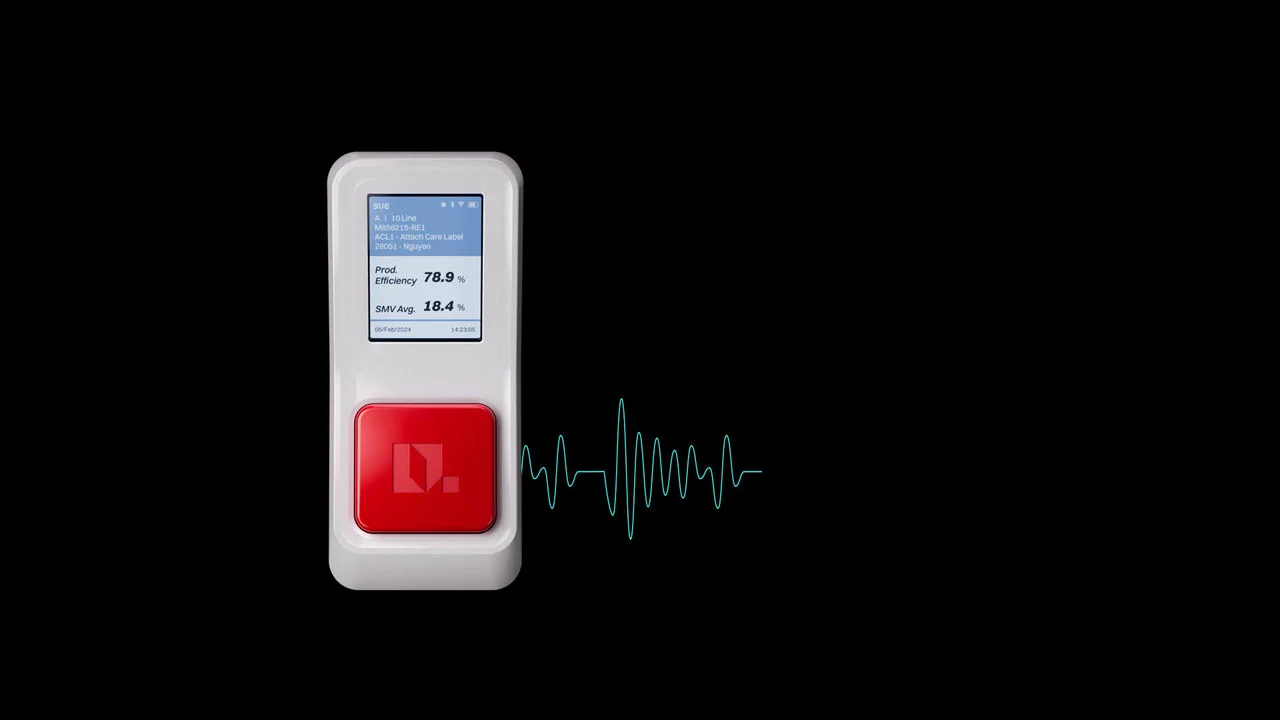
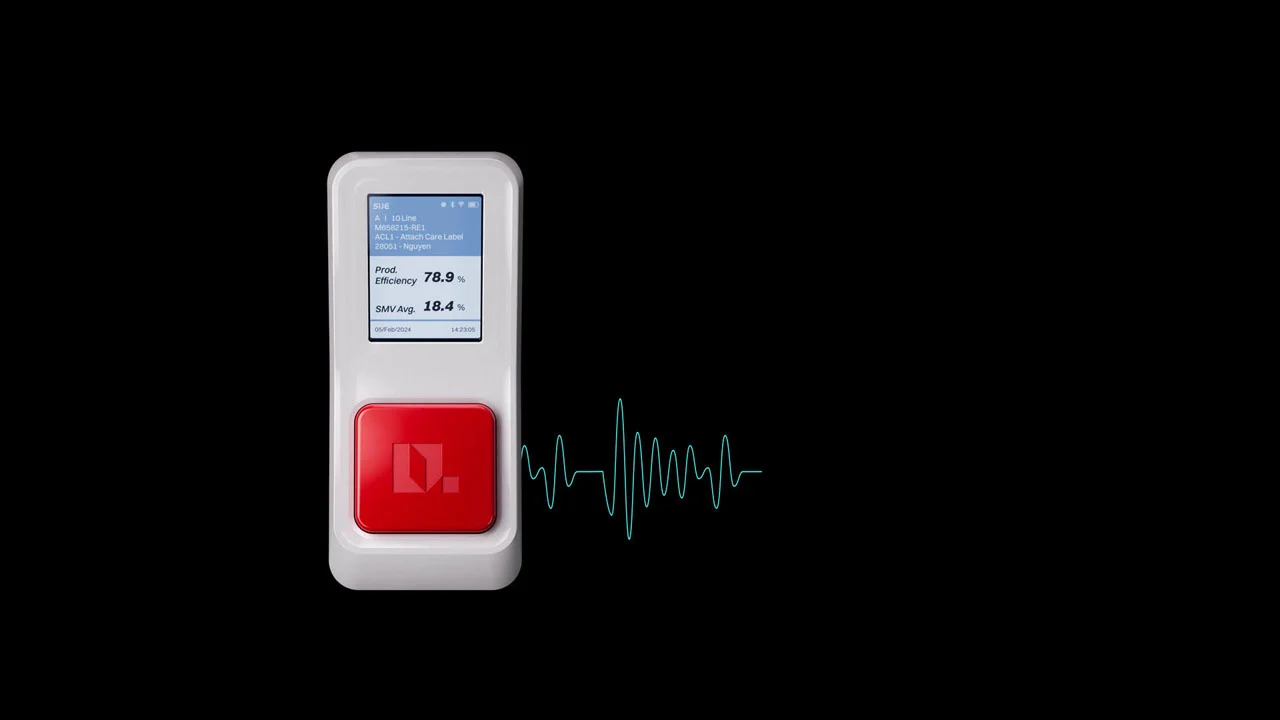
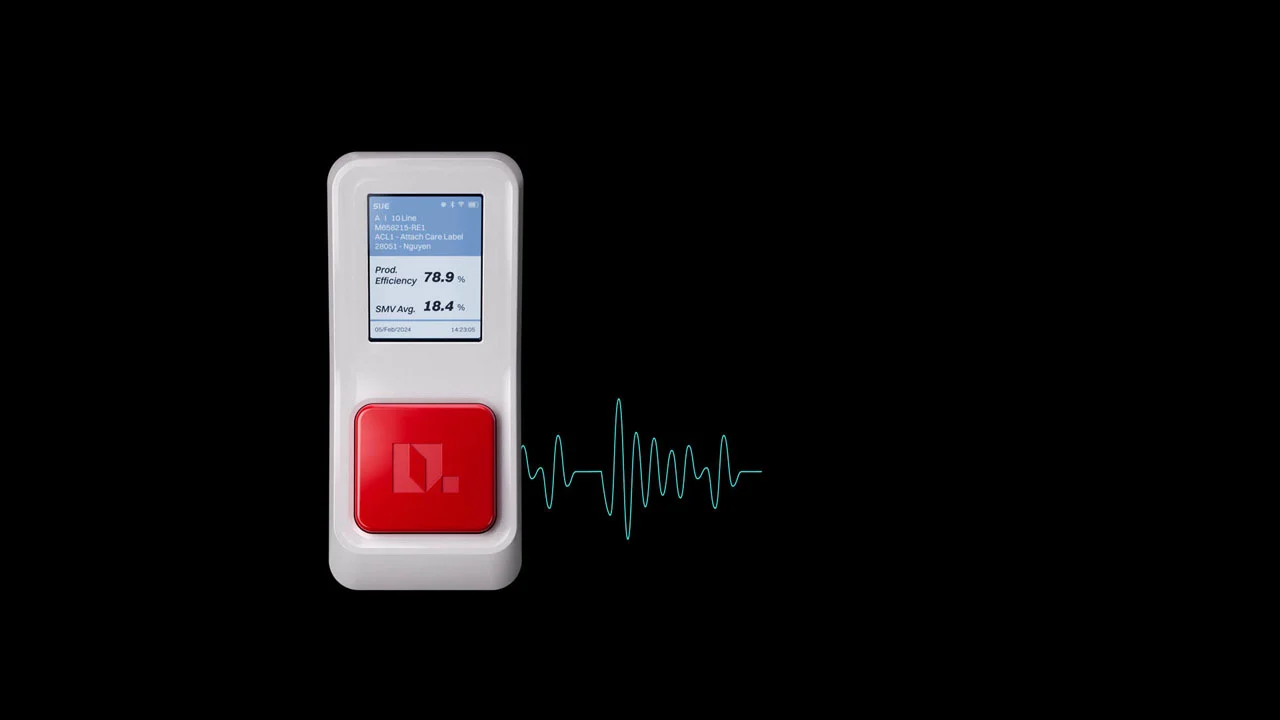
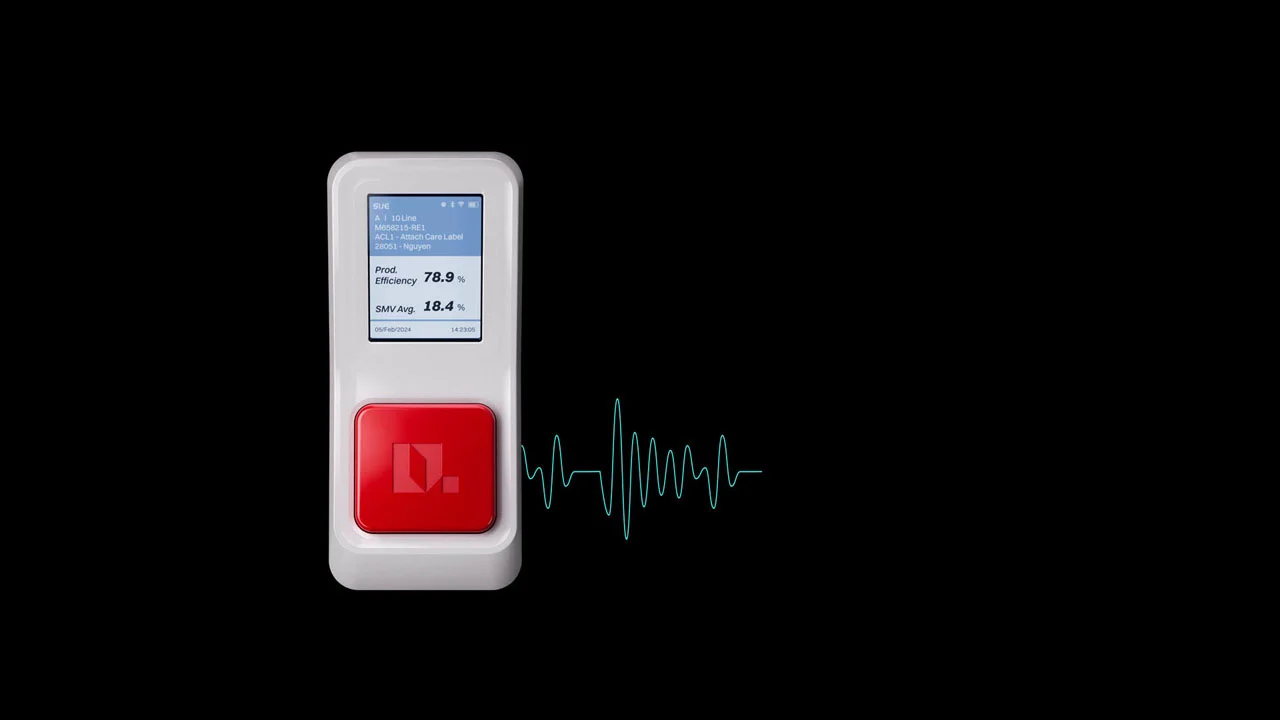
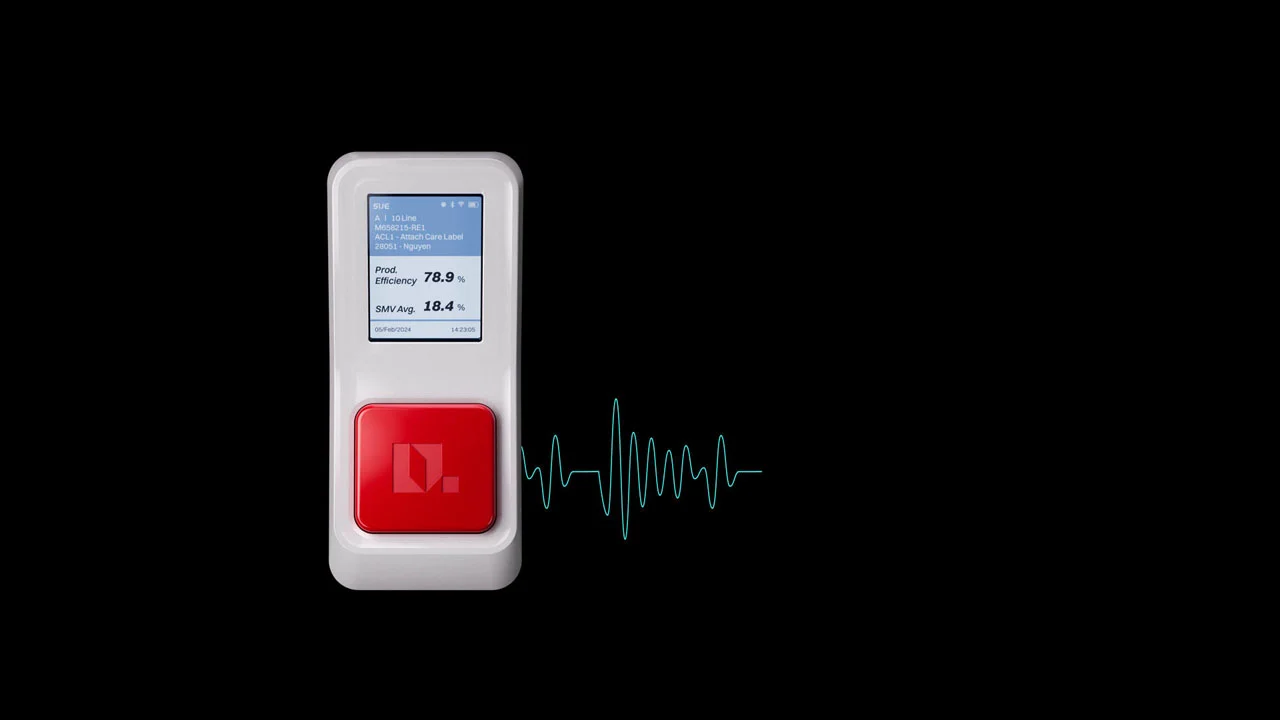
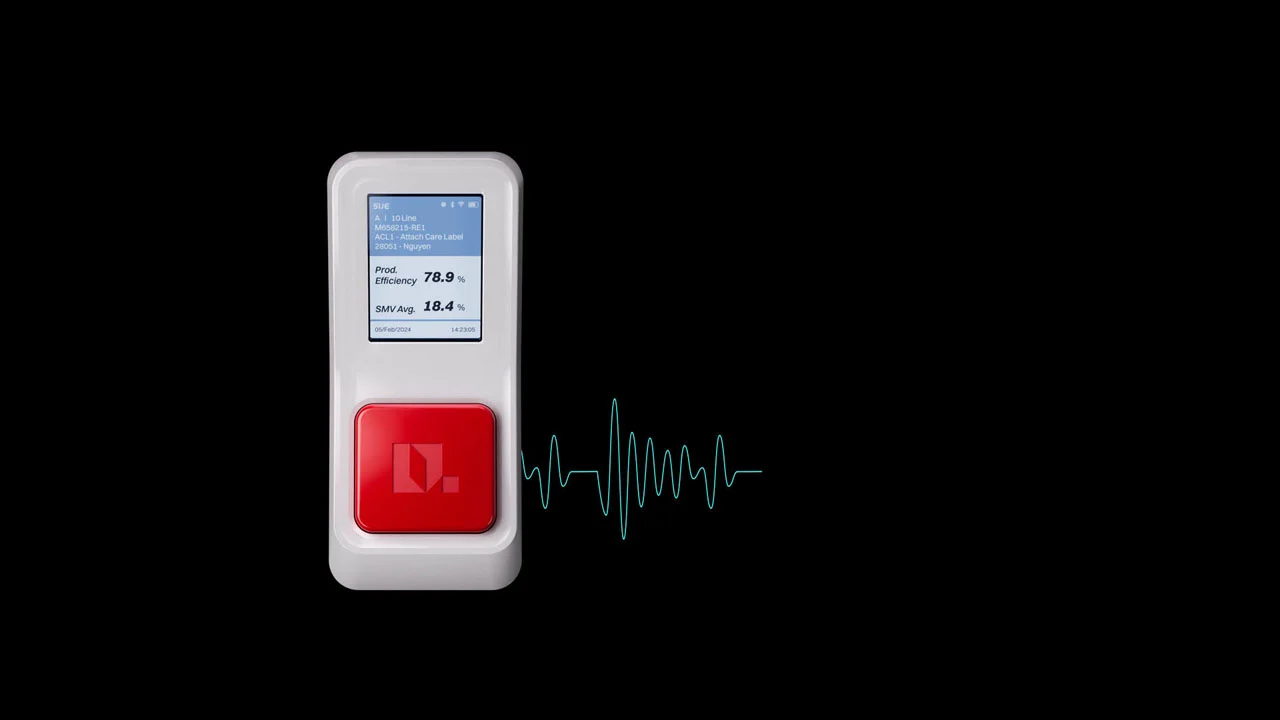
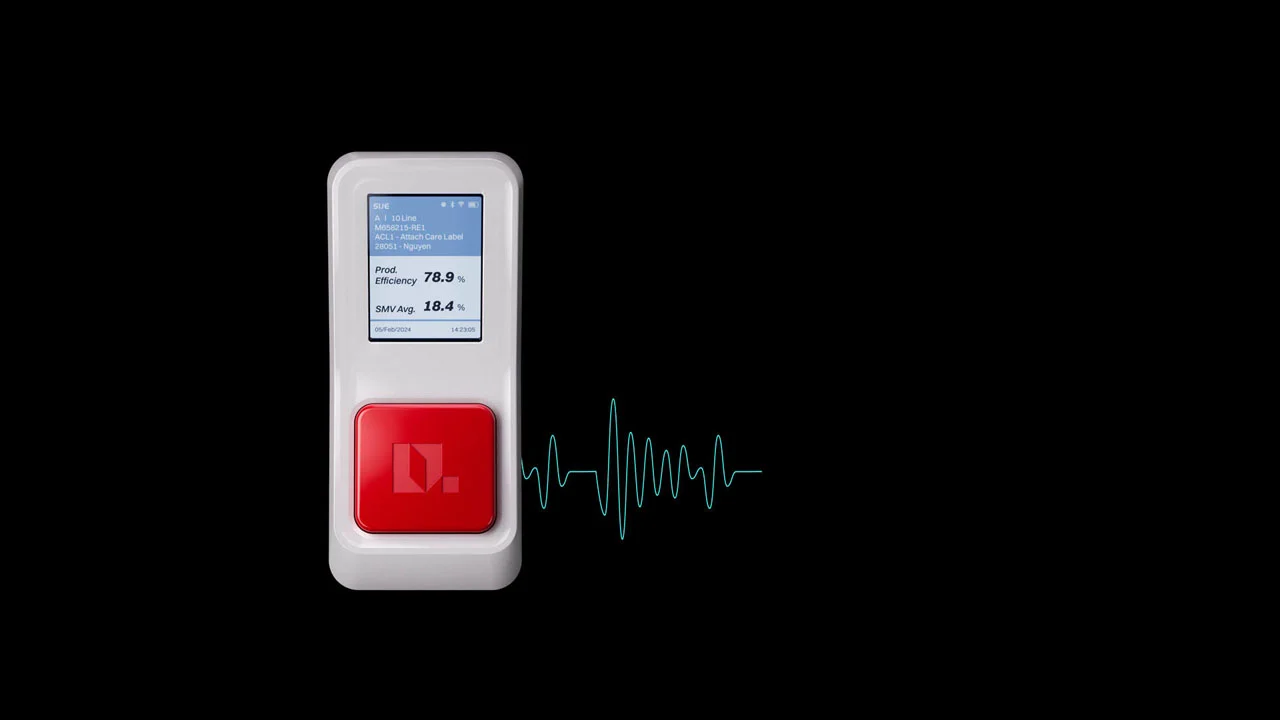
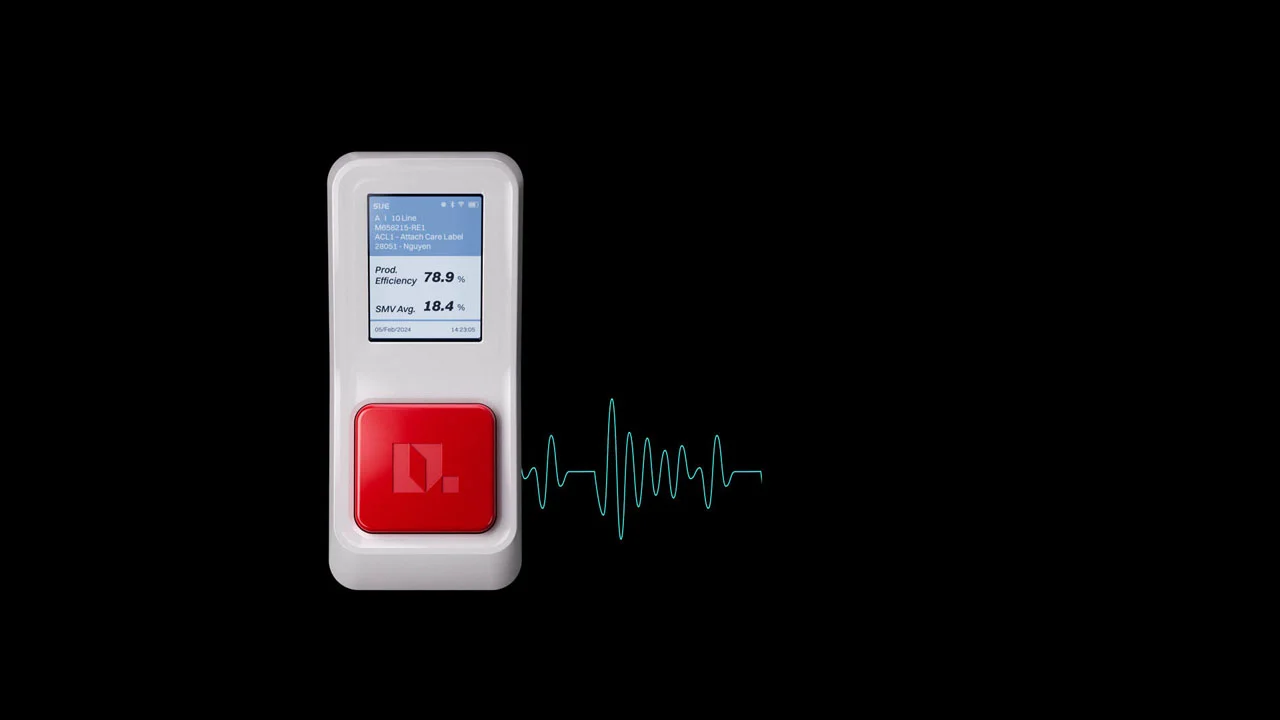
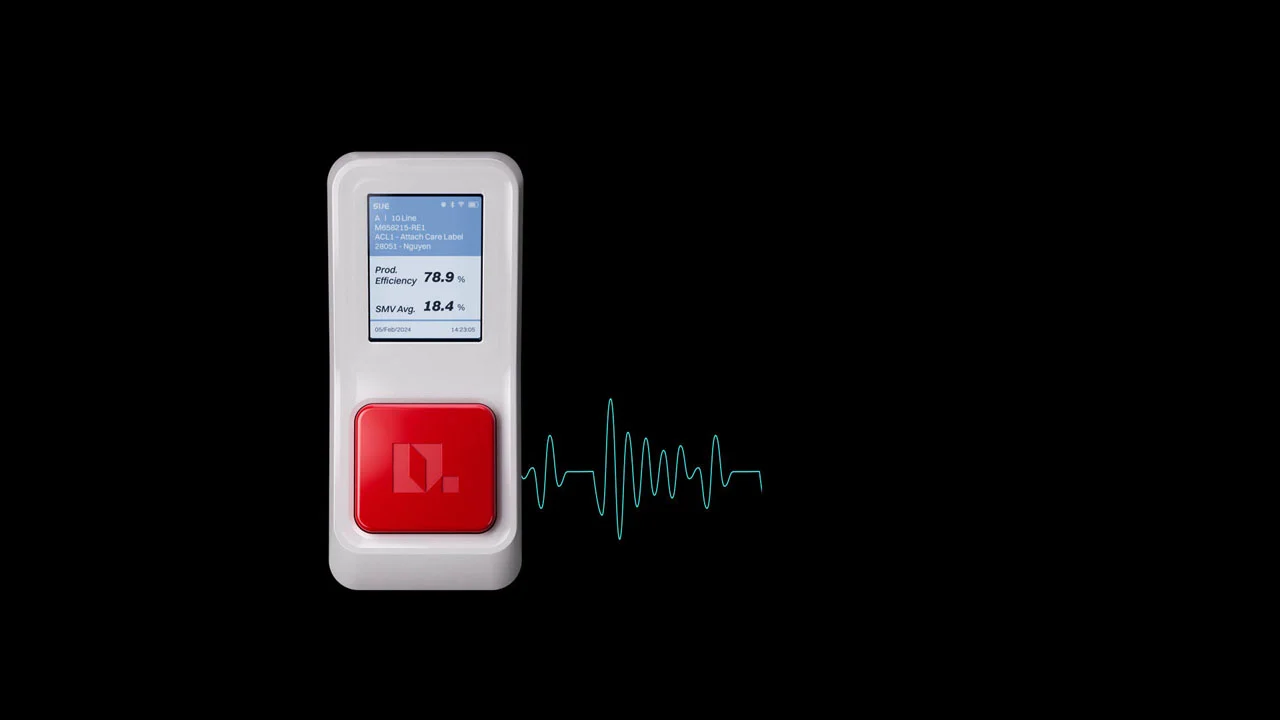
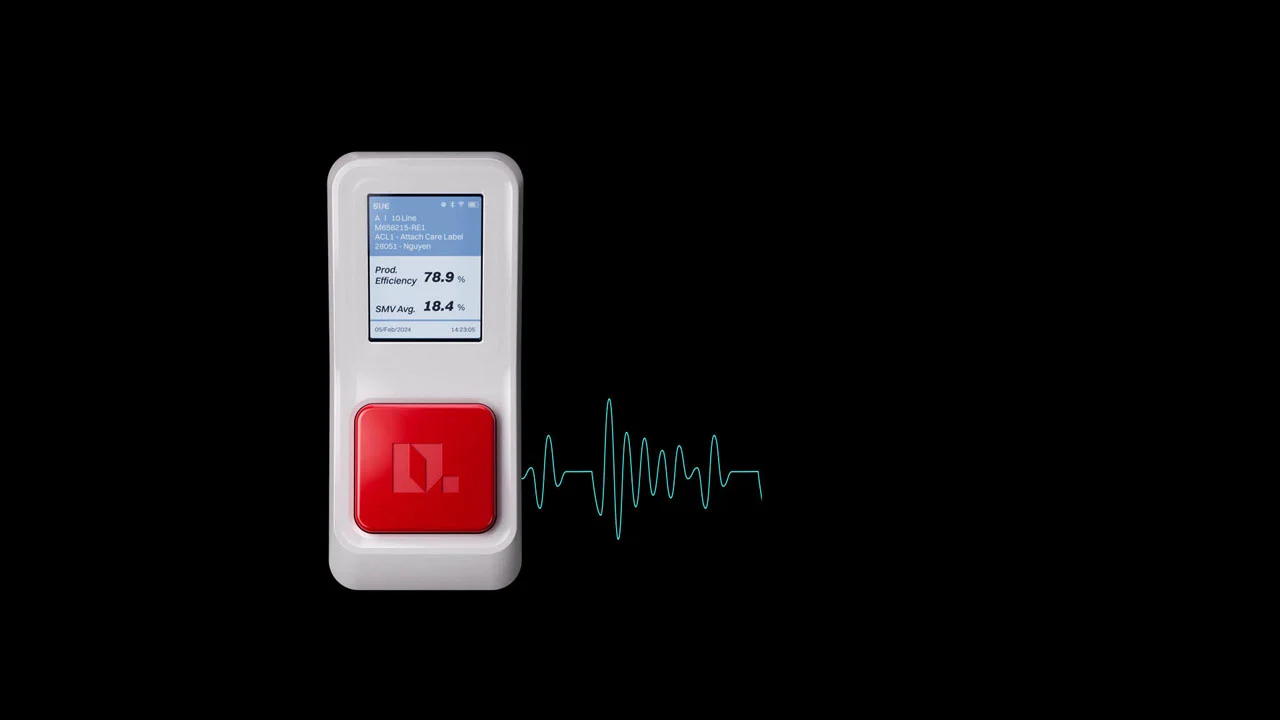
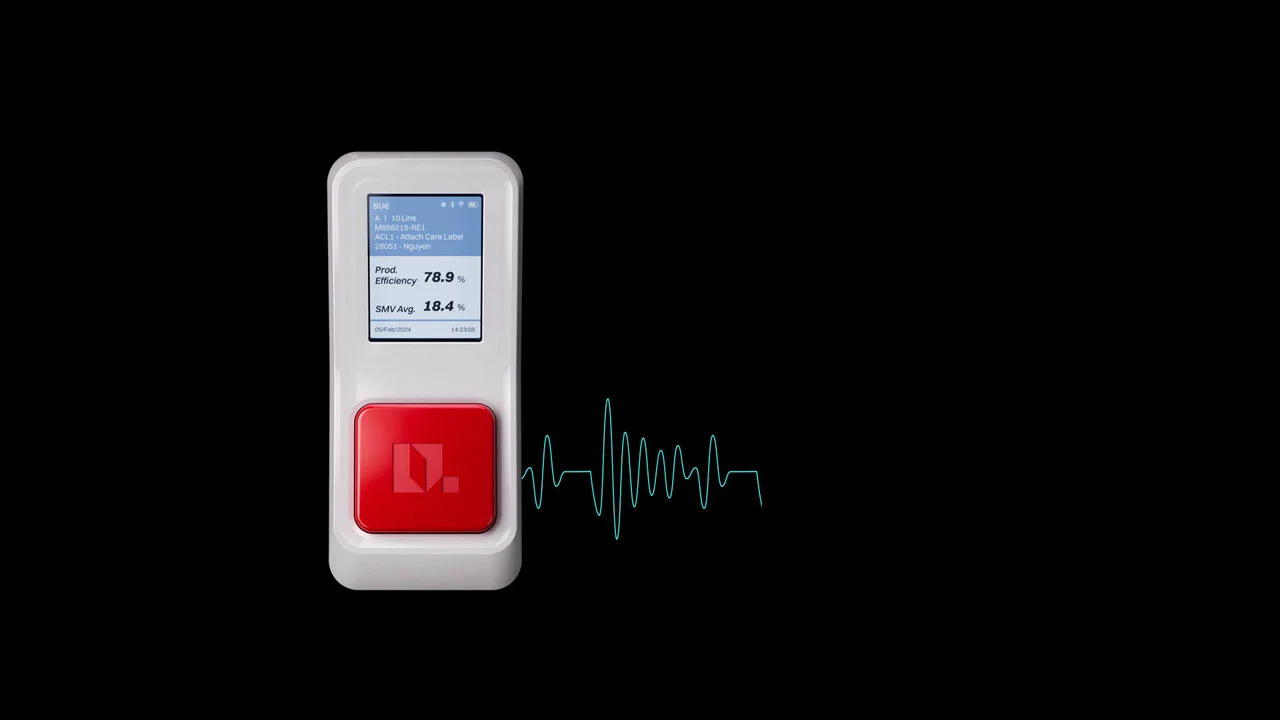
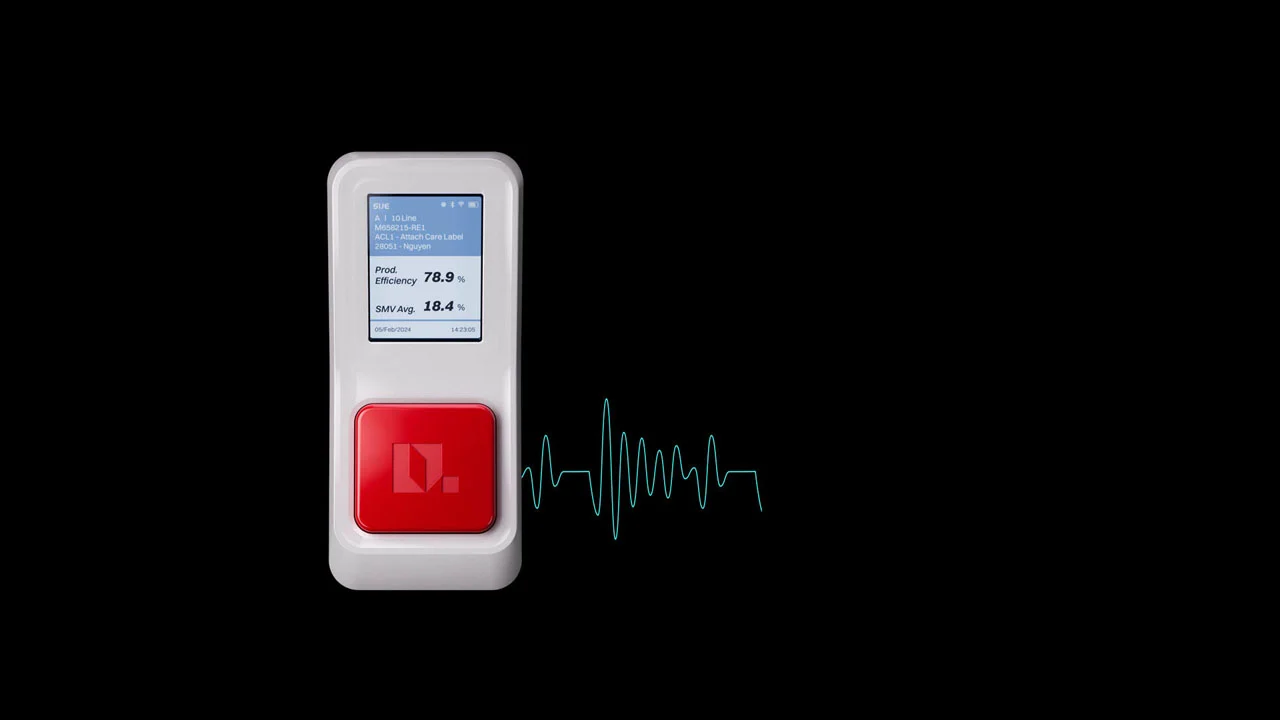
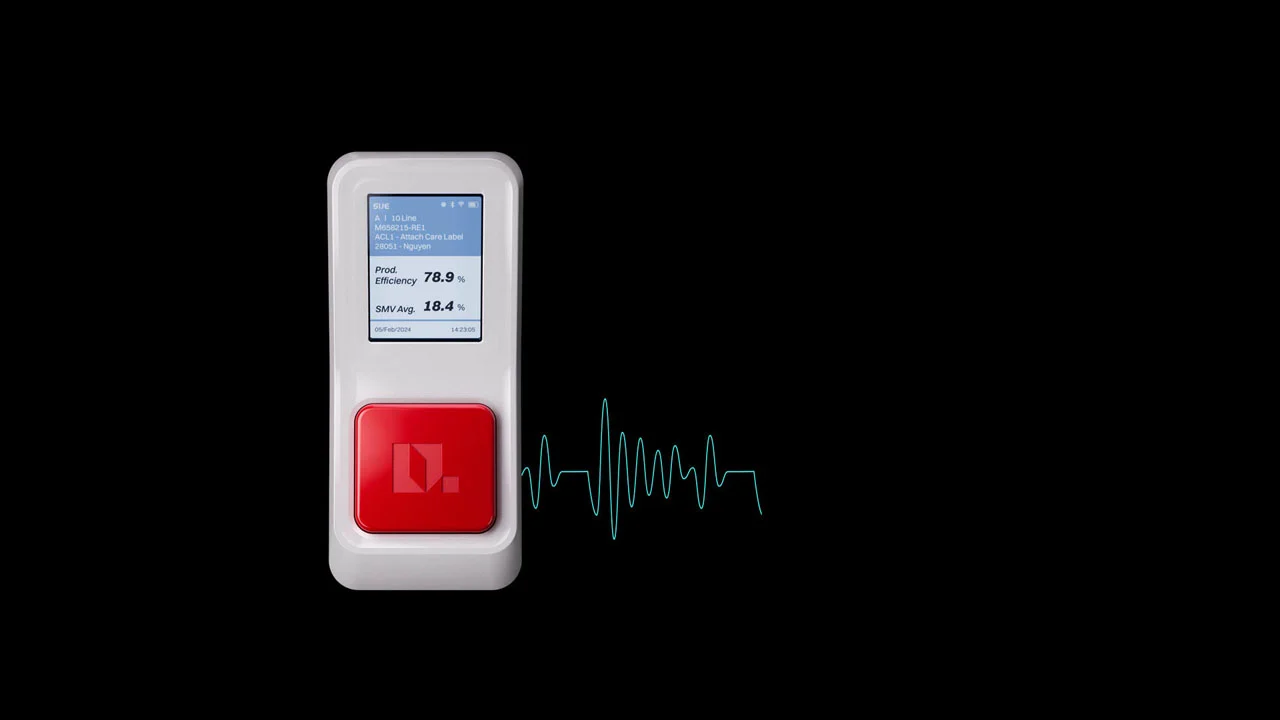
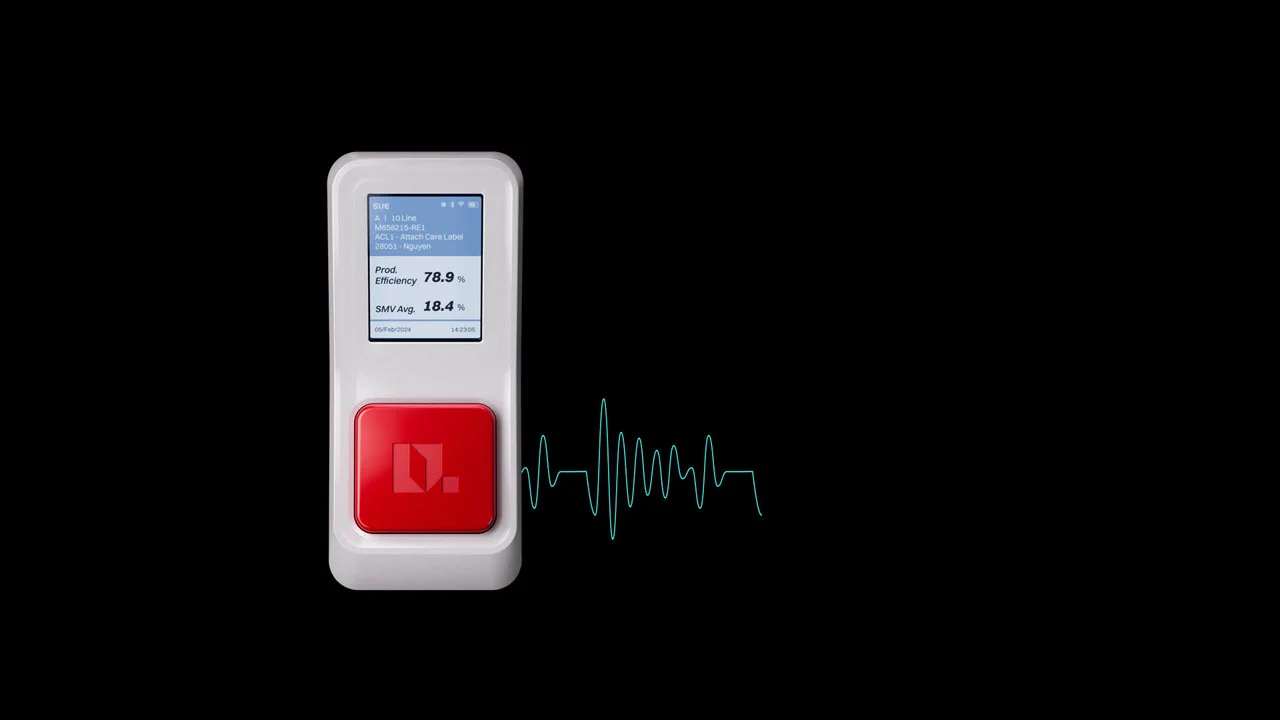
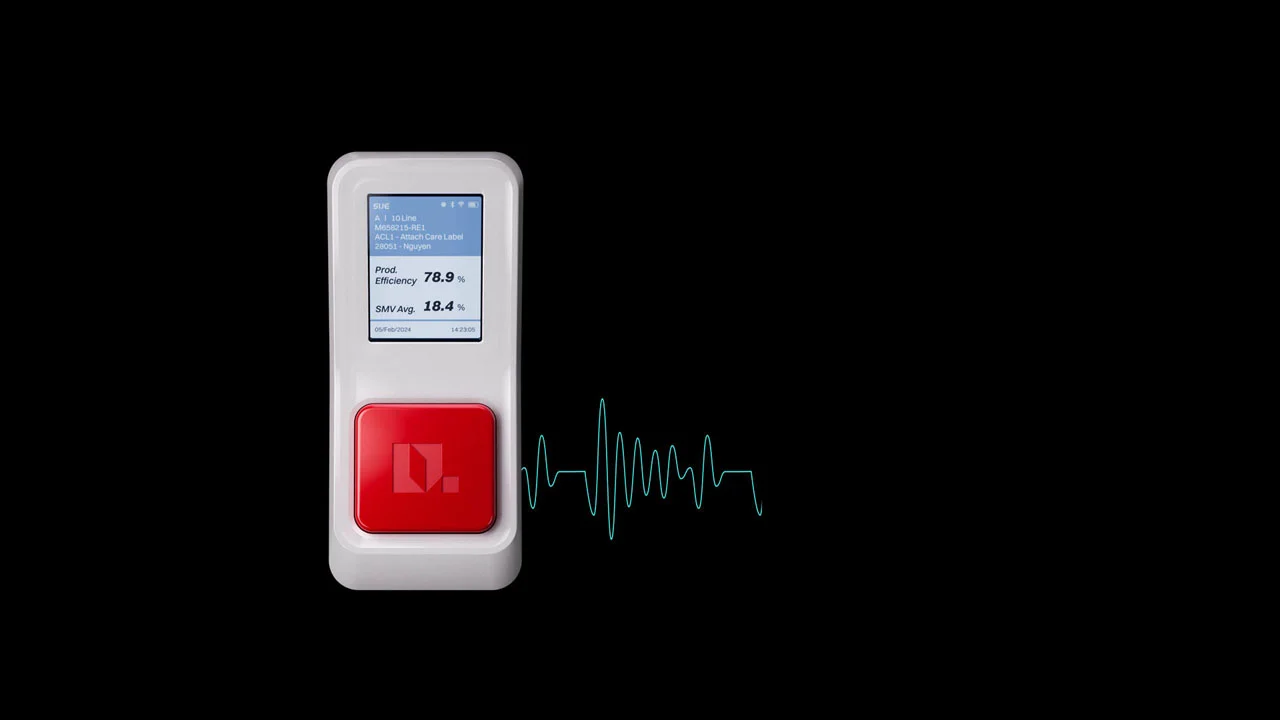
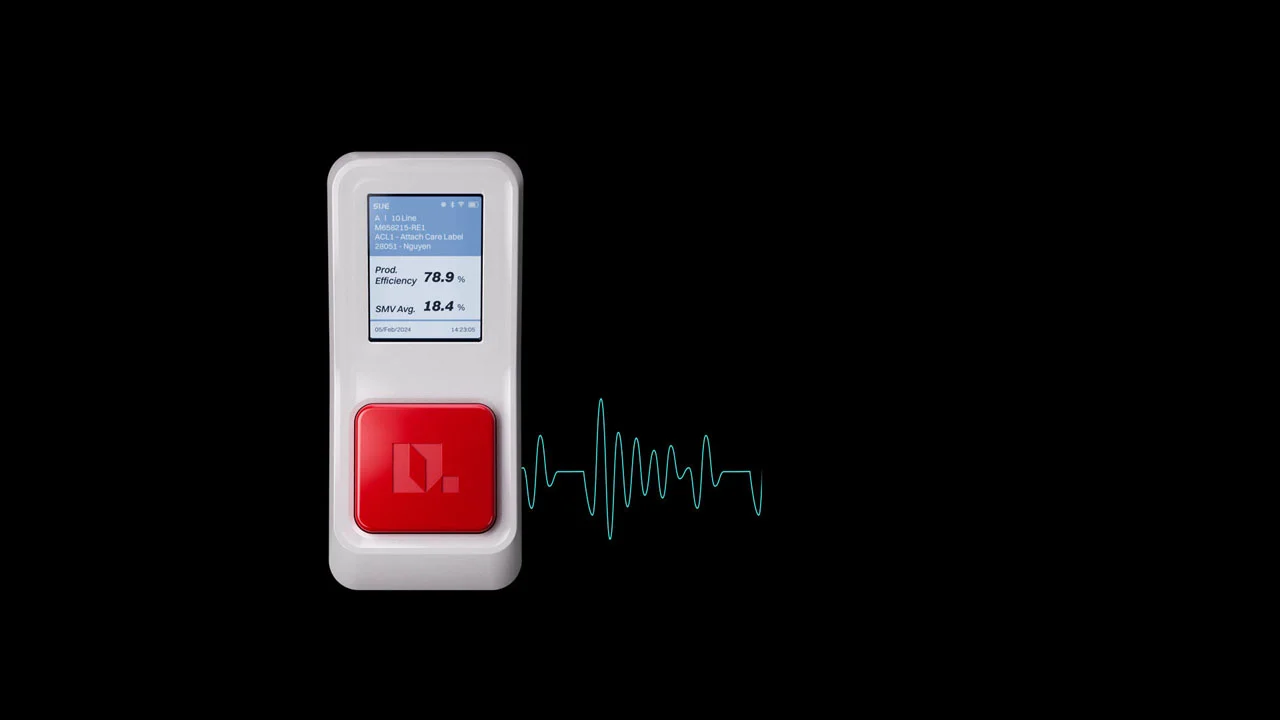
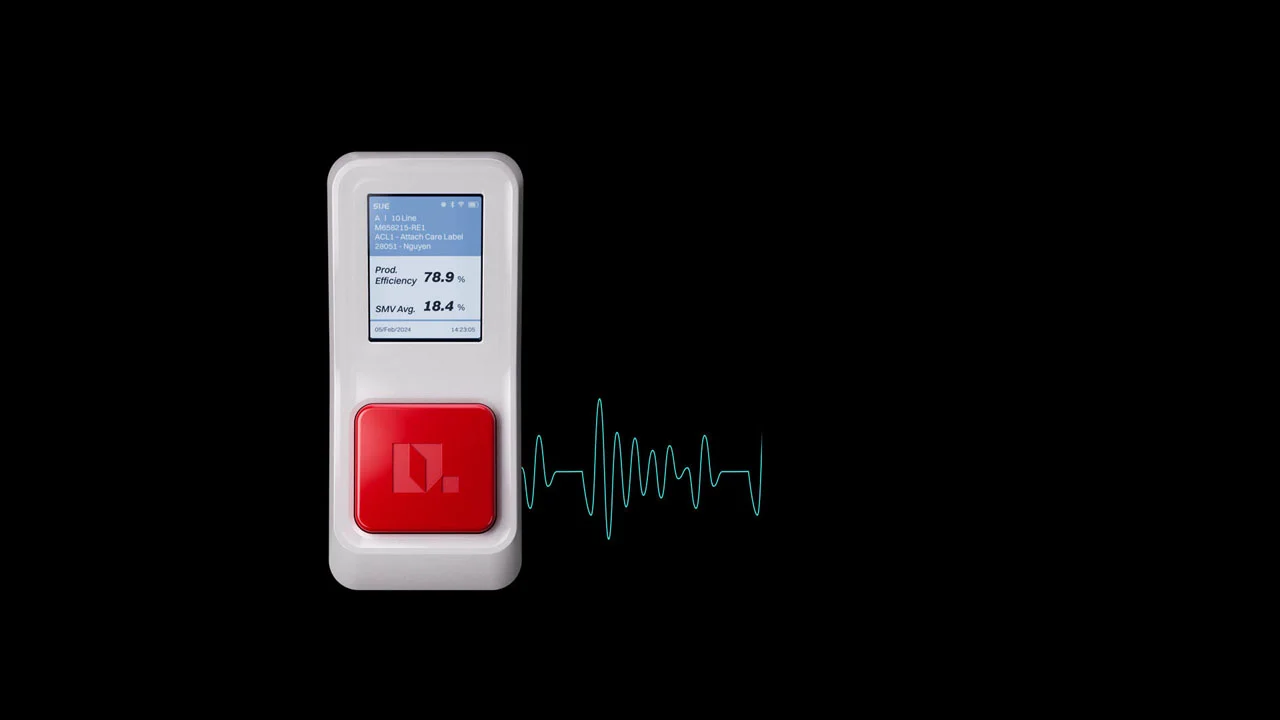
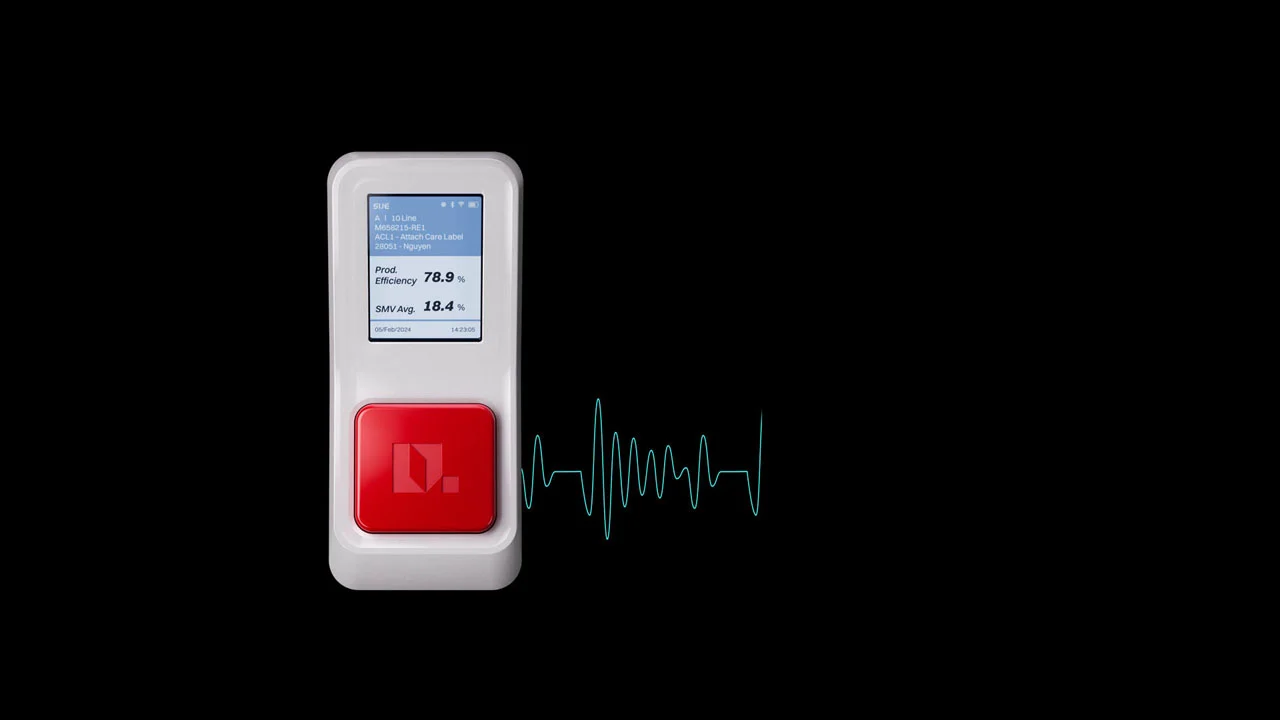
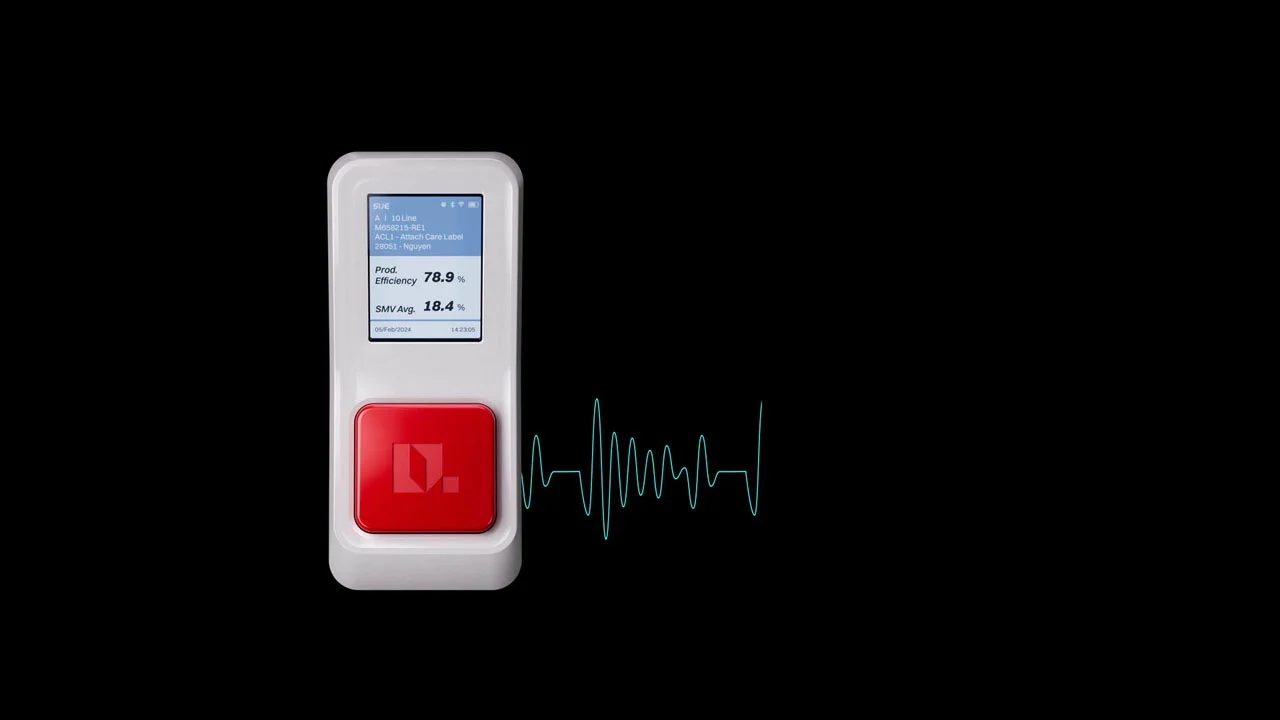
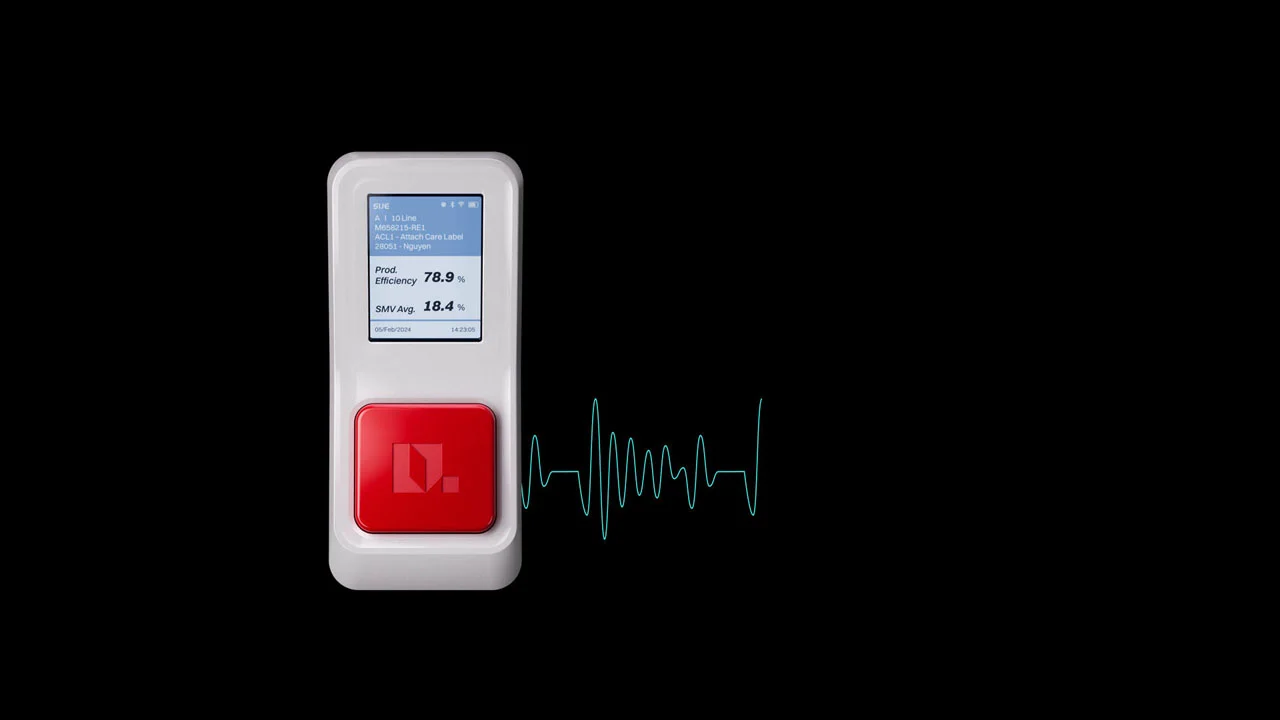
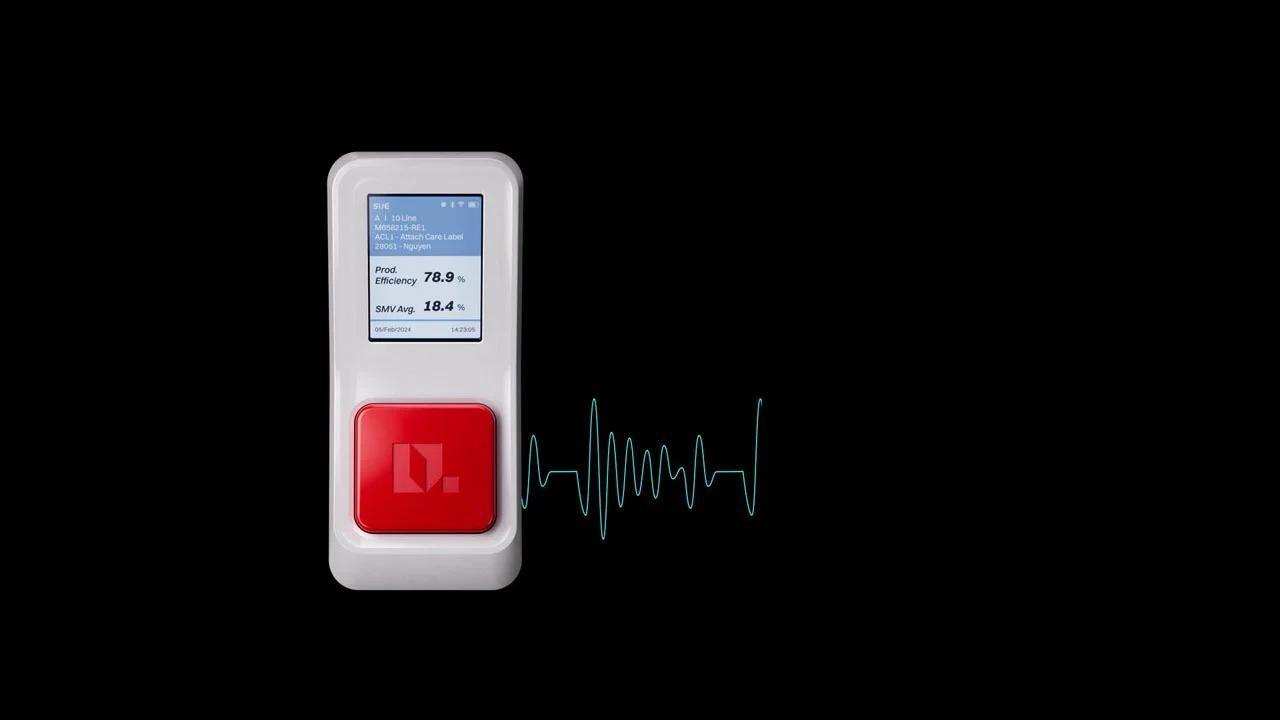
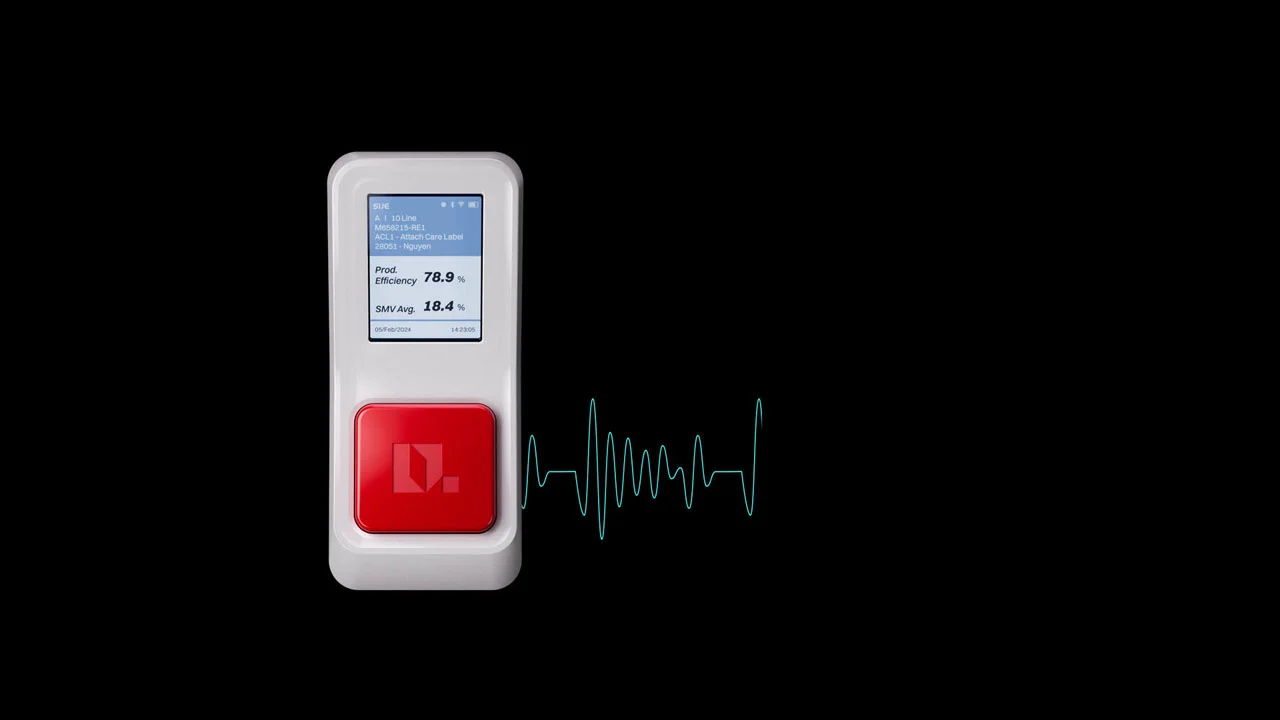
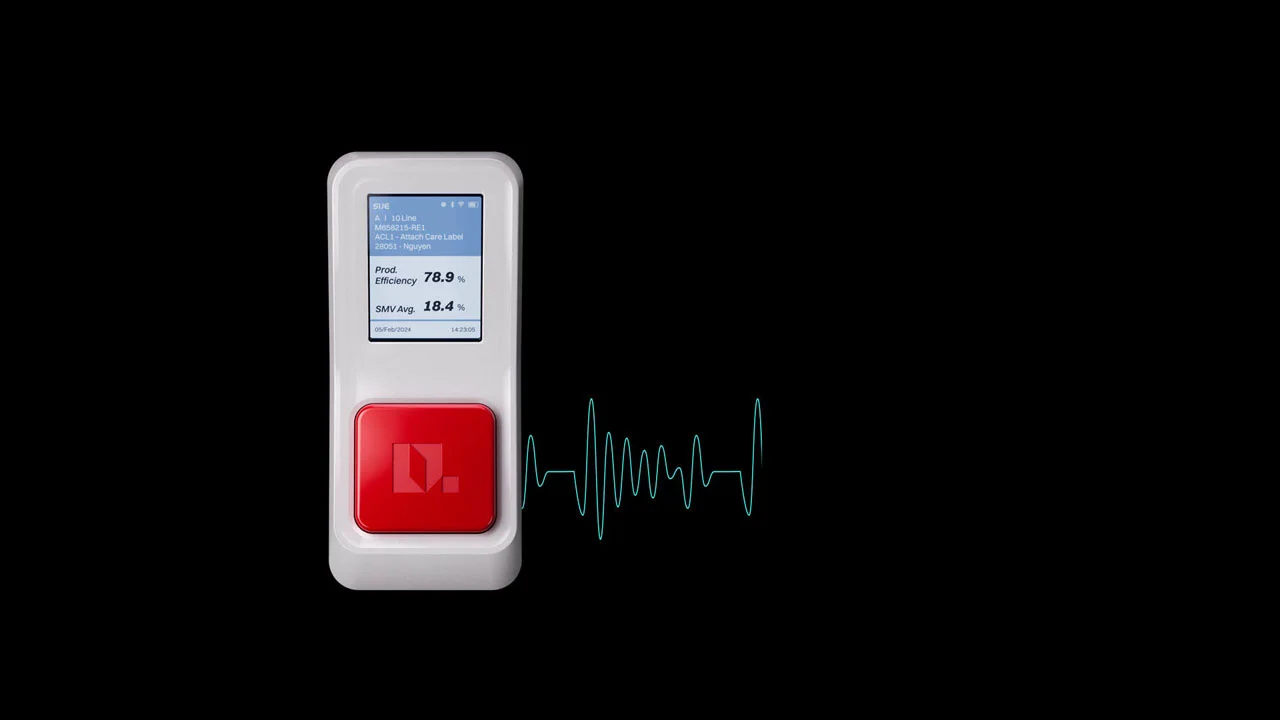
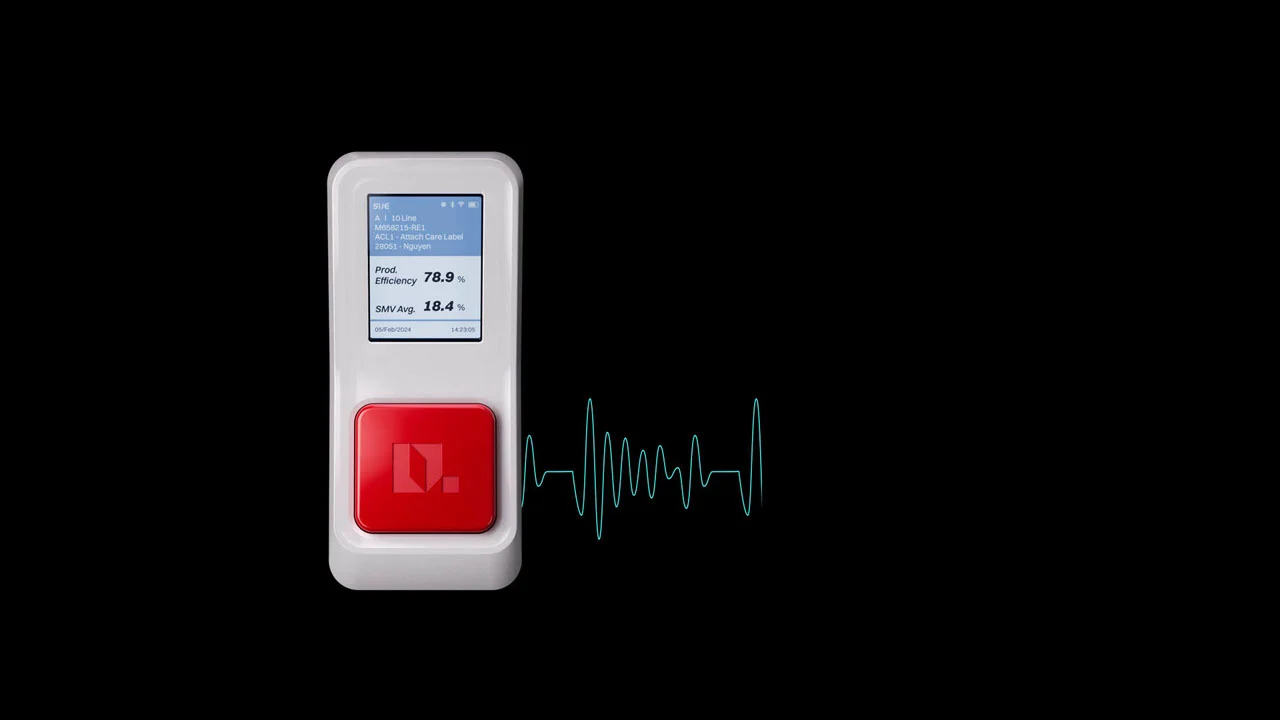
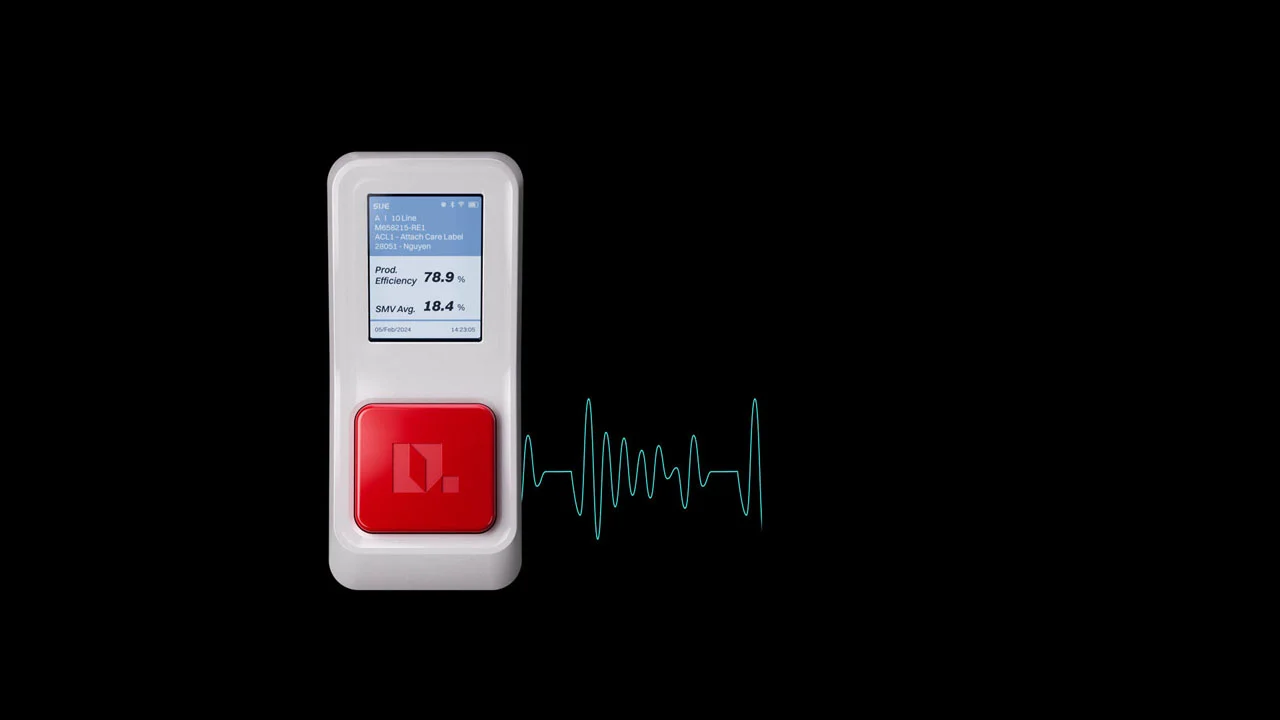
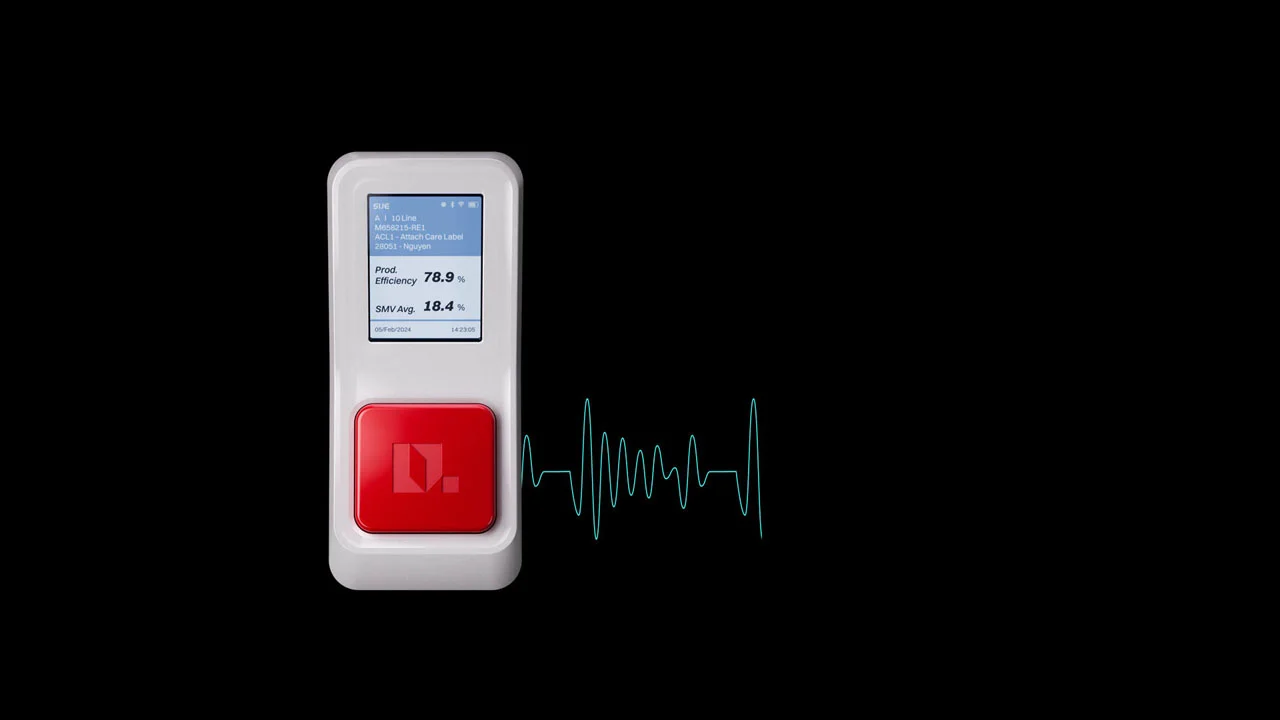
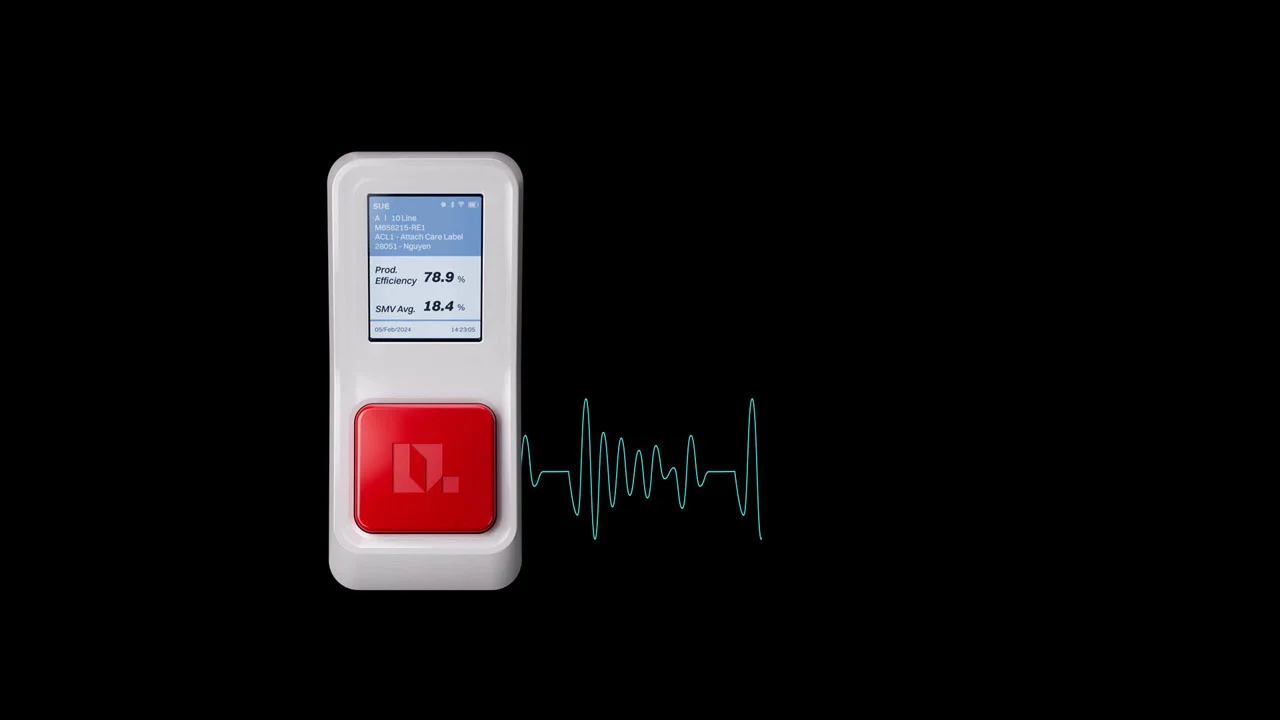
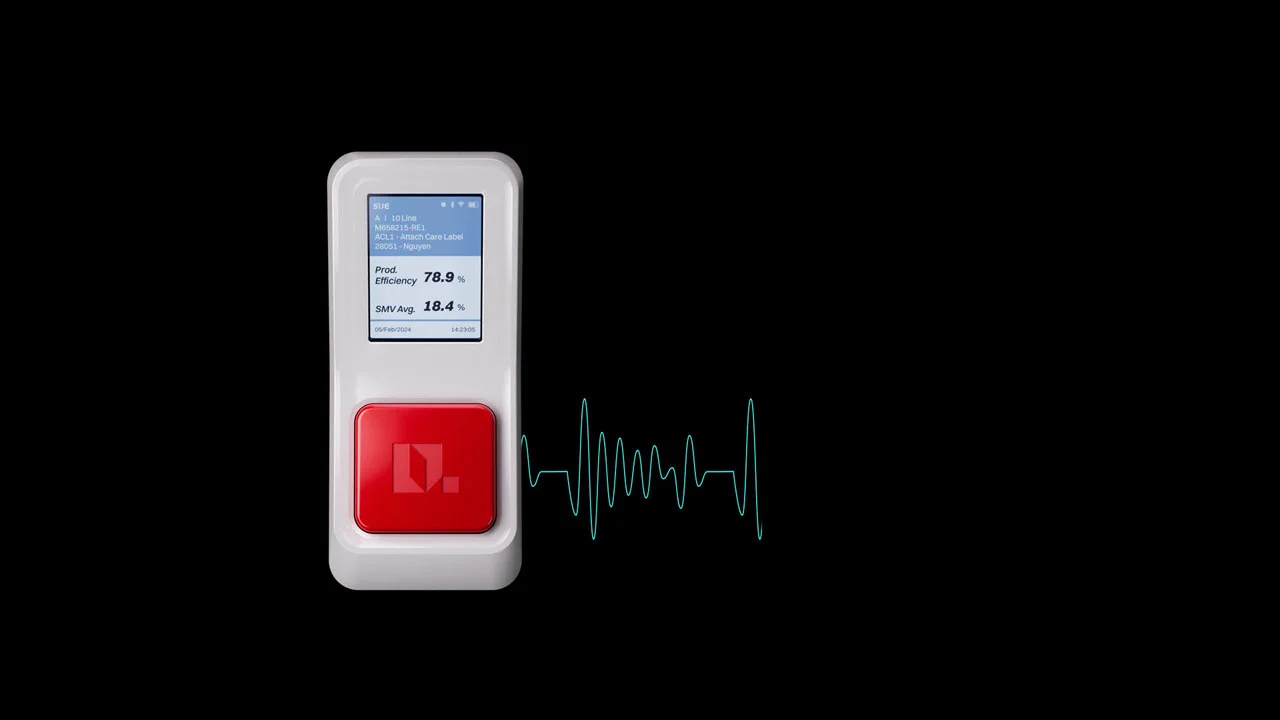
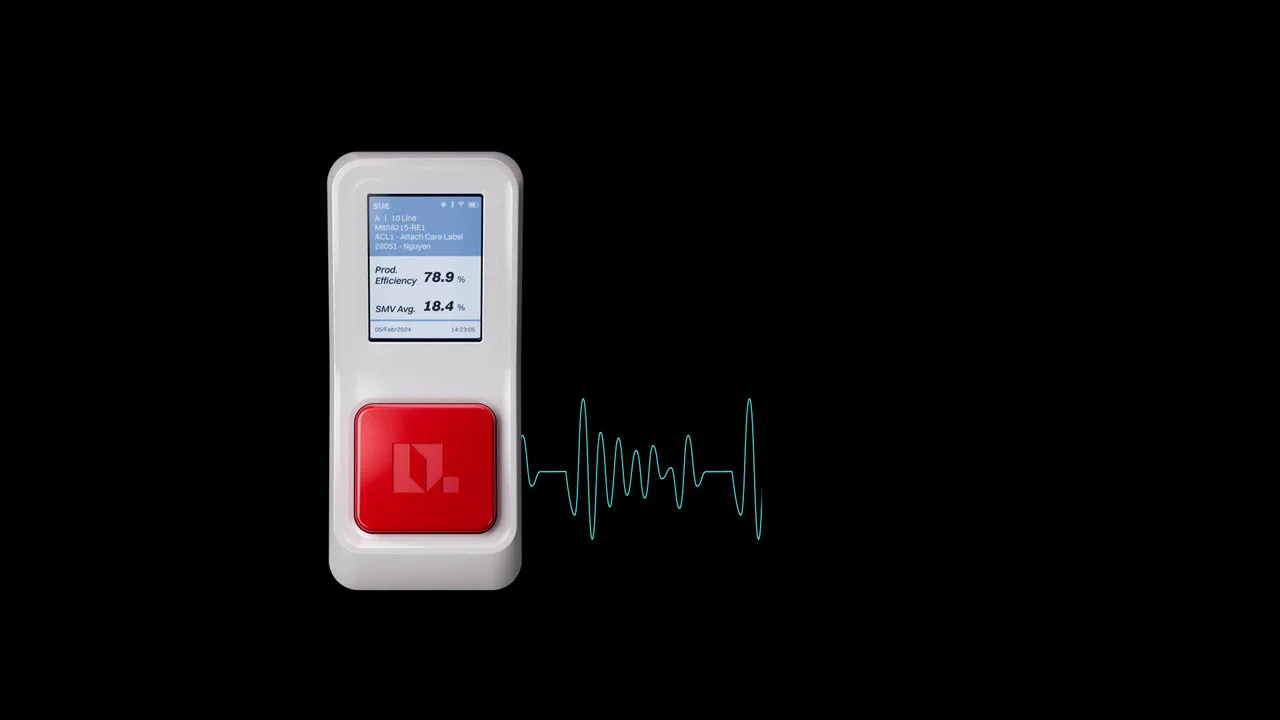
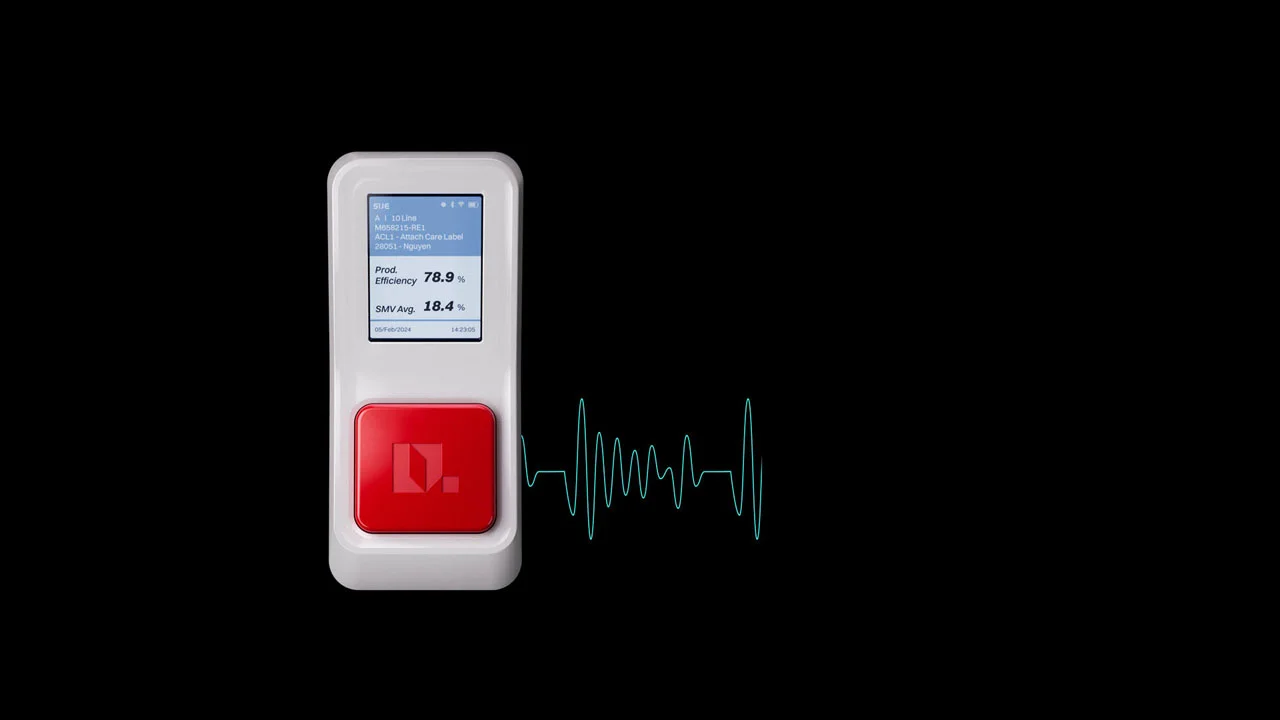
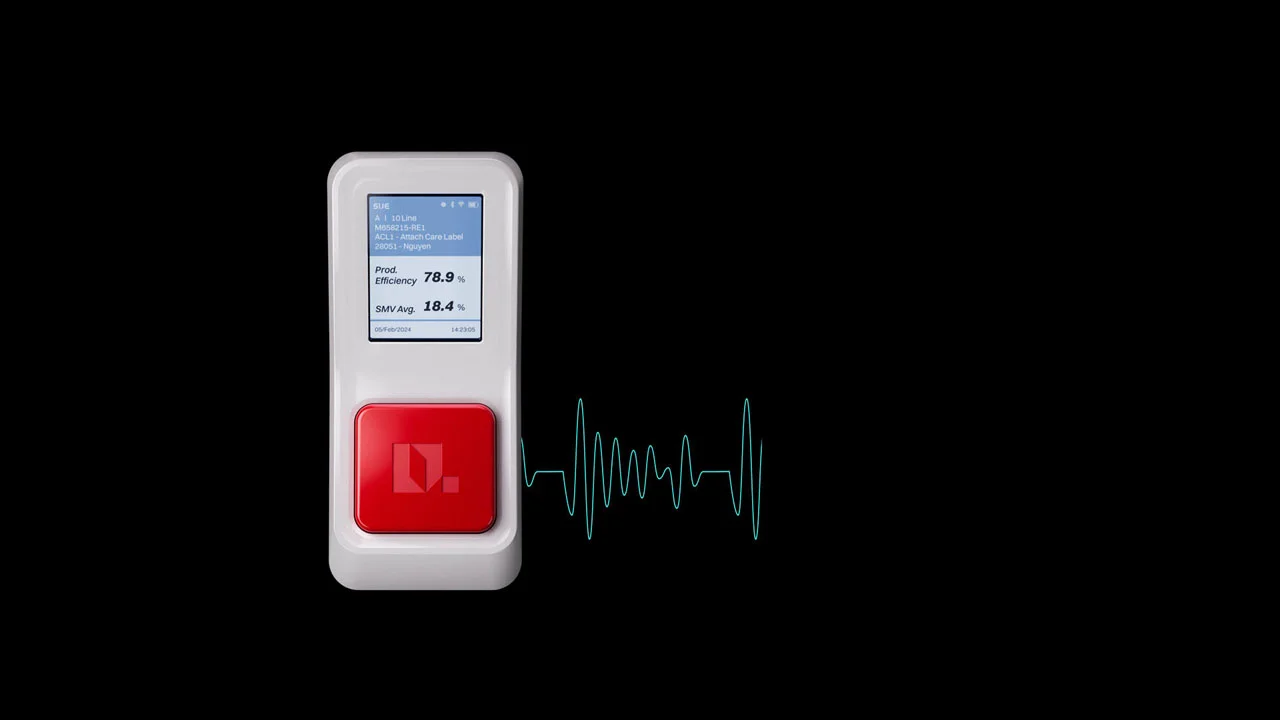
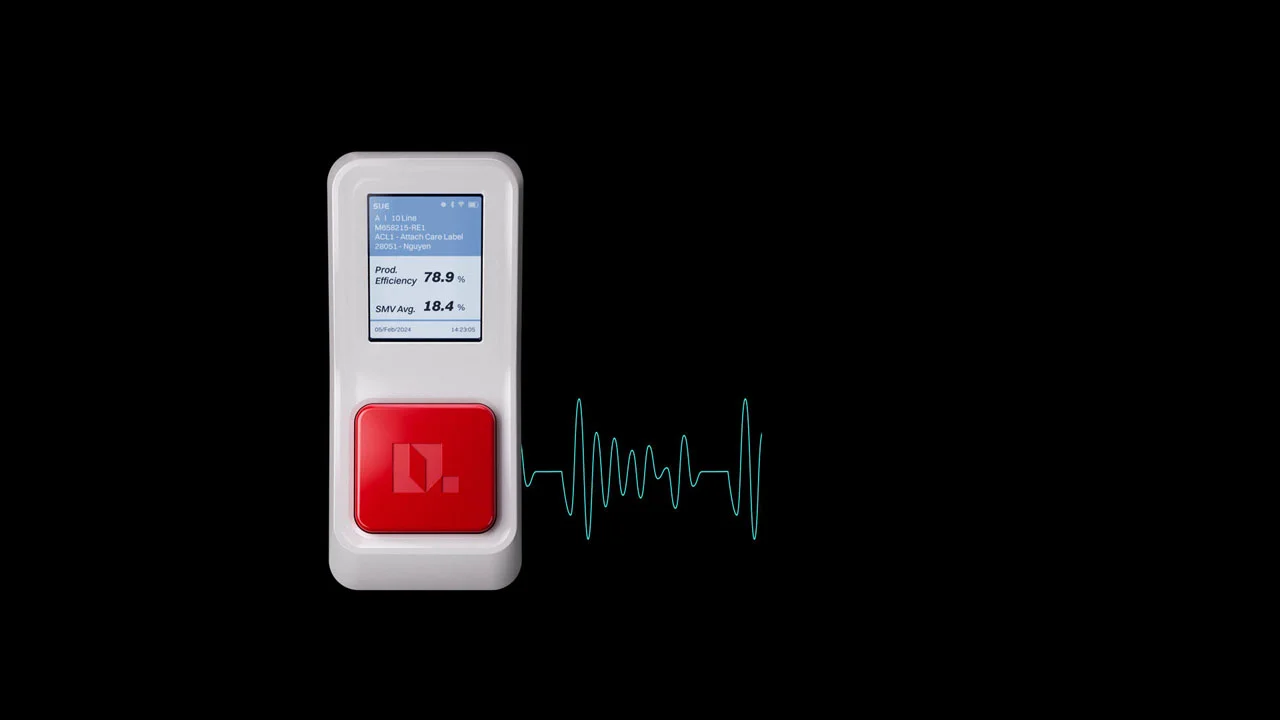
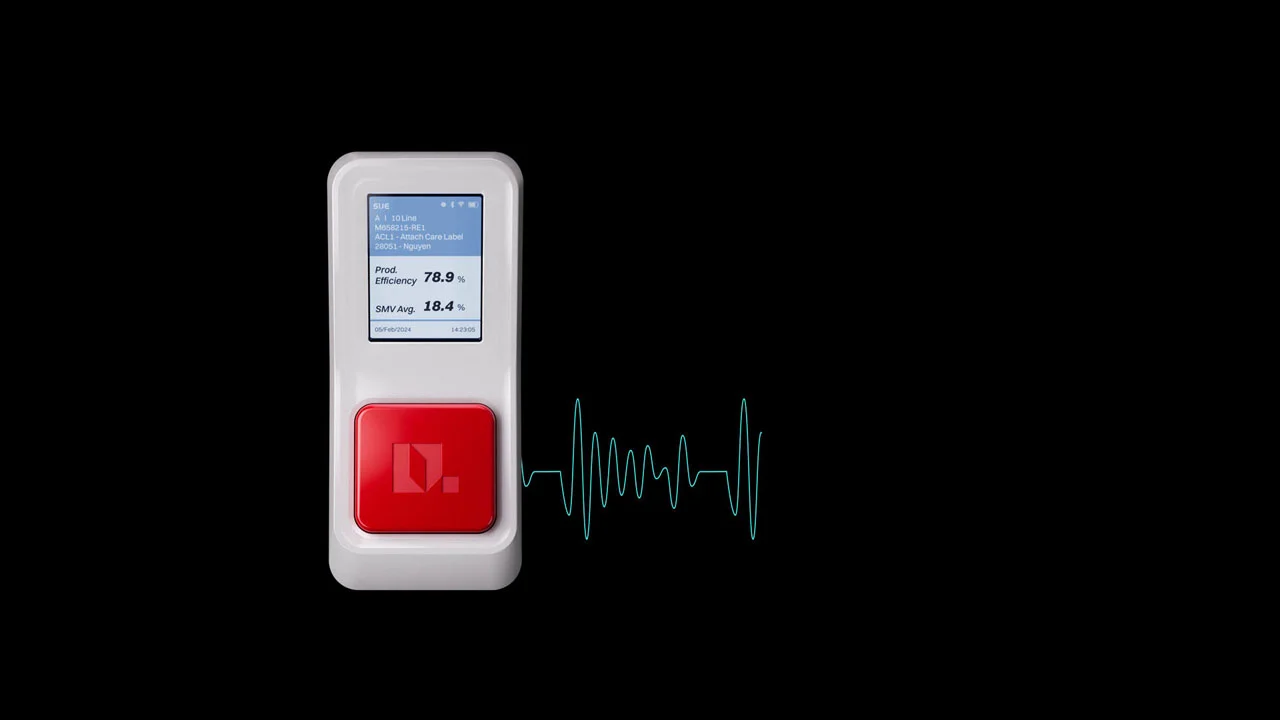
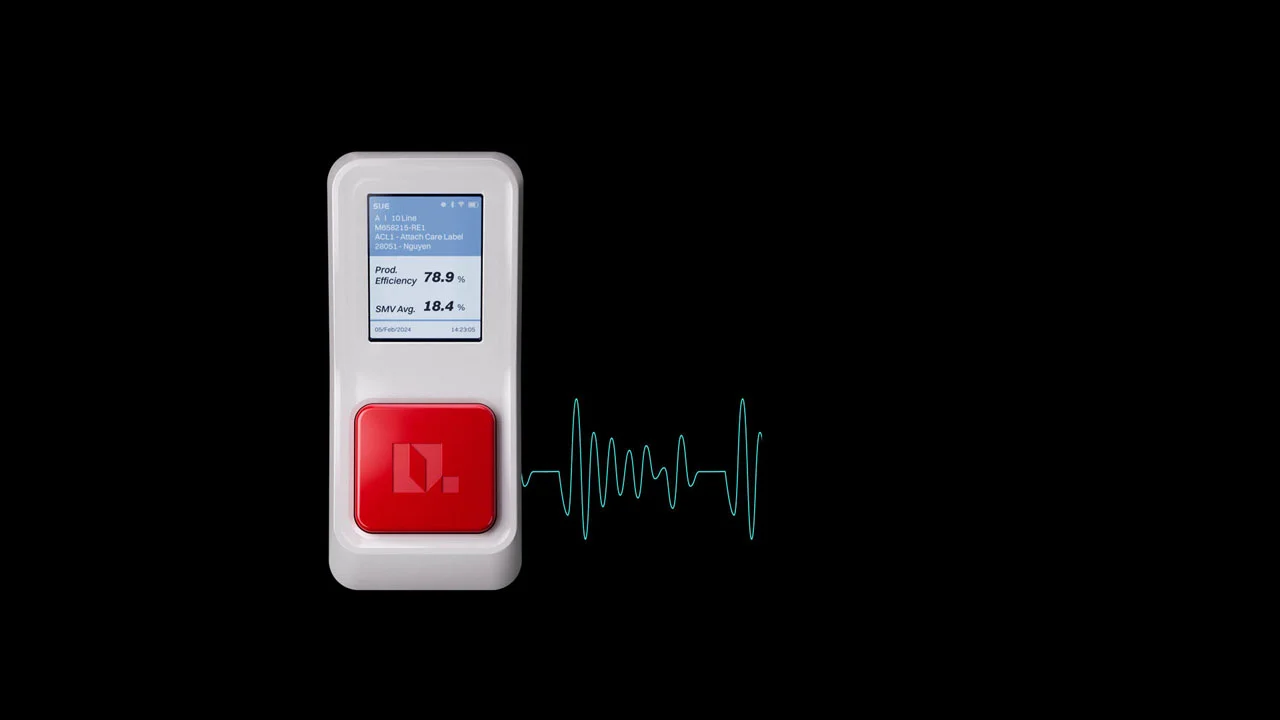
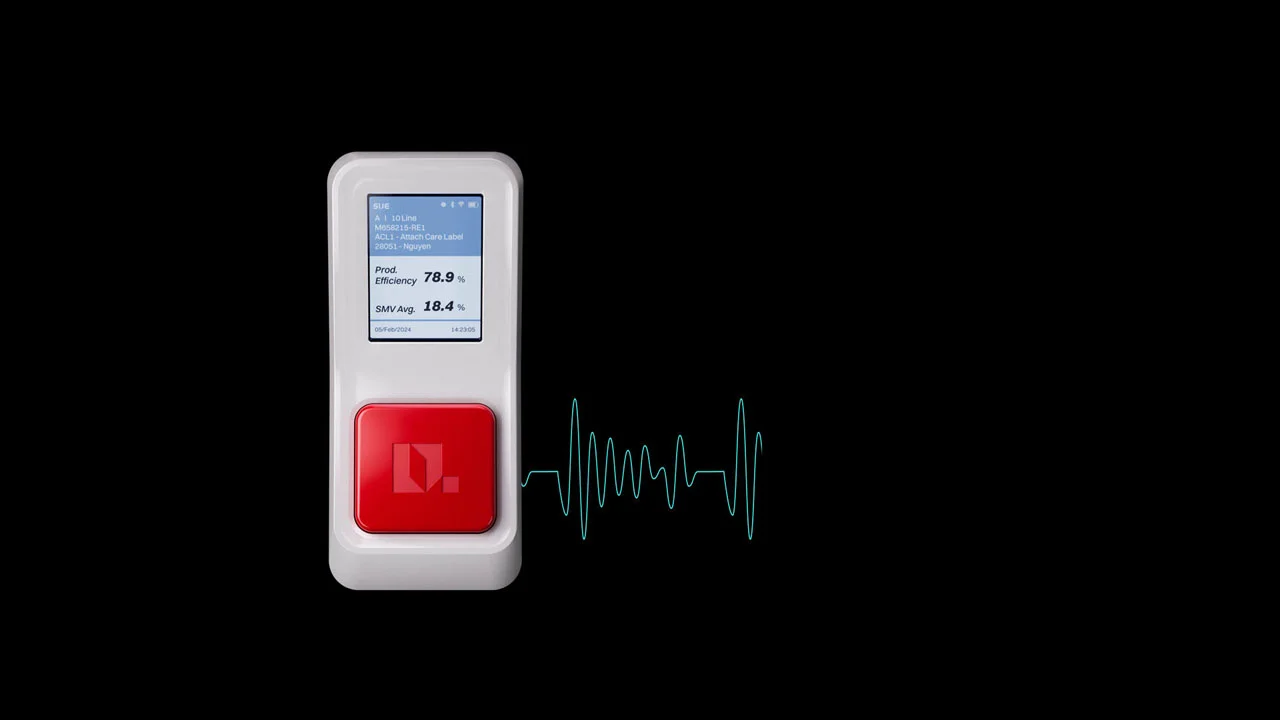
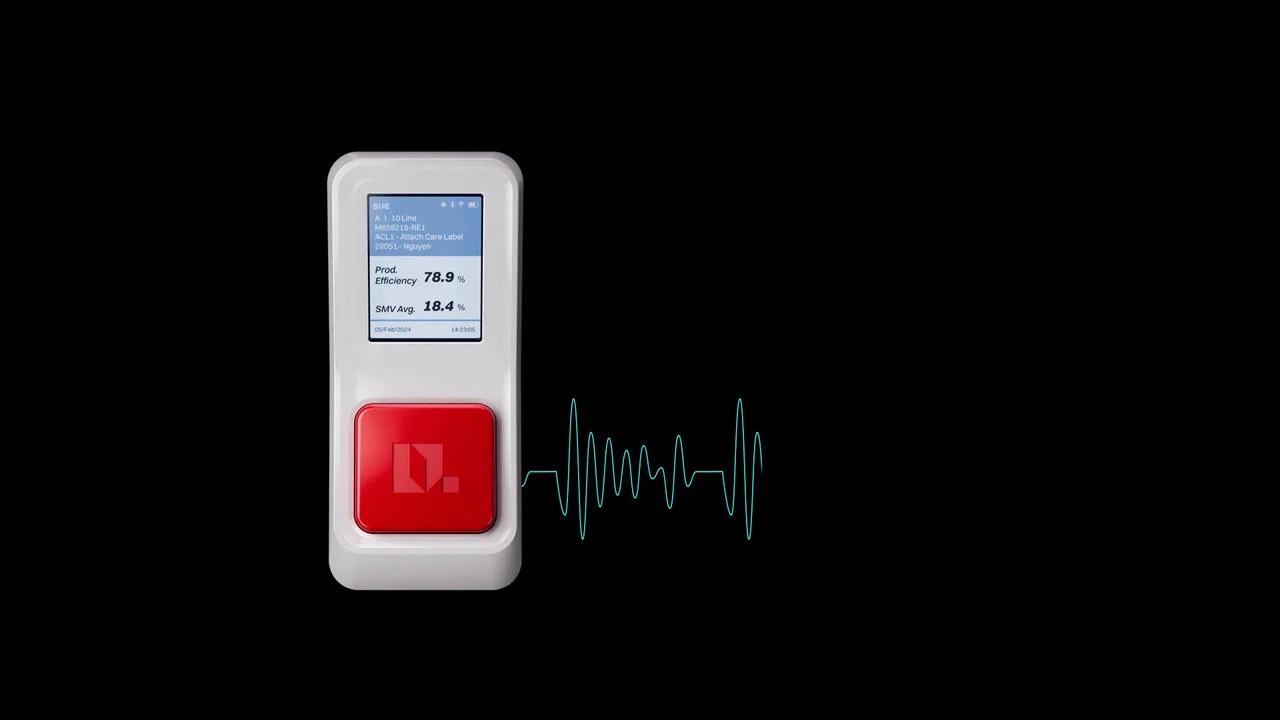
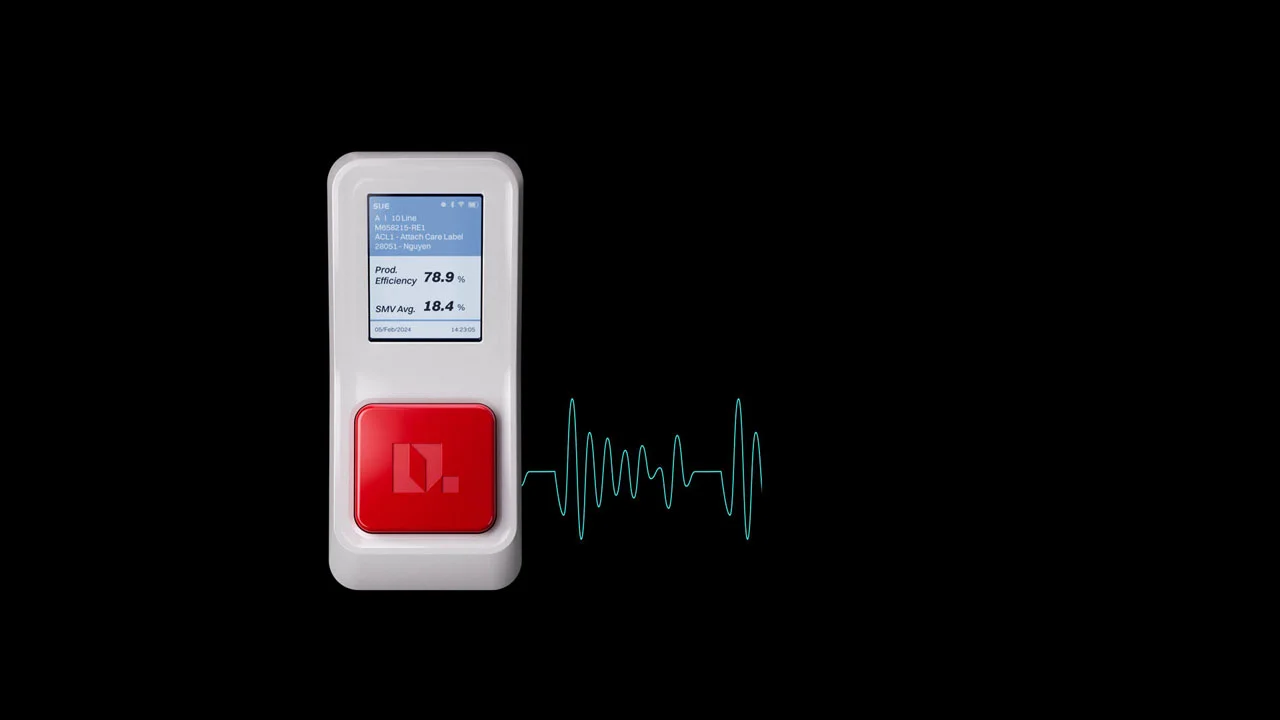
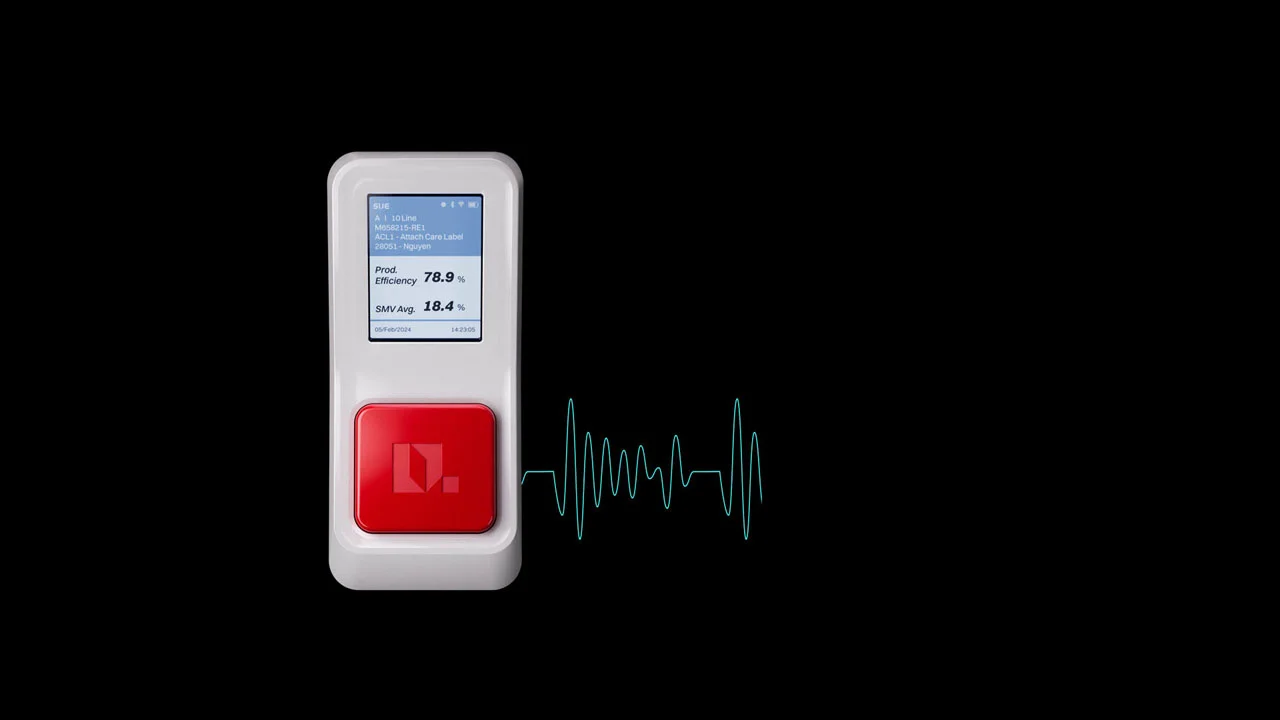
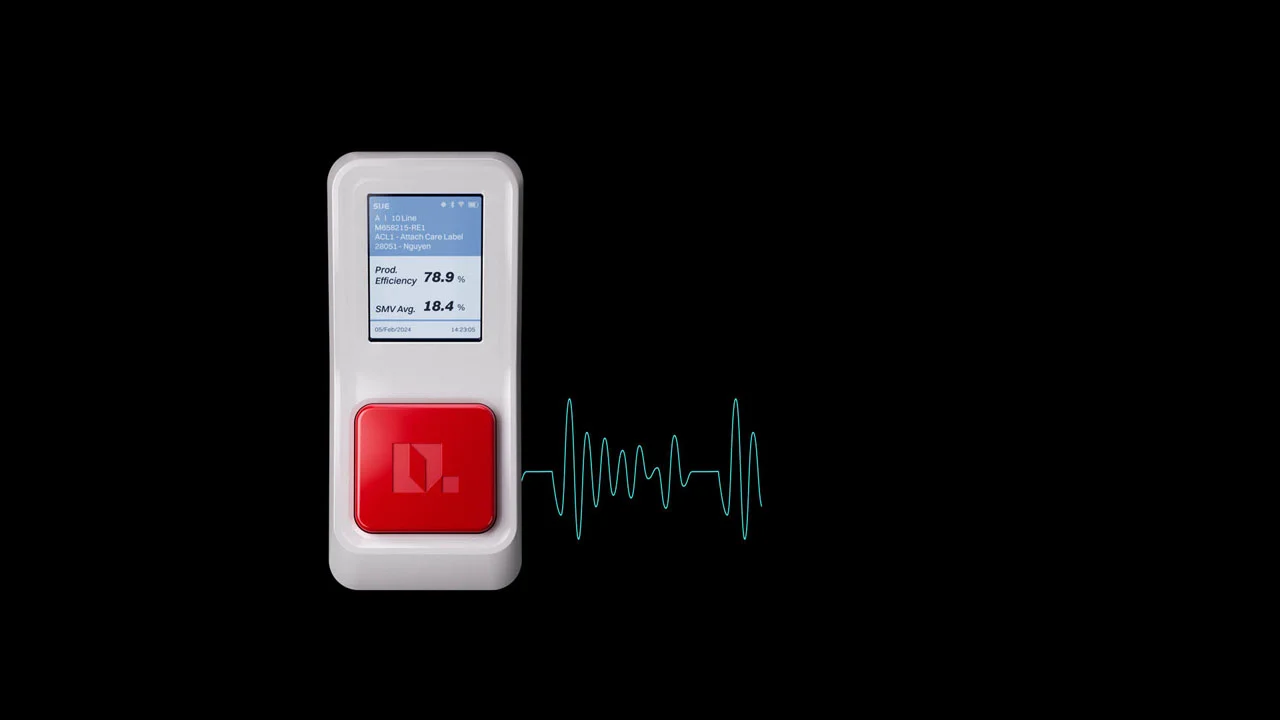
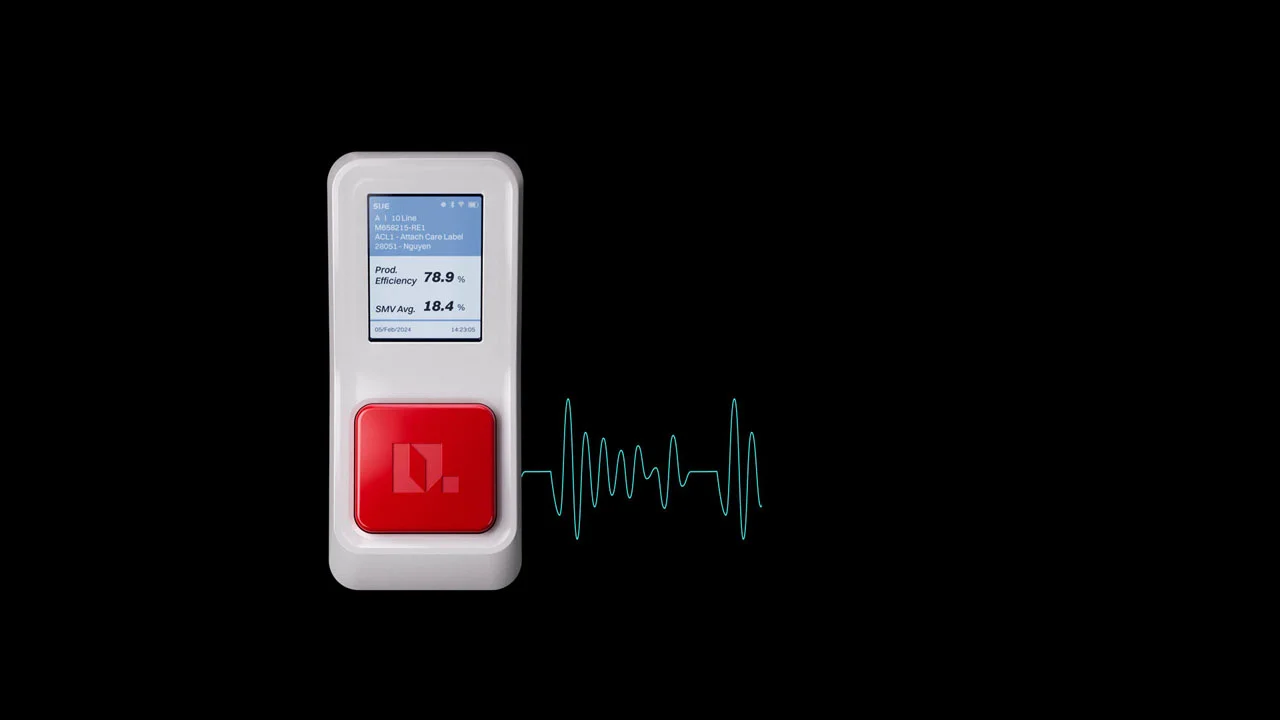
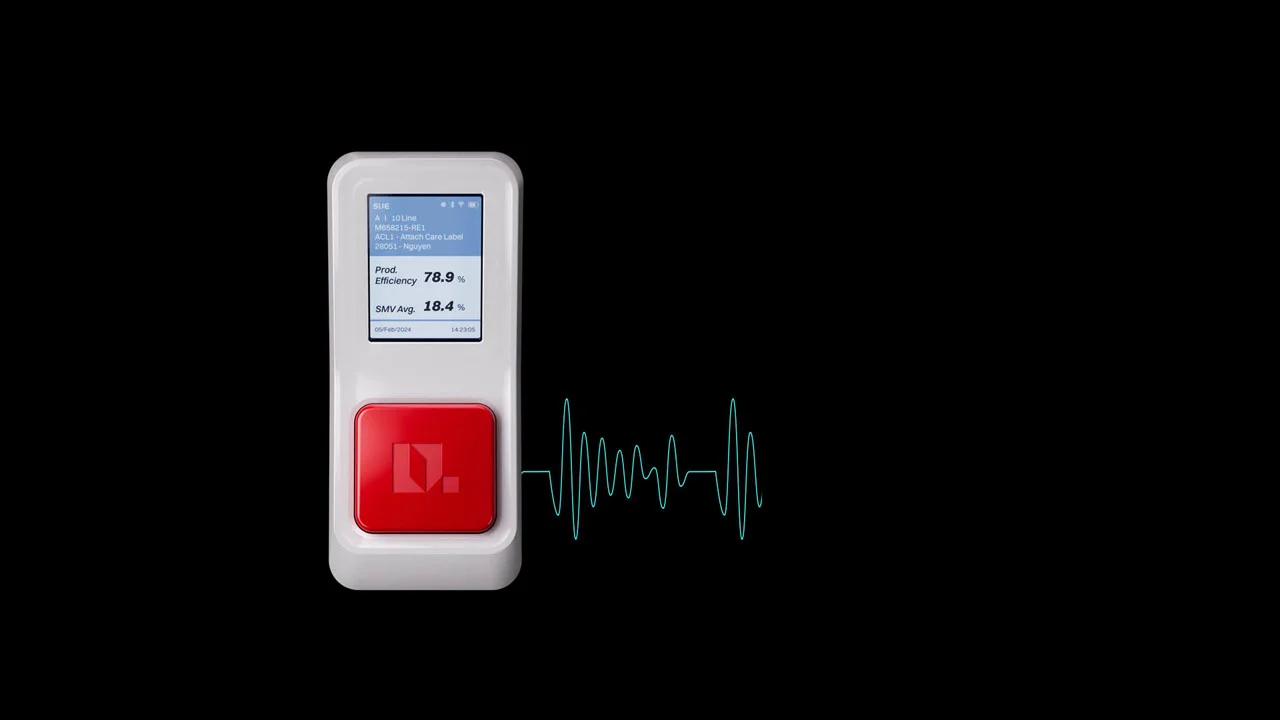
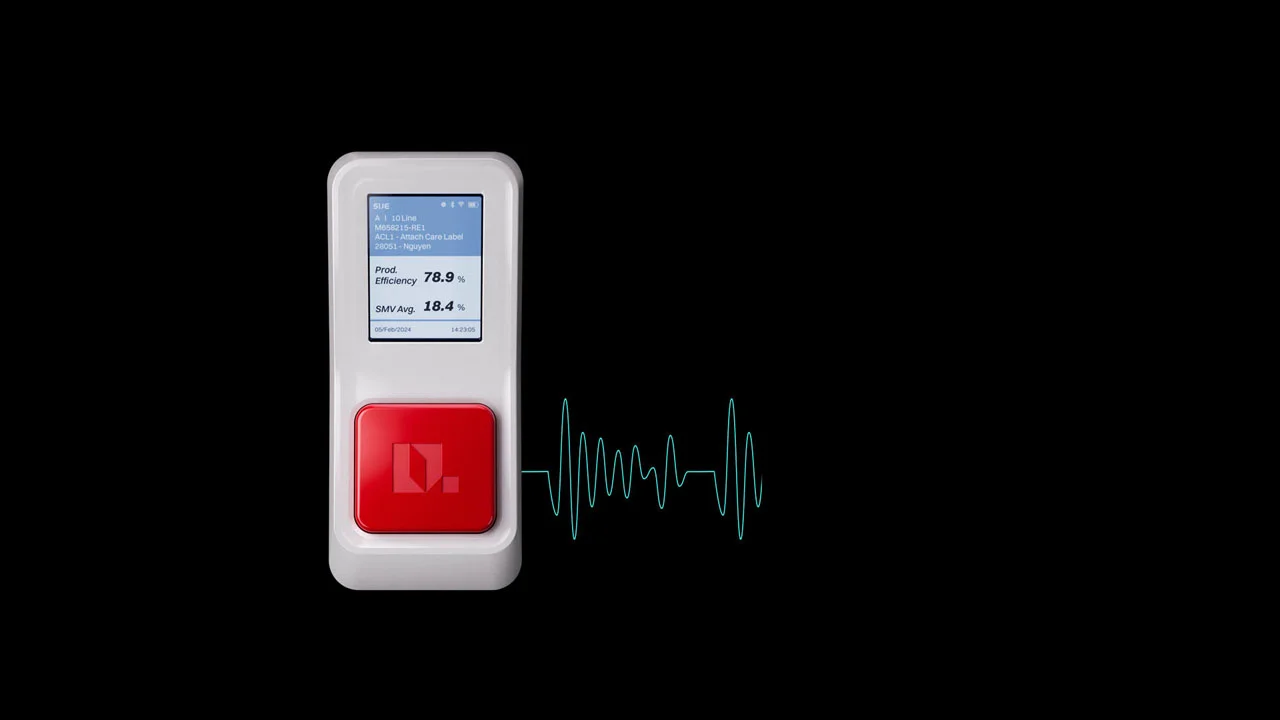
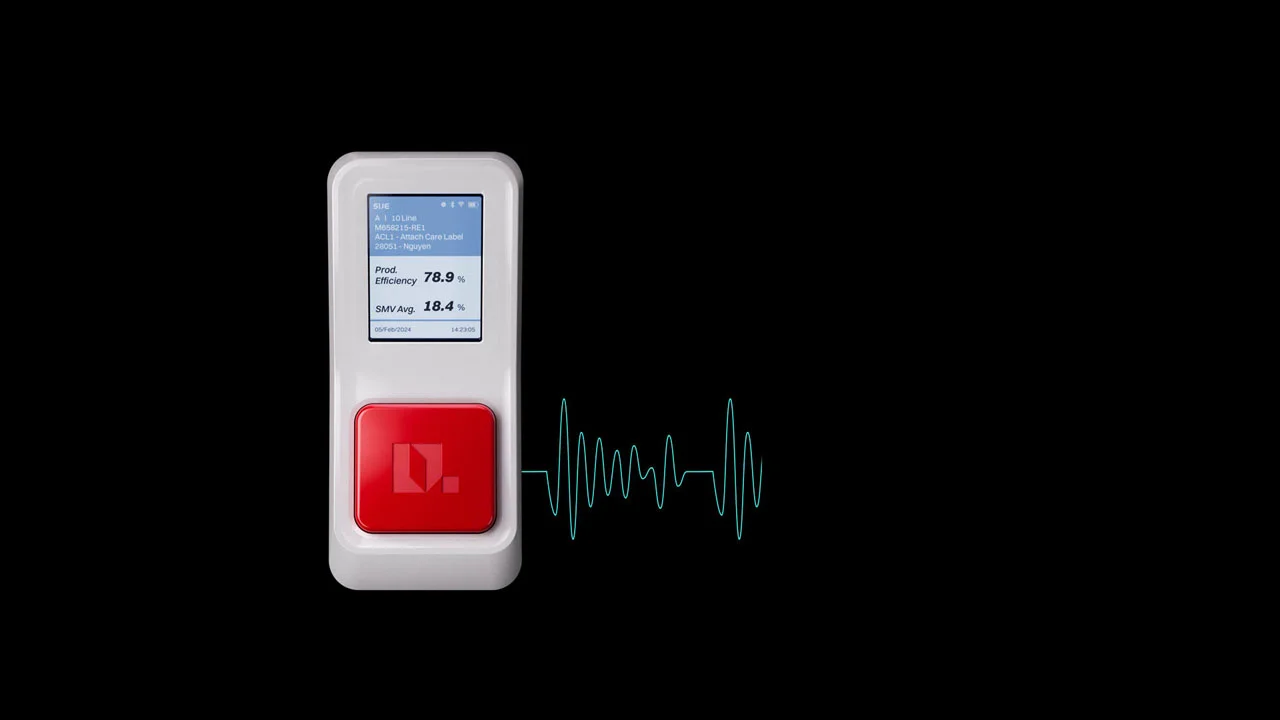
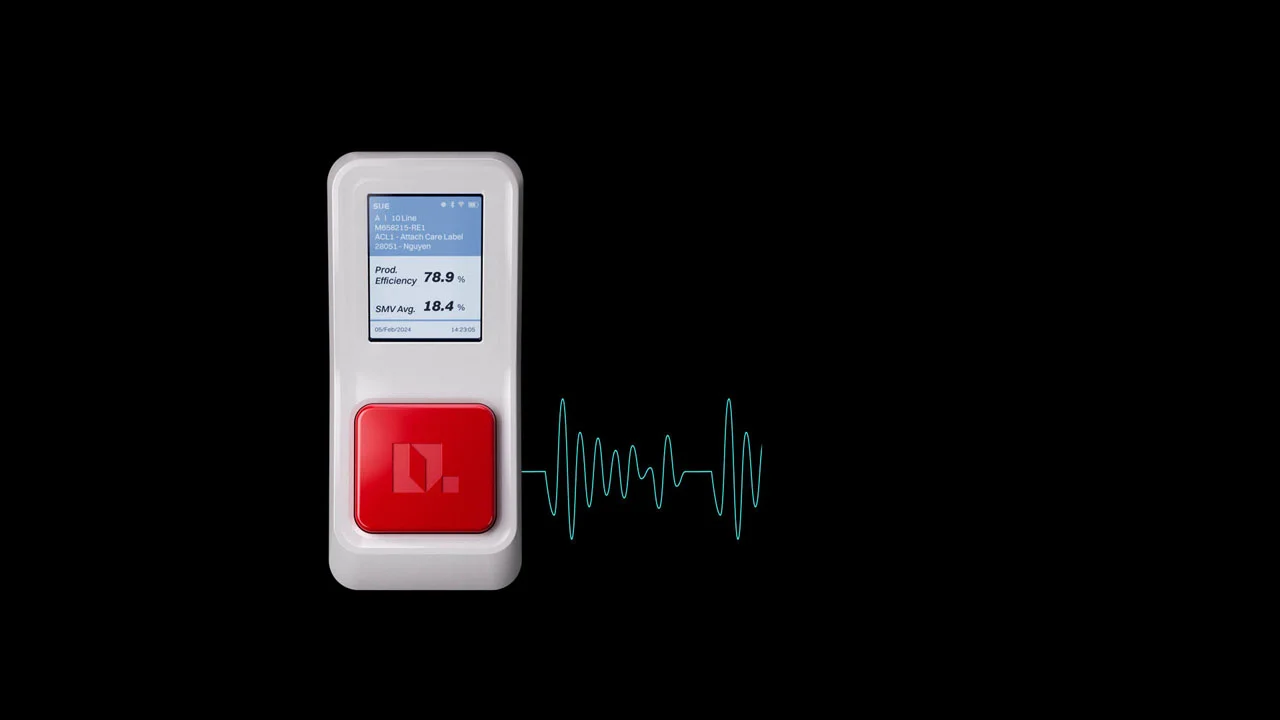
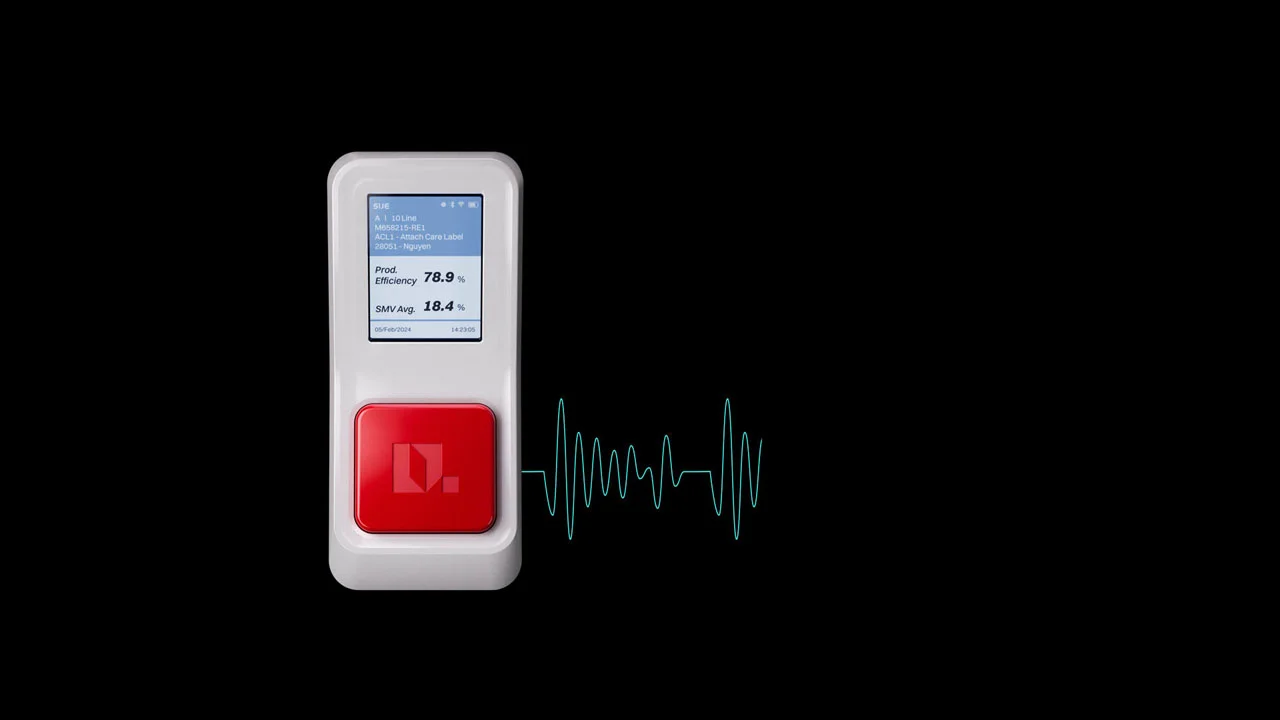
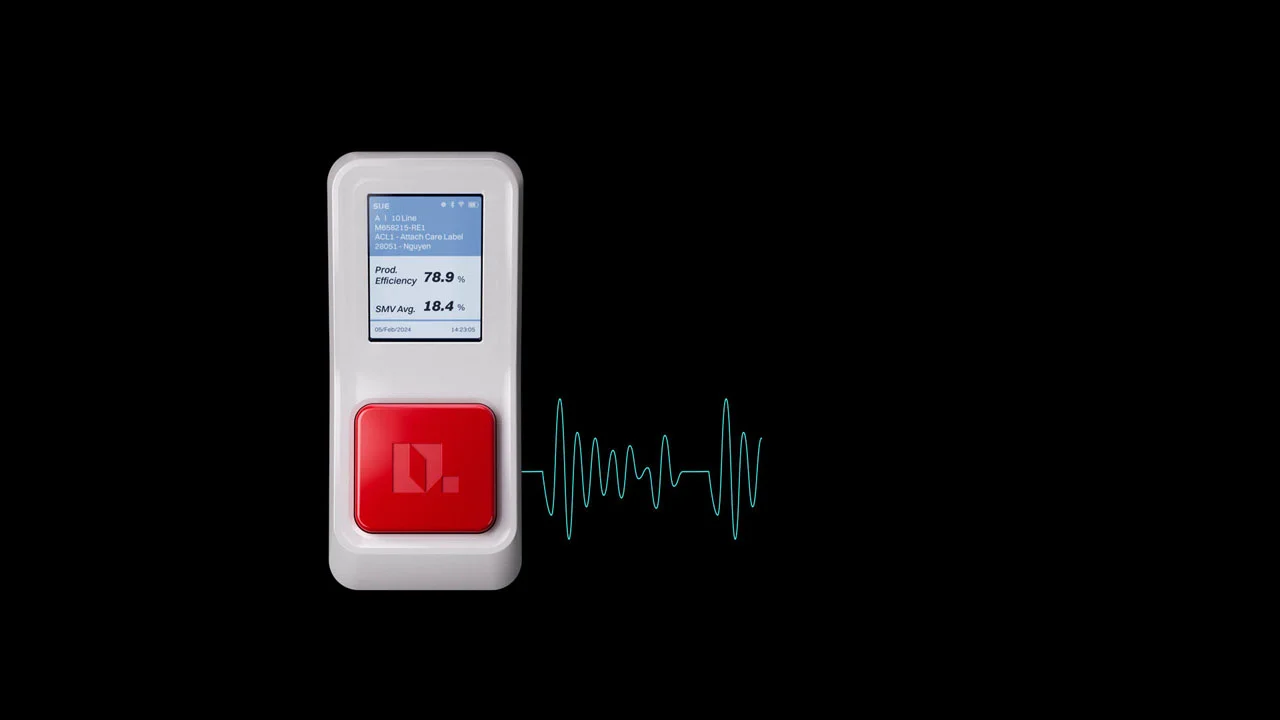
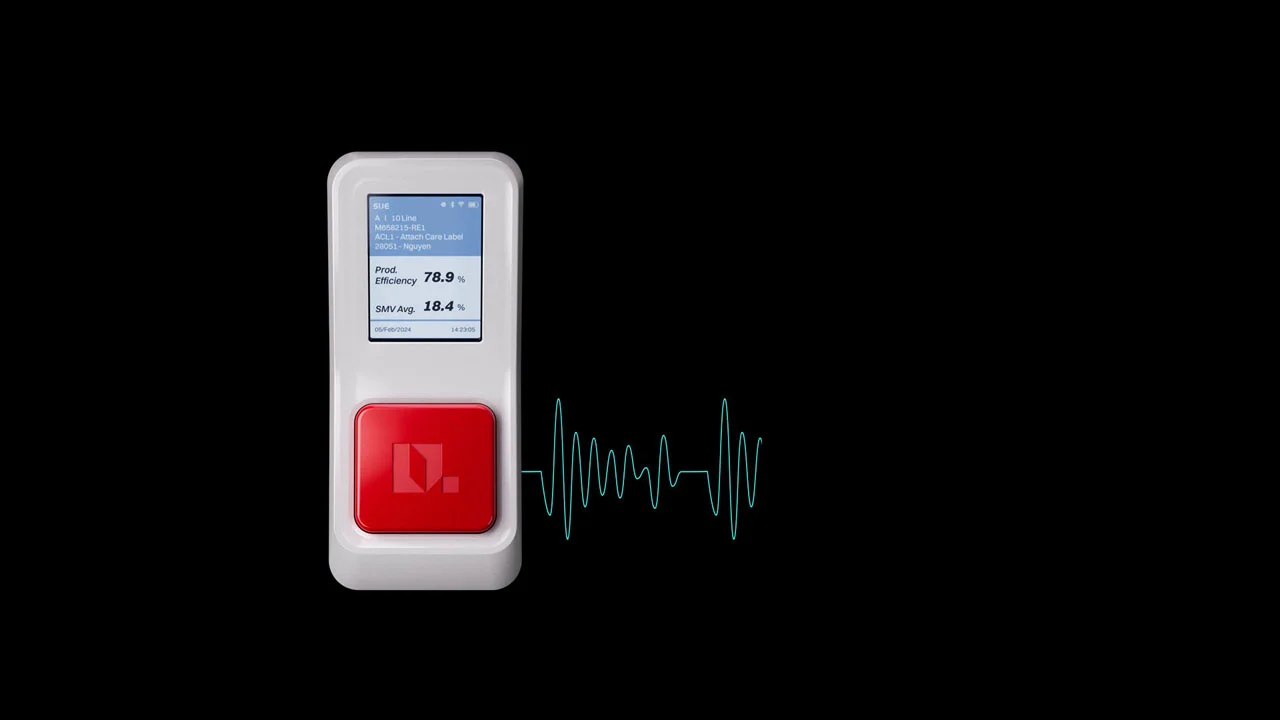
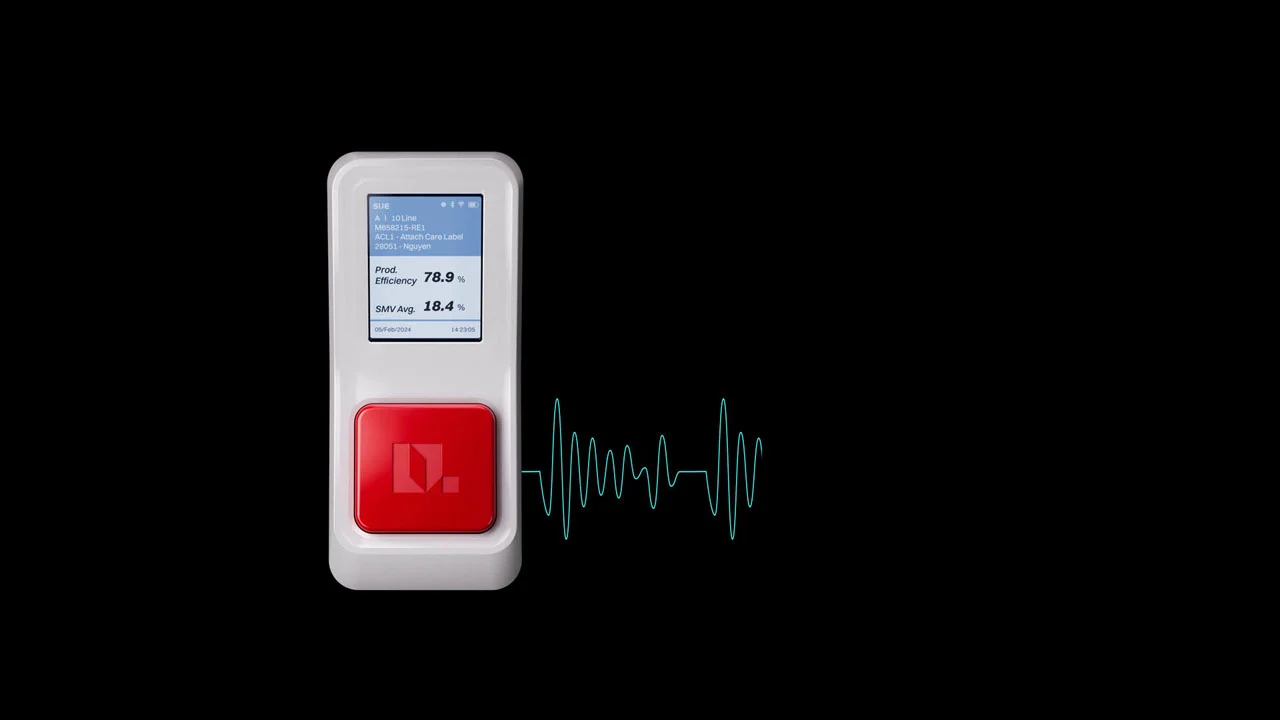
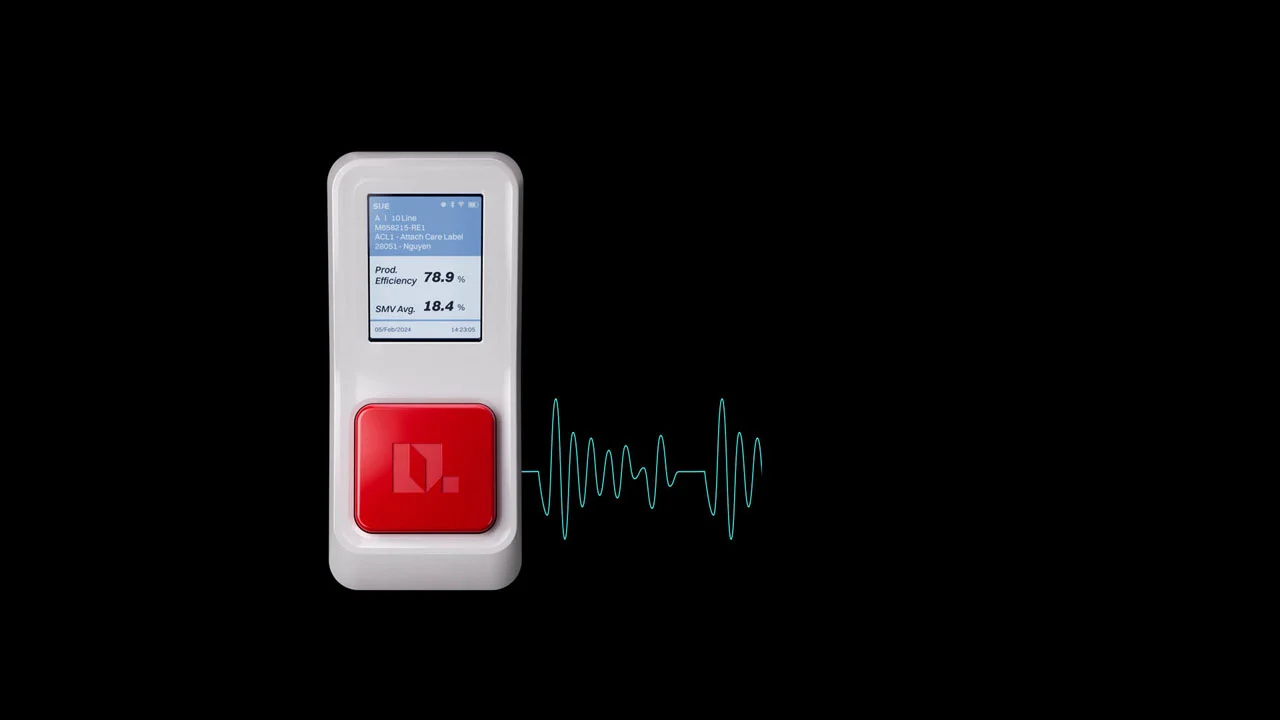
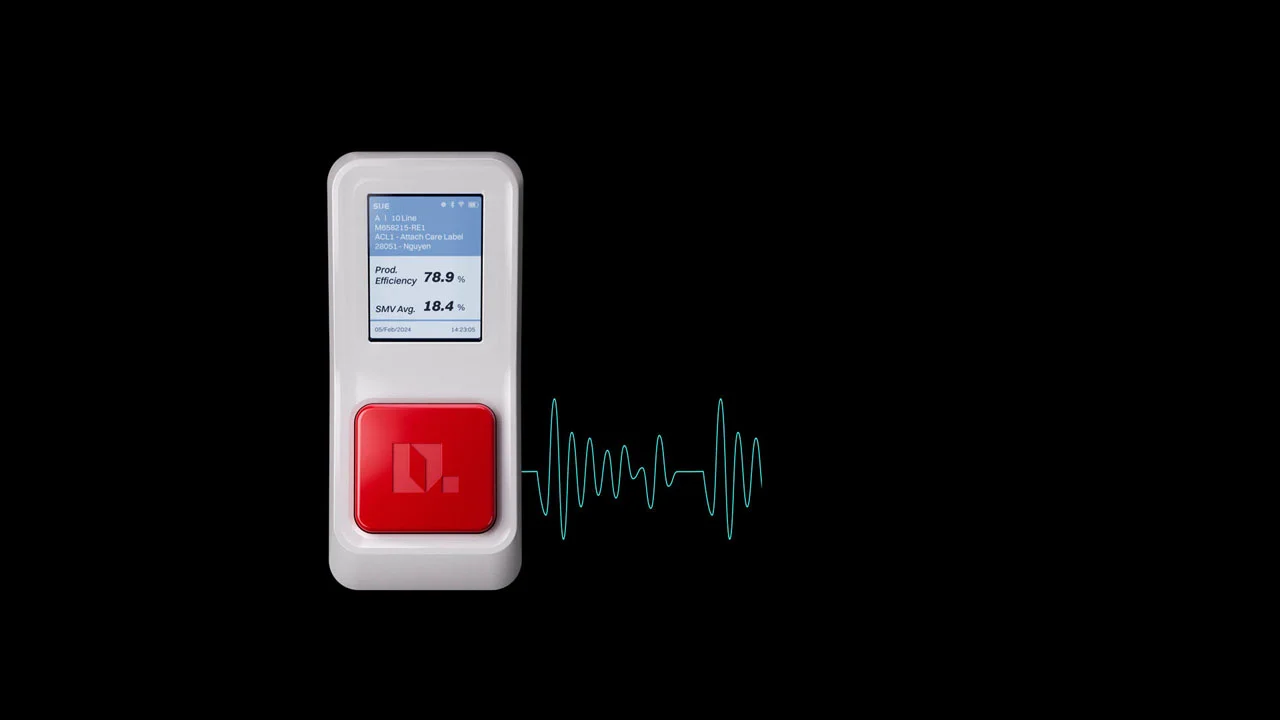
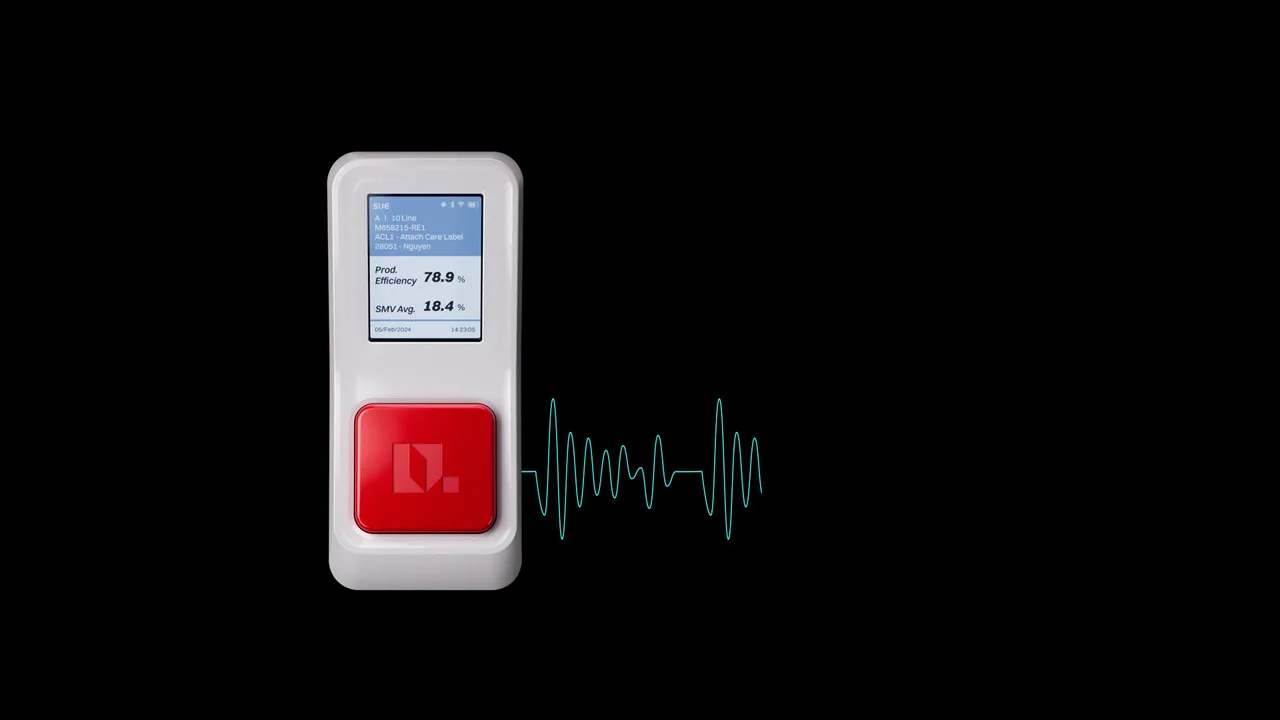
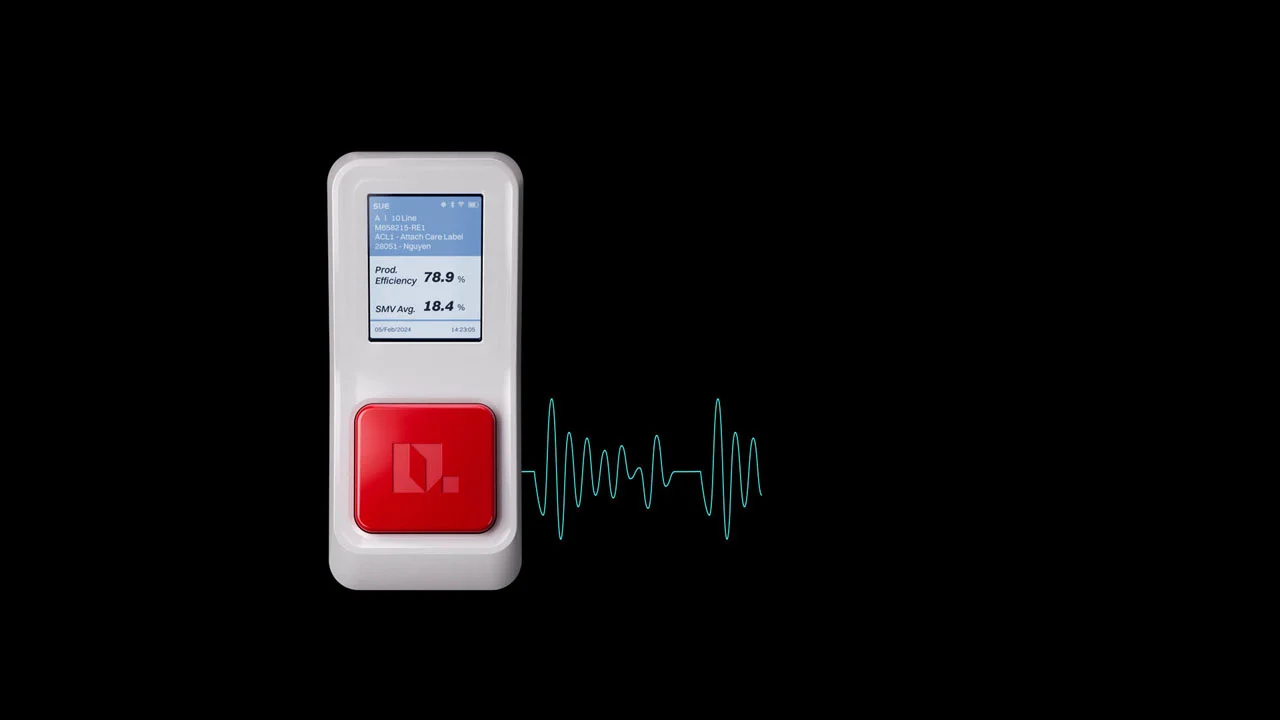
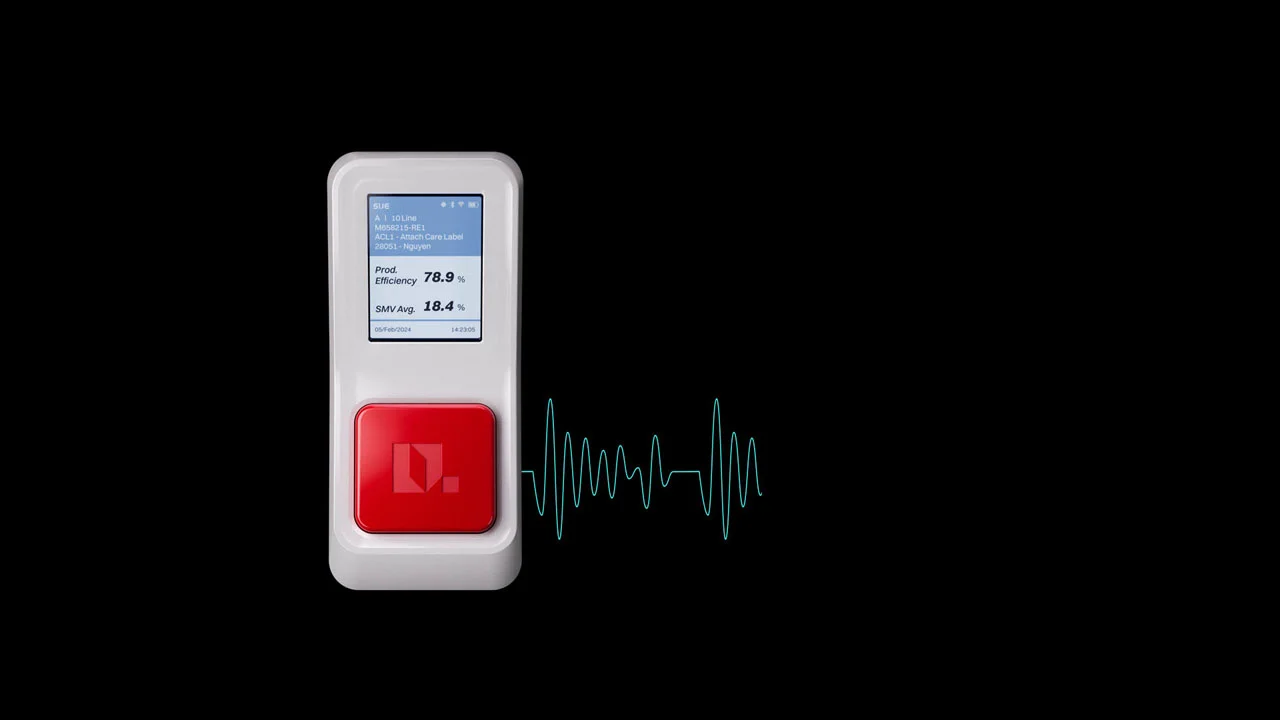
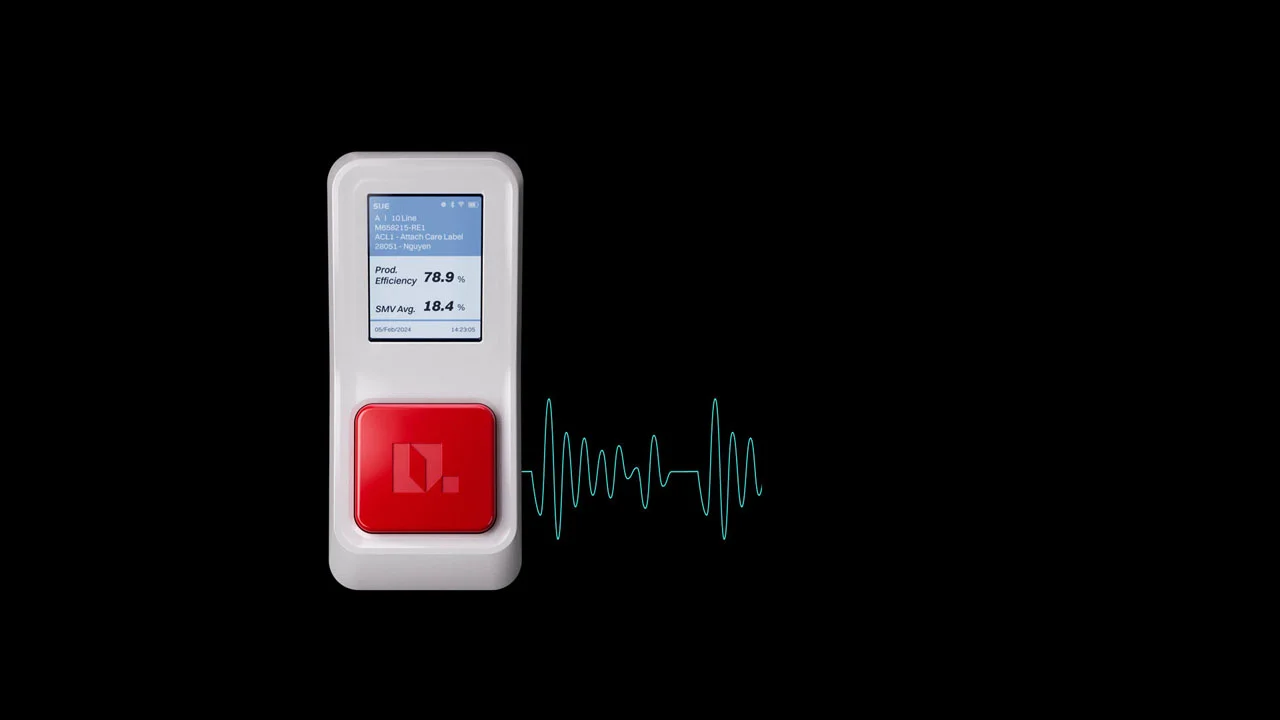
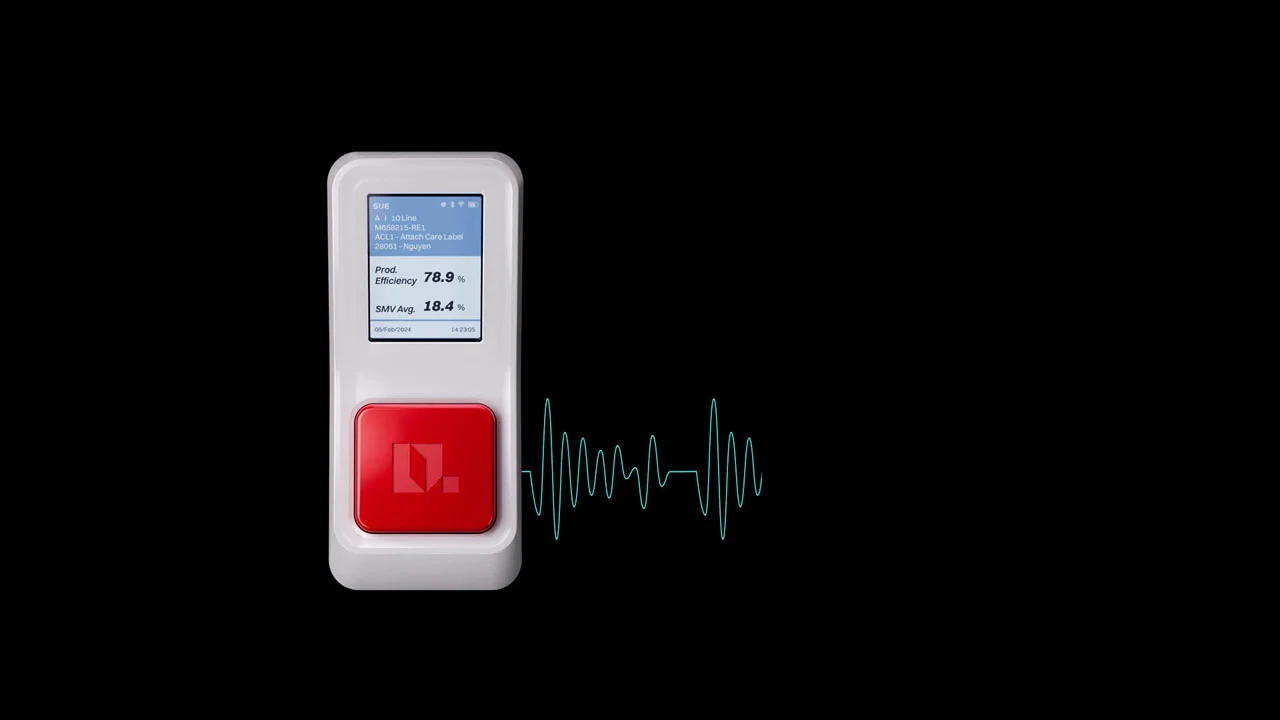
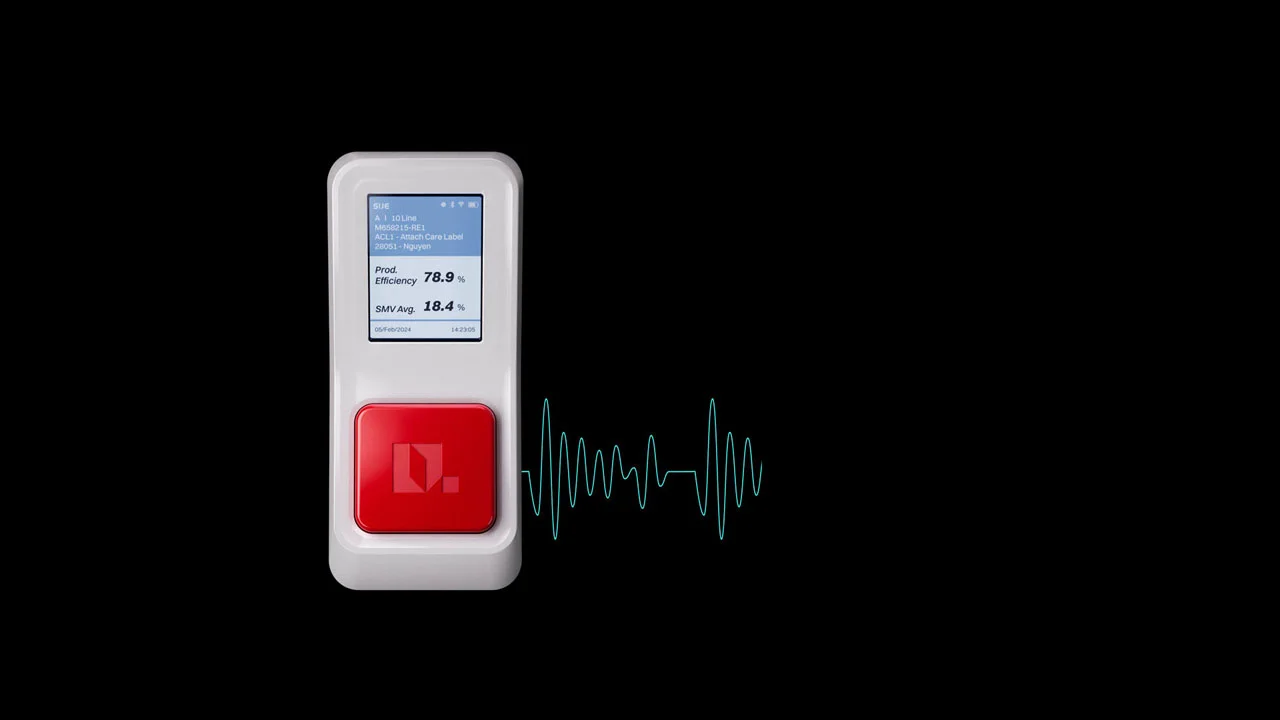
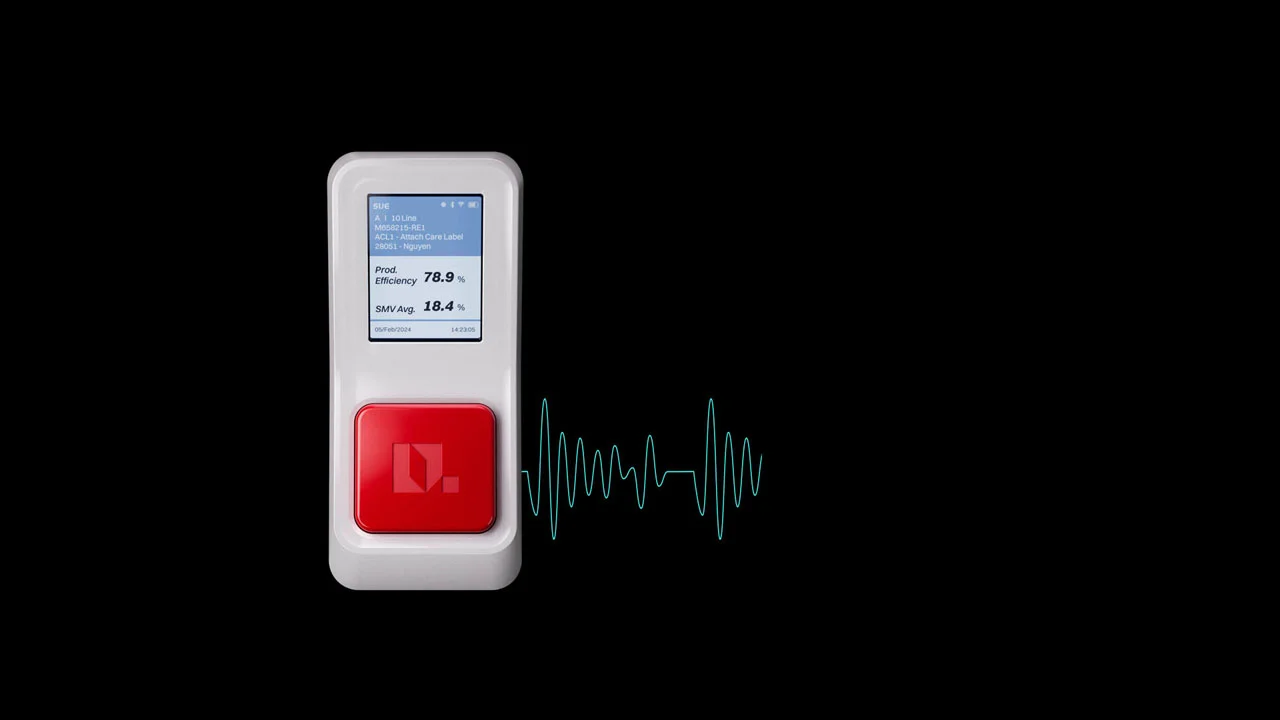
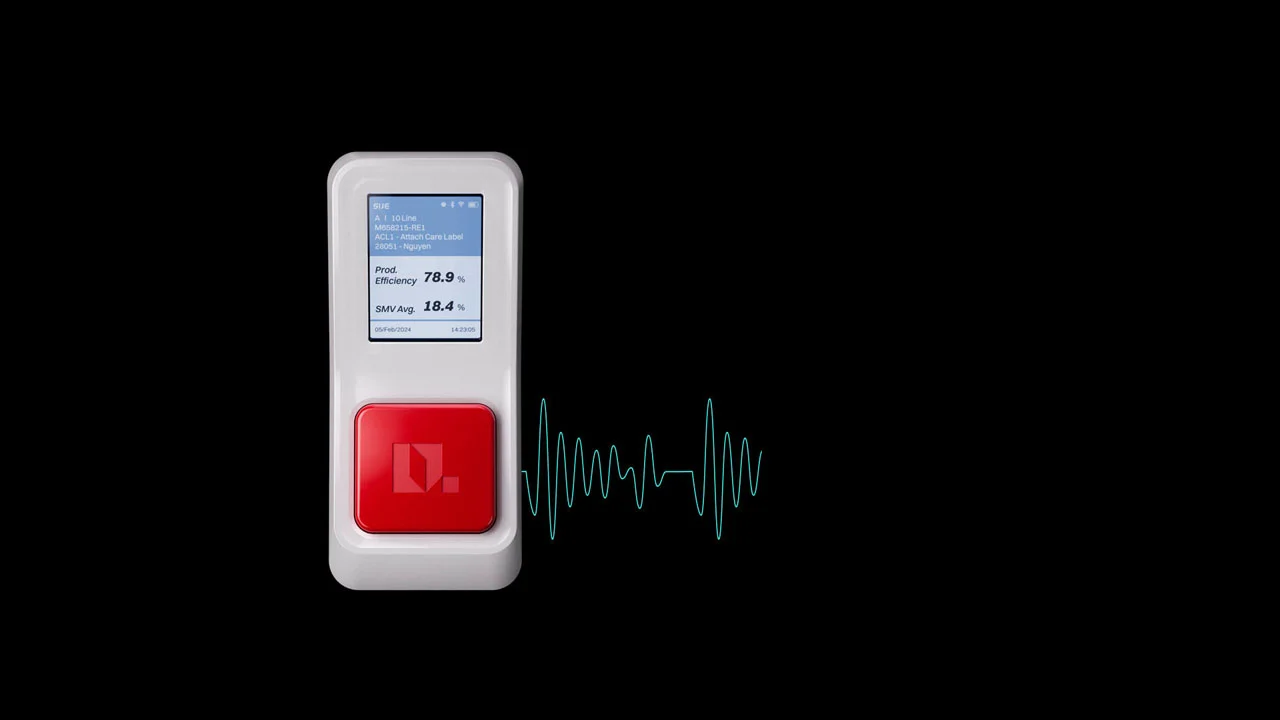
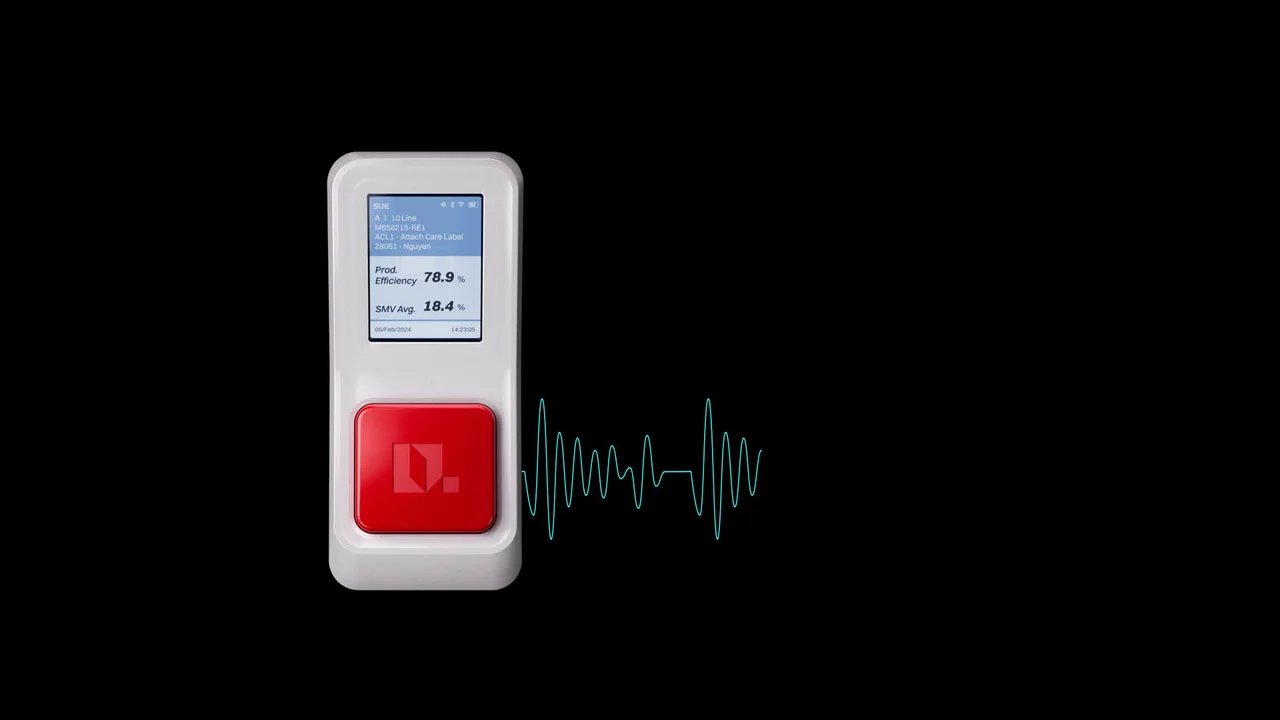
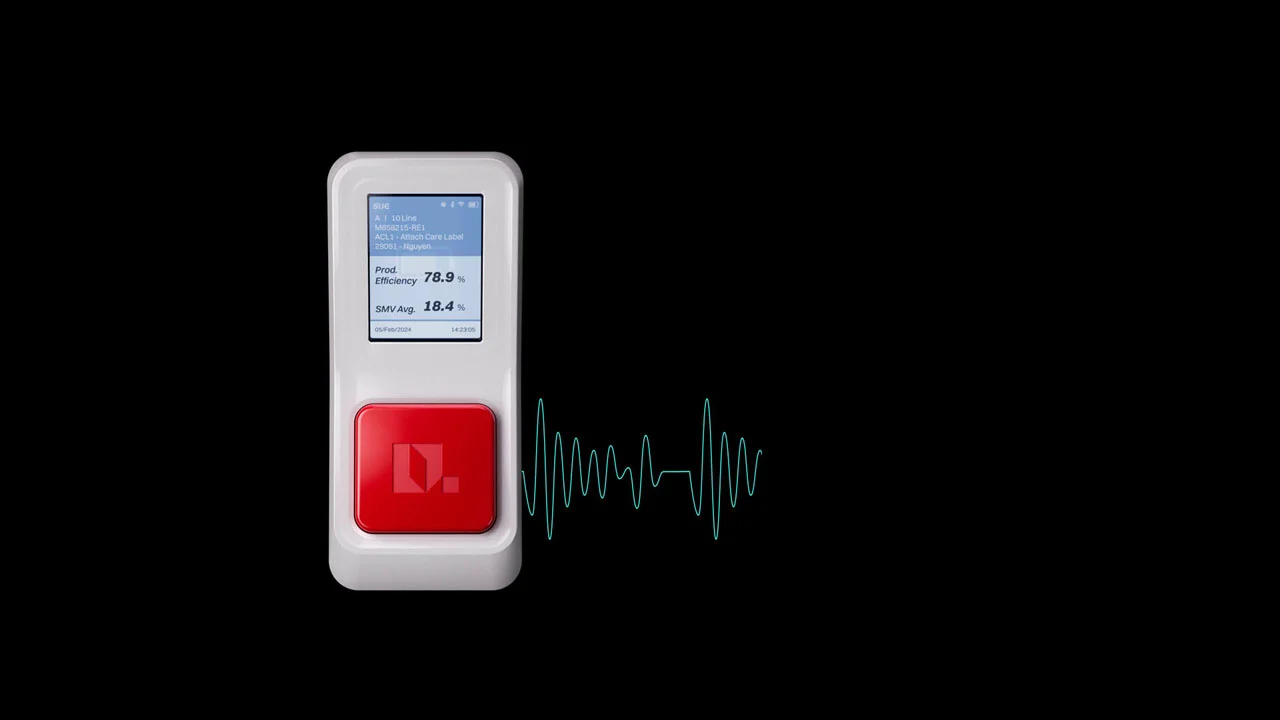
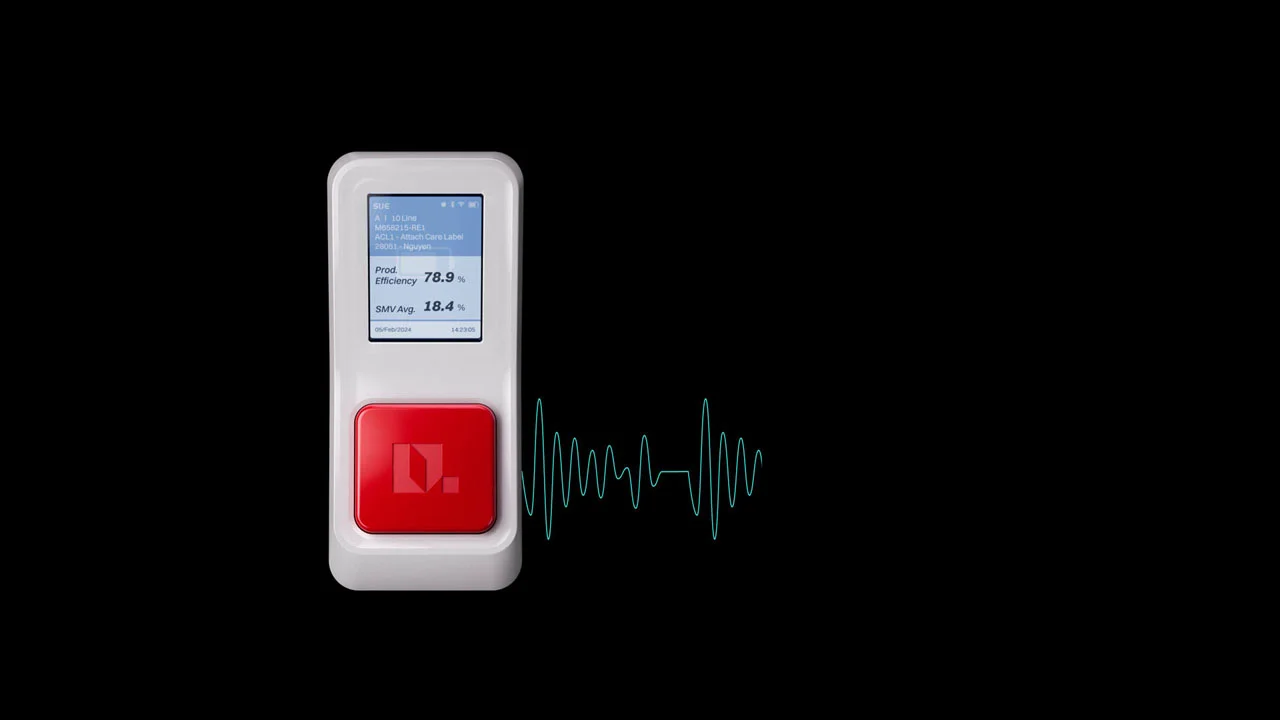
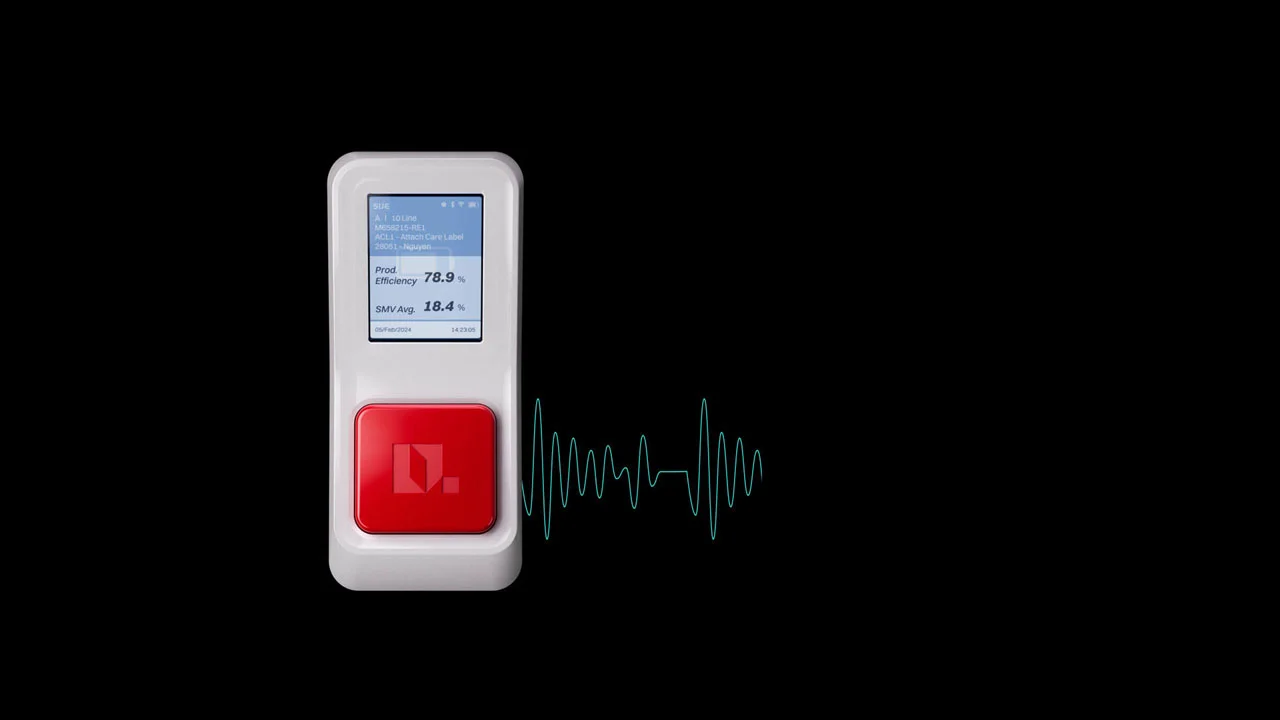
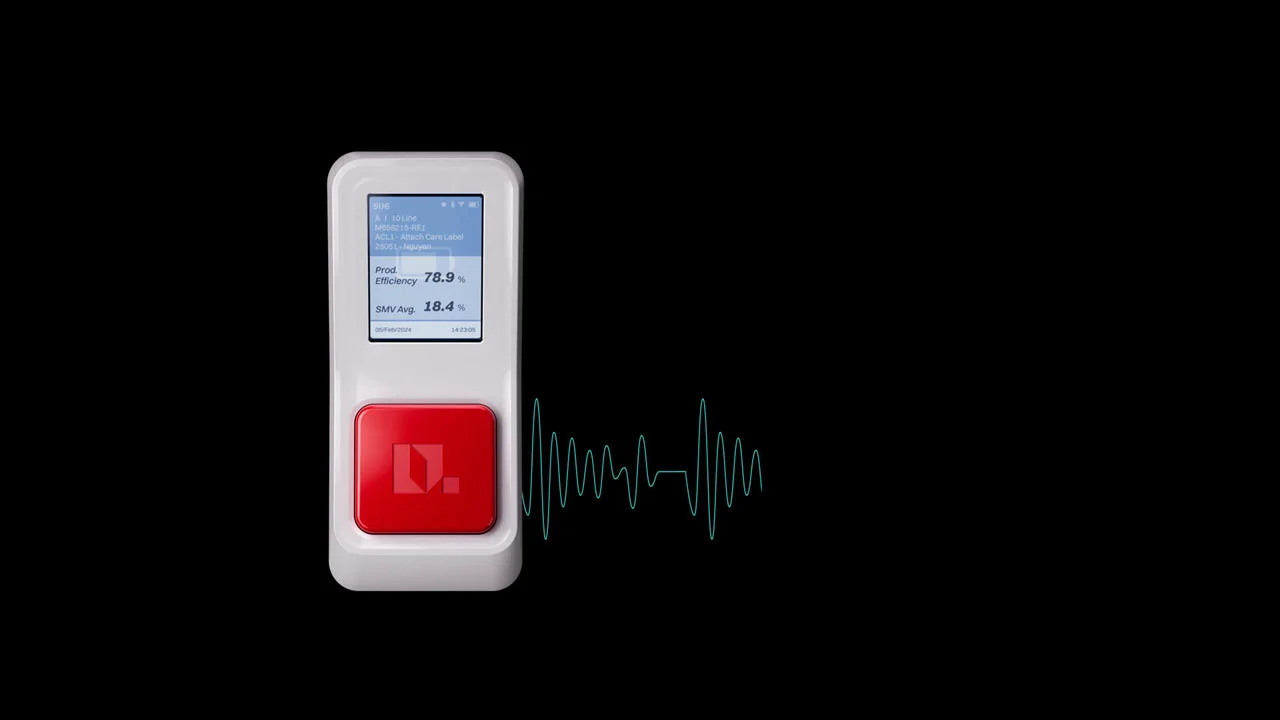
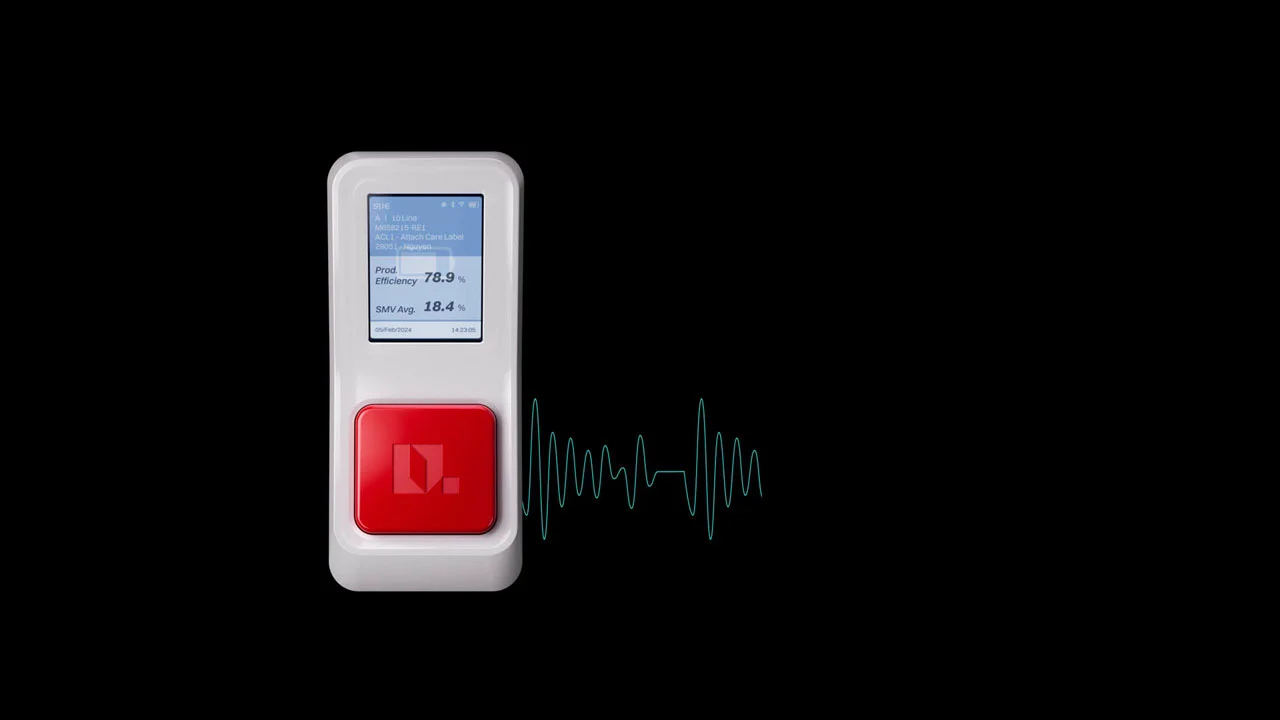
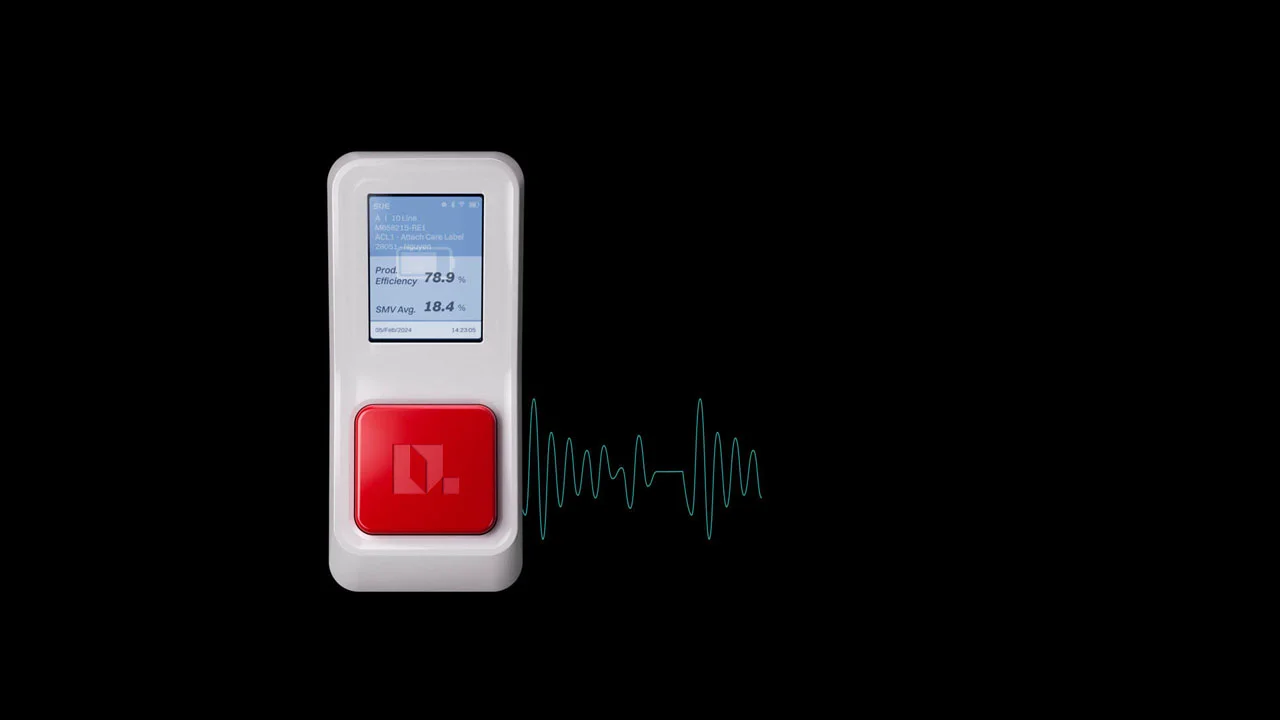
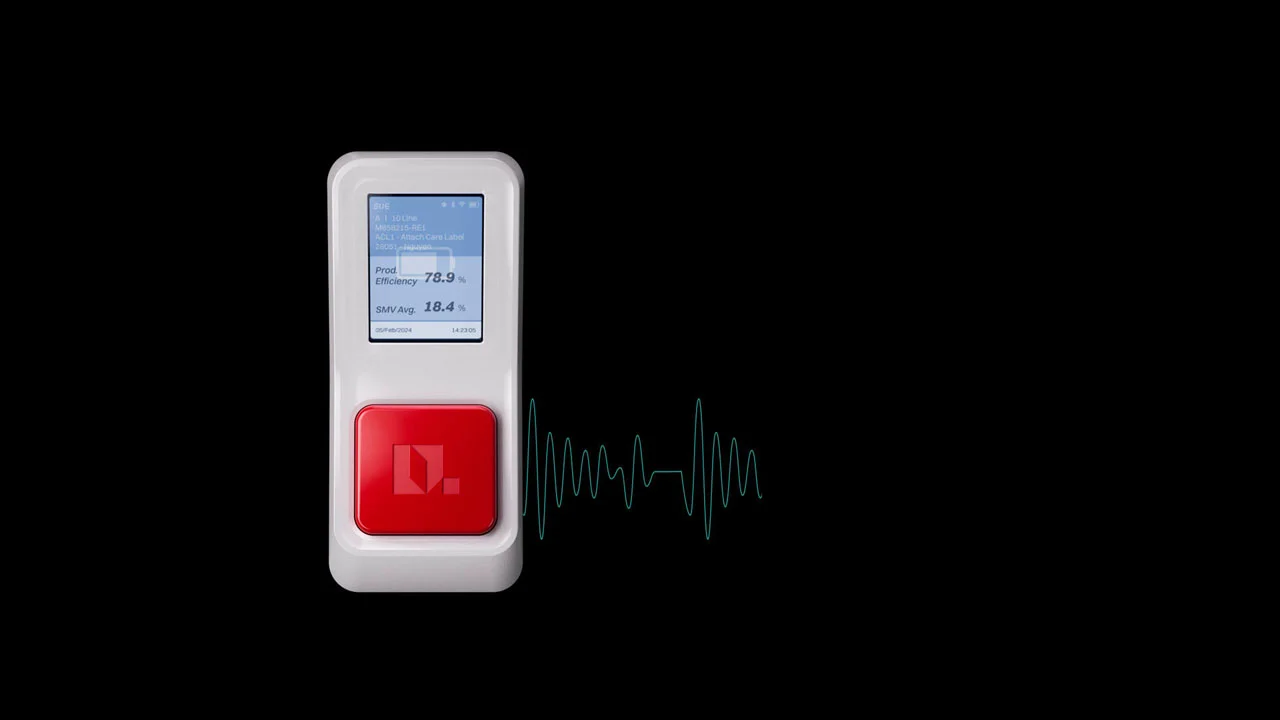
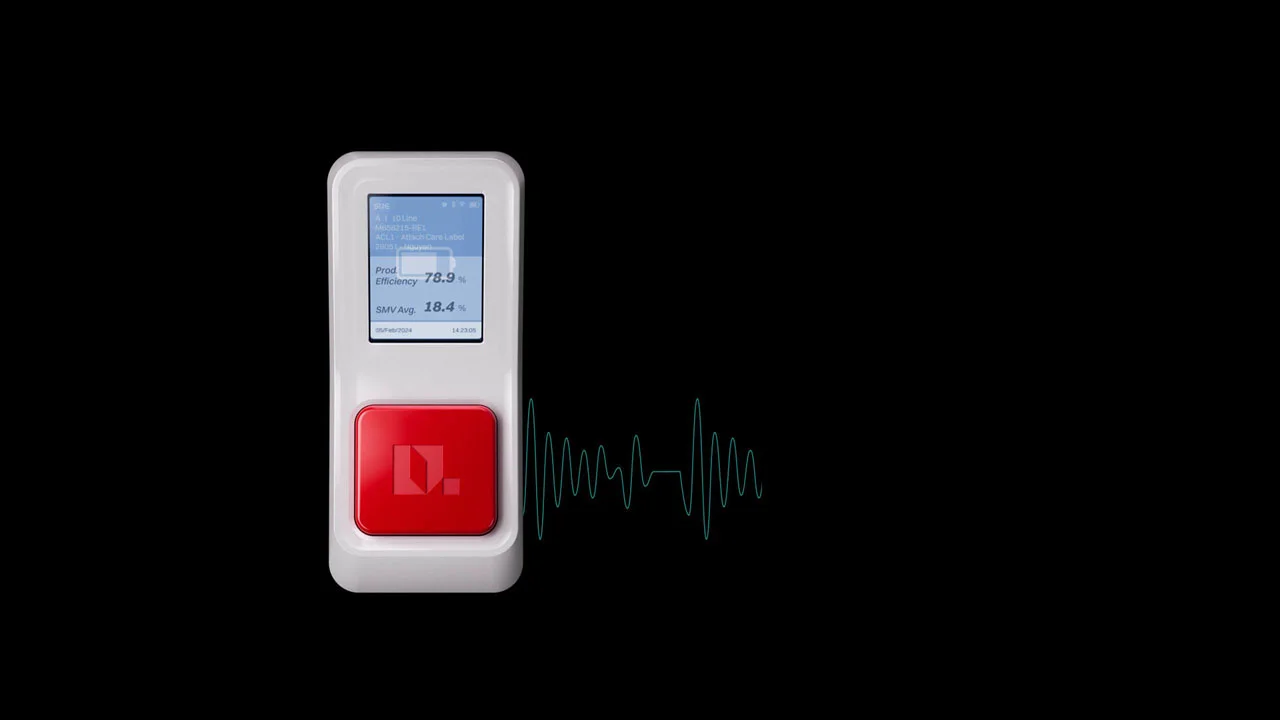
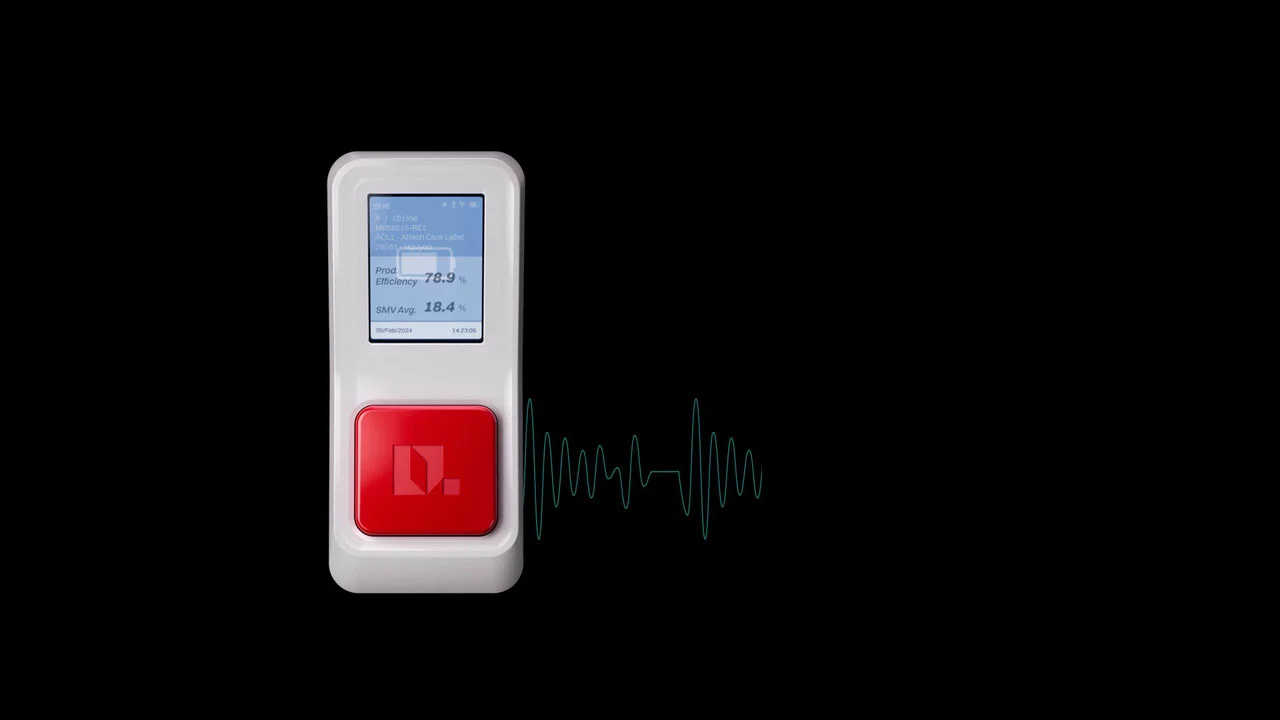
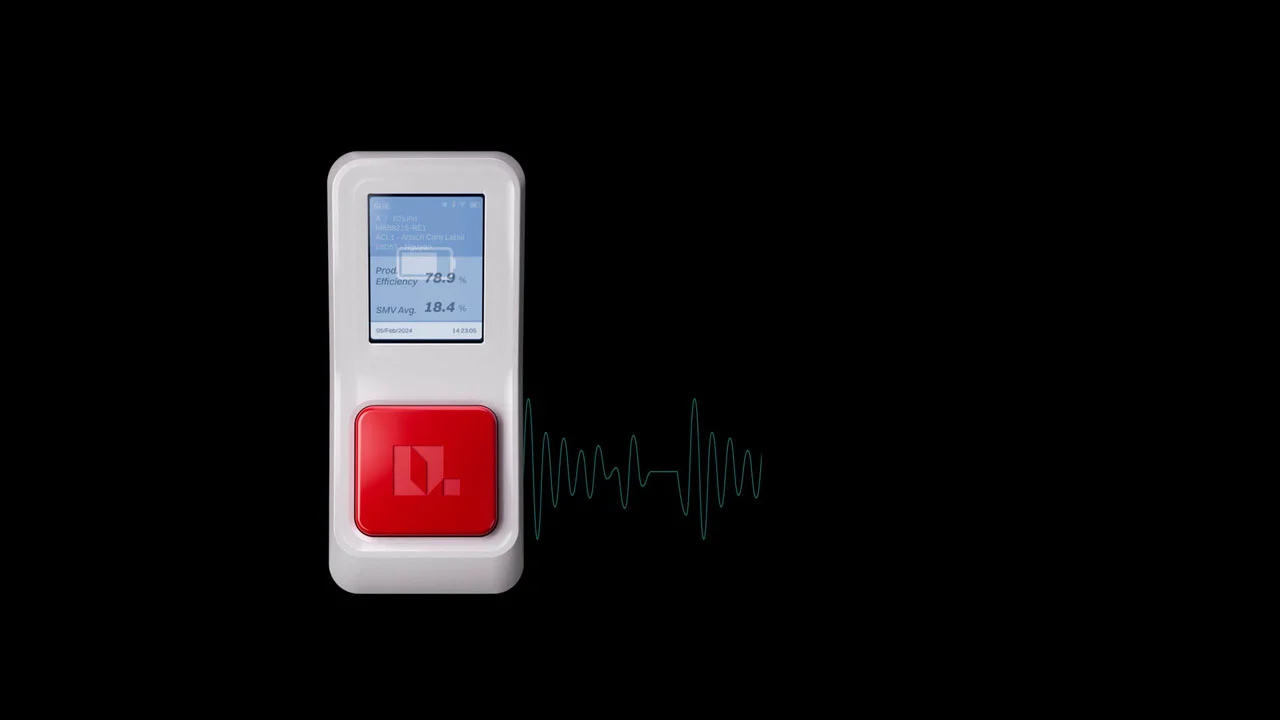
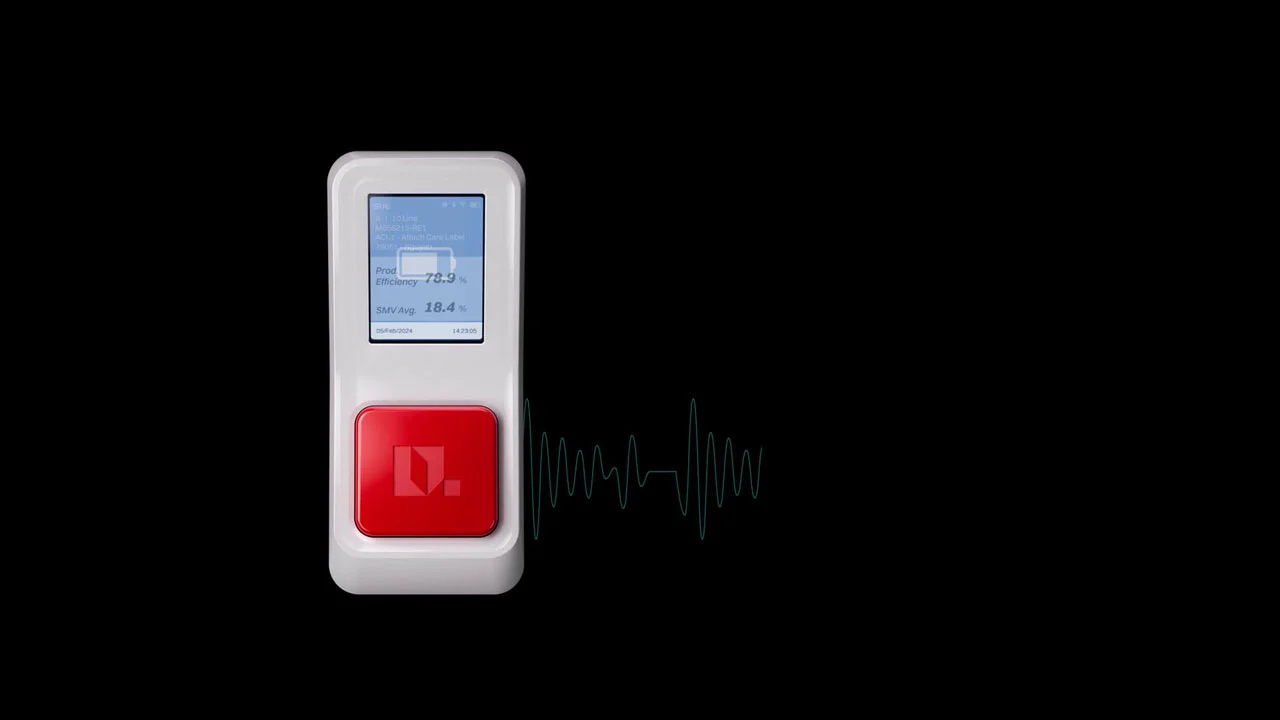
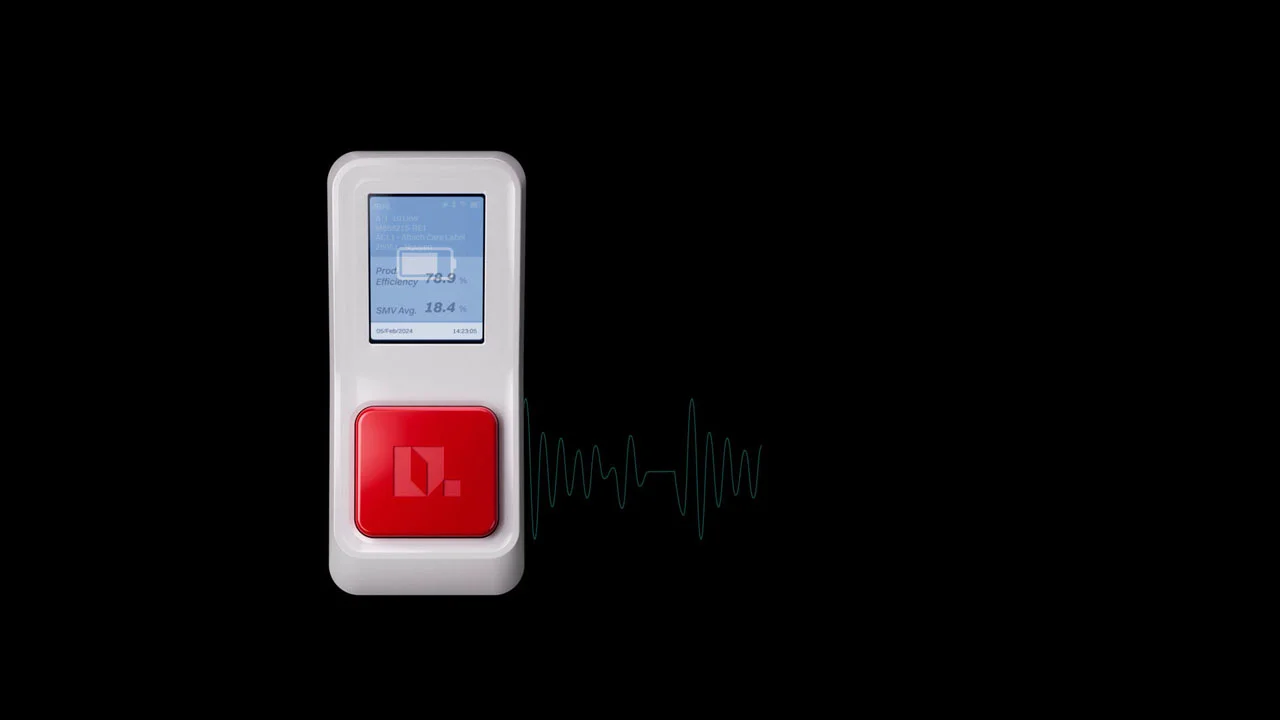
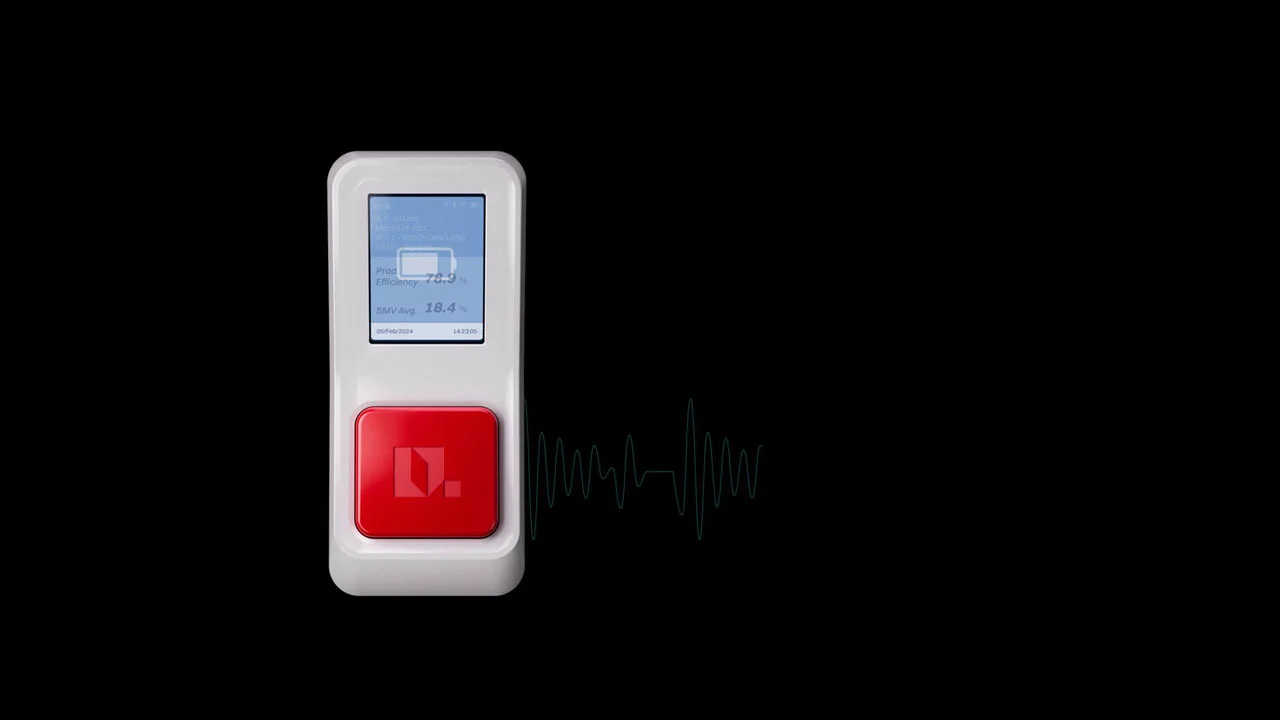
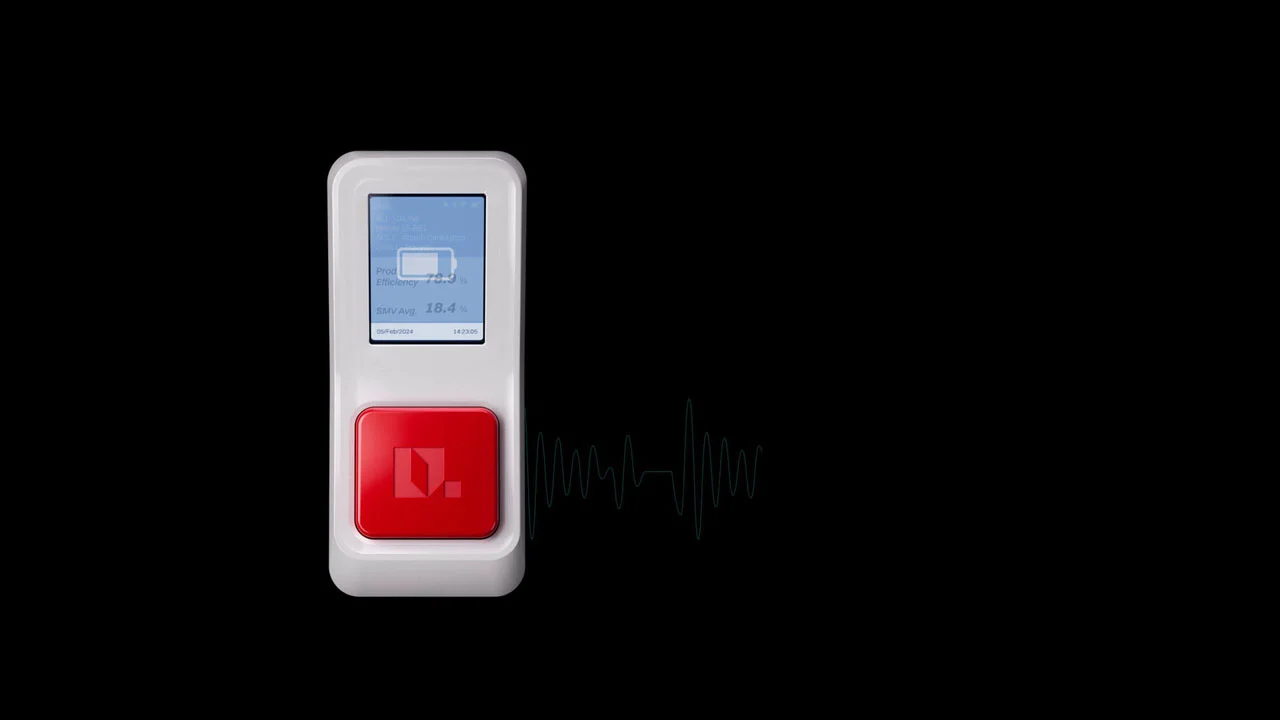
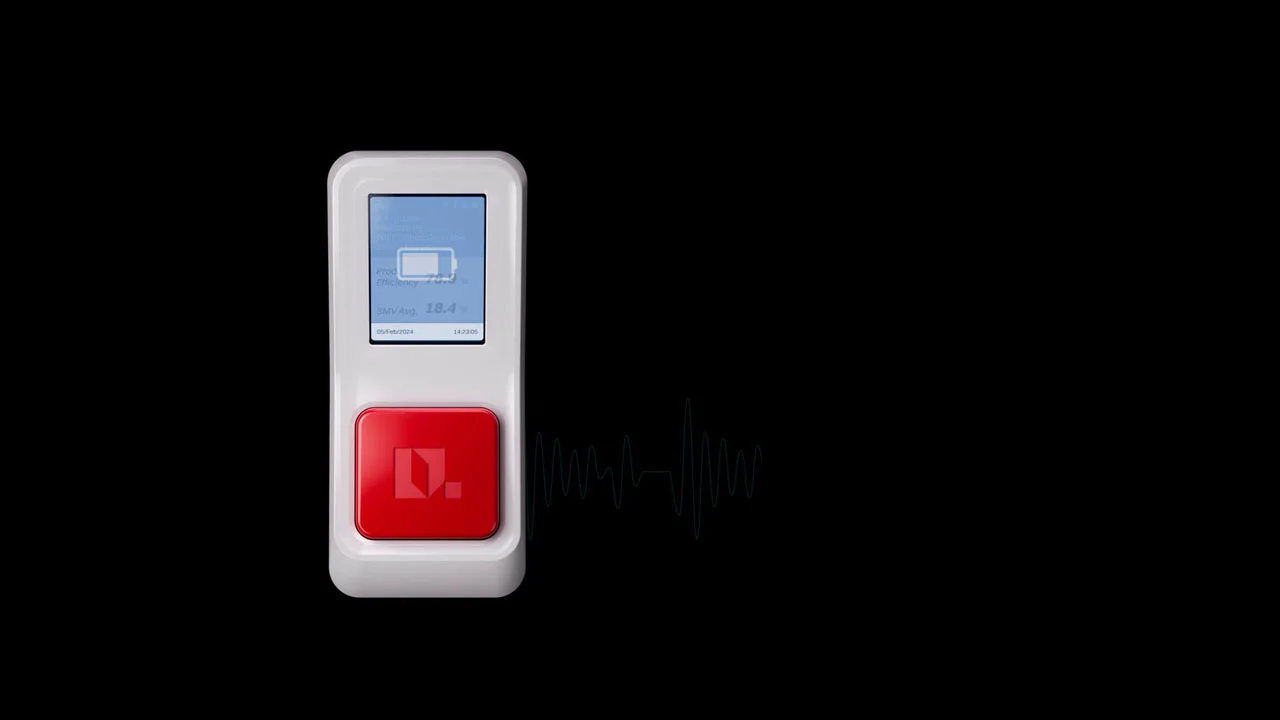
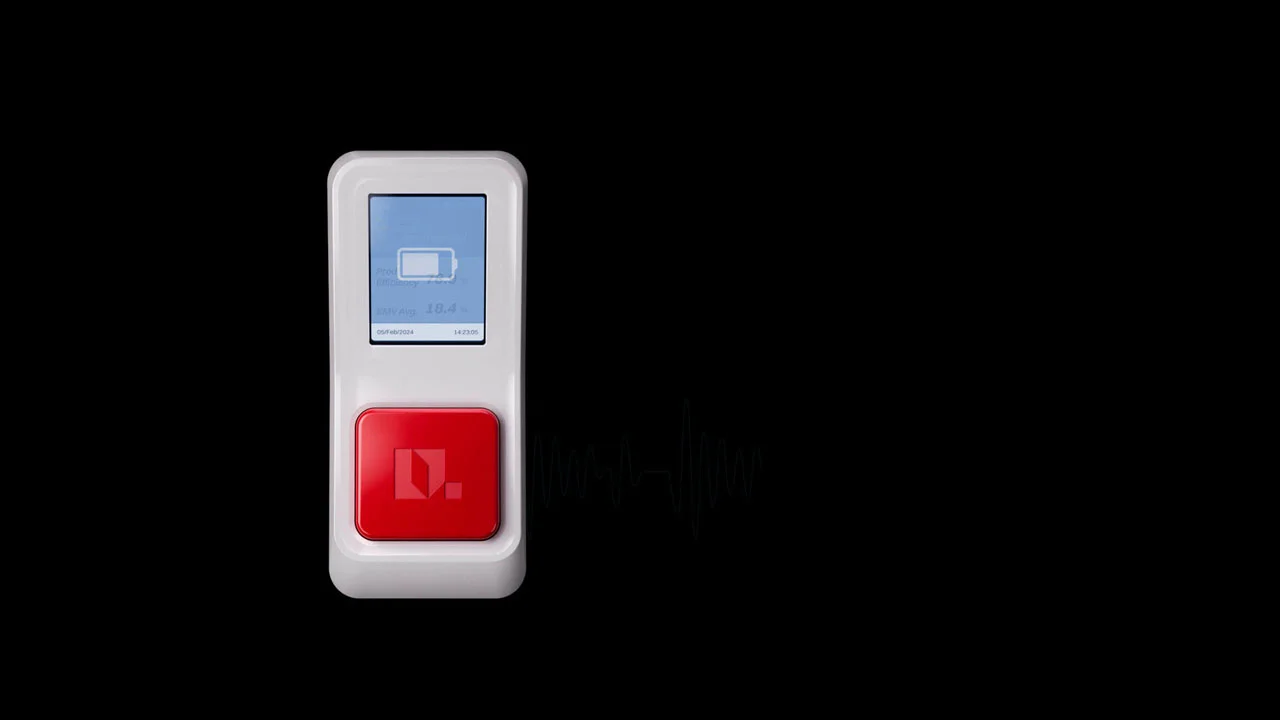
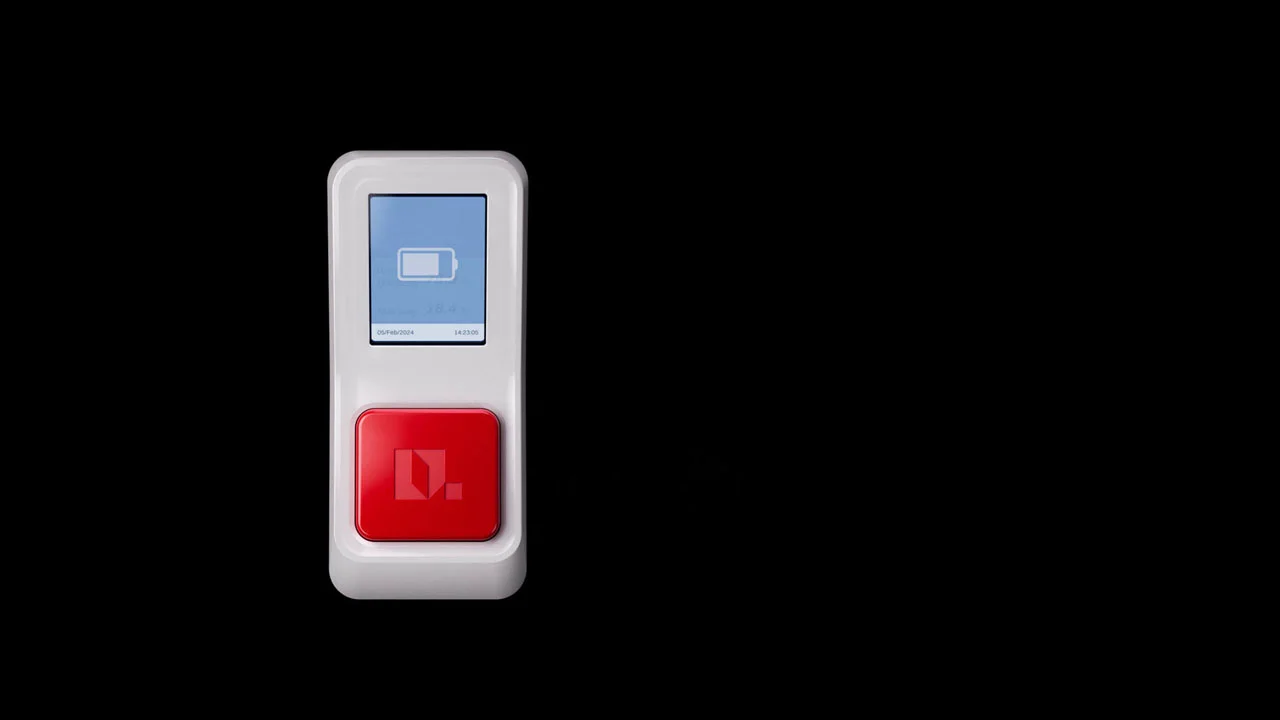
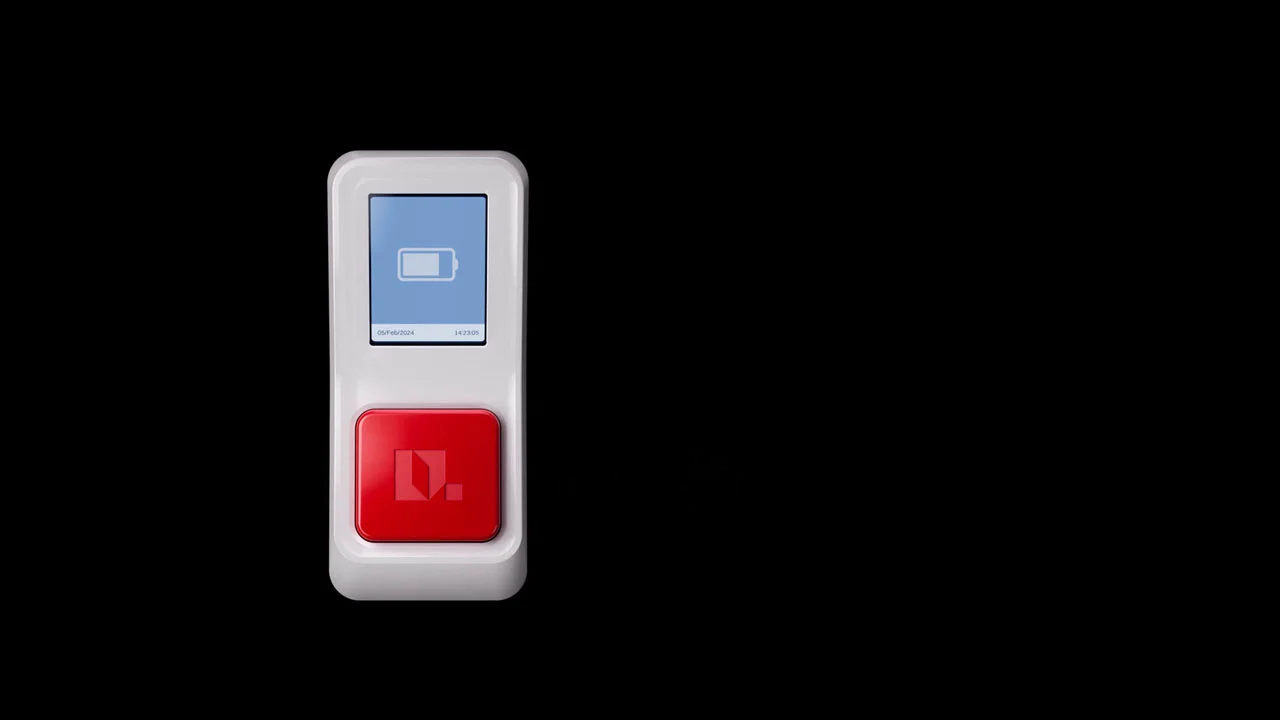
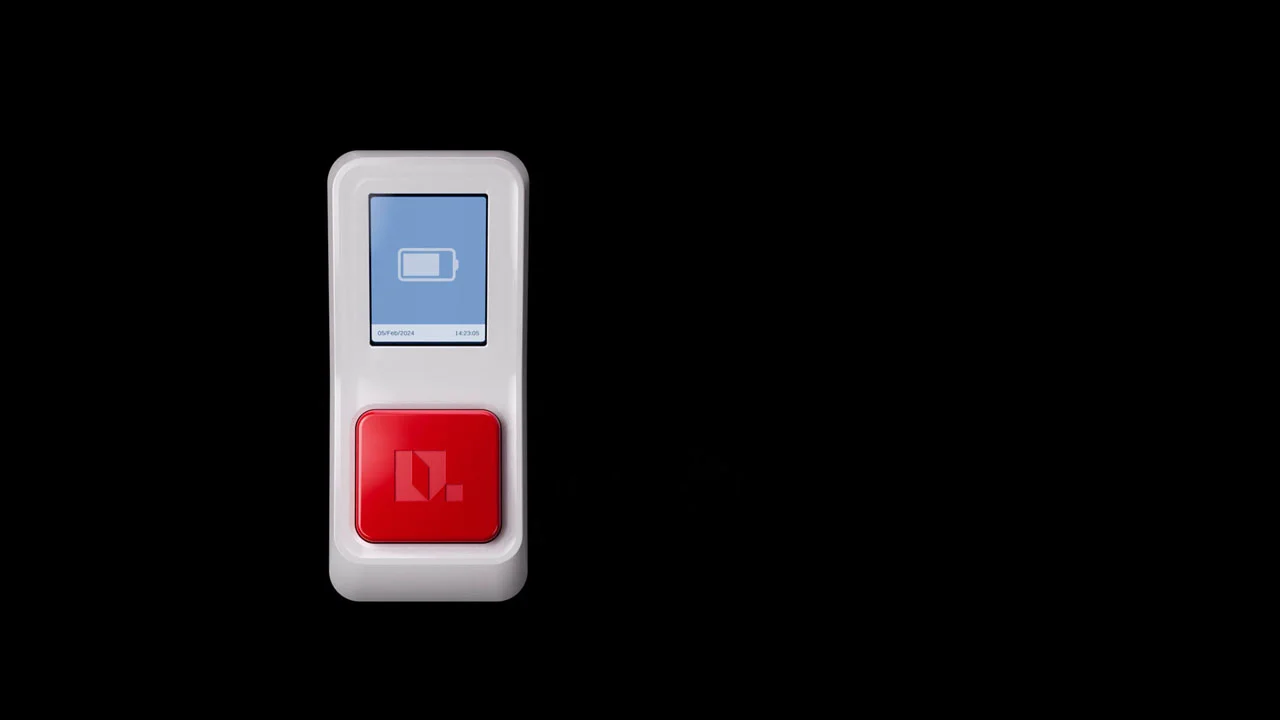
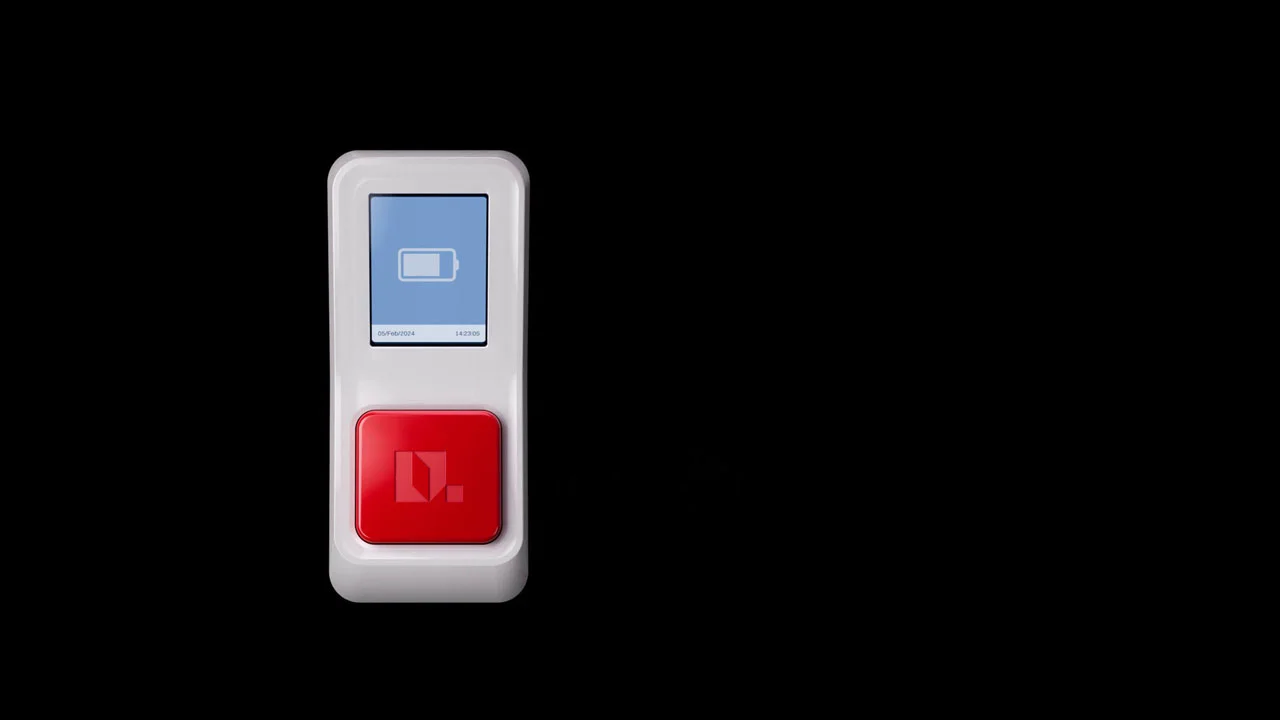
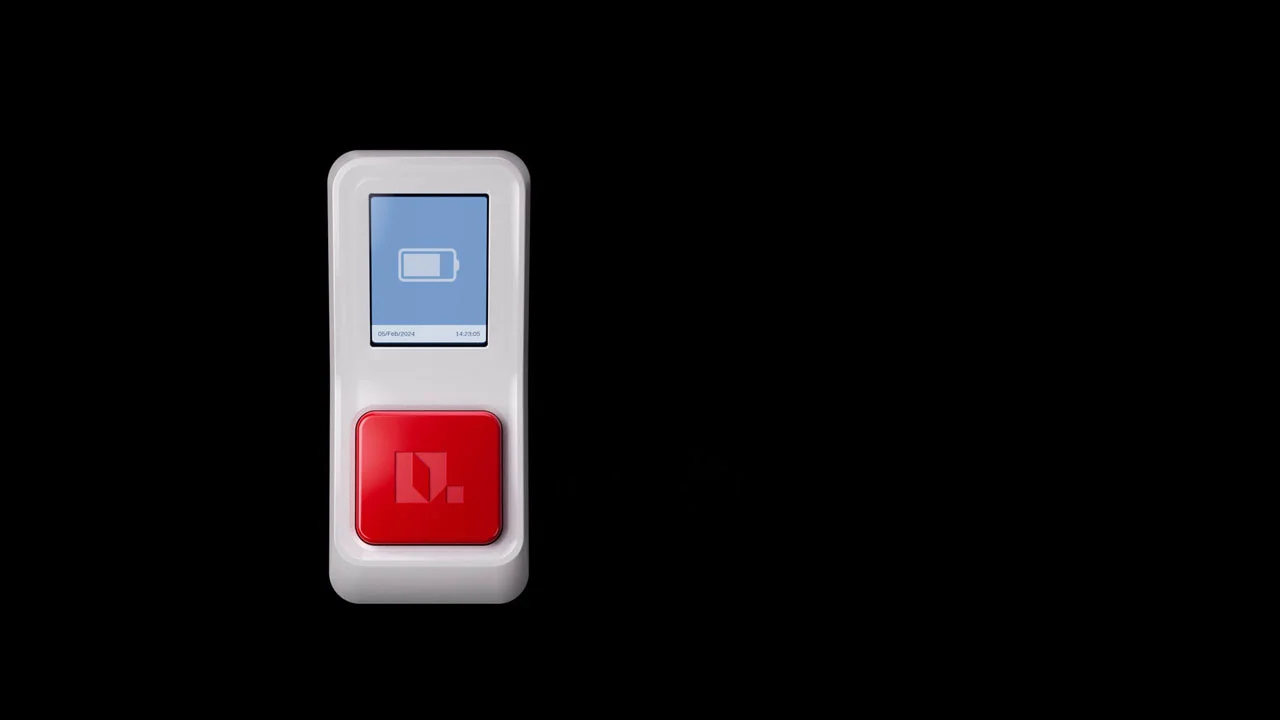
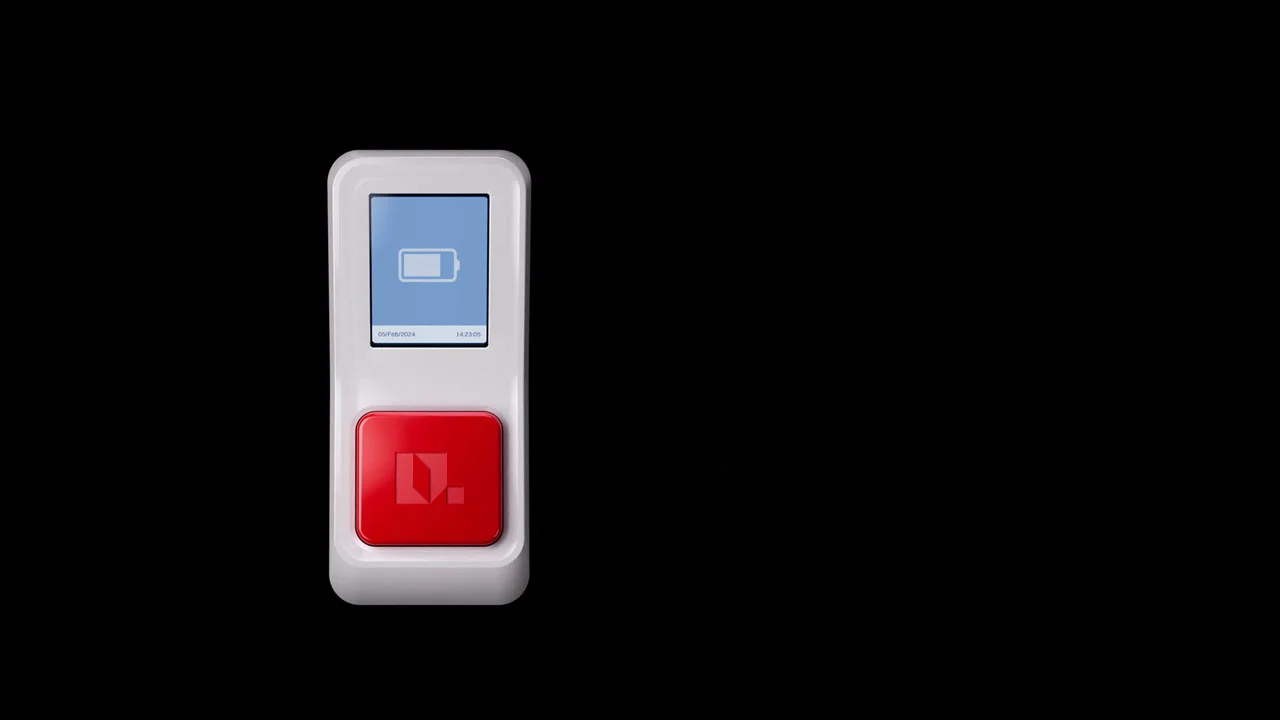
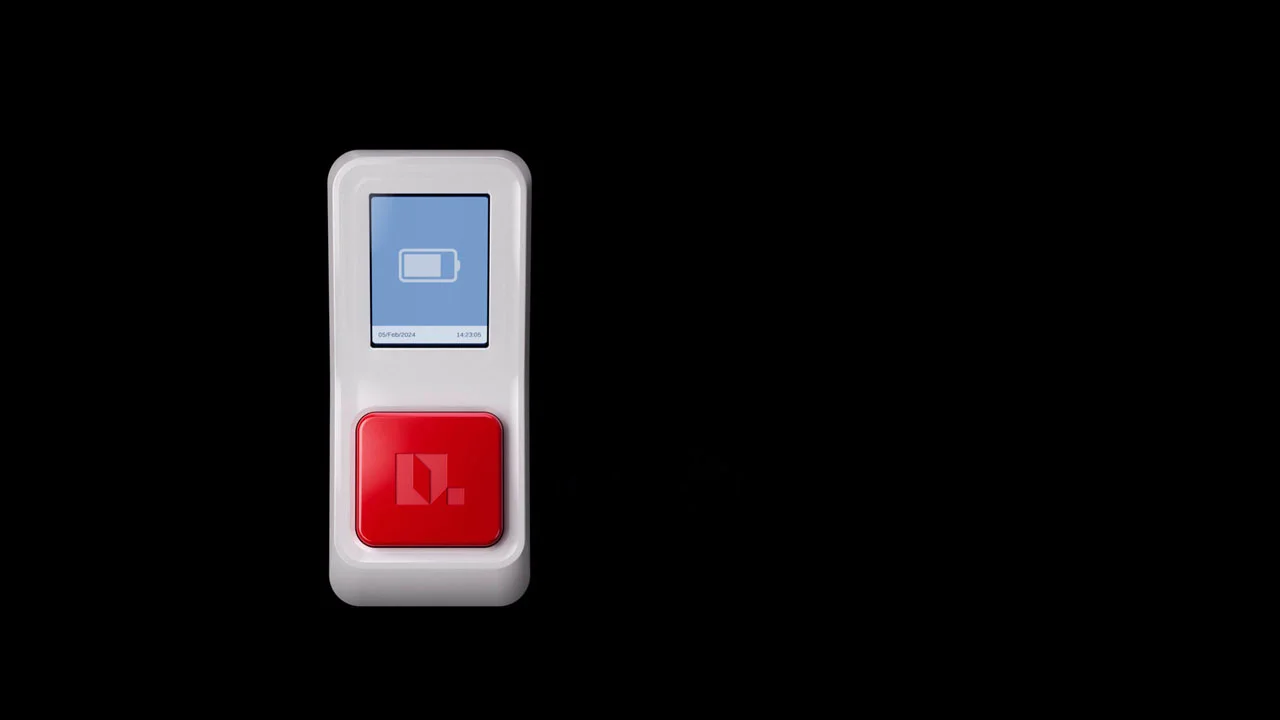
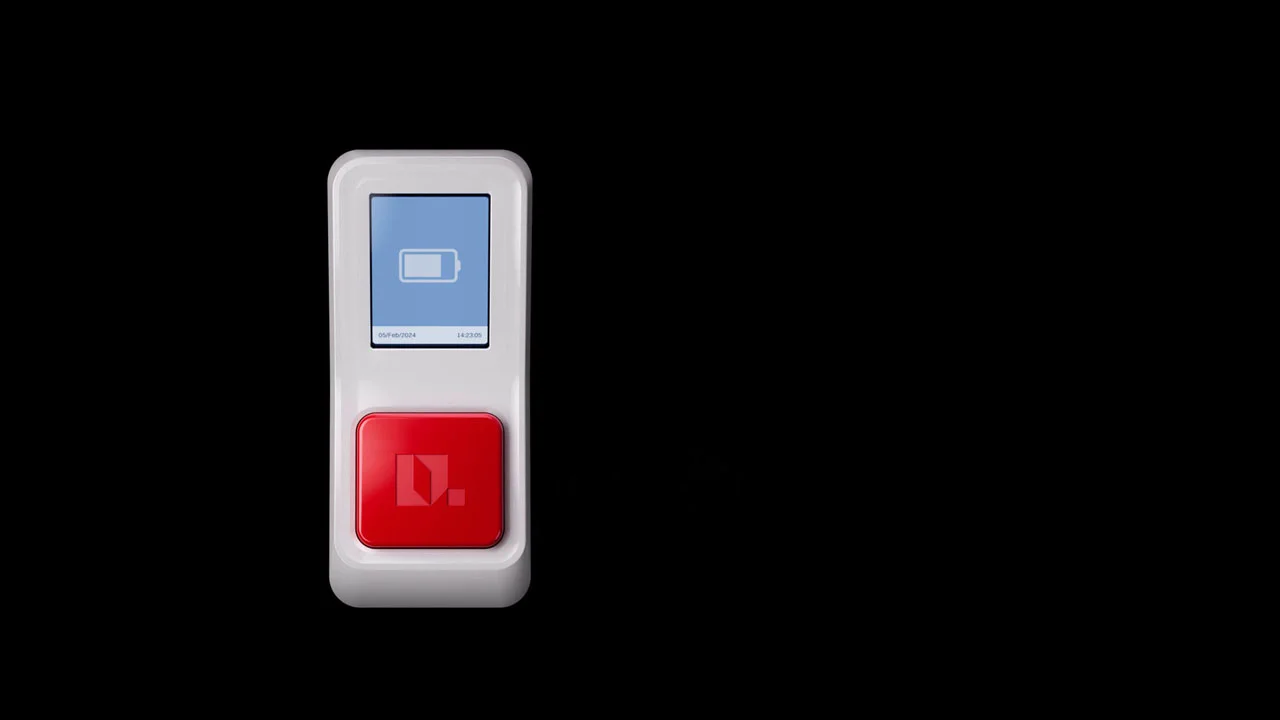
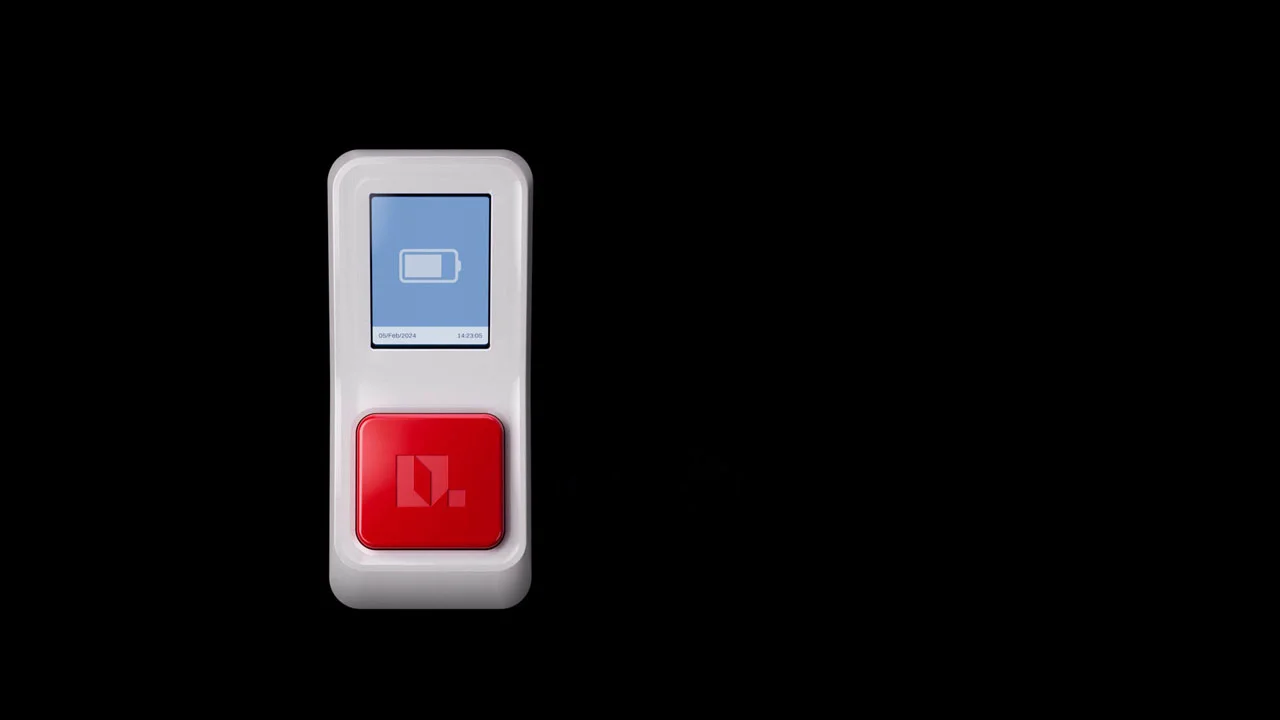
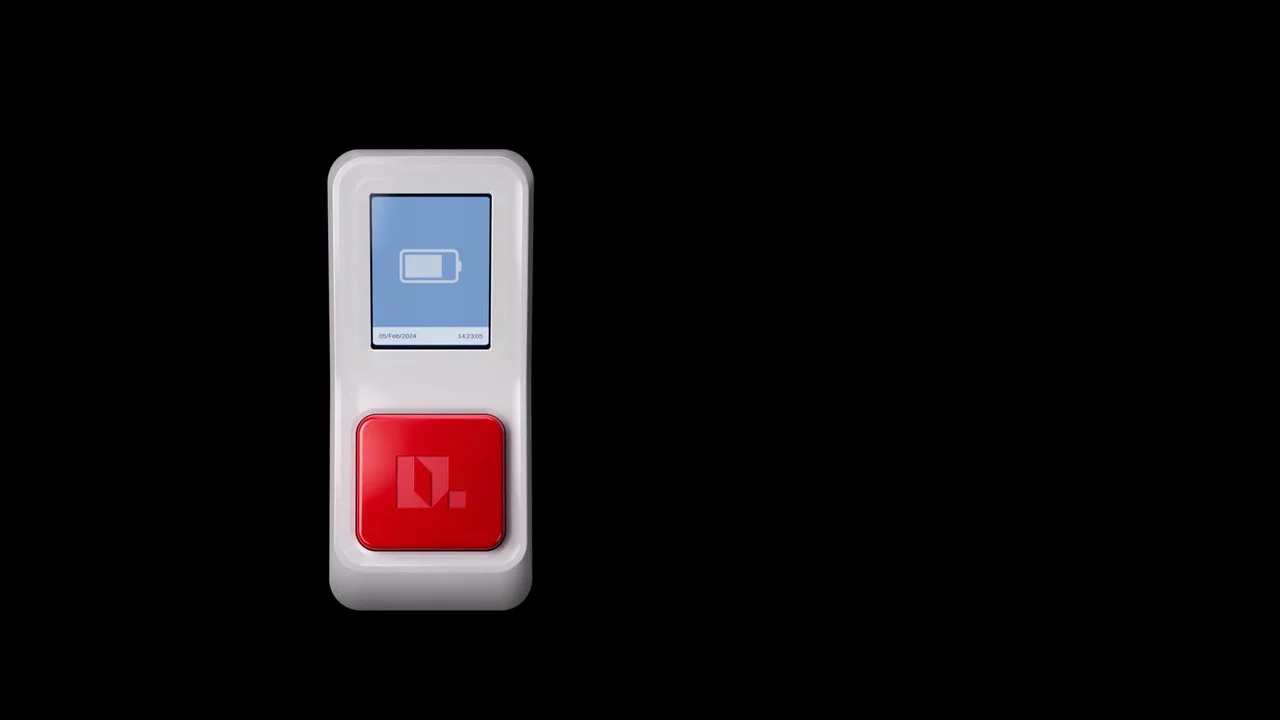
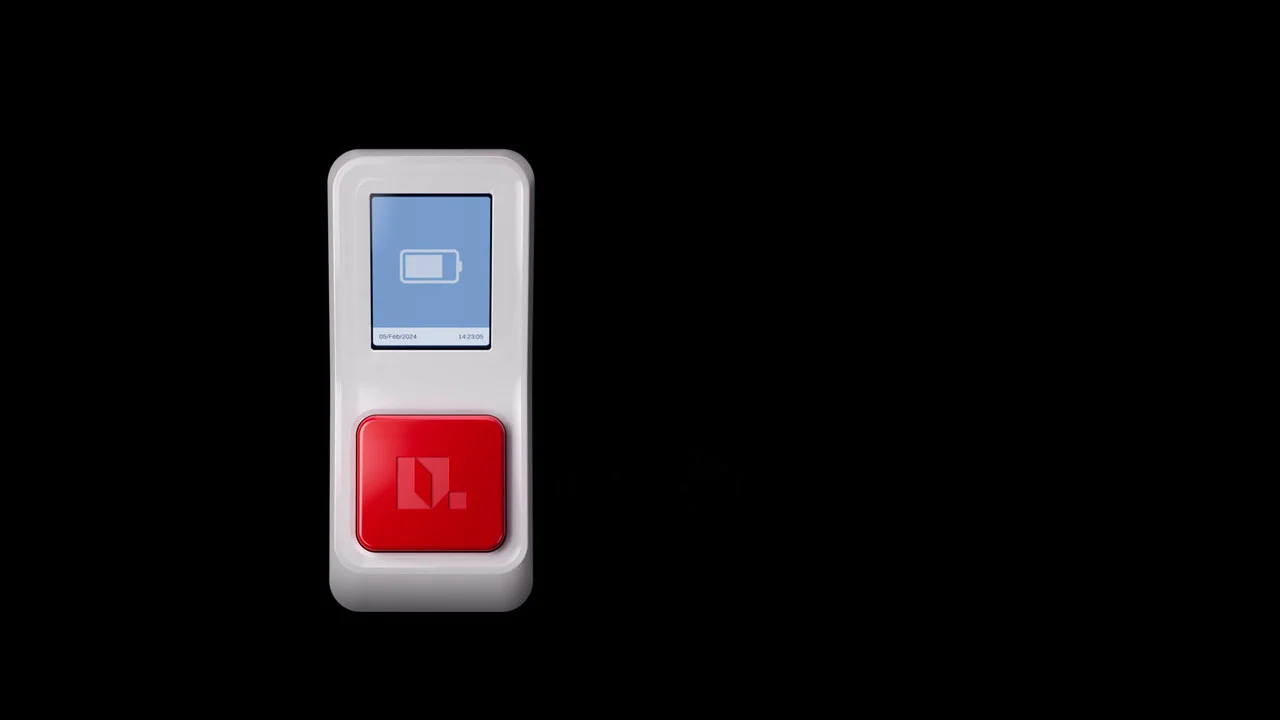
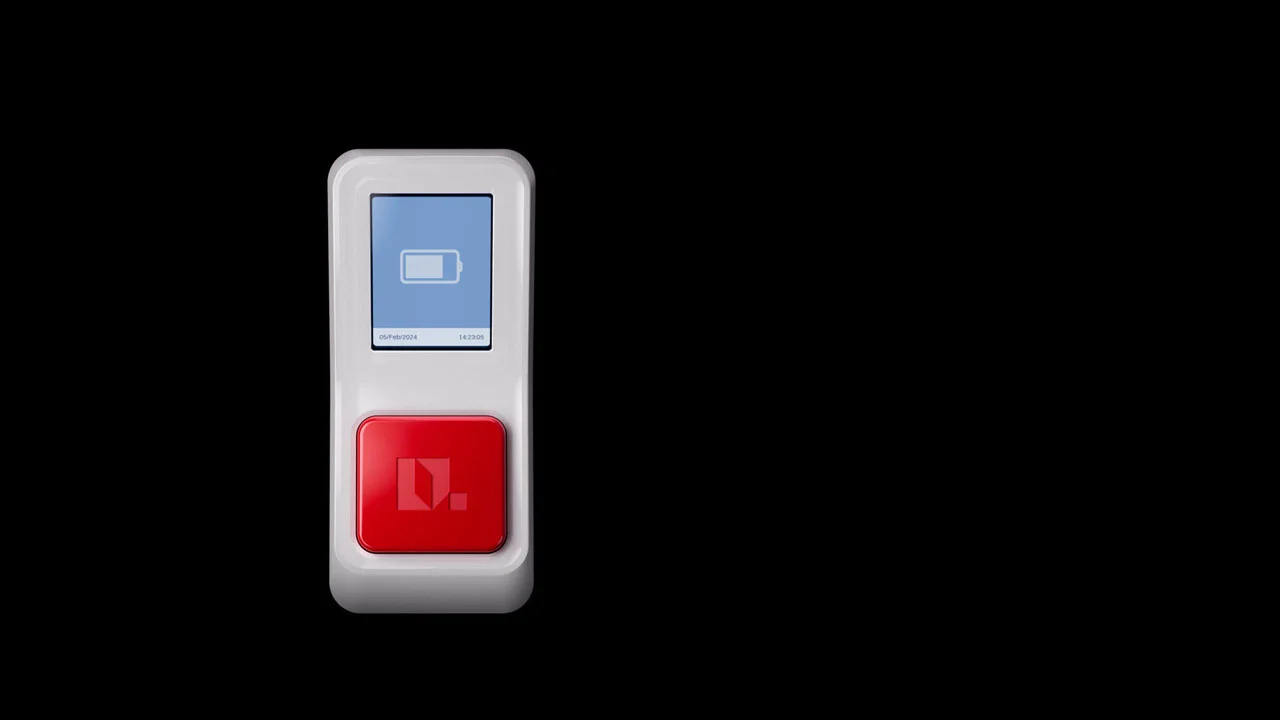
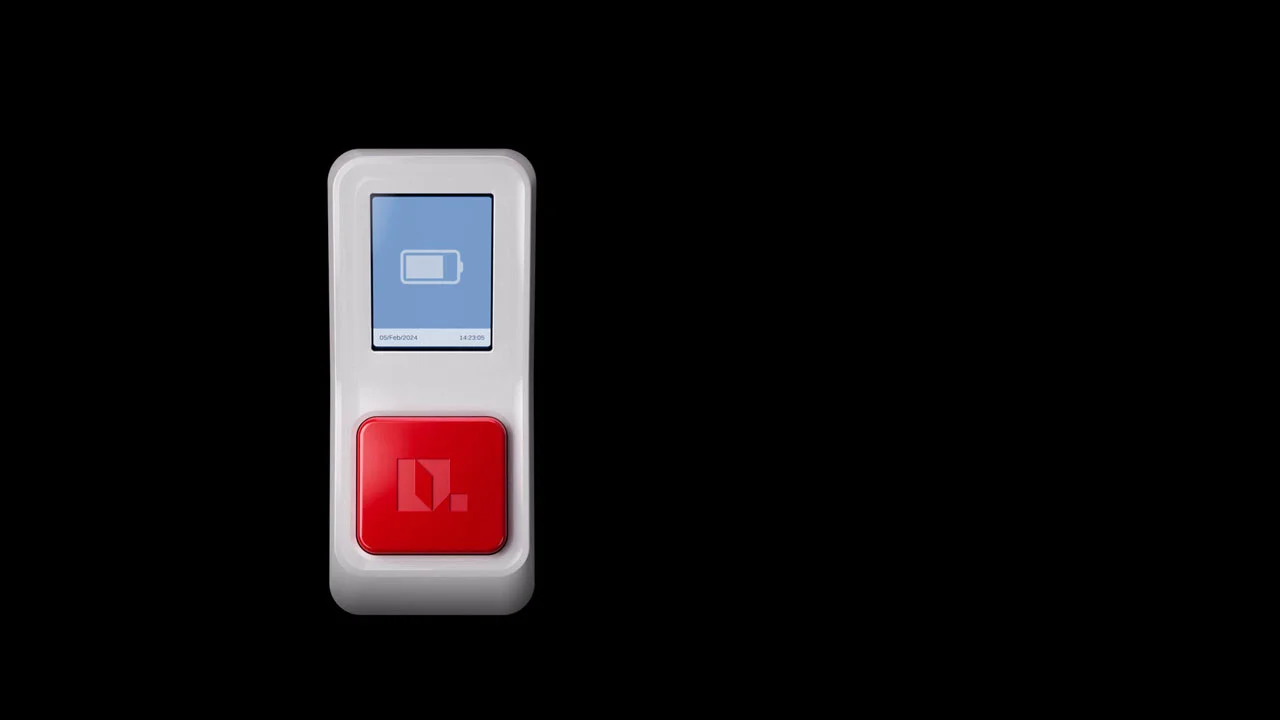
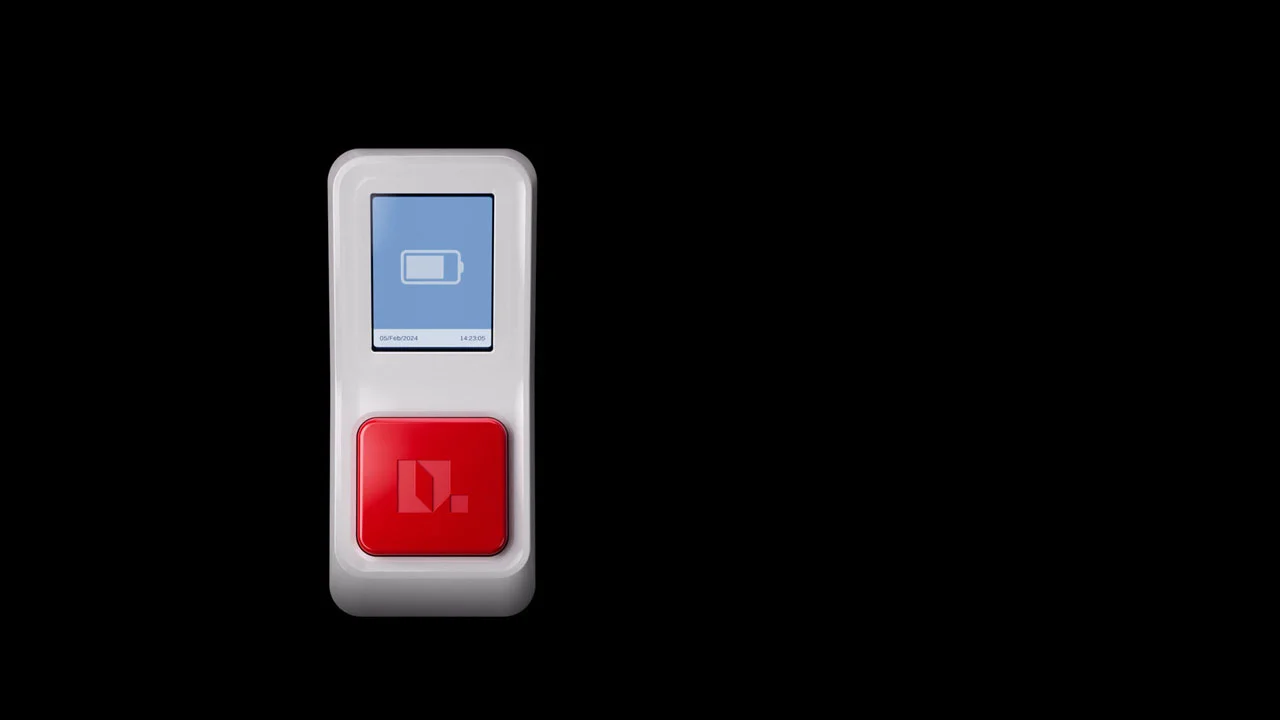
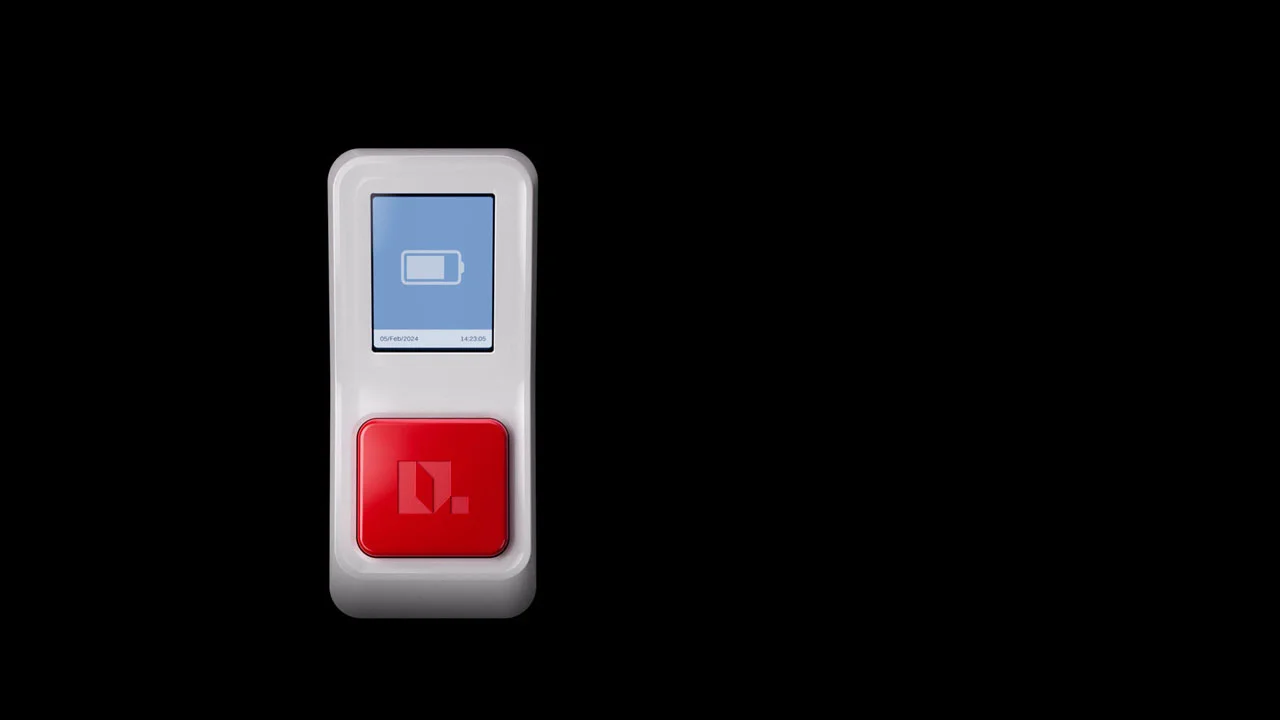
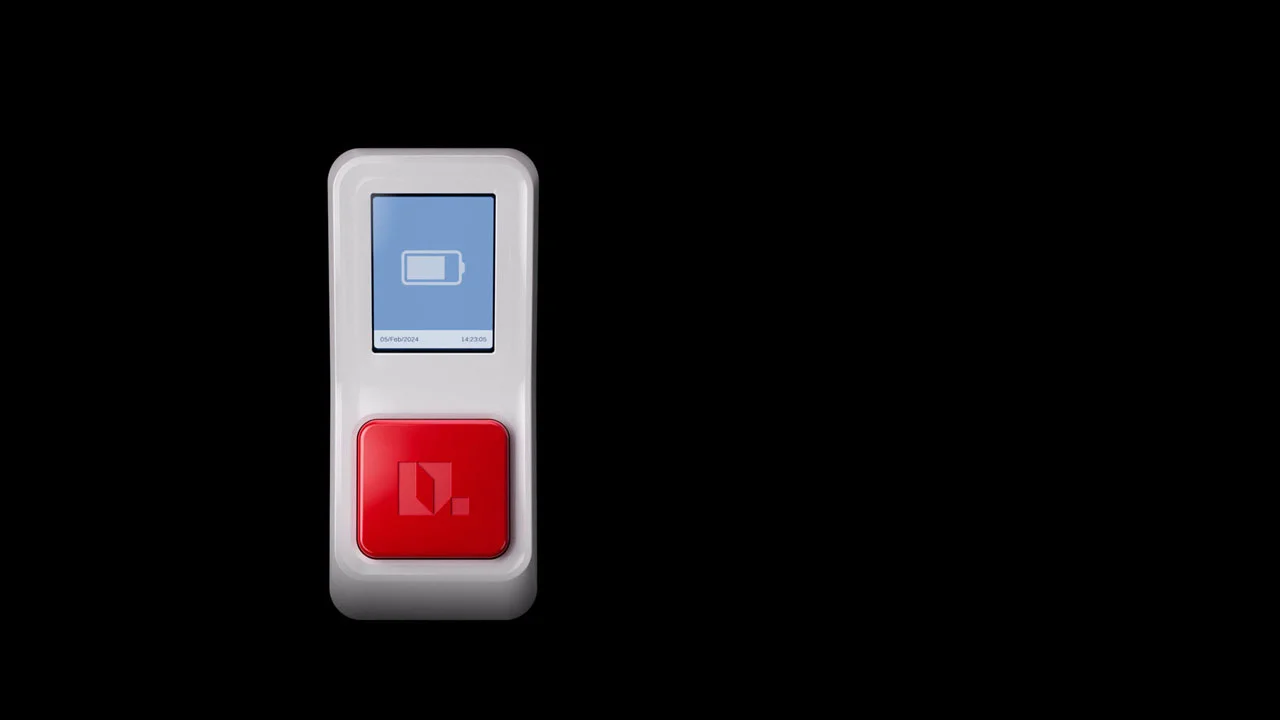
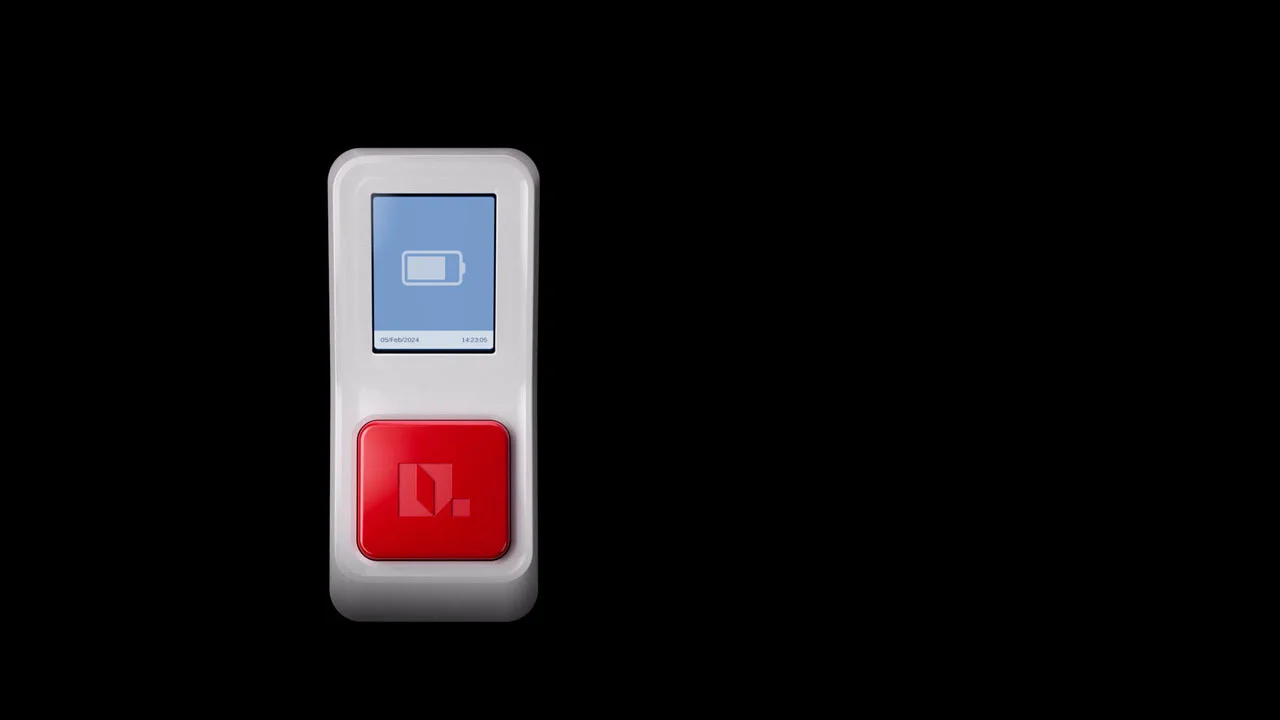
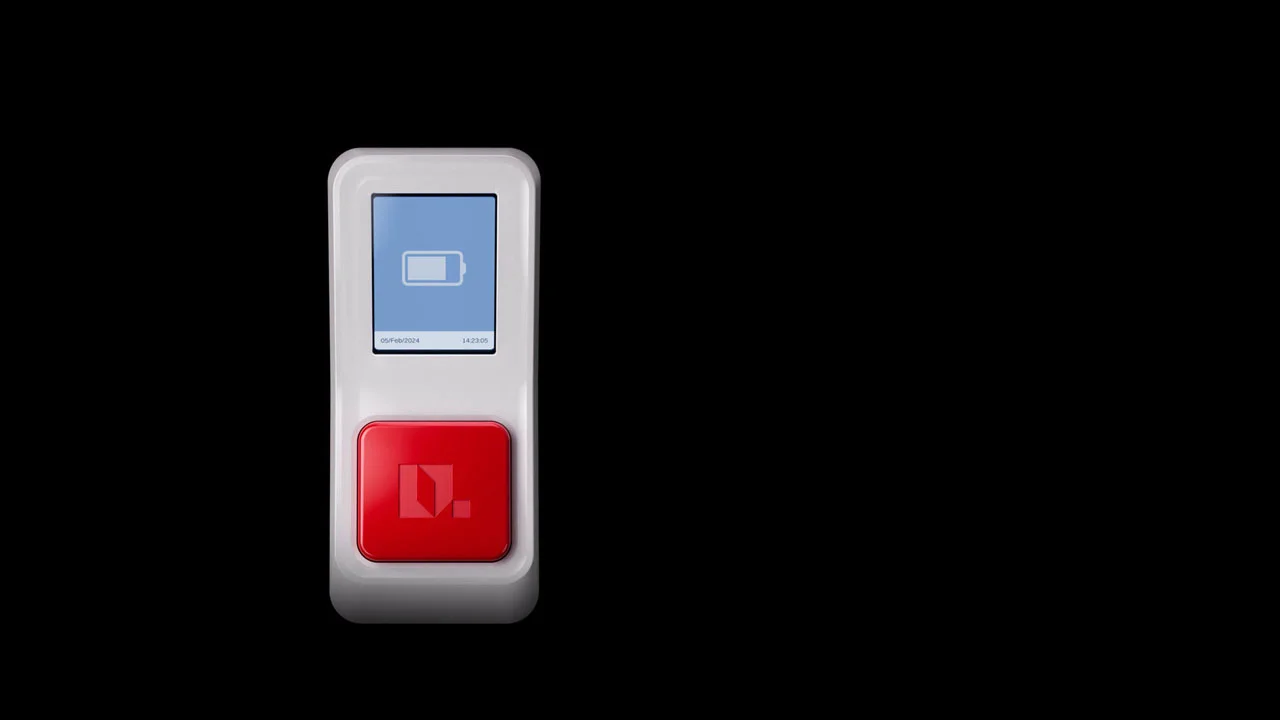
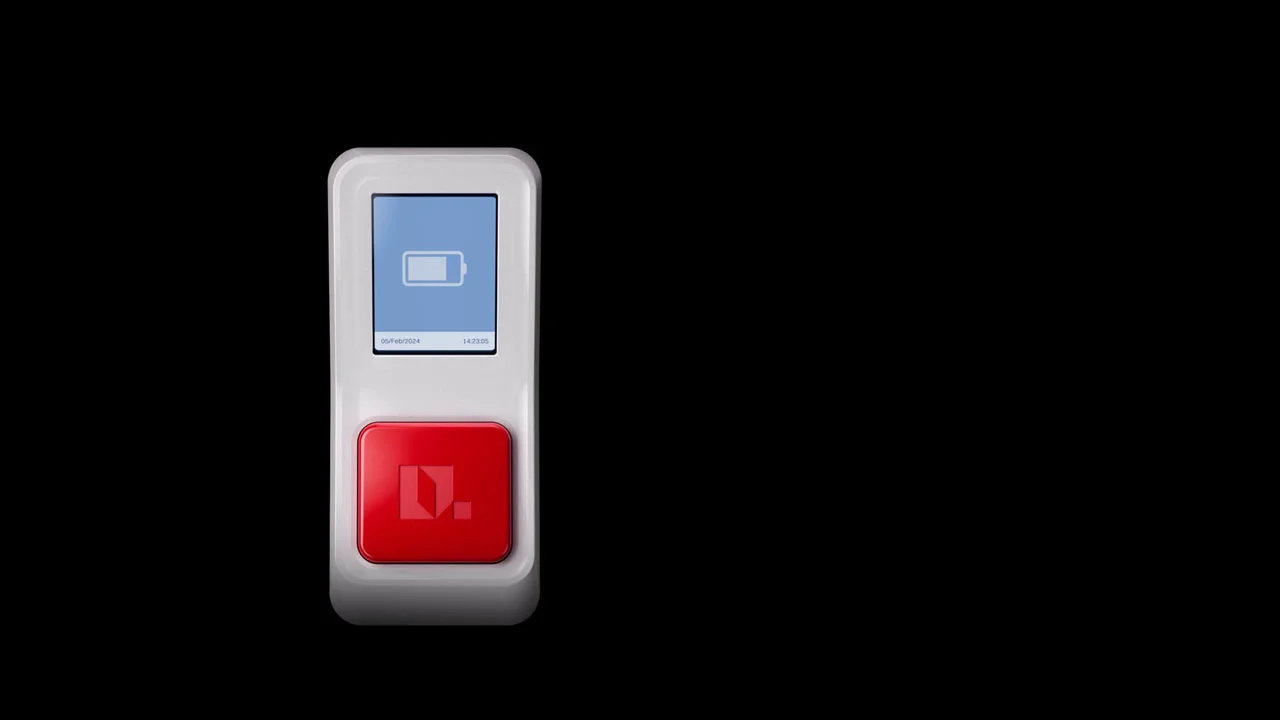
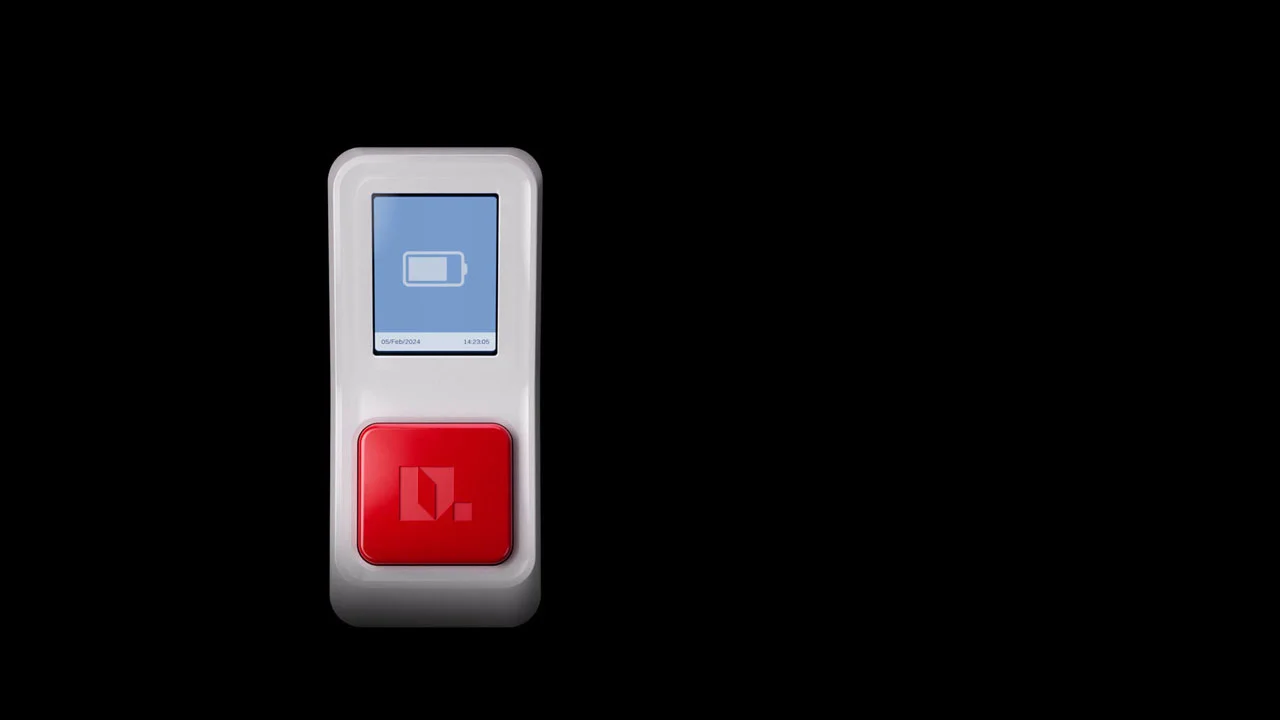
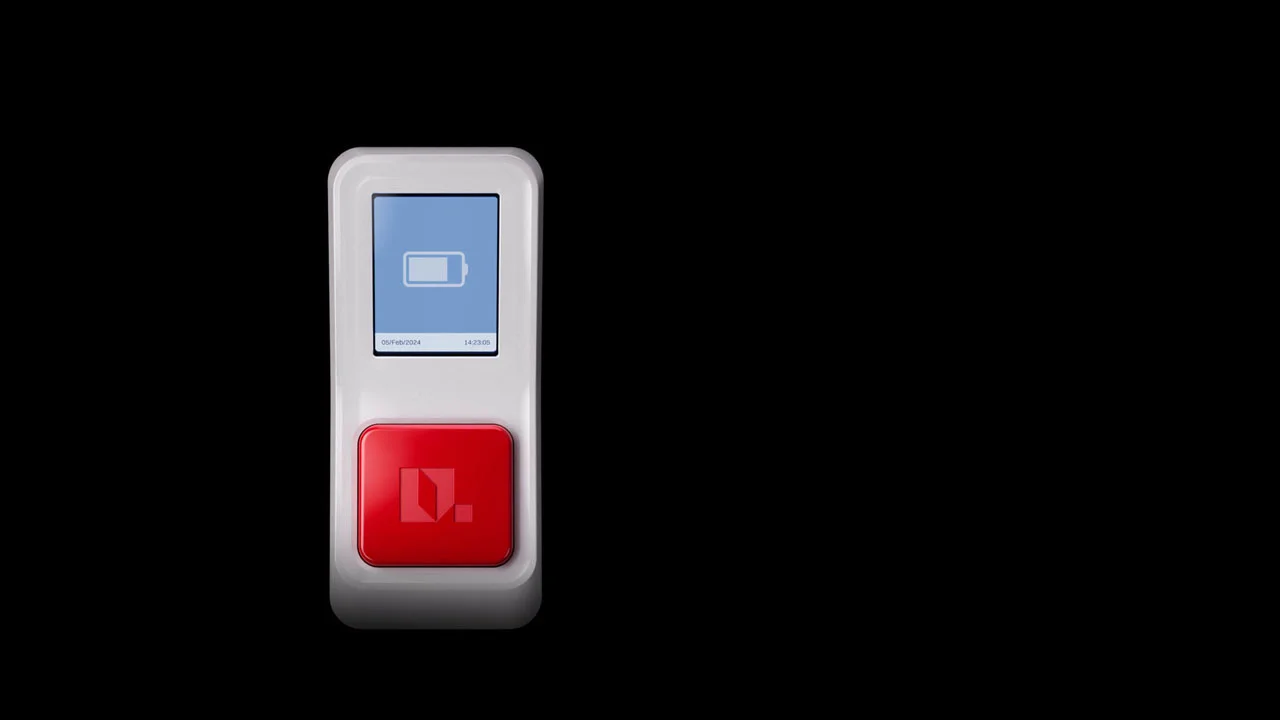
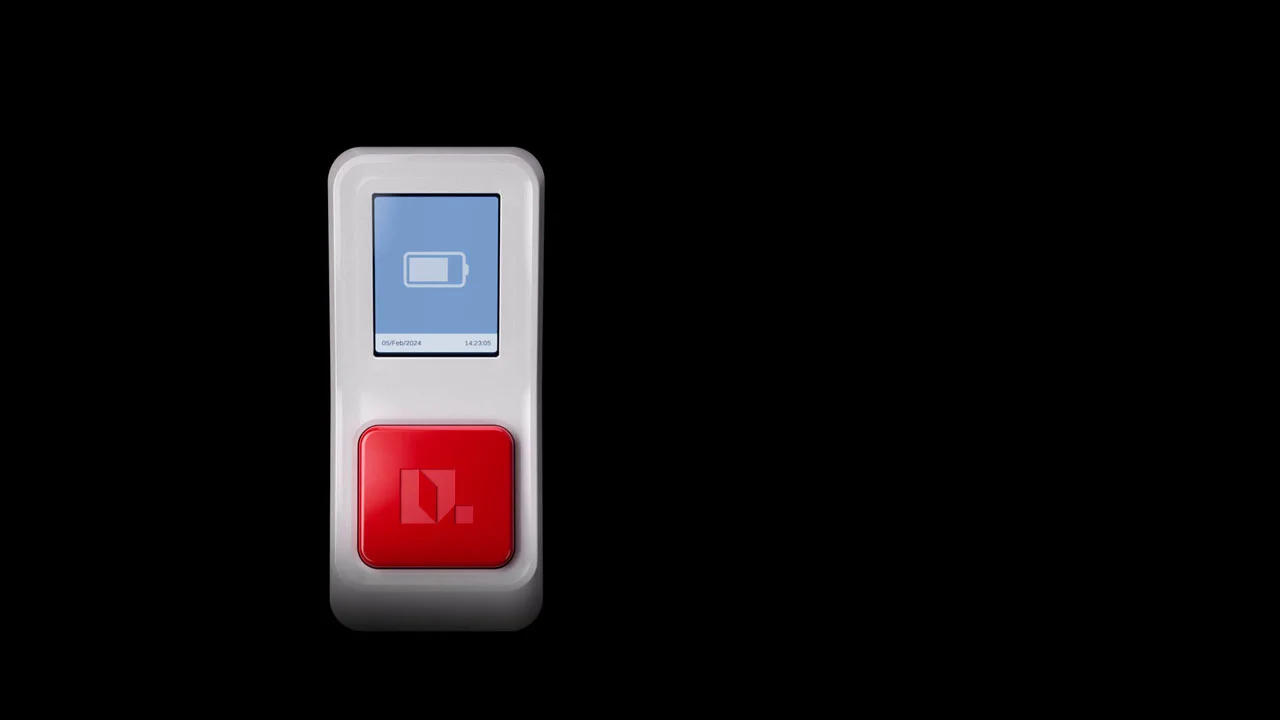
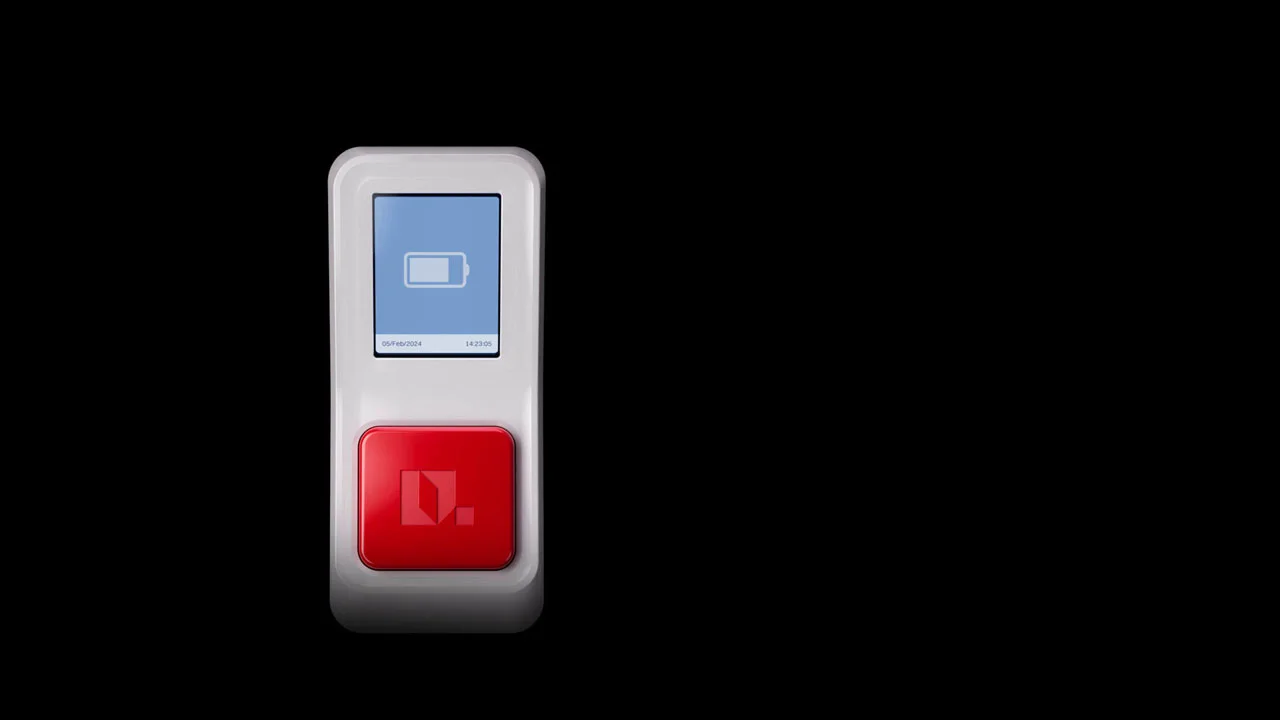
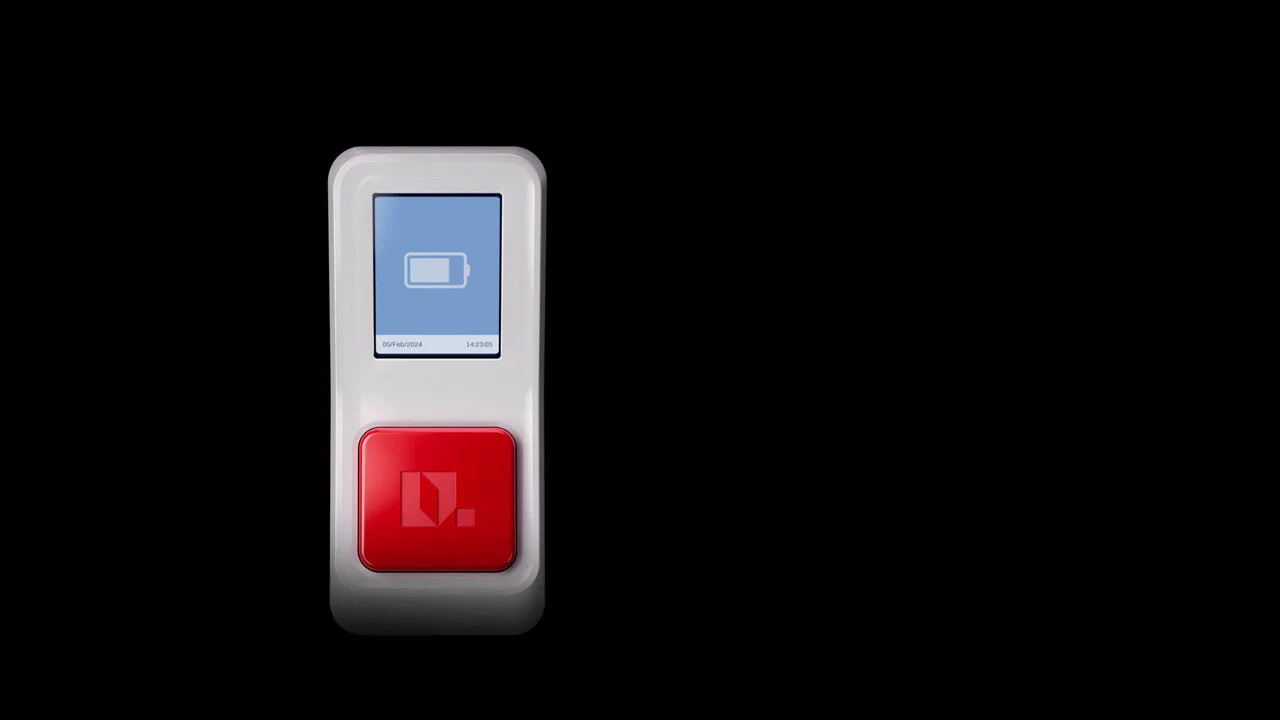
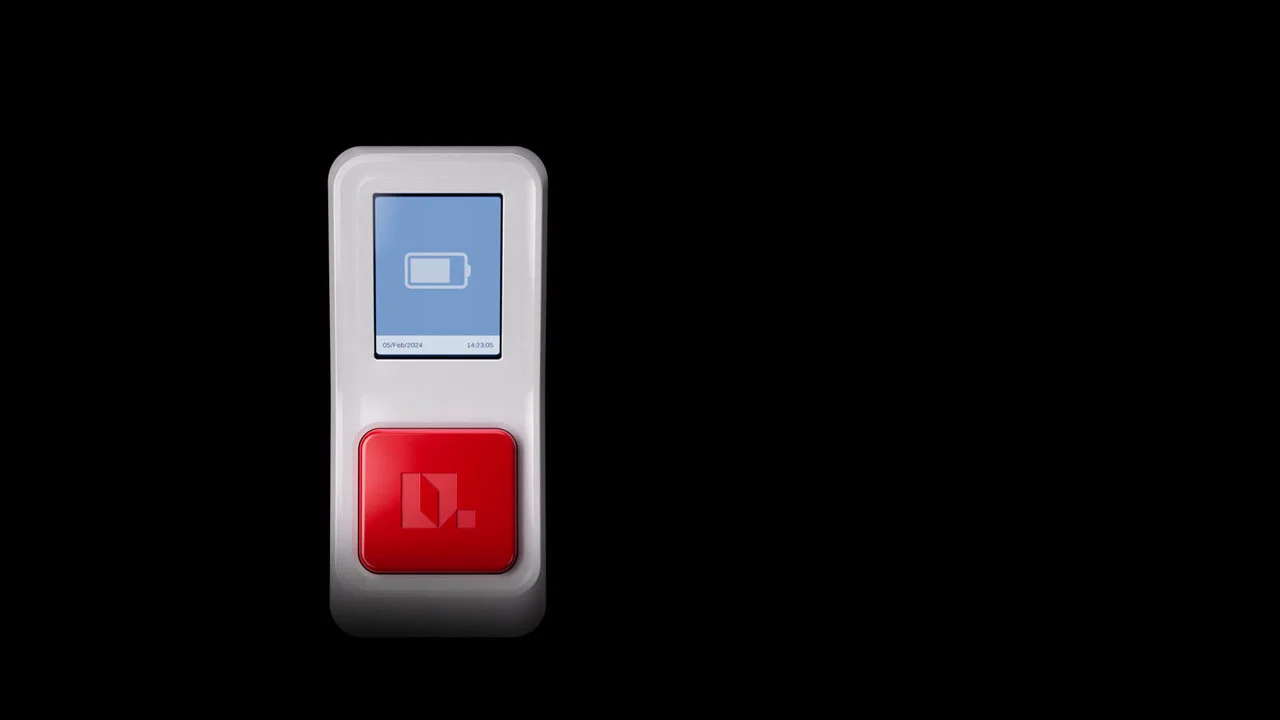
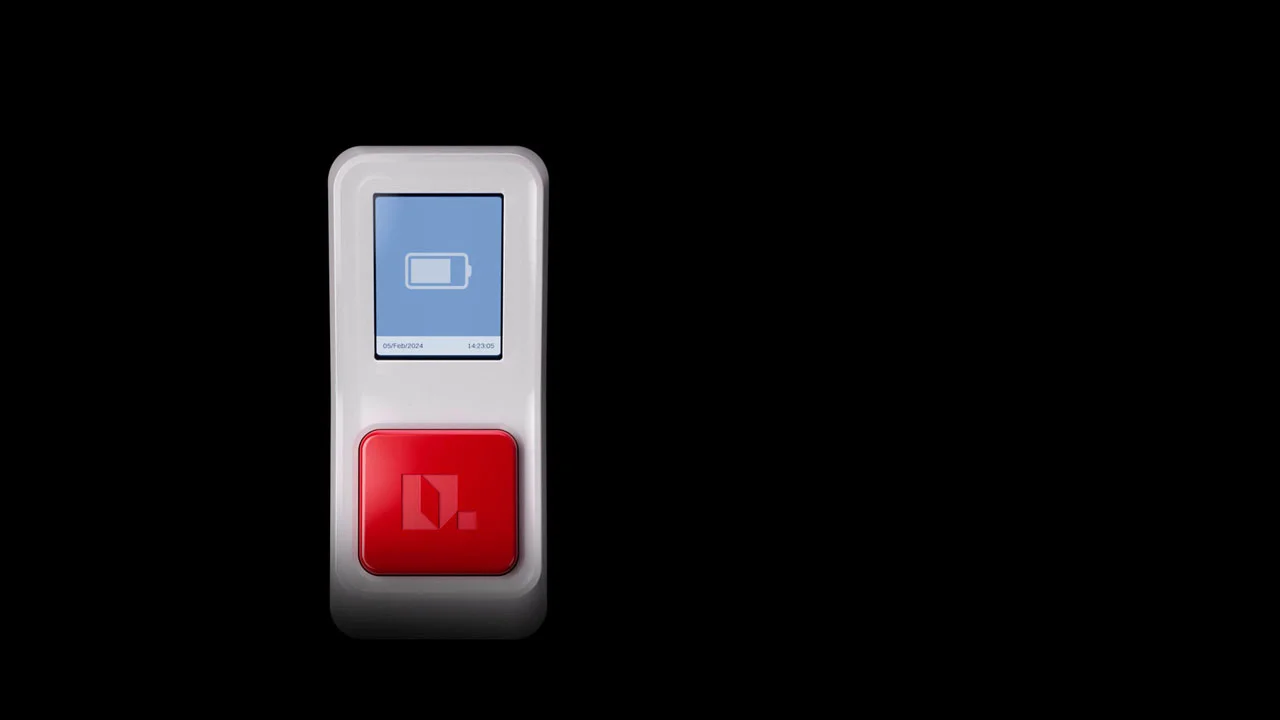
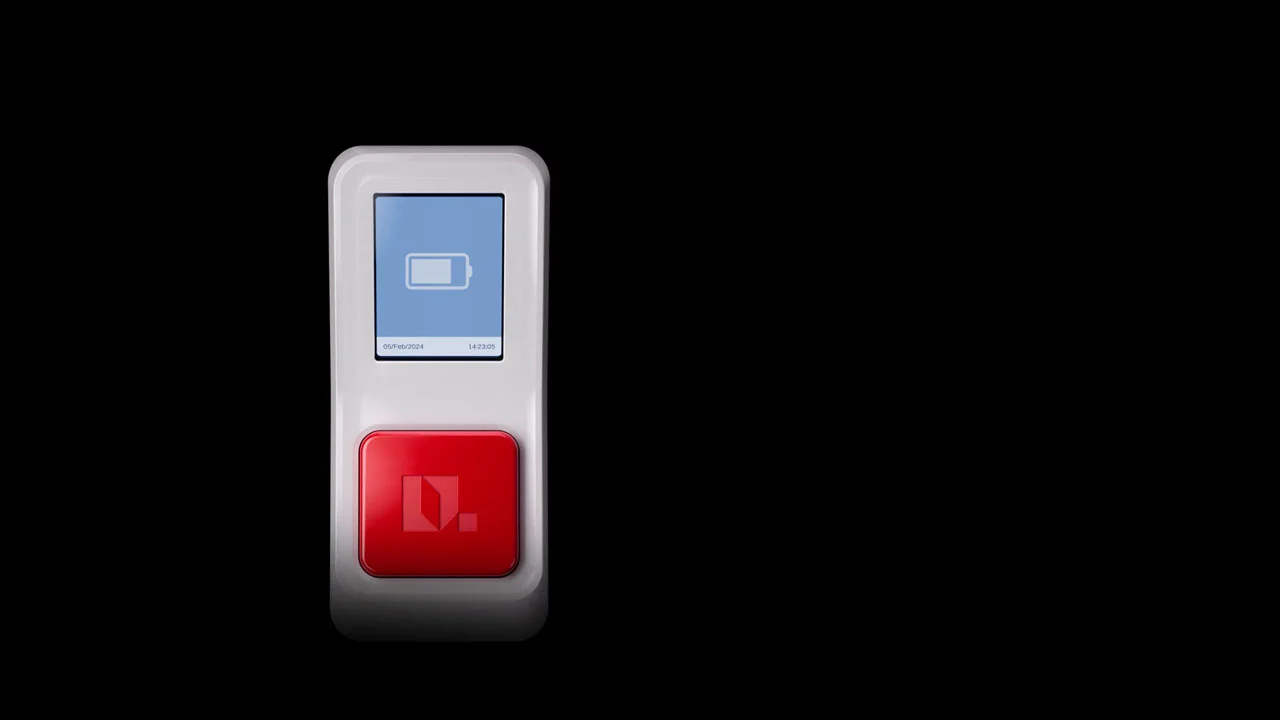
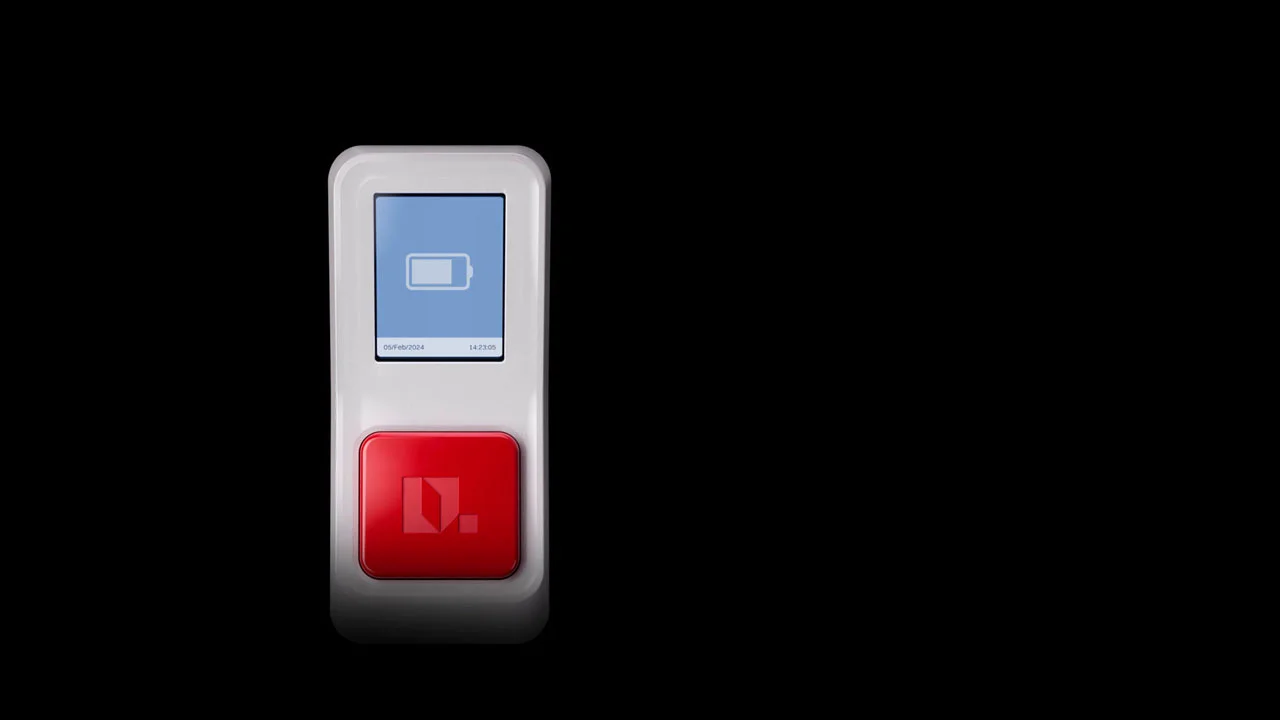
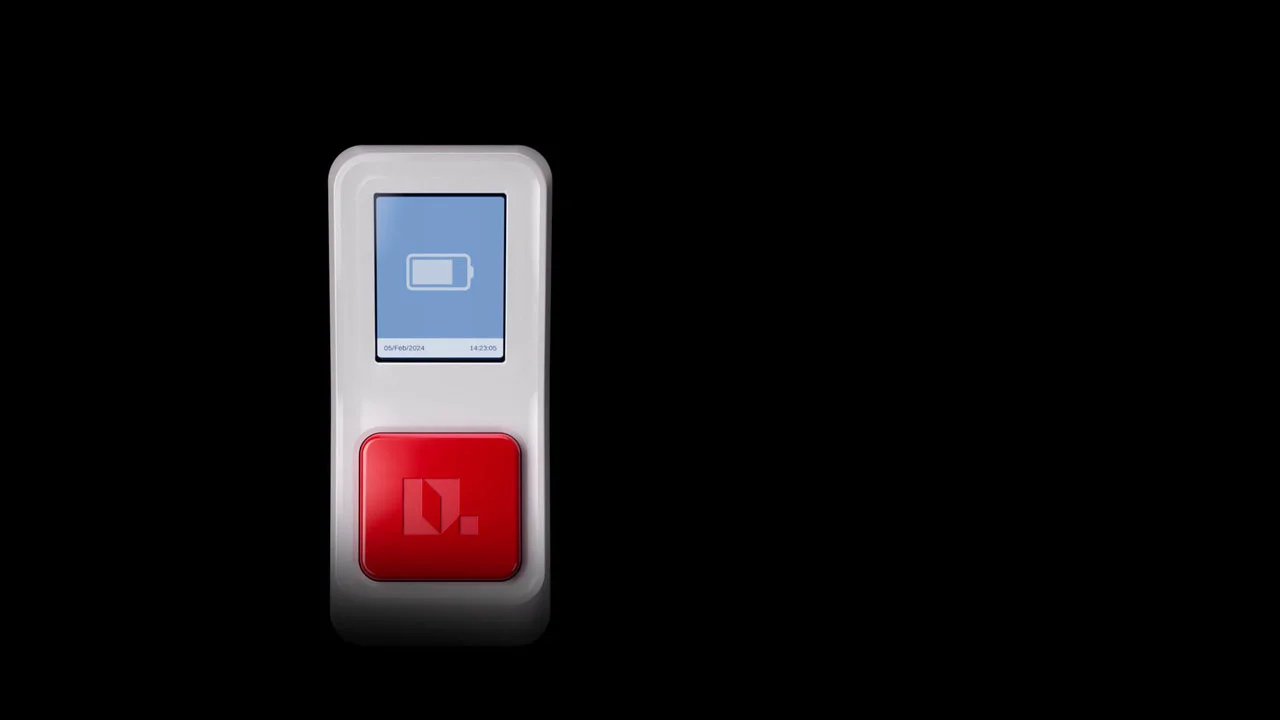
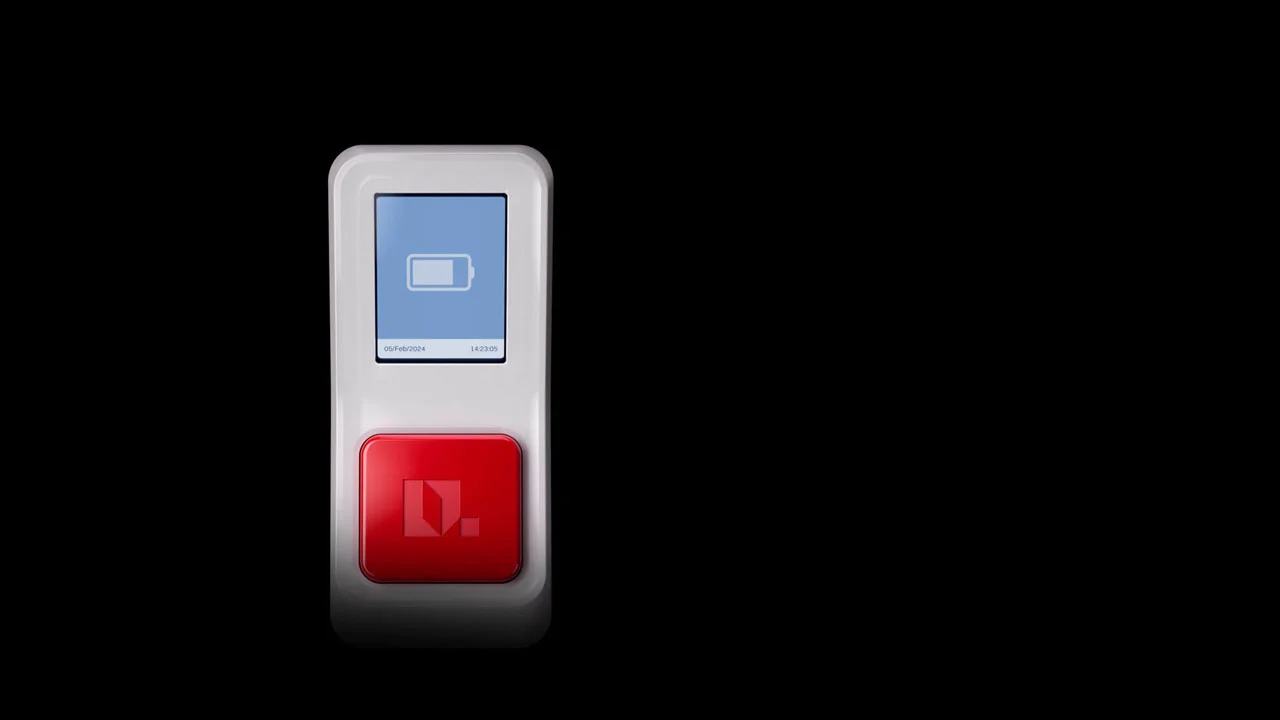
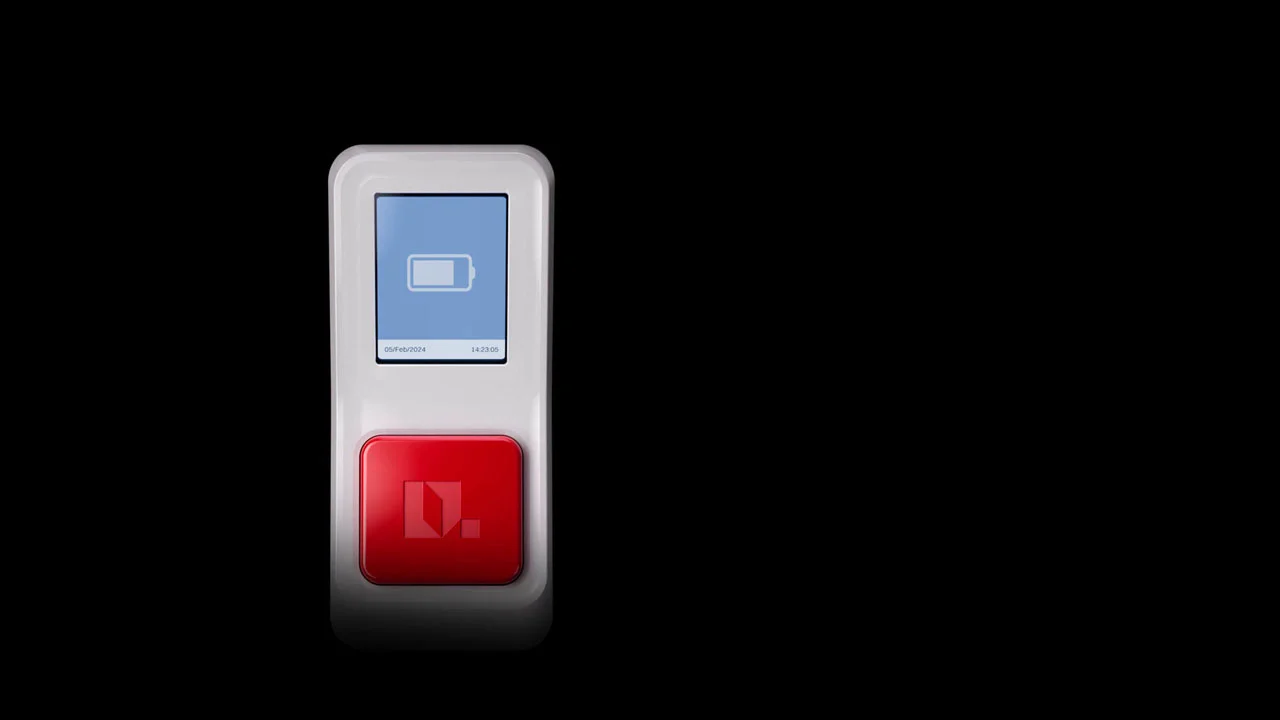
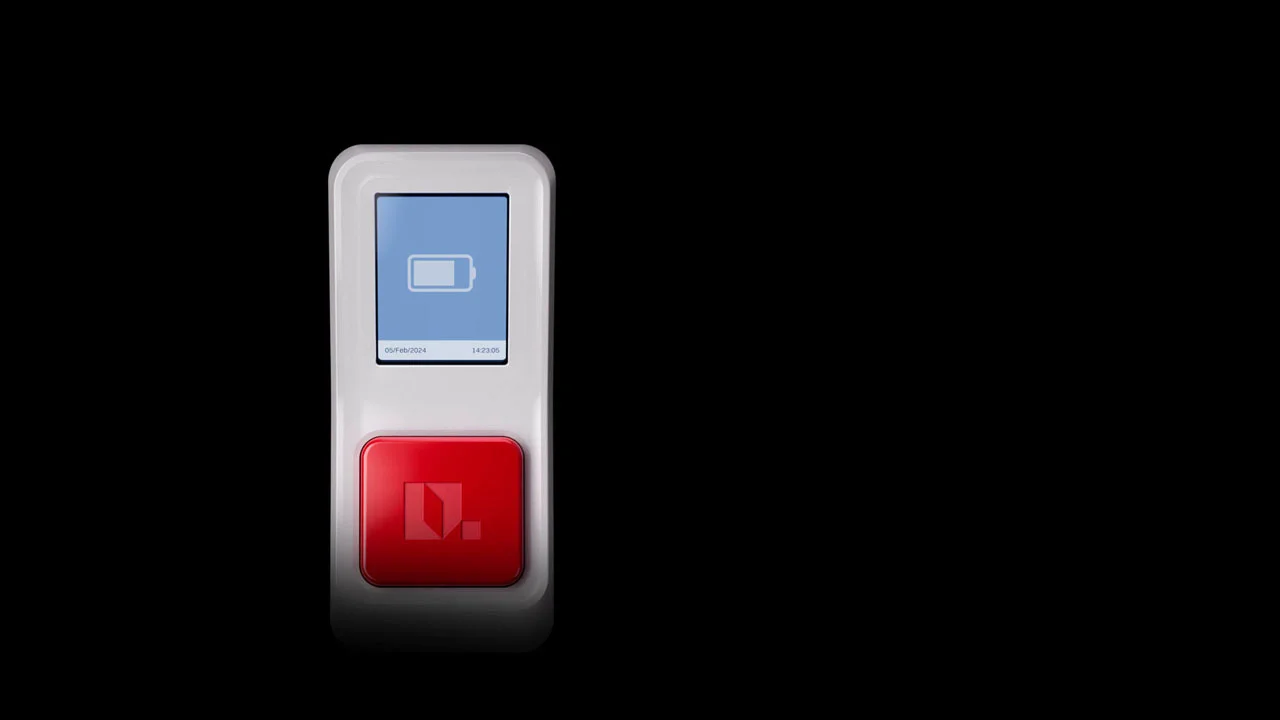
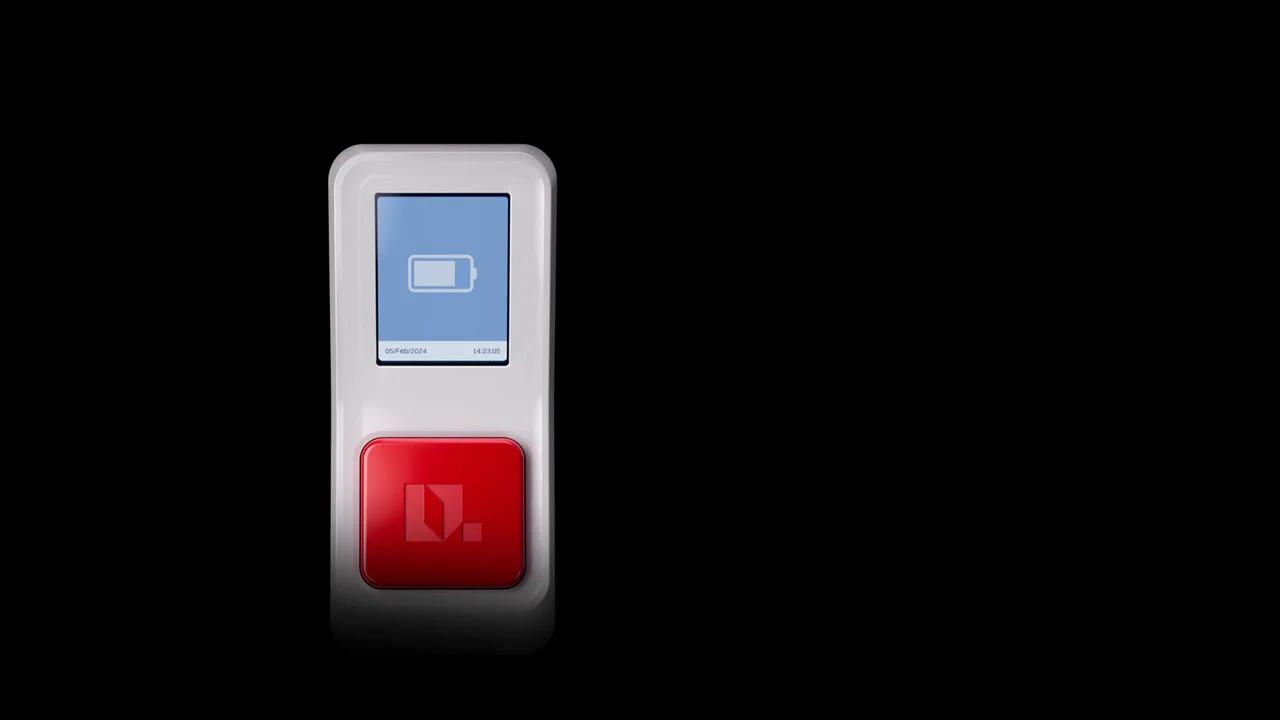
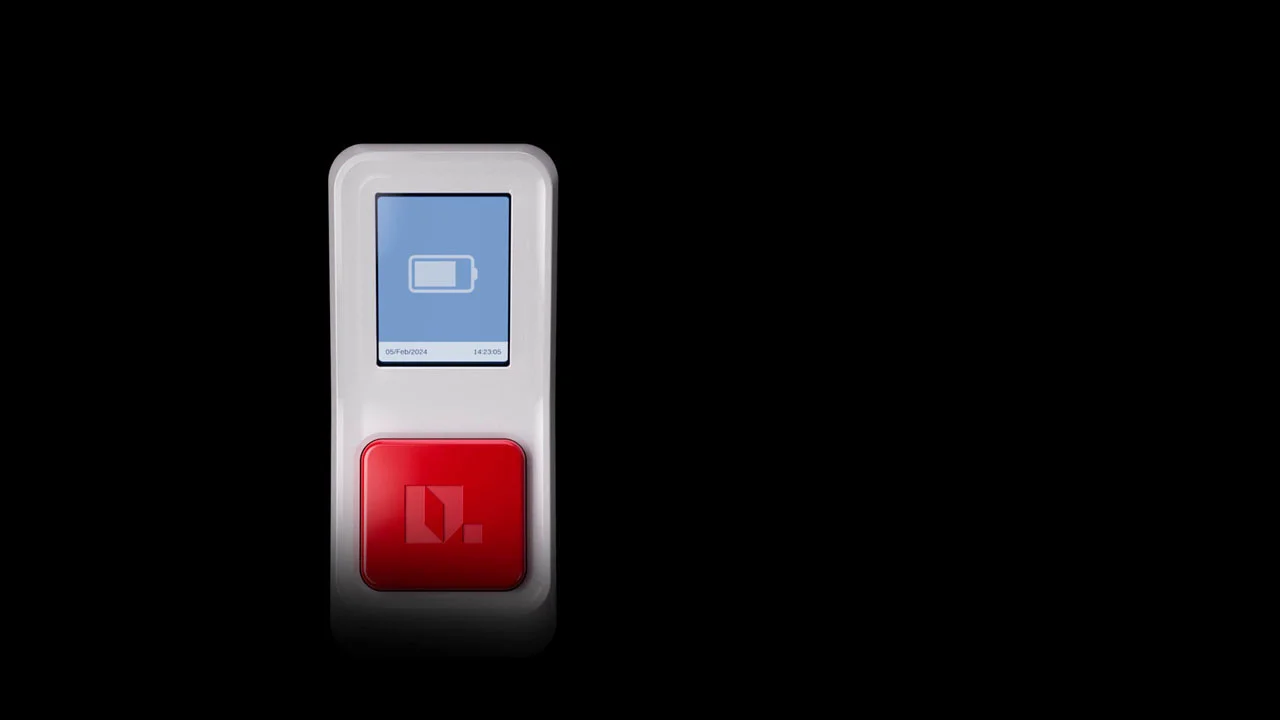
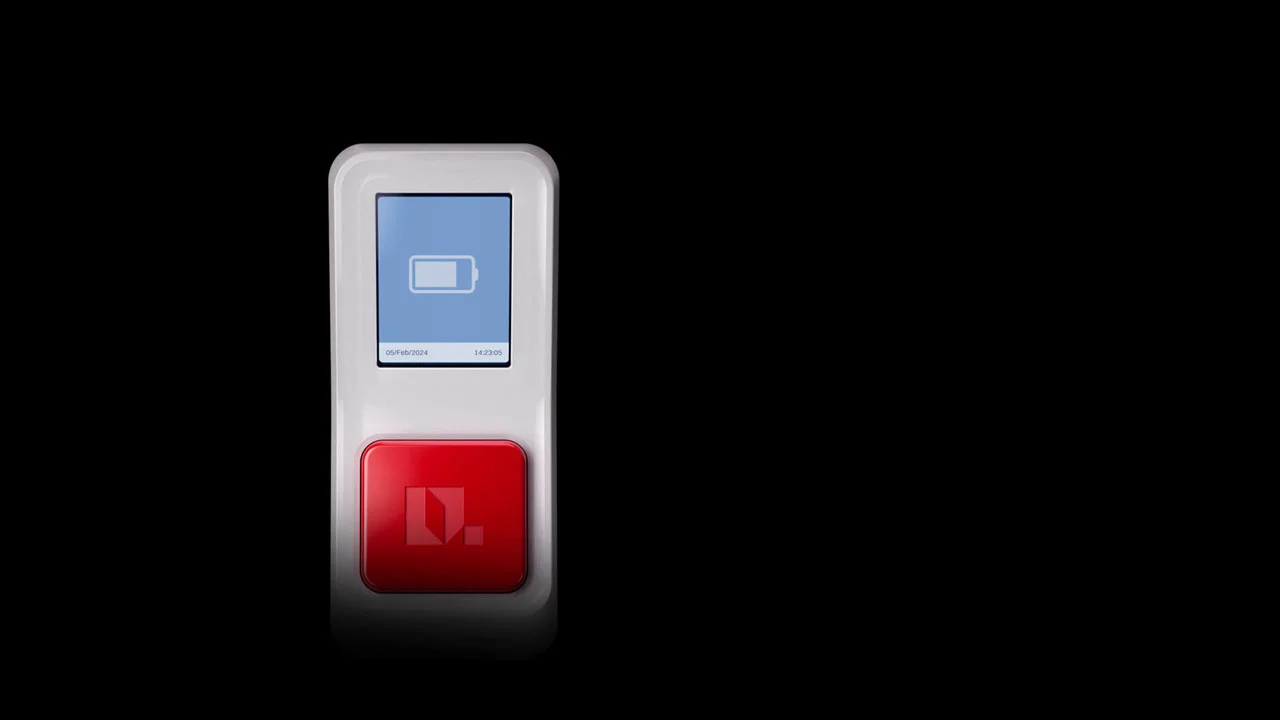
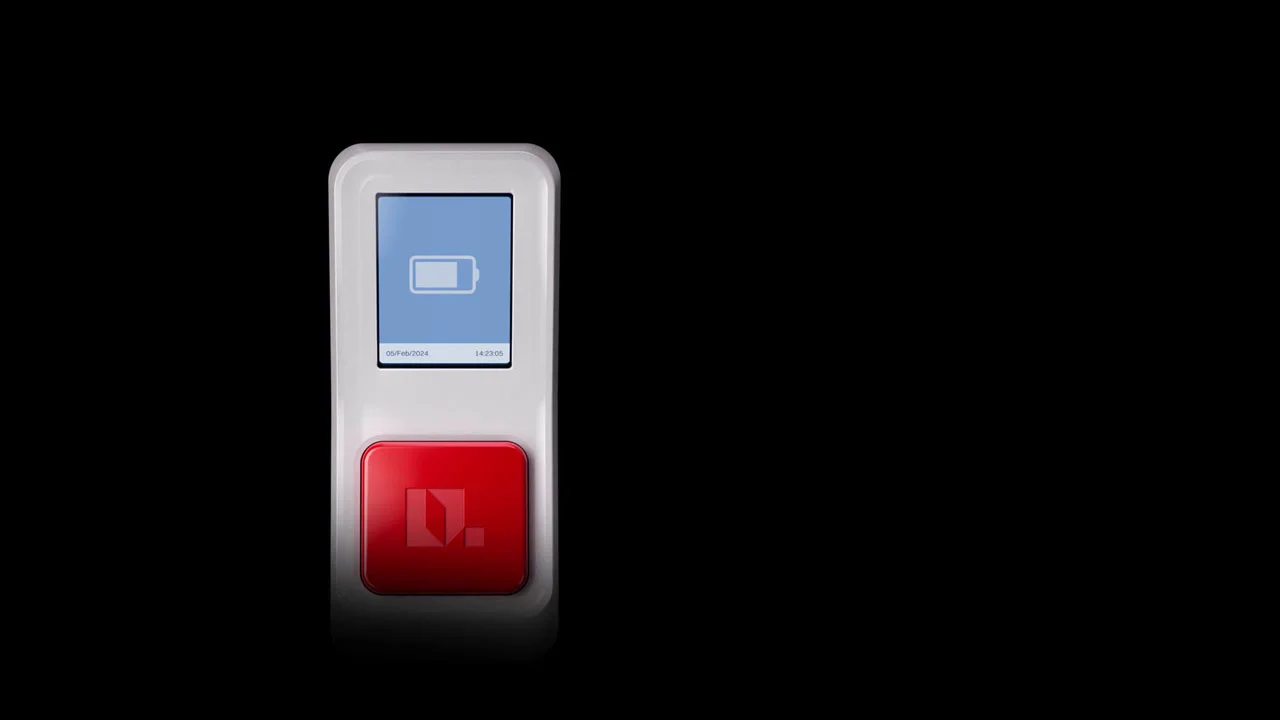
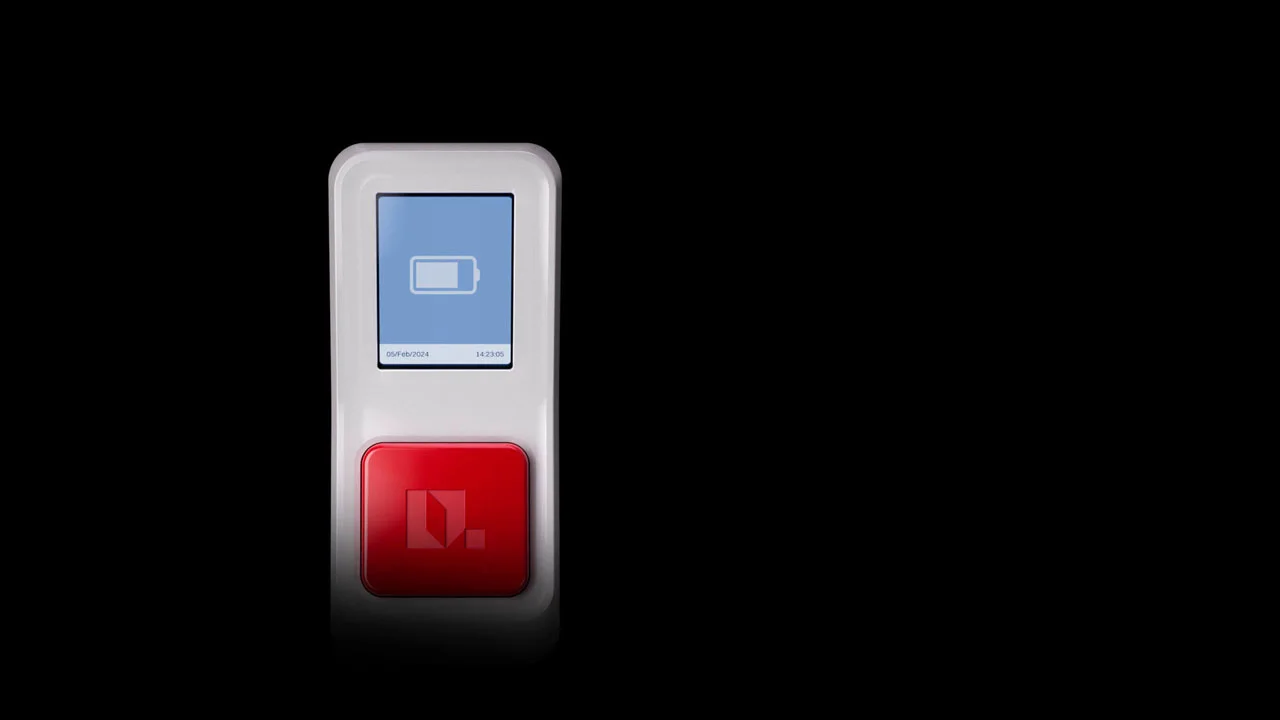
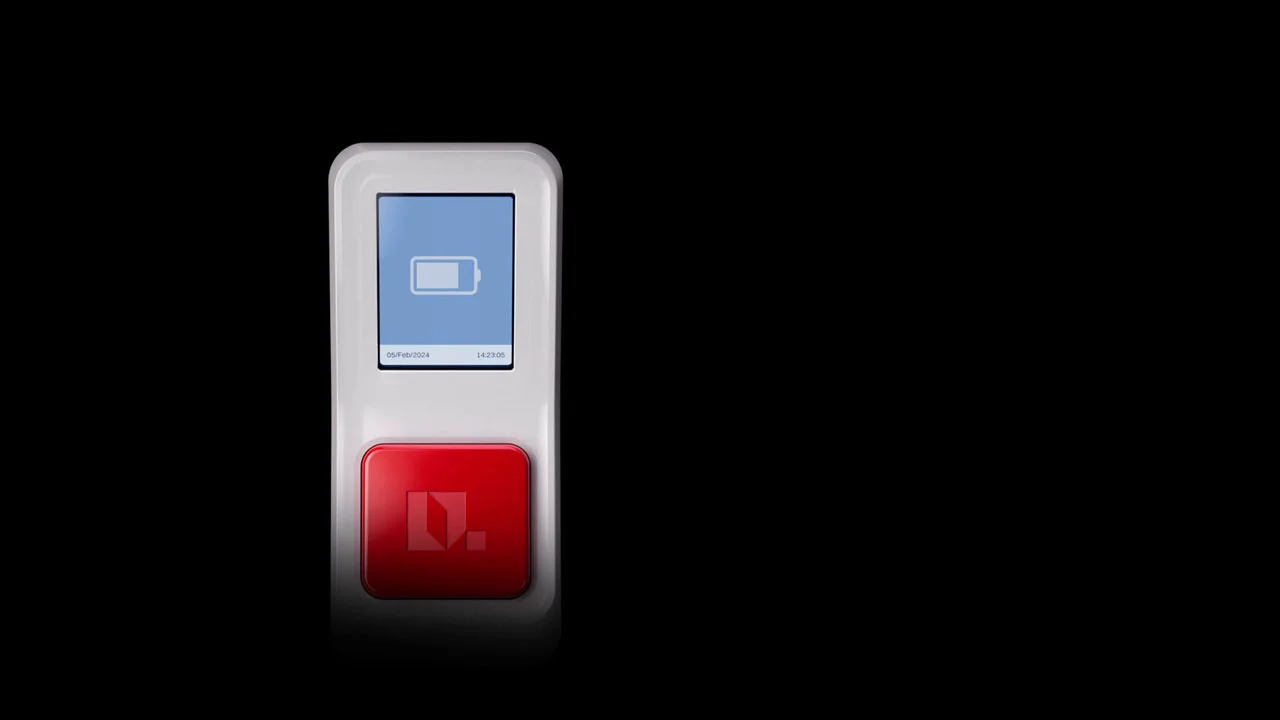
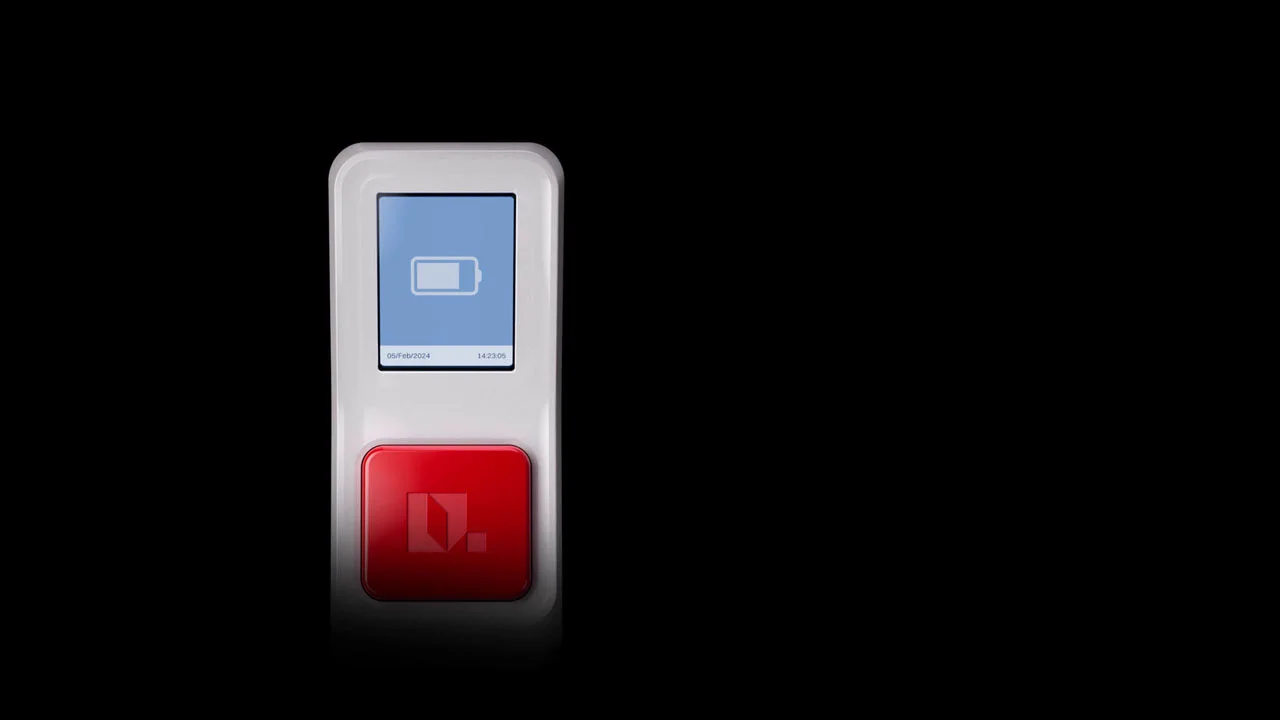
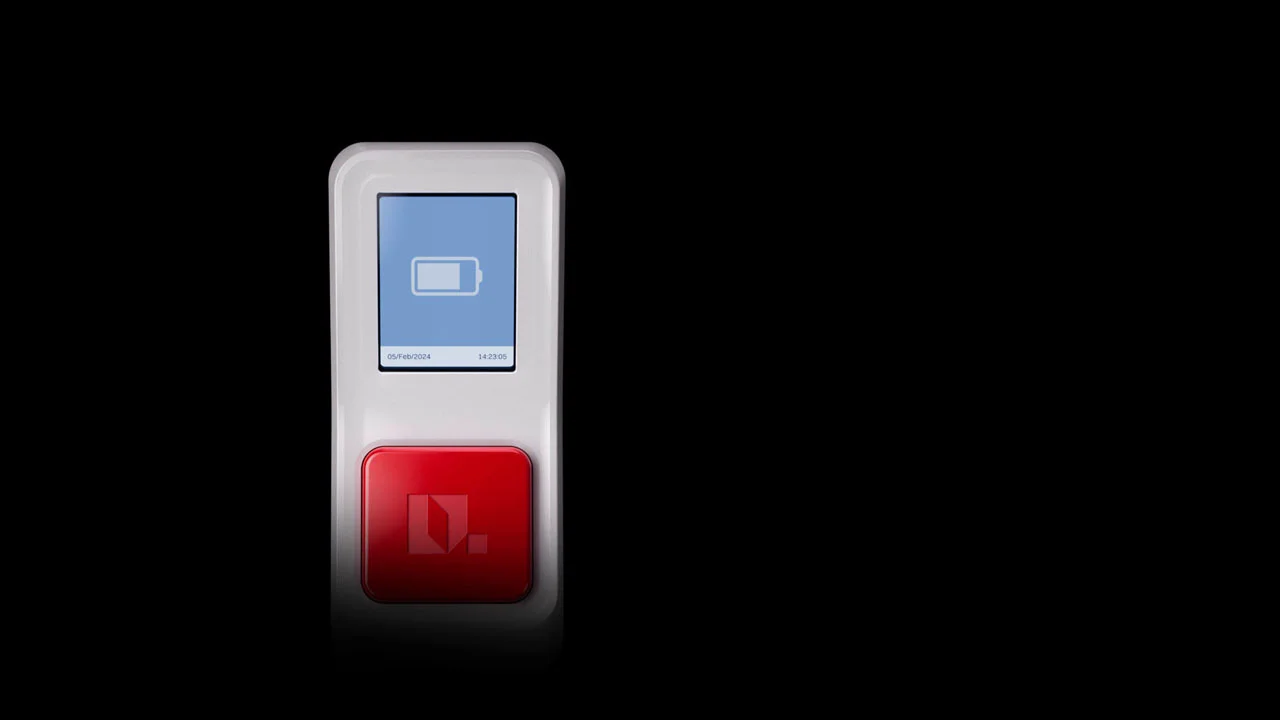
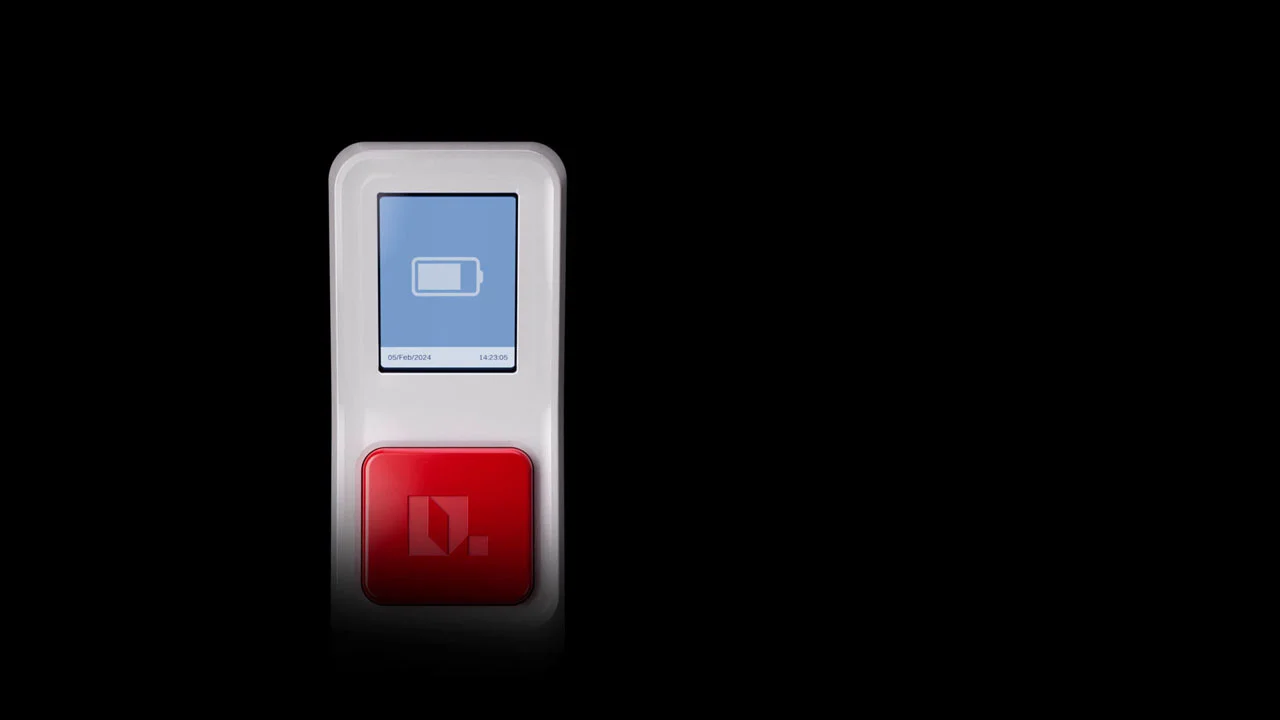
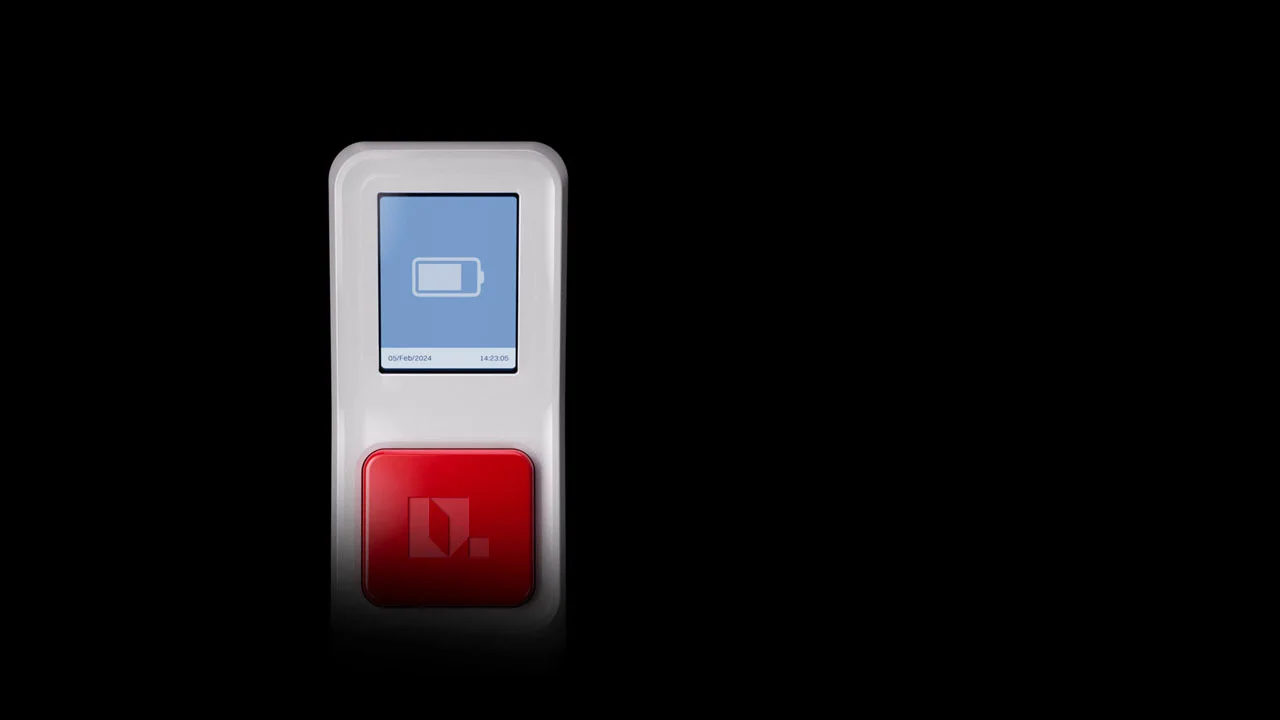
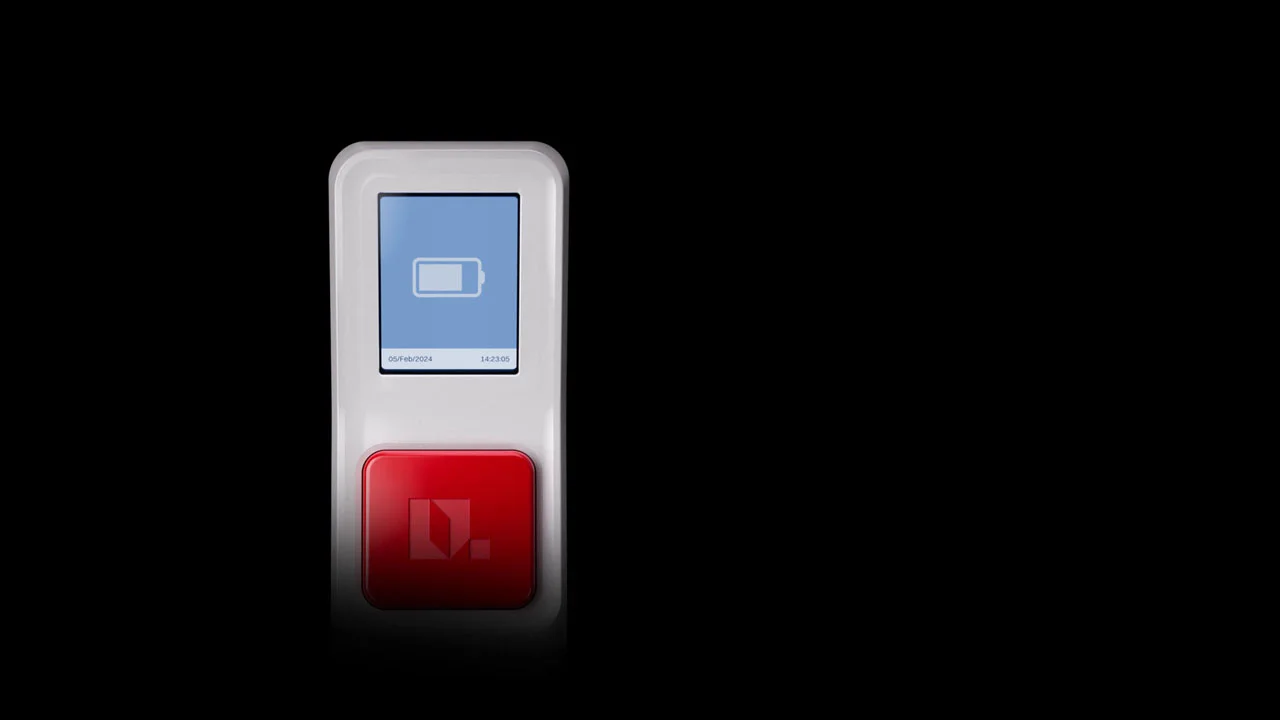
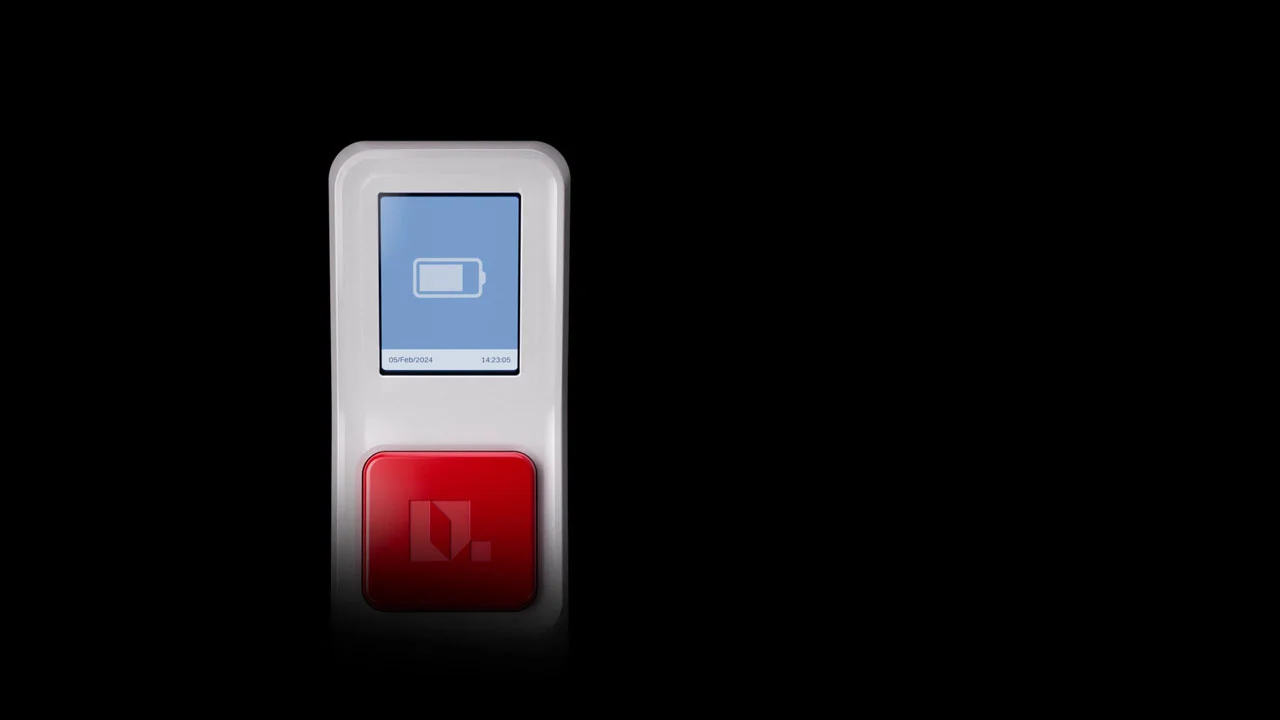
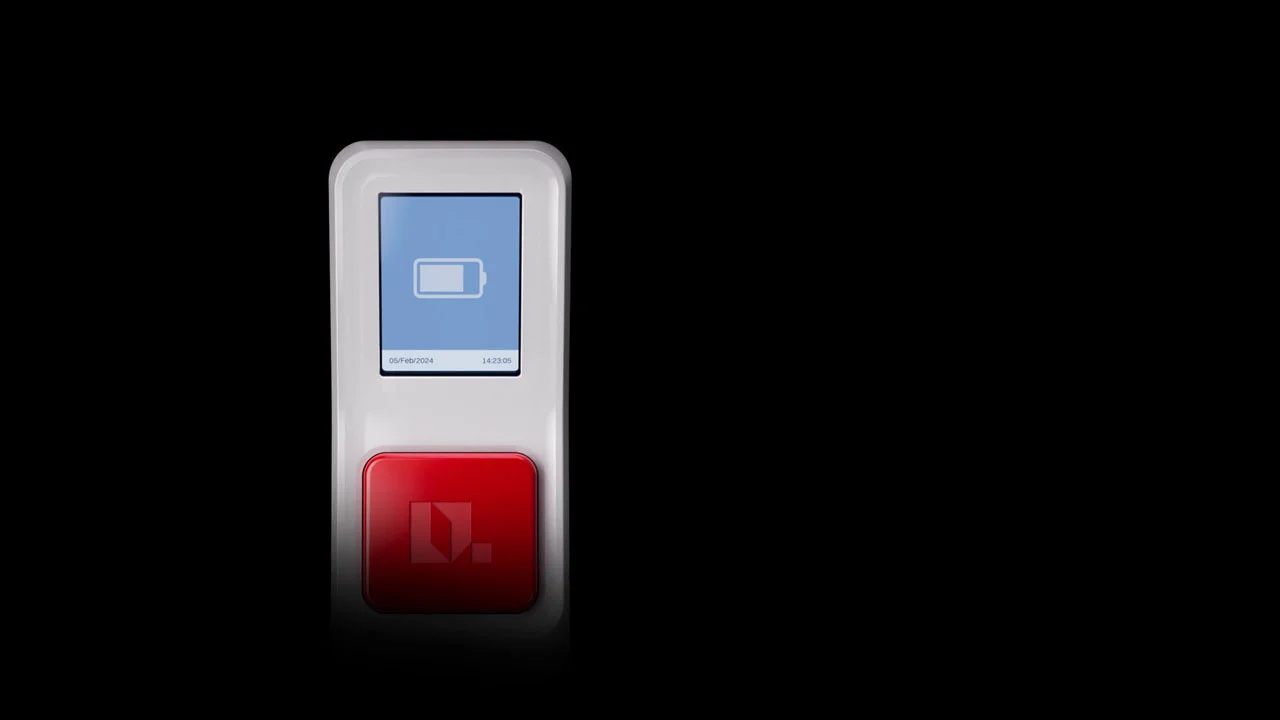
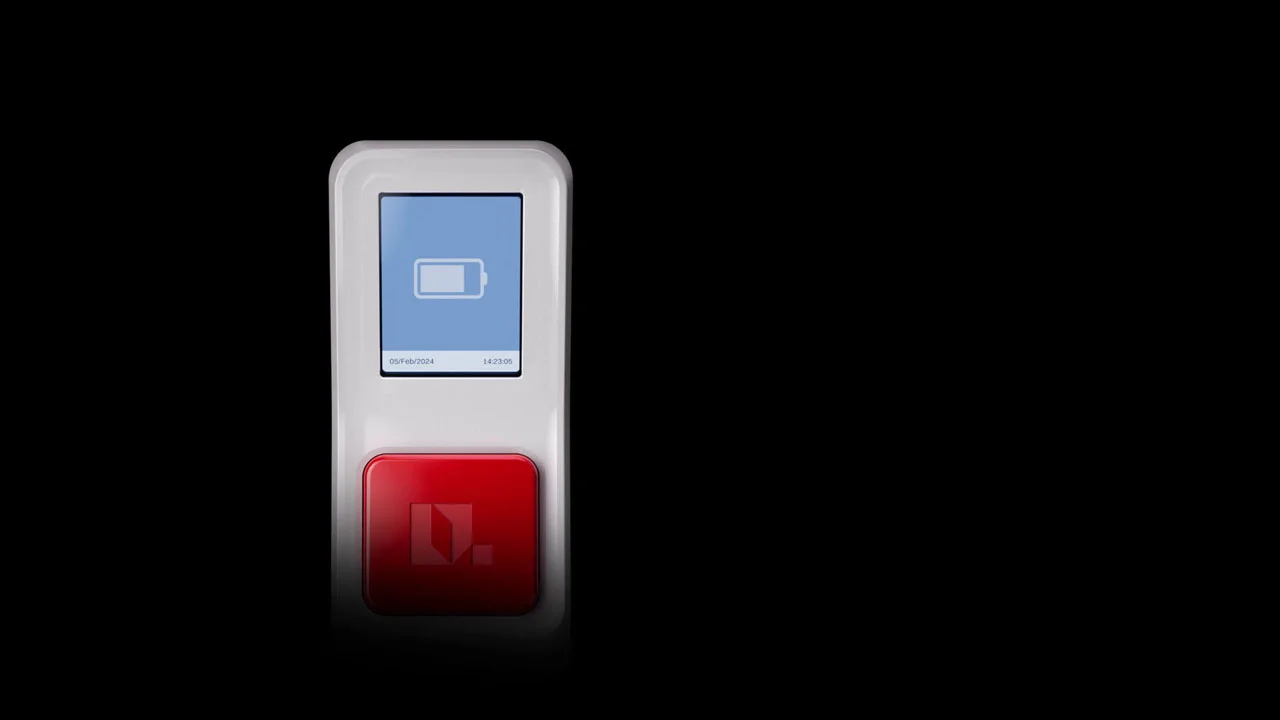
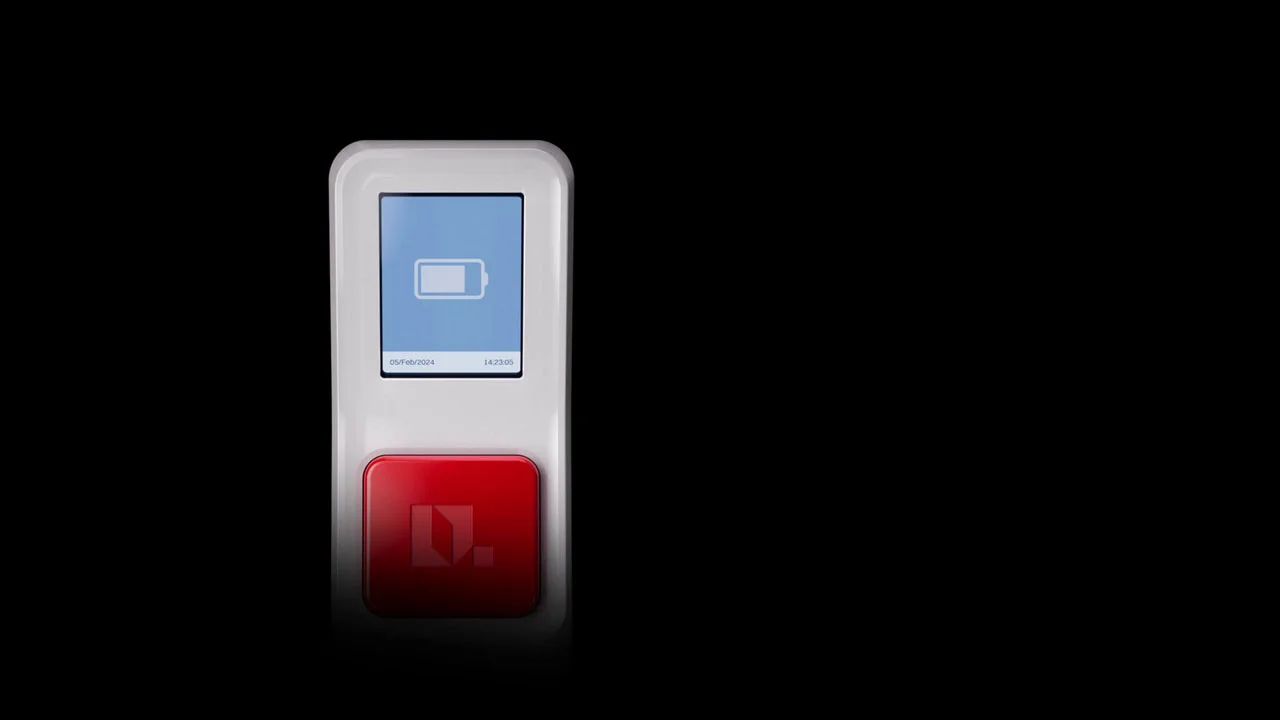
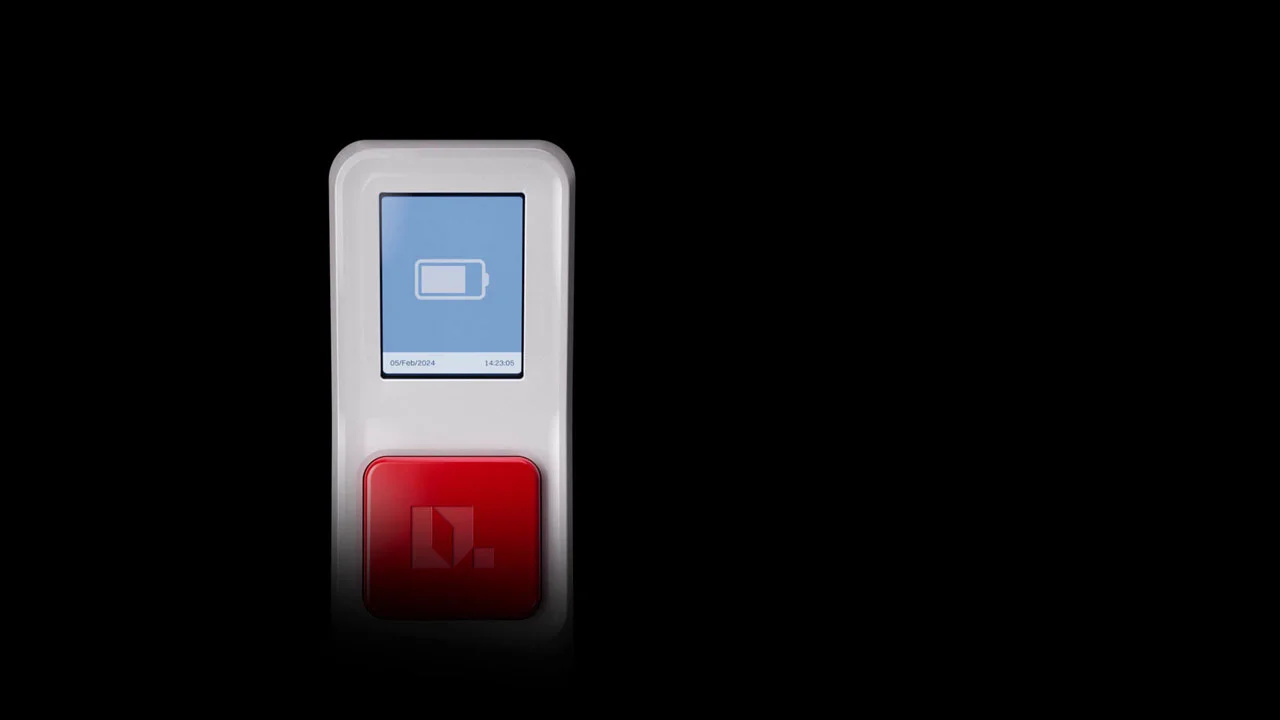
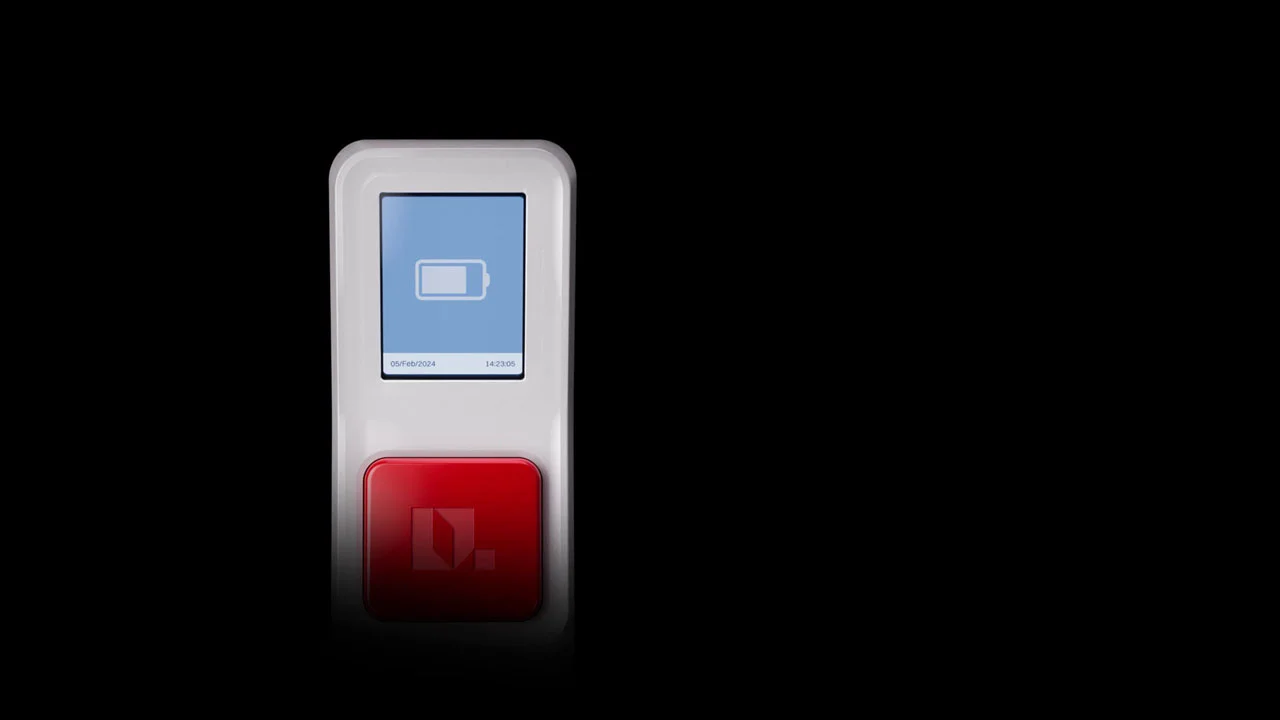
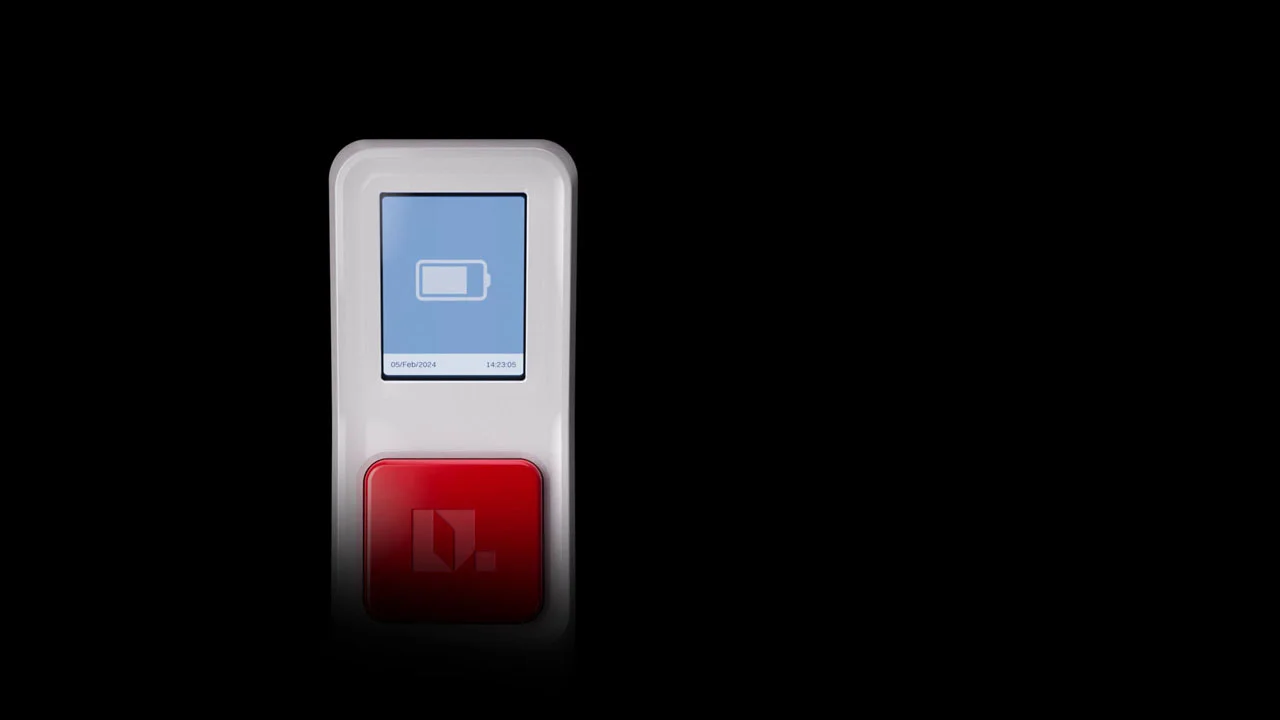
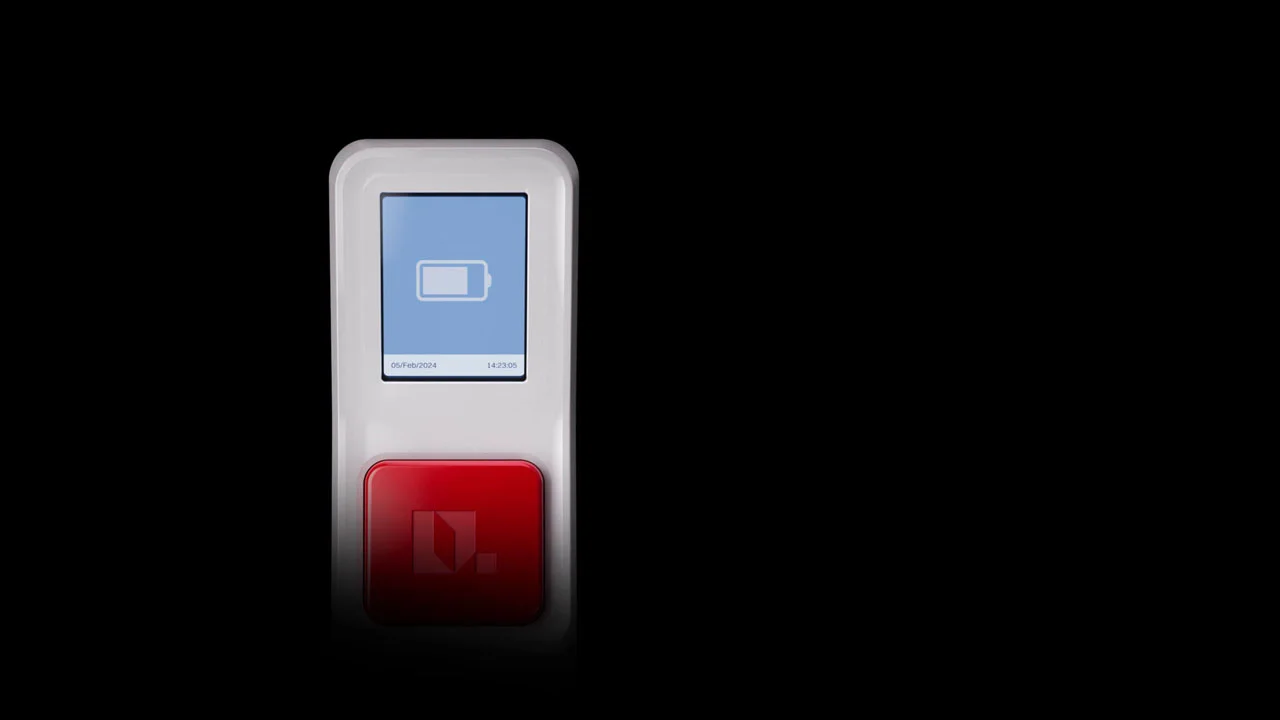
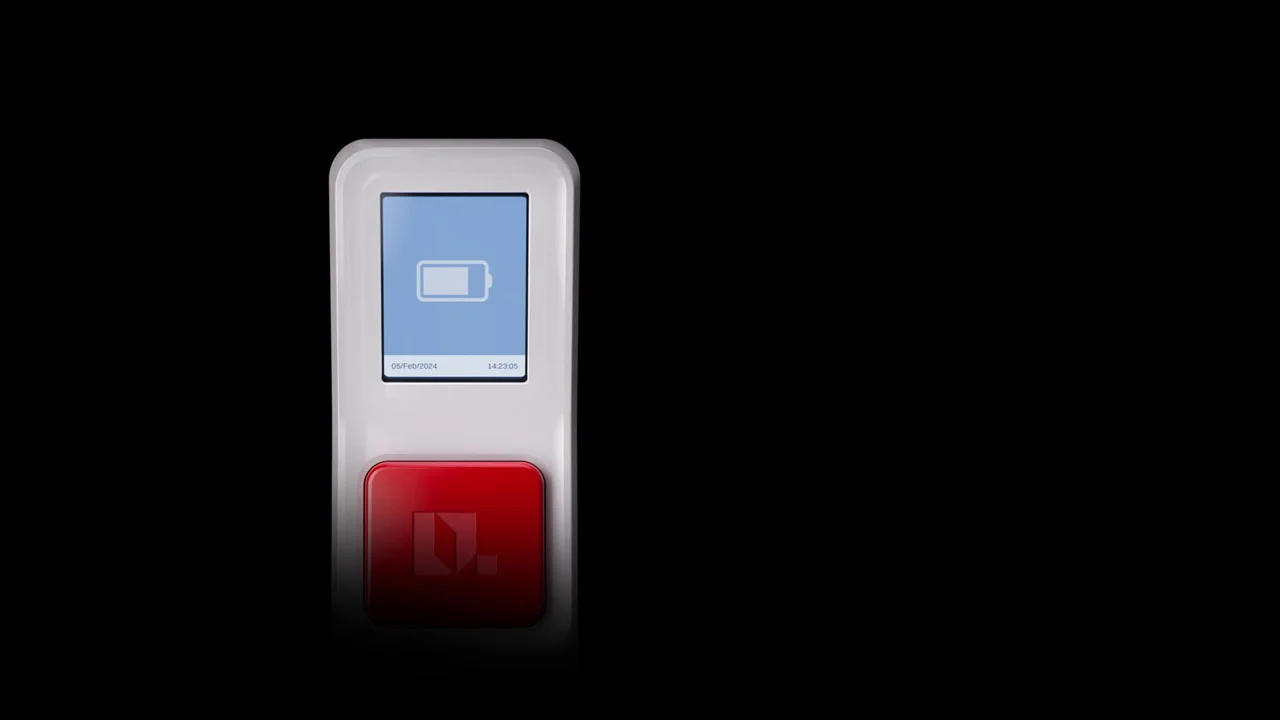
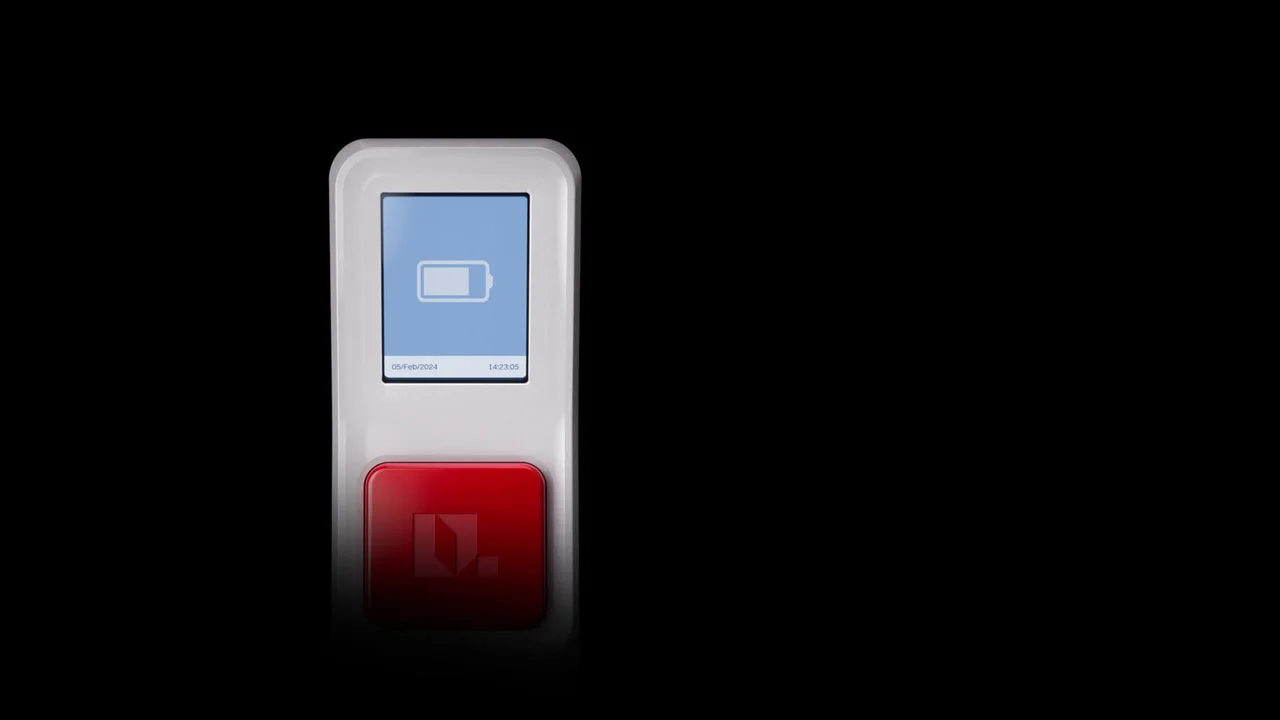
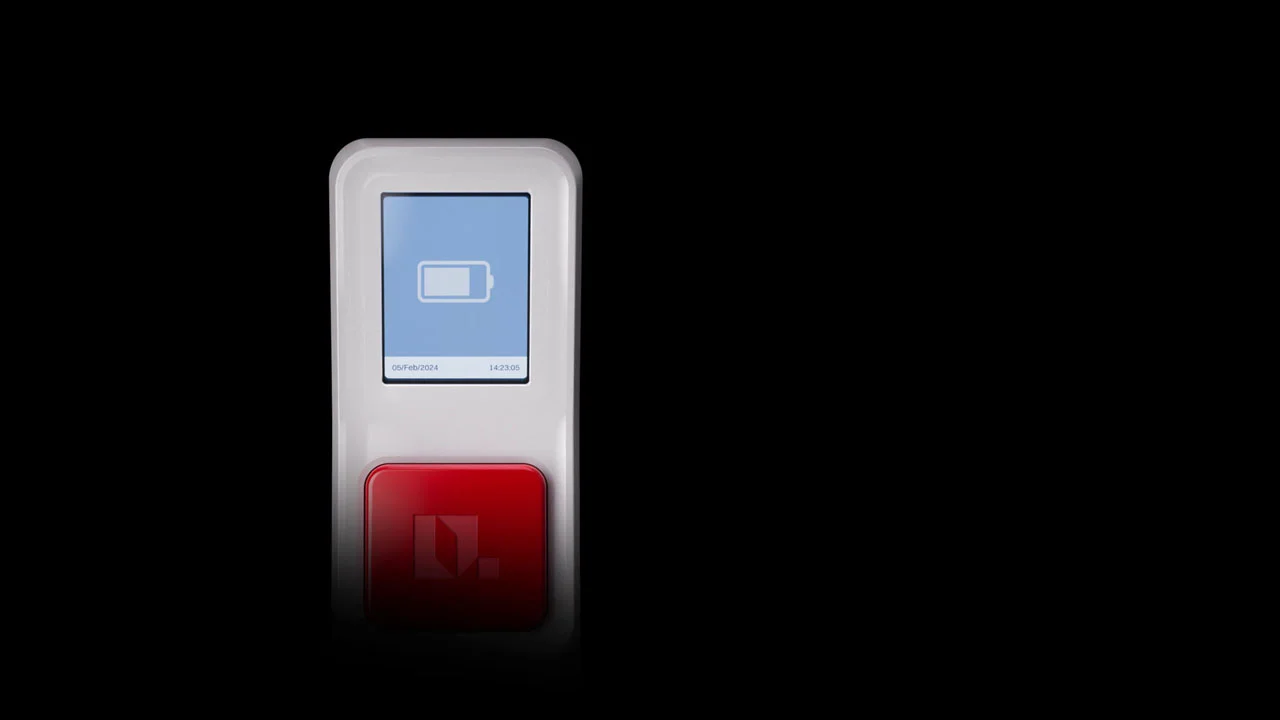
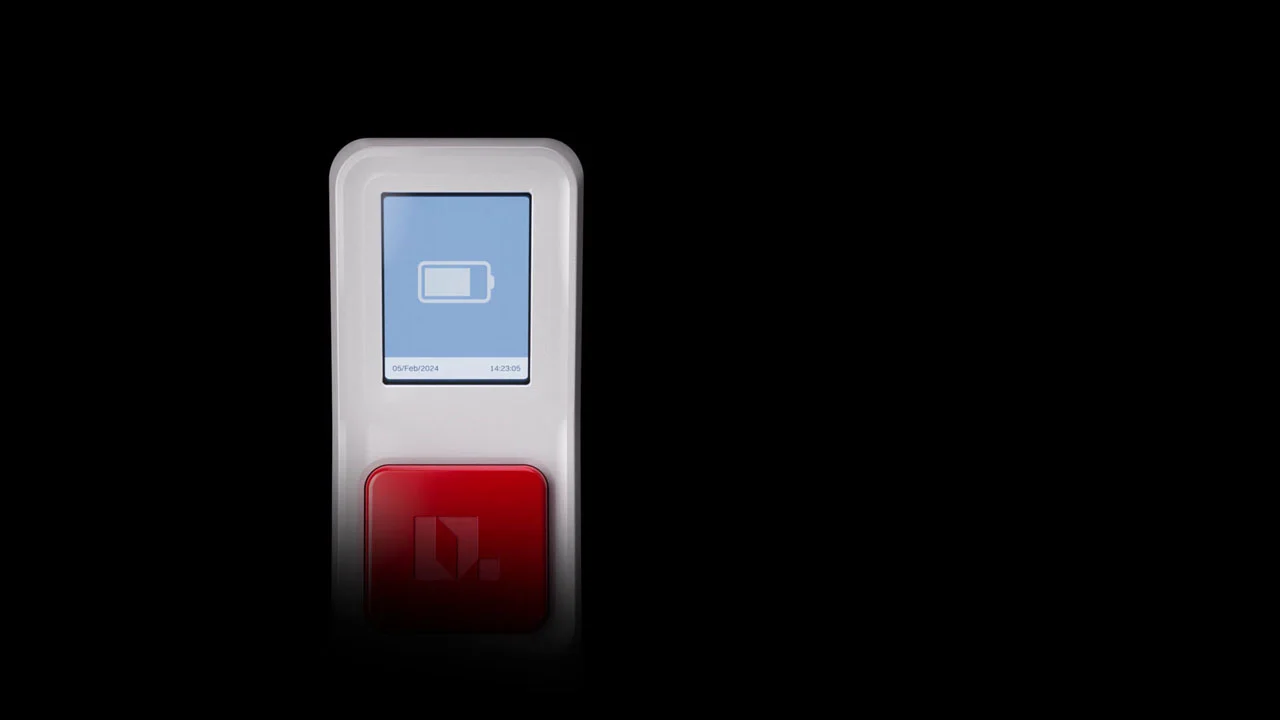
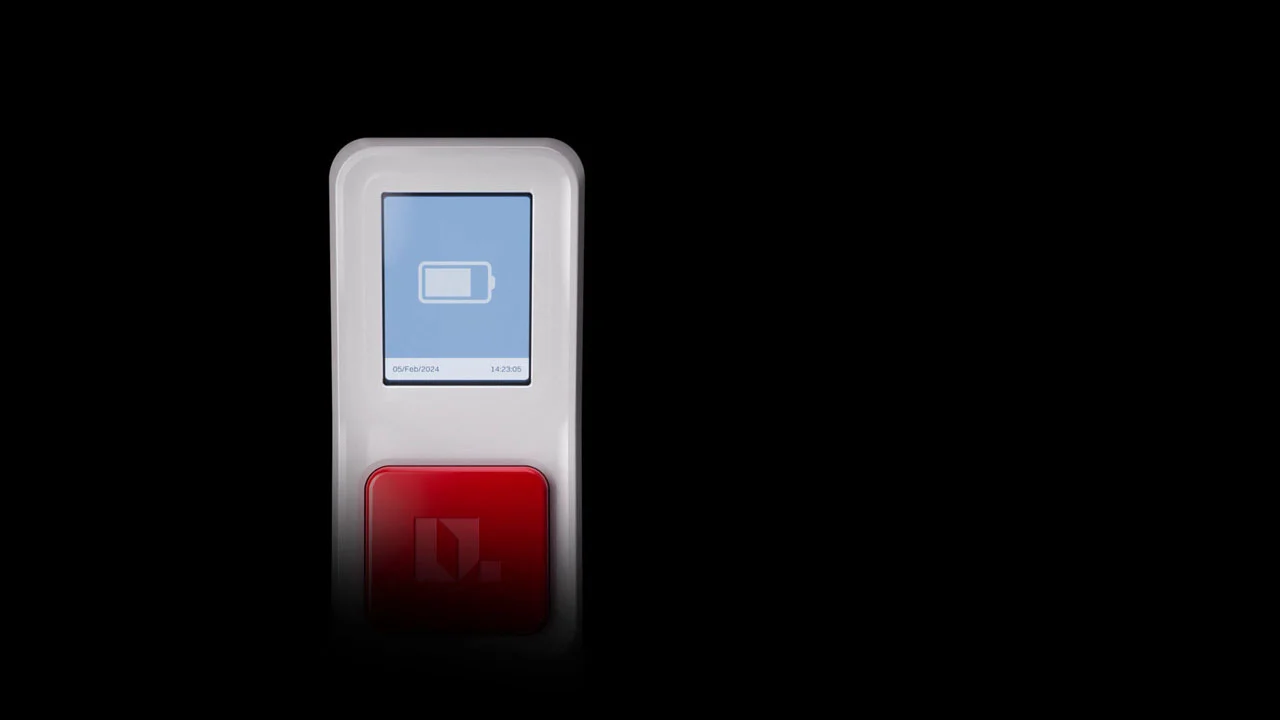
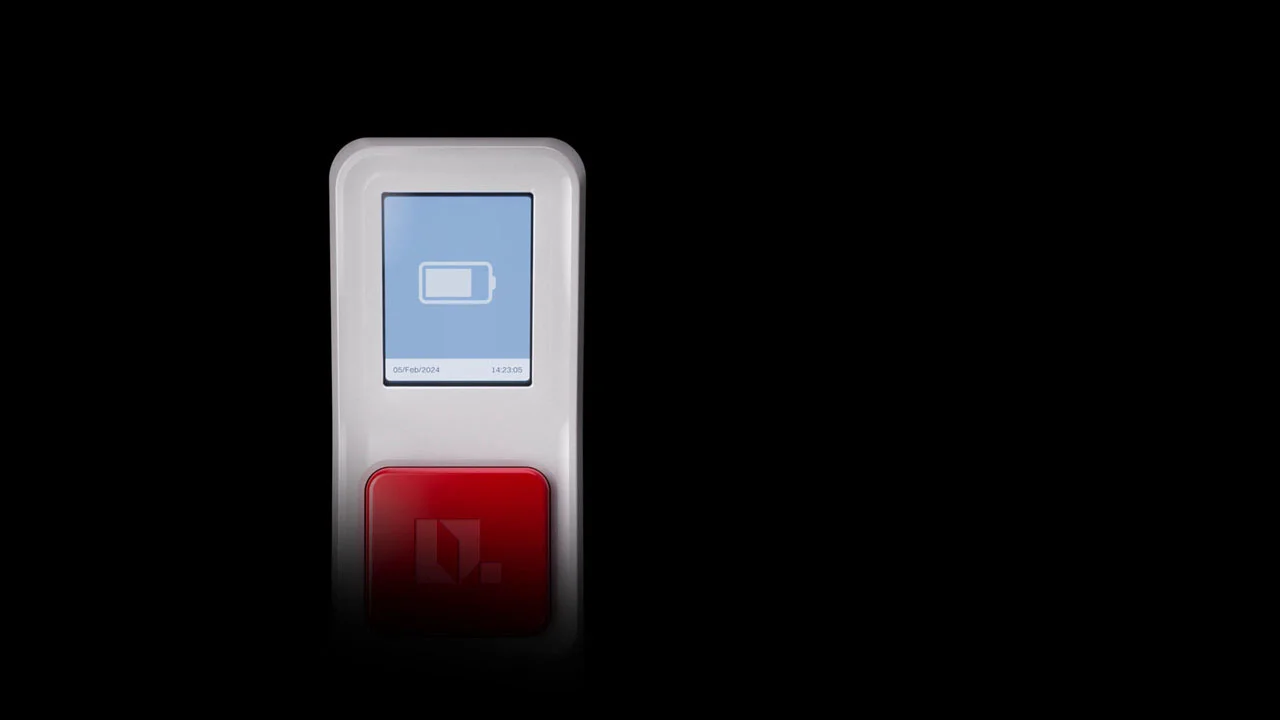
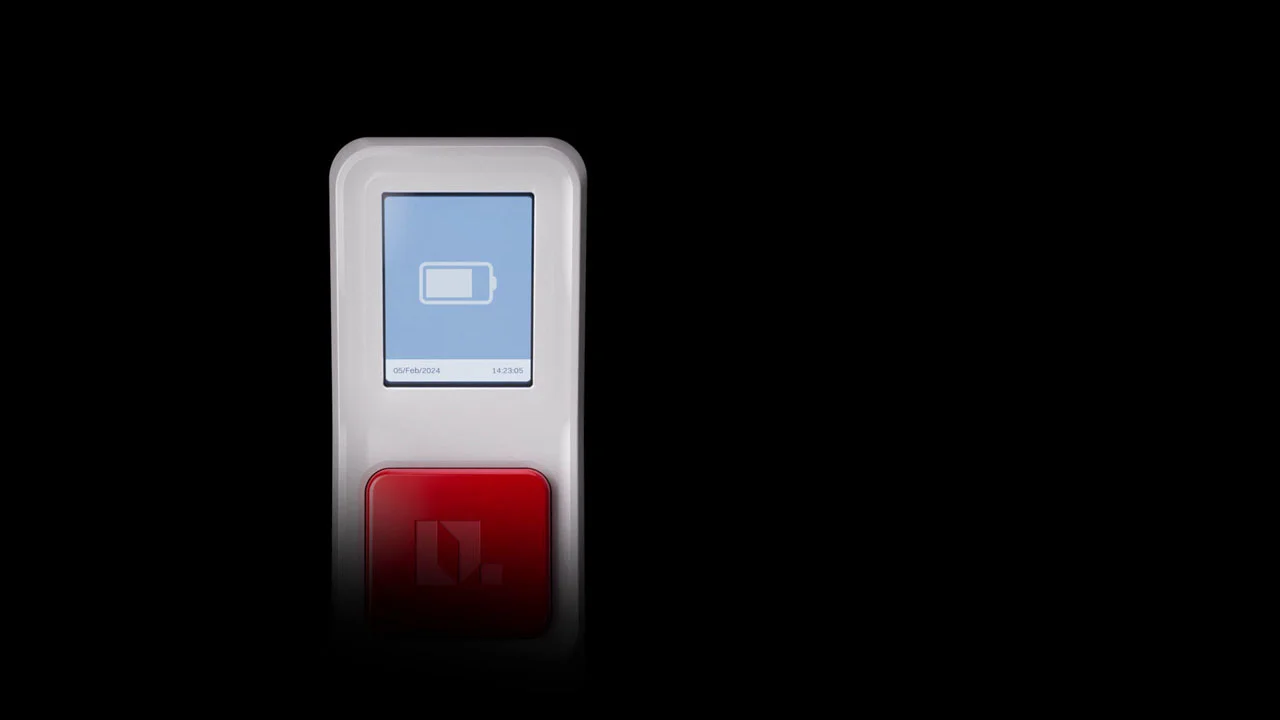
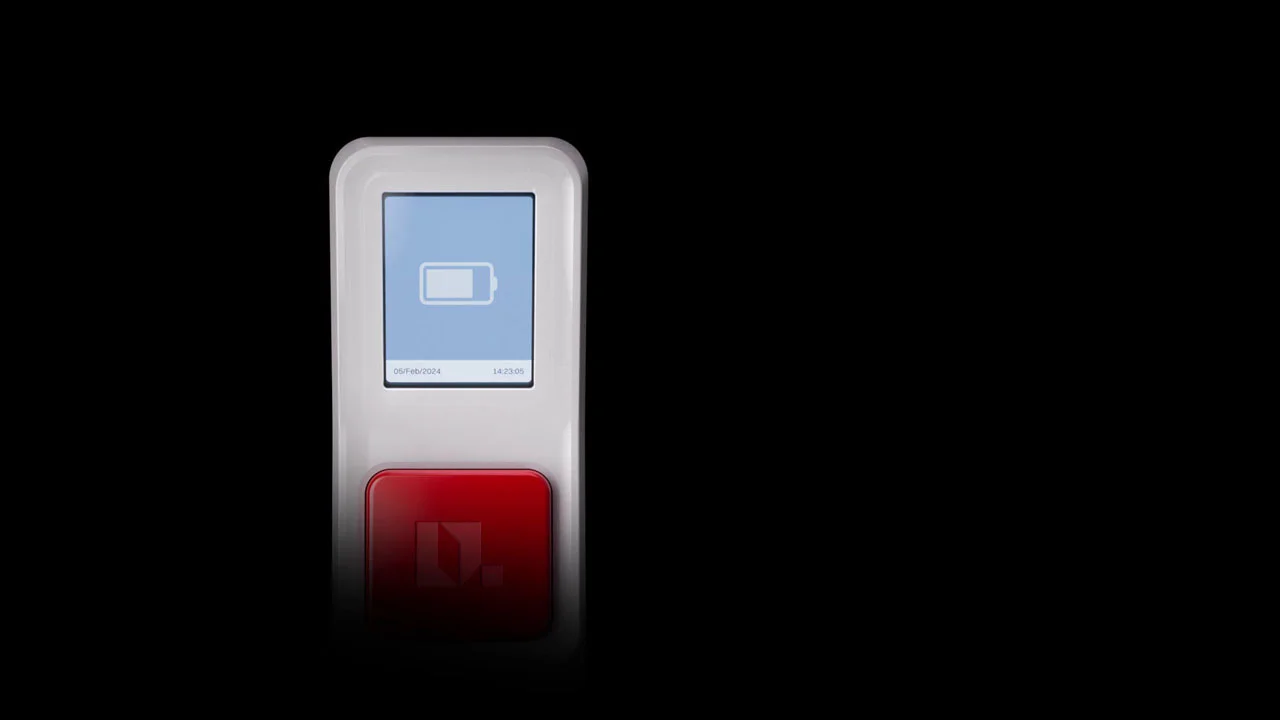
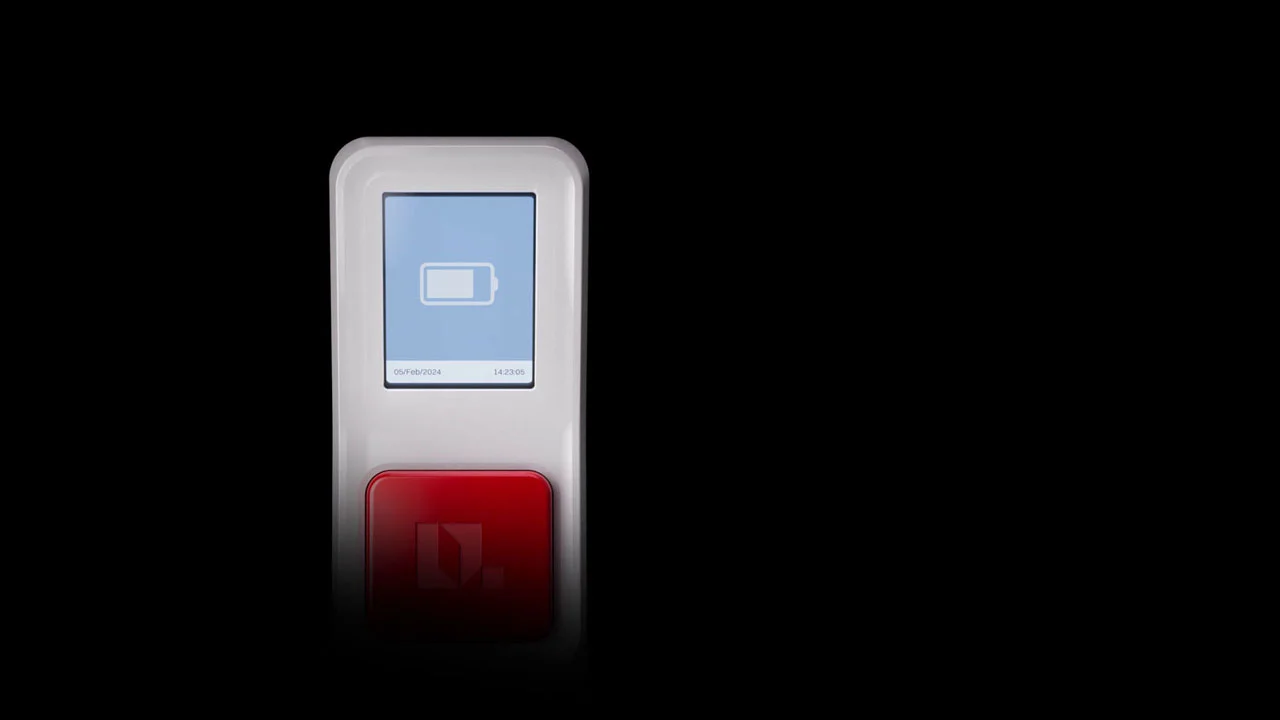
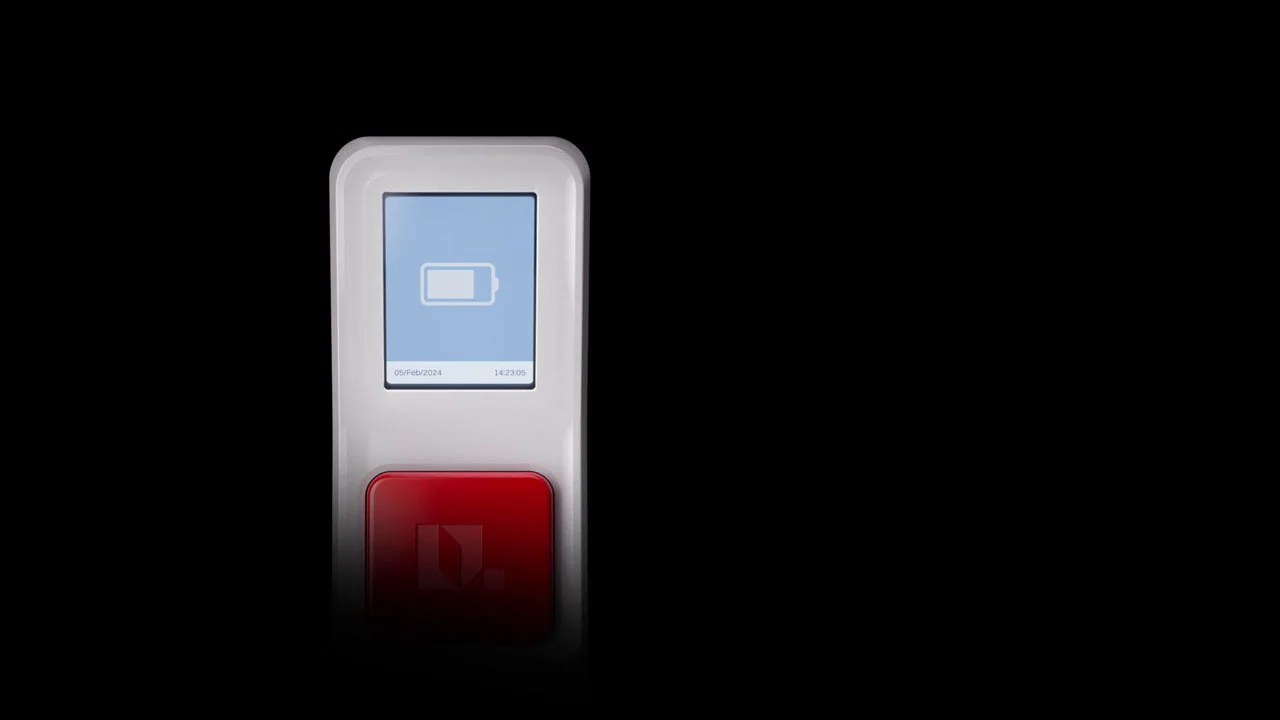
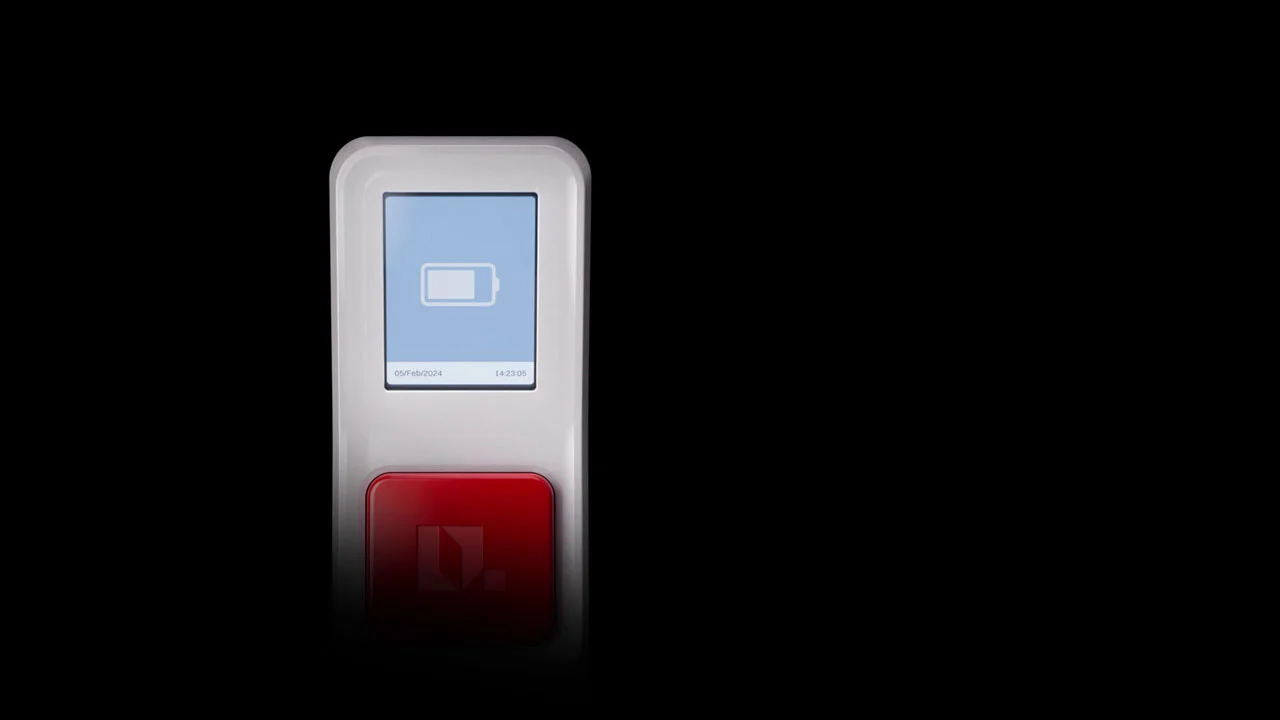
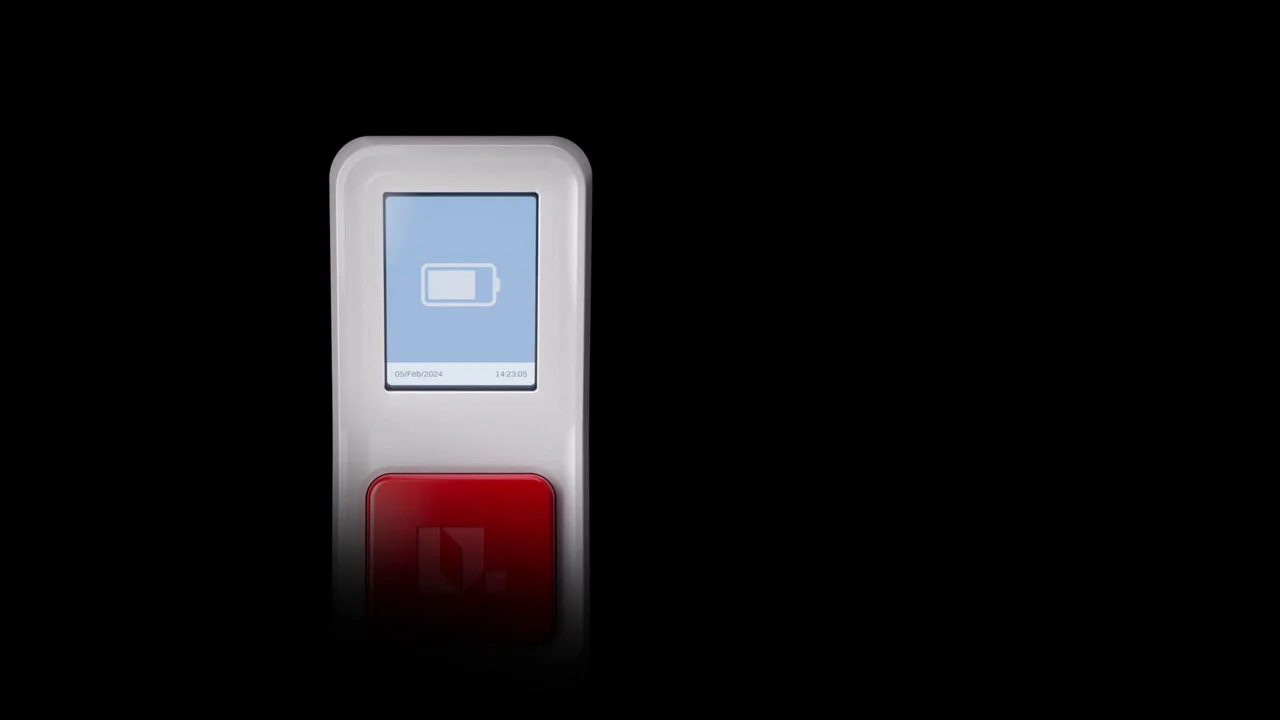
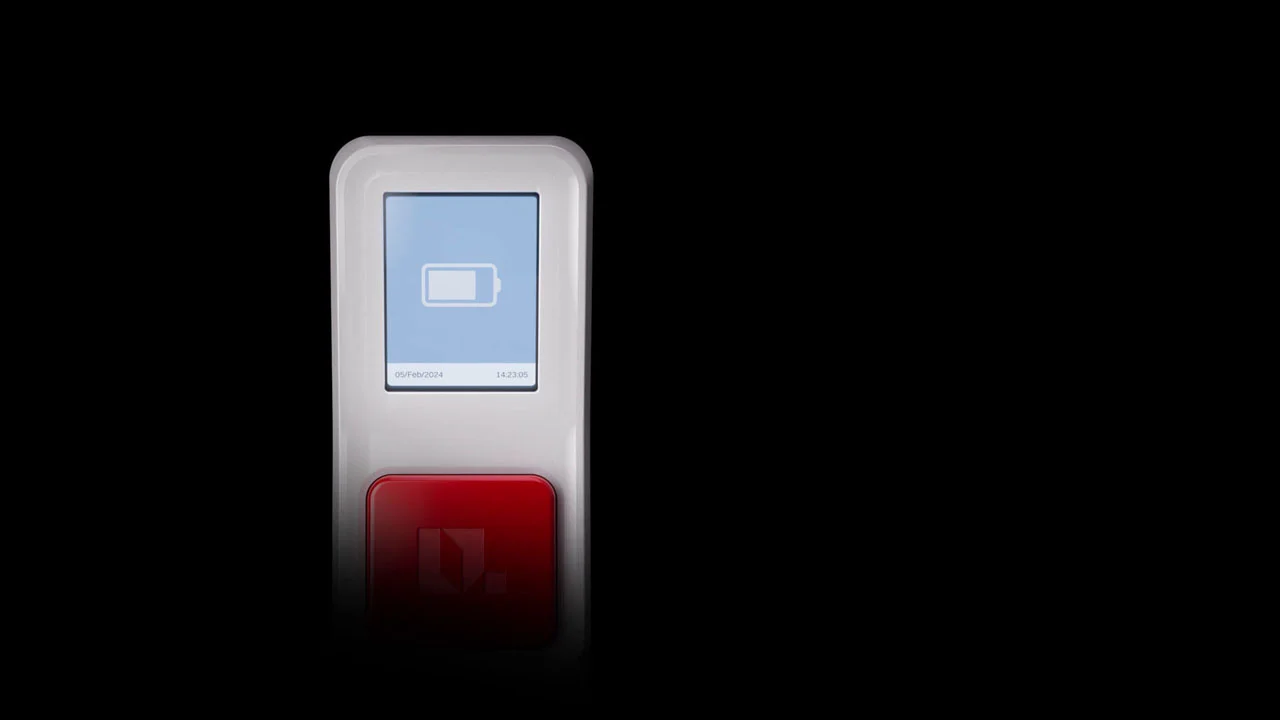
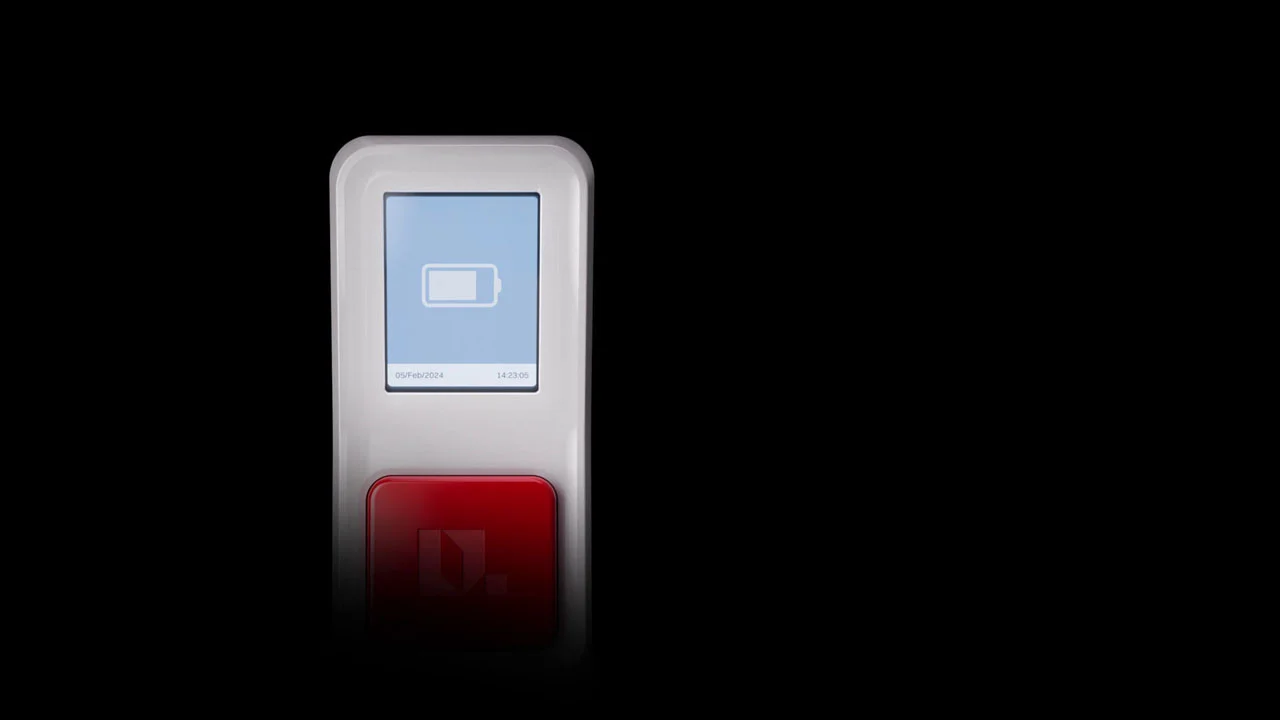
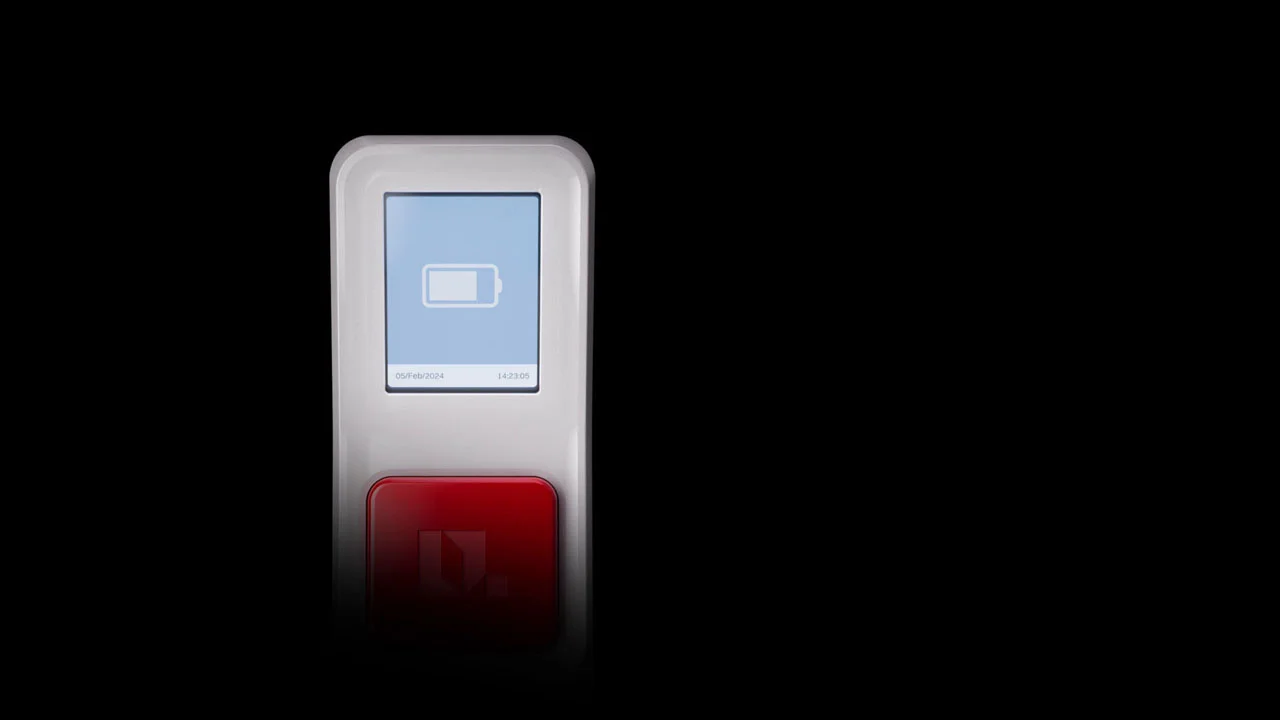
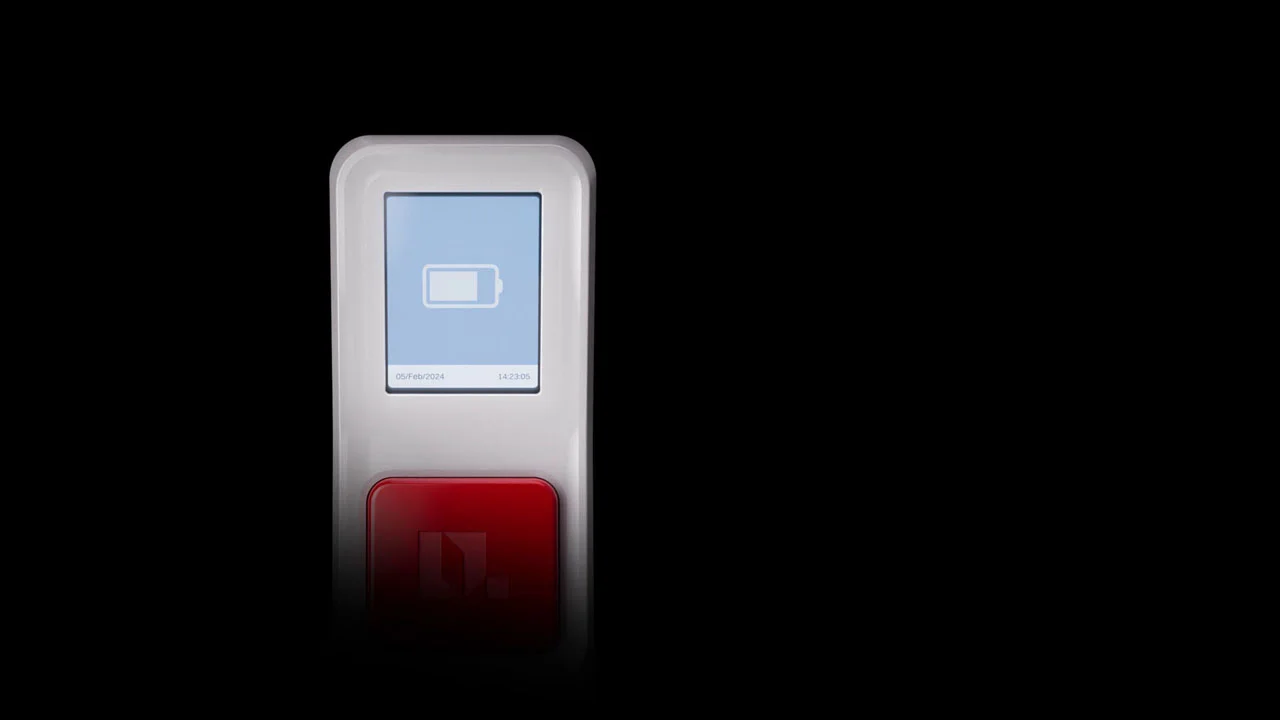
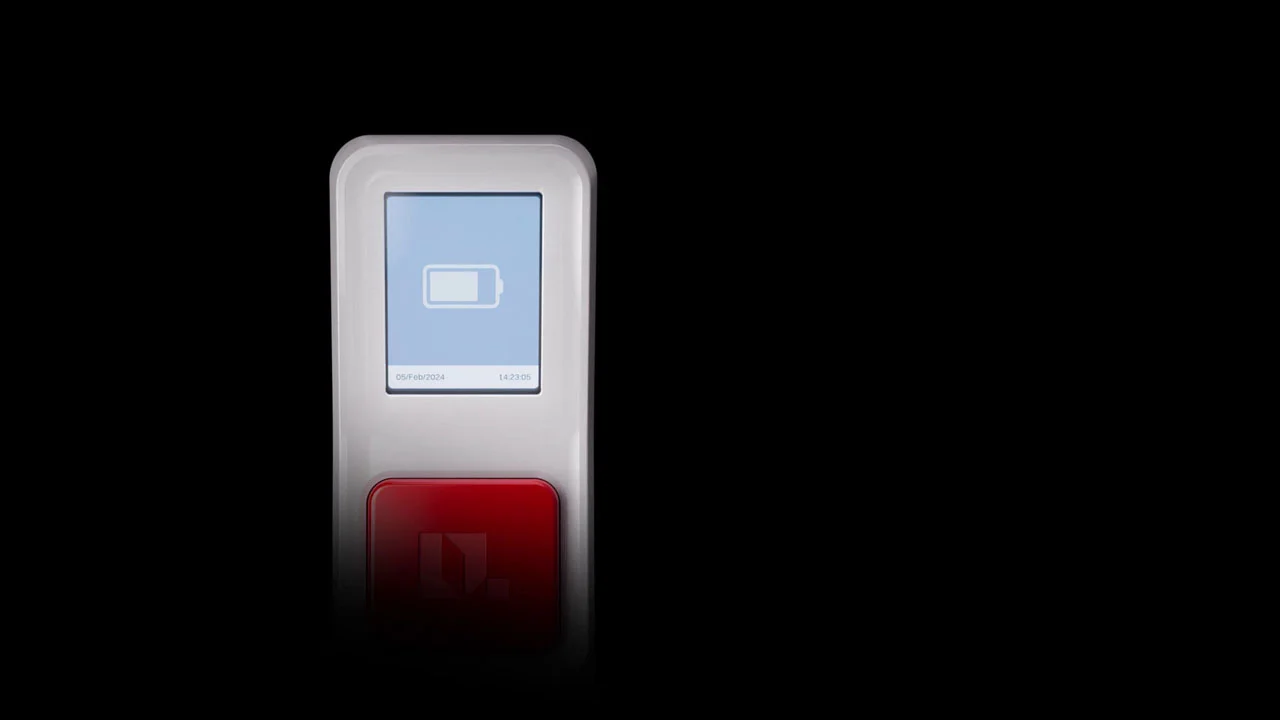
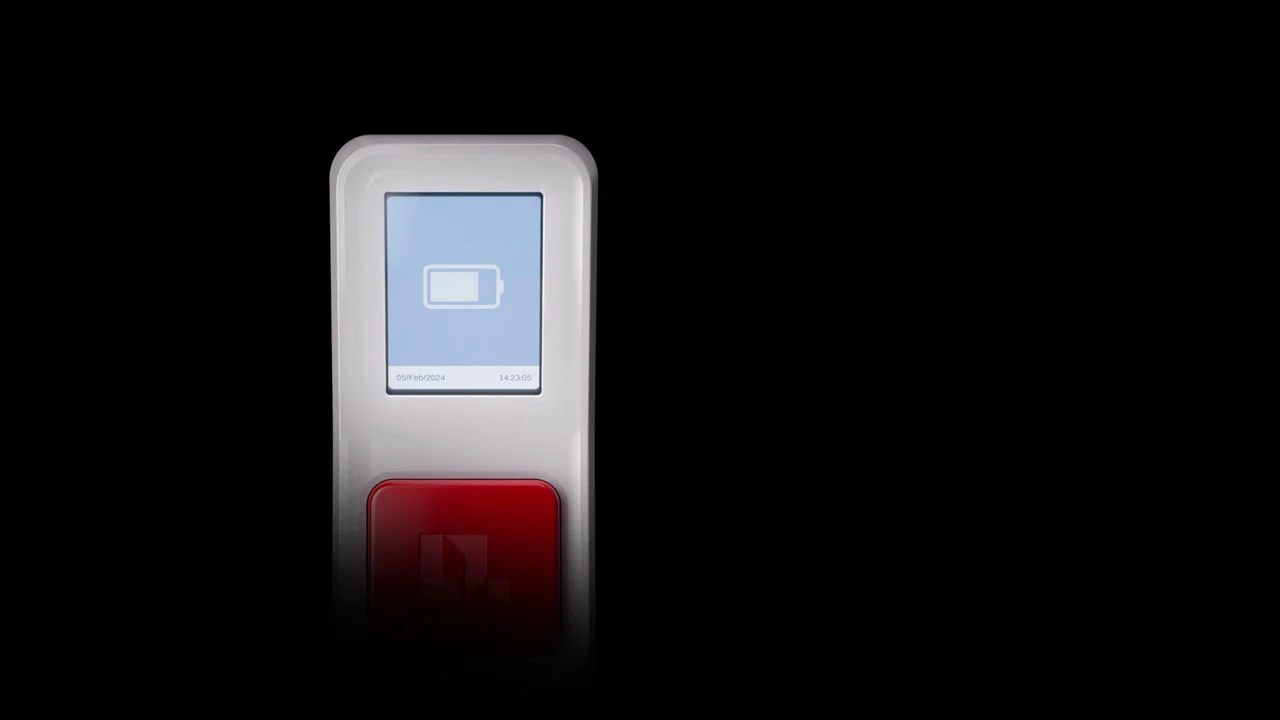
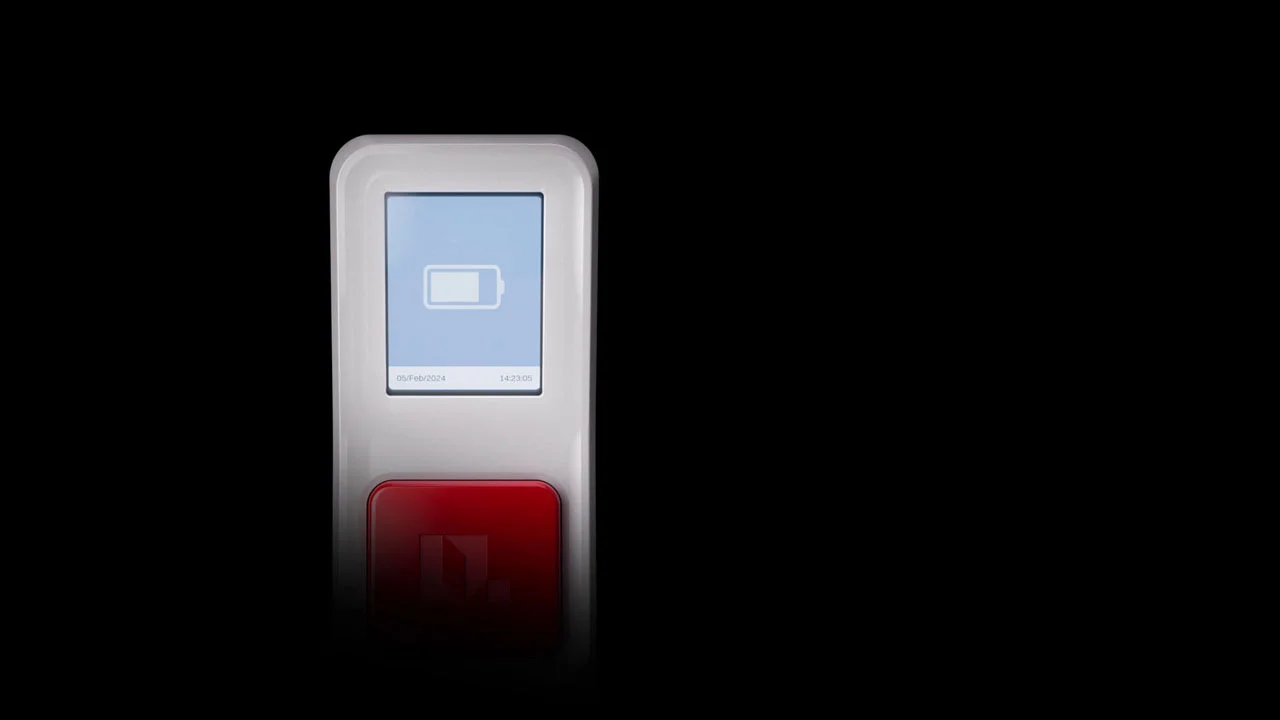
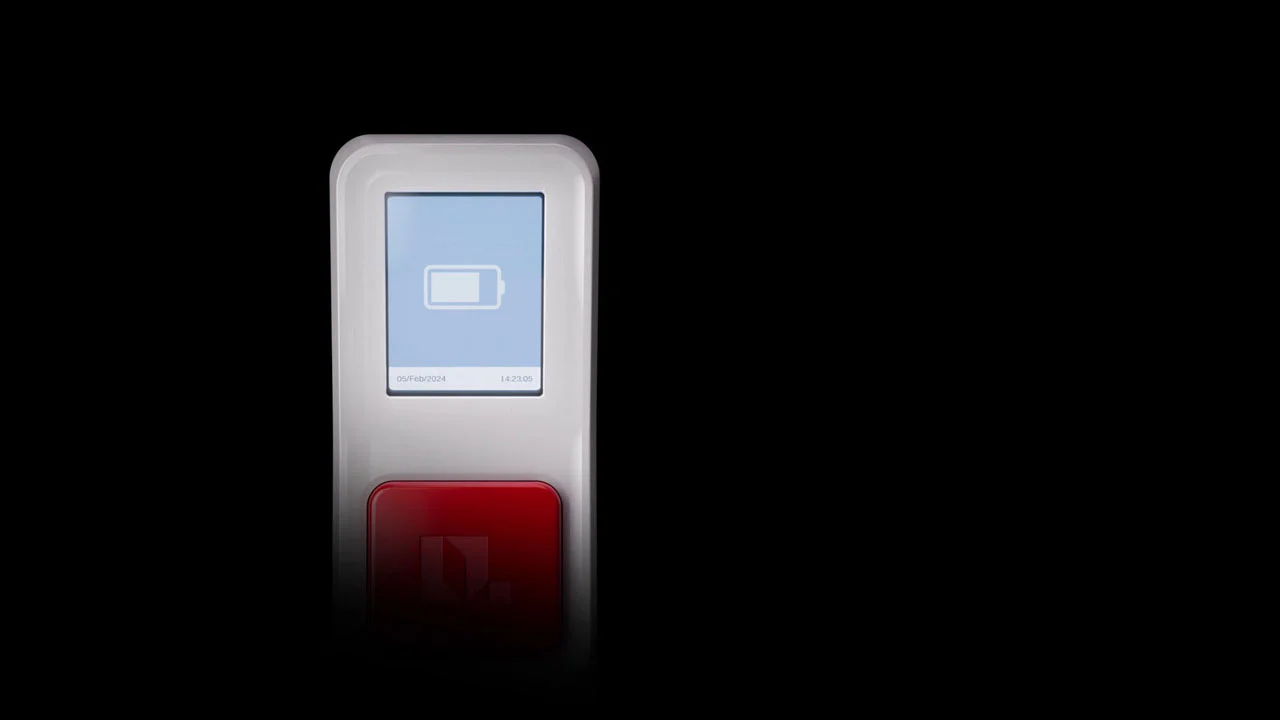
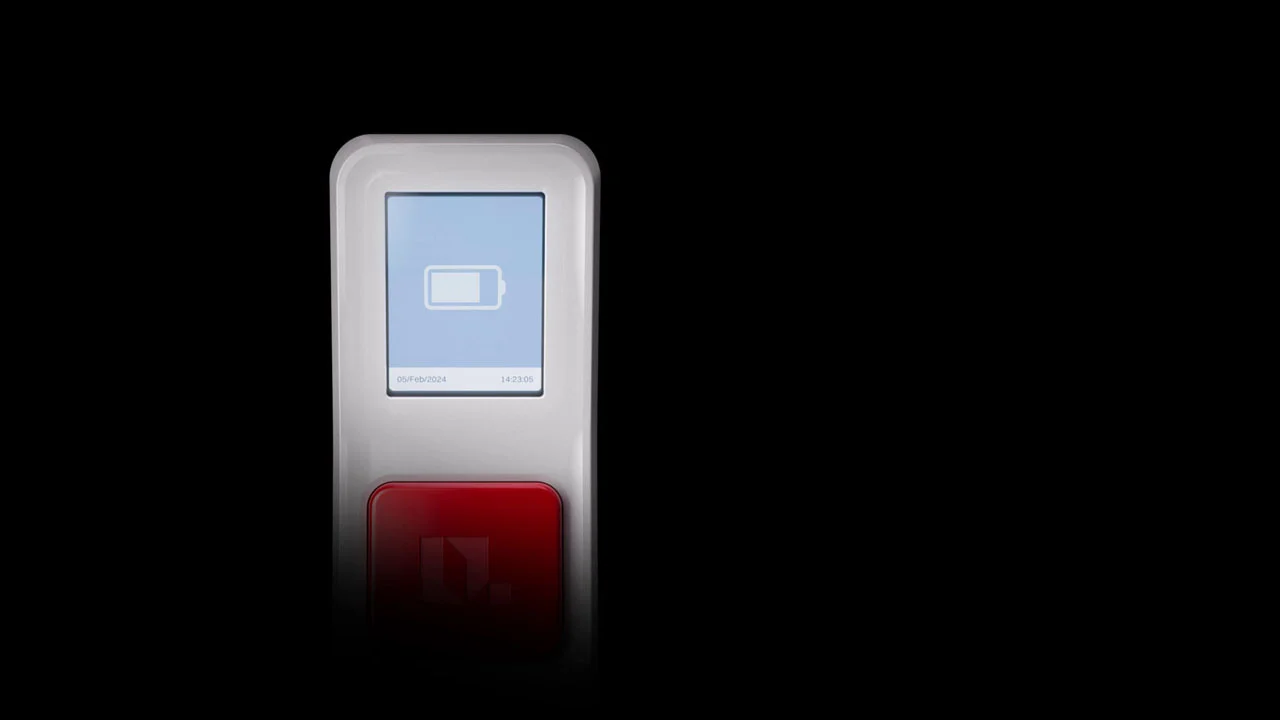
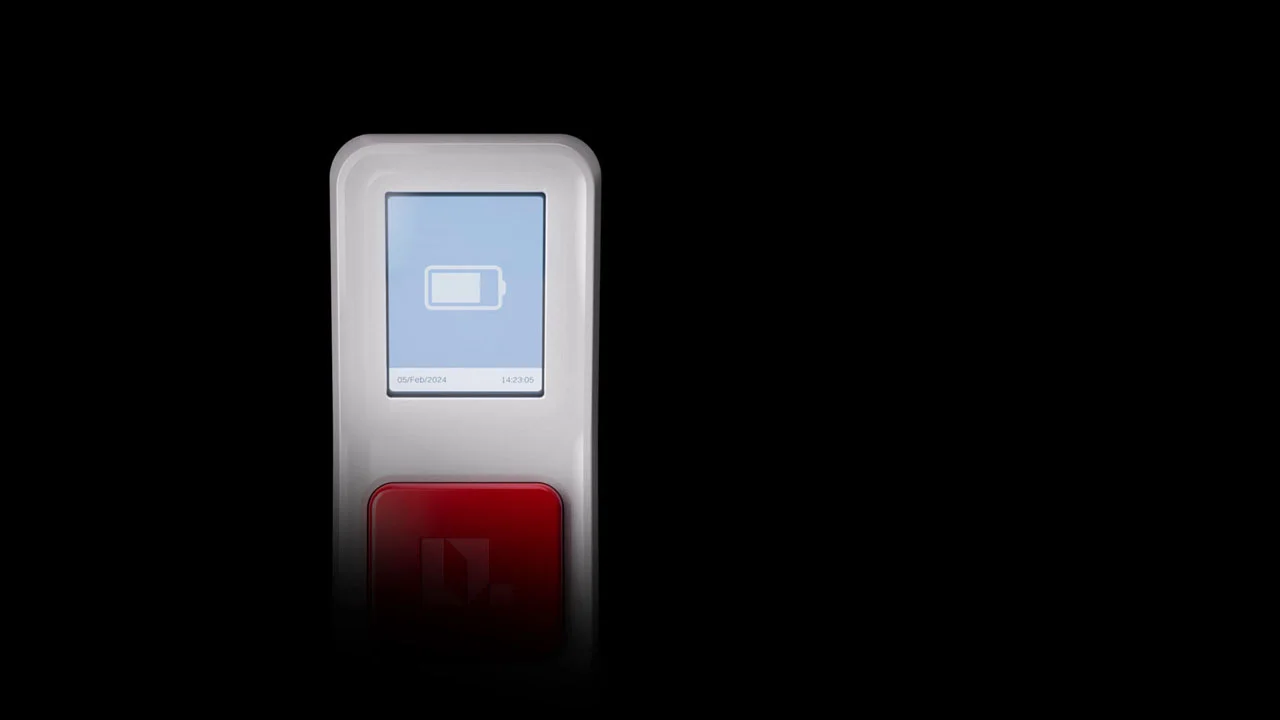
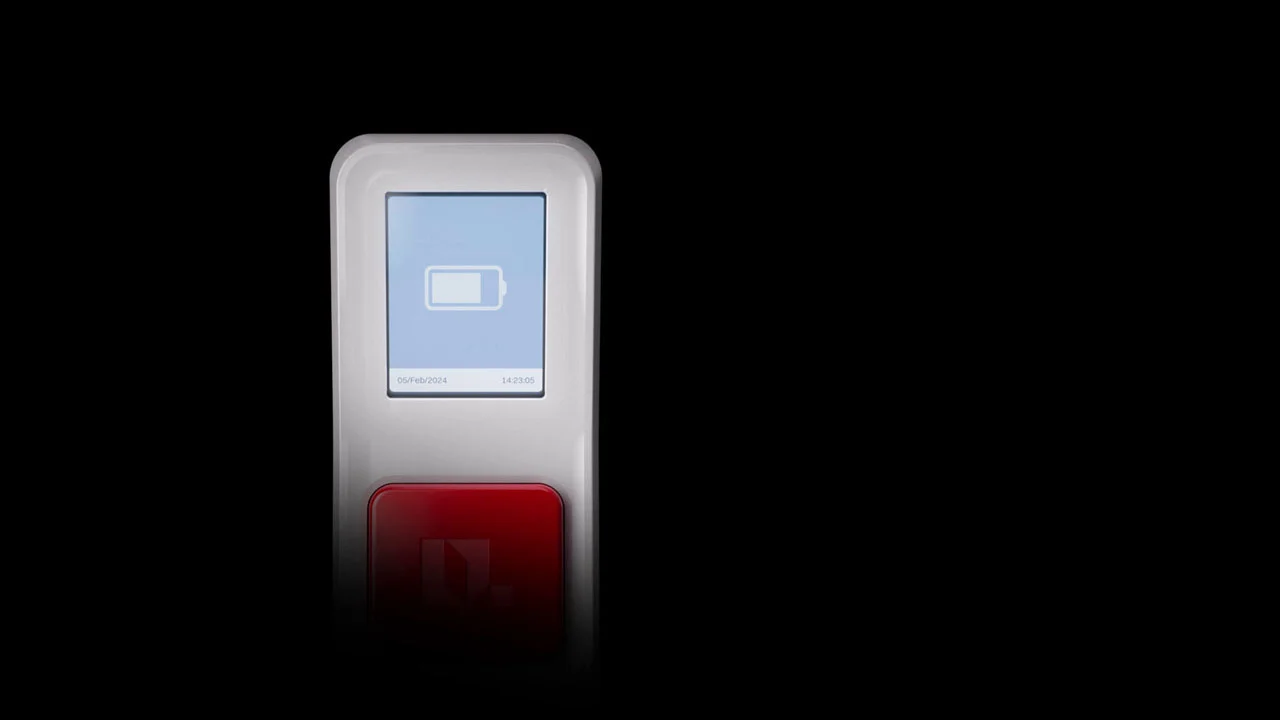
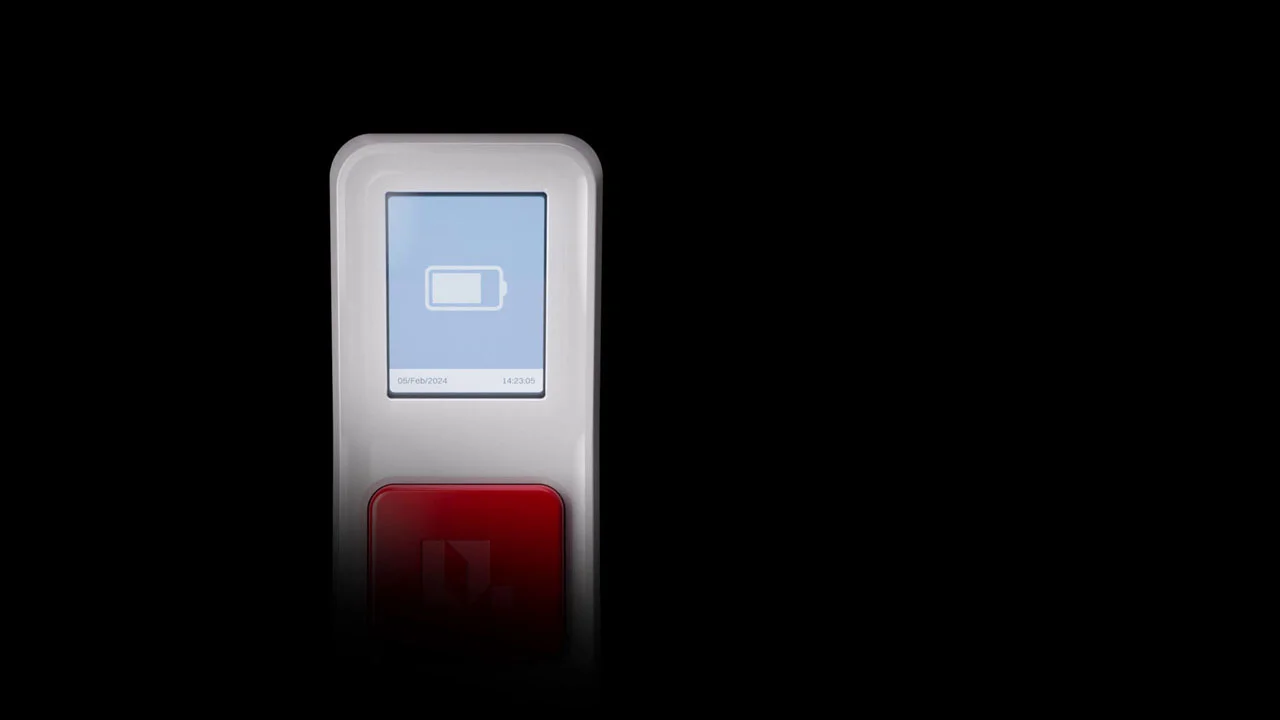
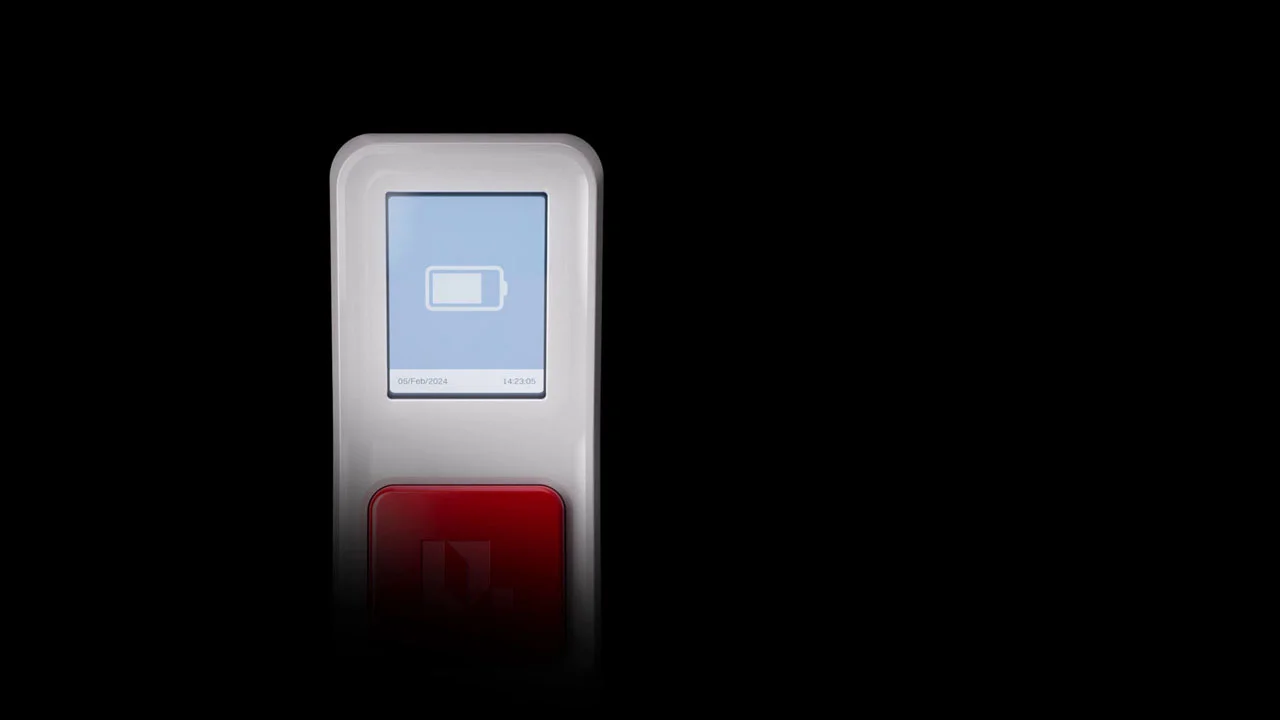
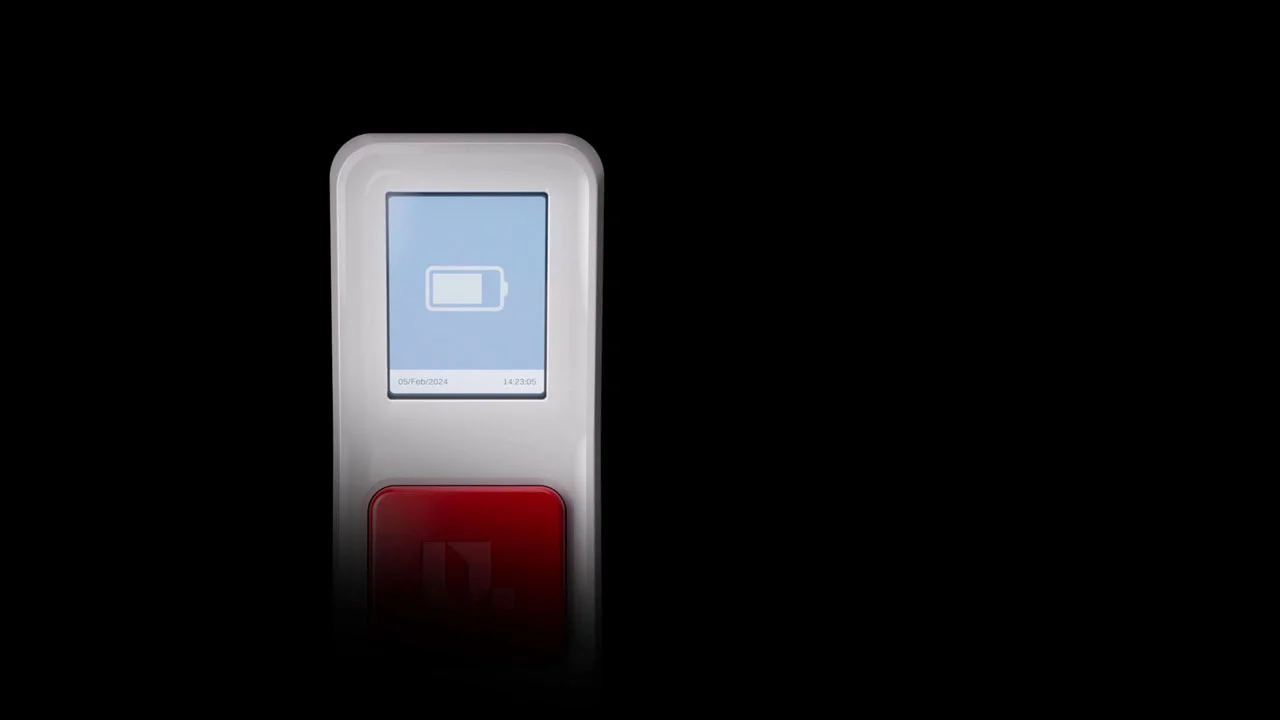
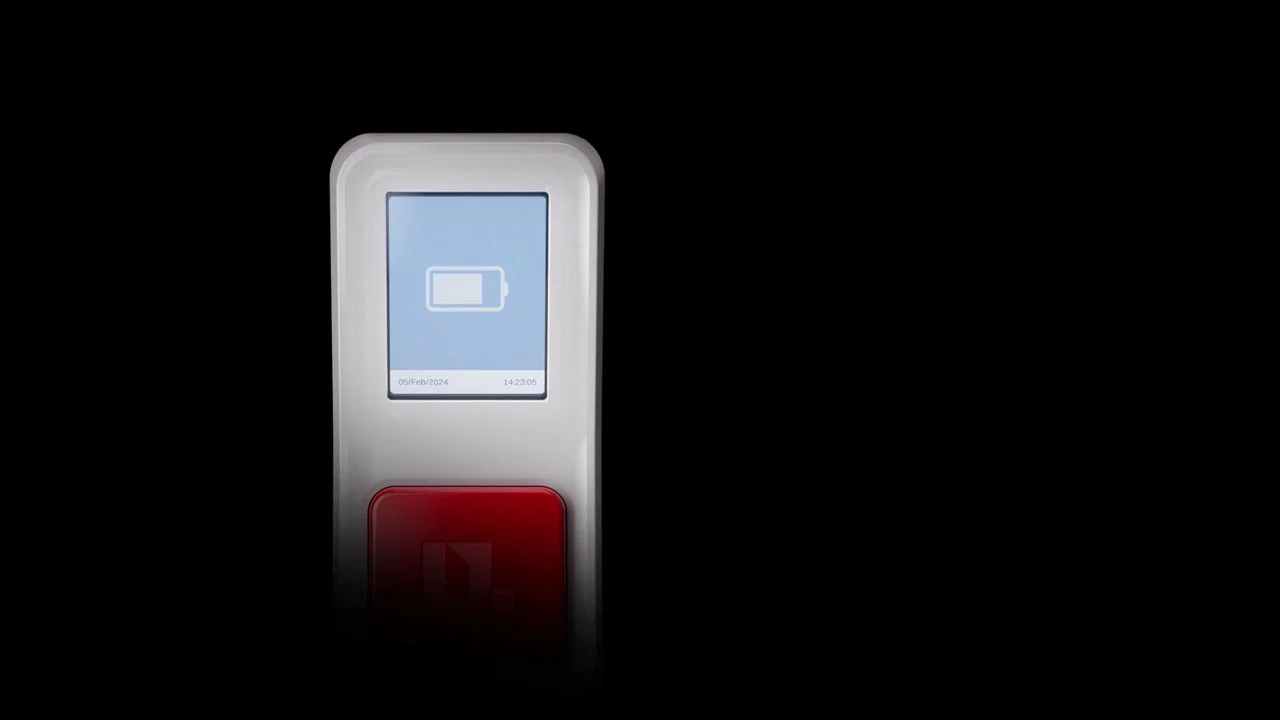
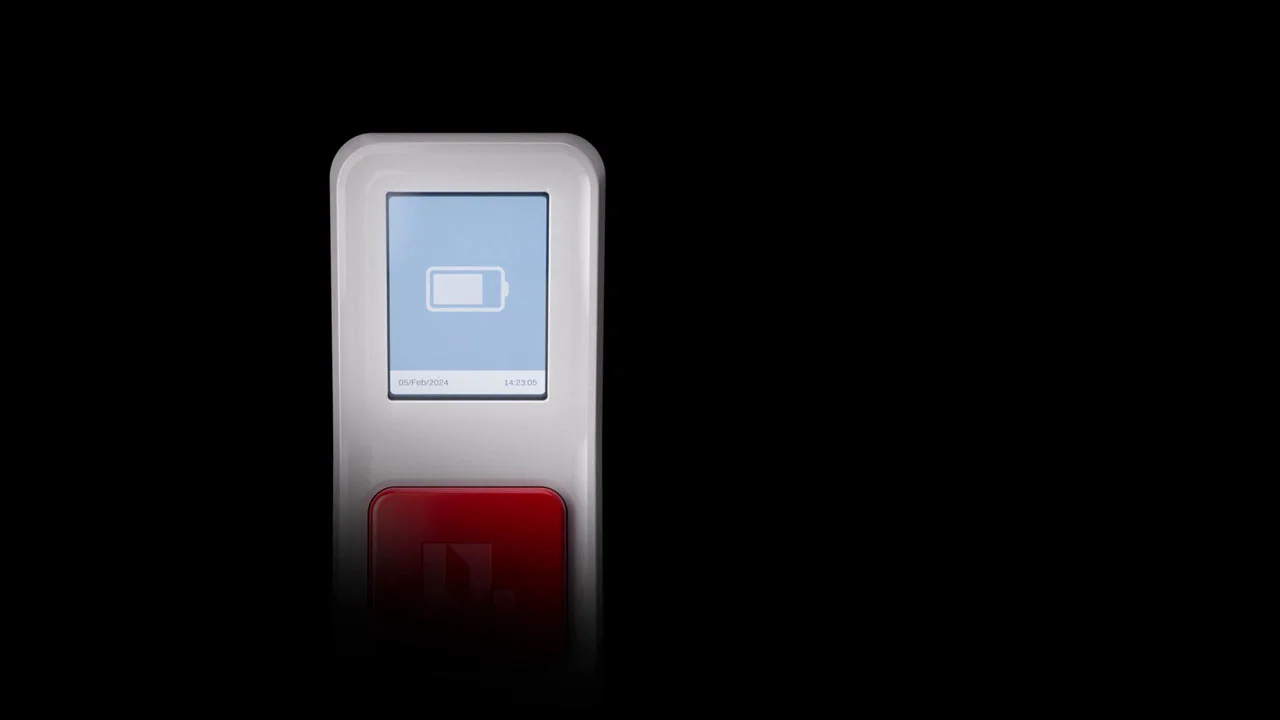
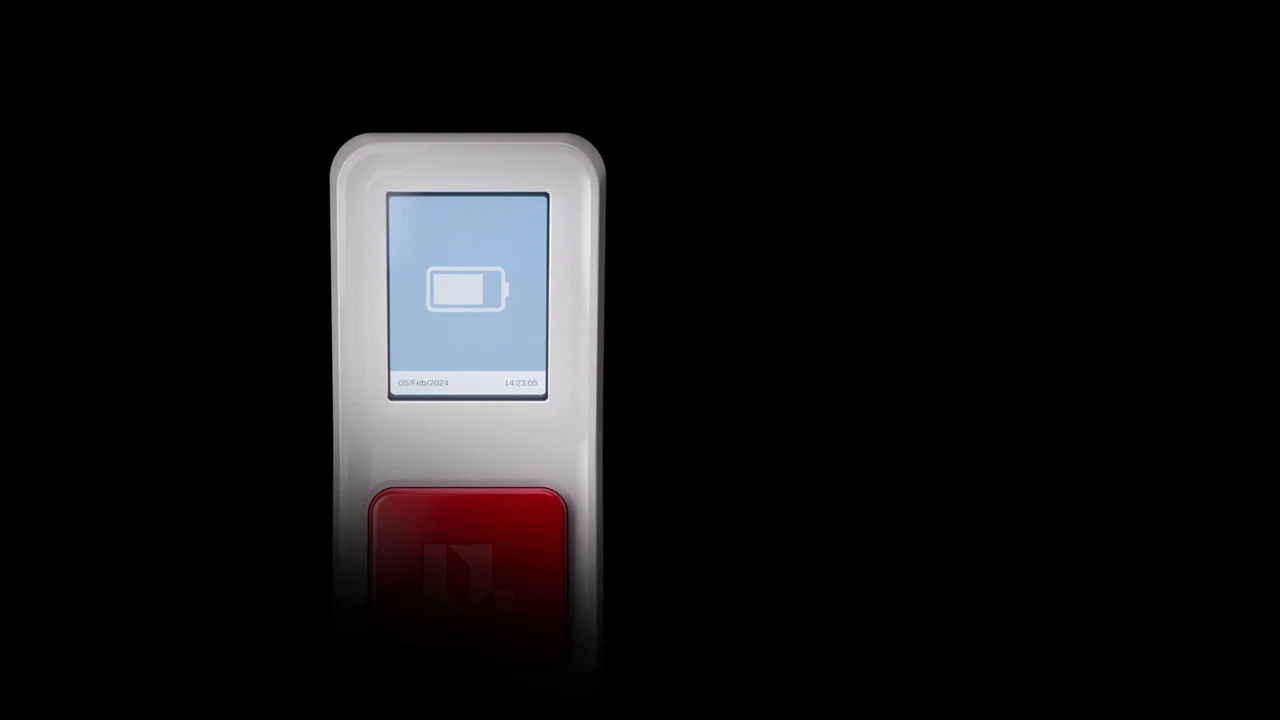
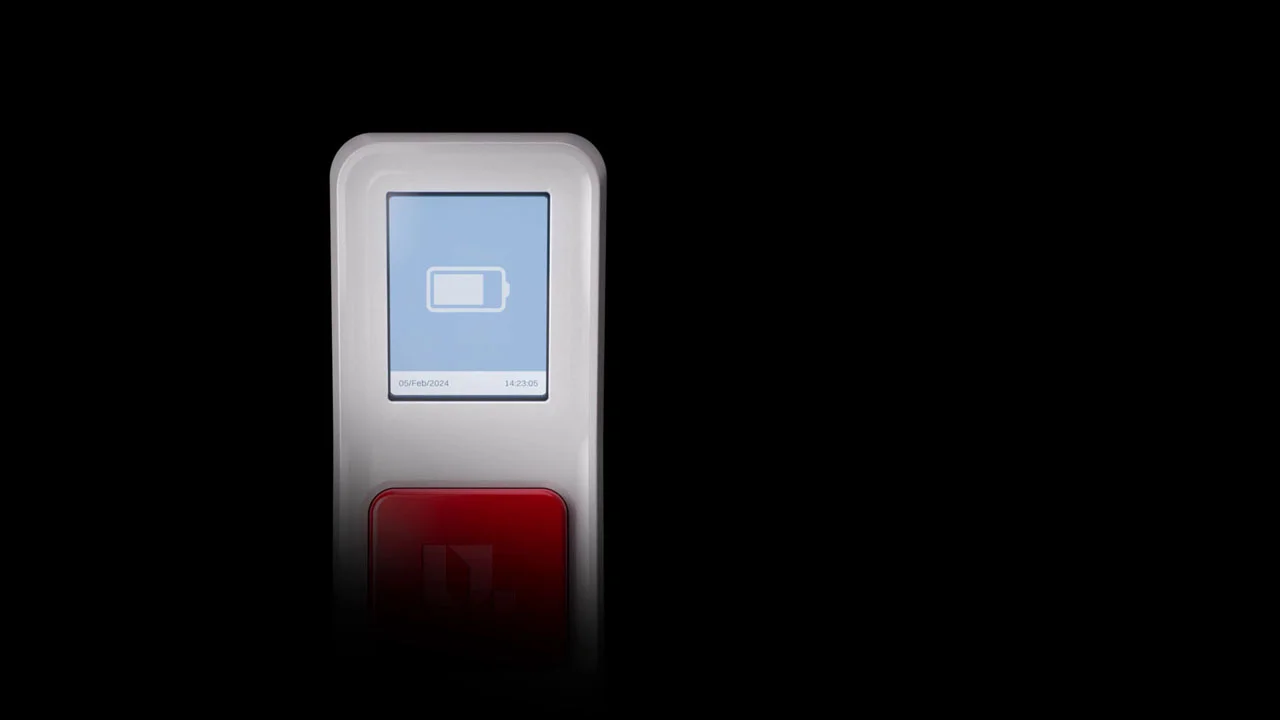
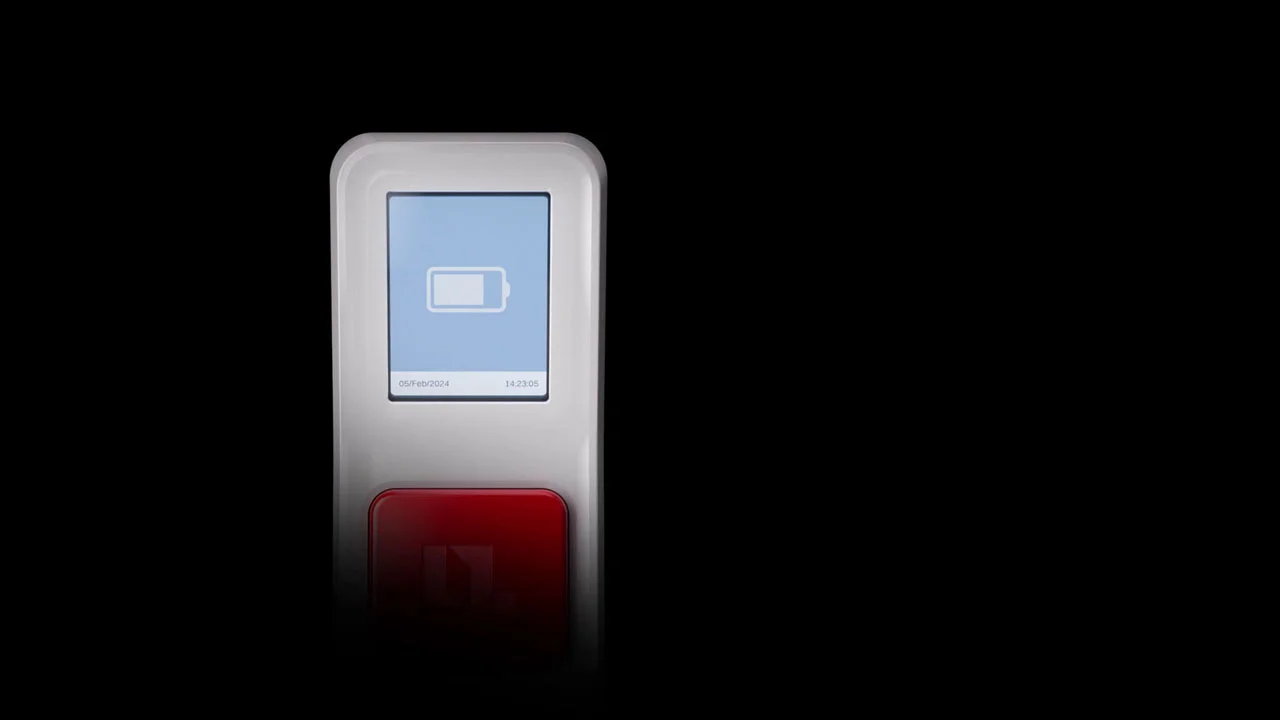
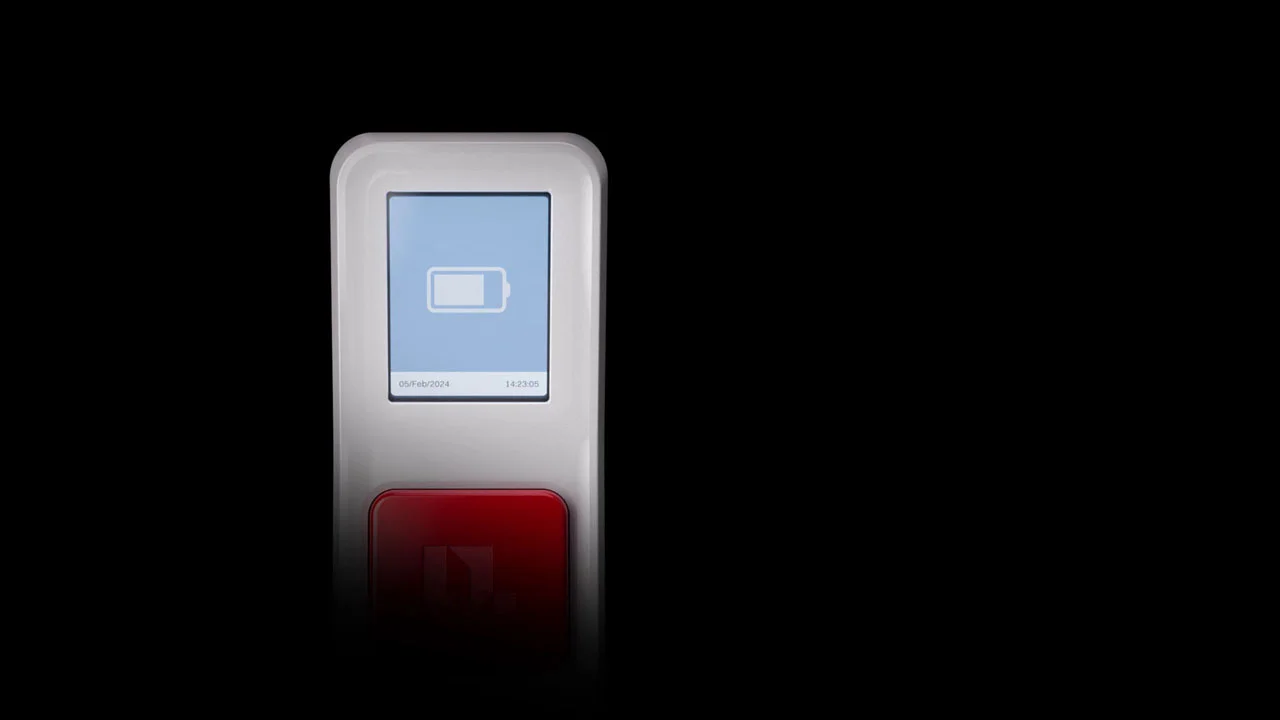
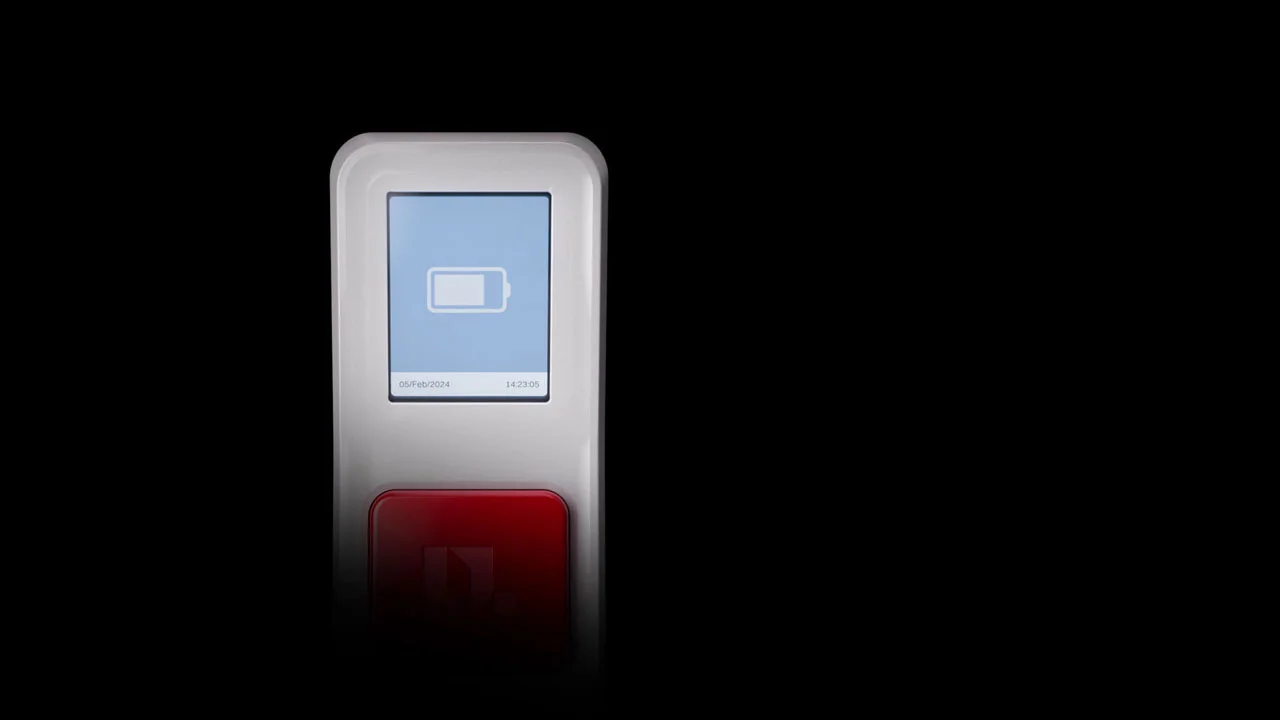
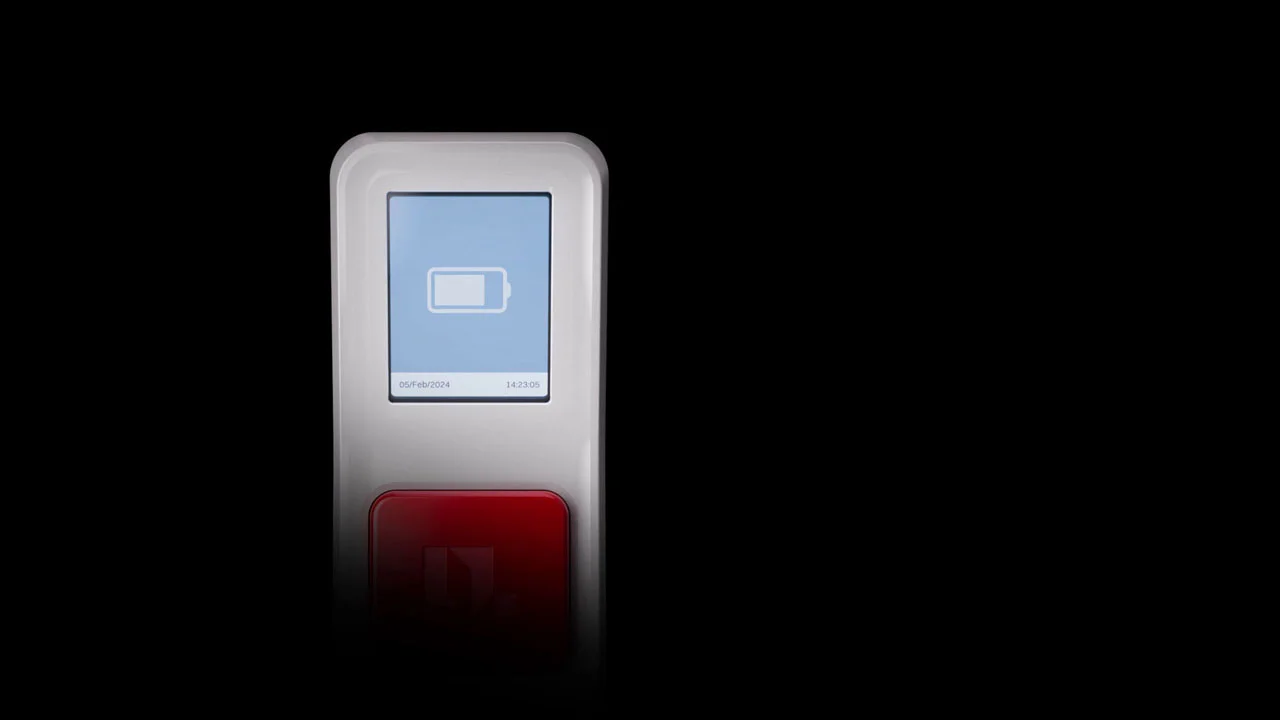
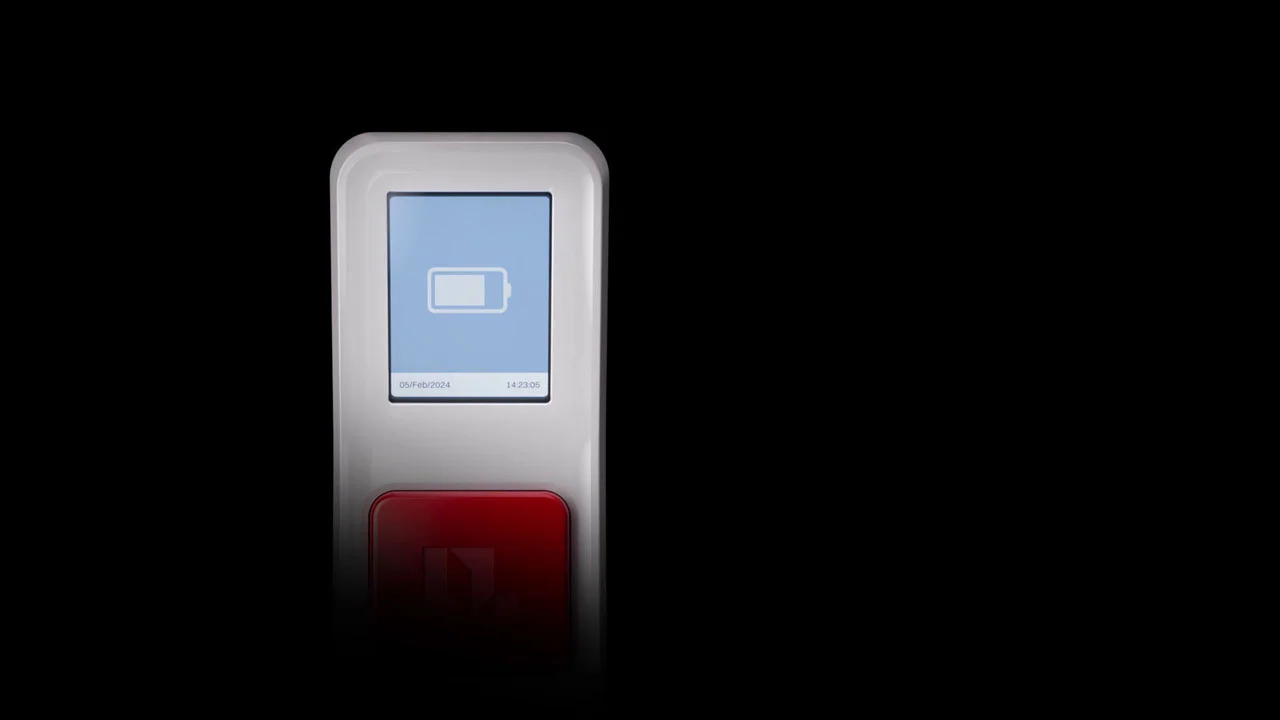
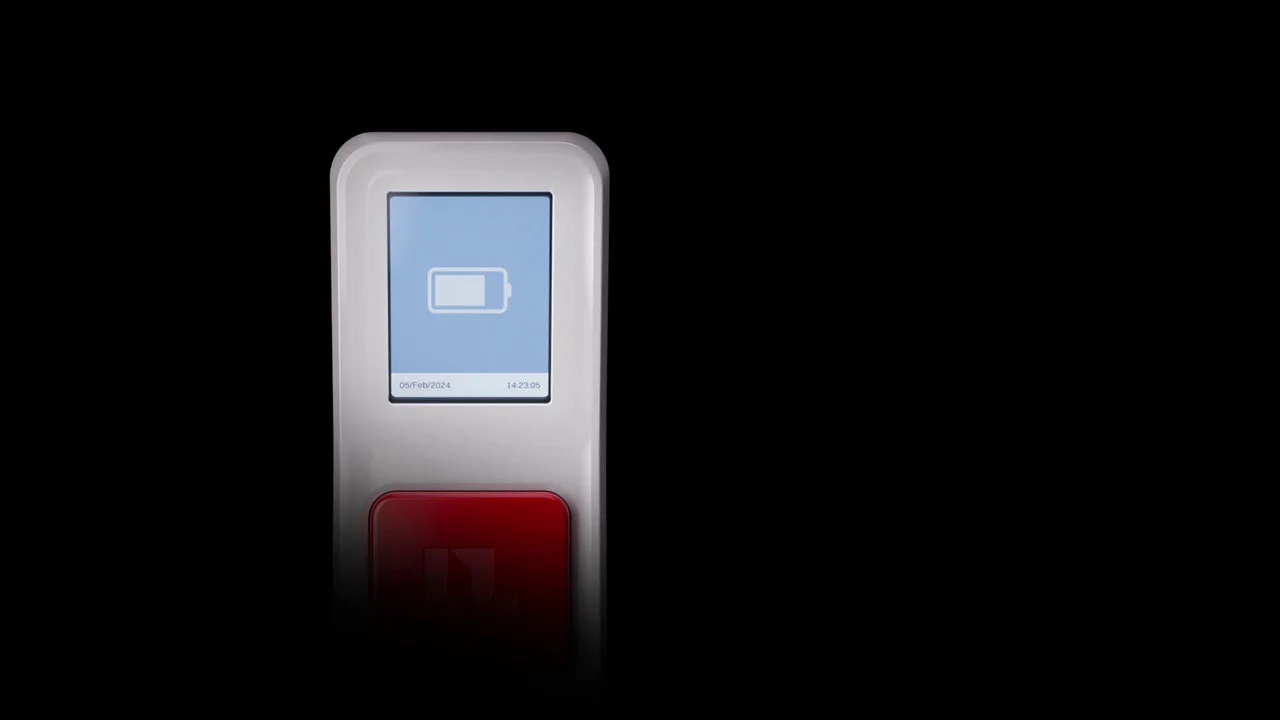
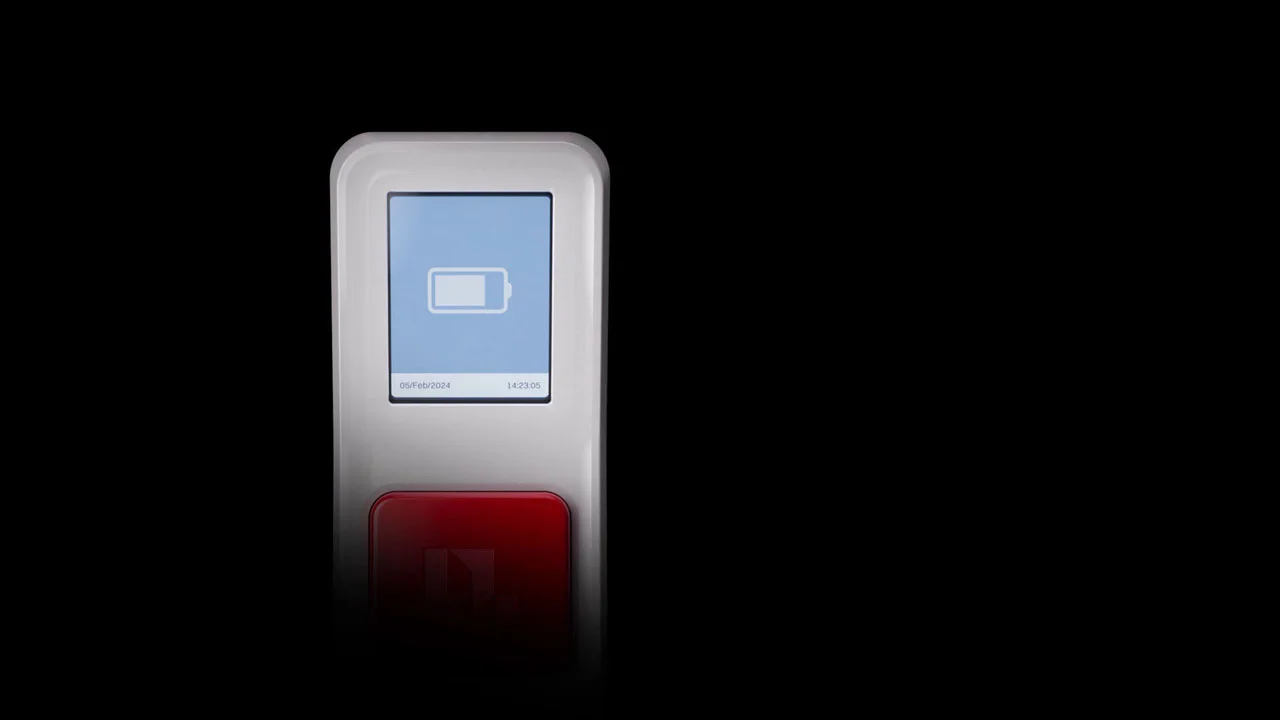
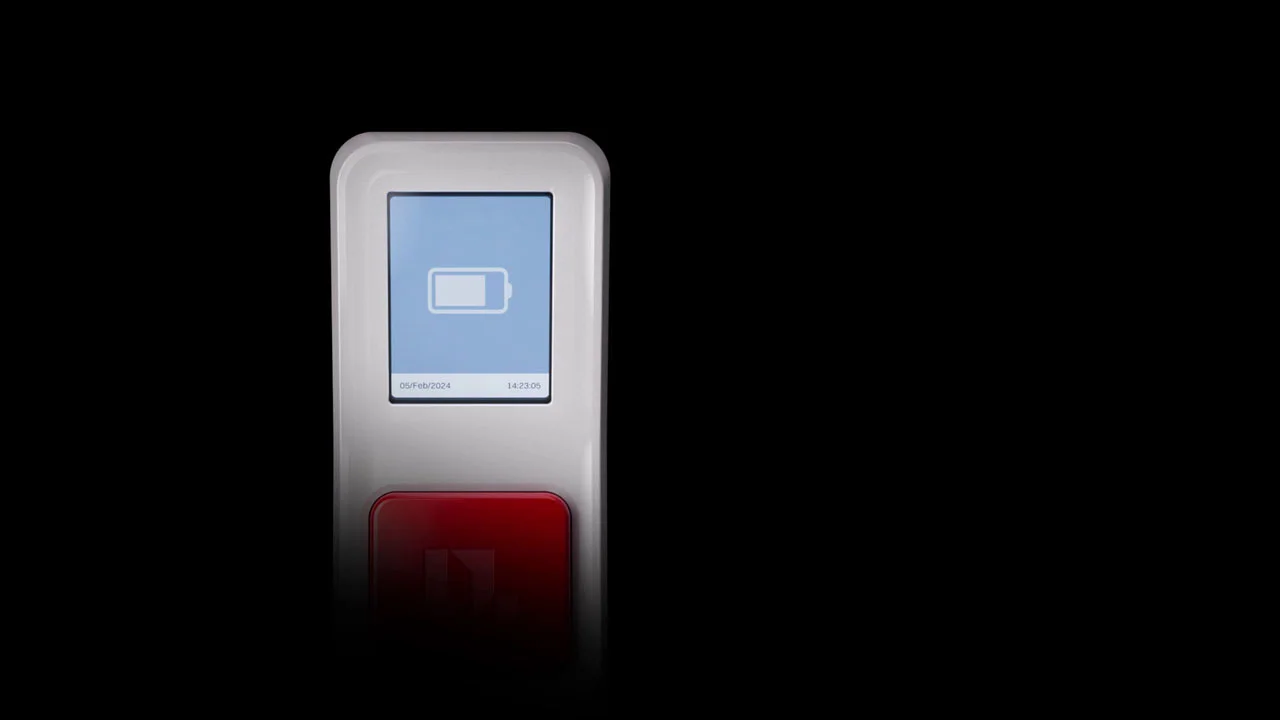
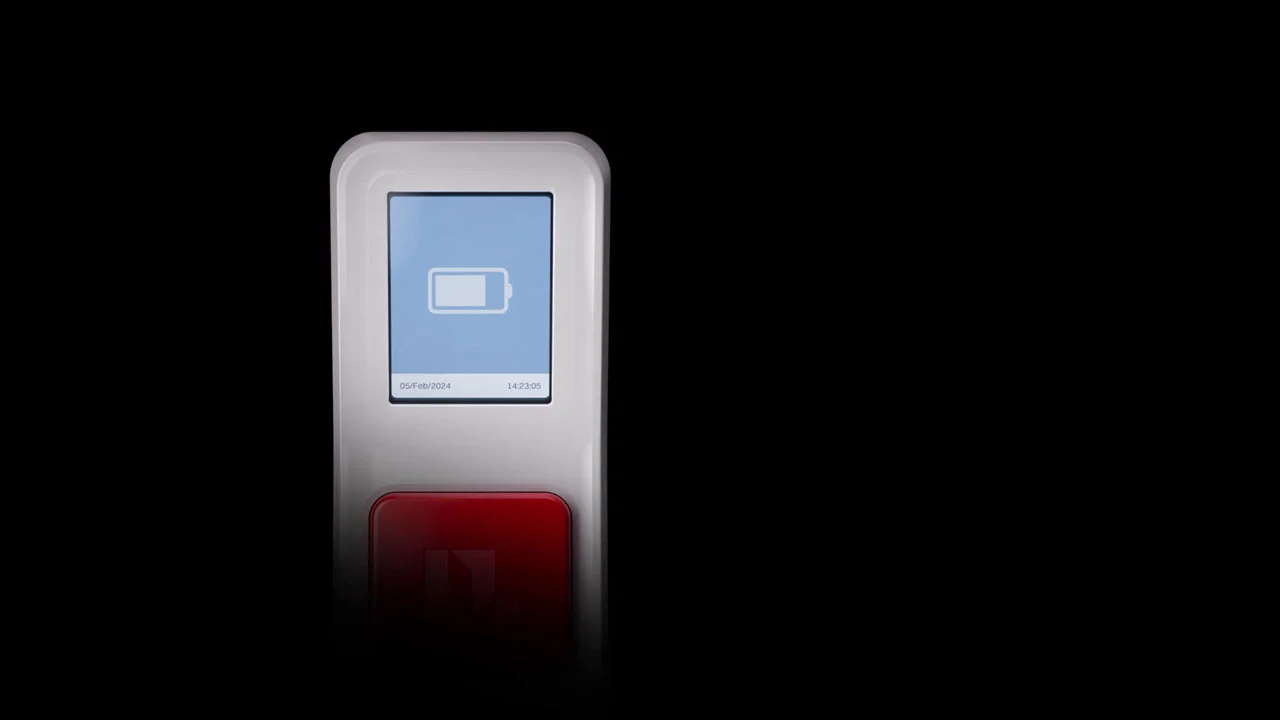
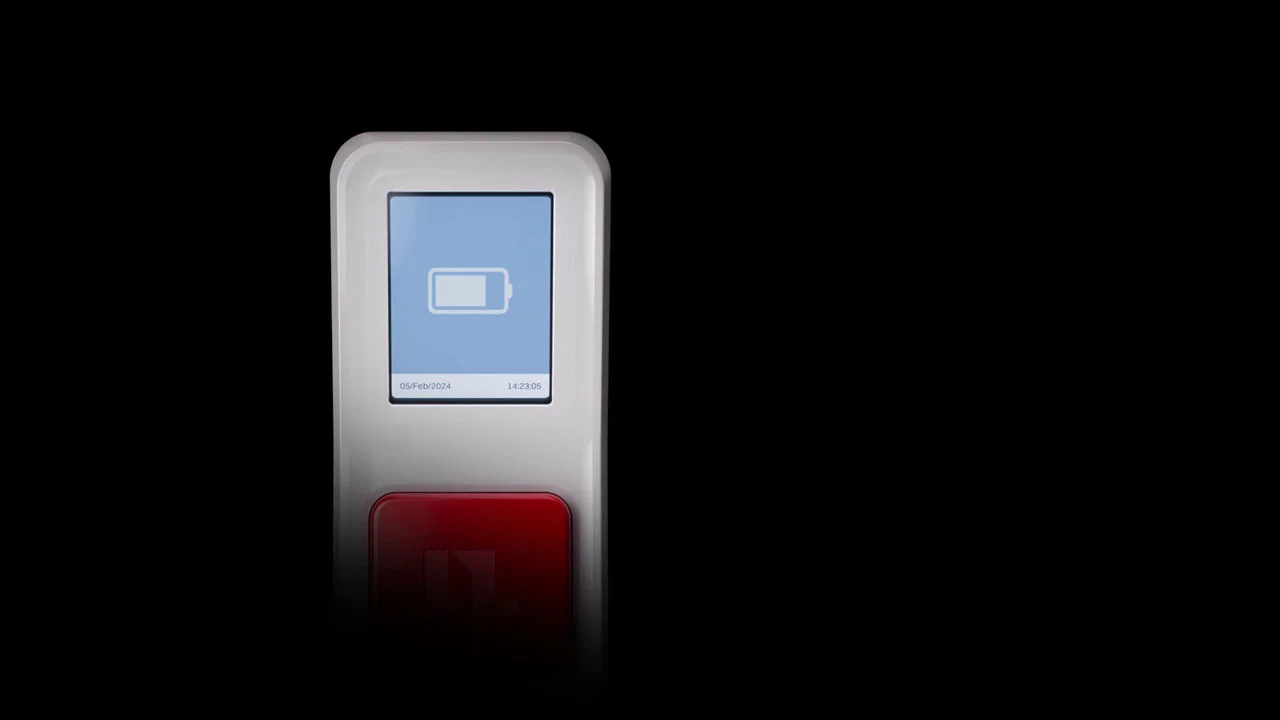
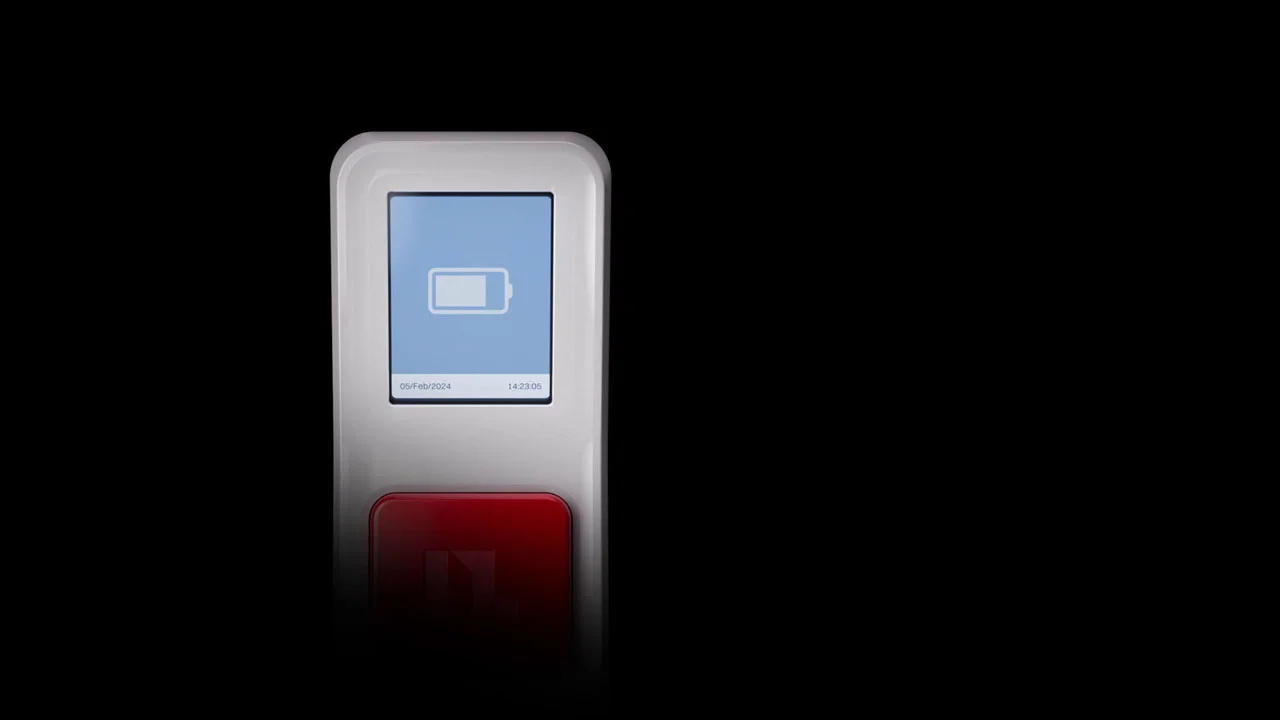
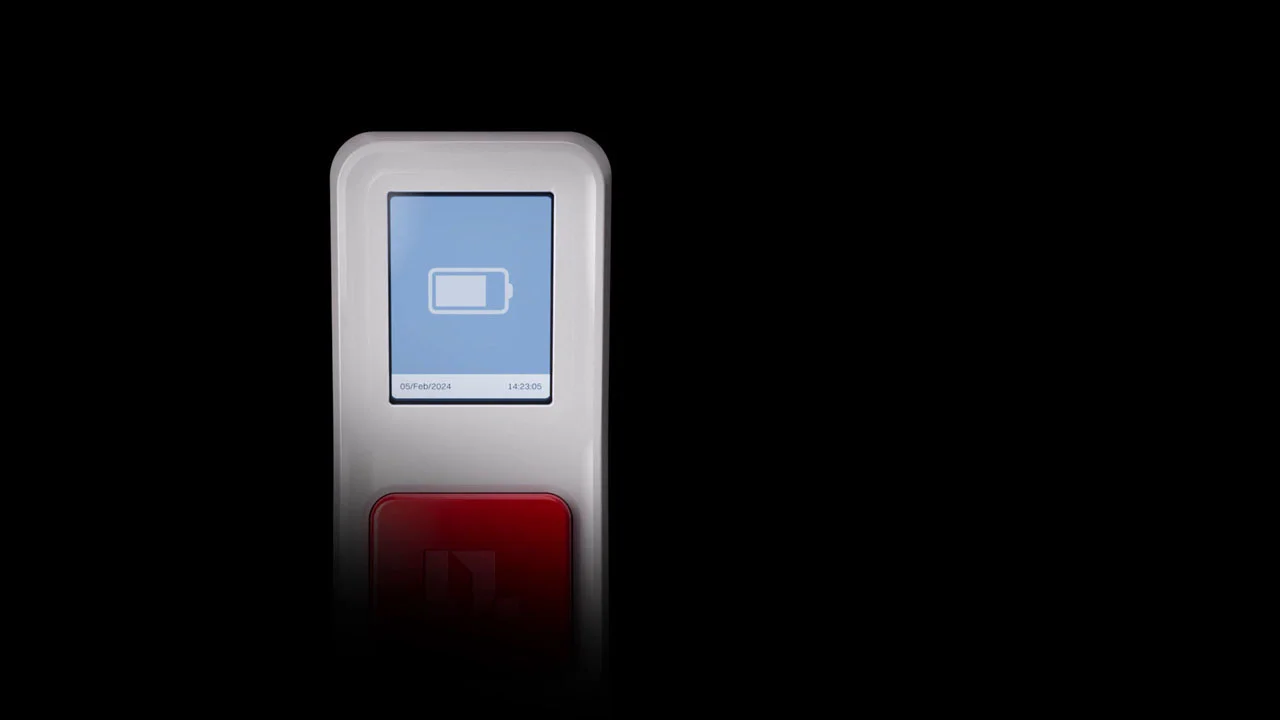
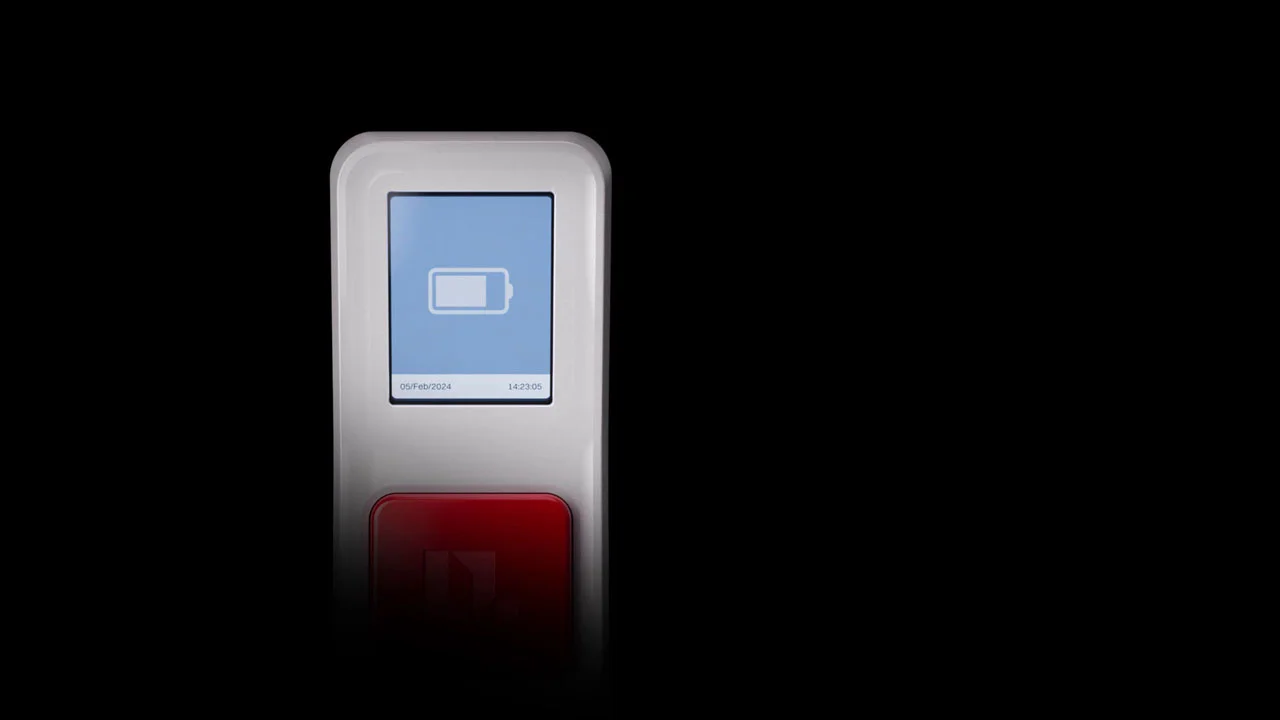
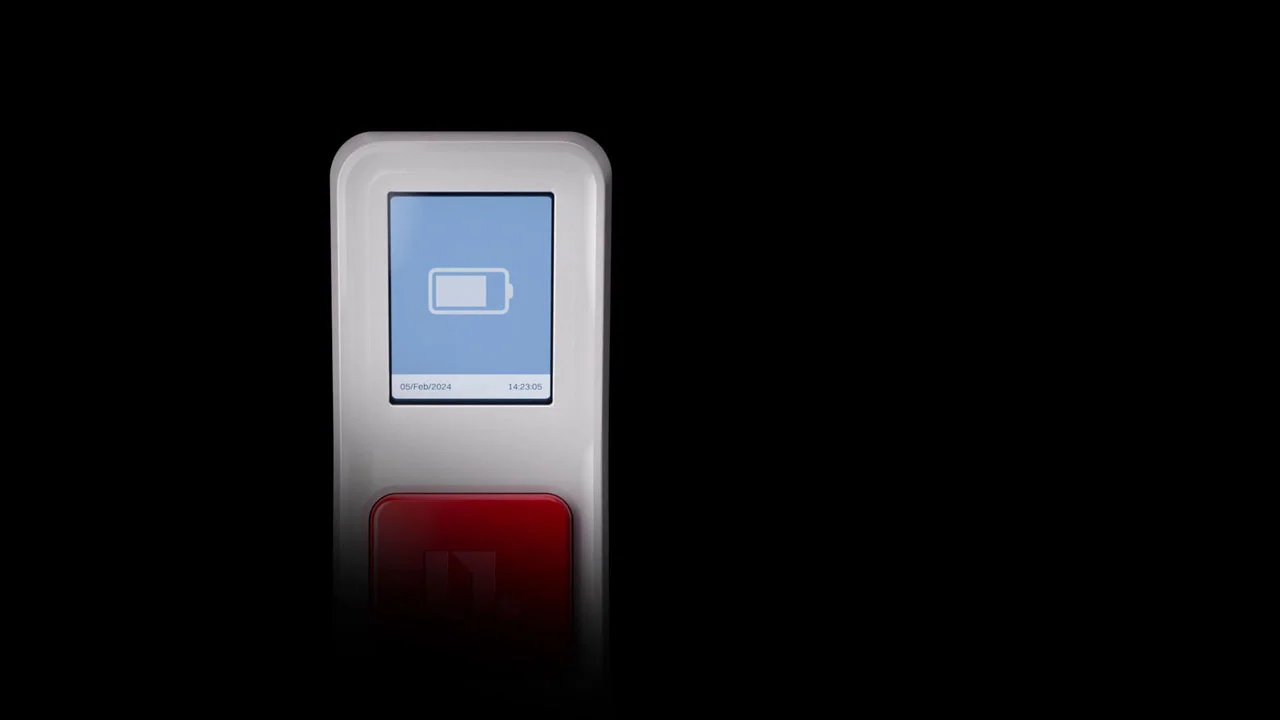
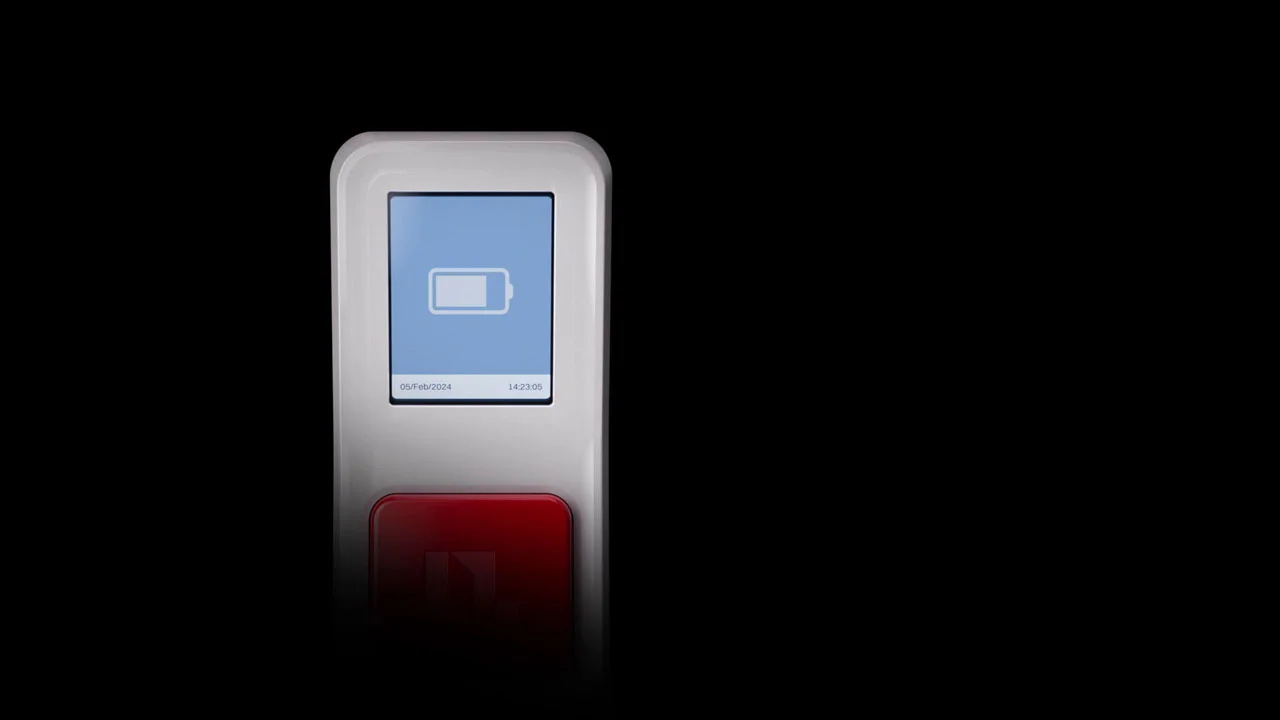
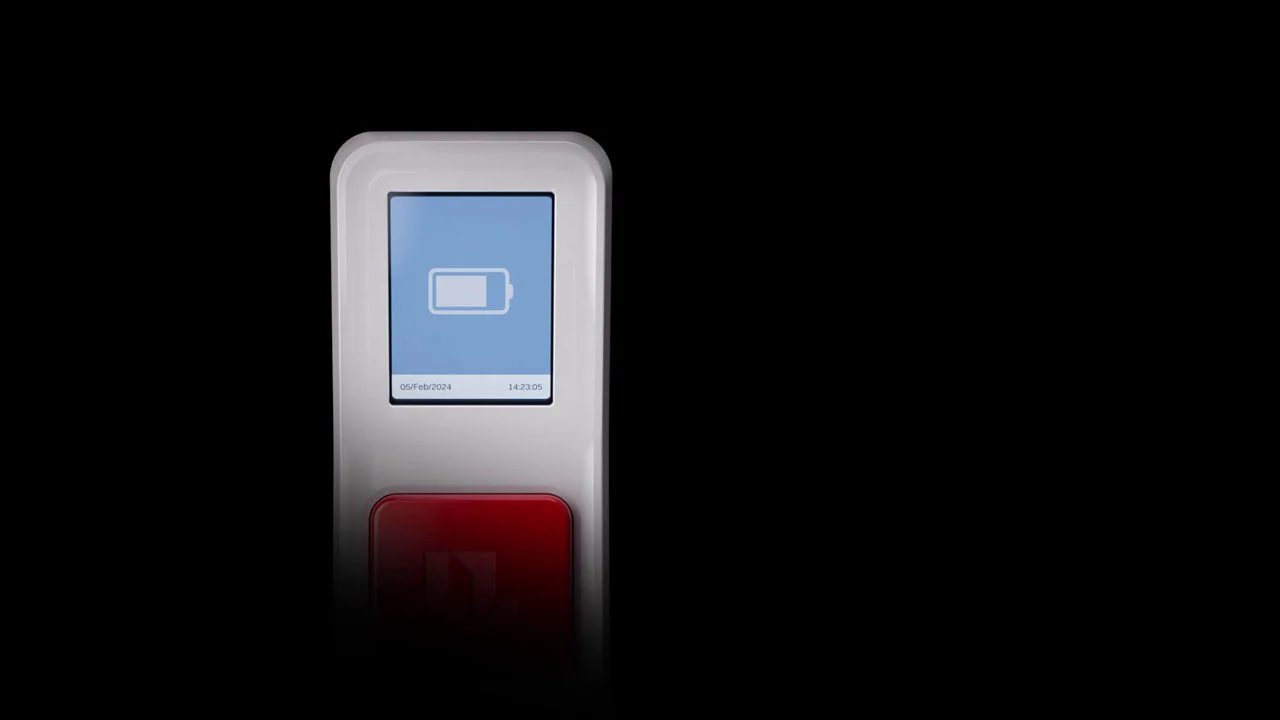
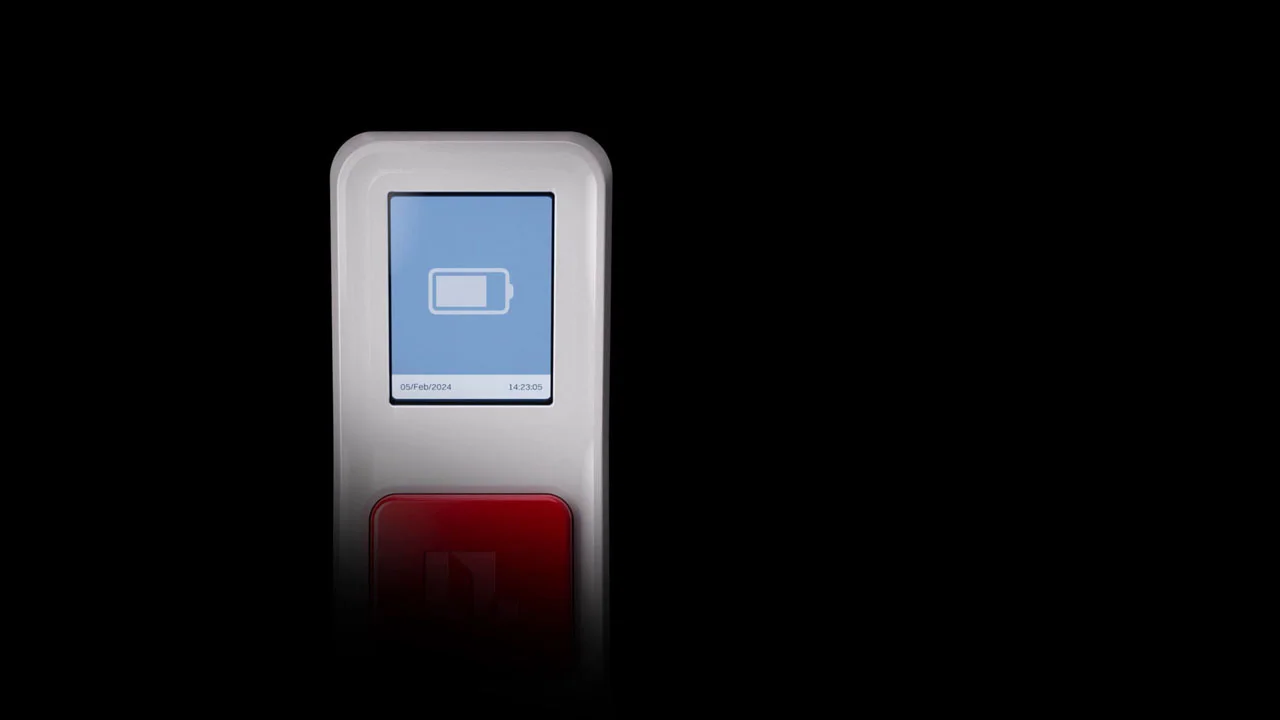
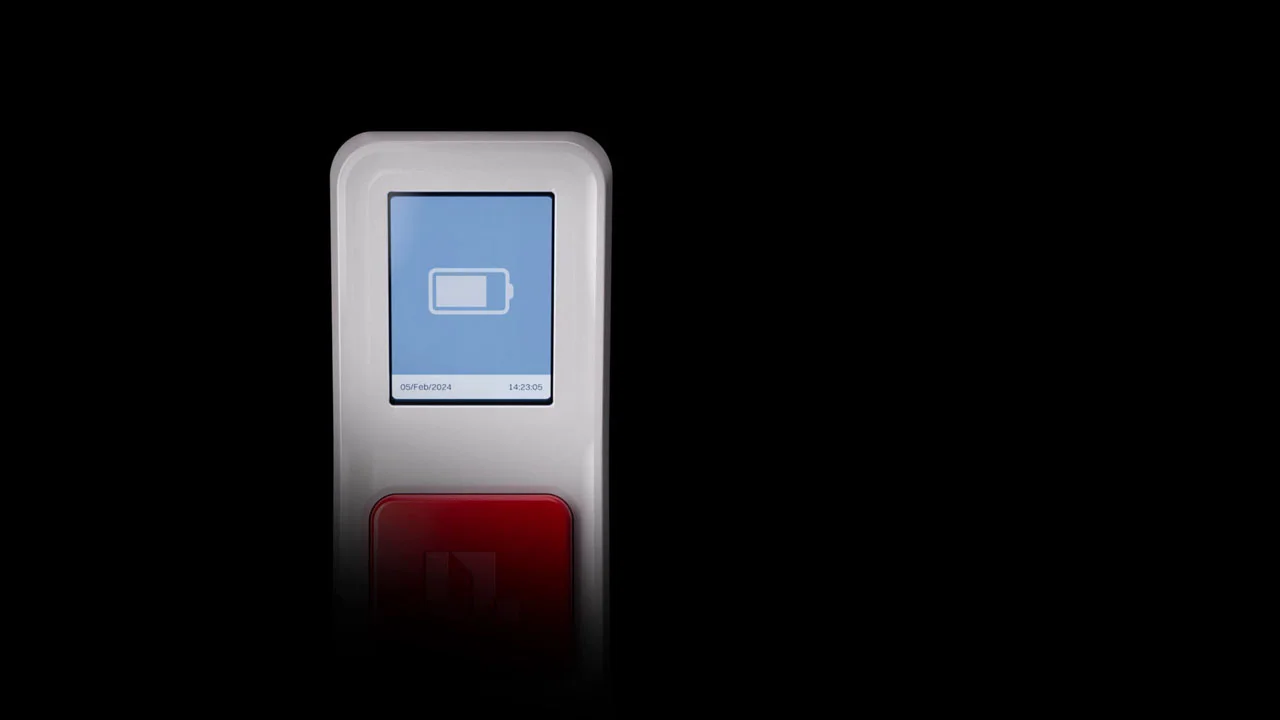
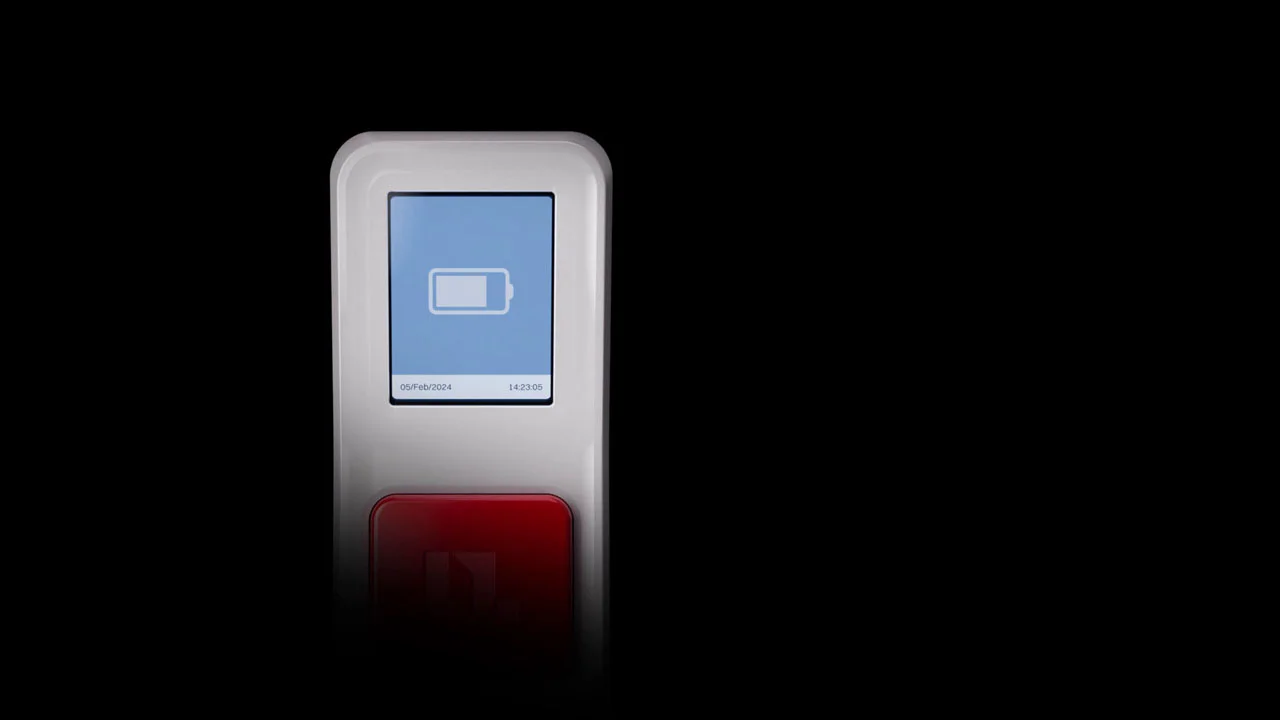
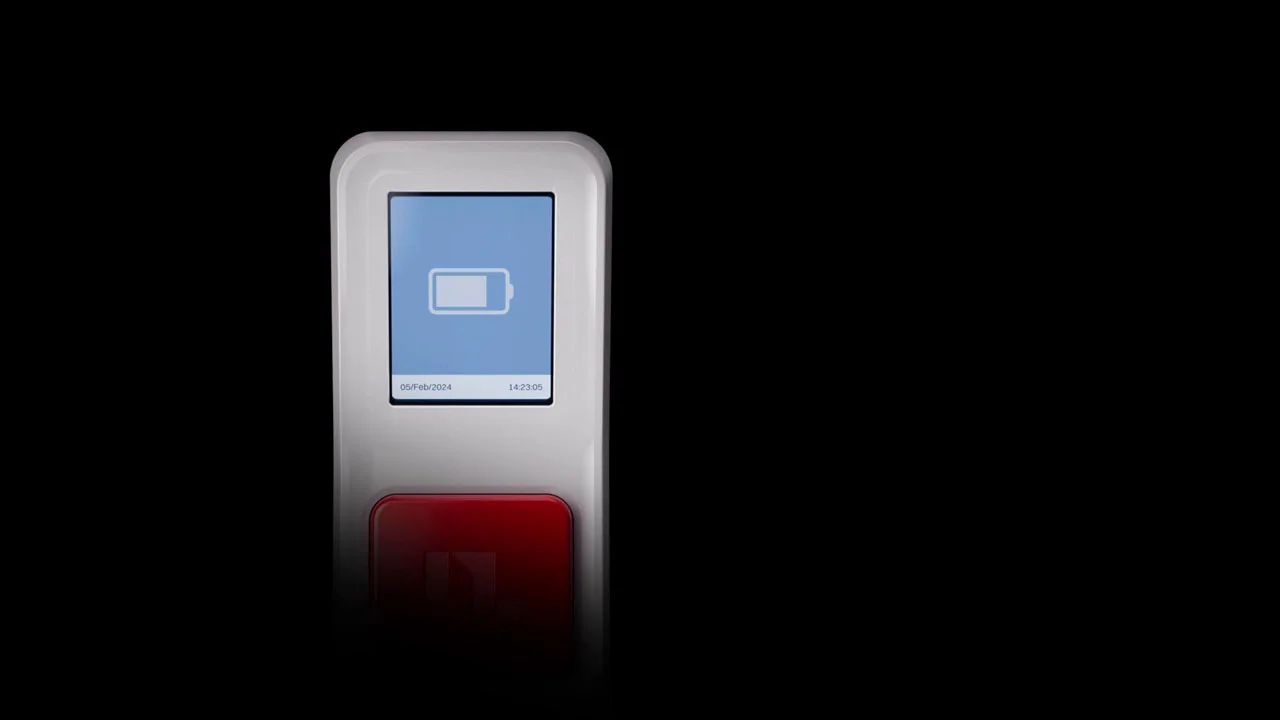
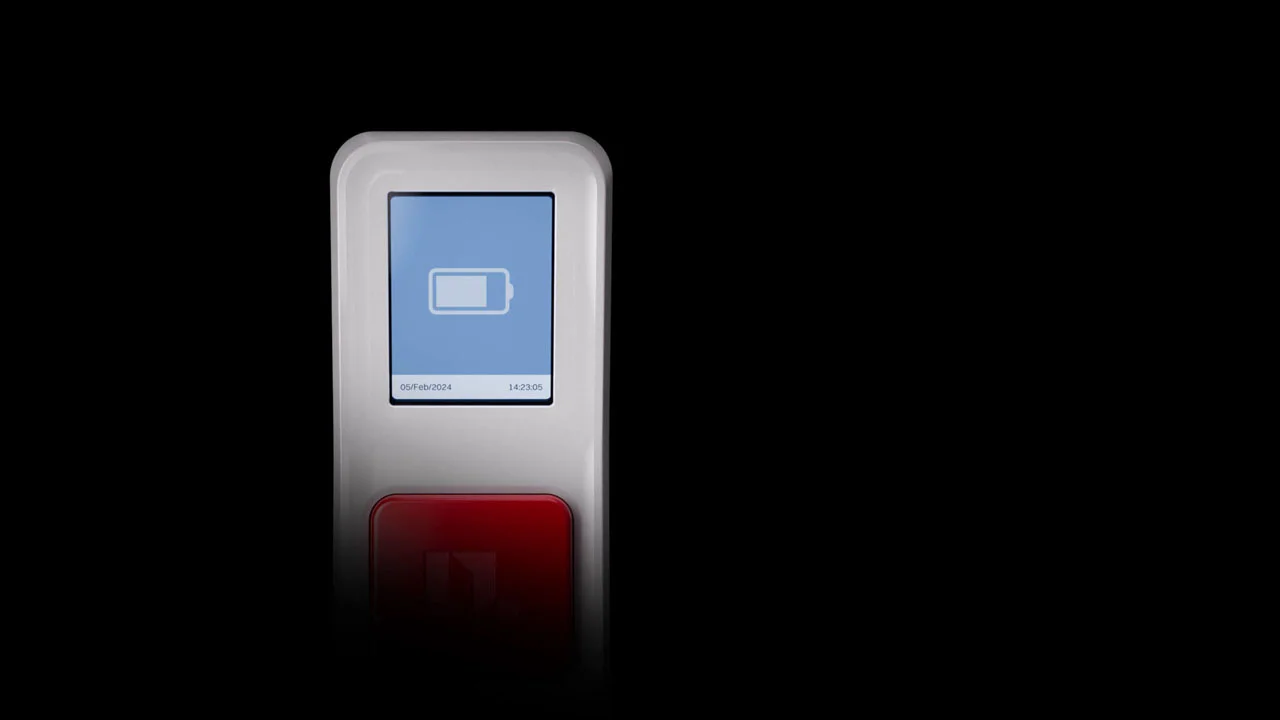
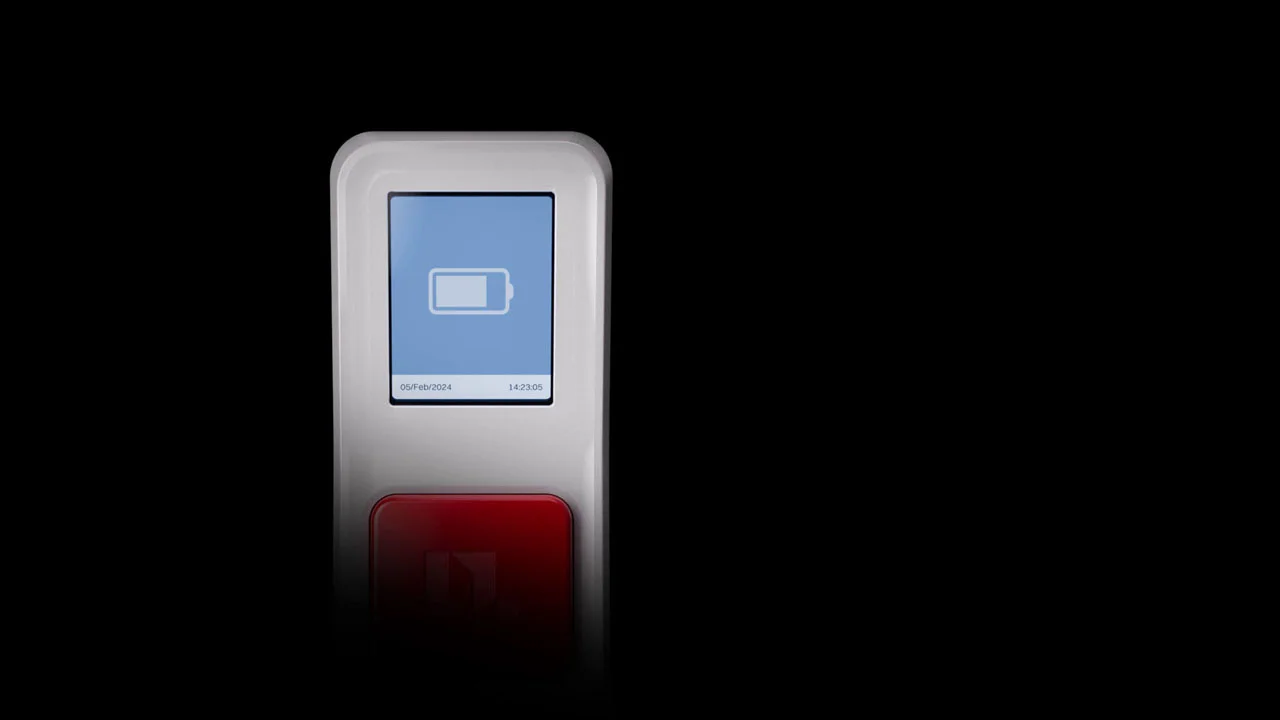
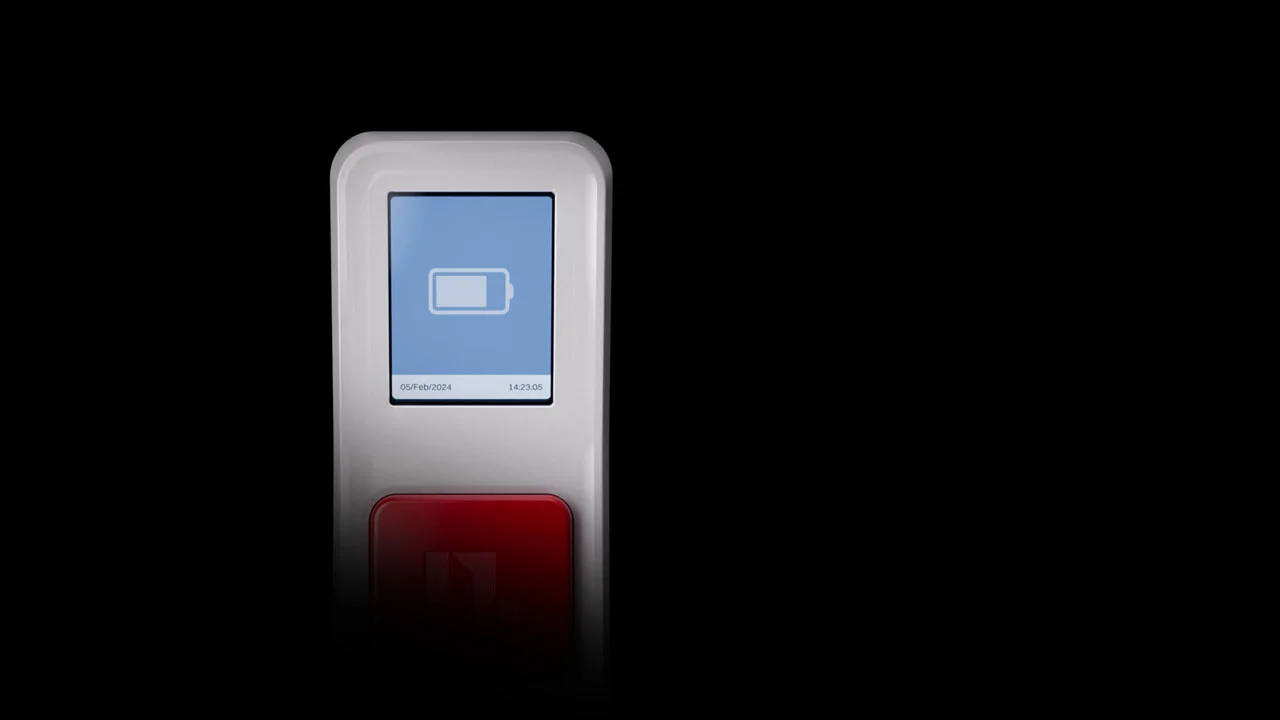
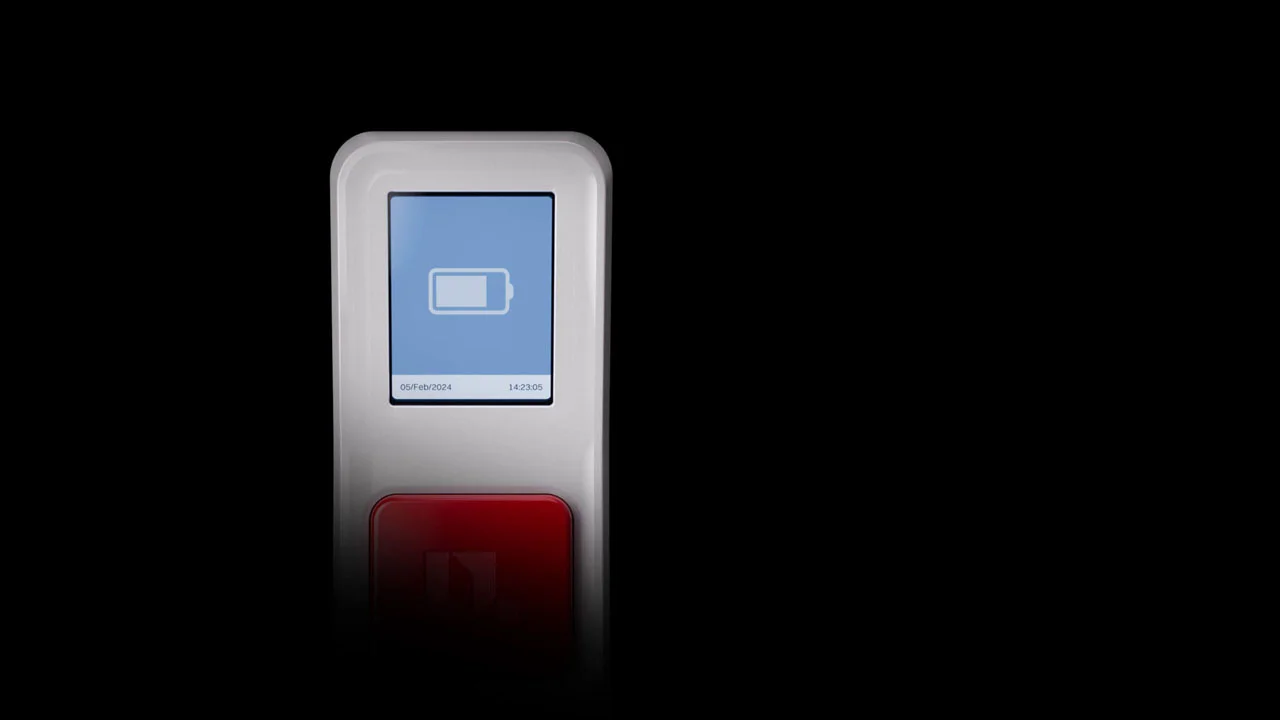
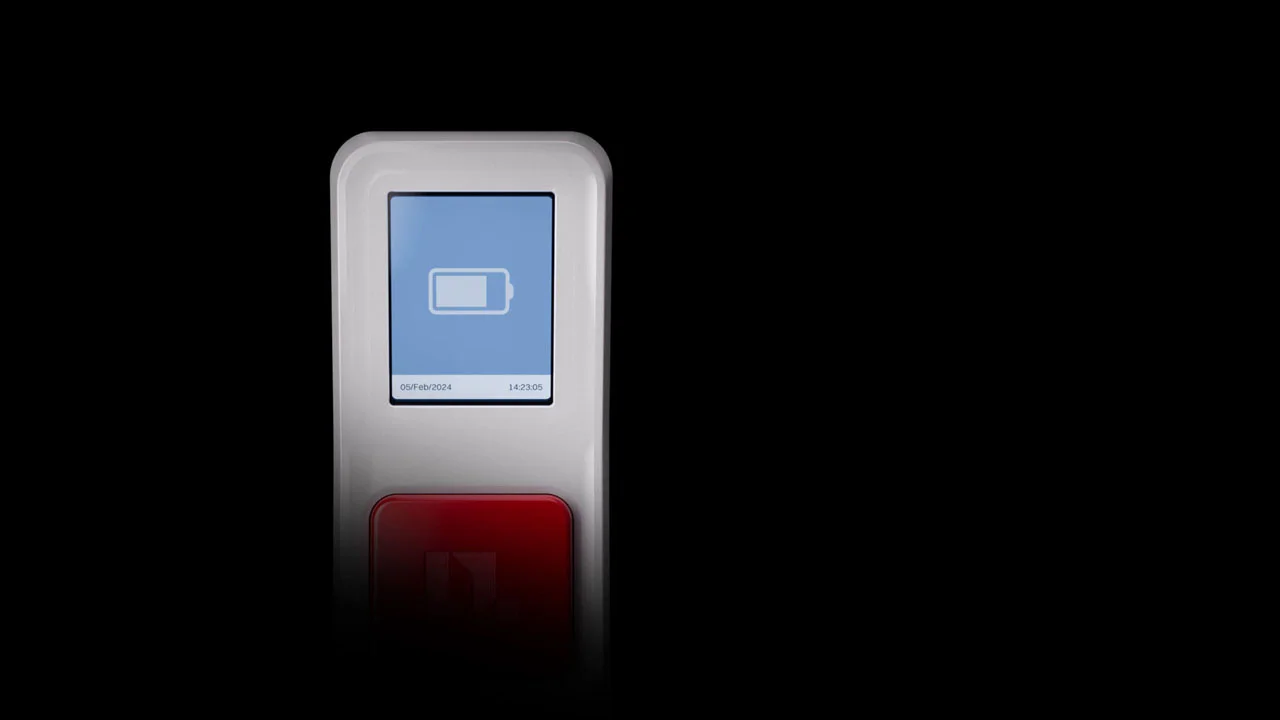
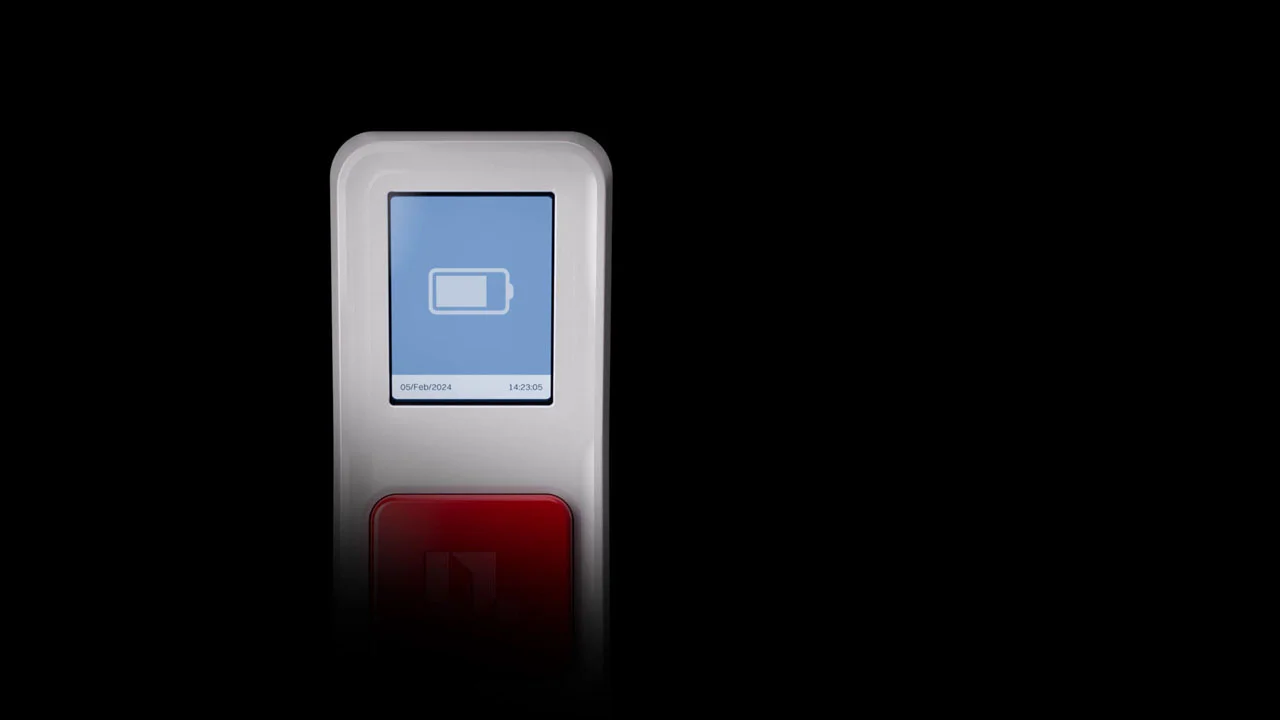
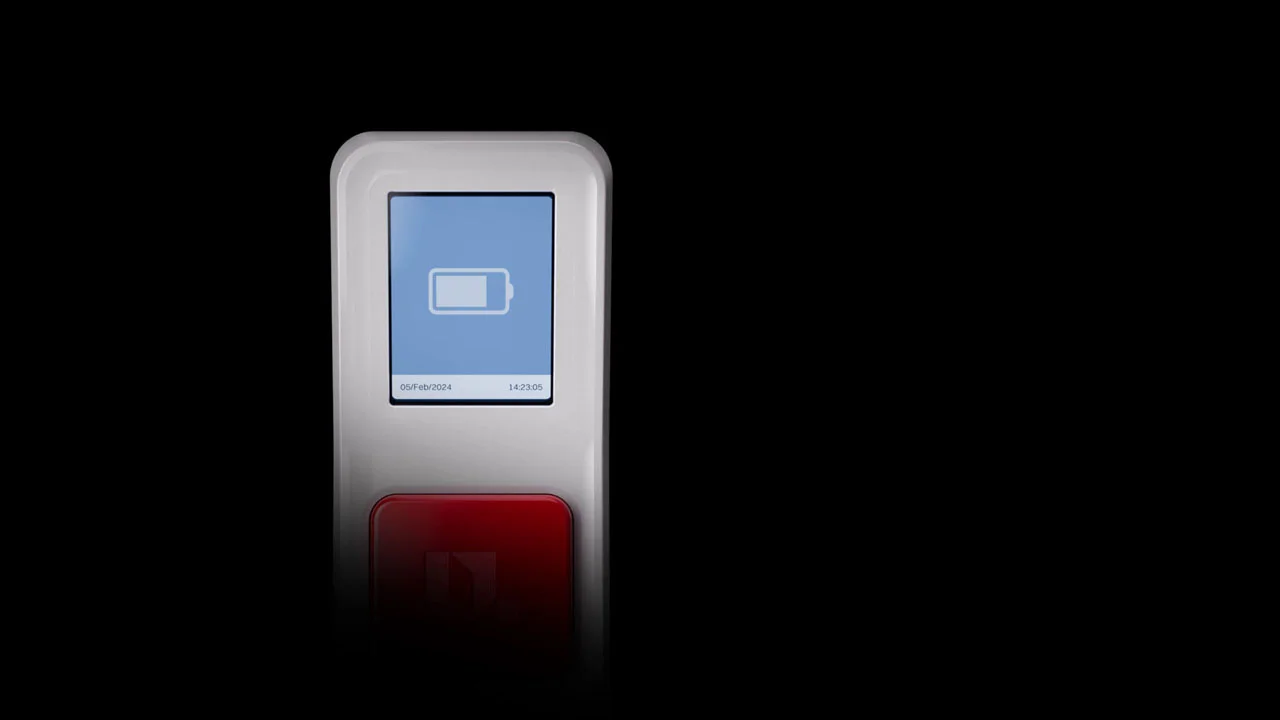
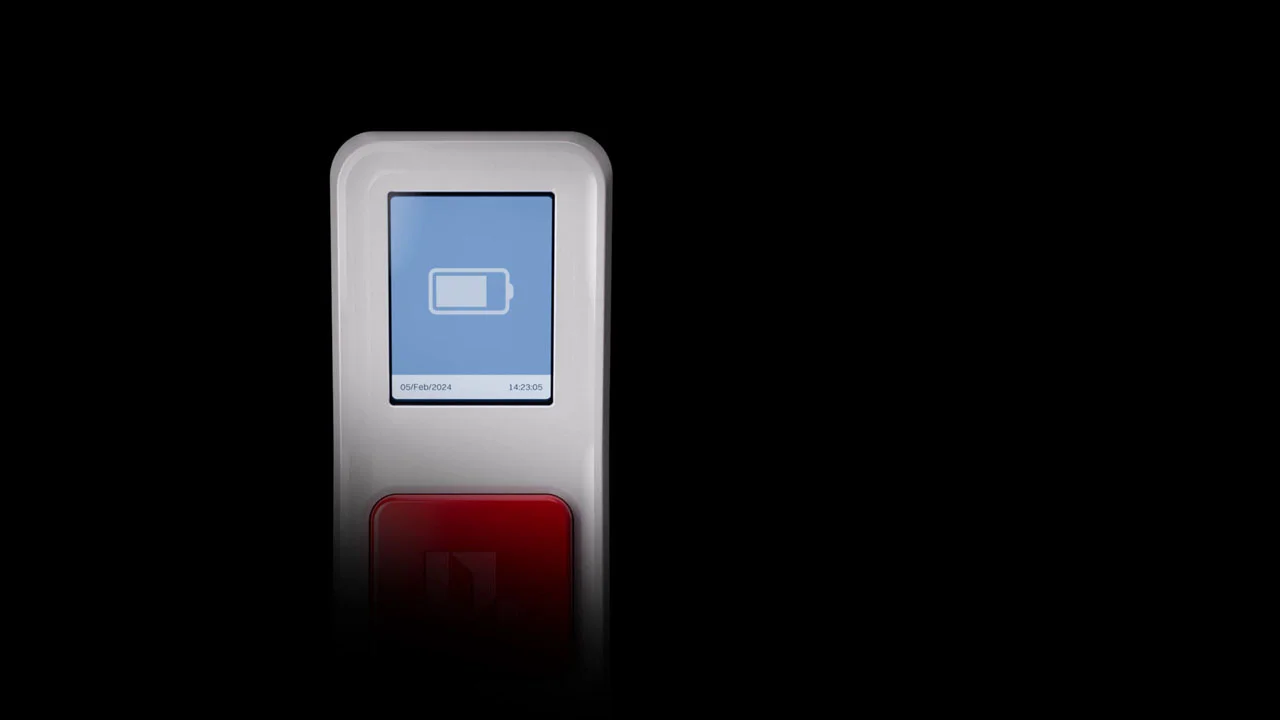
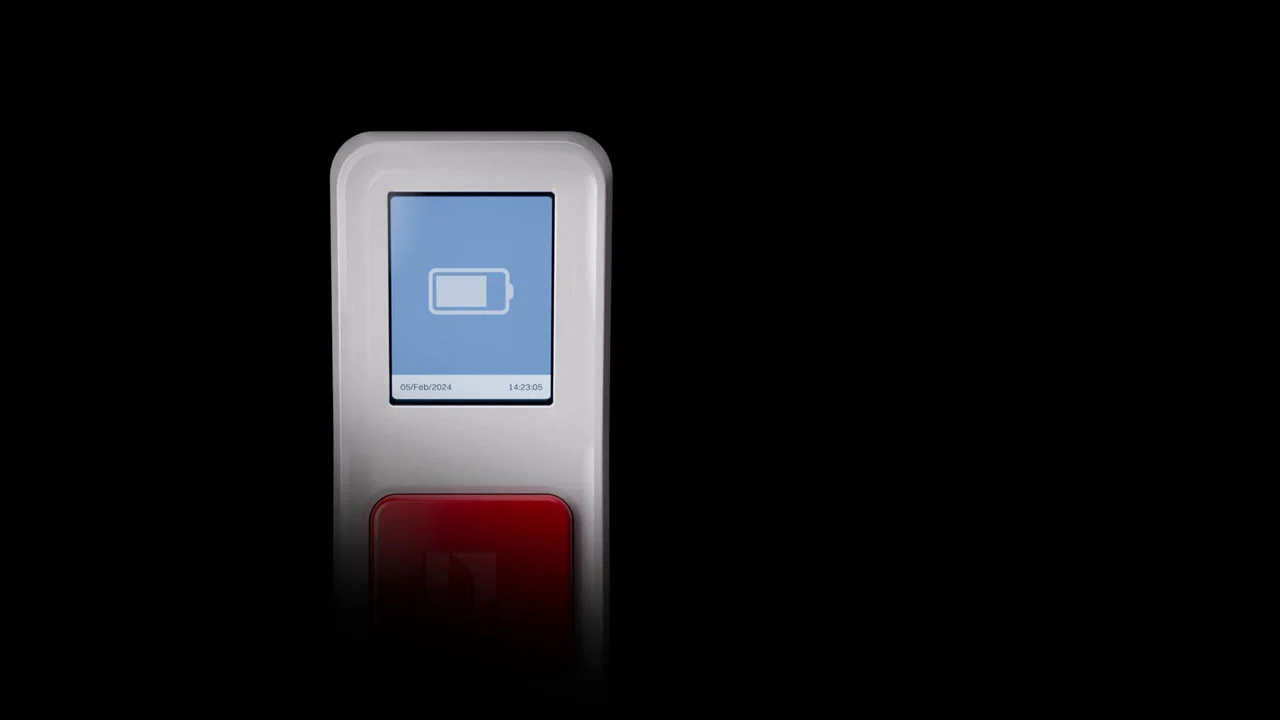
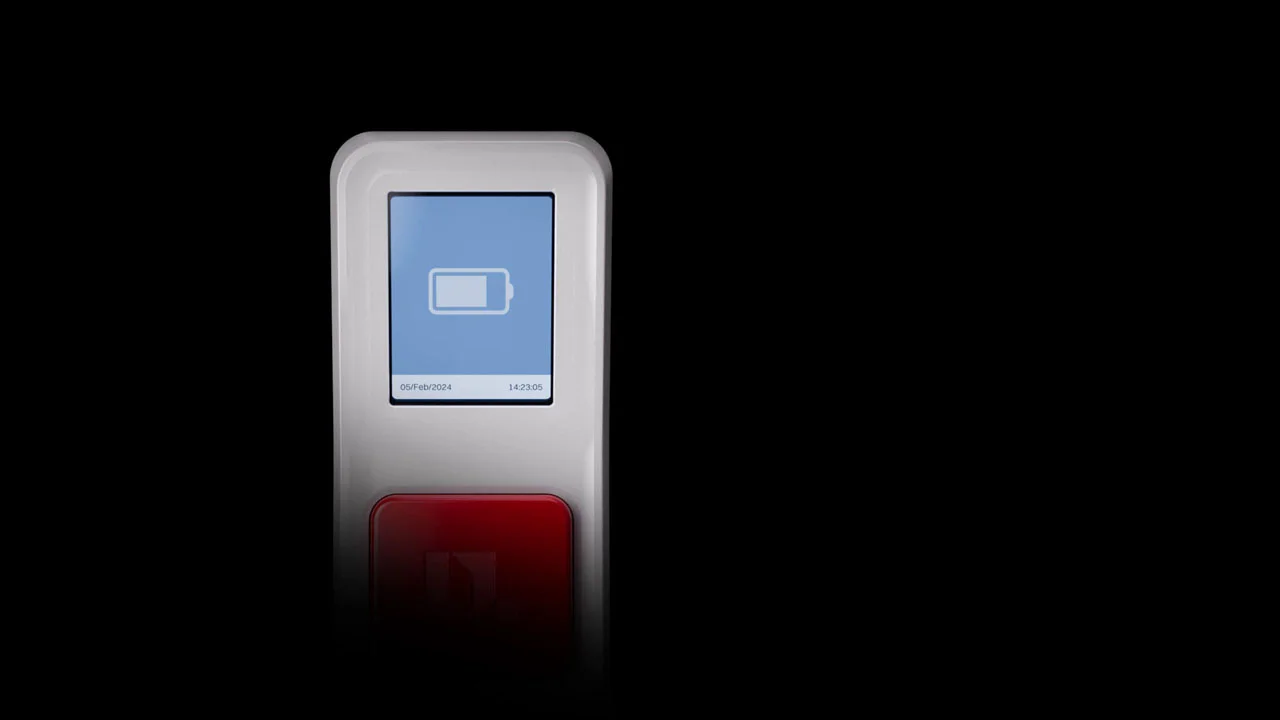
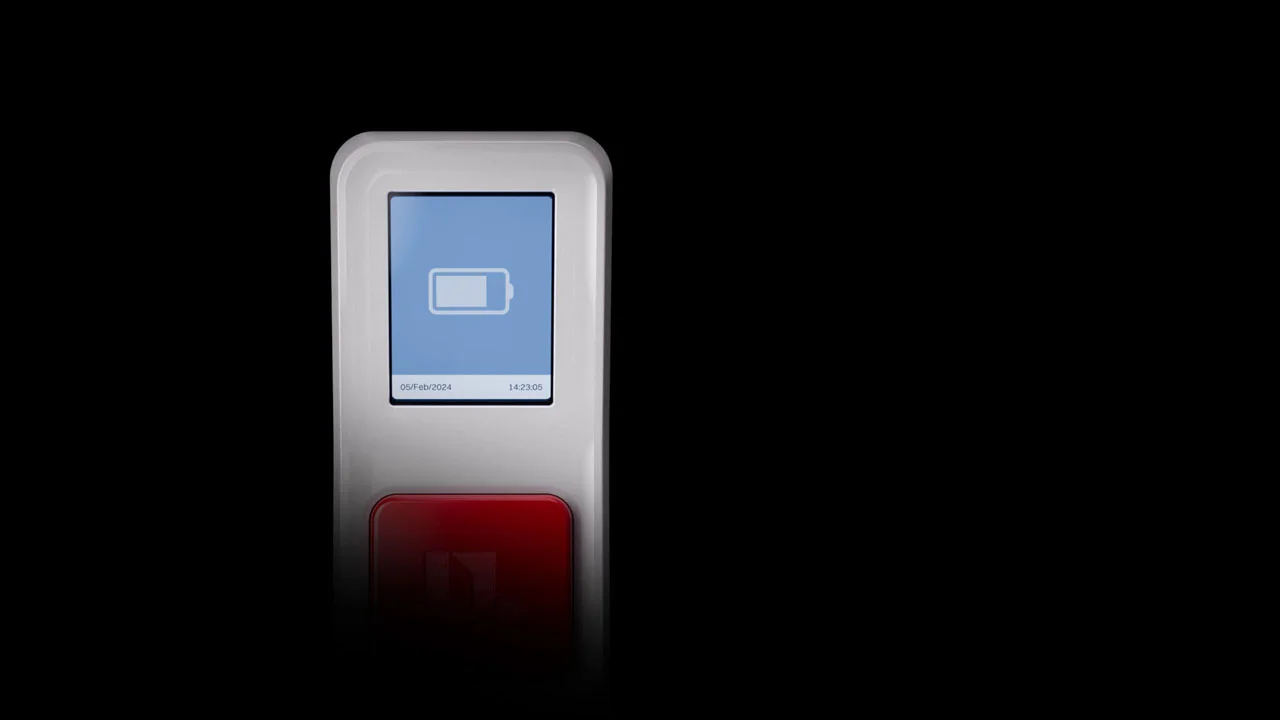
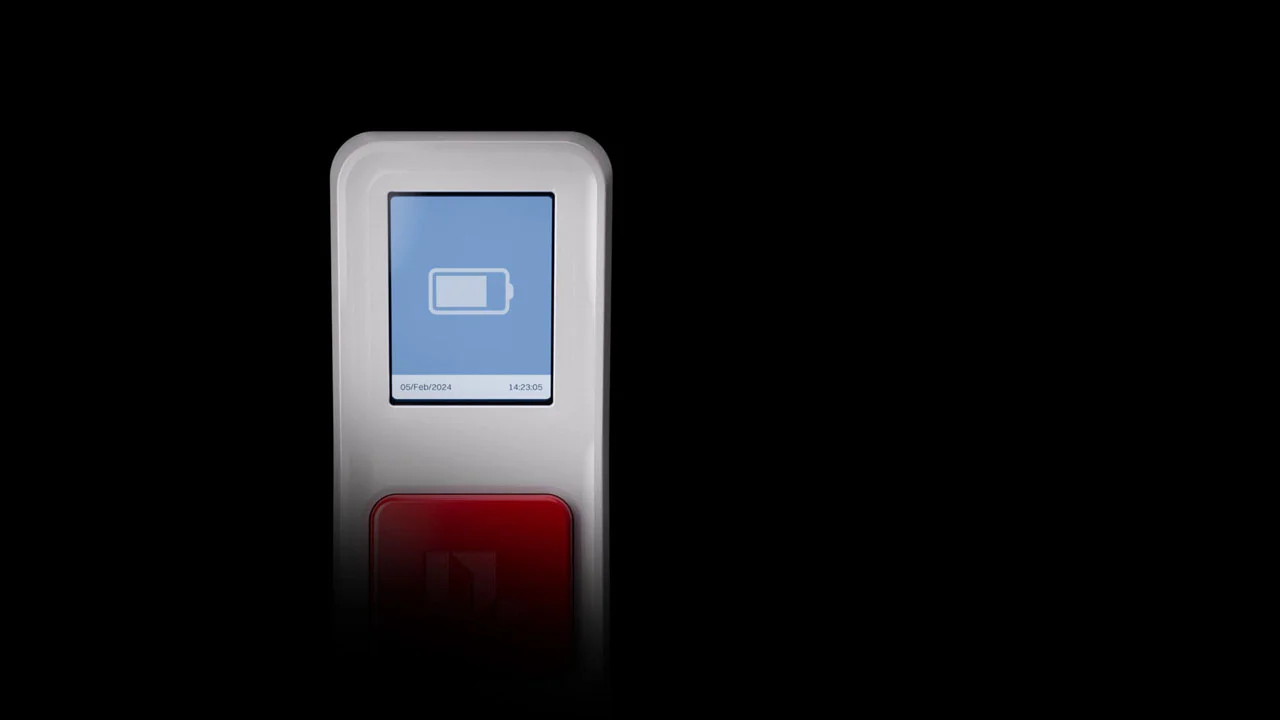
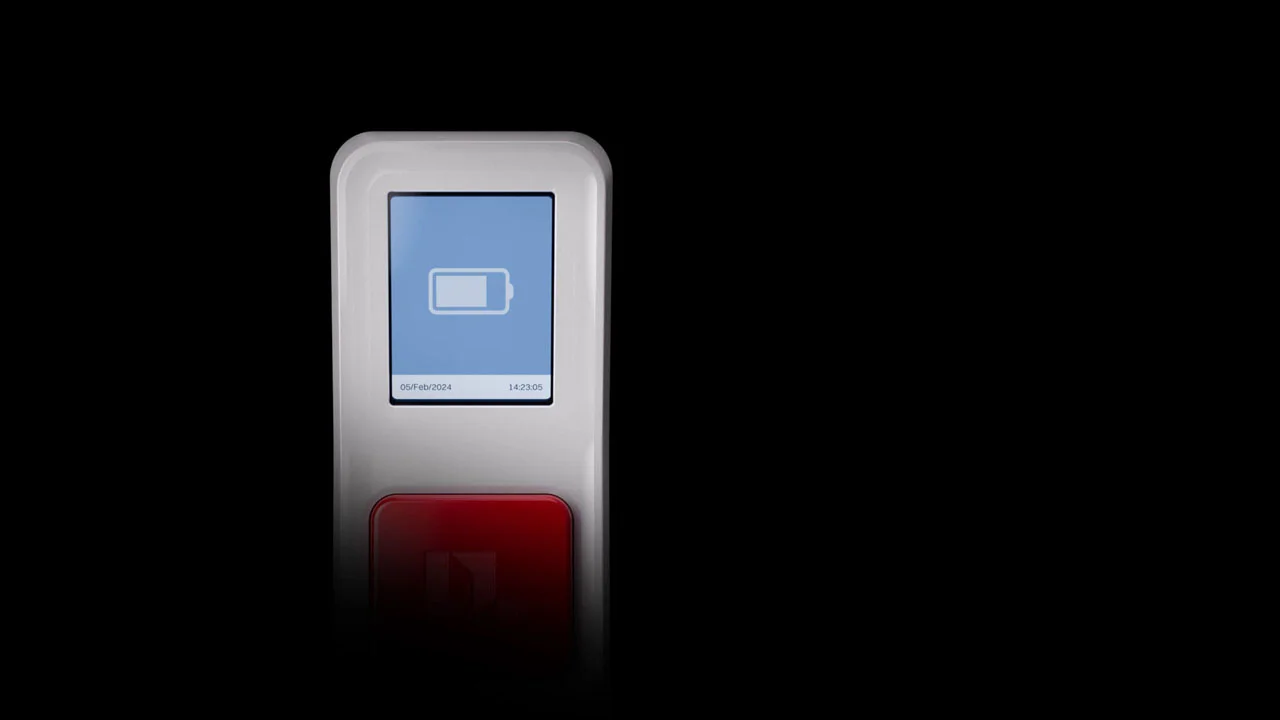
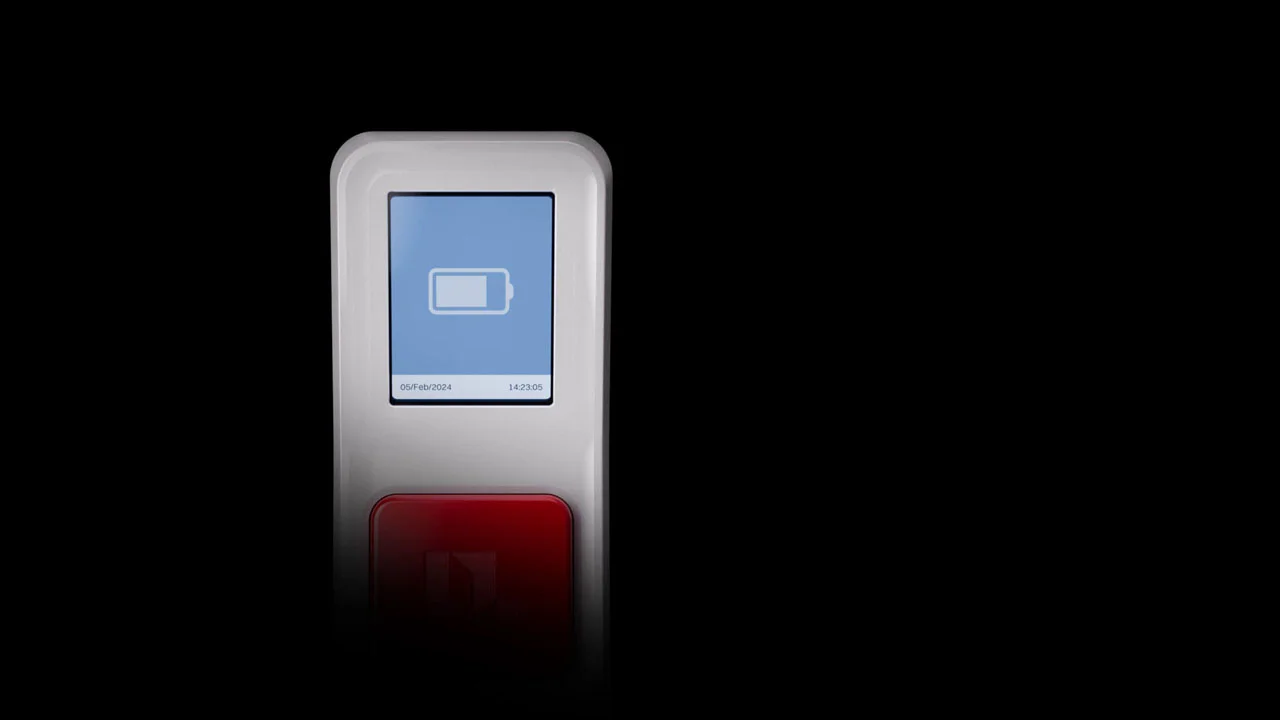
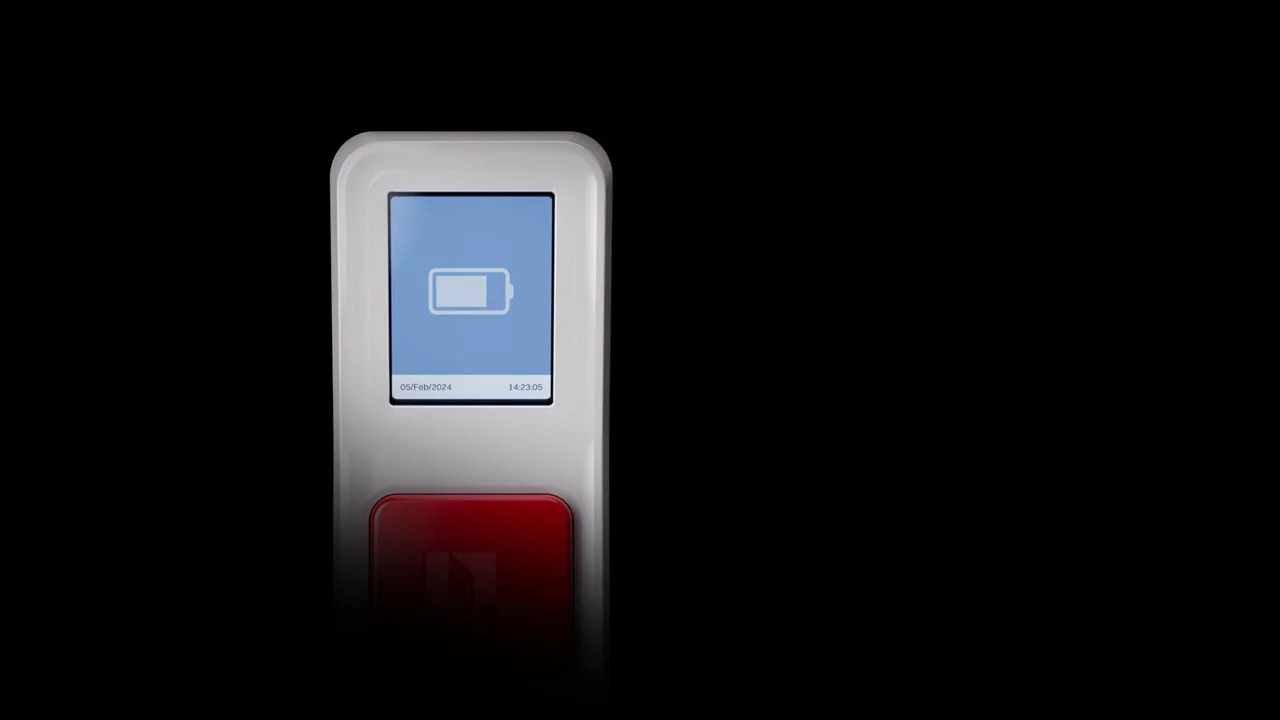
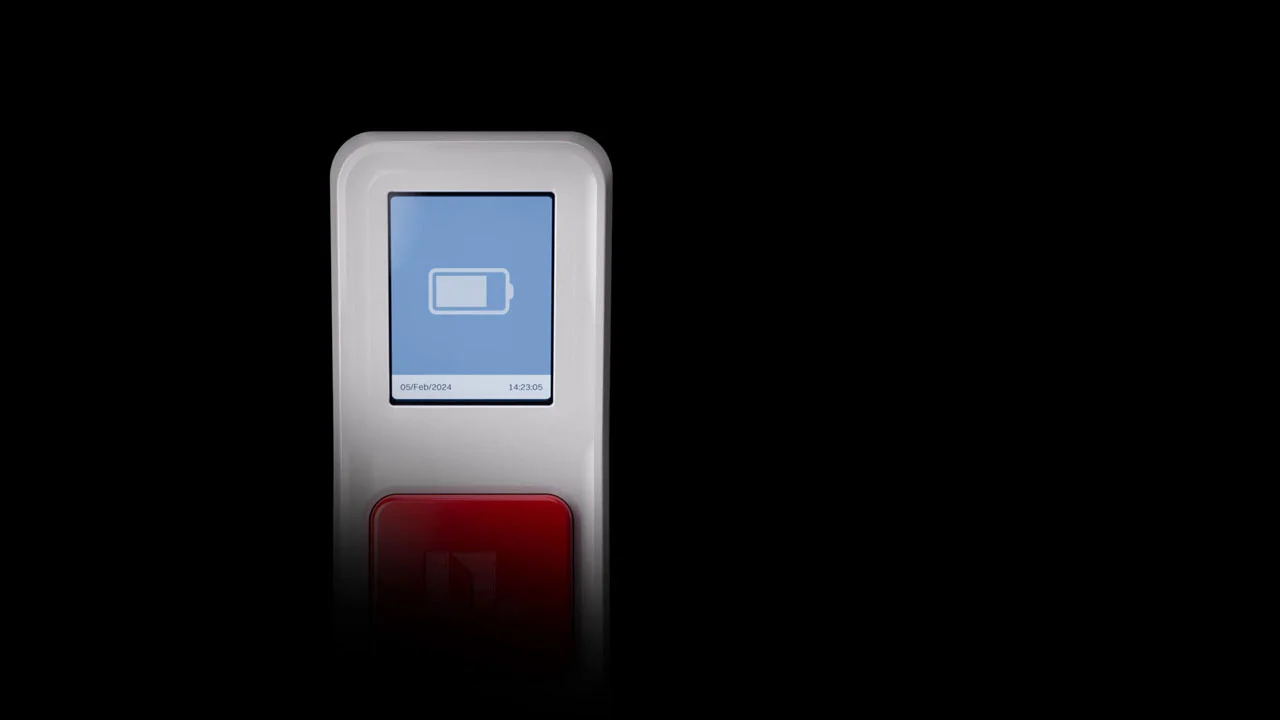
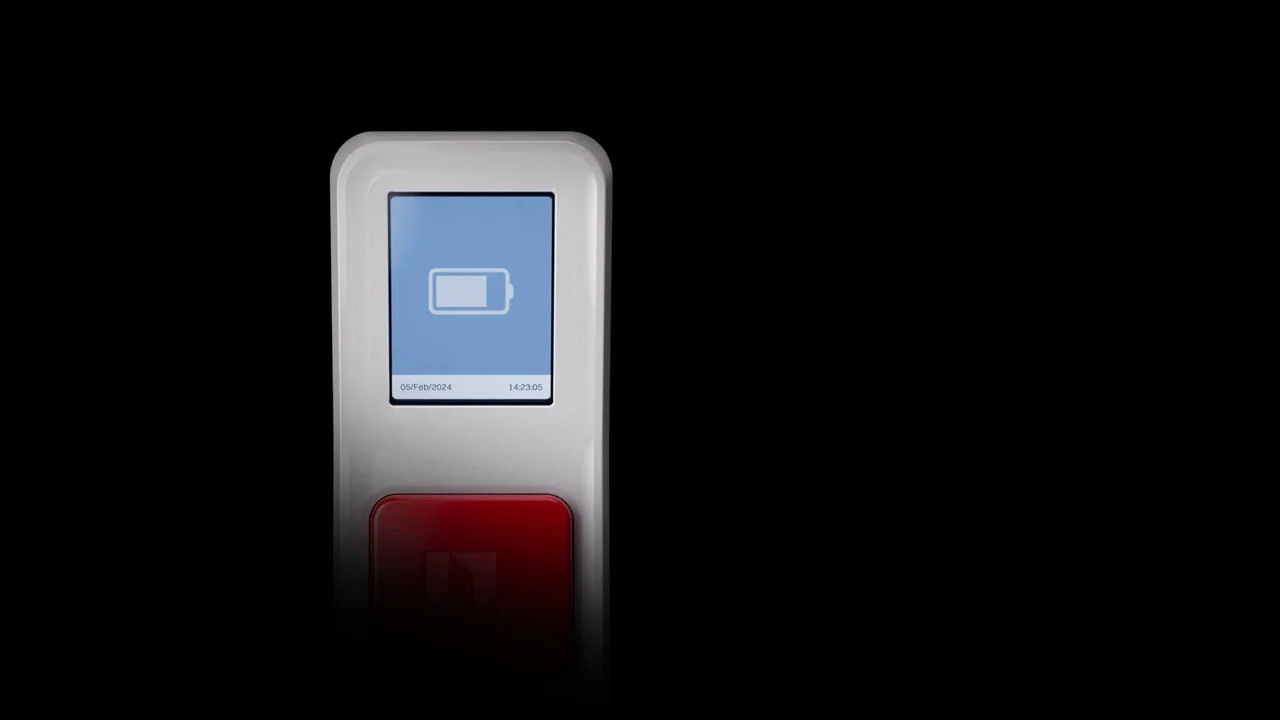
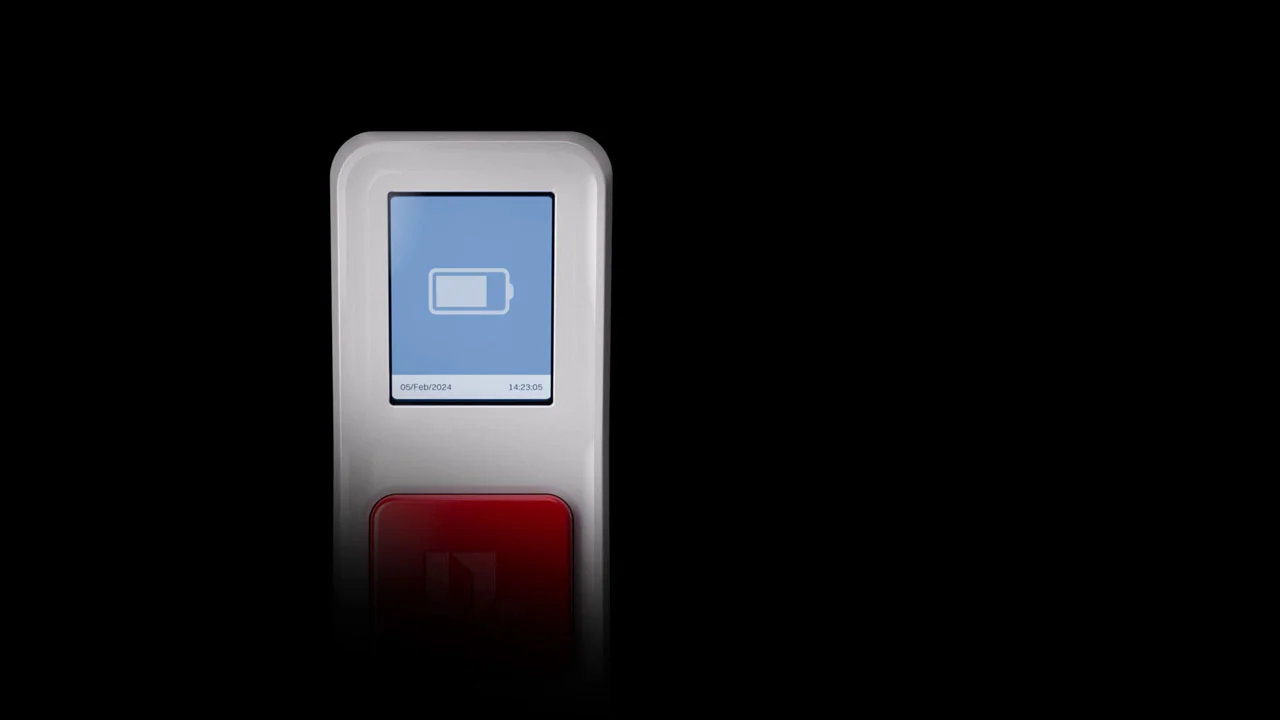
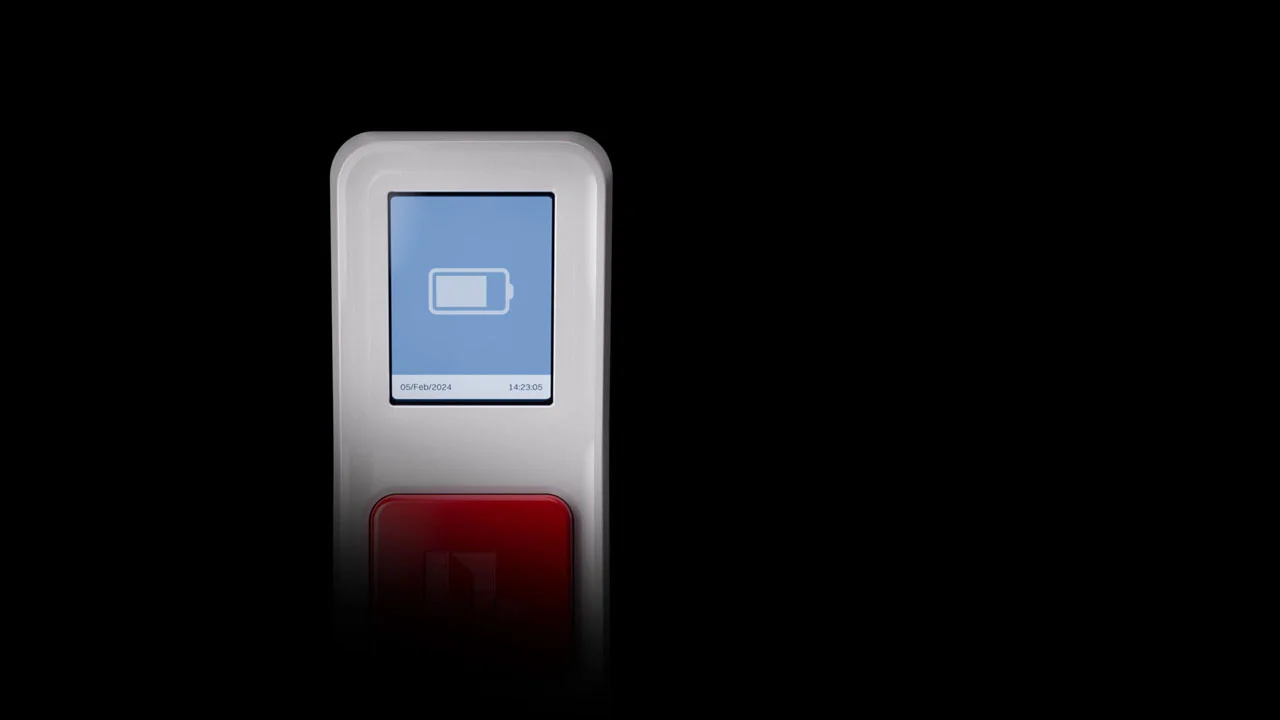
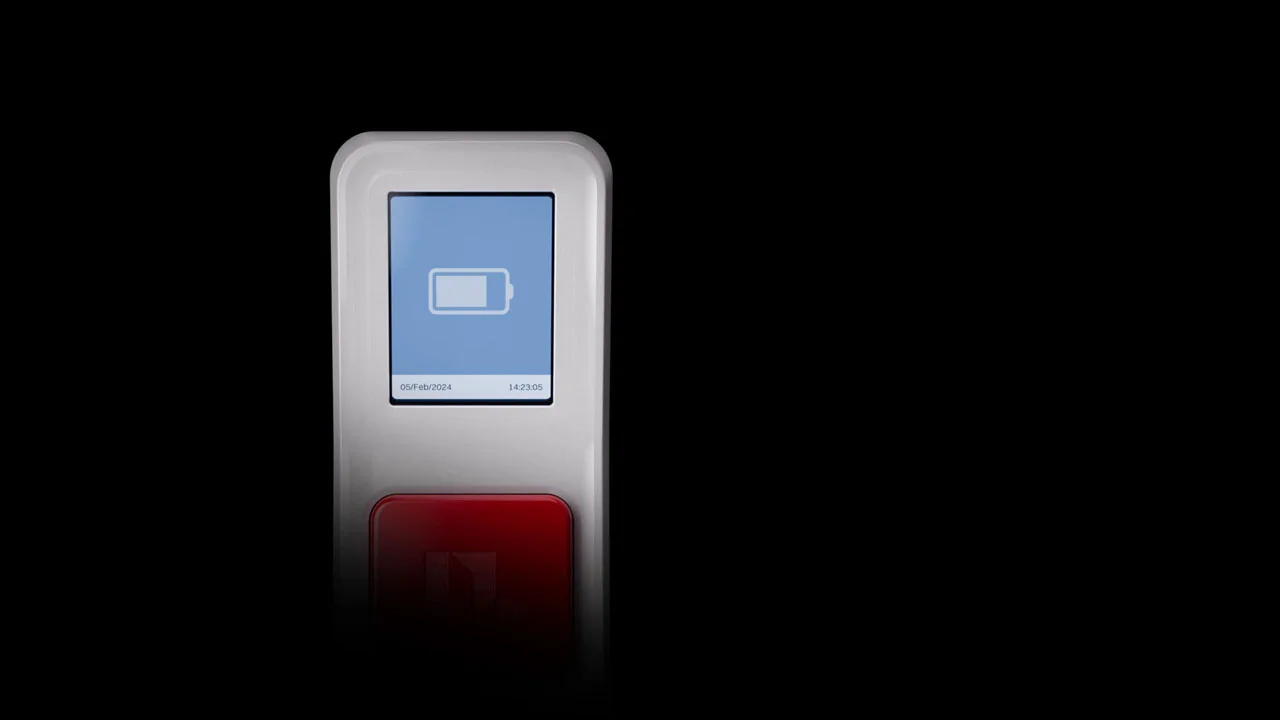
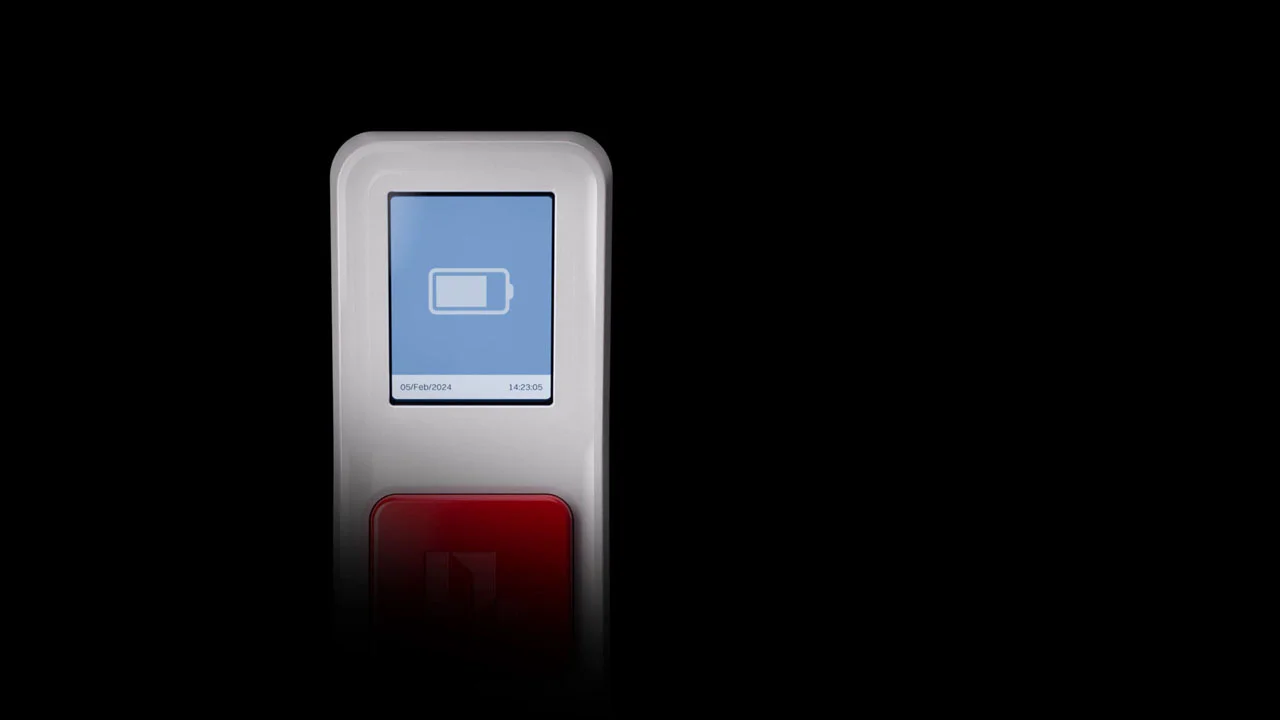
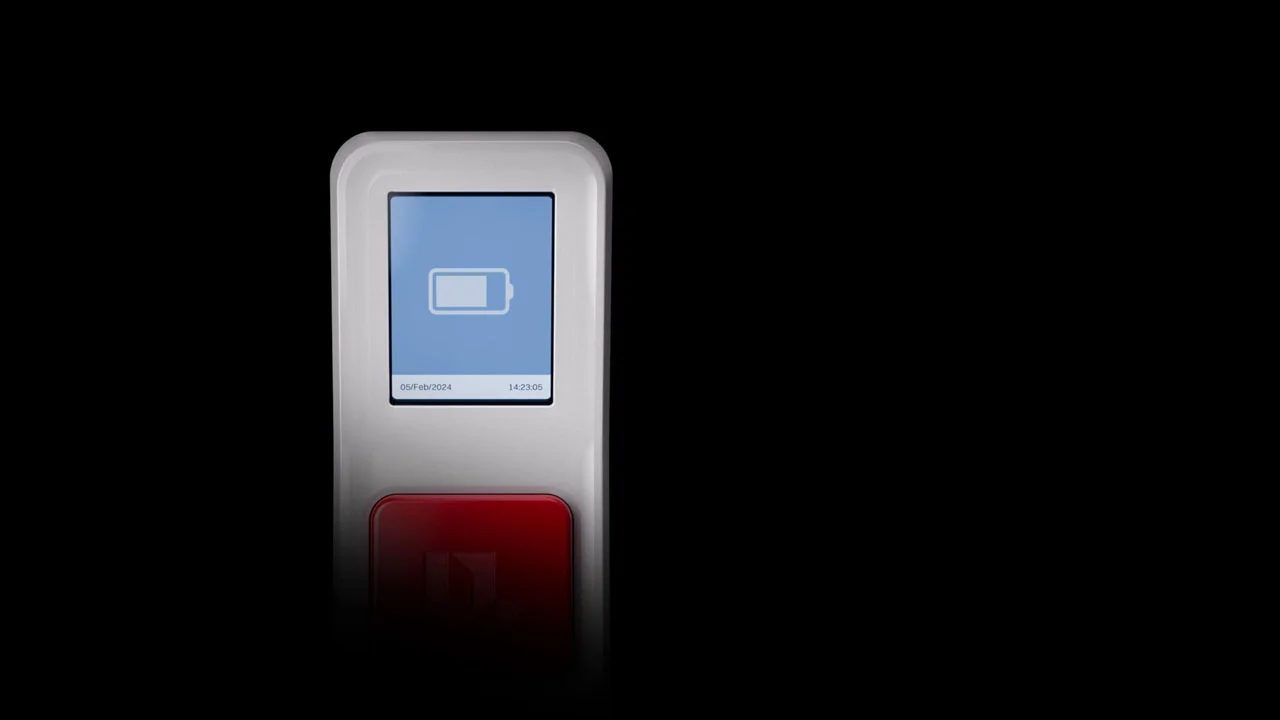
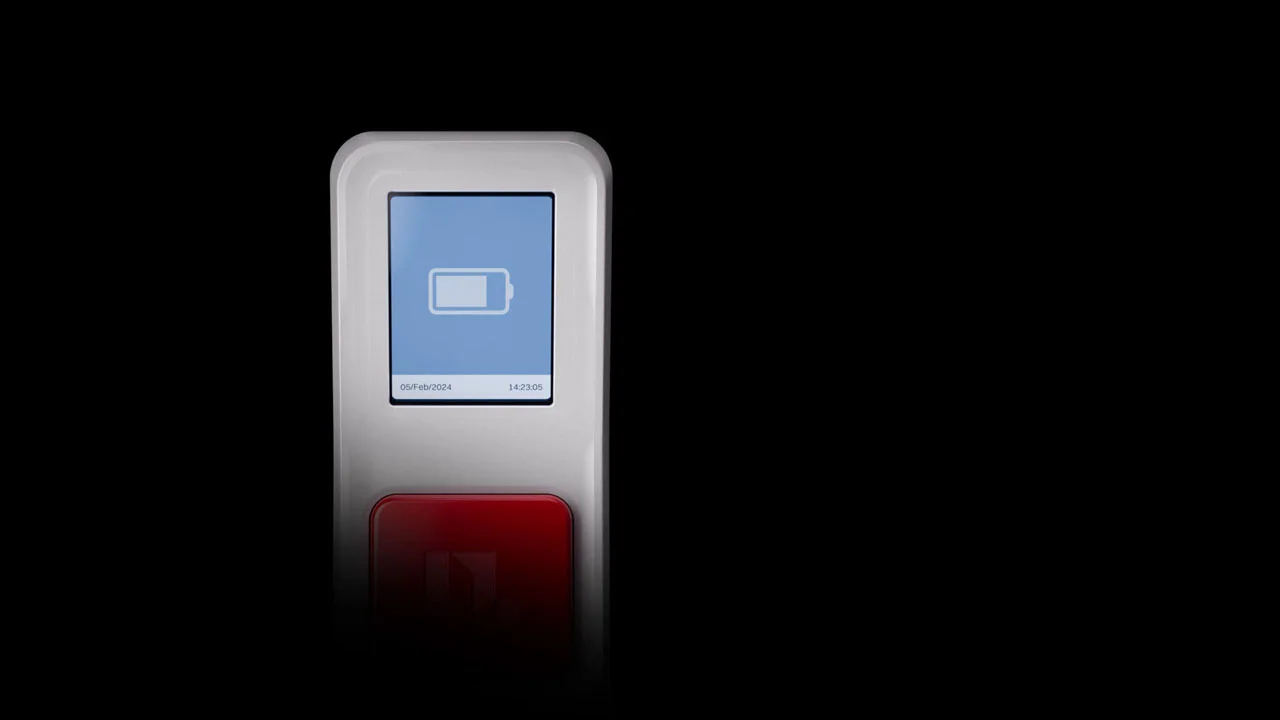
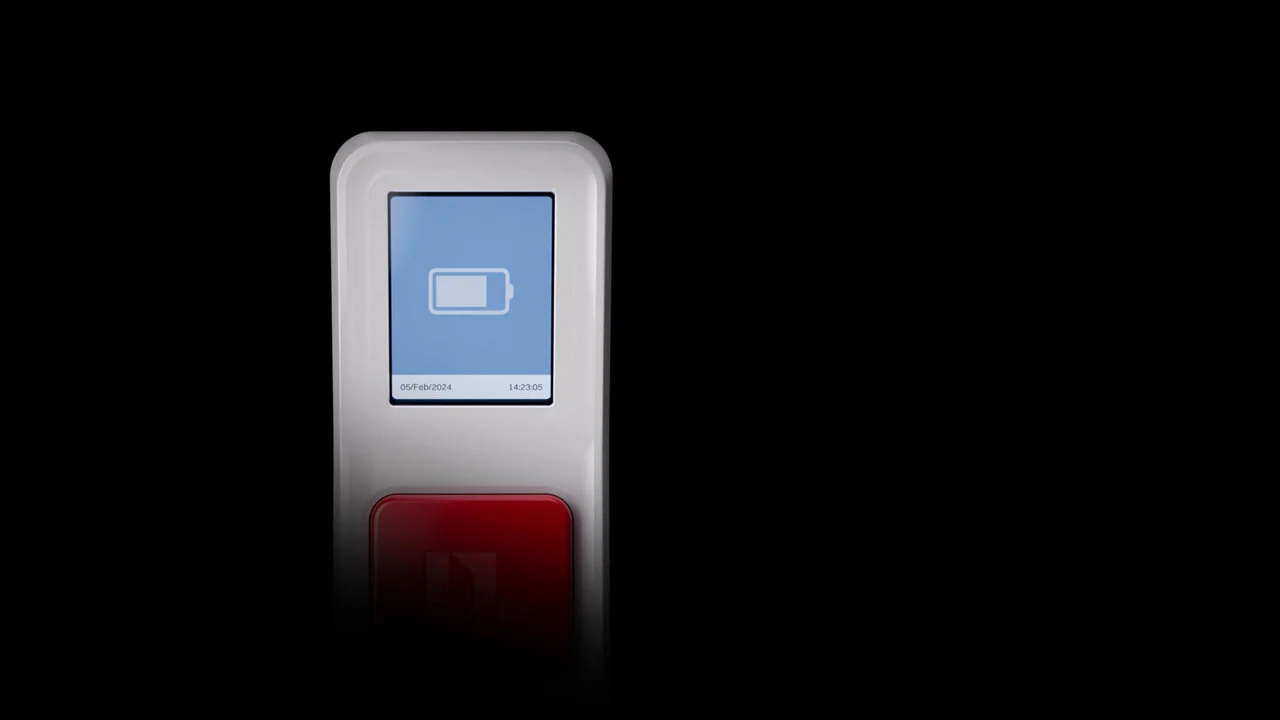
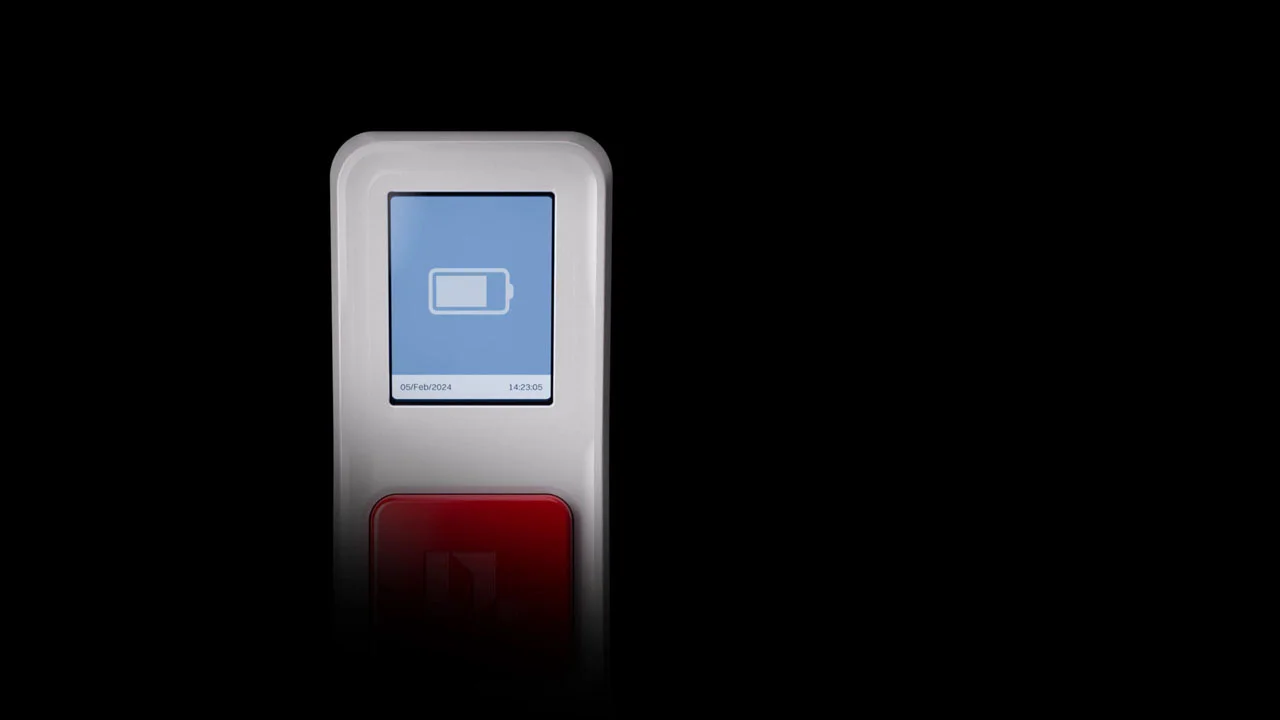
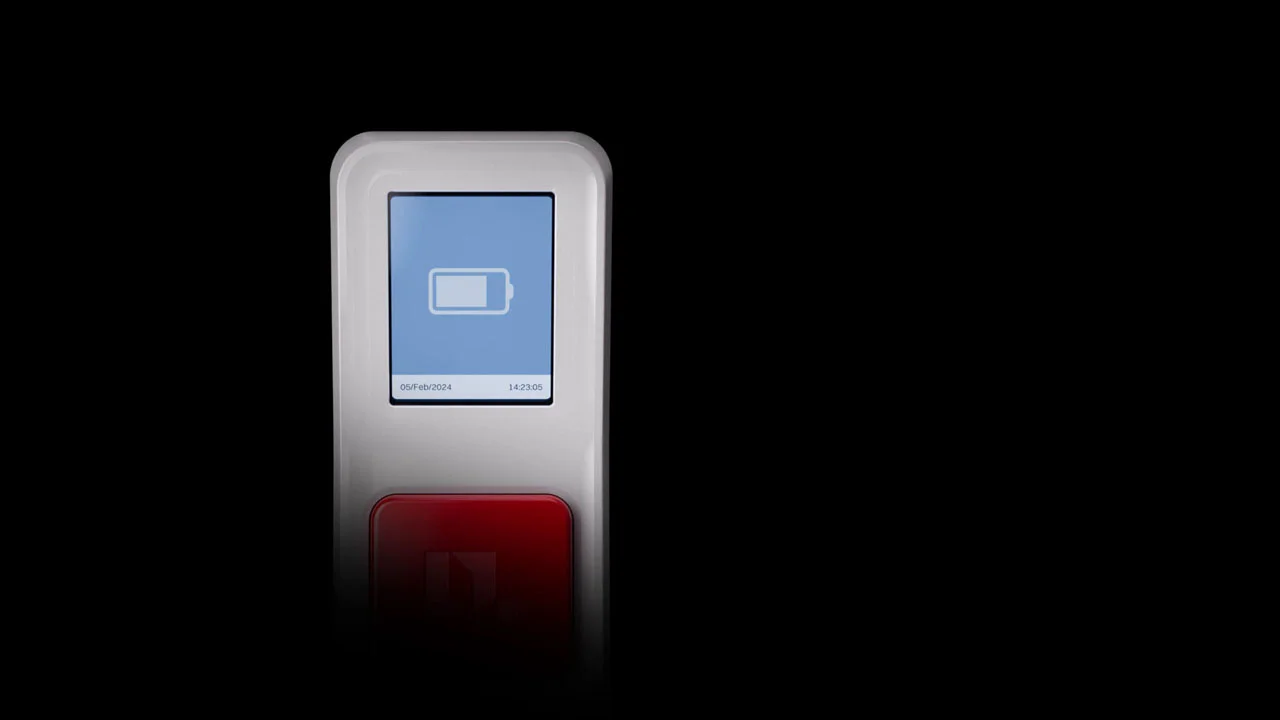
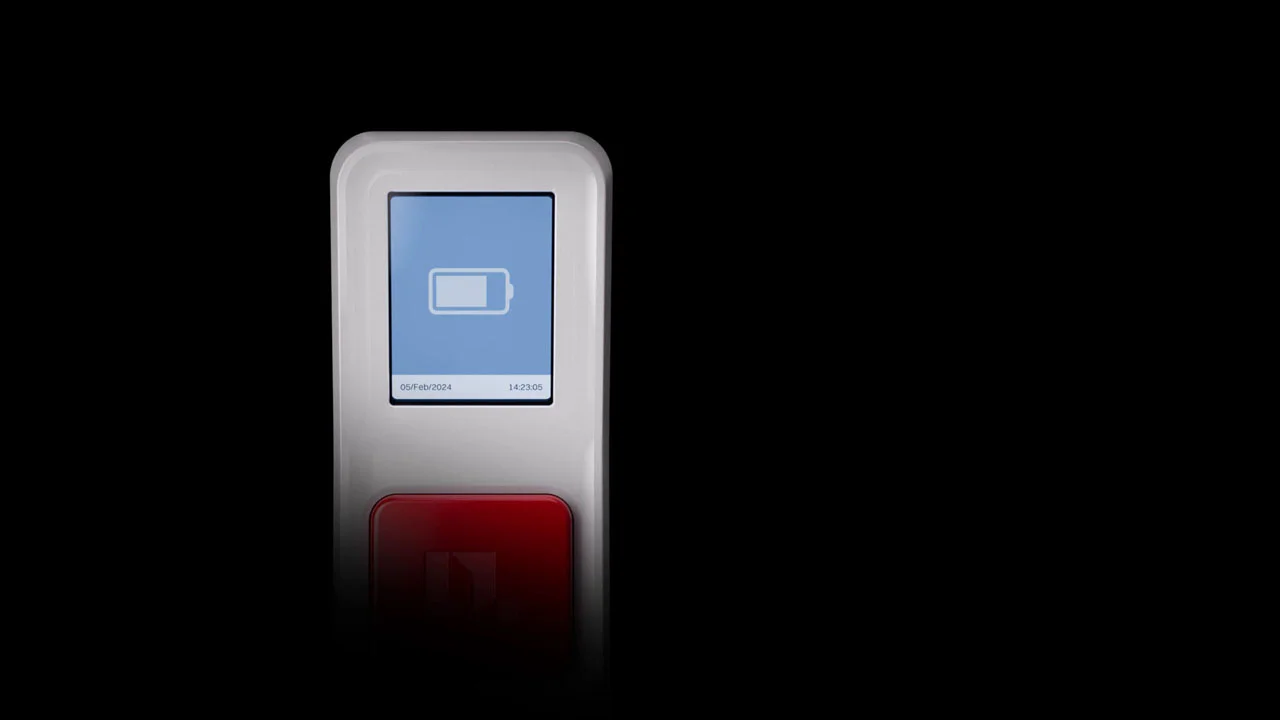
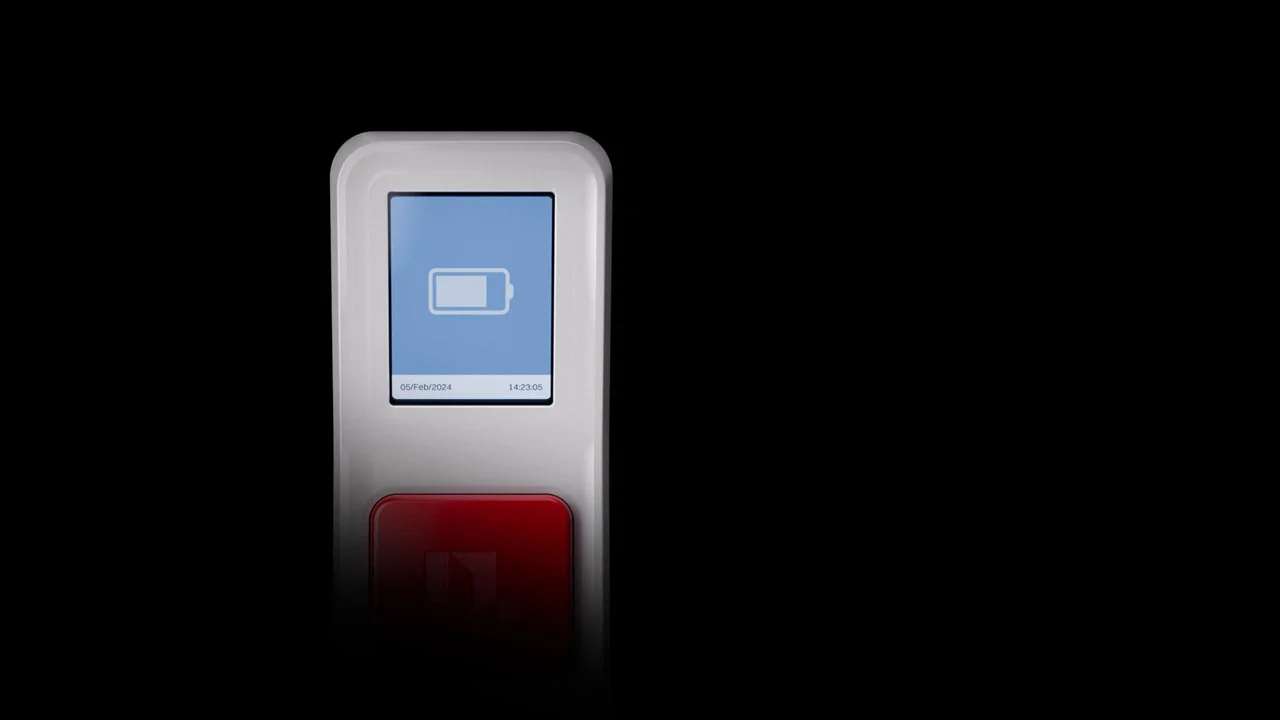
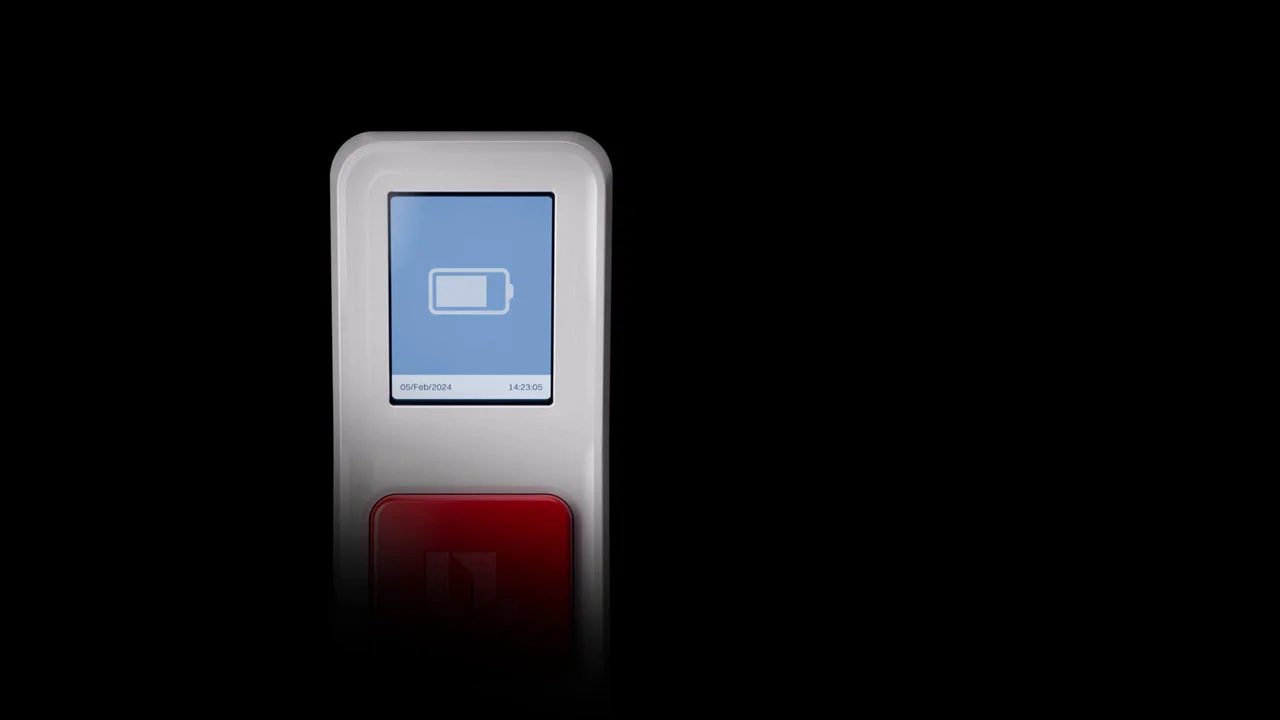
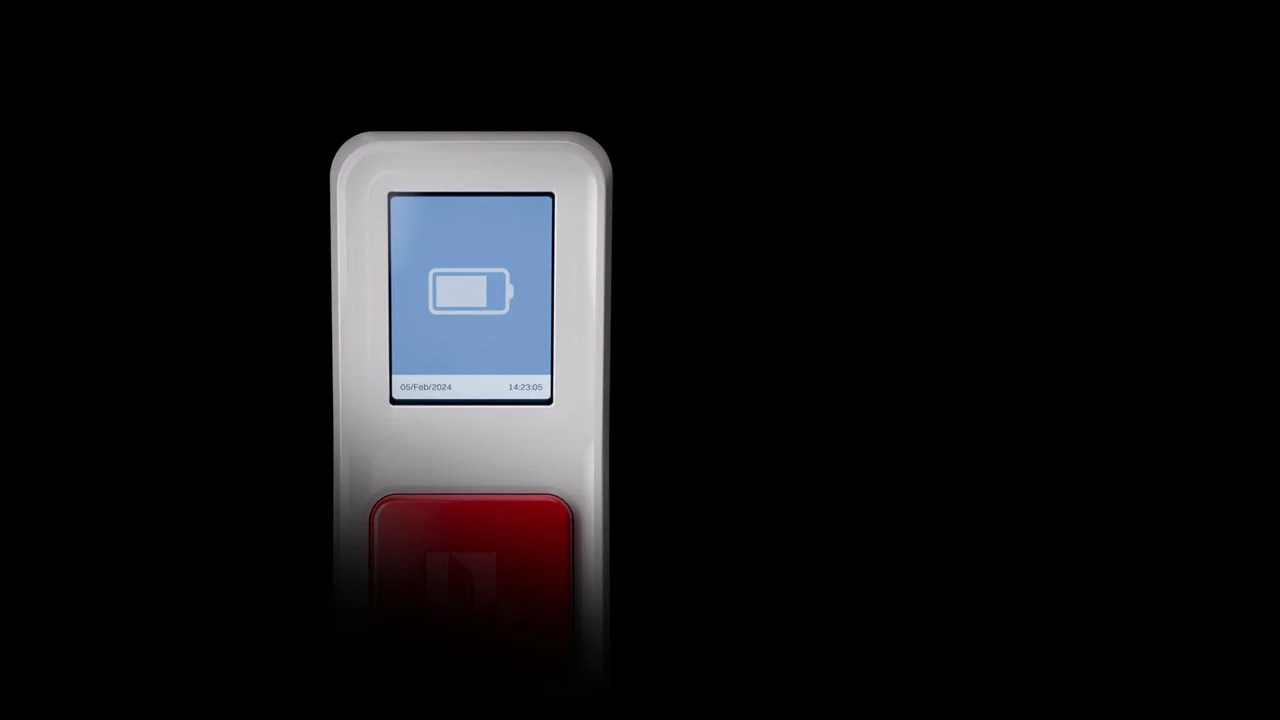
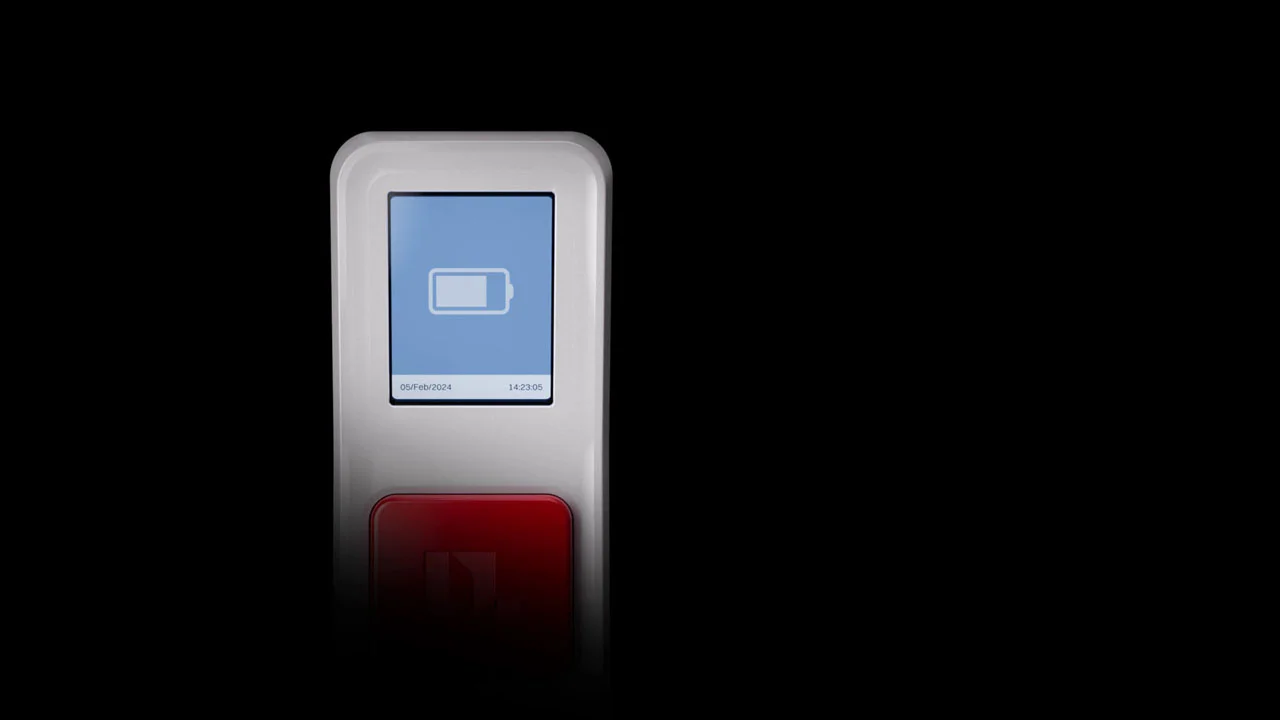
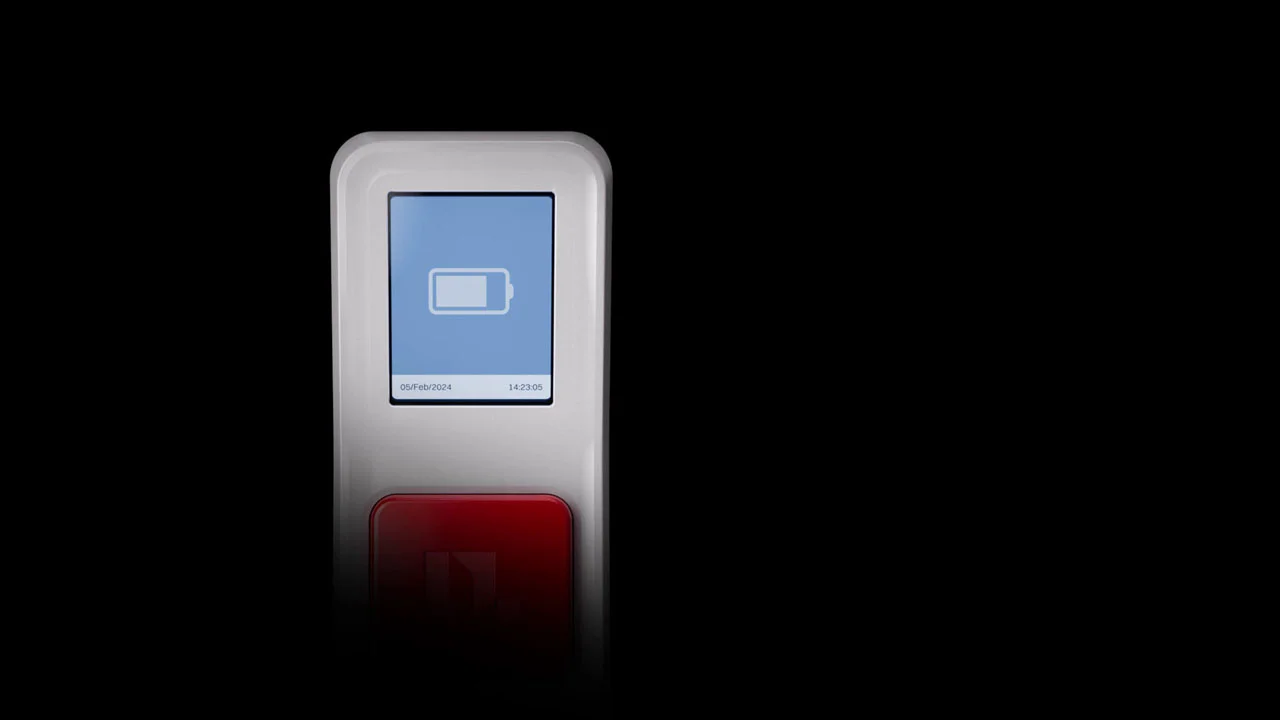
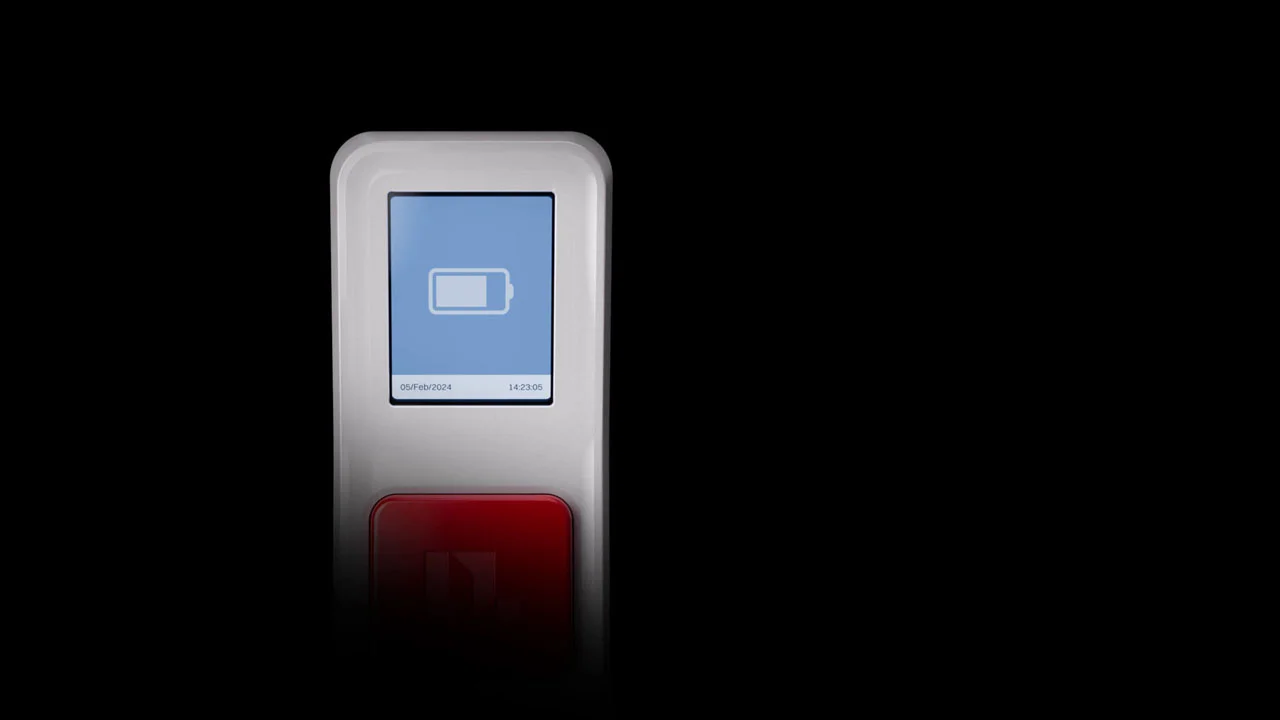
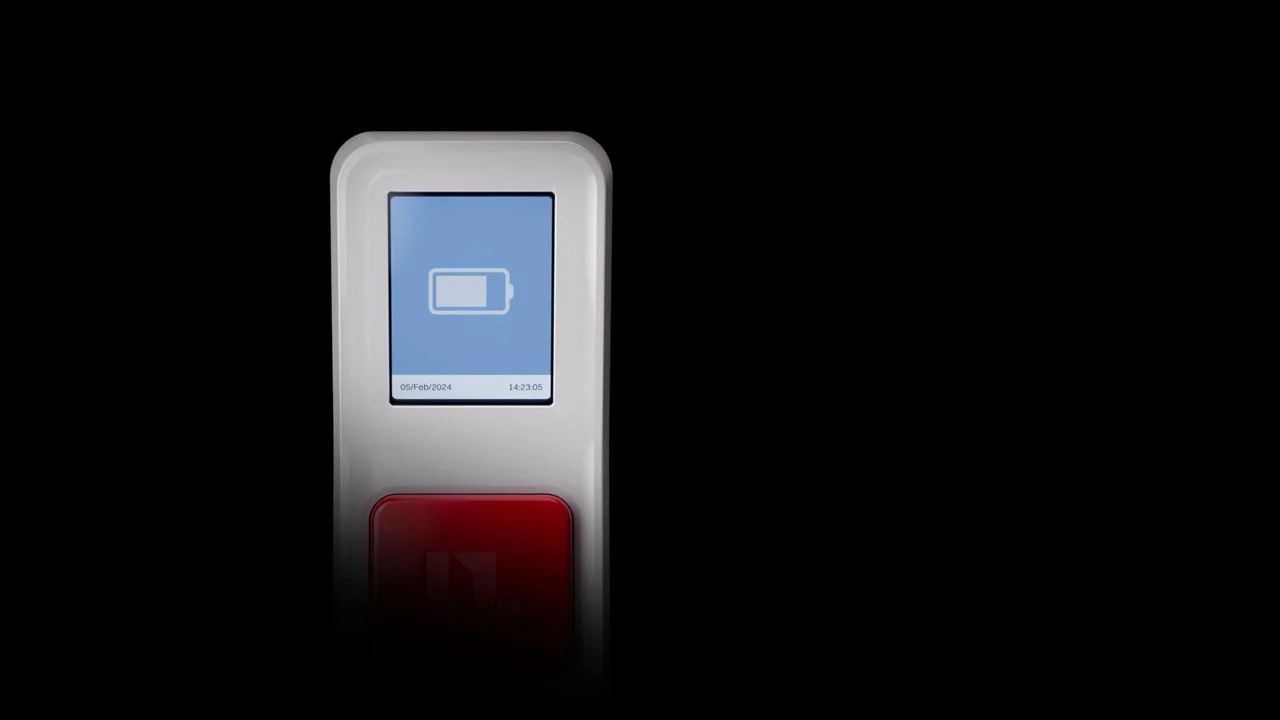
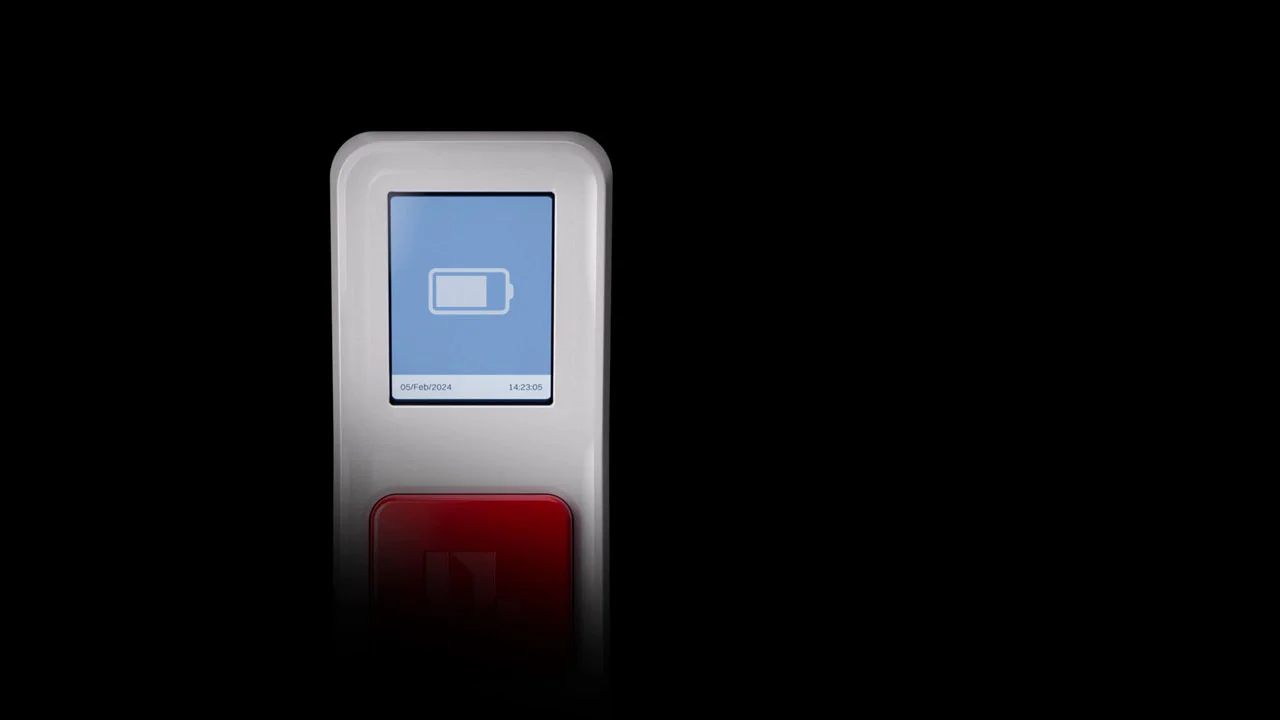
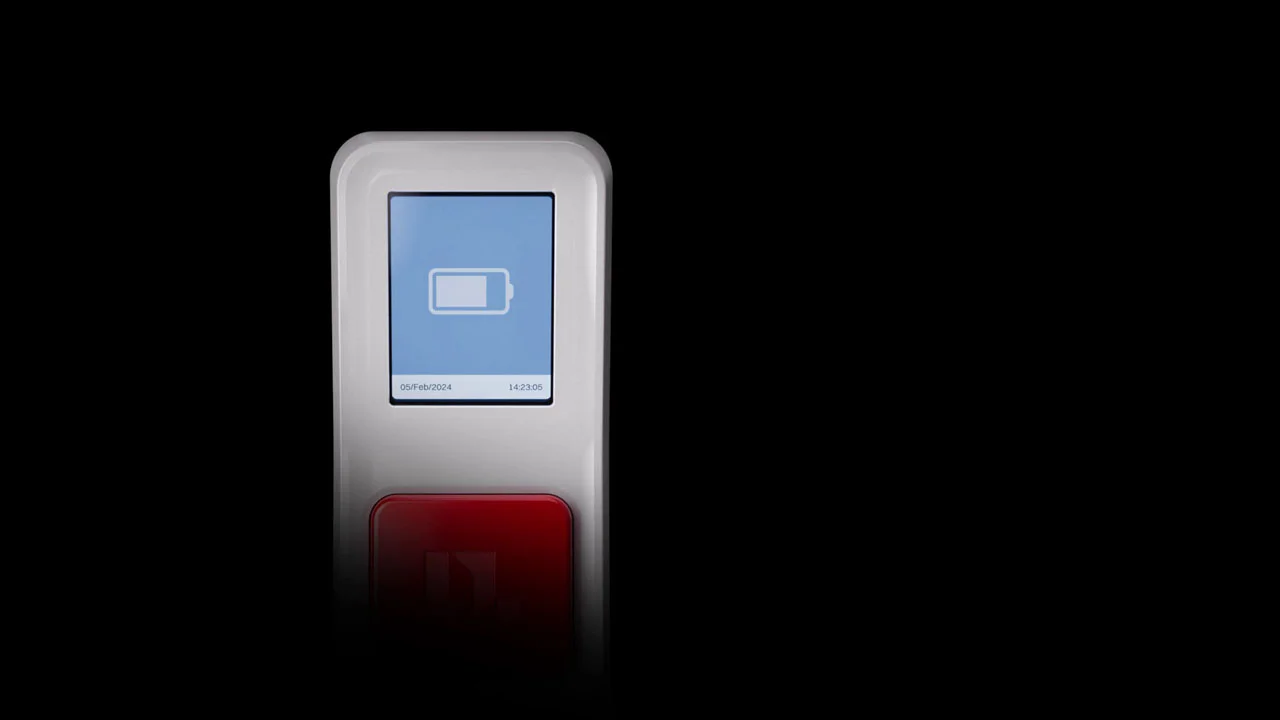
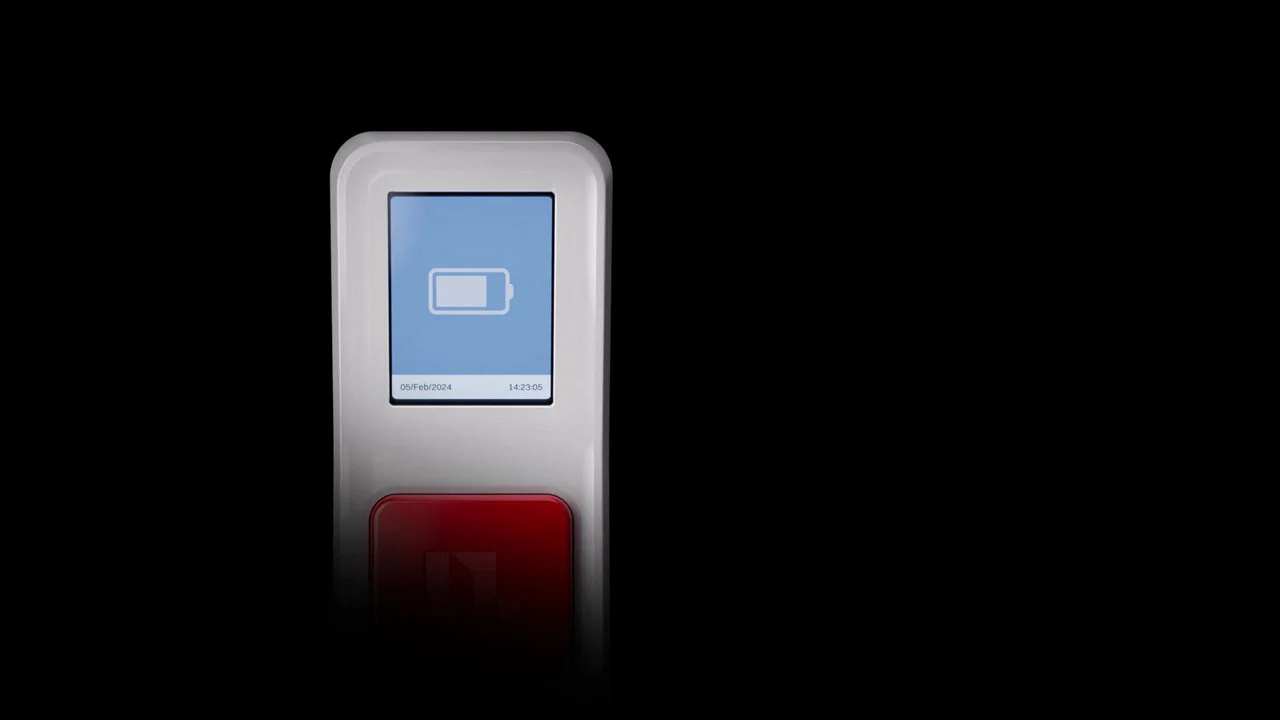
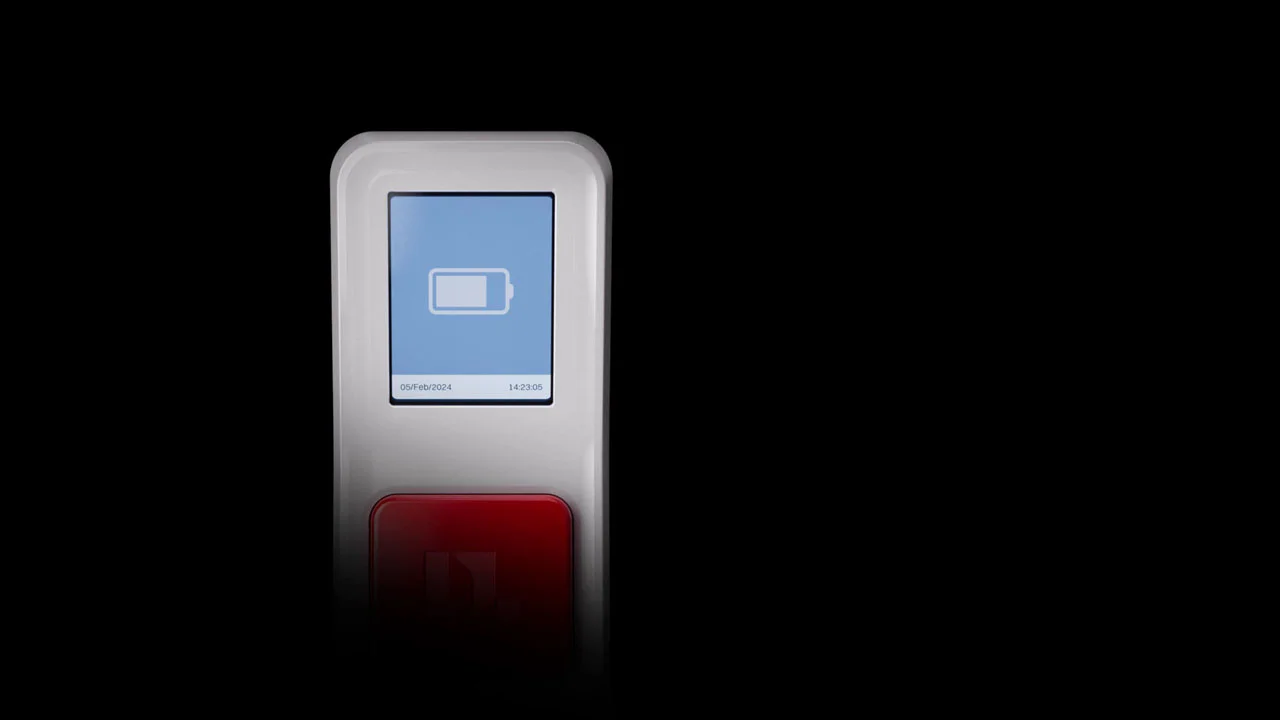
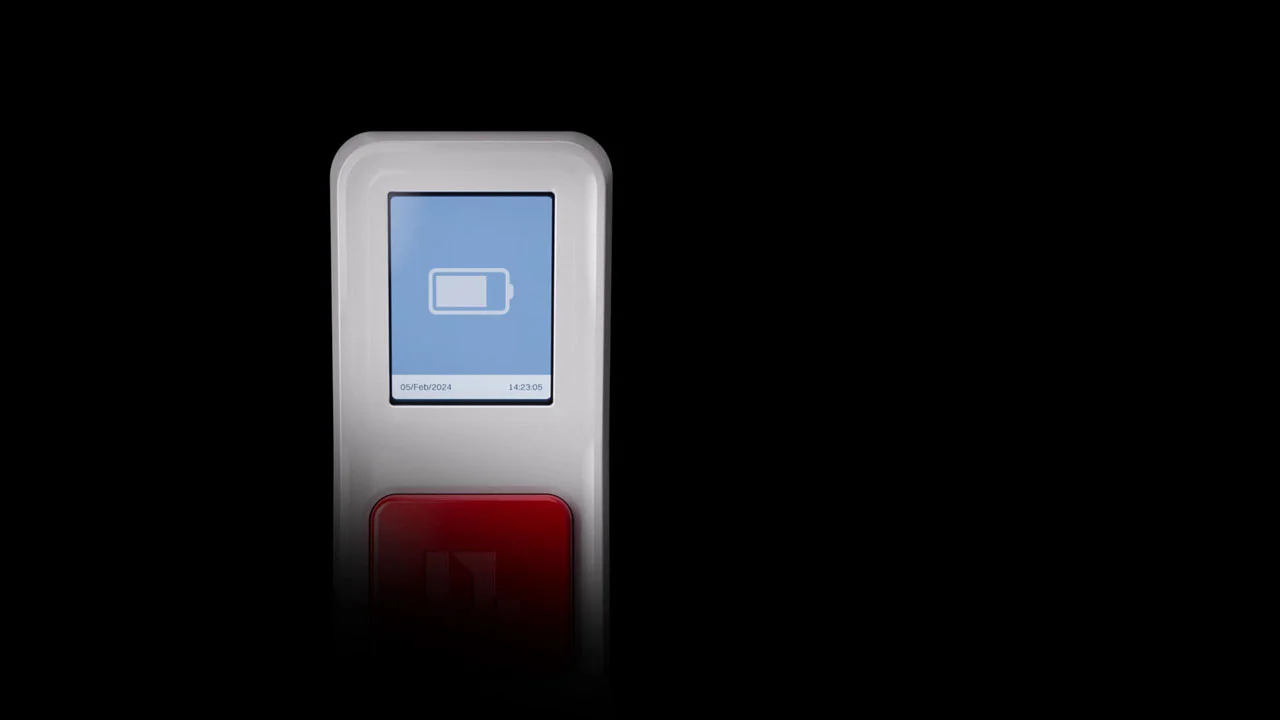
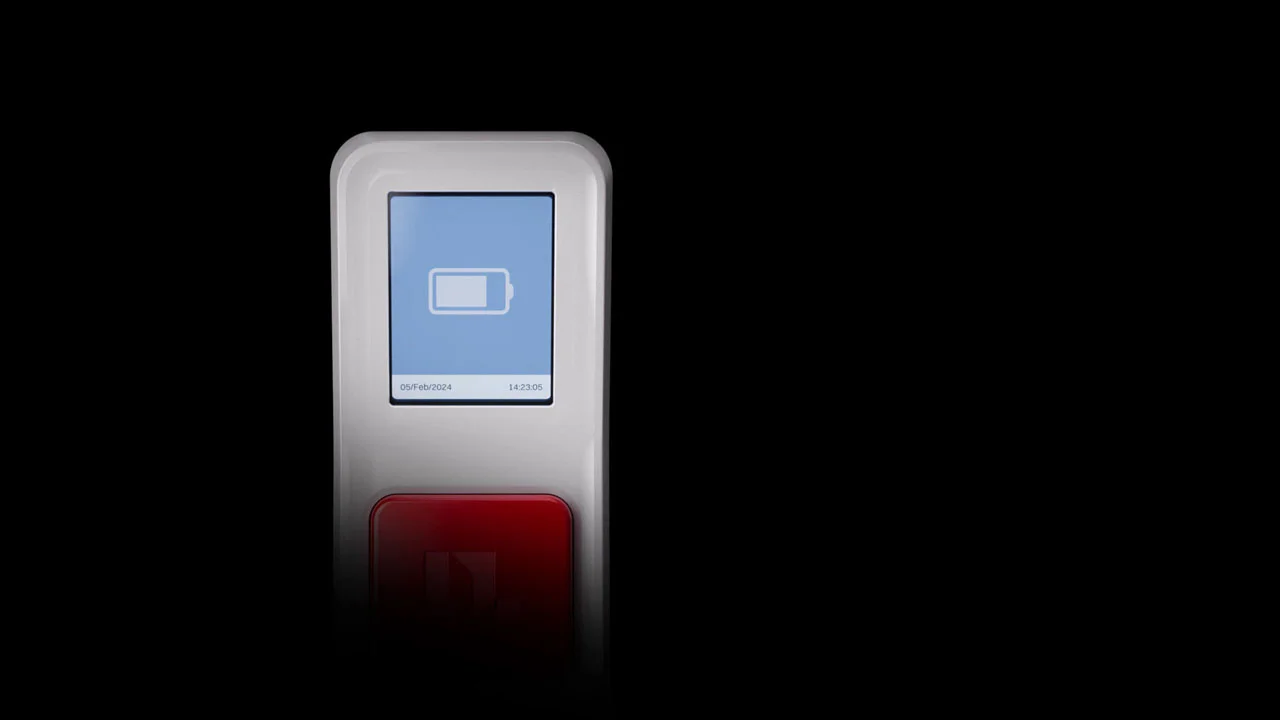
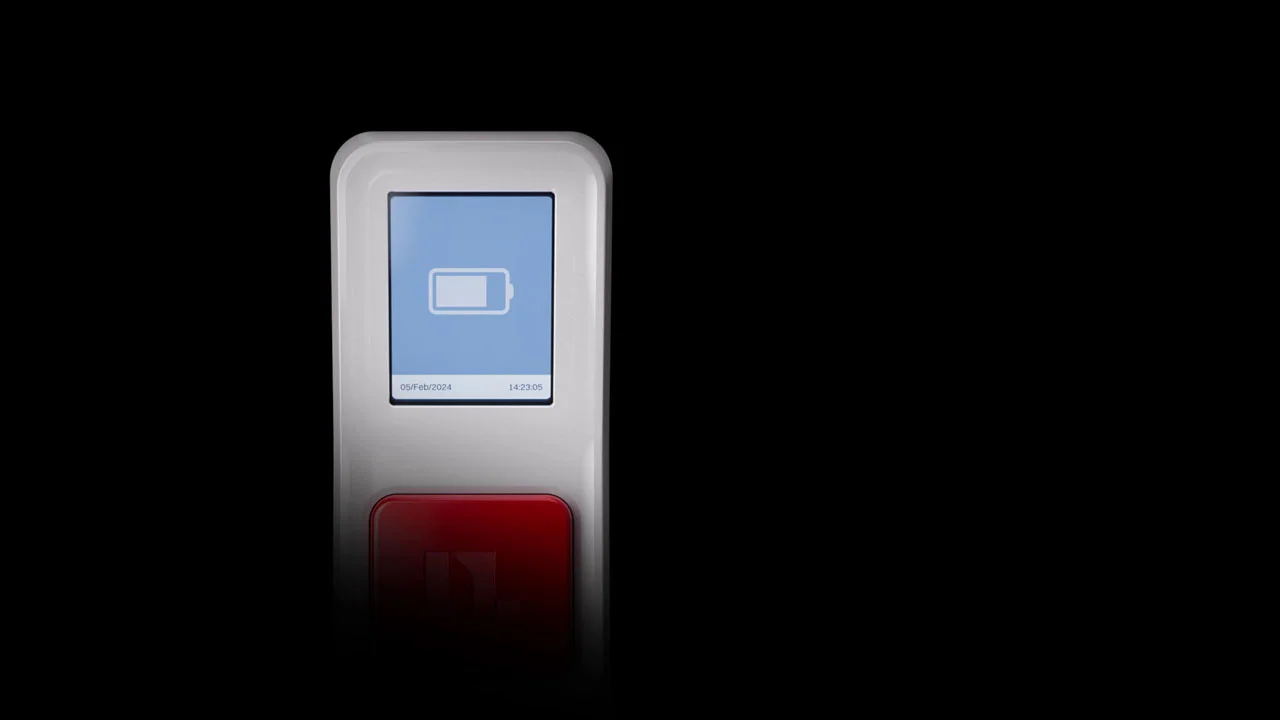
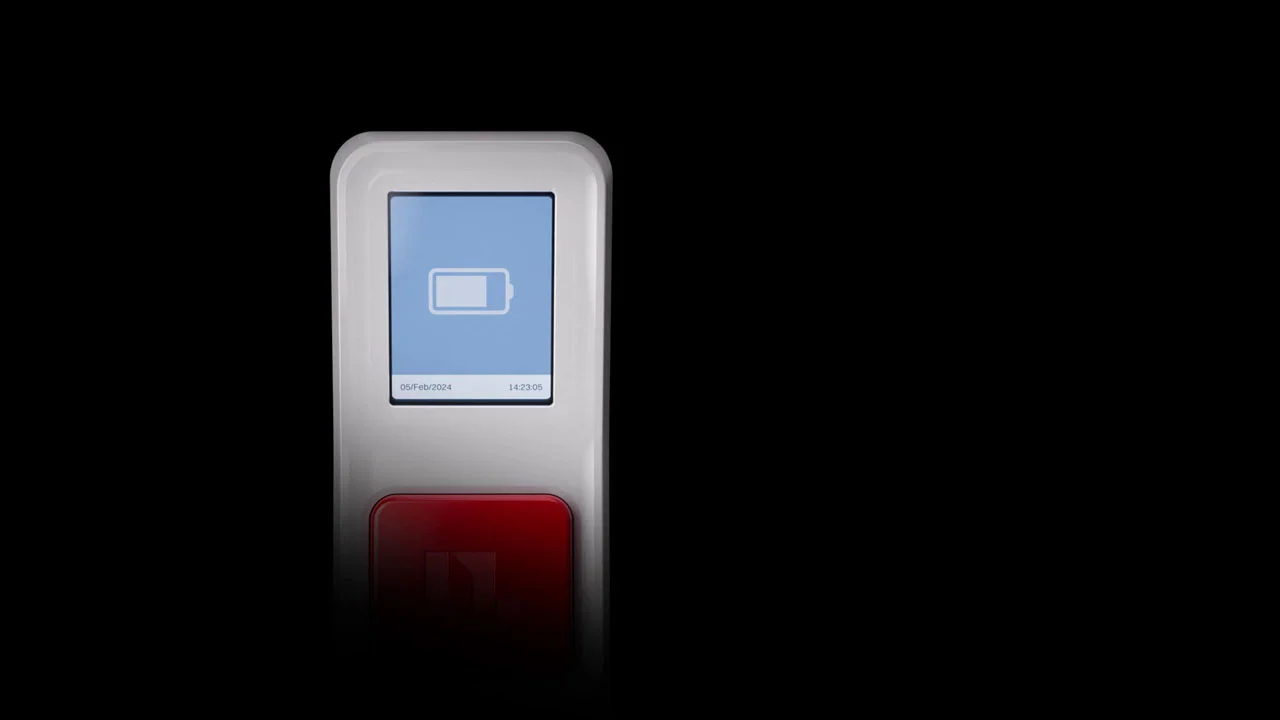
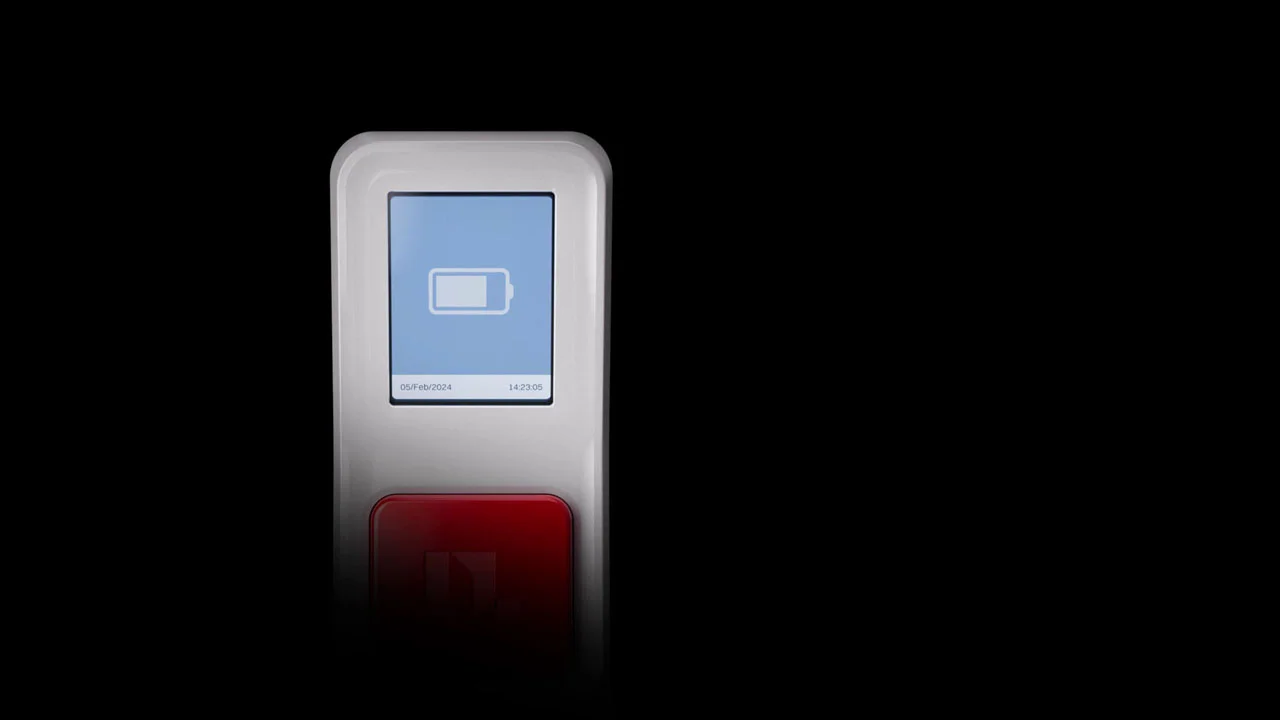
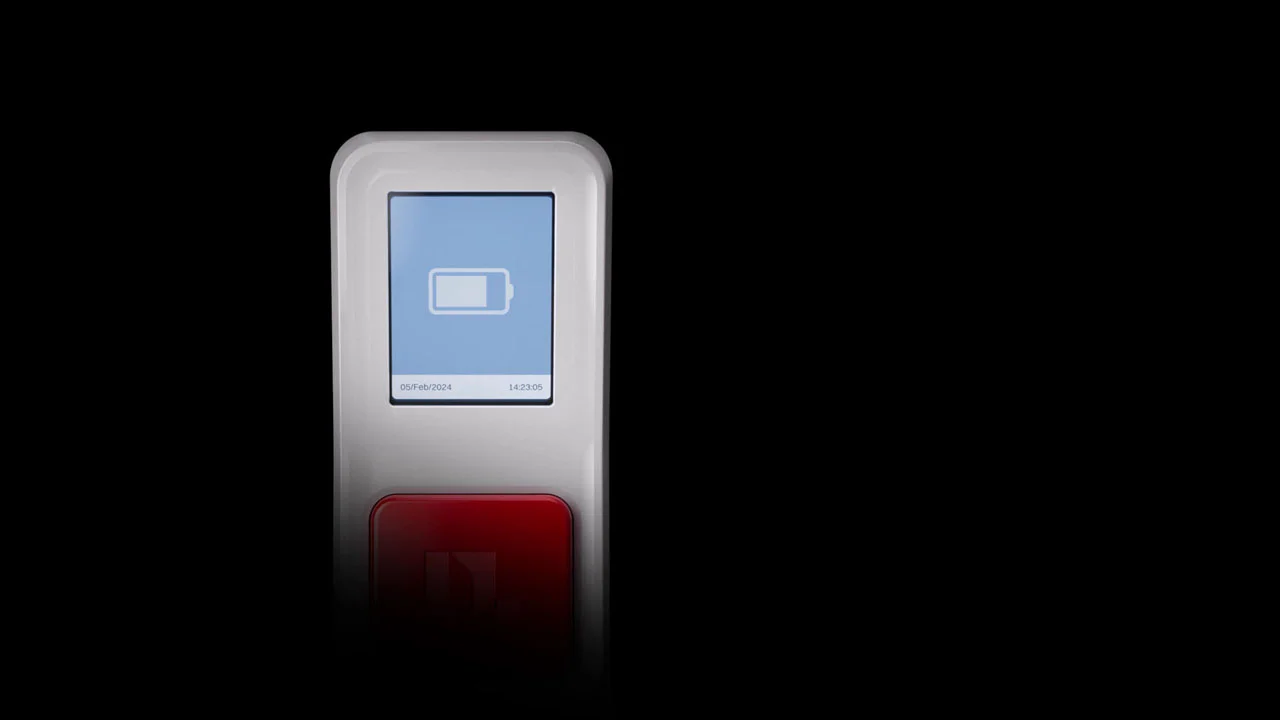
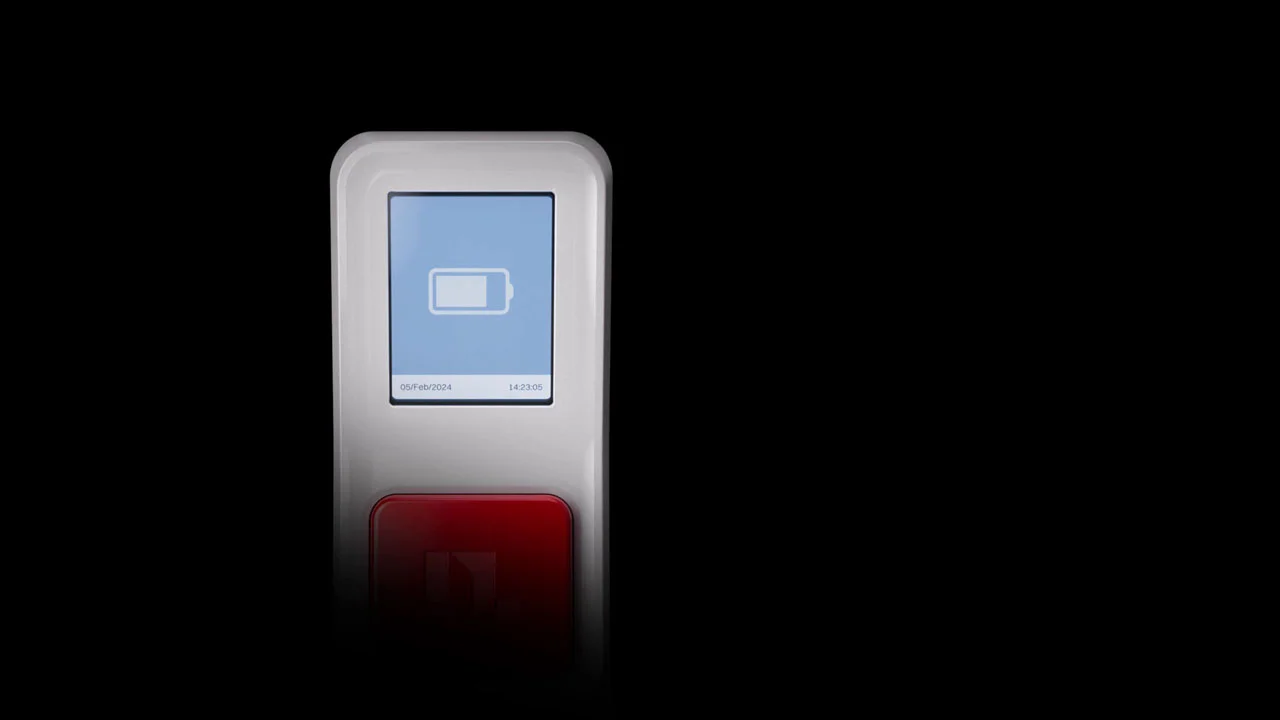
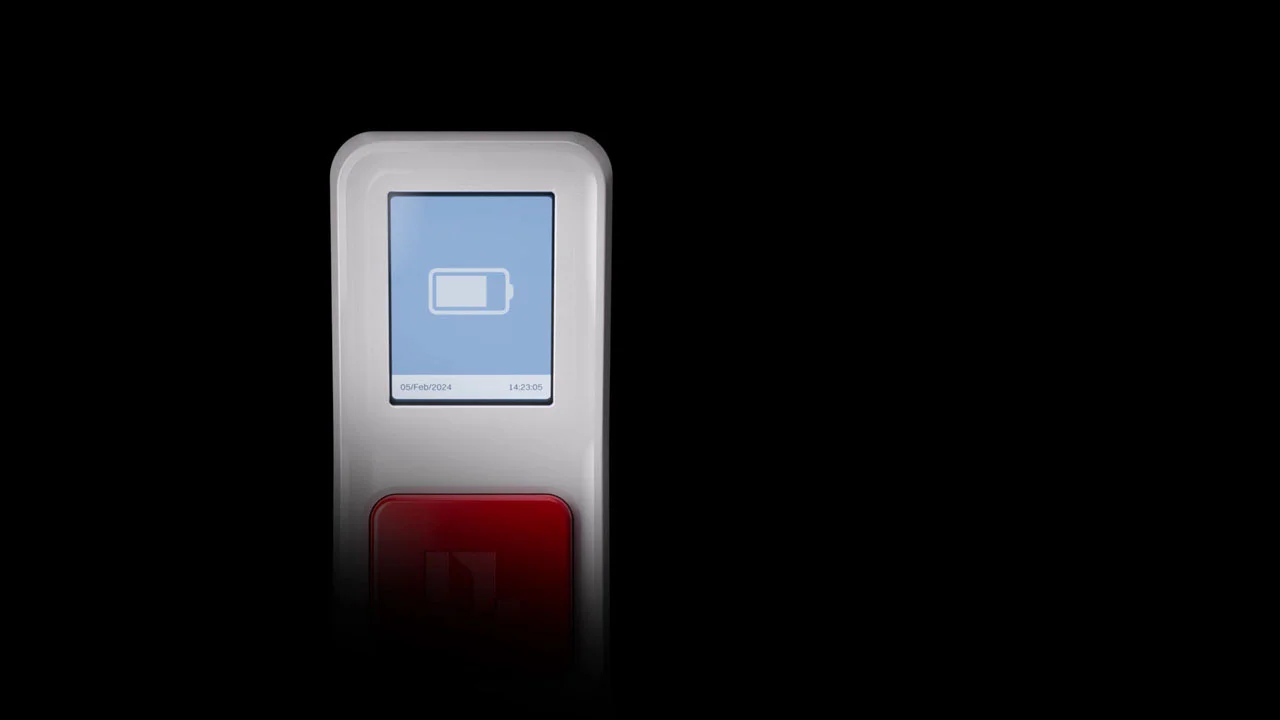
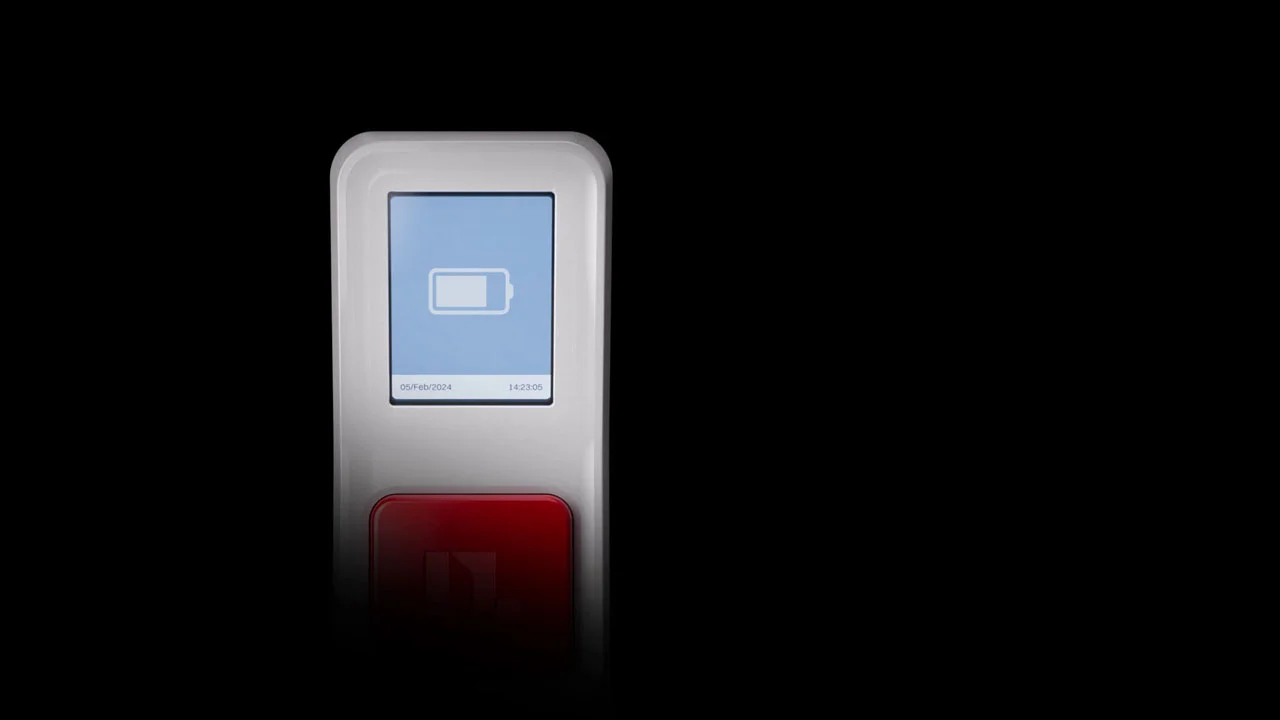
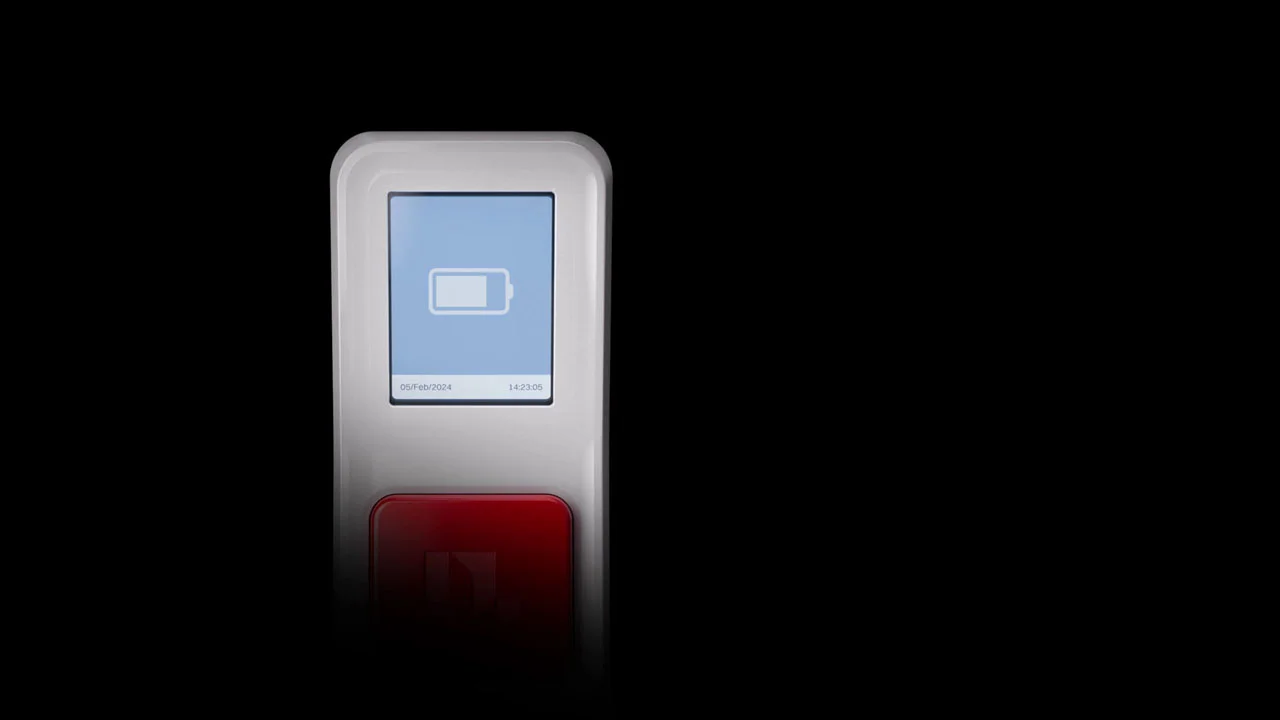
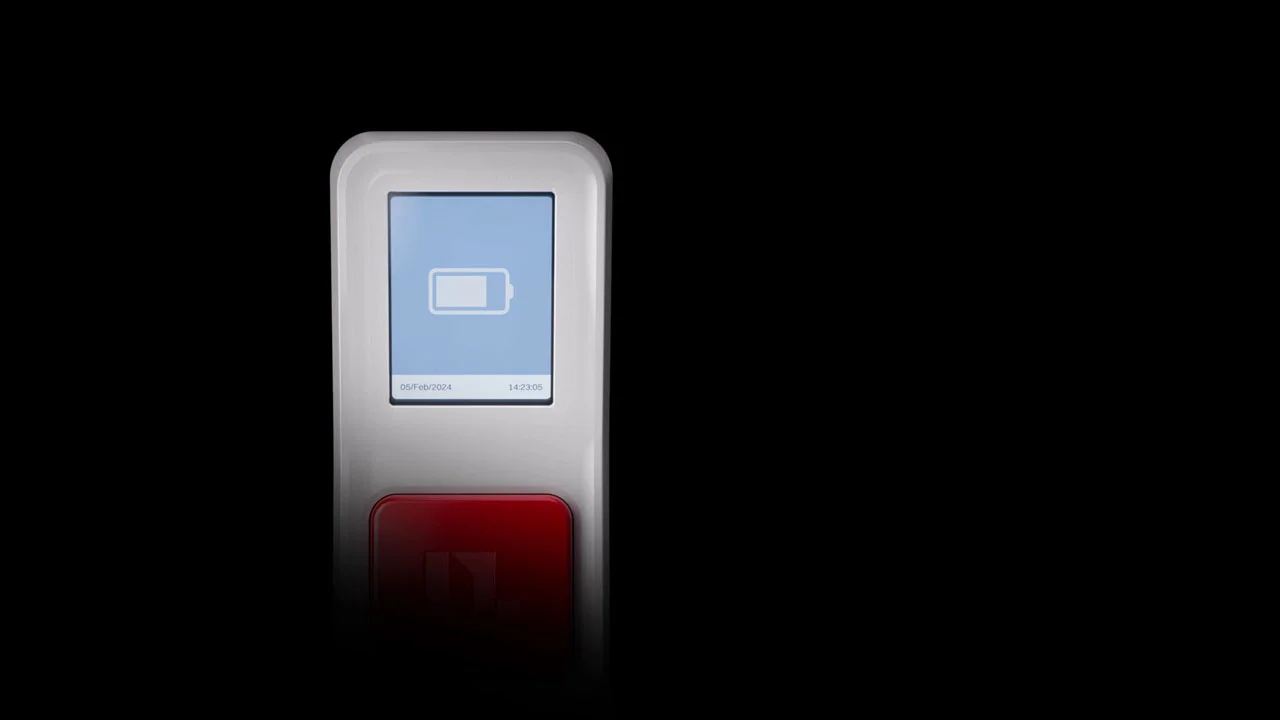
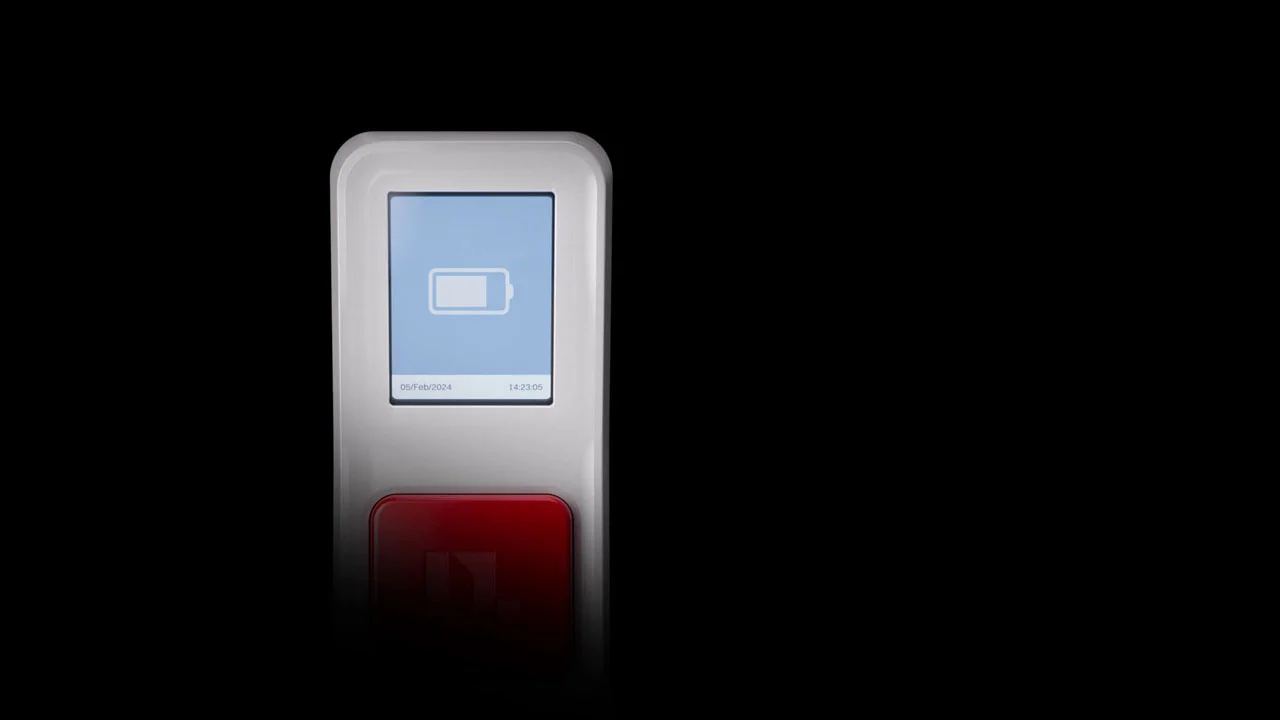
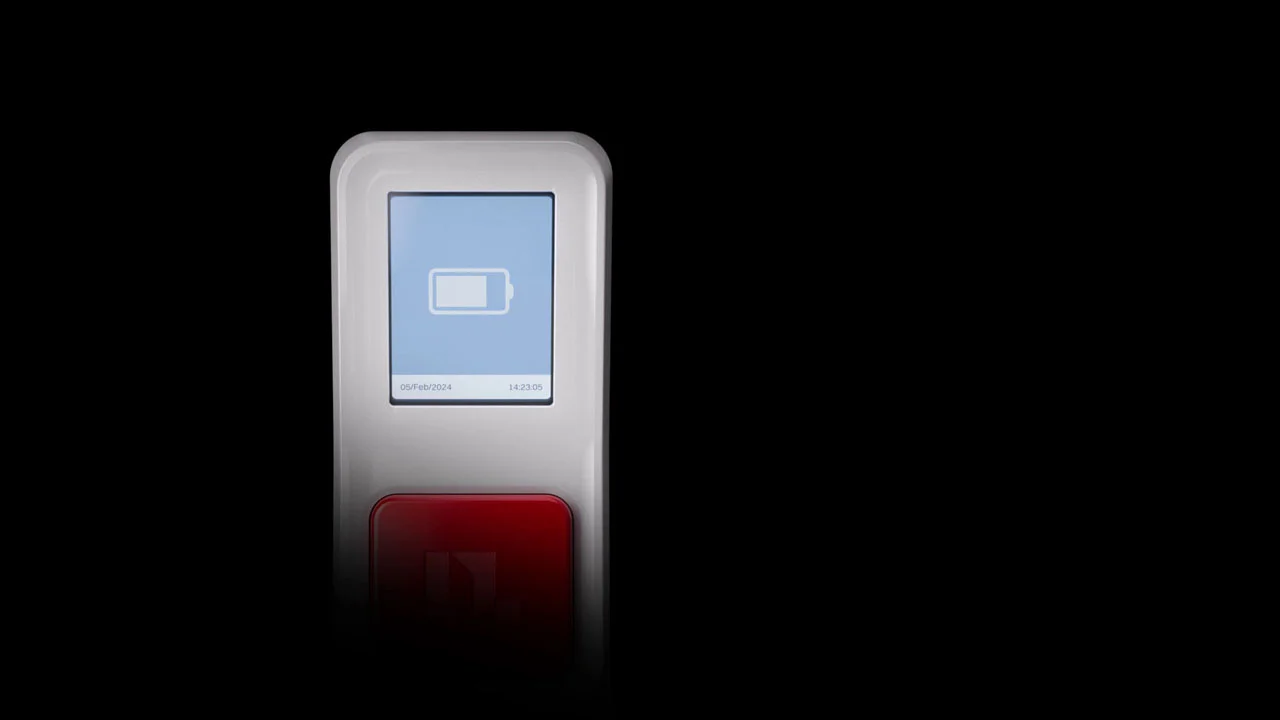
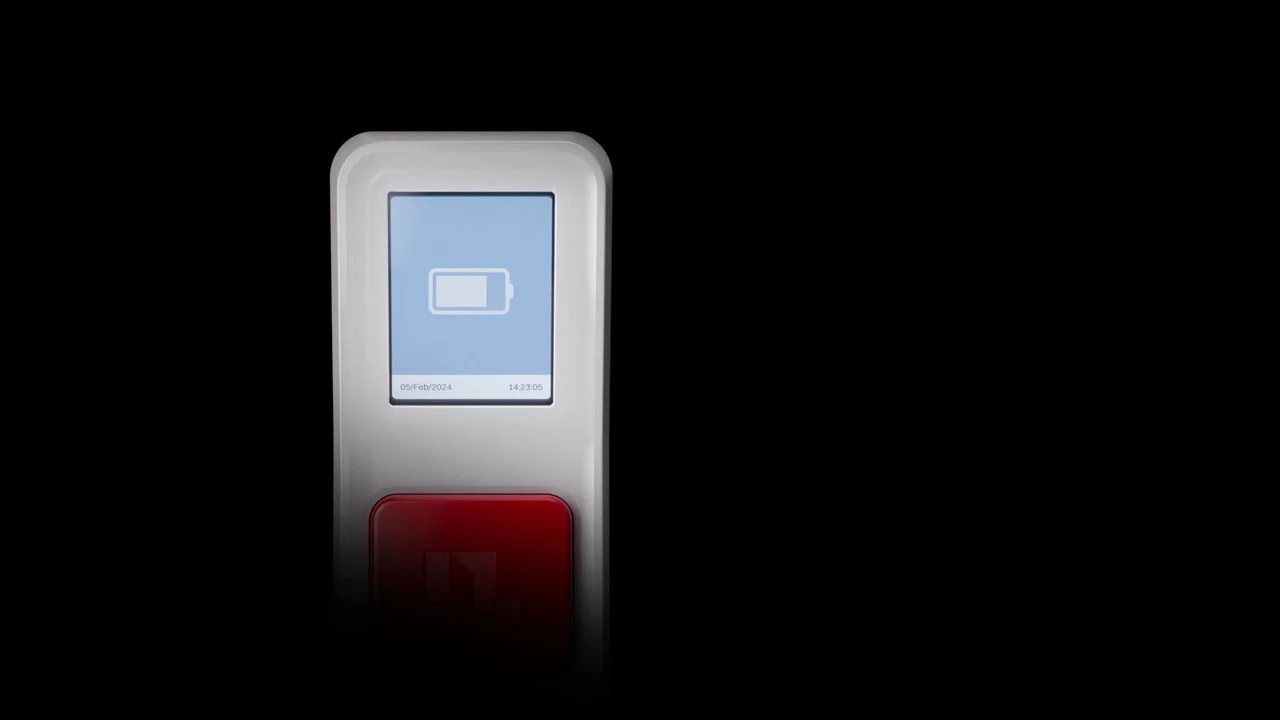
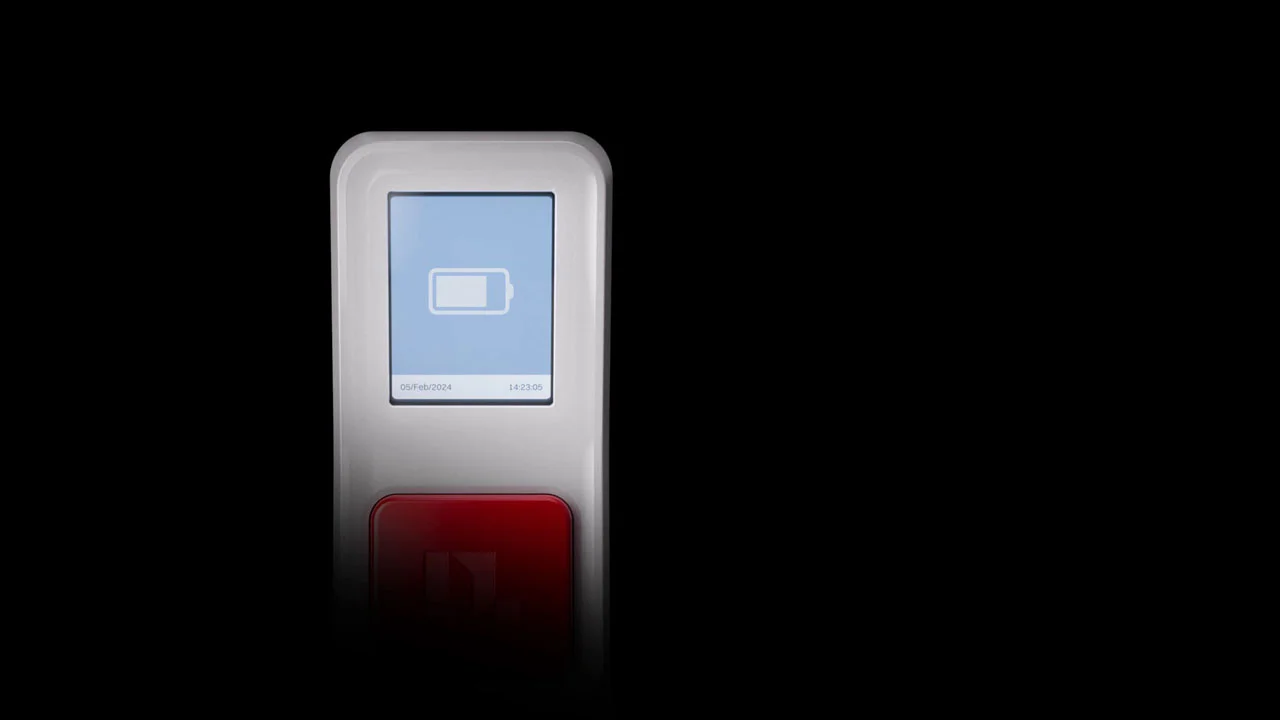
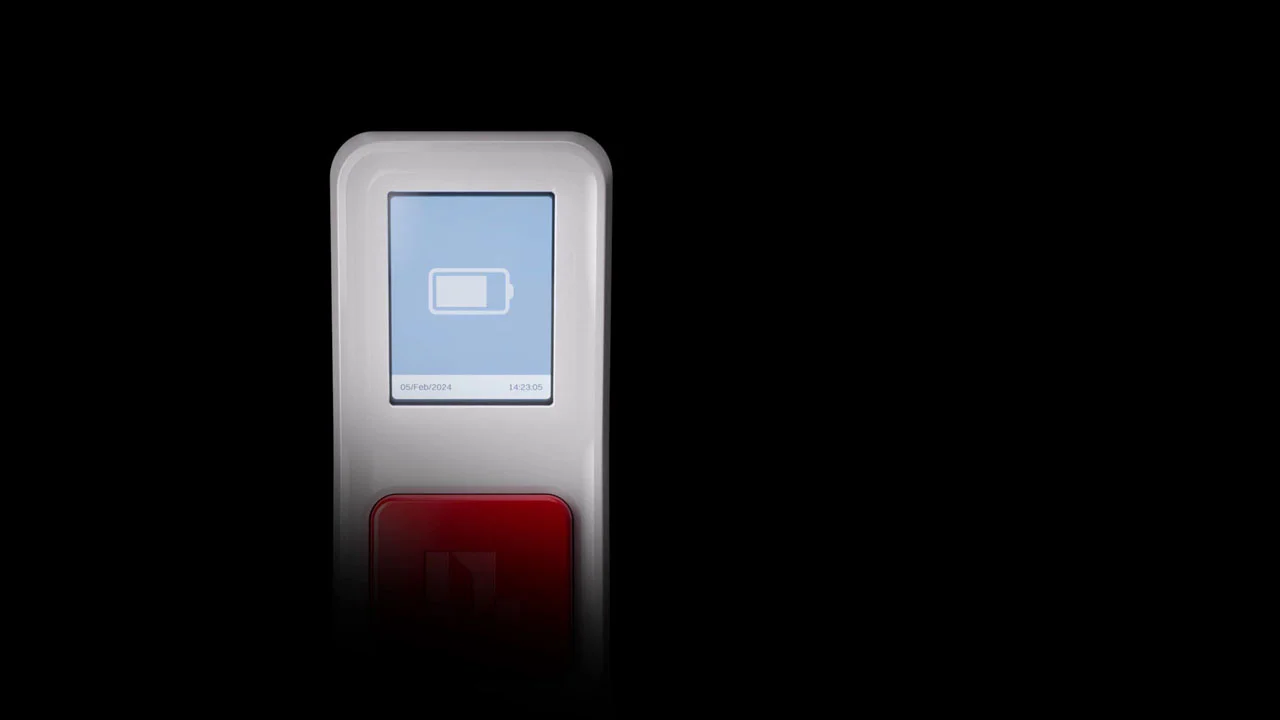
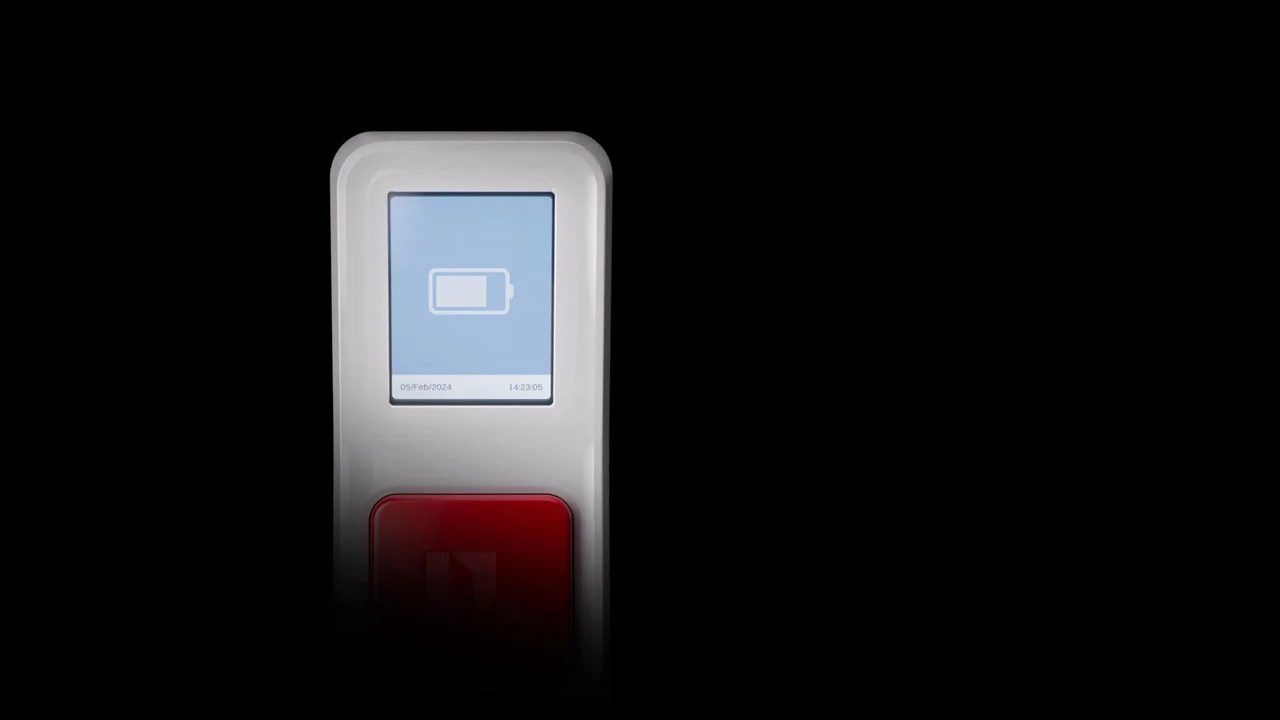
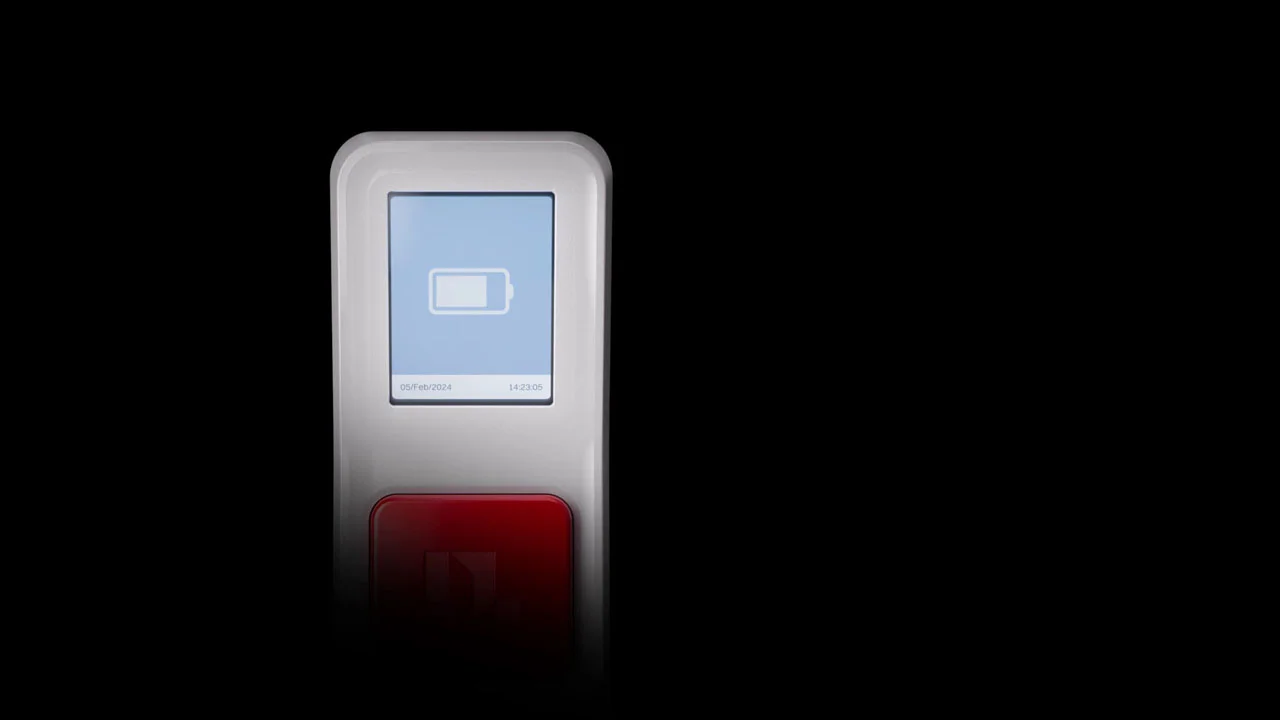
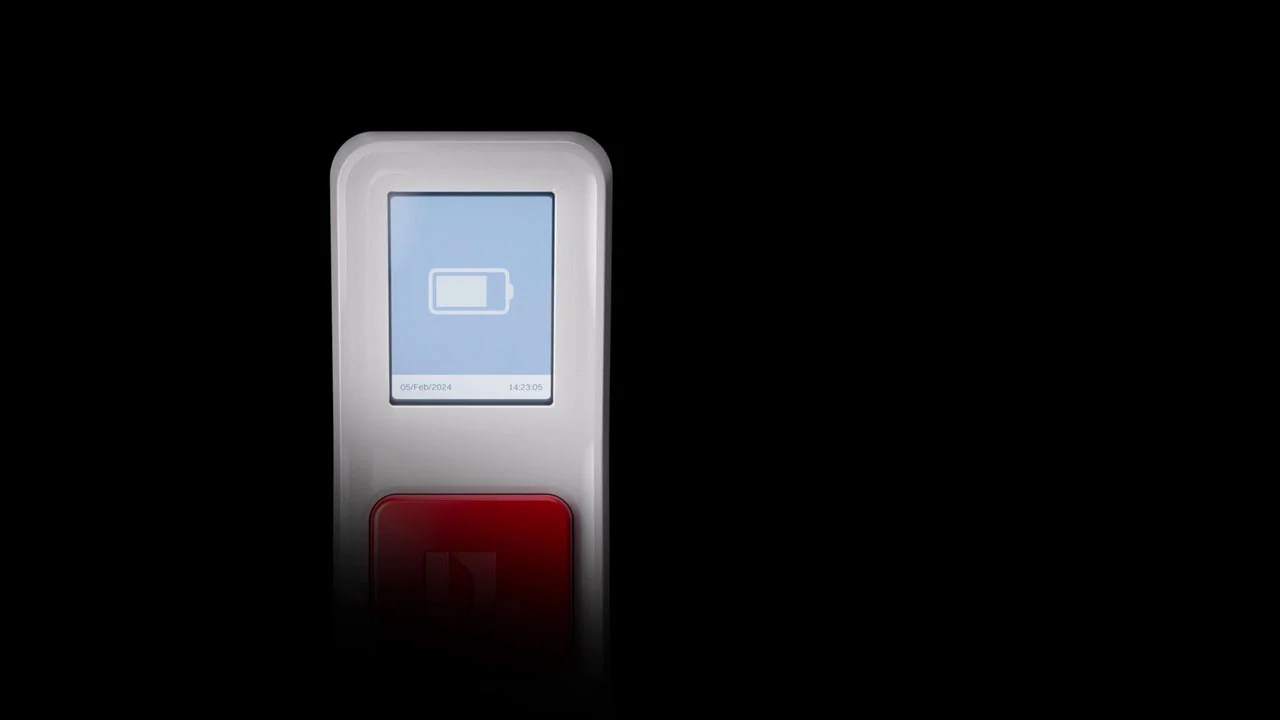
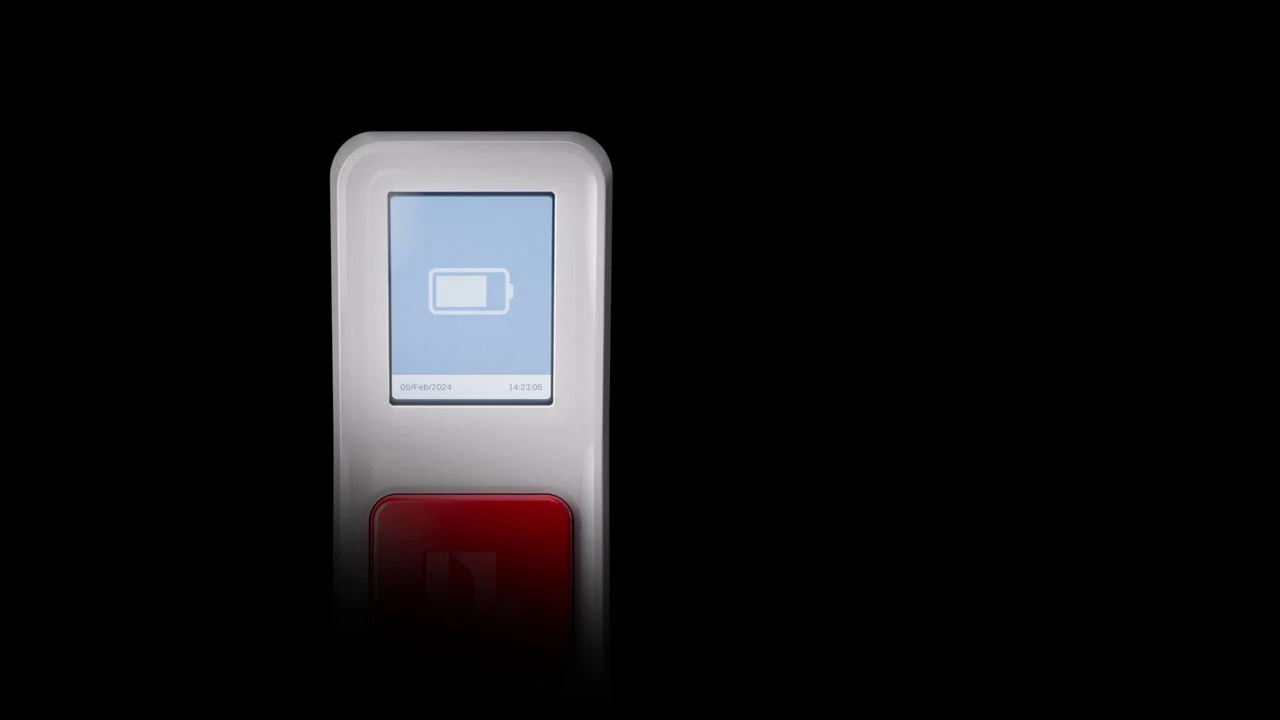
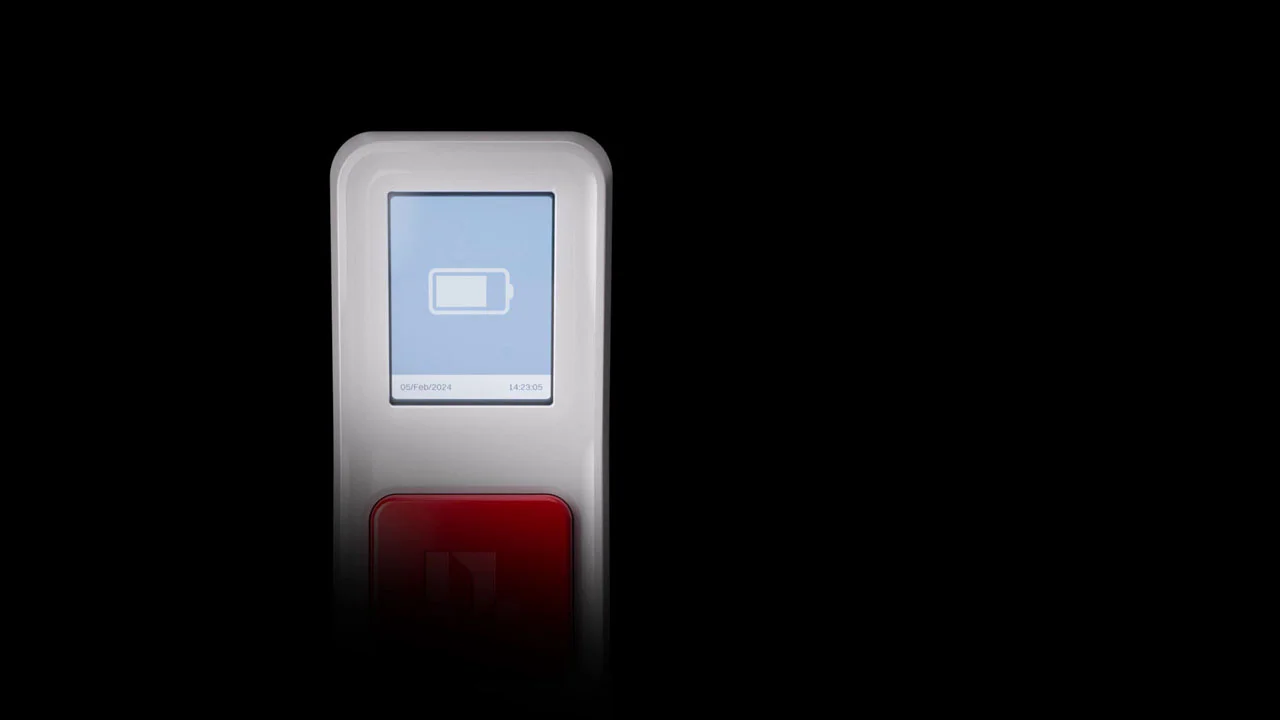
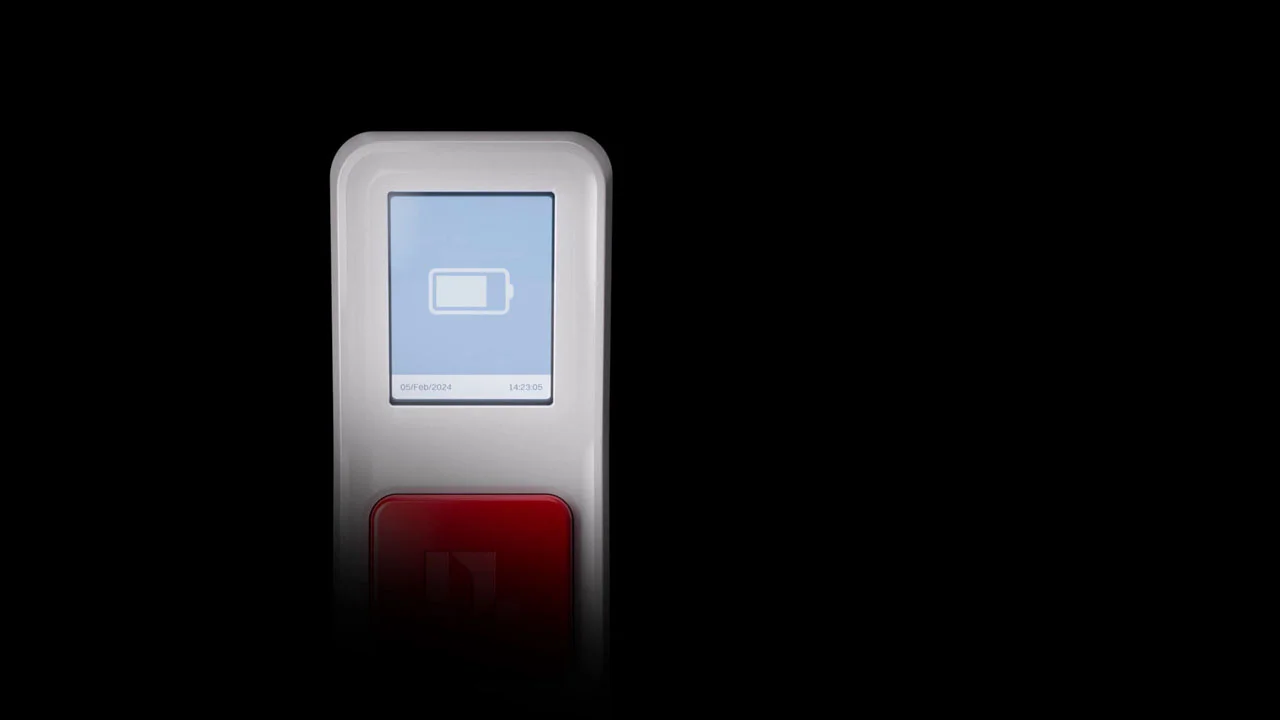
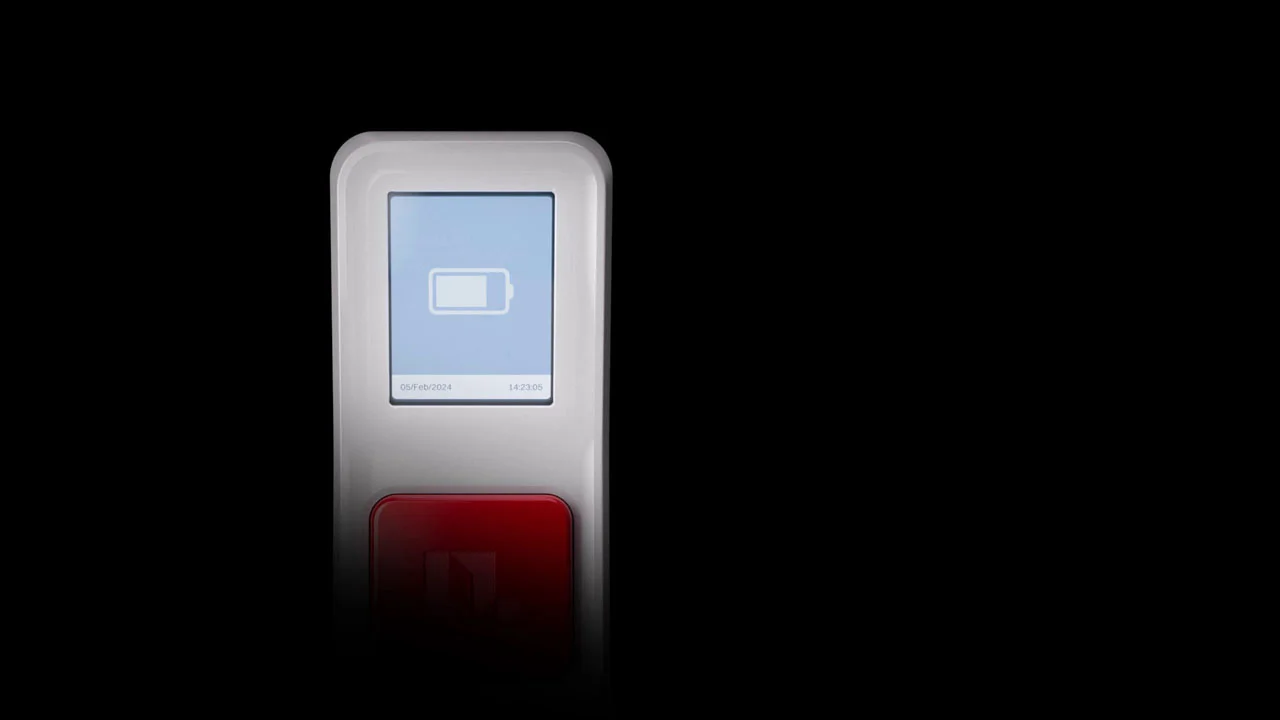
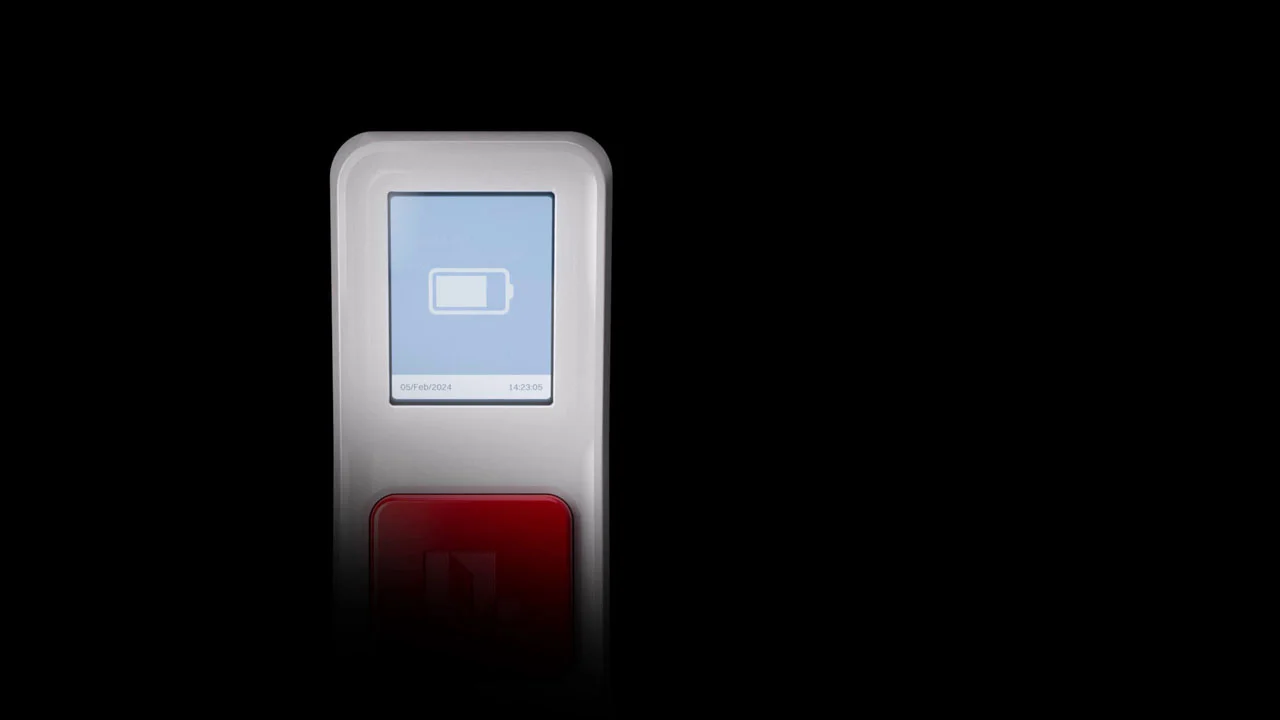
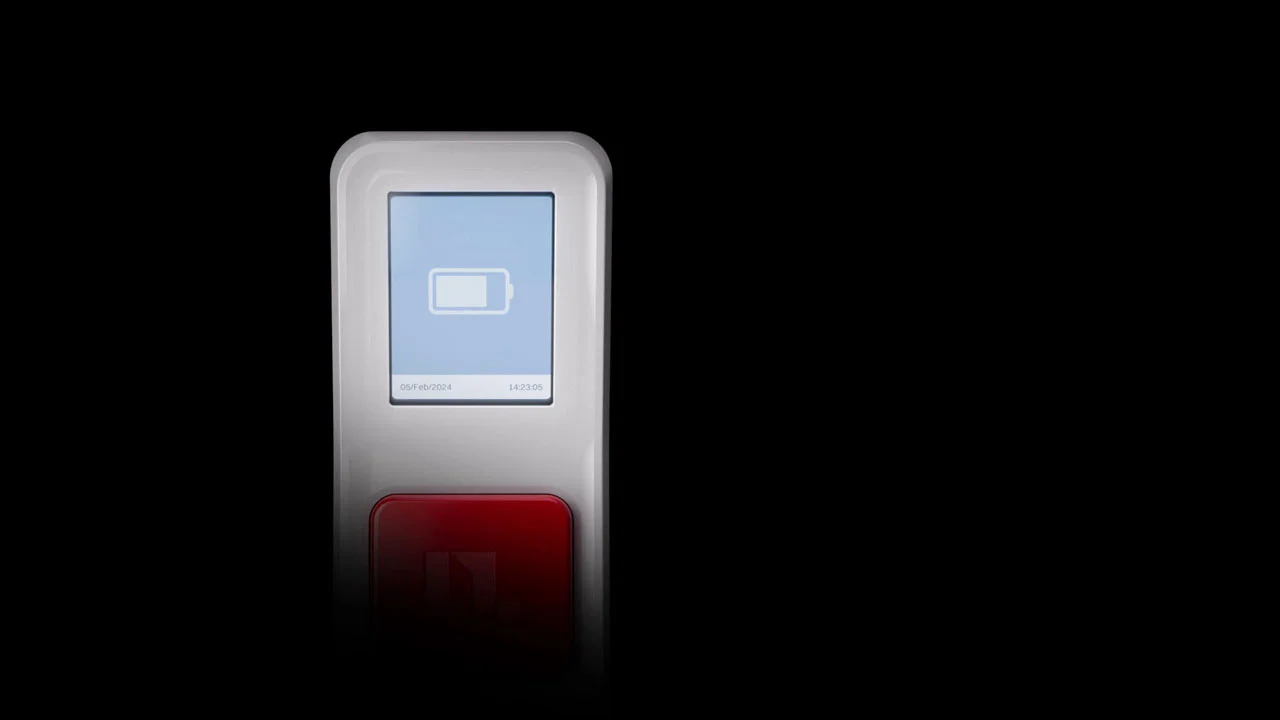
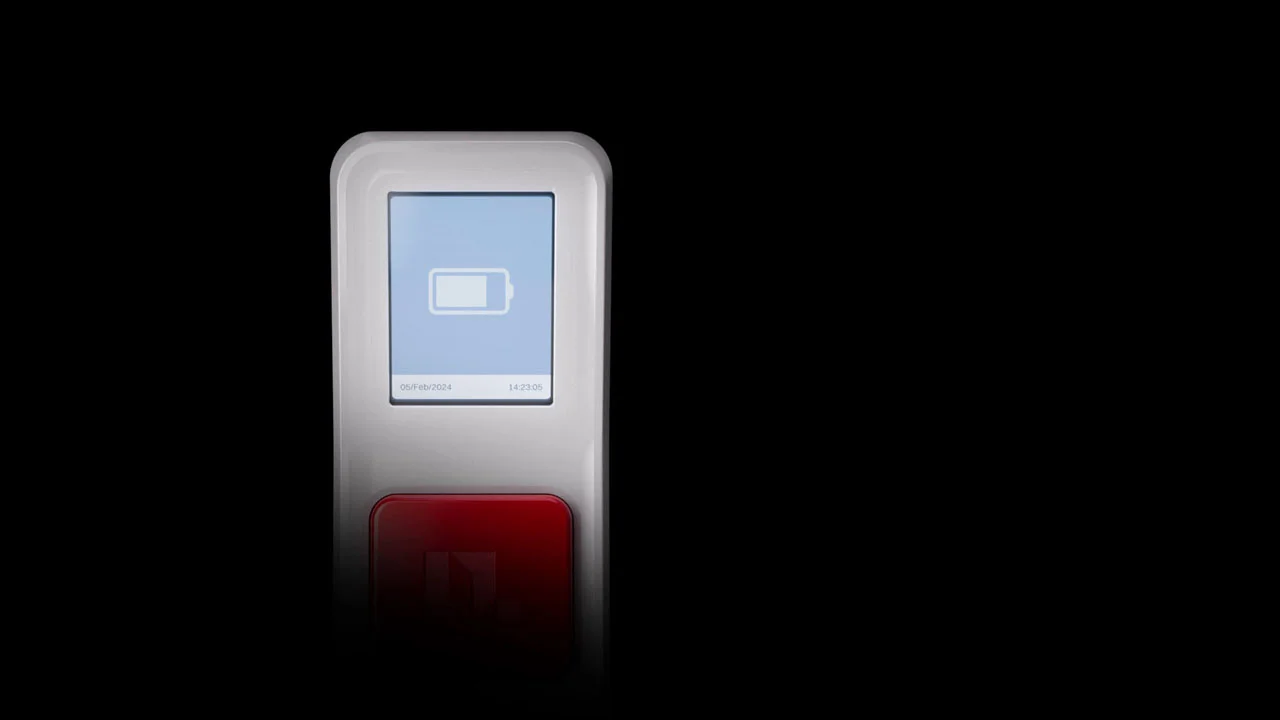
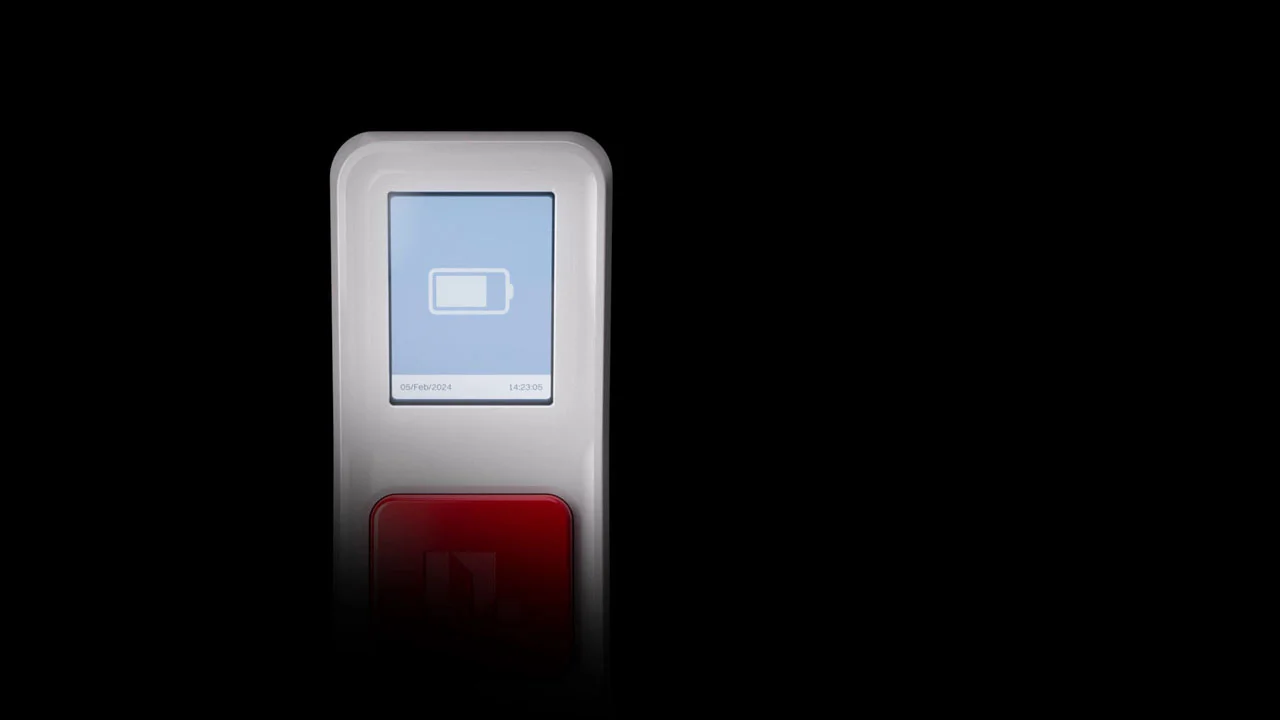
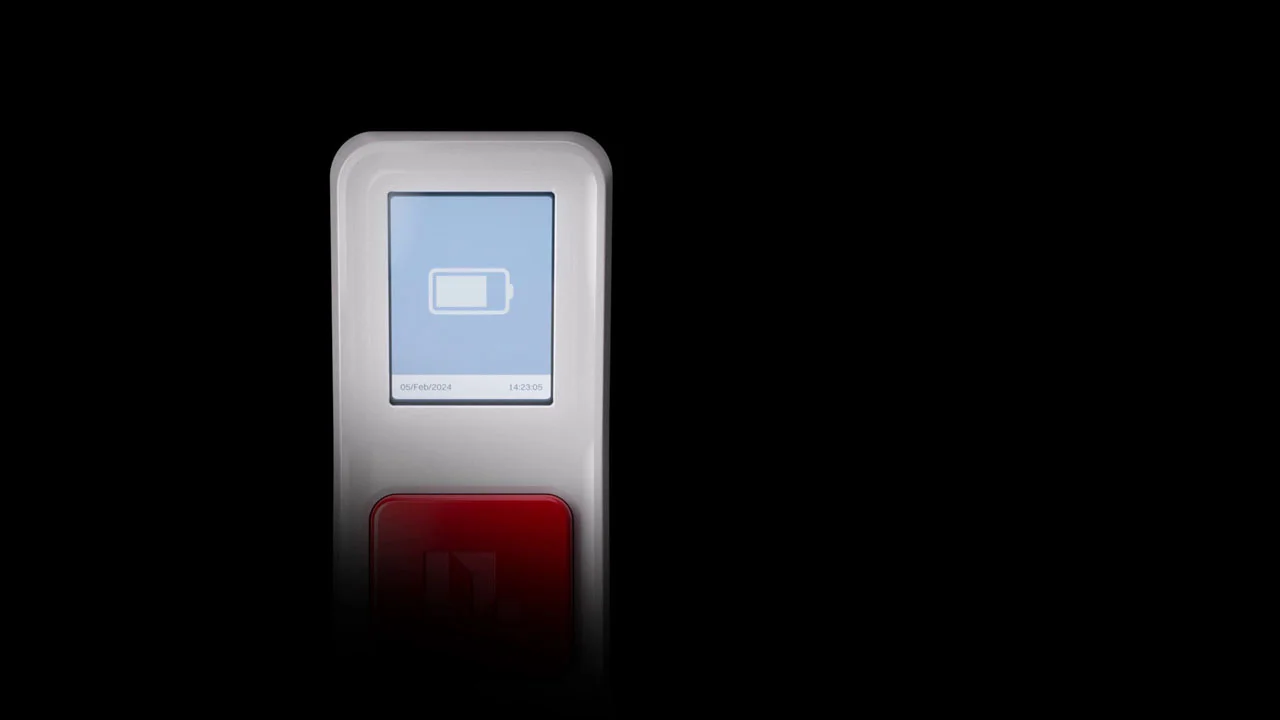
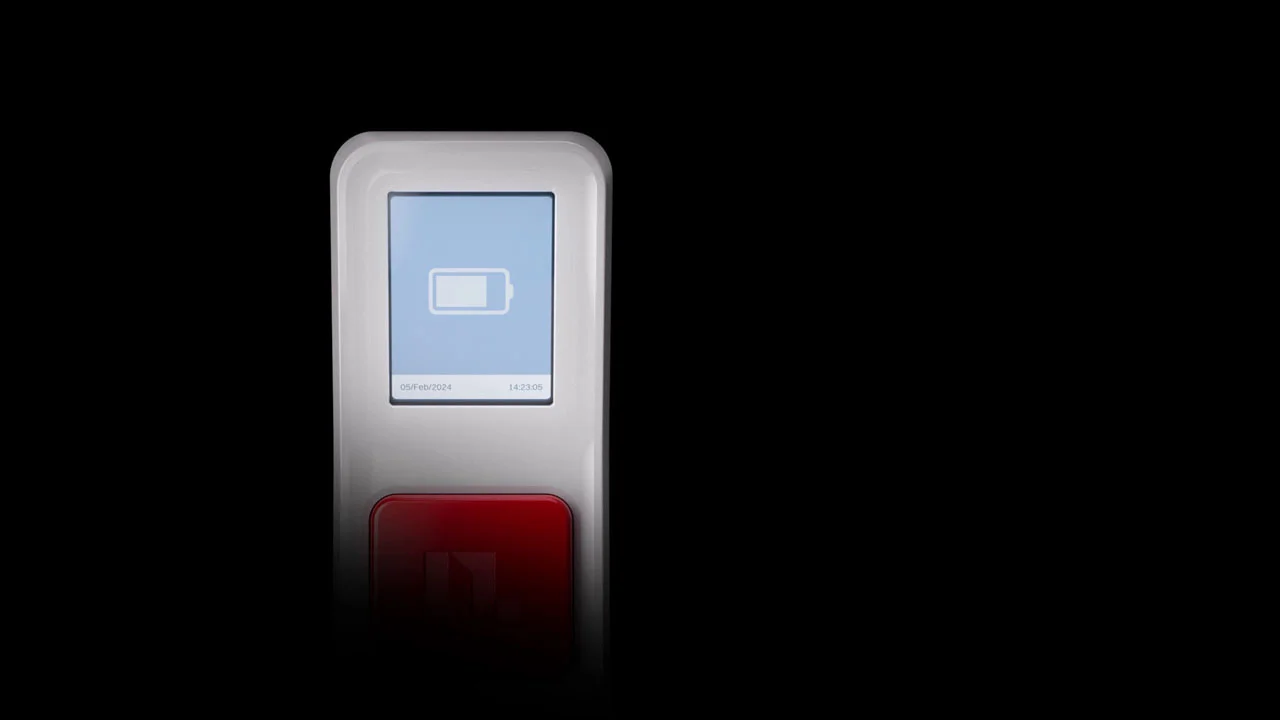
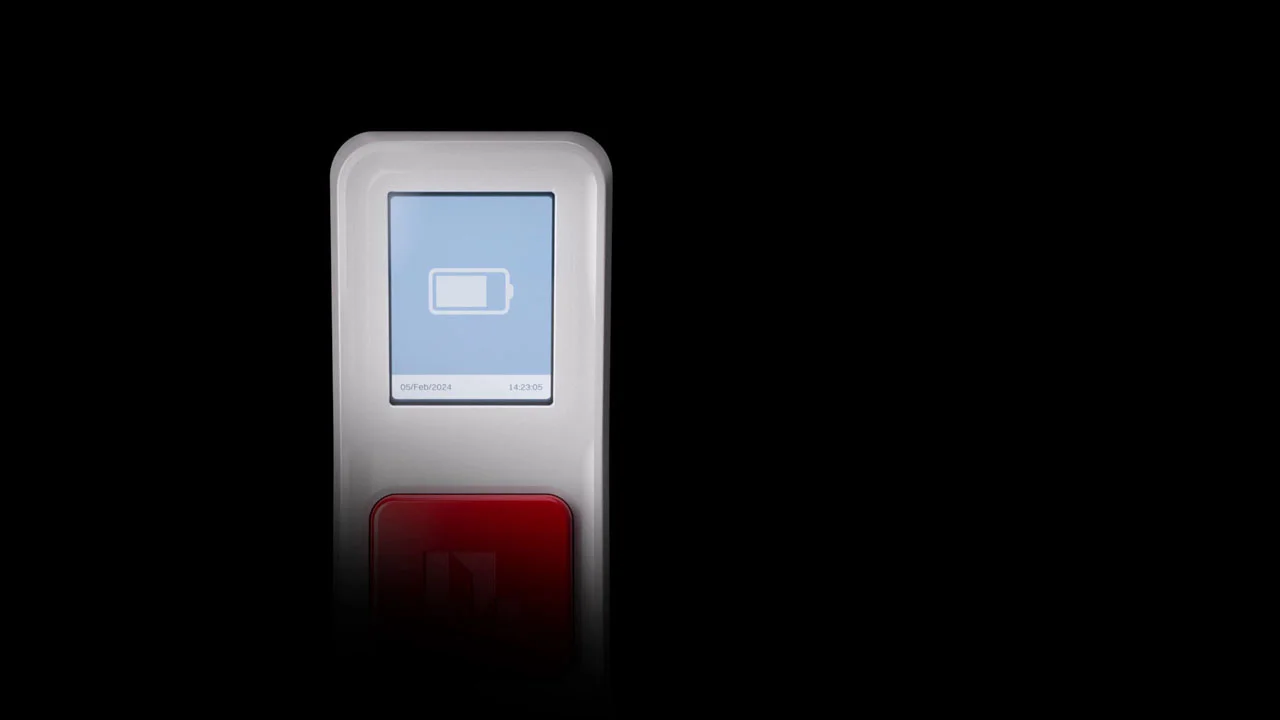
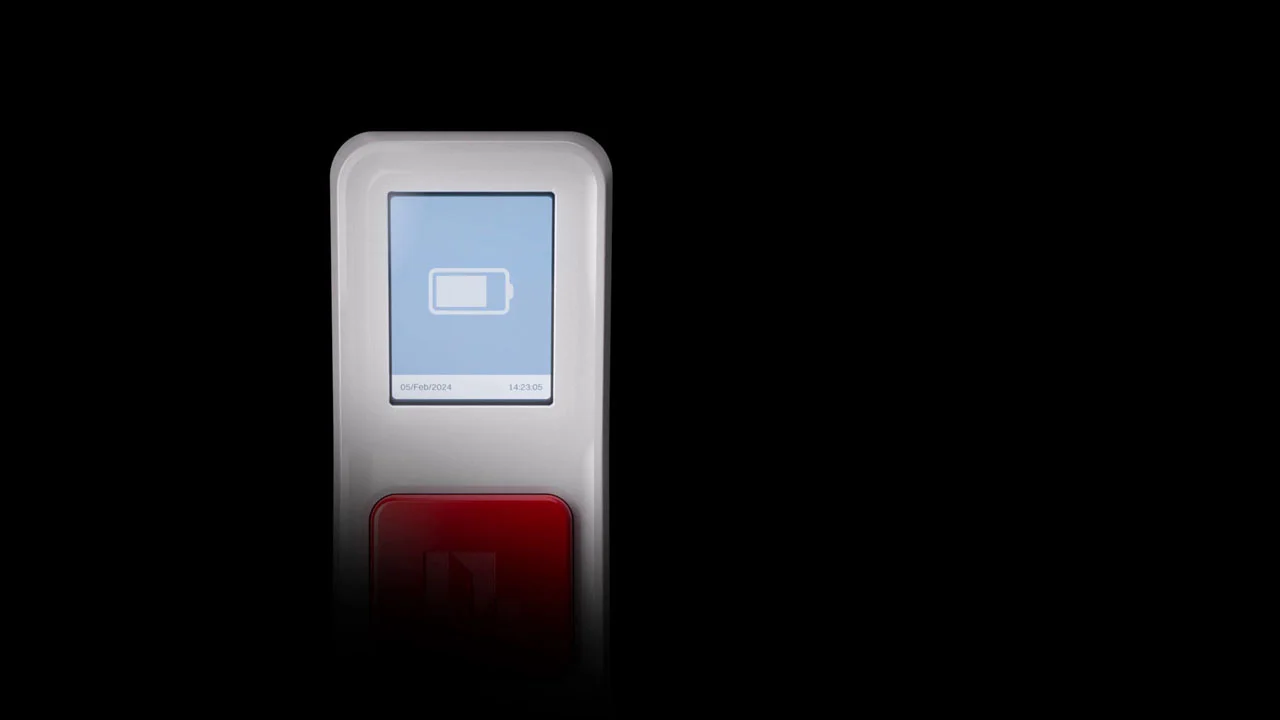
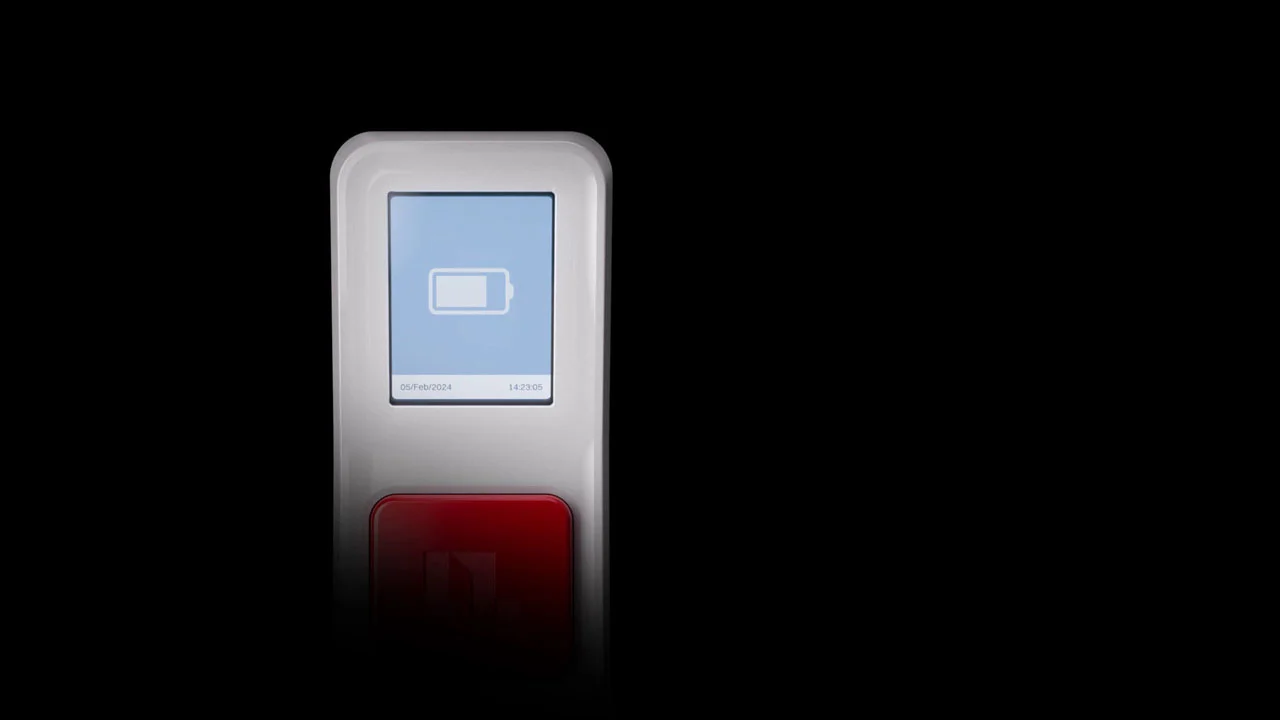
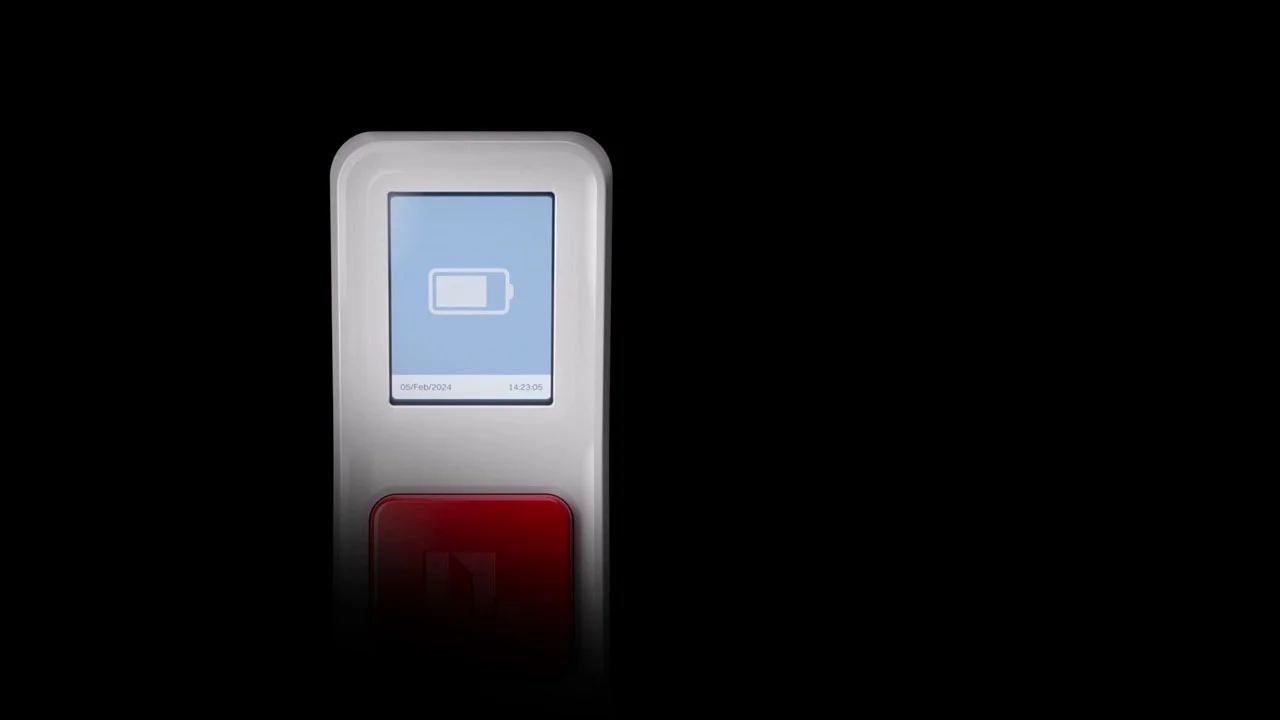
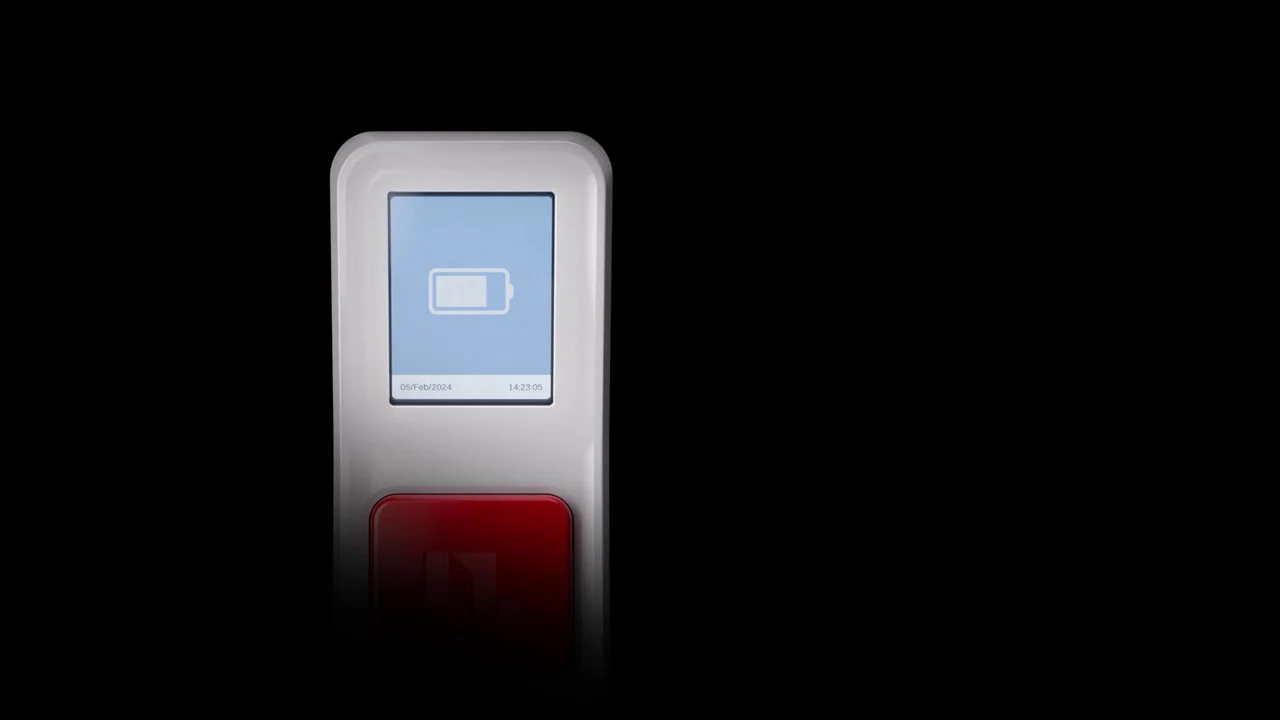
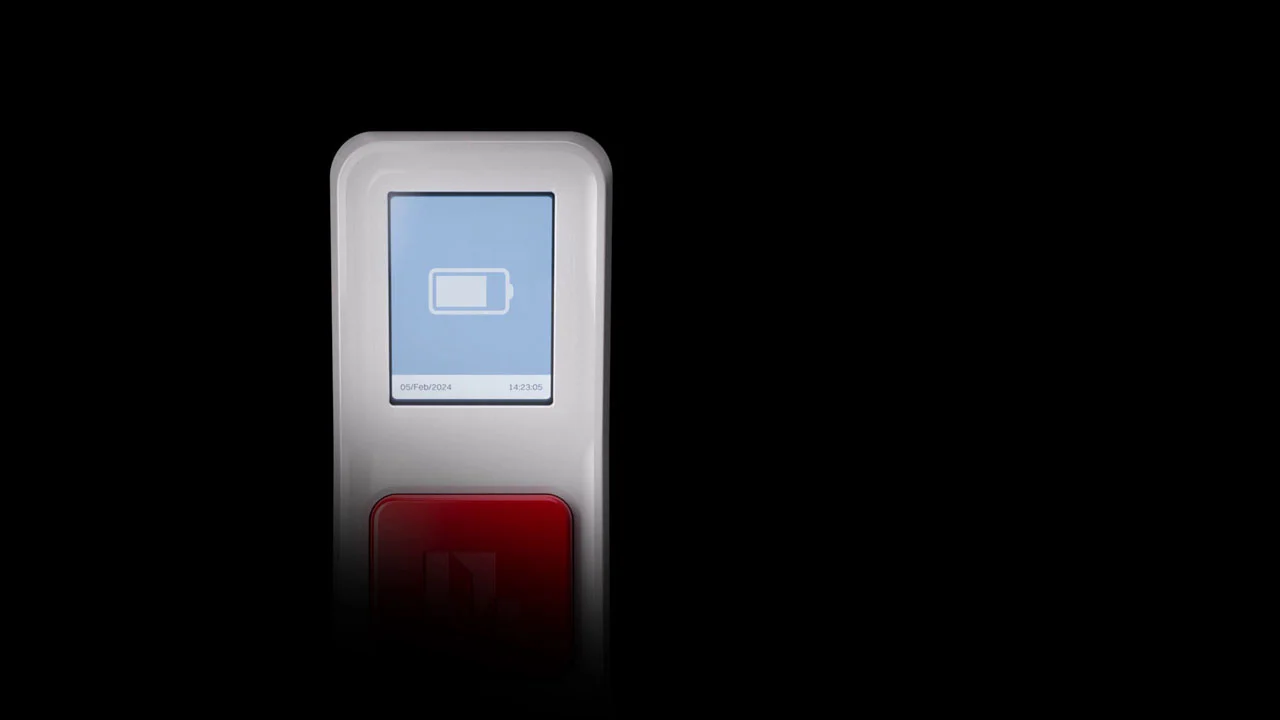
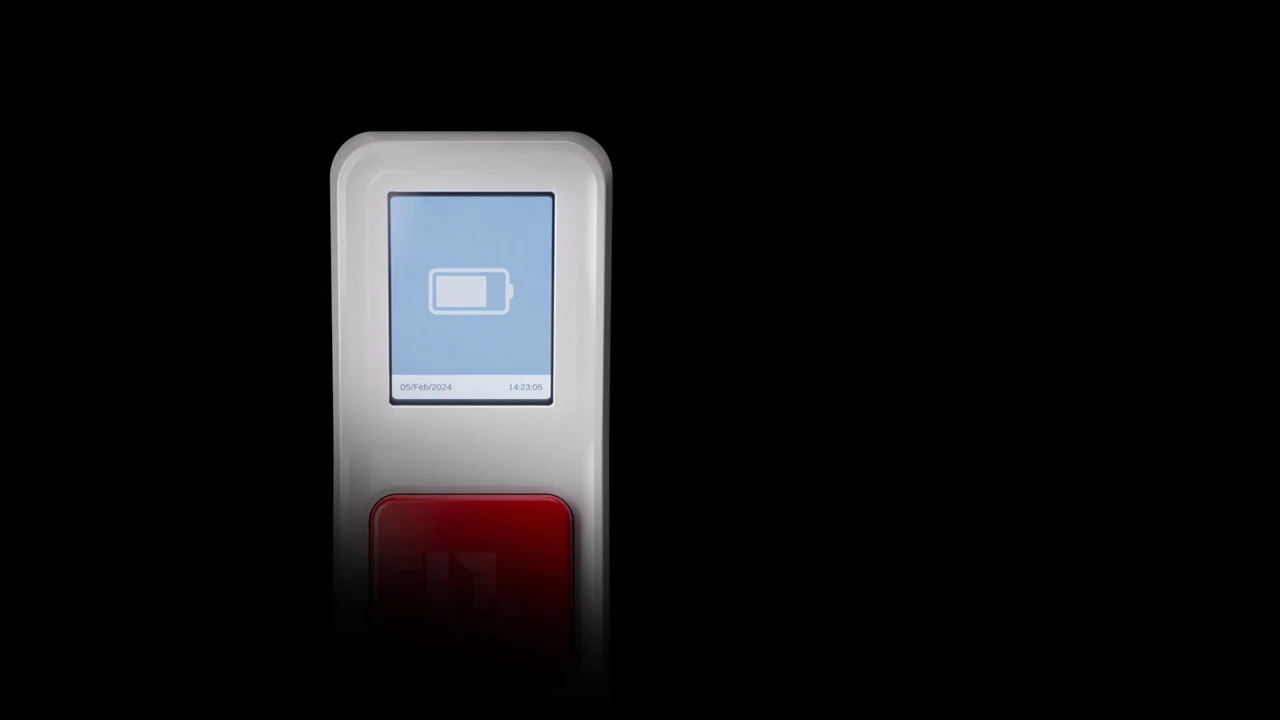
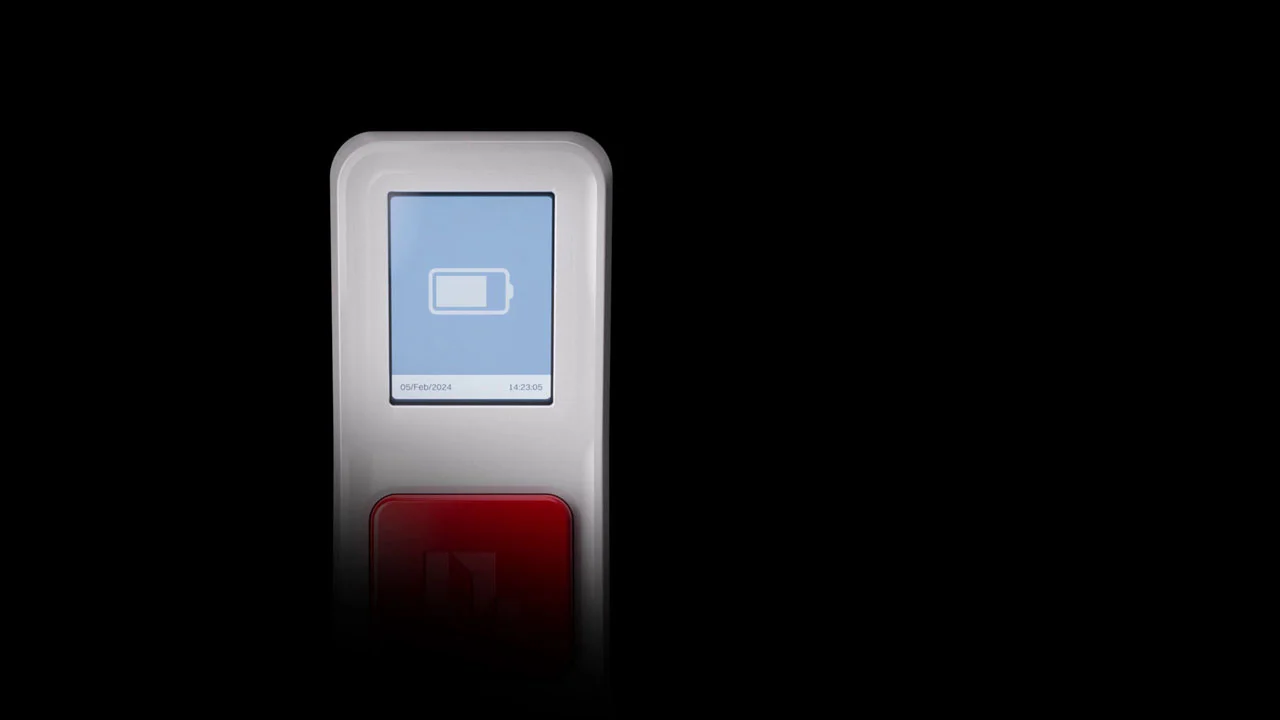
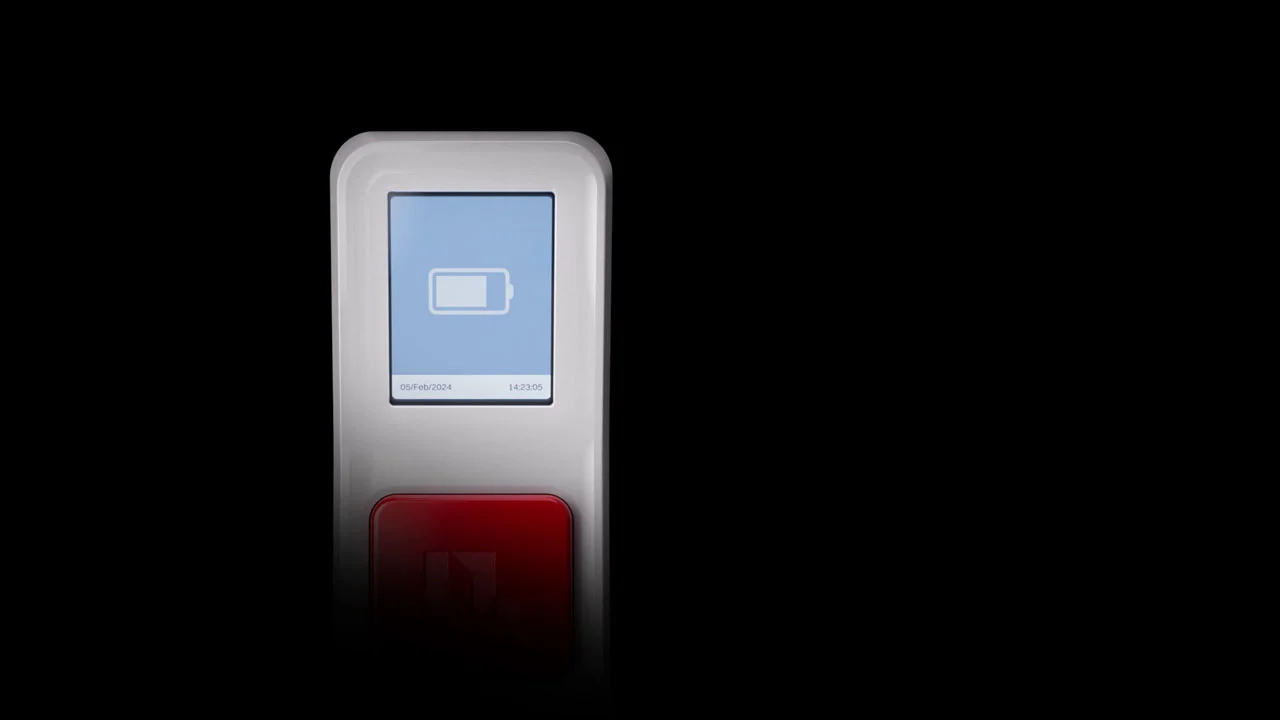
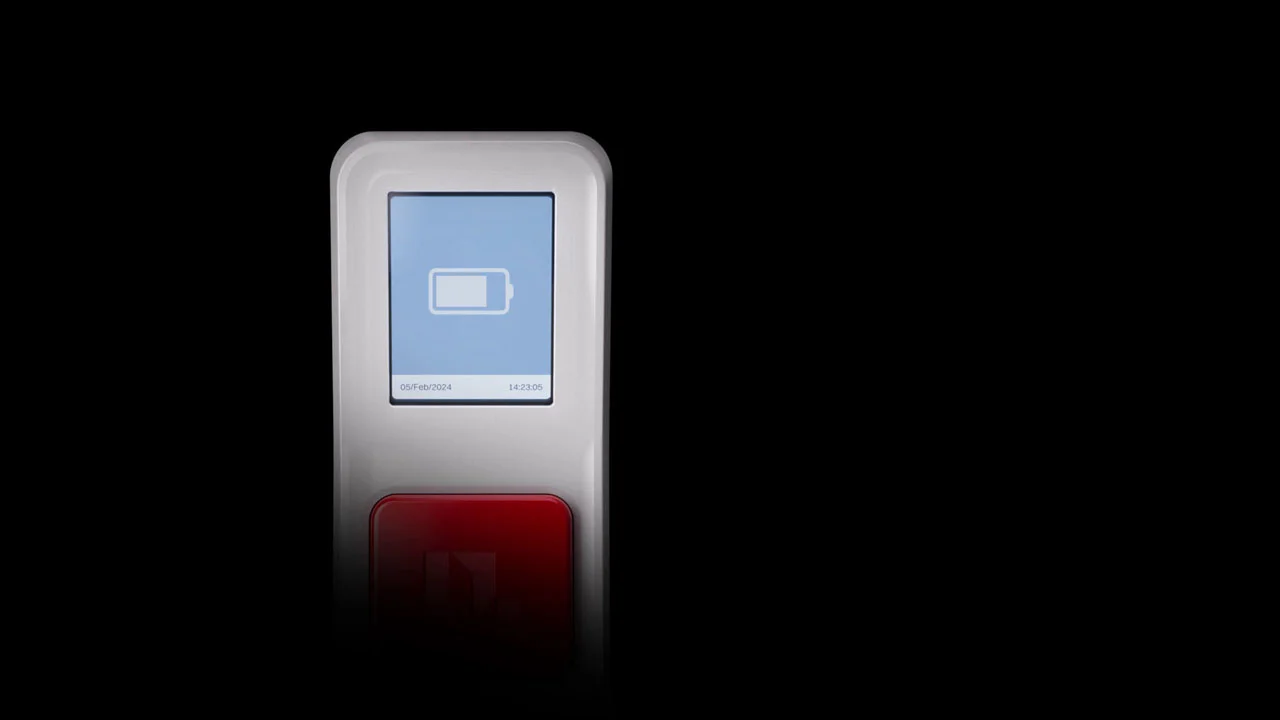
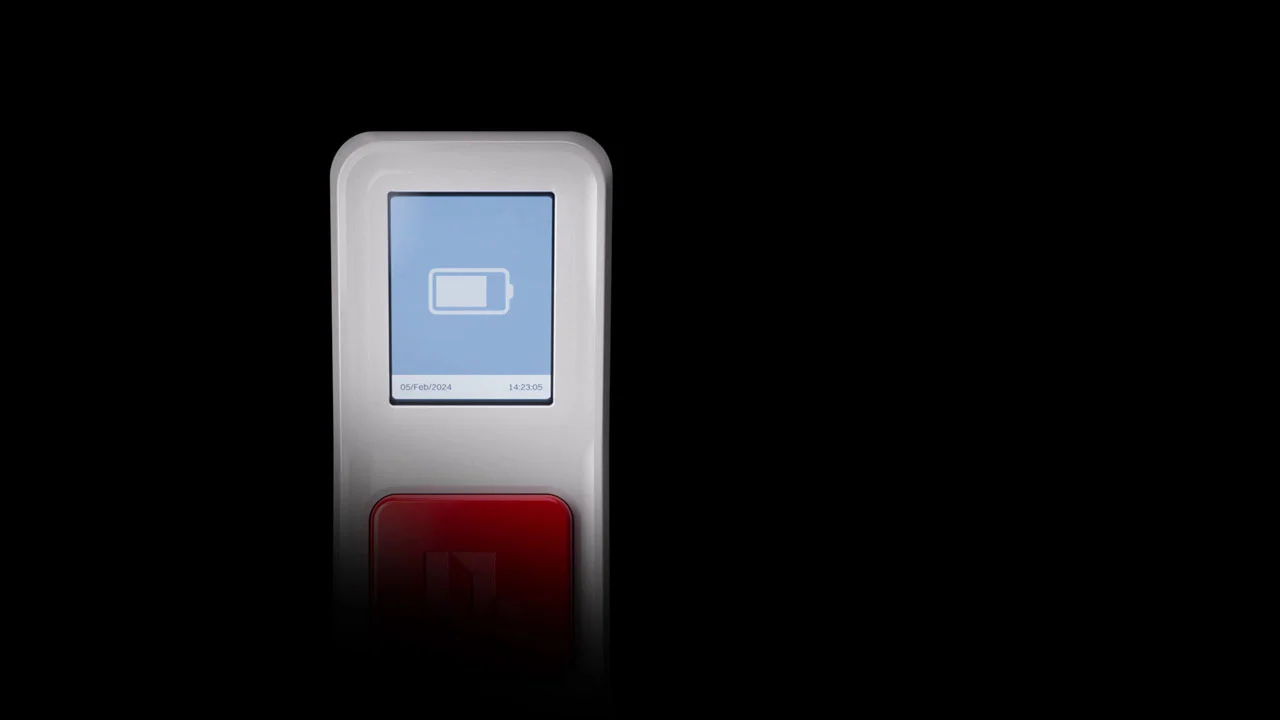
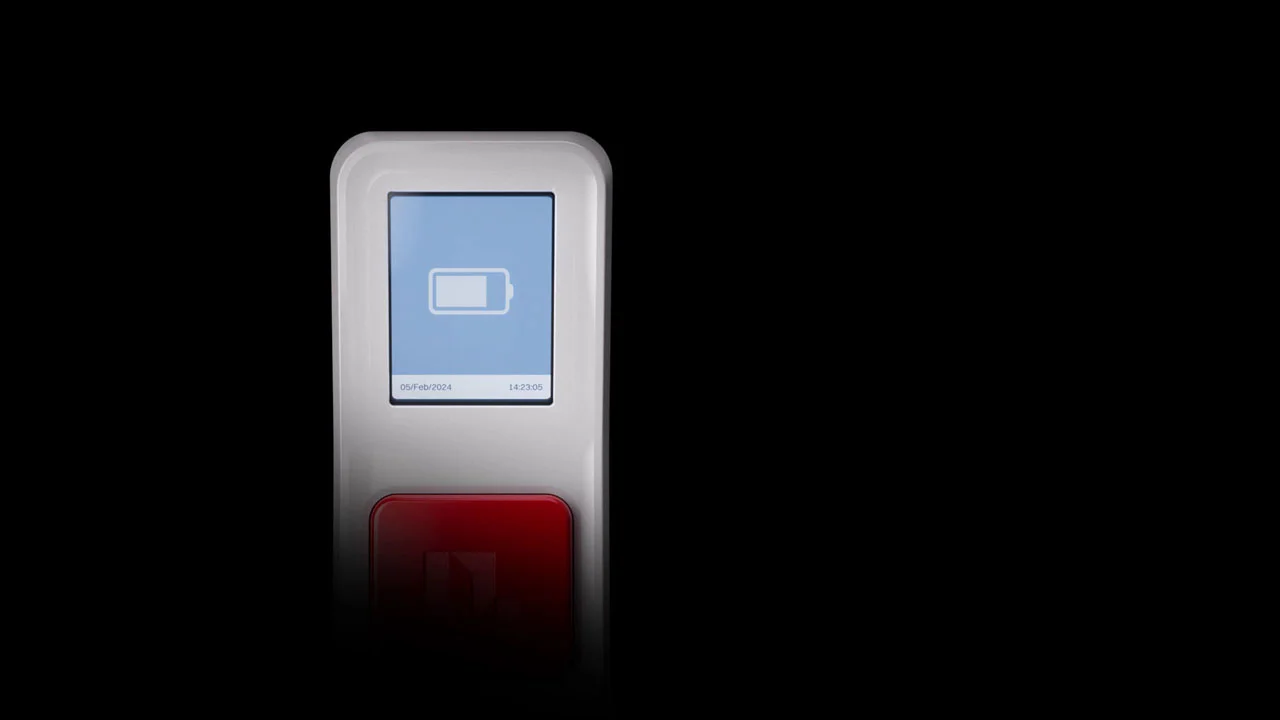
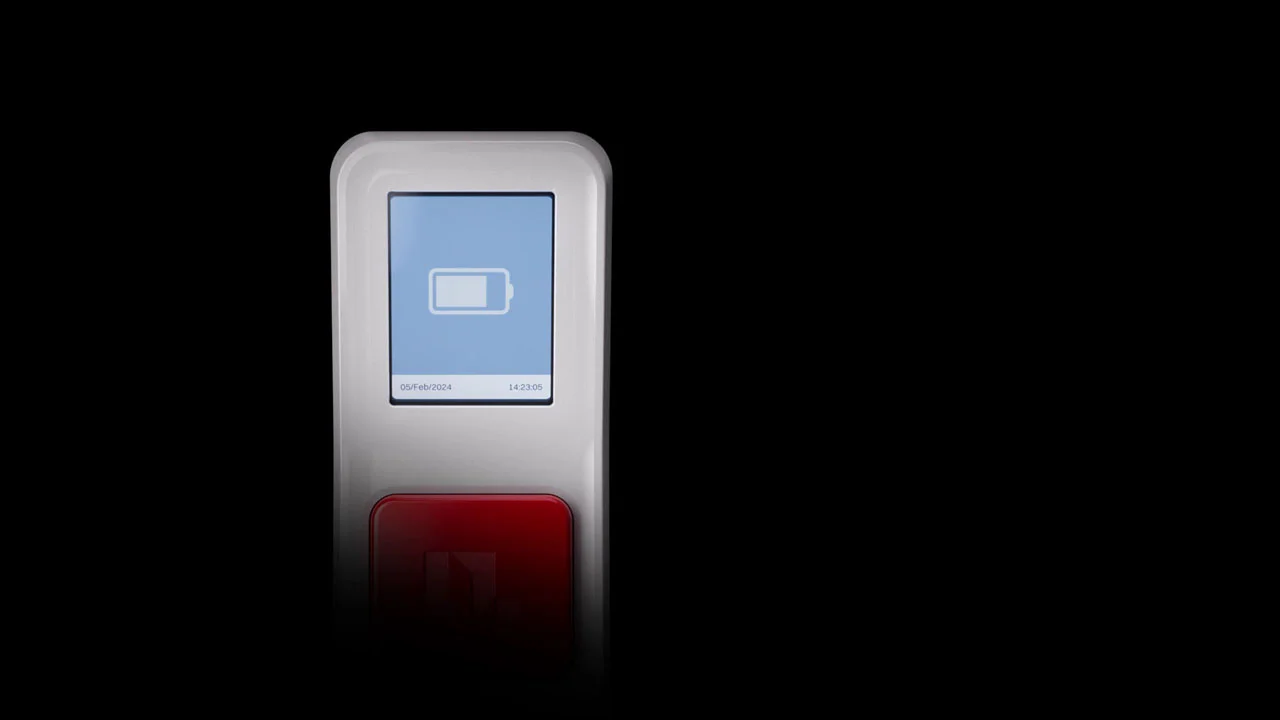
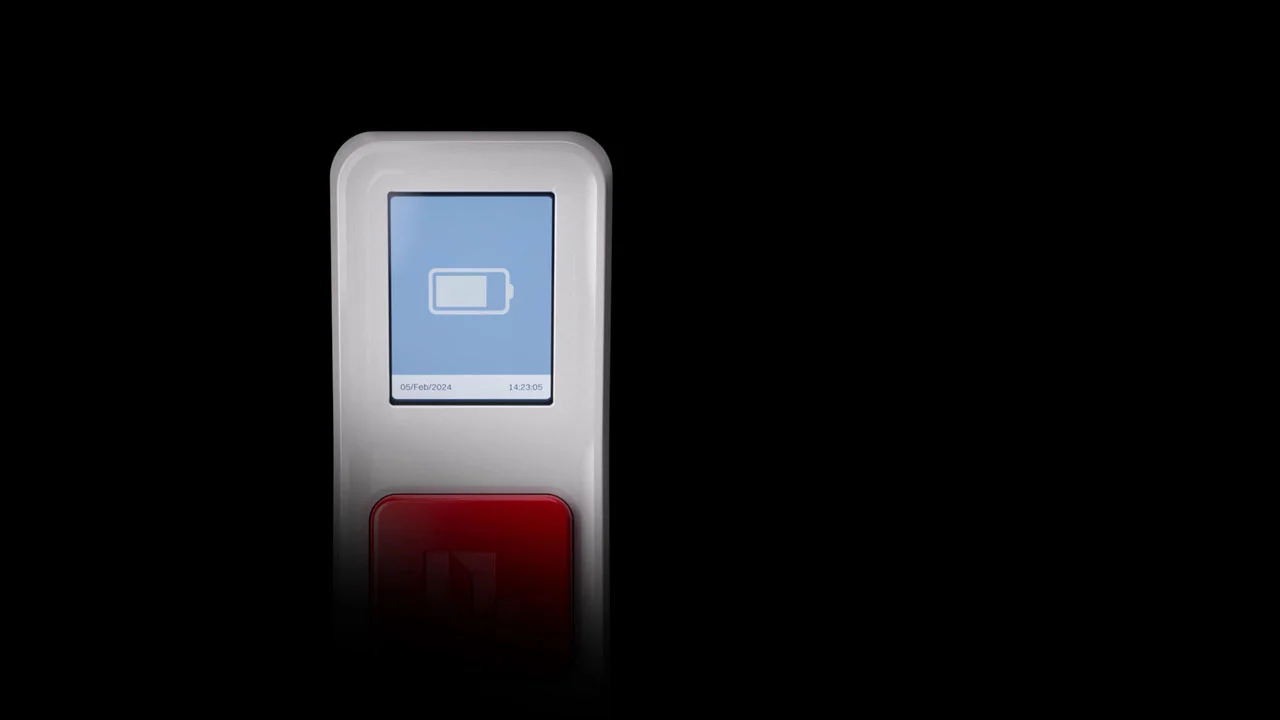
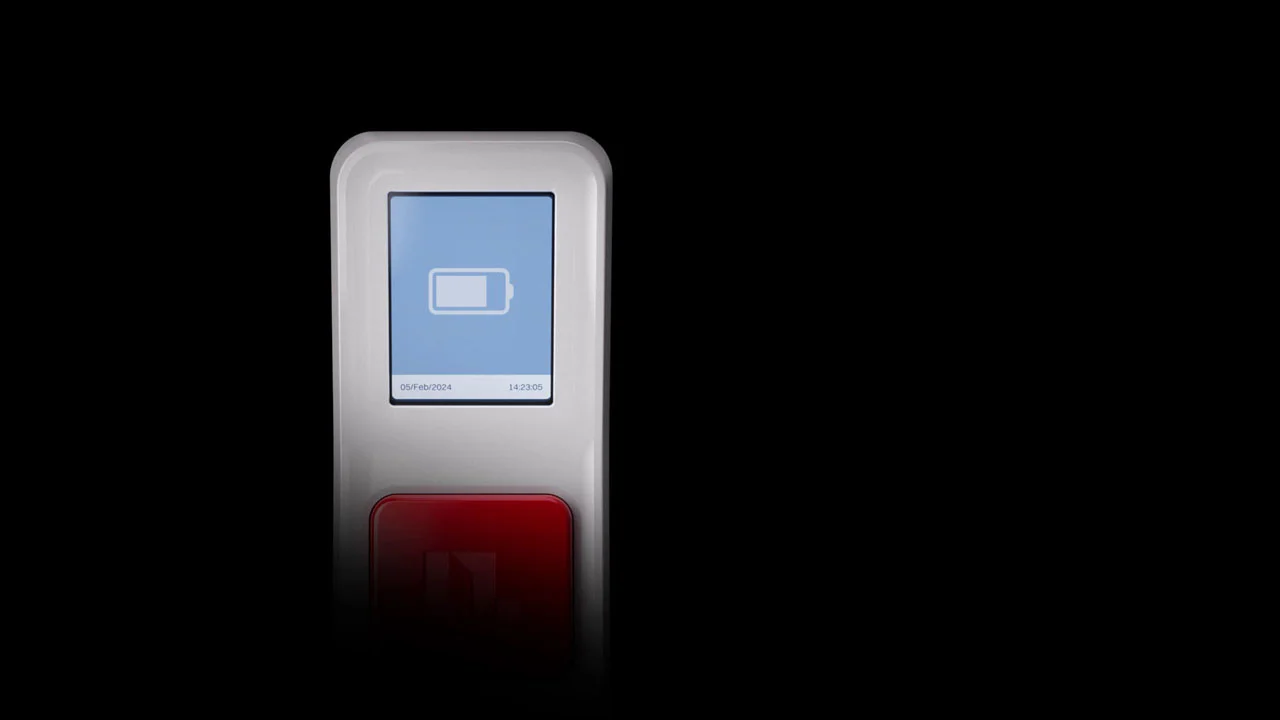
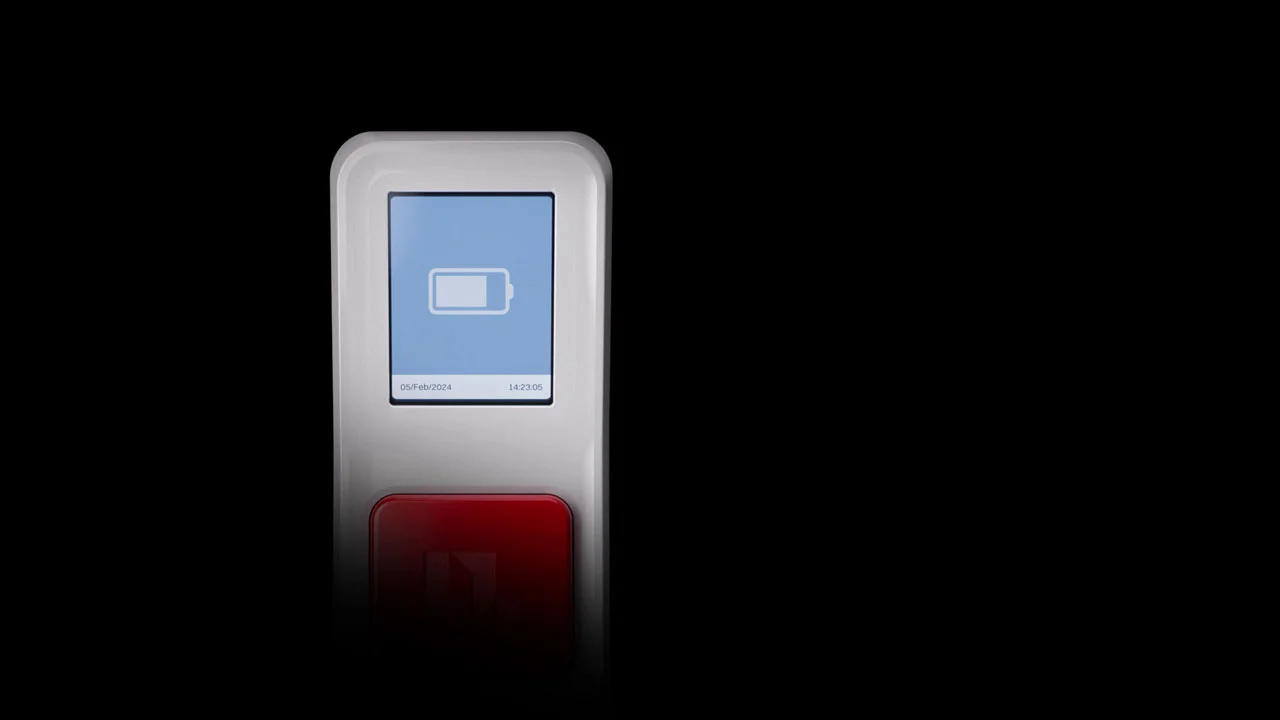
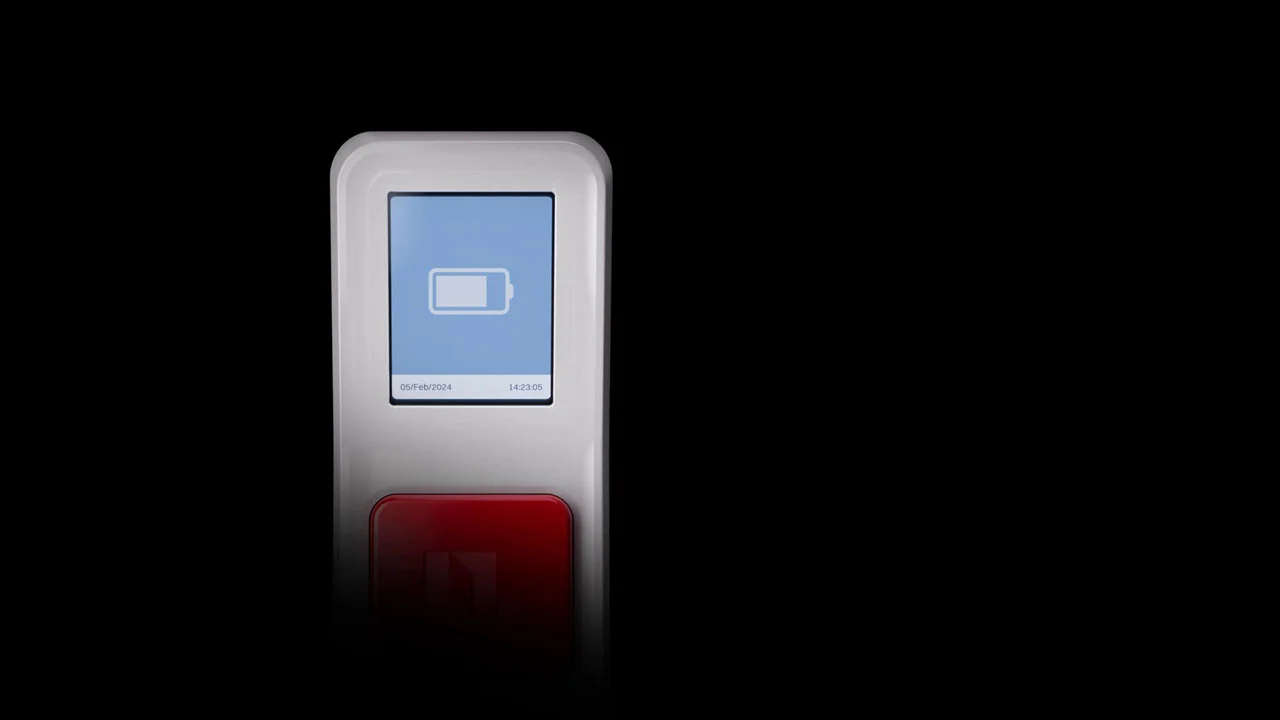
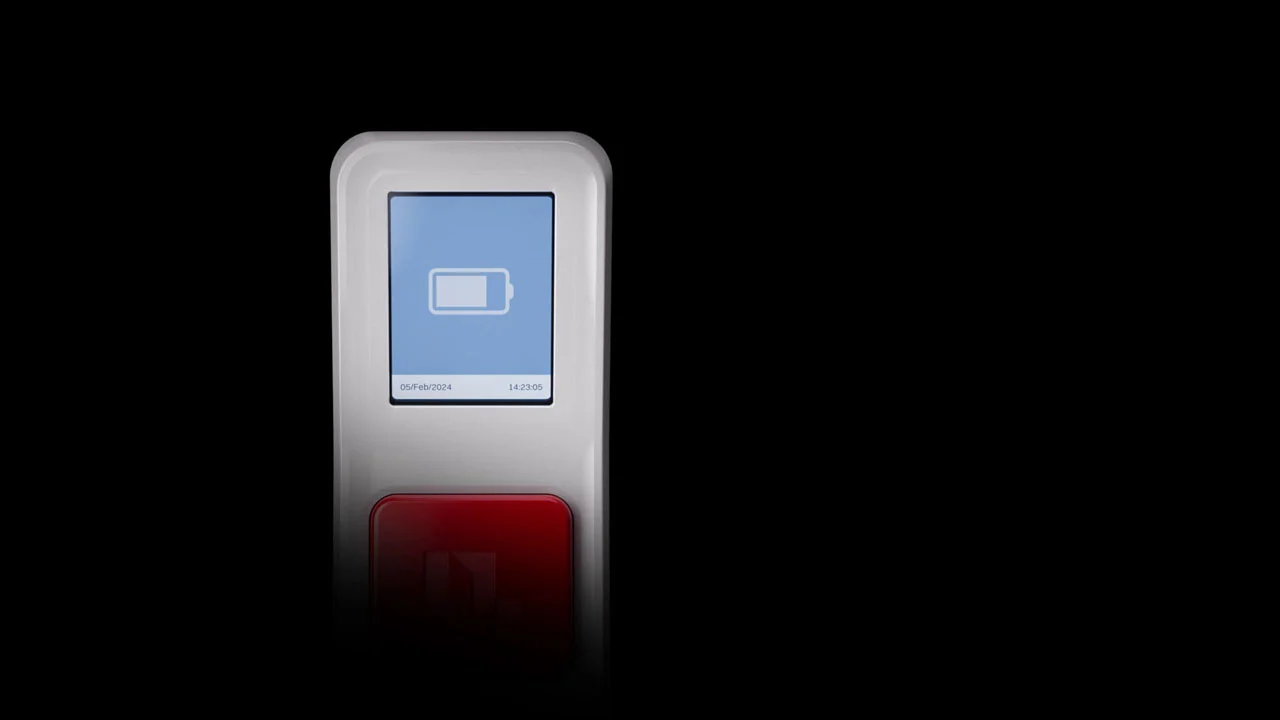
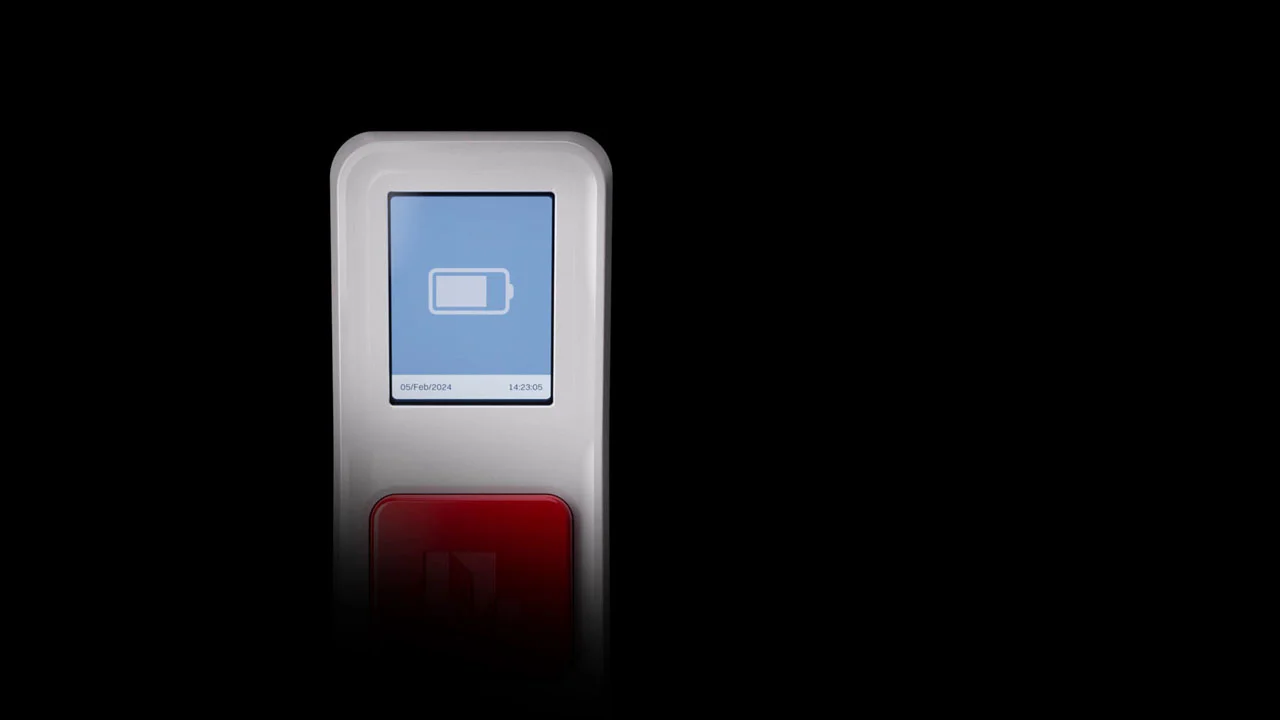
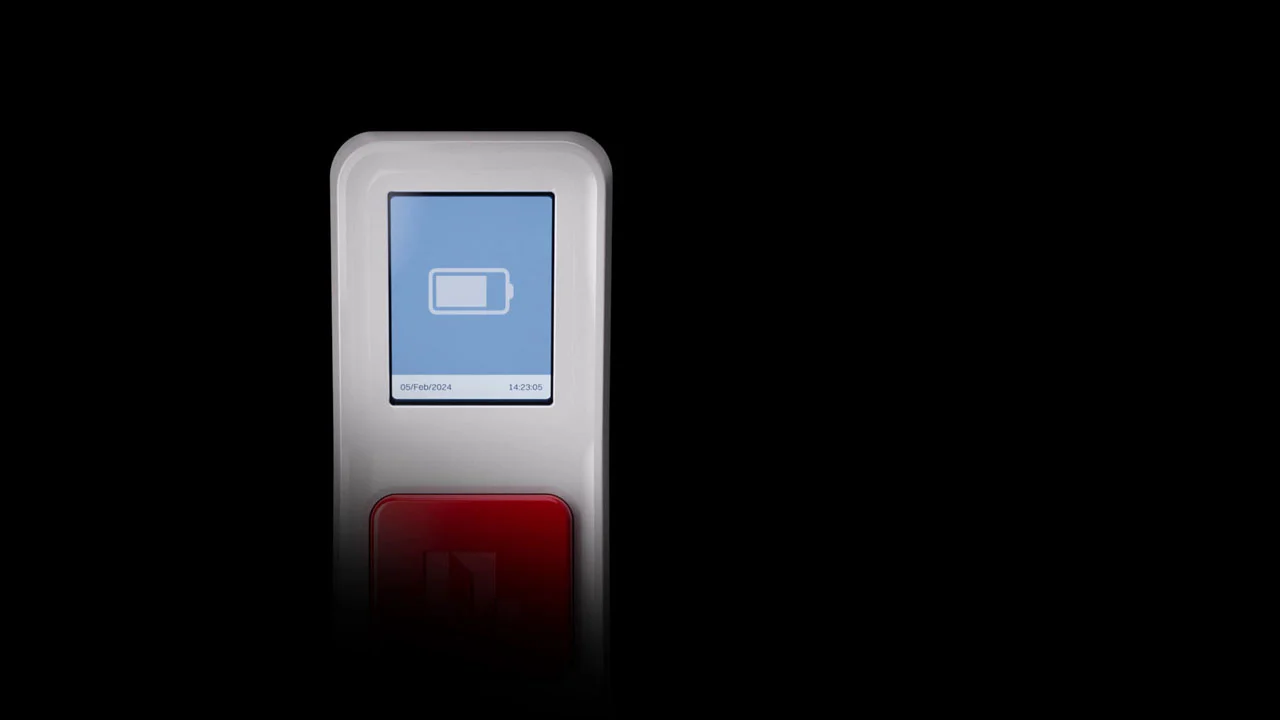
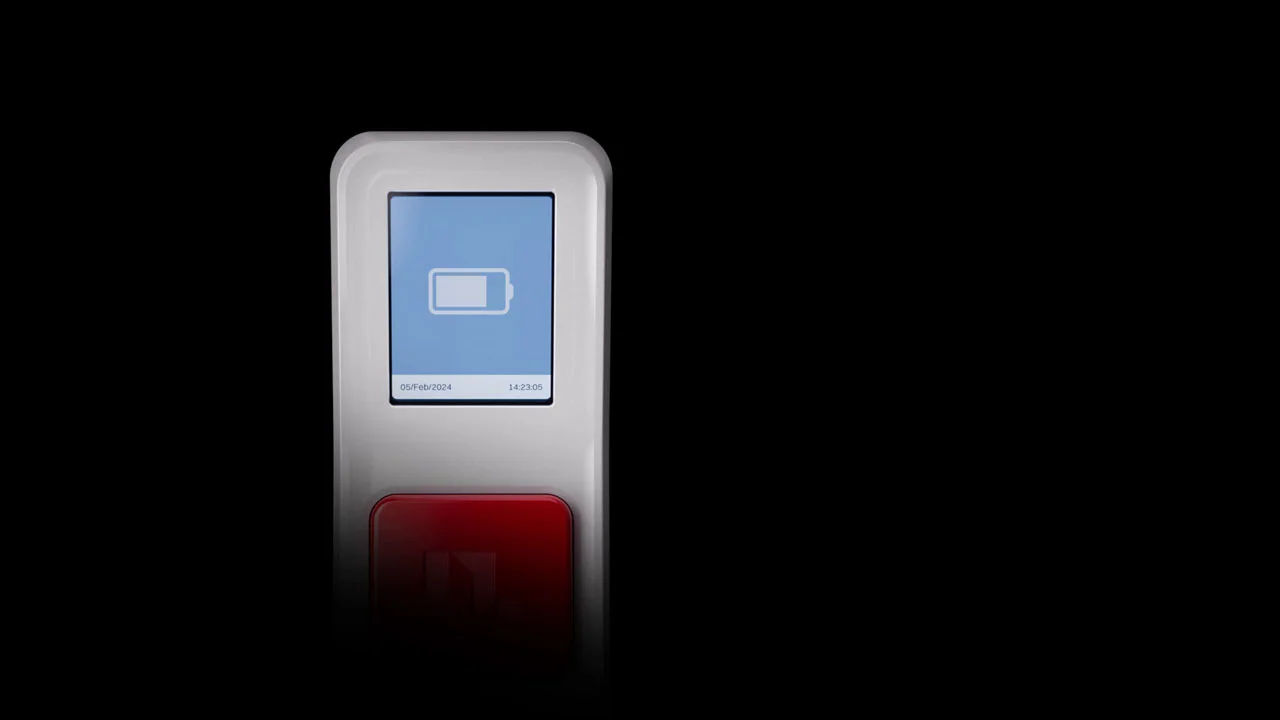
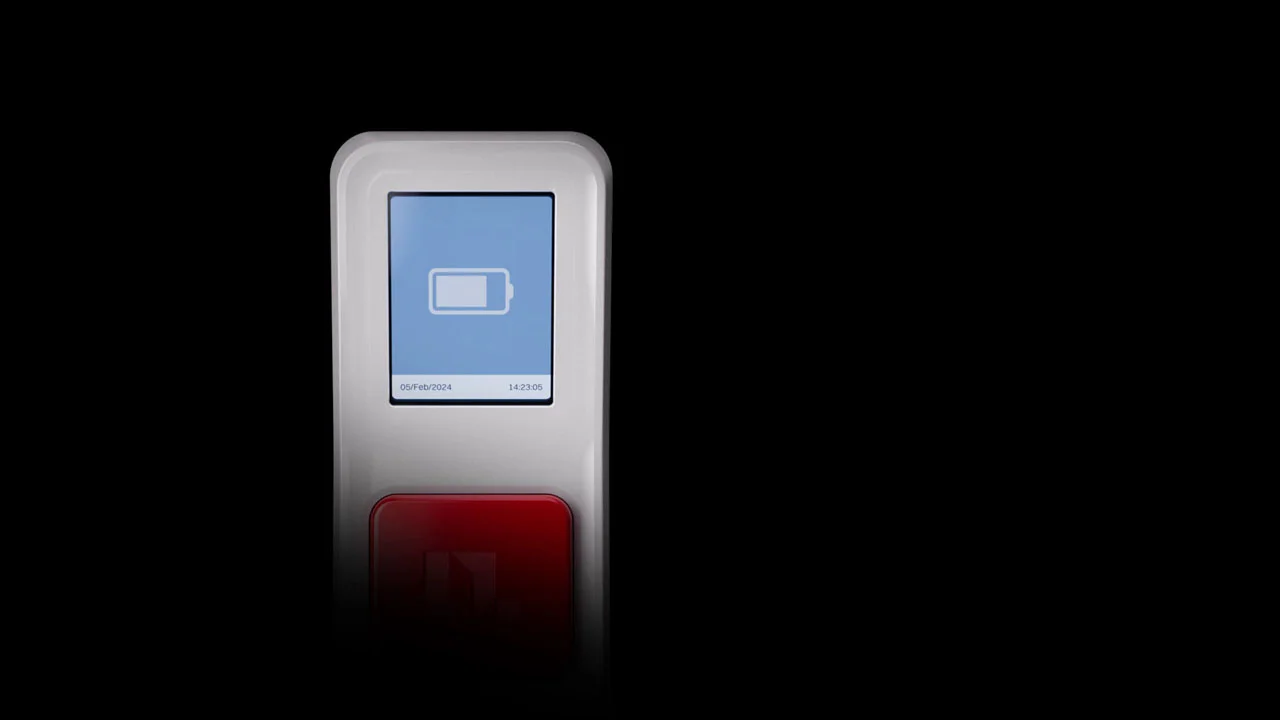
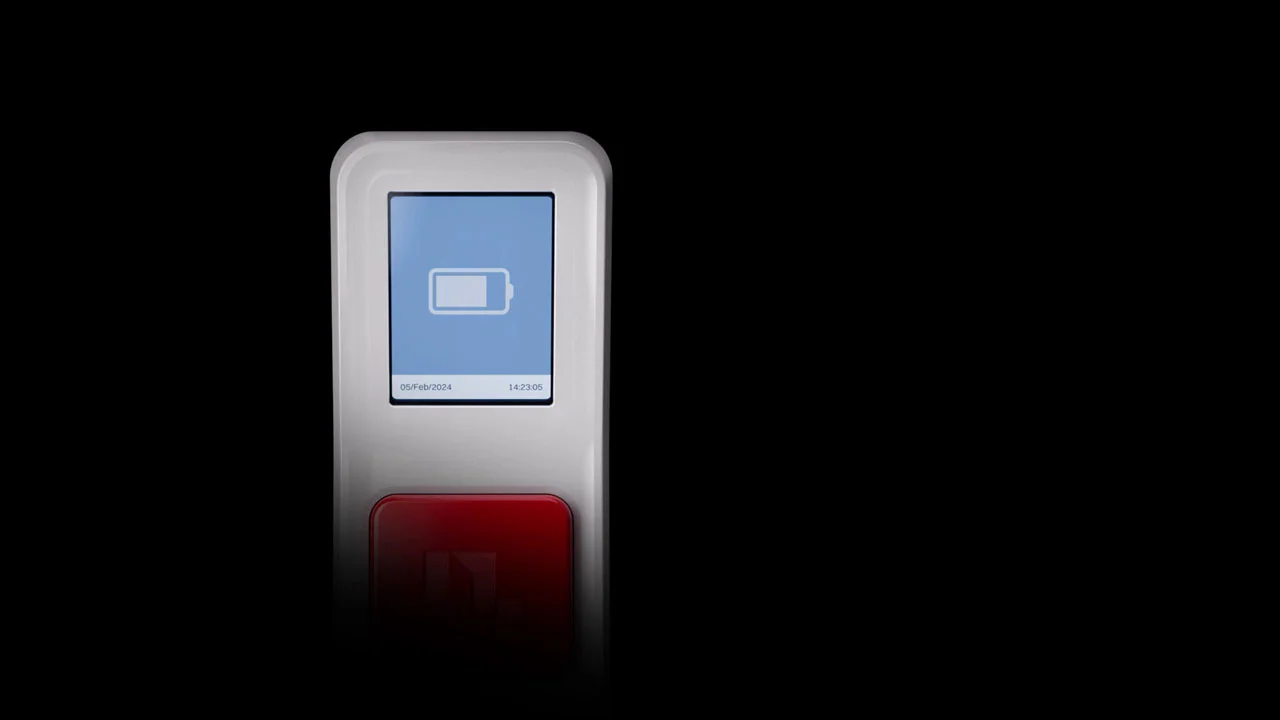
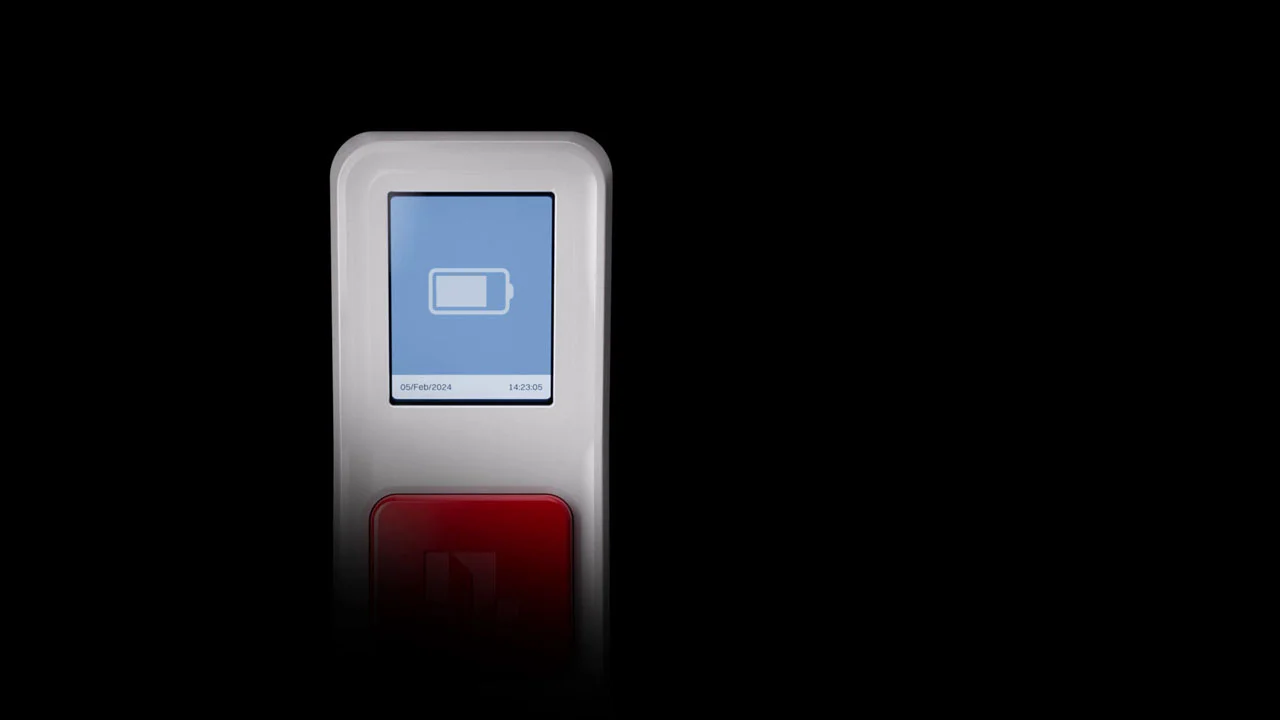
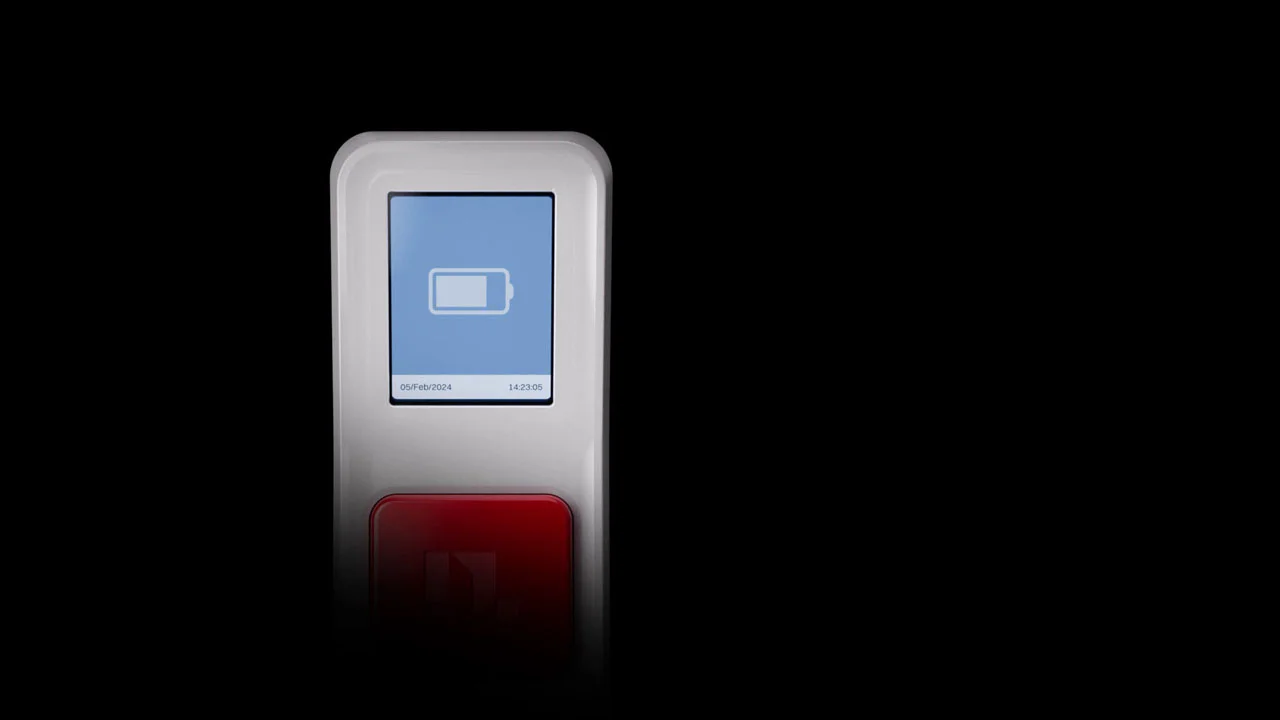
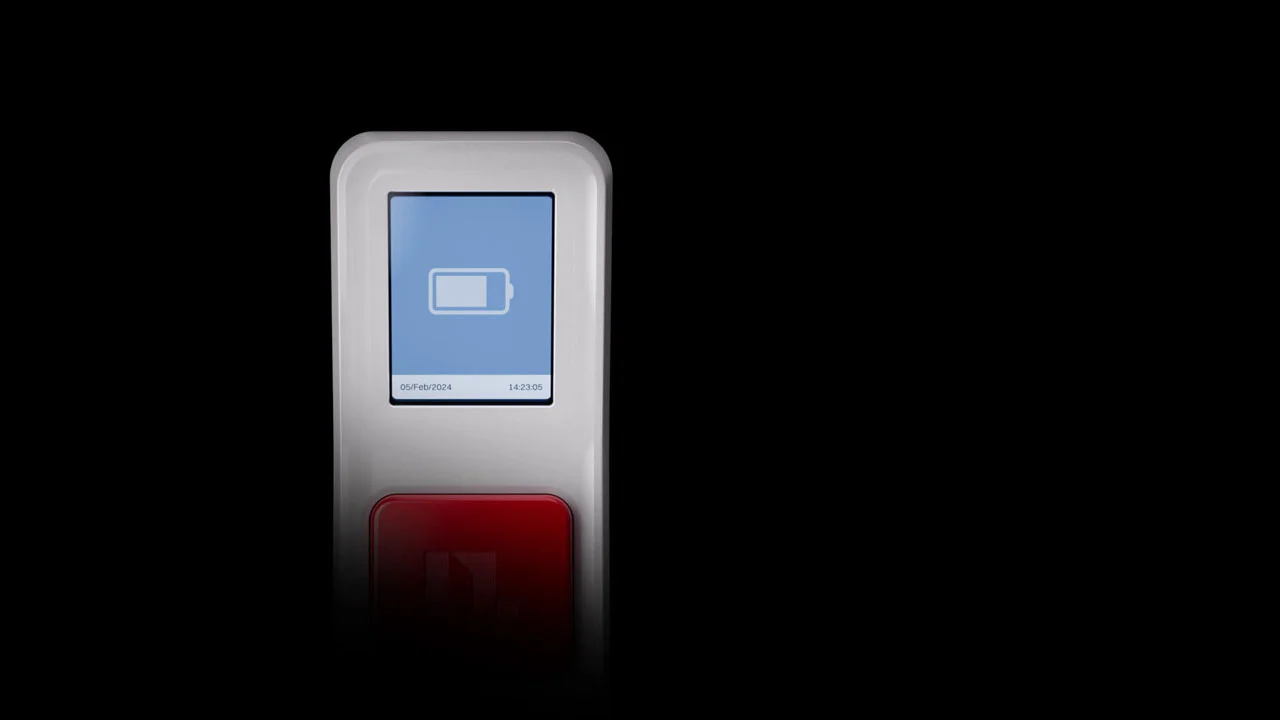
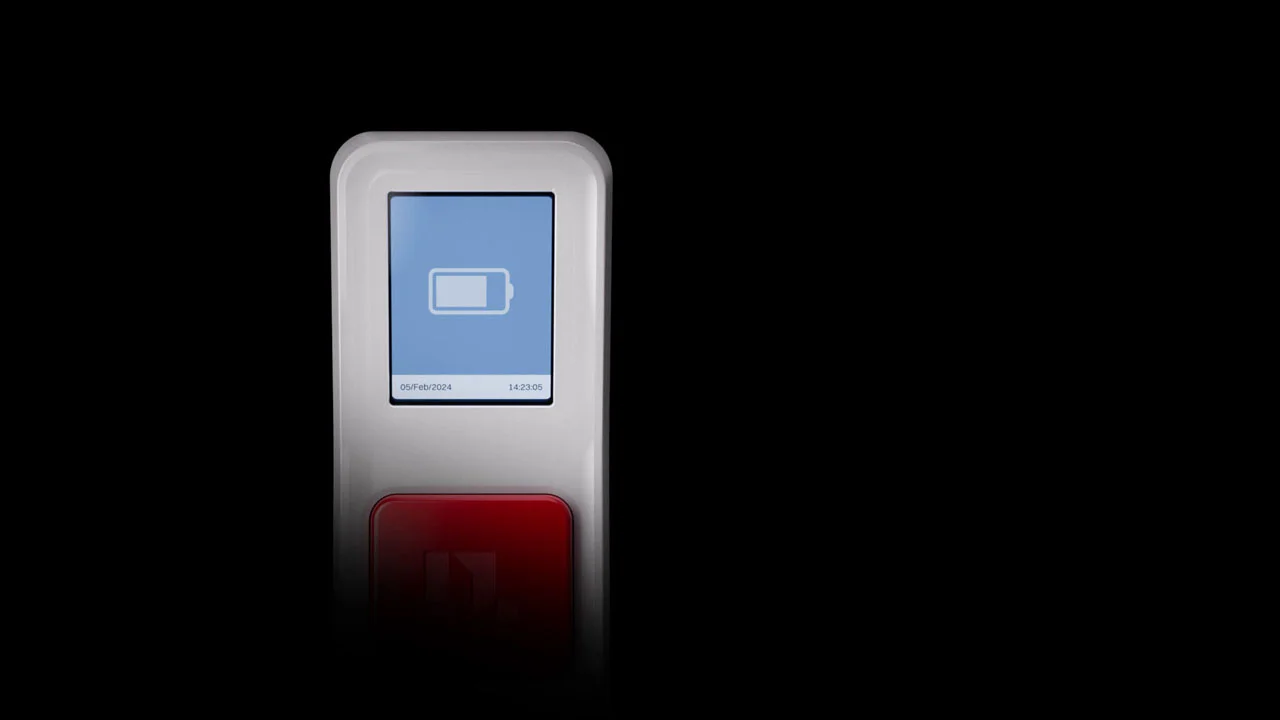
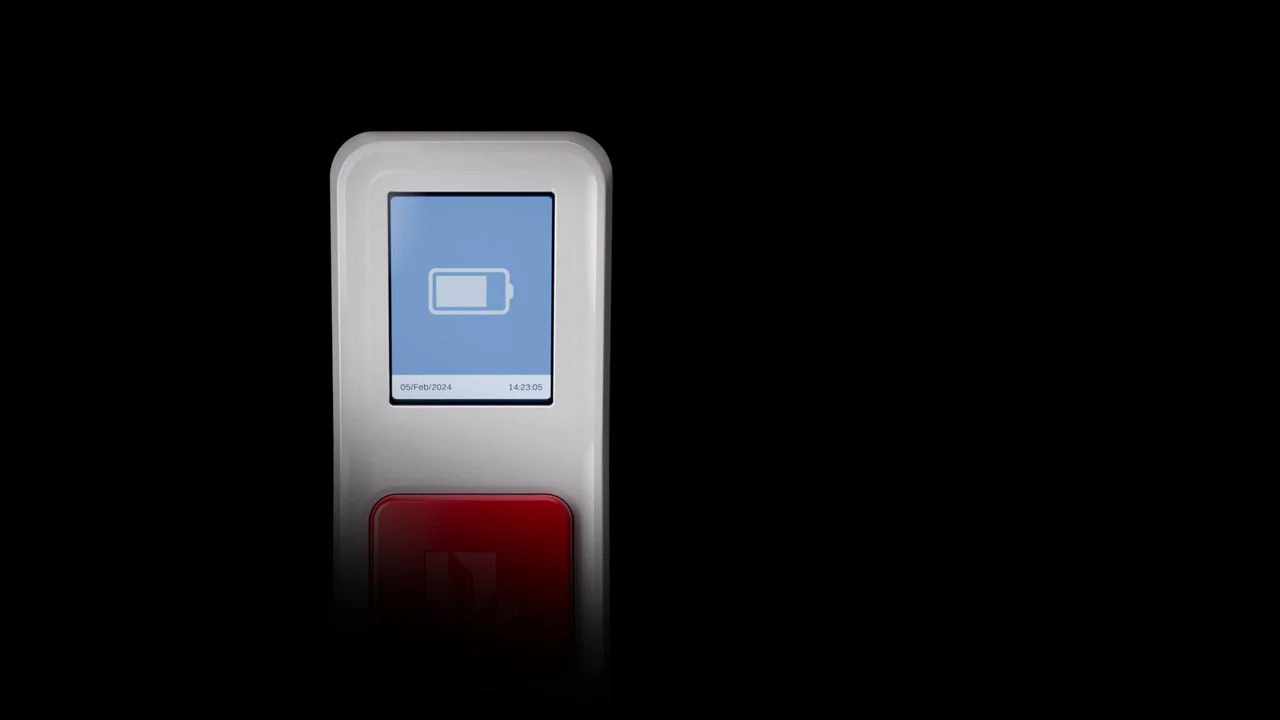
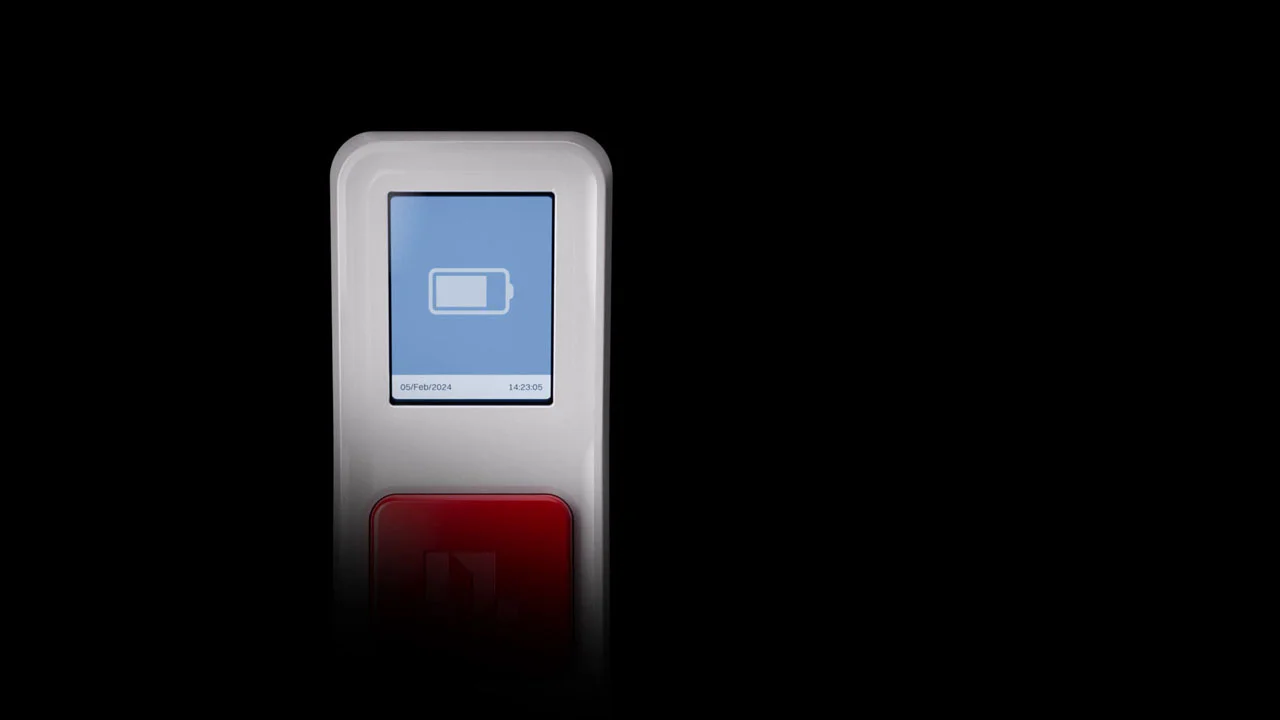
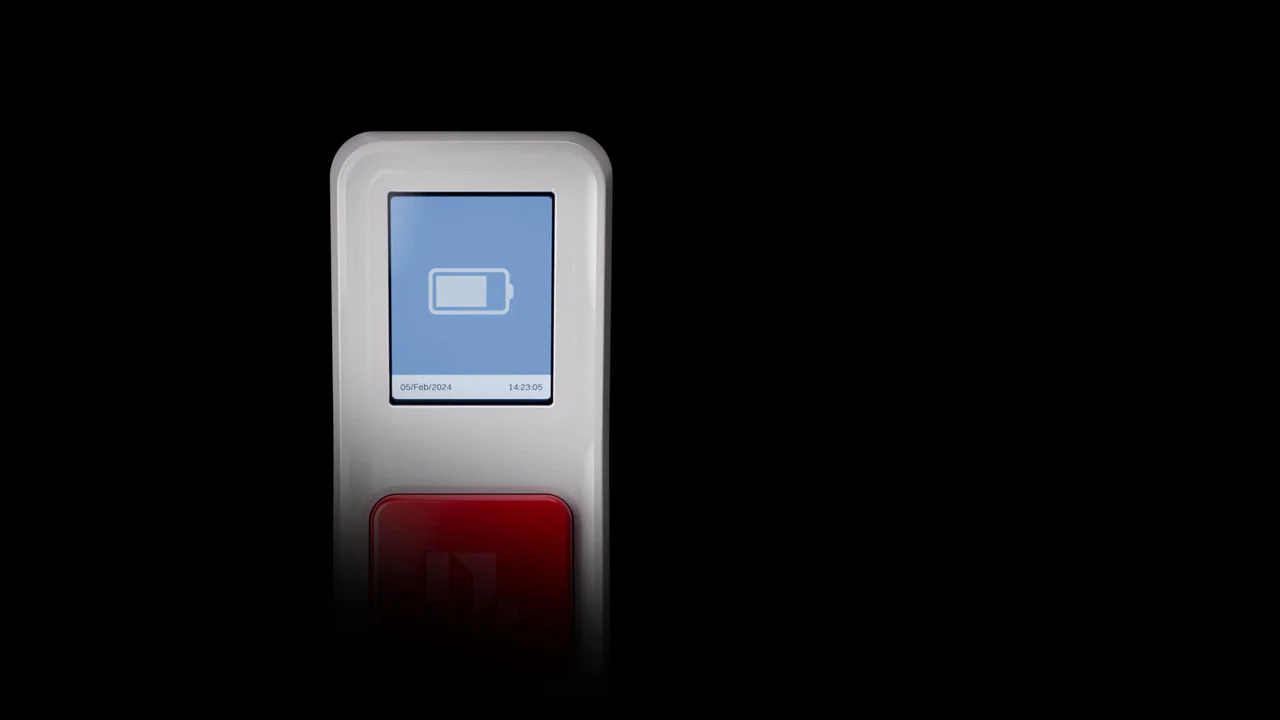
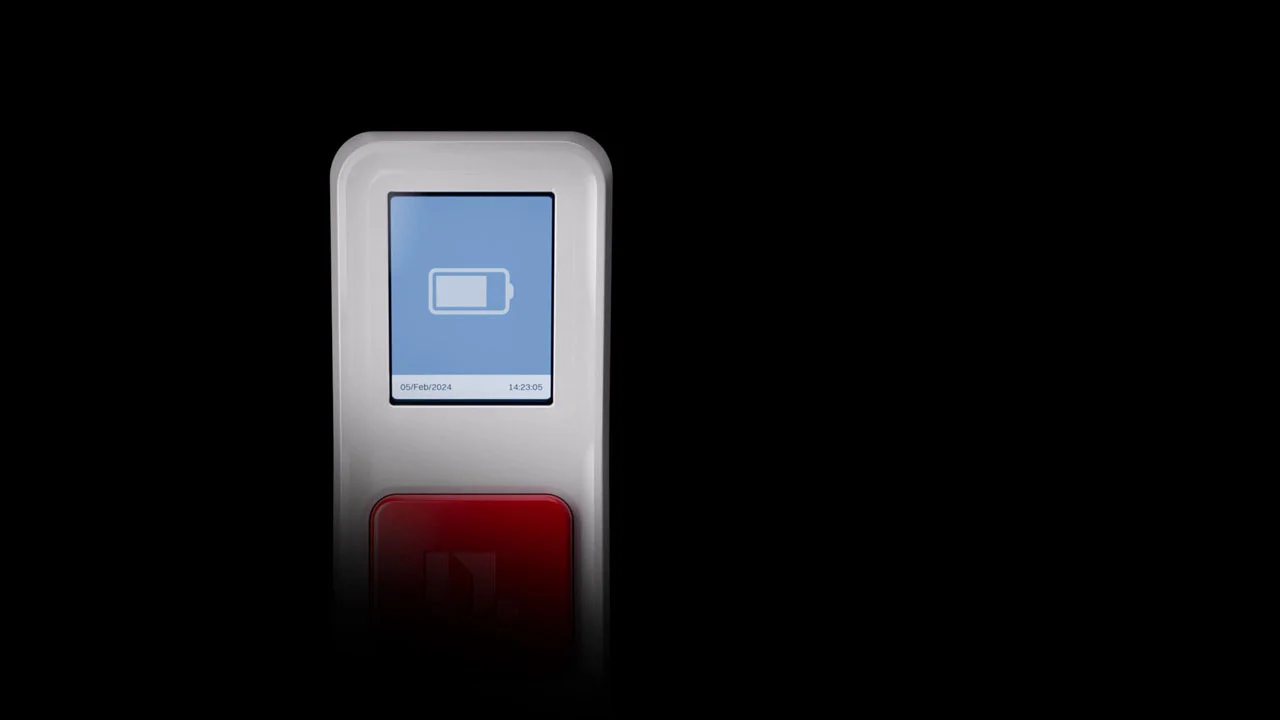
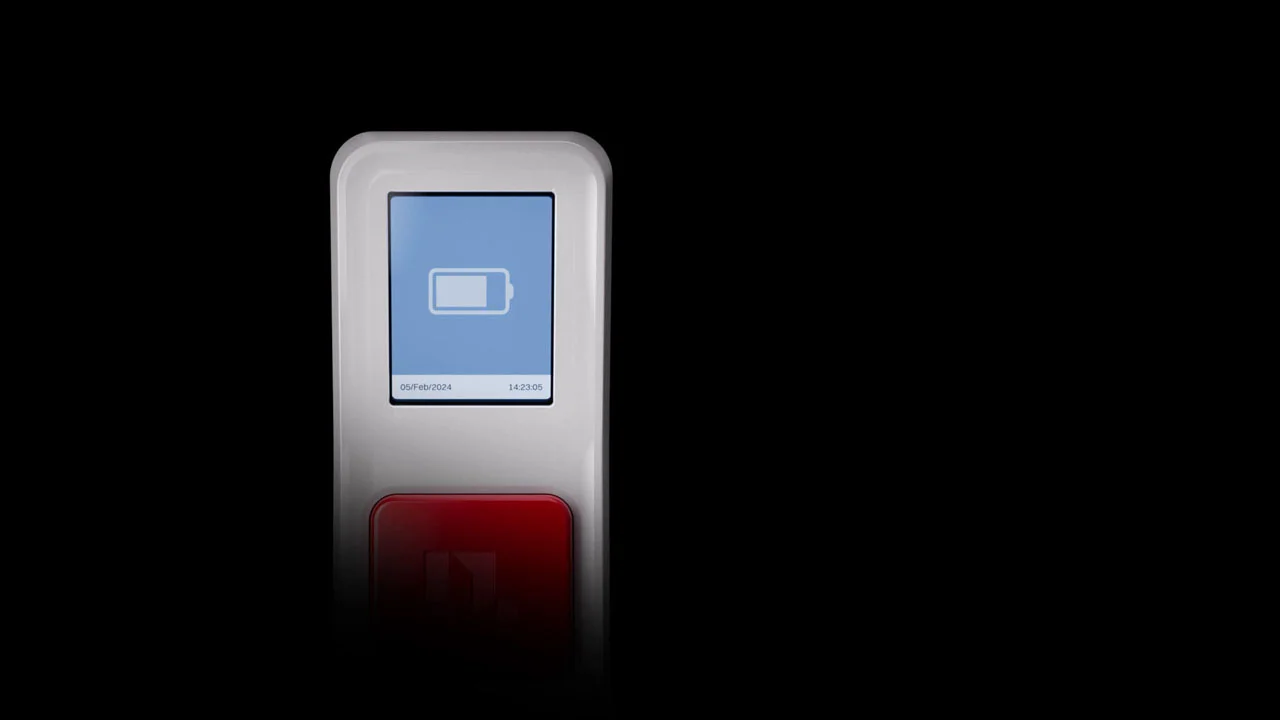
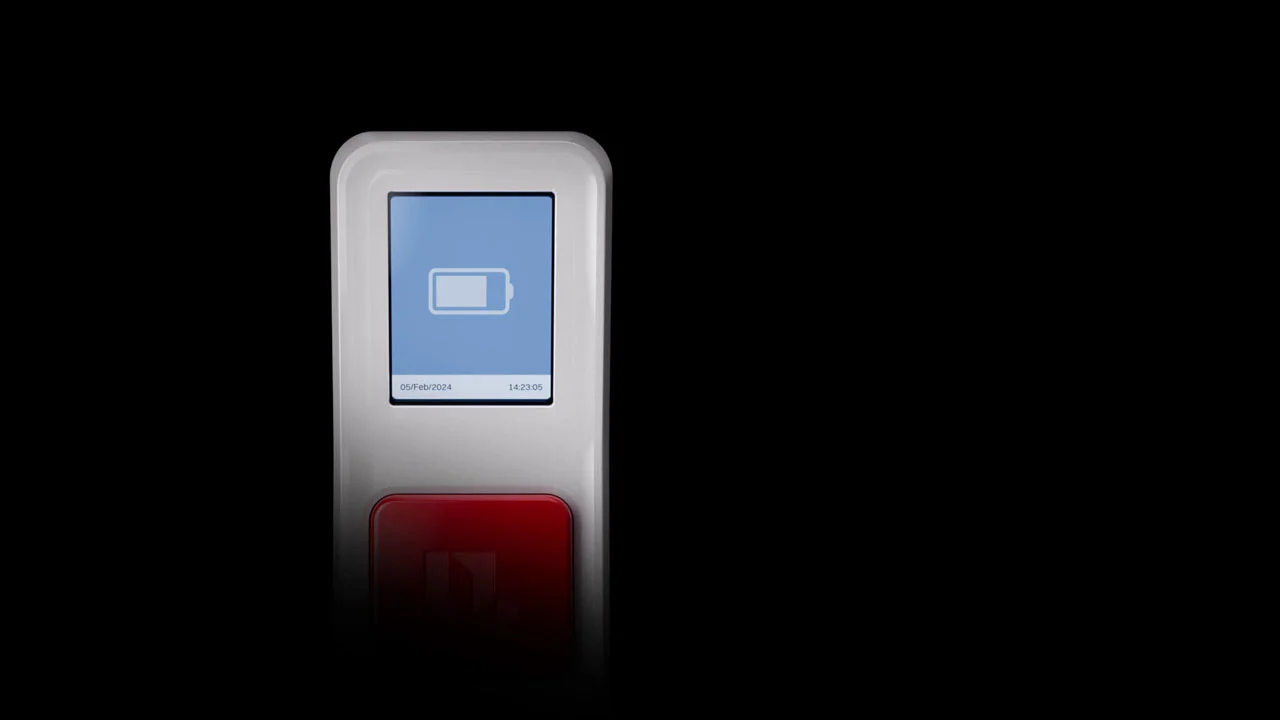
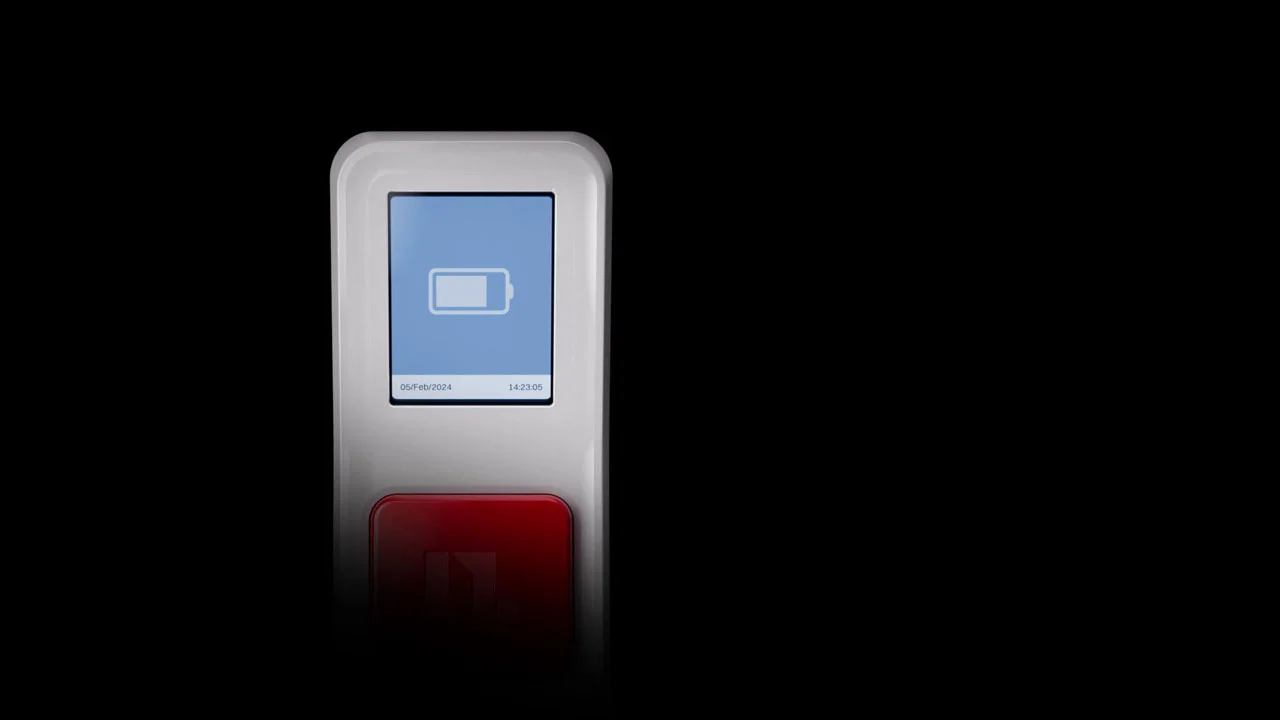
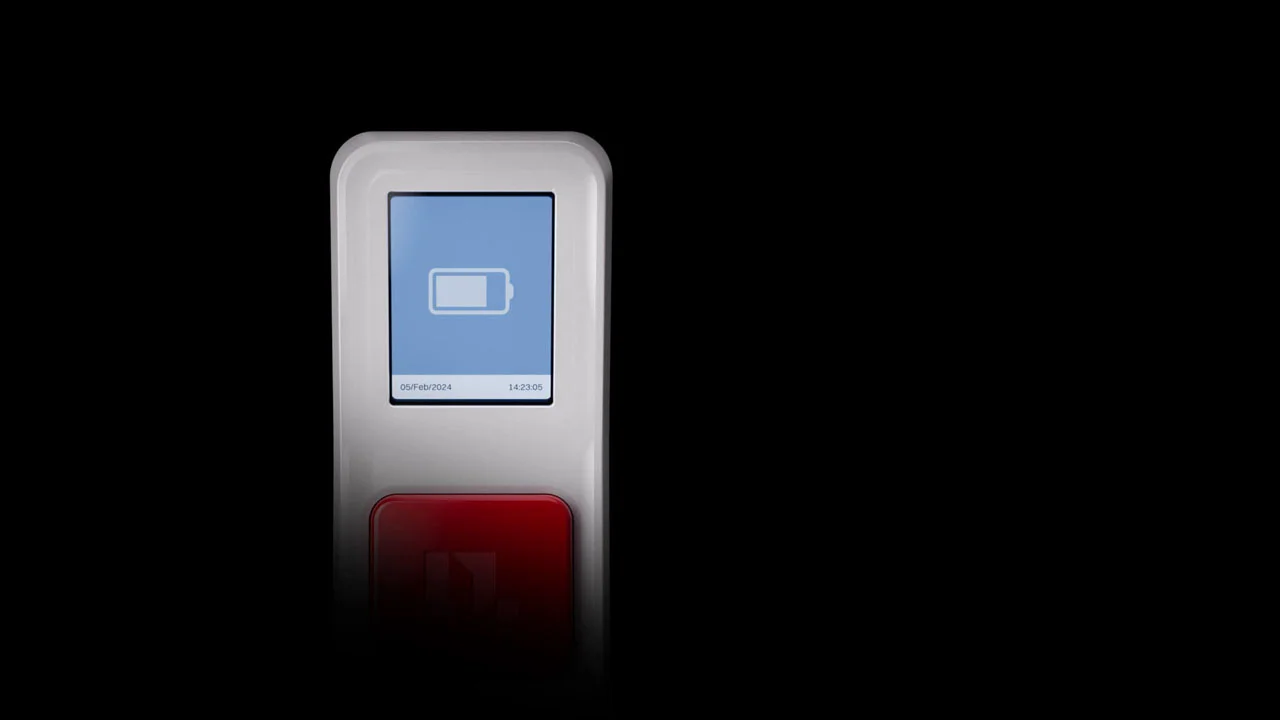
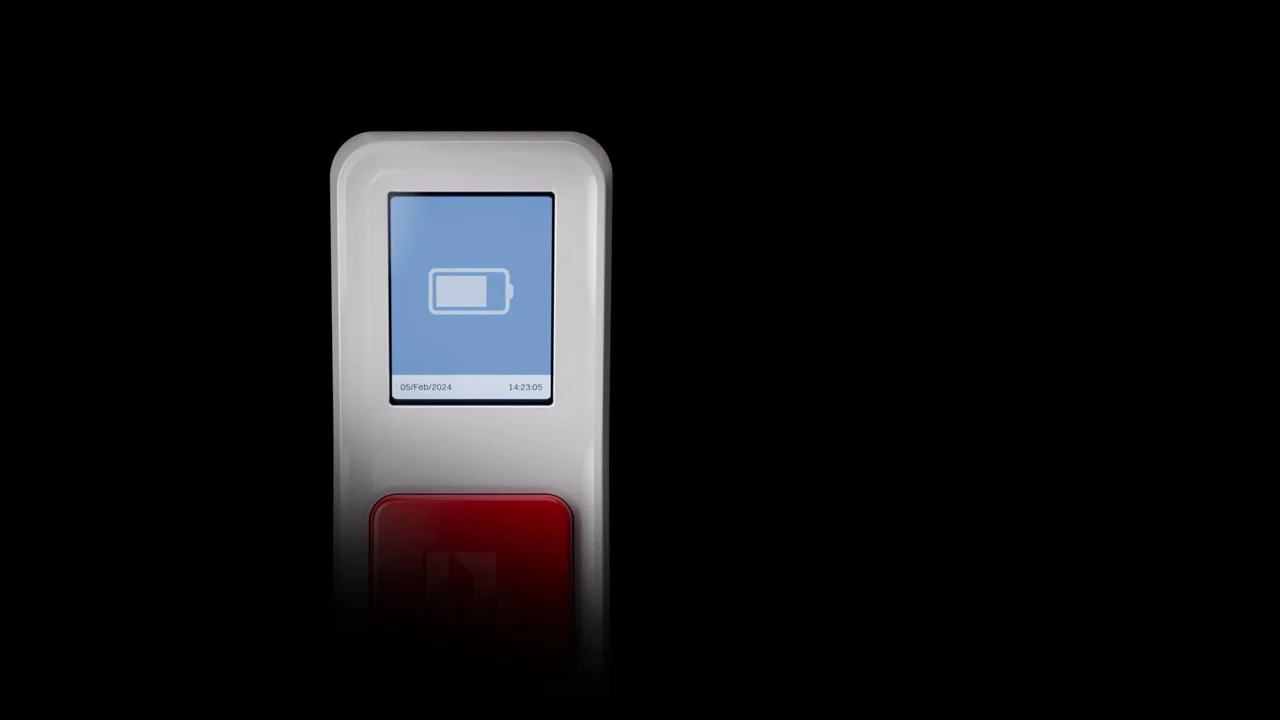
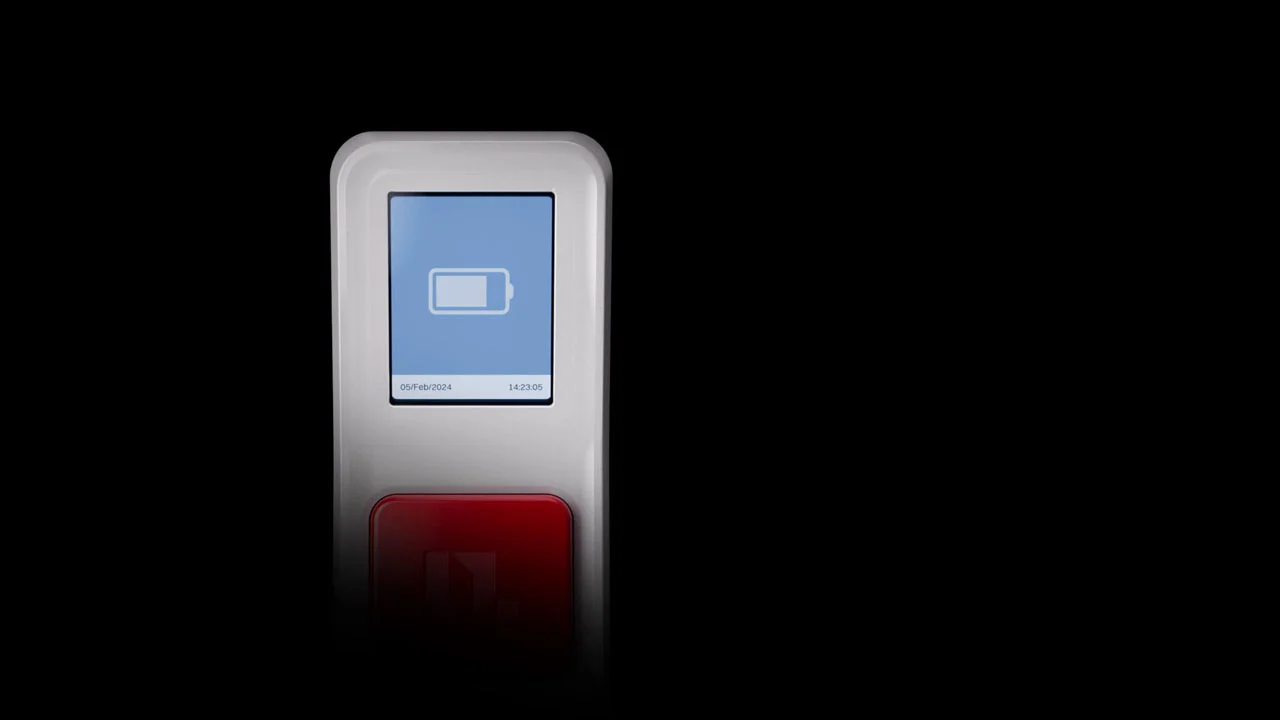
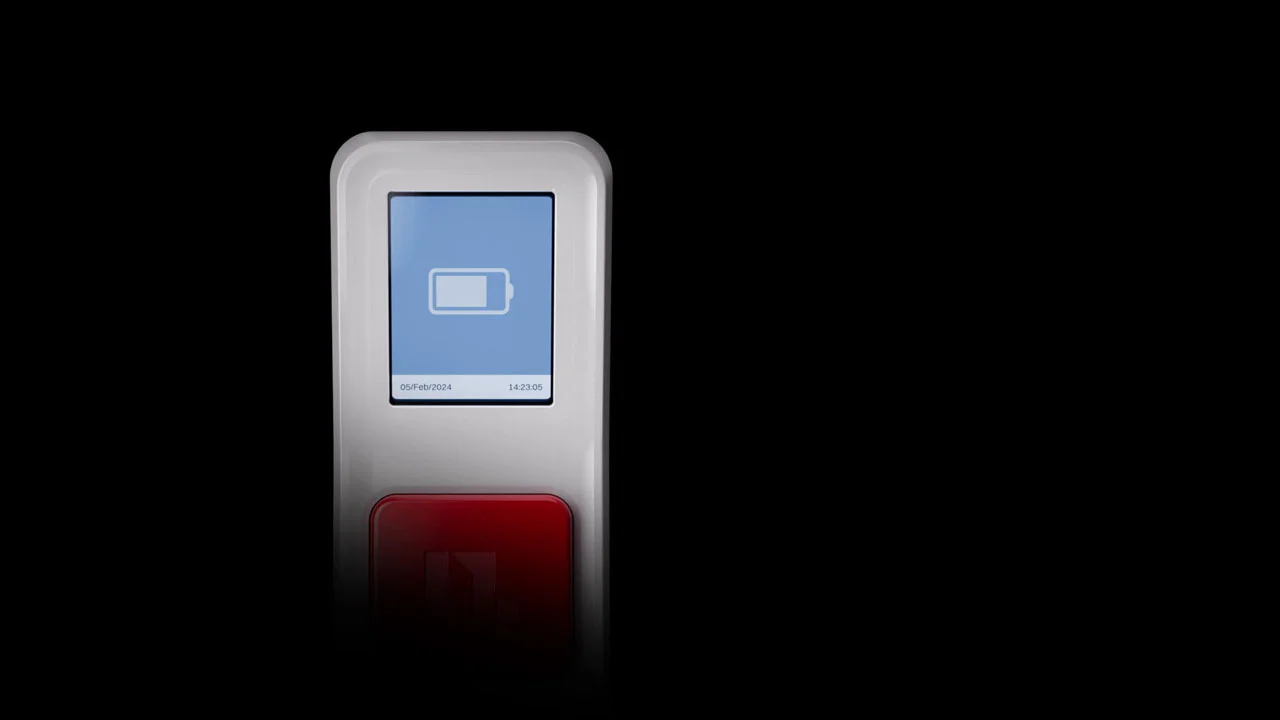
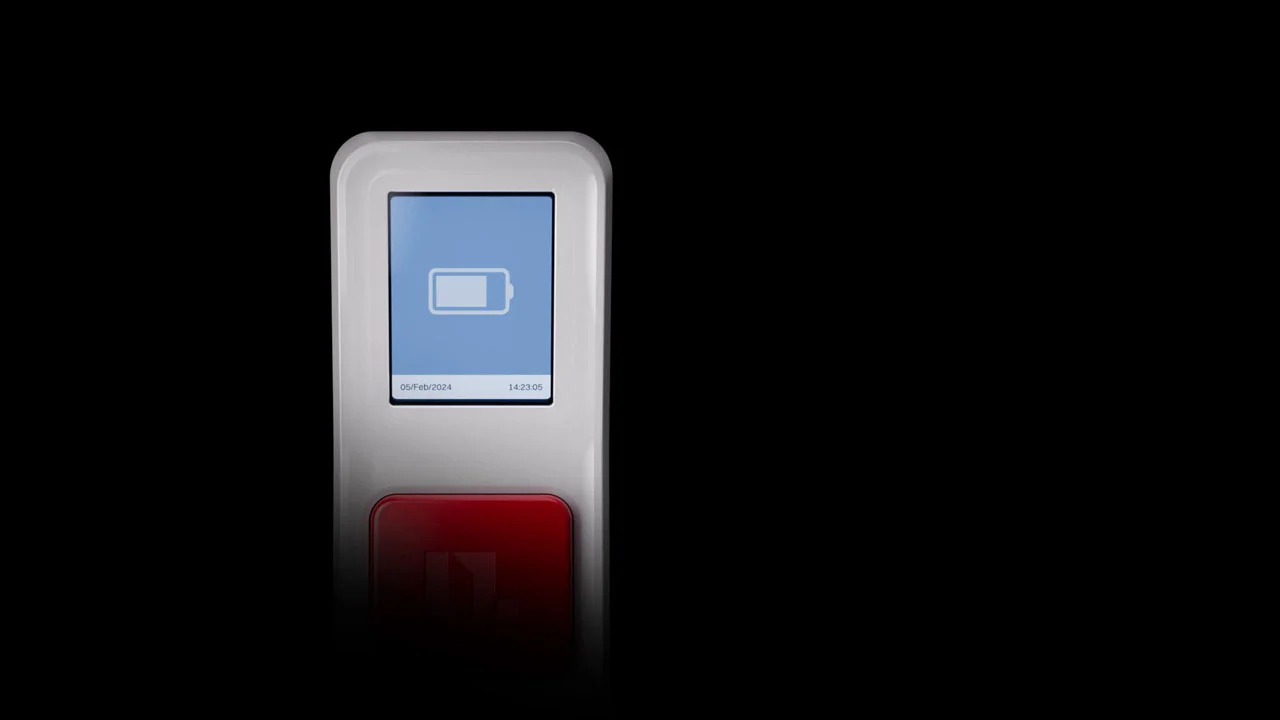
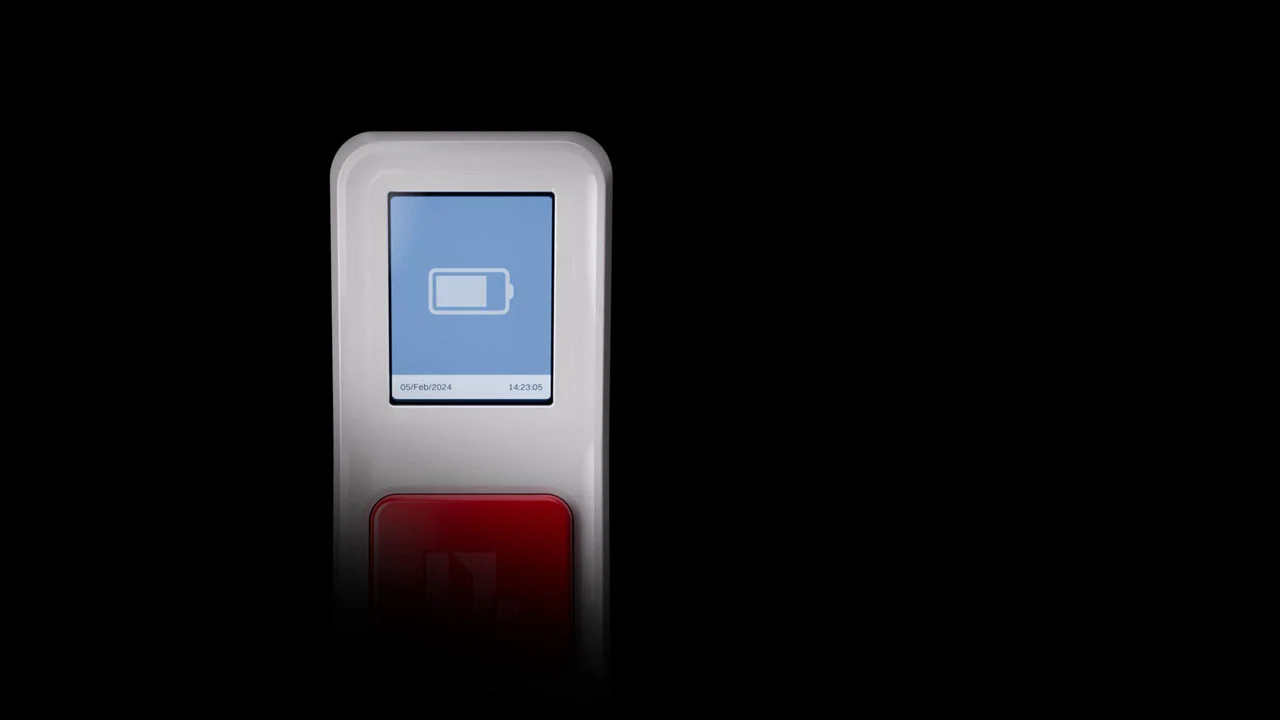
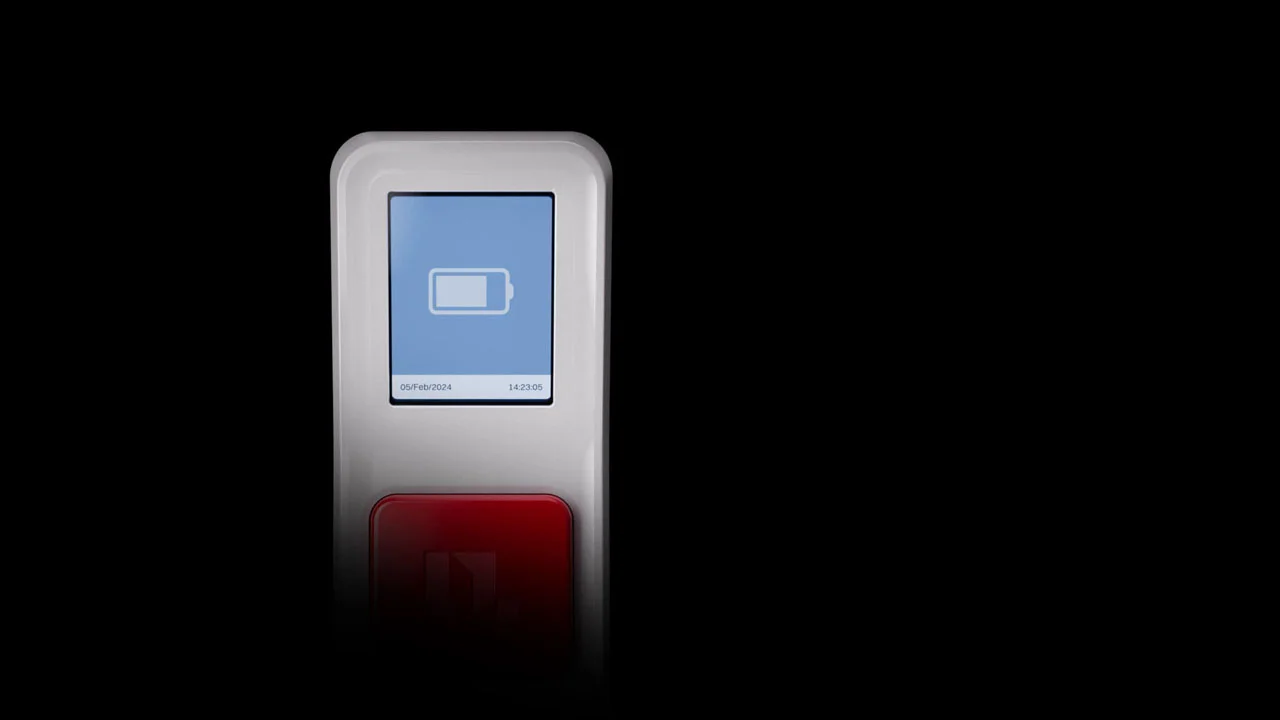
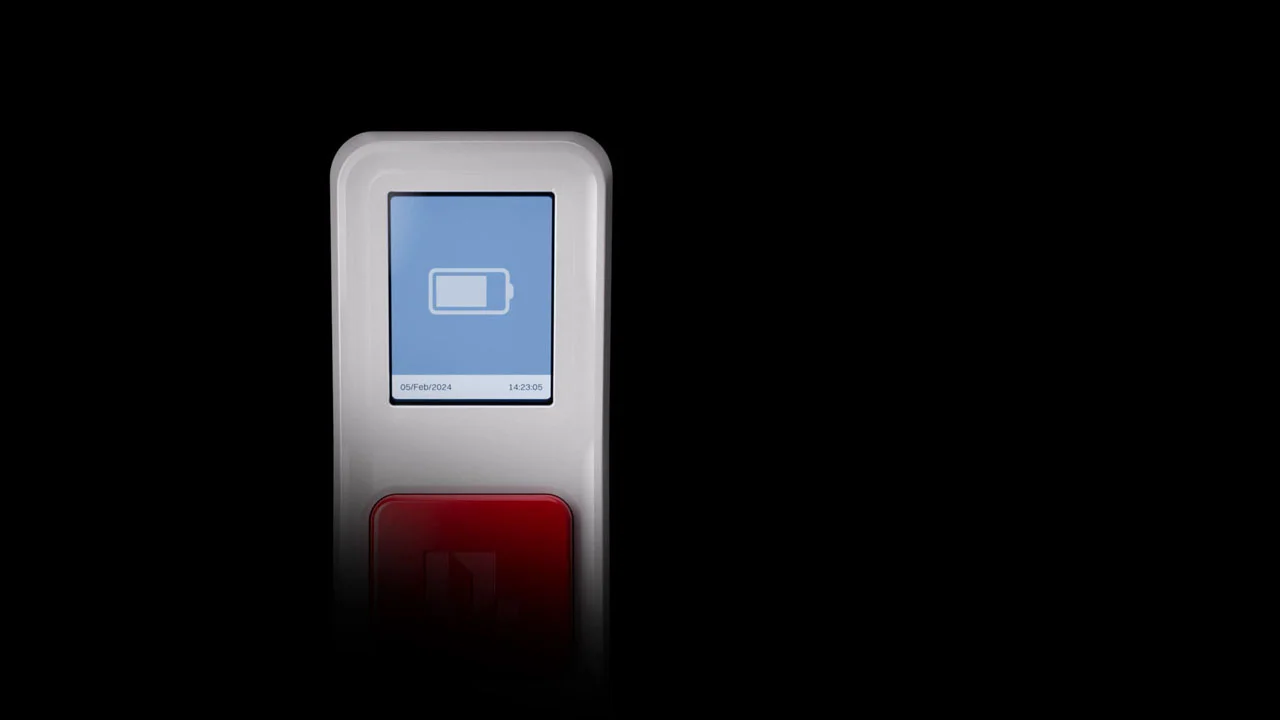
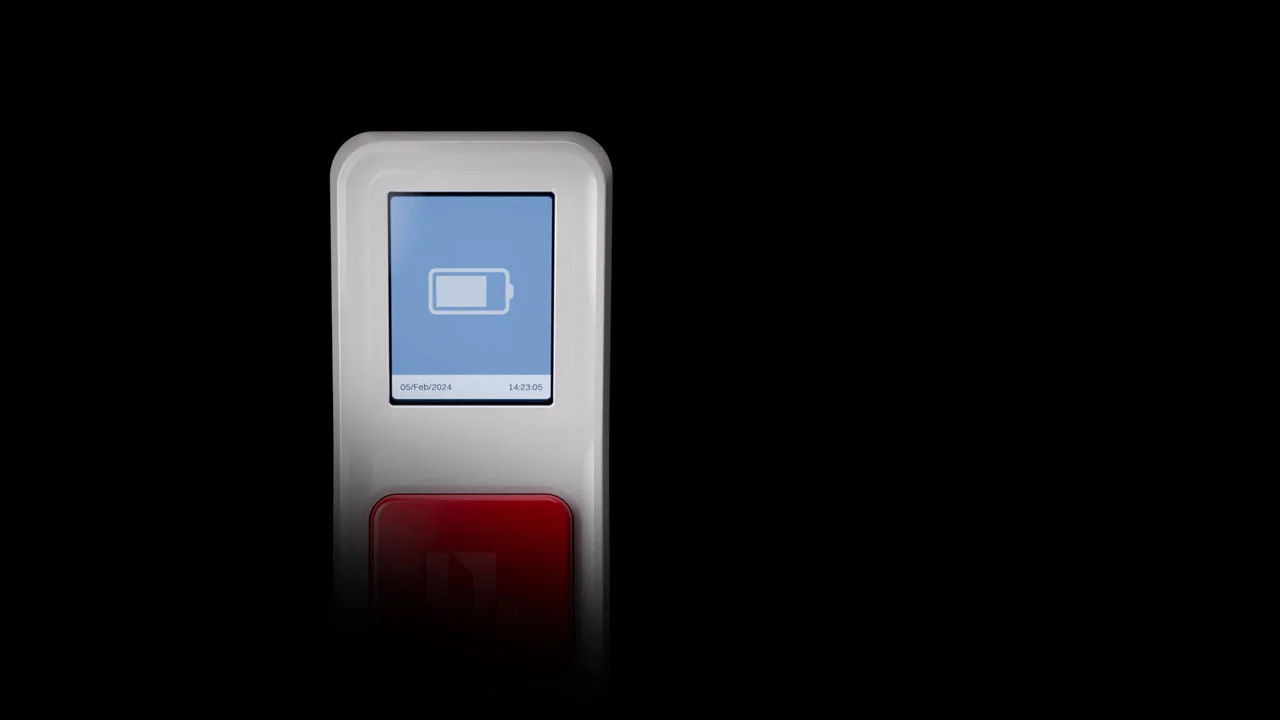
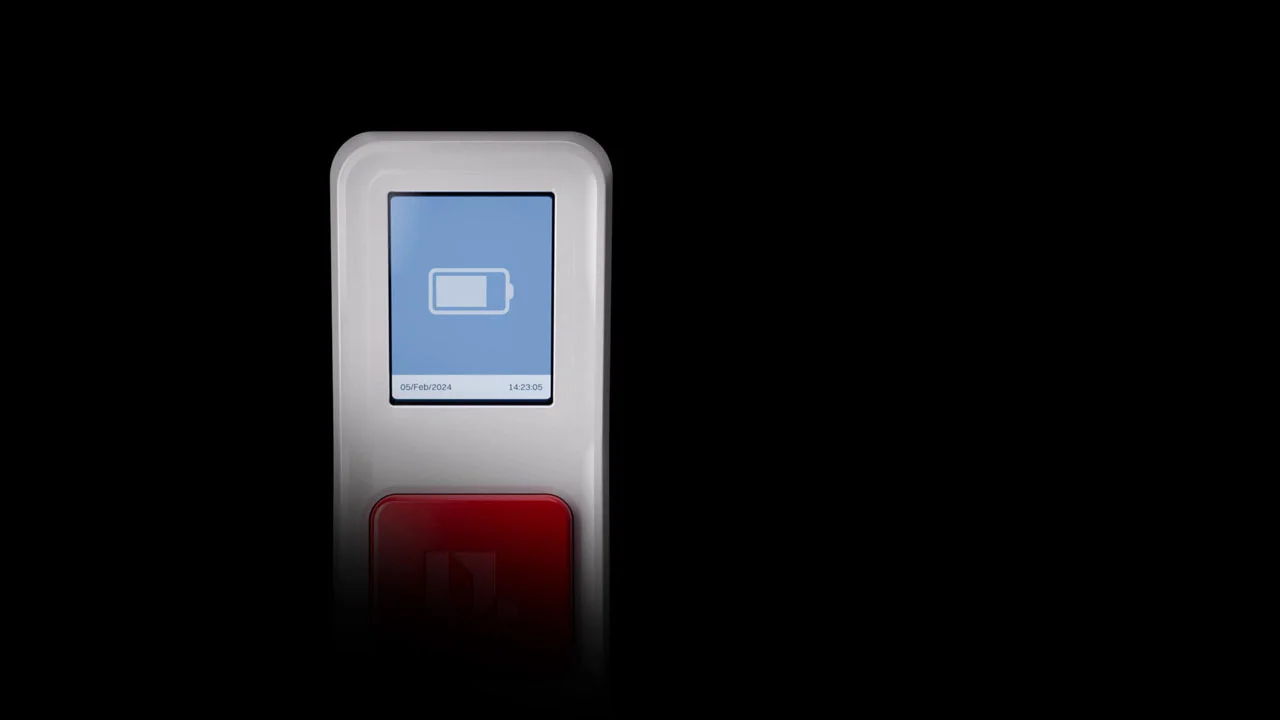
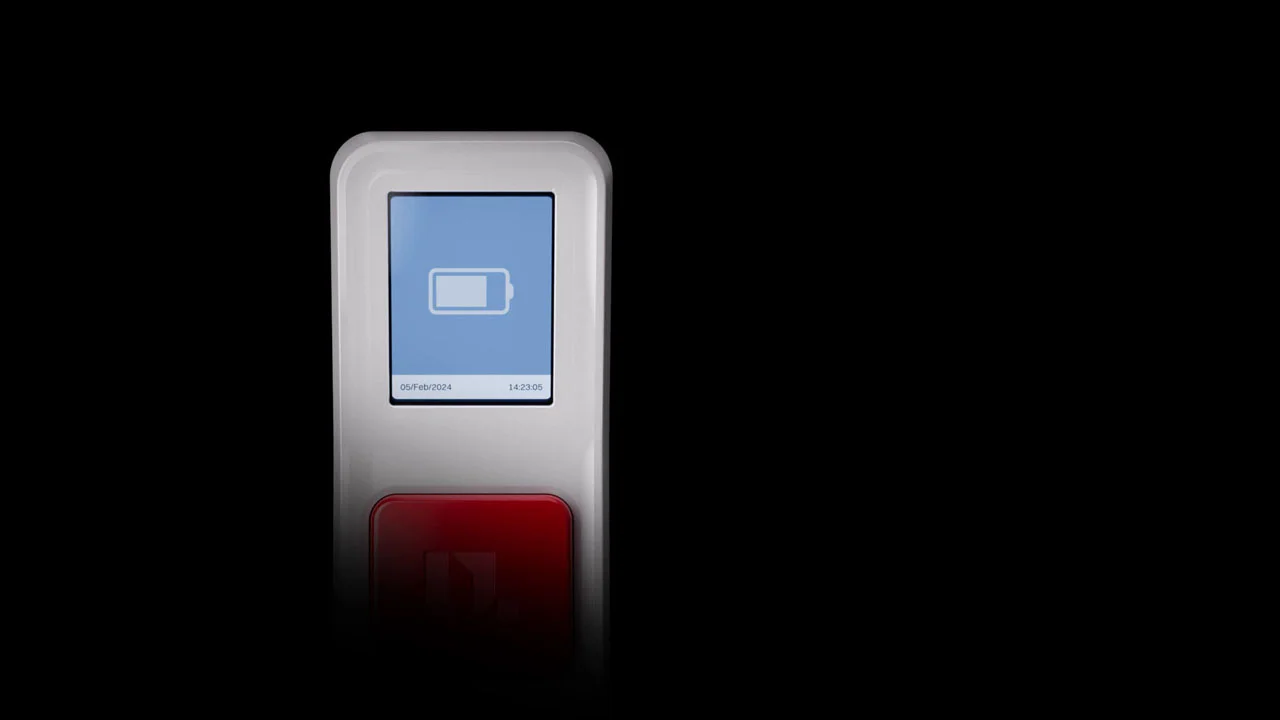
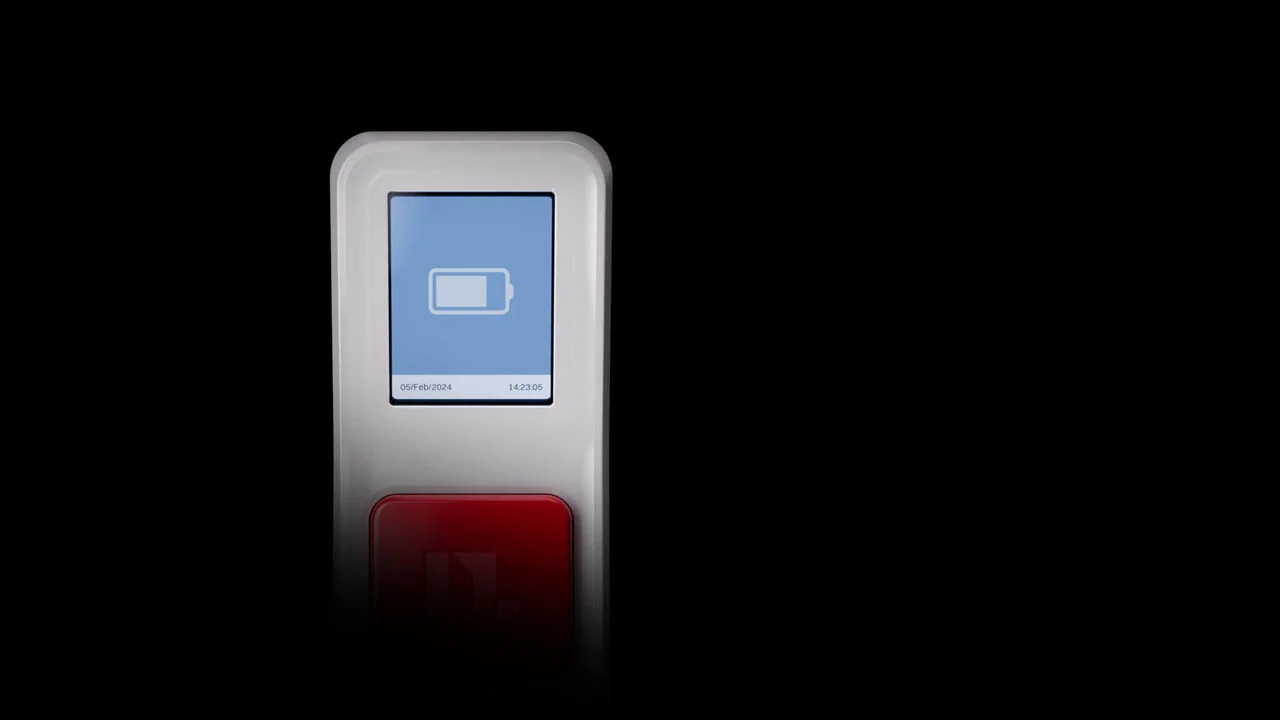
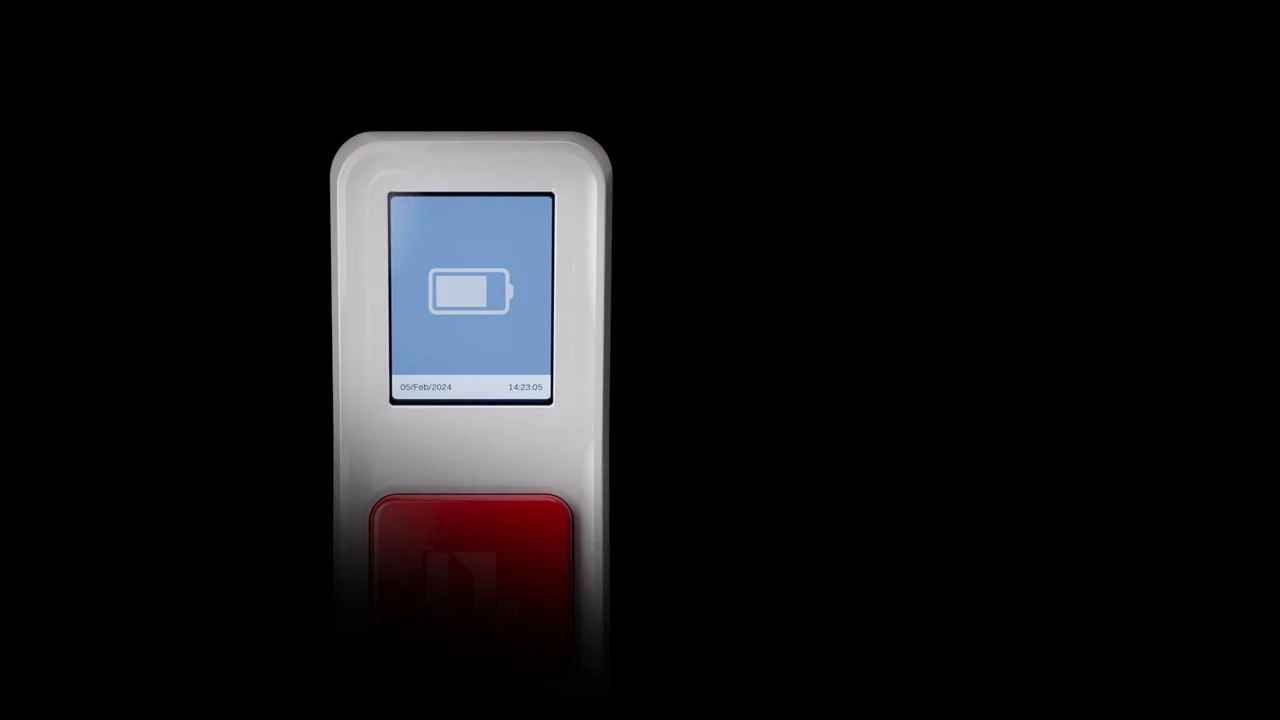
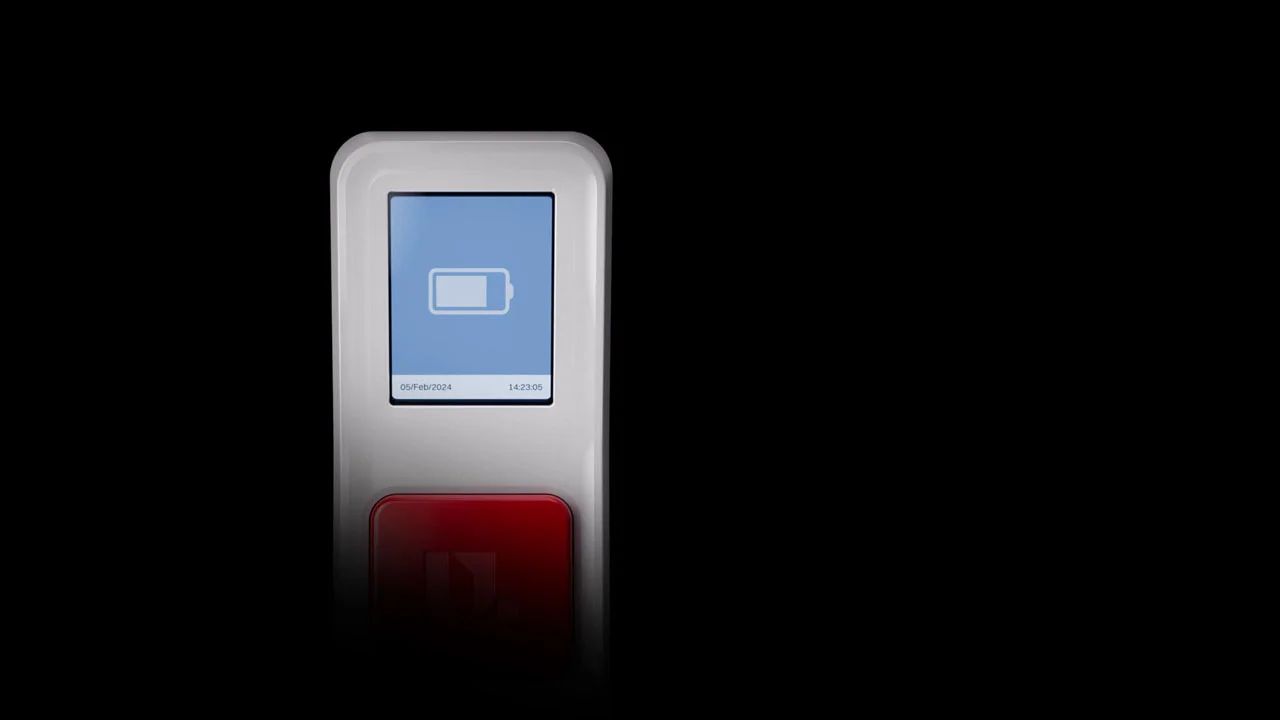
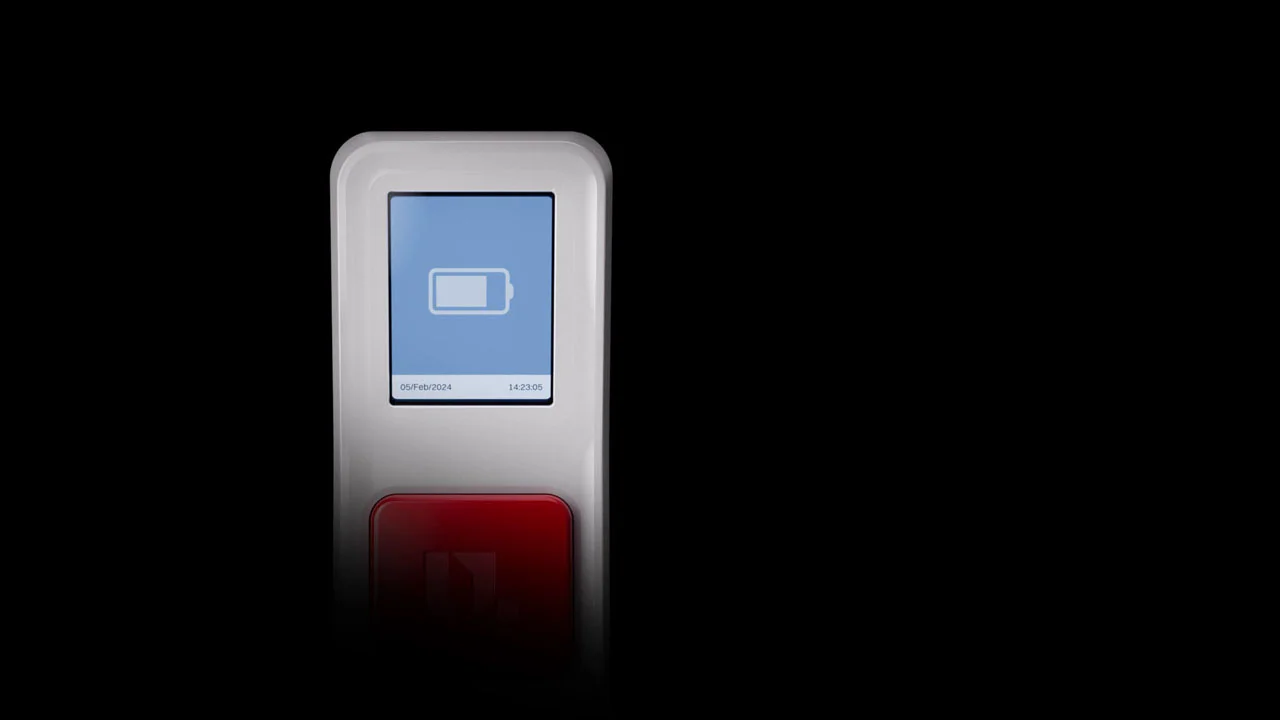
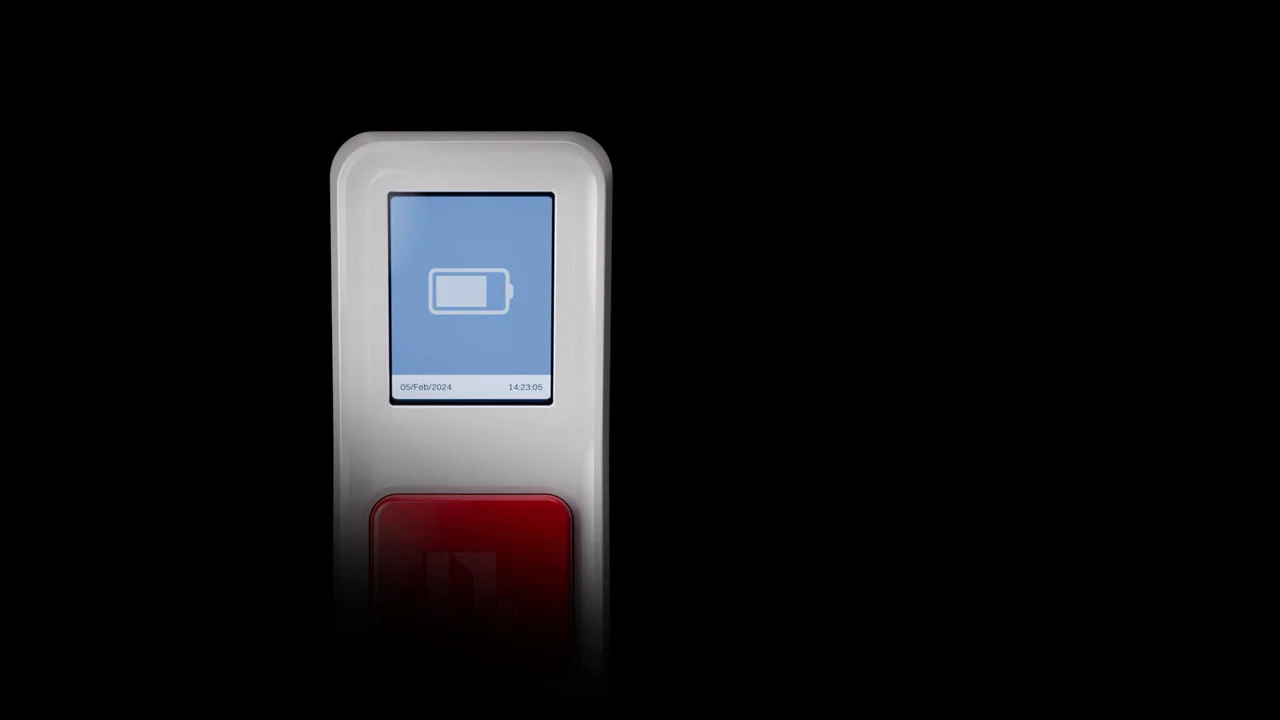
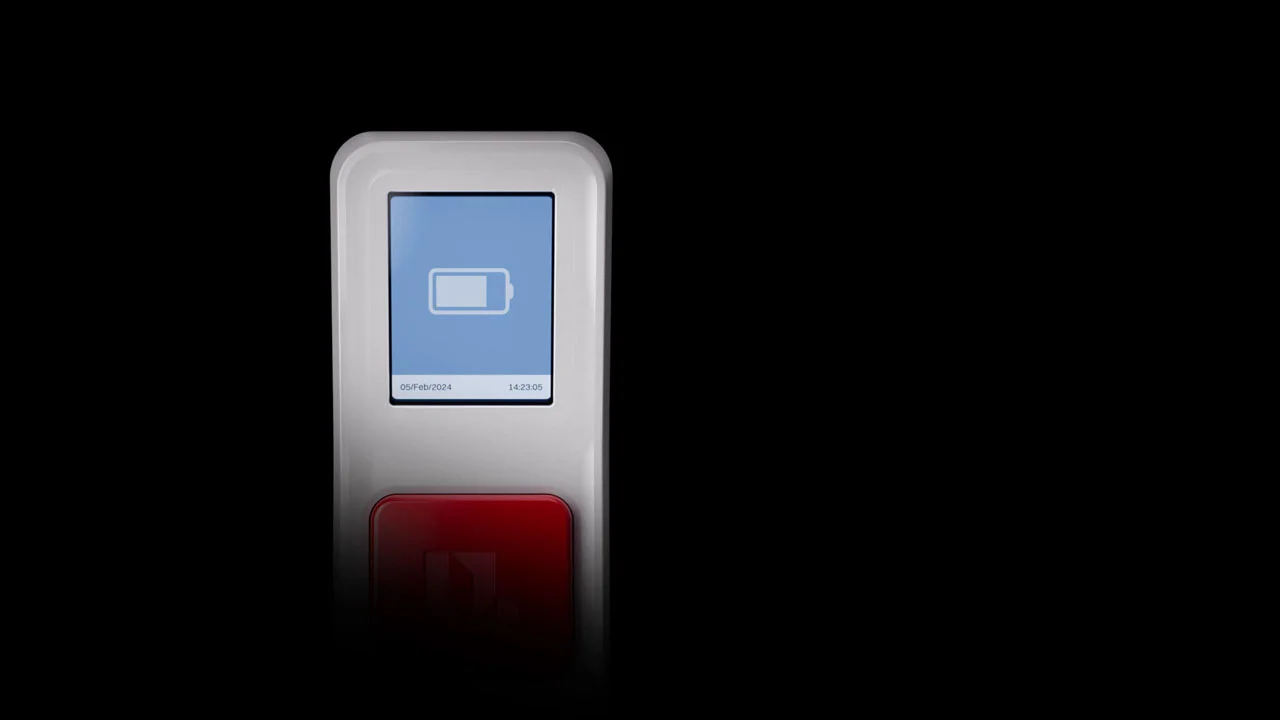
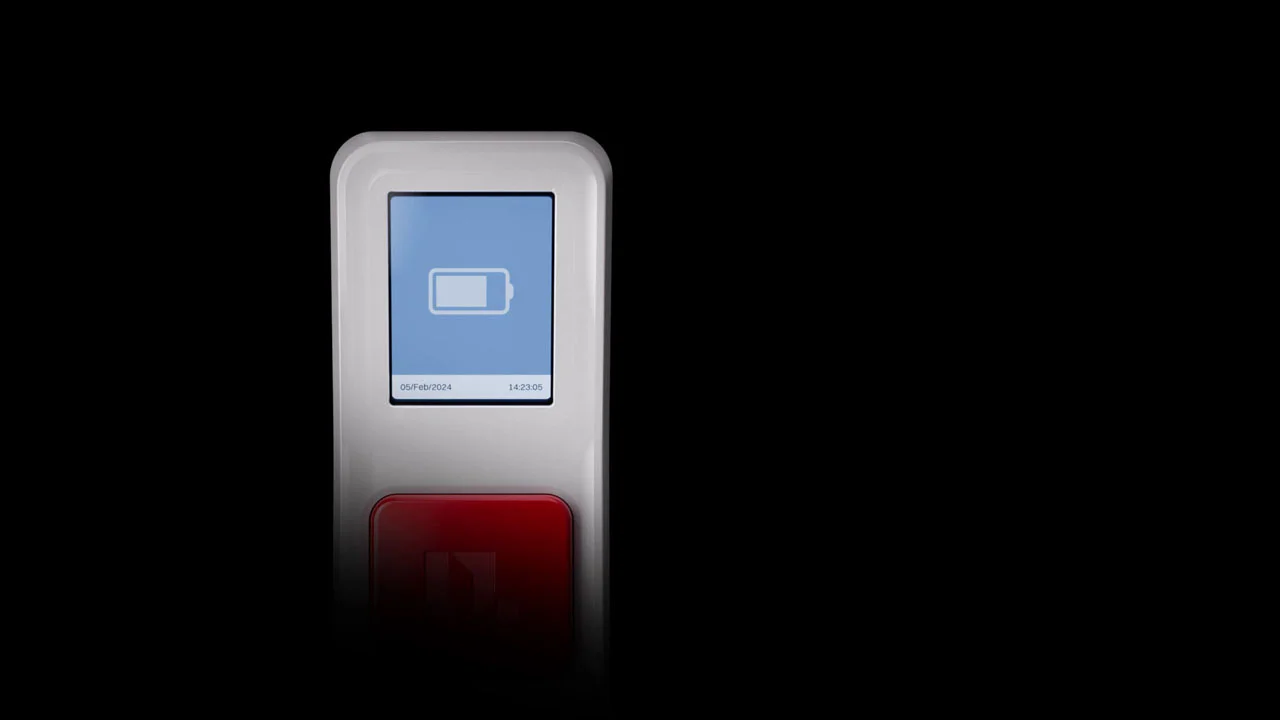
Pressing the red button in every time it's compeleted will update the production quantity and average SMV in real time.
Productivity can be measured accurately by collecting 30 vibration datas per second of sewing machines while production.
Even if there is a power outage at the factory, or the internet is disconnected, we operate stably through the battery to collect data without any issues.

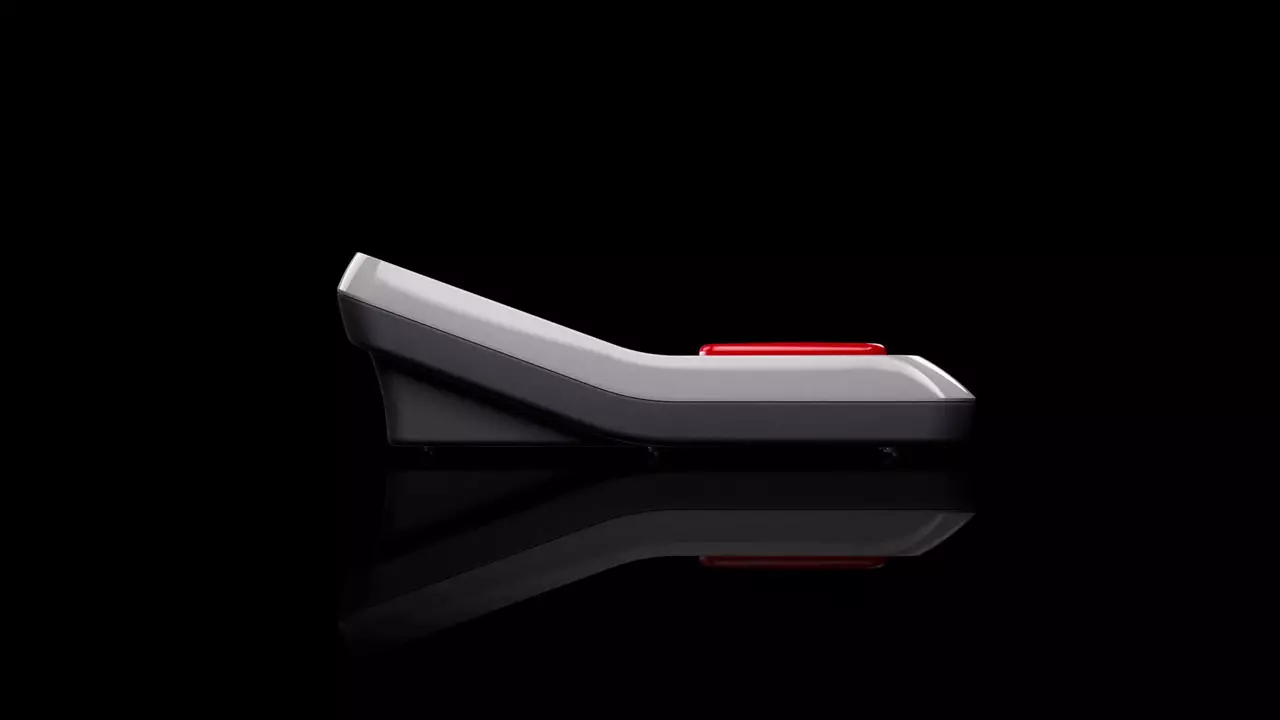
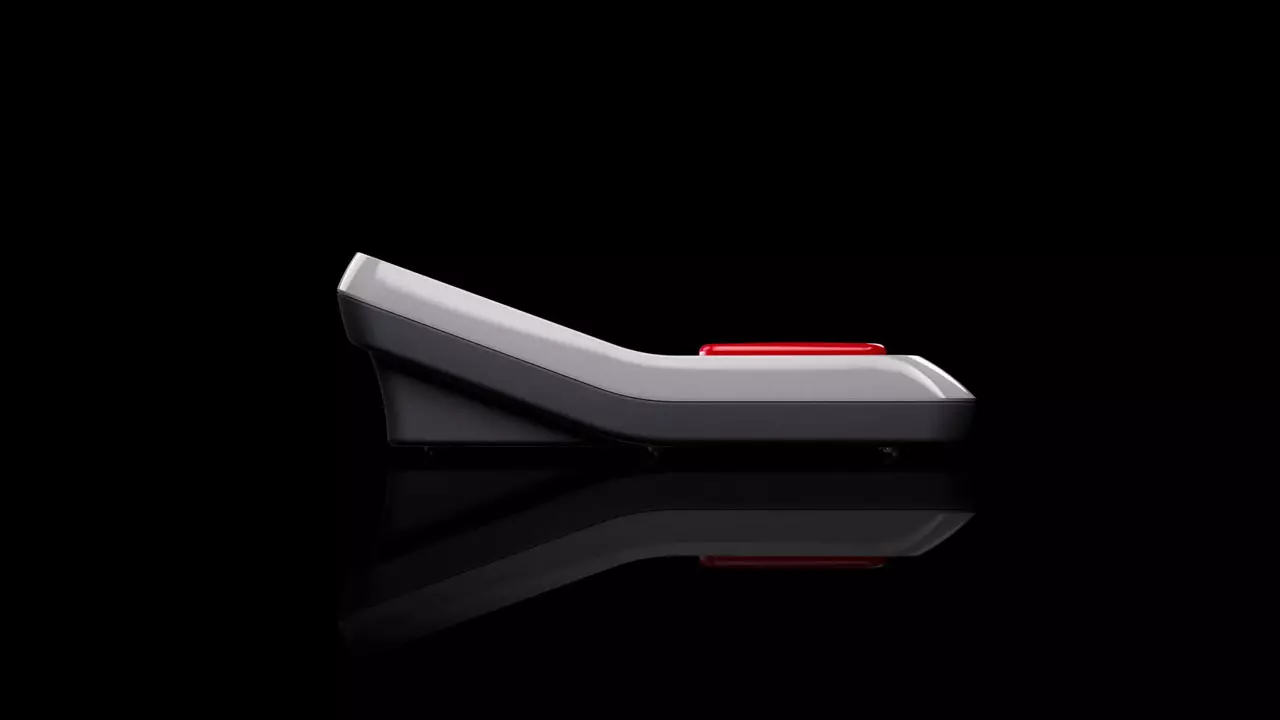
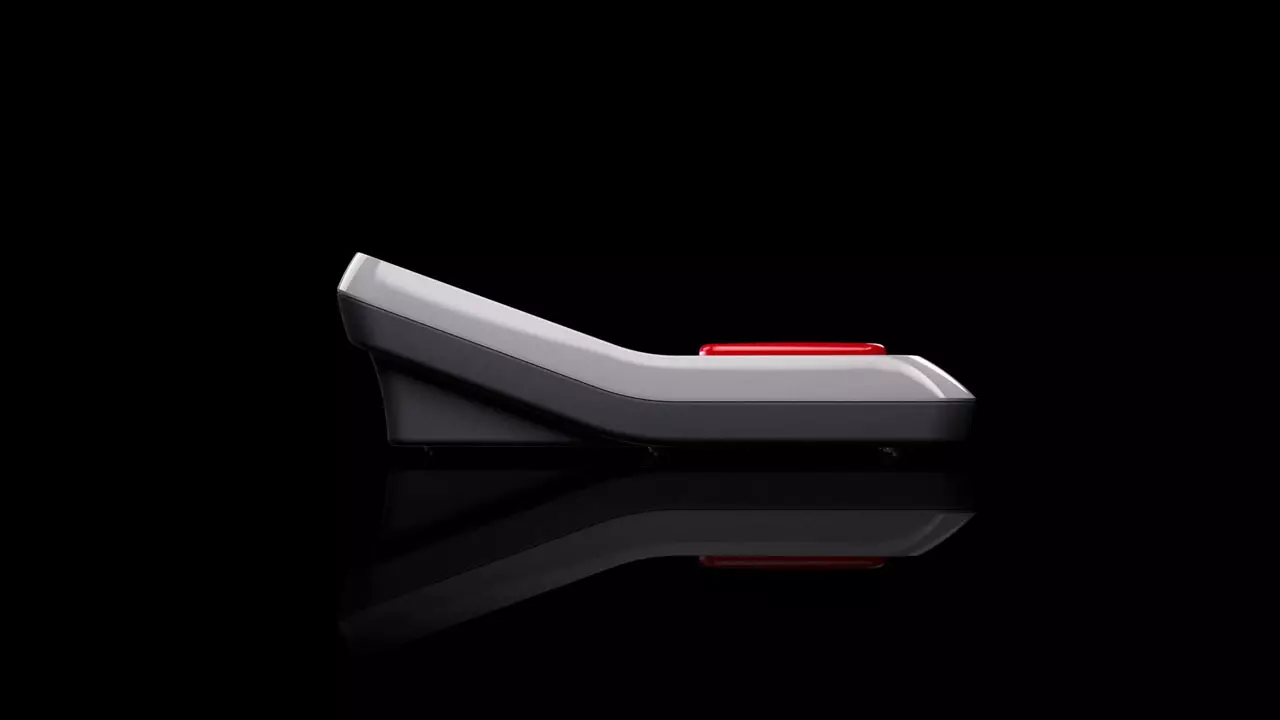
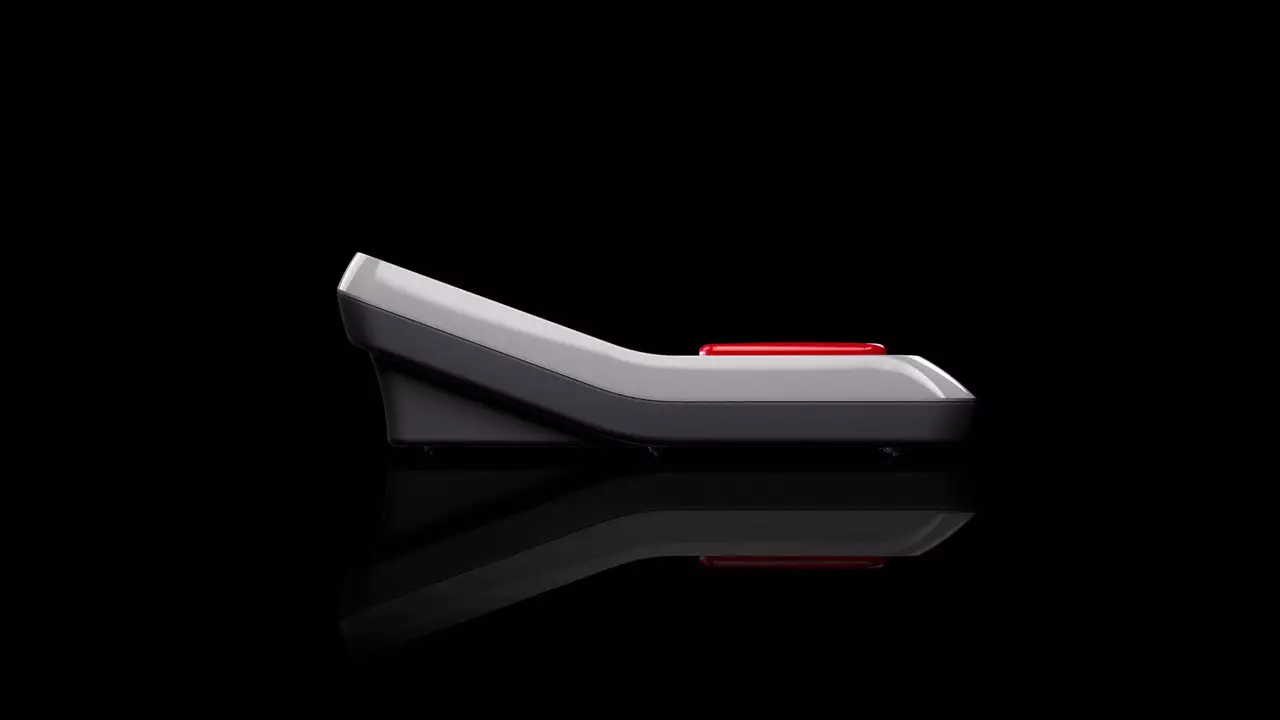
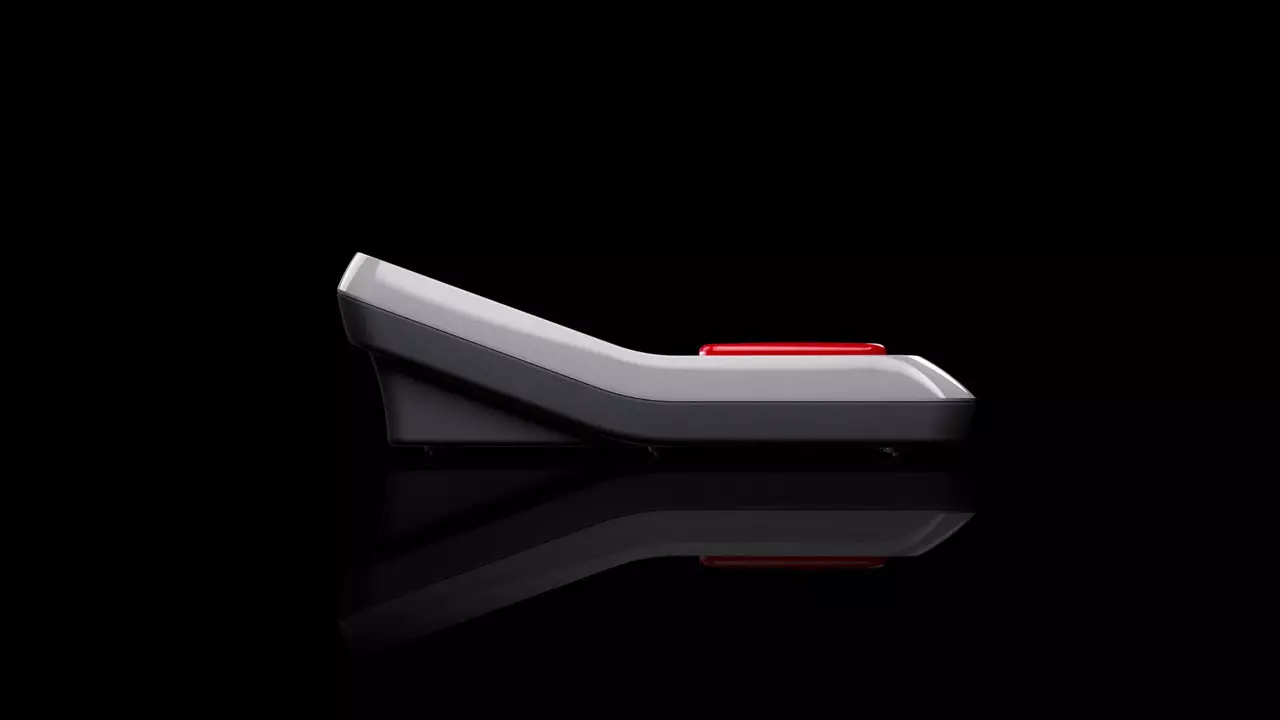
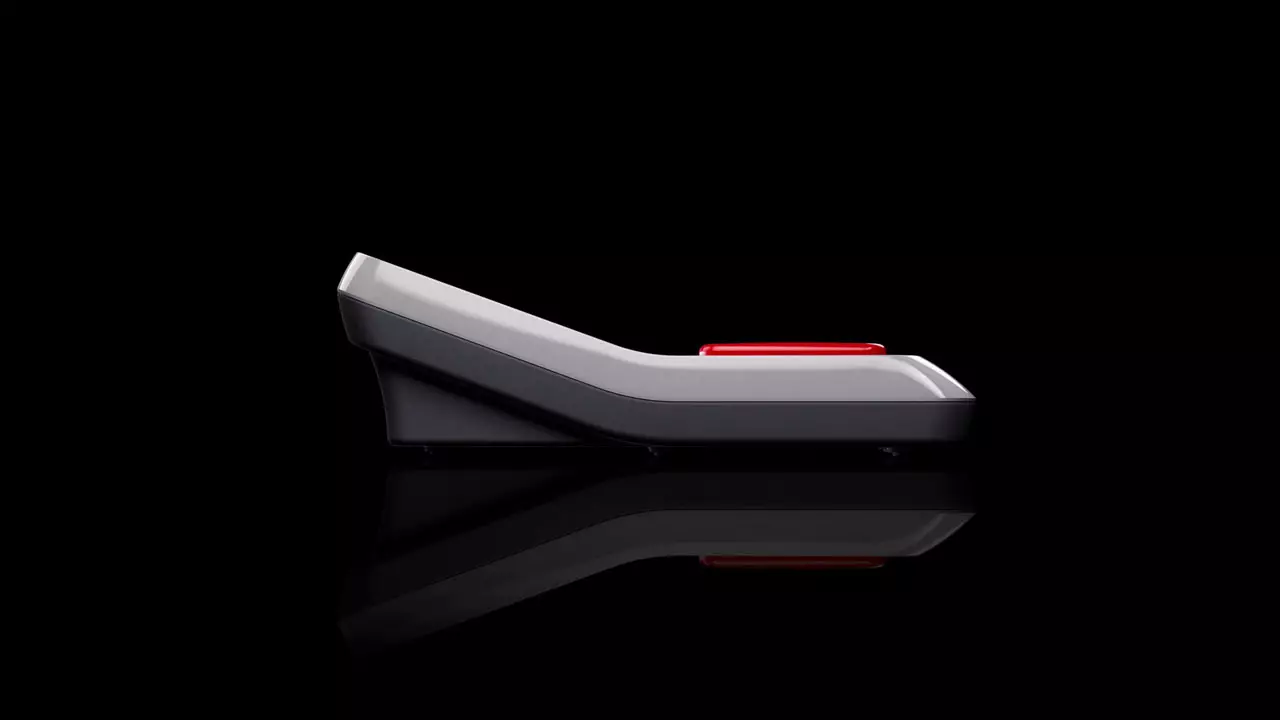
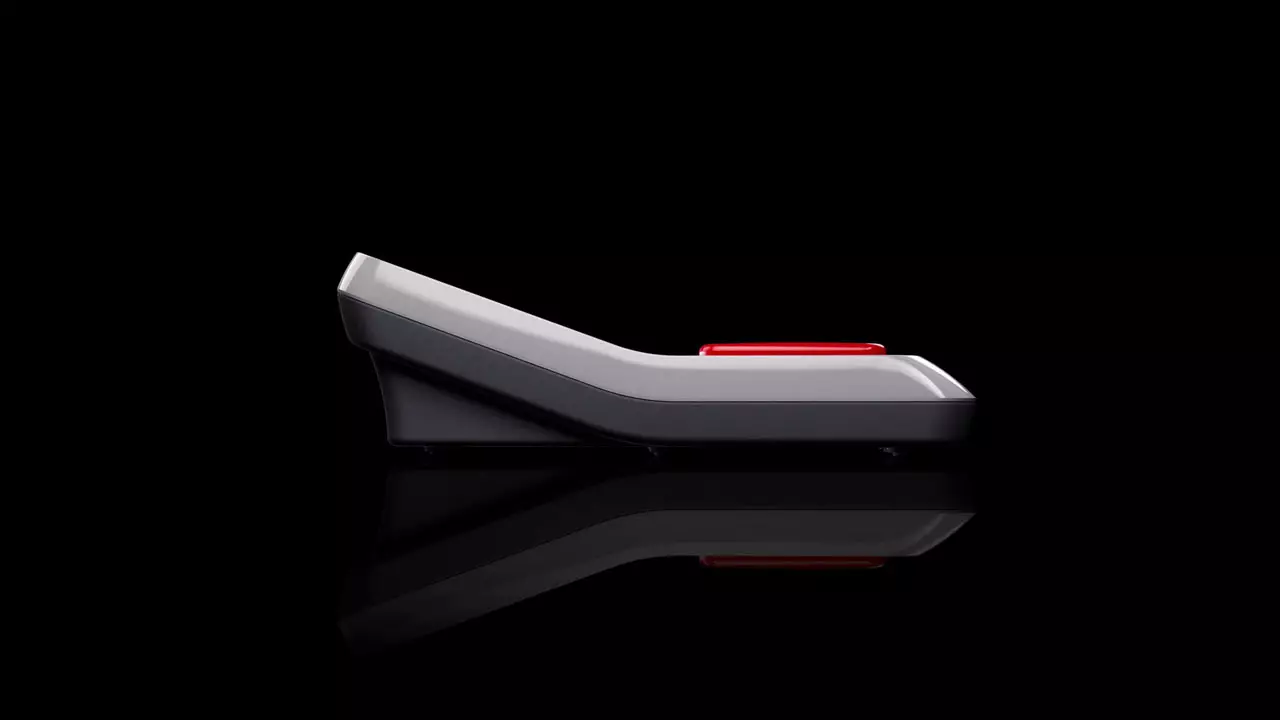
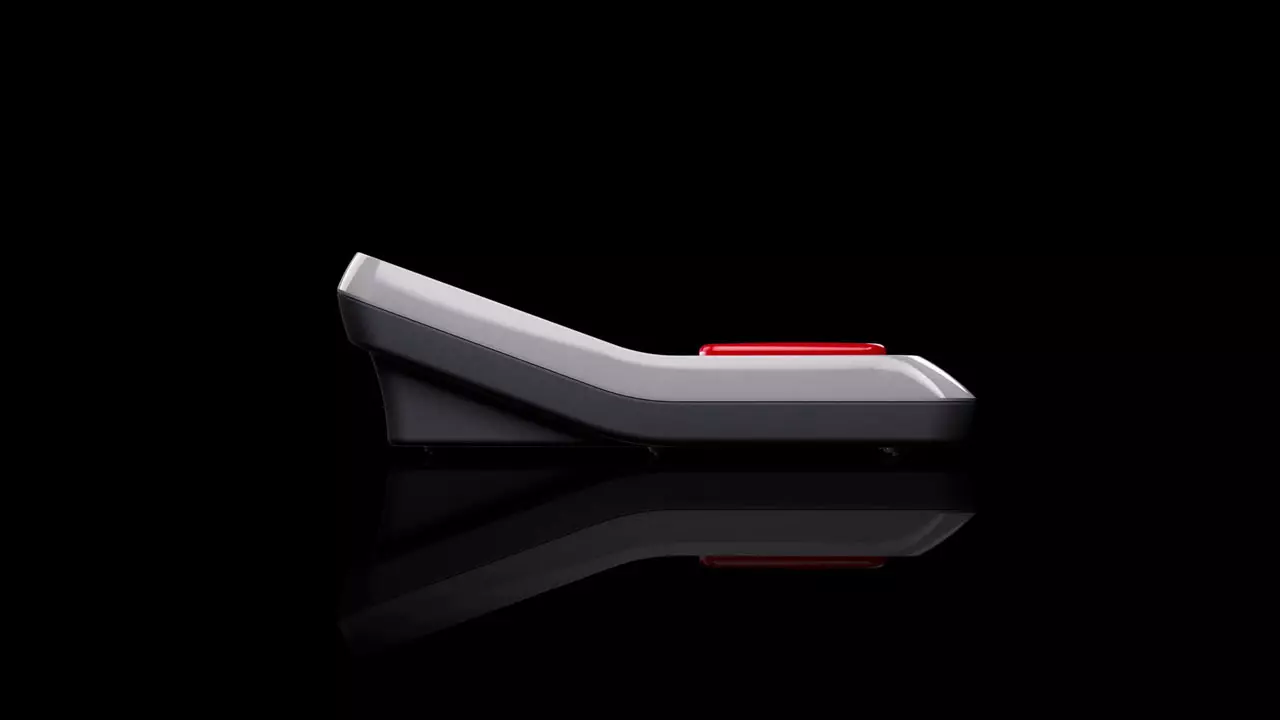
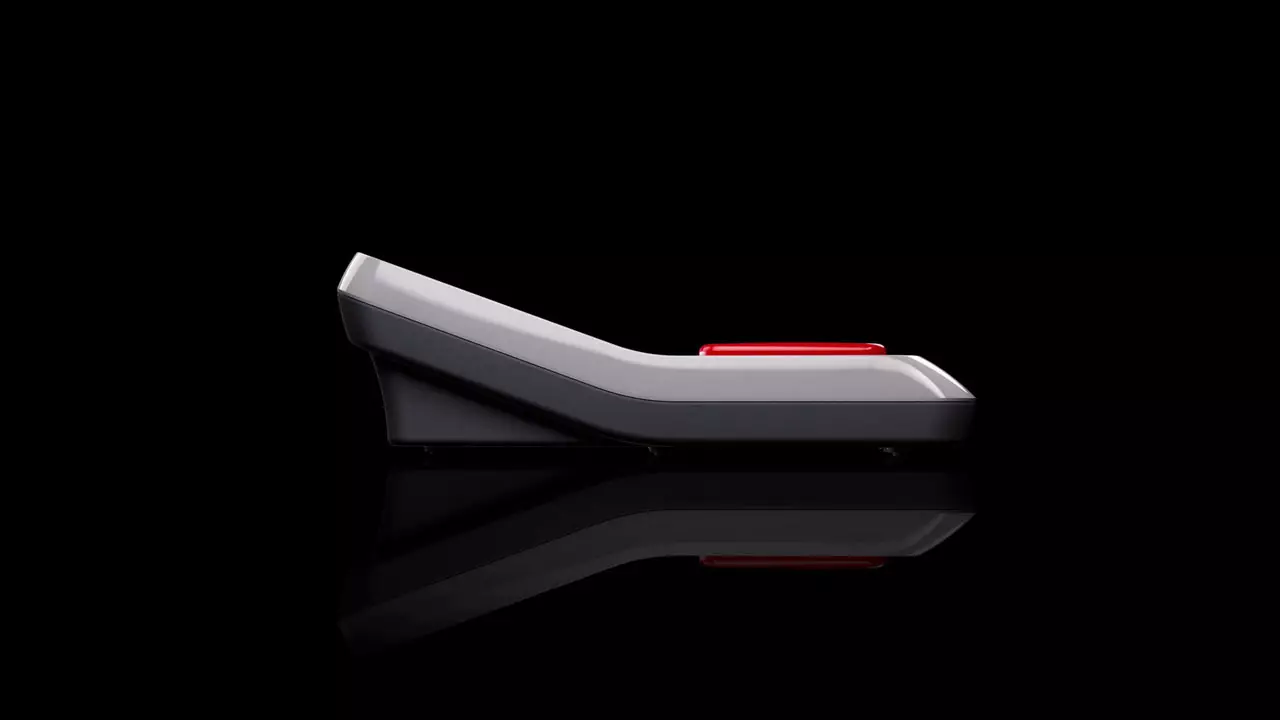
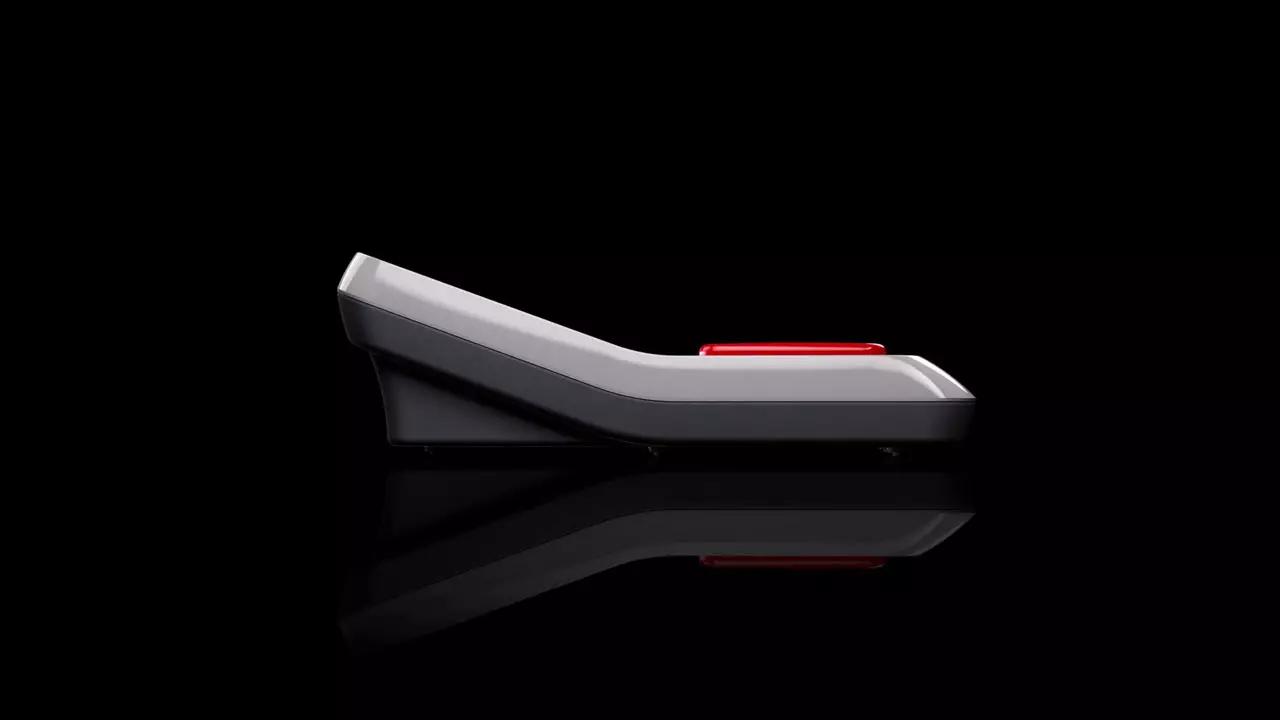
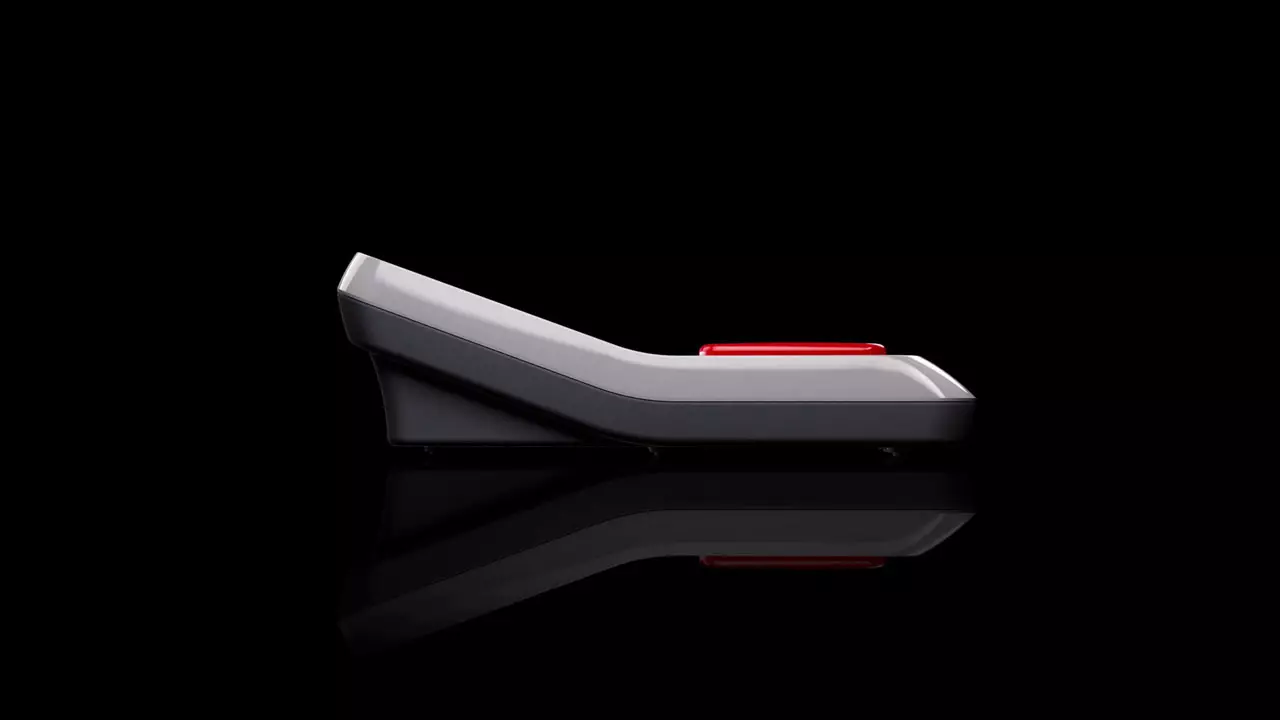
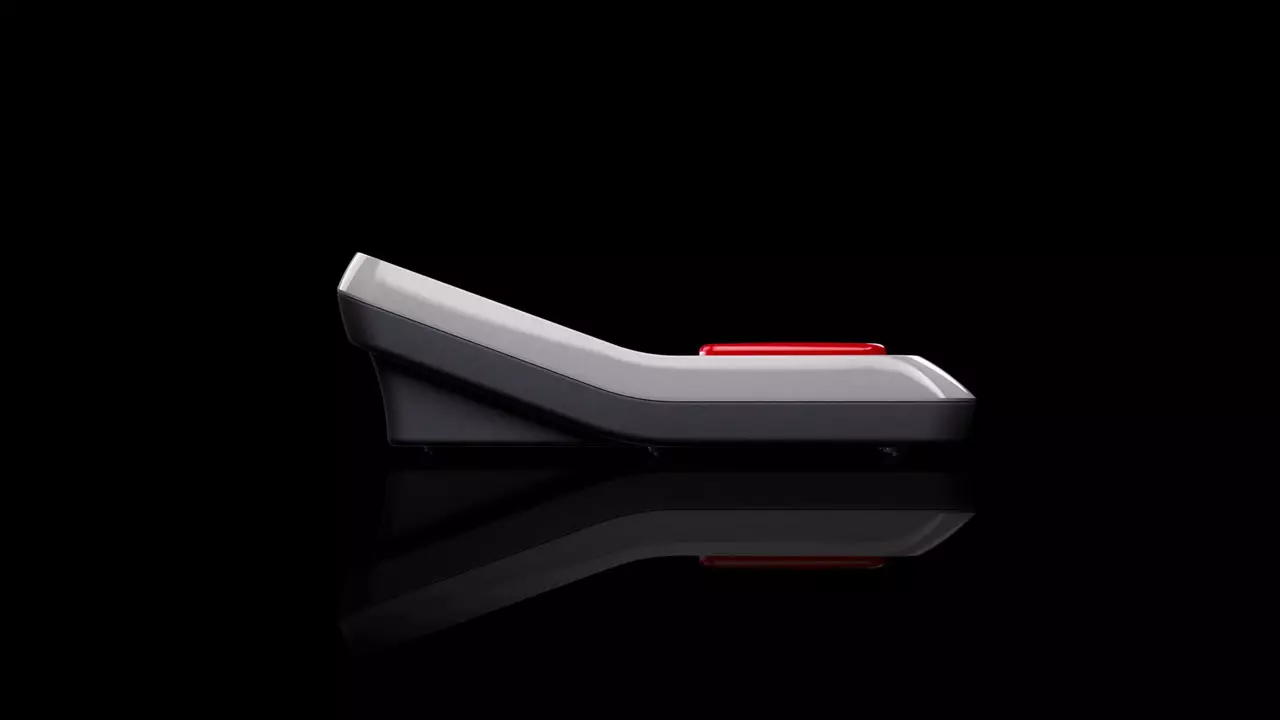
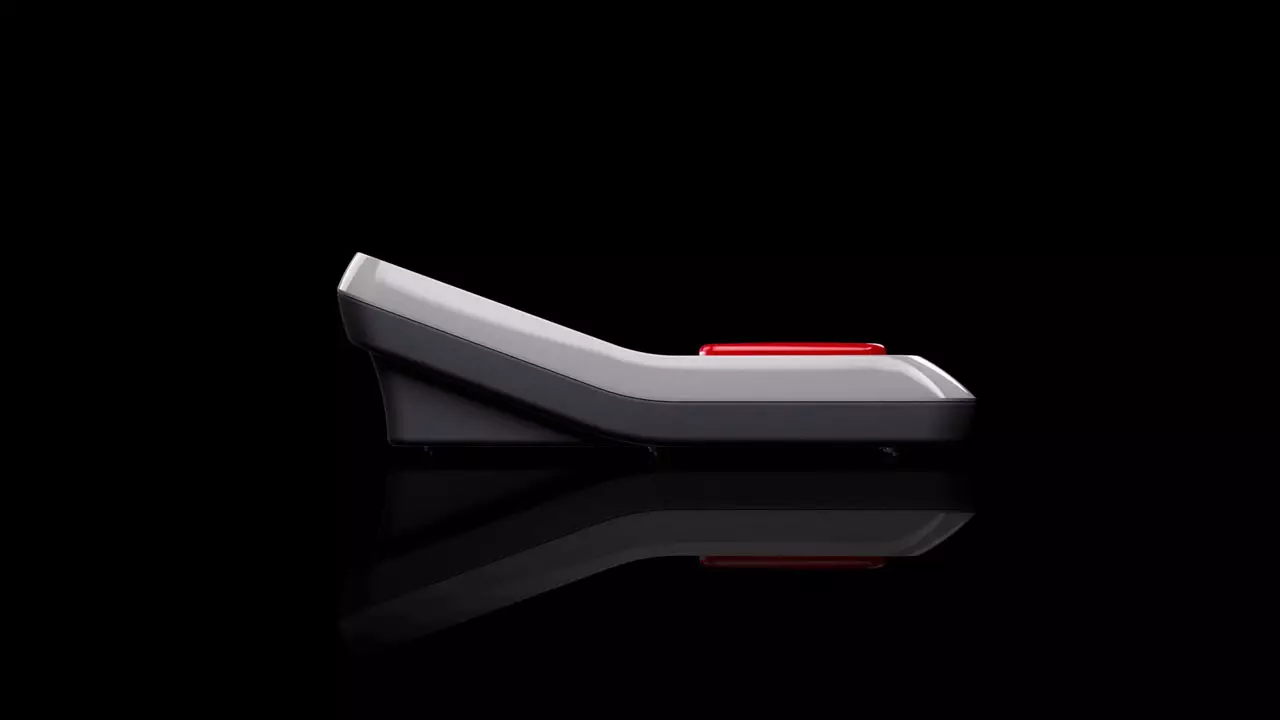
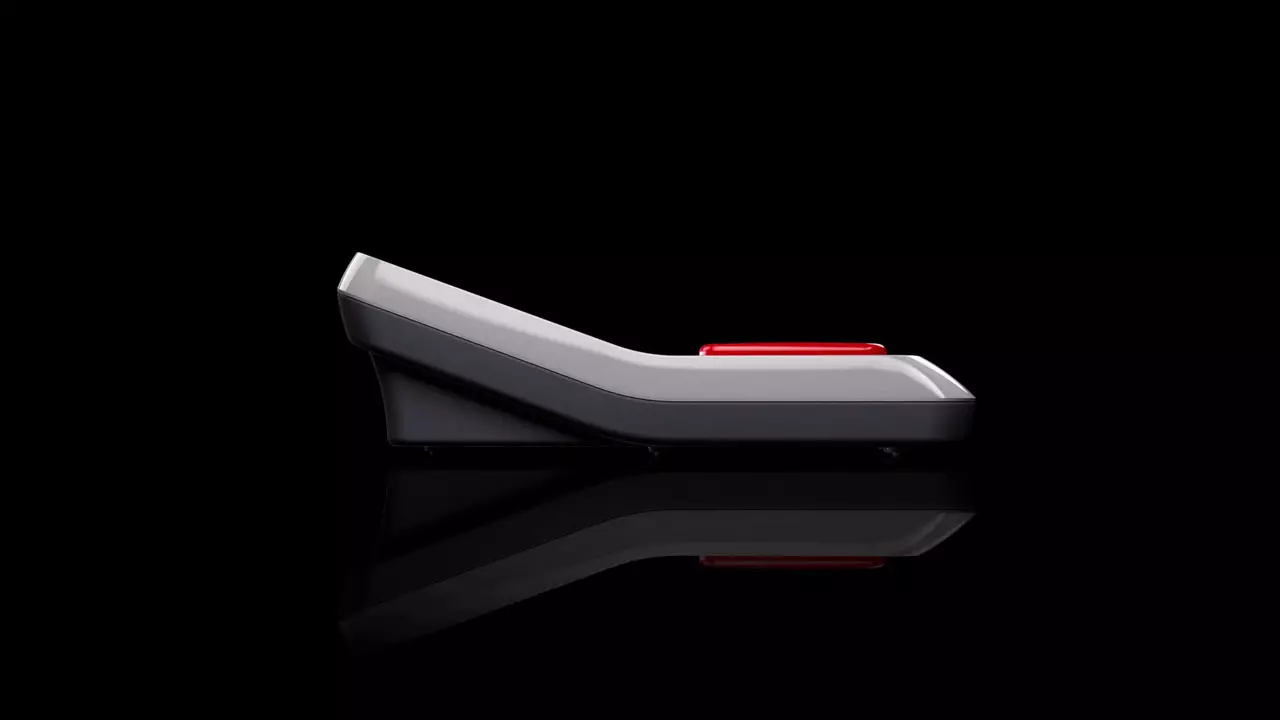
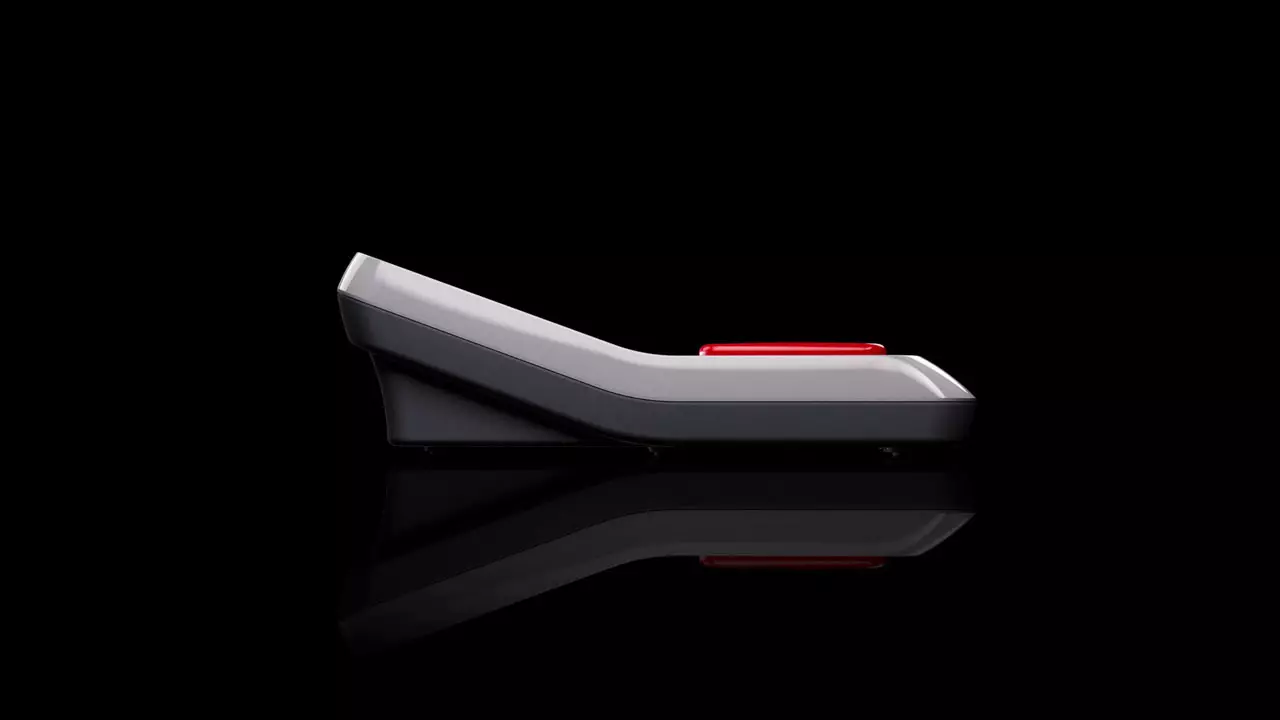
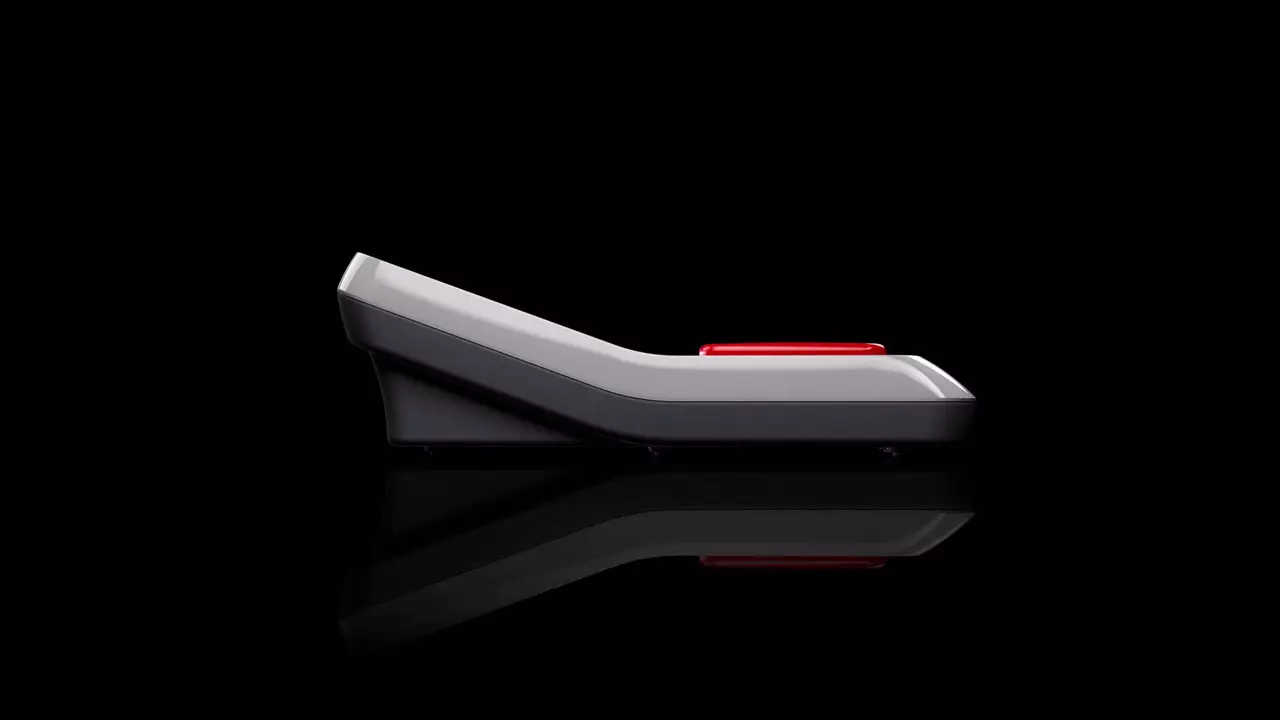
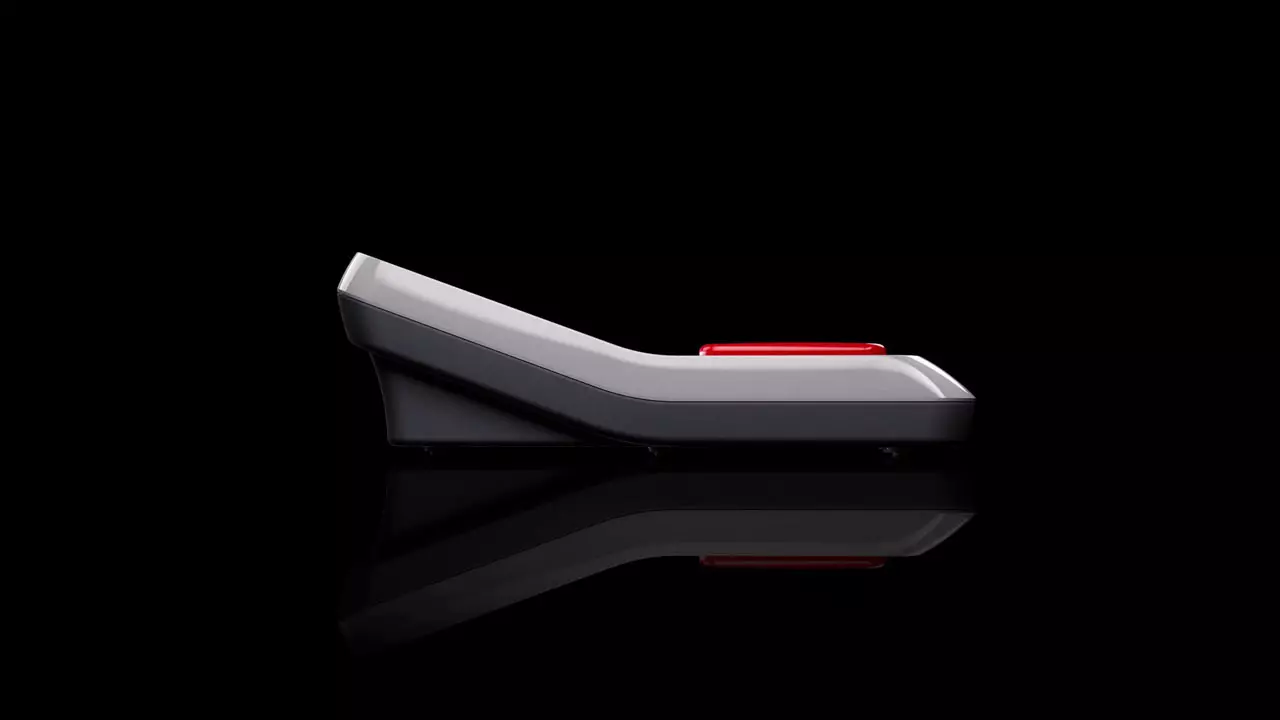
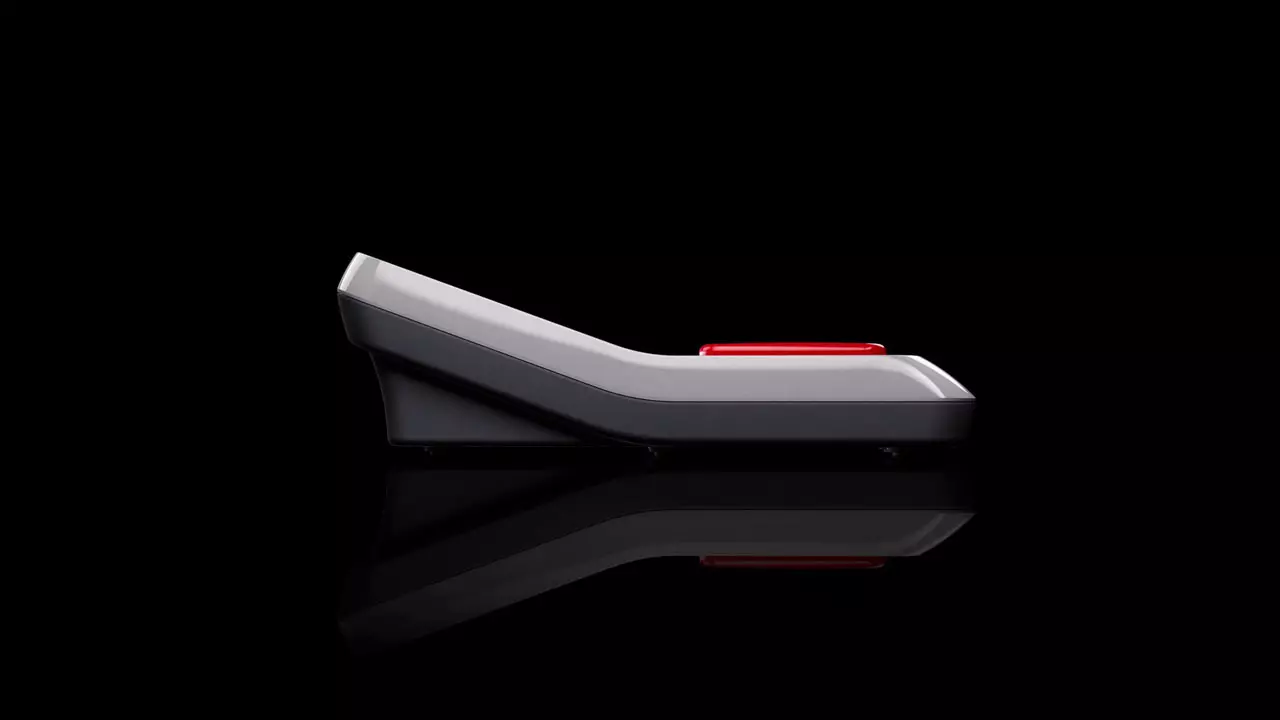
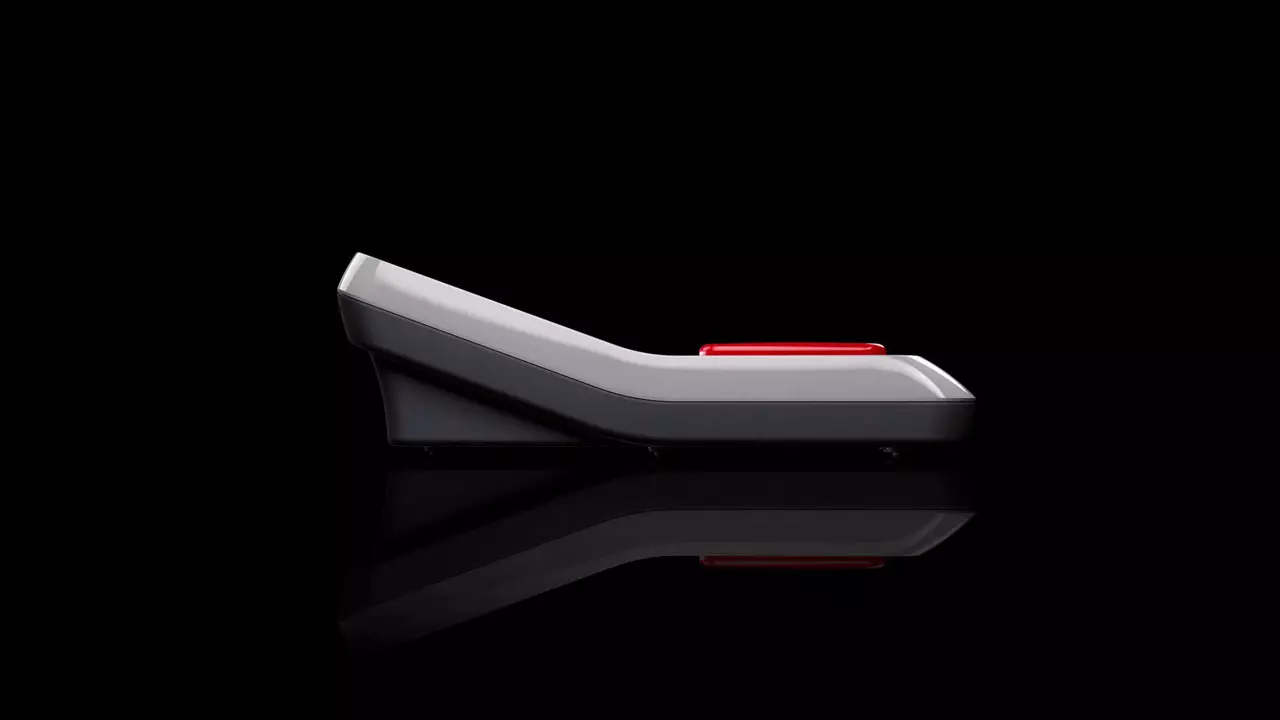
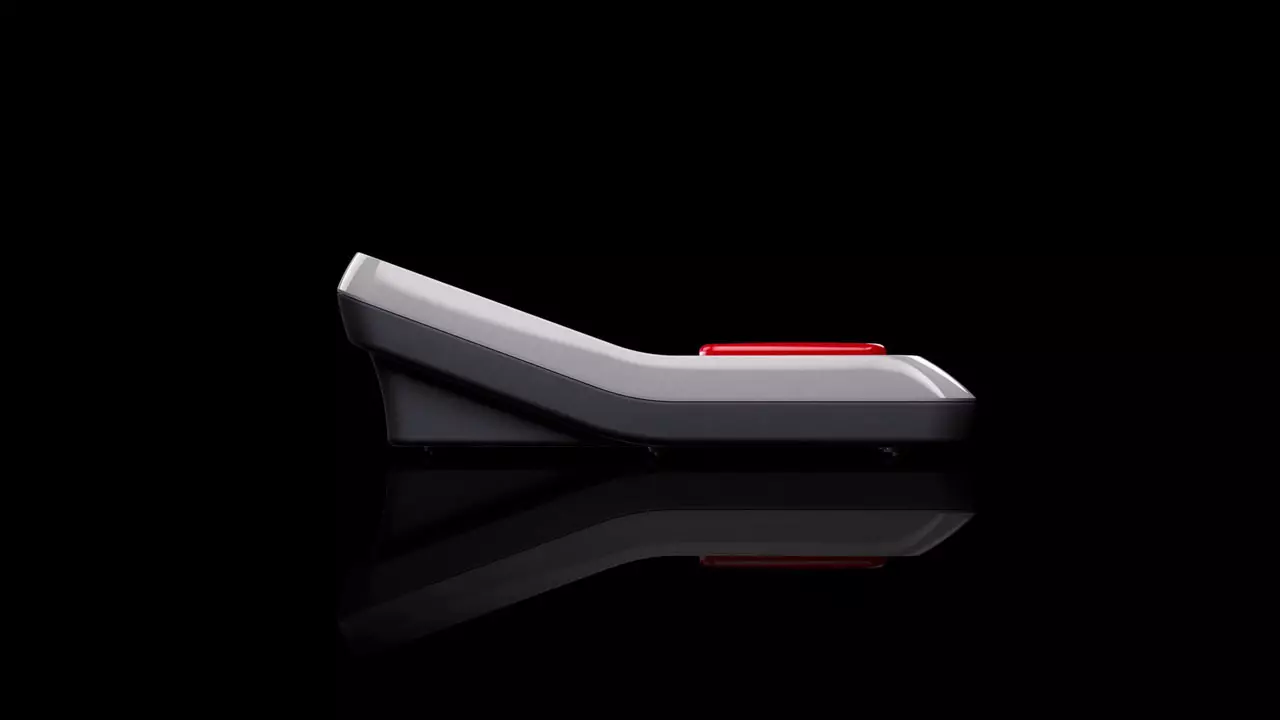
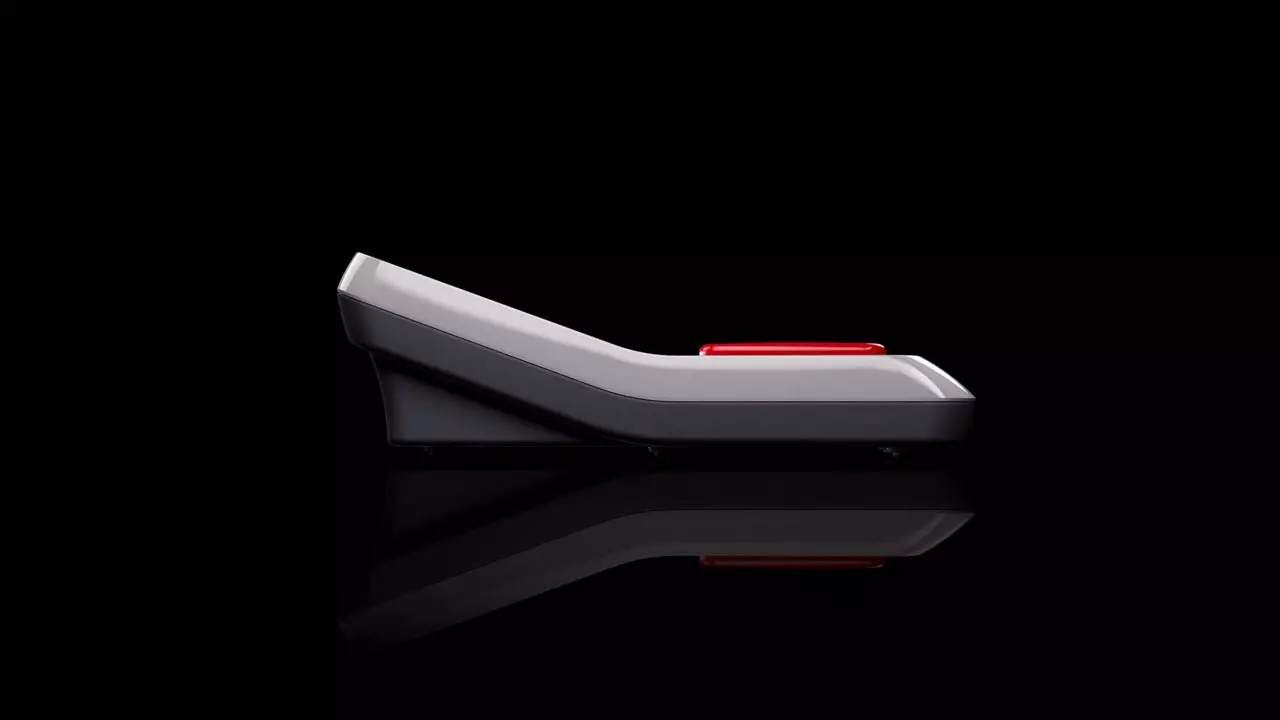
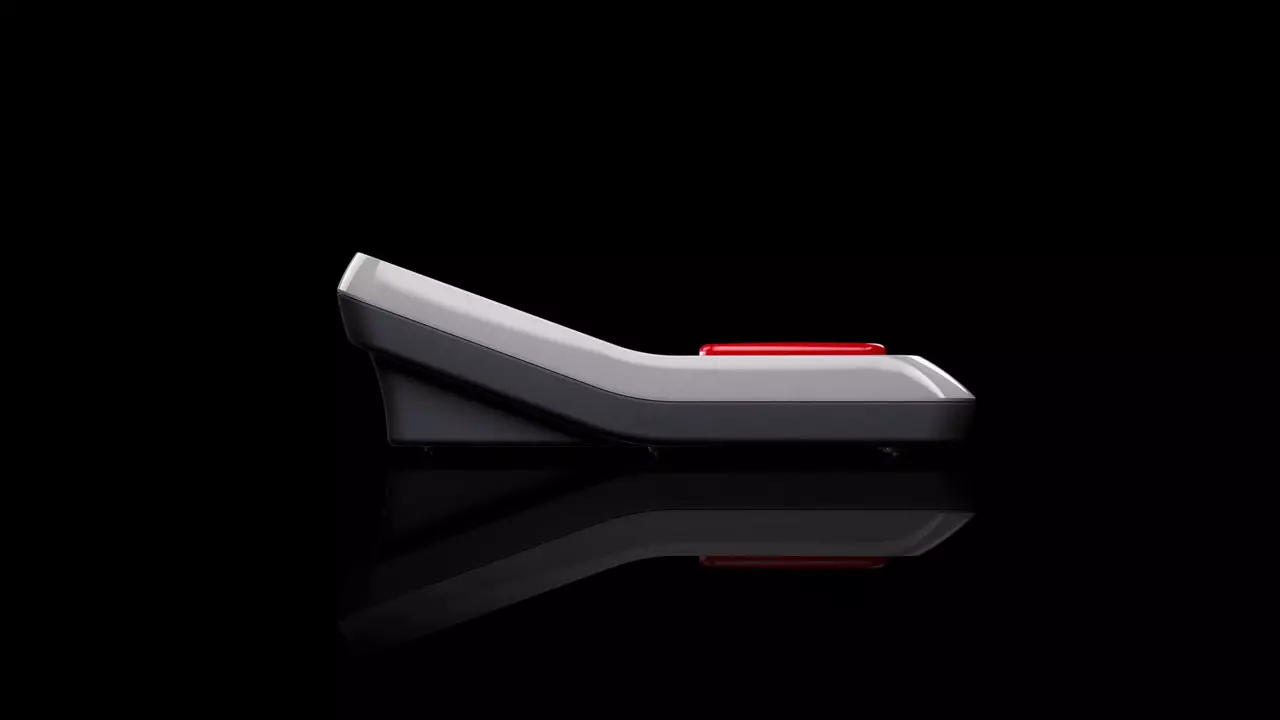
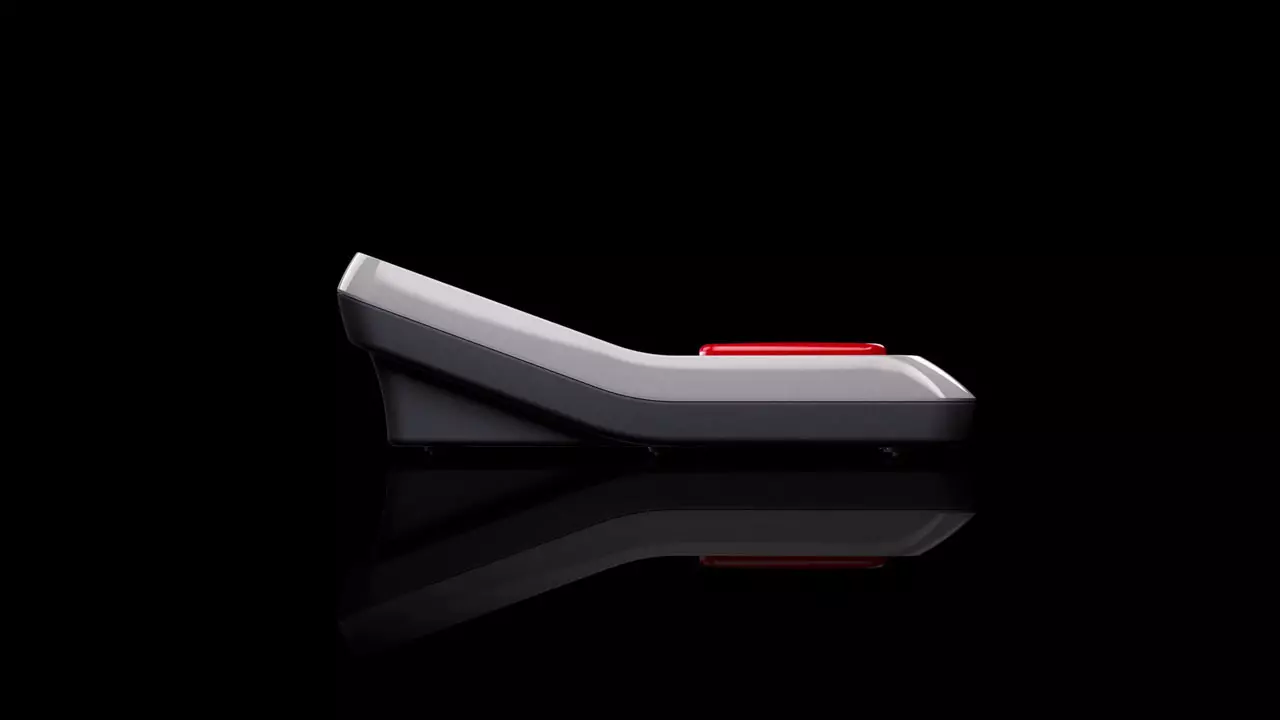
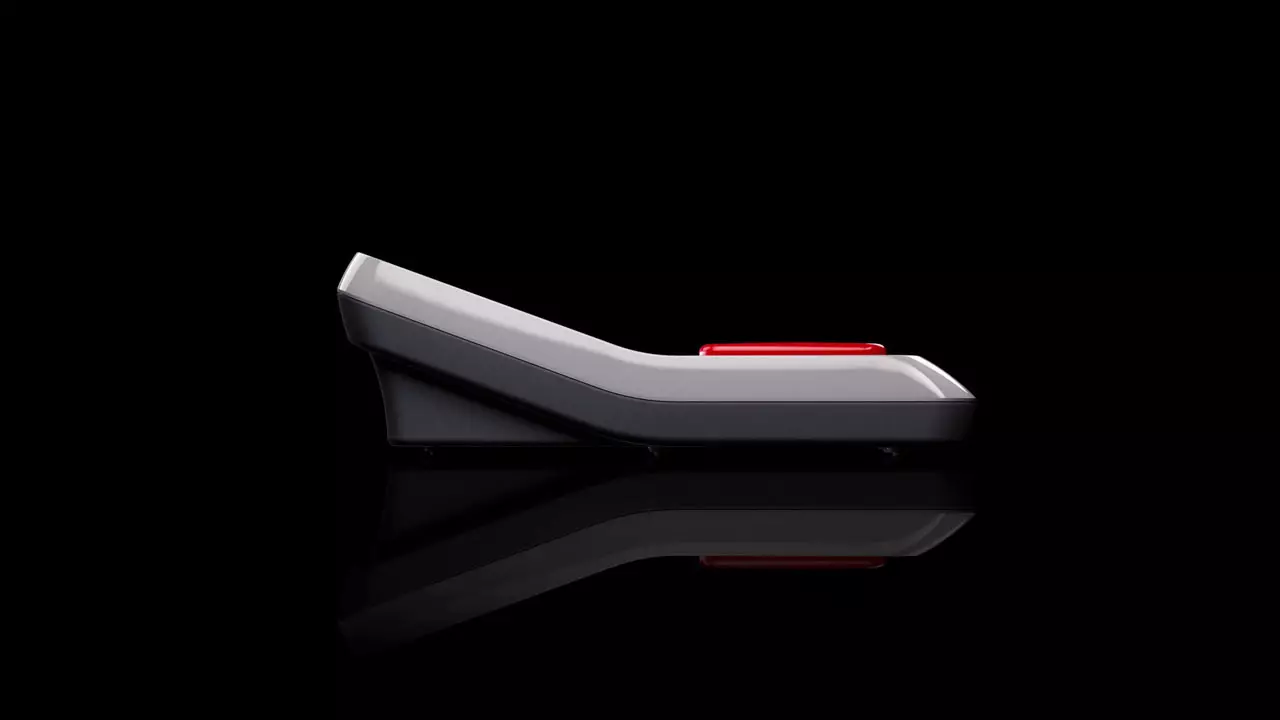
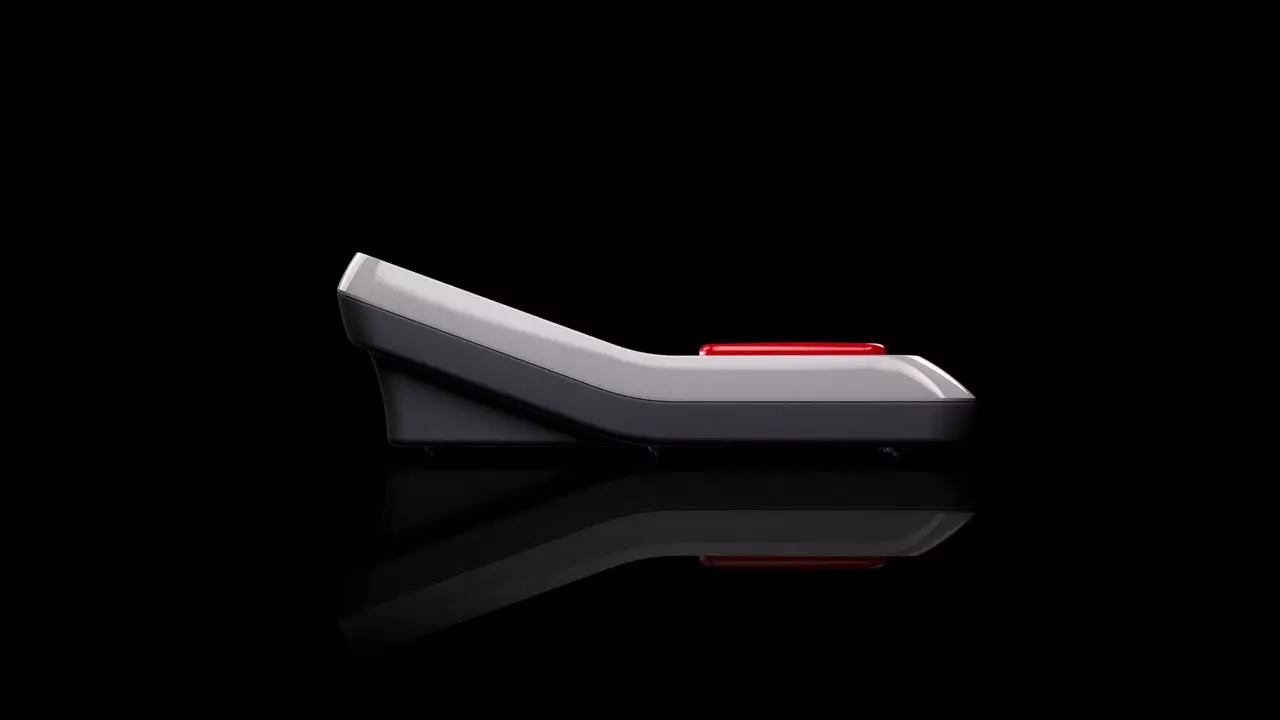
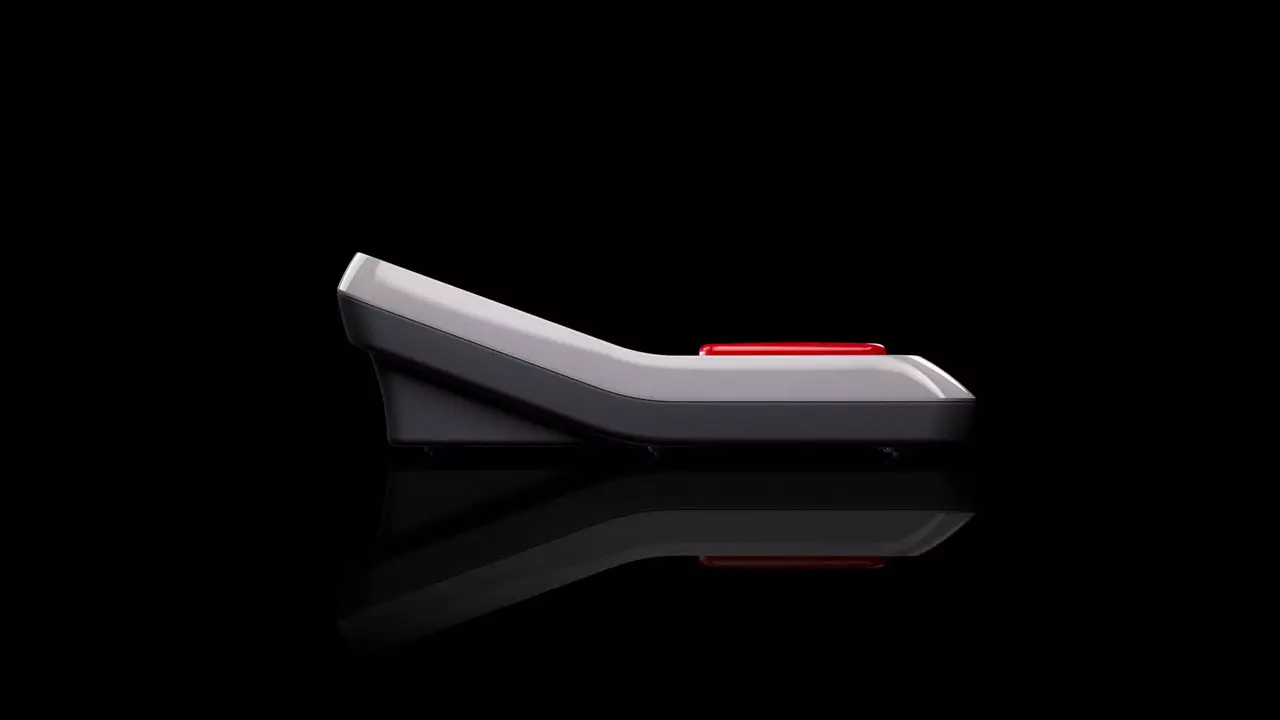
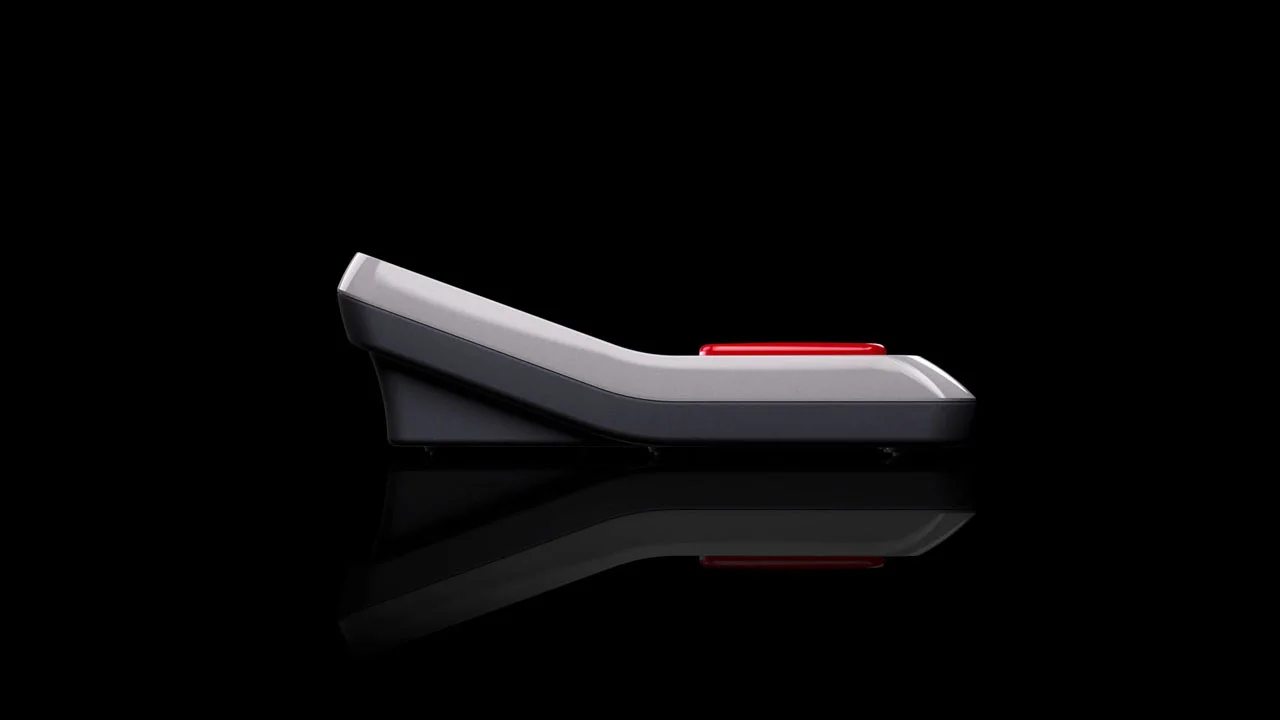
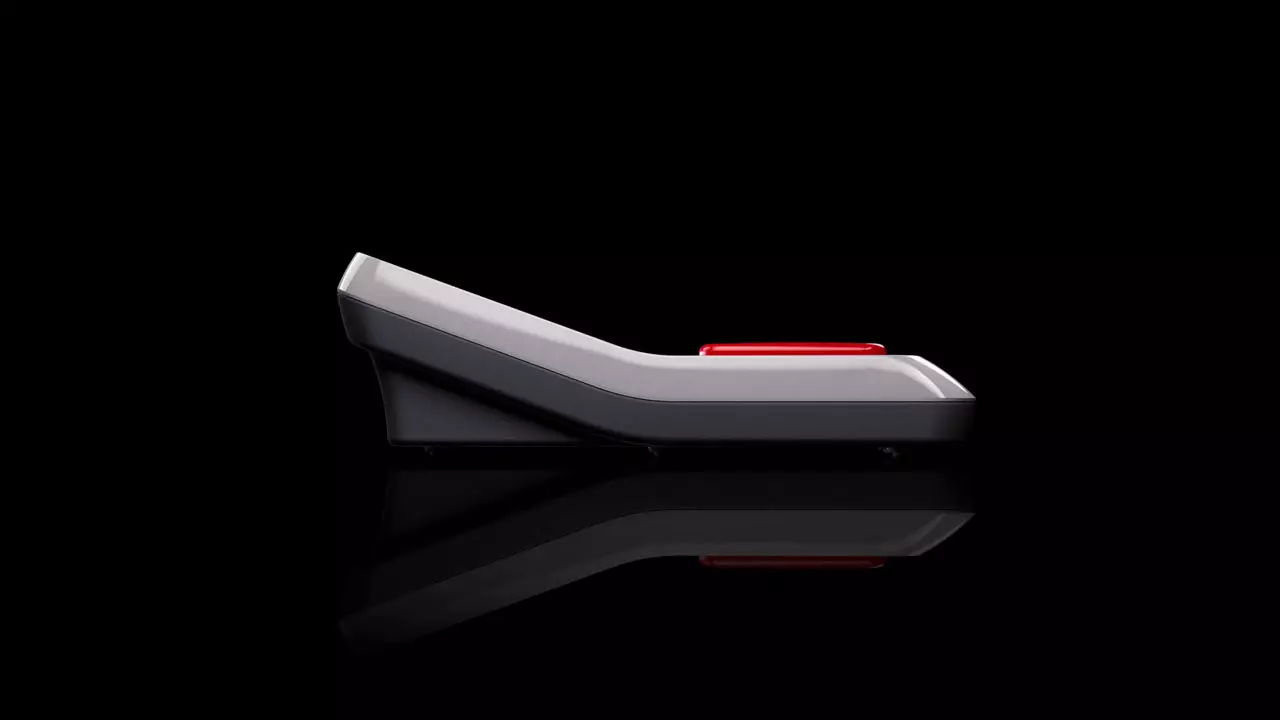
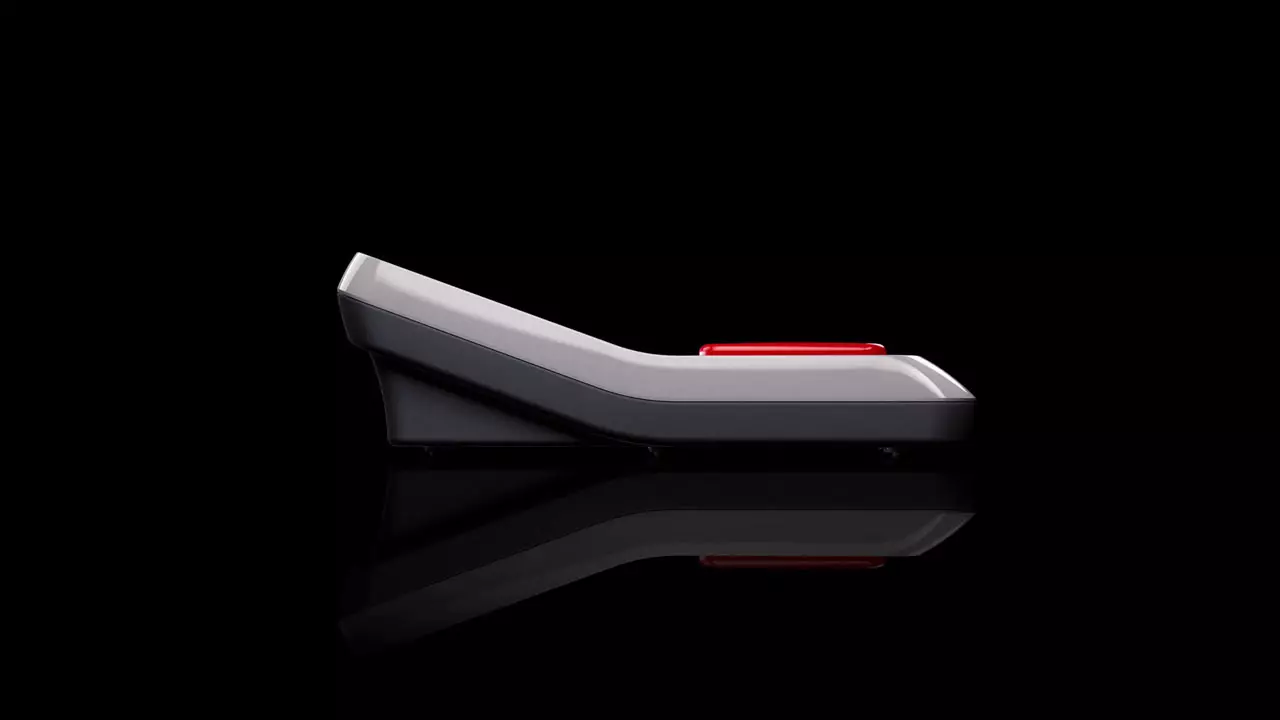
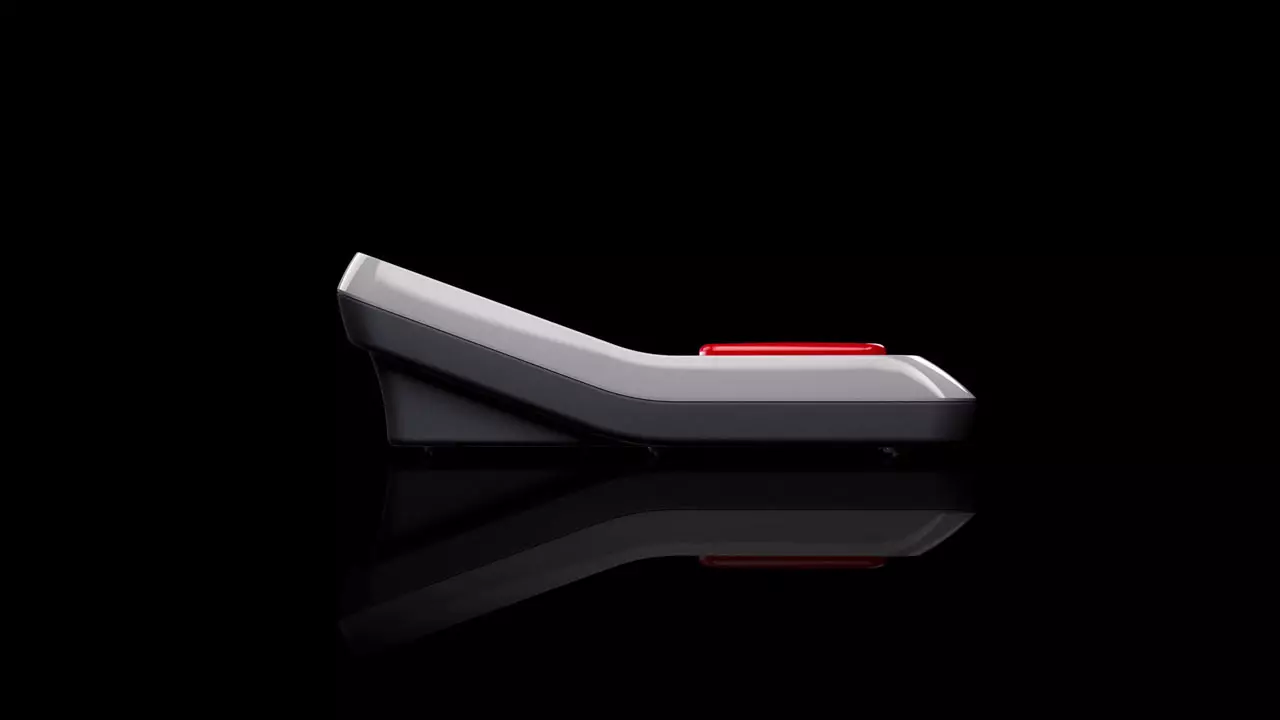
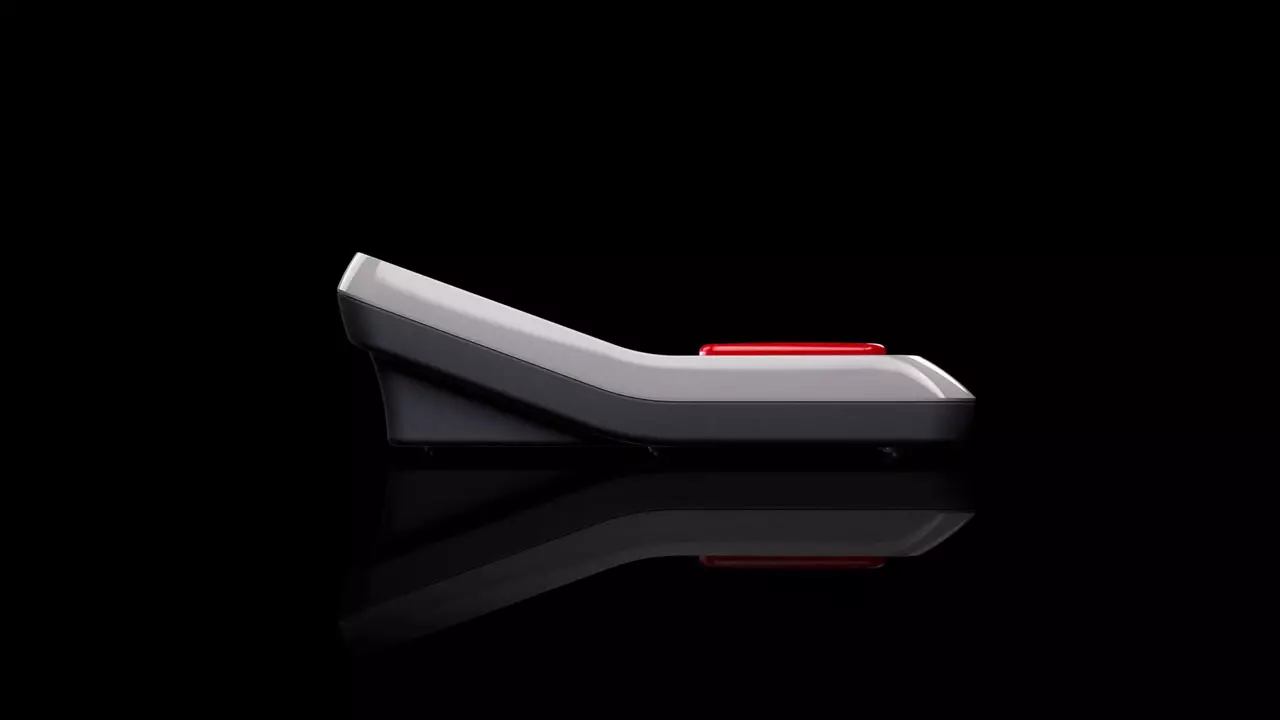
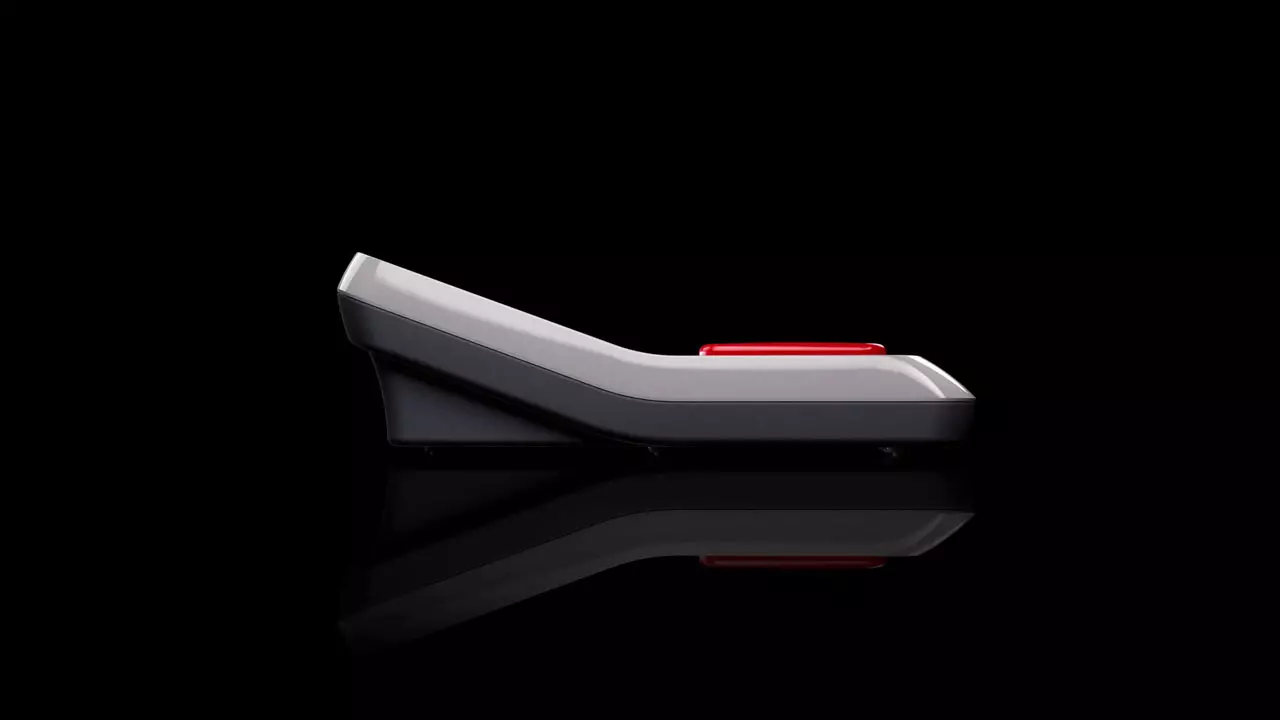
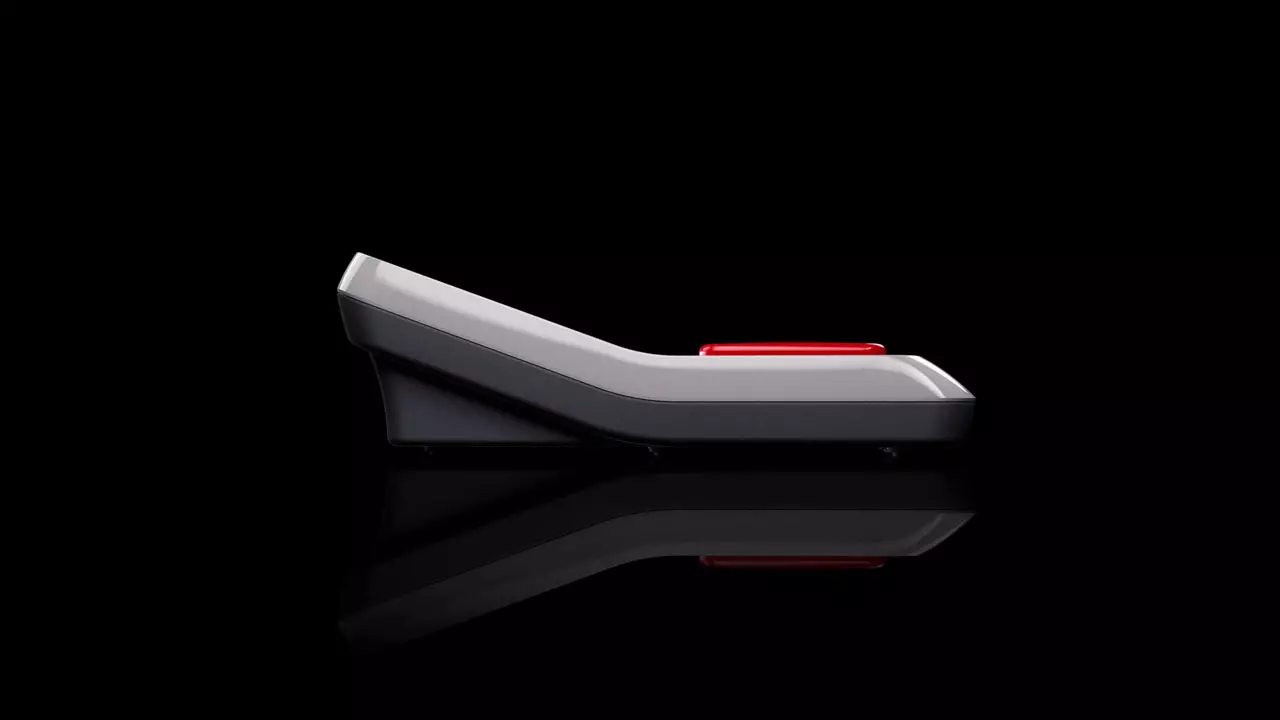
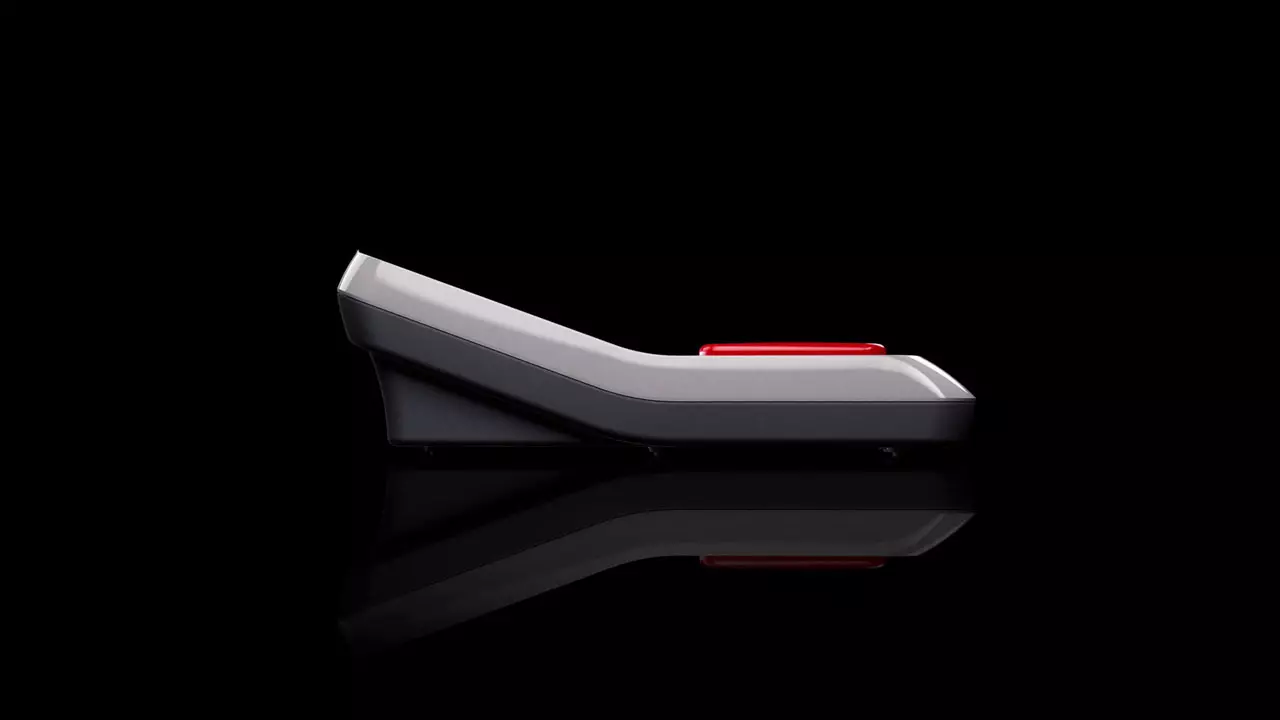
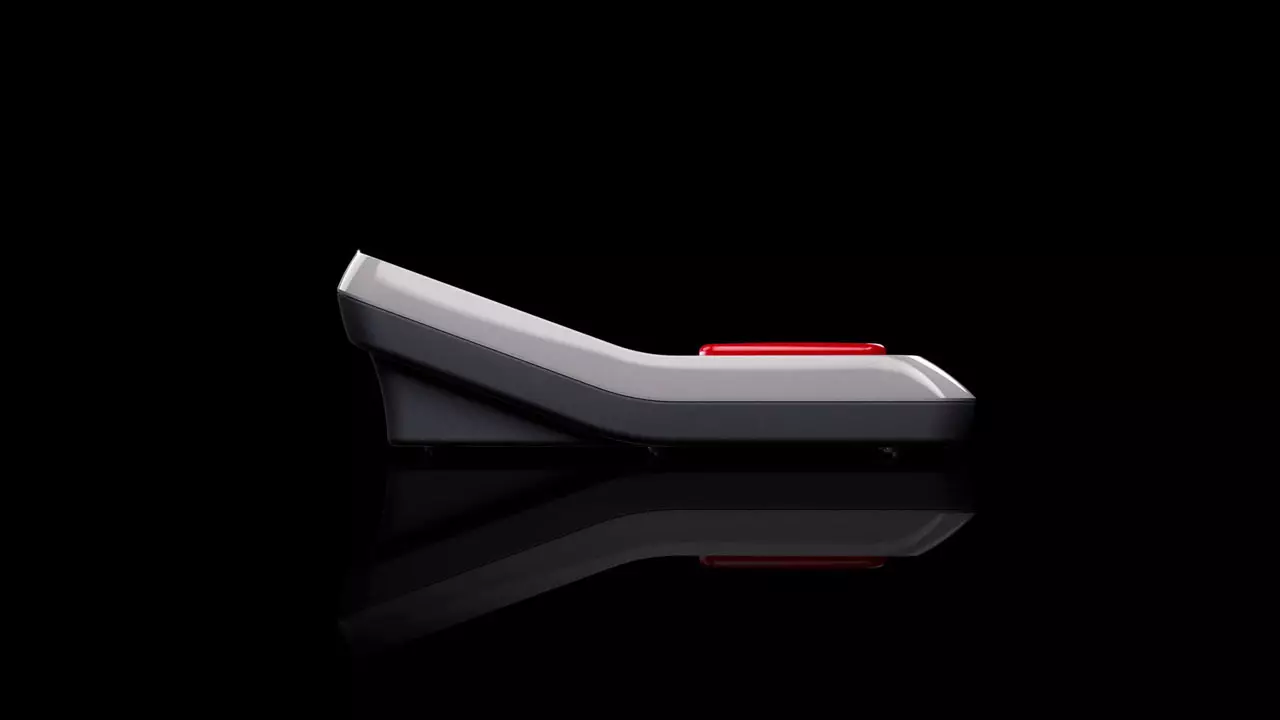
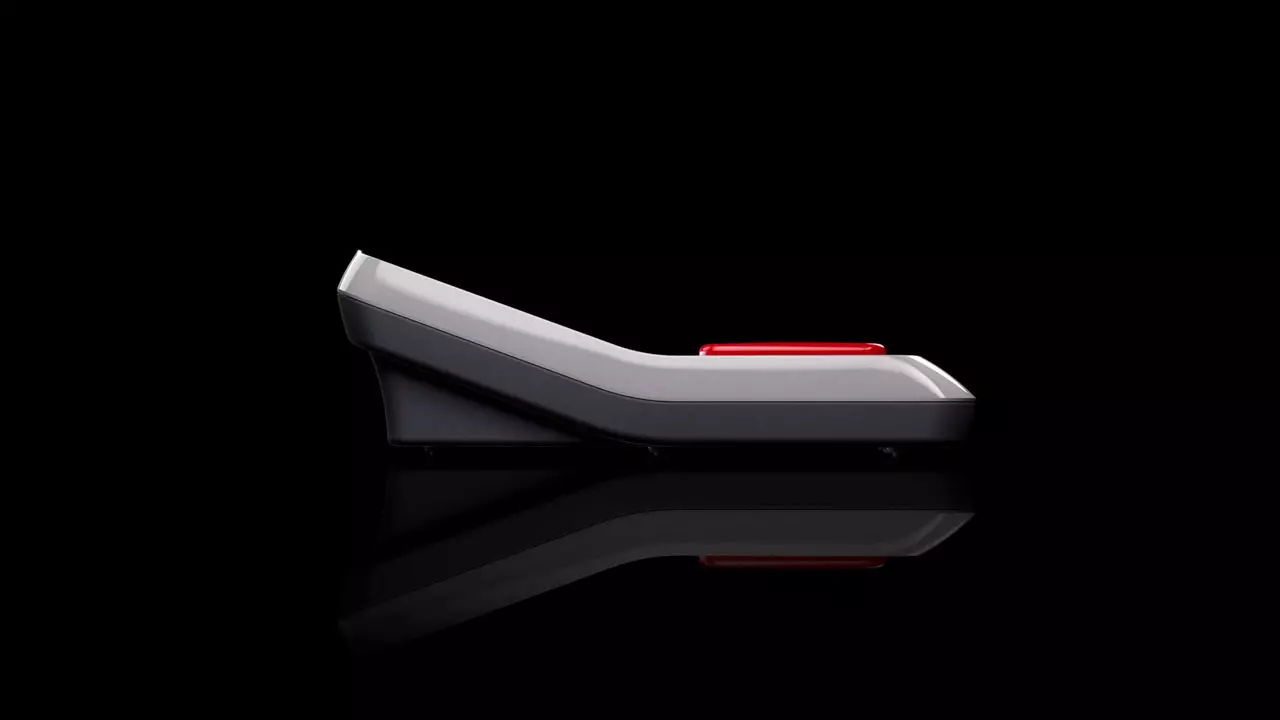
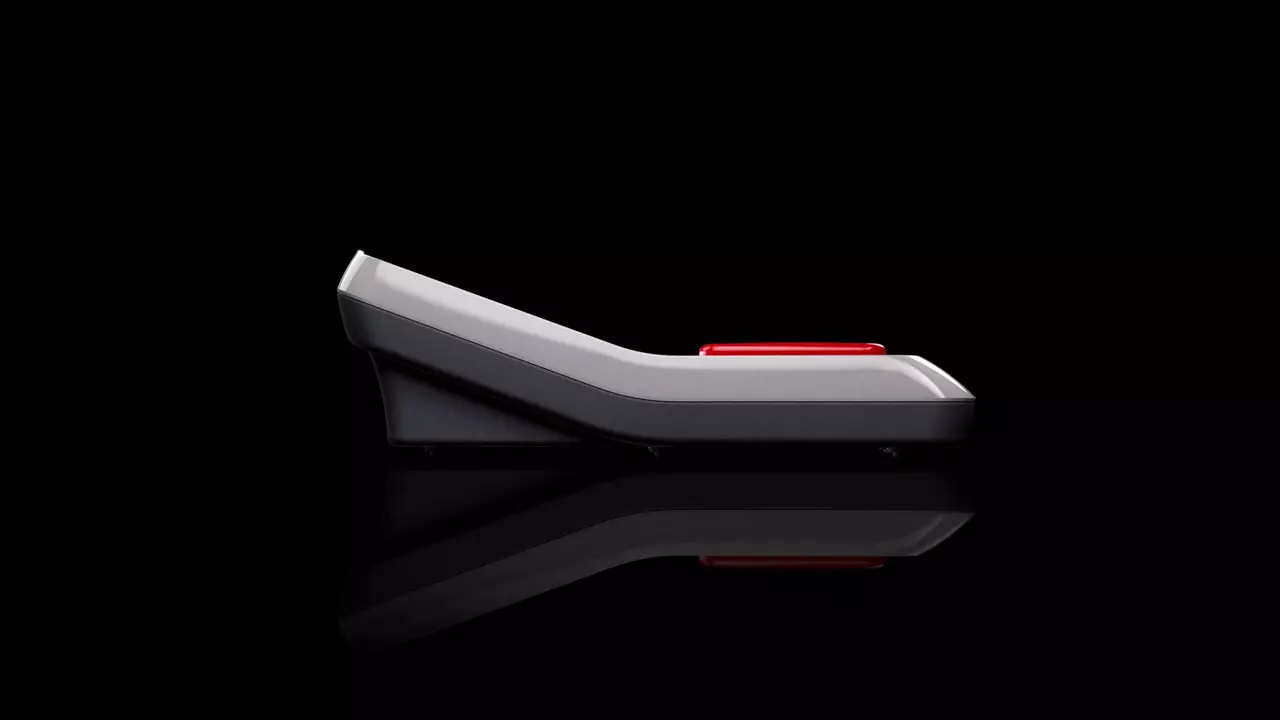
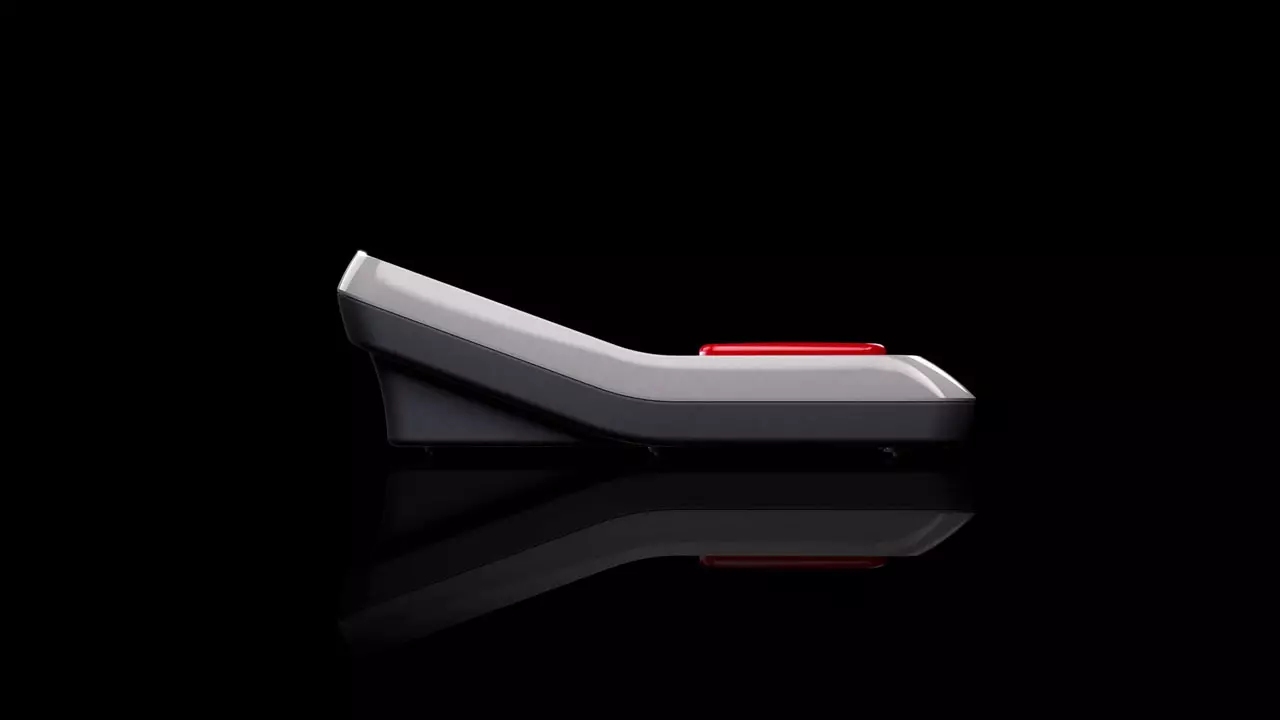
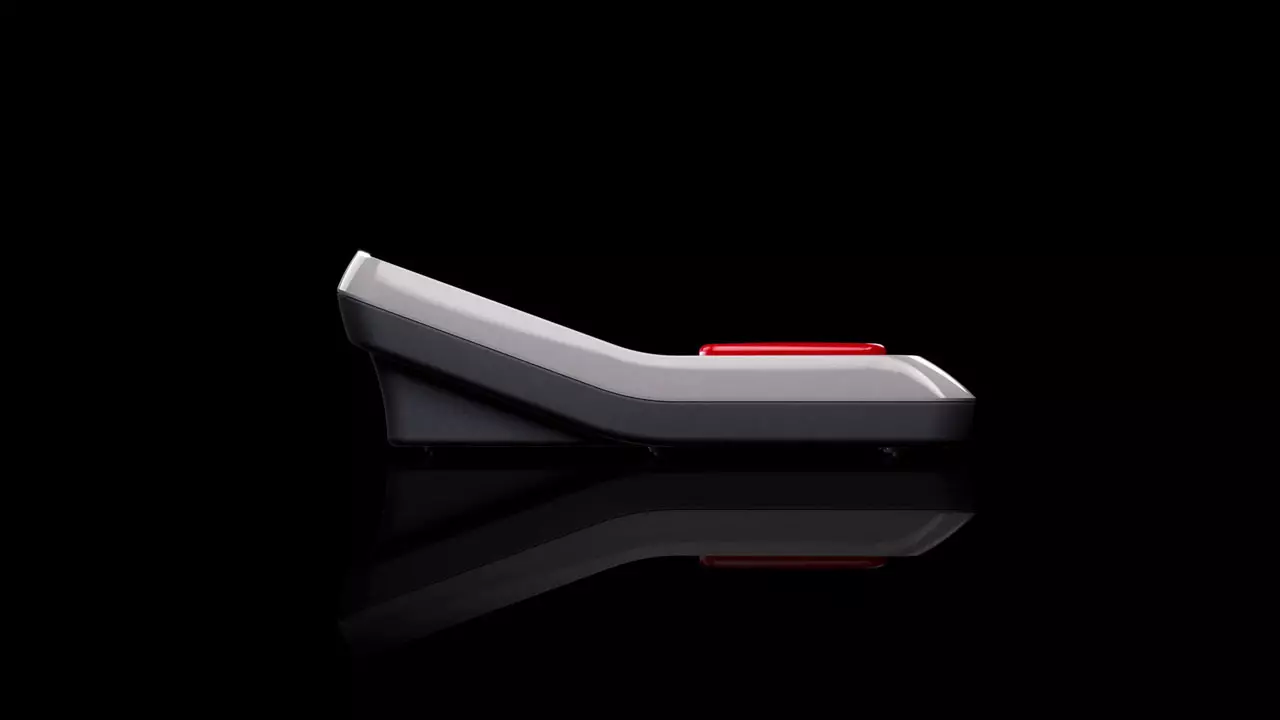
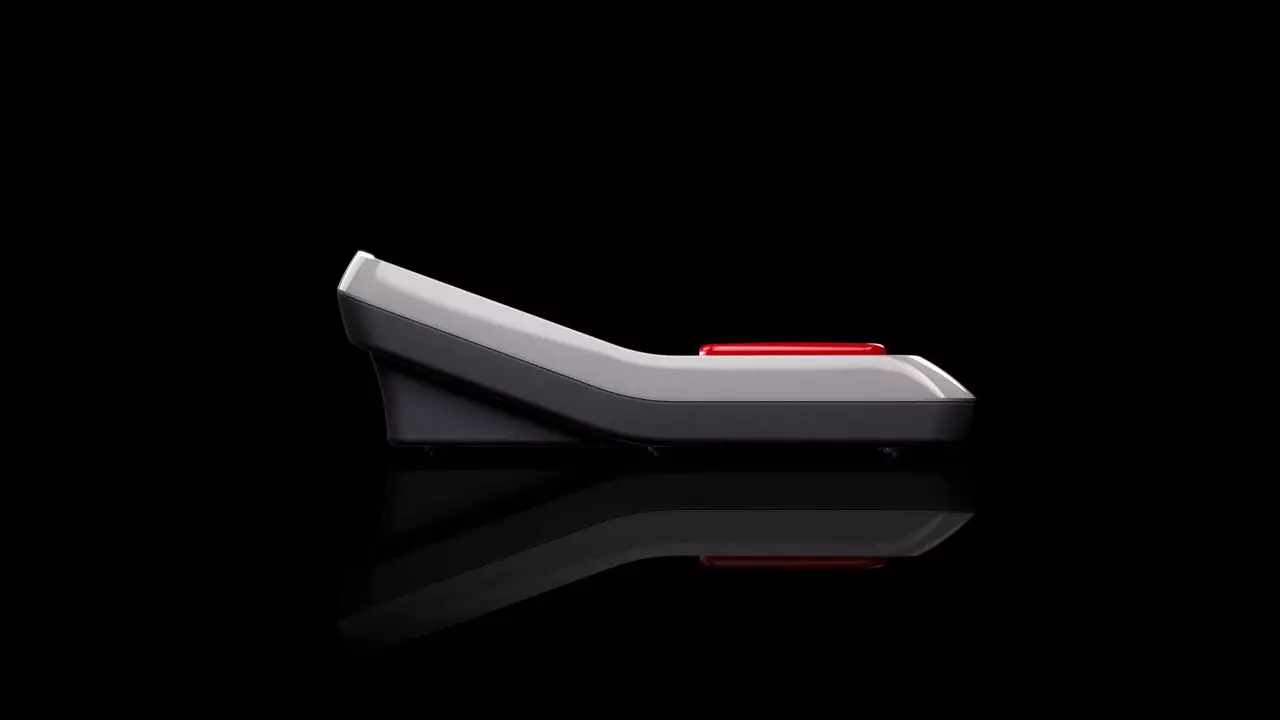
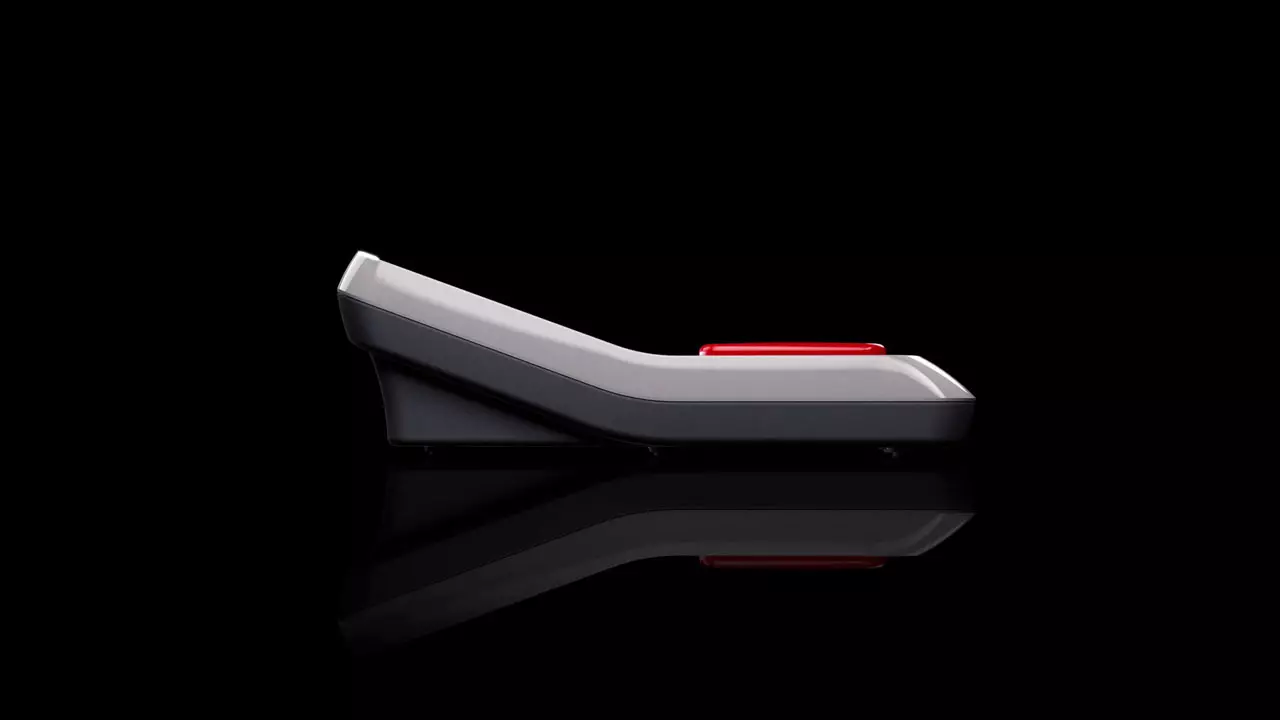
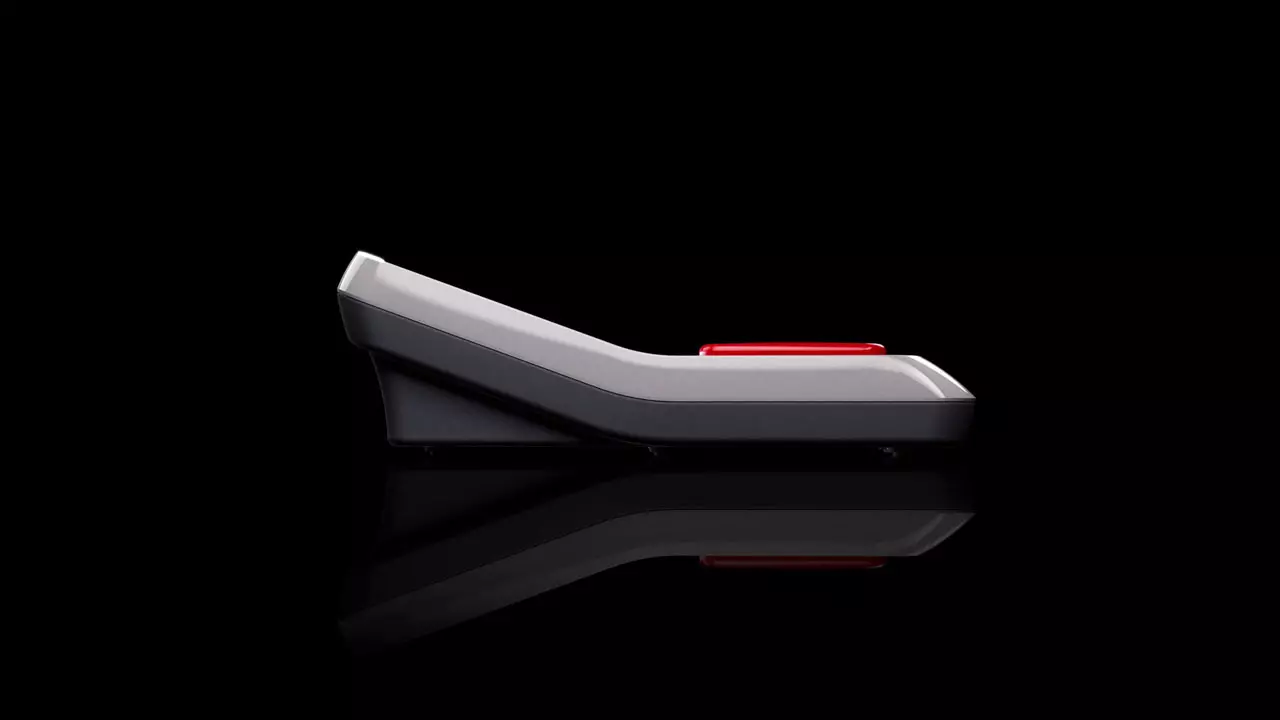
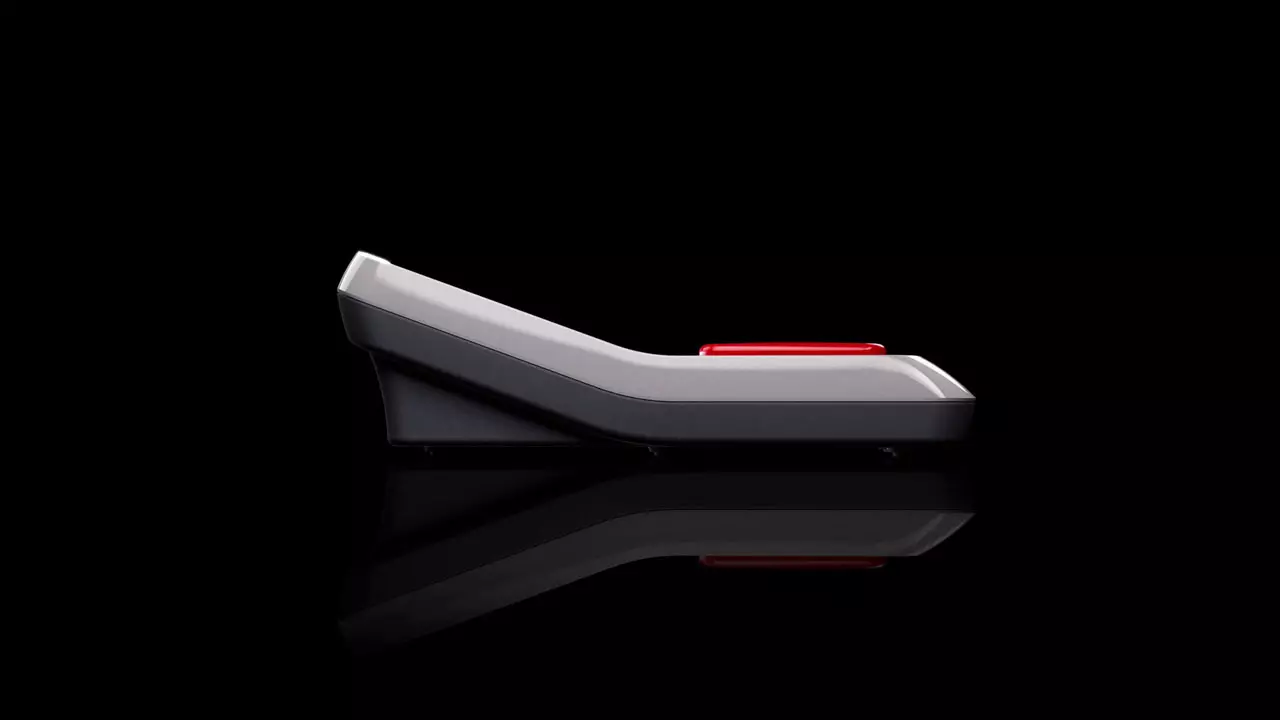
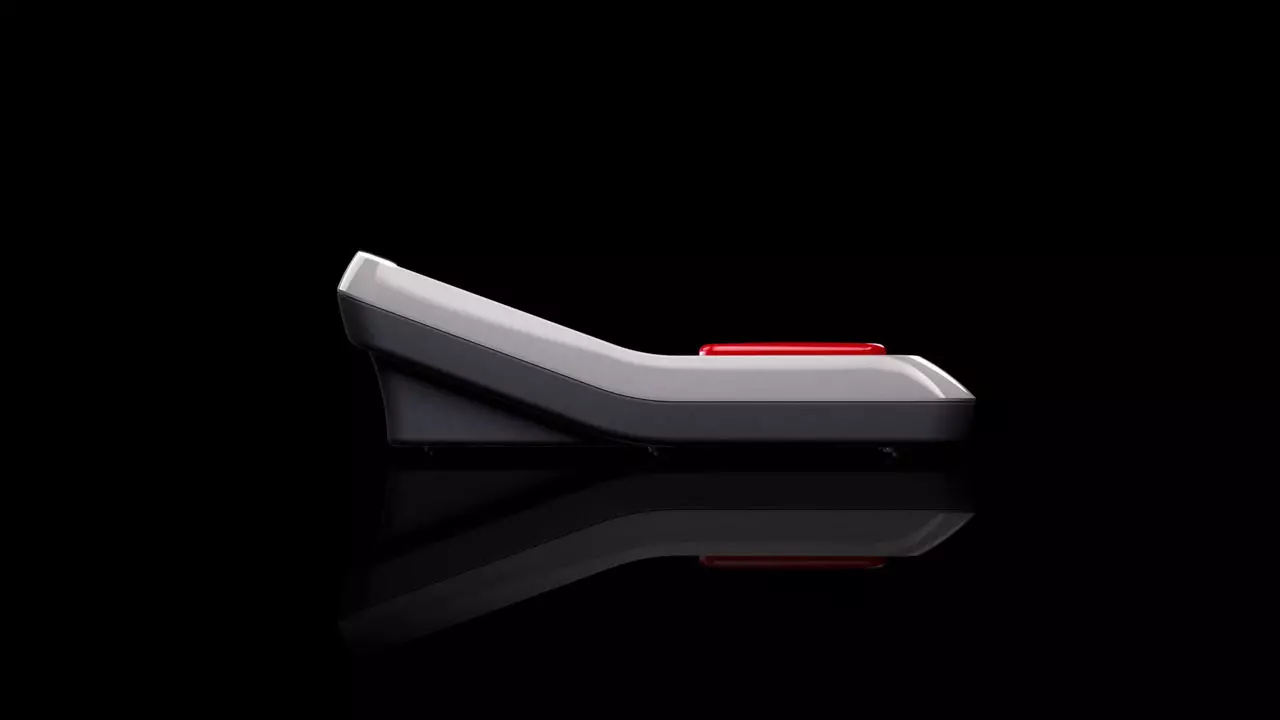
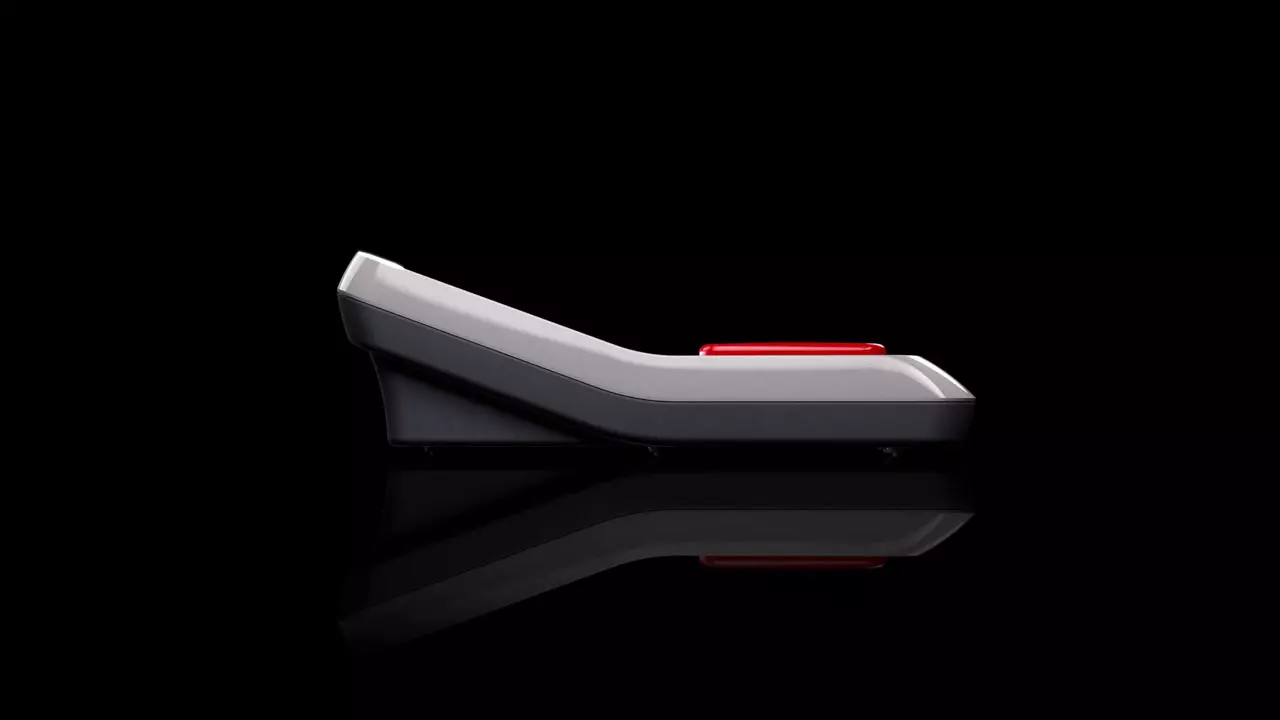
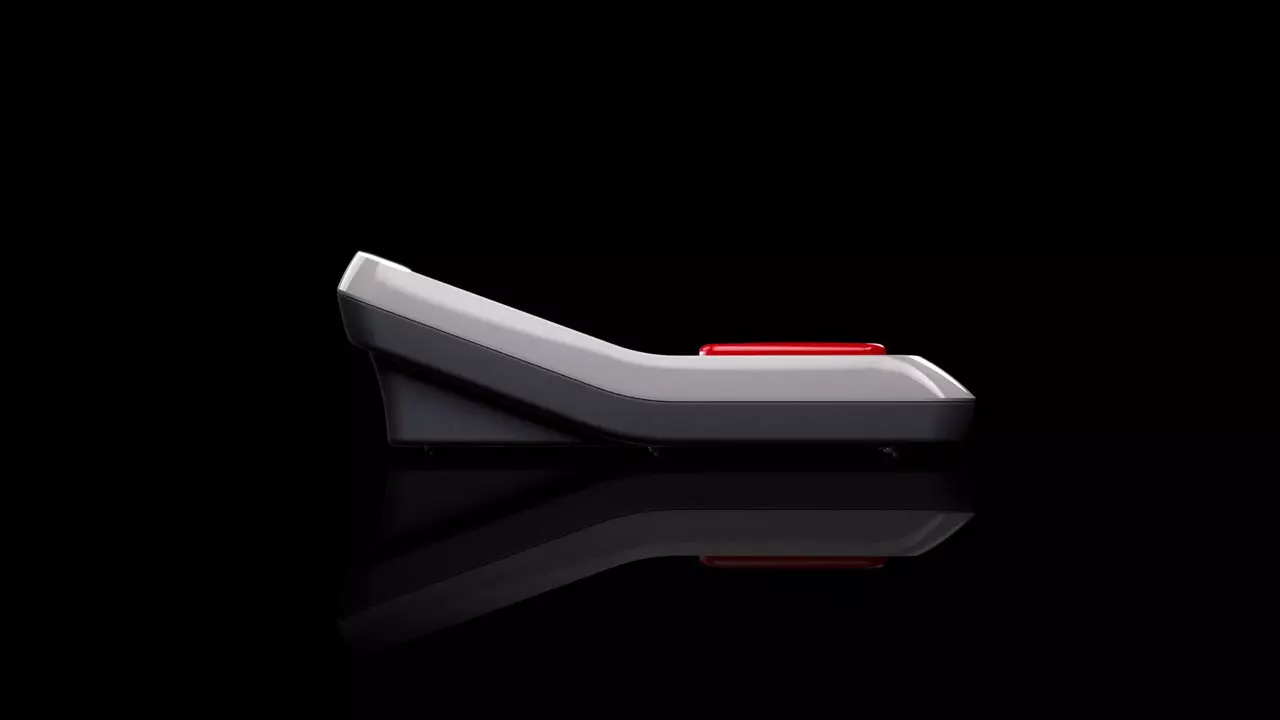
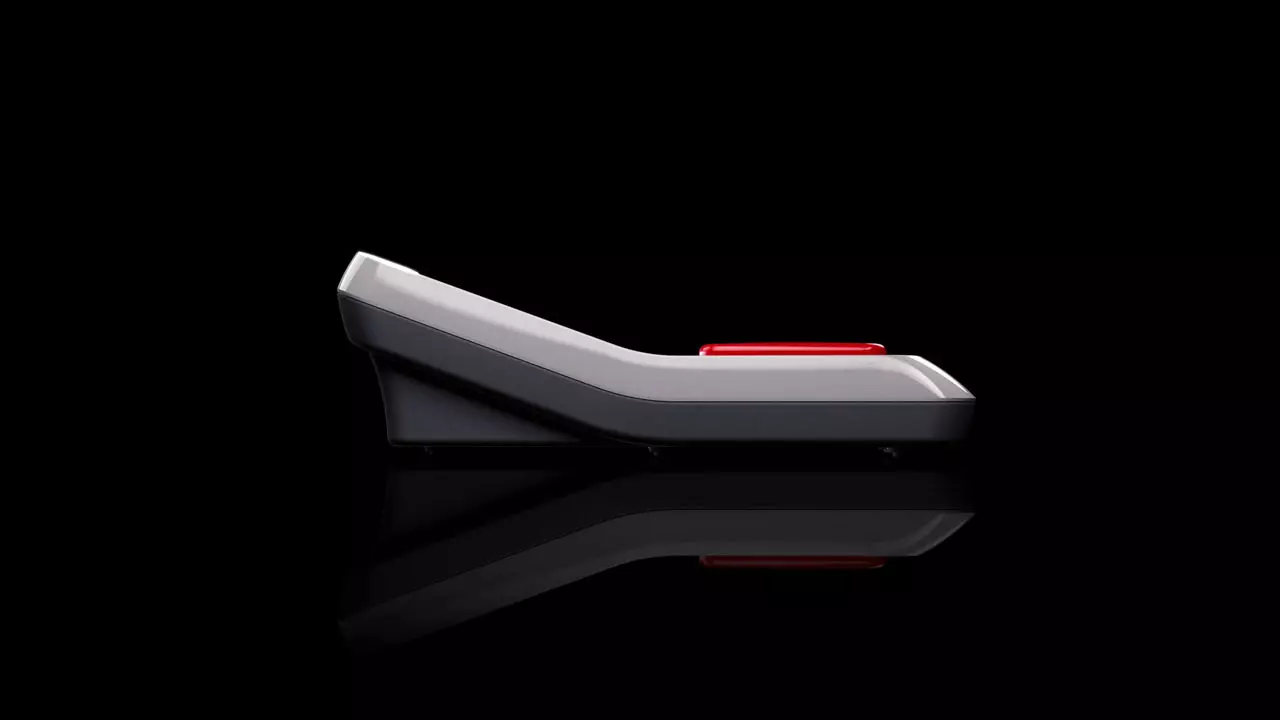
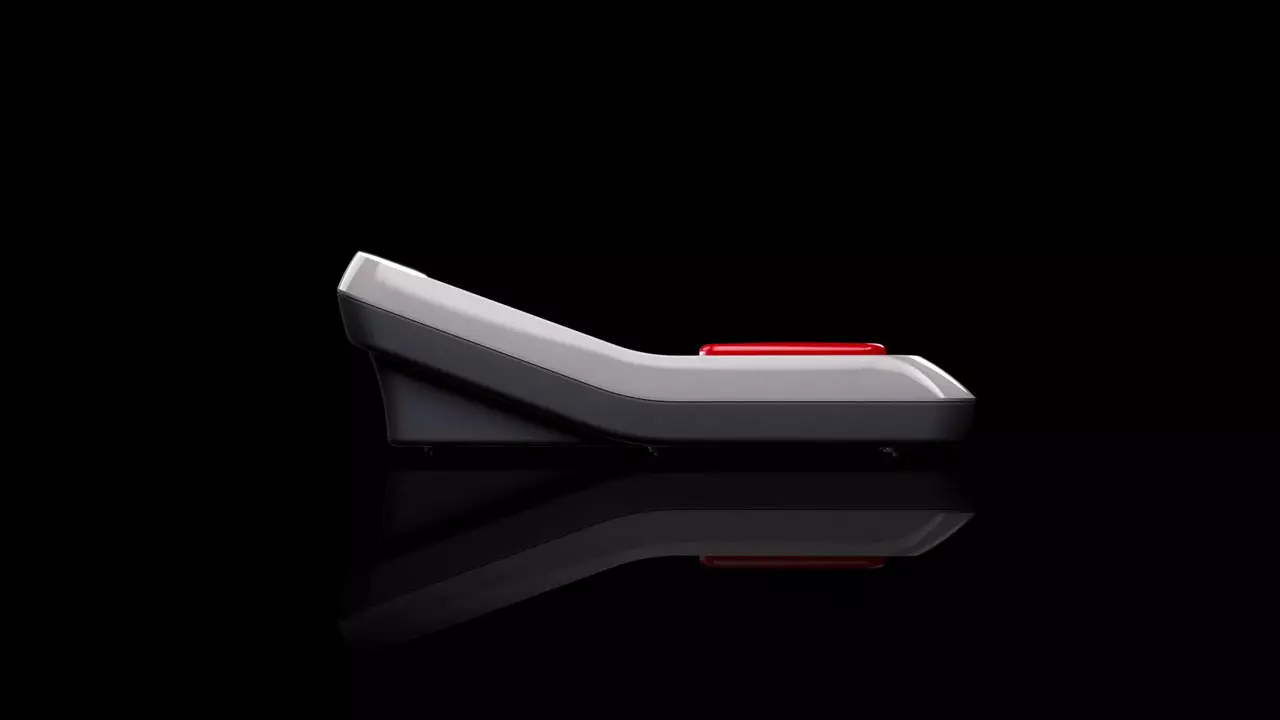
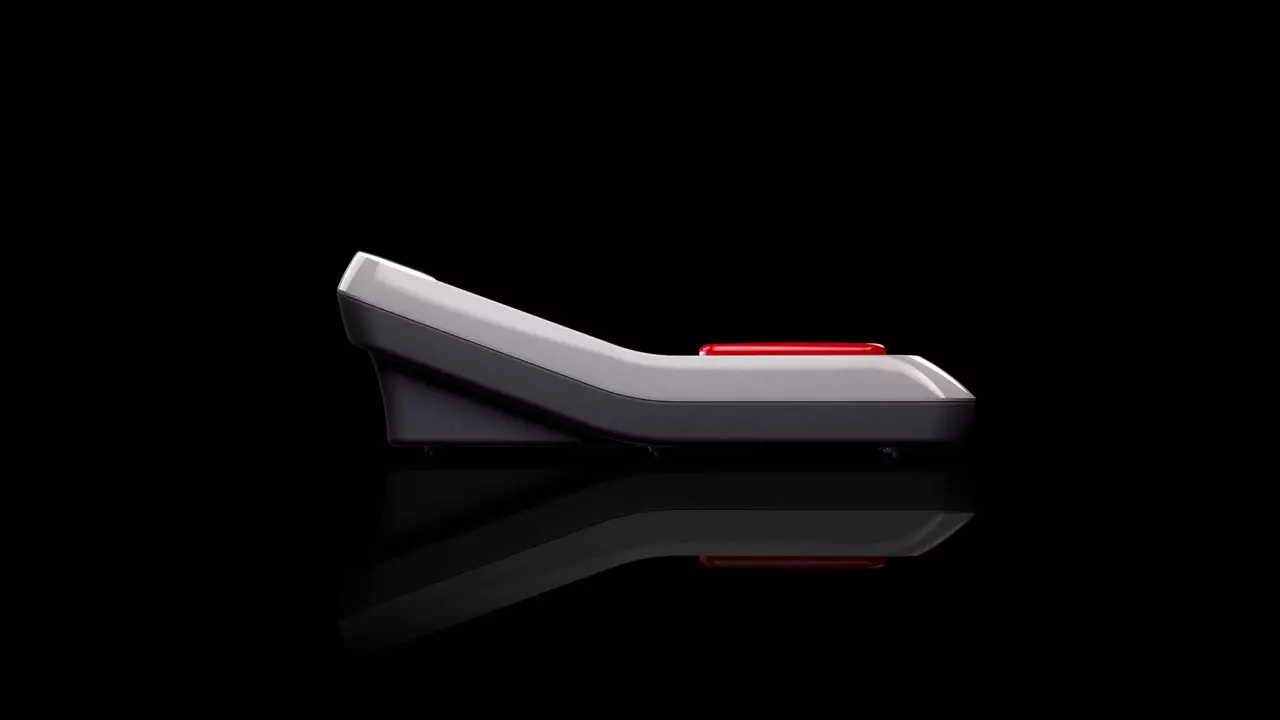
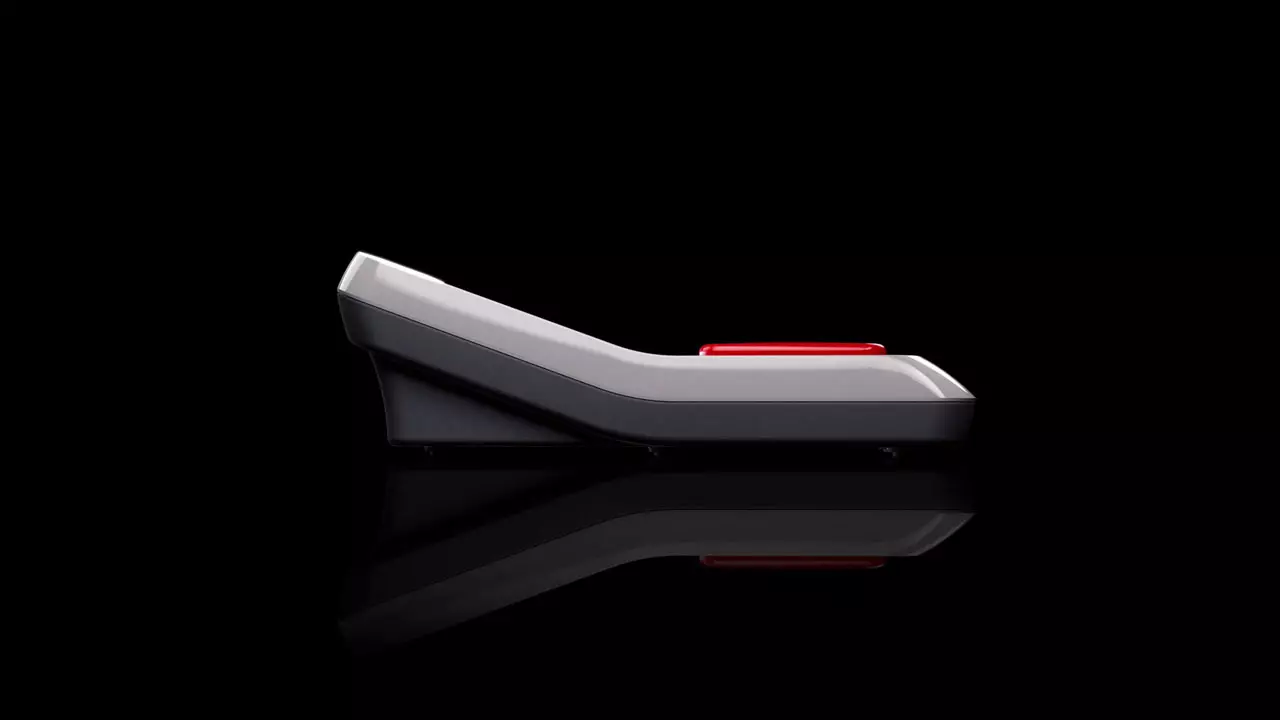
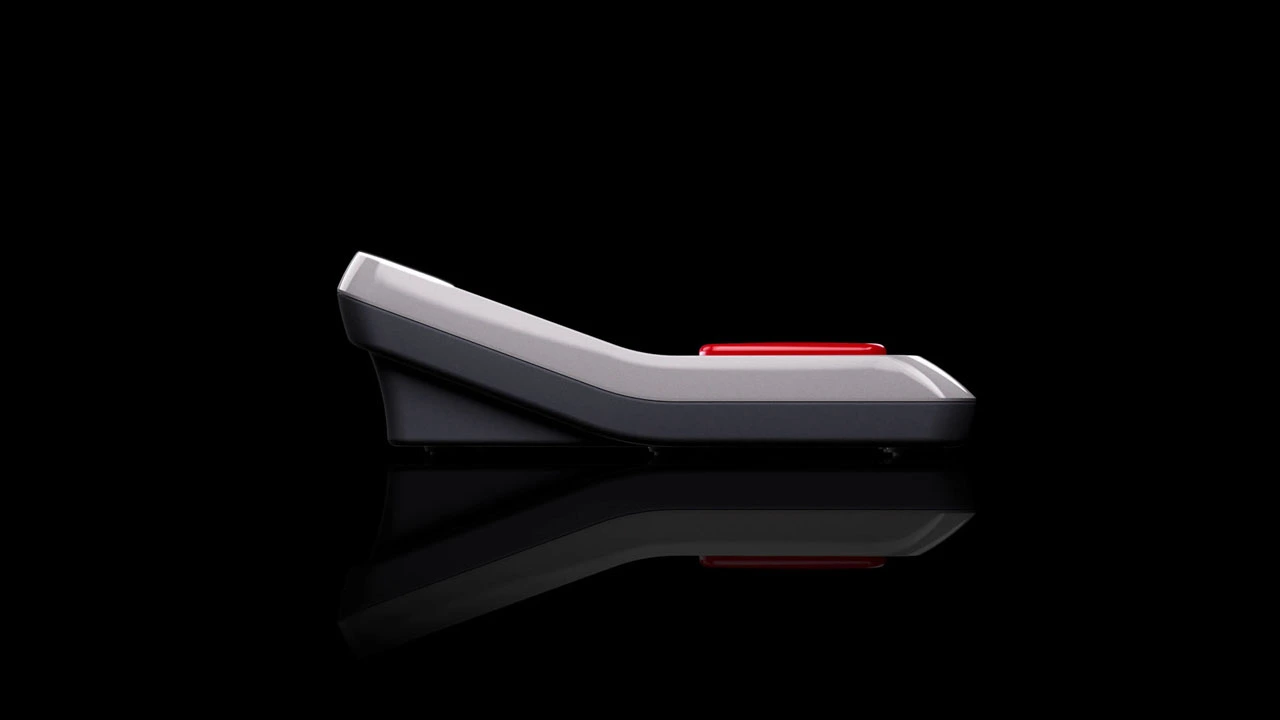
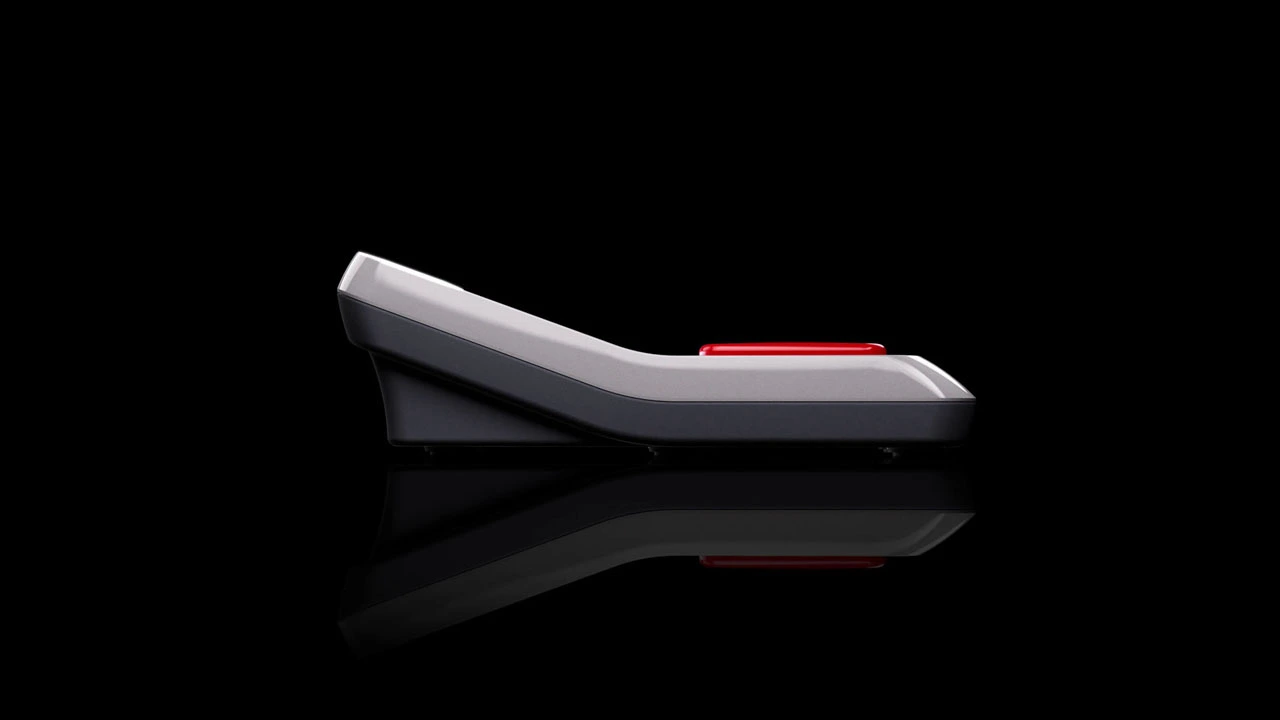
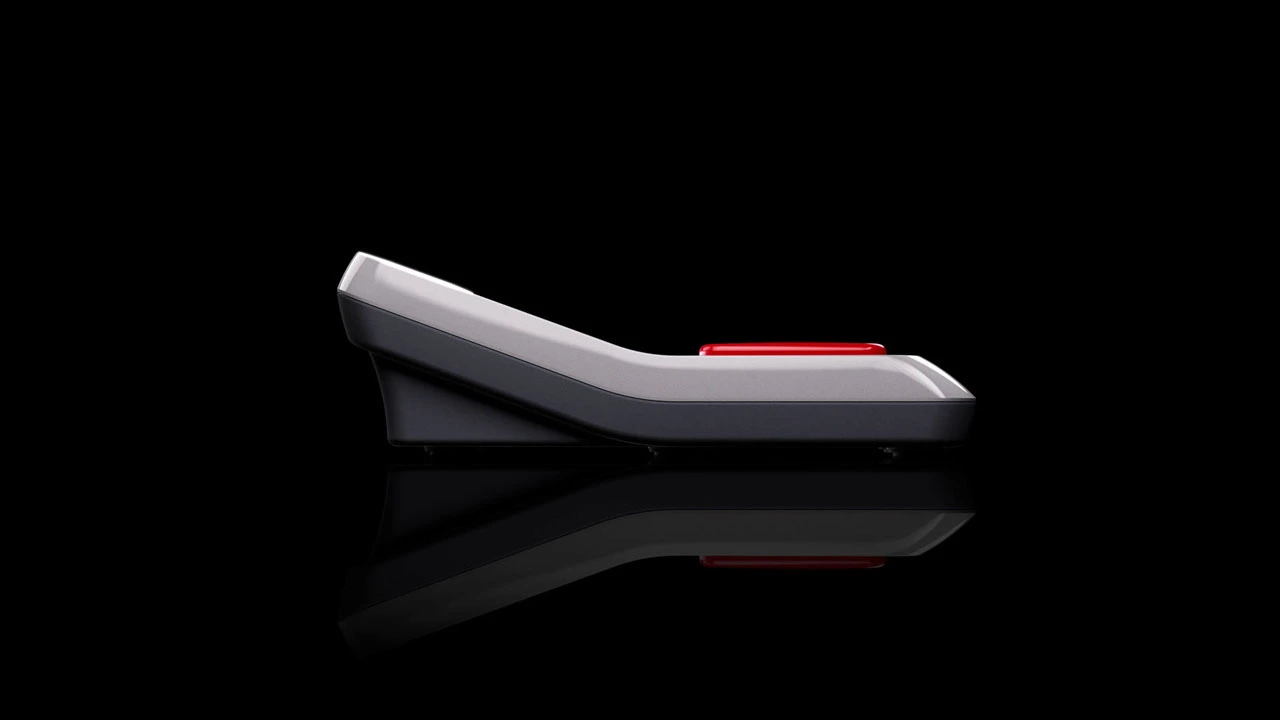
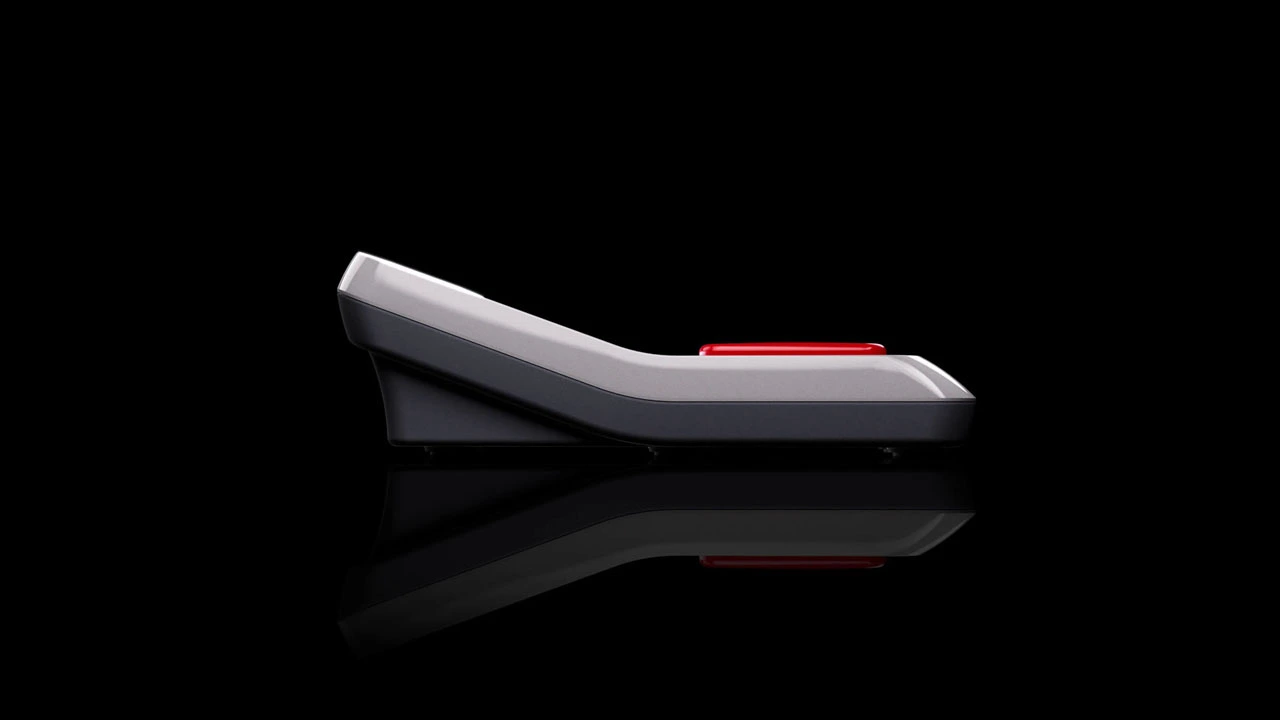
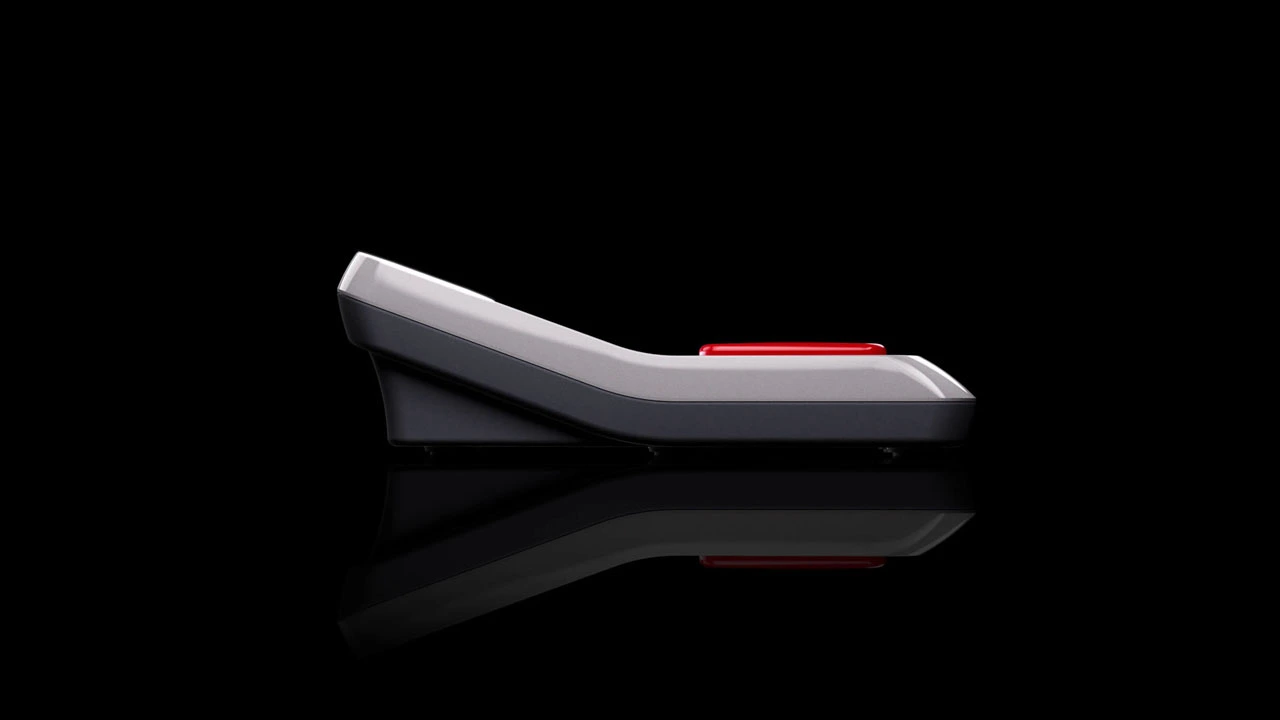
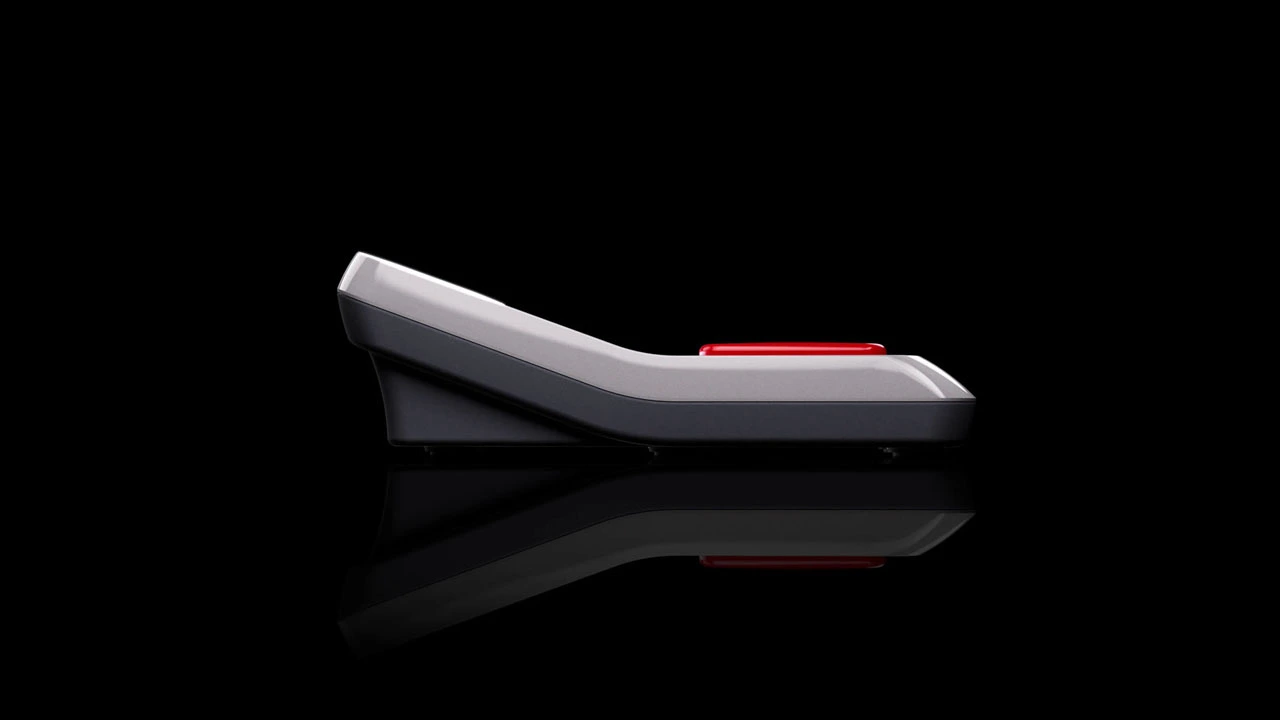
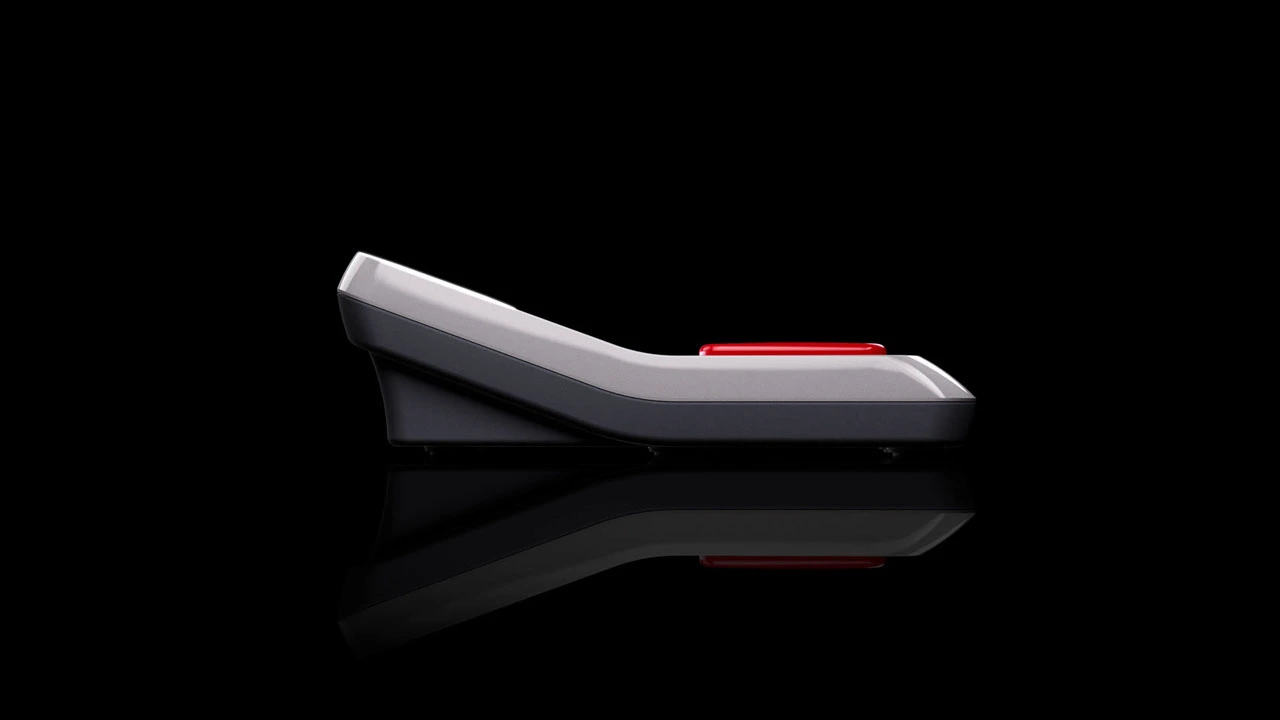
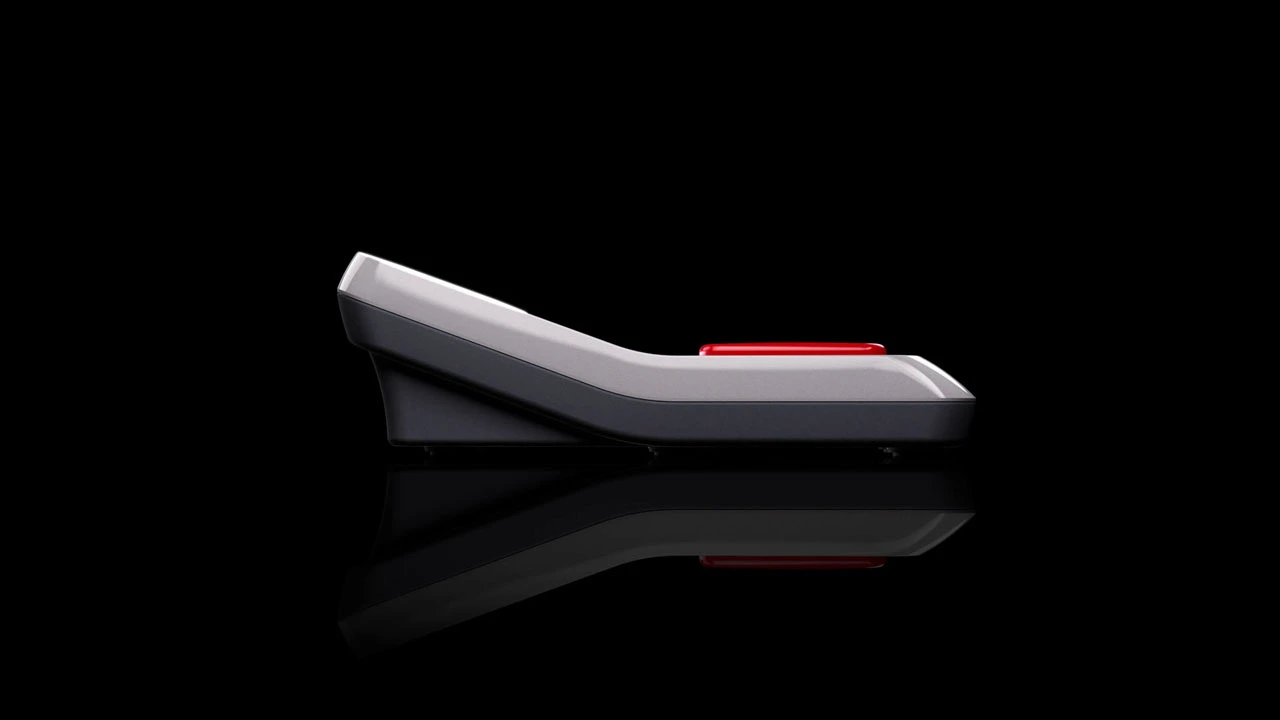
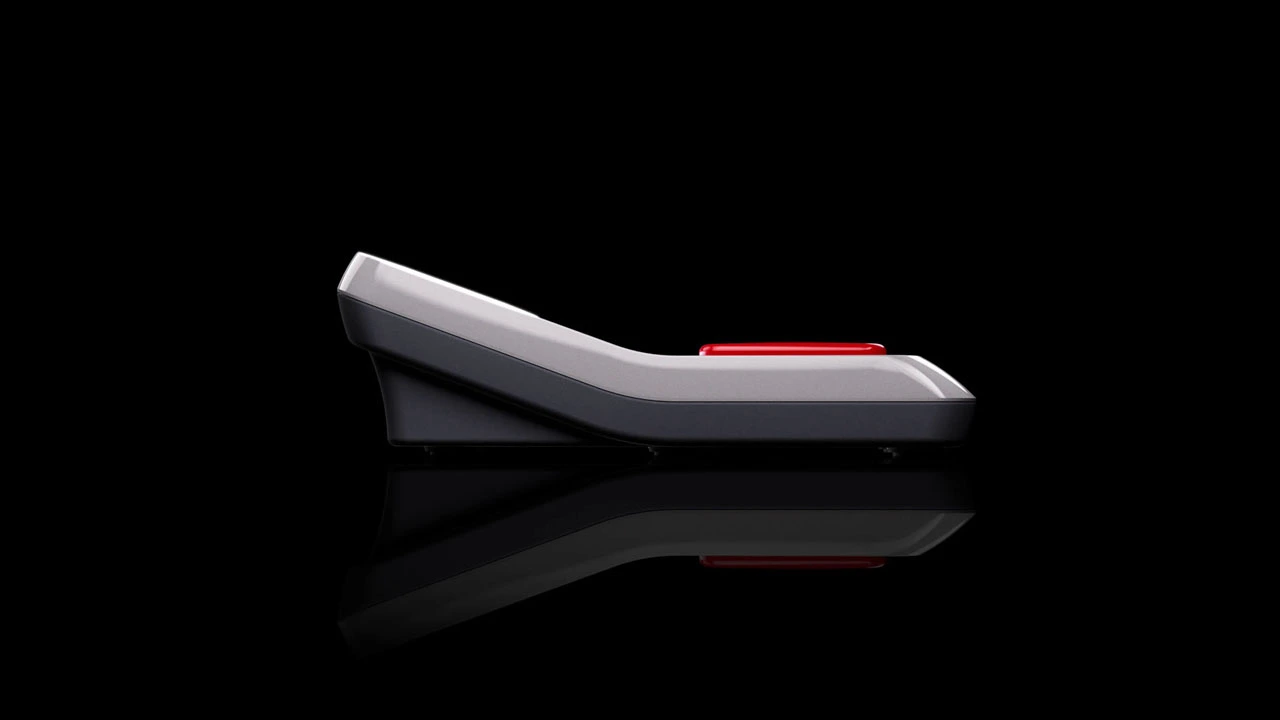
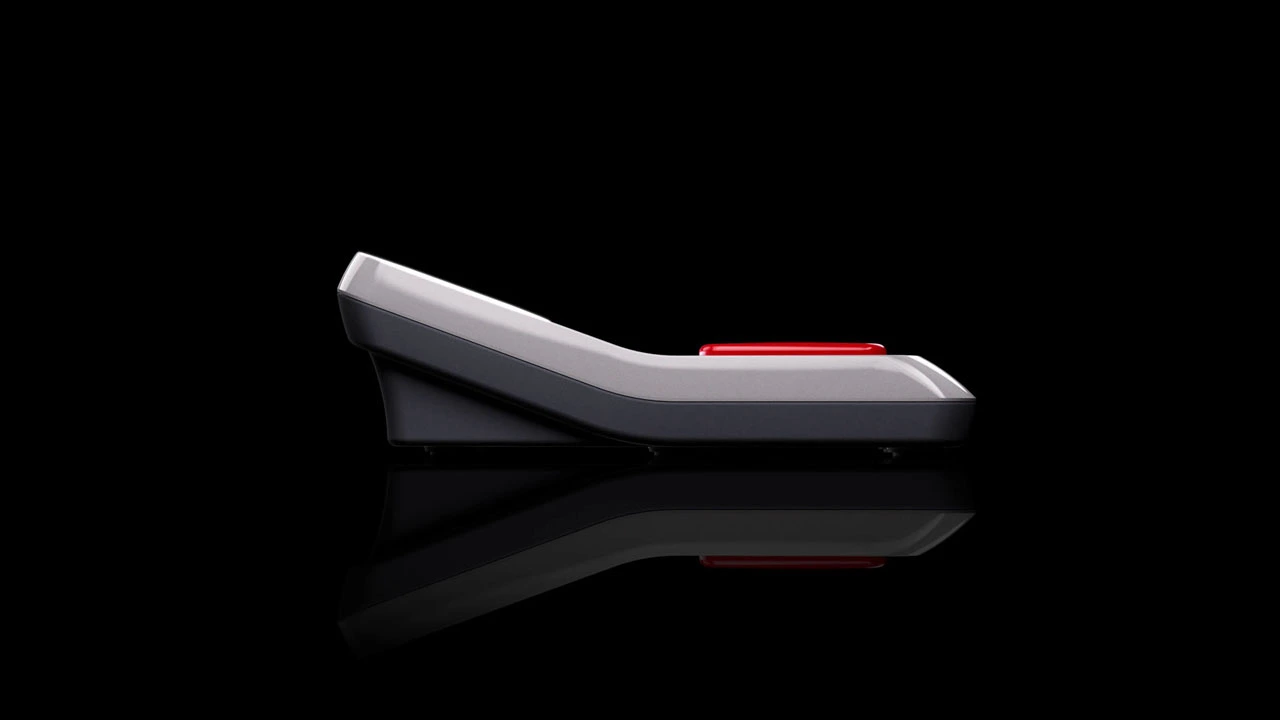
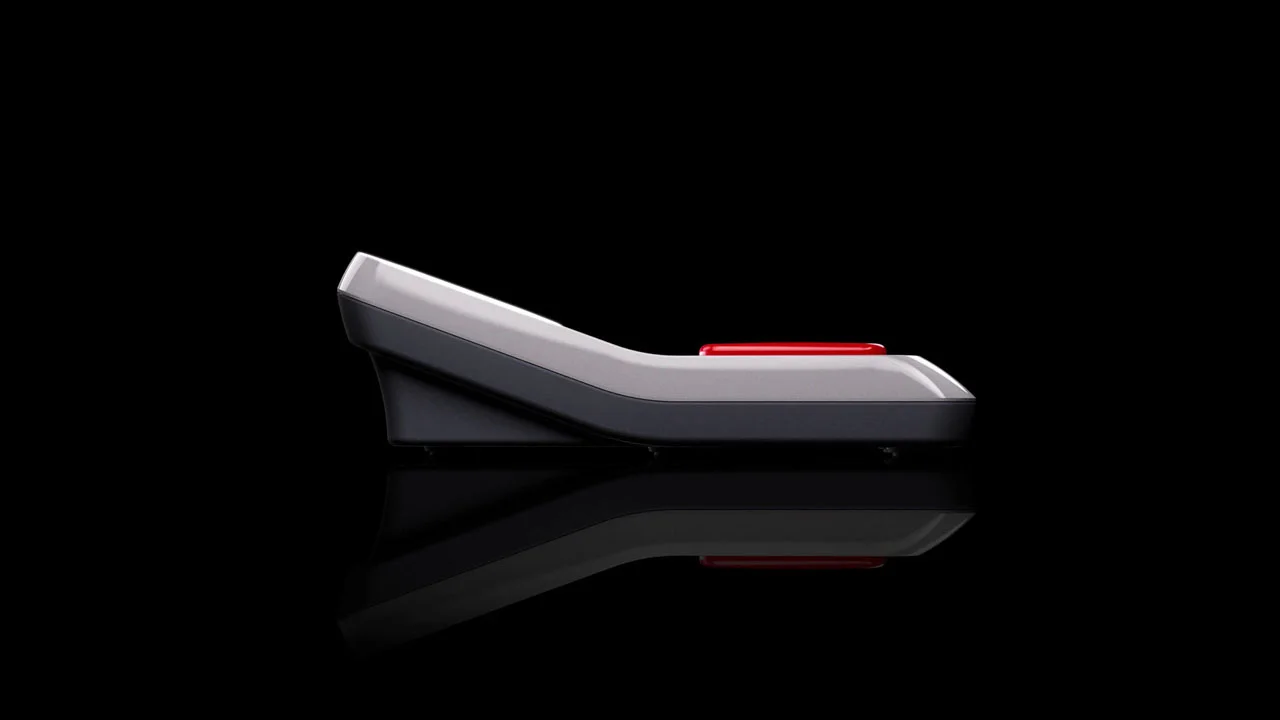
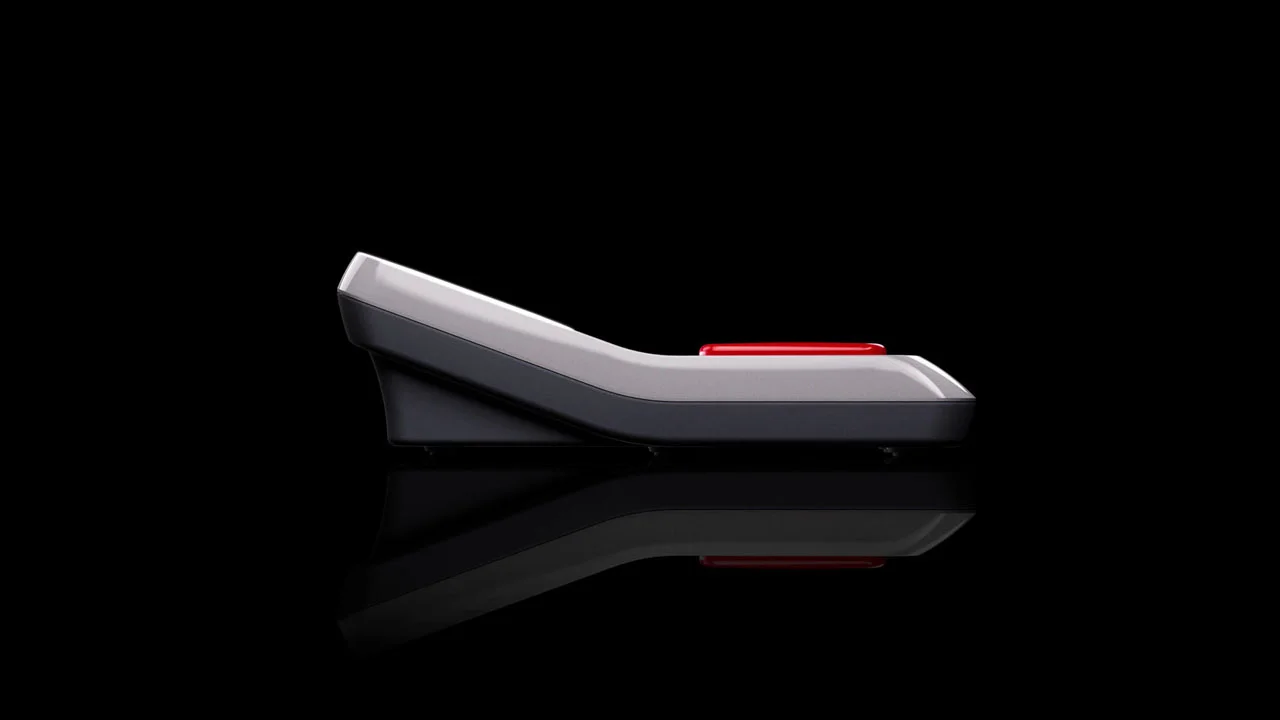
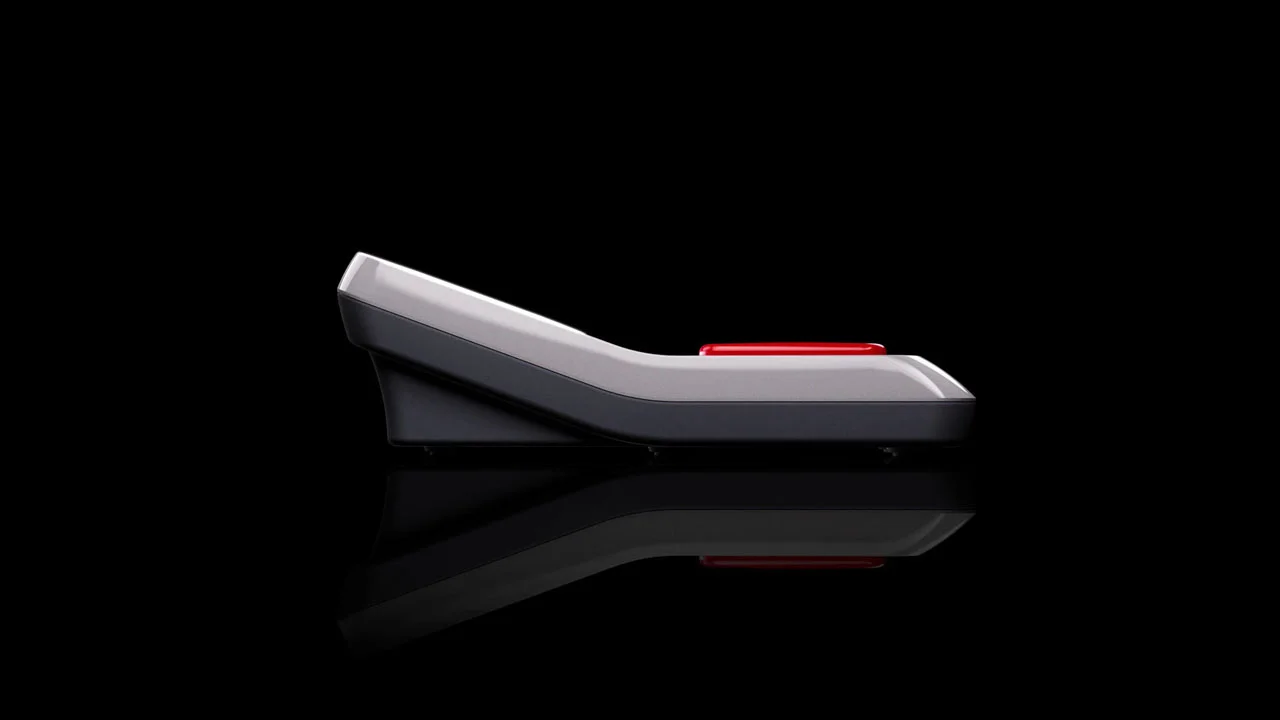
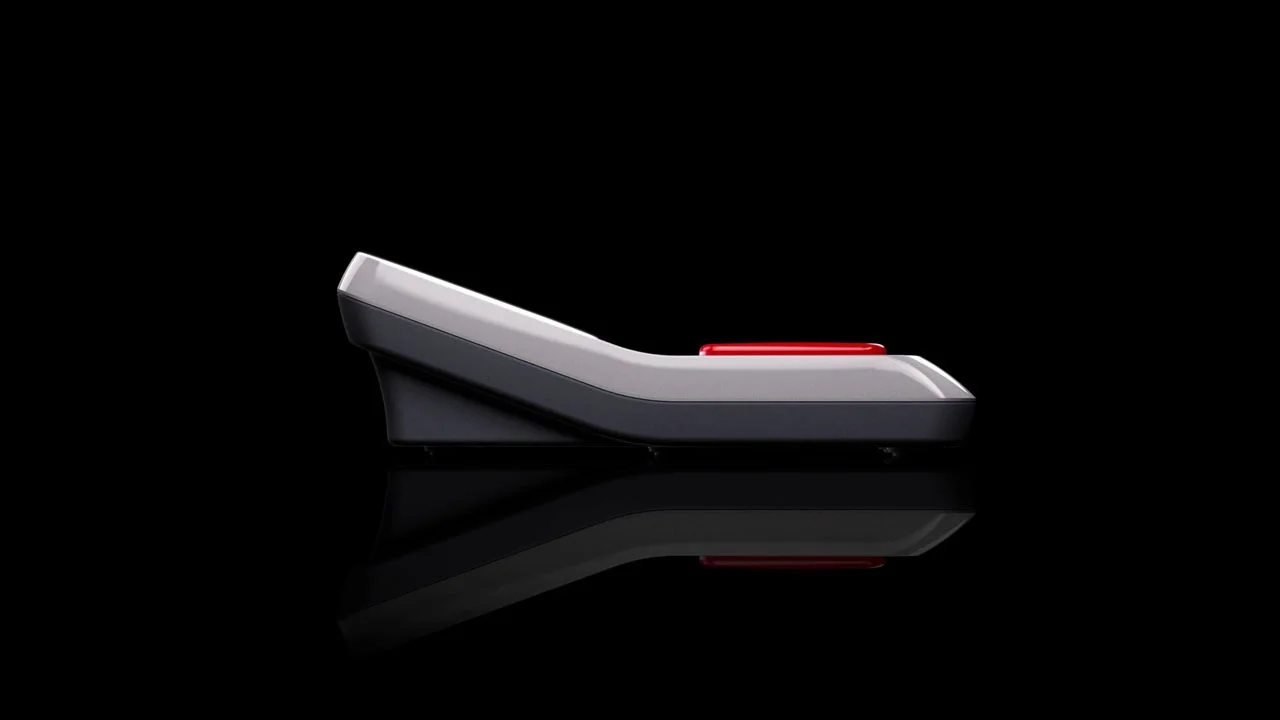
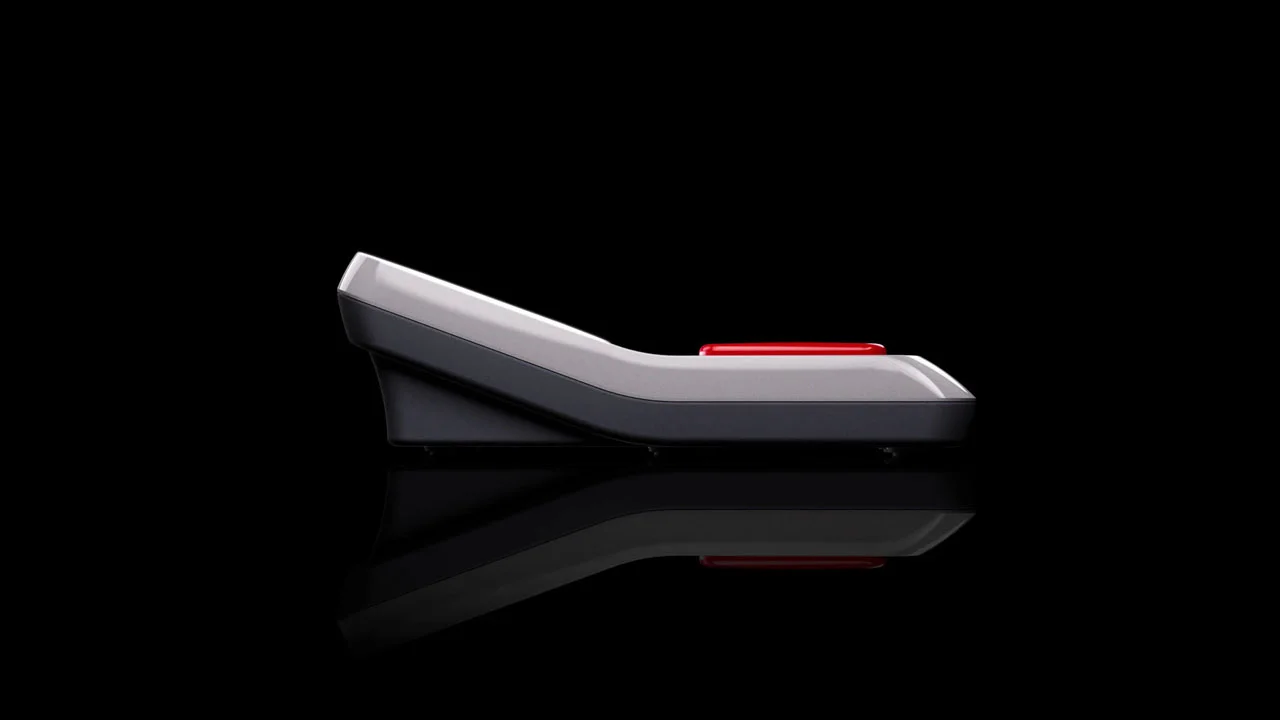
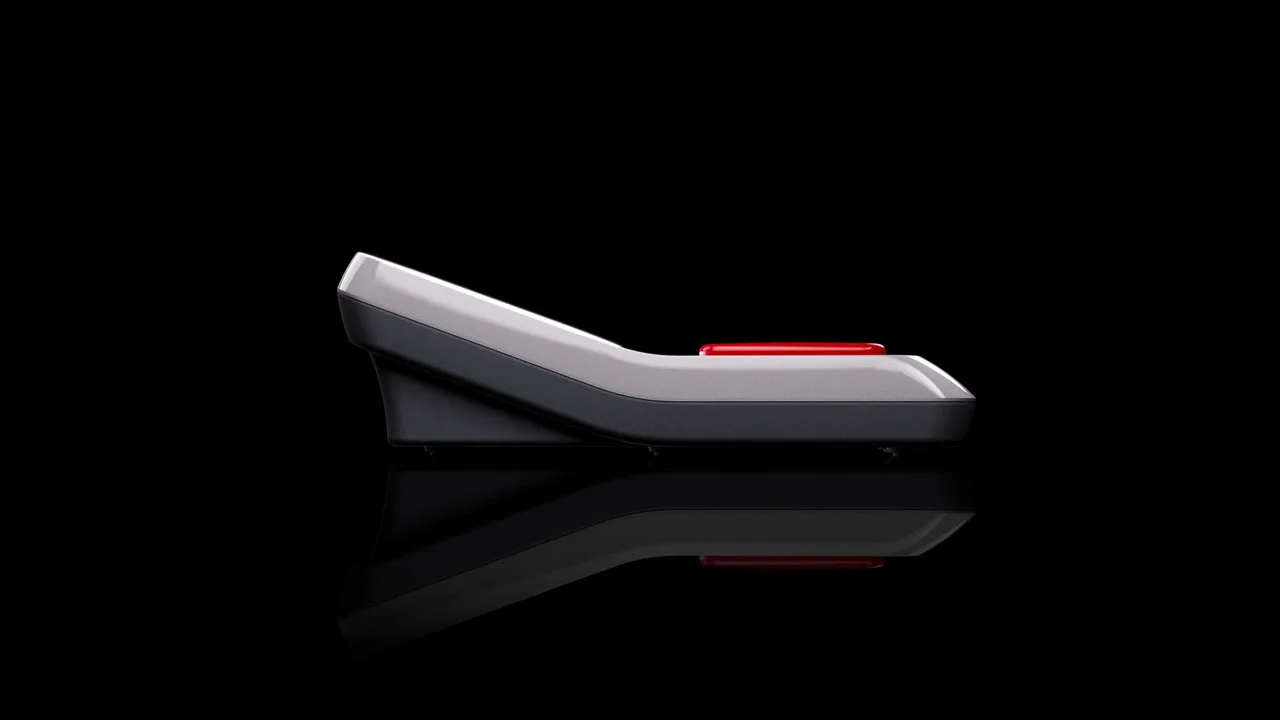
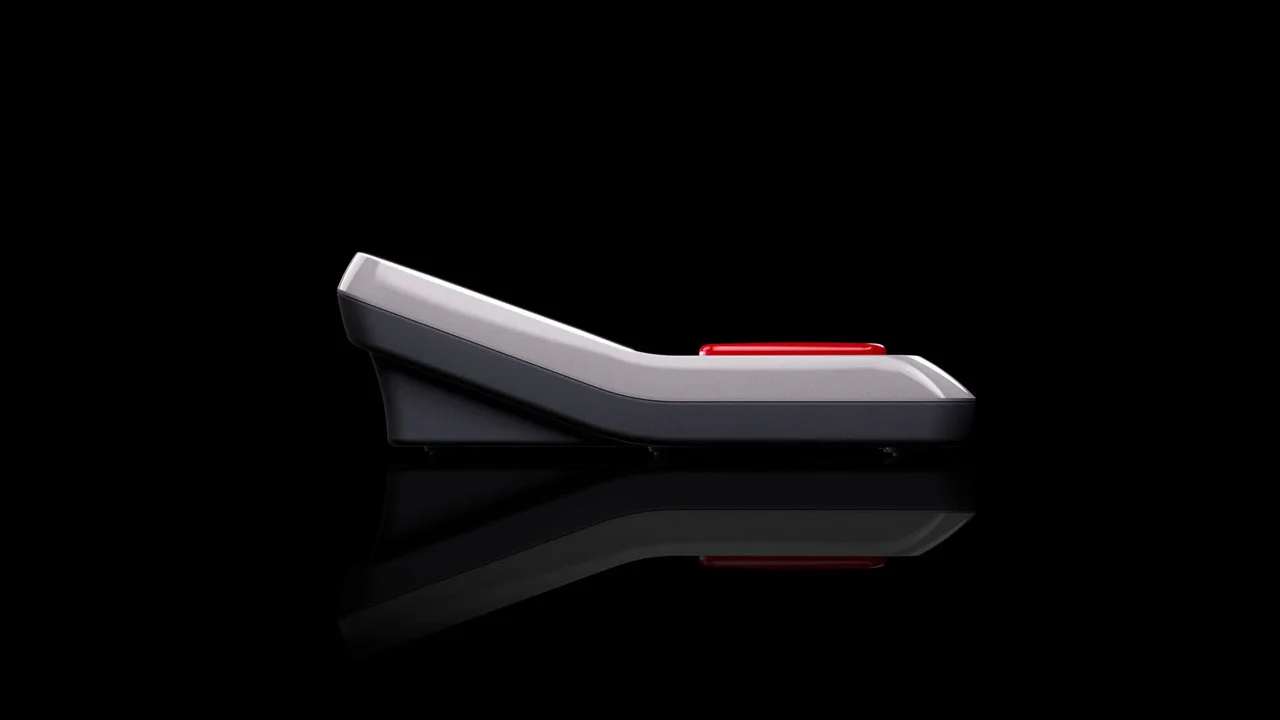
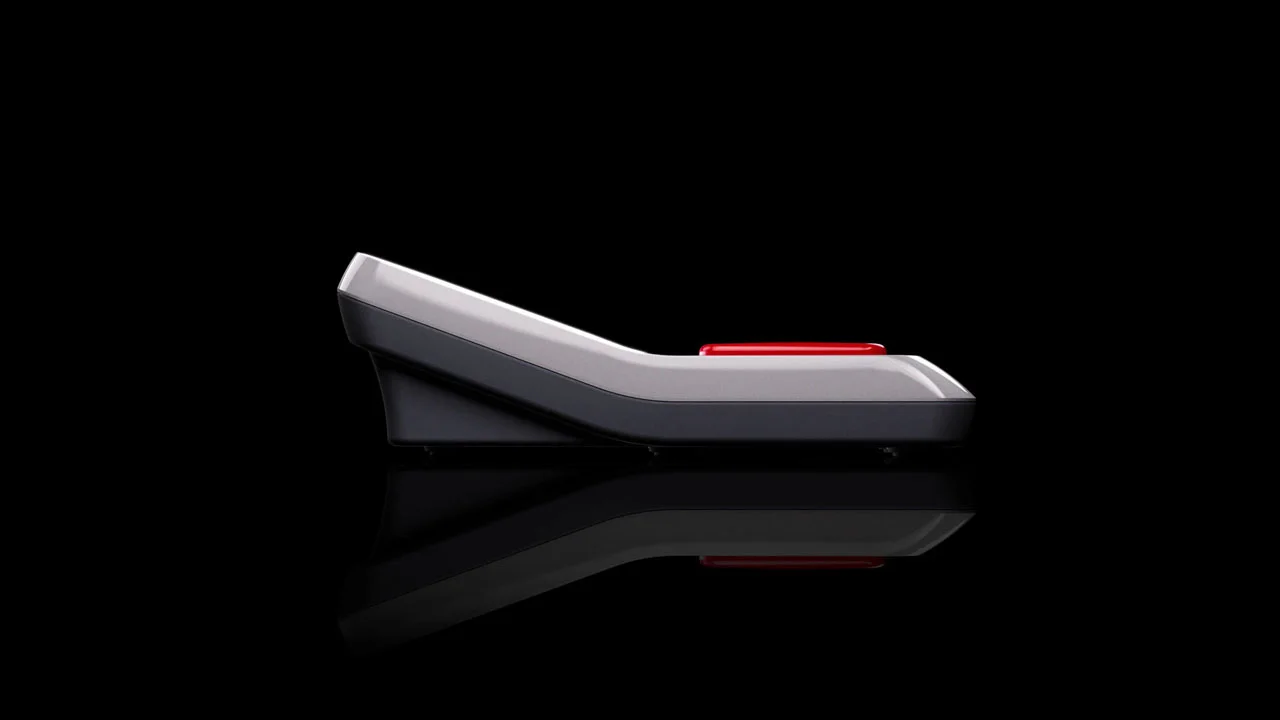
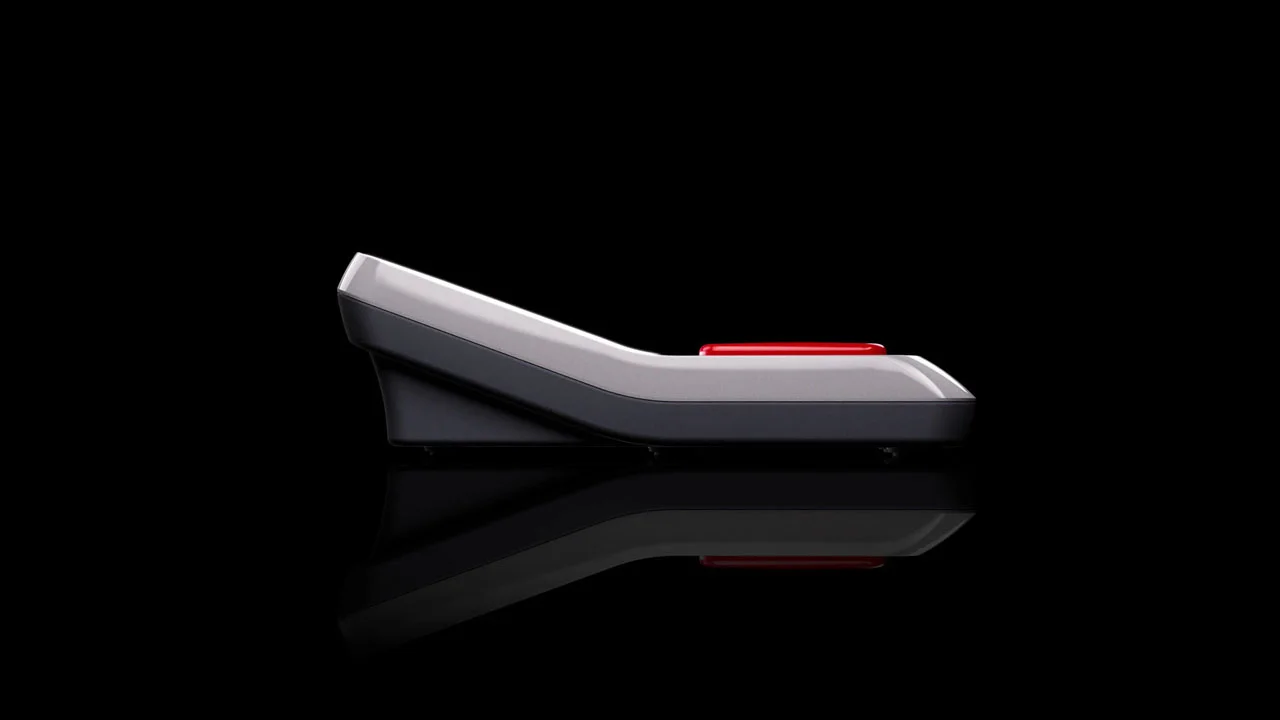
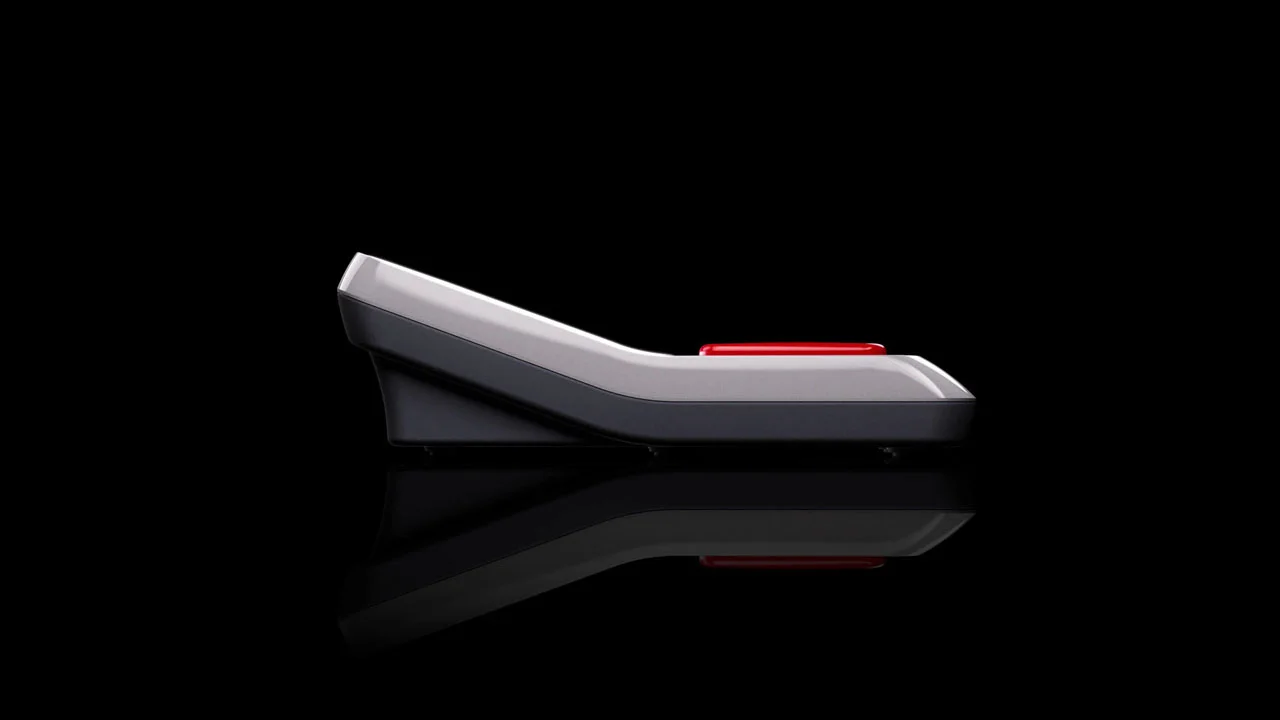
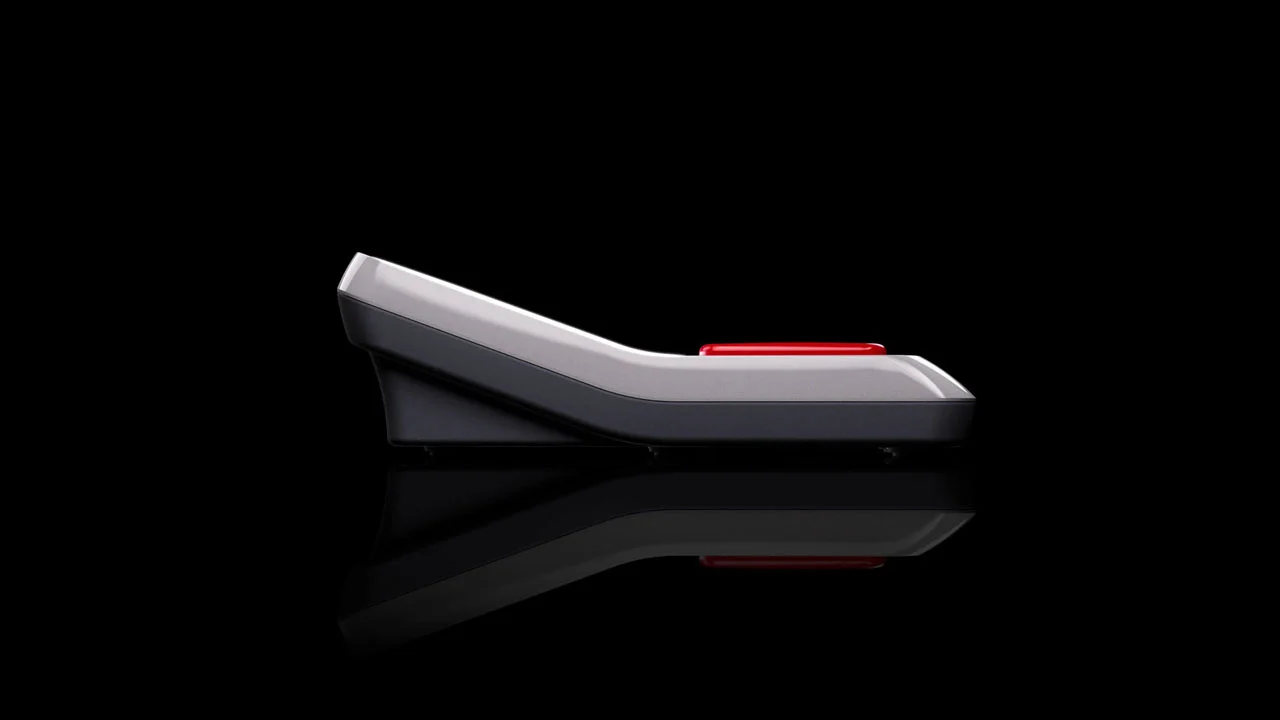
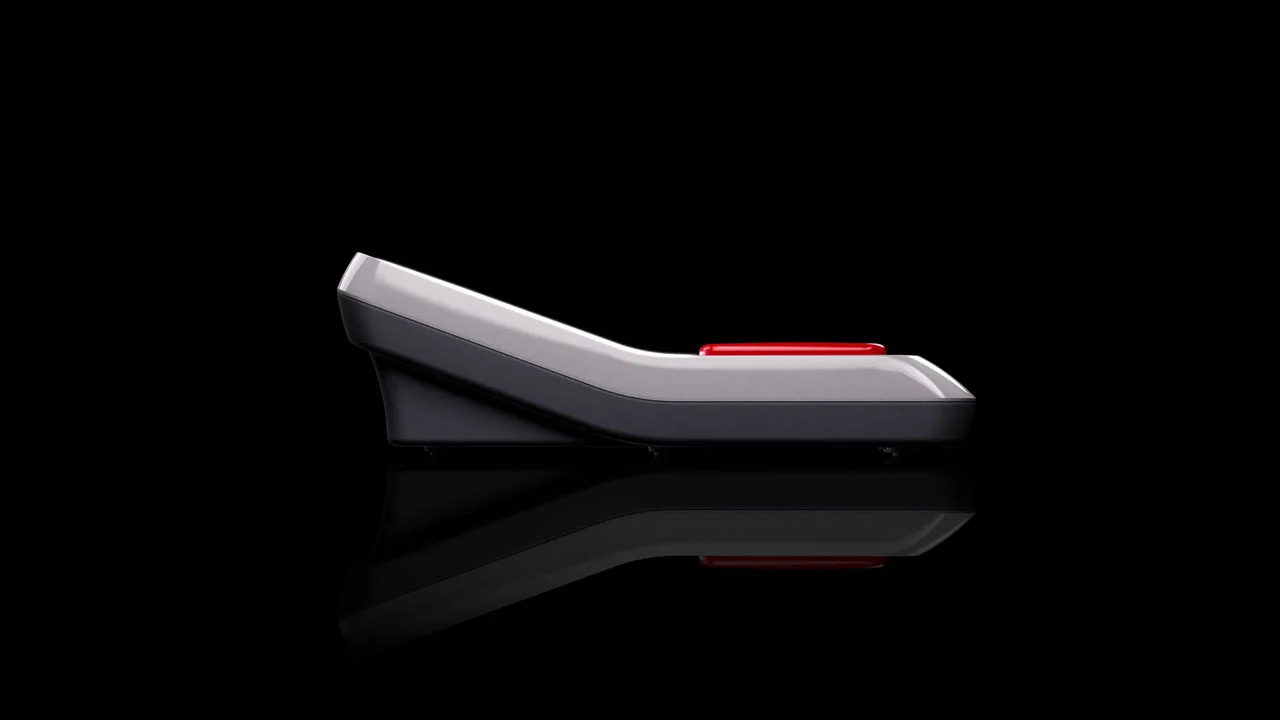
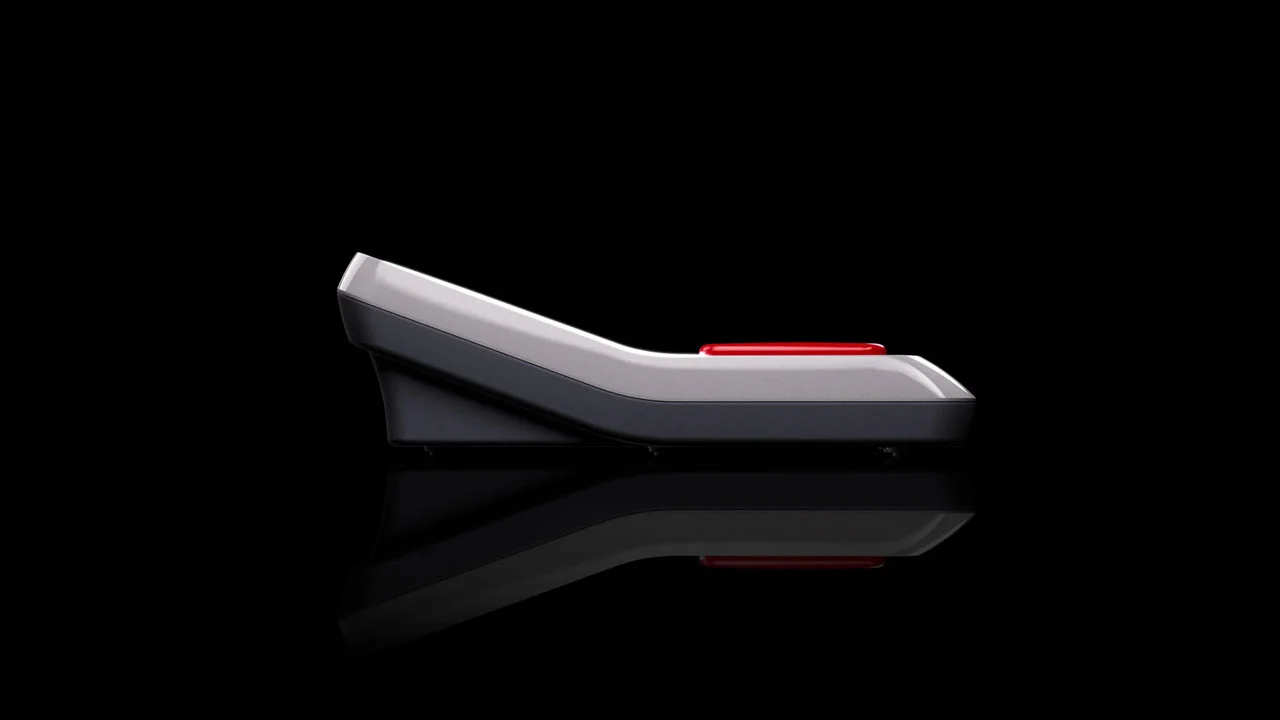
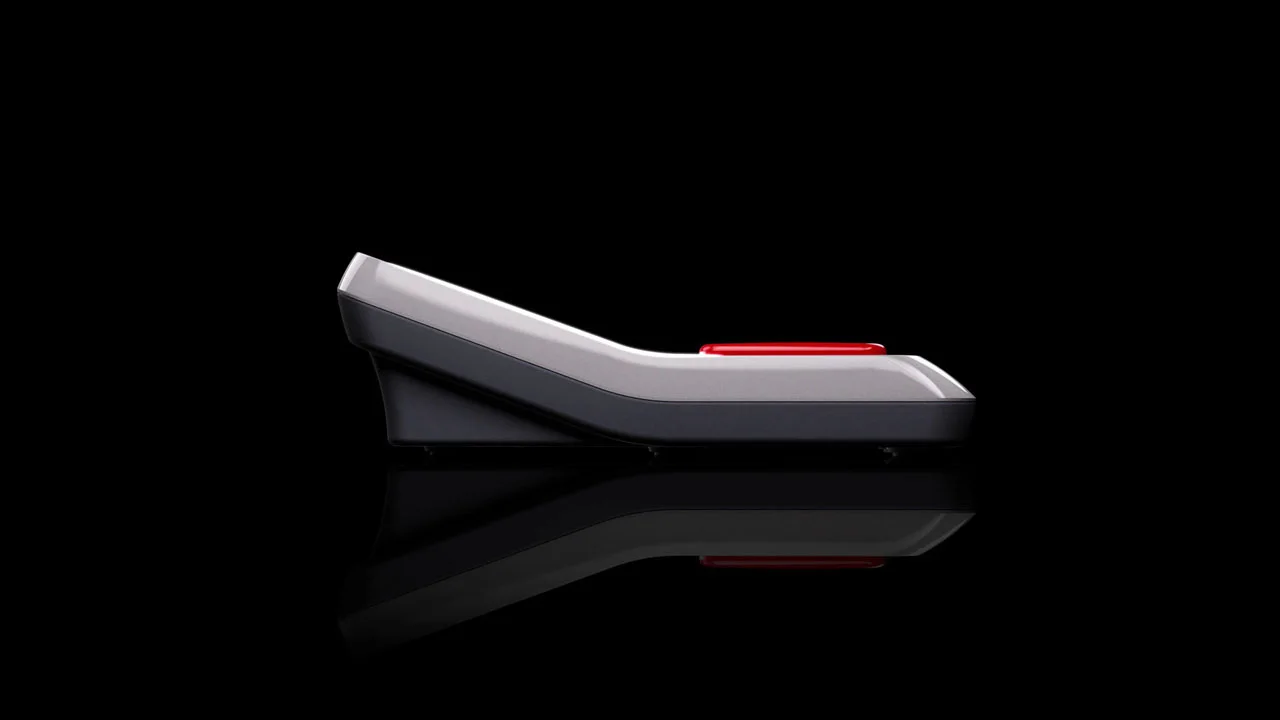
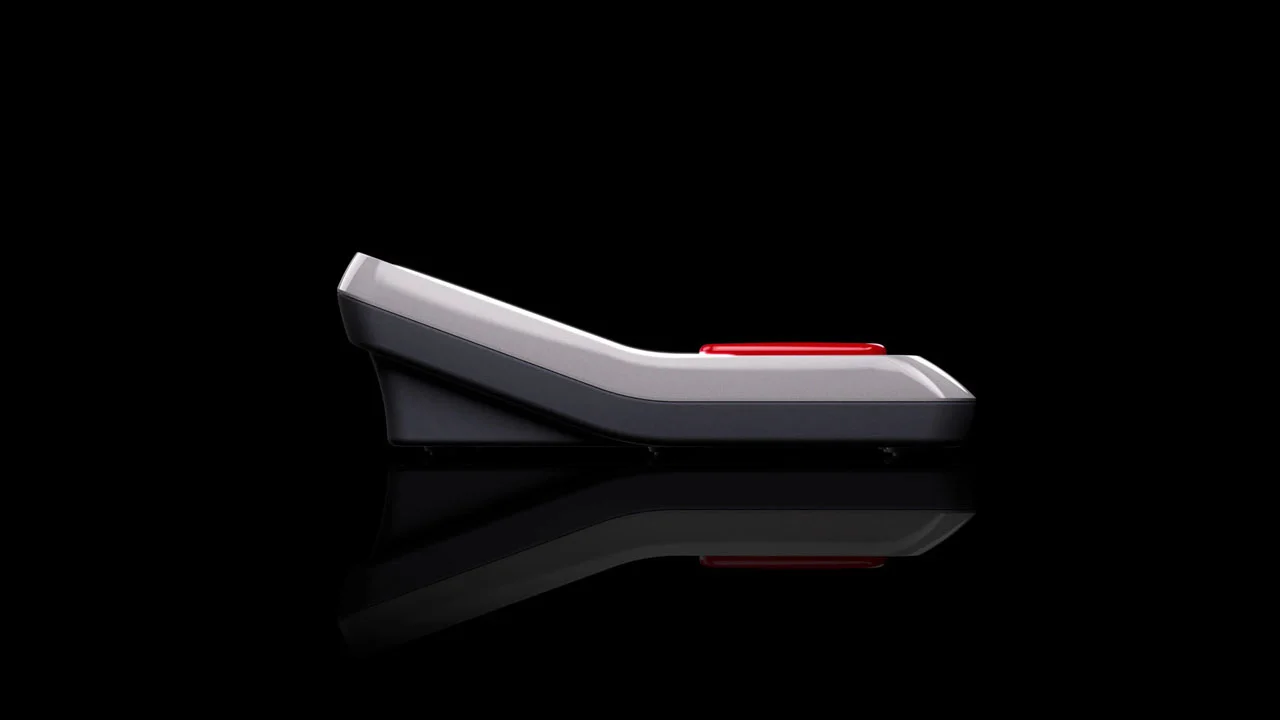
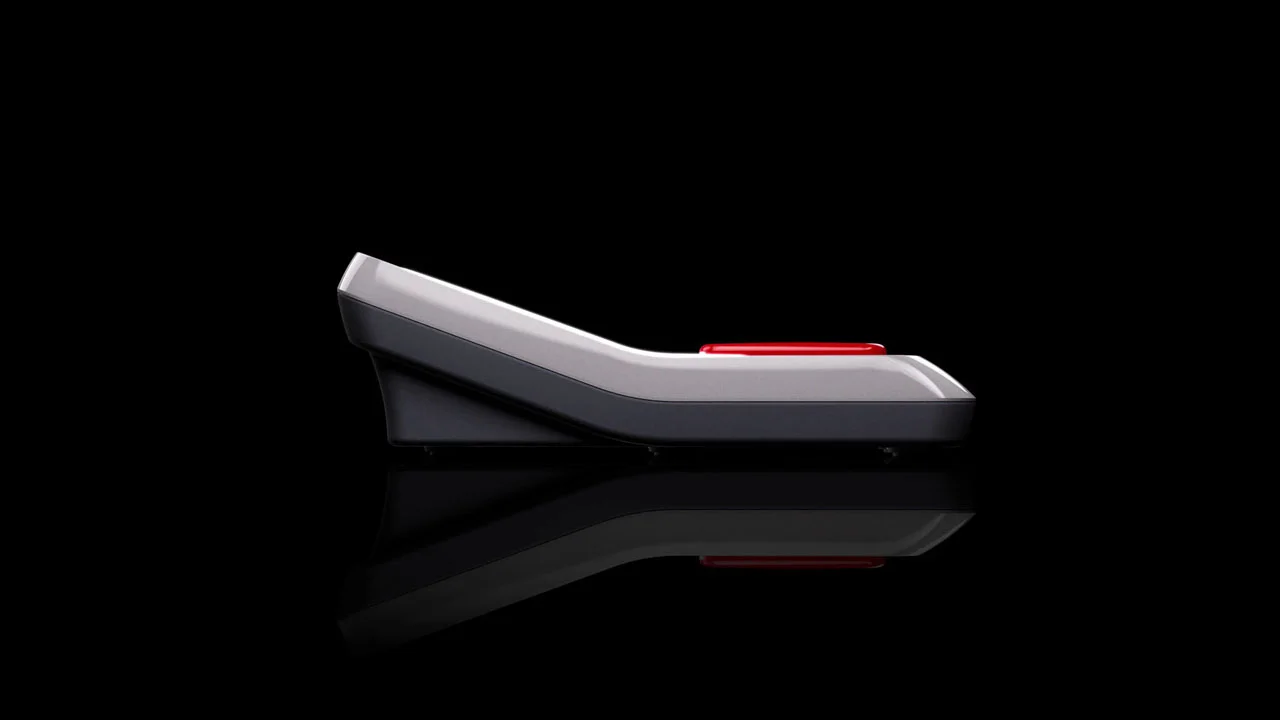
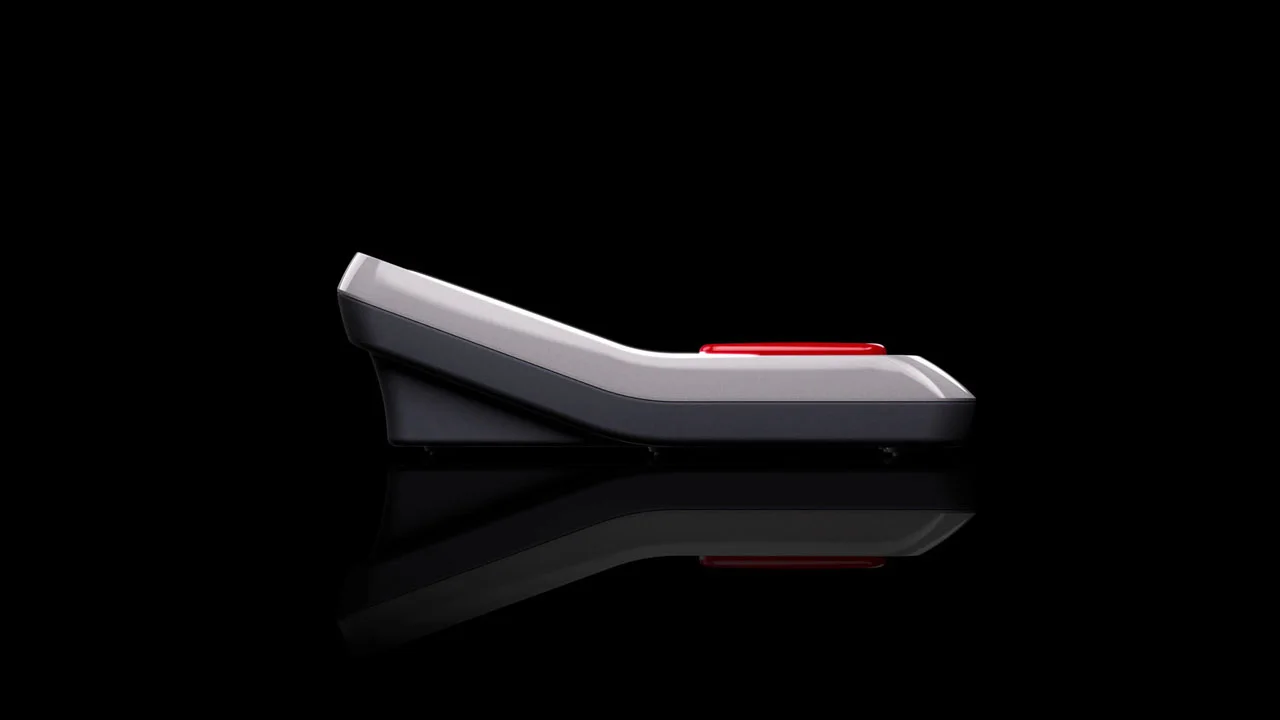
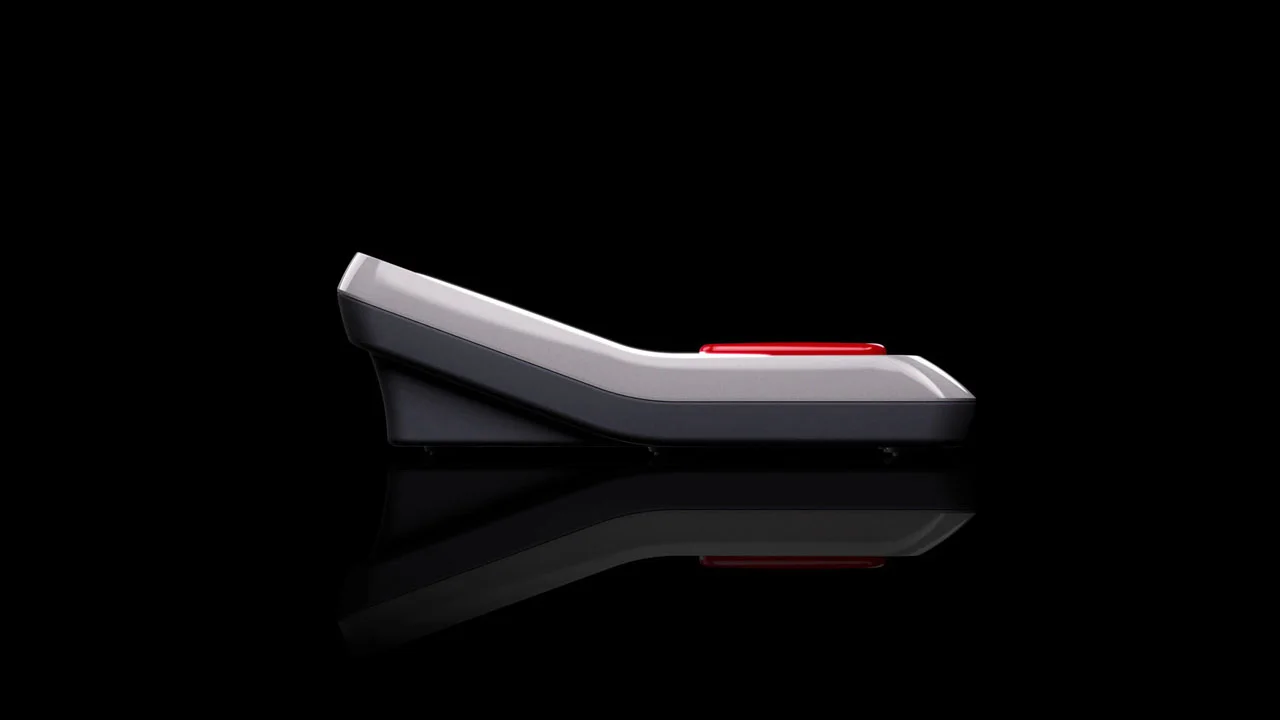
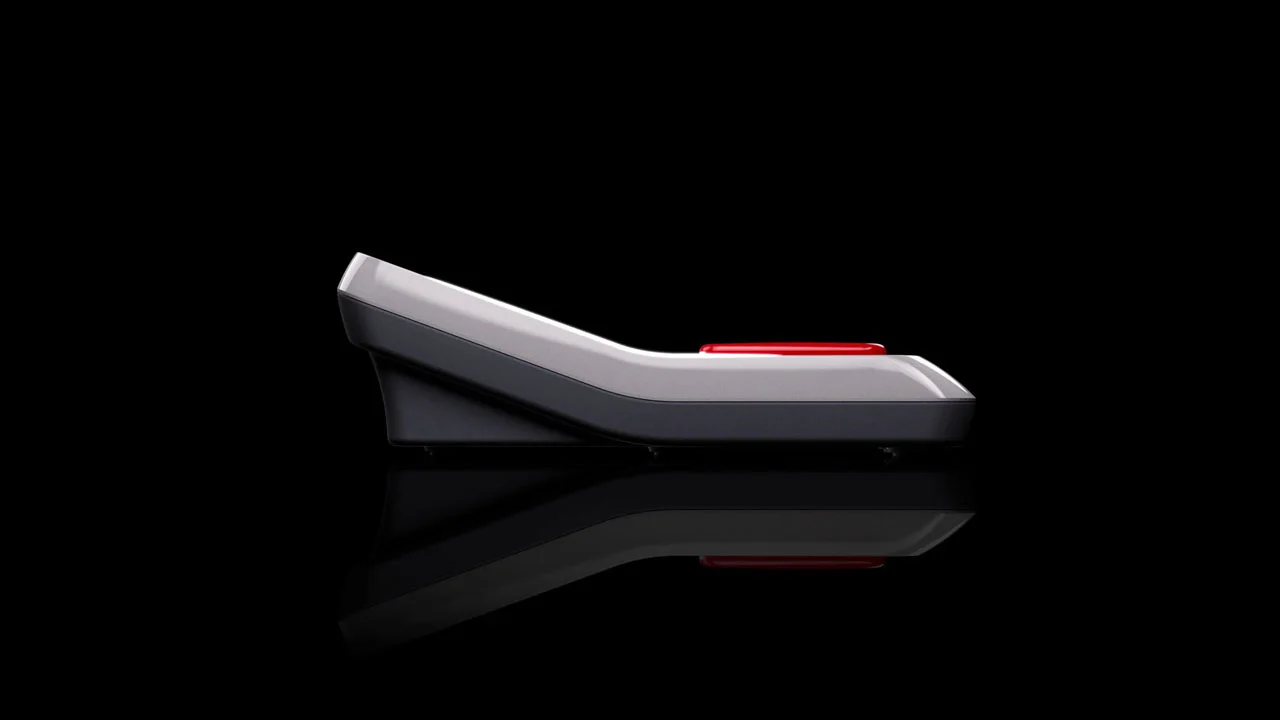
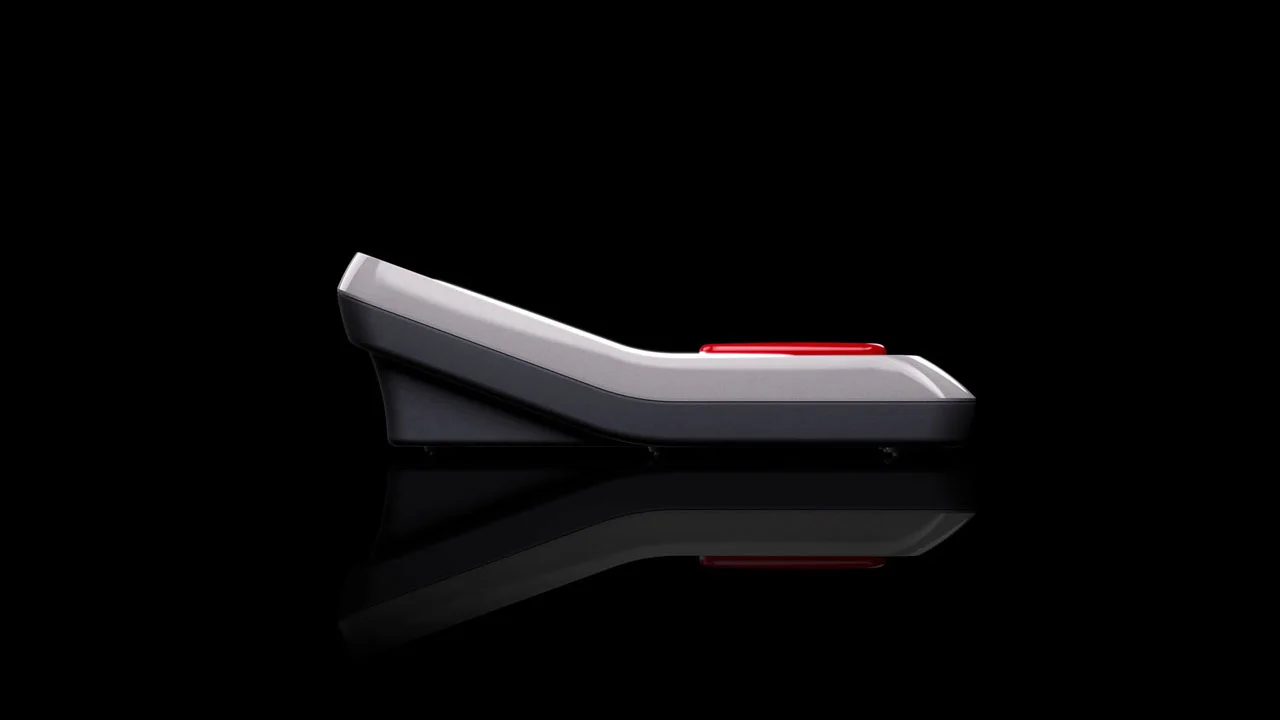
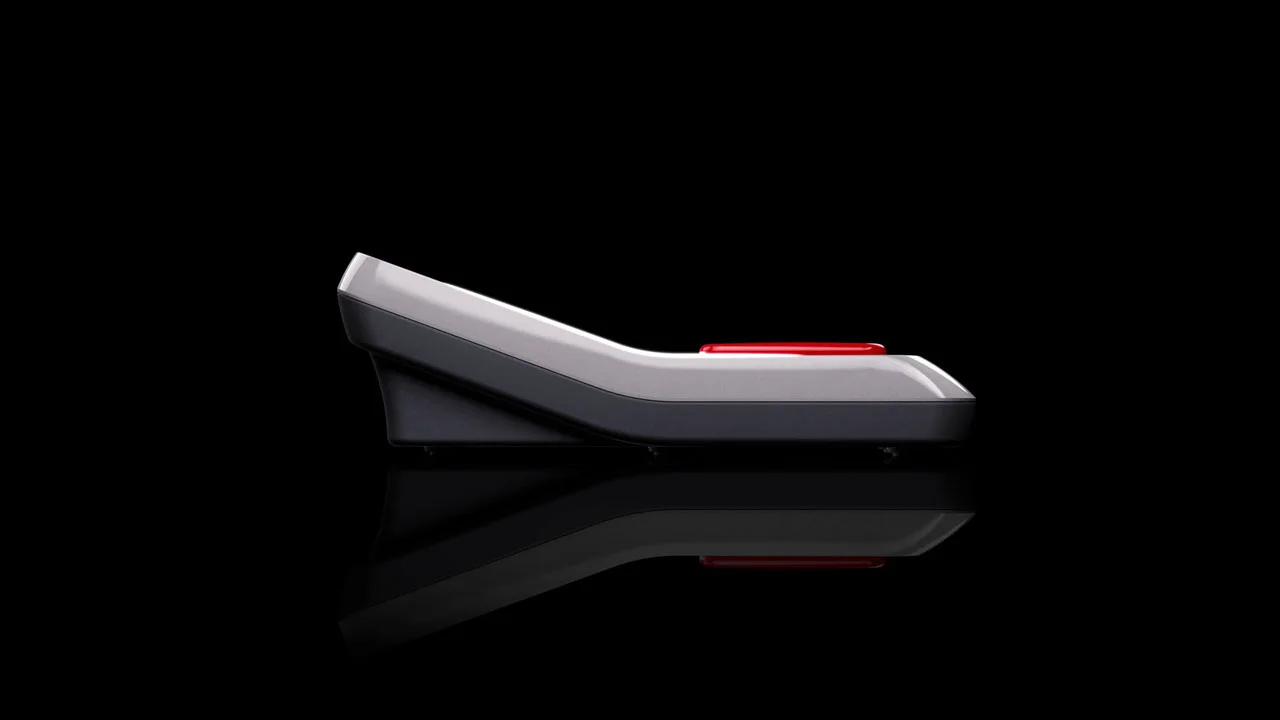
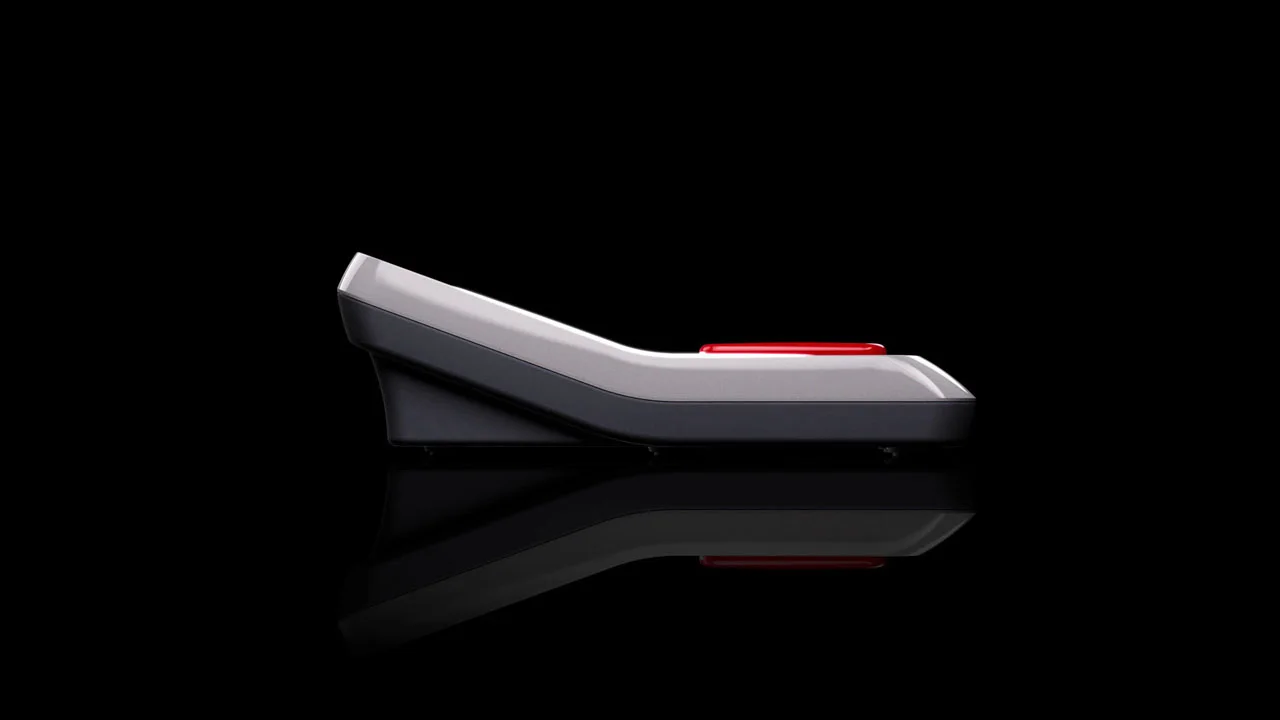
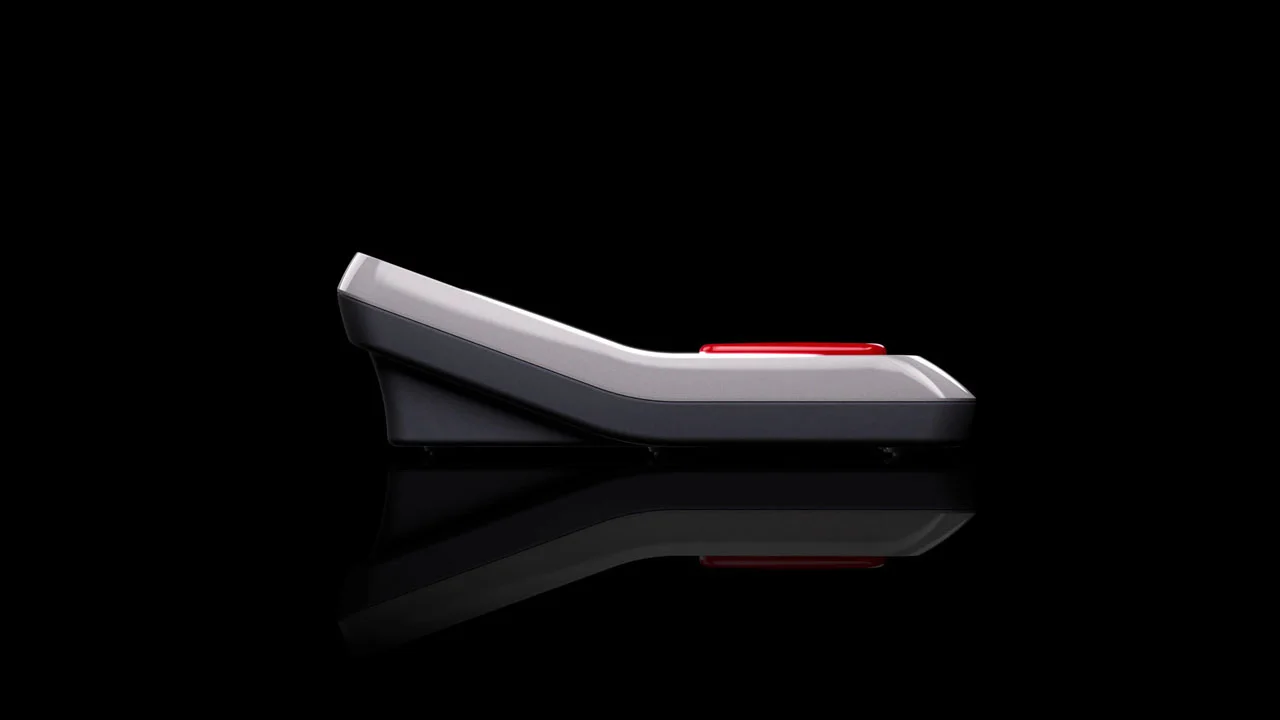
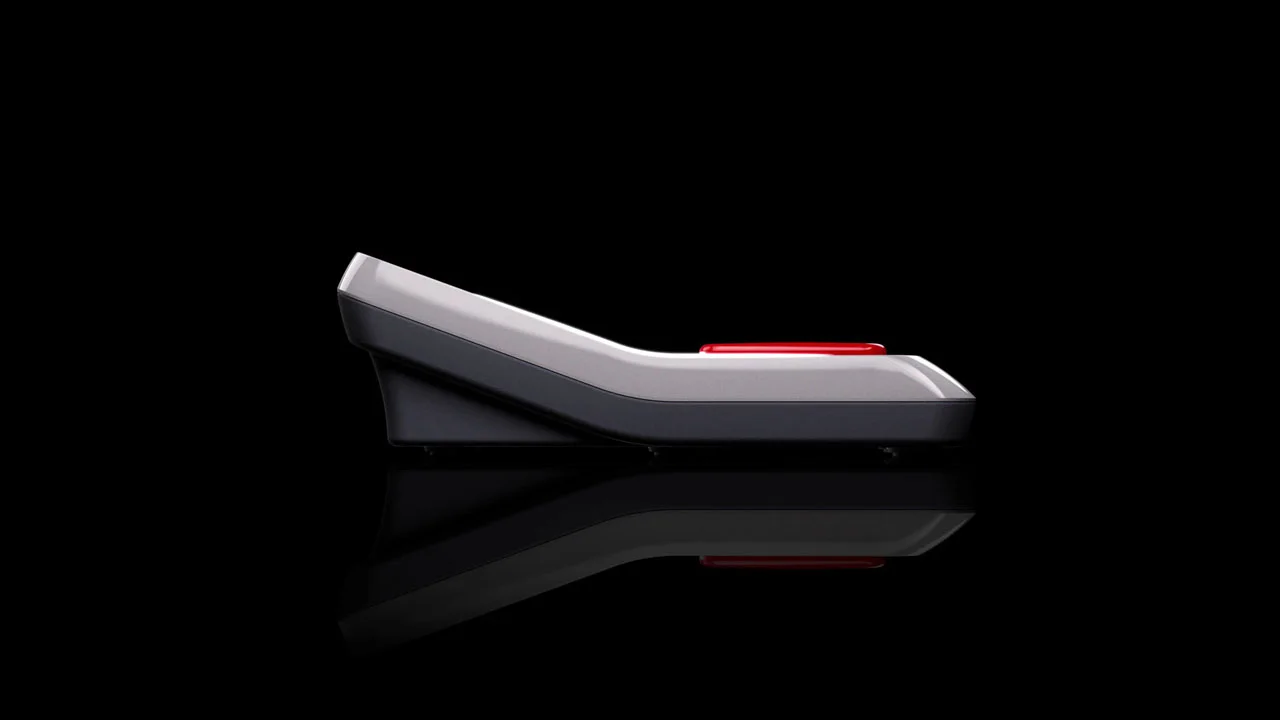
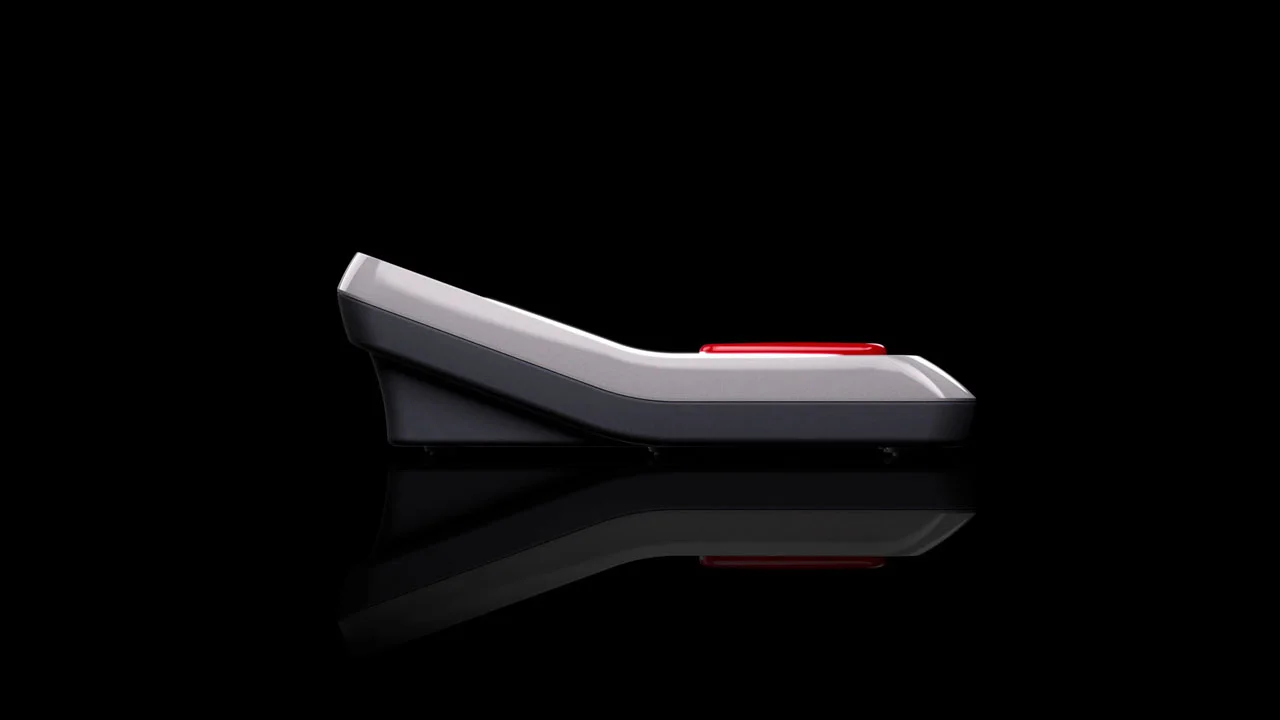
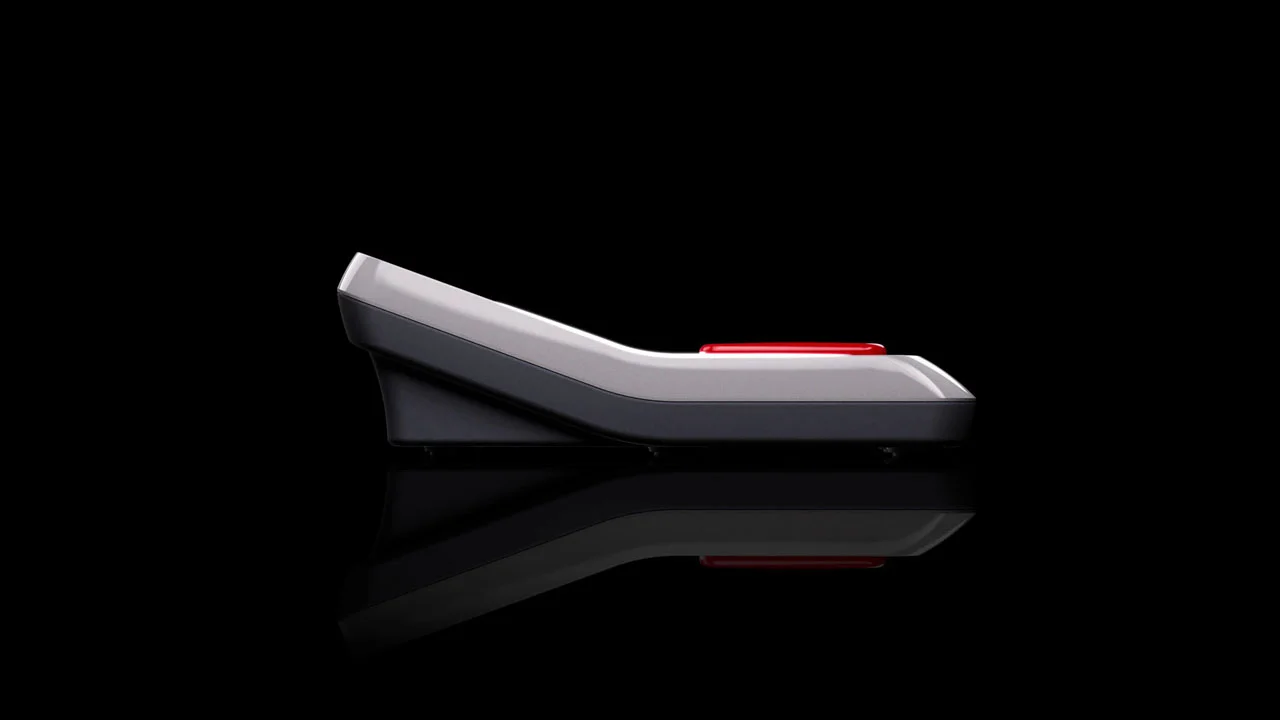
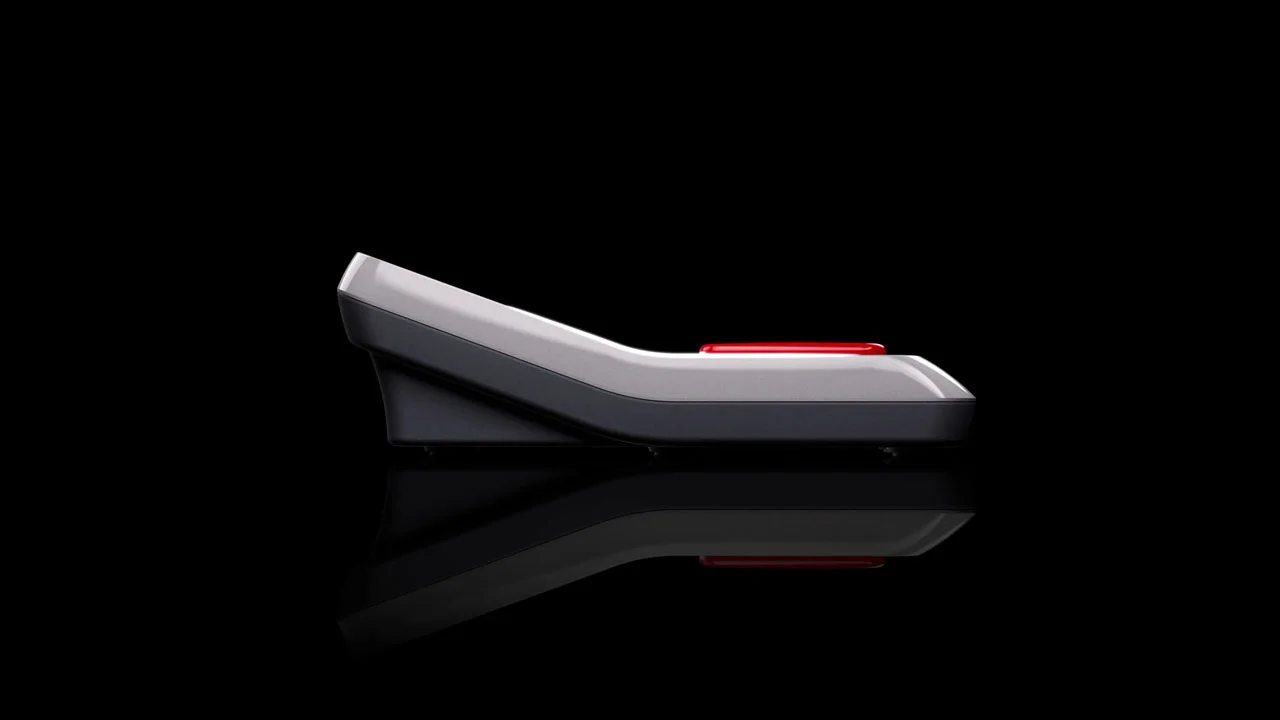
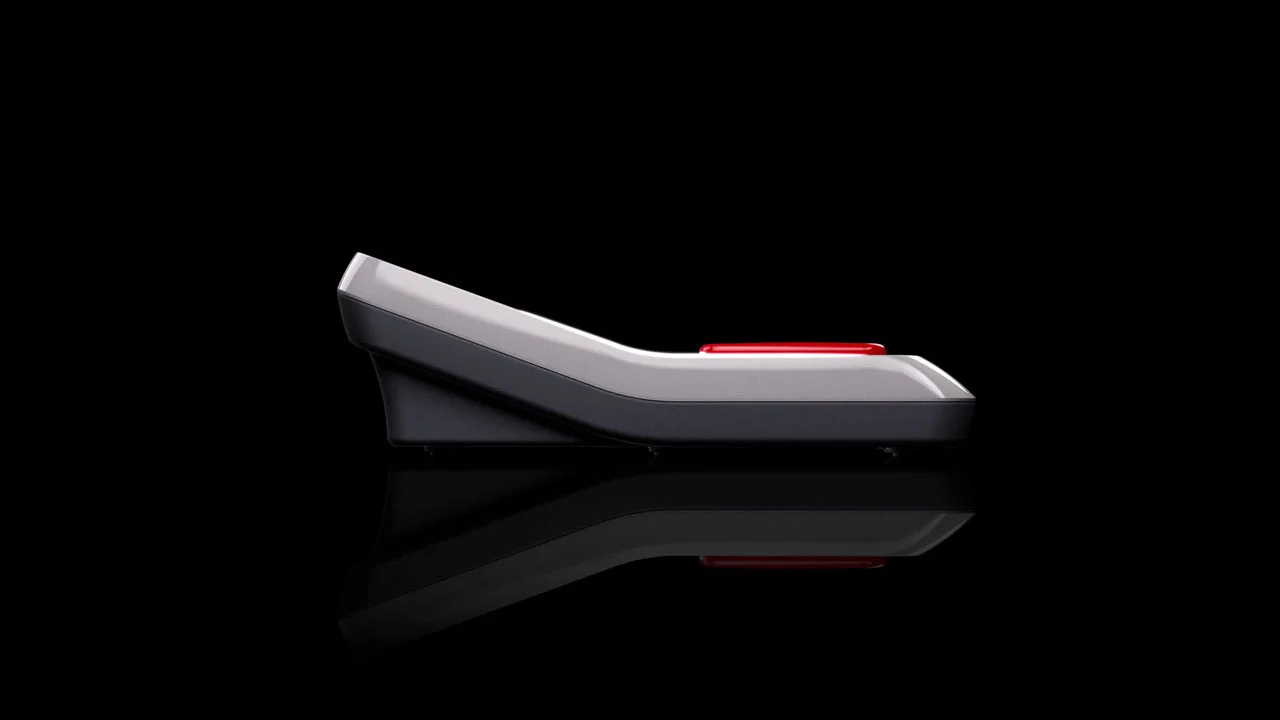
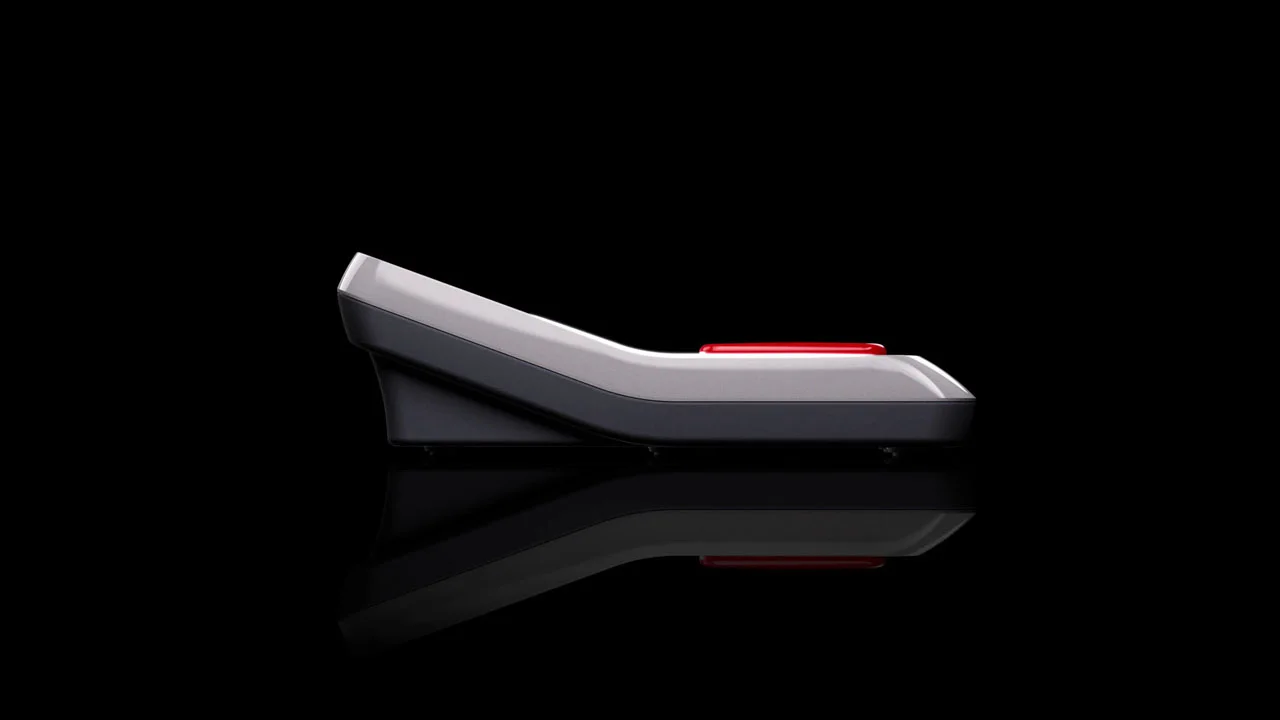
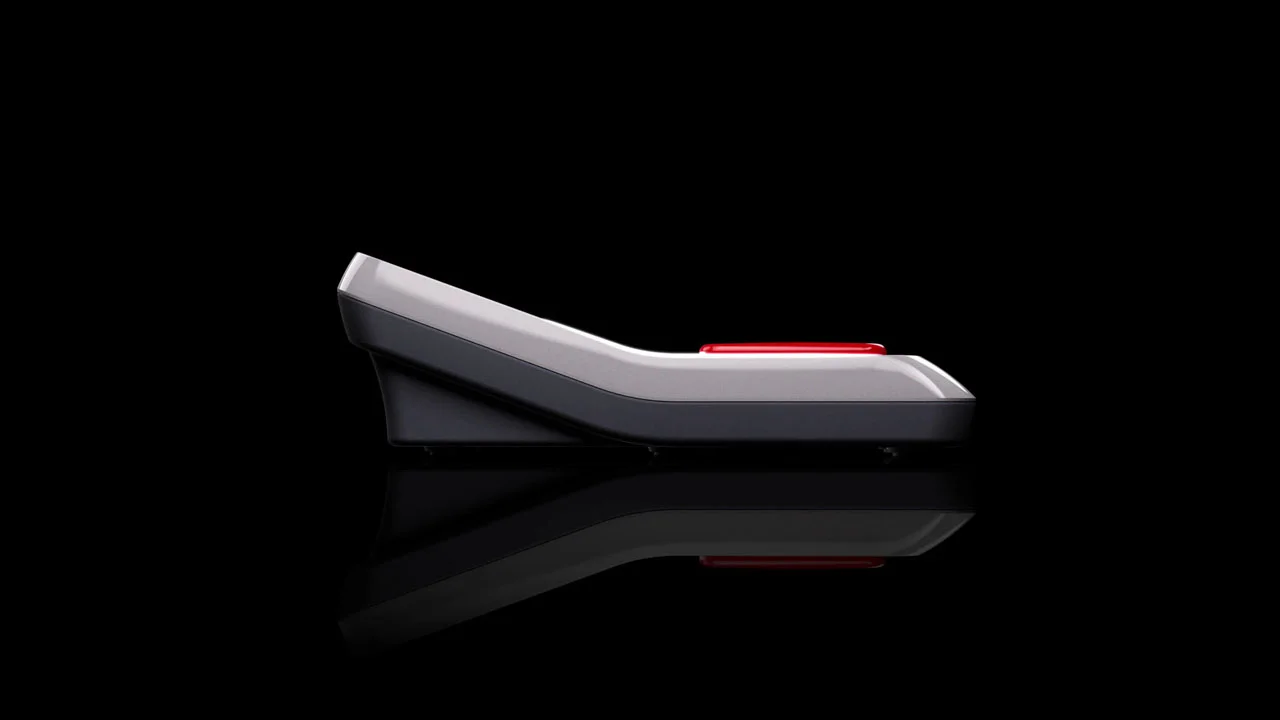
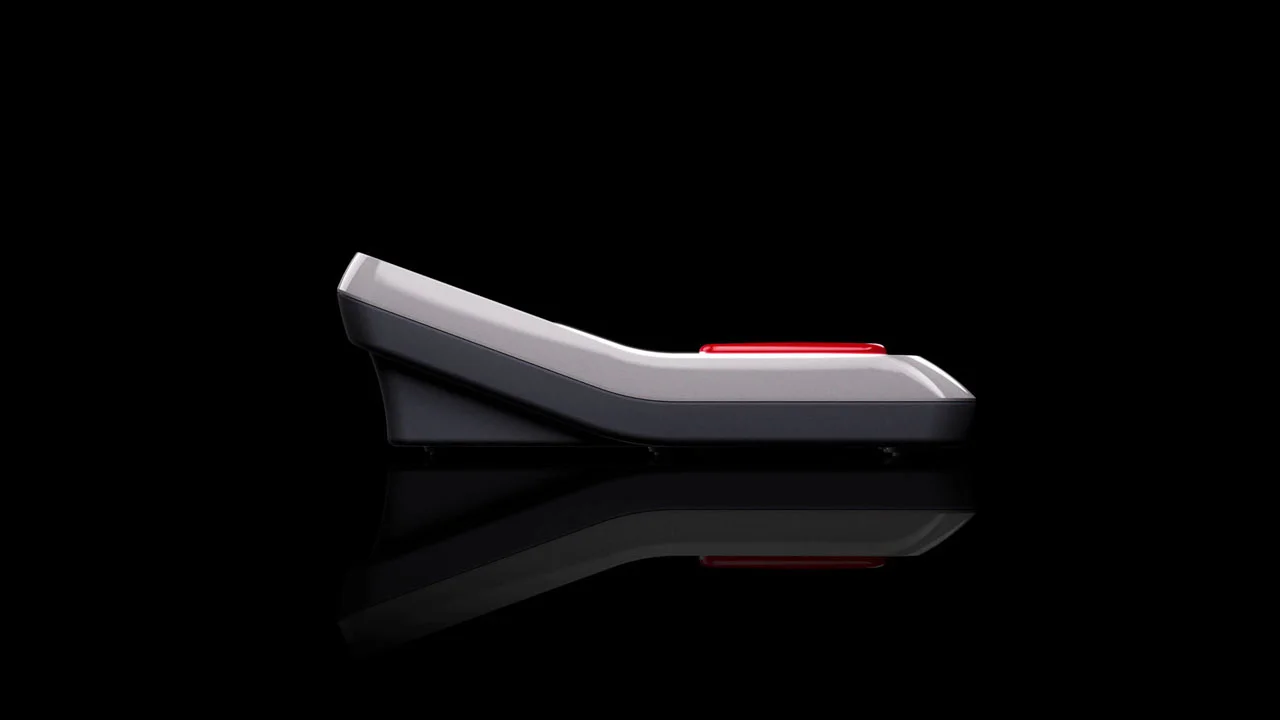
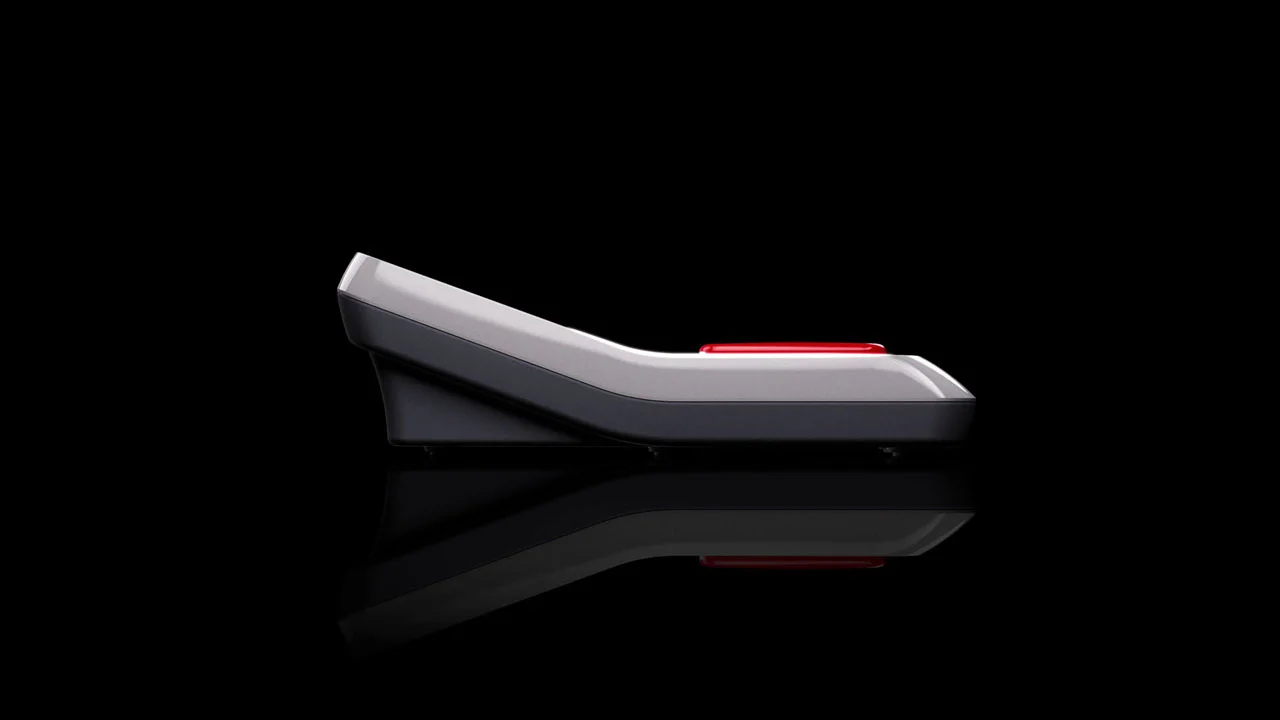
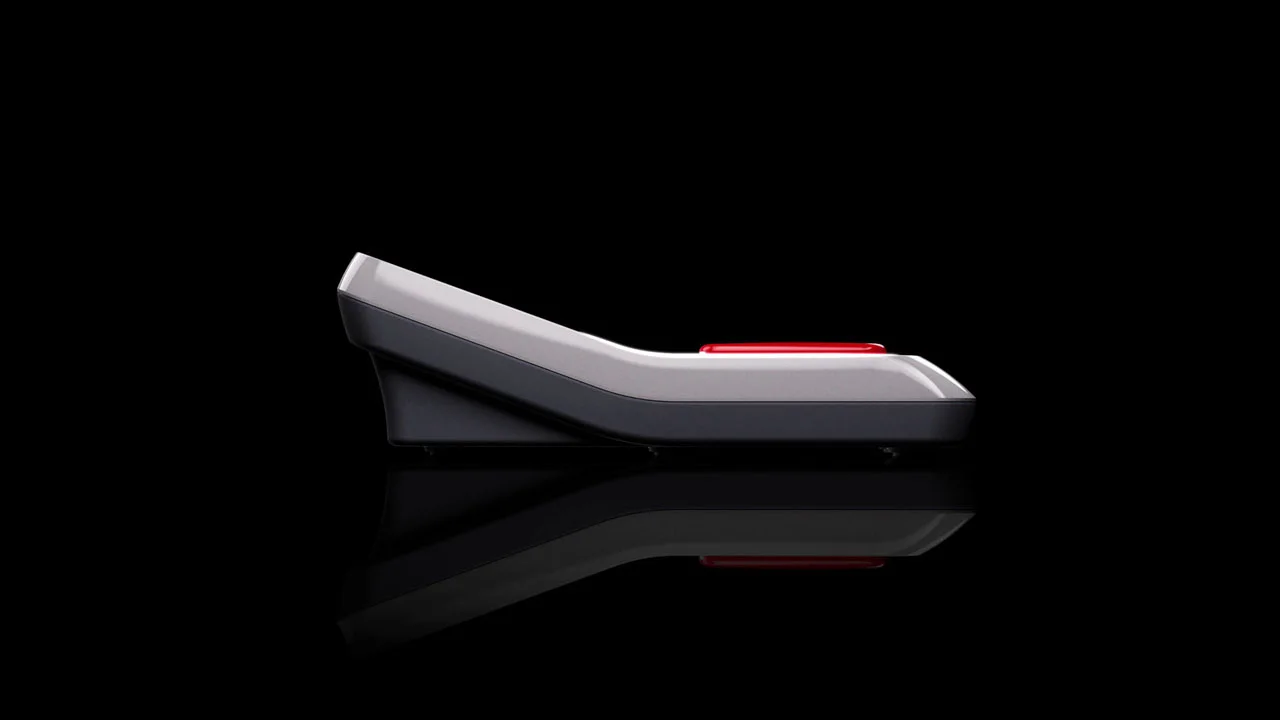
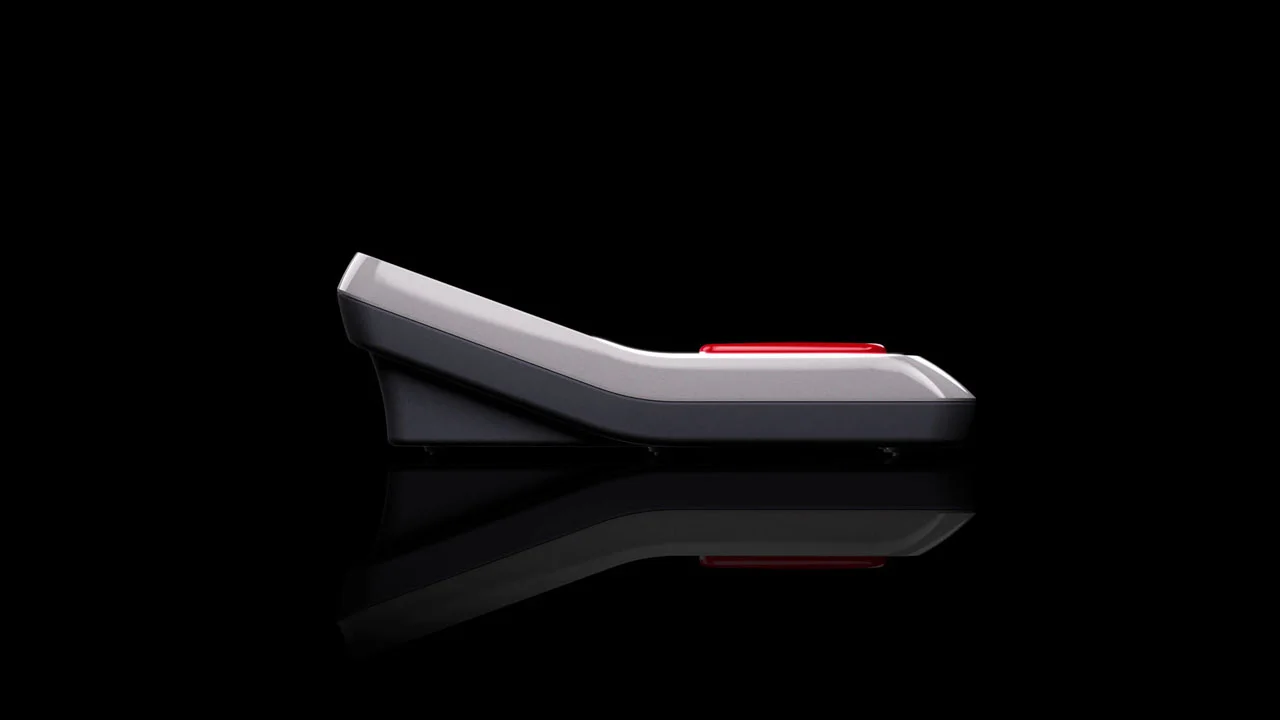
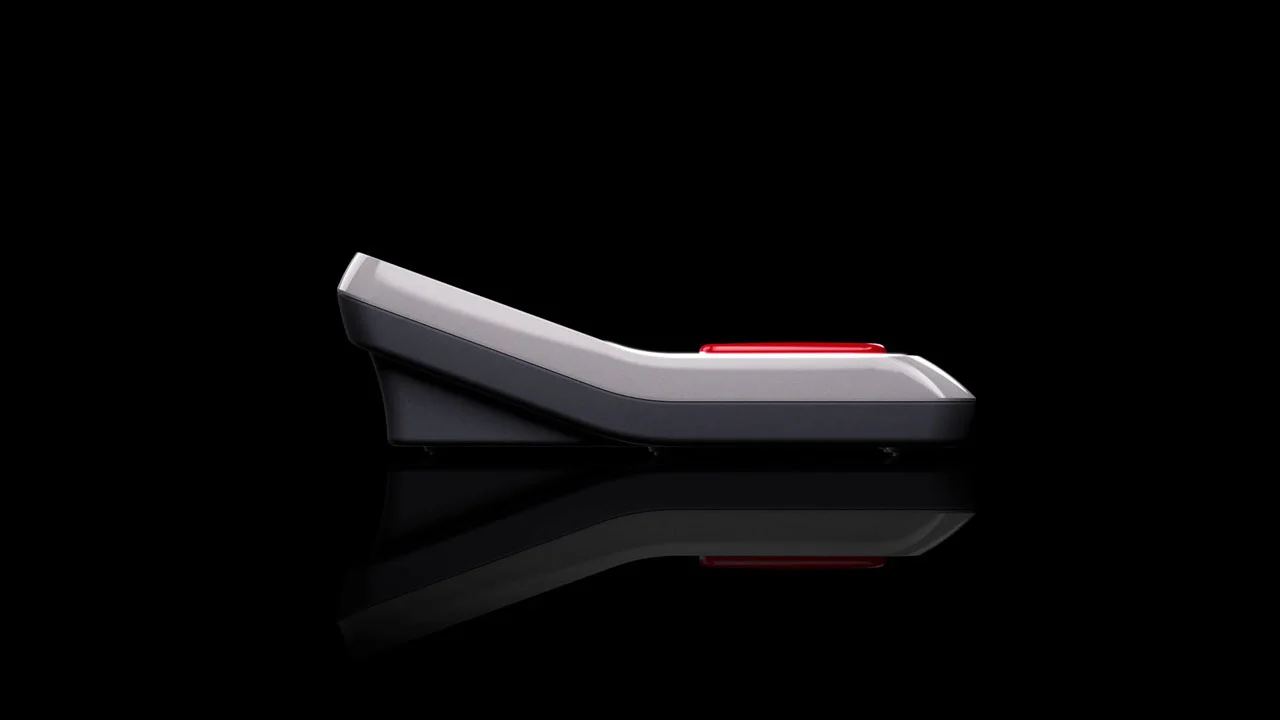
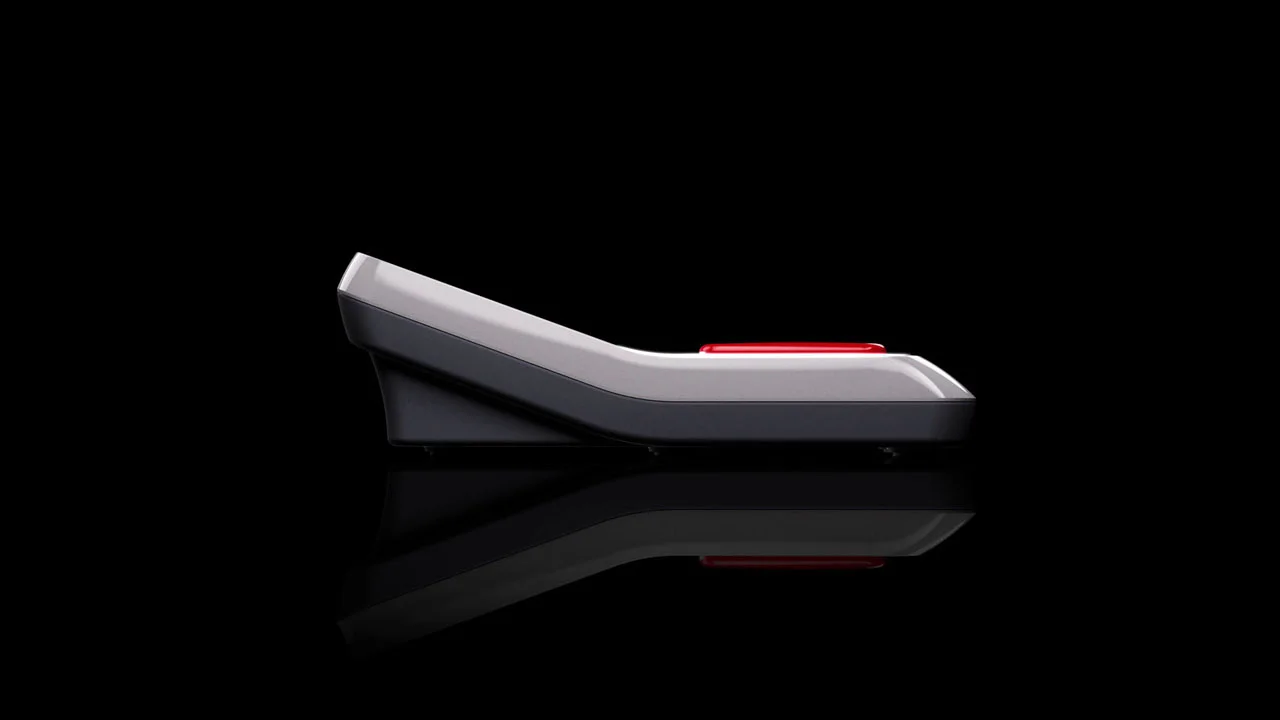
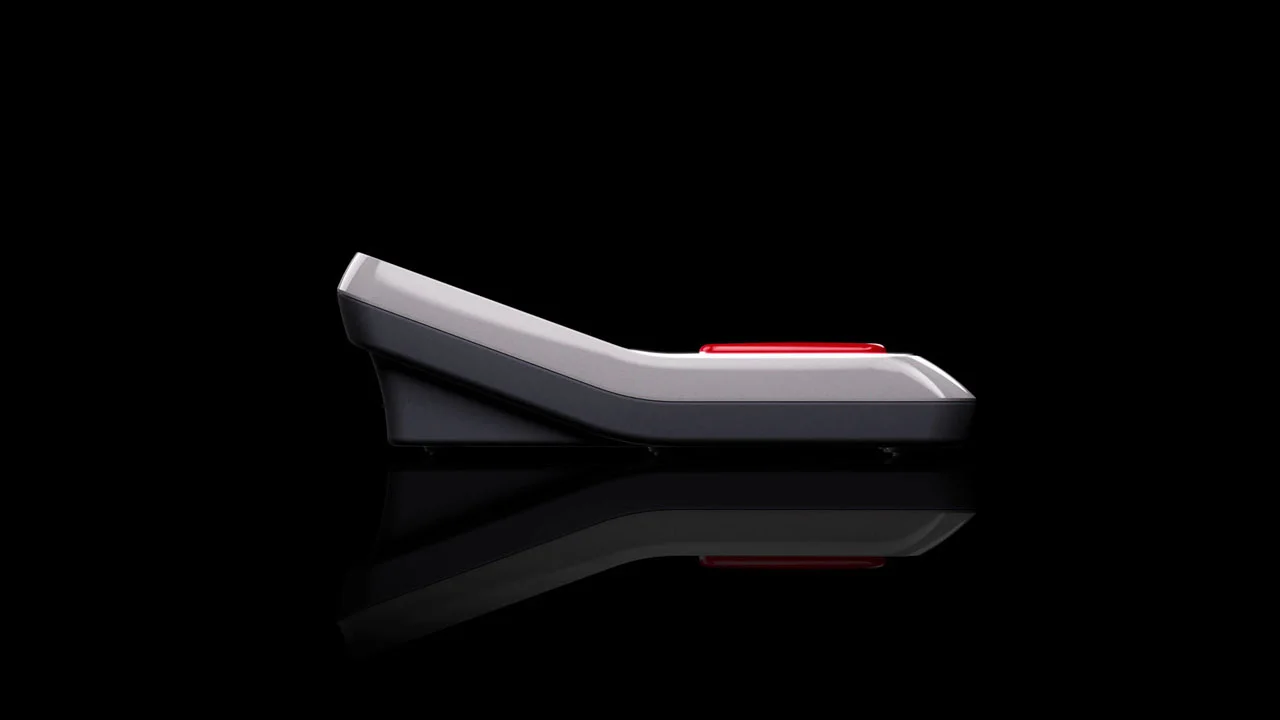
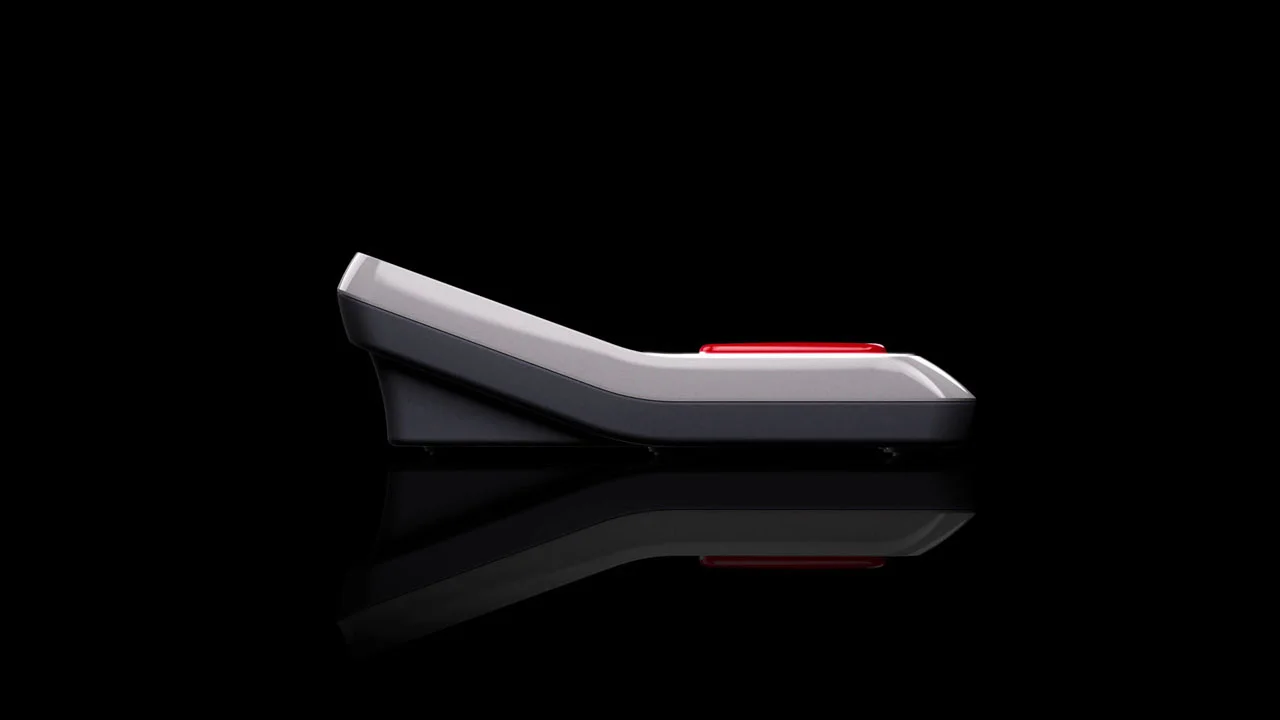
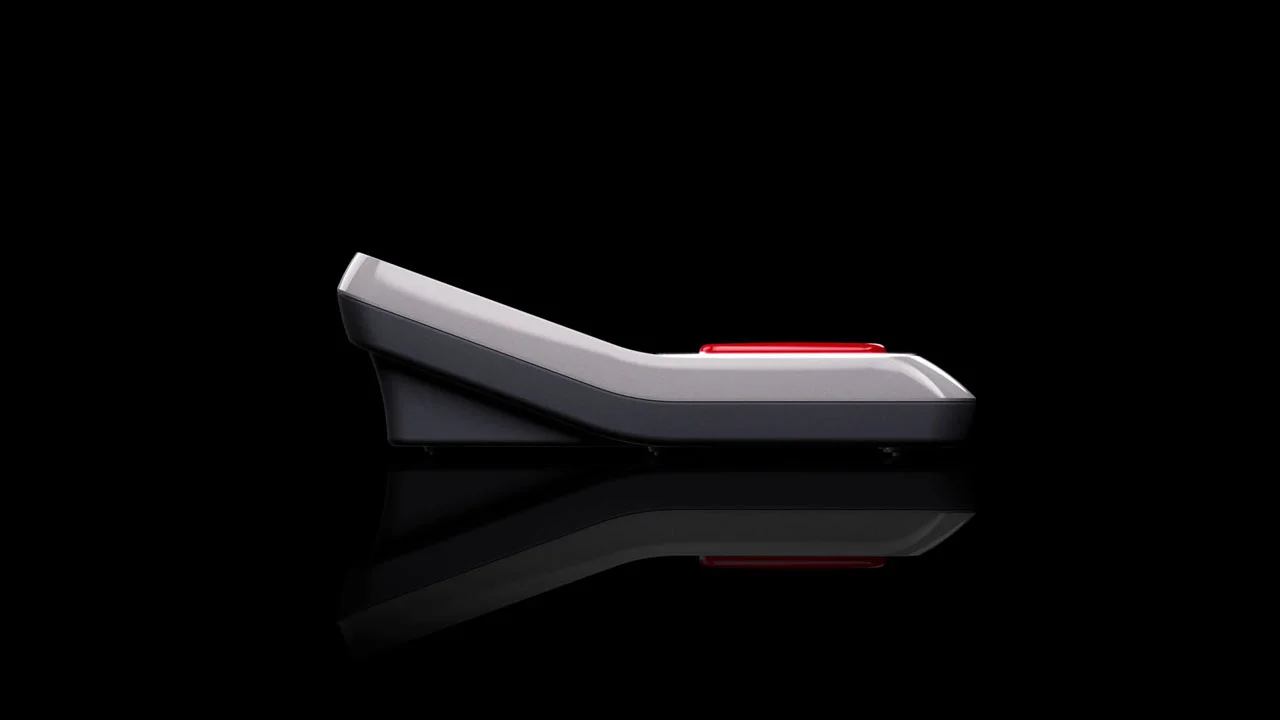
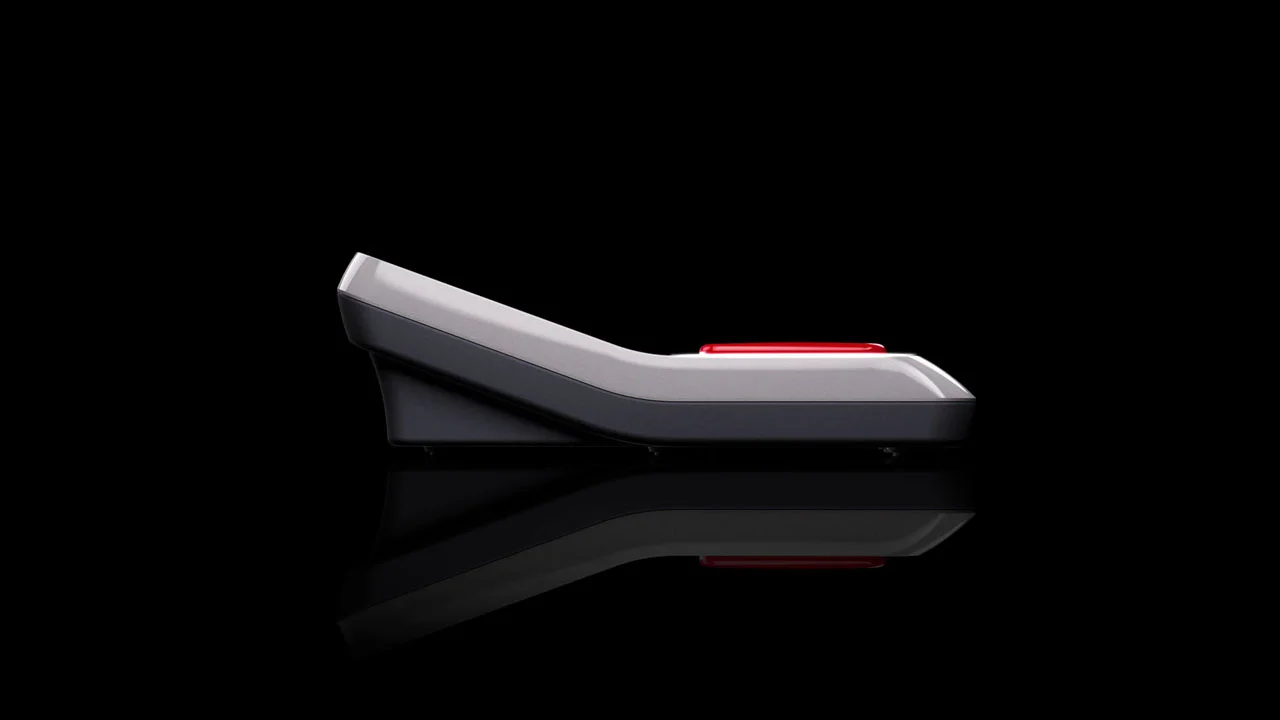
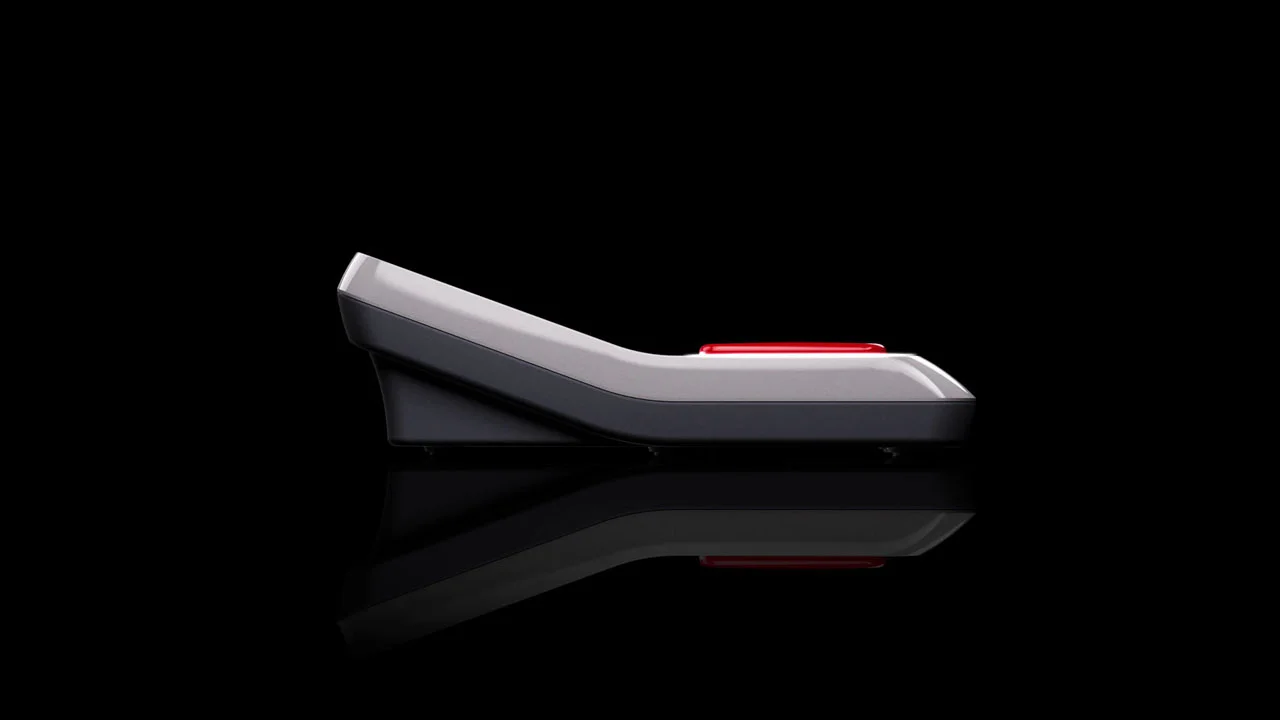
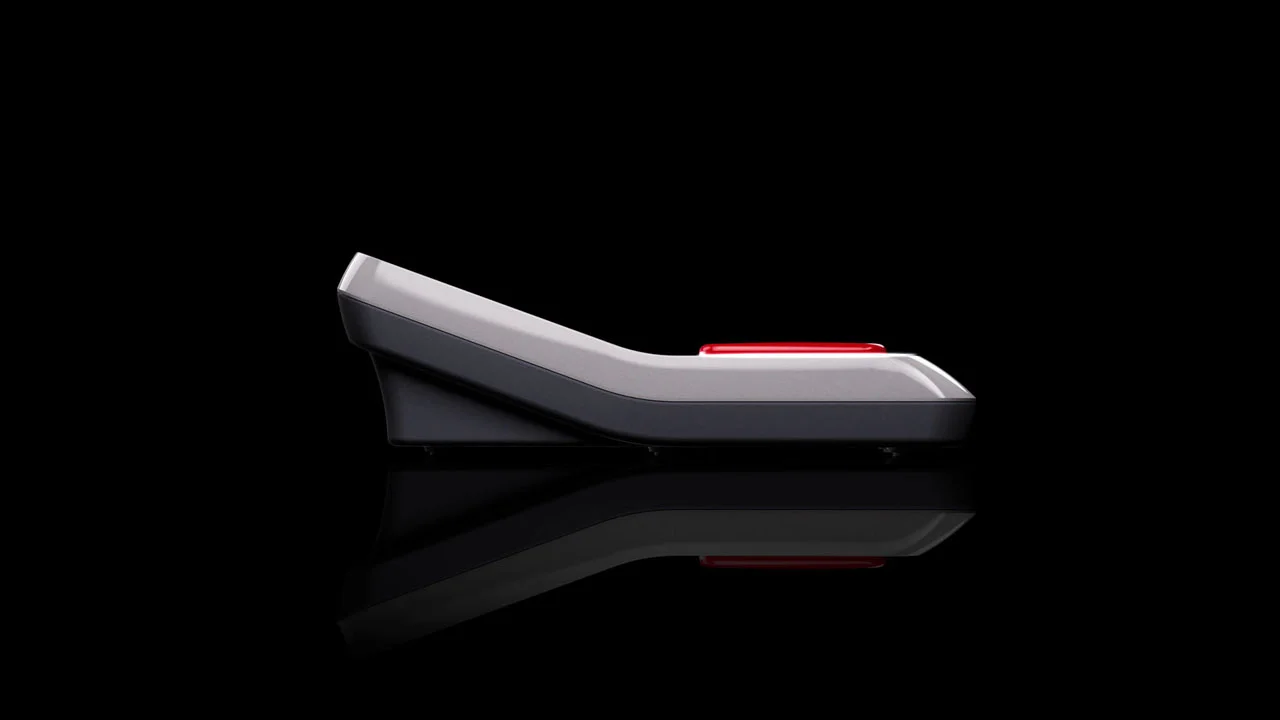
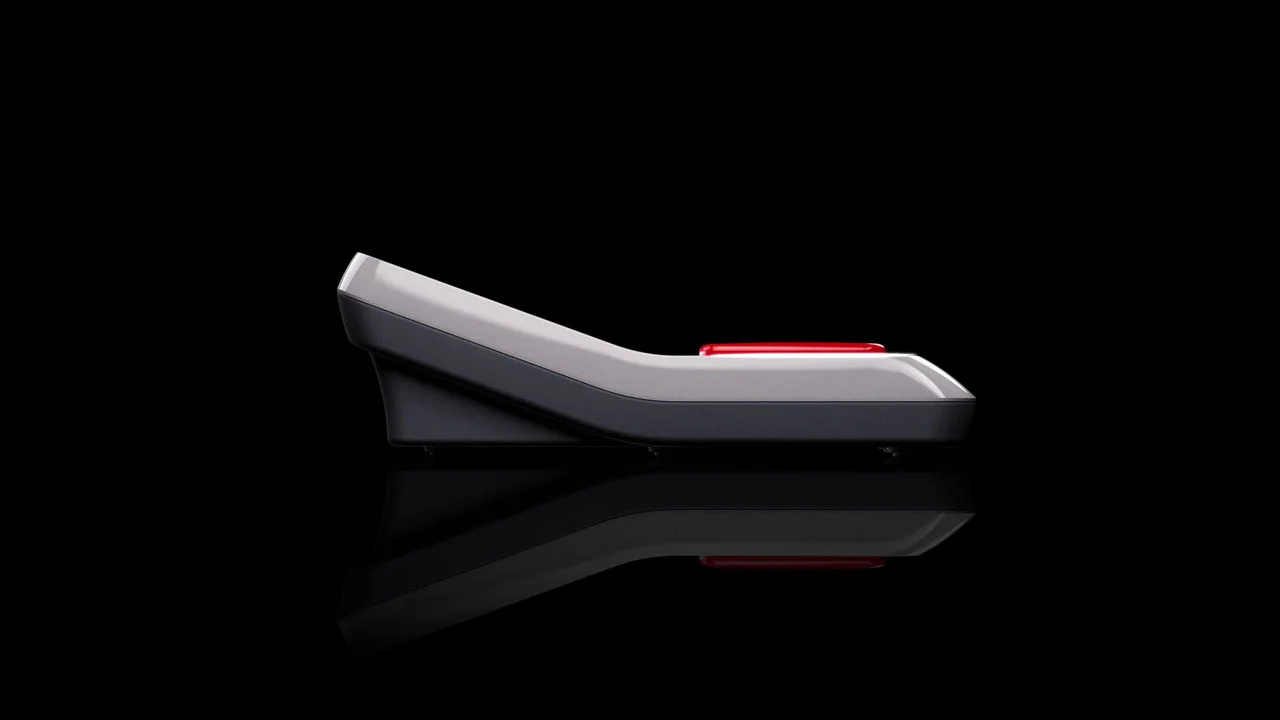
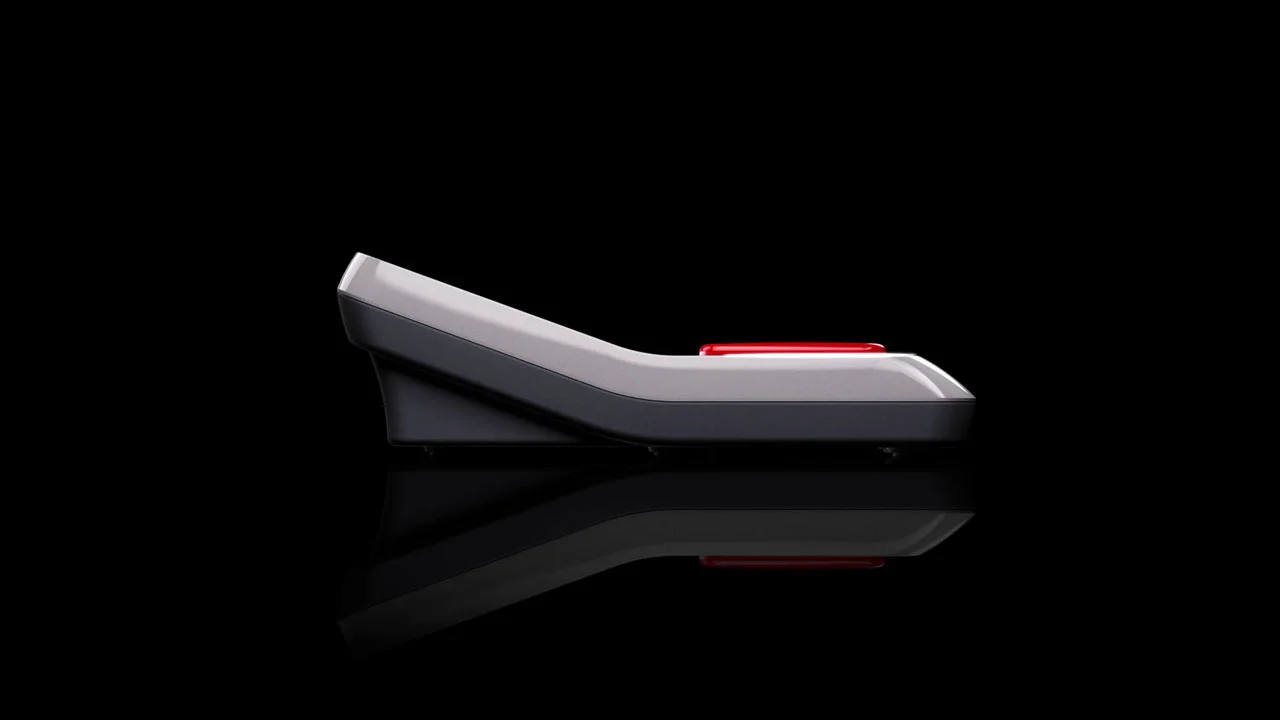
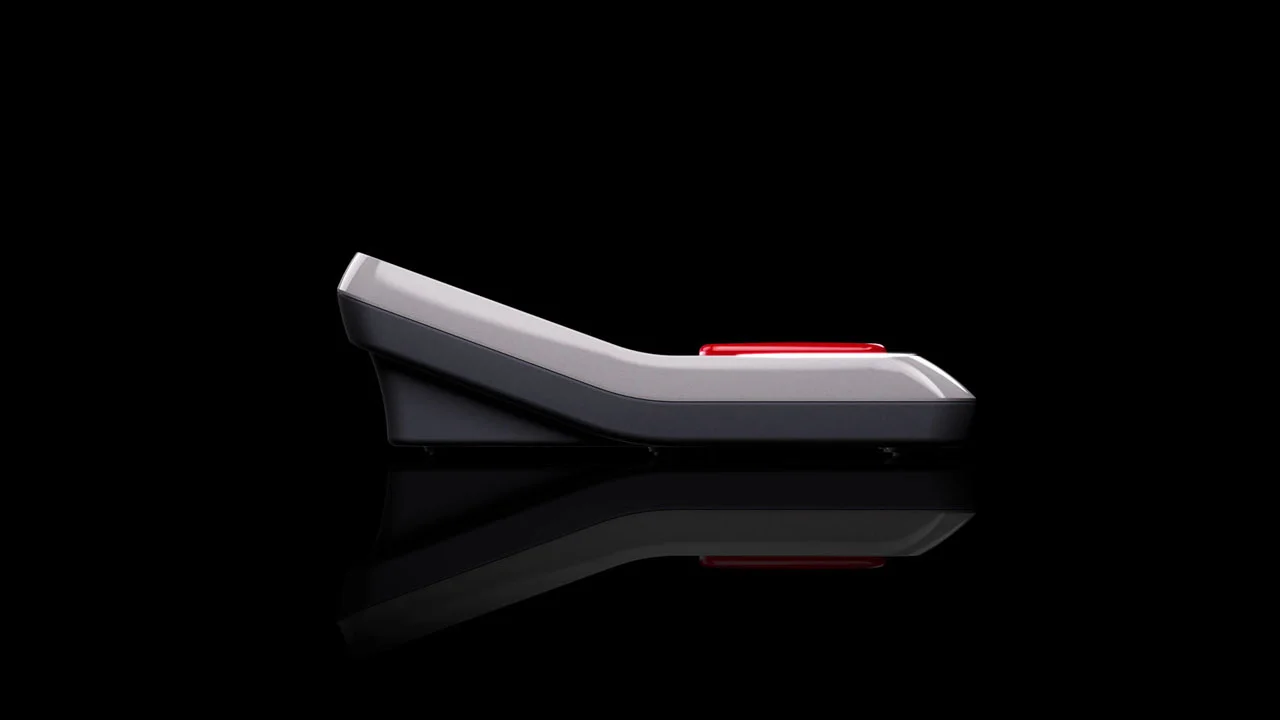
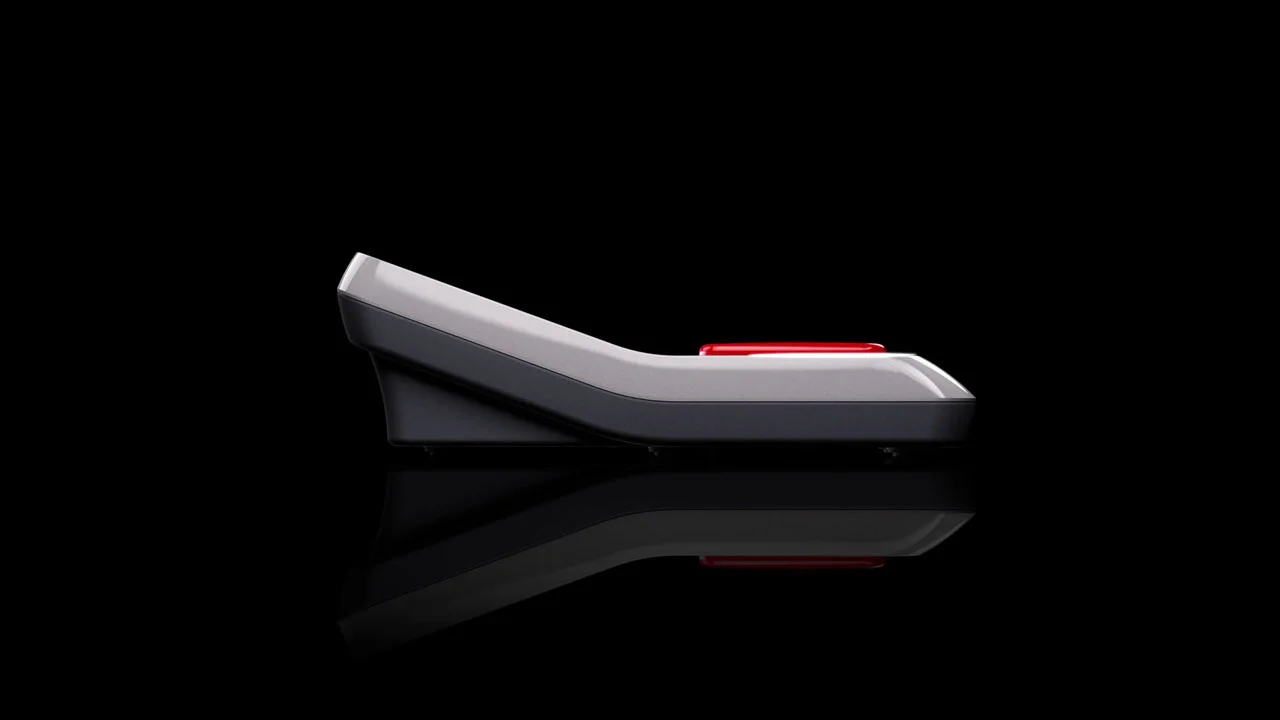
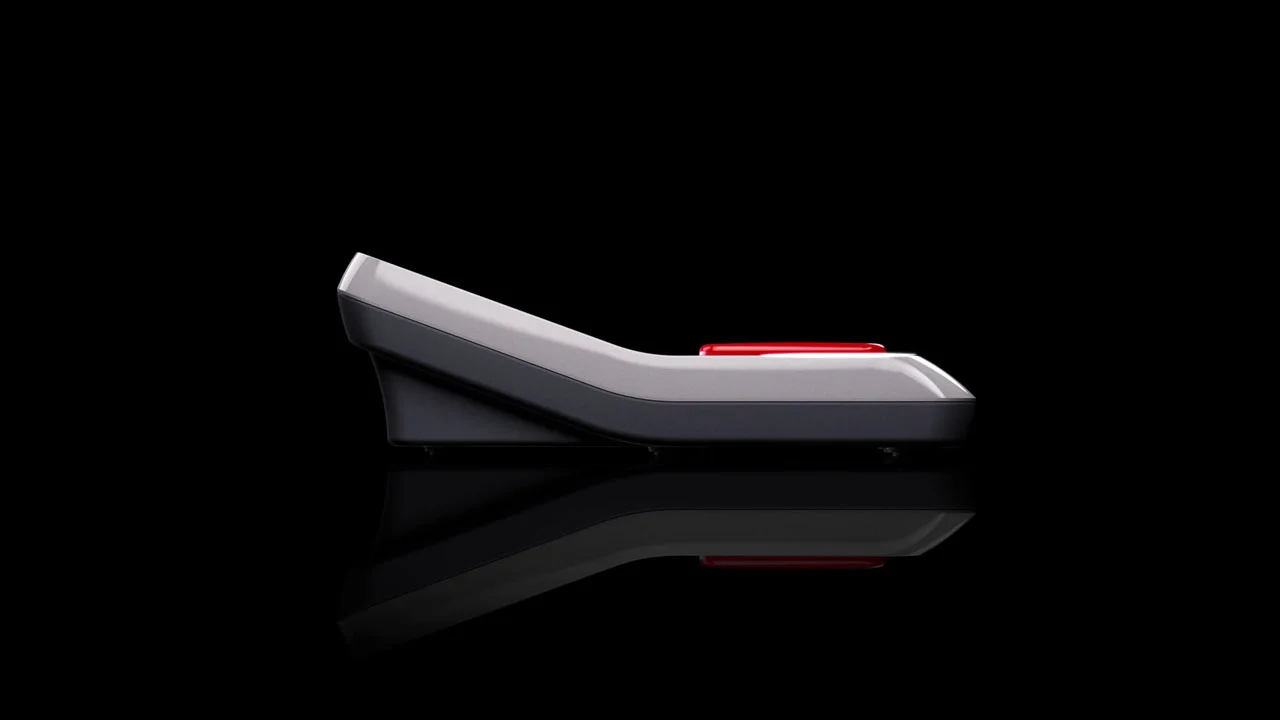
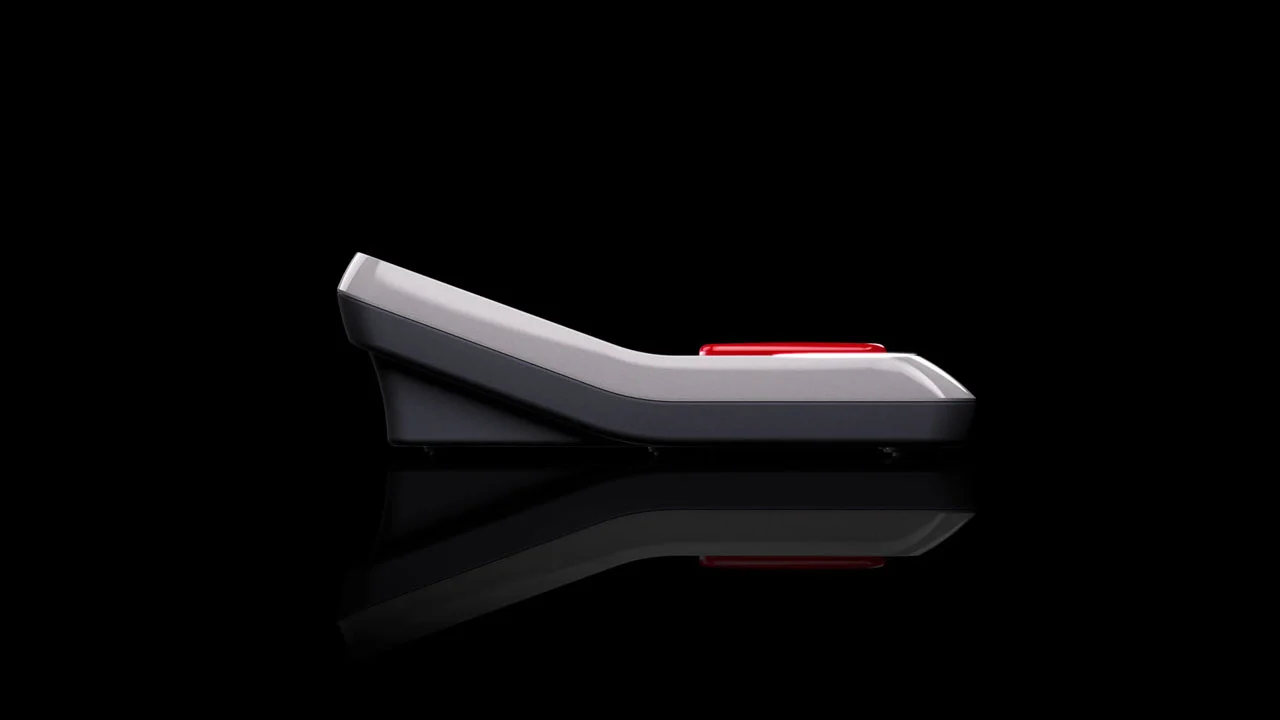
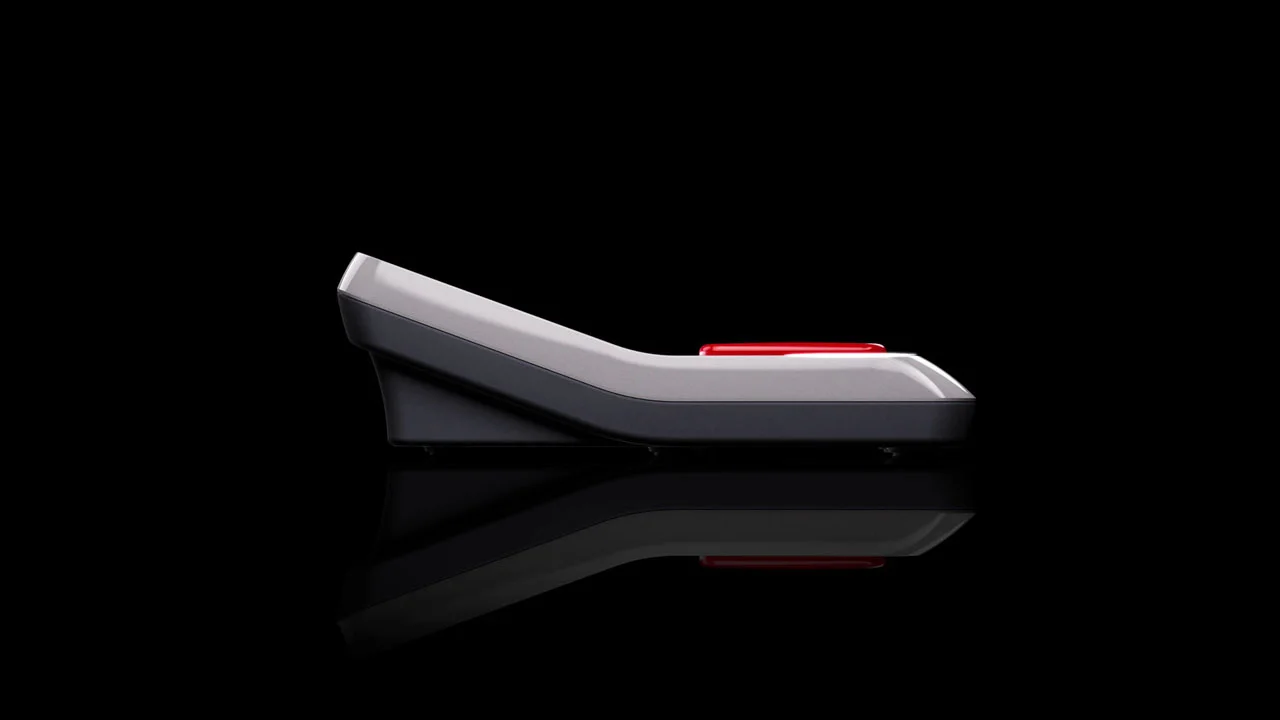
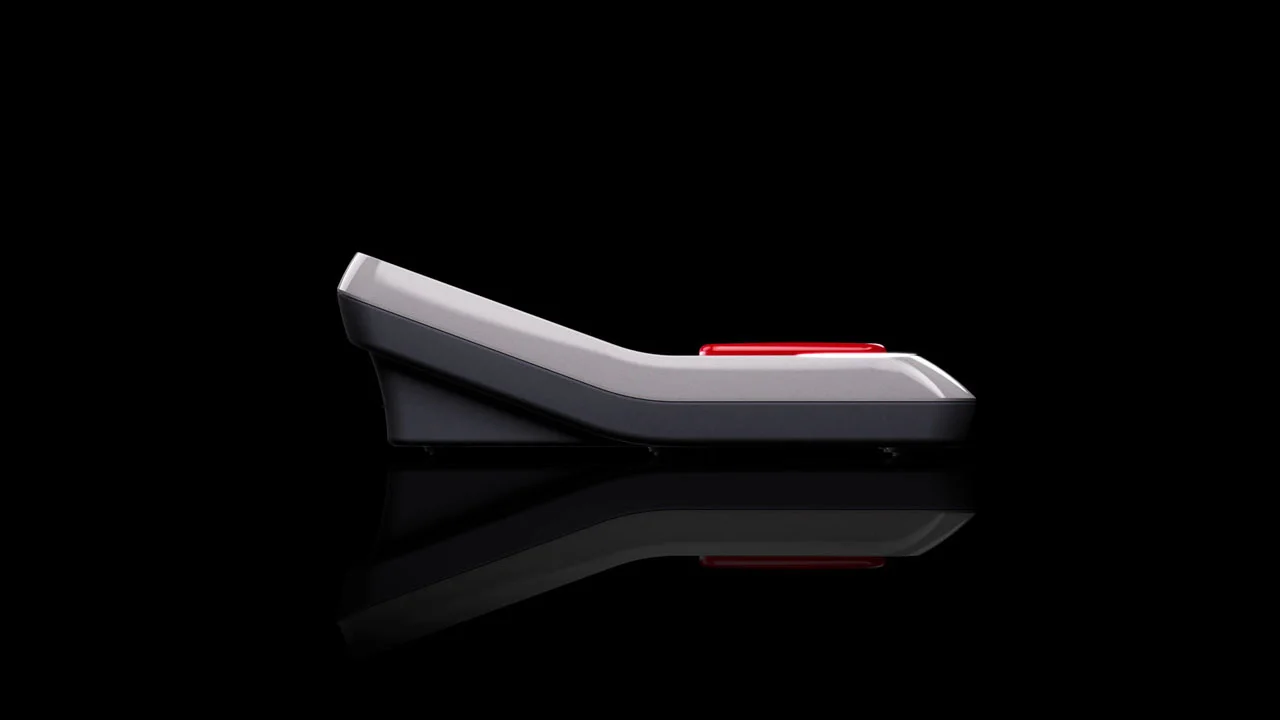
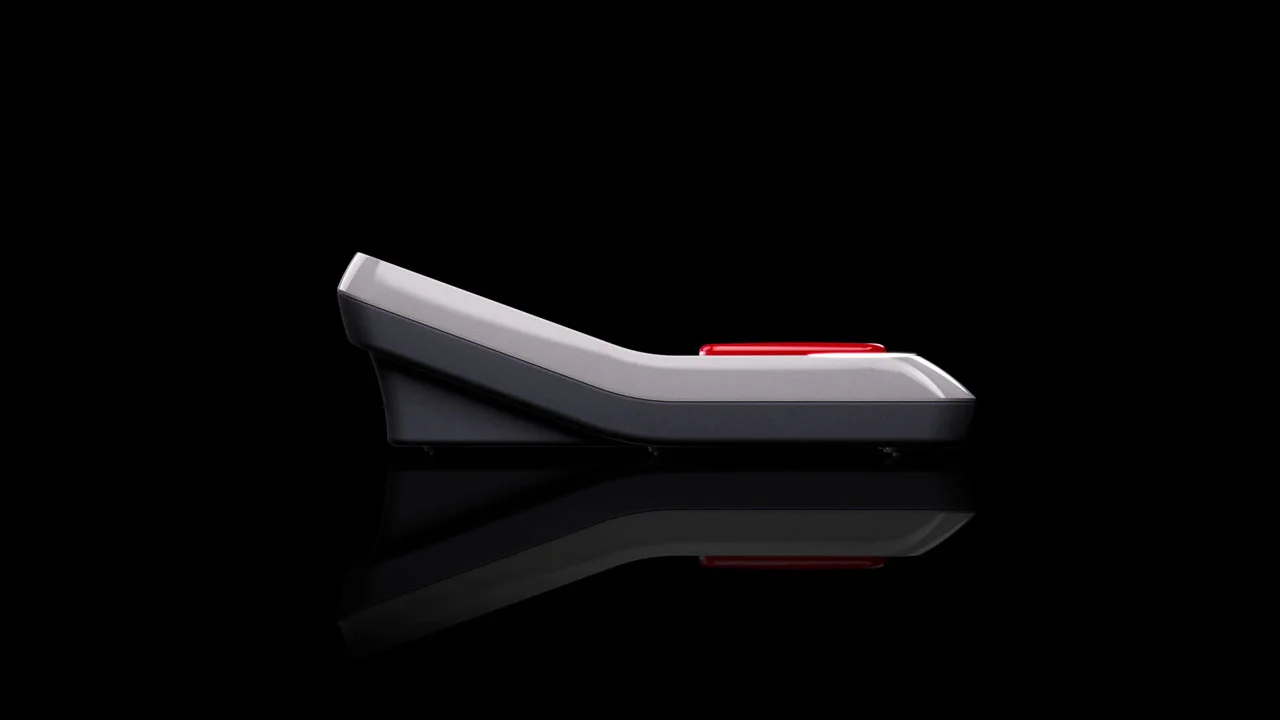
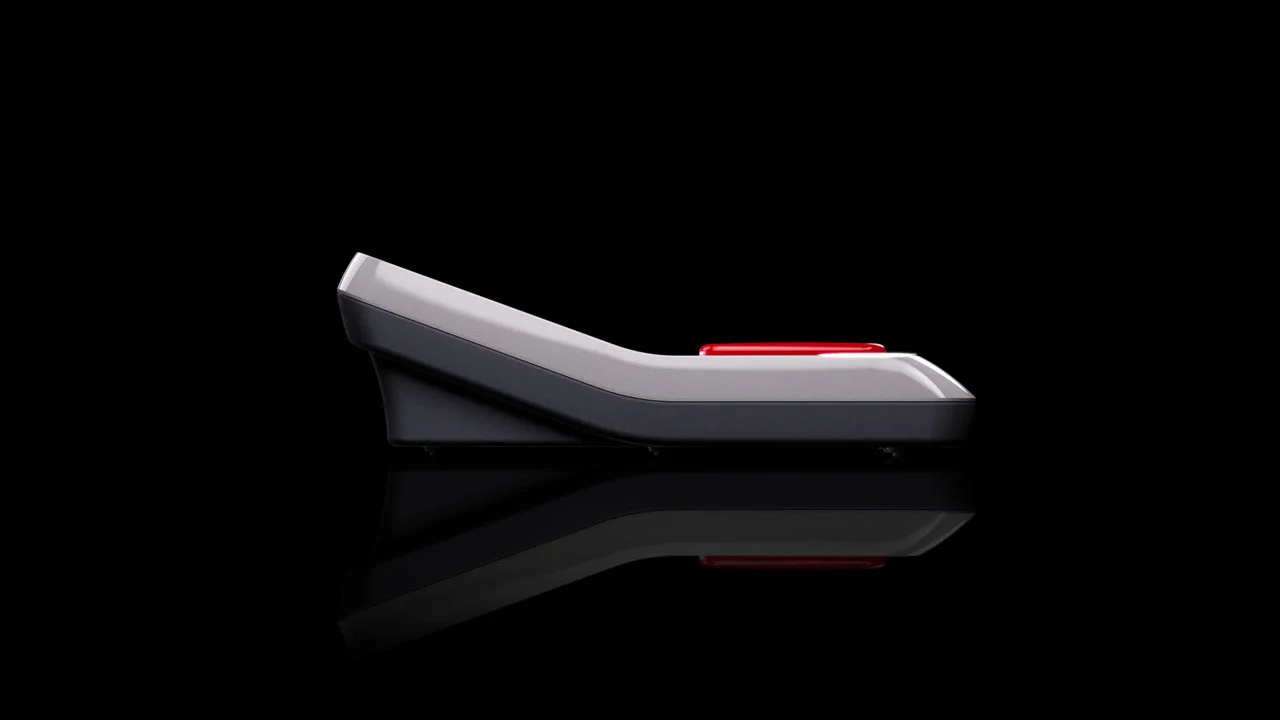
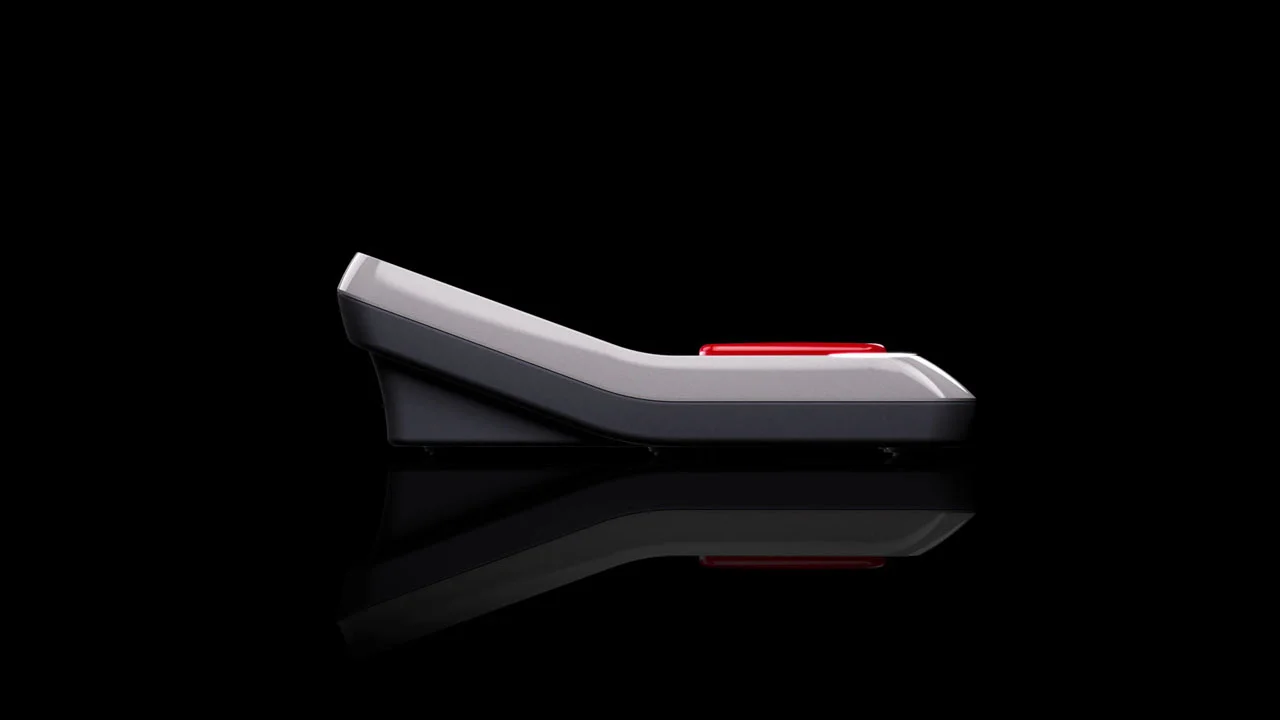
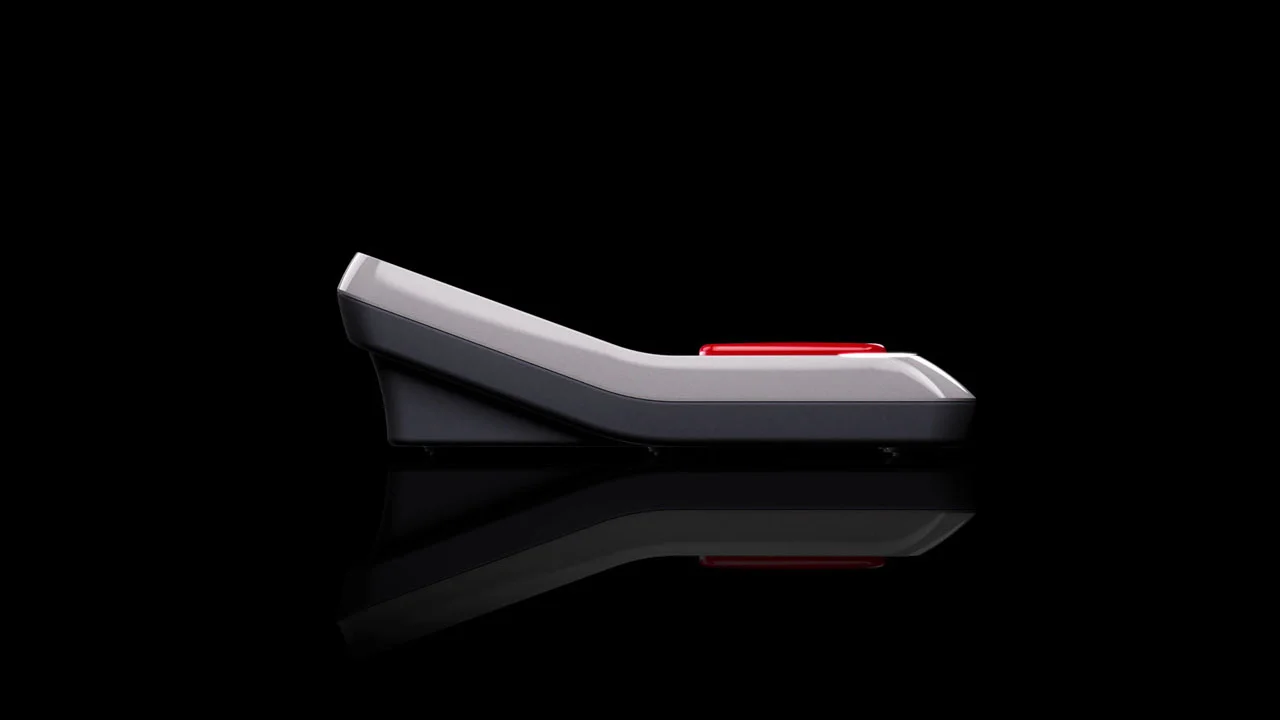
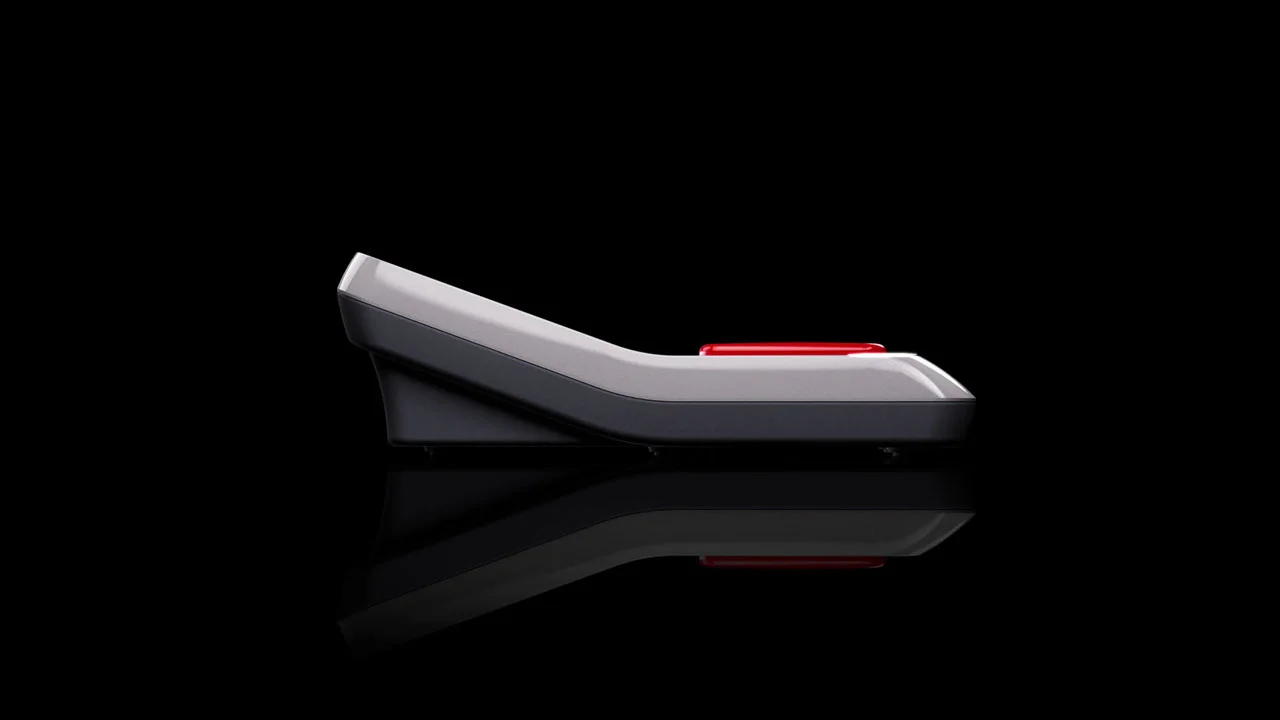
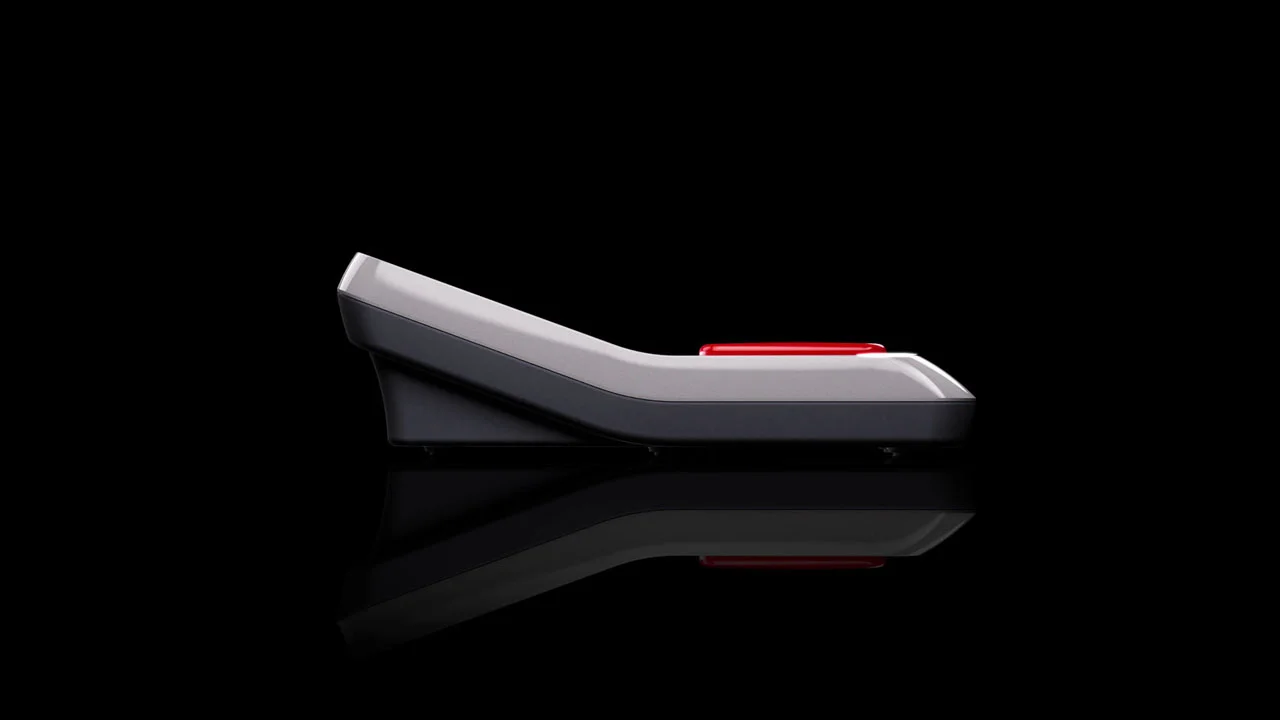
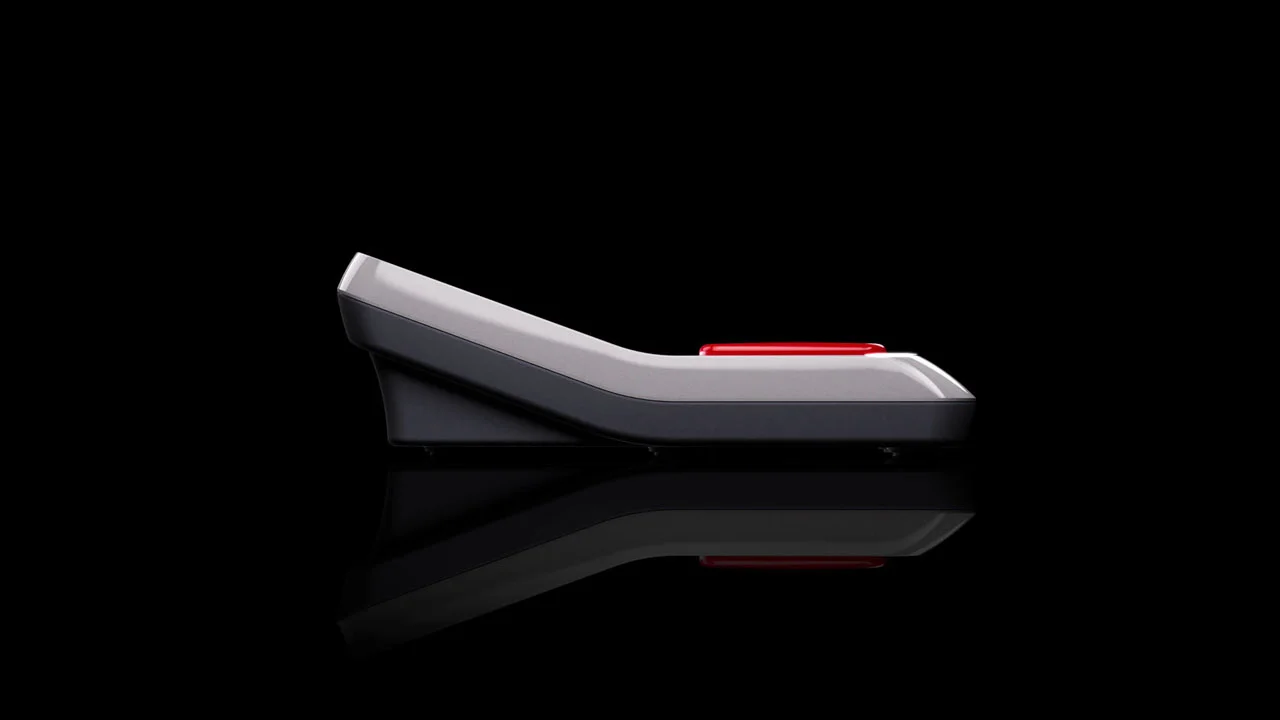
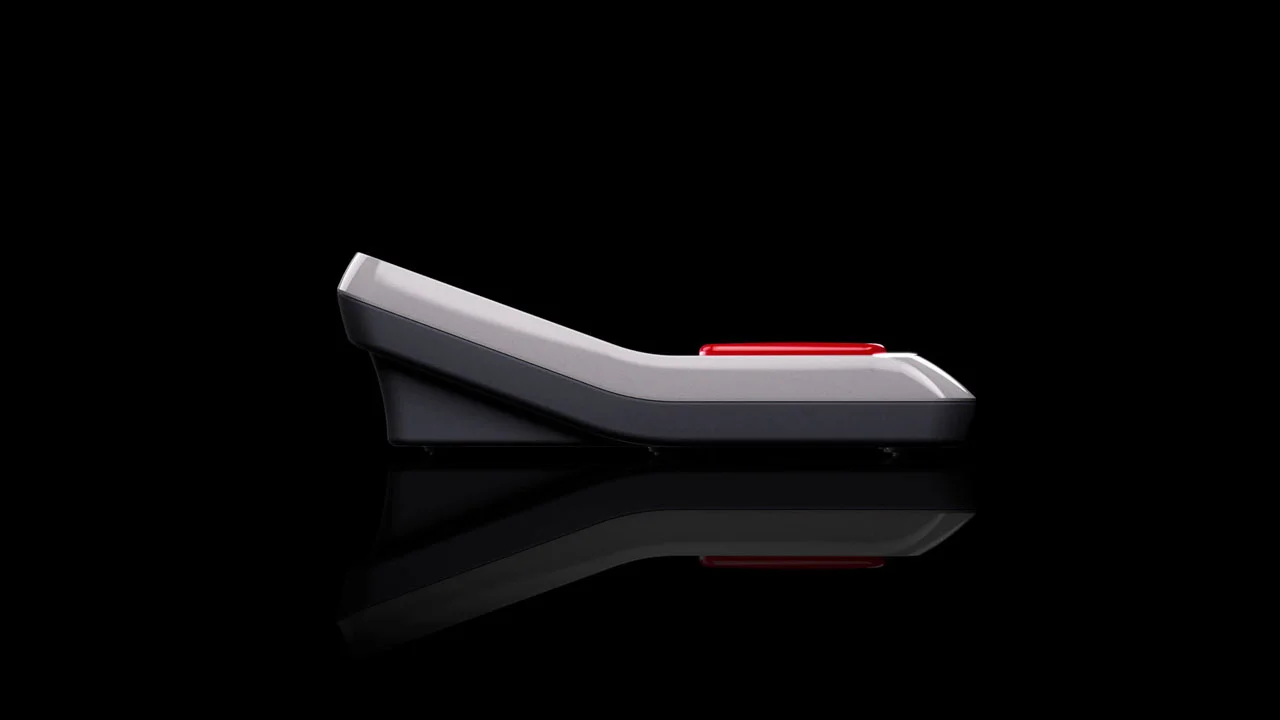
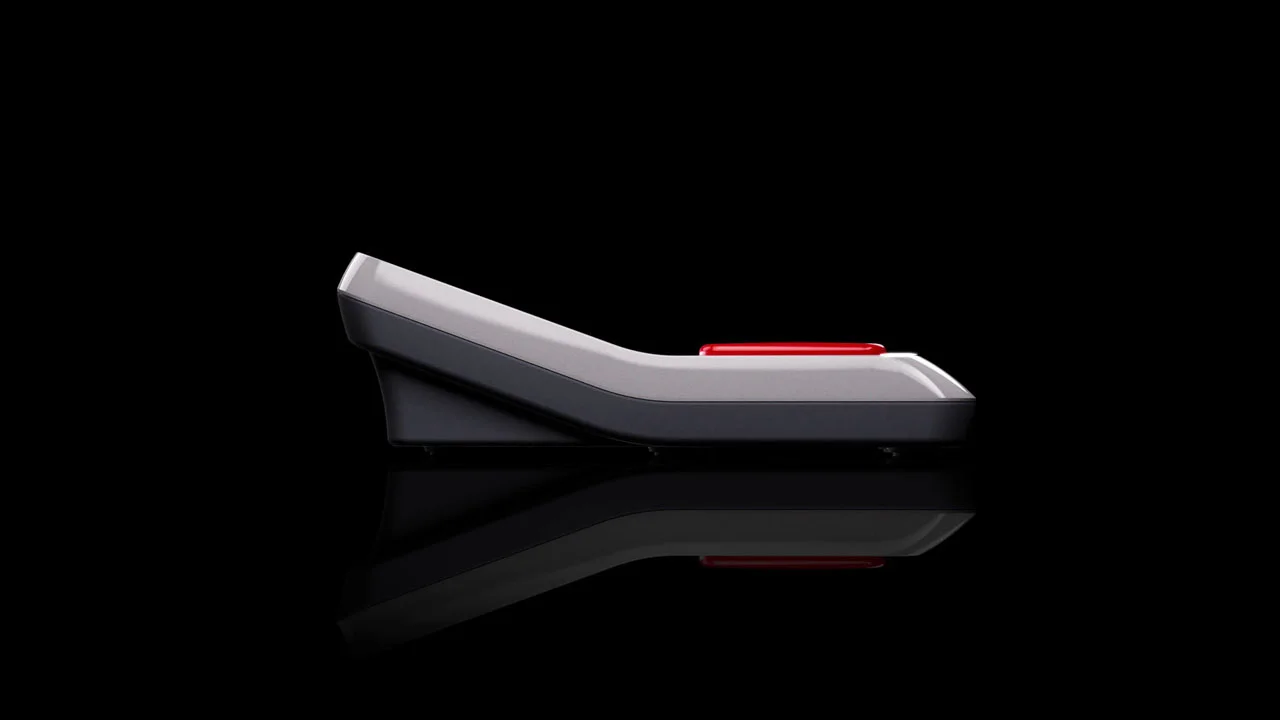
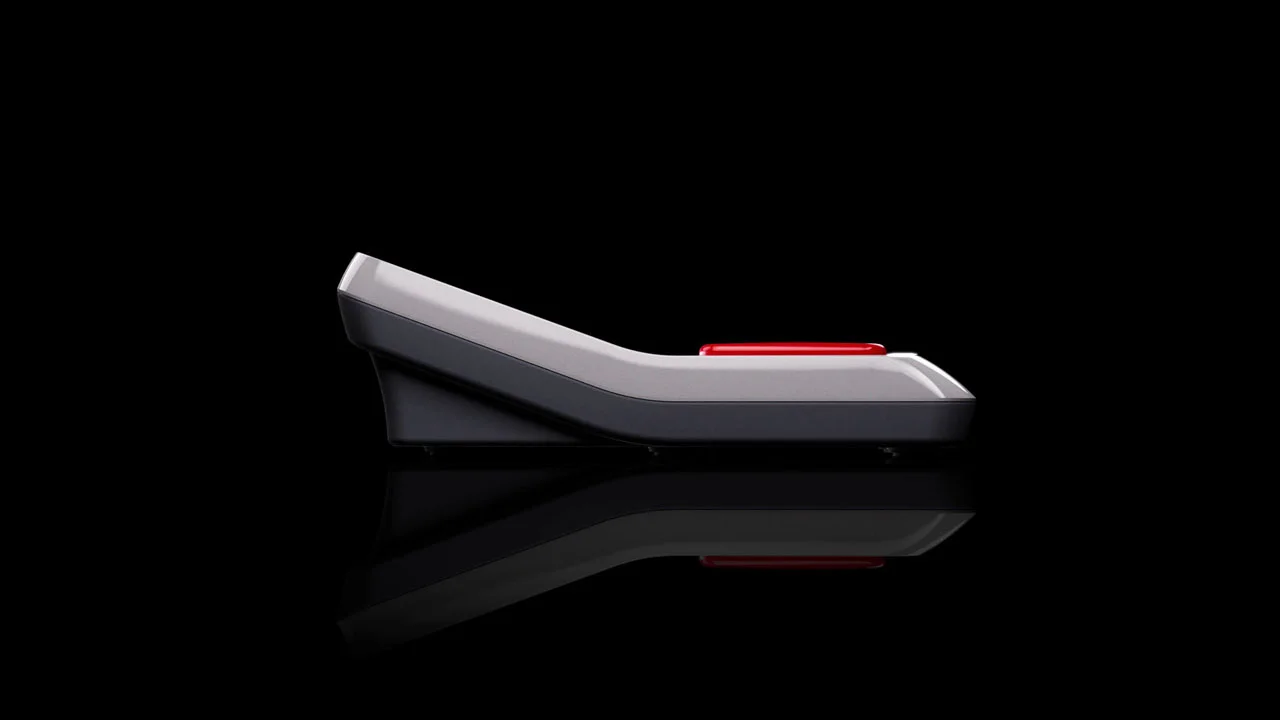
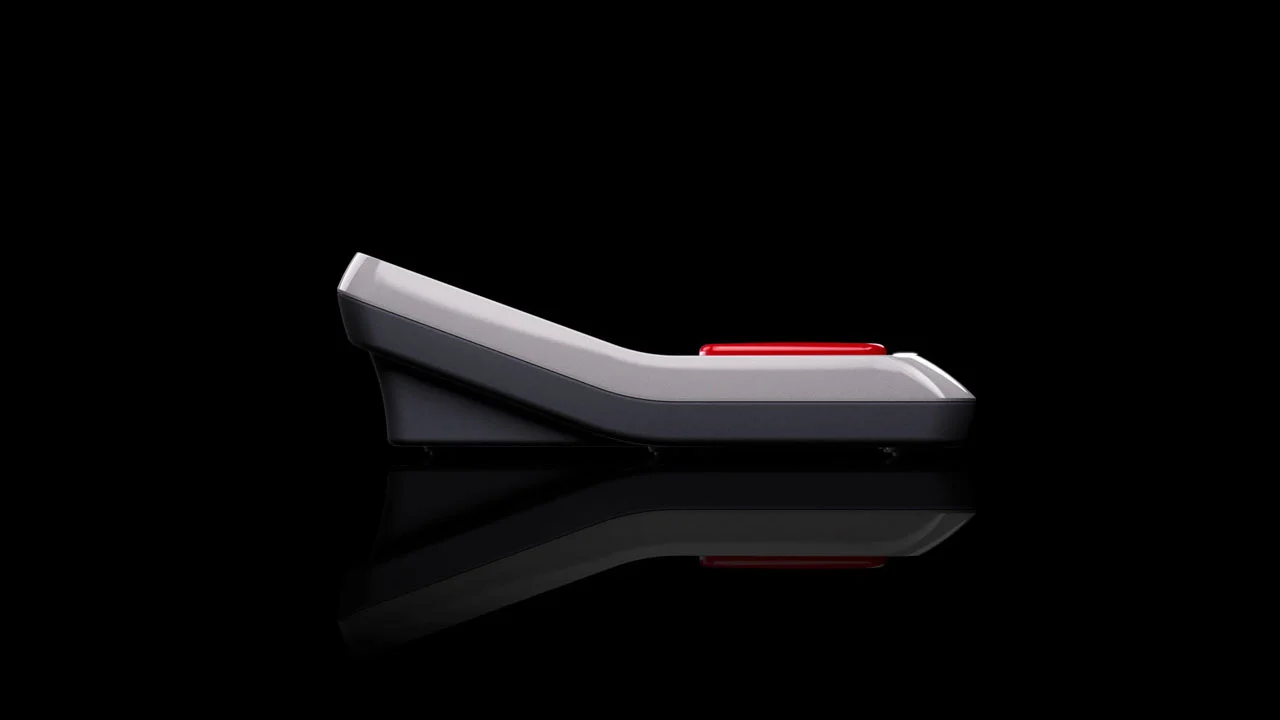
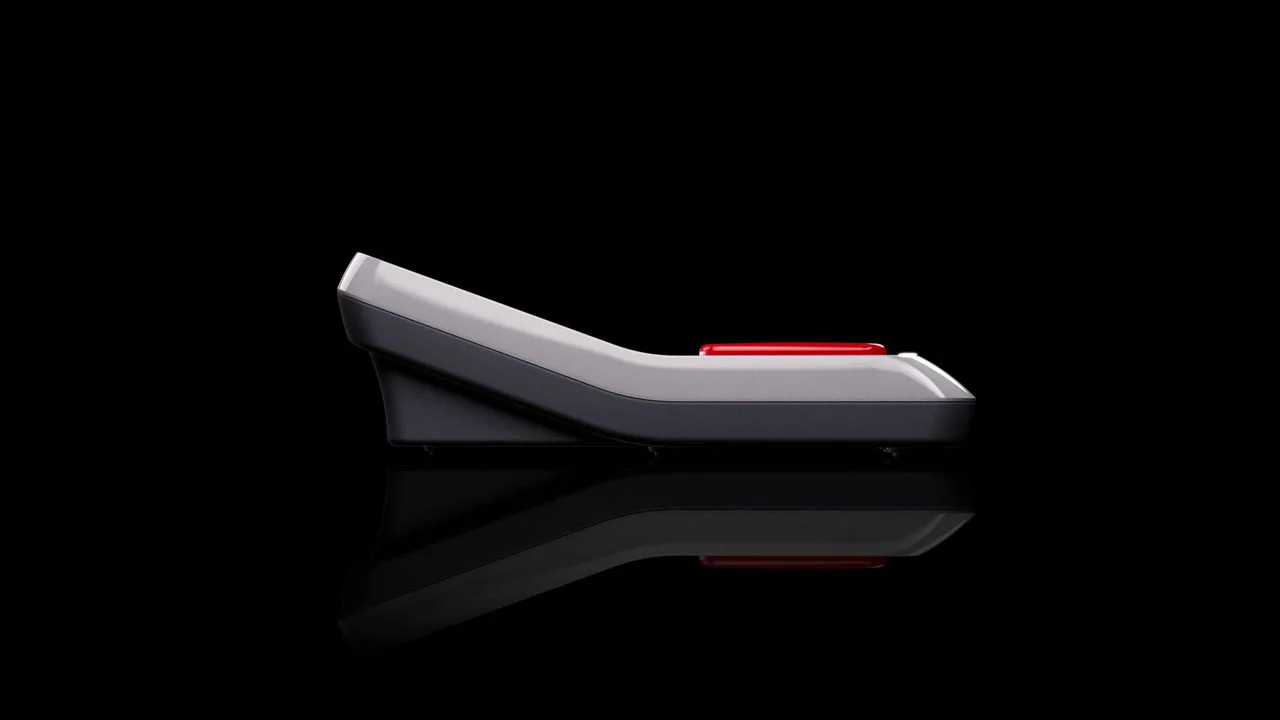
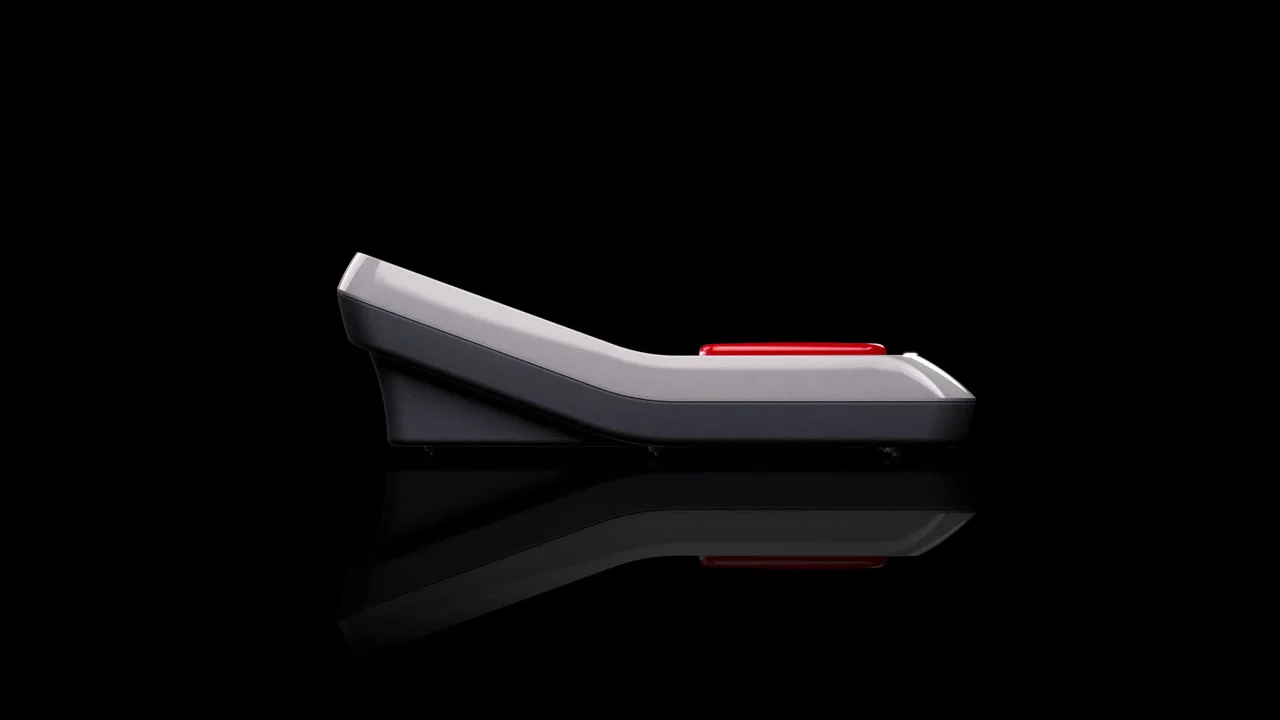
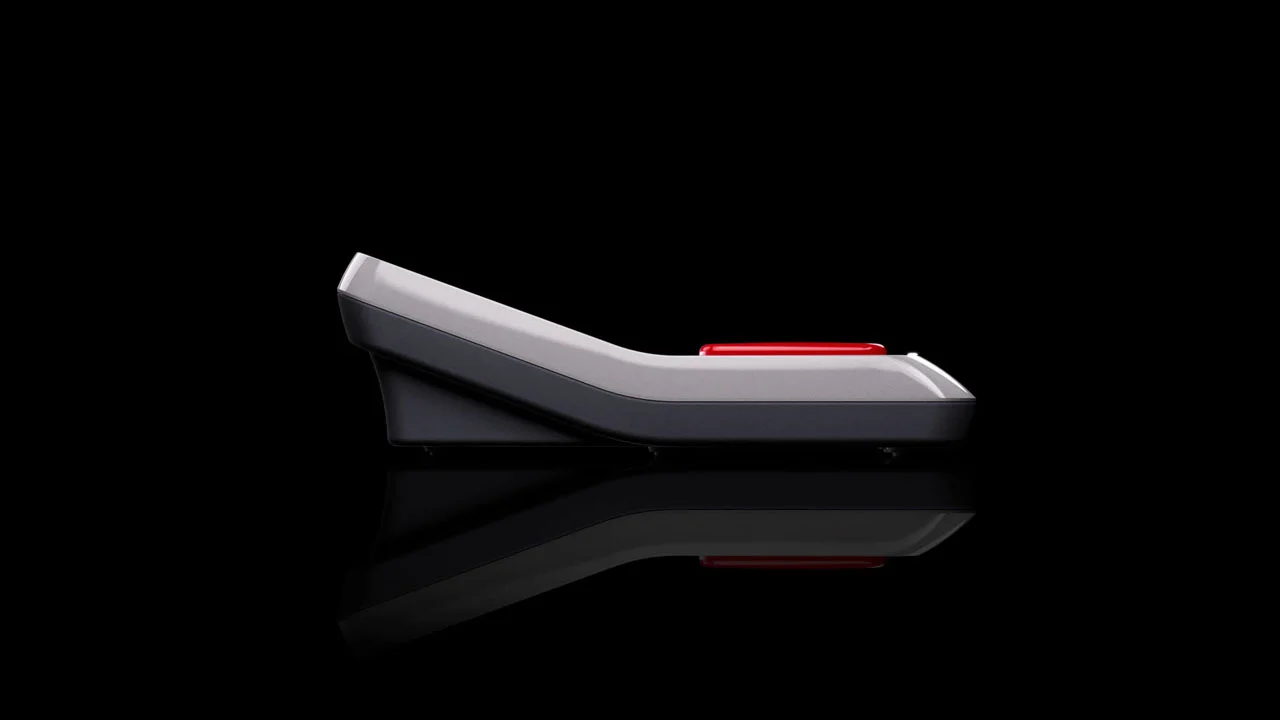
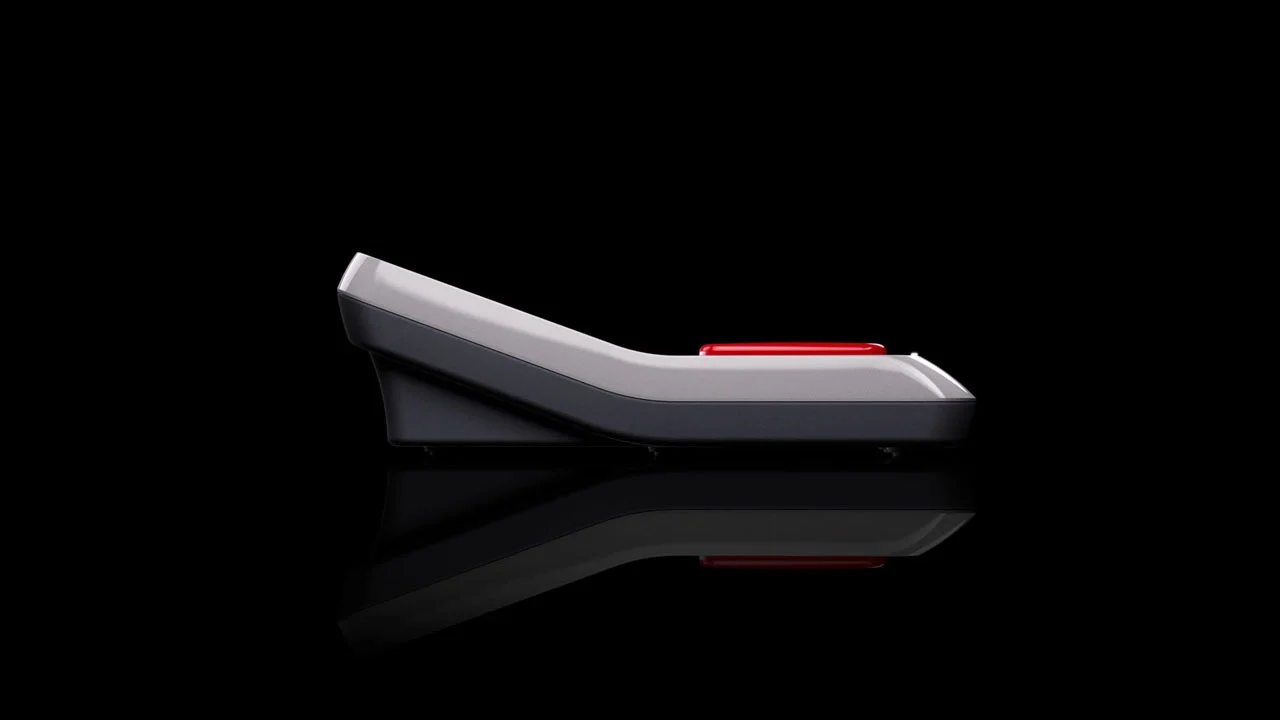
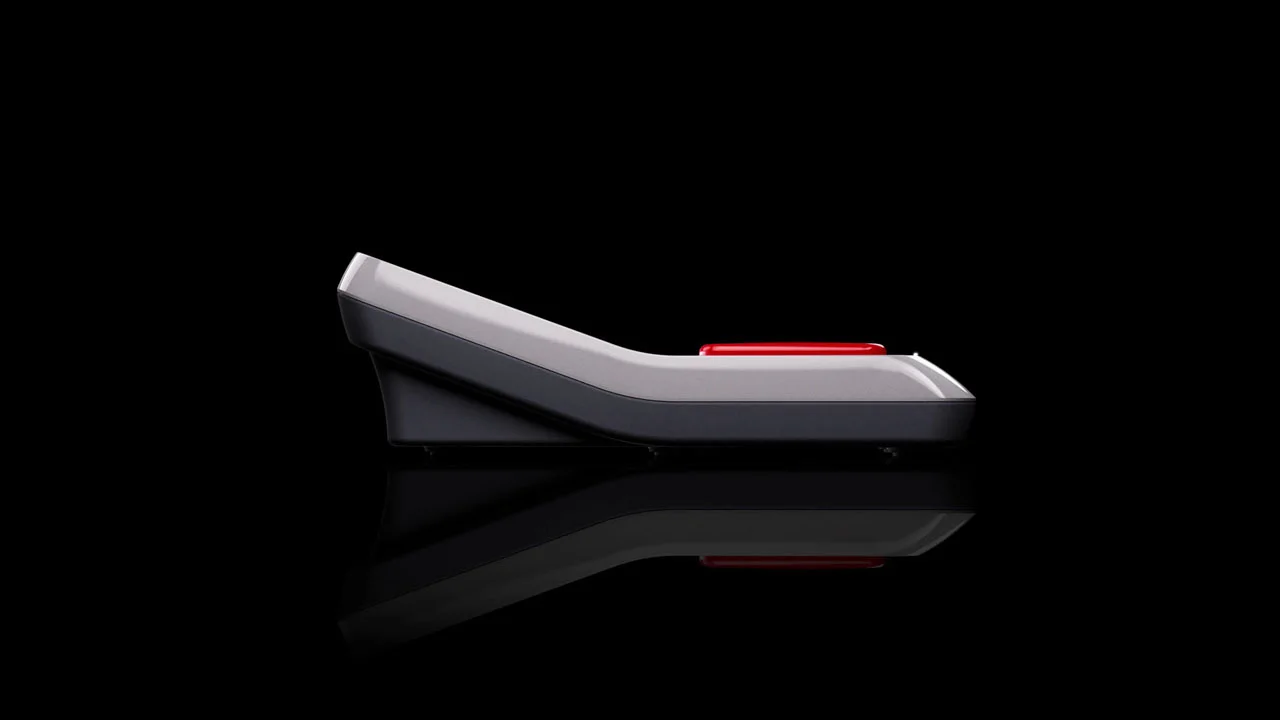
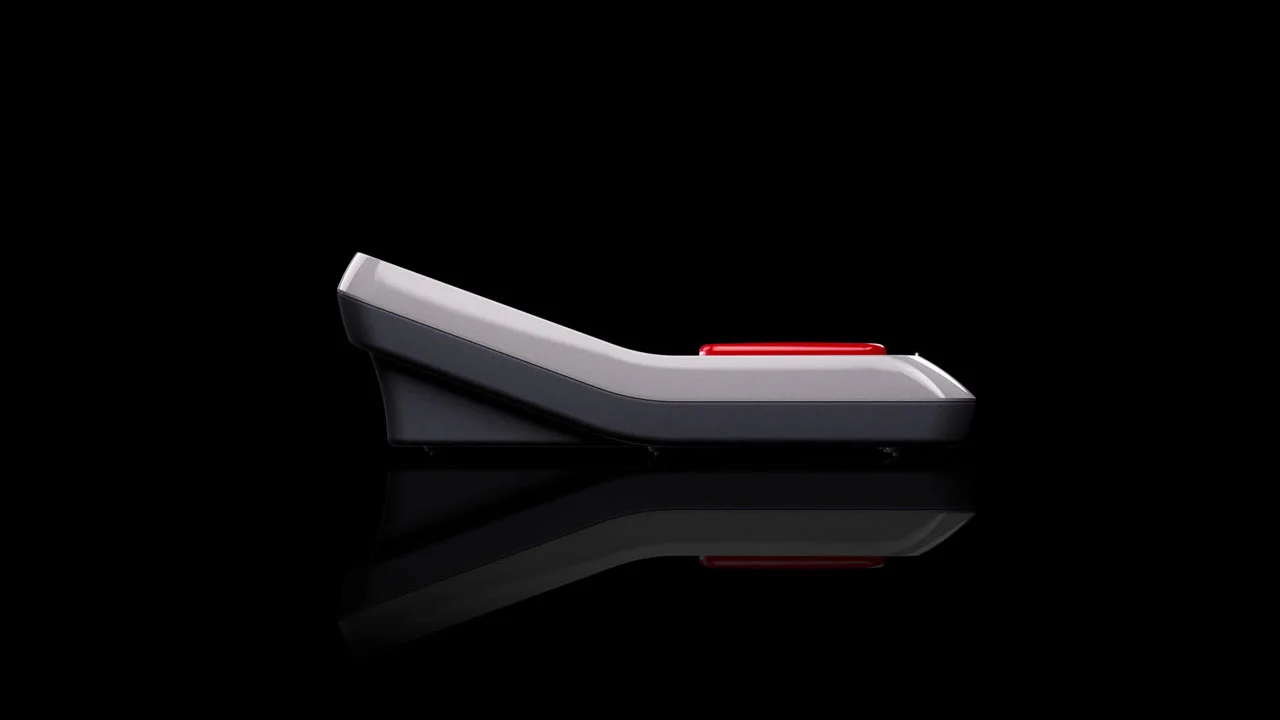
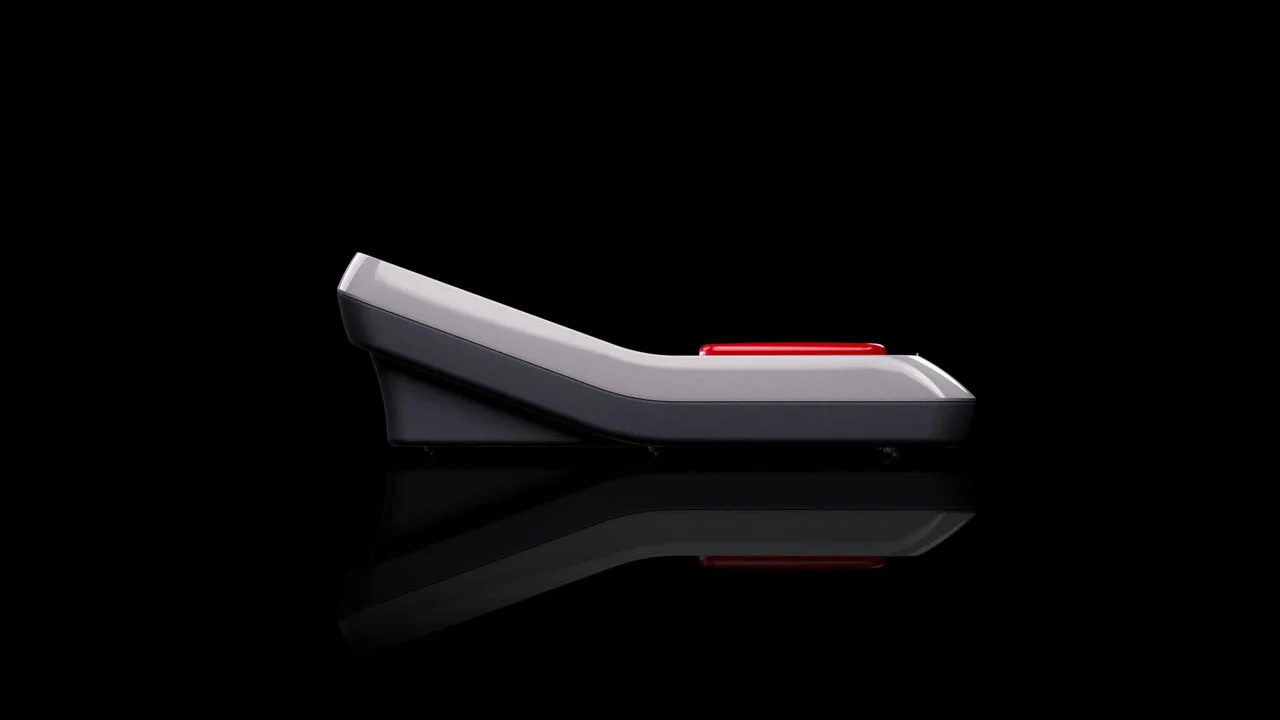
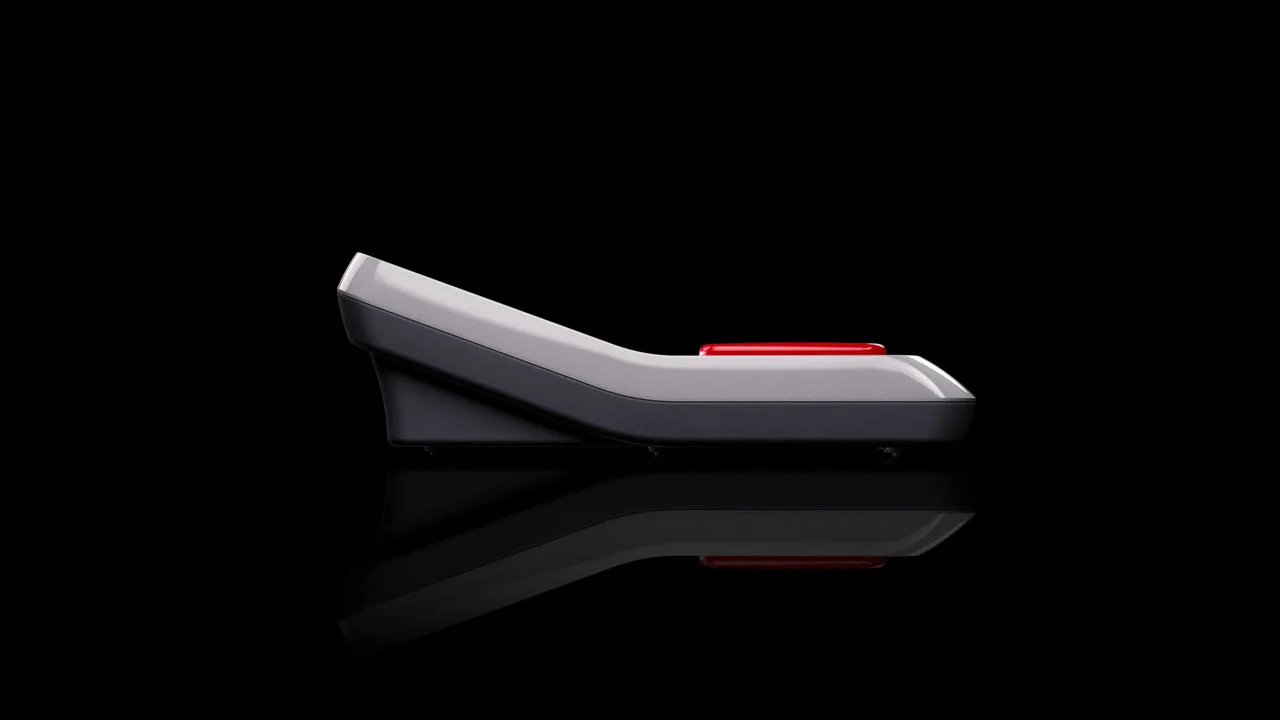
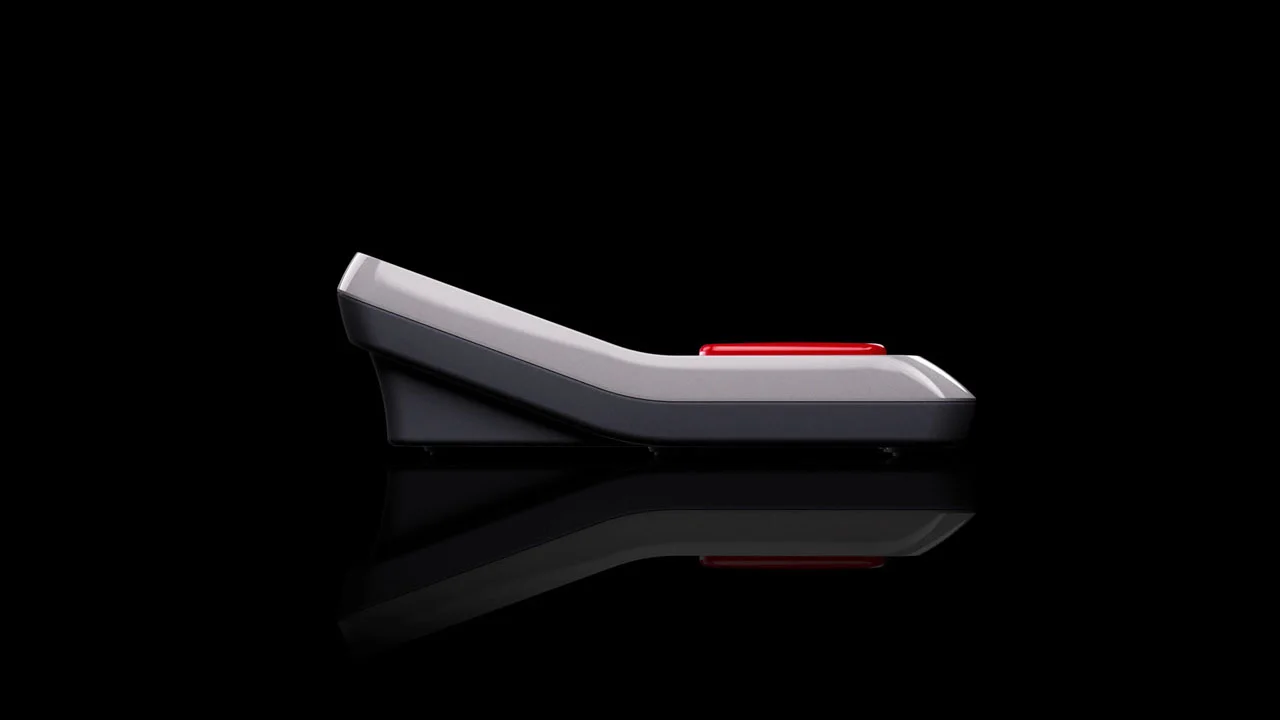
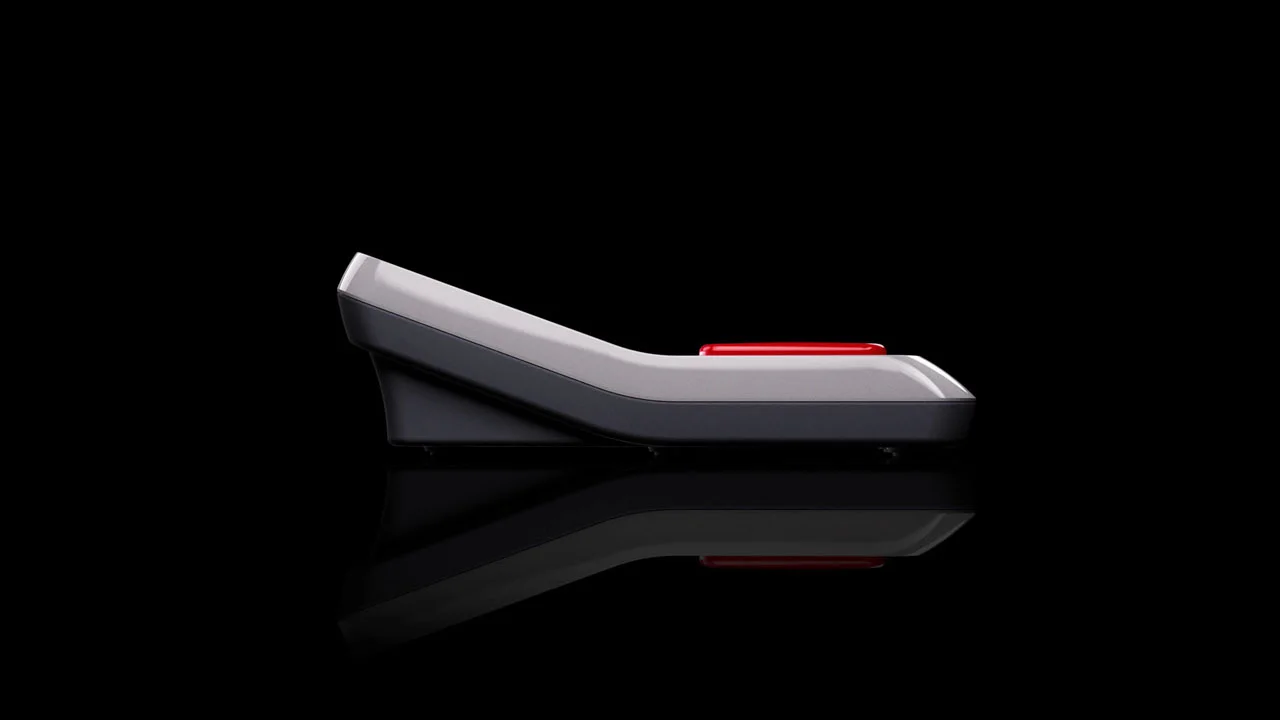
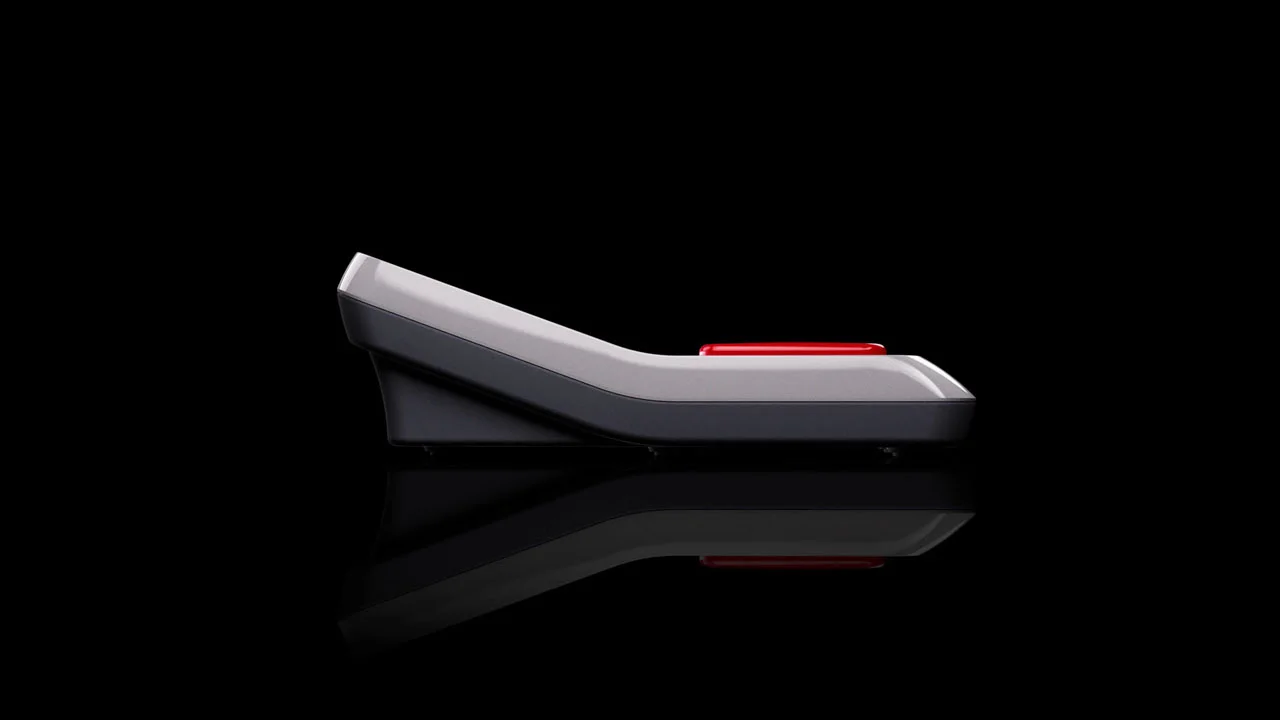
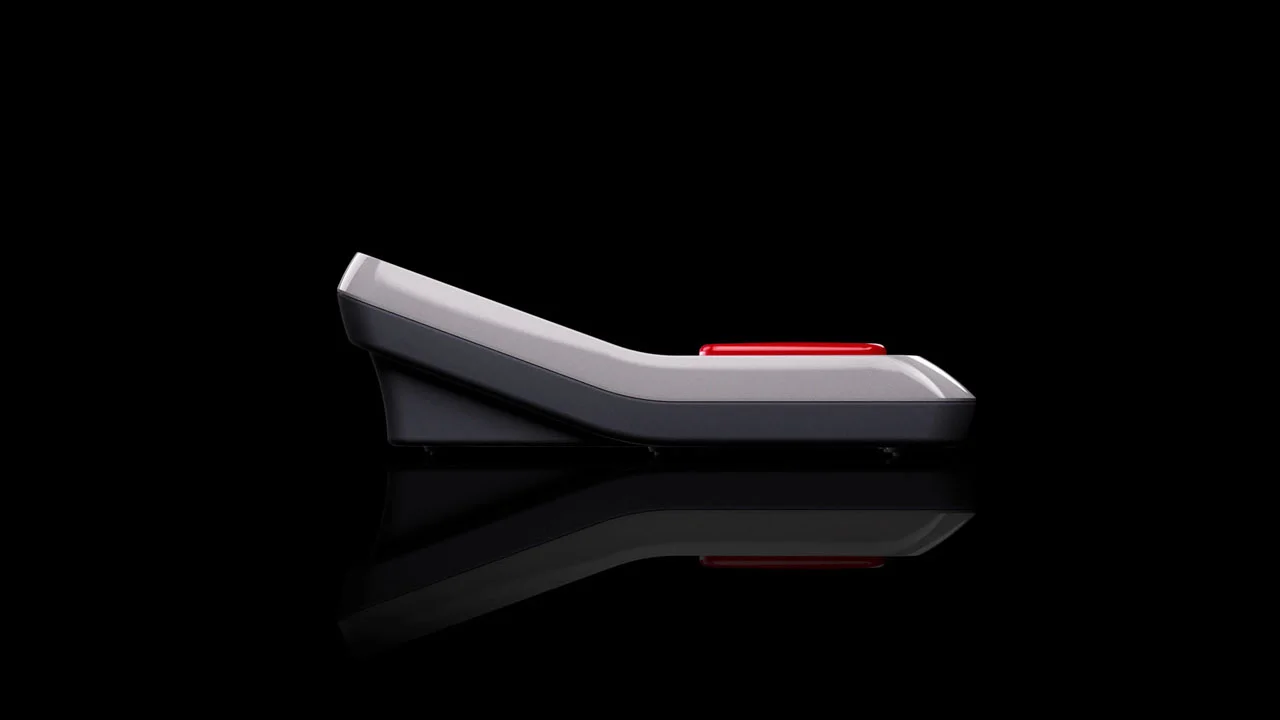
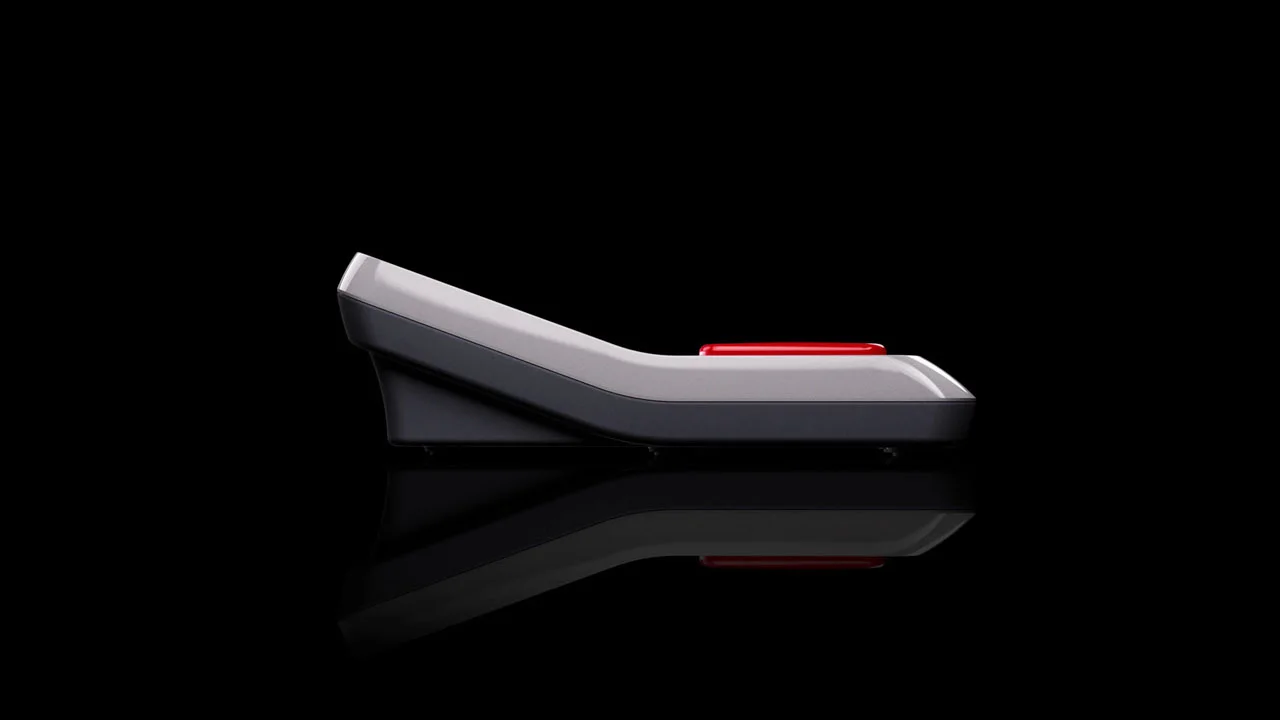
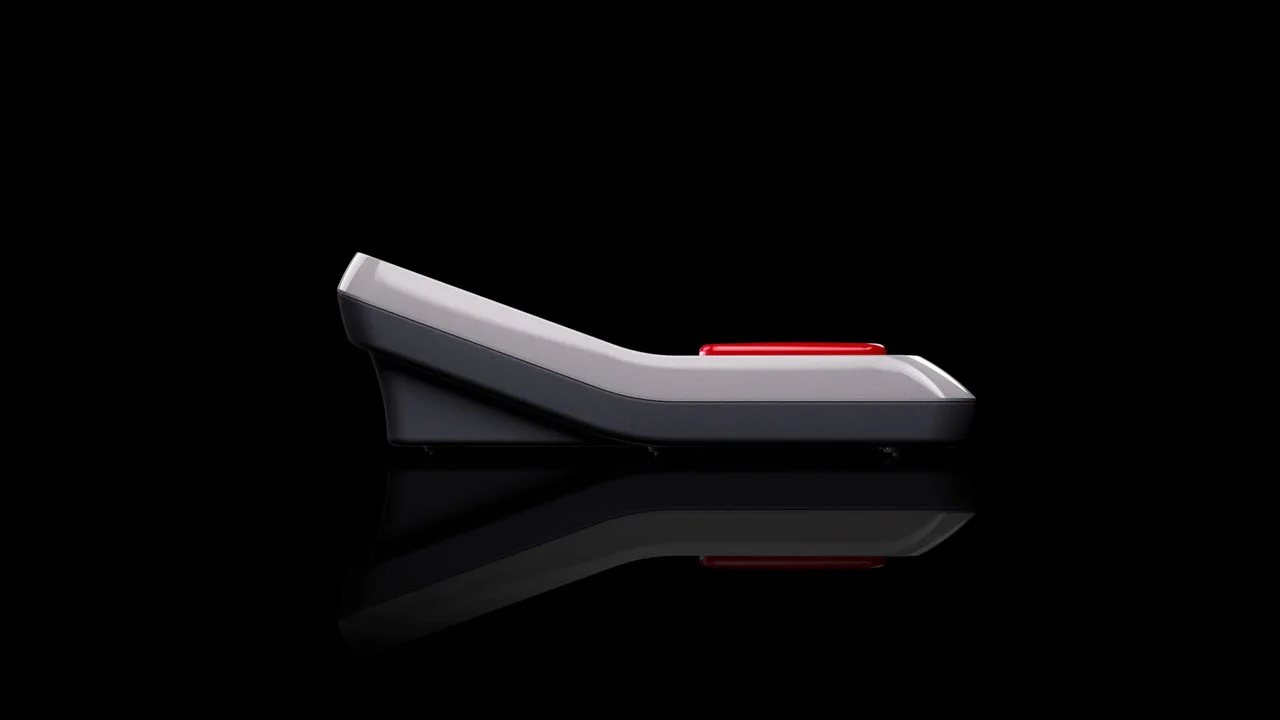
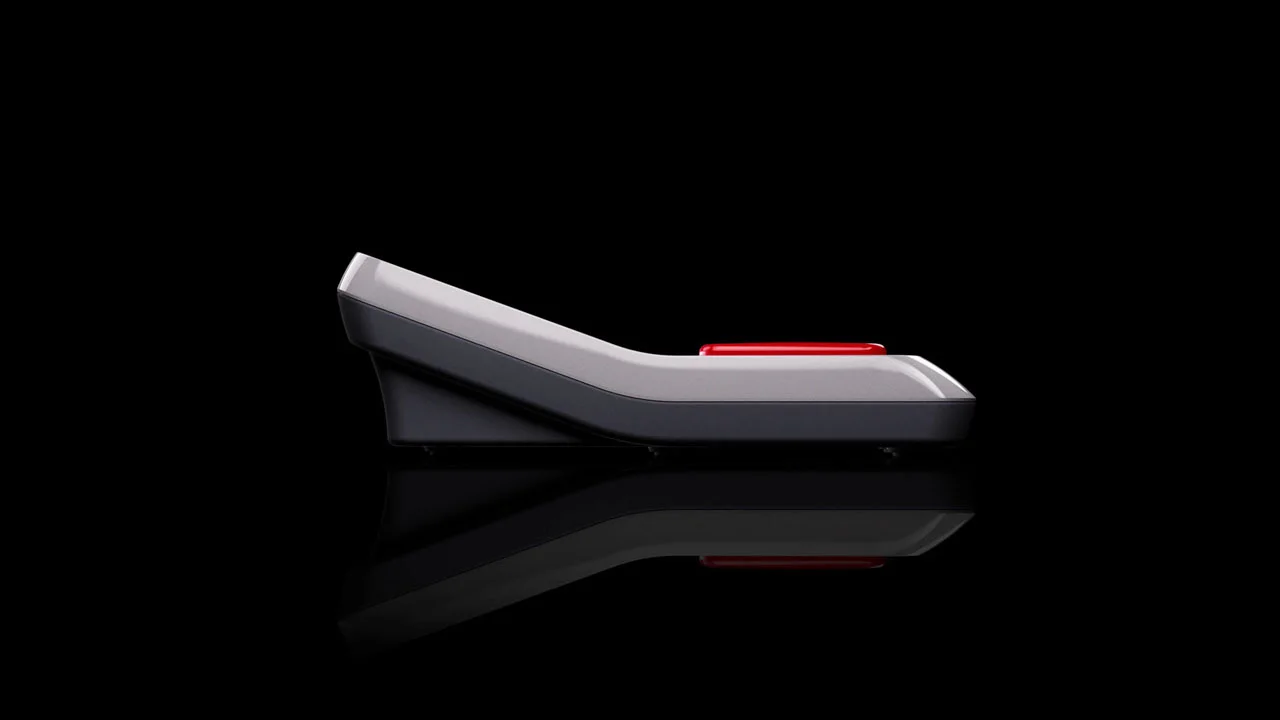
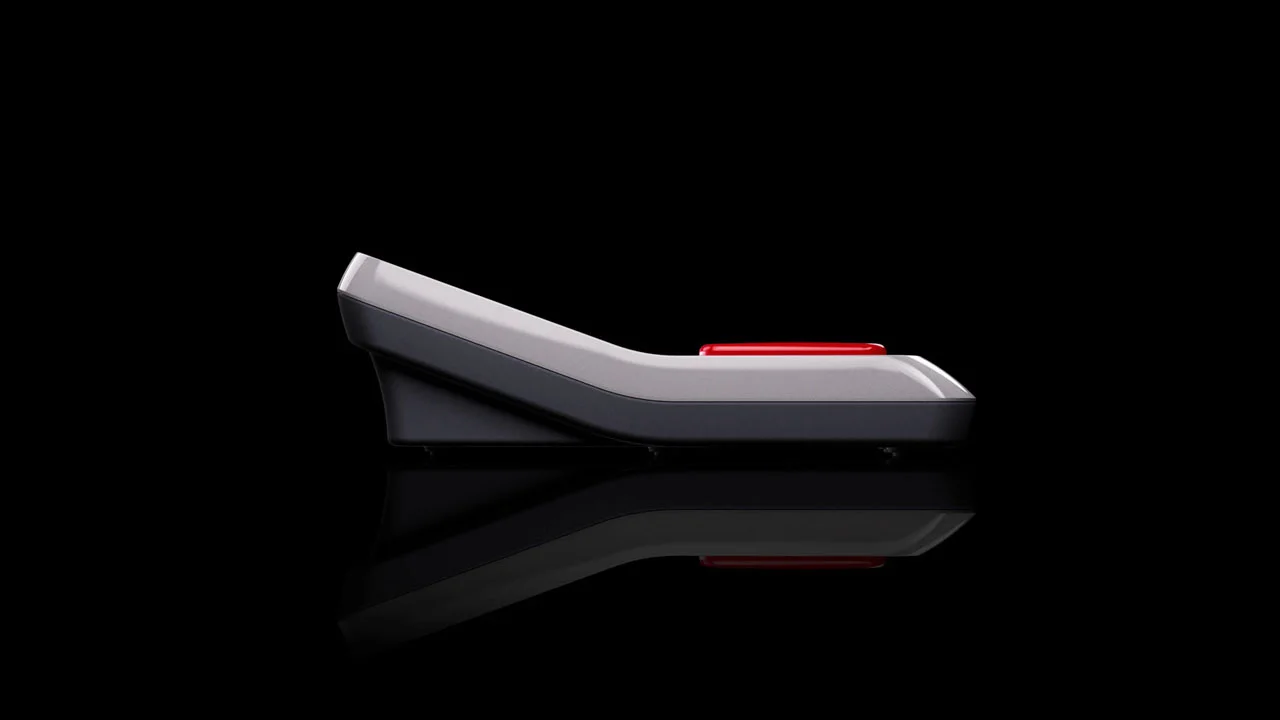
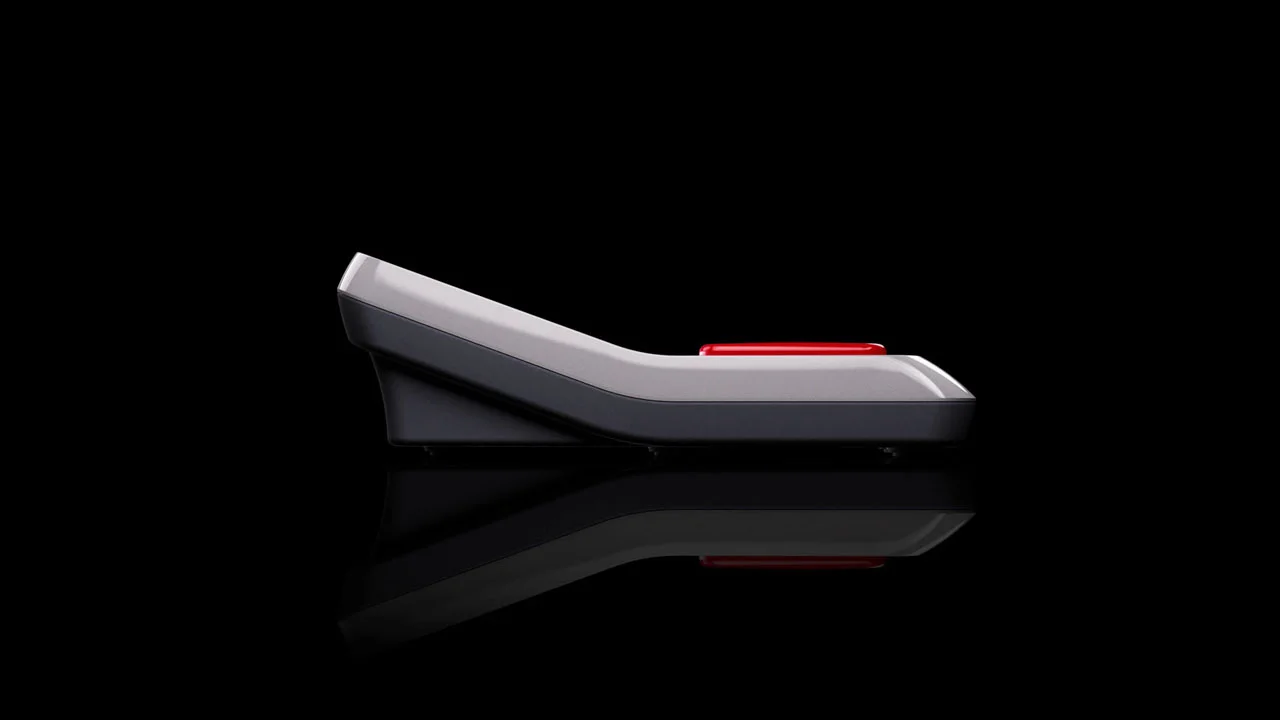
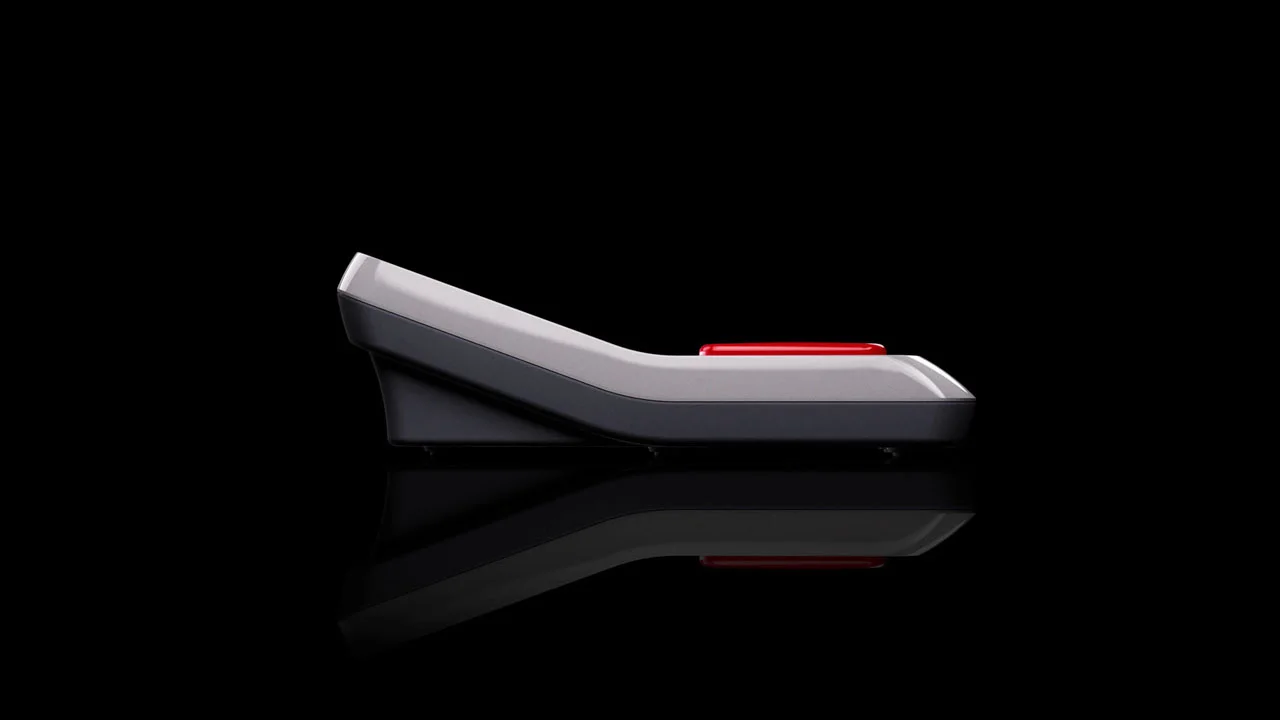
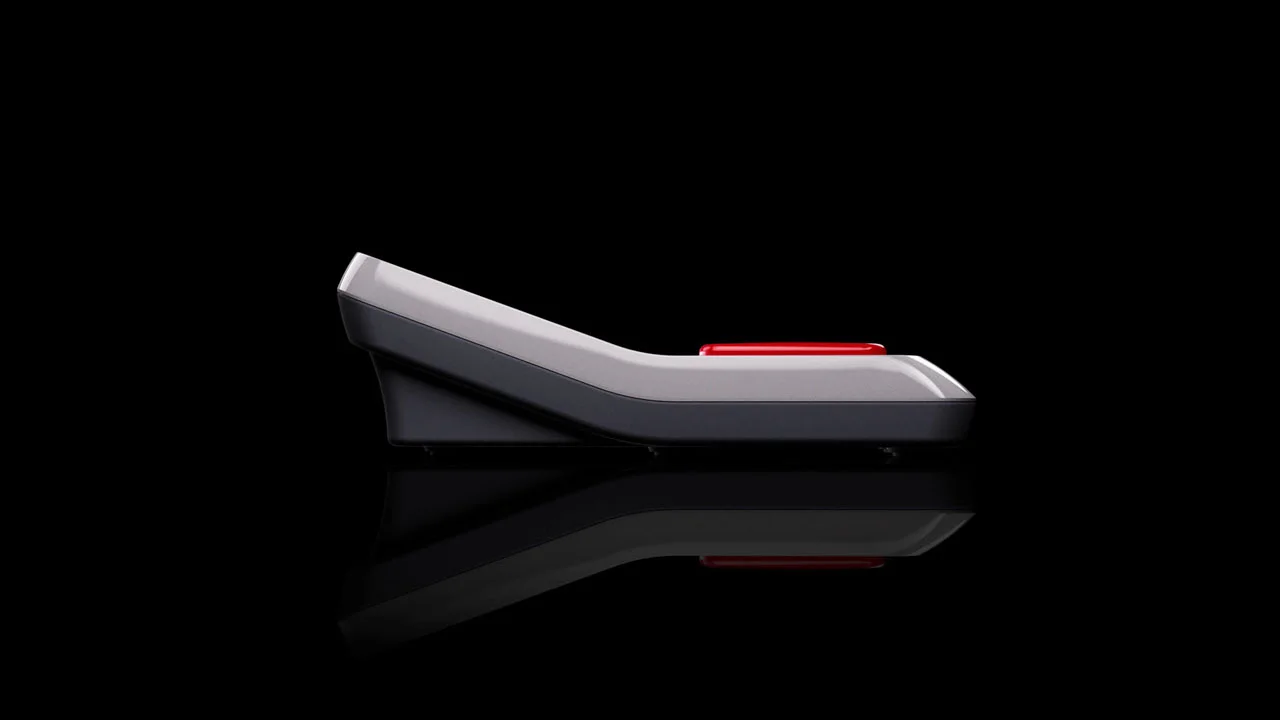
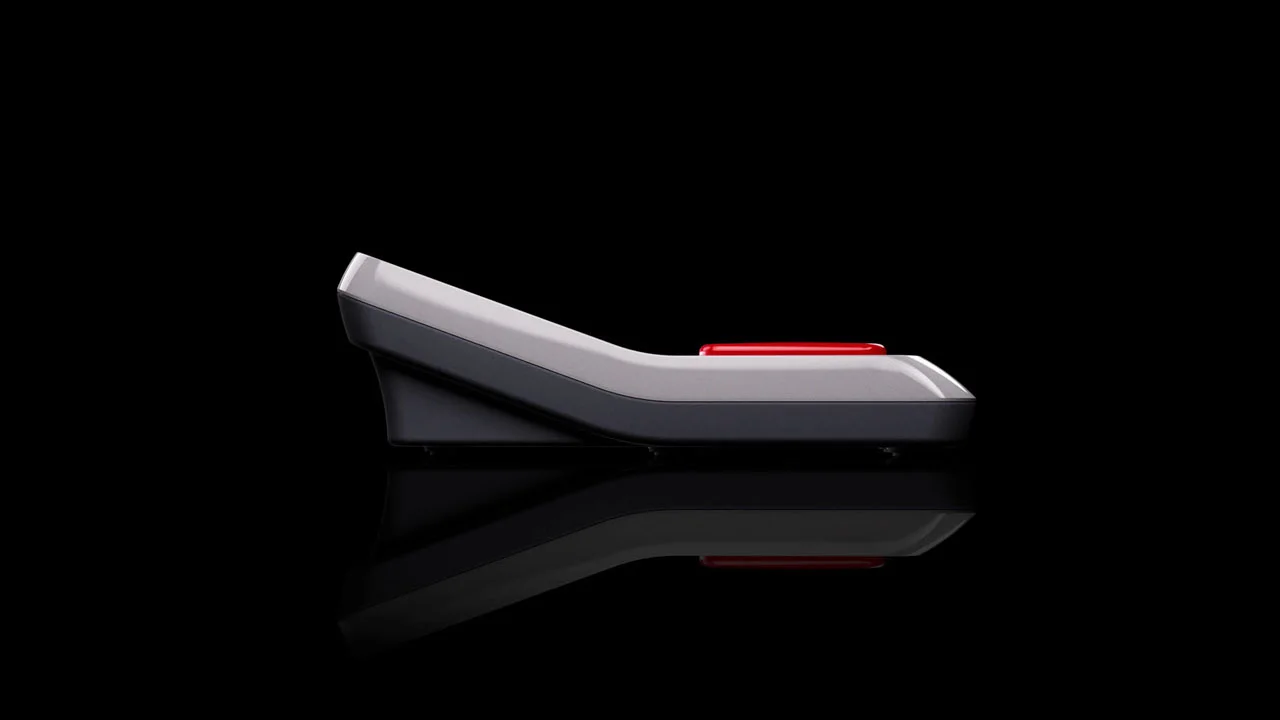
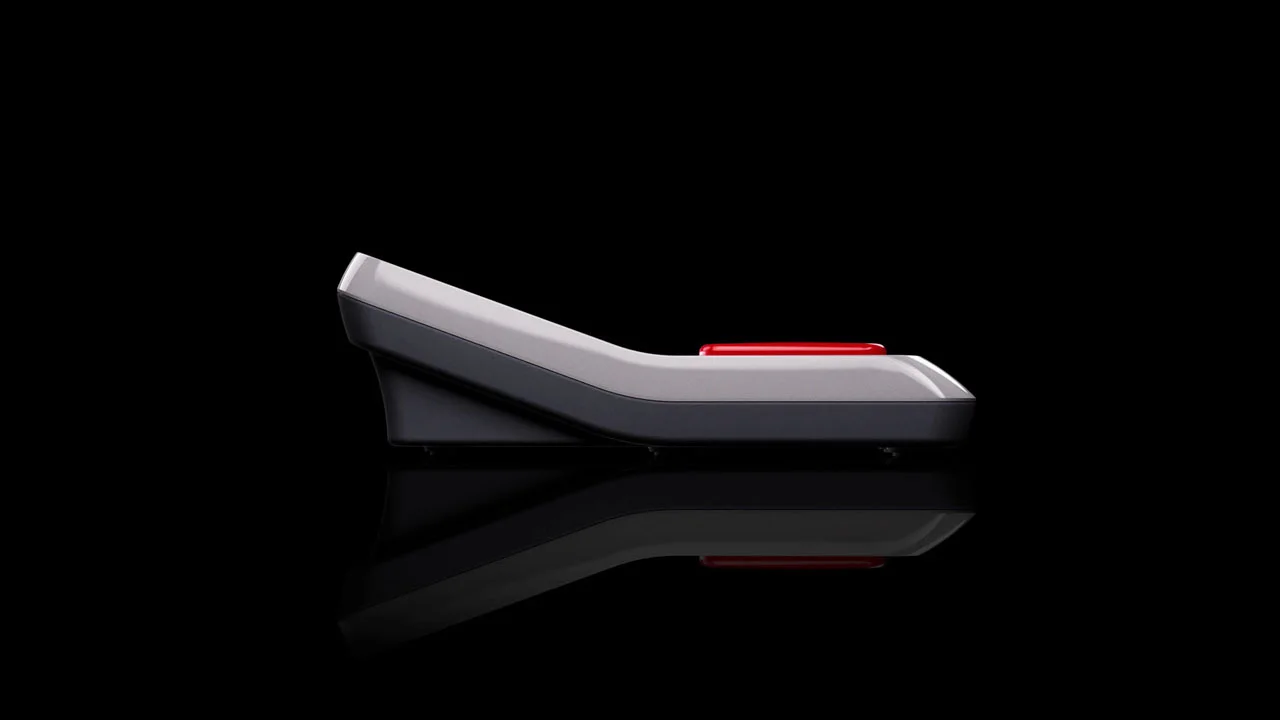
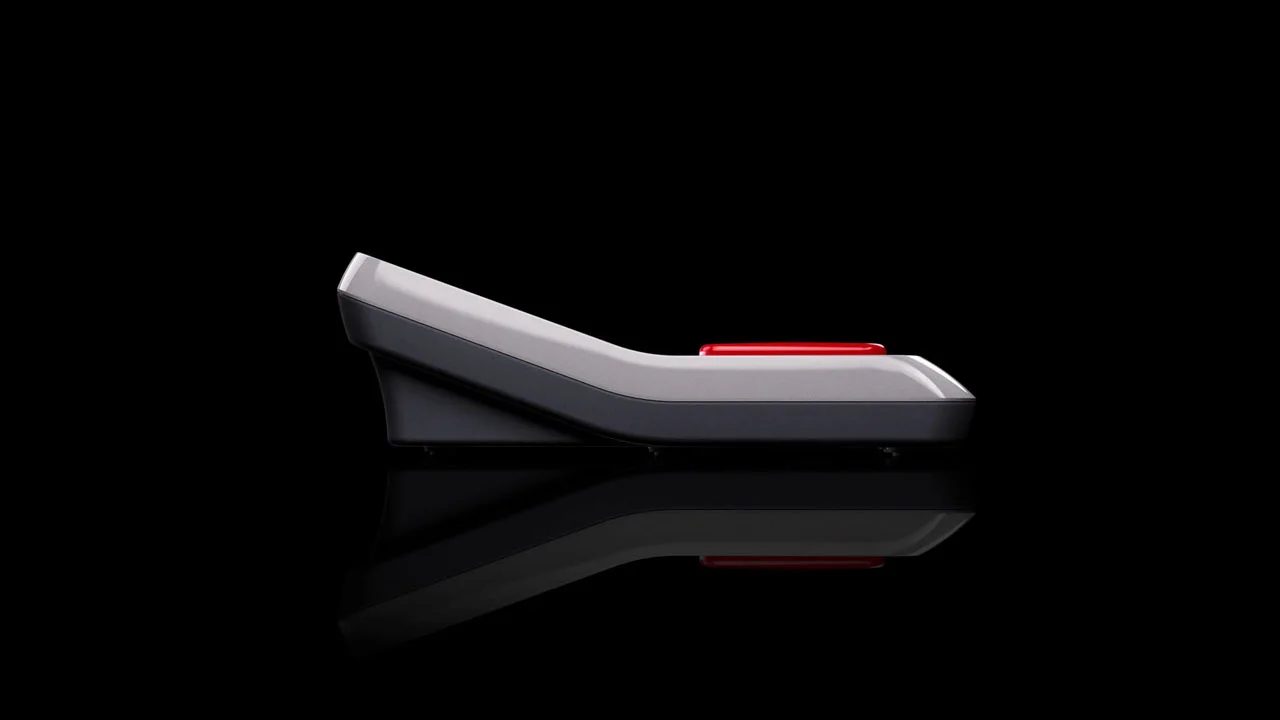
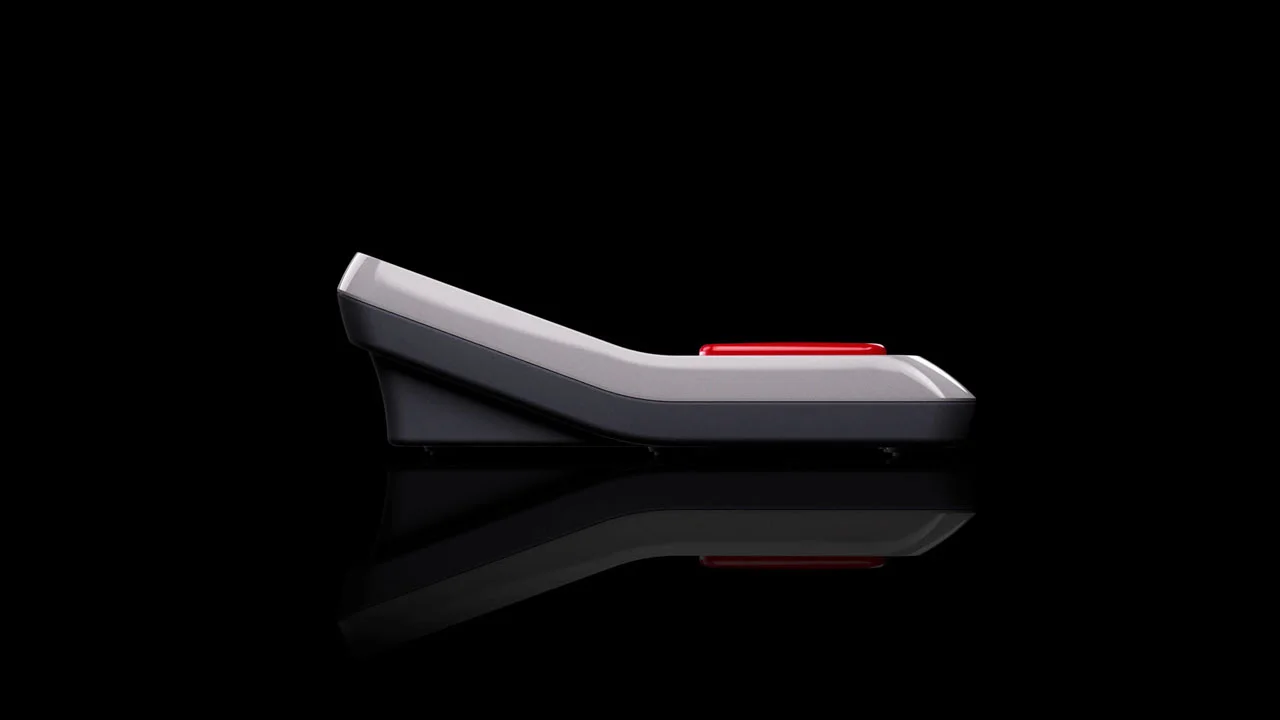
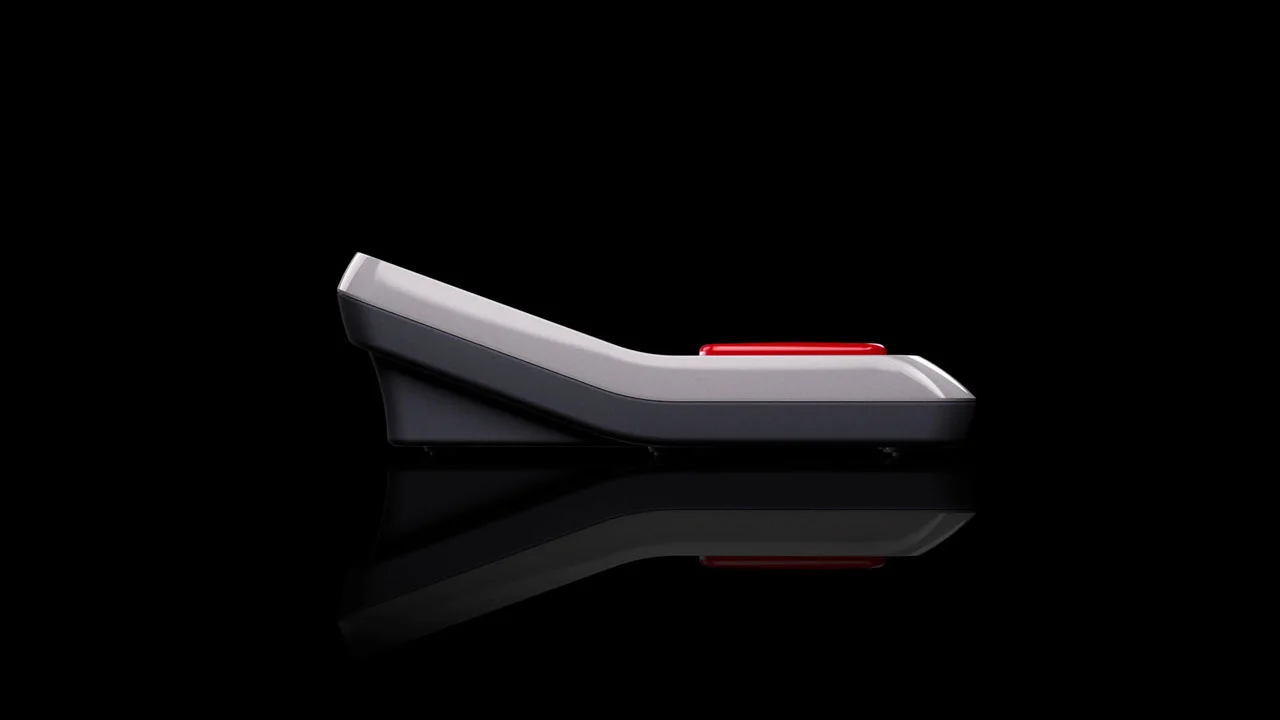
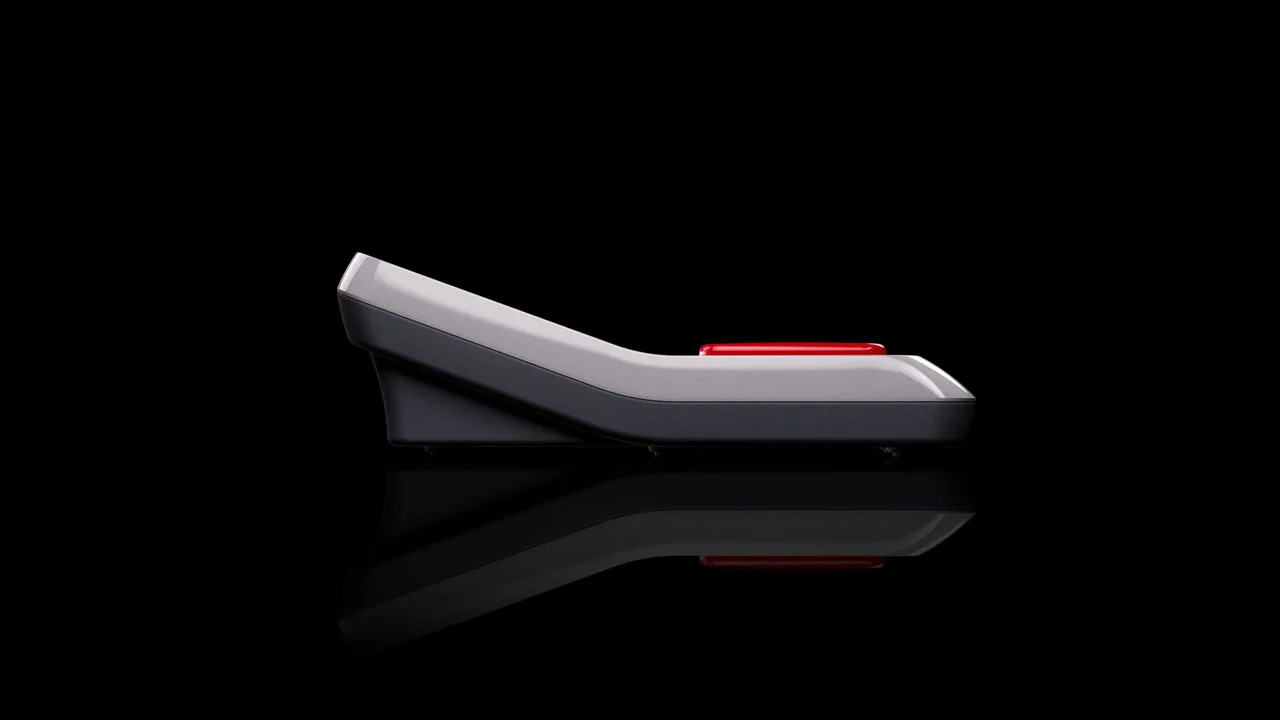
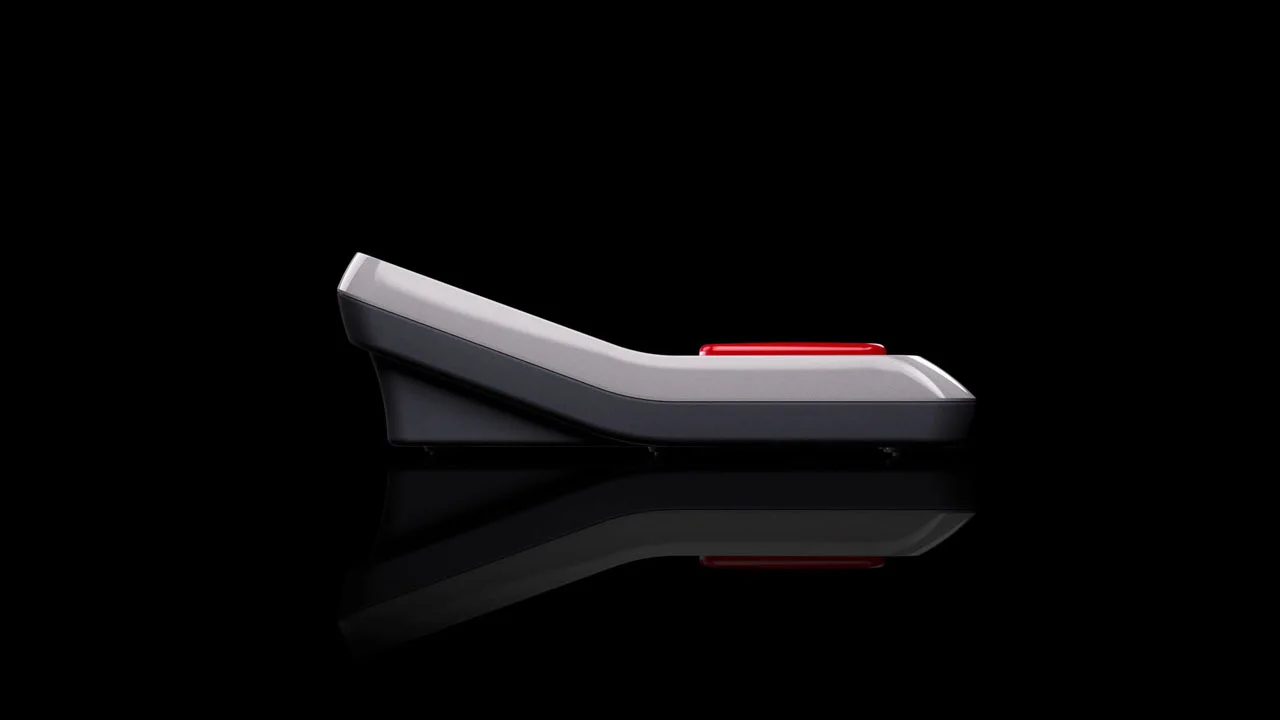
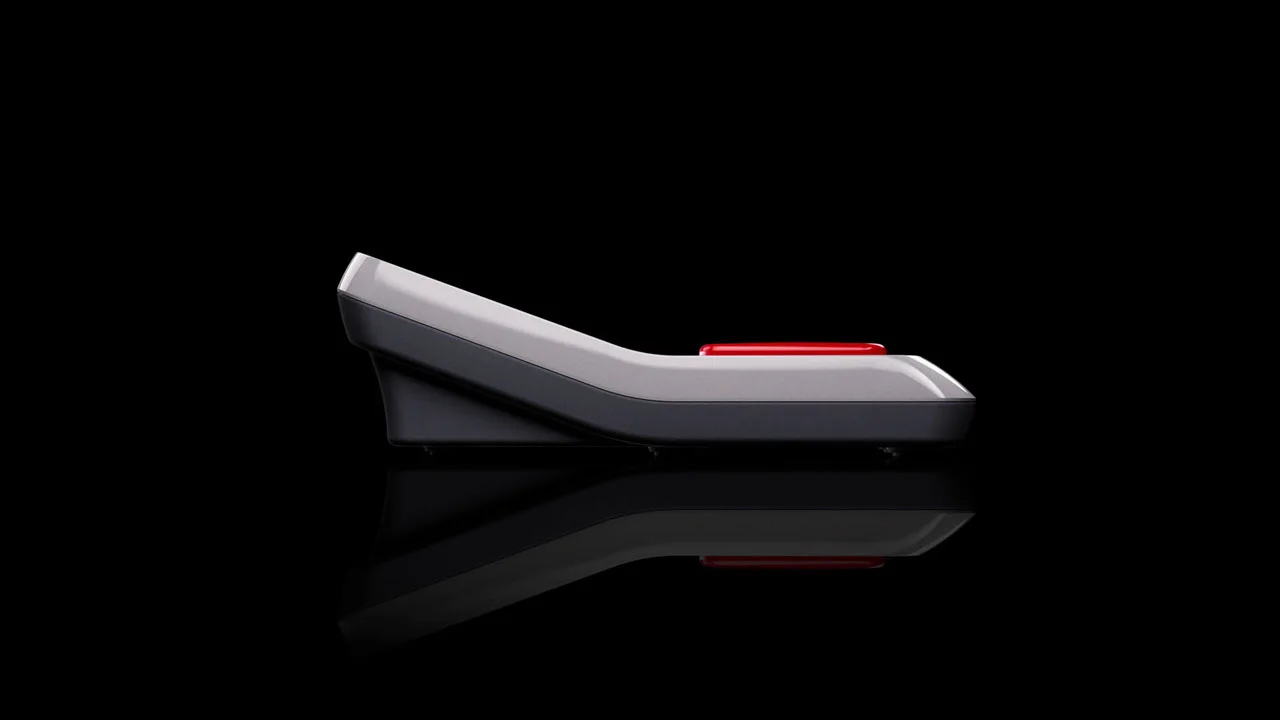
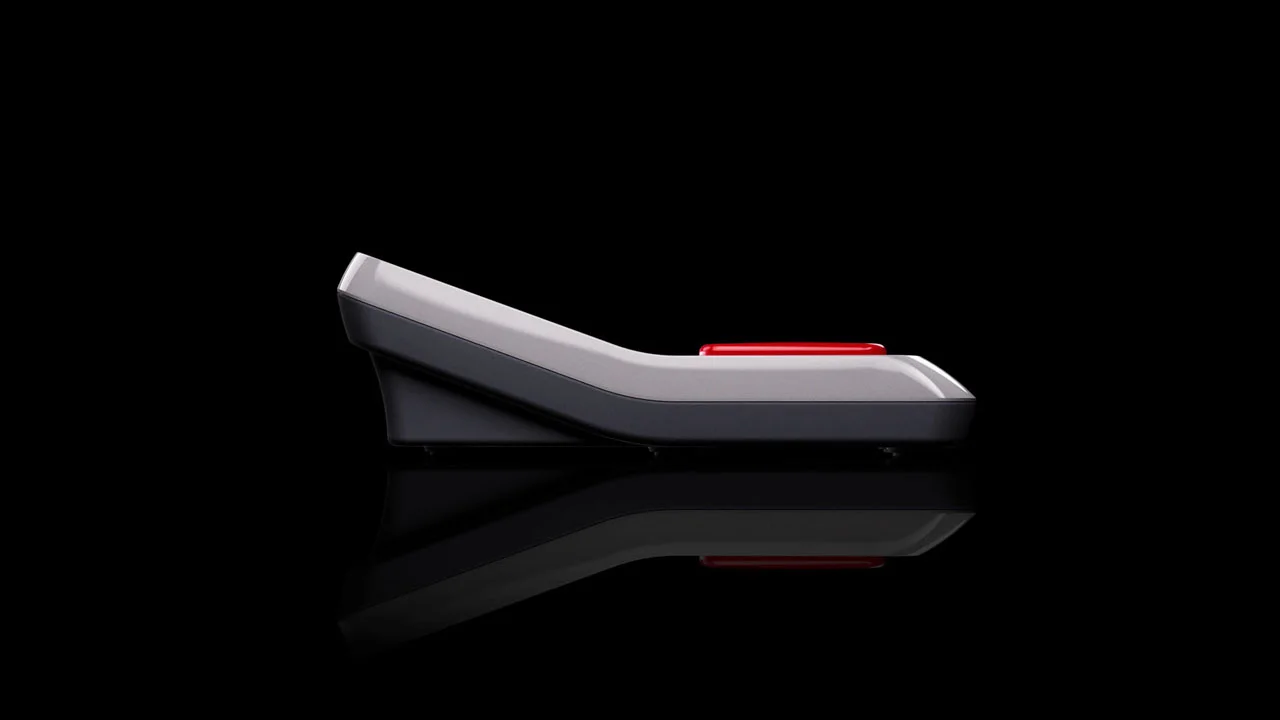
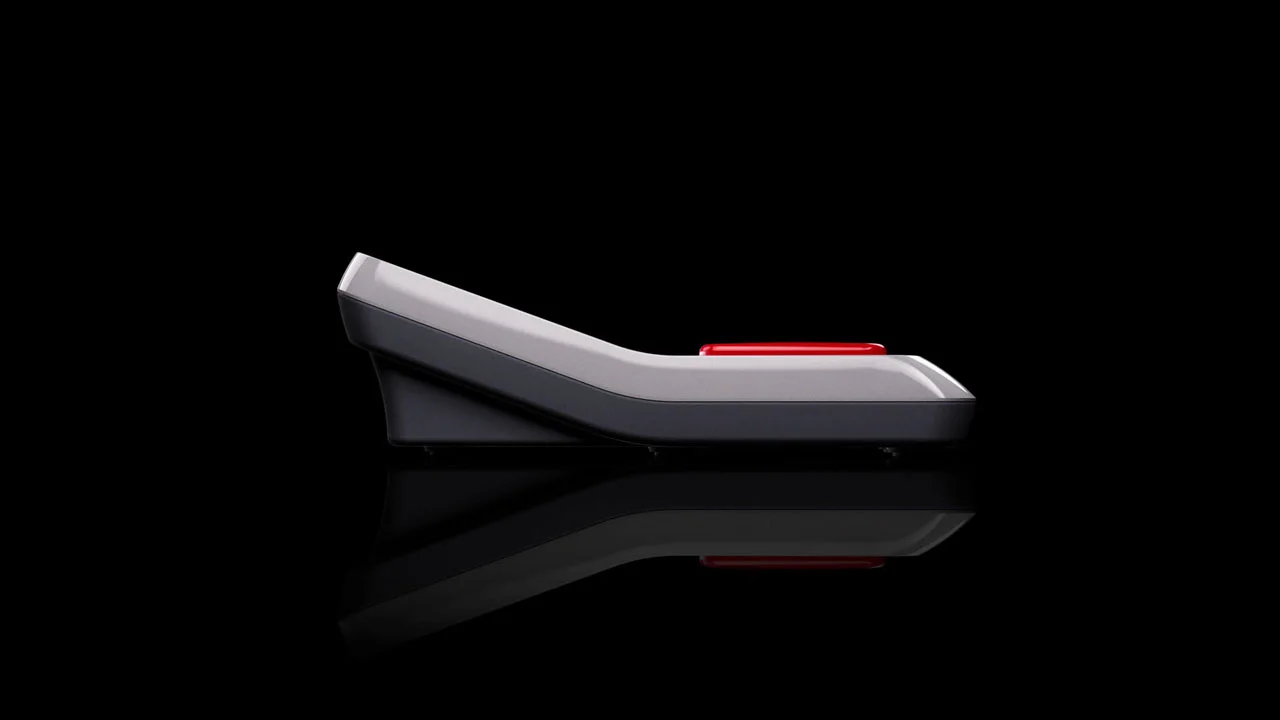
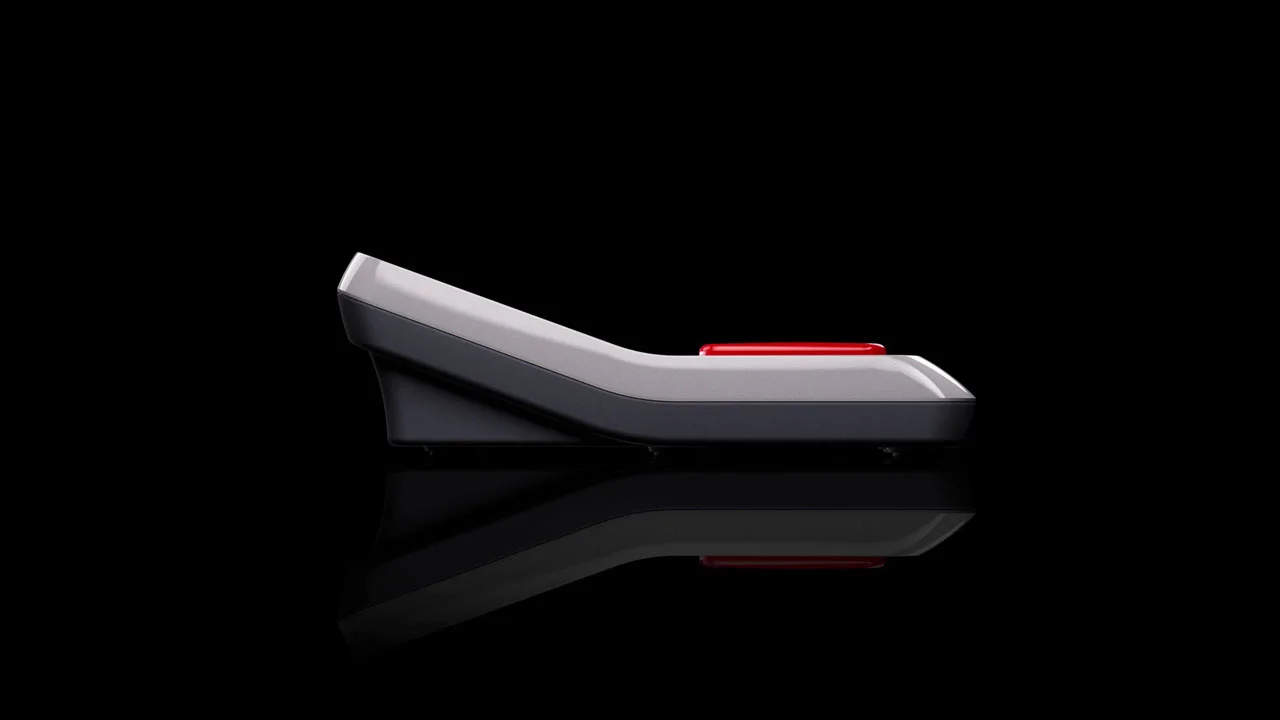
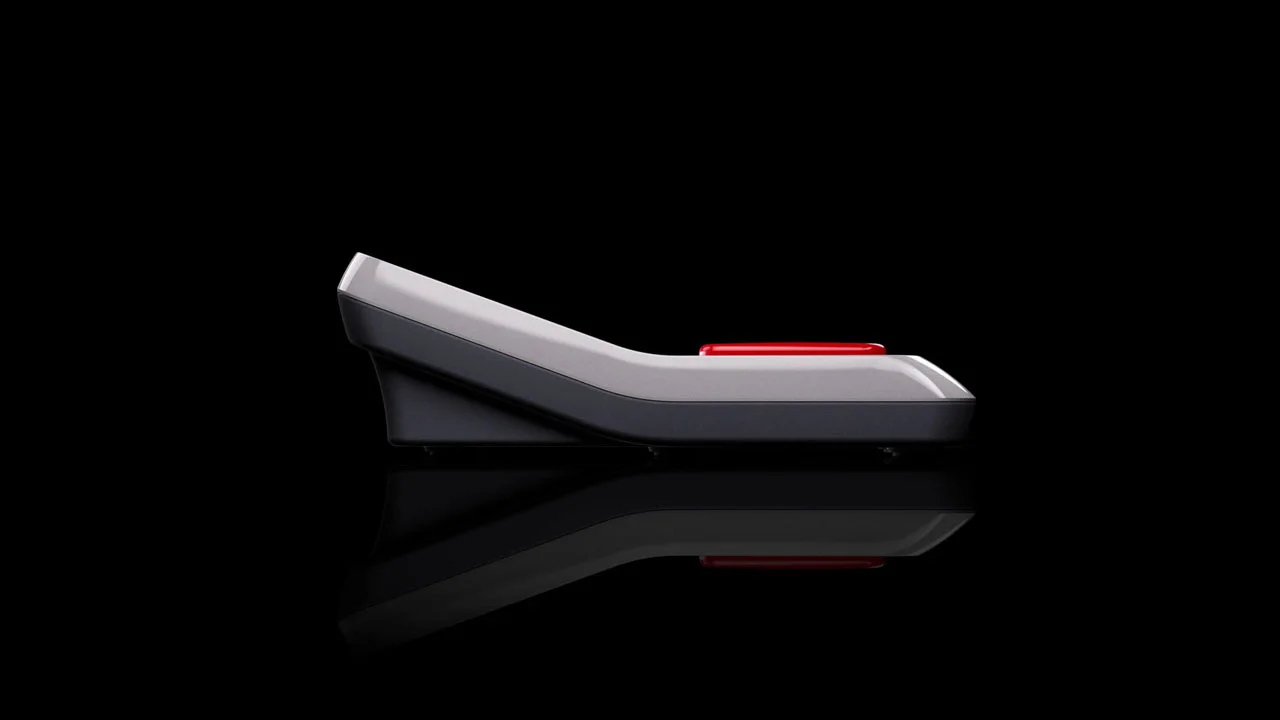
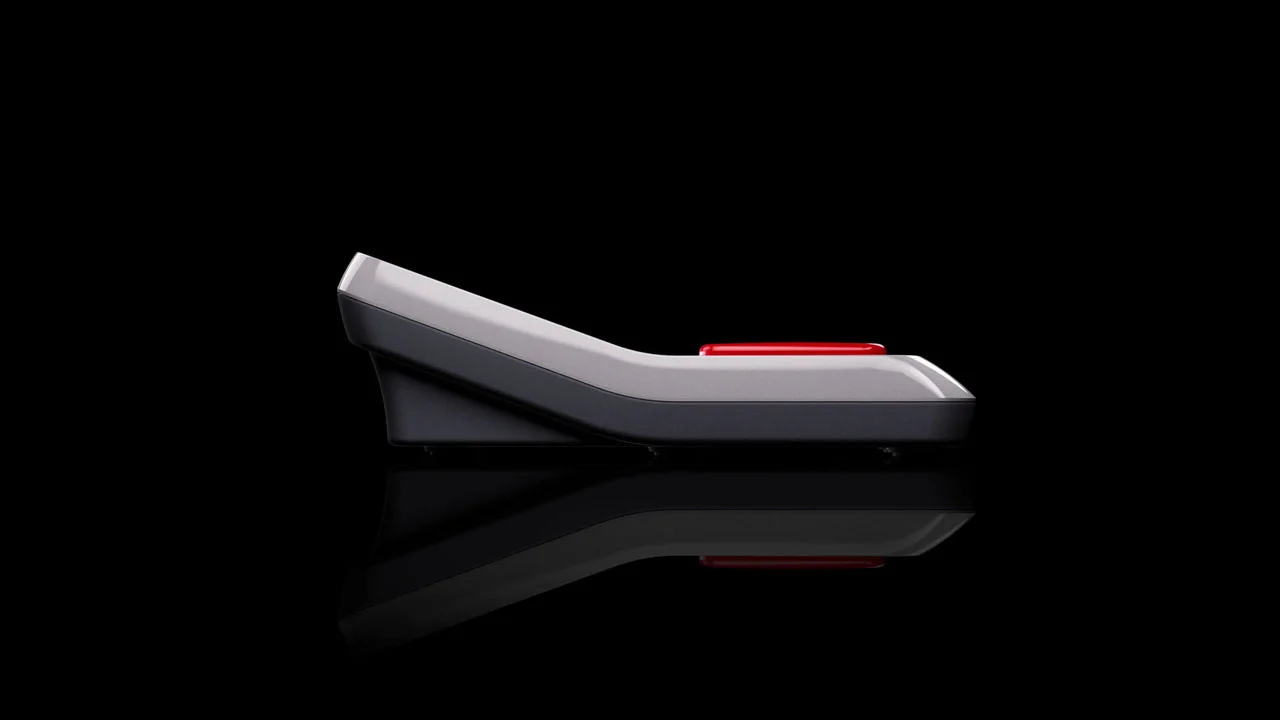
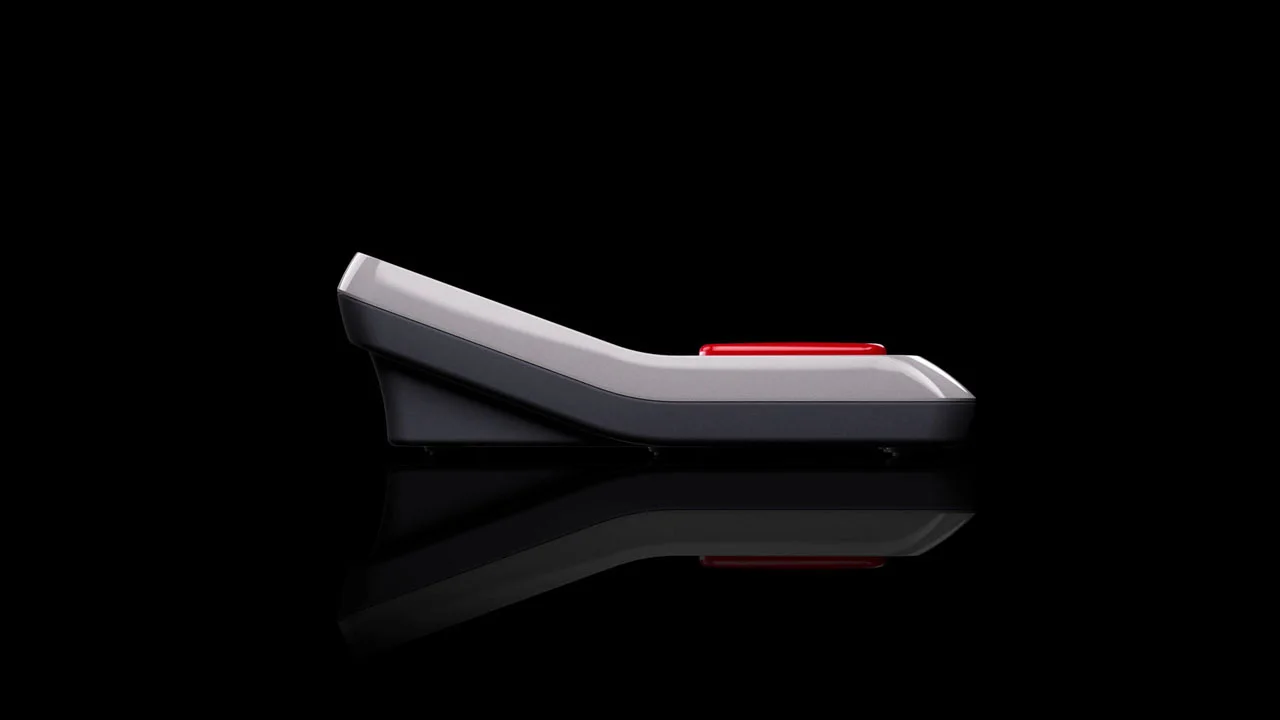
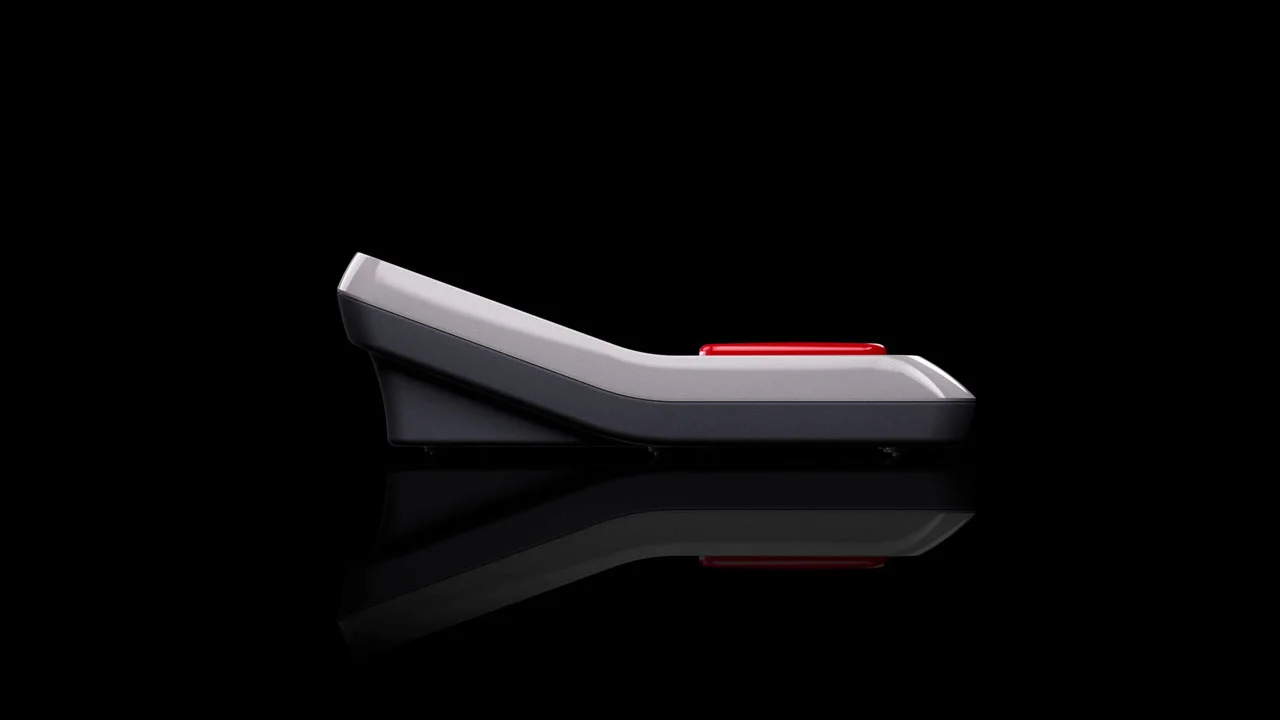
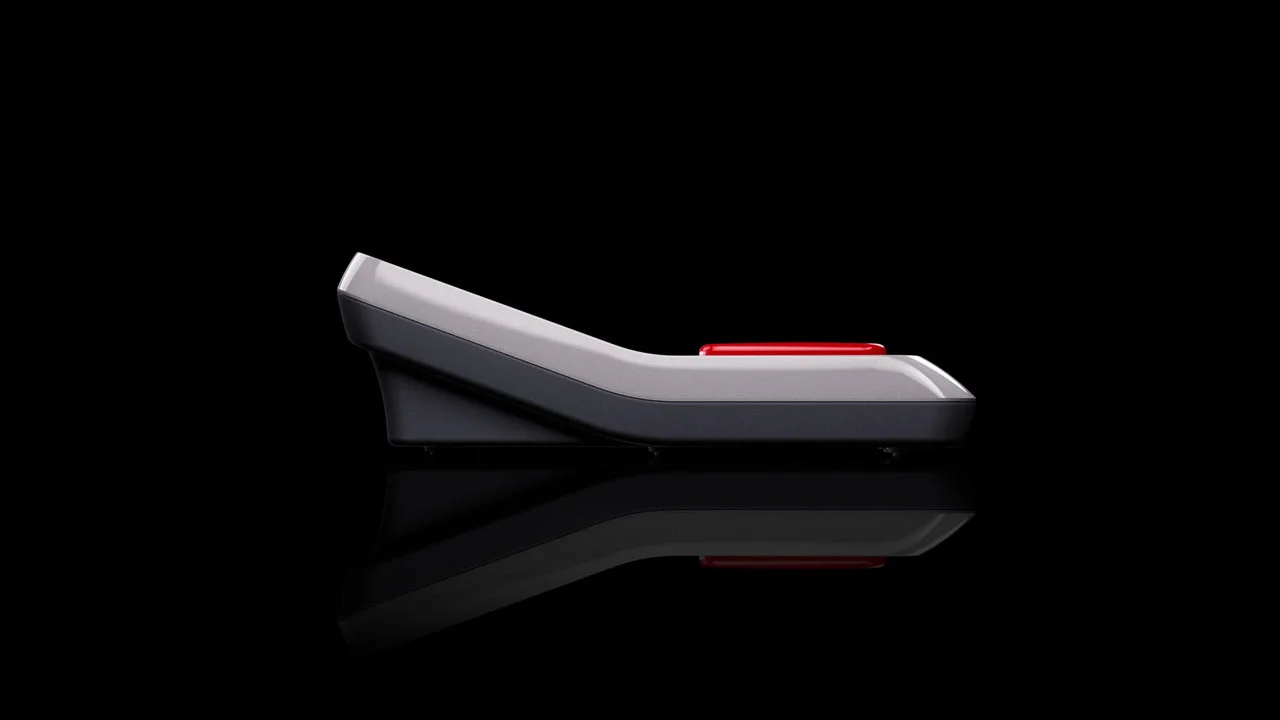
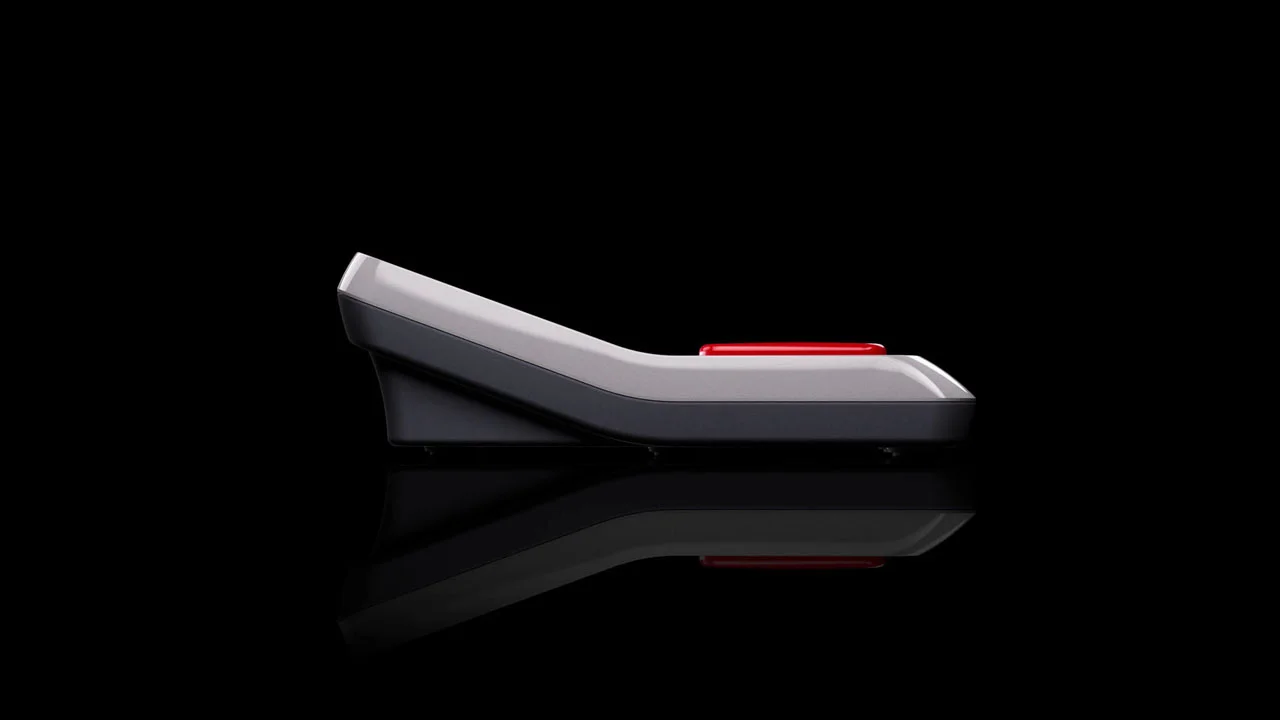
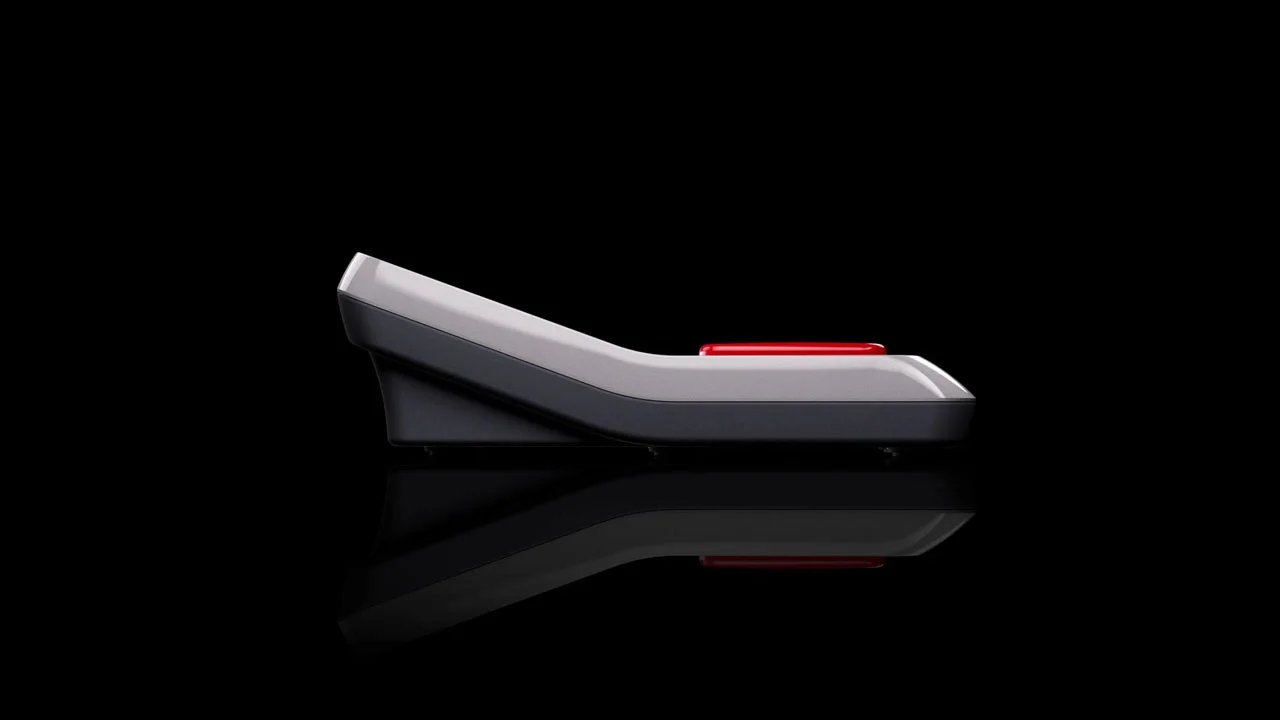
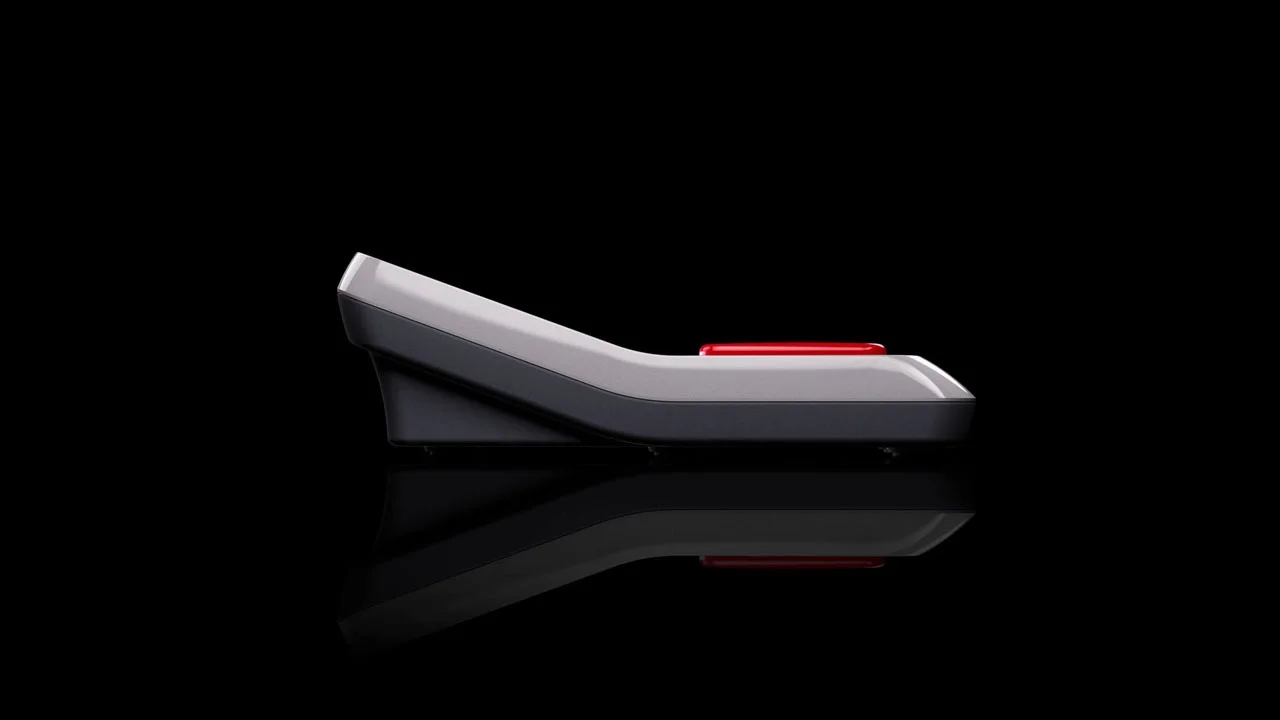
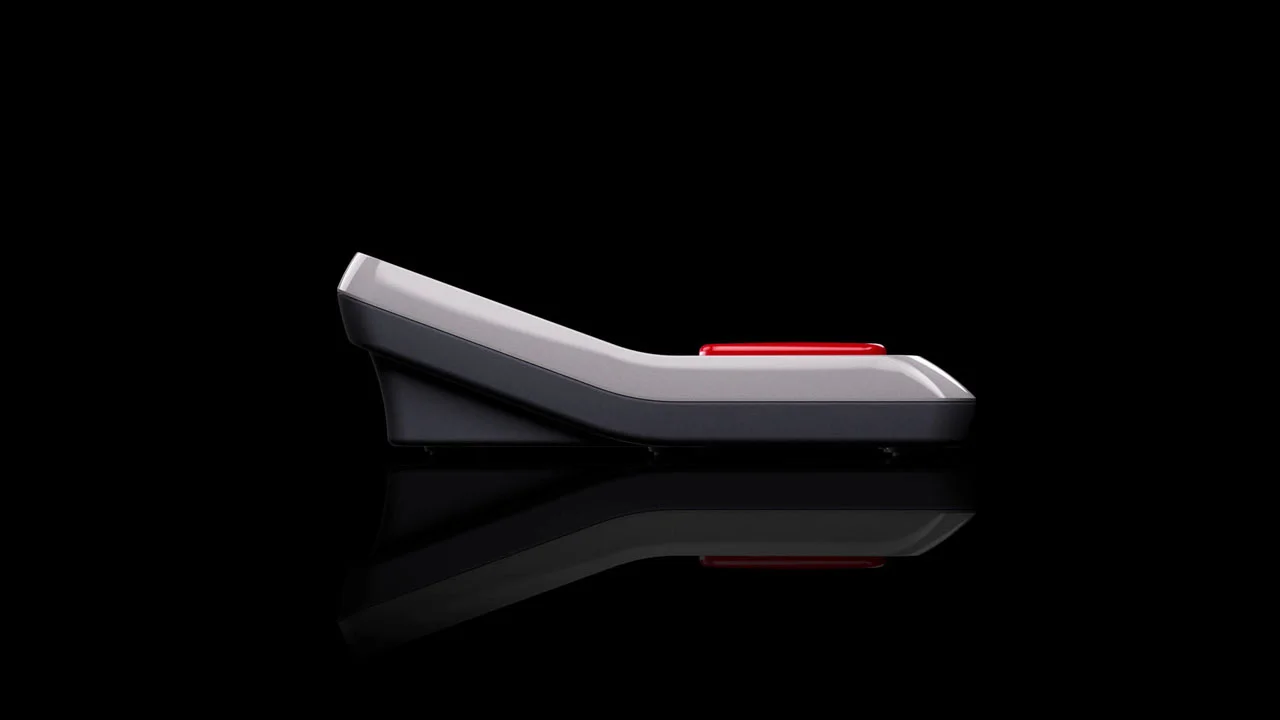
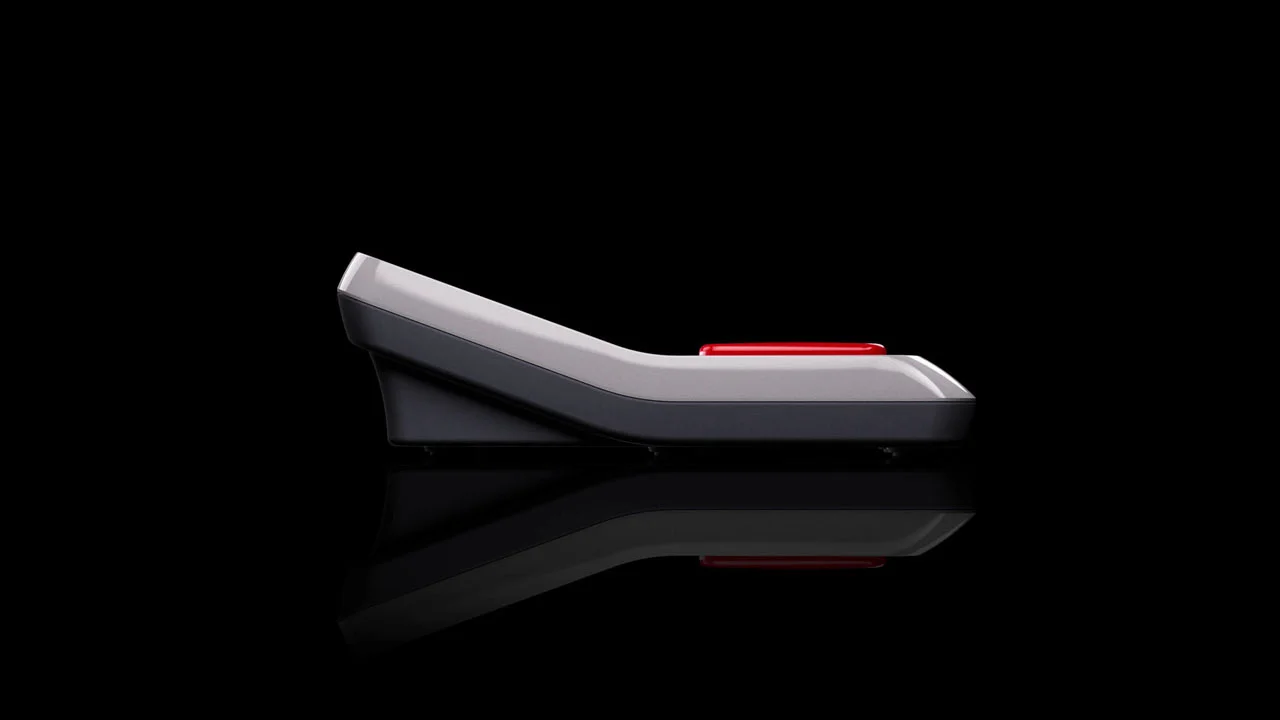
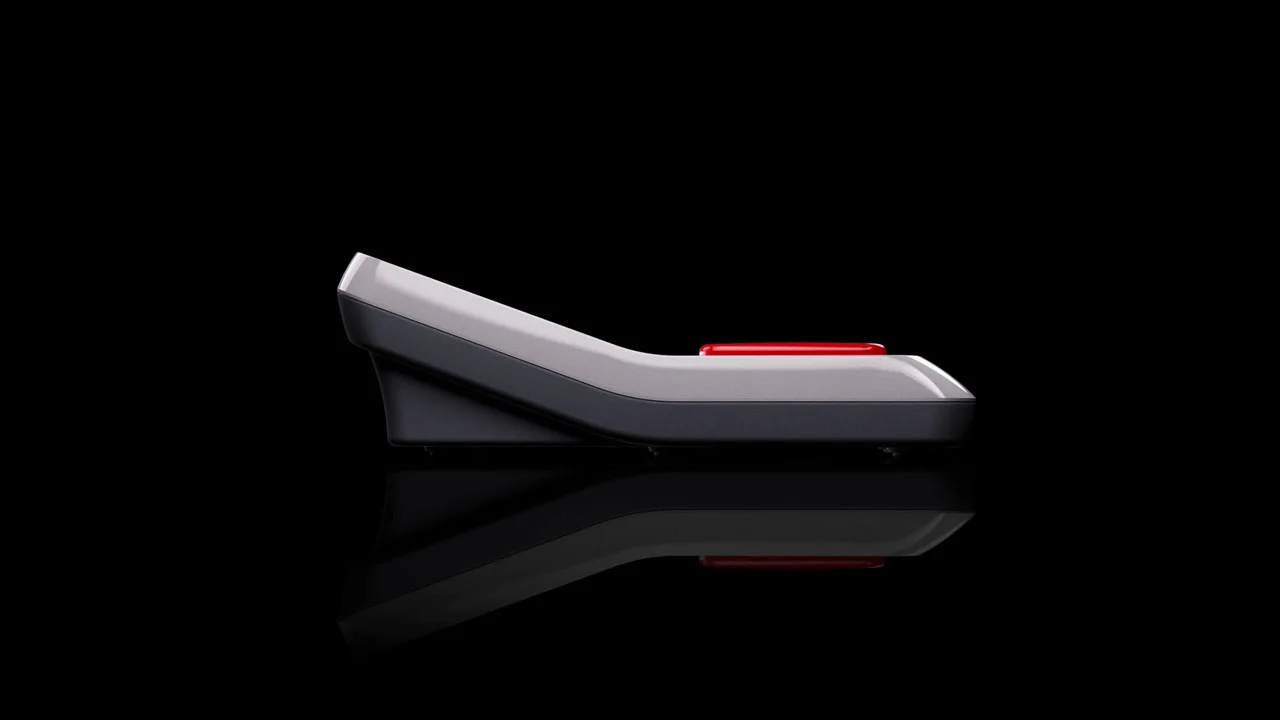
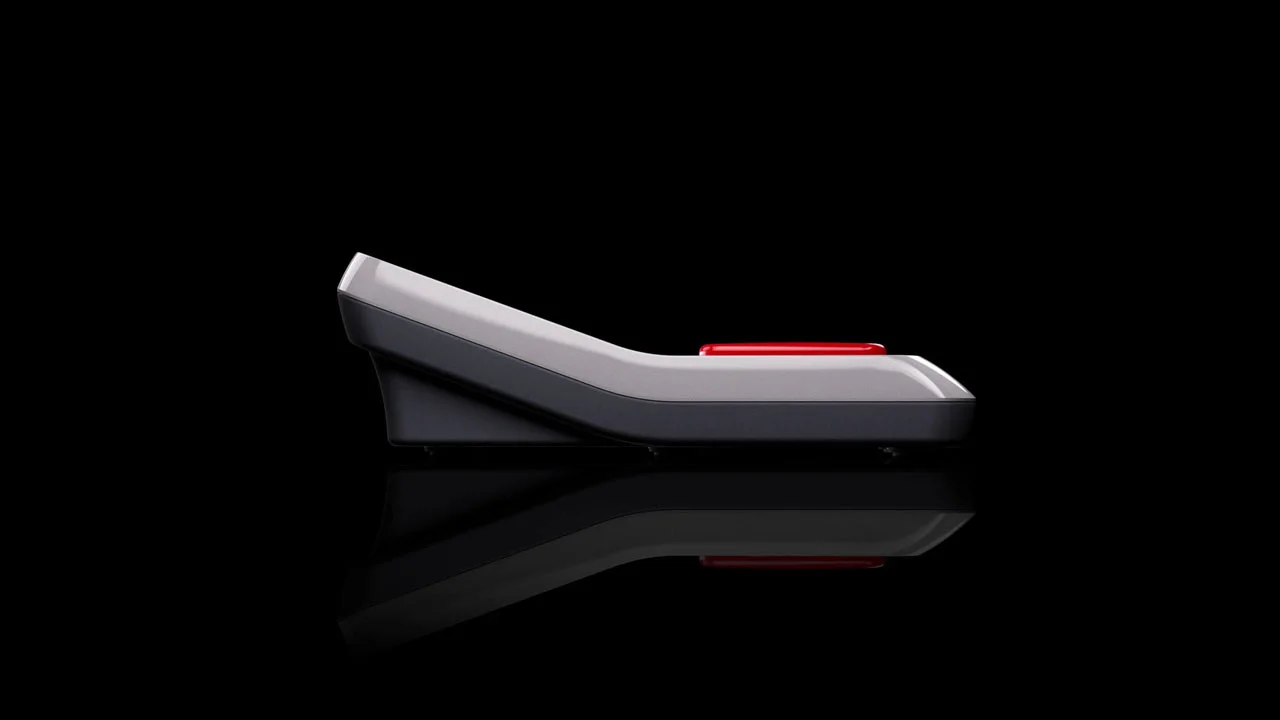
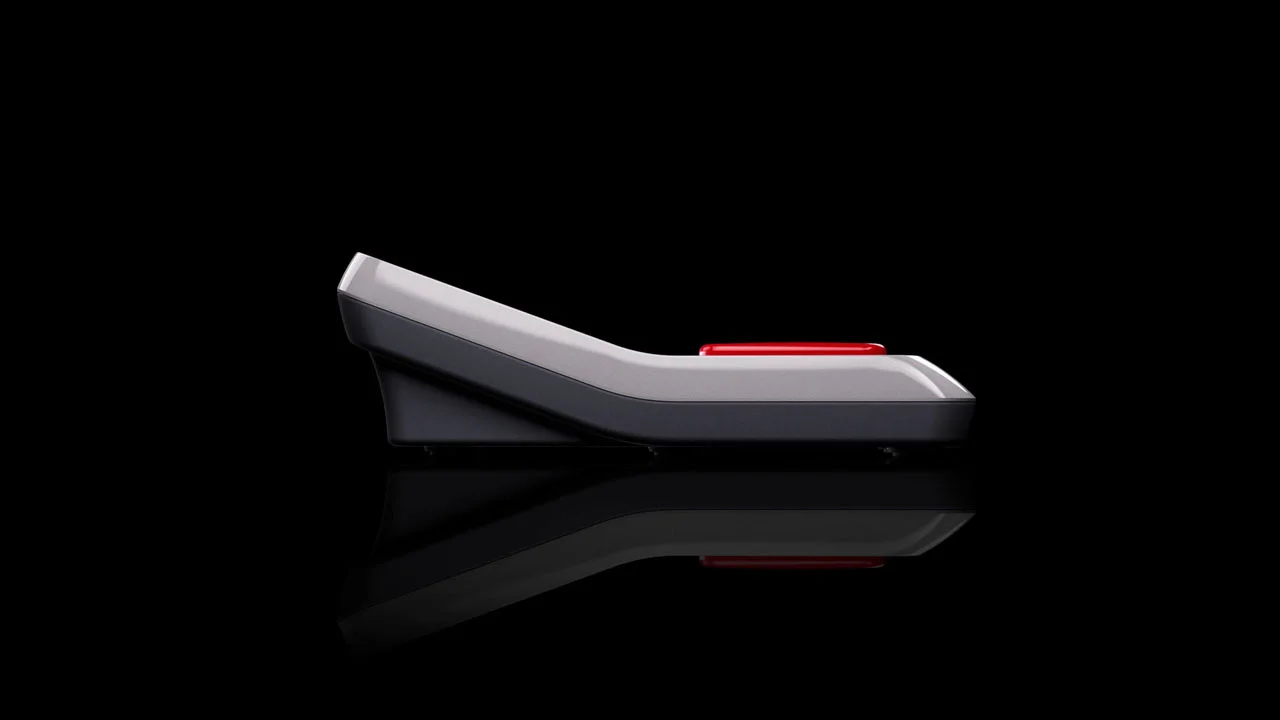
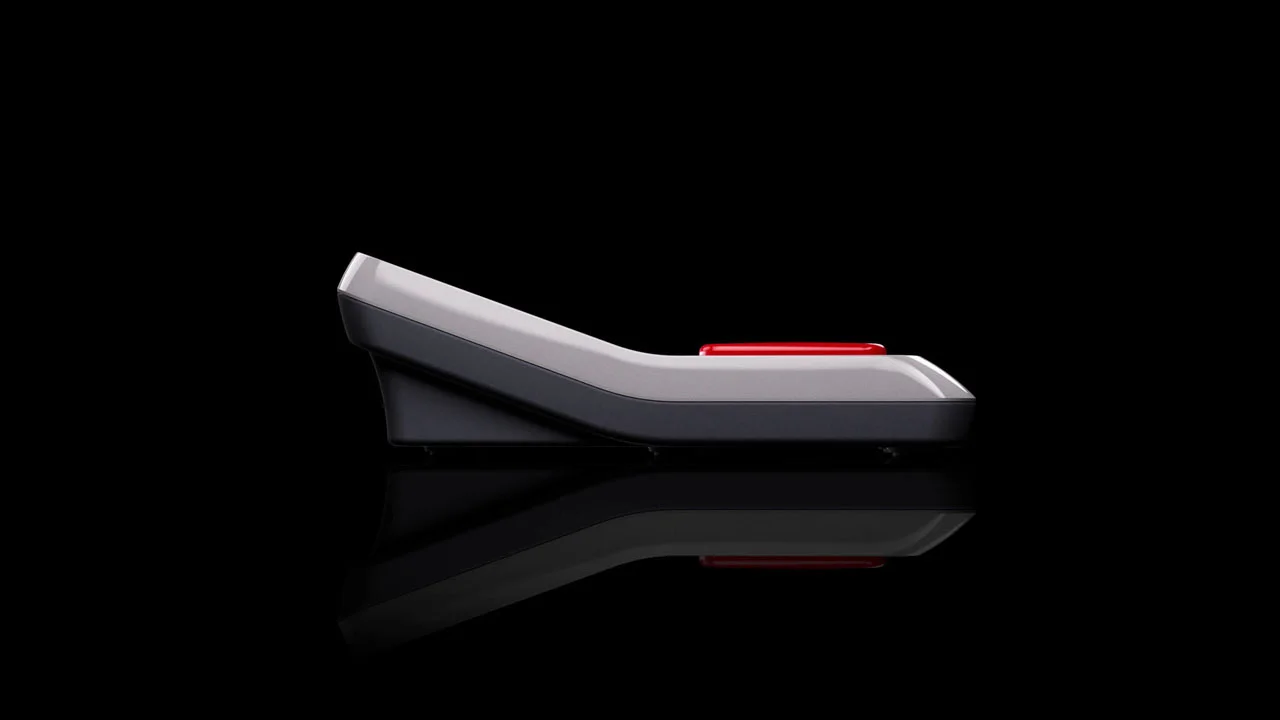
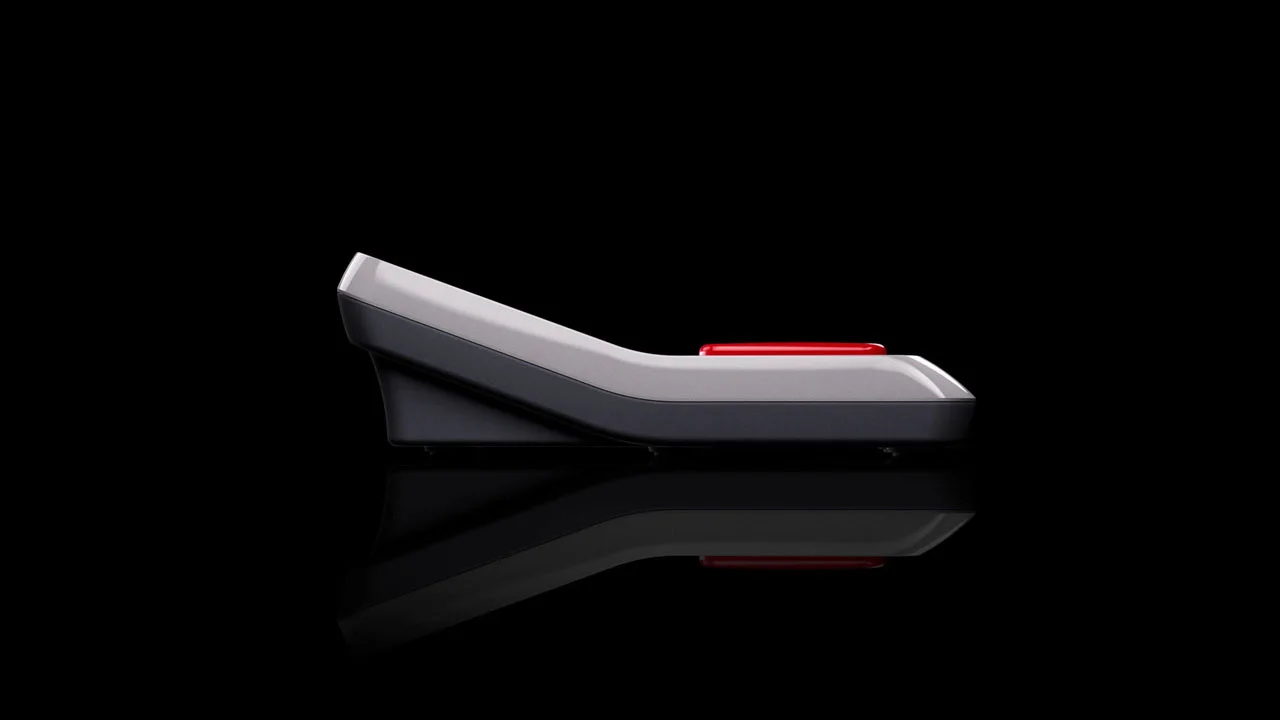
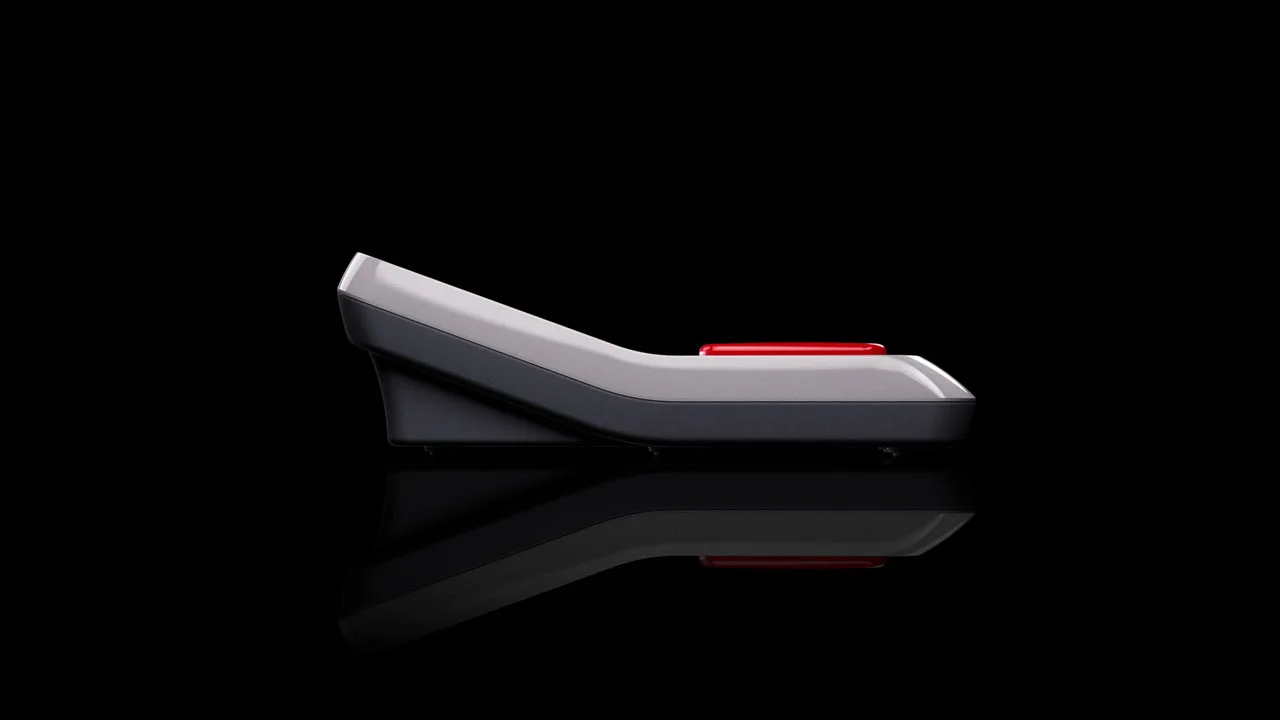
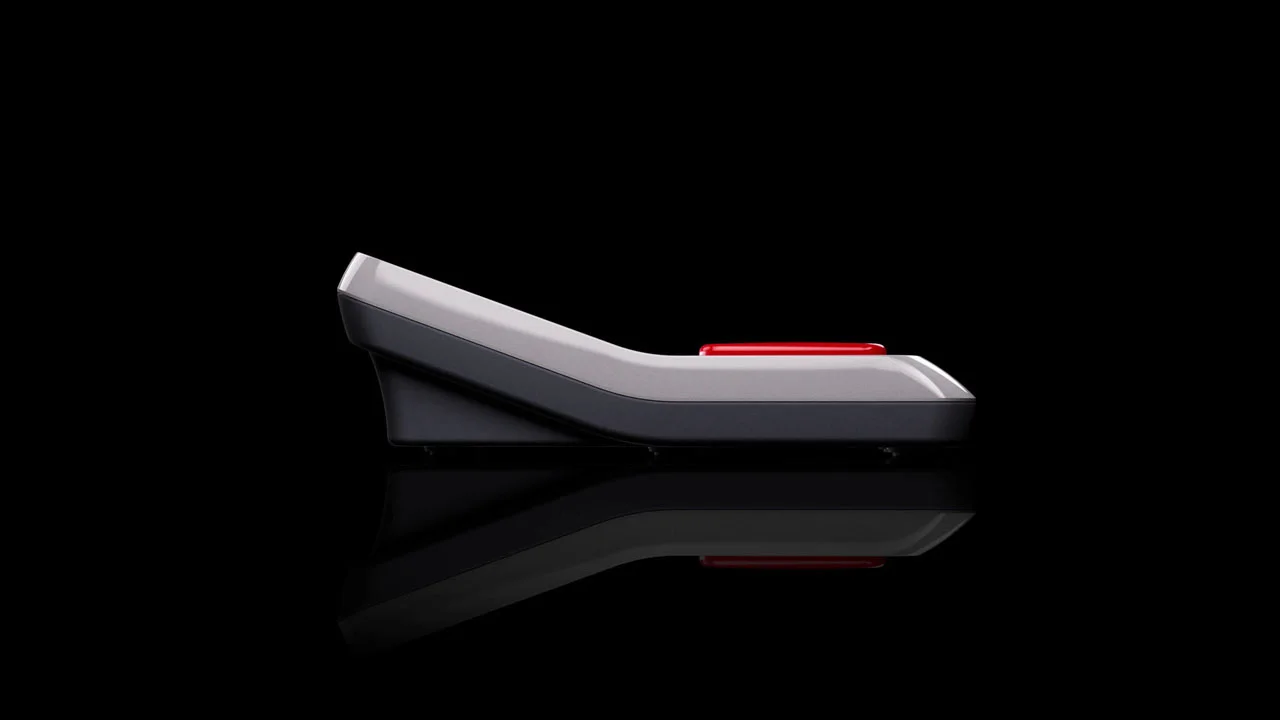
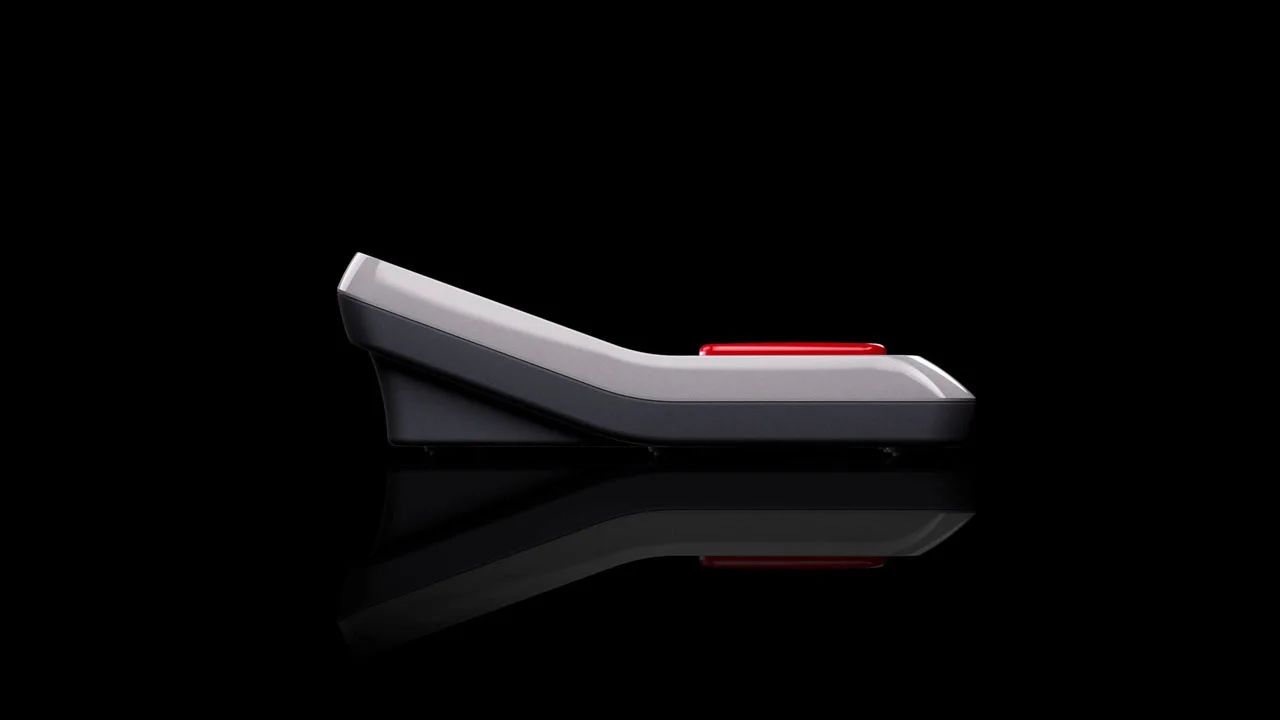
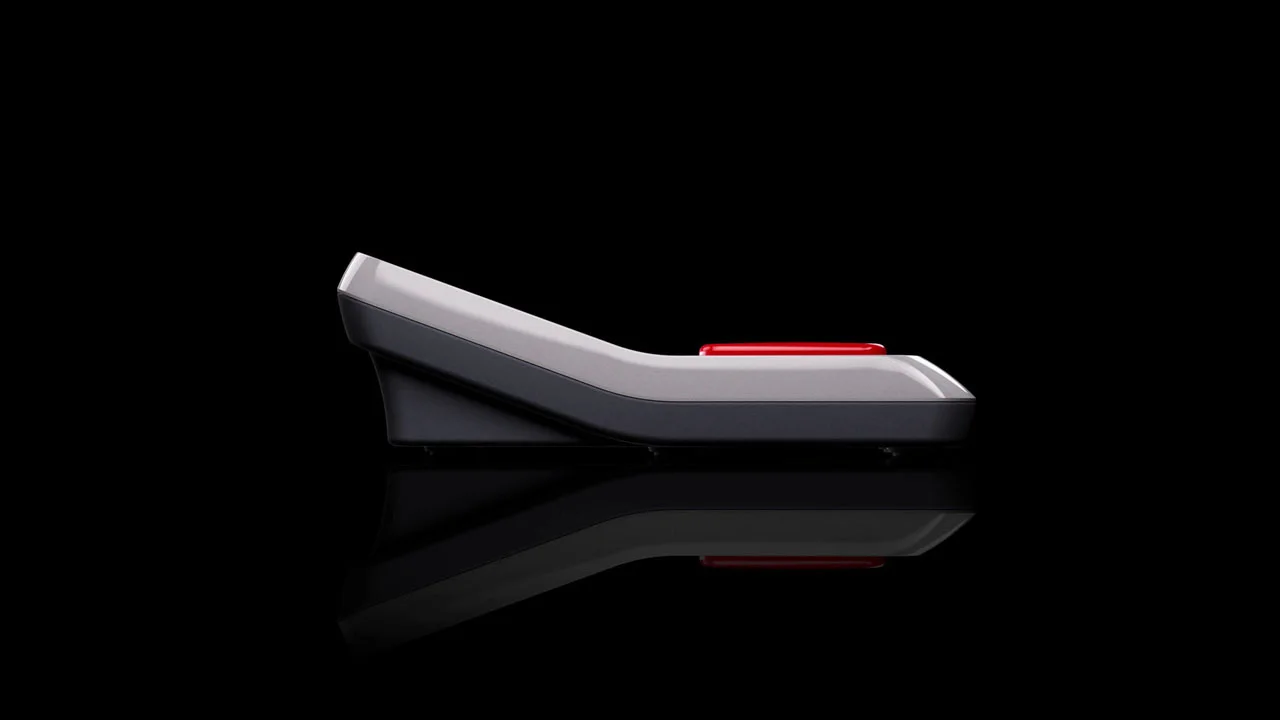
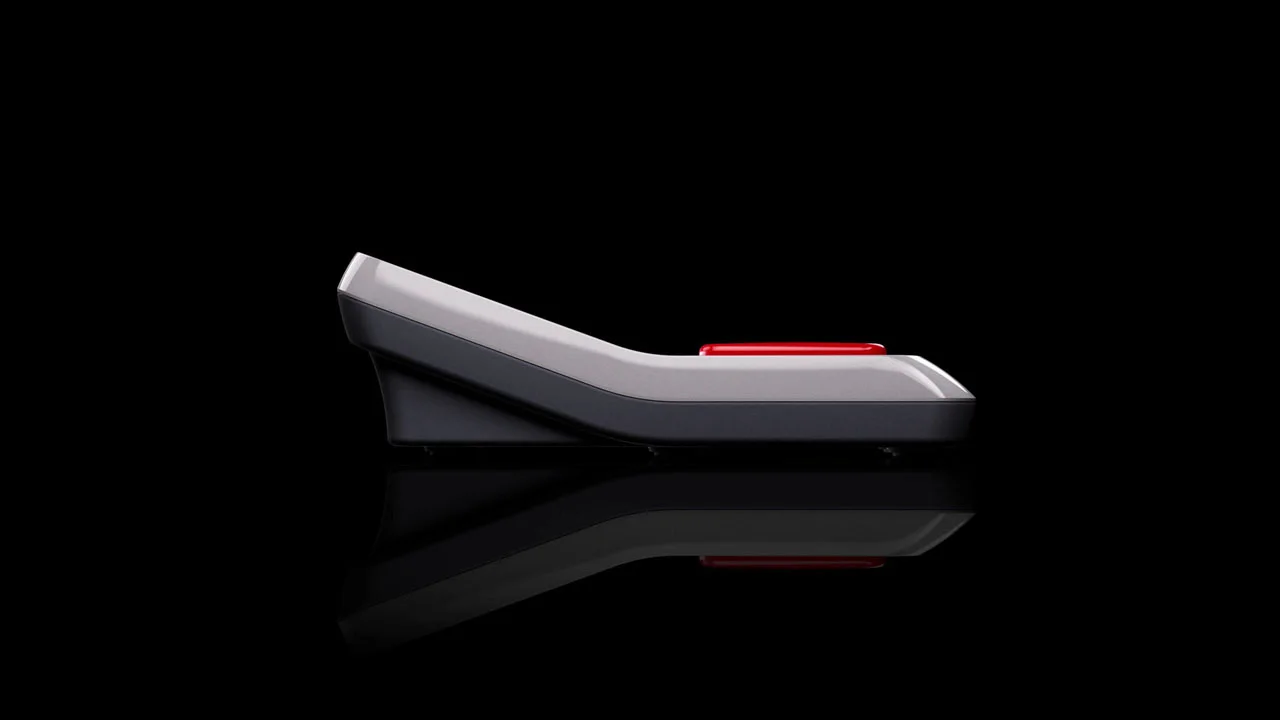
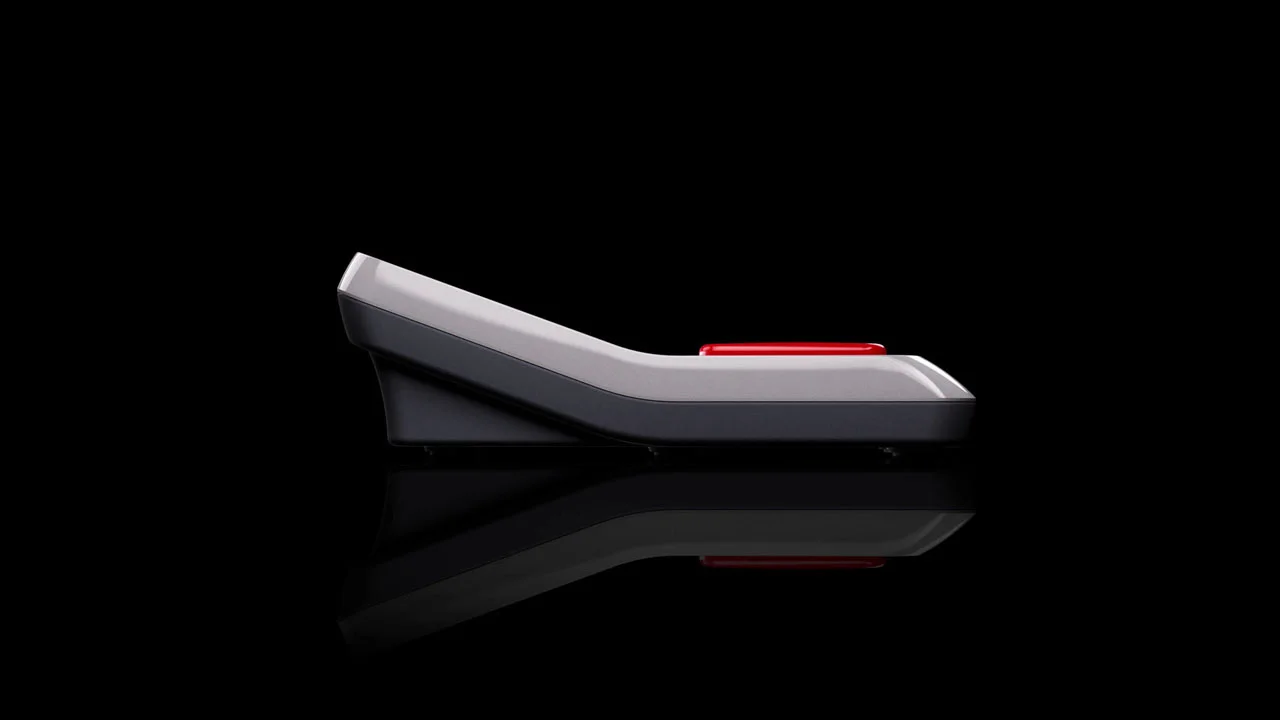
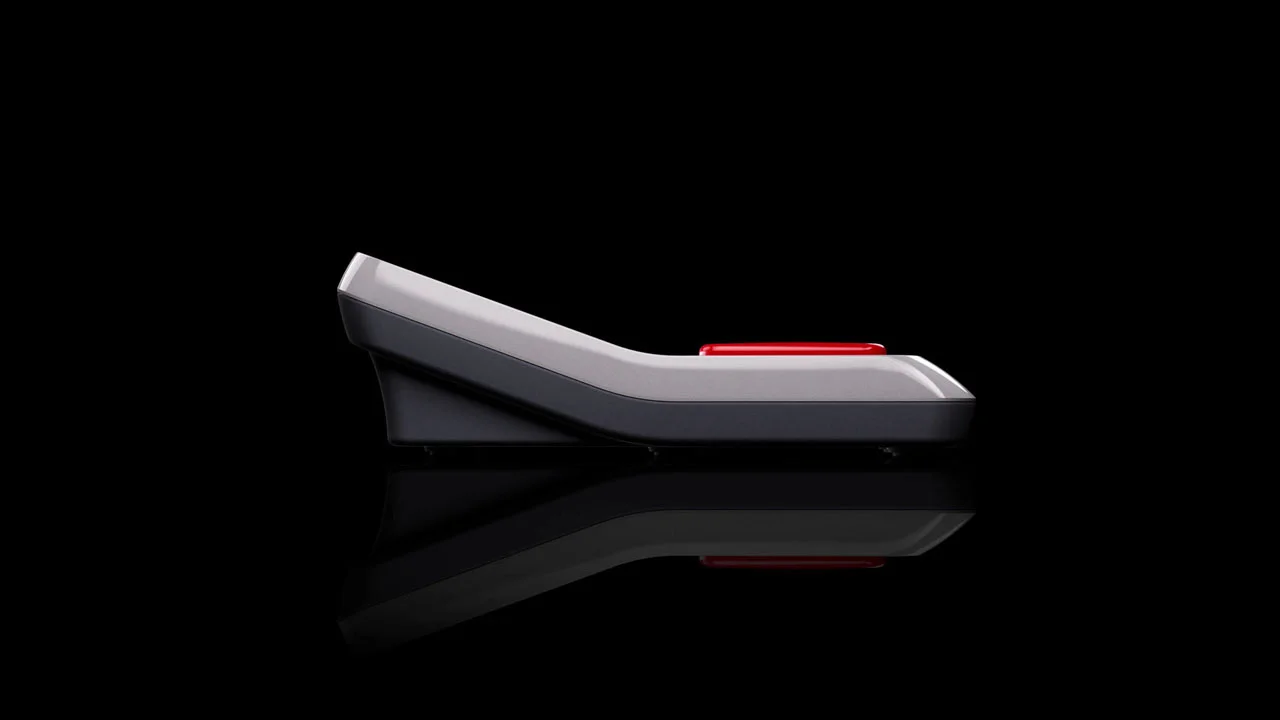
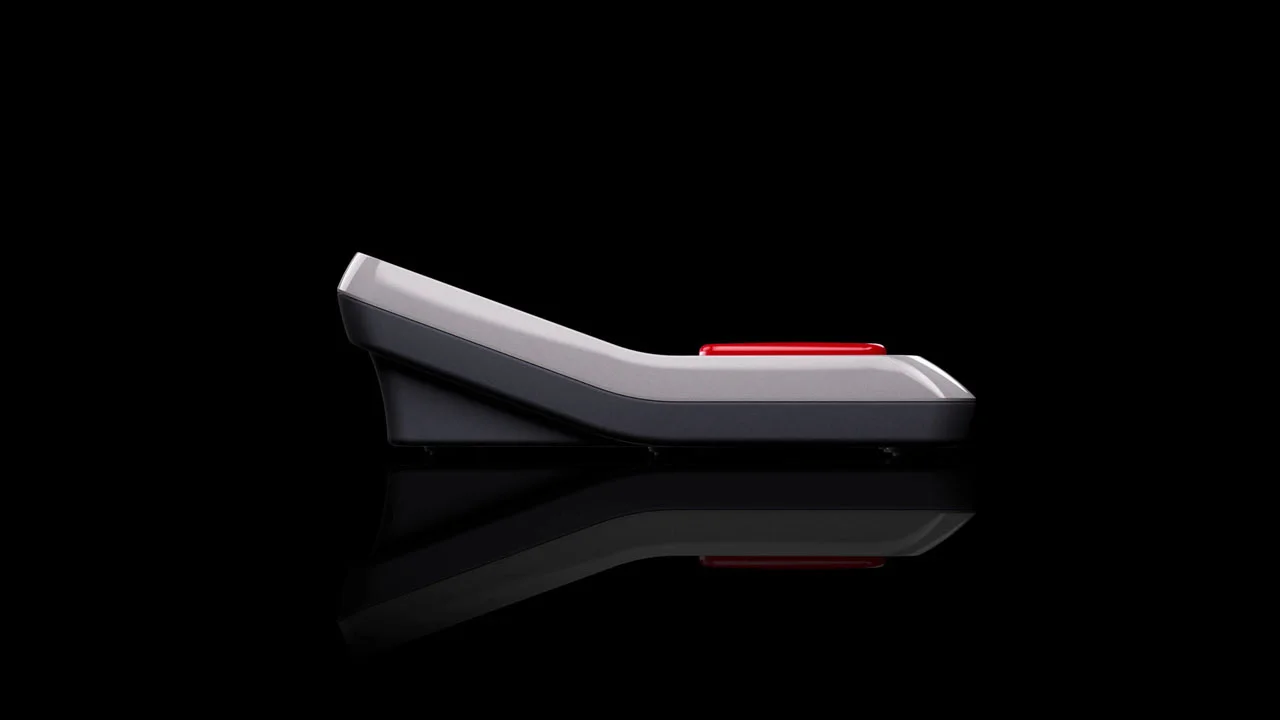
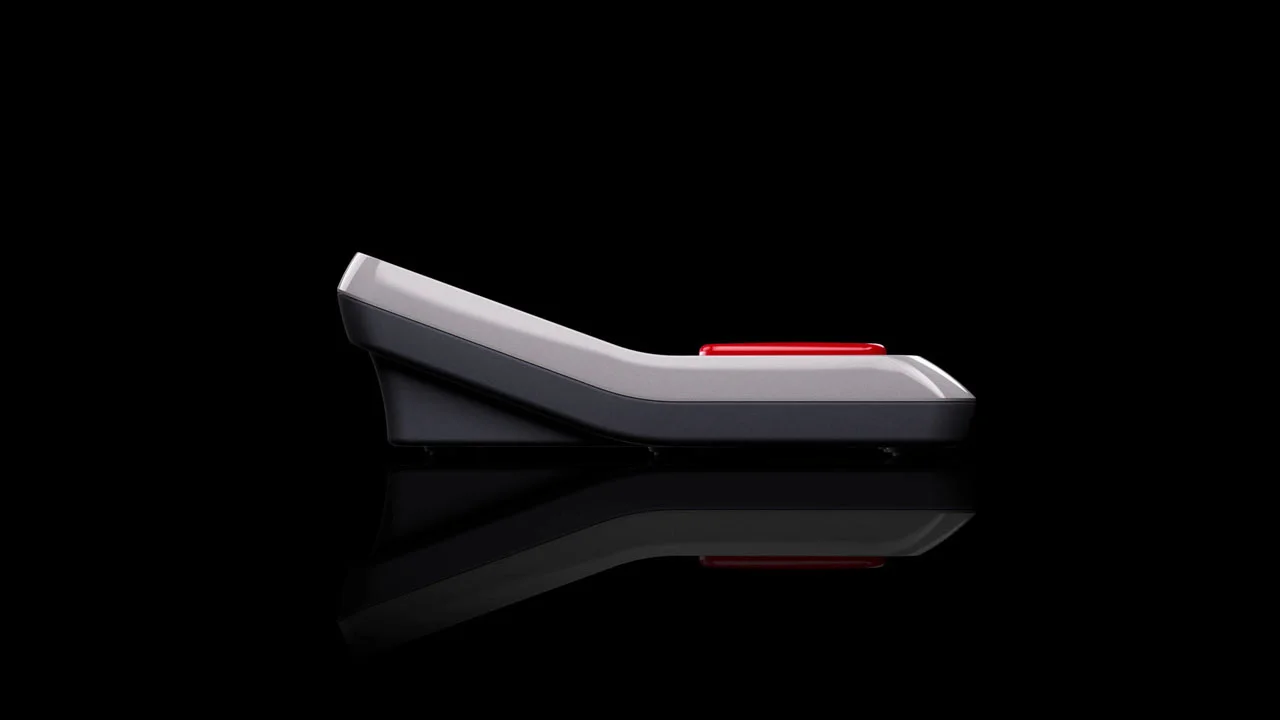
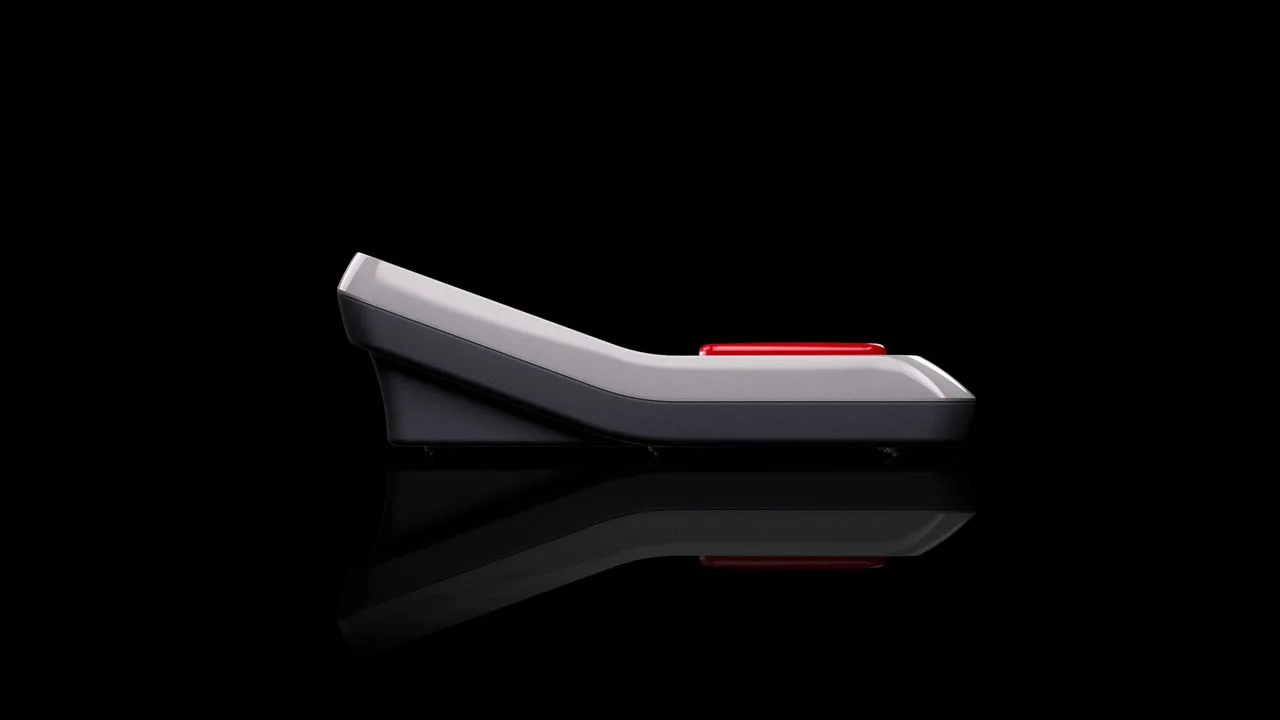
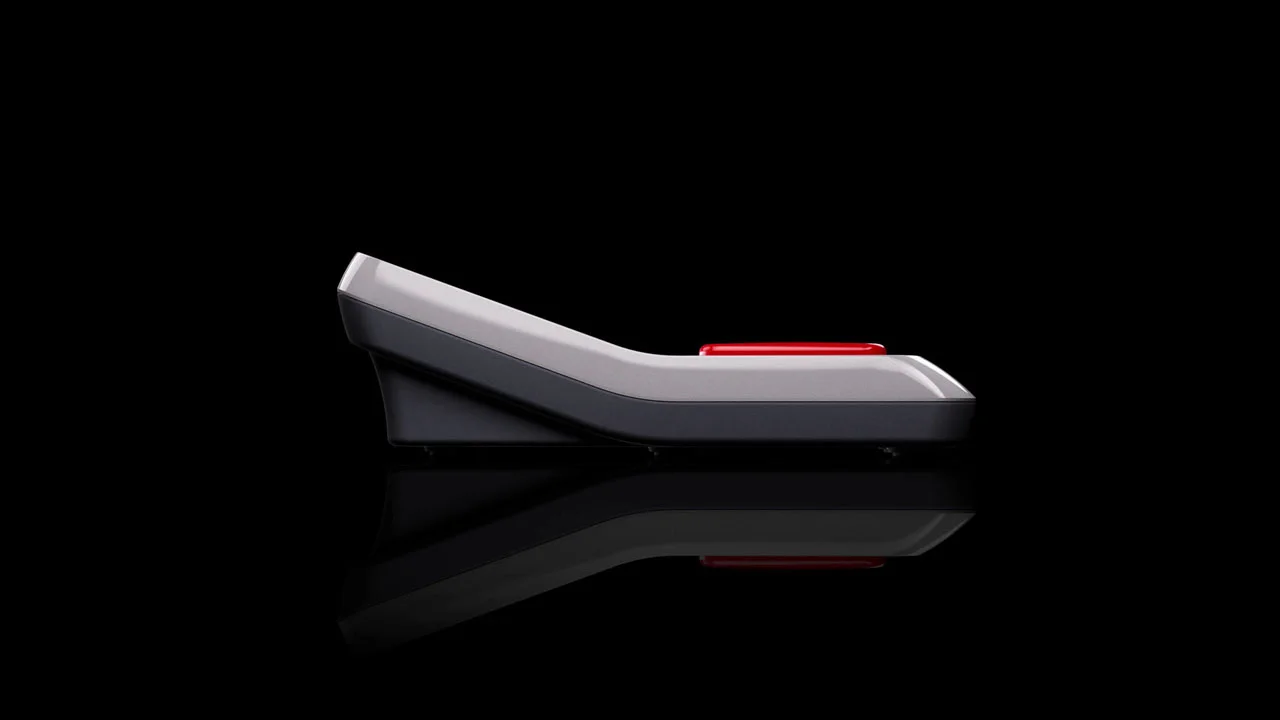
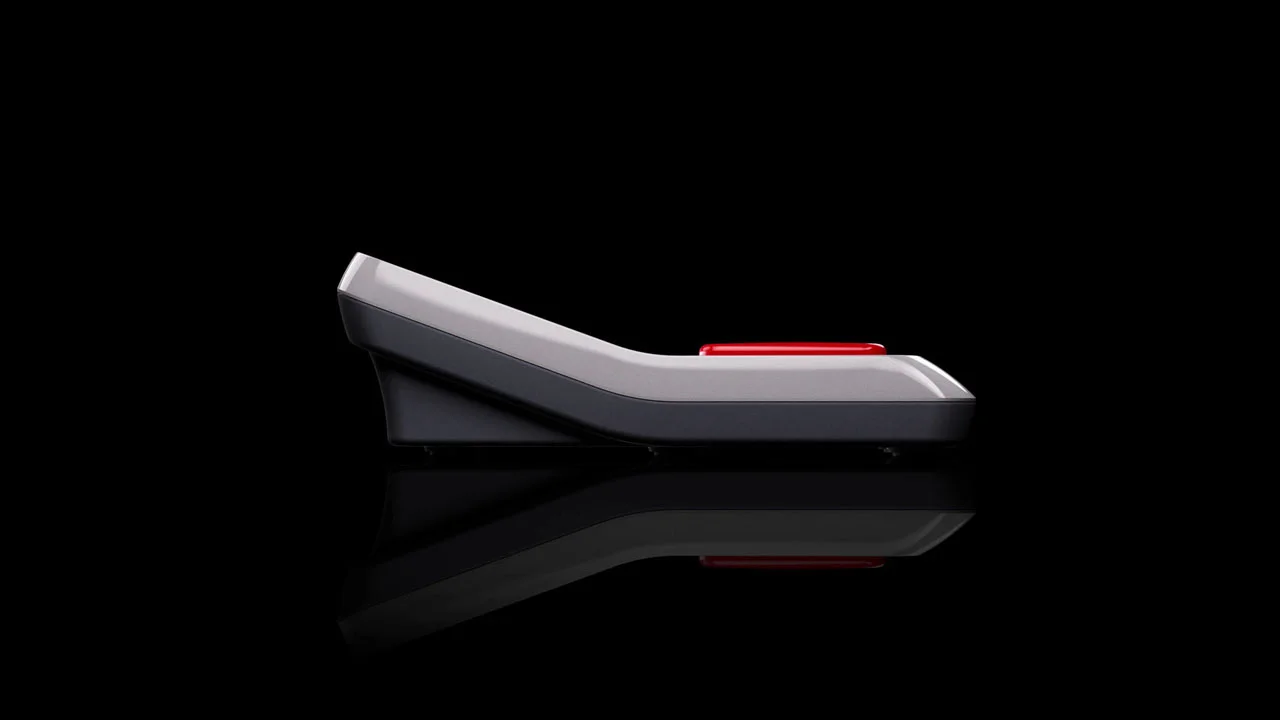
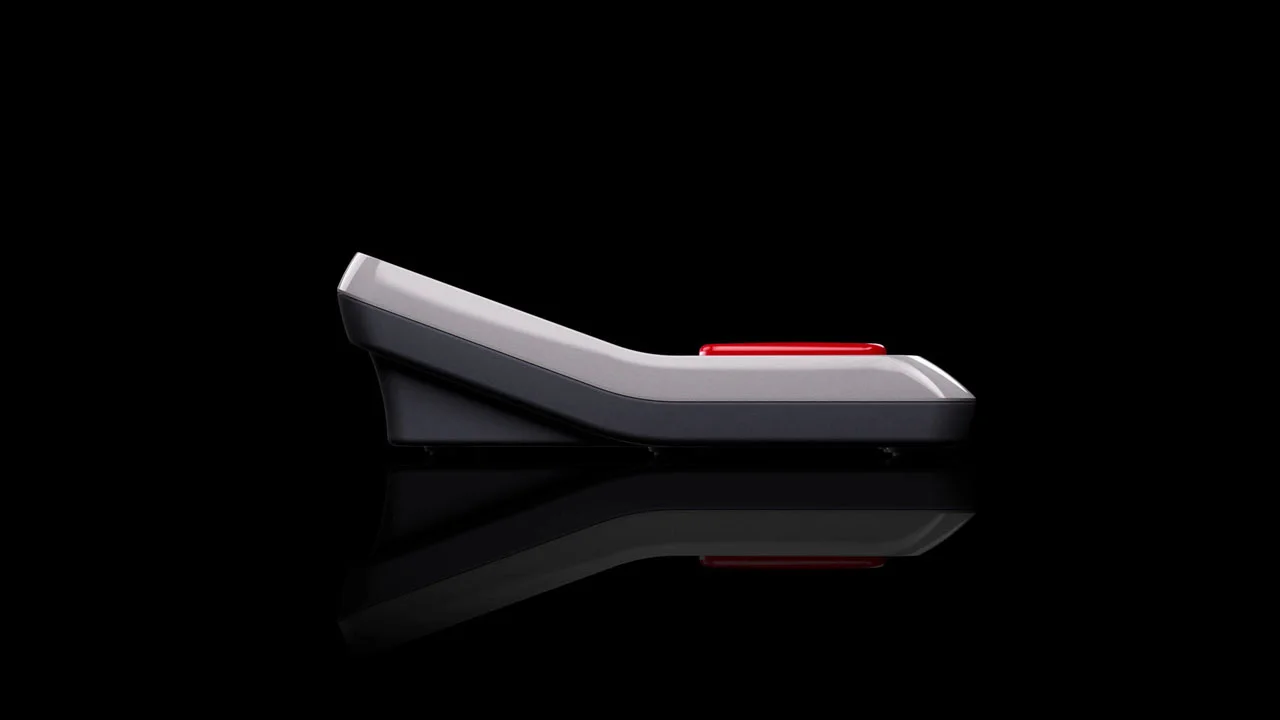
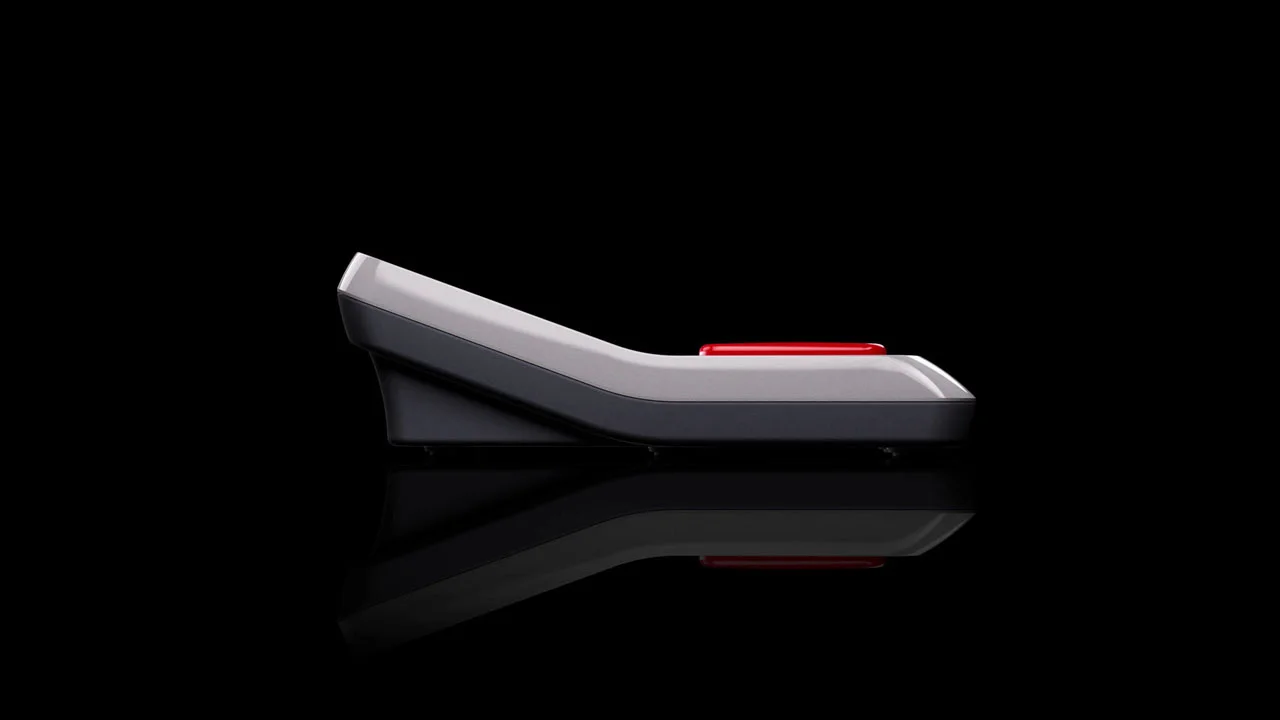
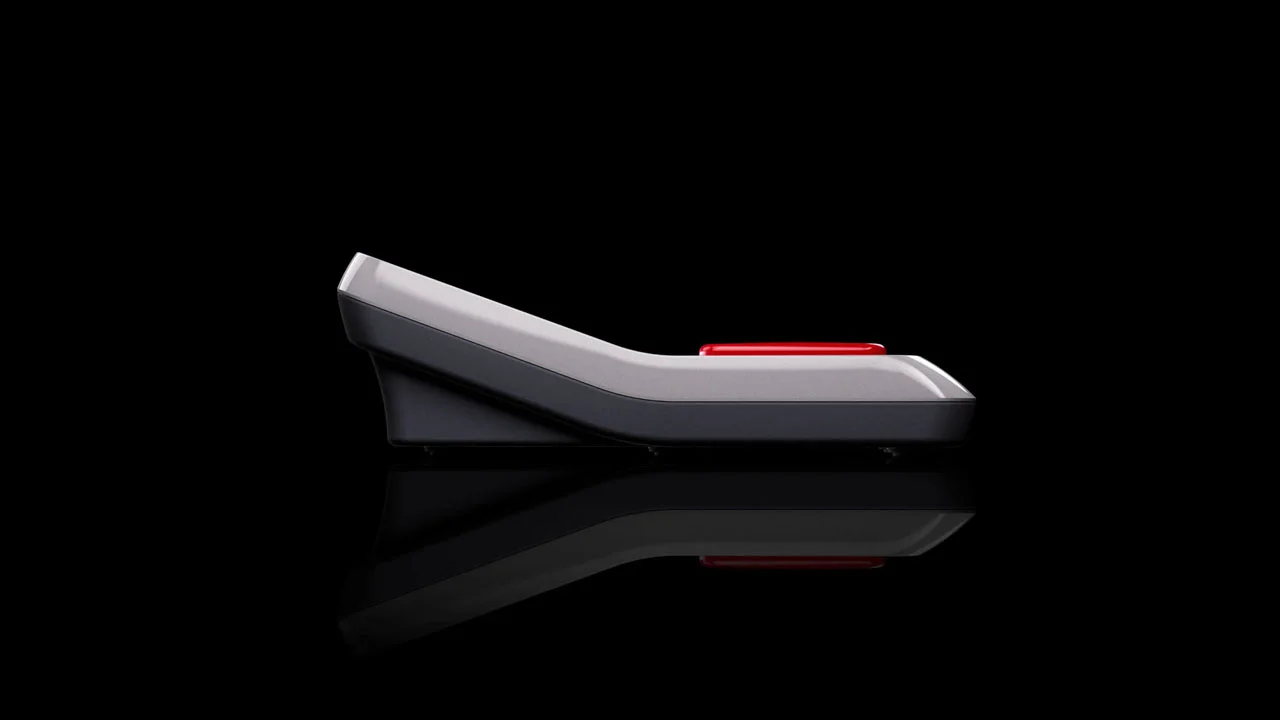
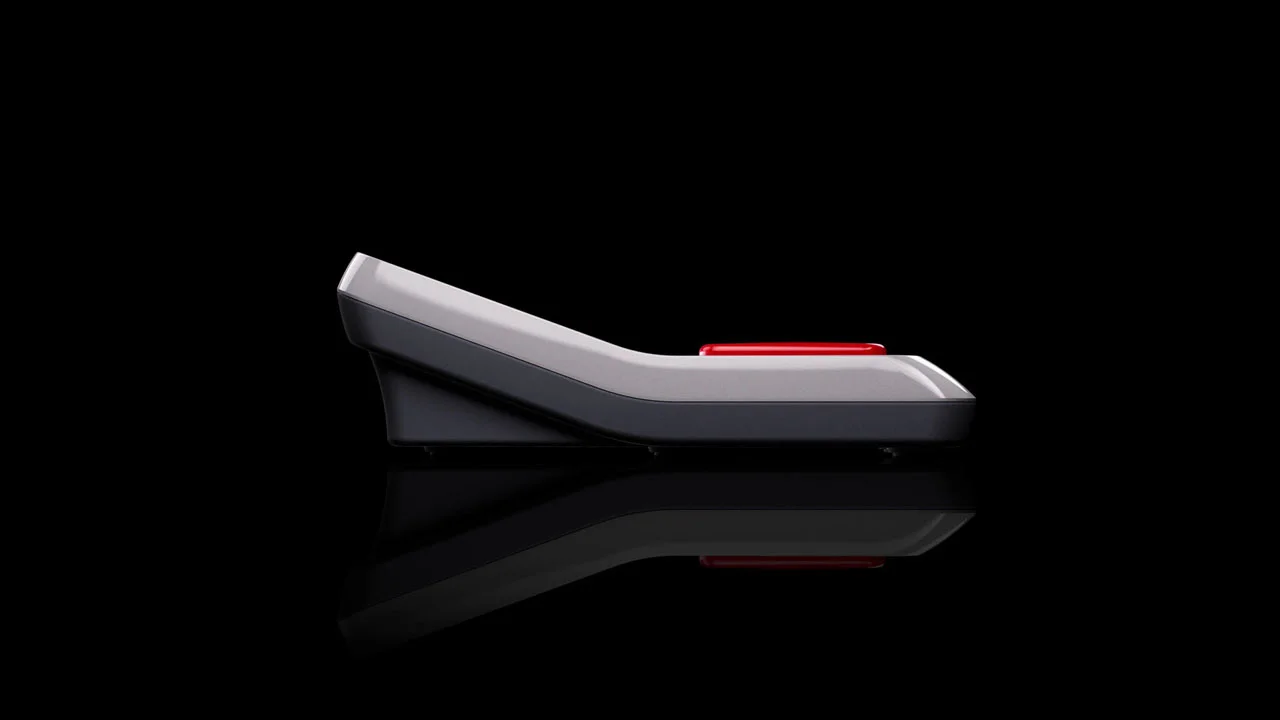
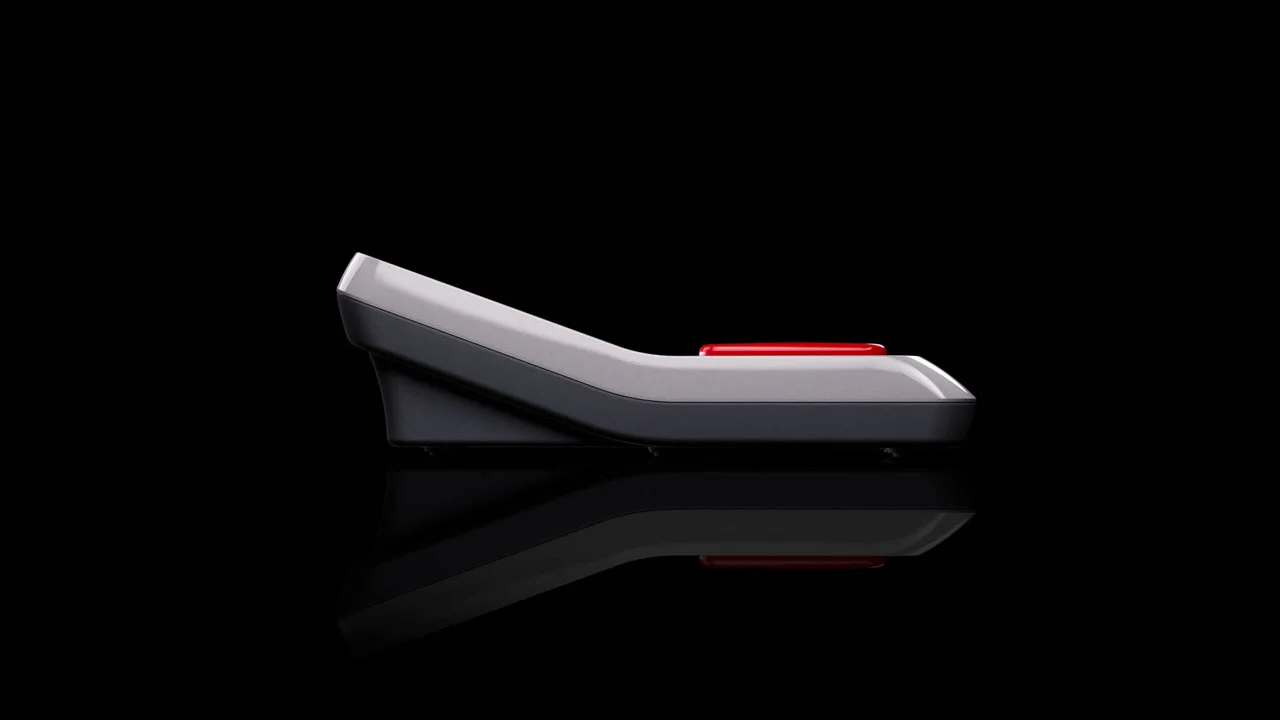
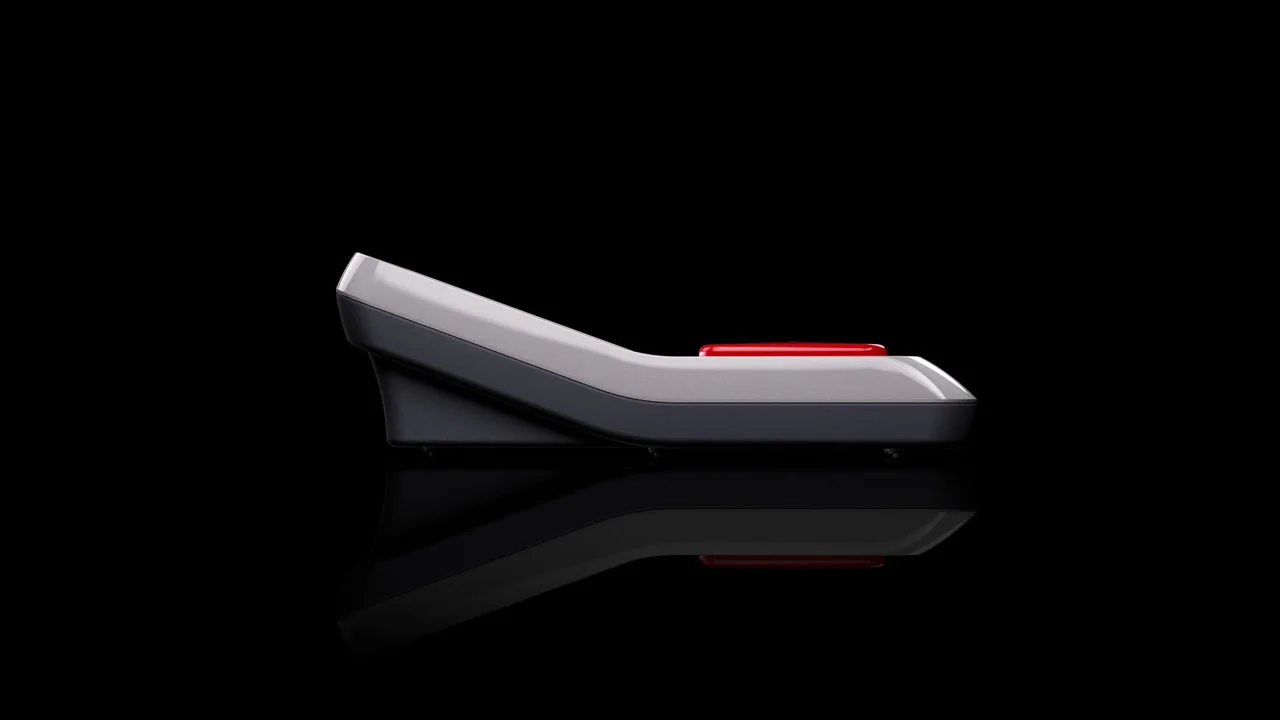
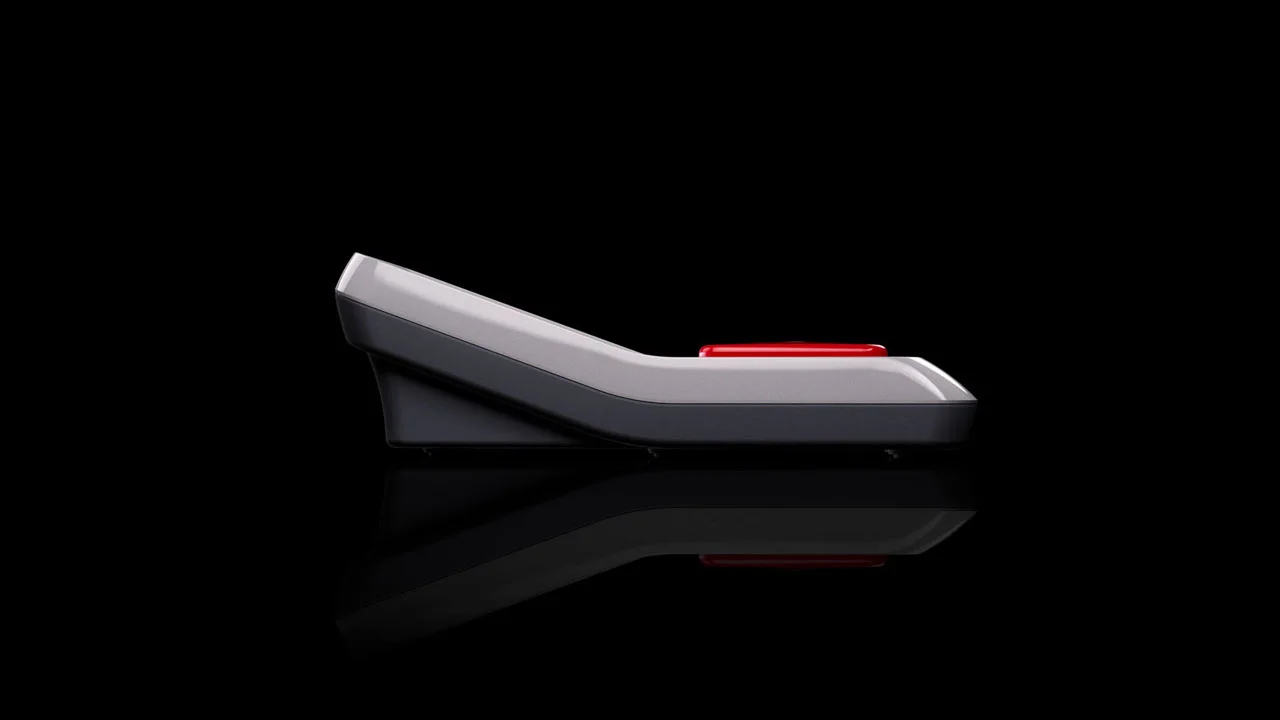
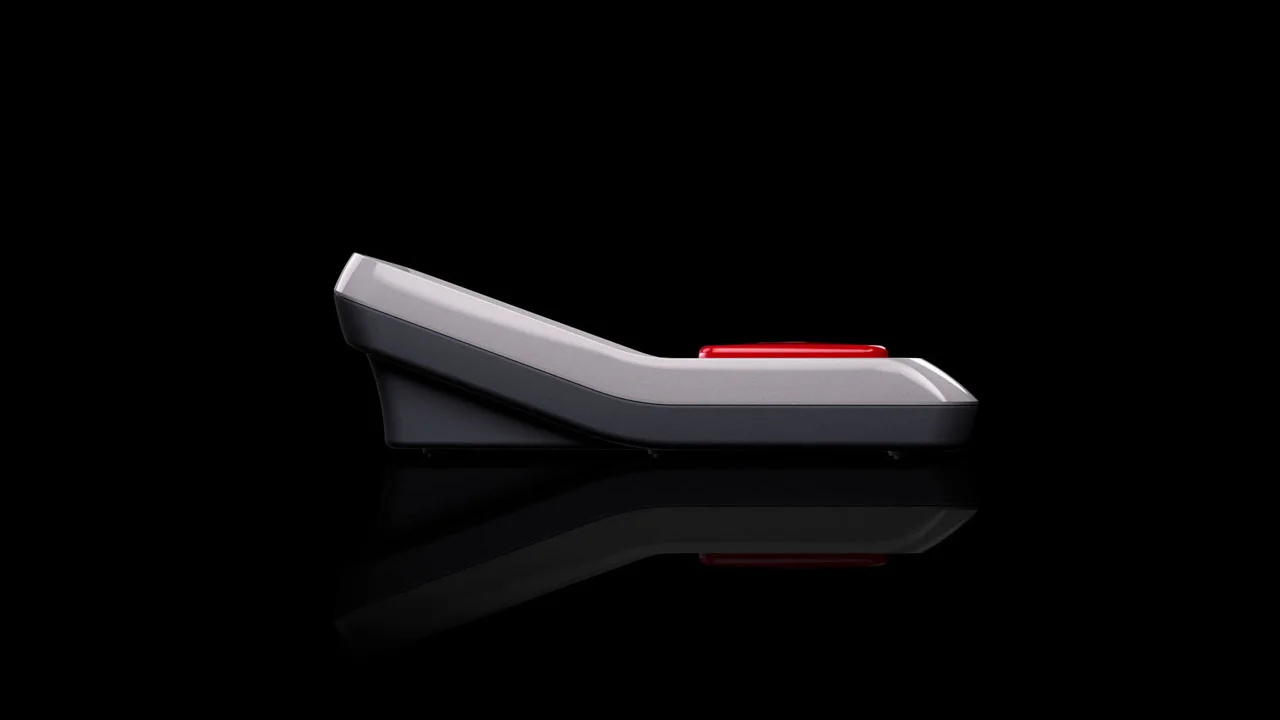
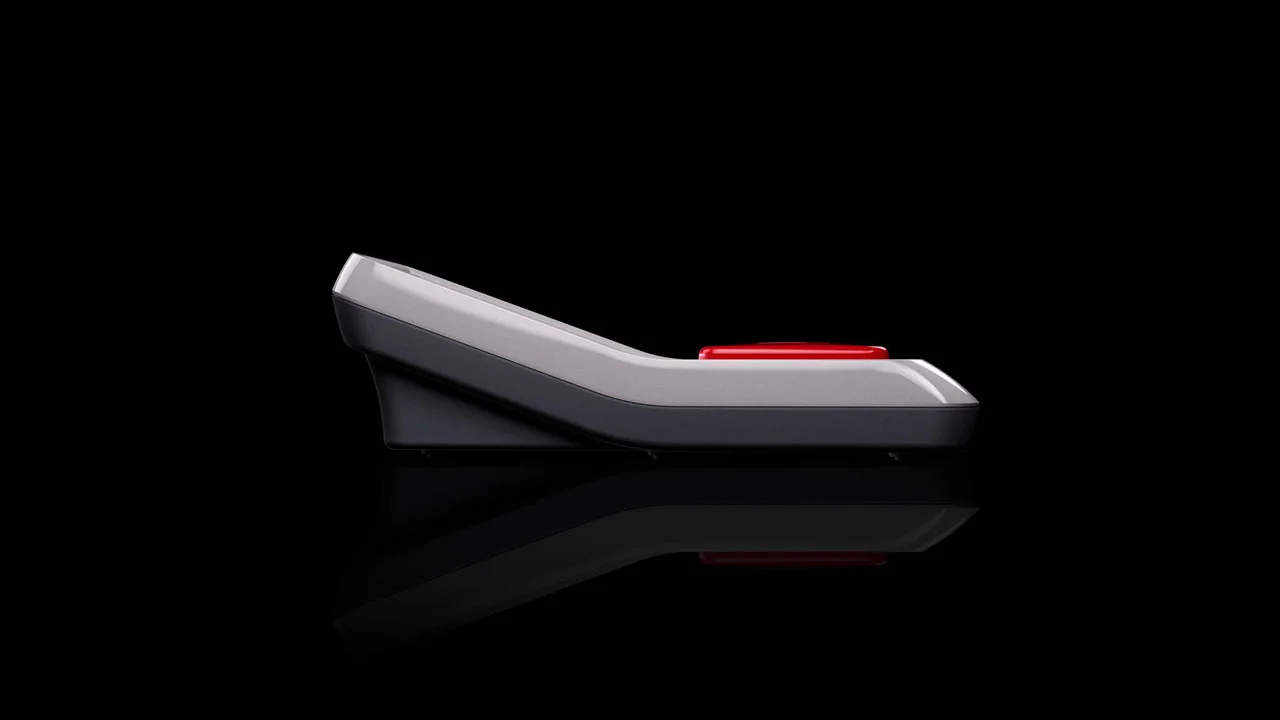
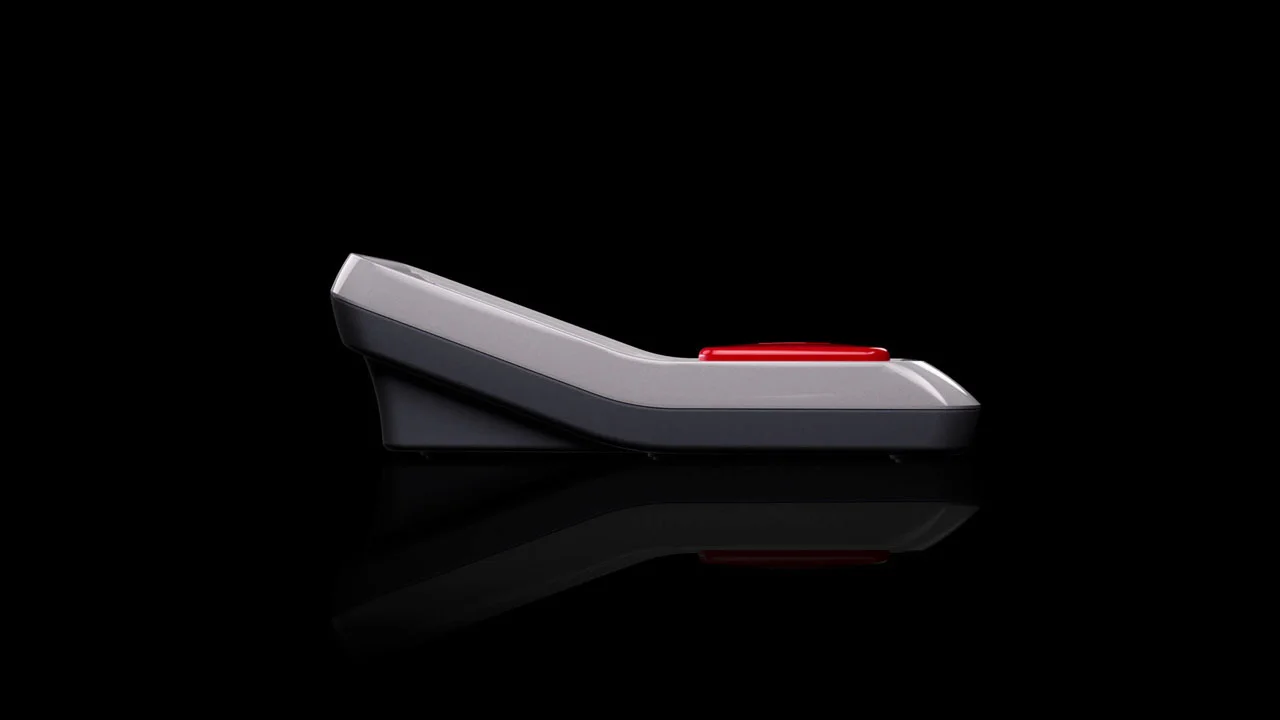
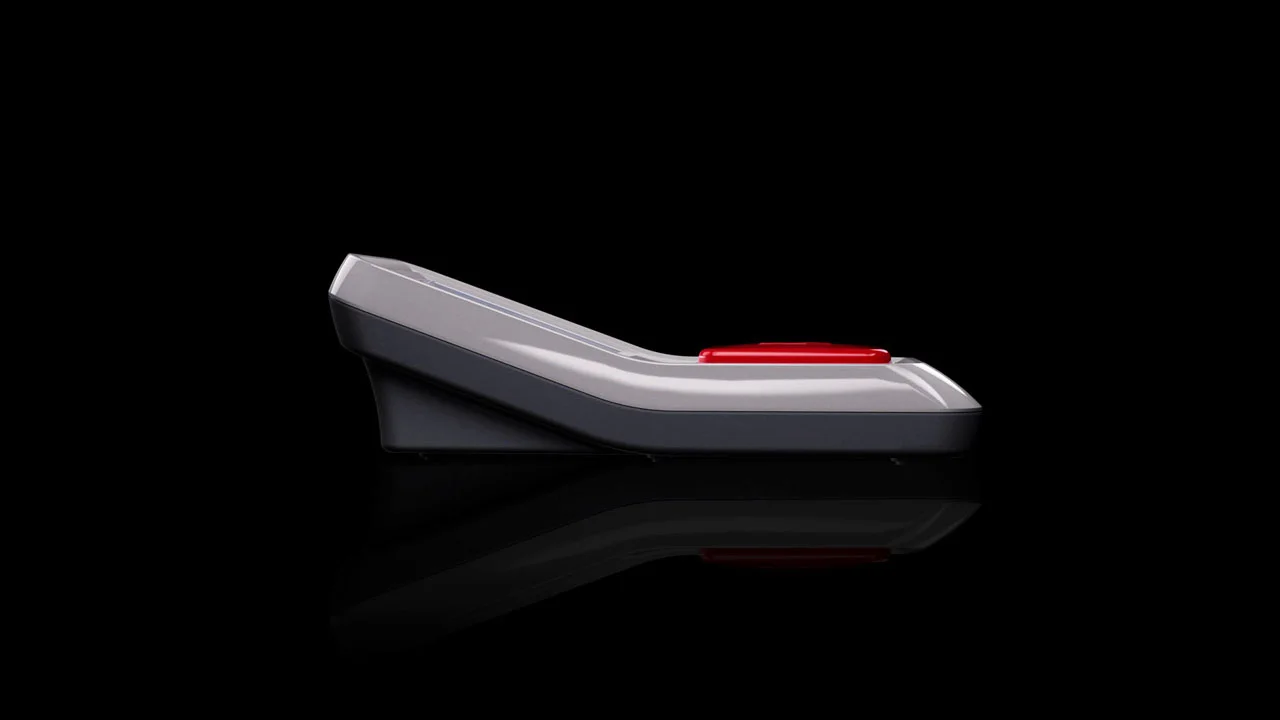
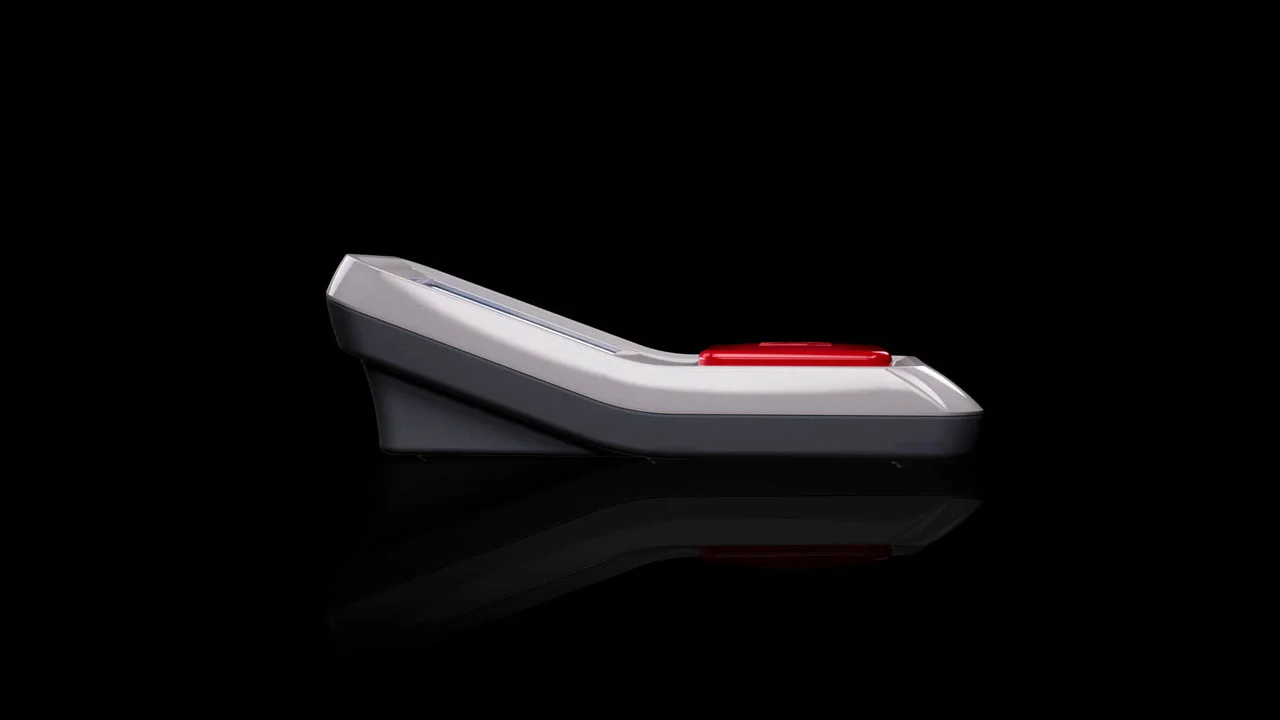
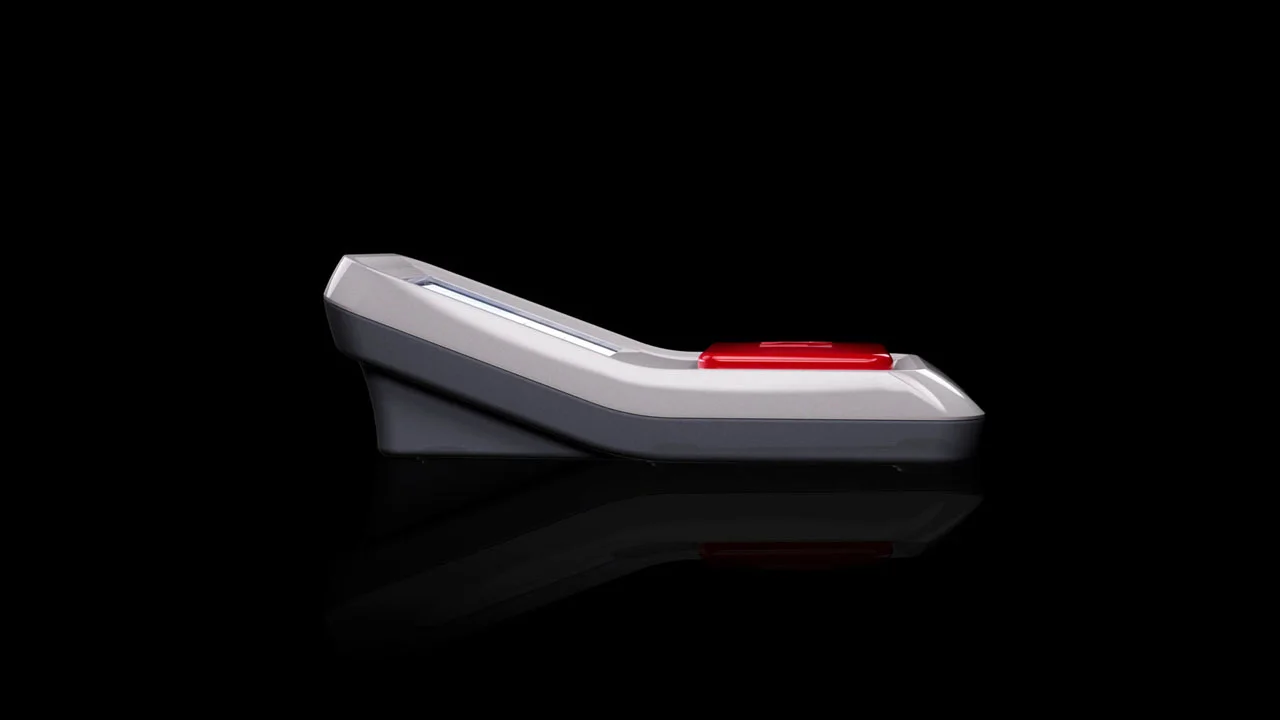
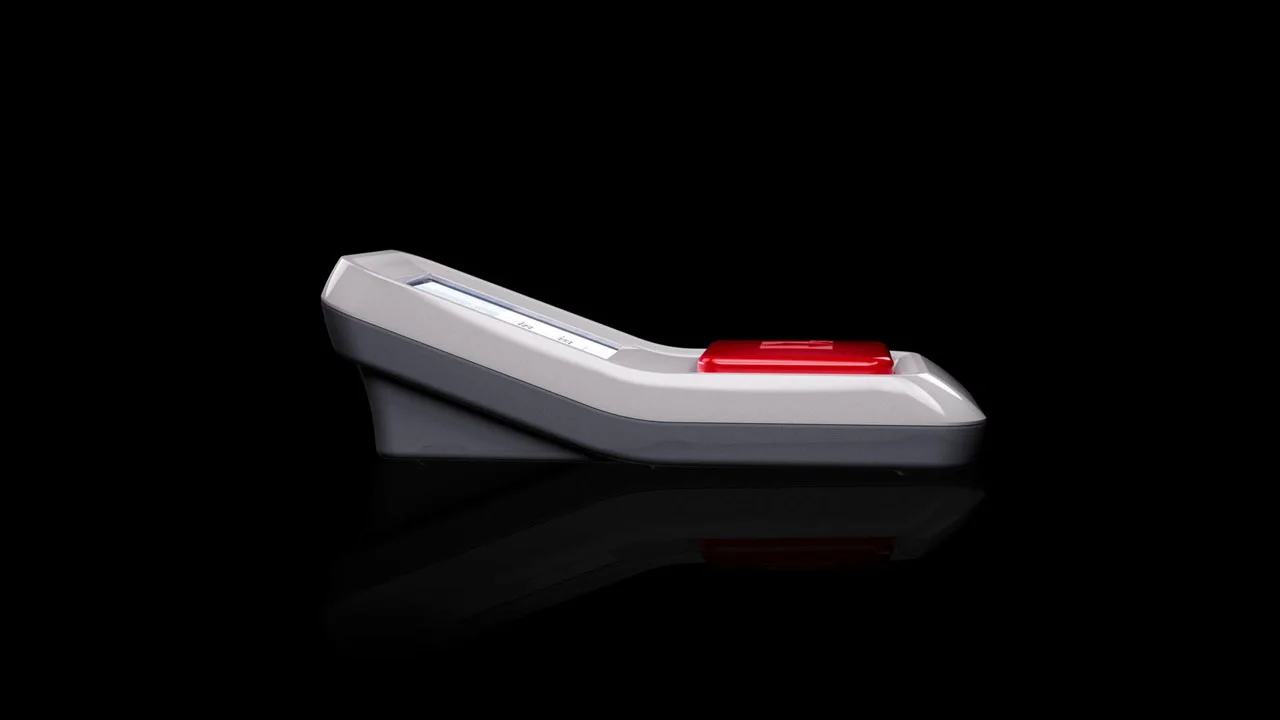
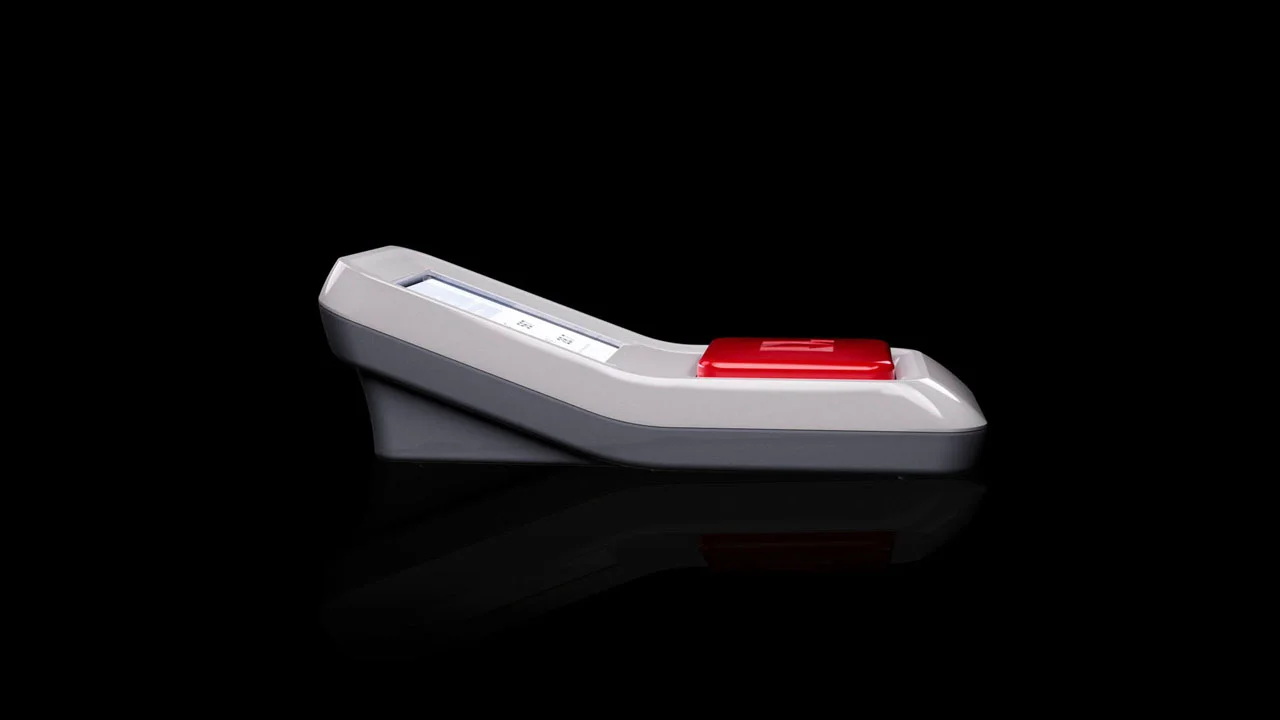
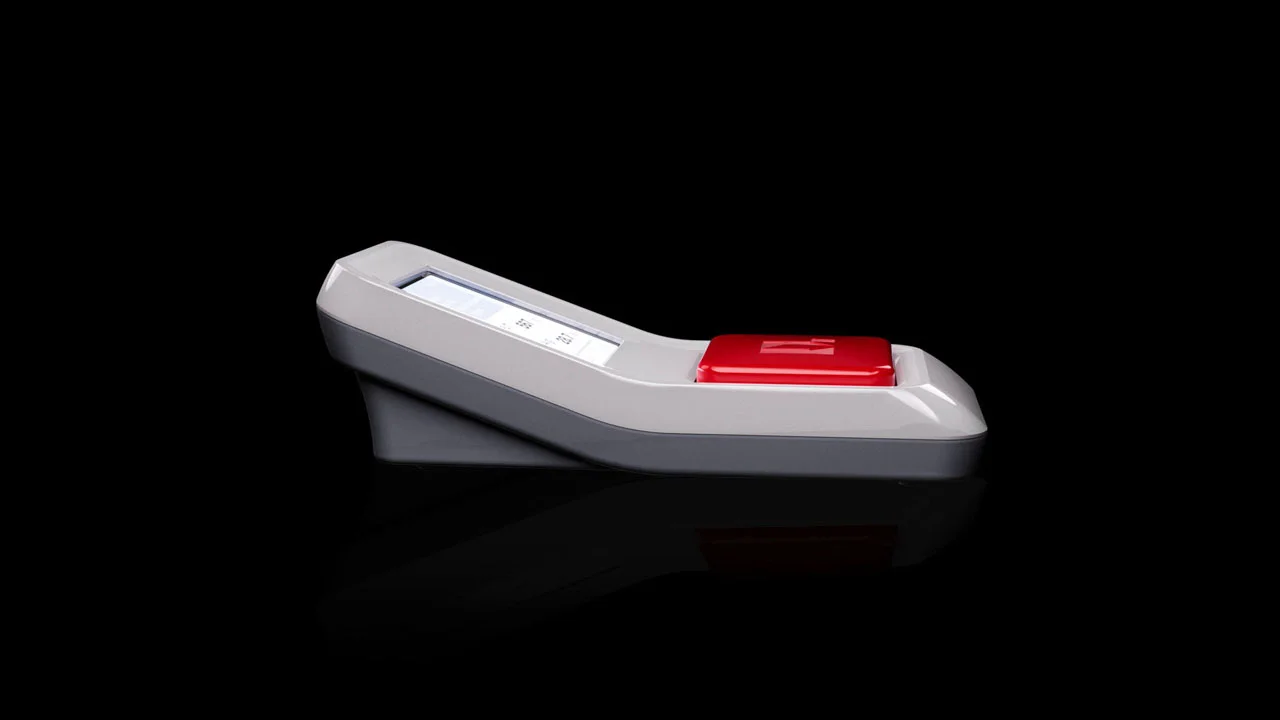
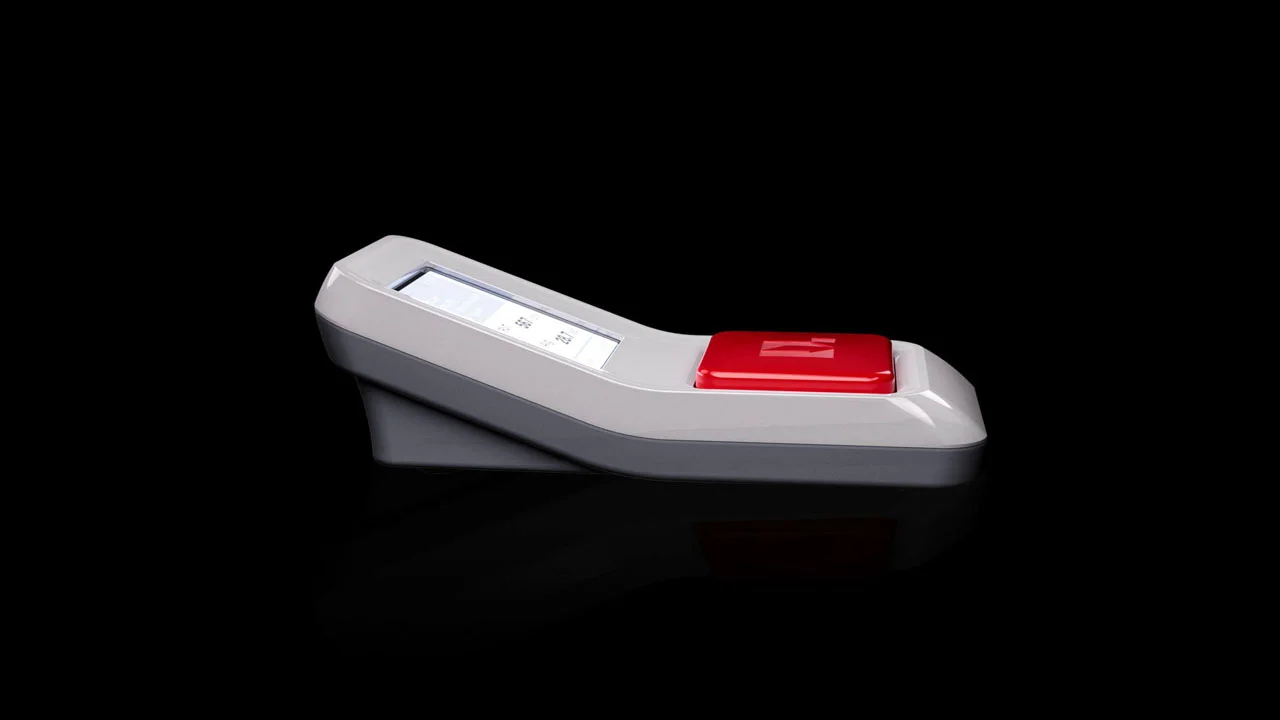
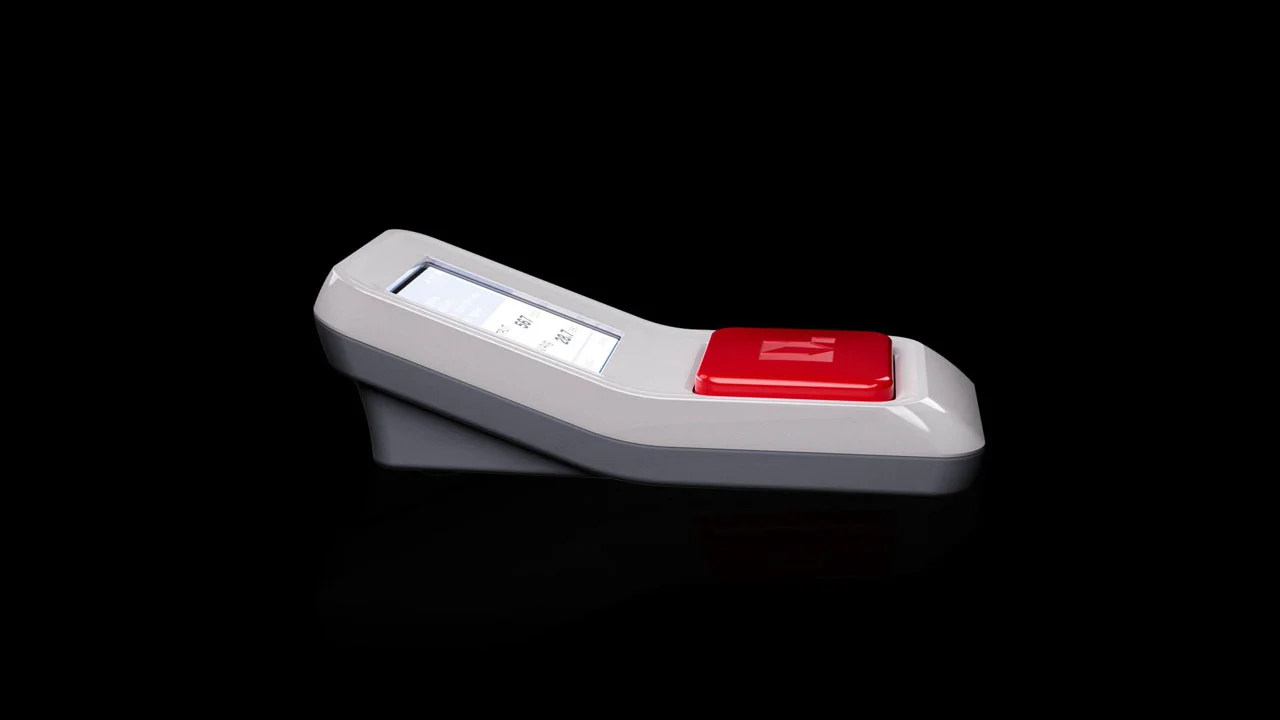
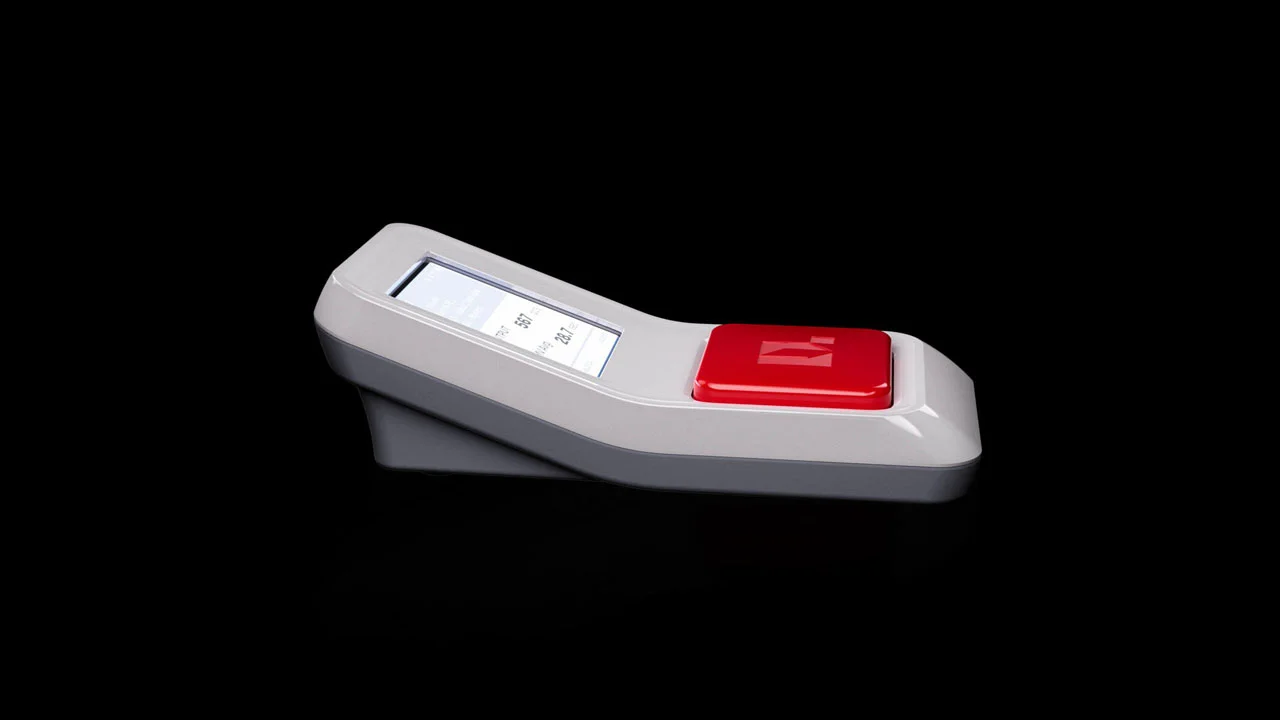
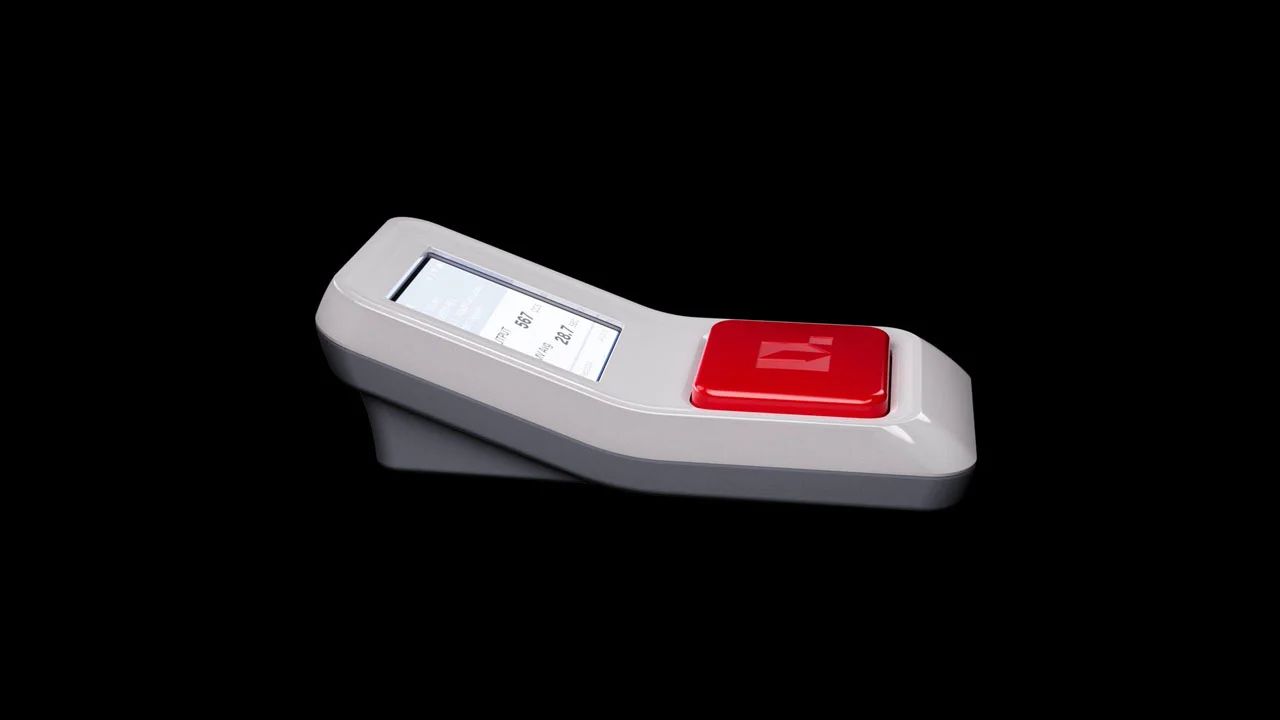
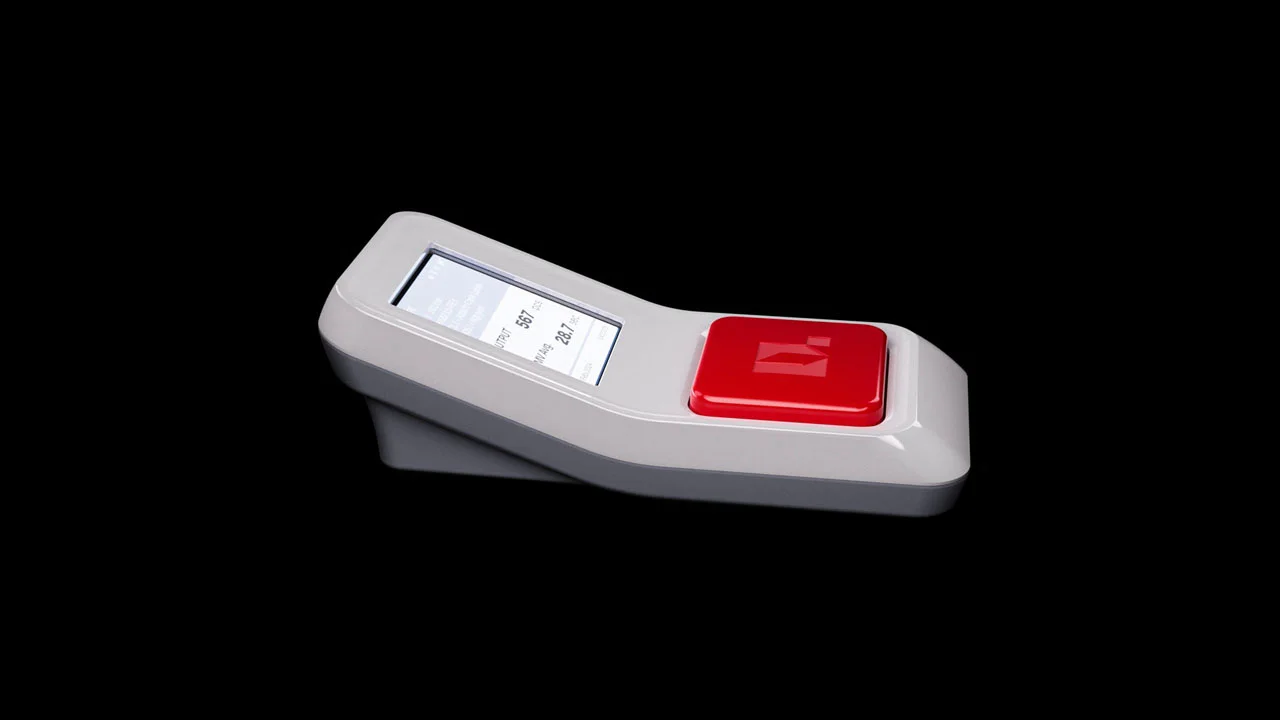
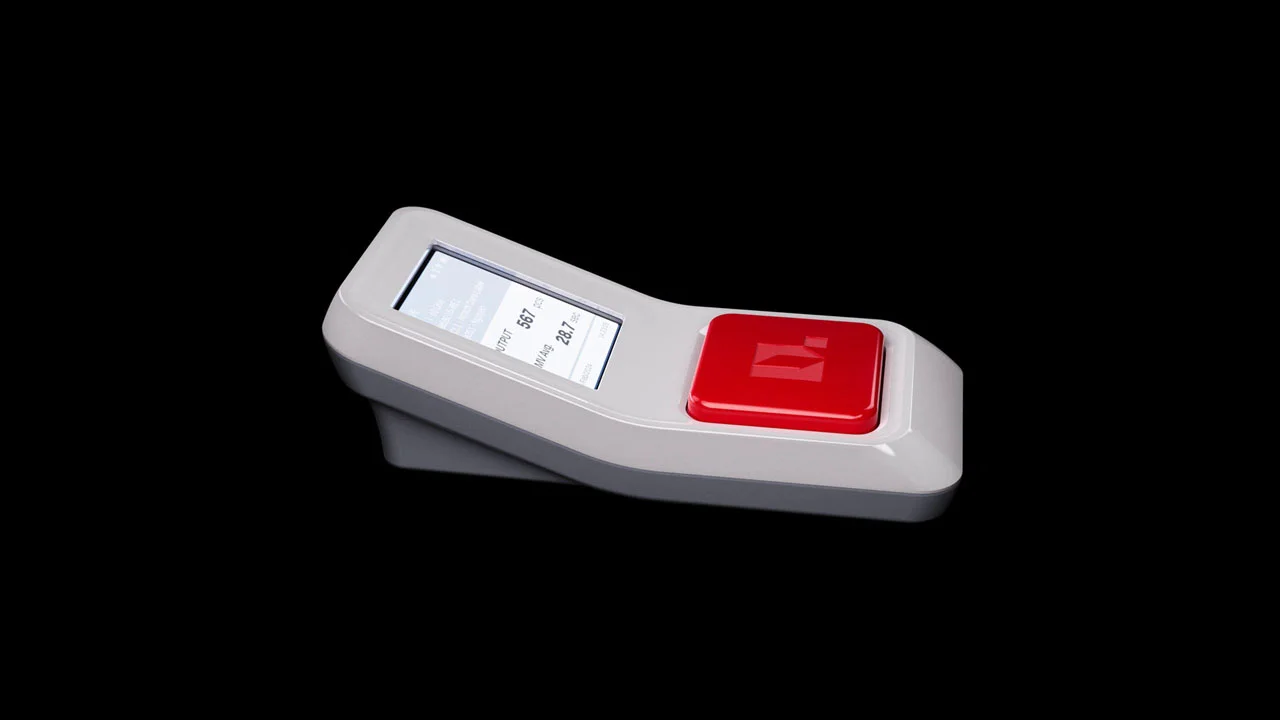
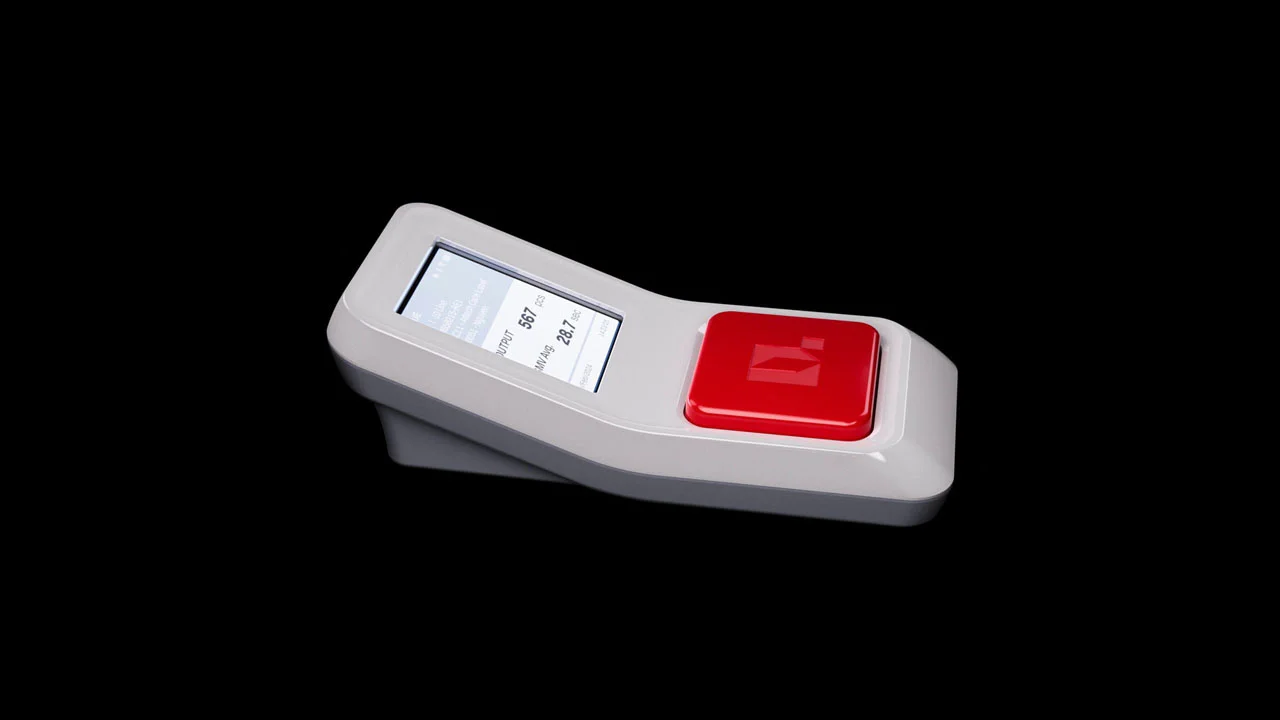
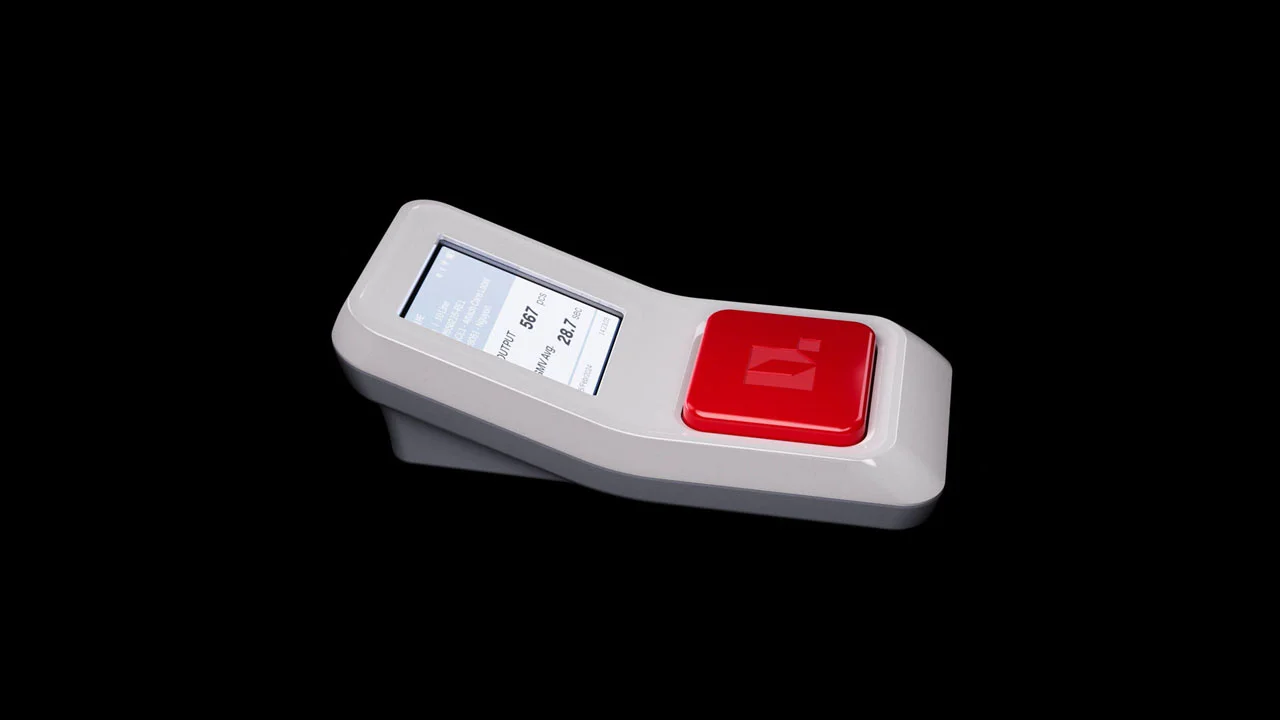
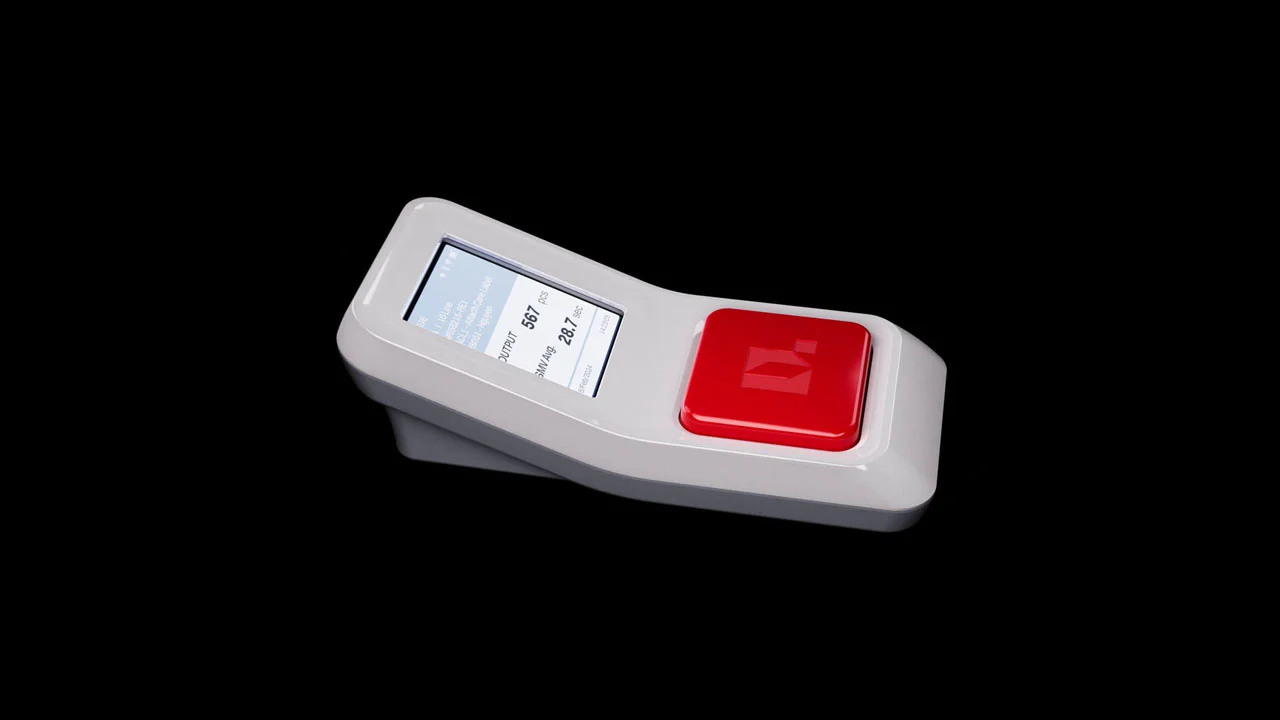
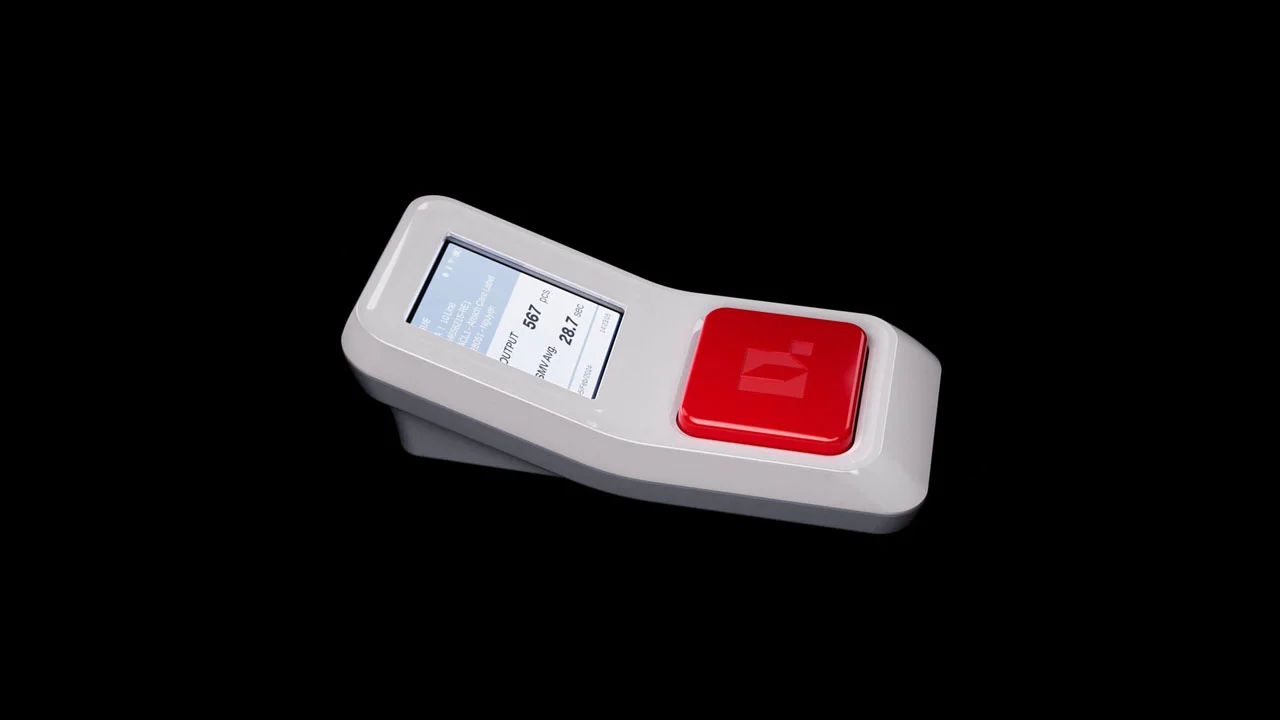
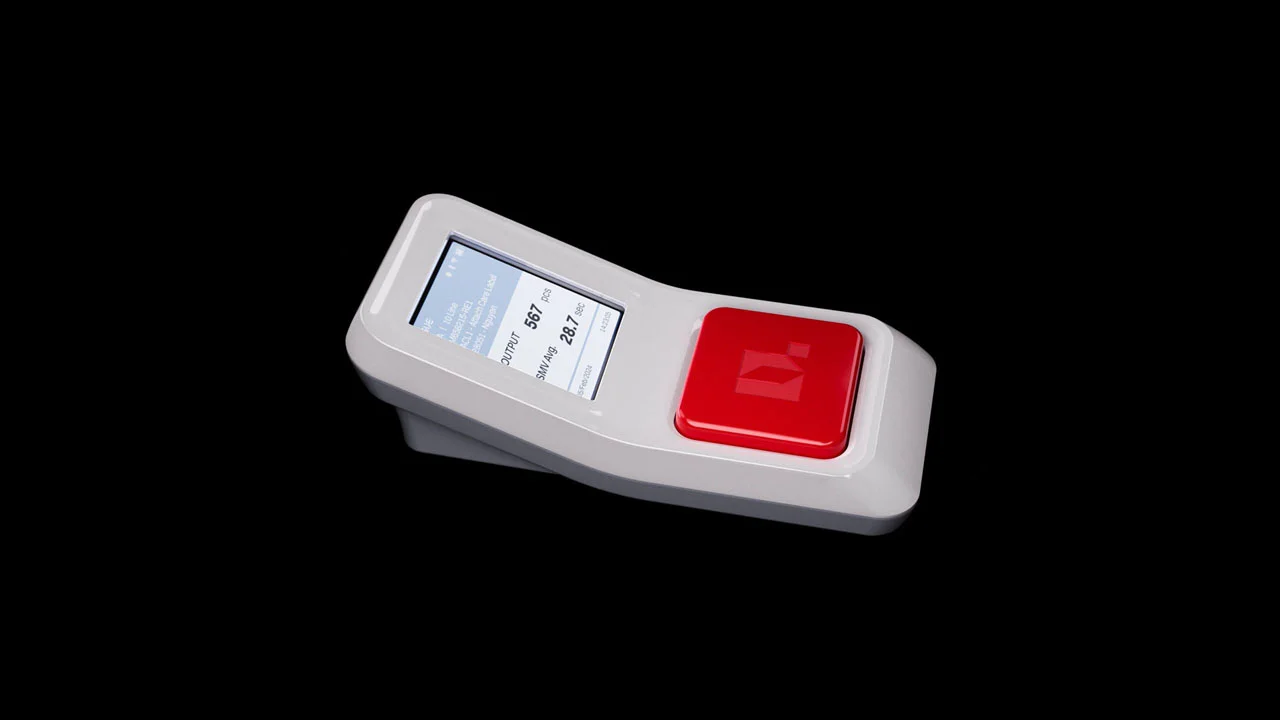
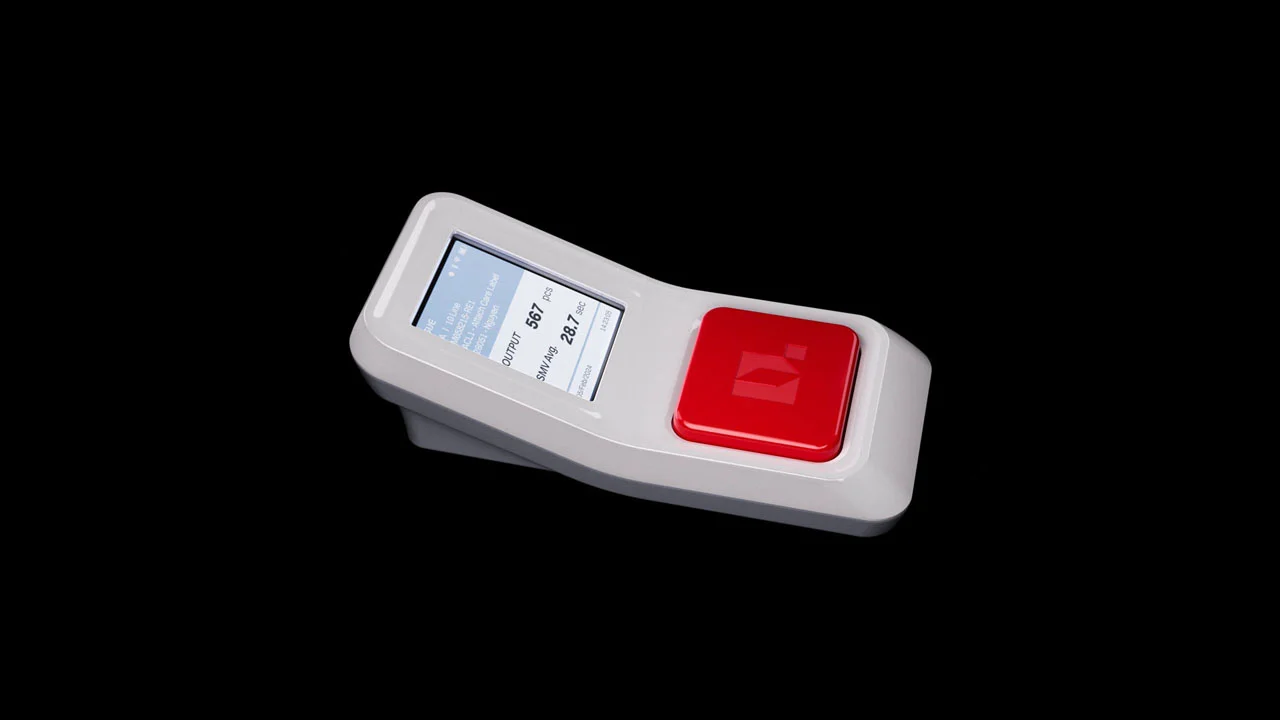
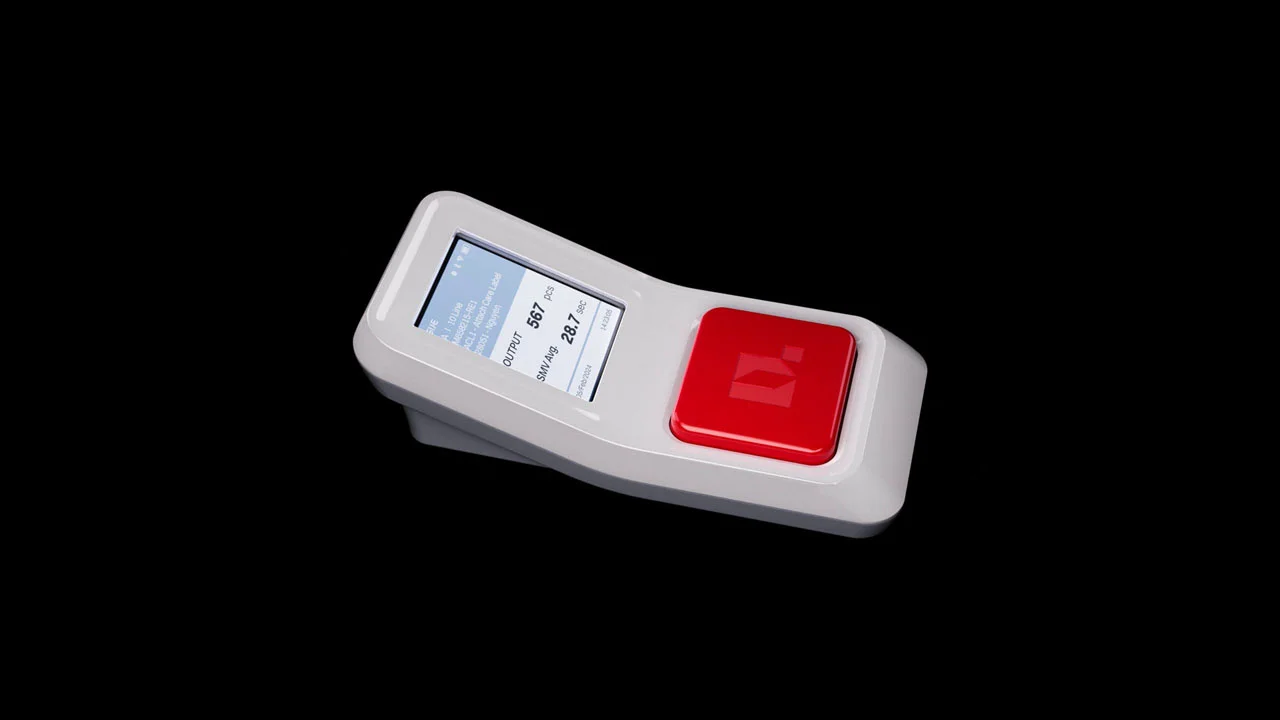
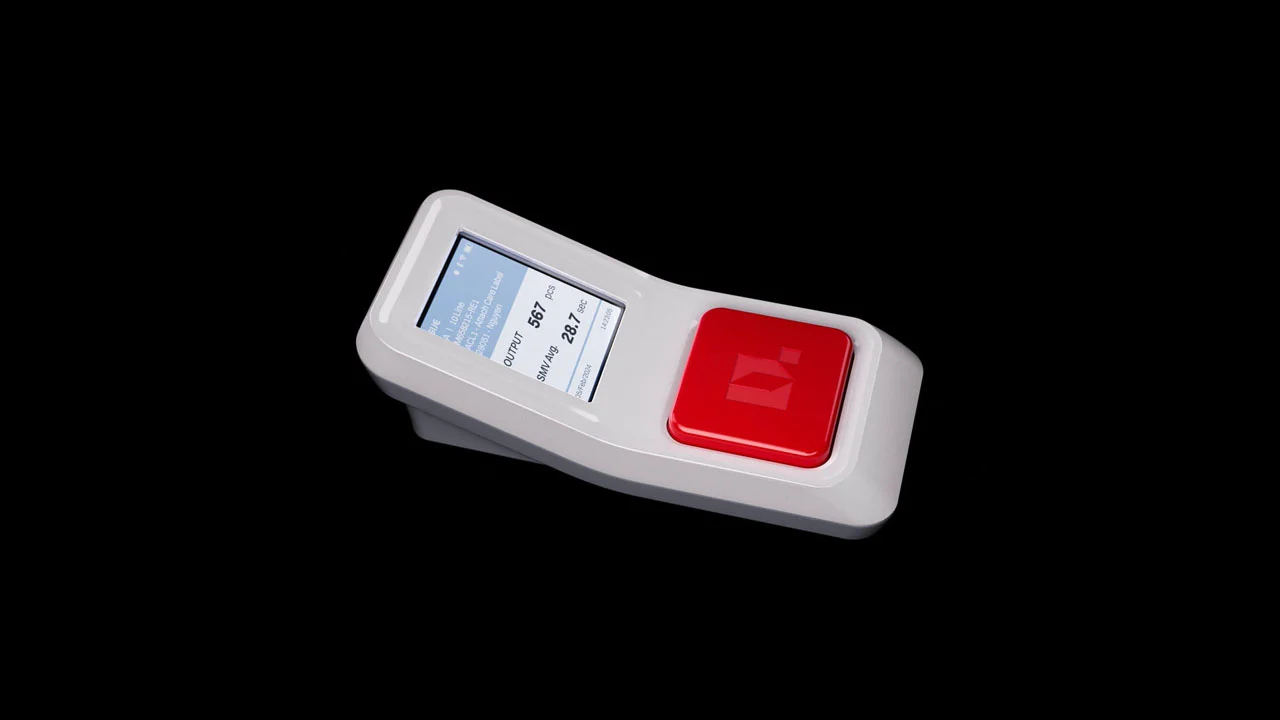
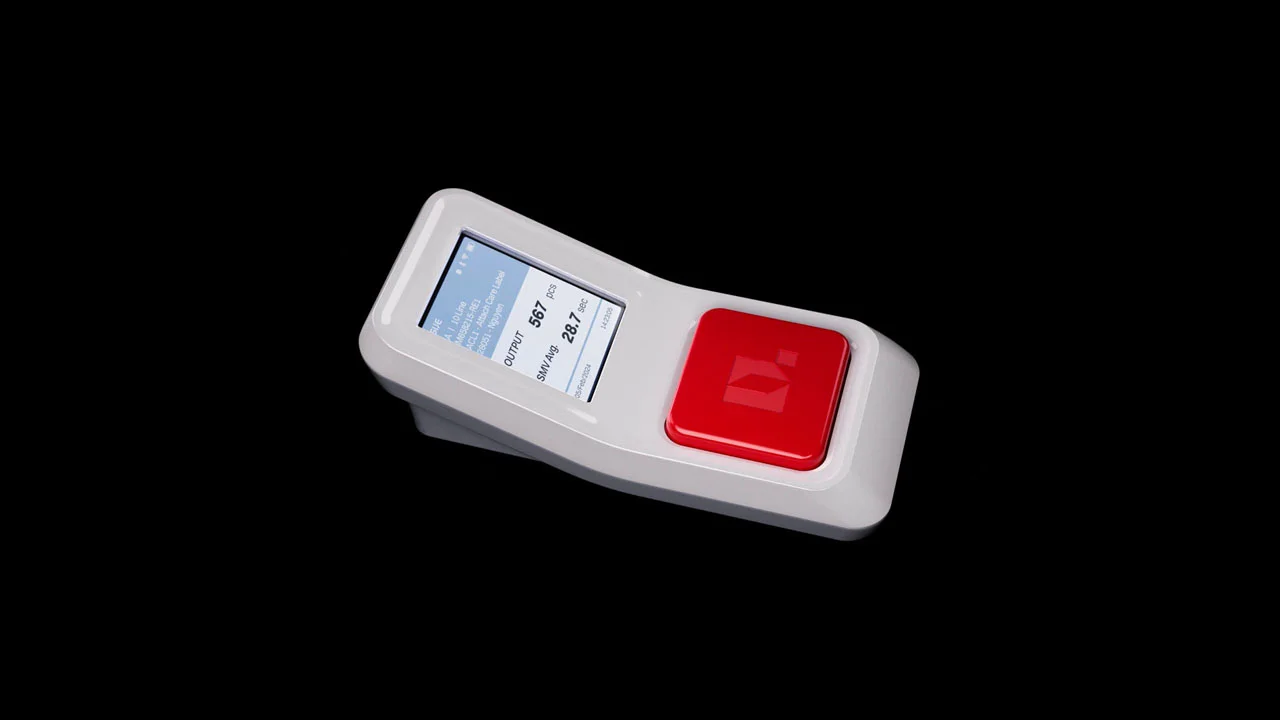
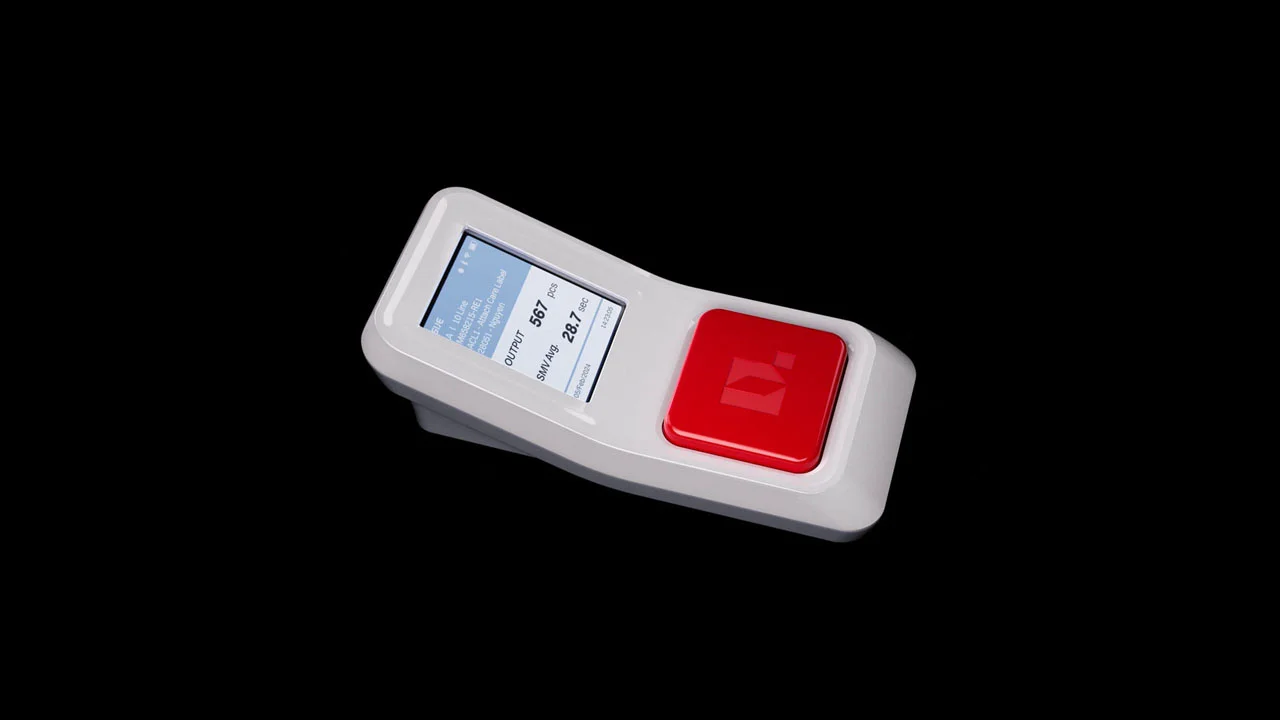
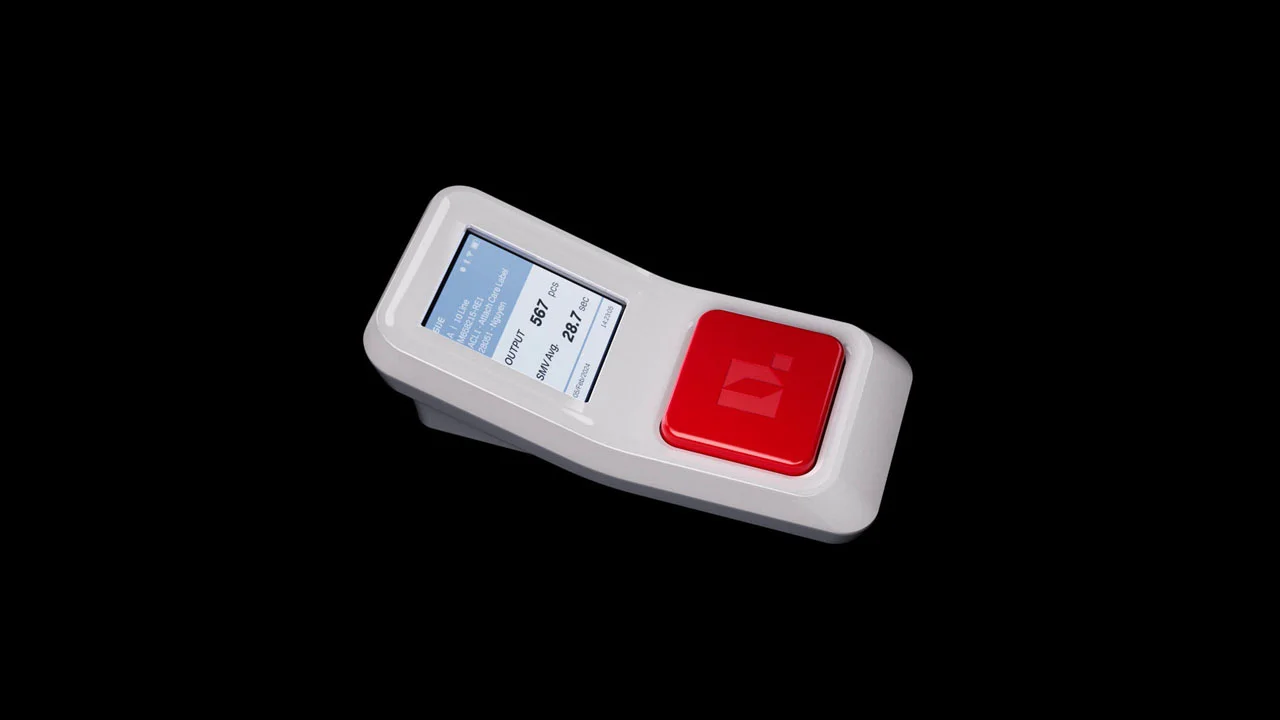
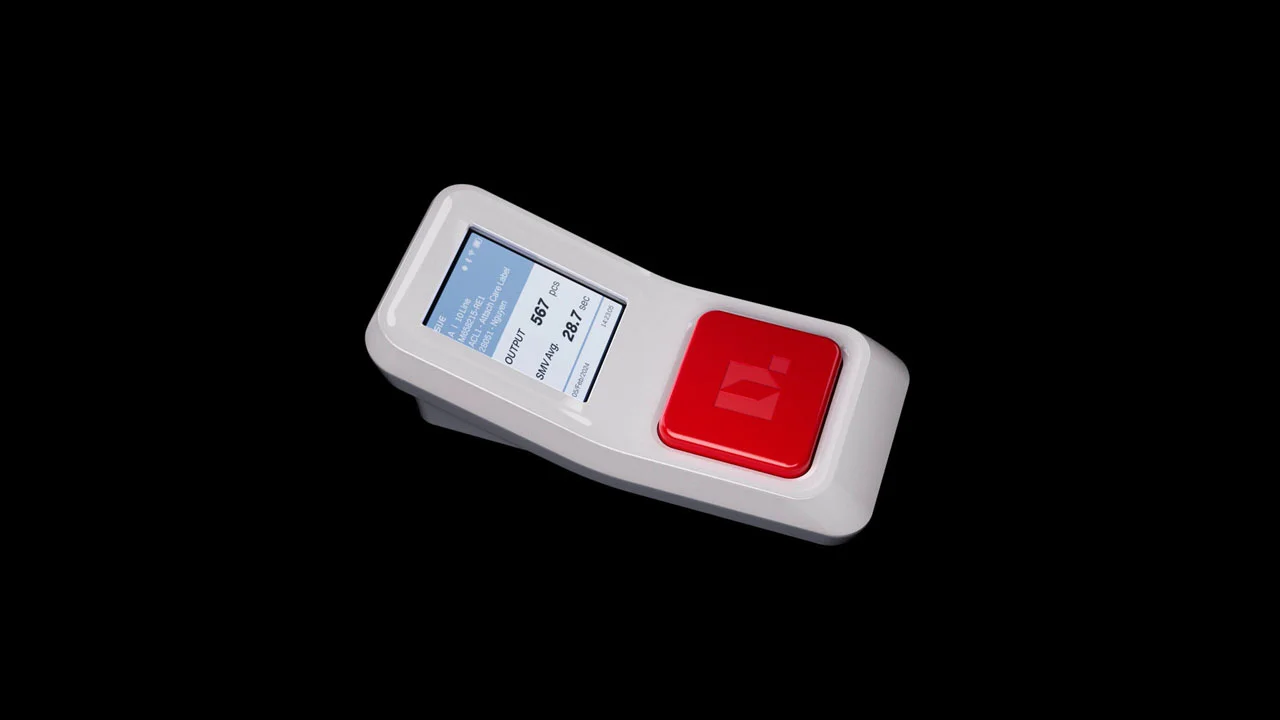
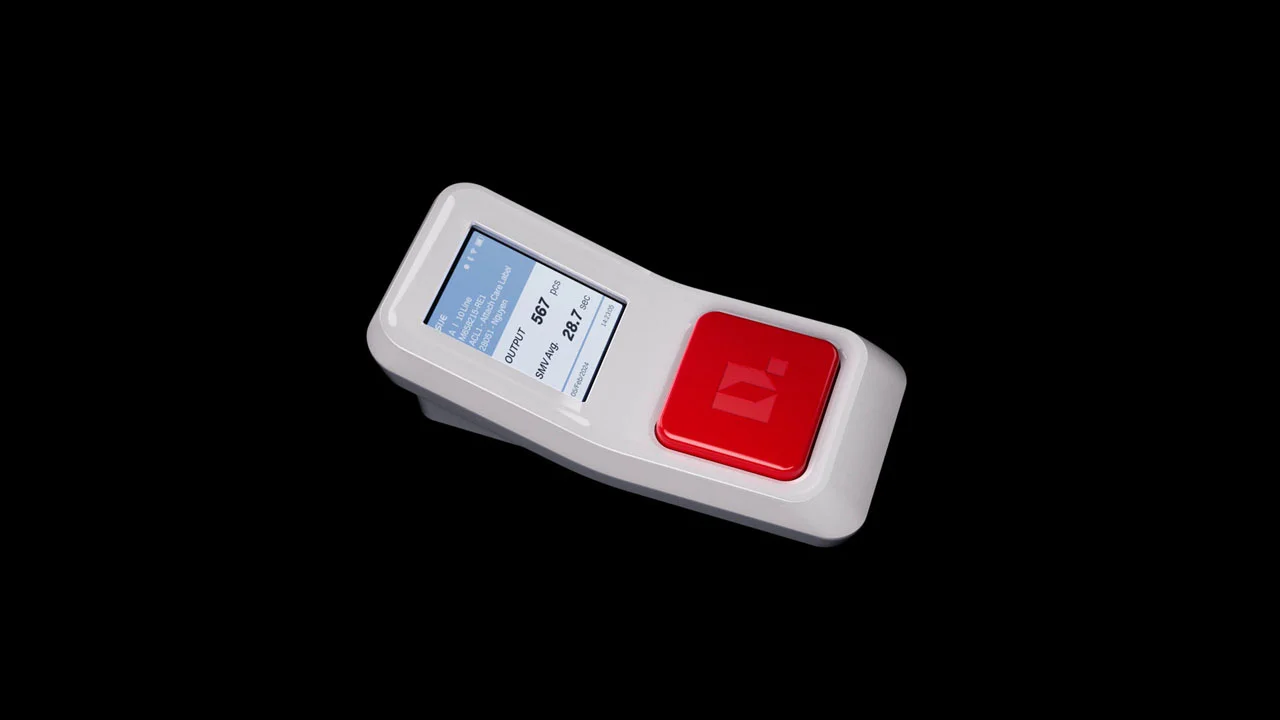
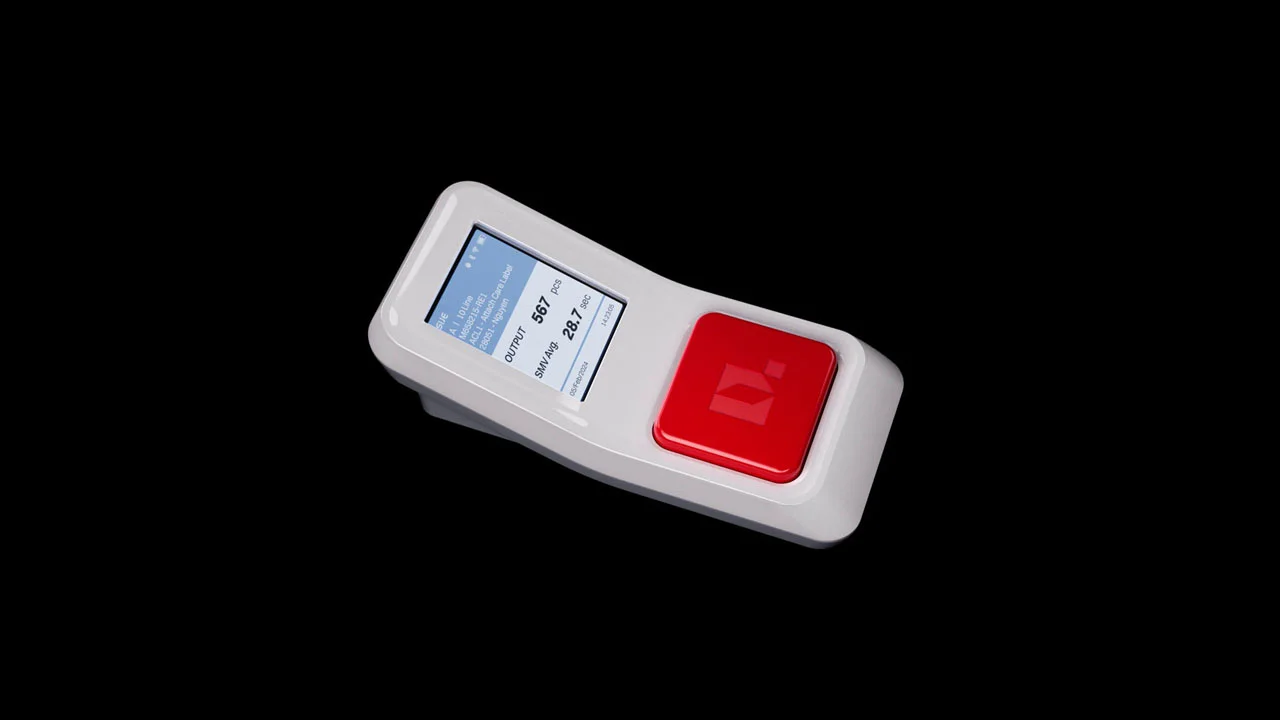
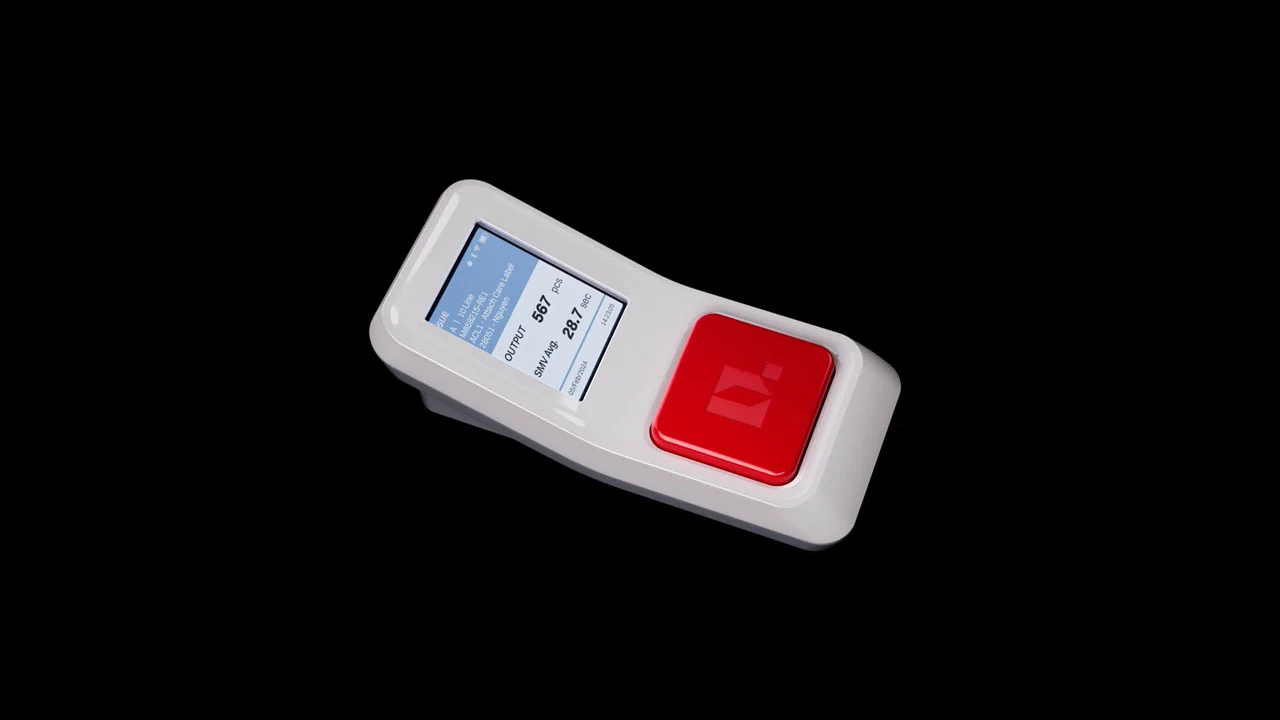
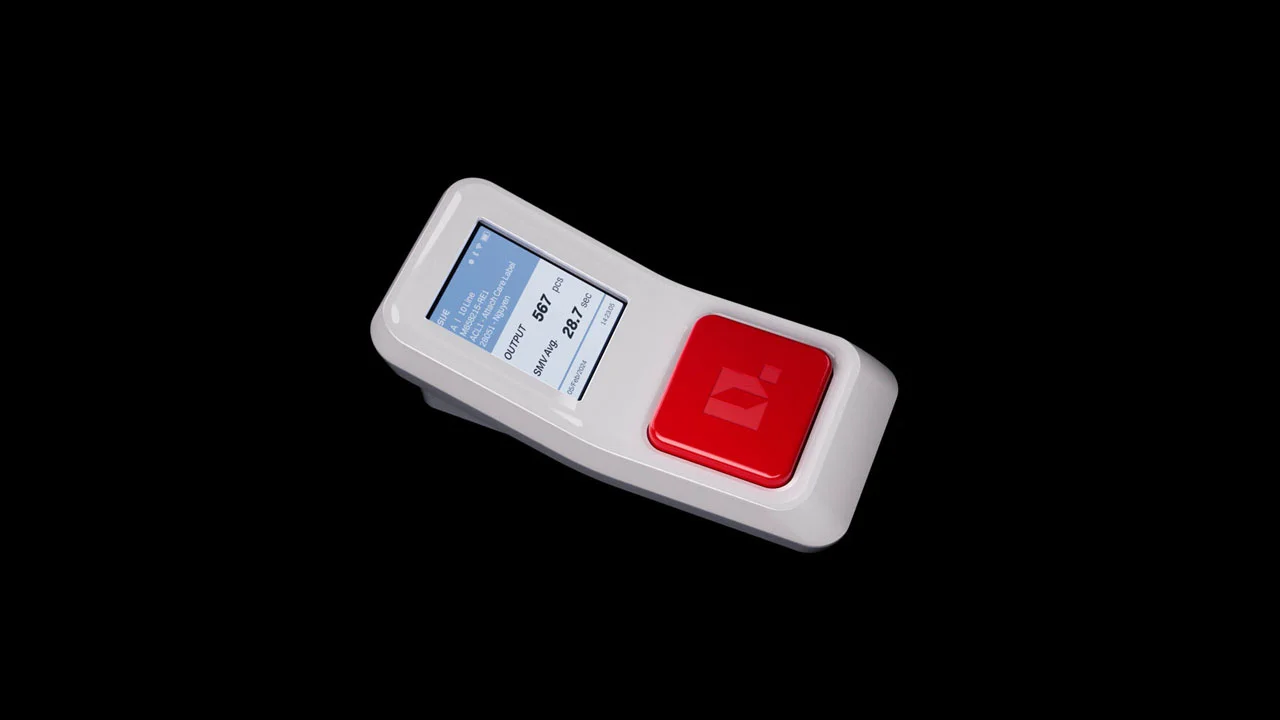
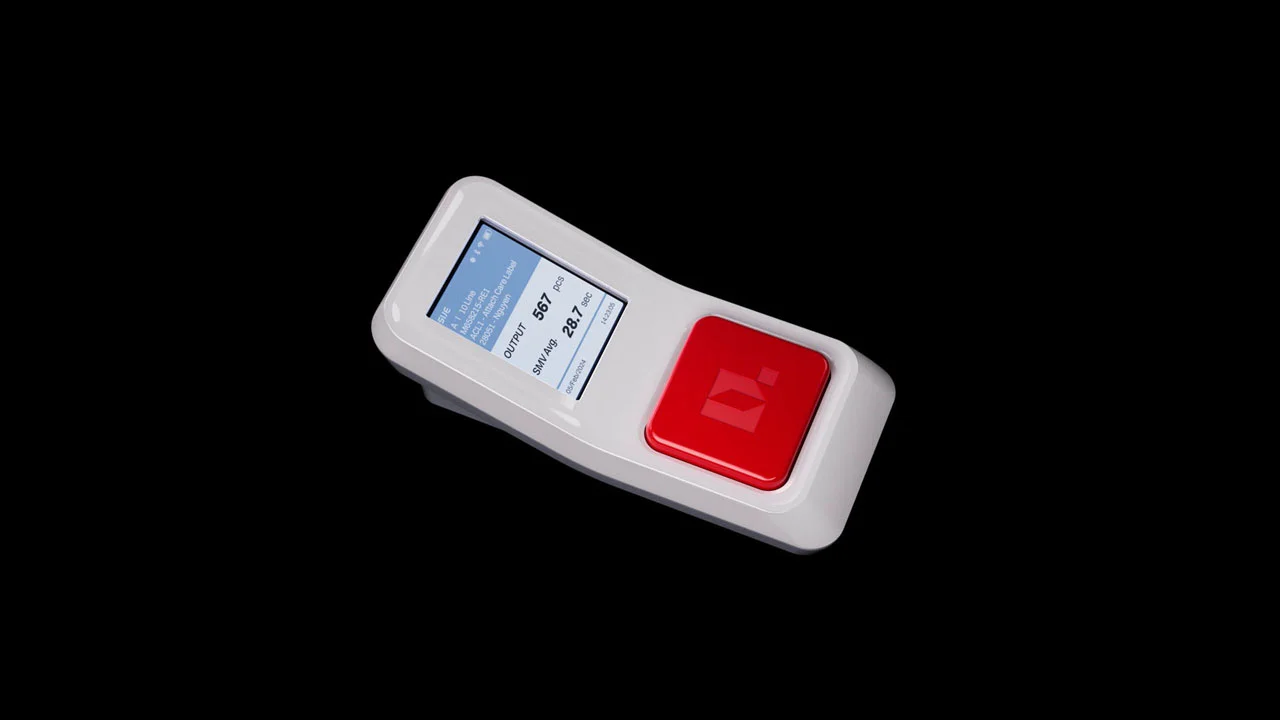
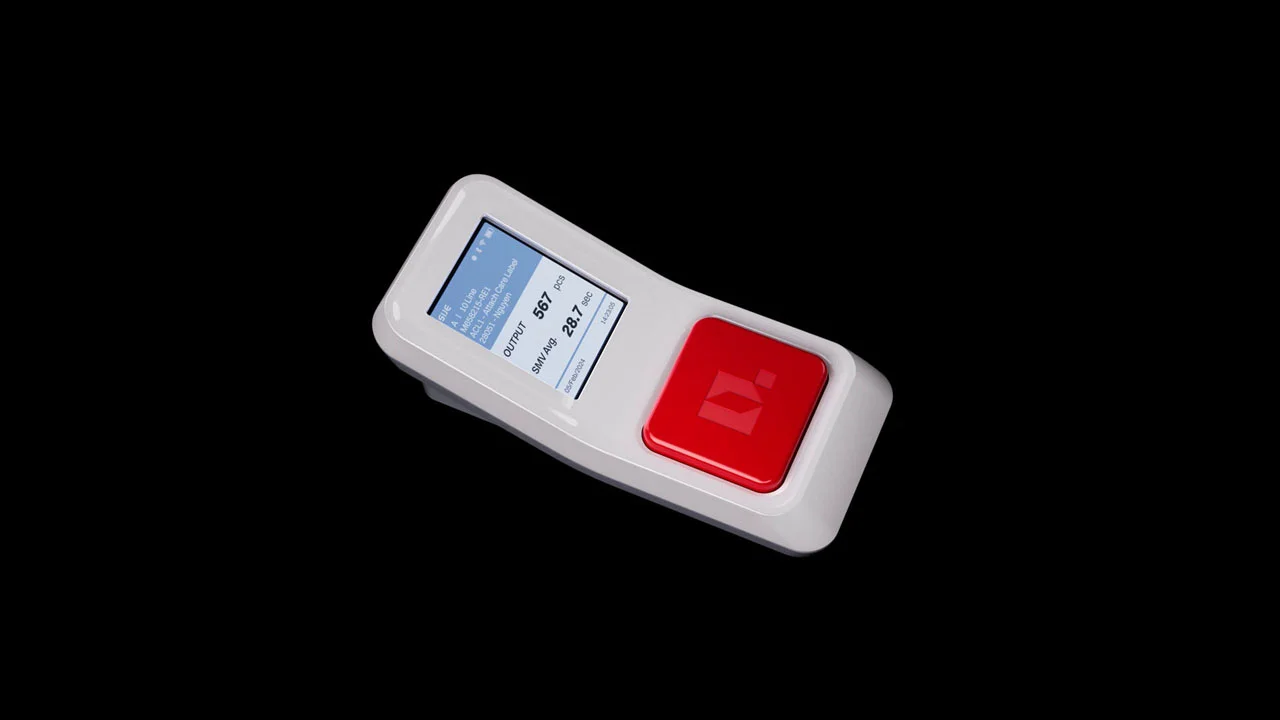
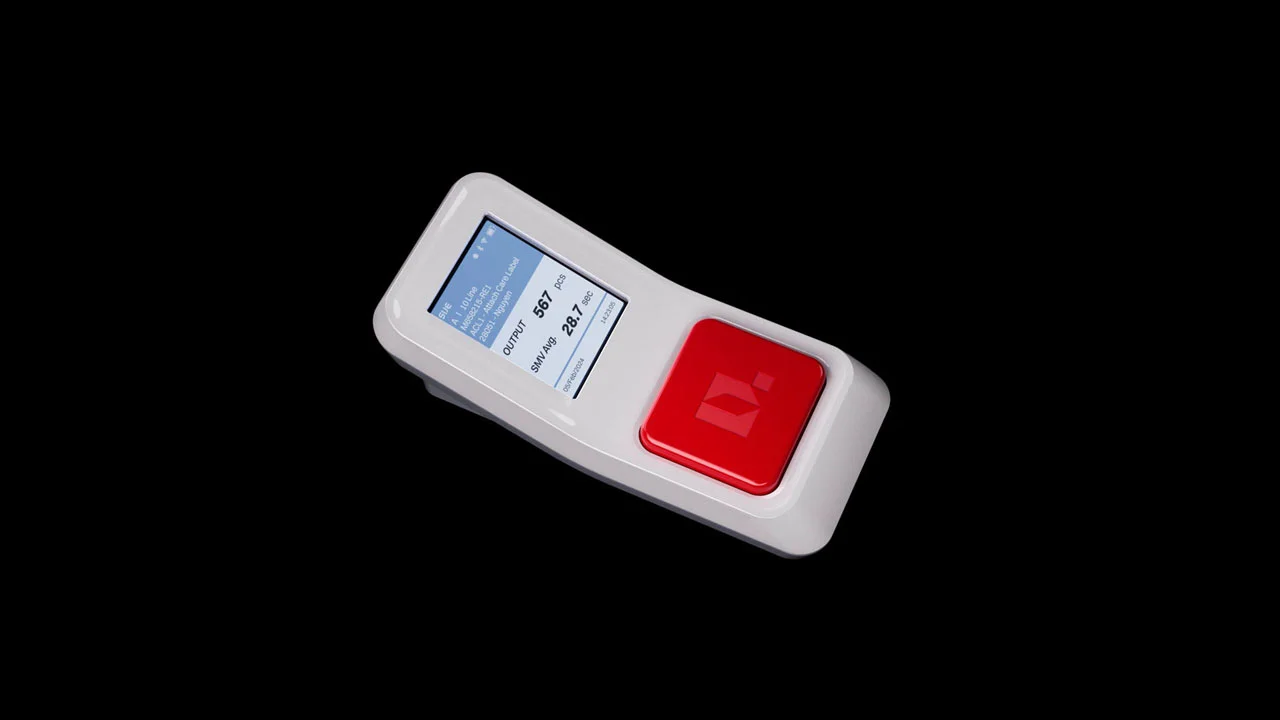
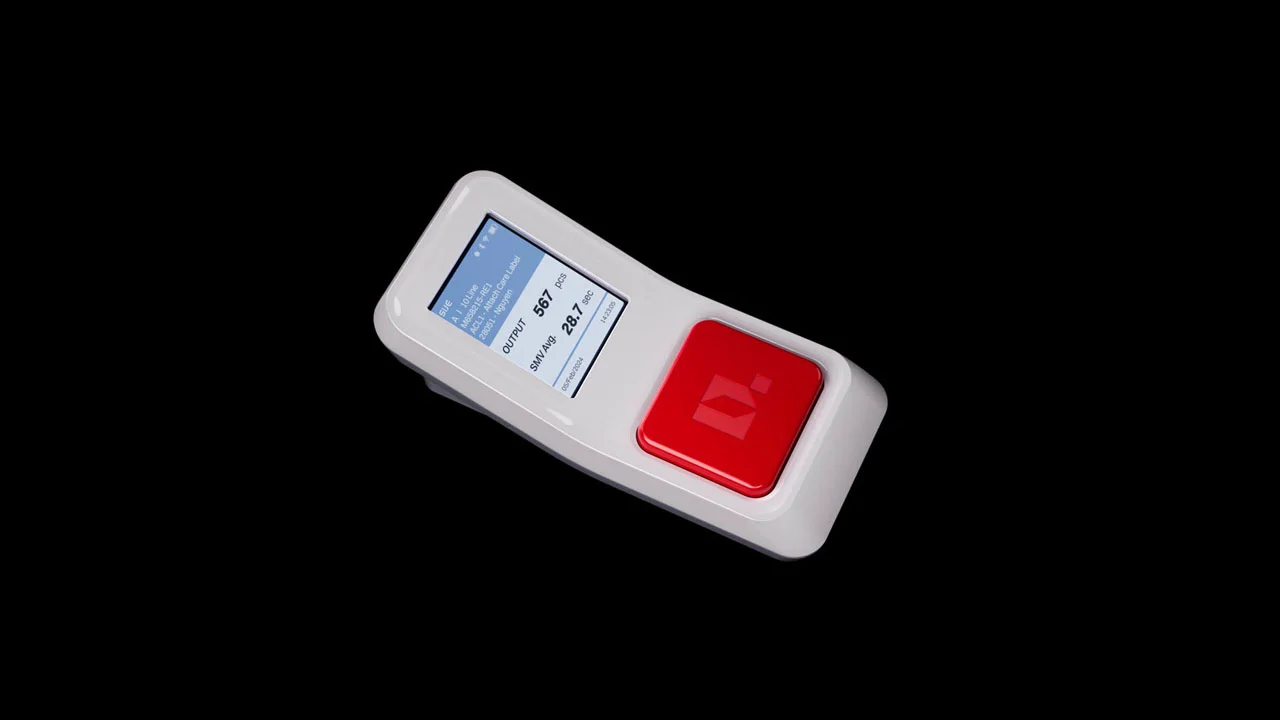
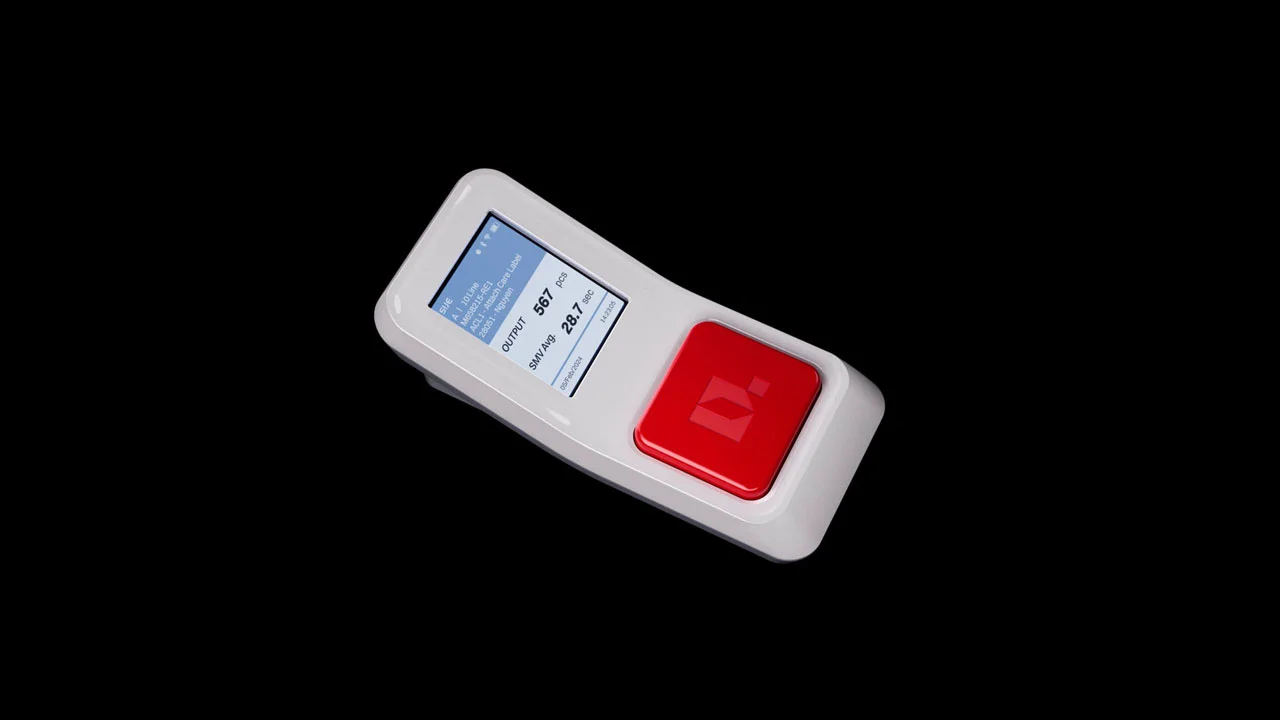
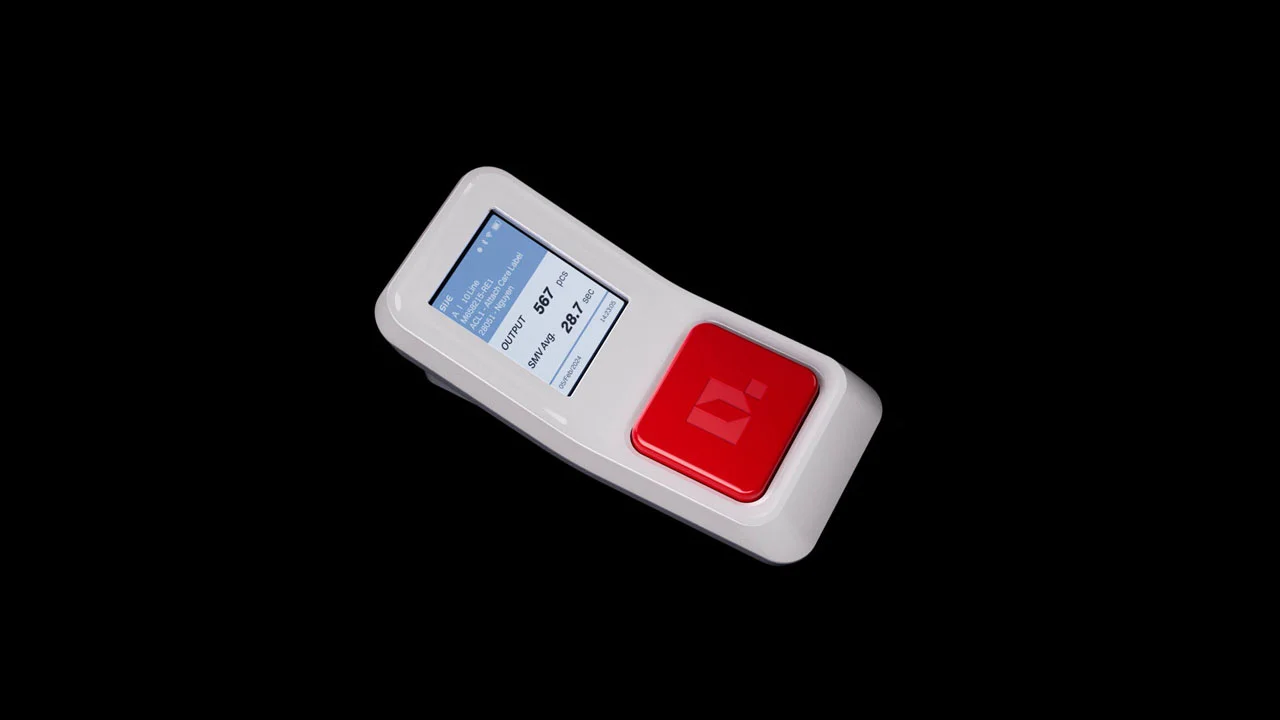
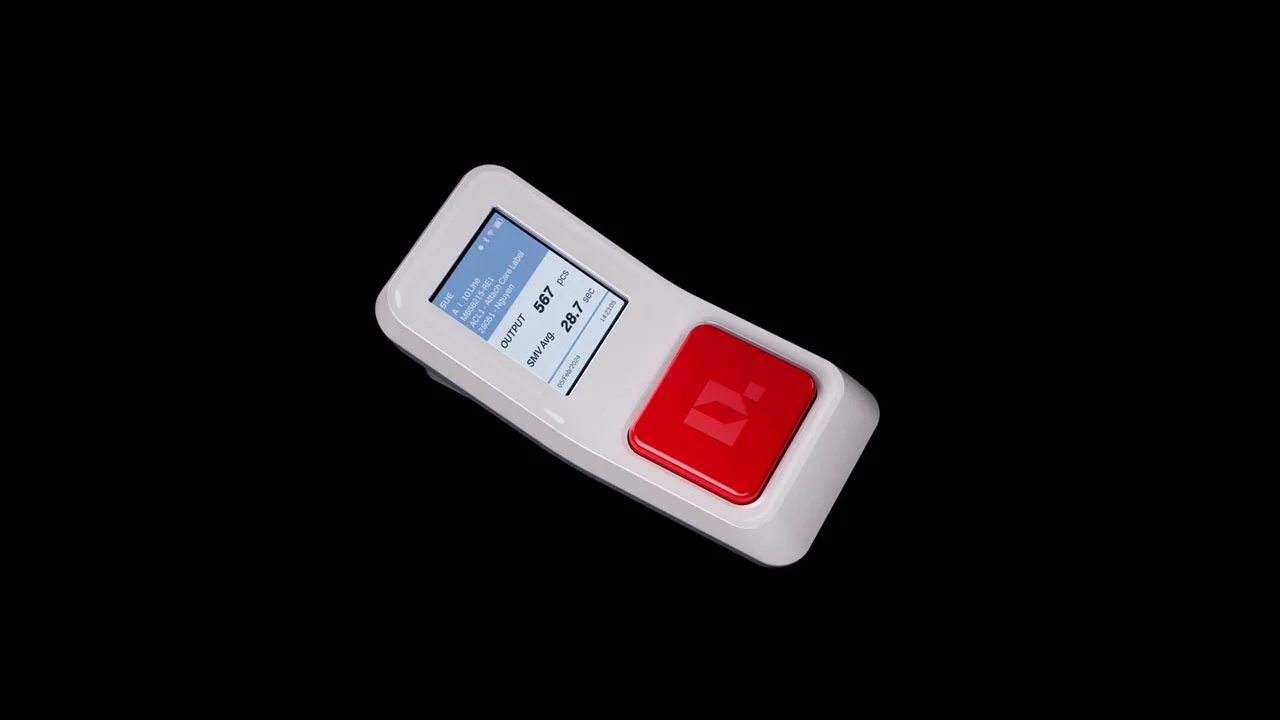
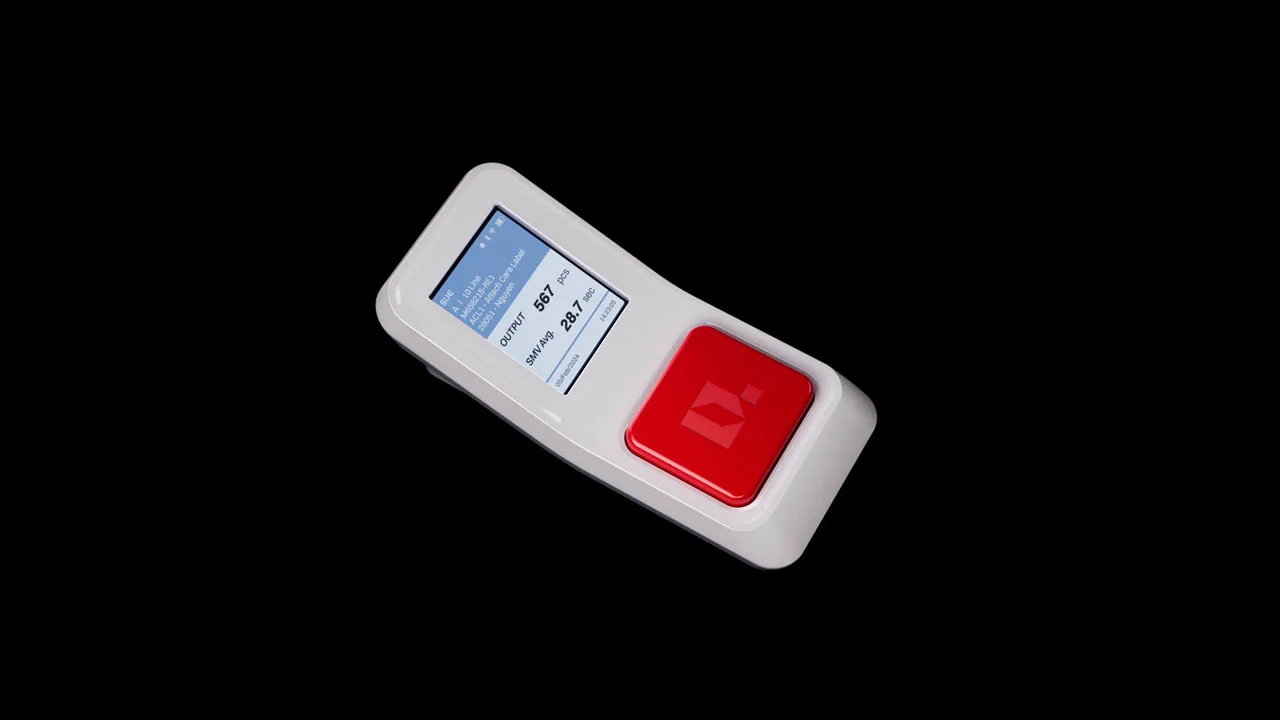
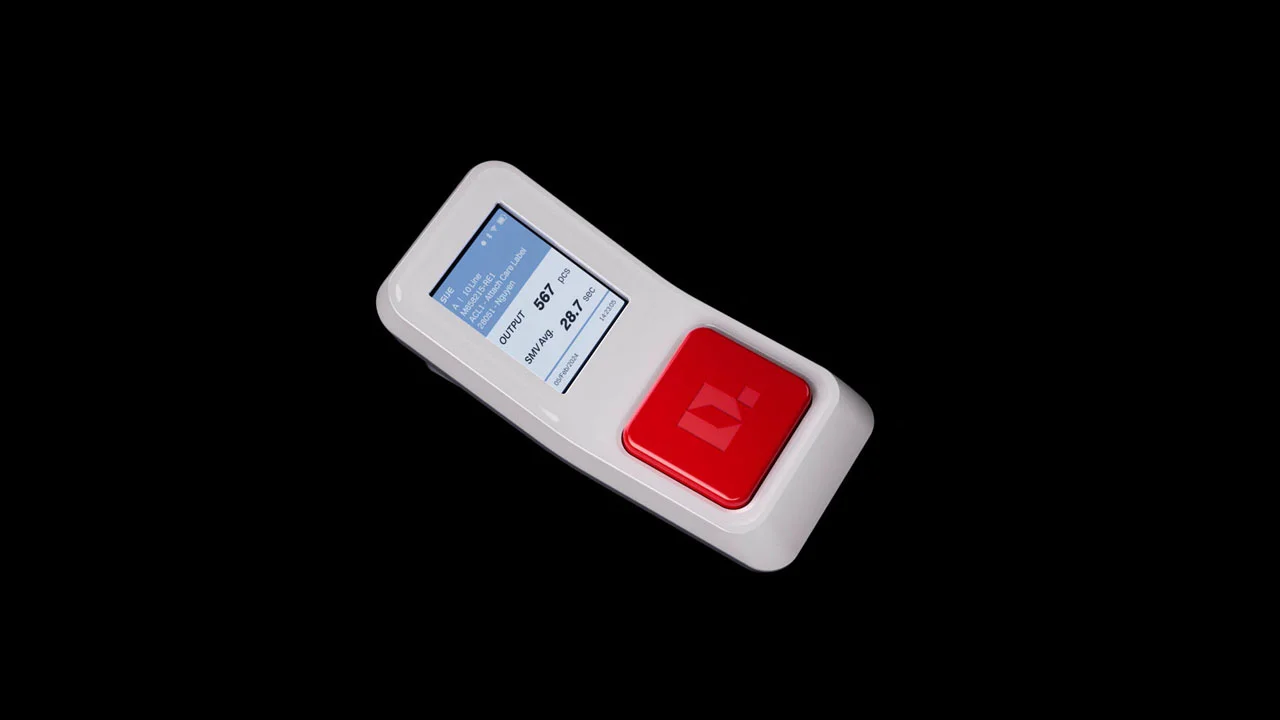
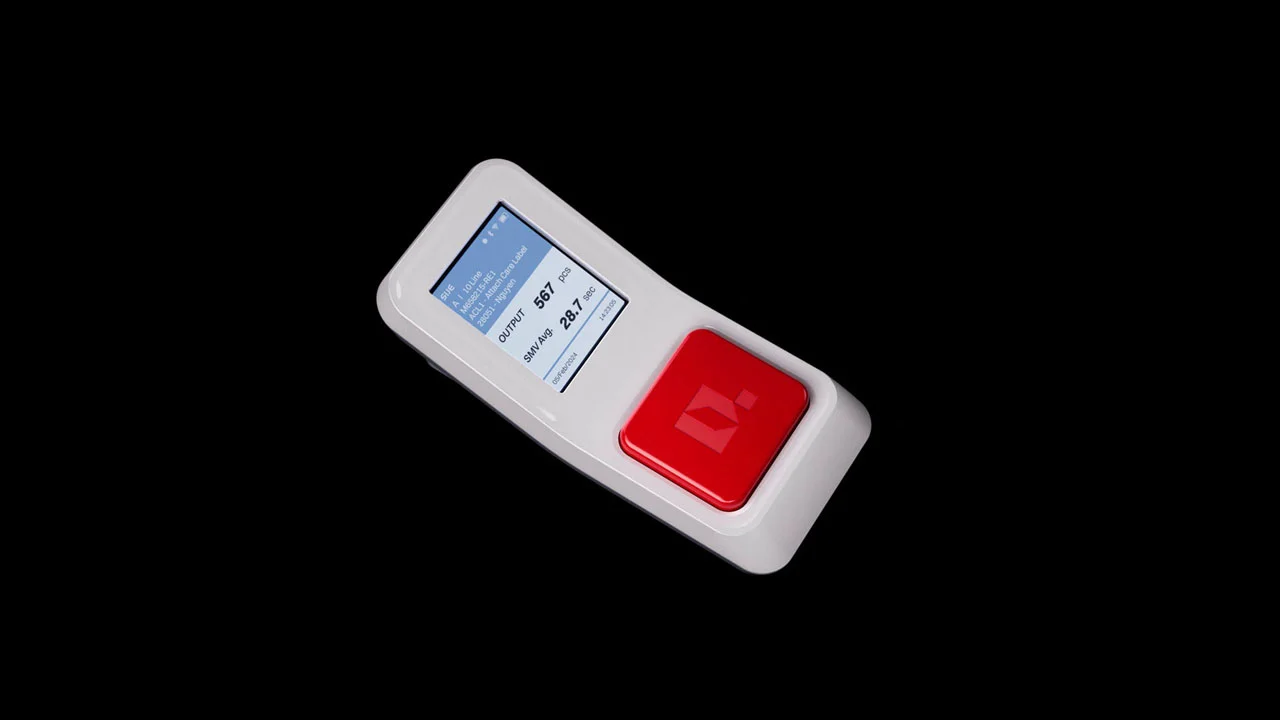
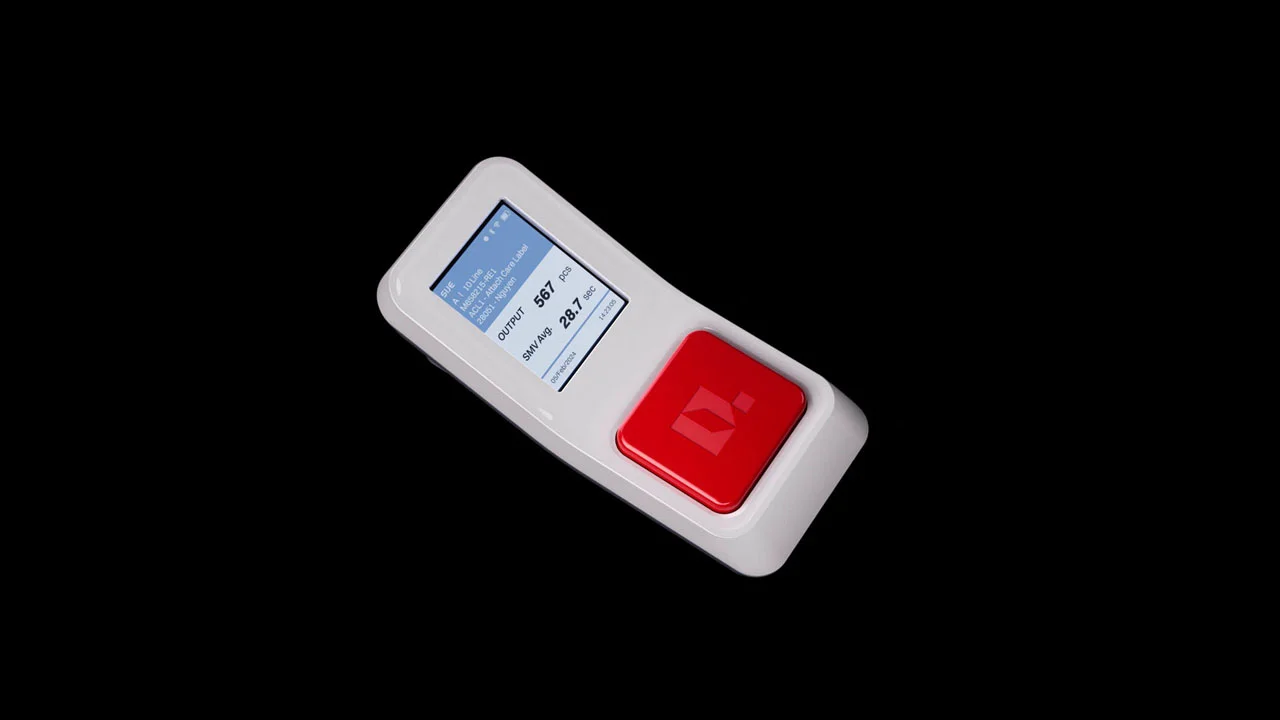
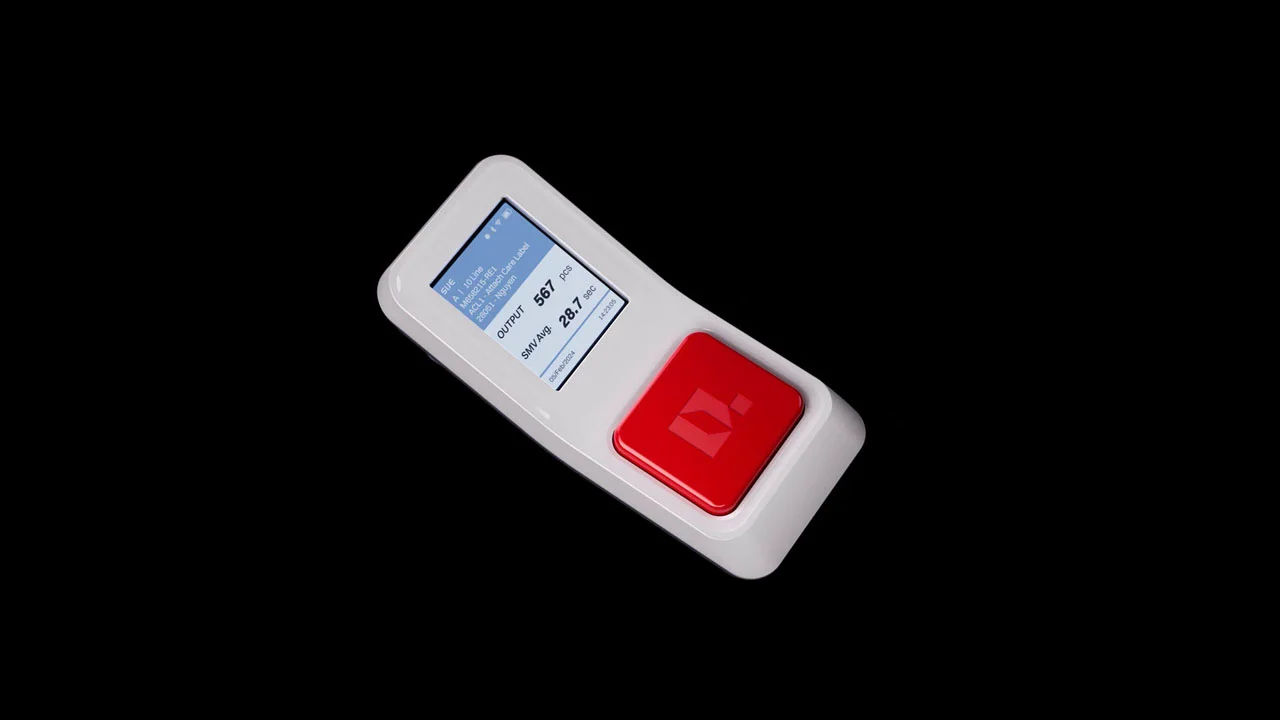
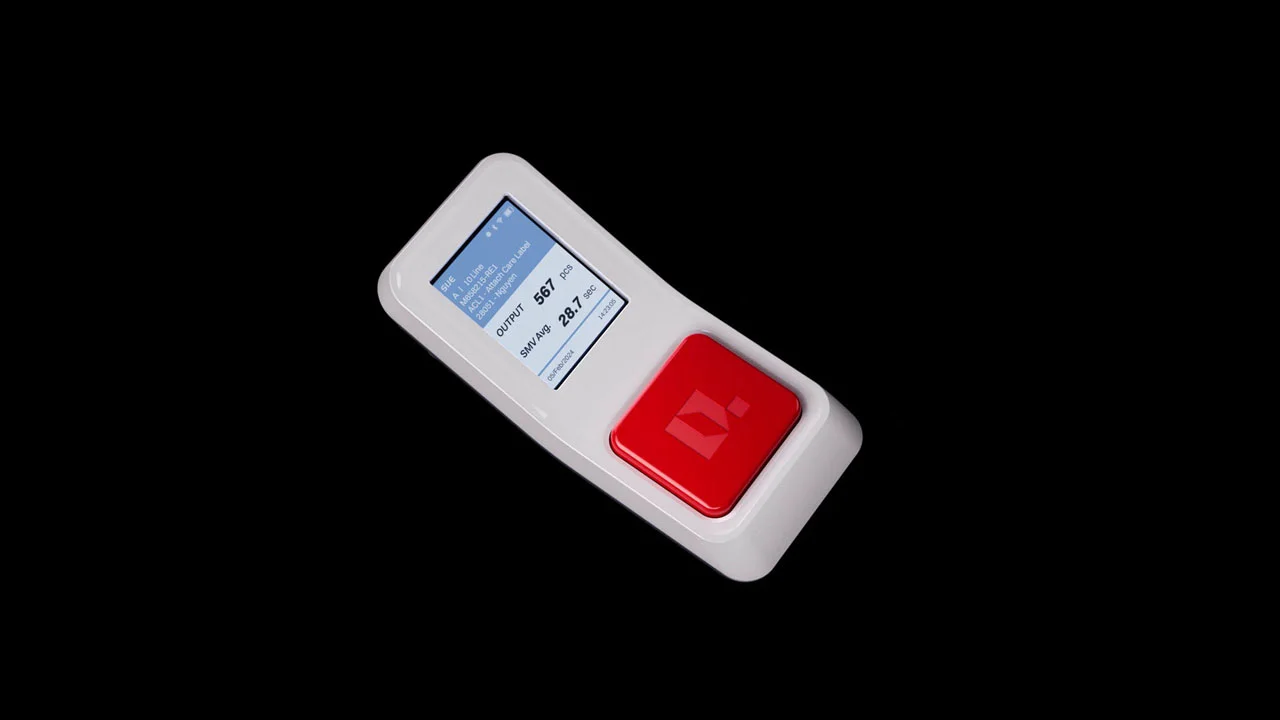
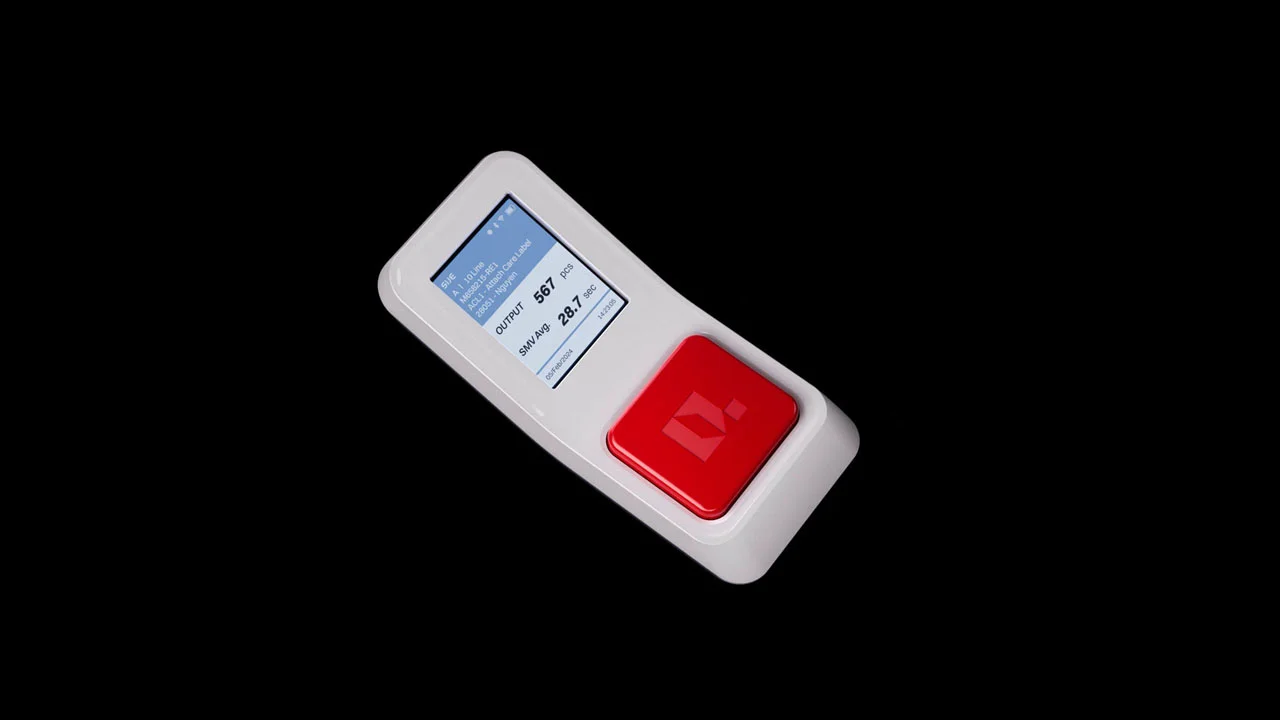
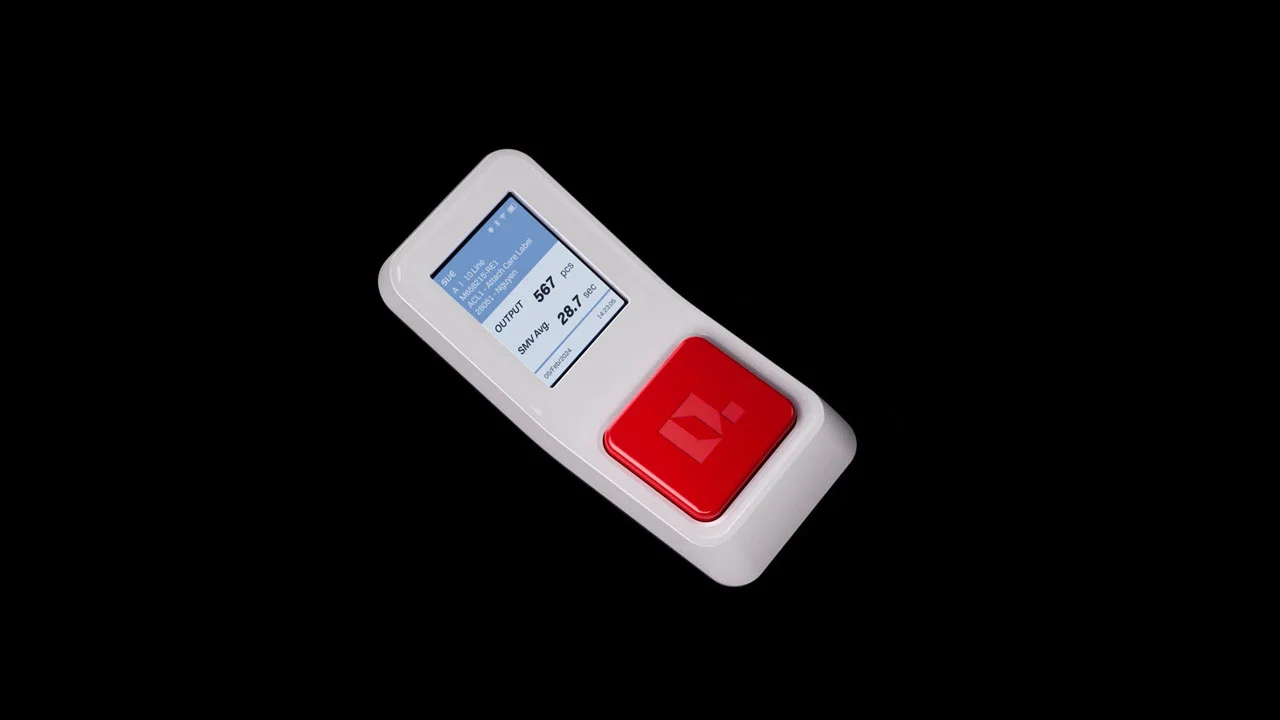
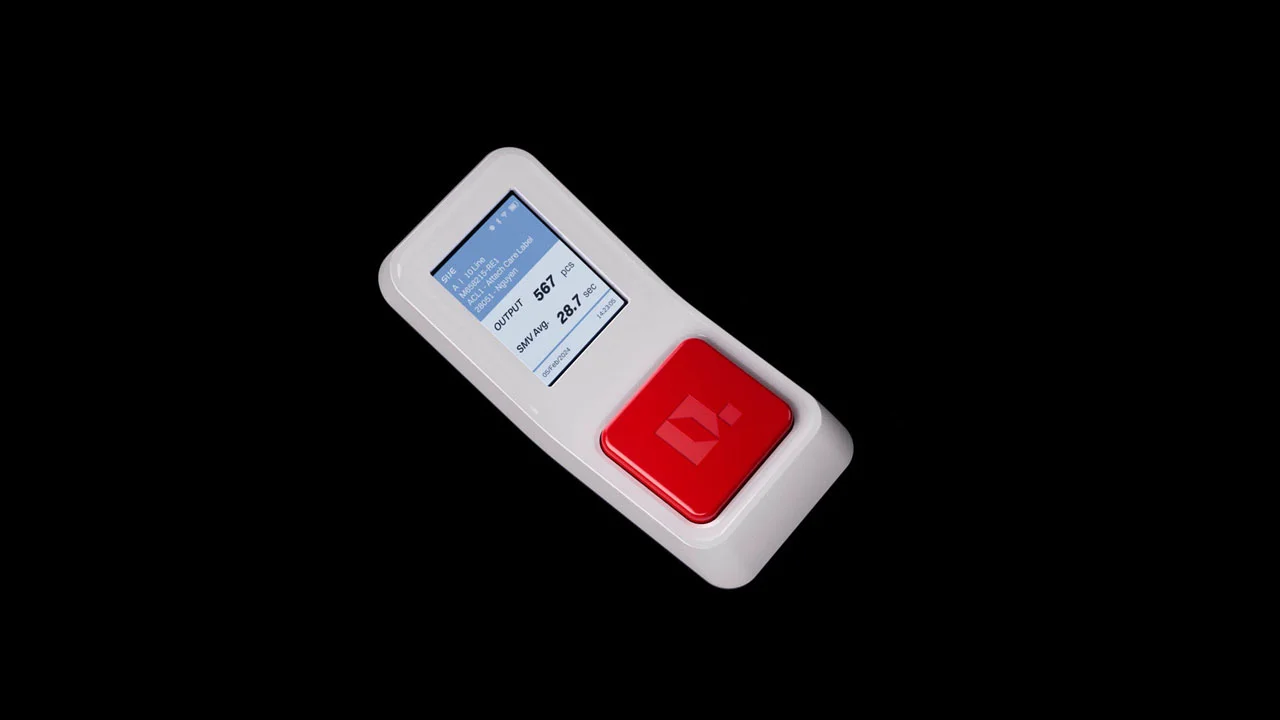
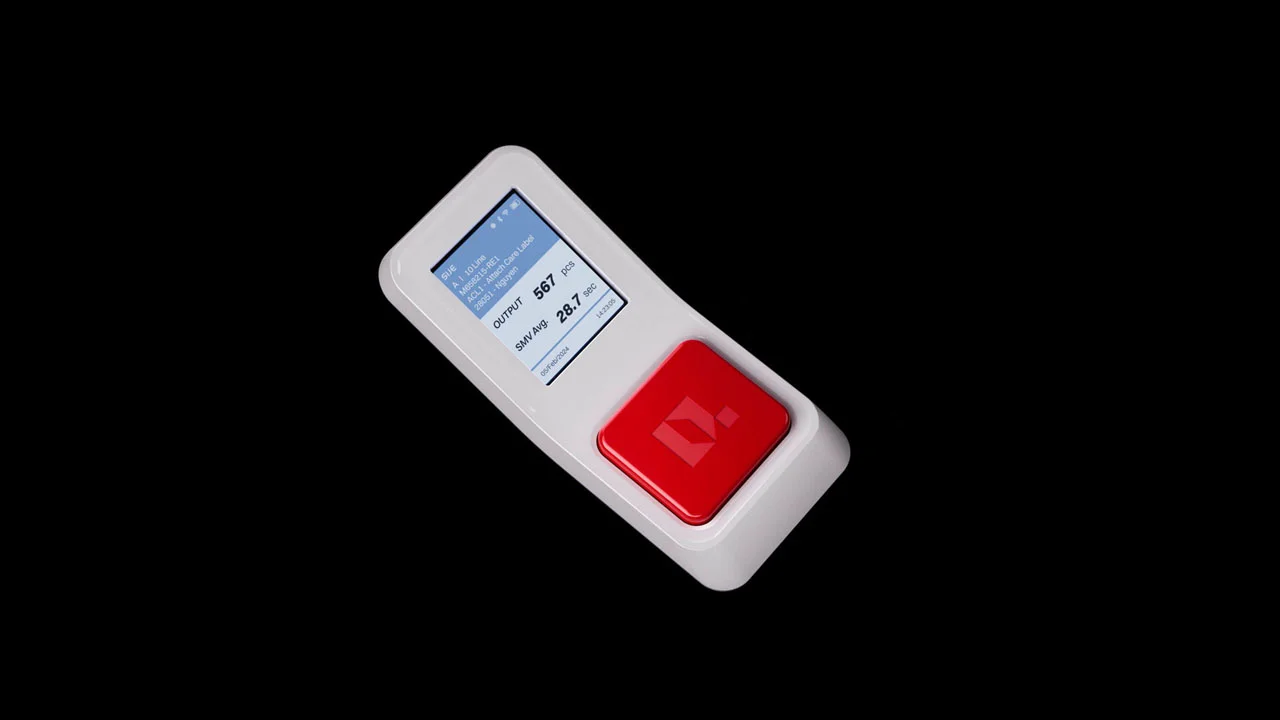
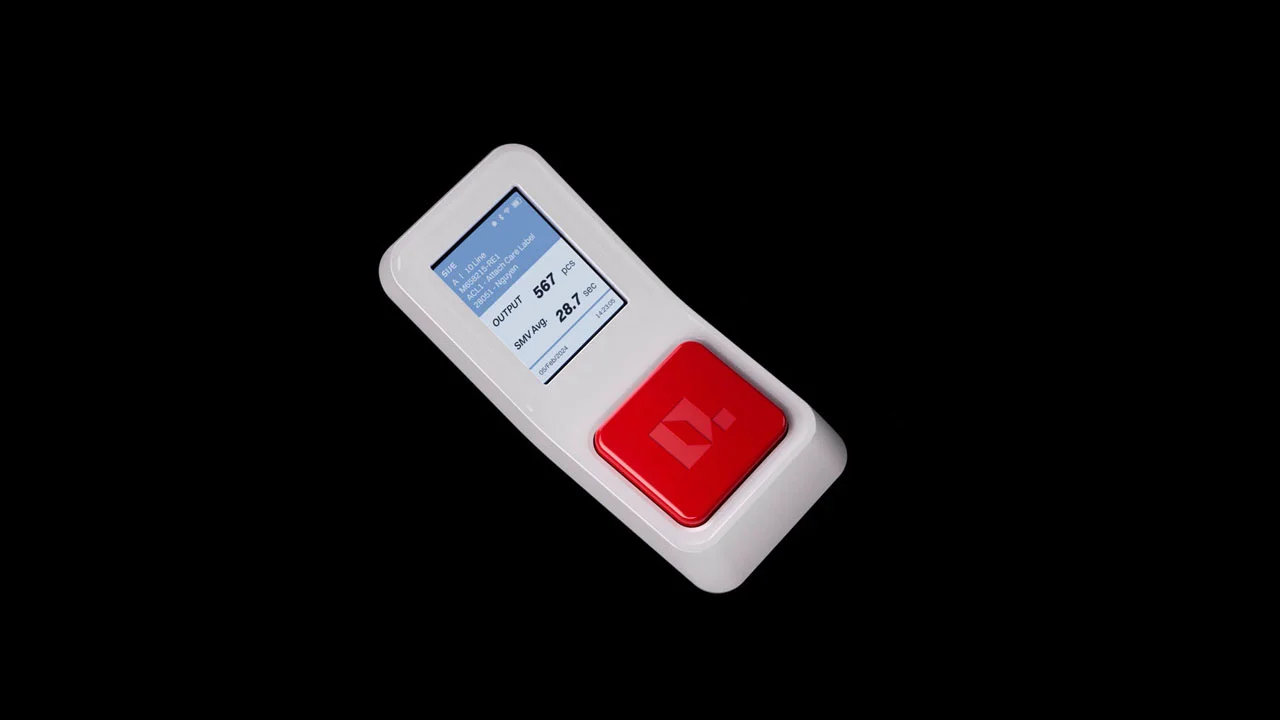
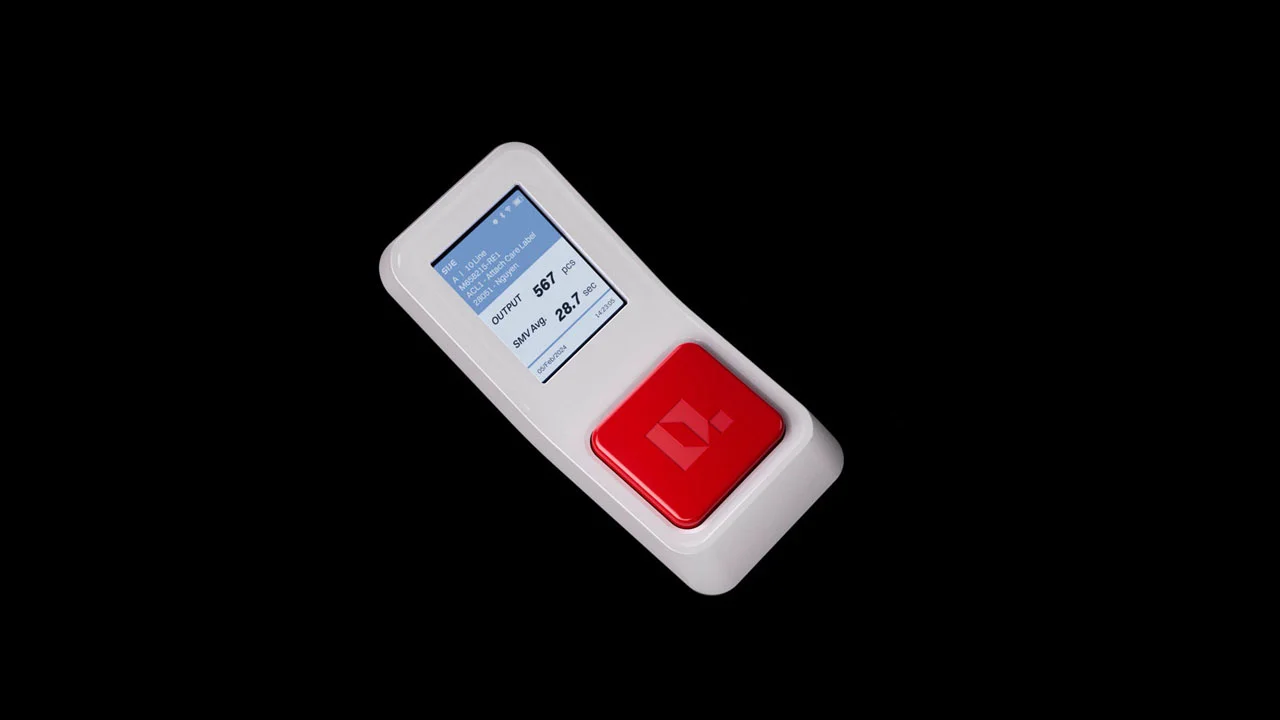
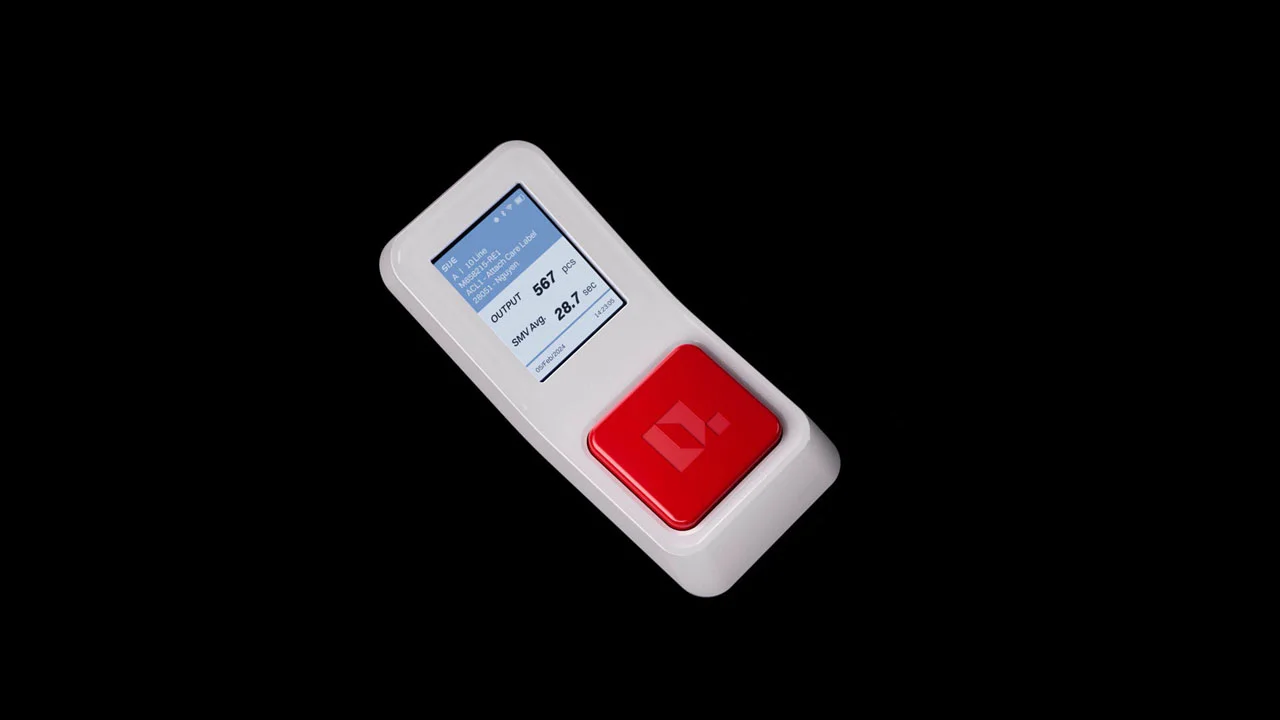
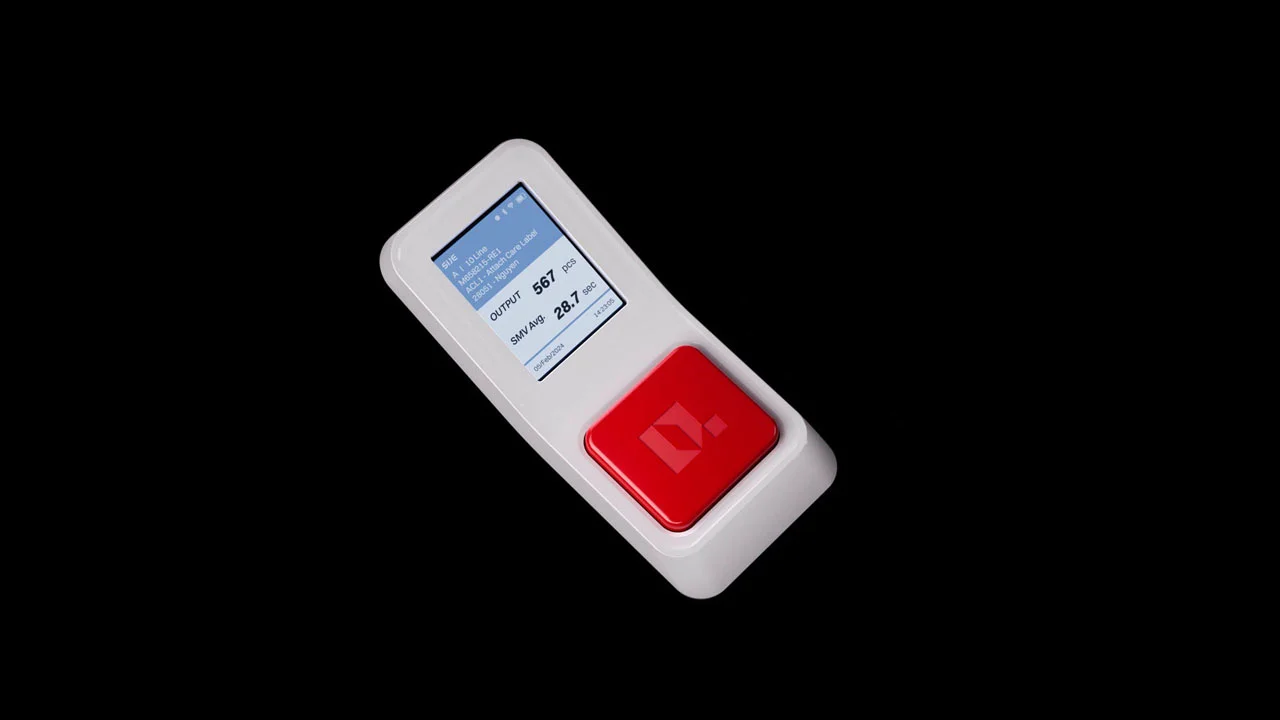
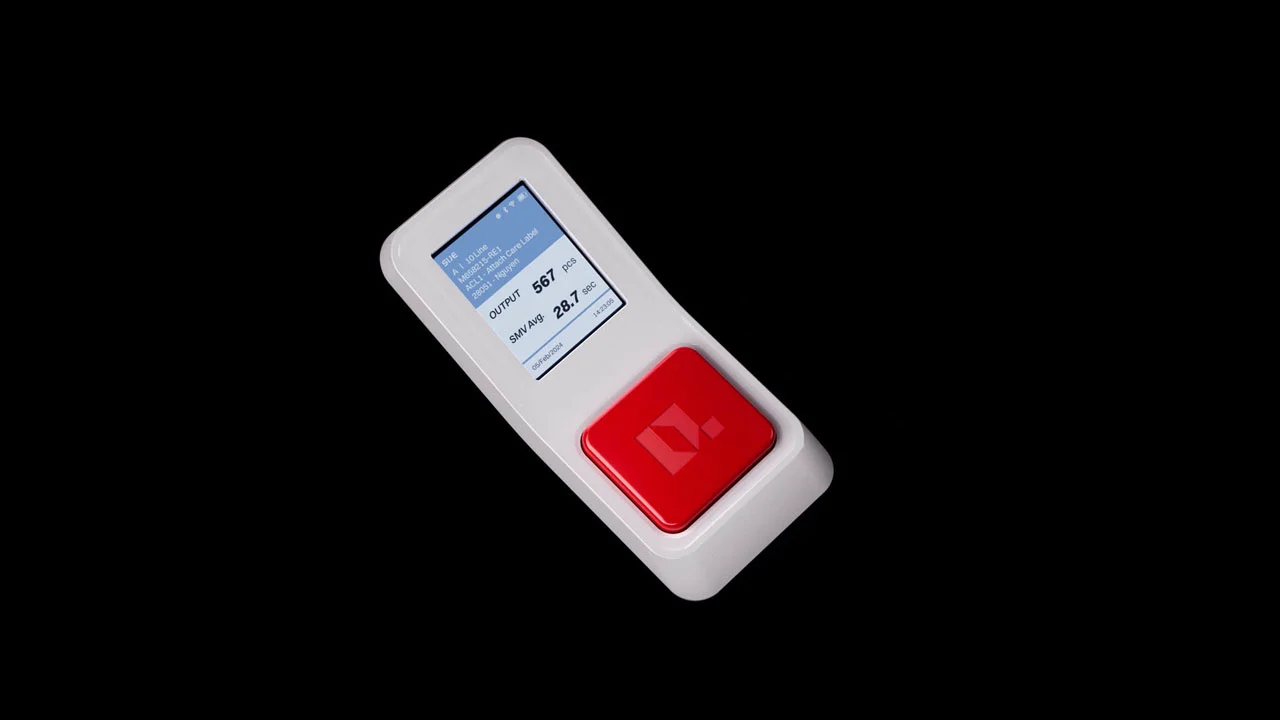
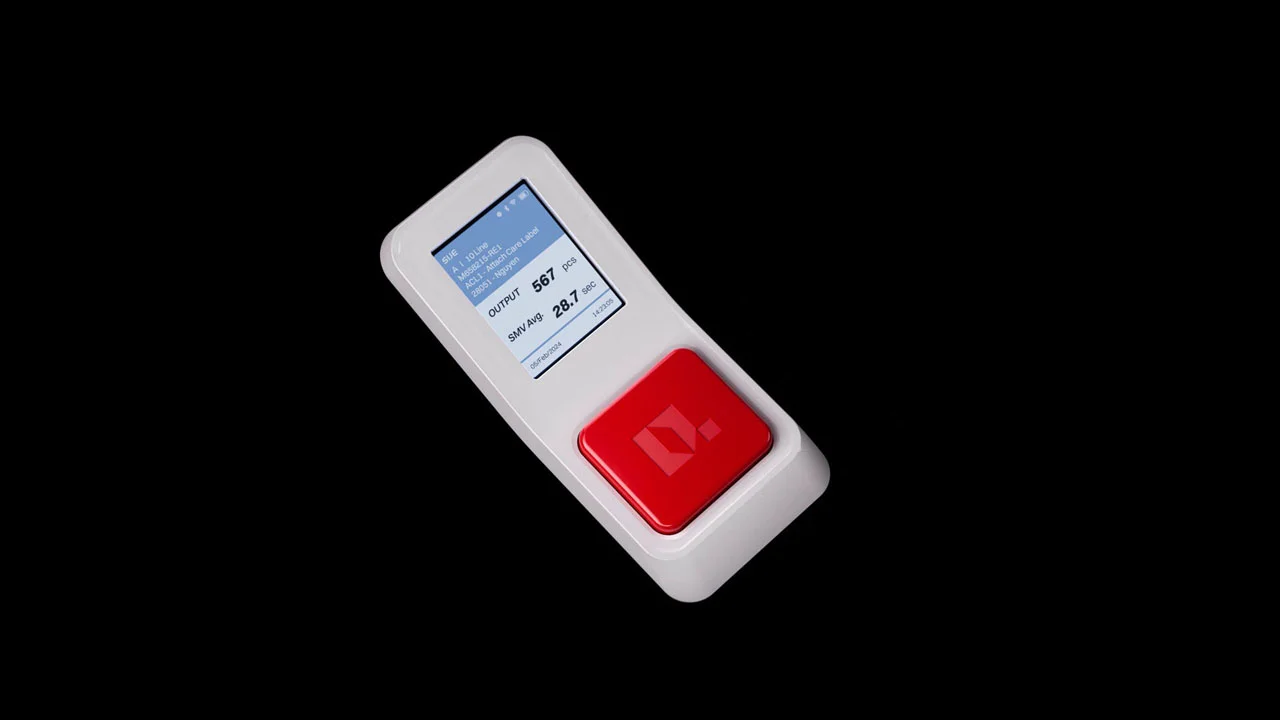
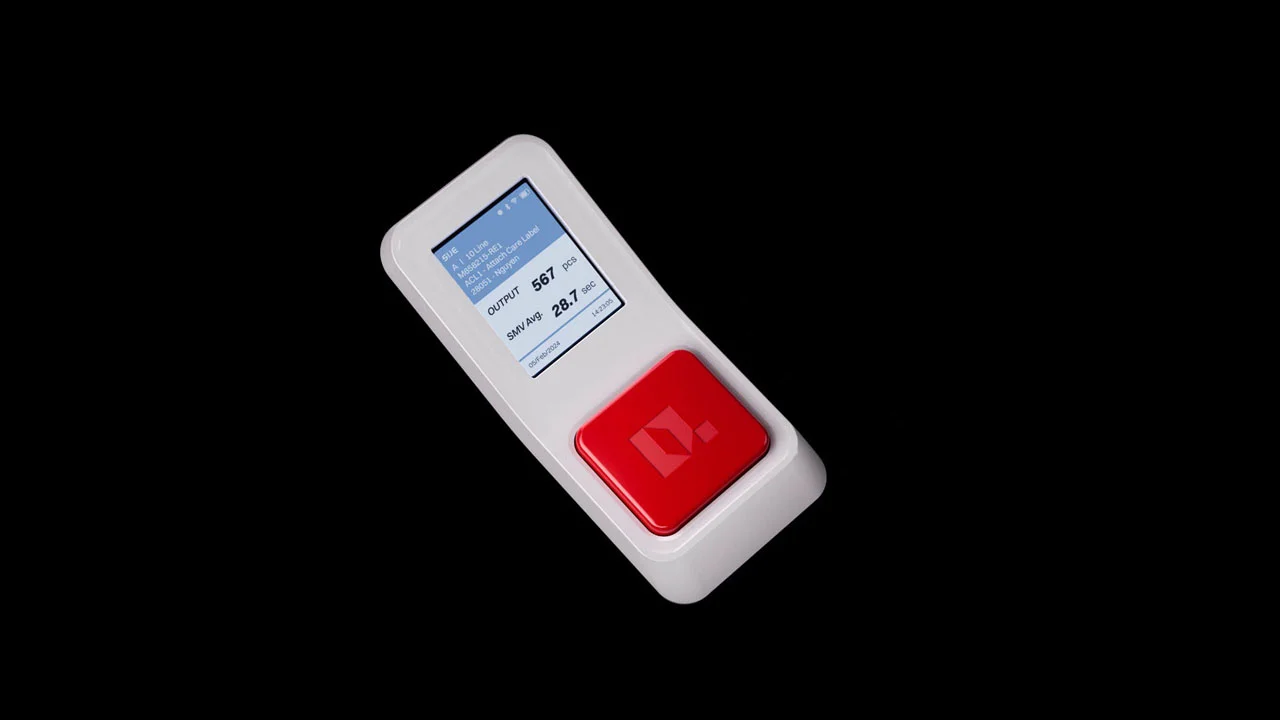
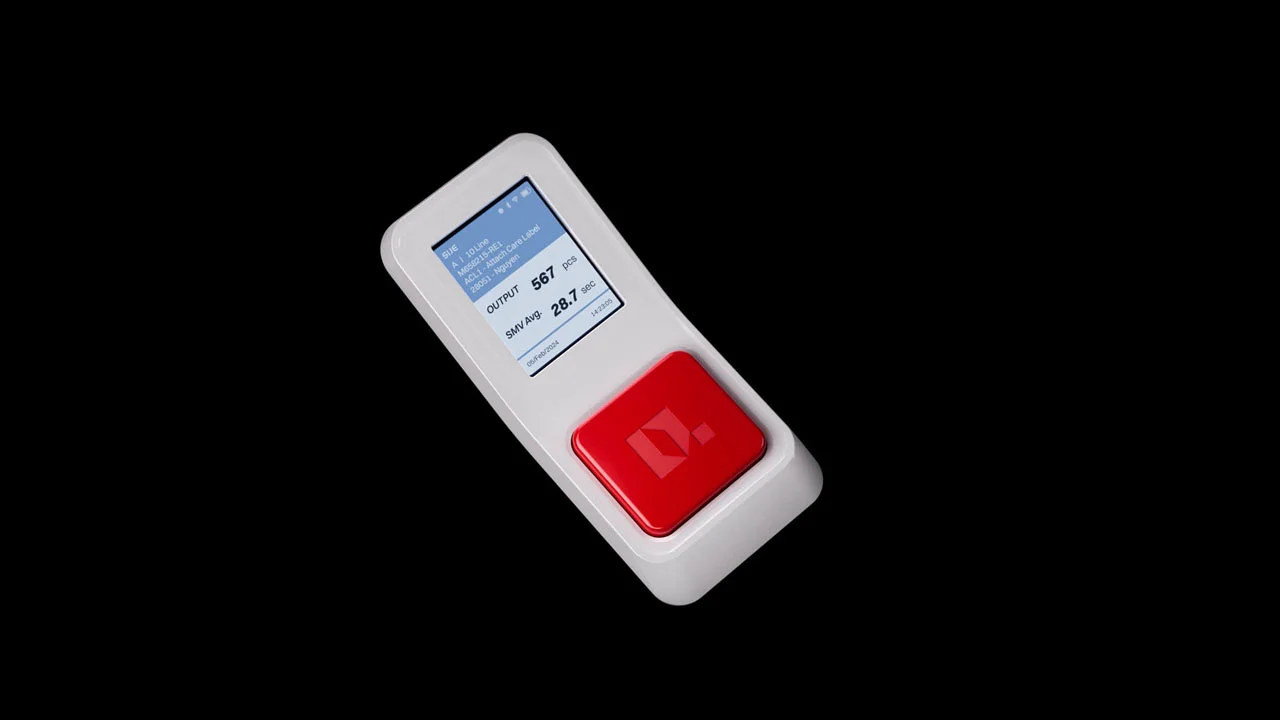
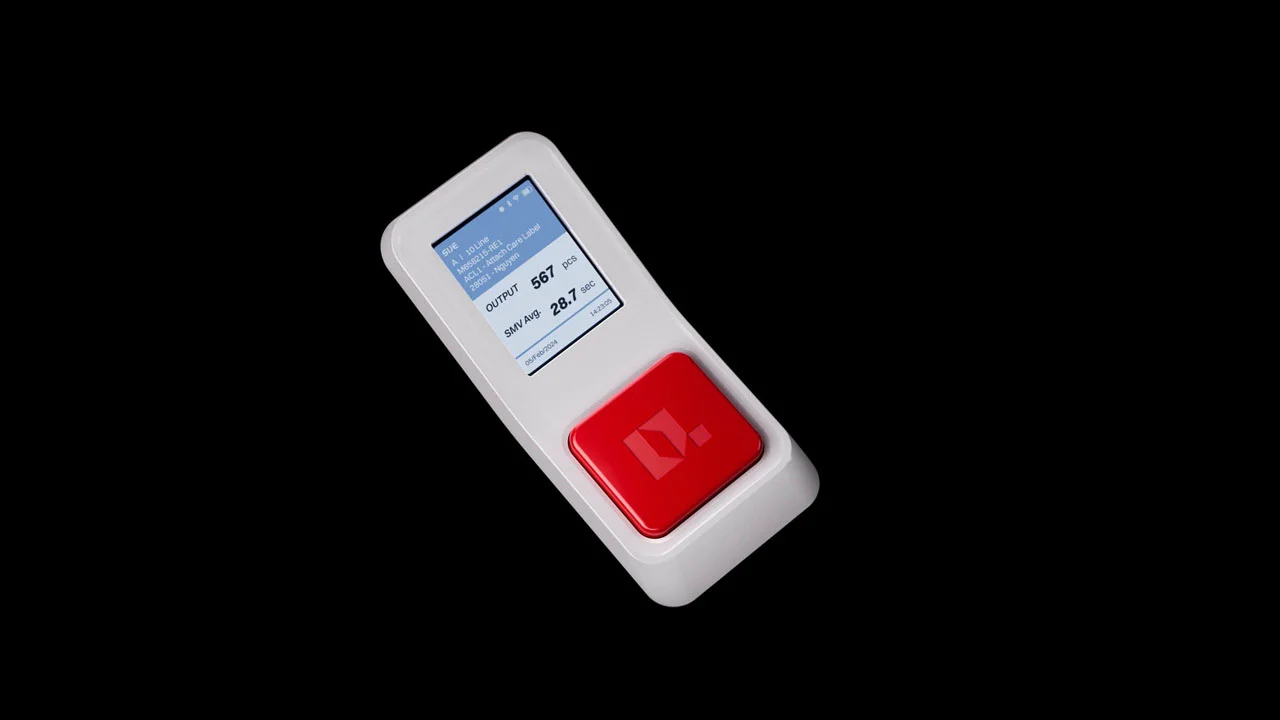
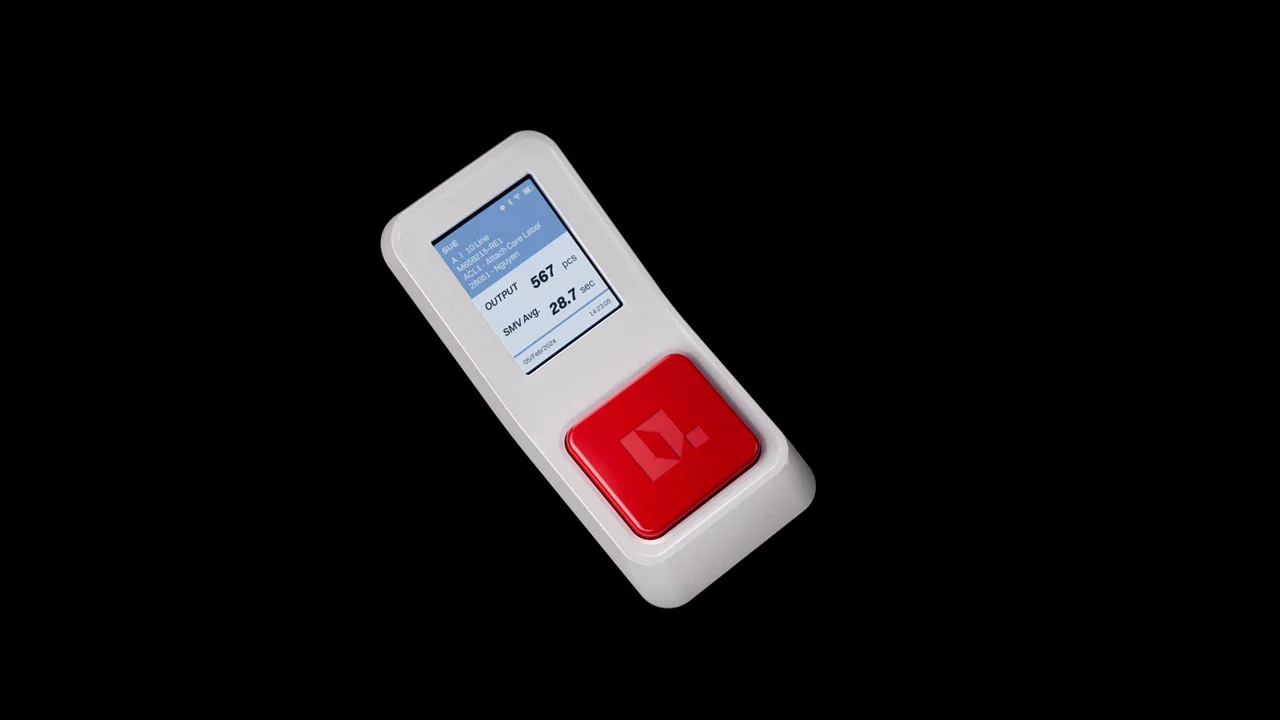
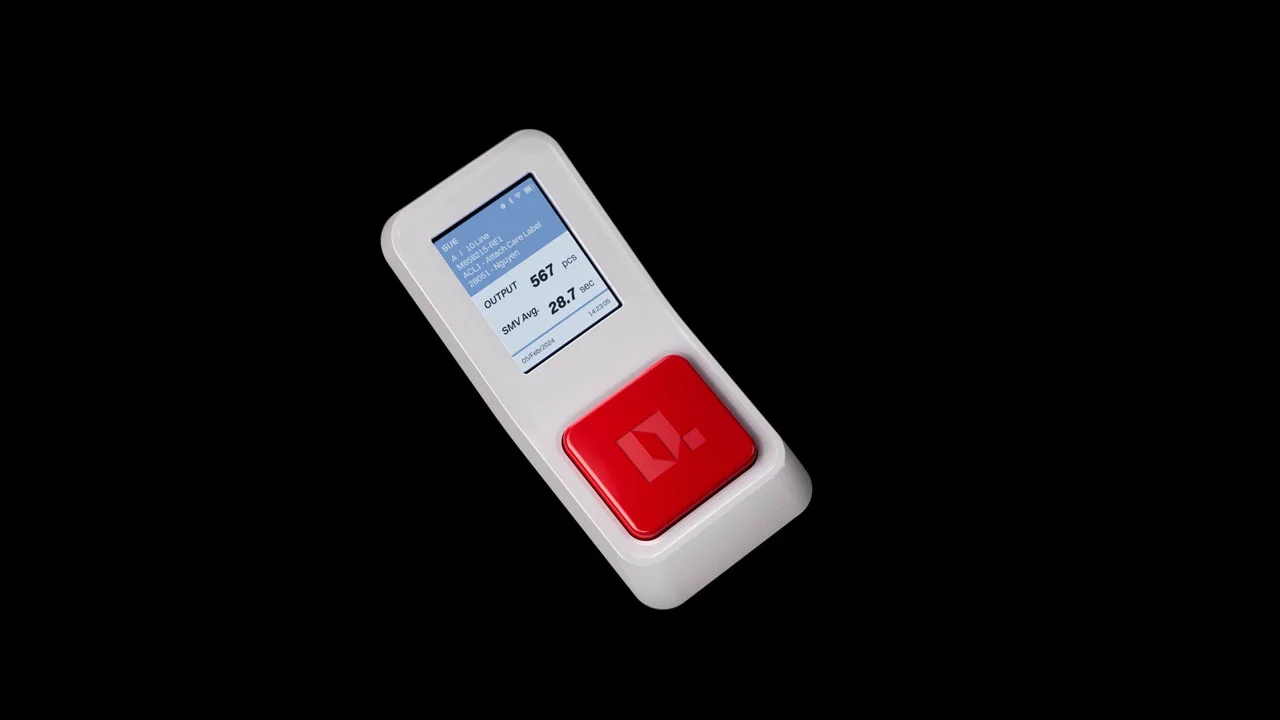
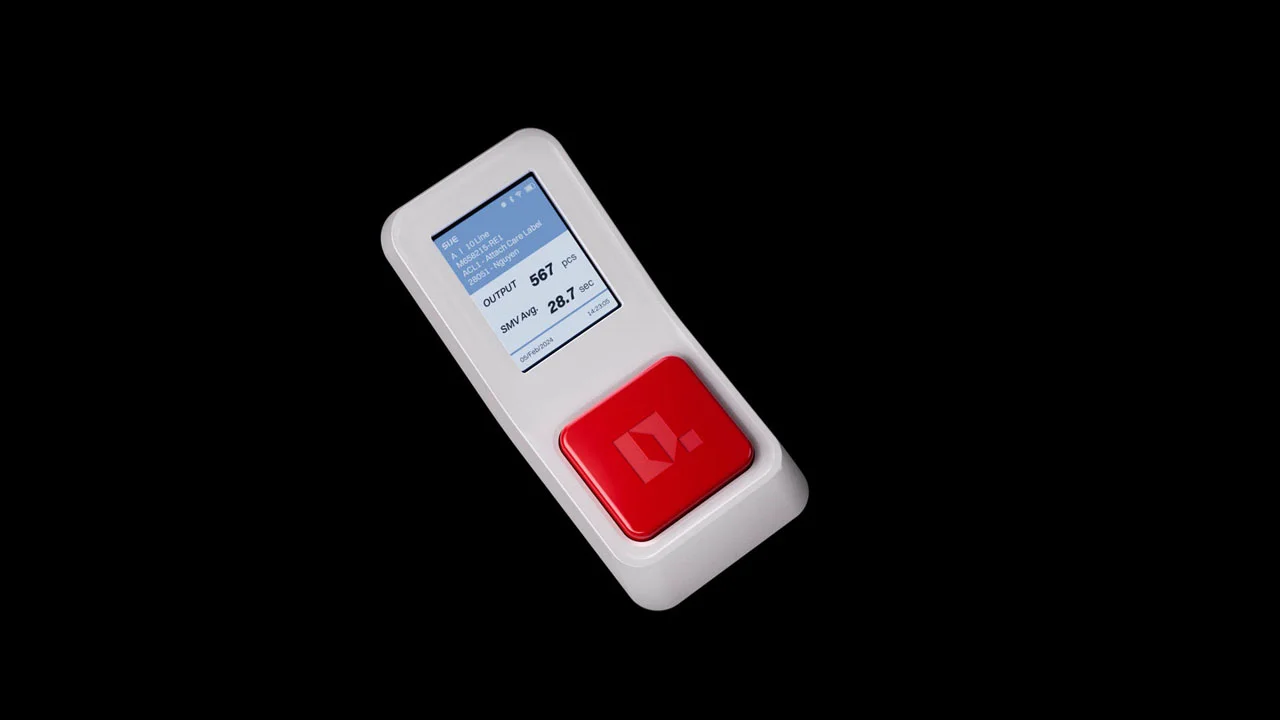
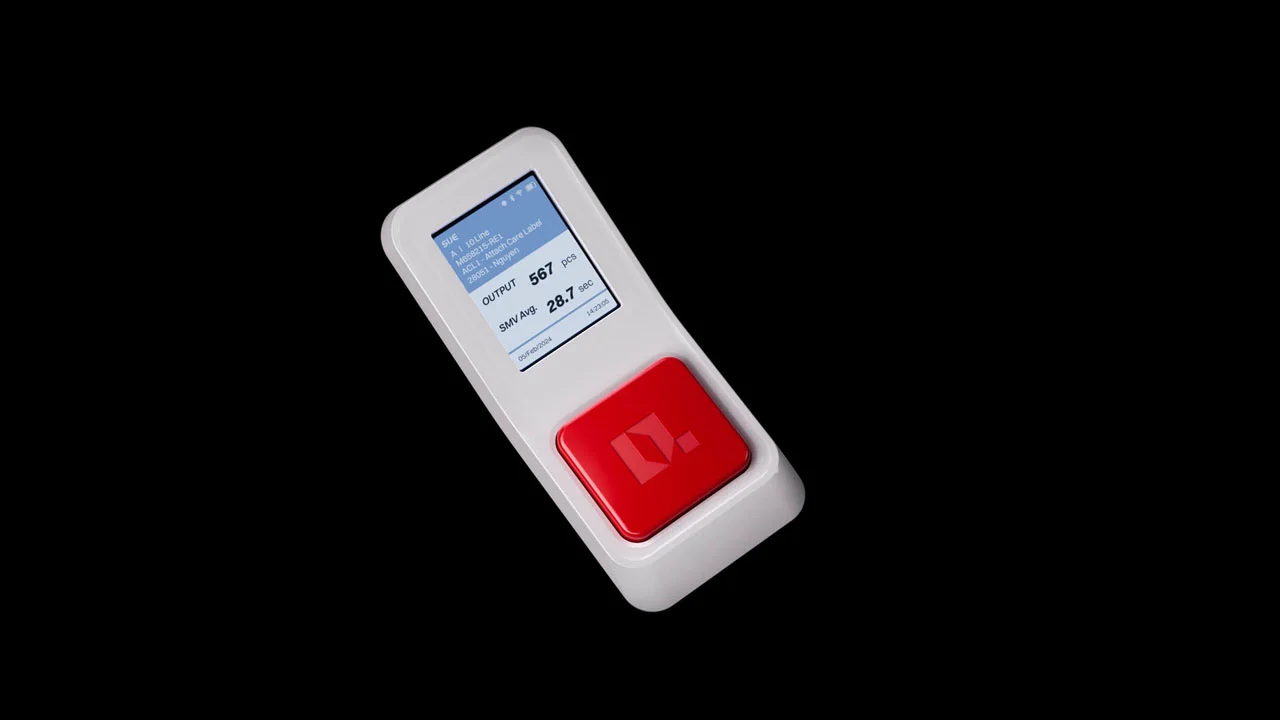
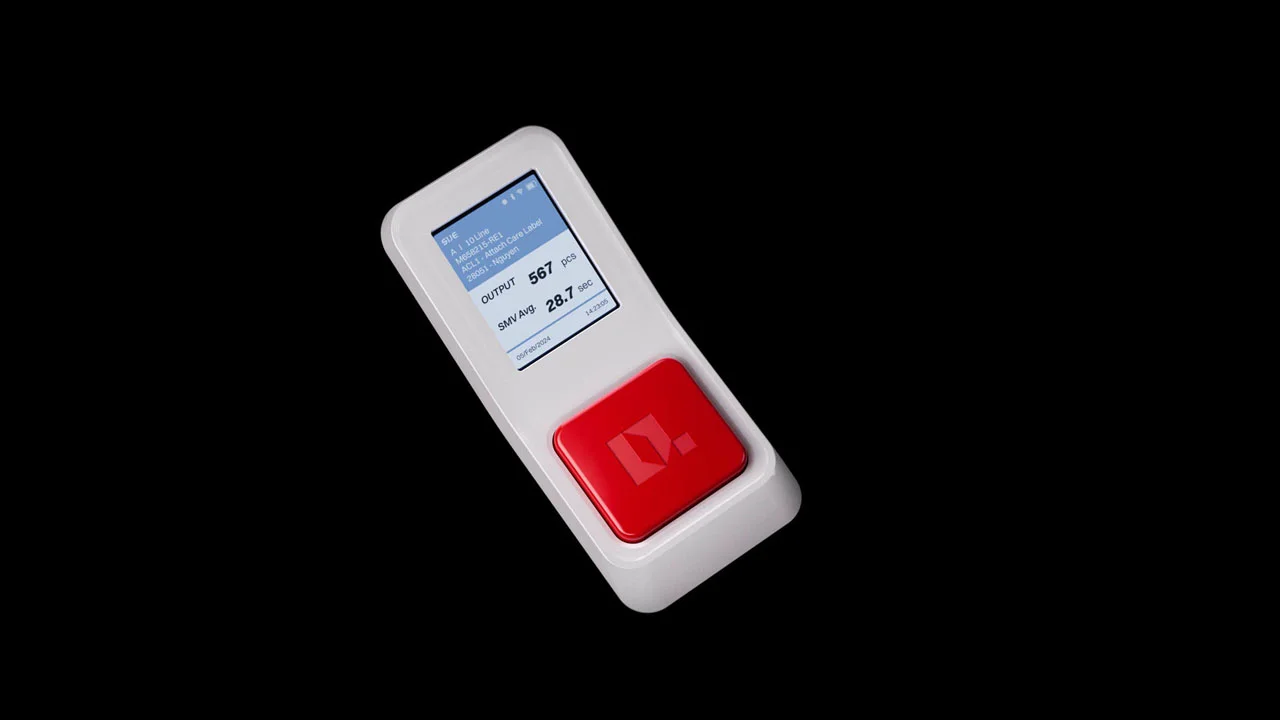
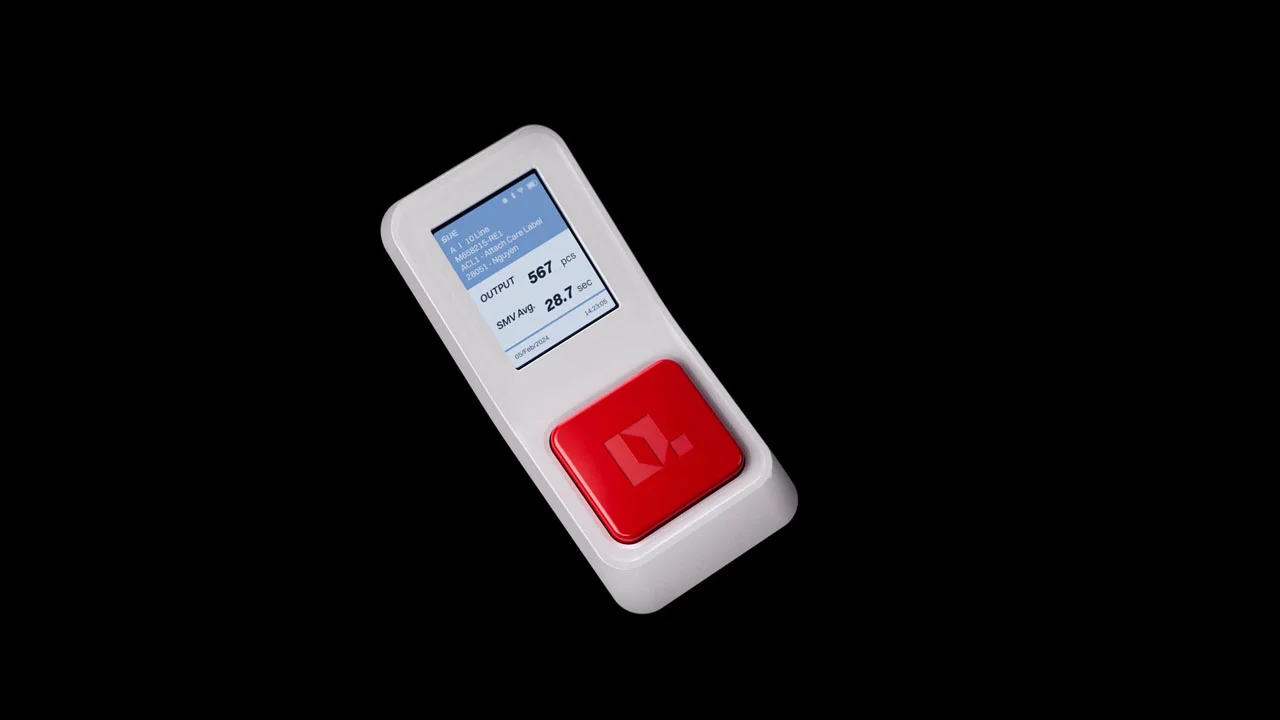
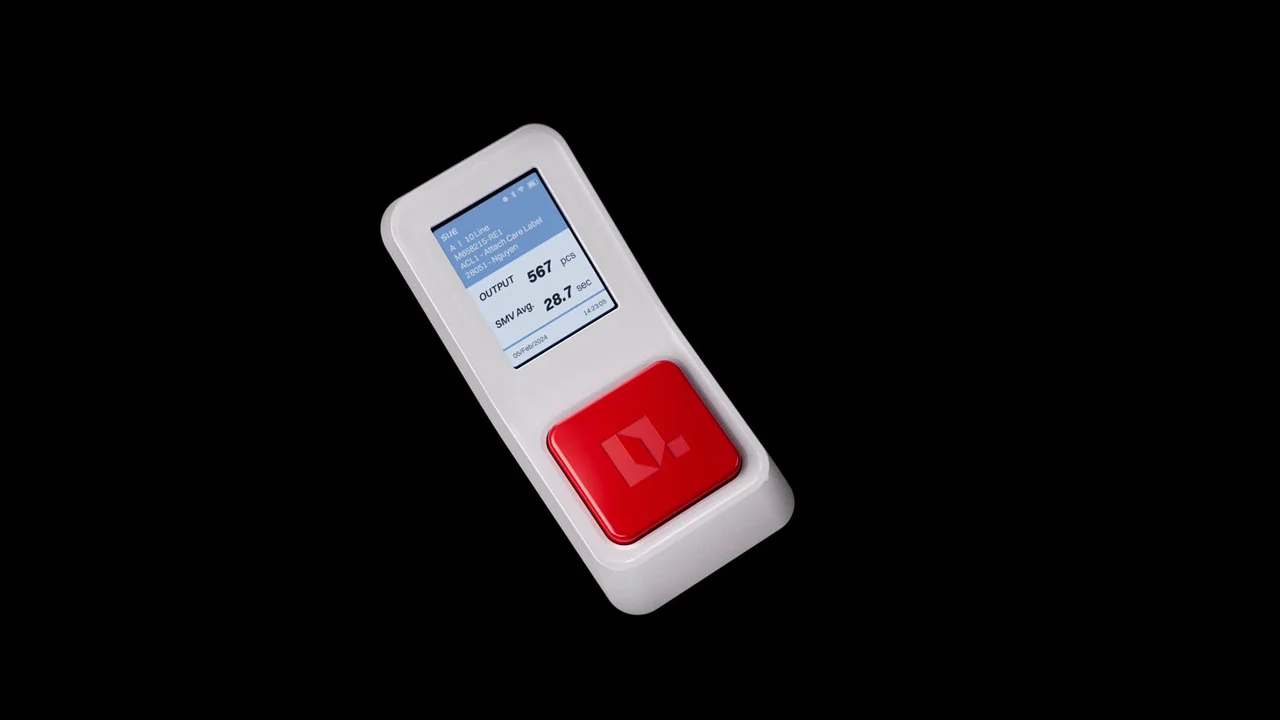
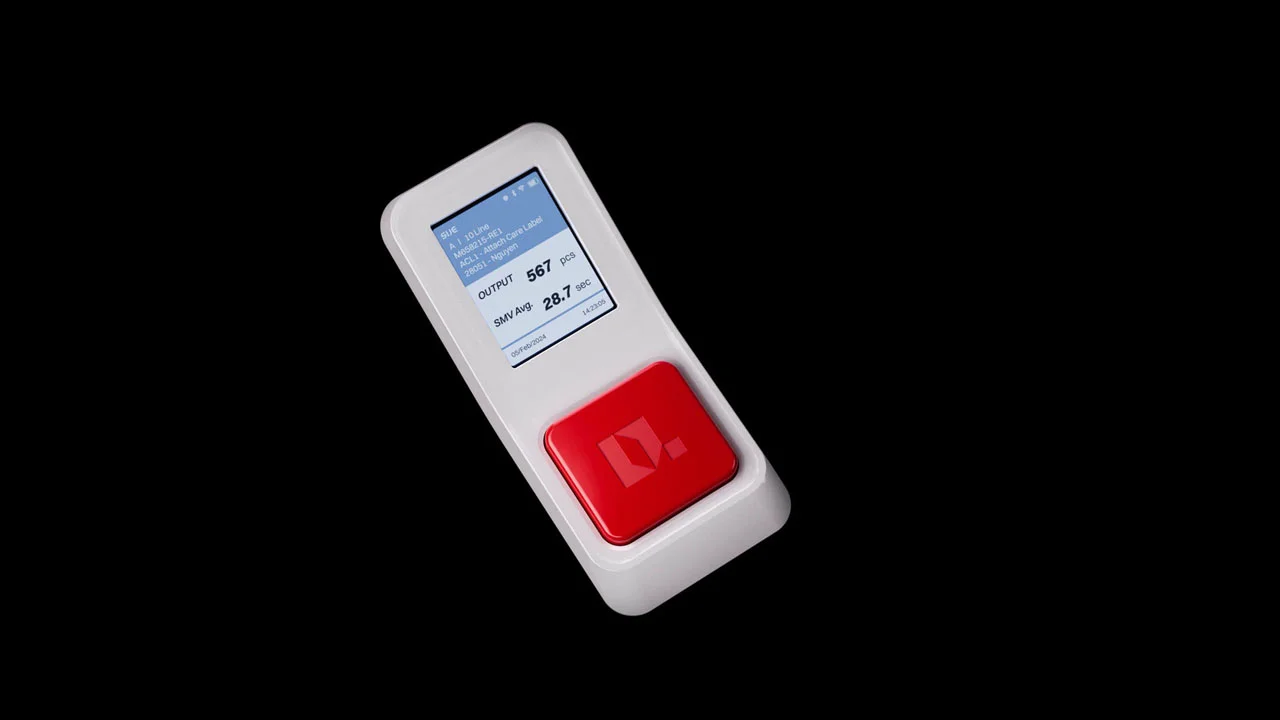
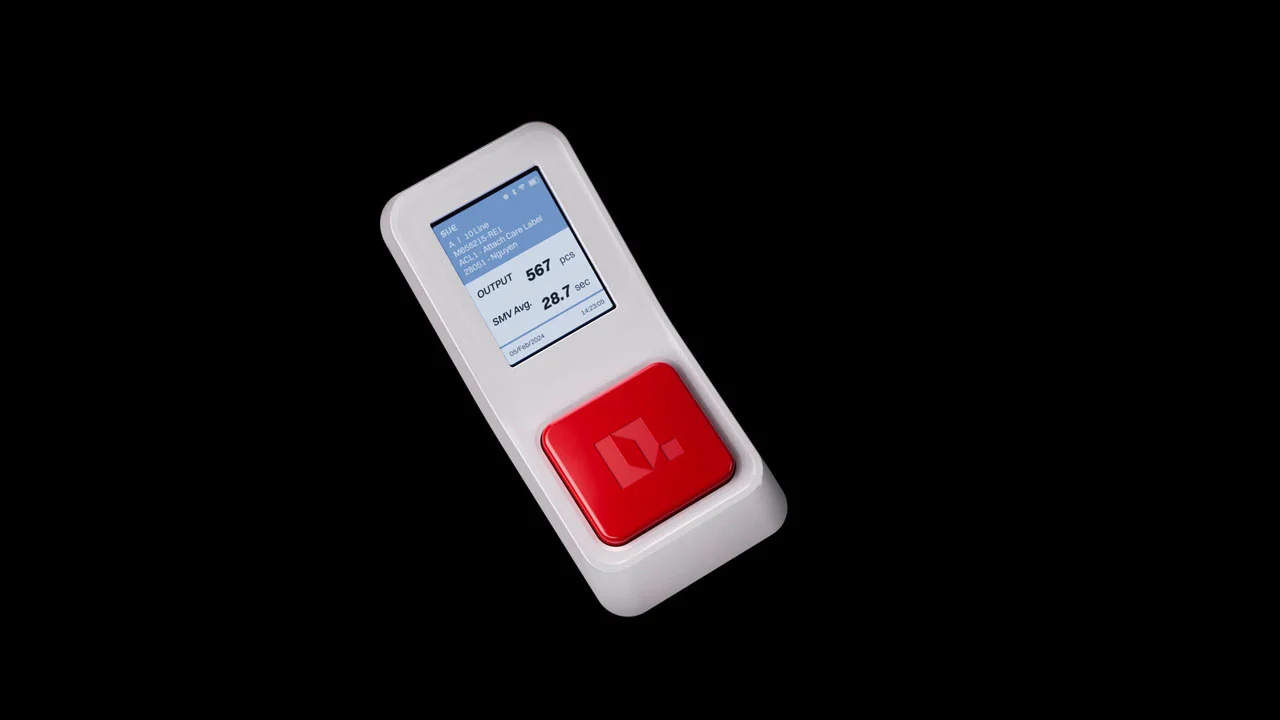
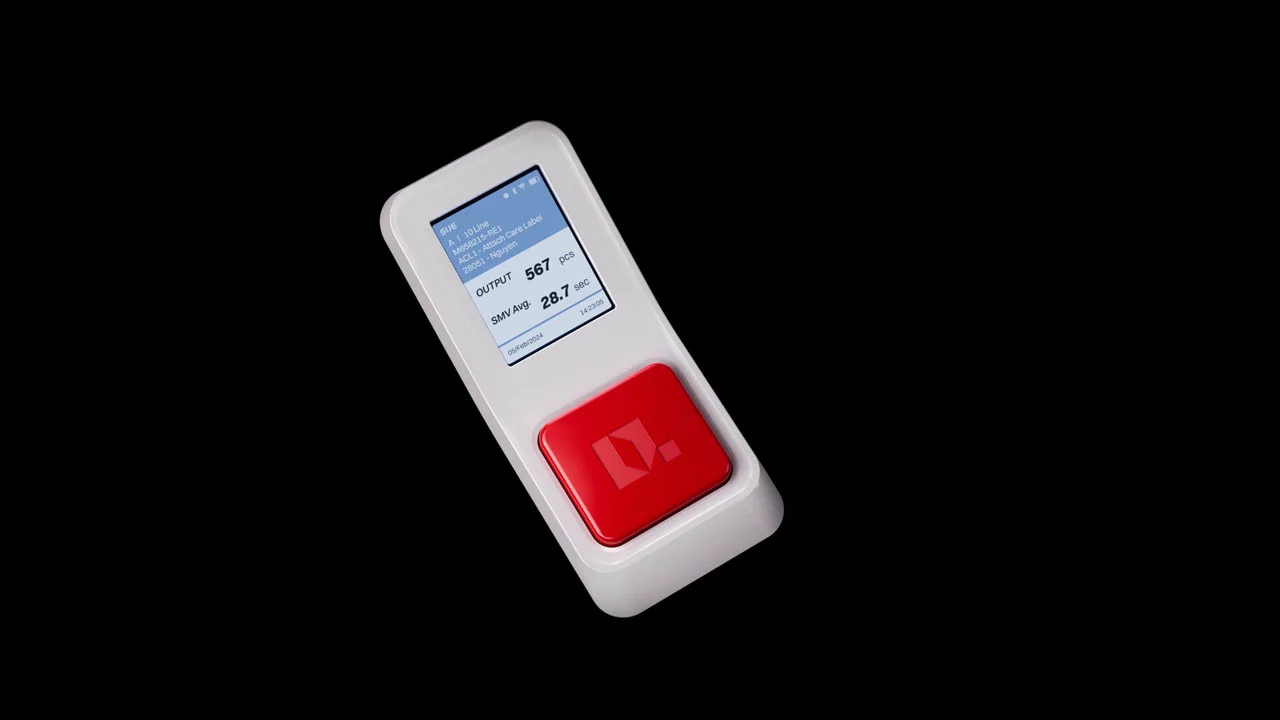
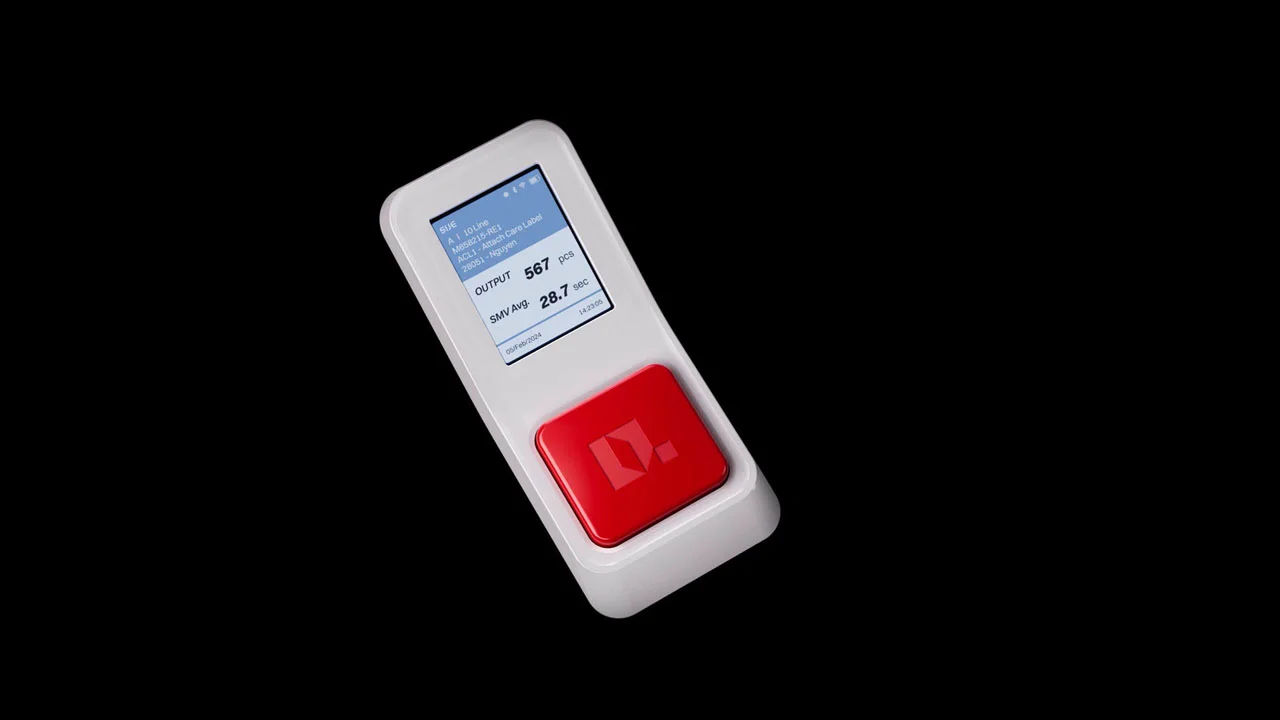
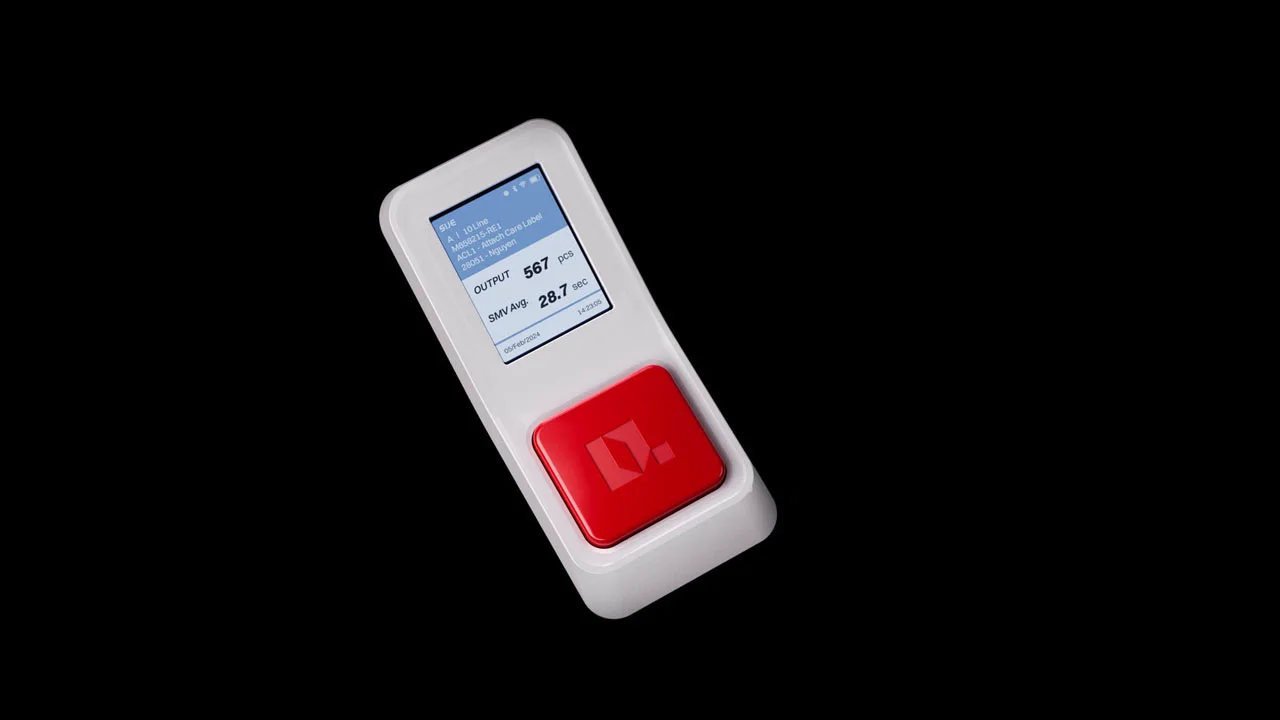
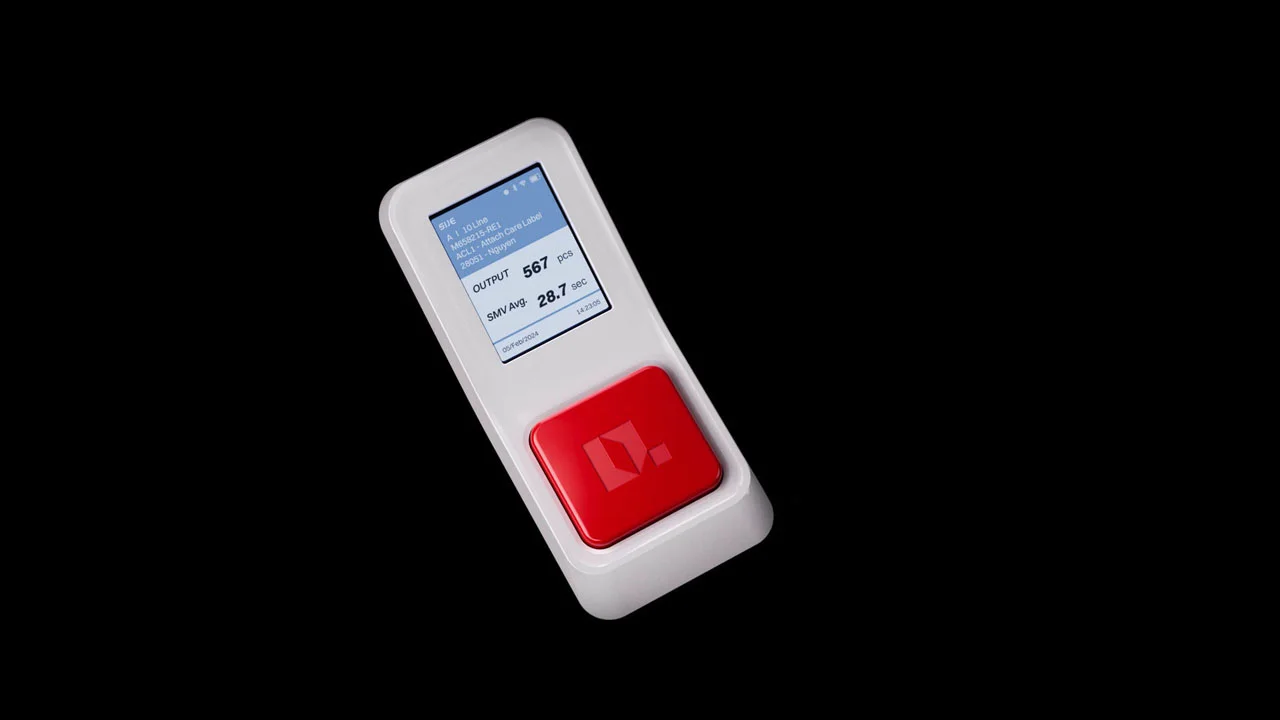
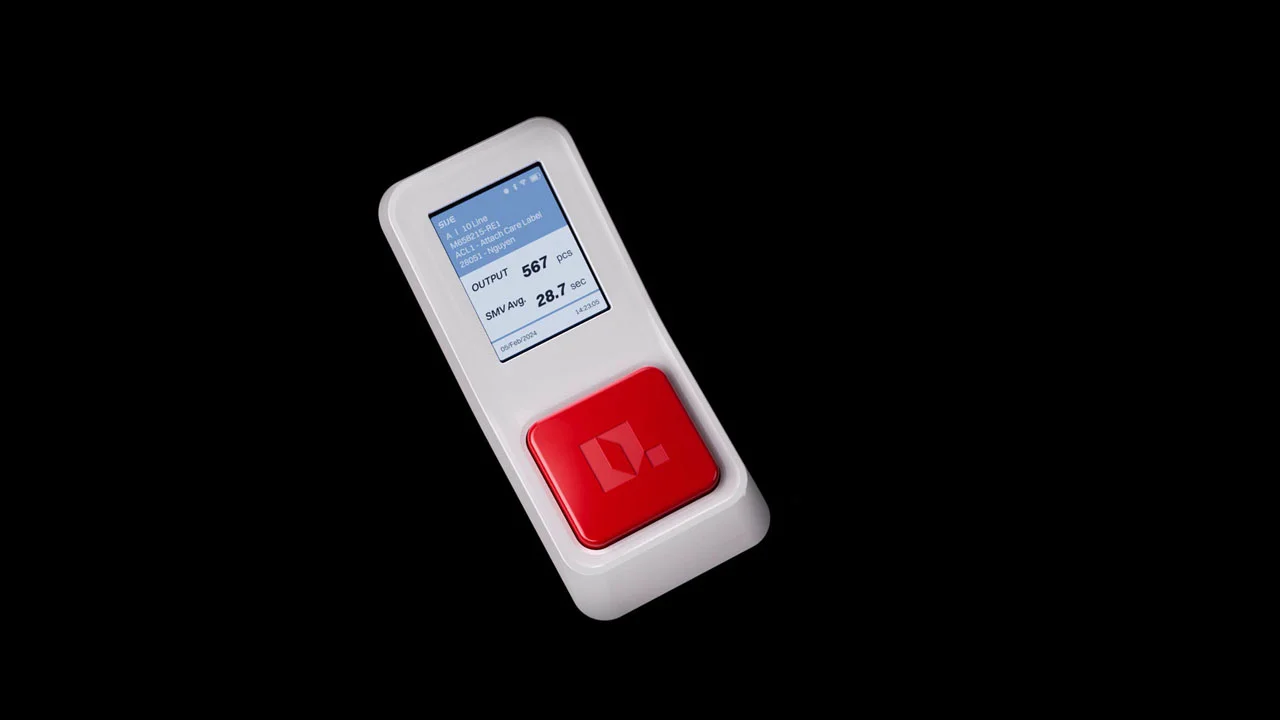
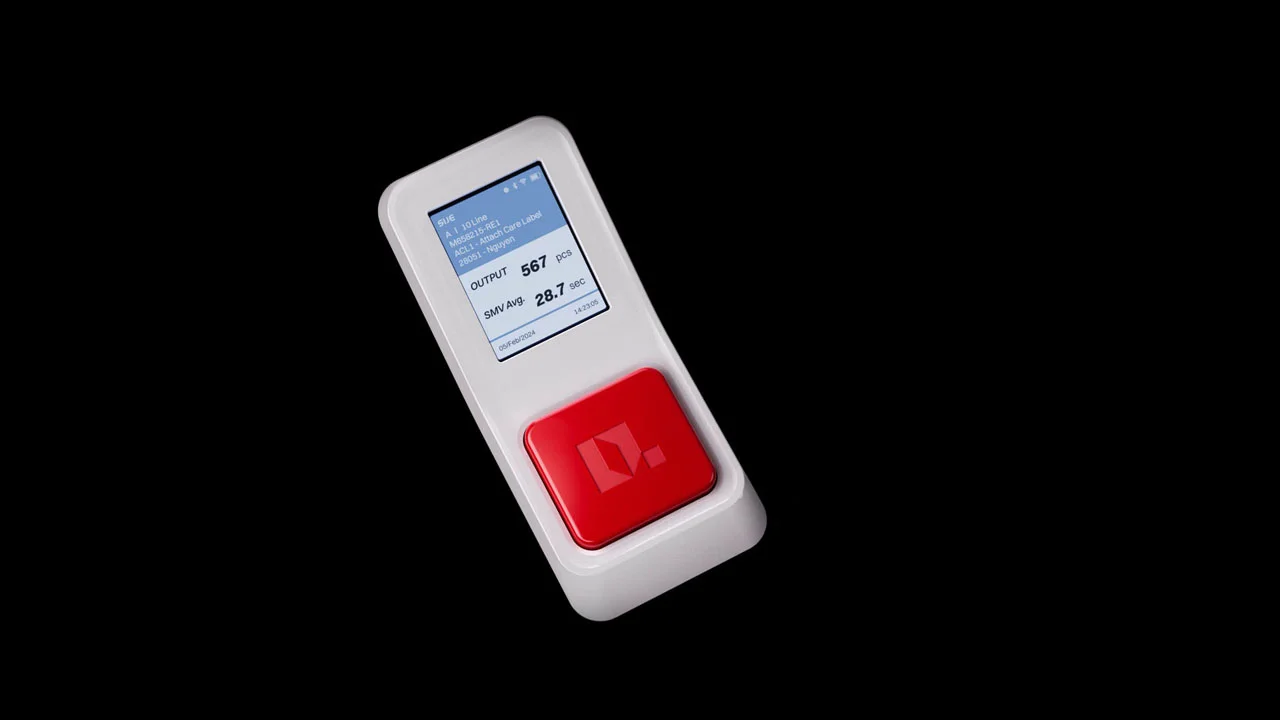
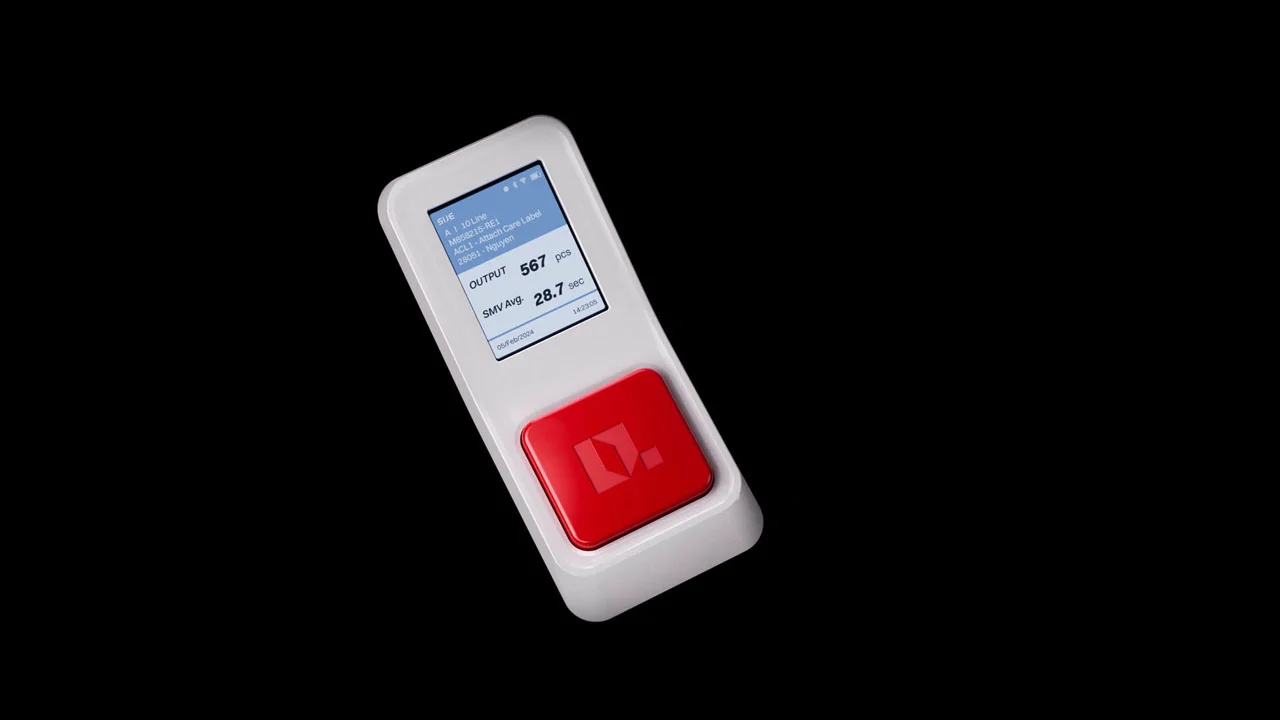
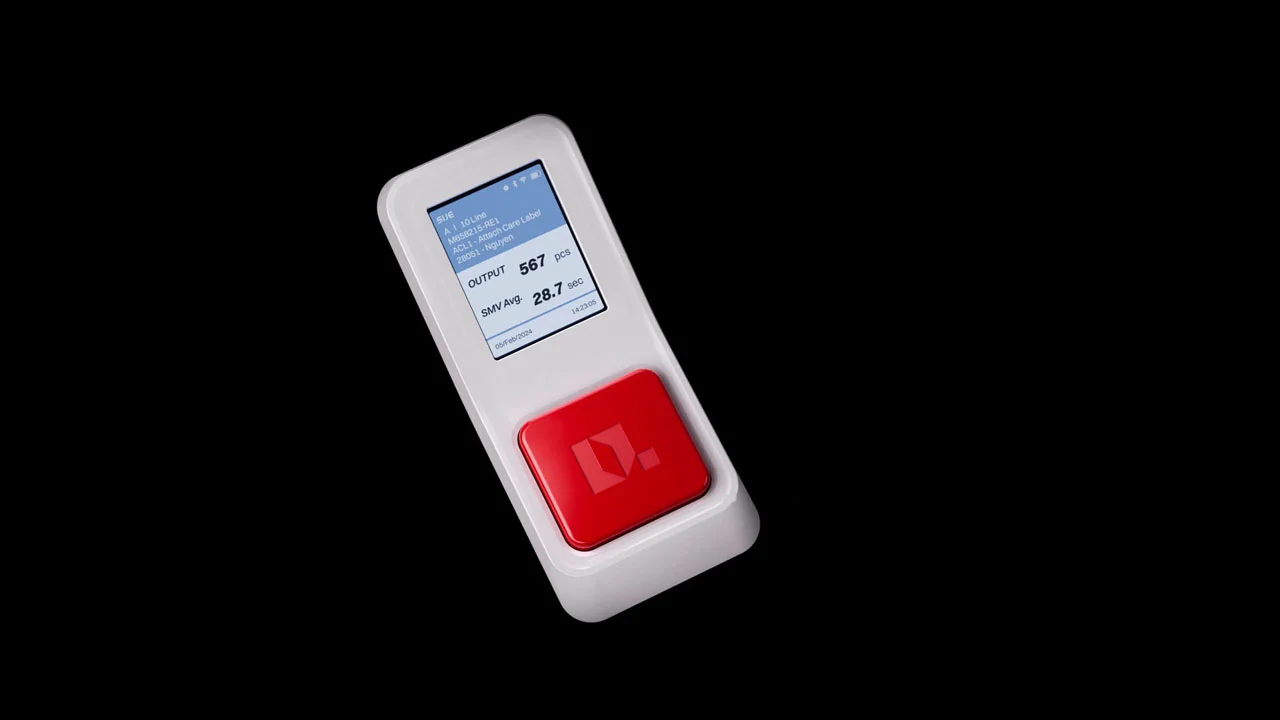
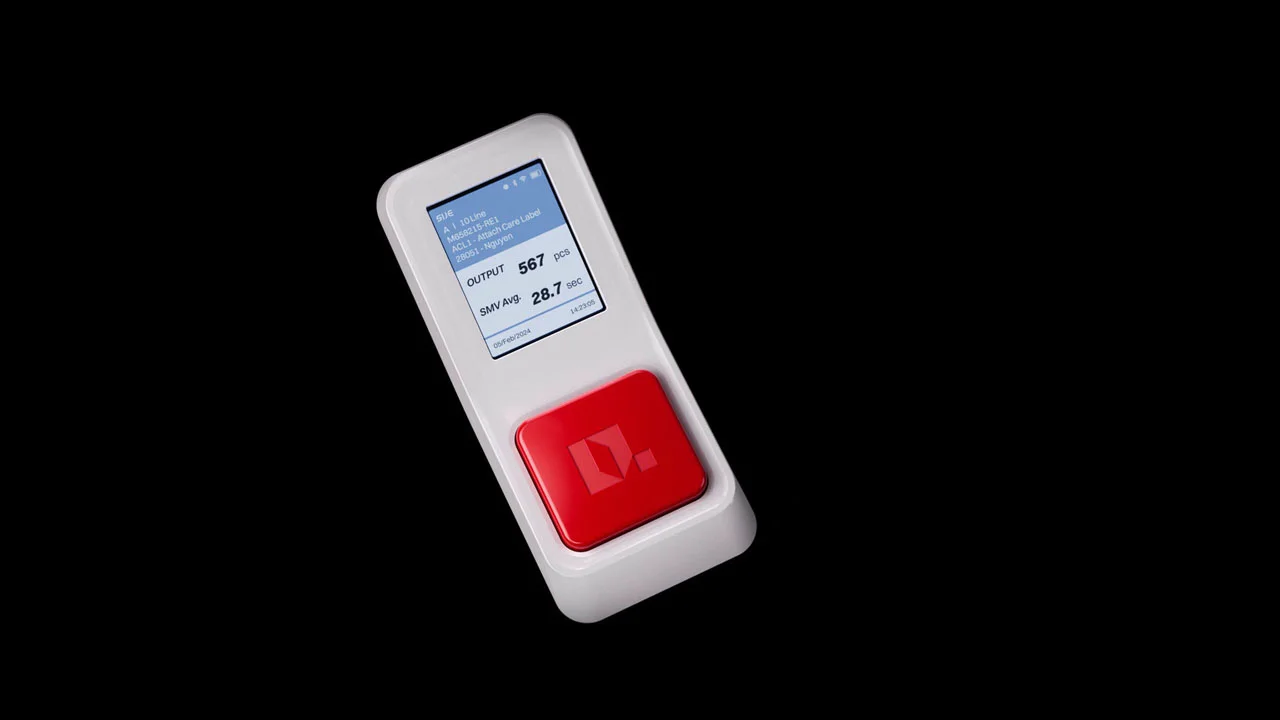
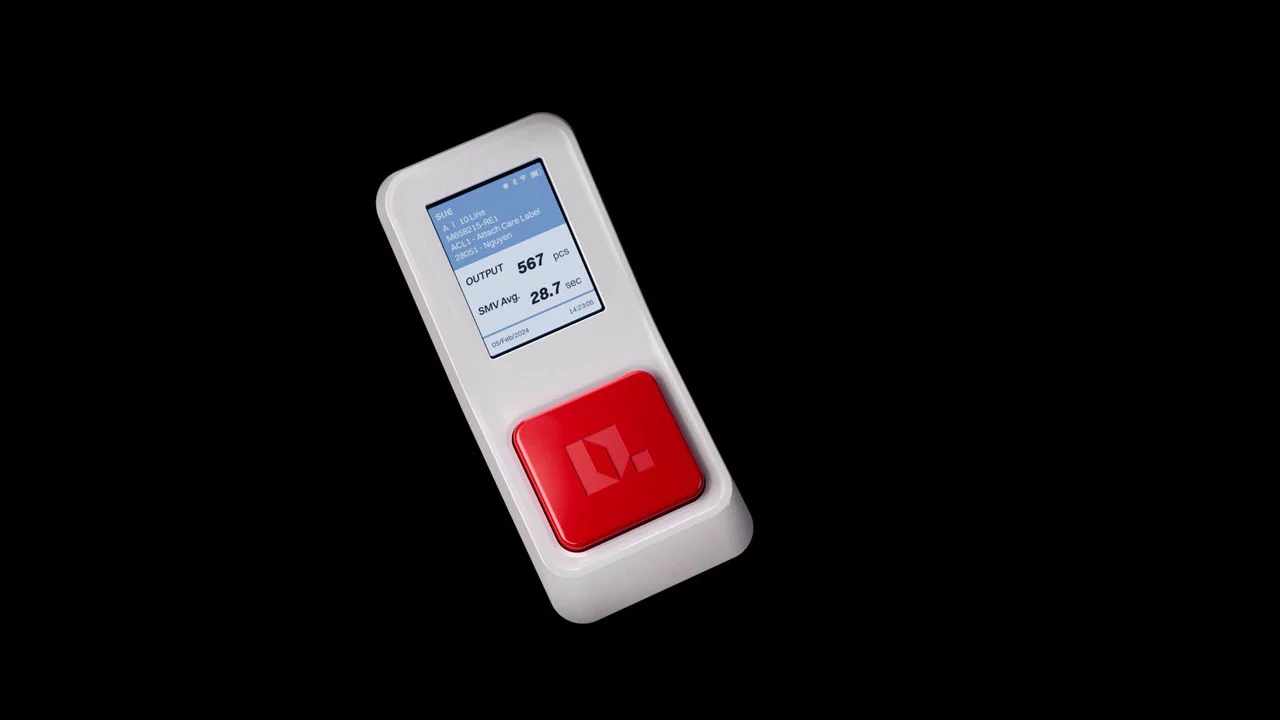
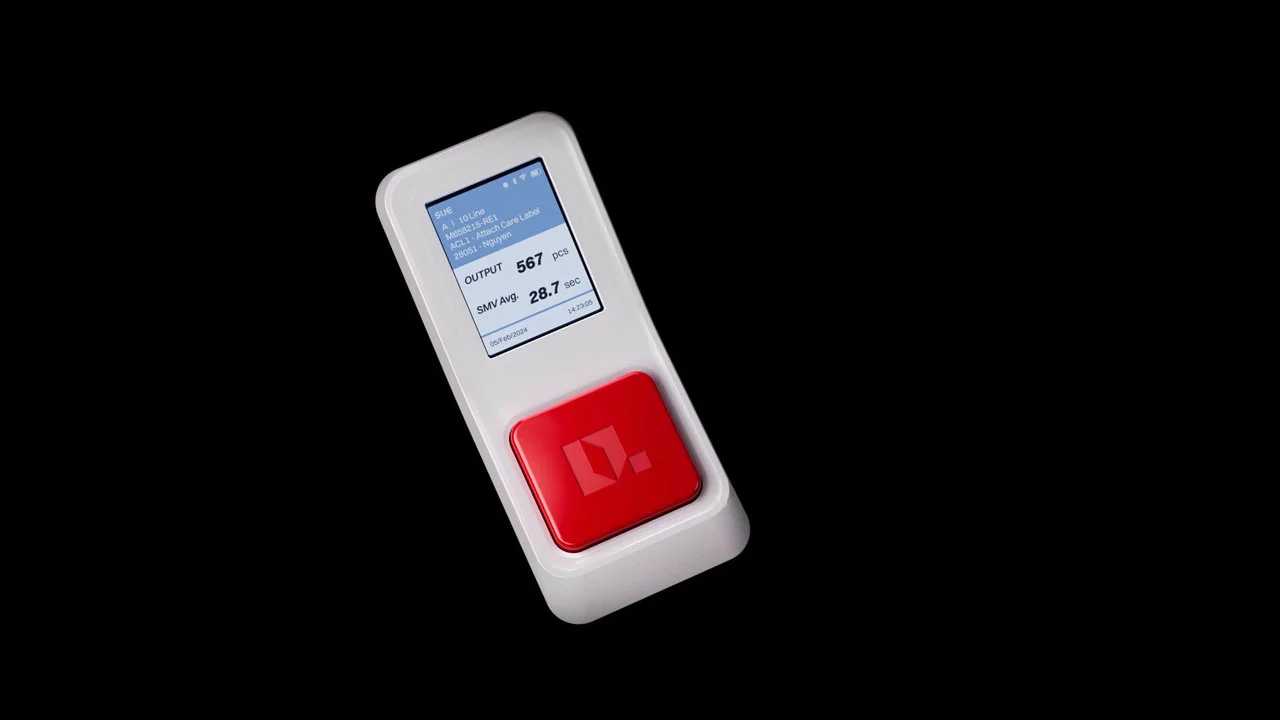
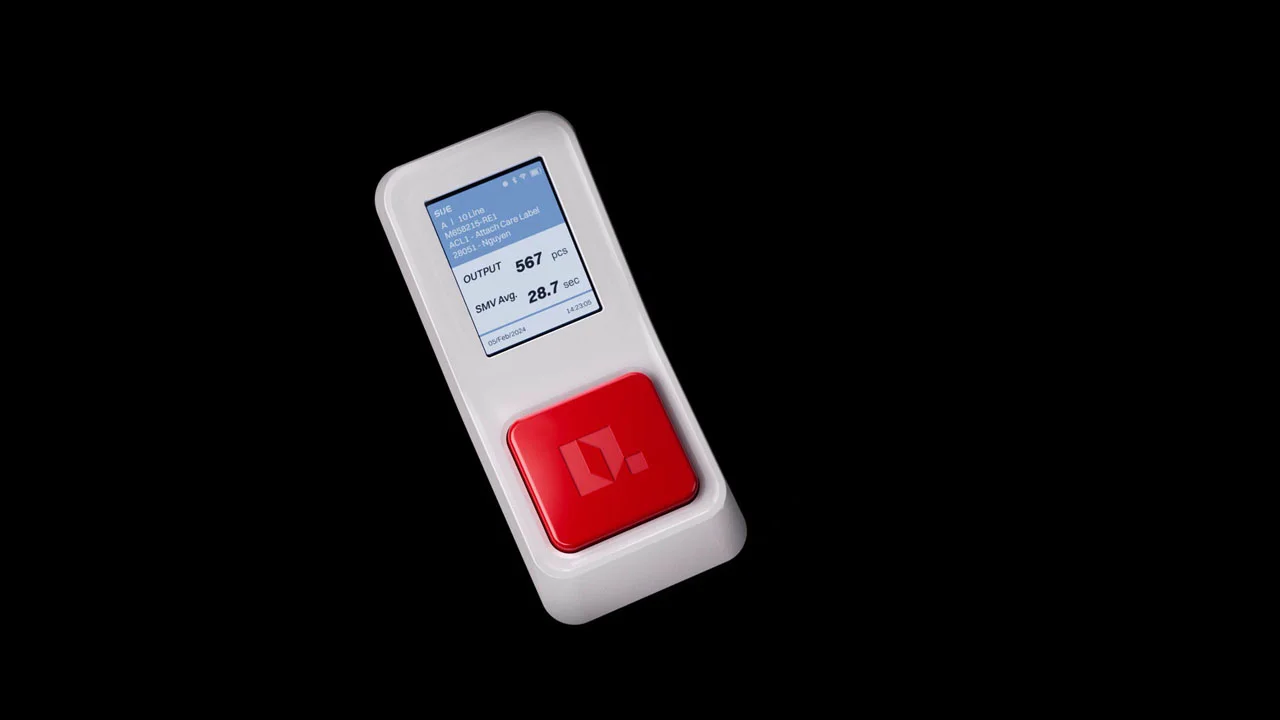
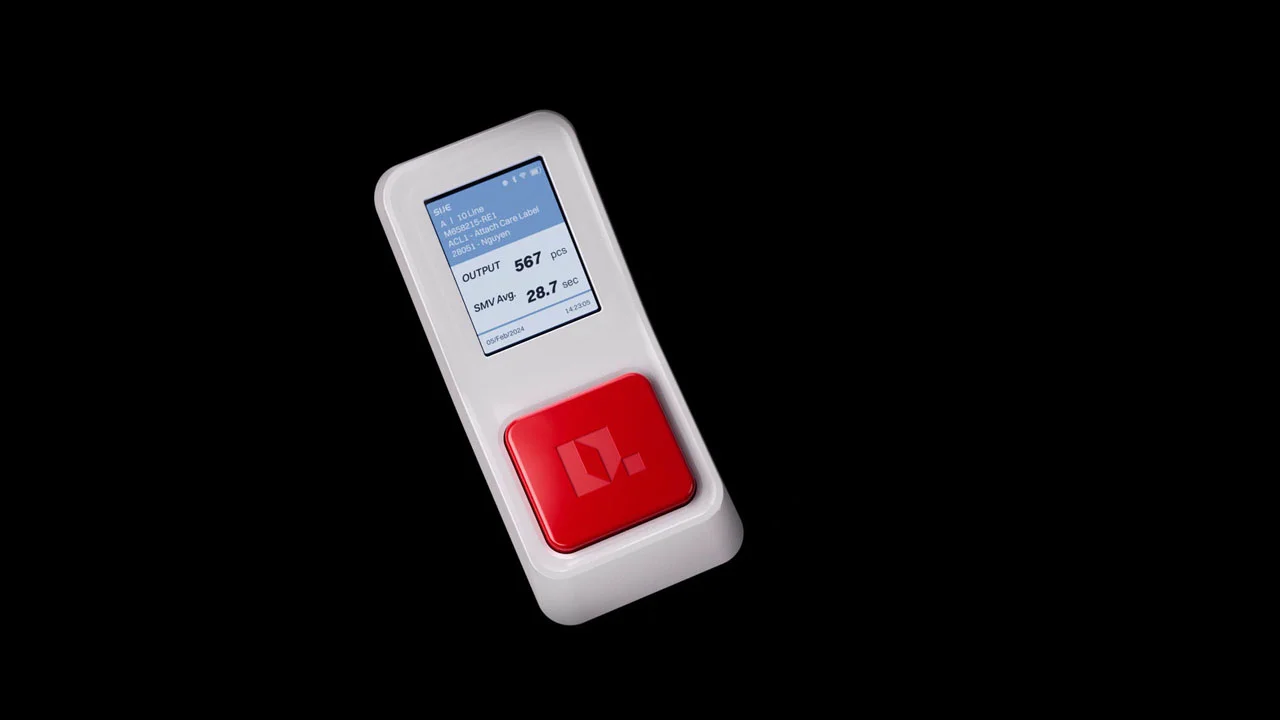
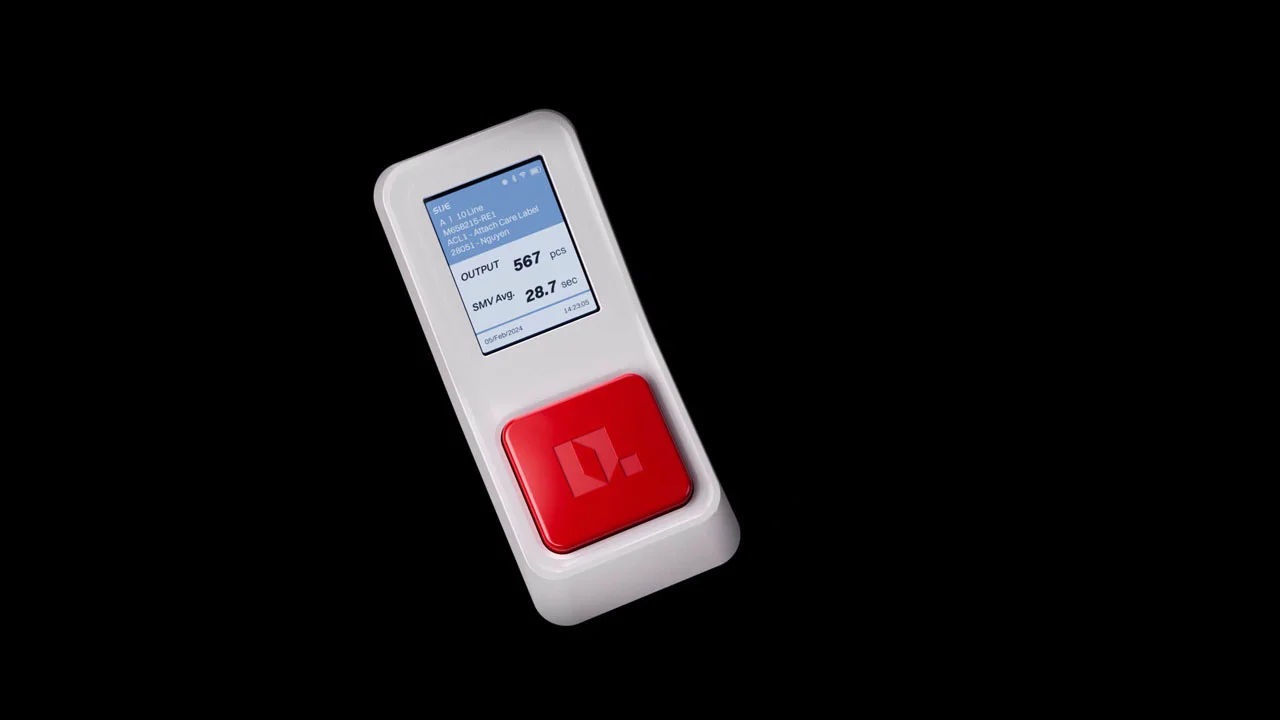
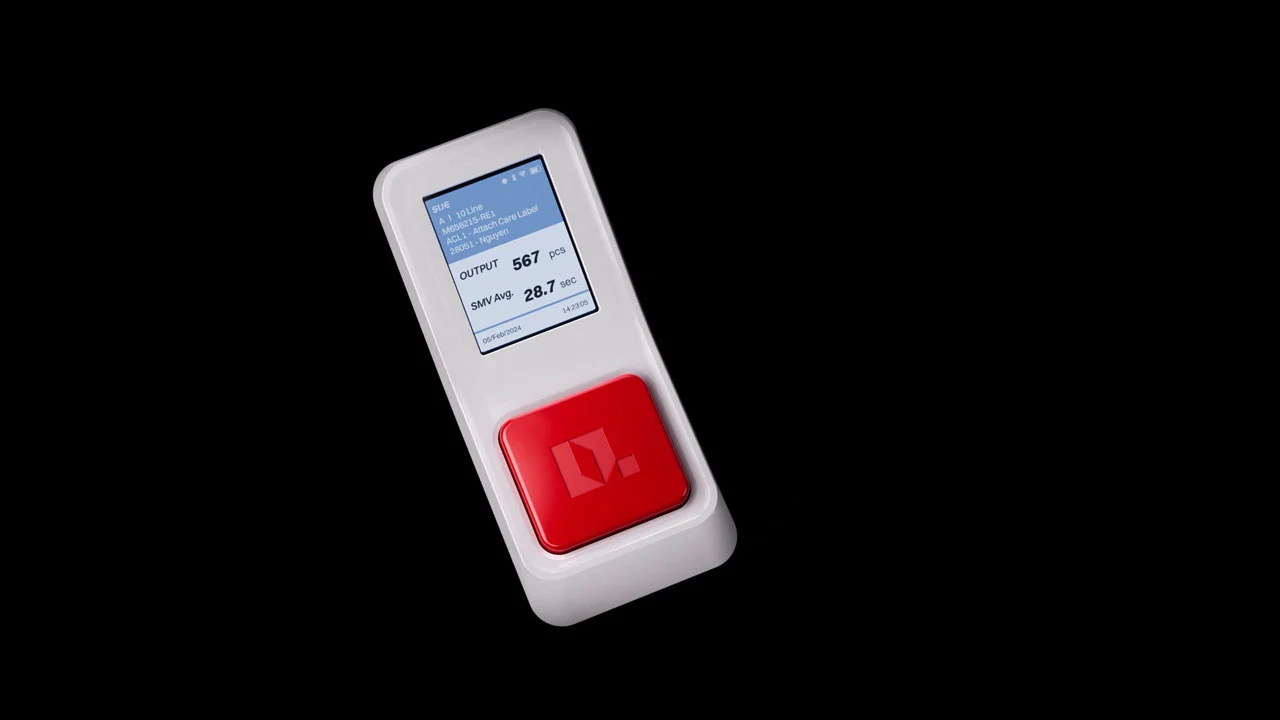
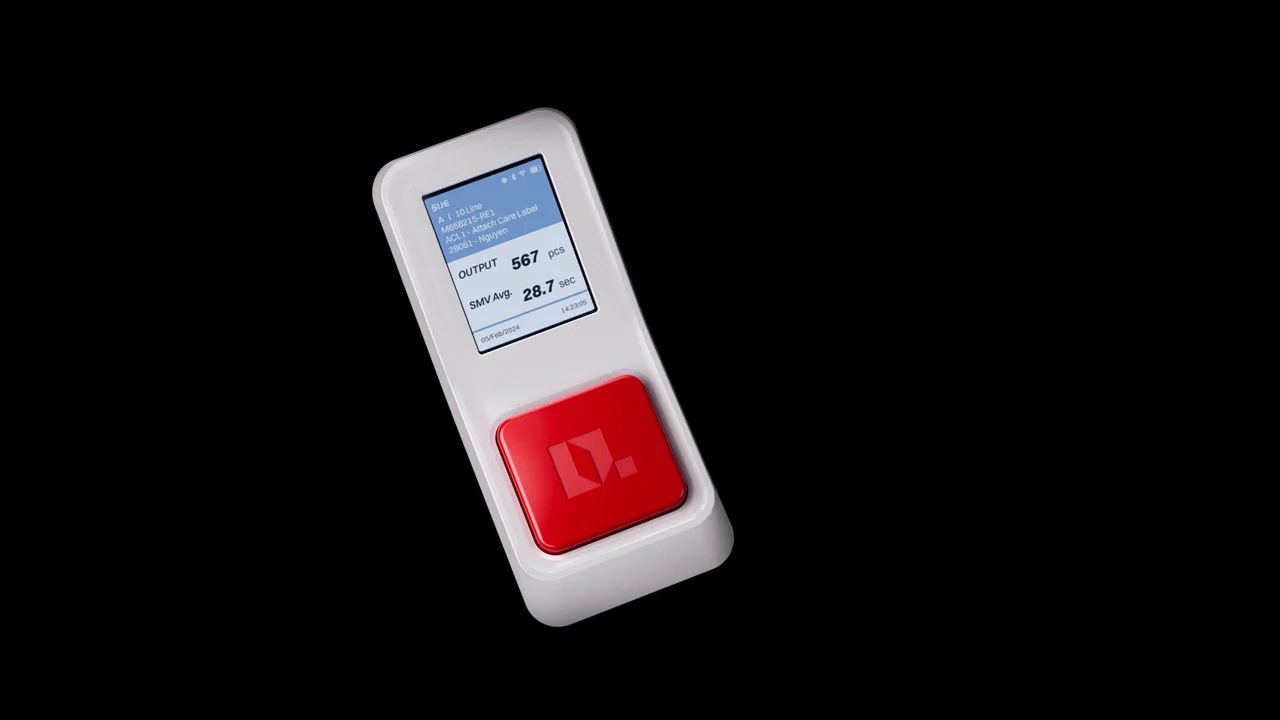
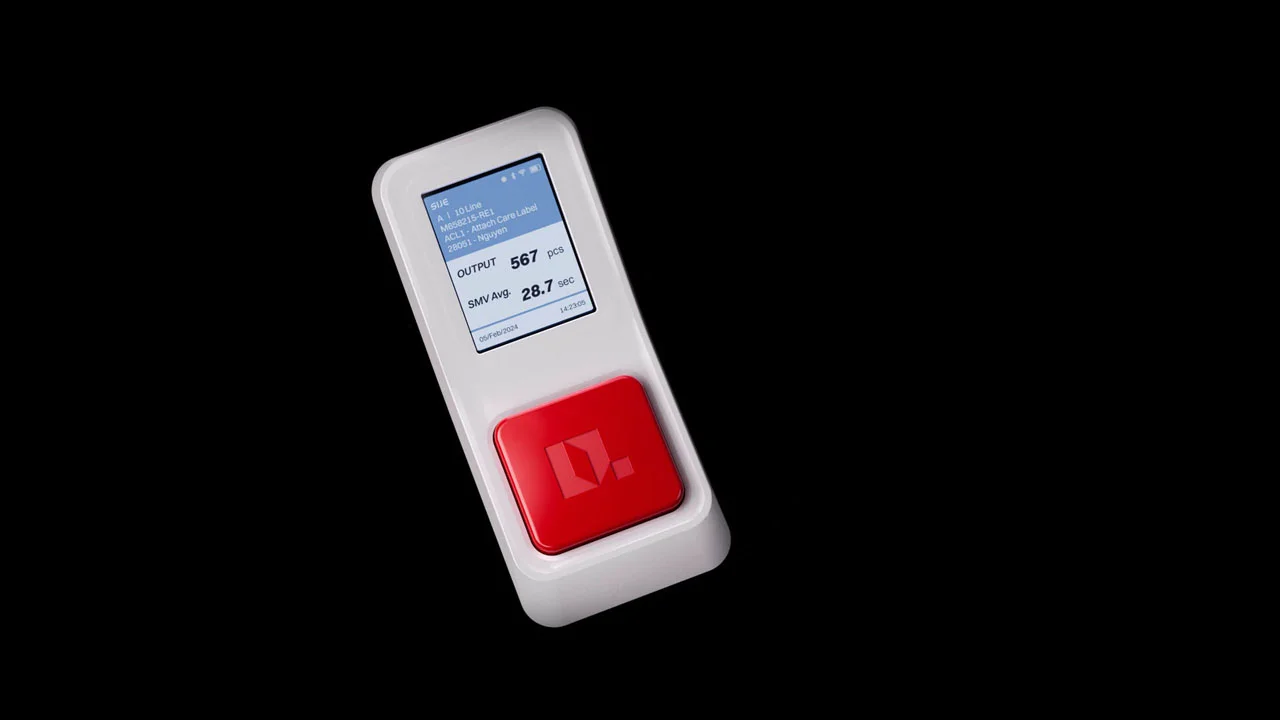
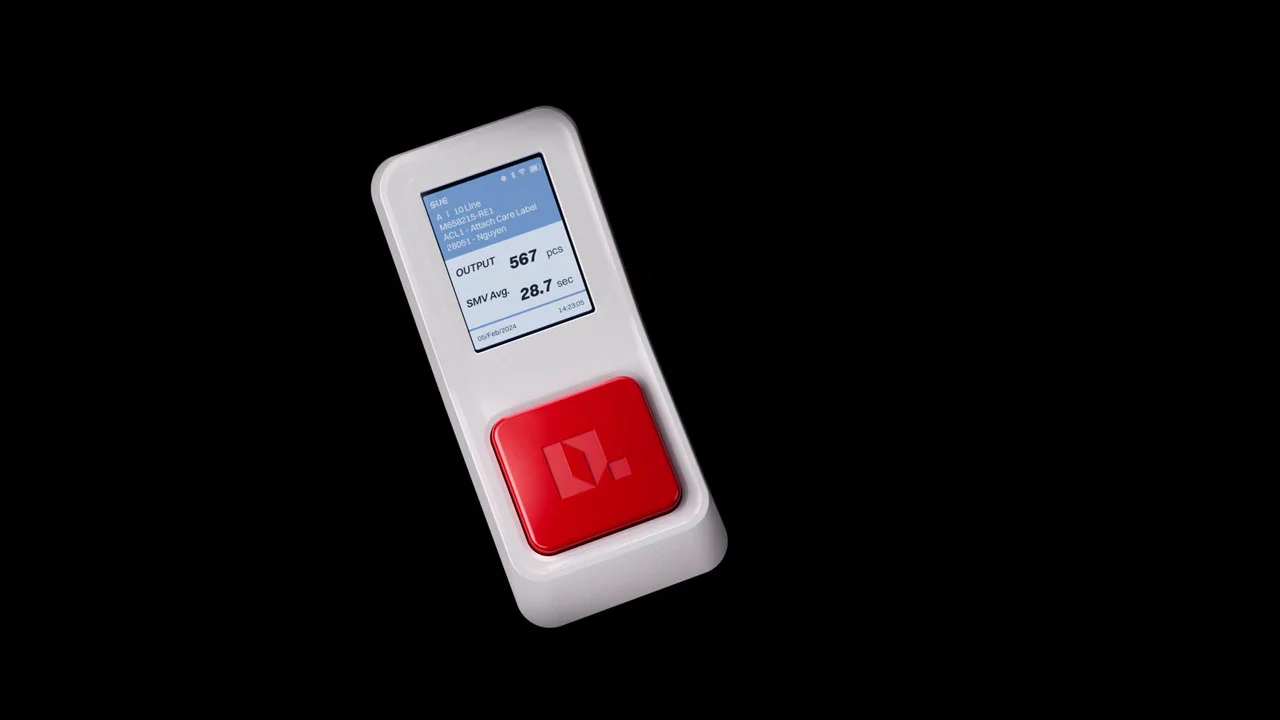
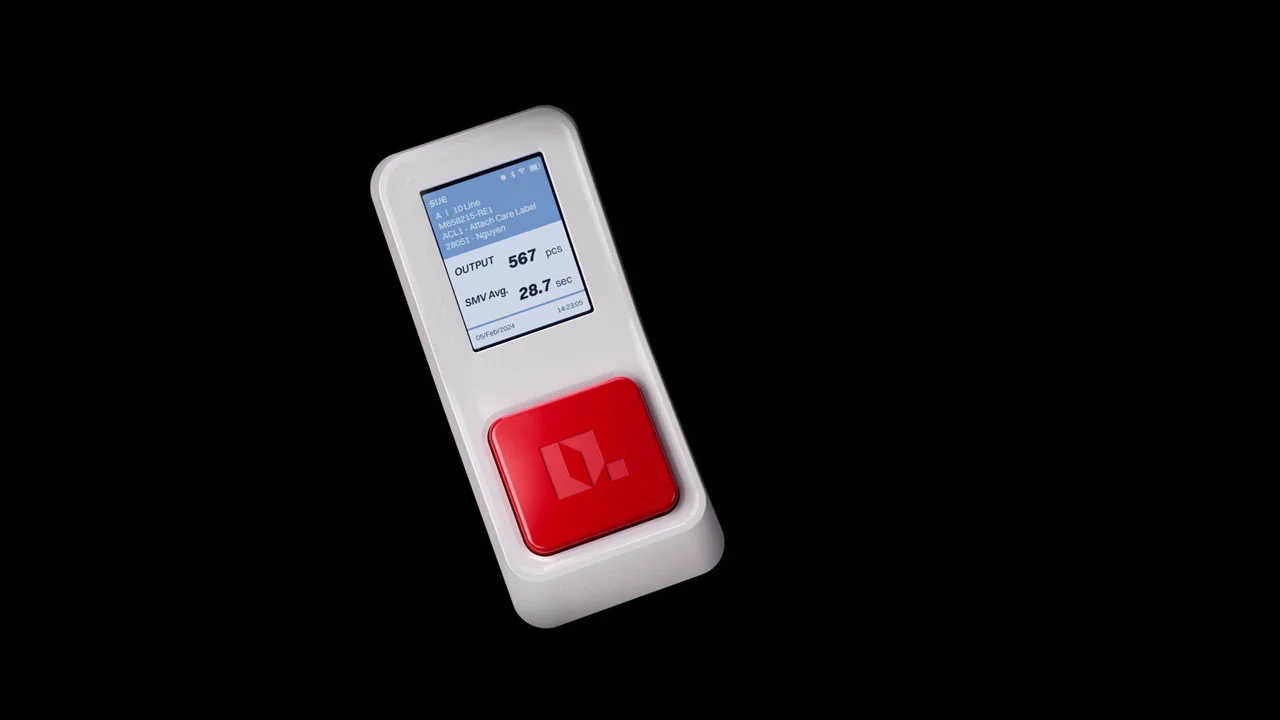
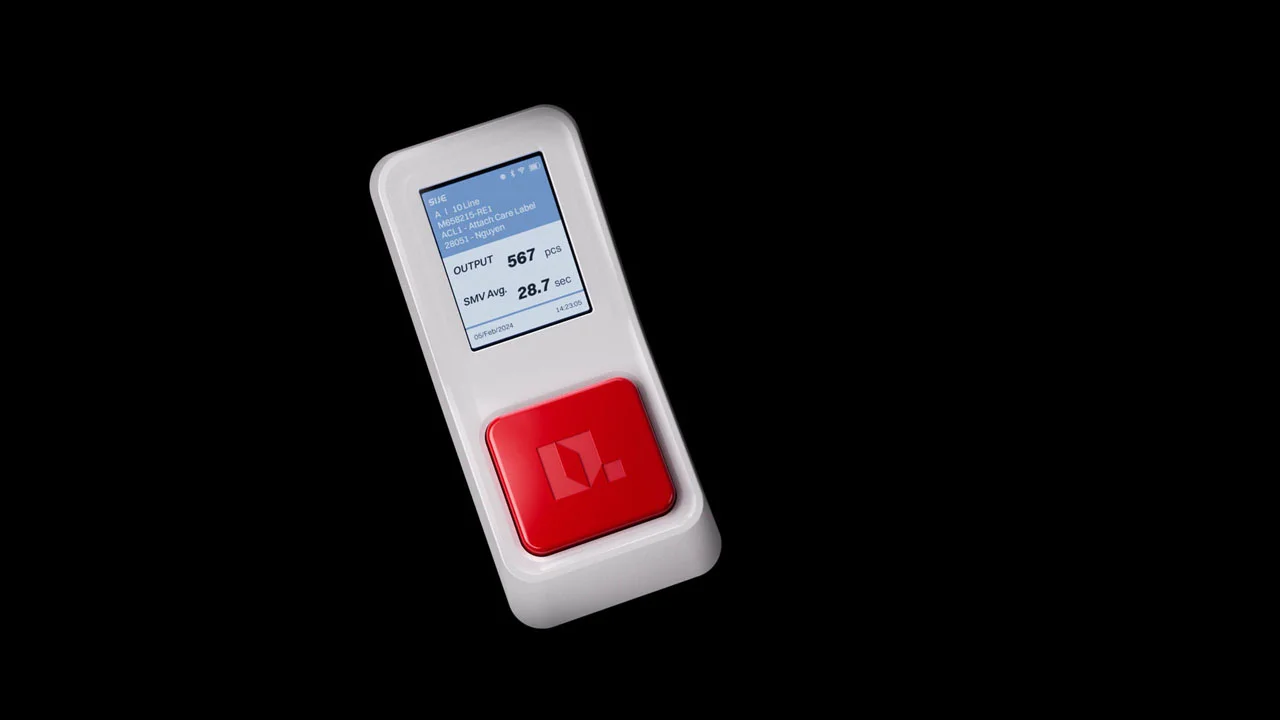
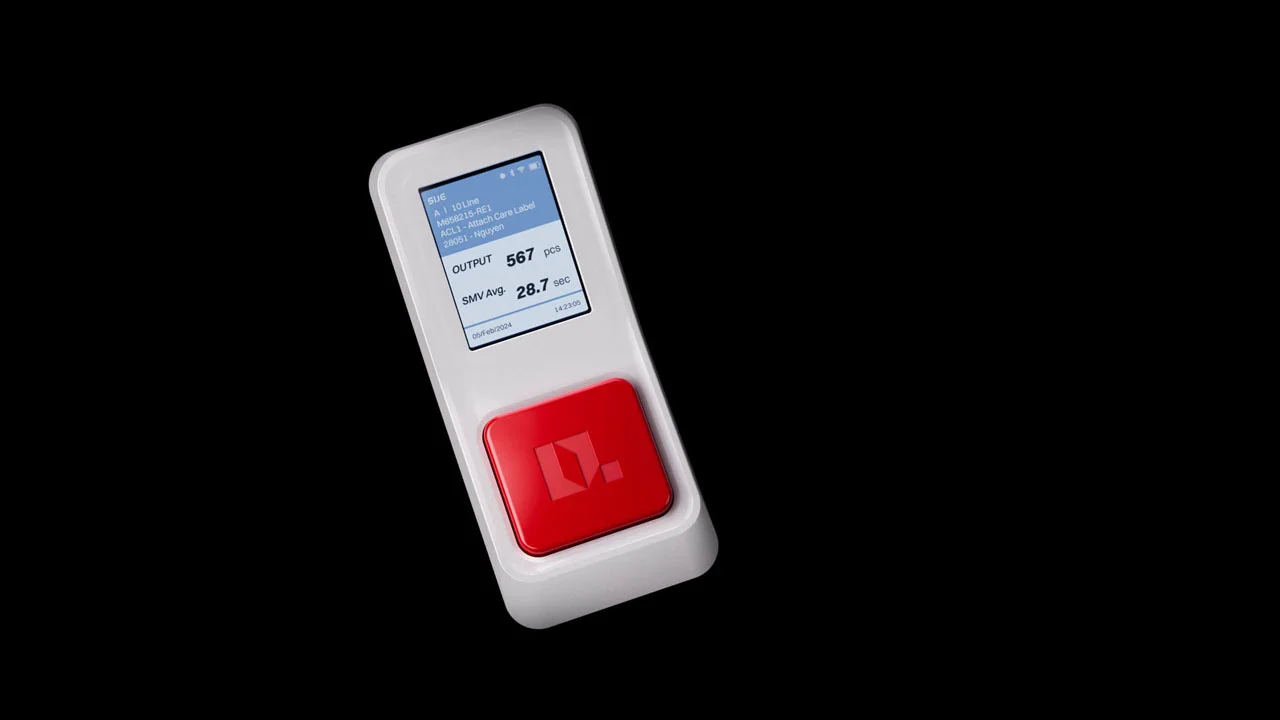
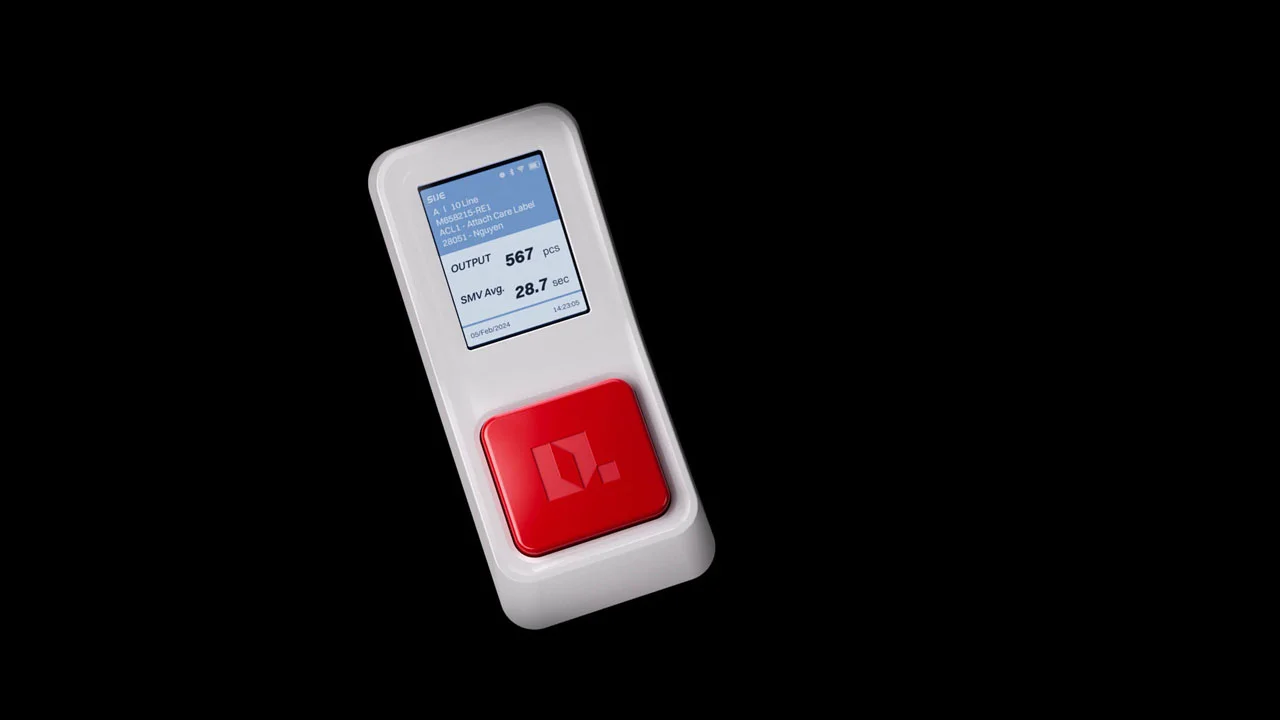
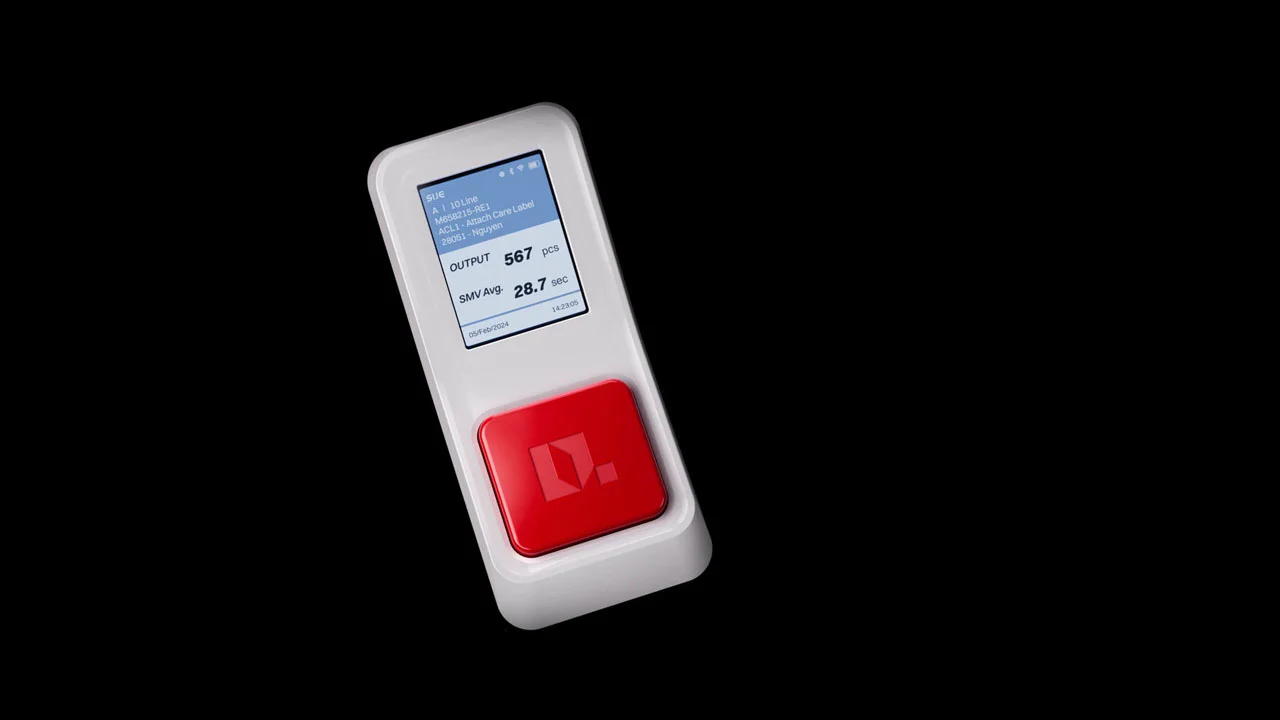
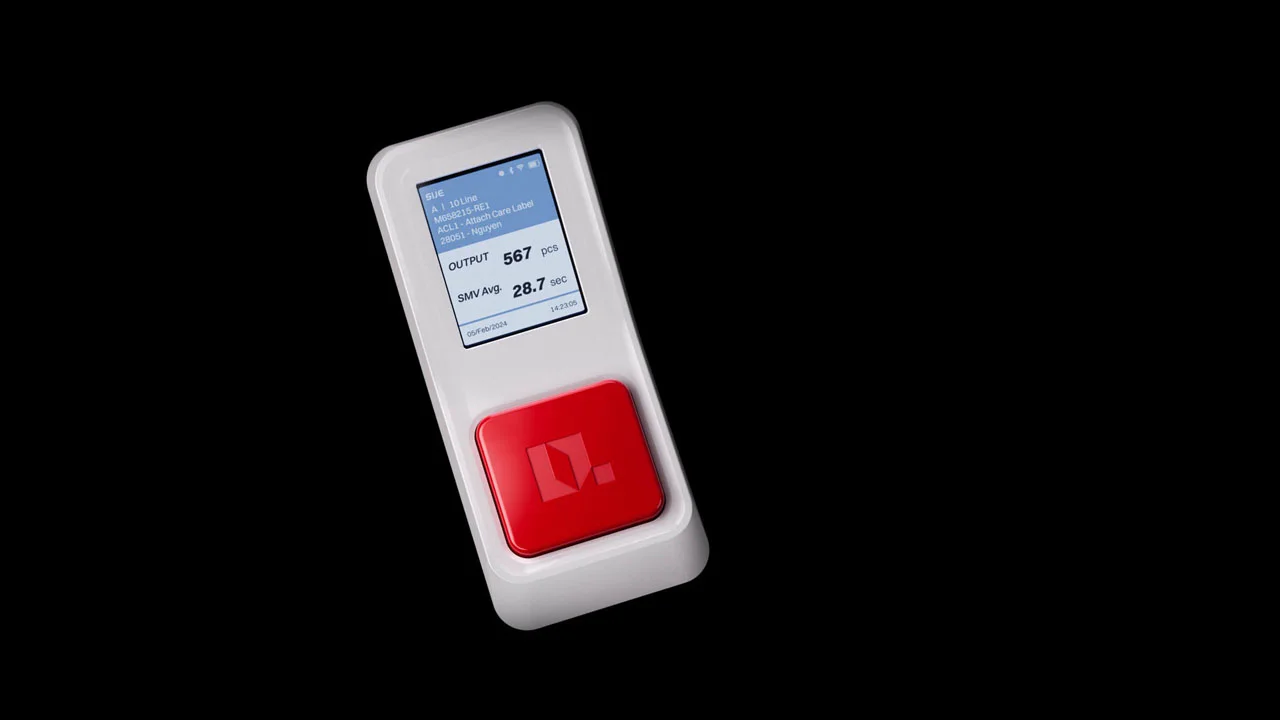
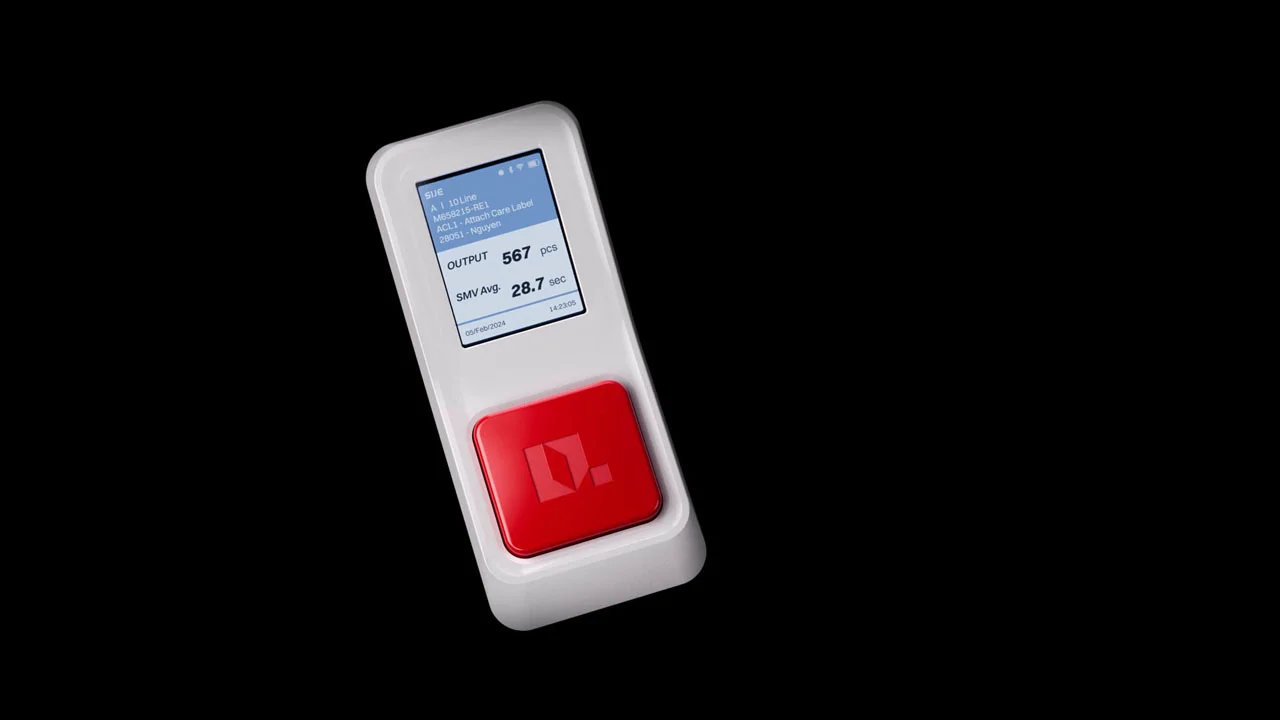
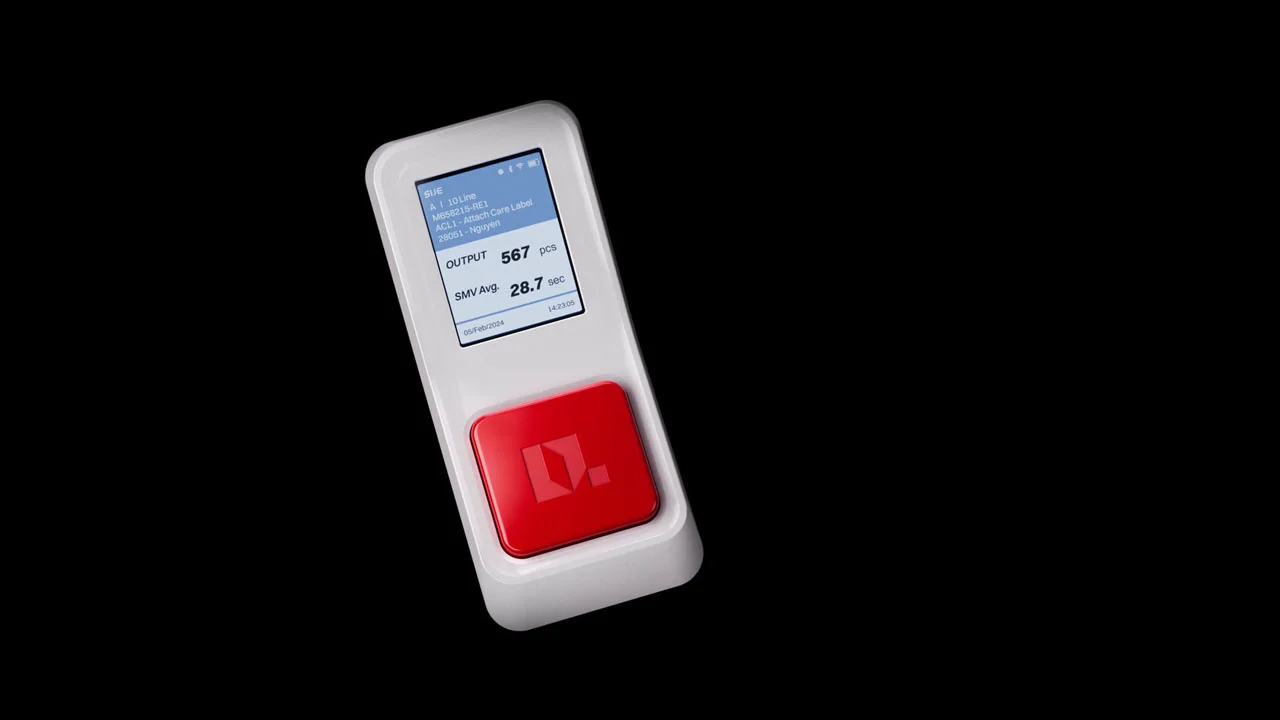
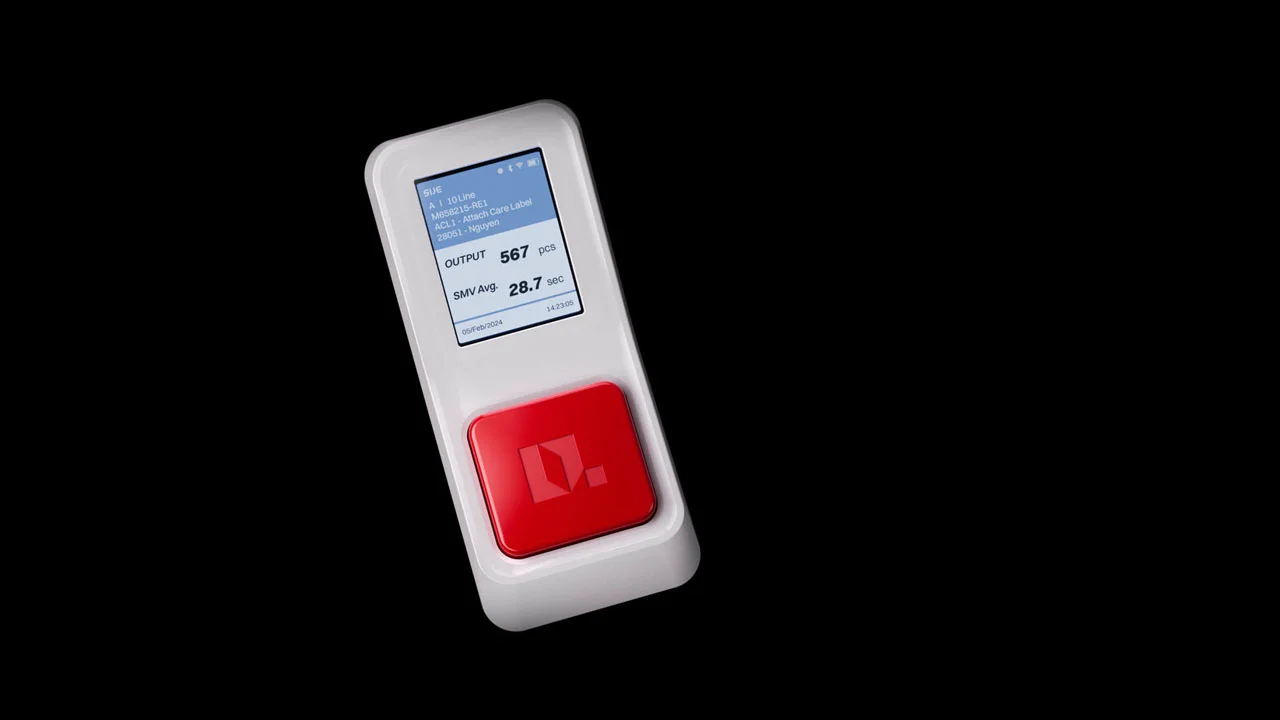
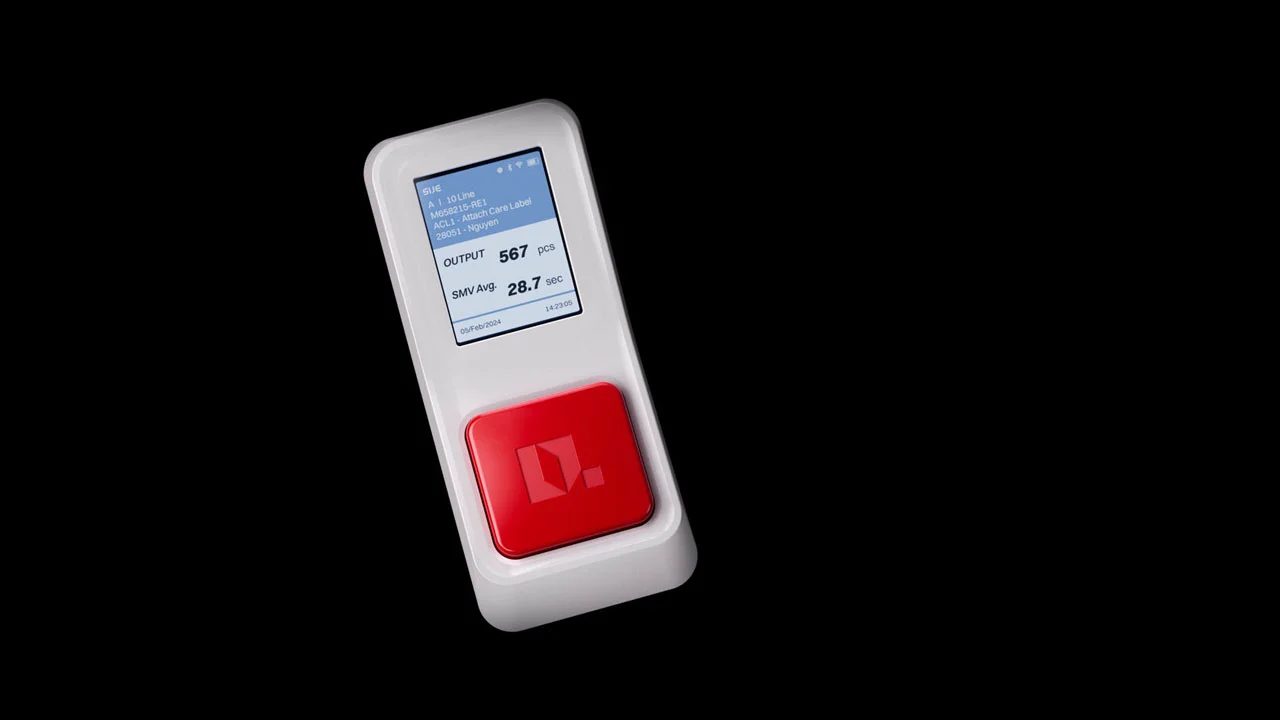
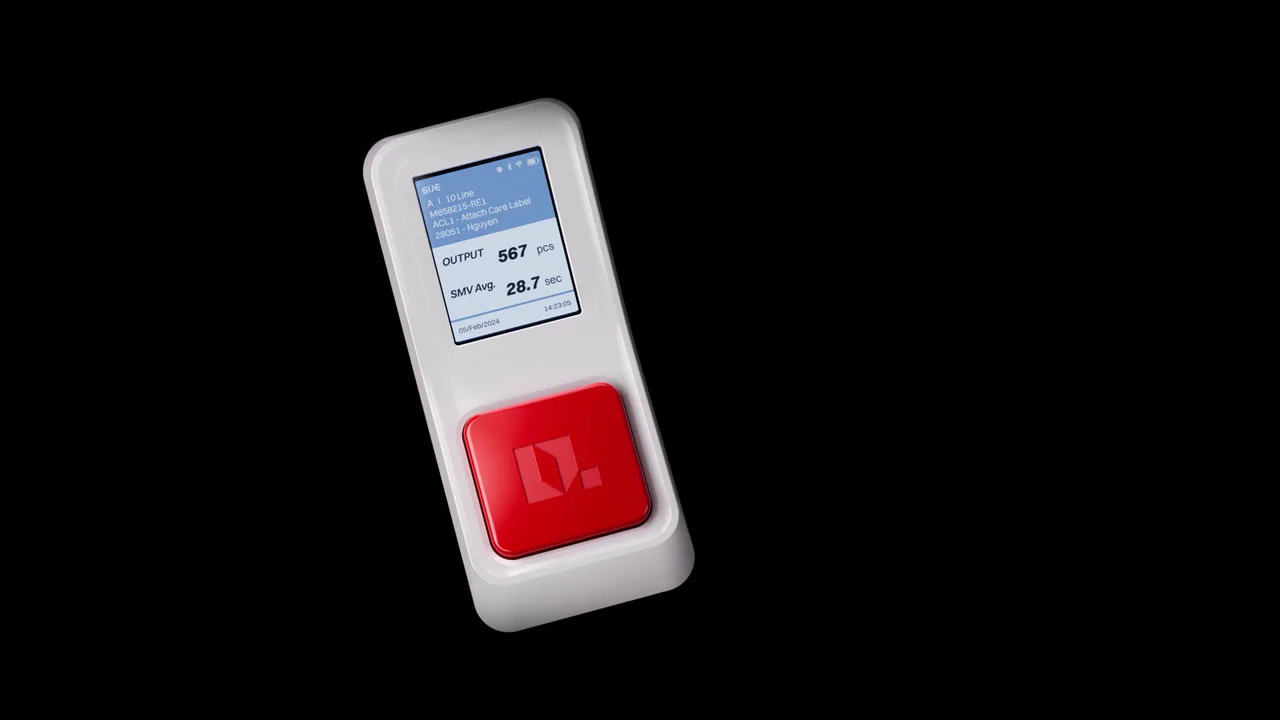
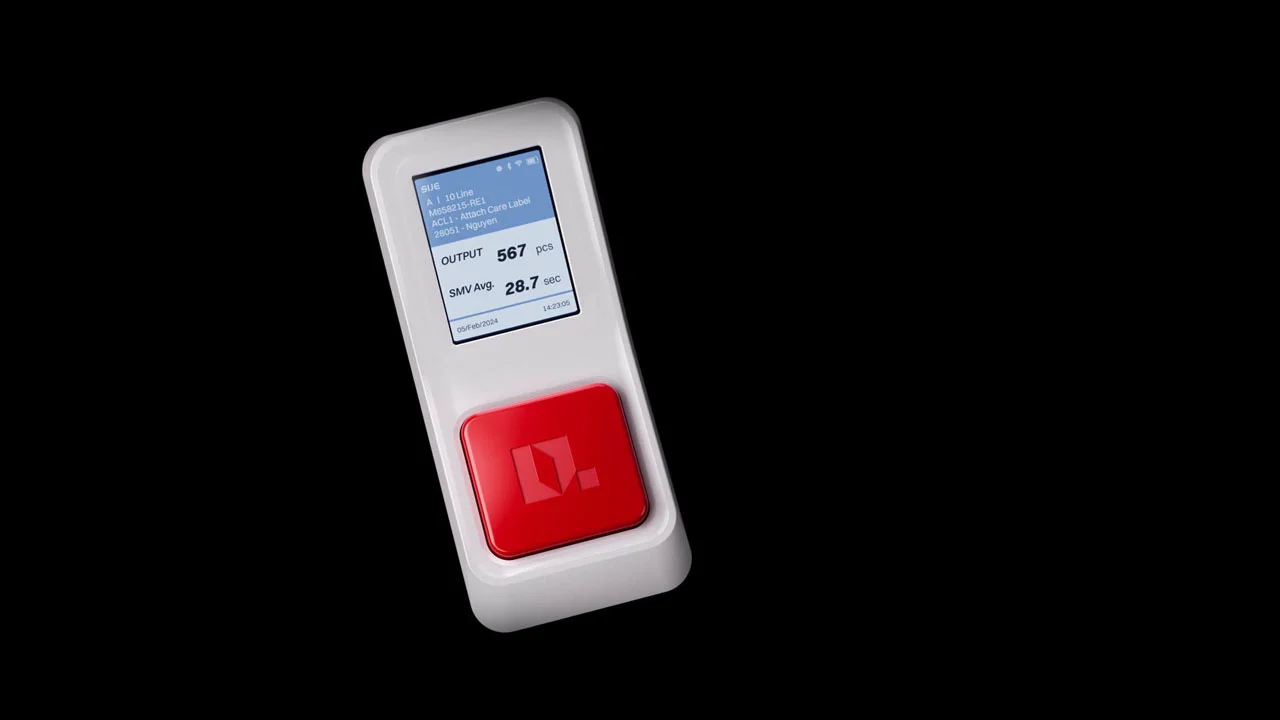
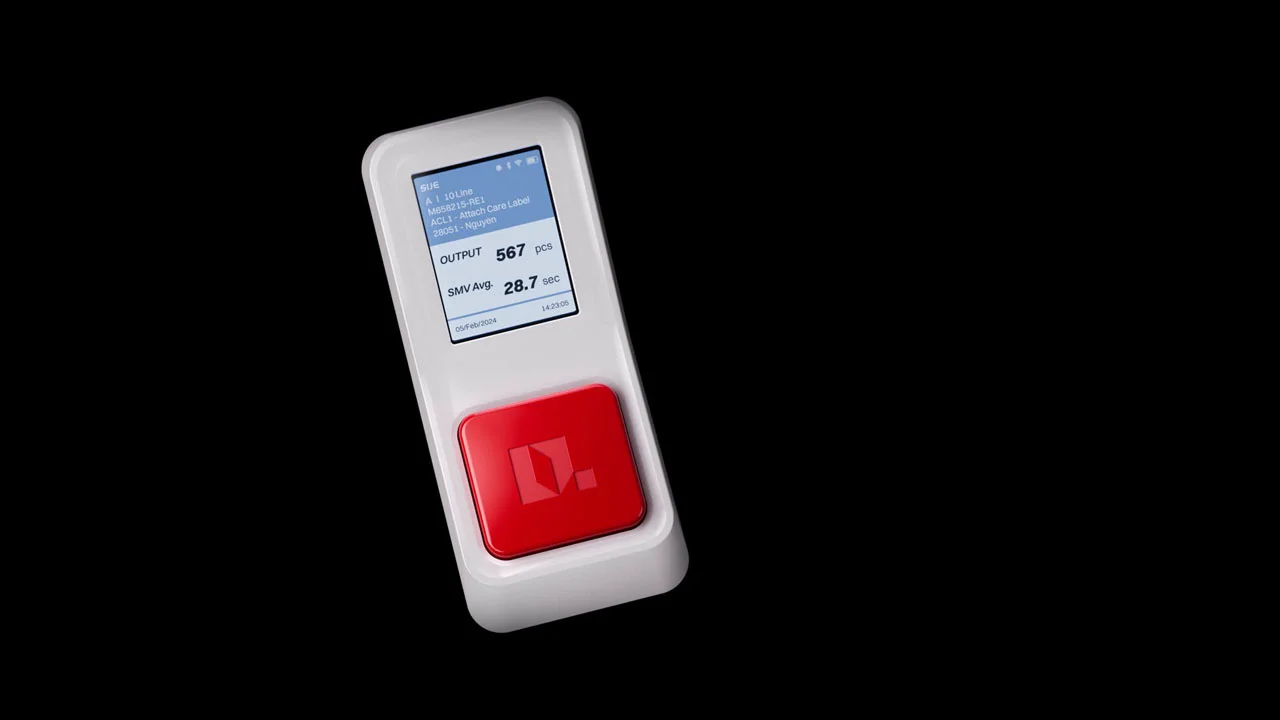
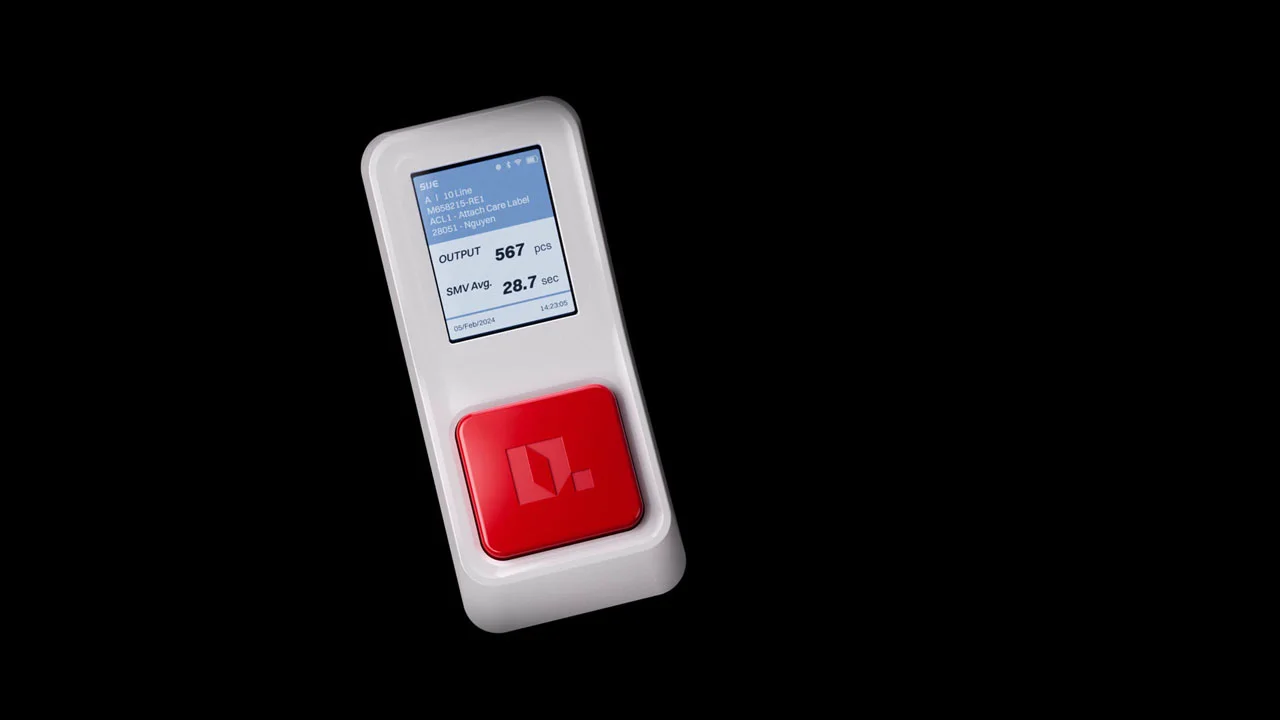
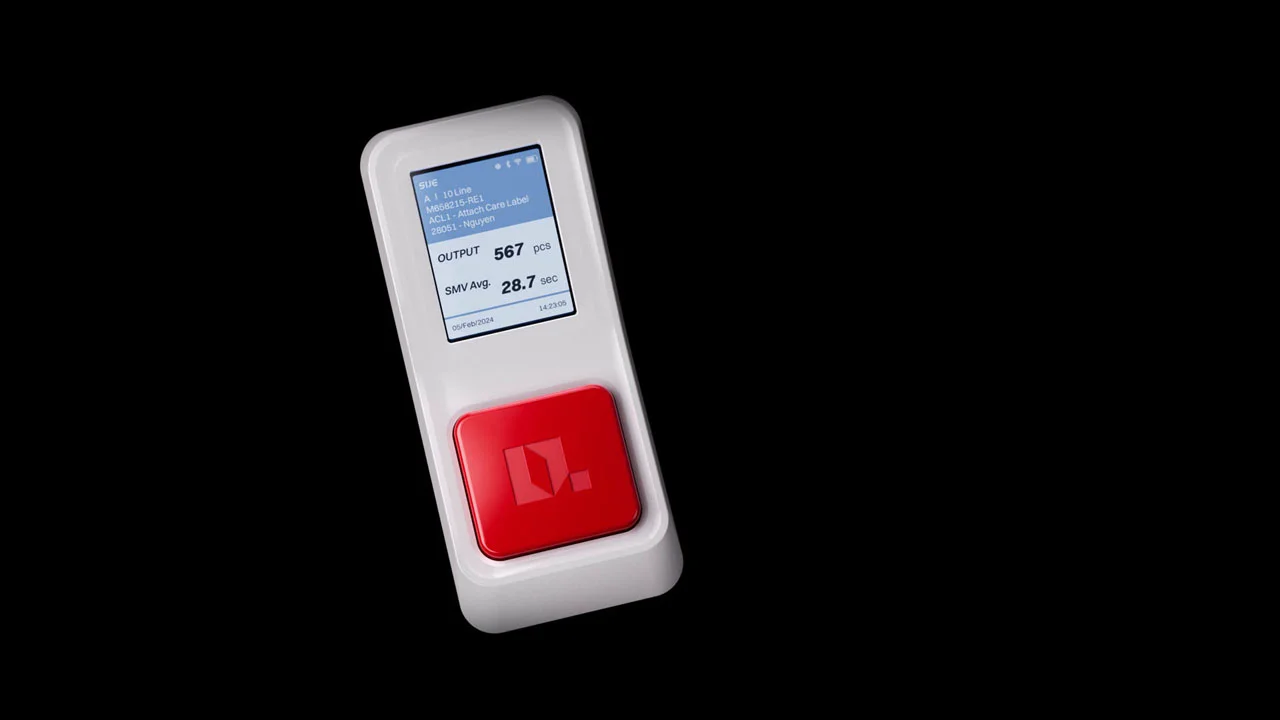
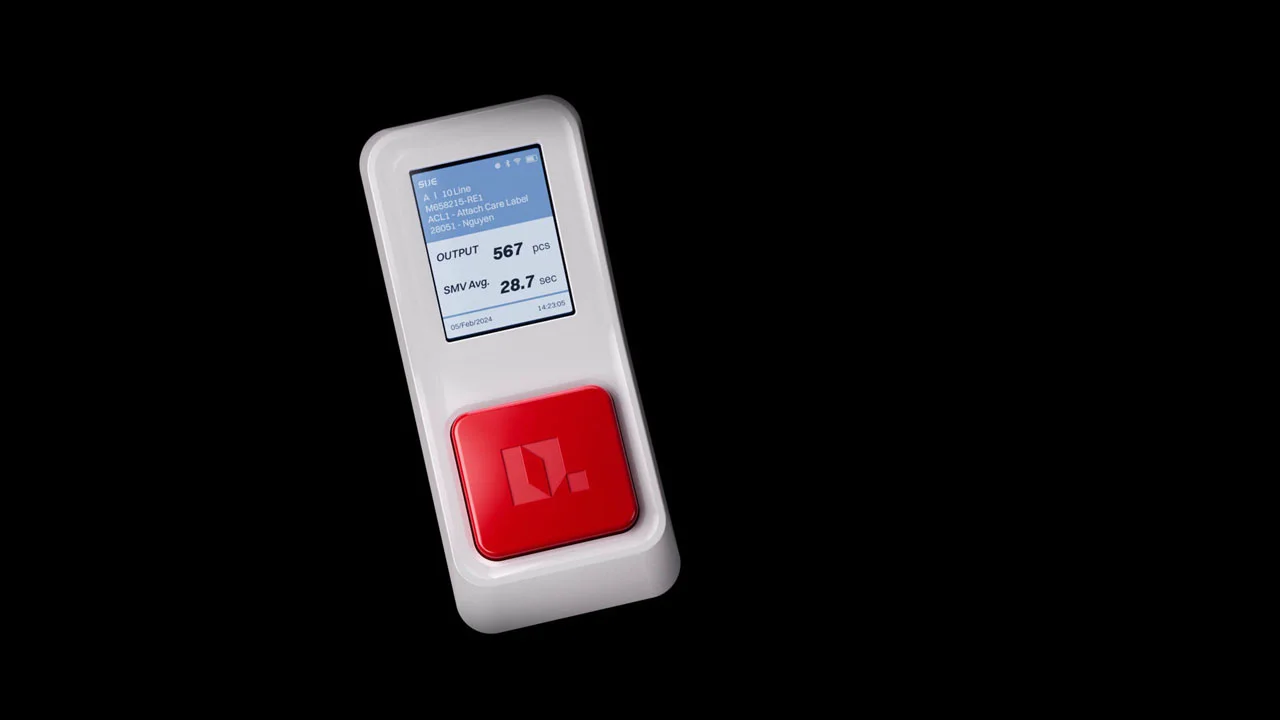
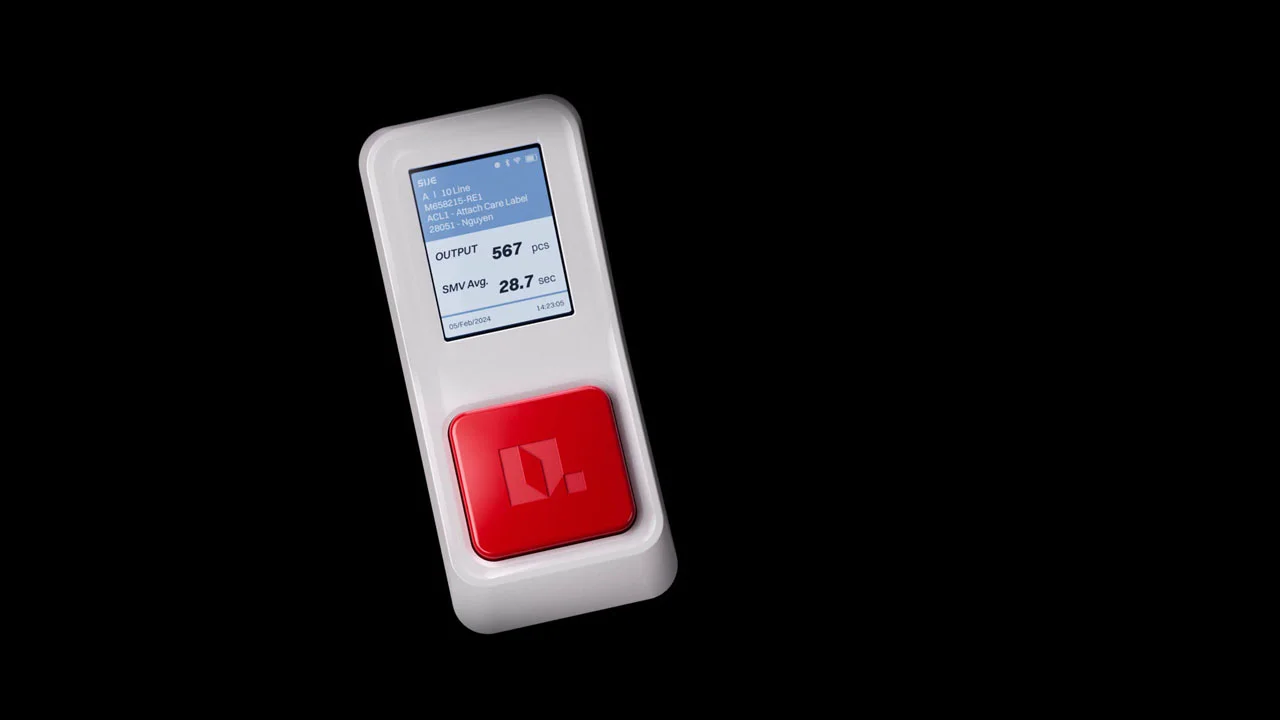
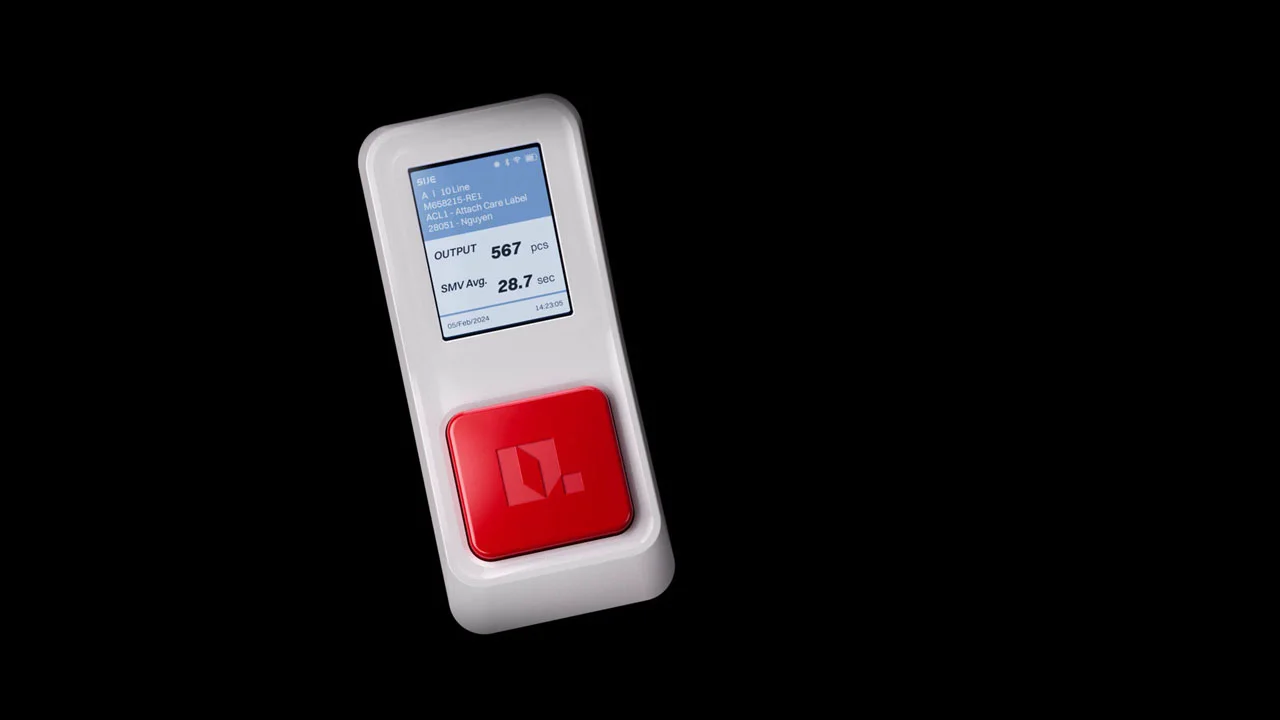
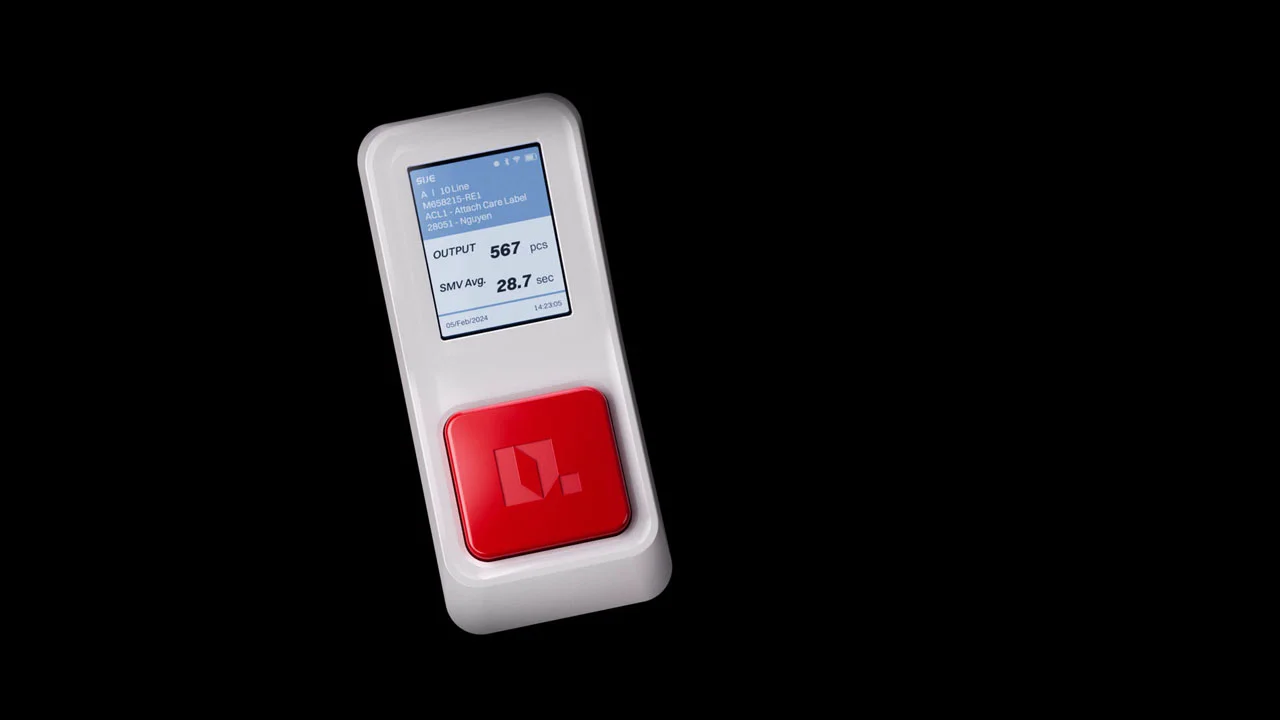
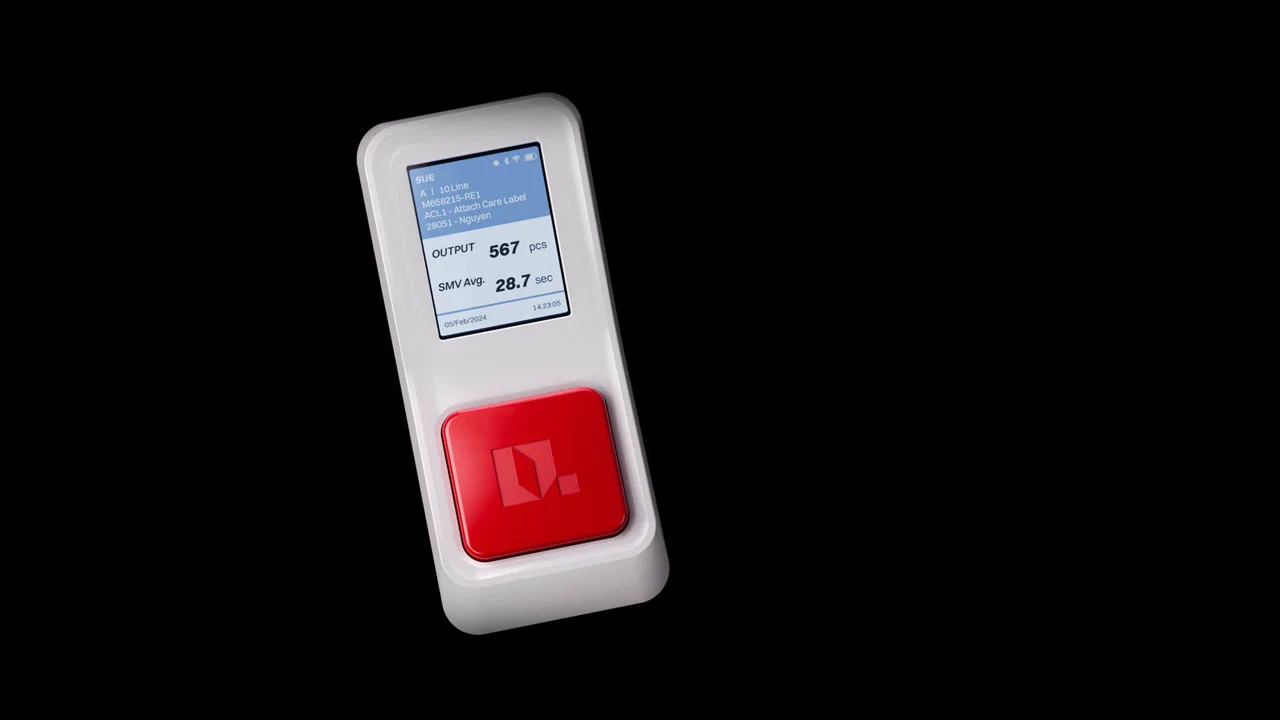
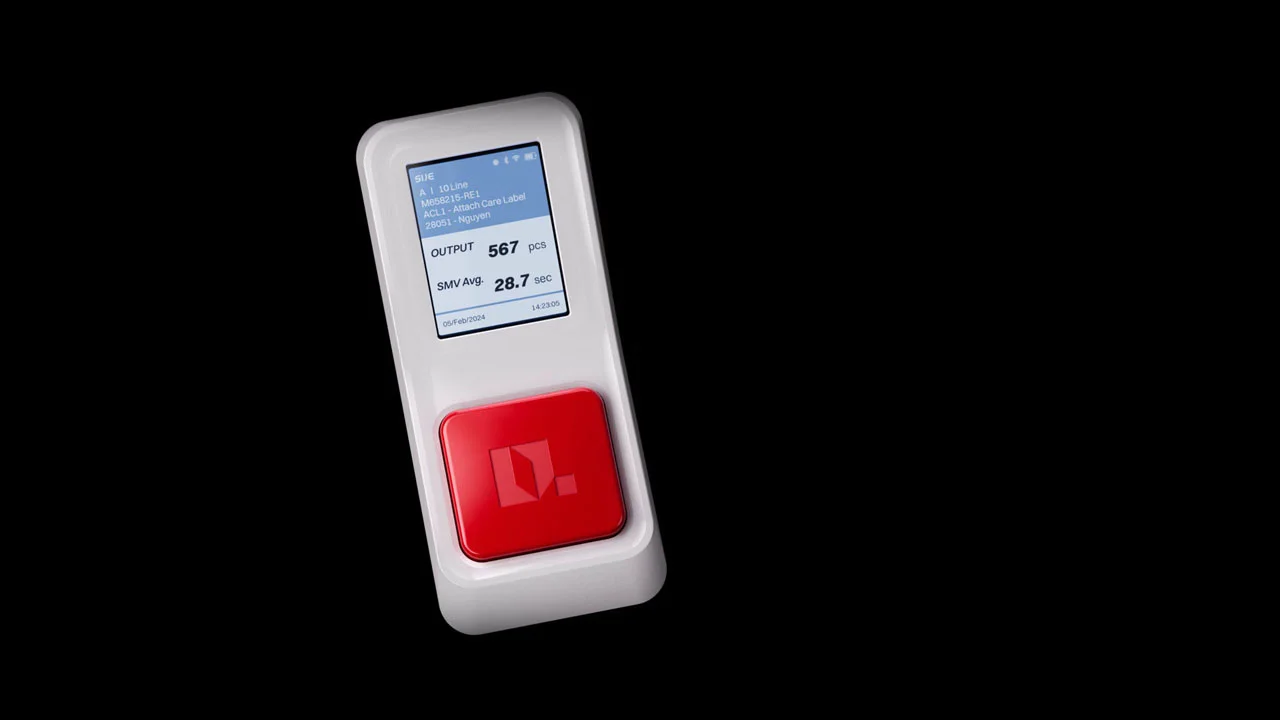
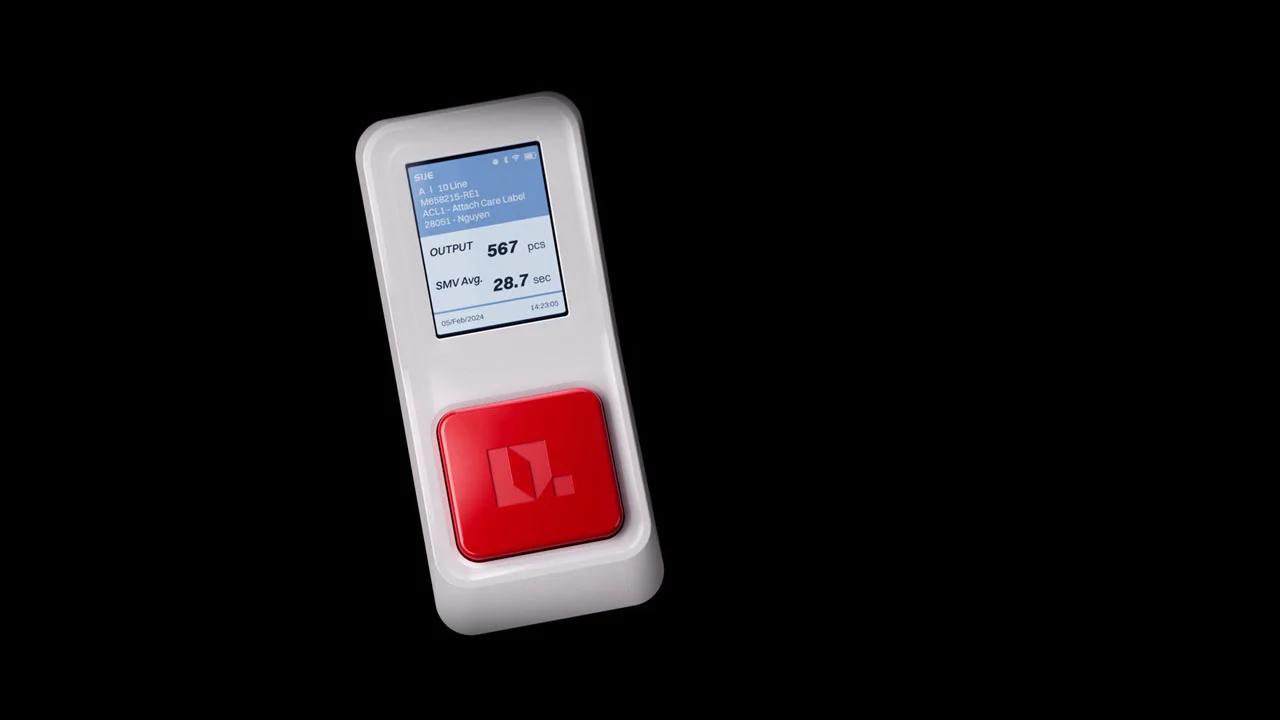
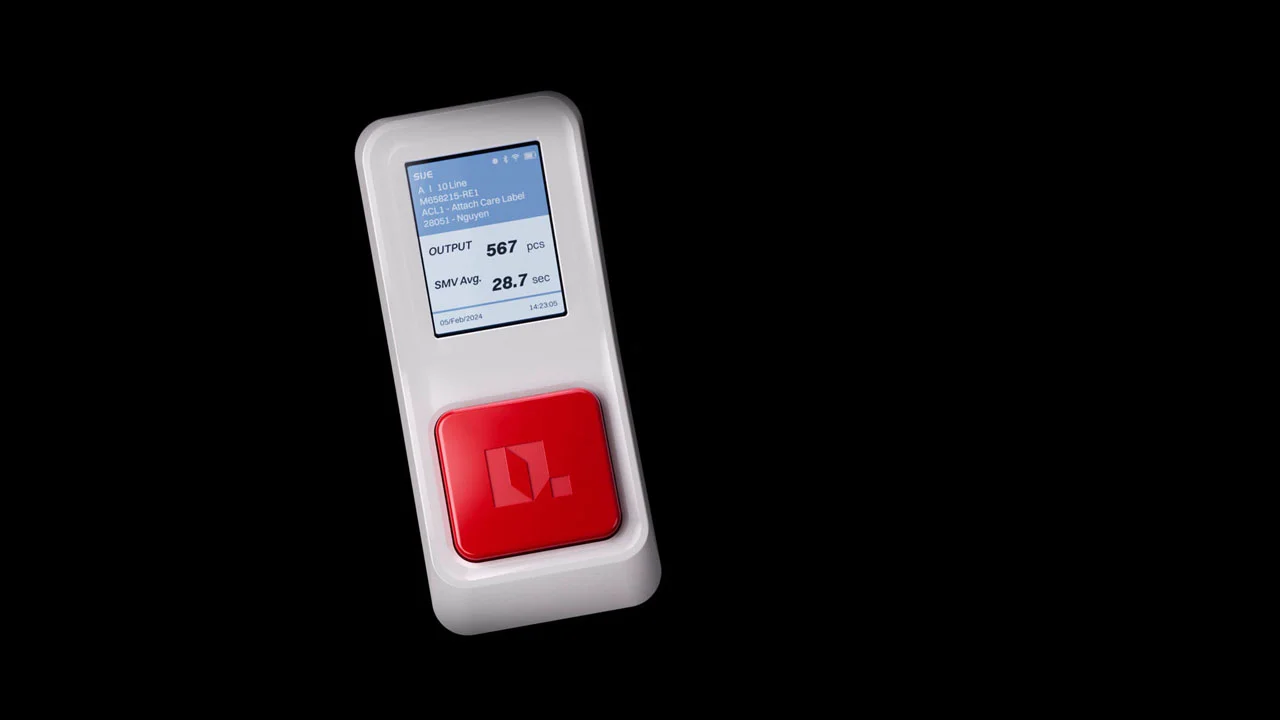
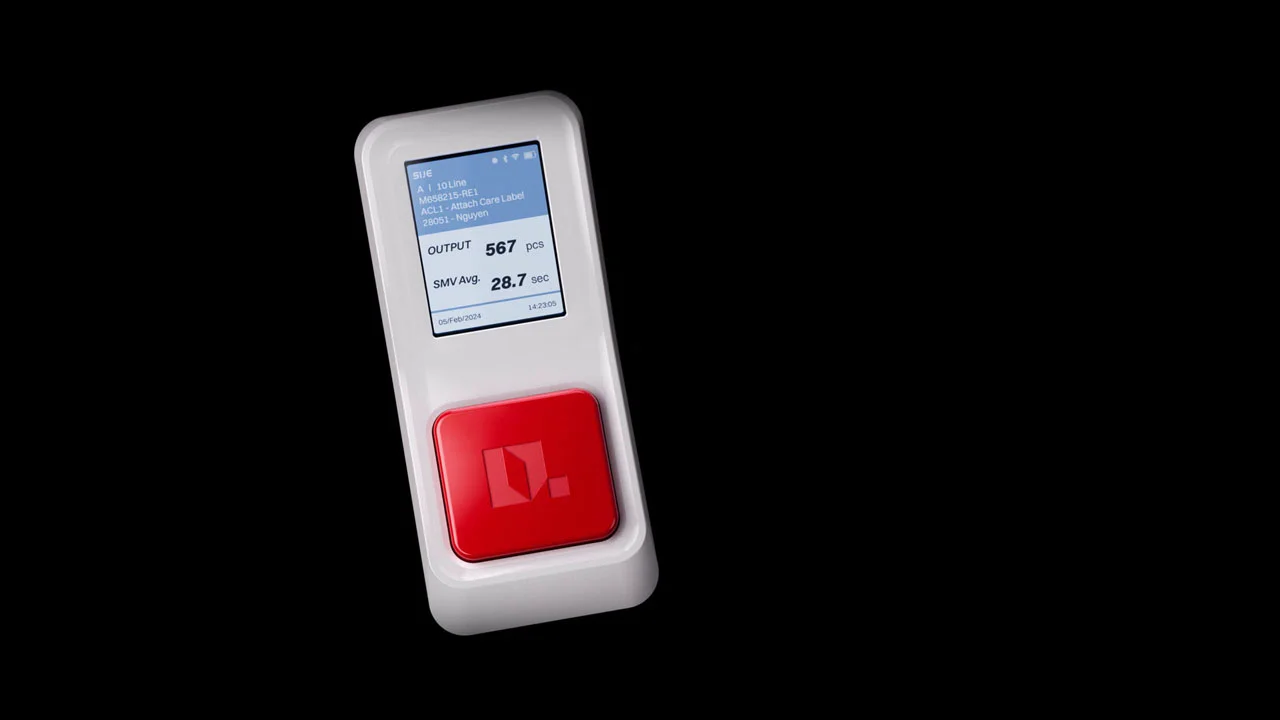
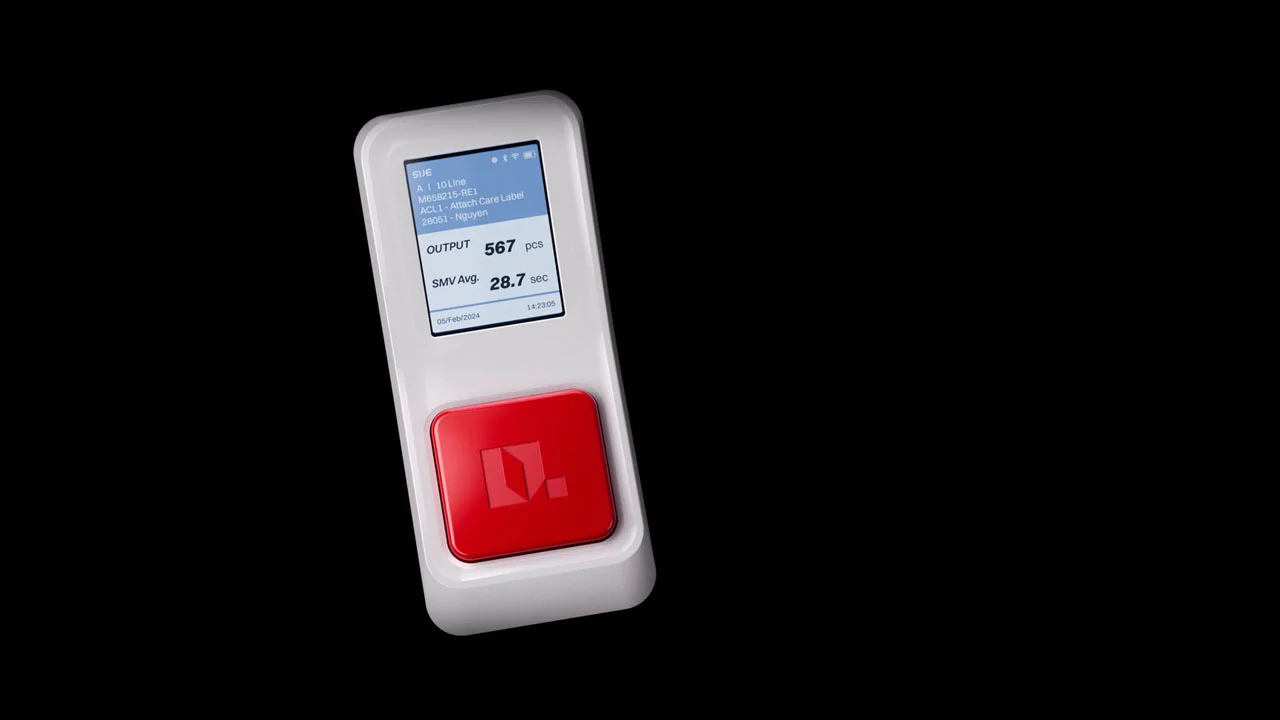
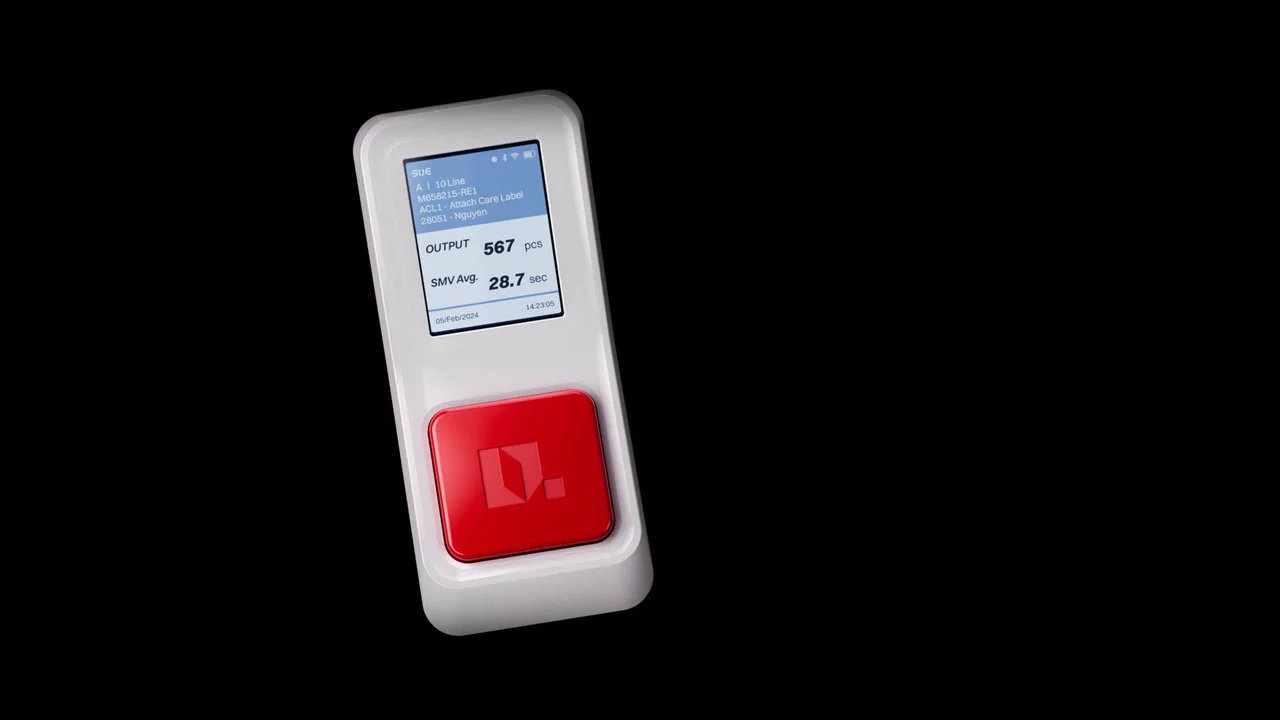
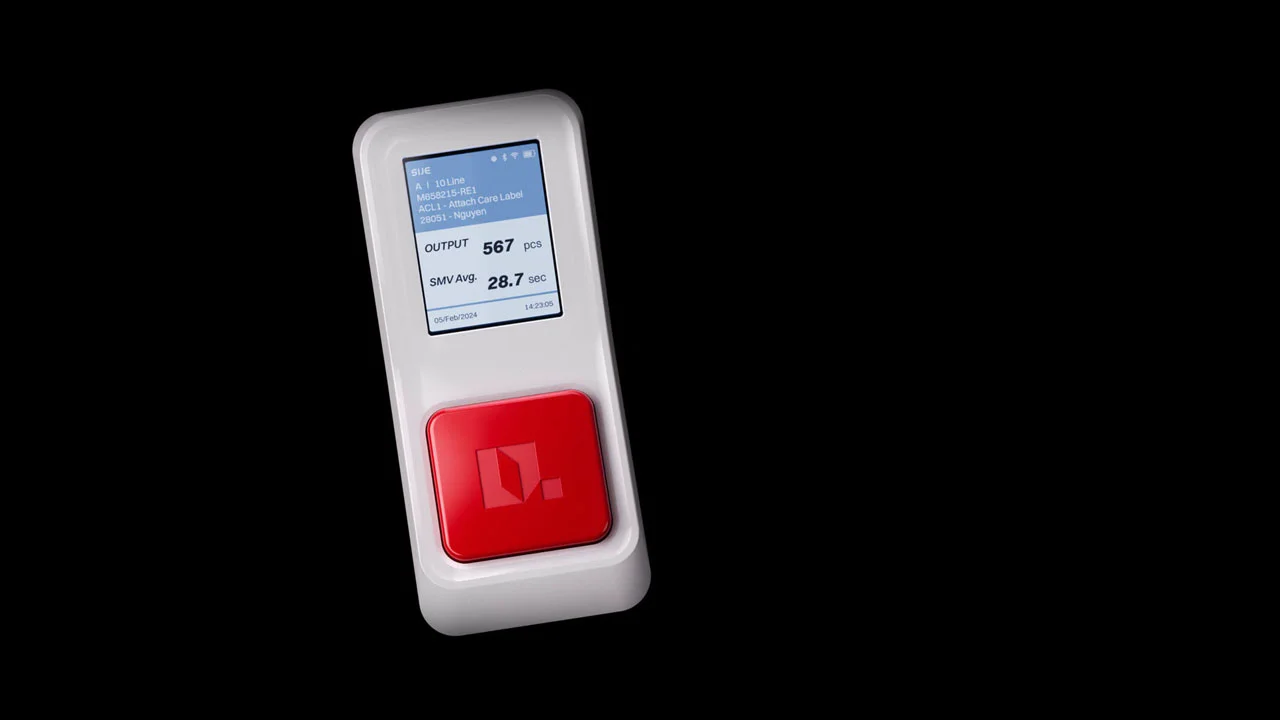
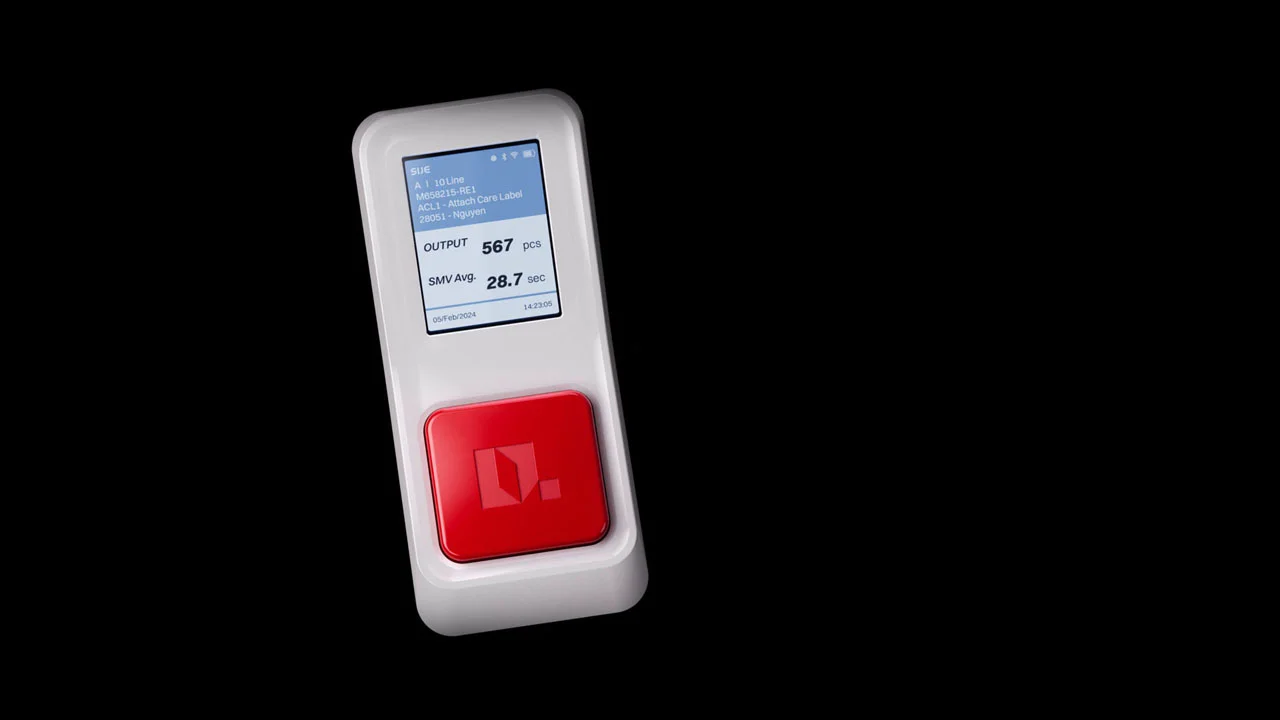
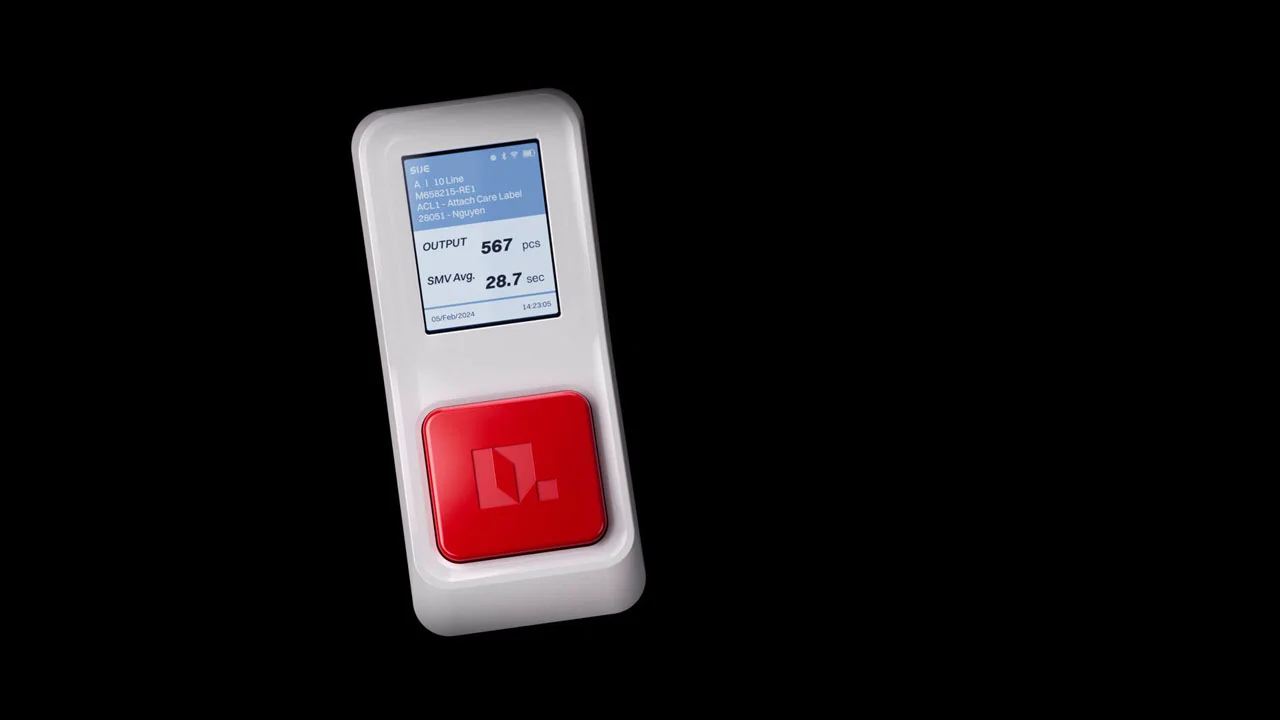
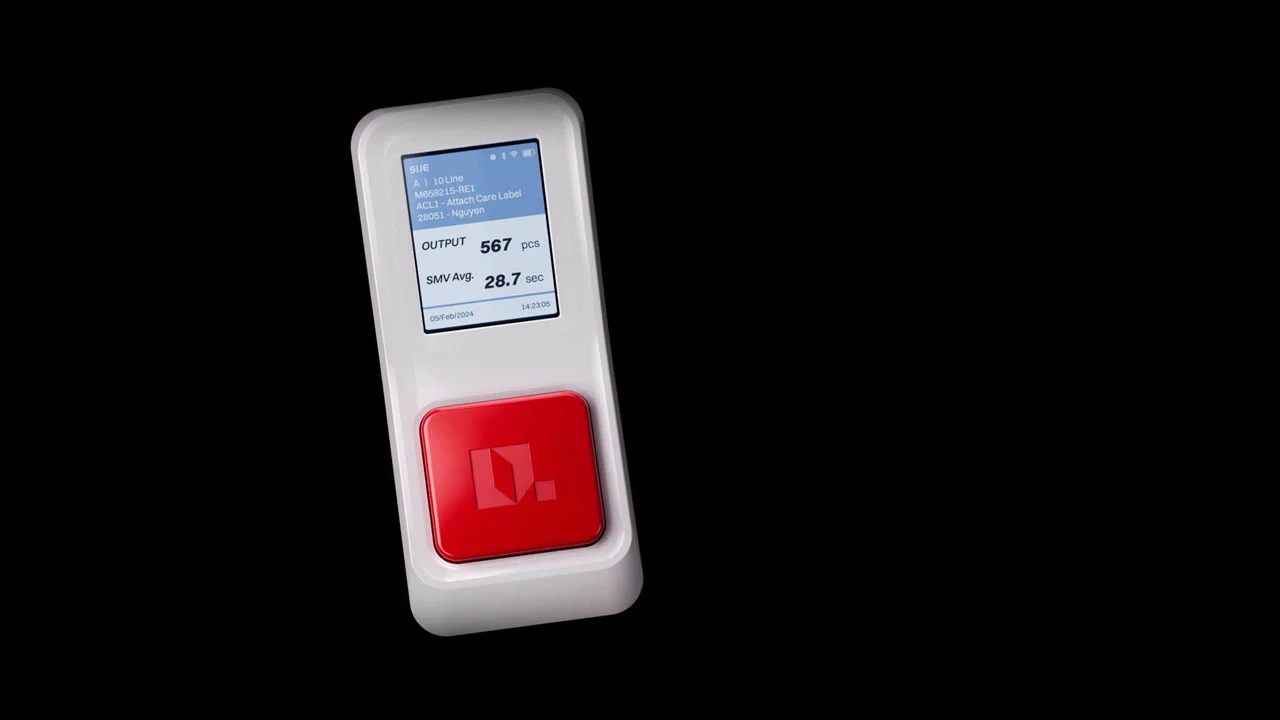
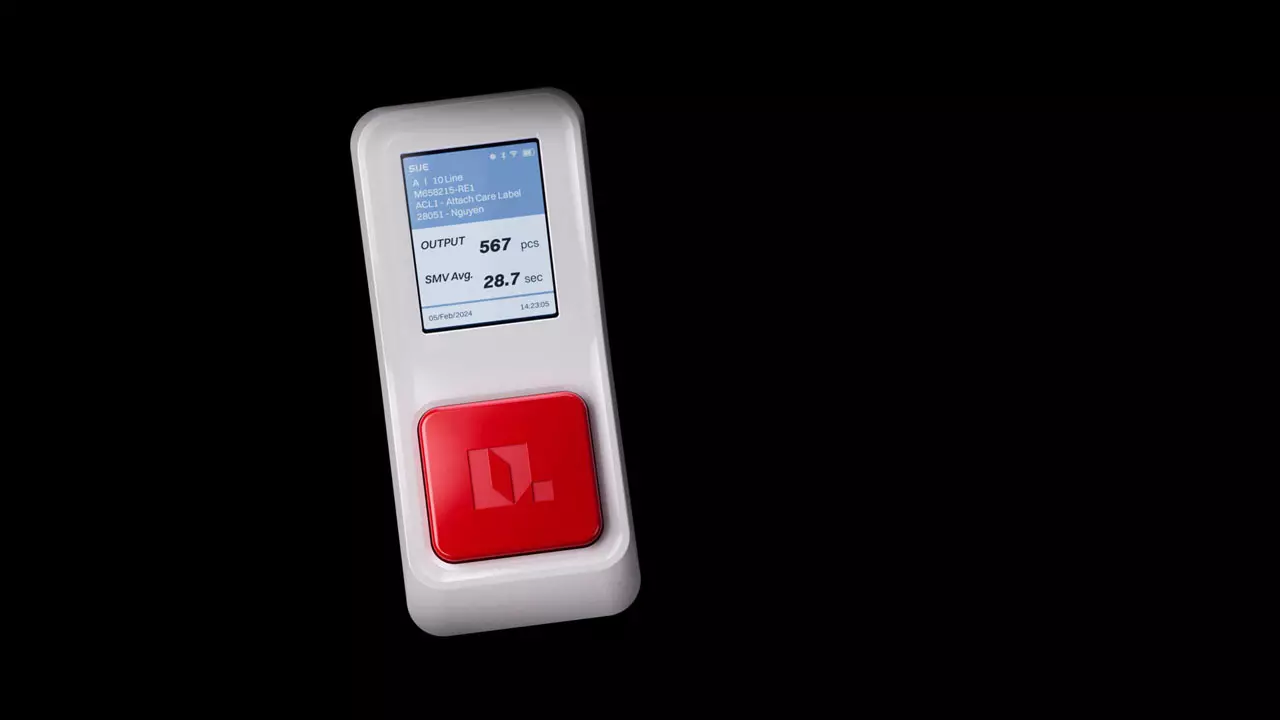
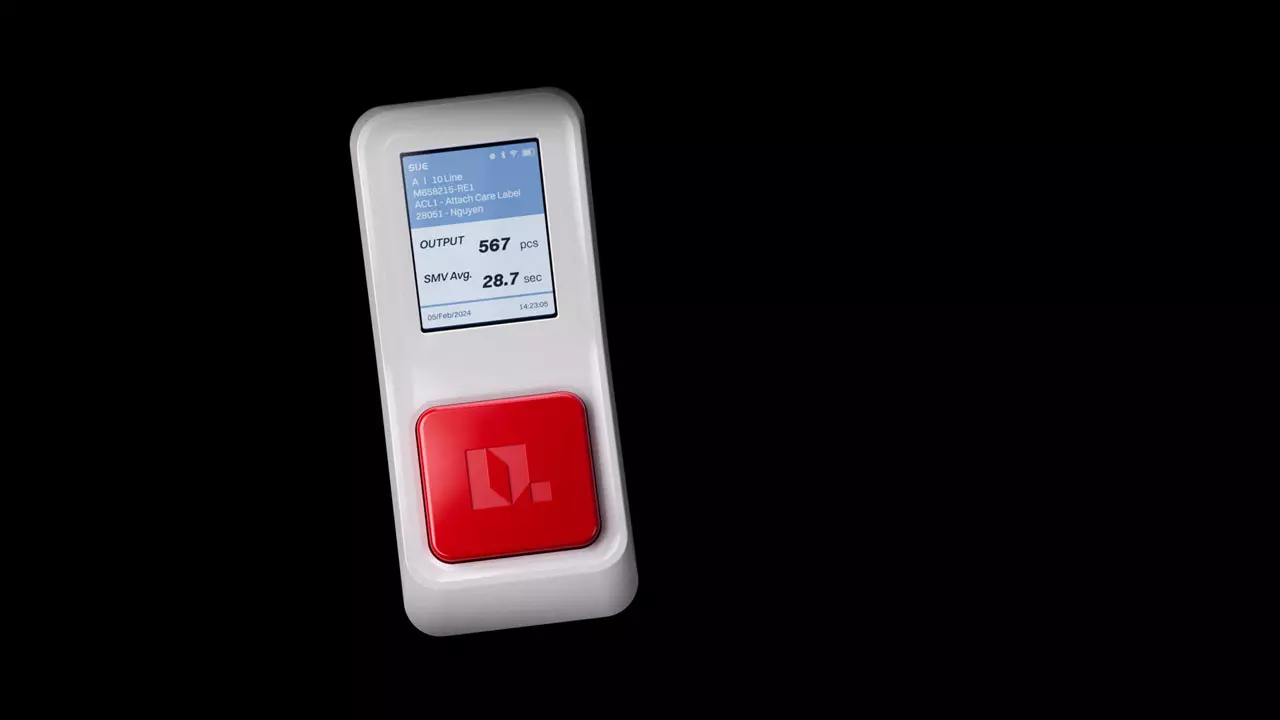
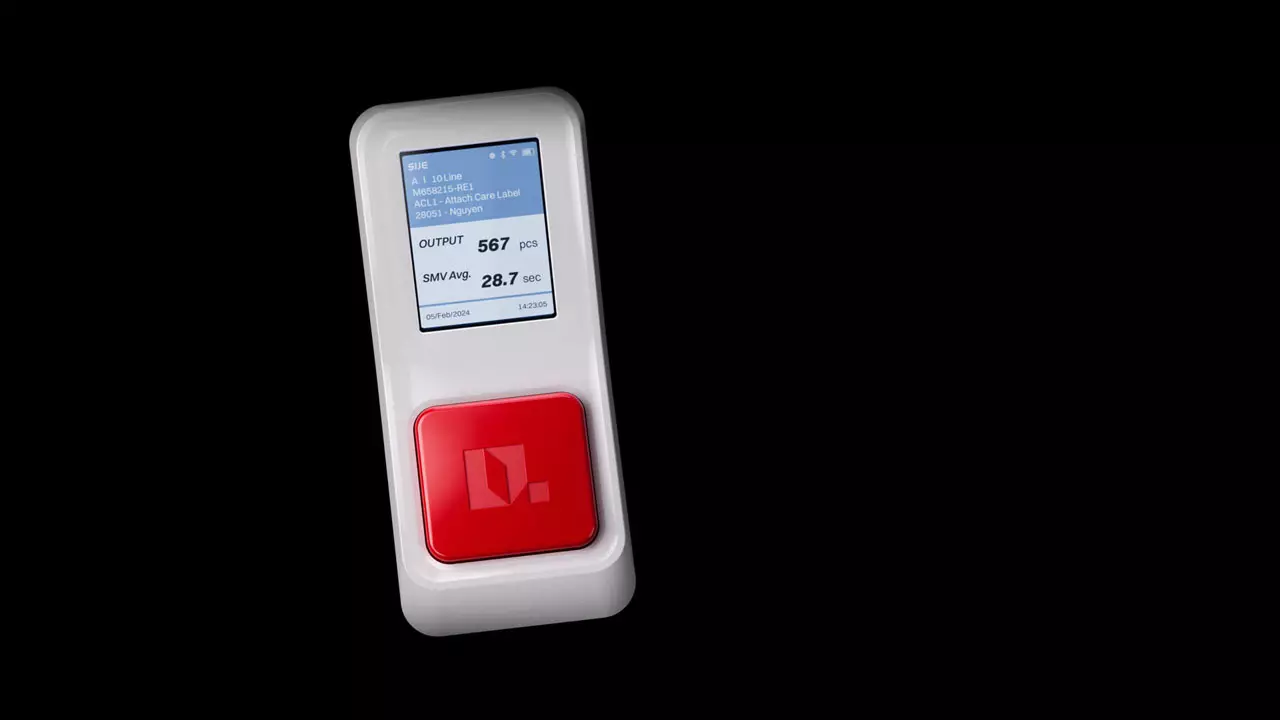
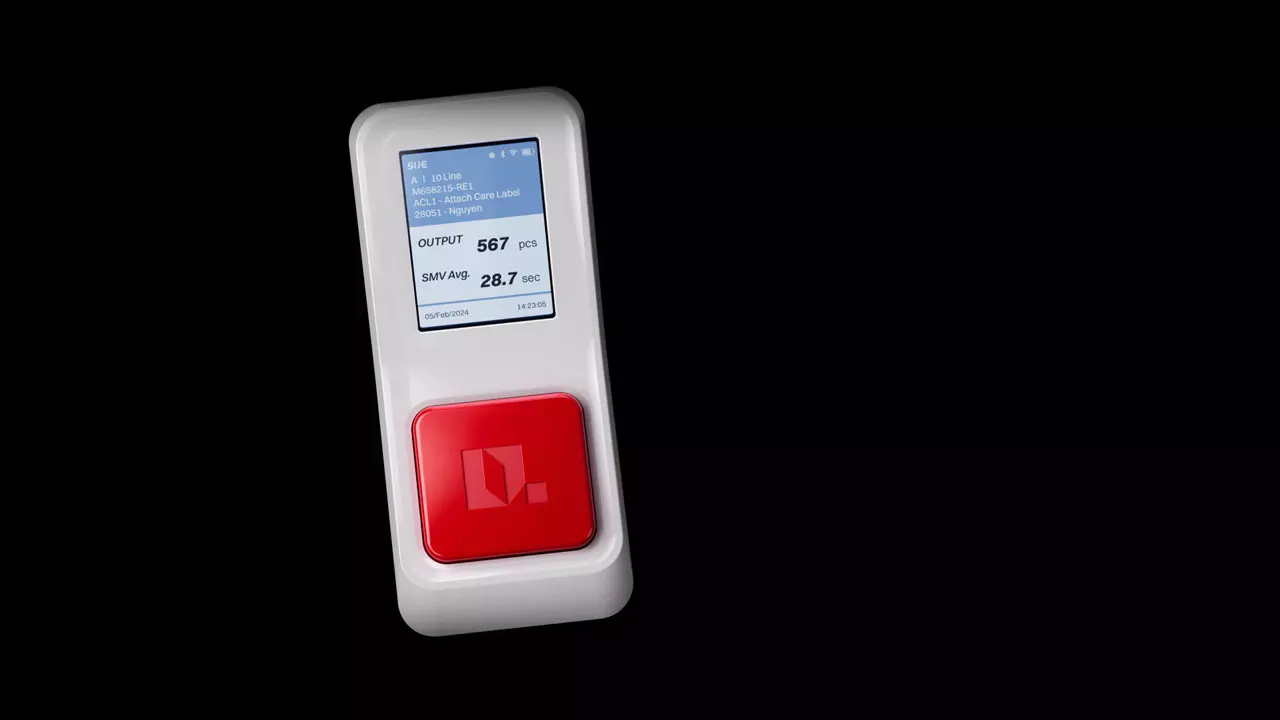
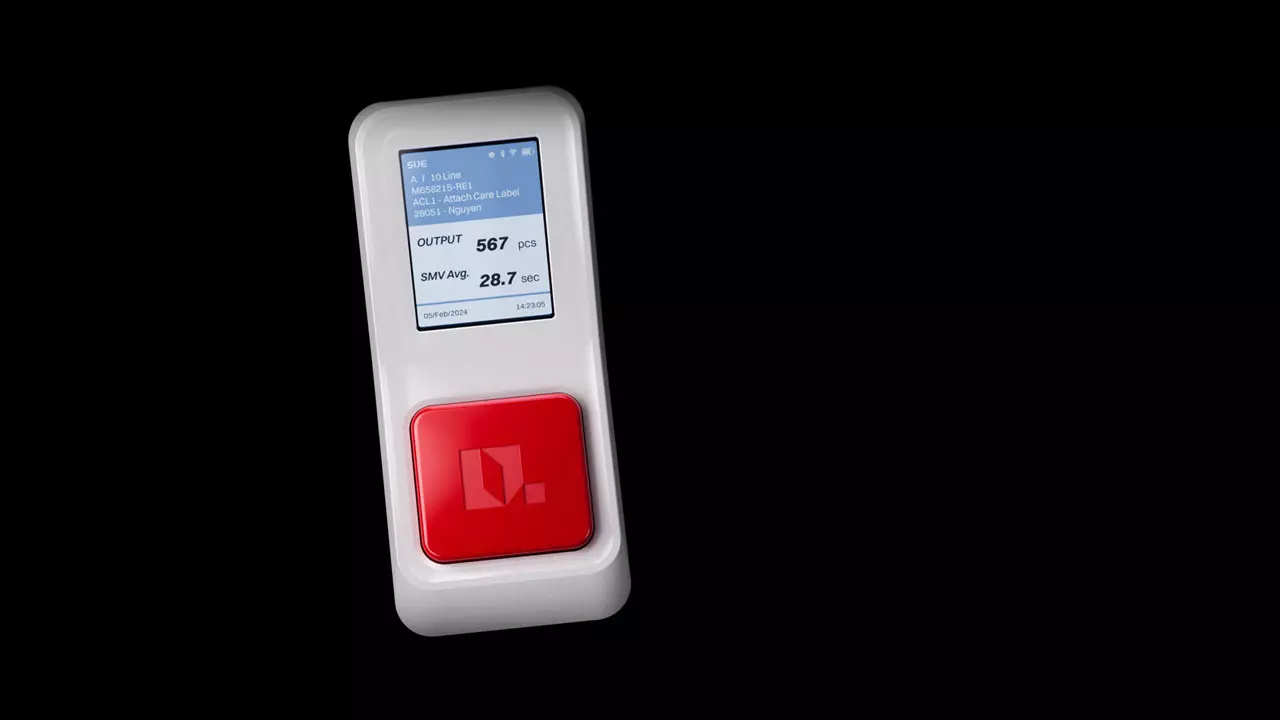
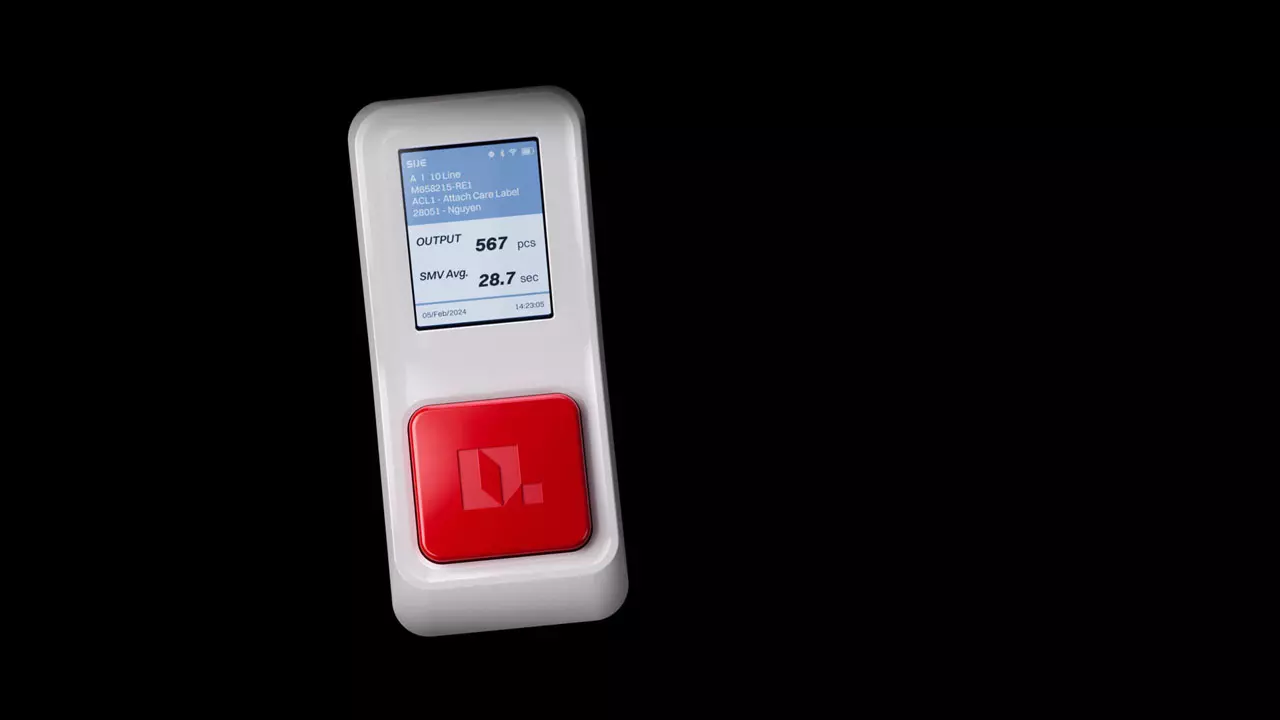
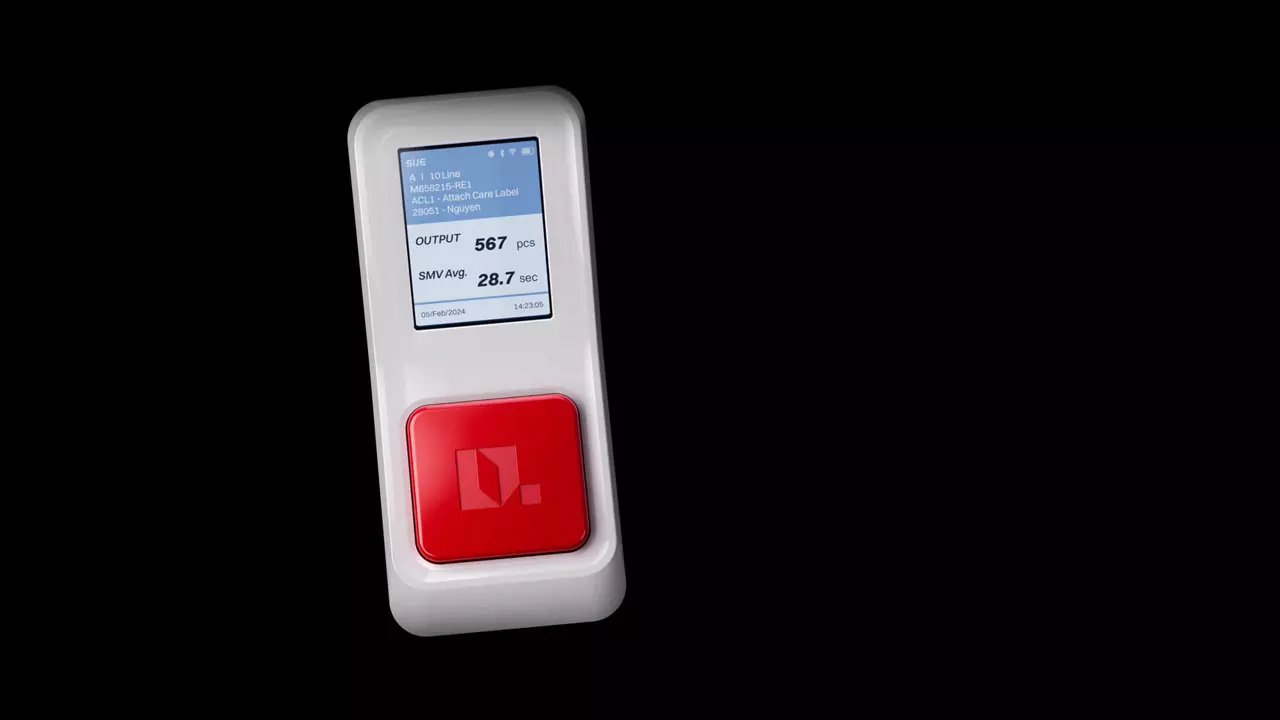
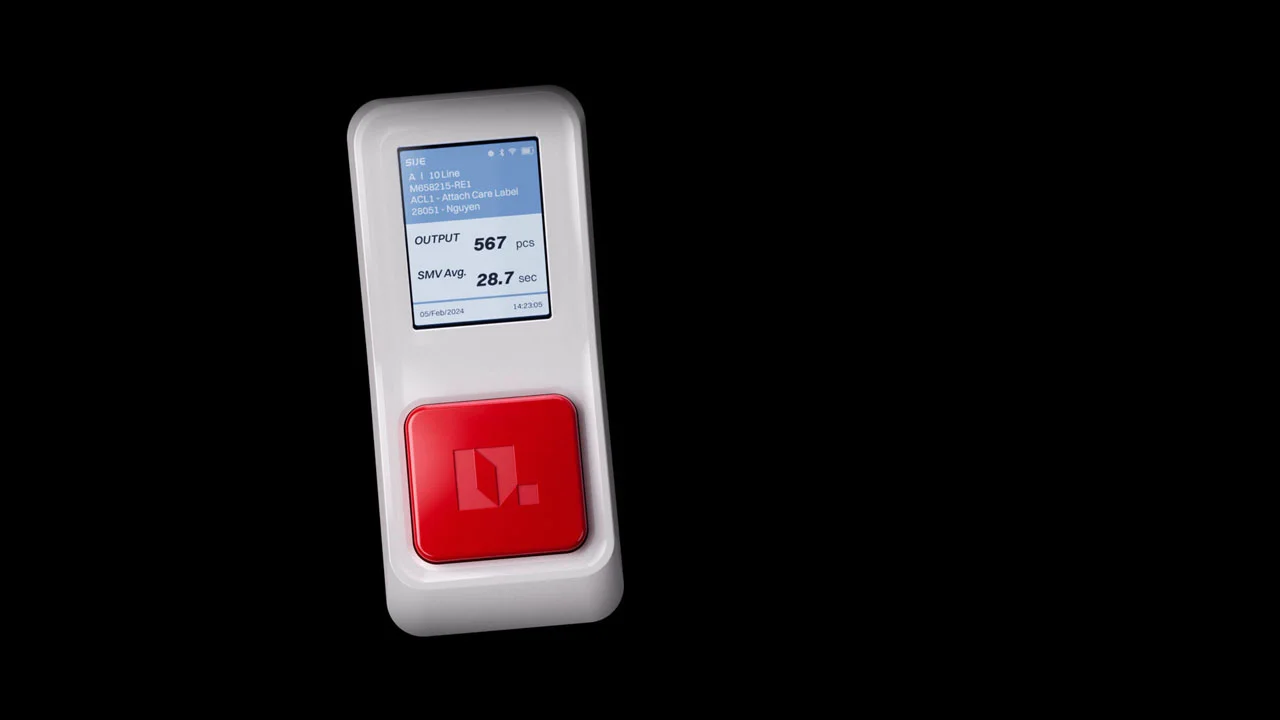
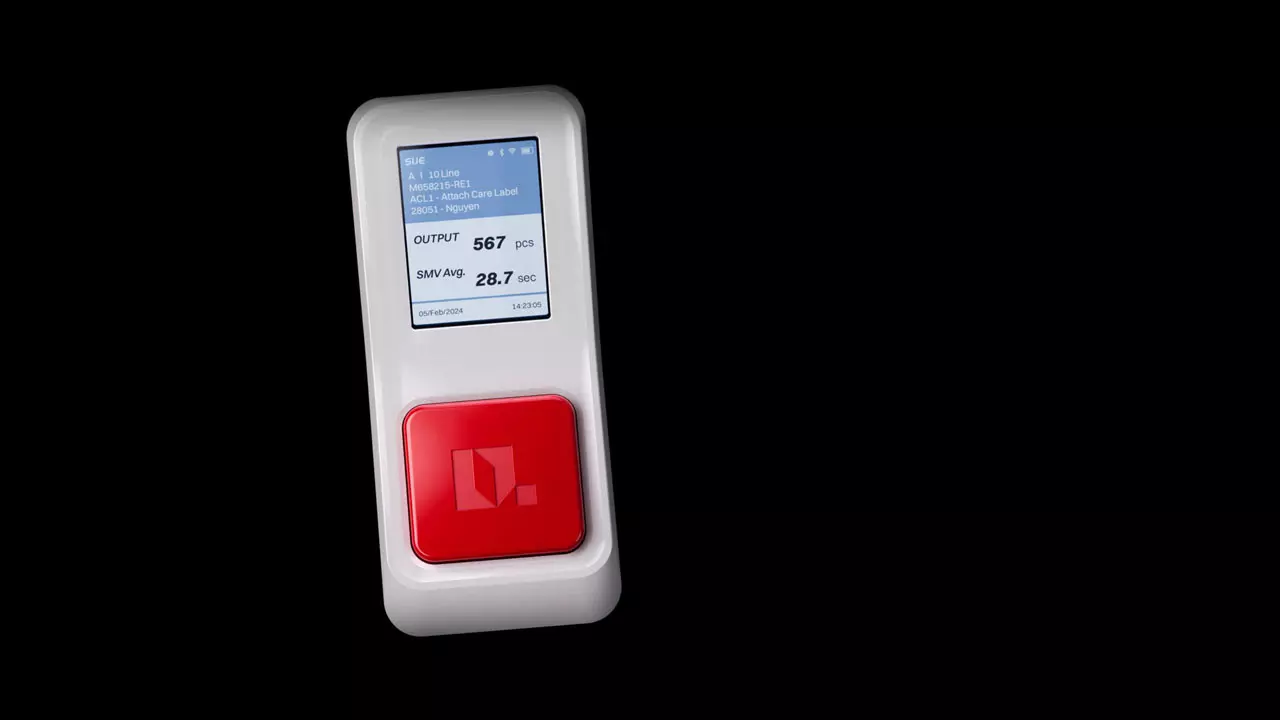
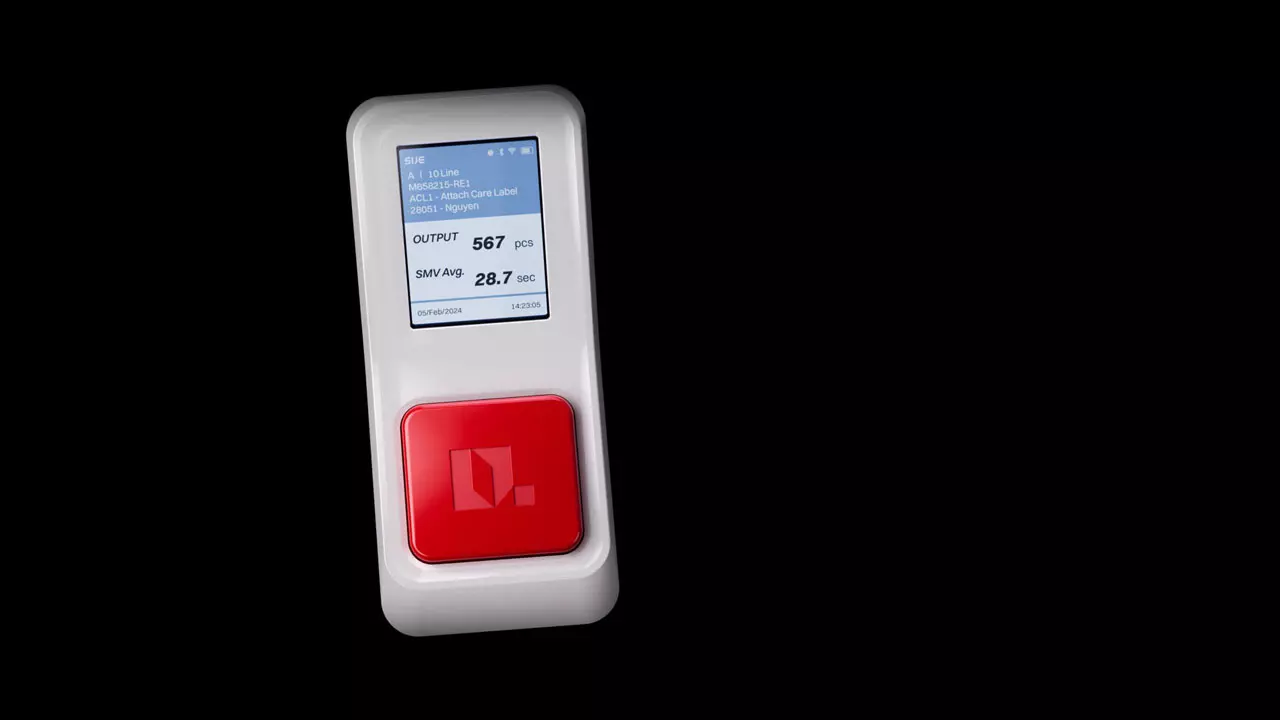
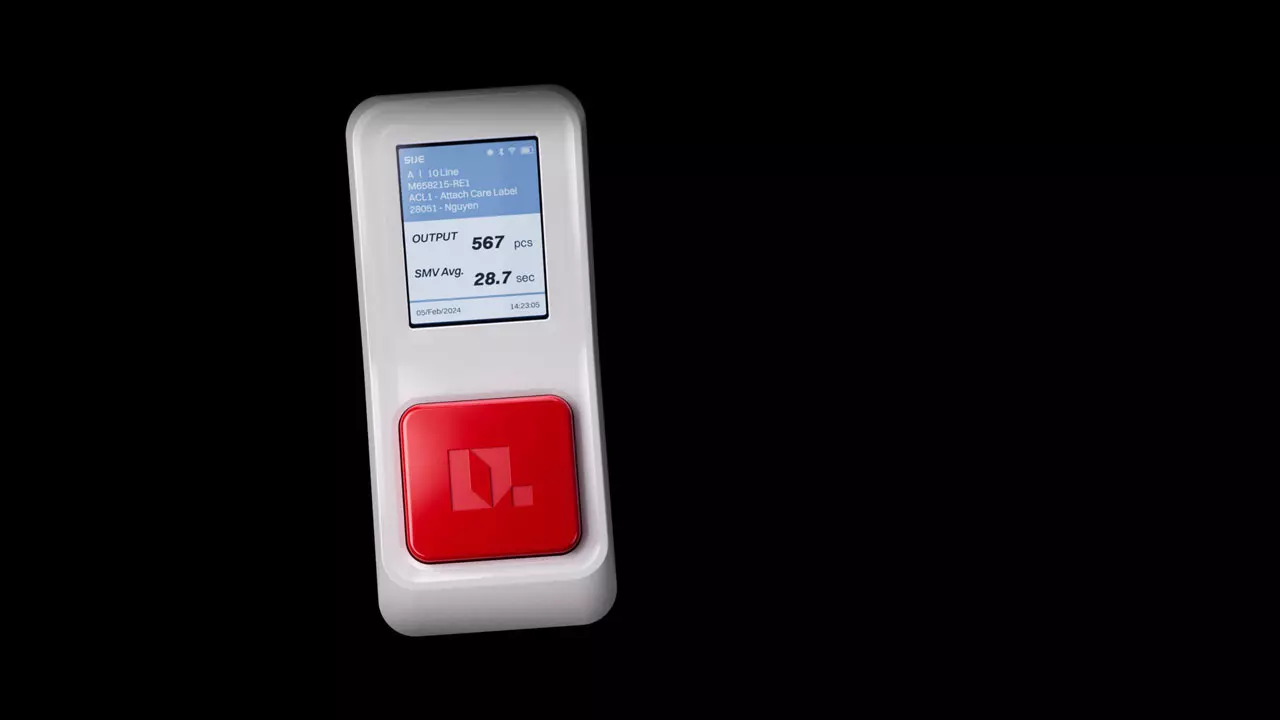
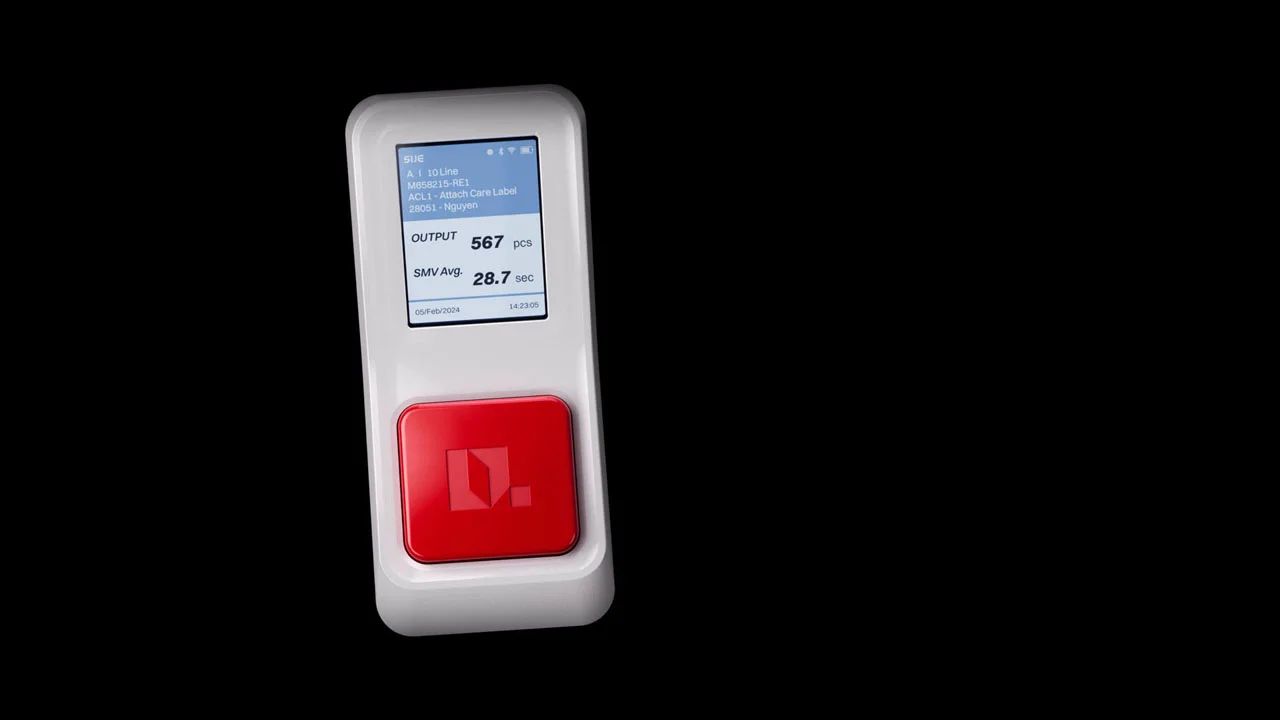
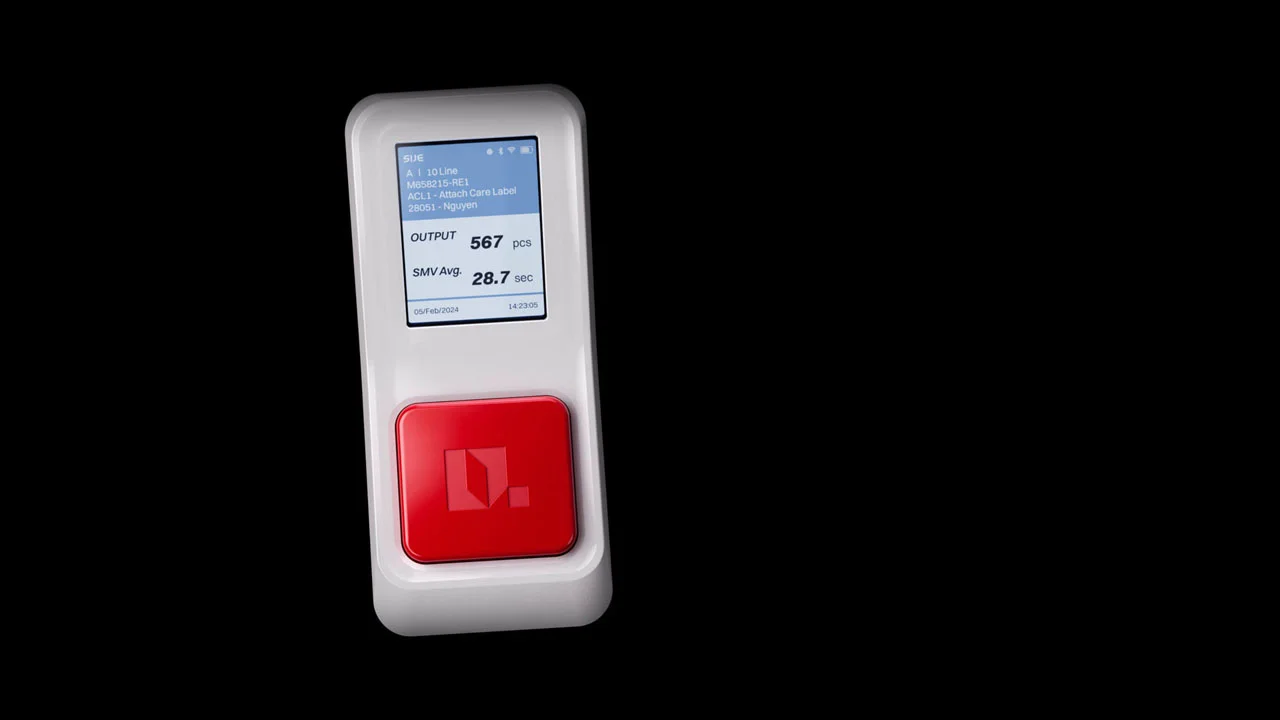
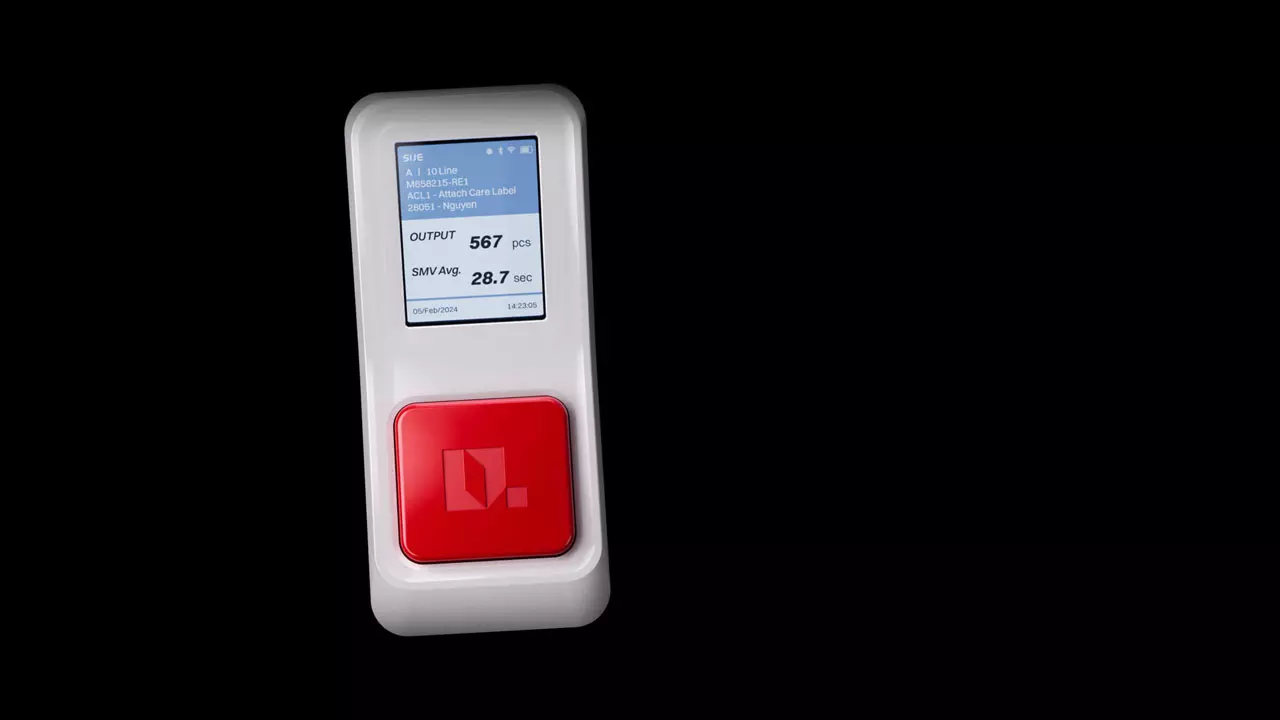
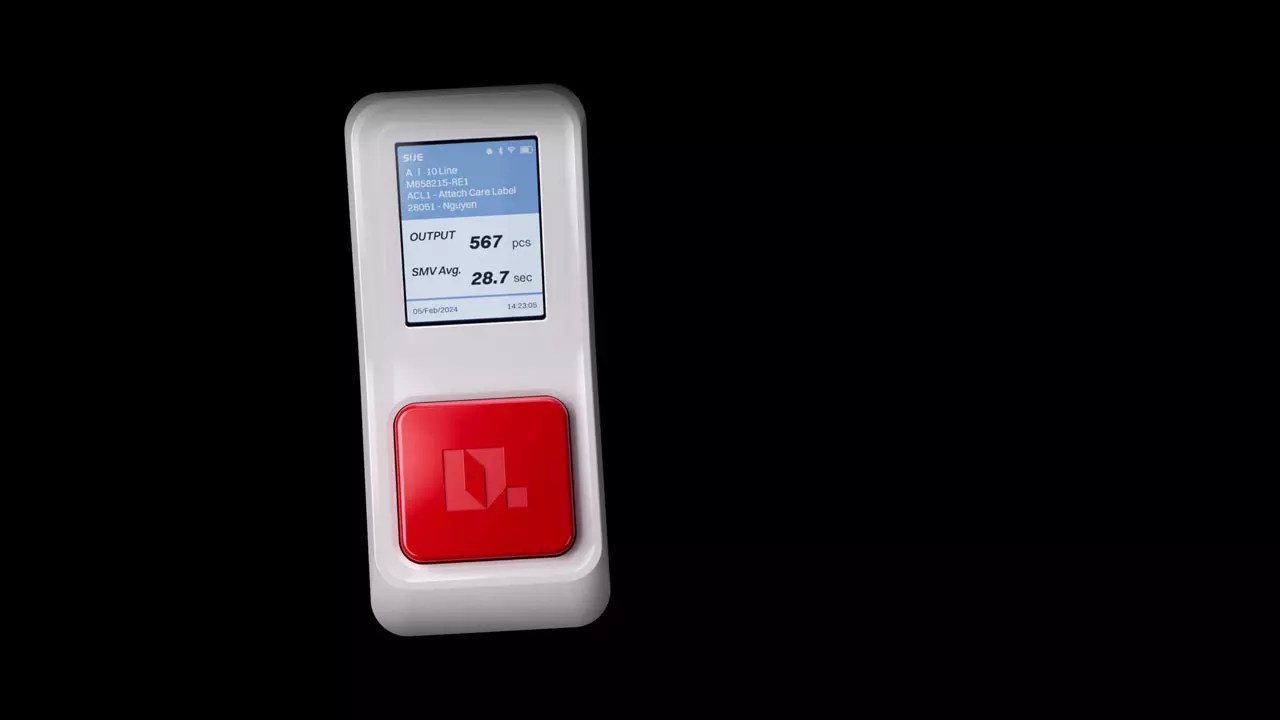
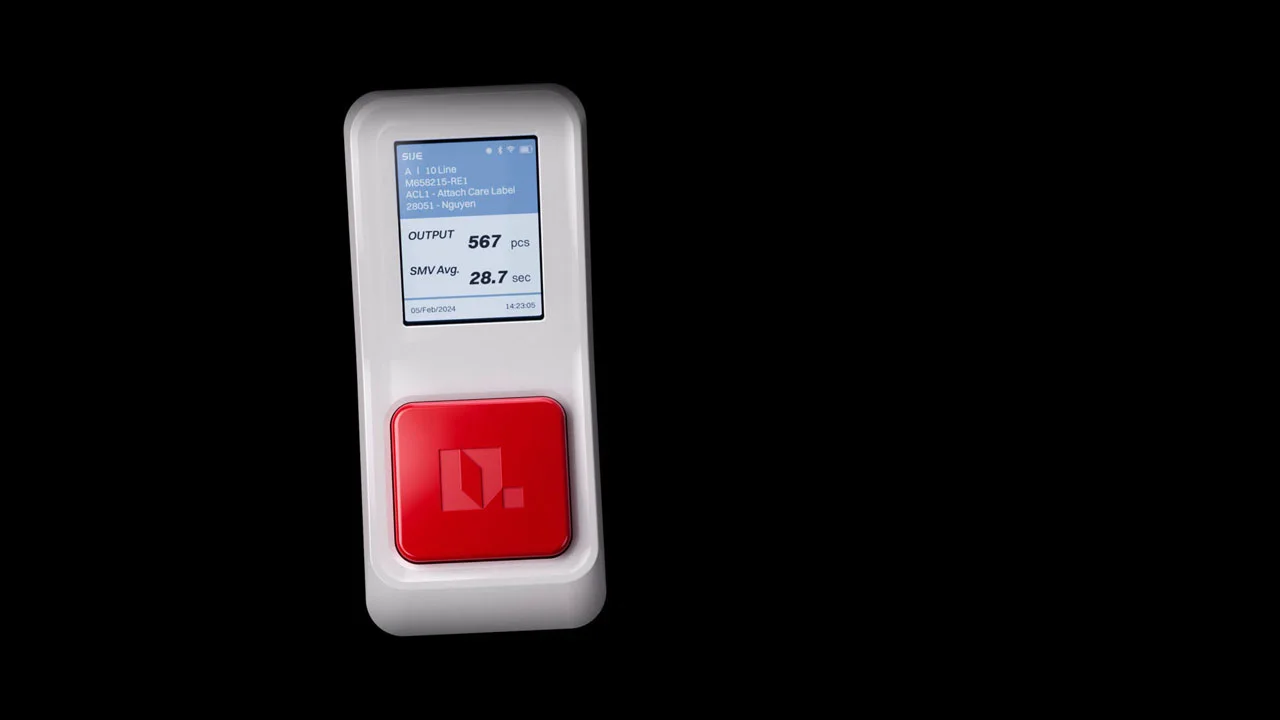
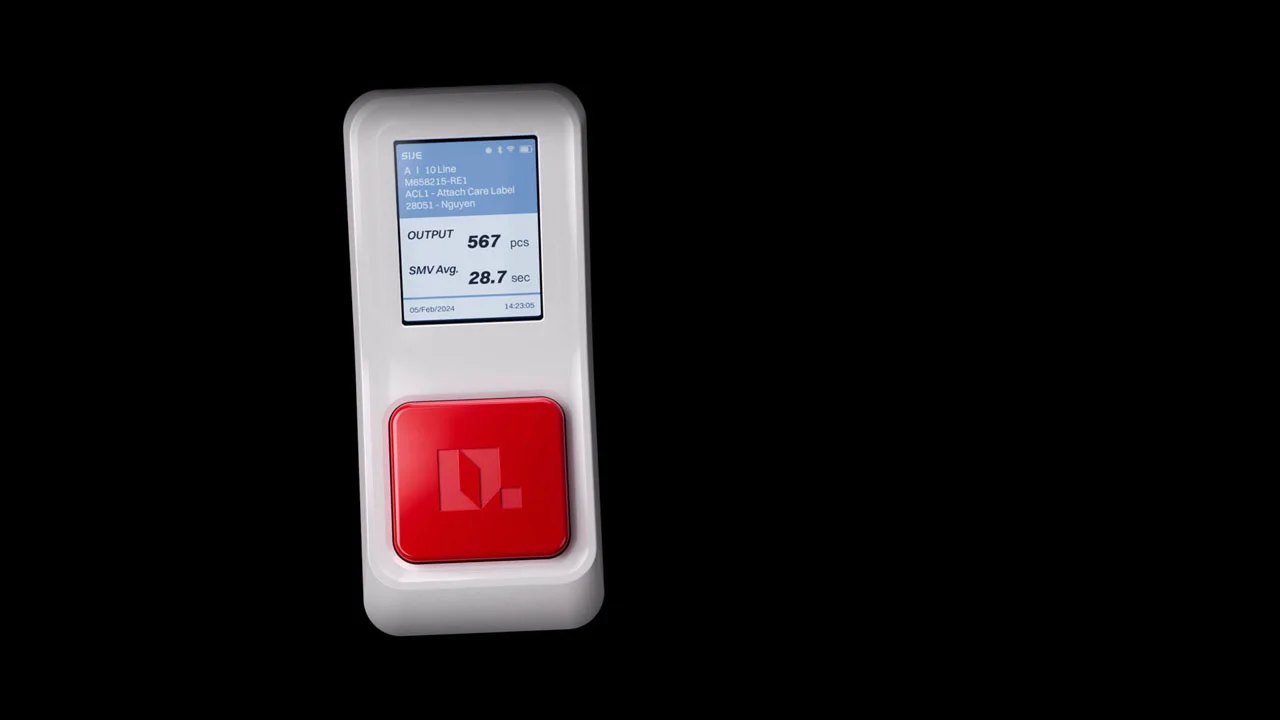
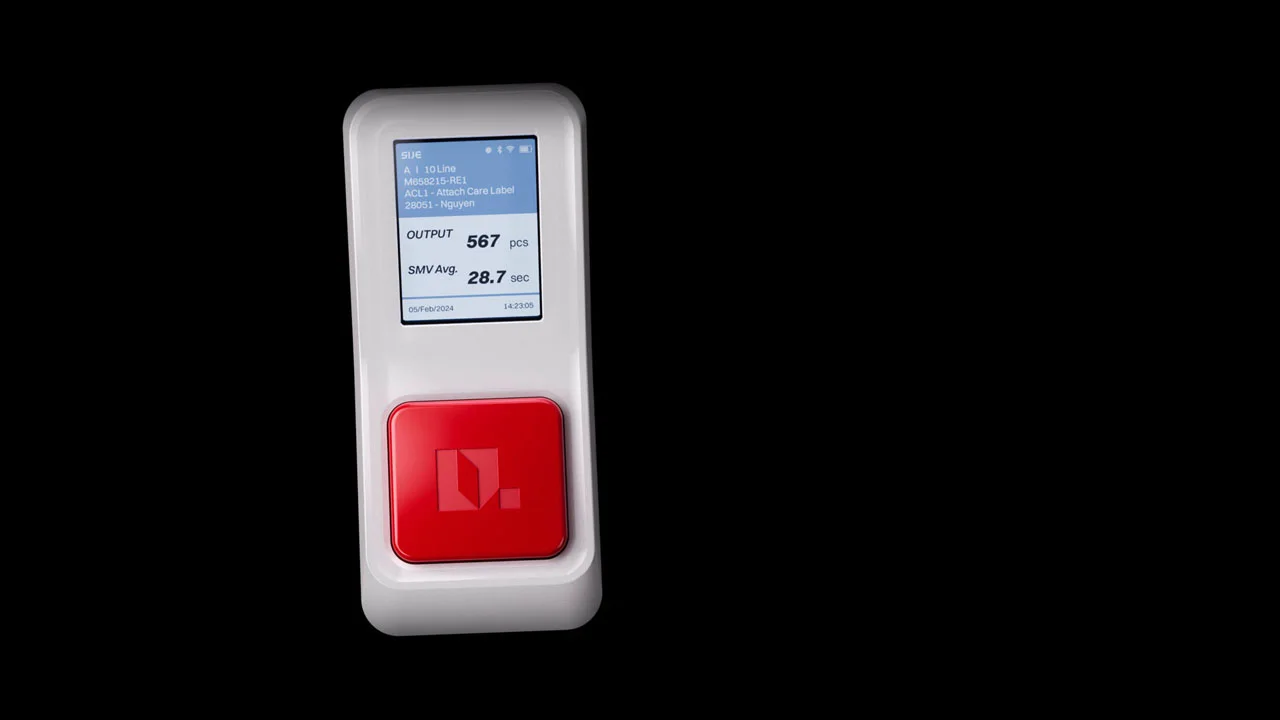
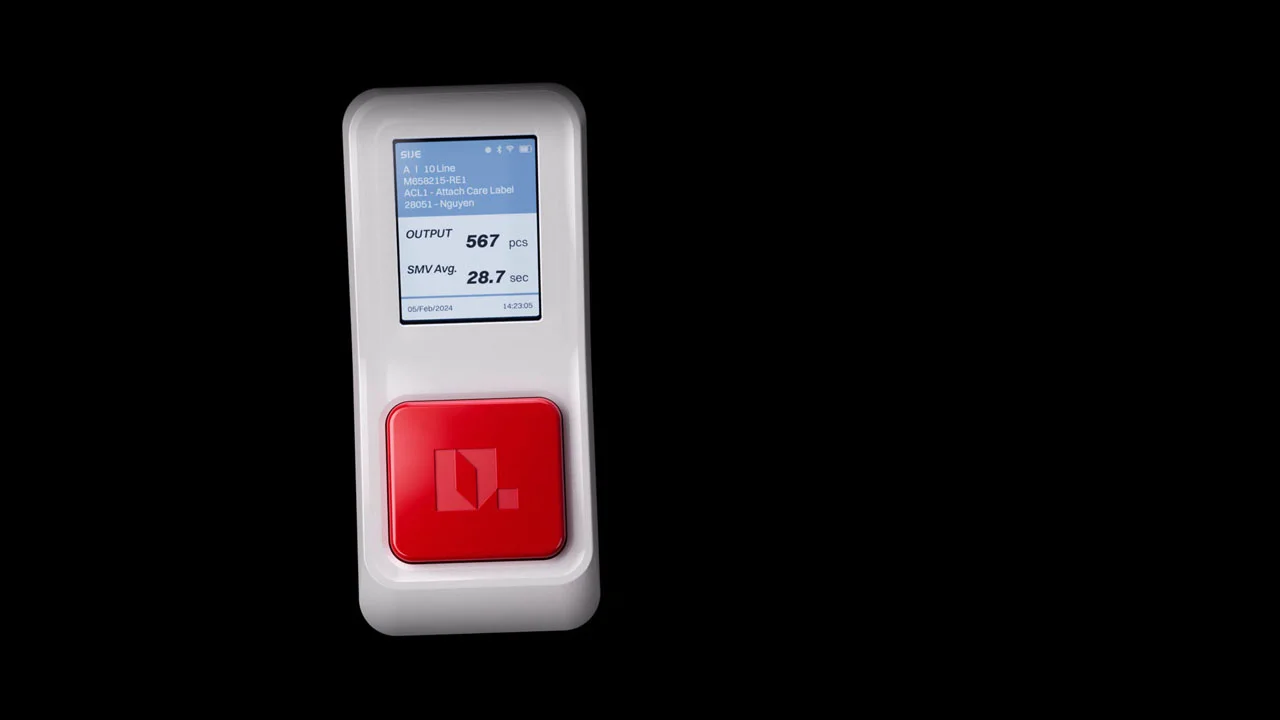
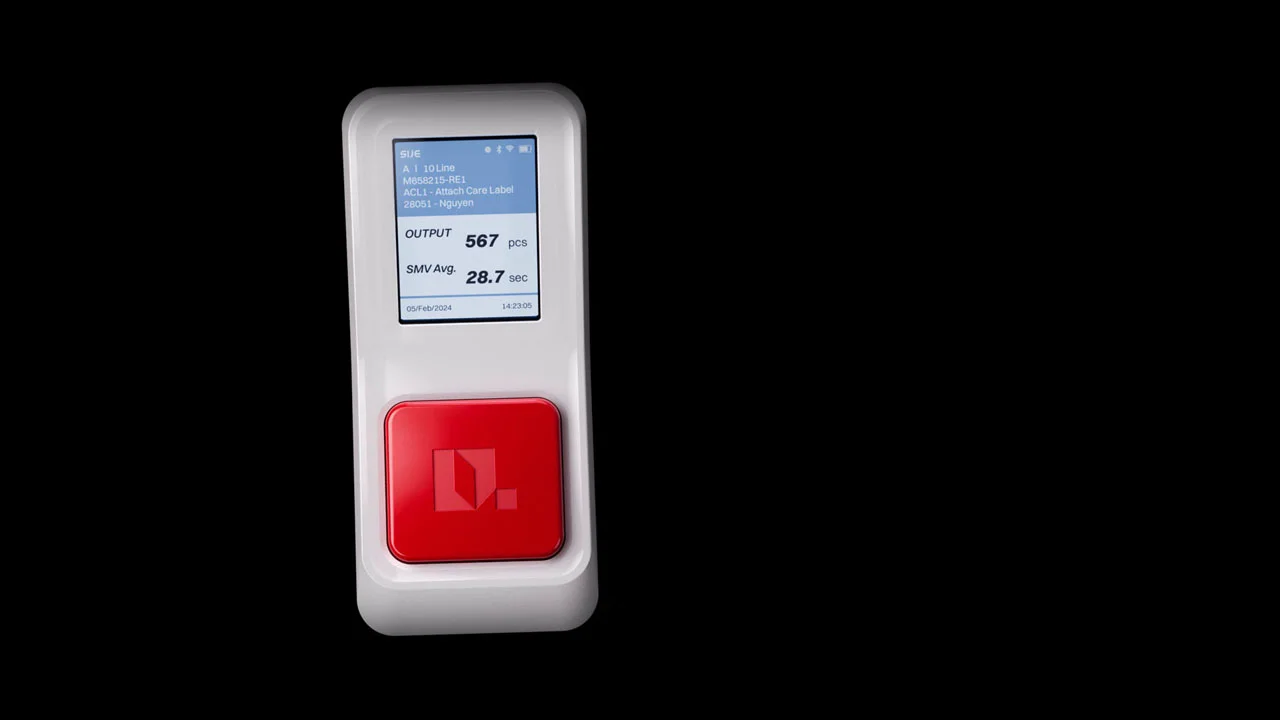
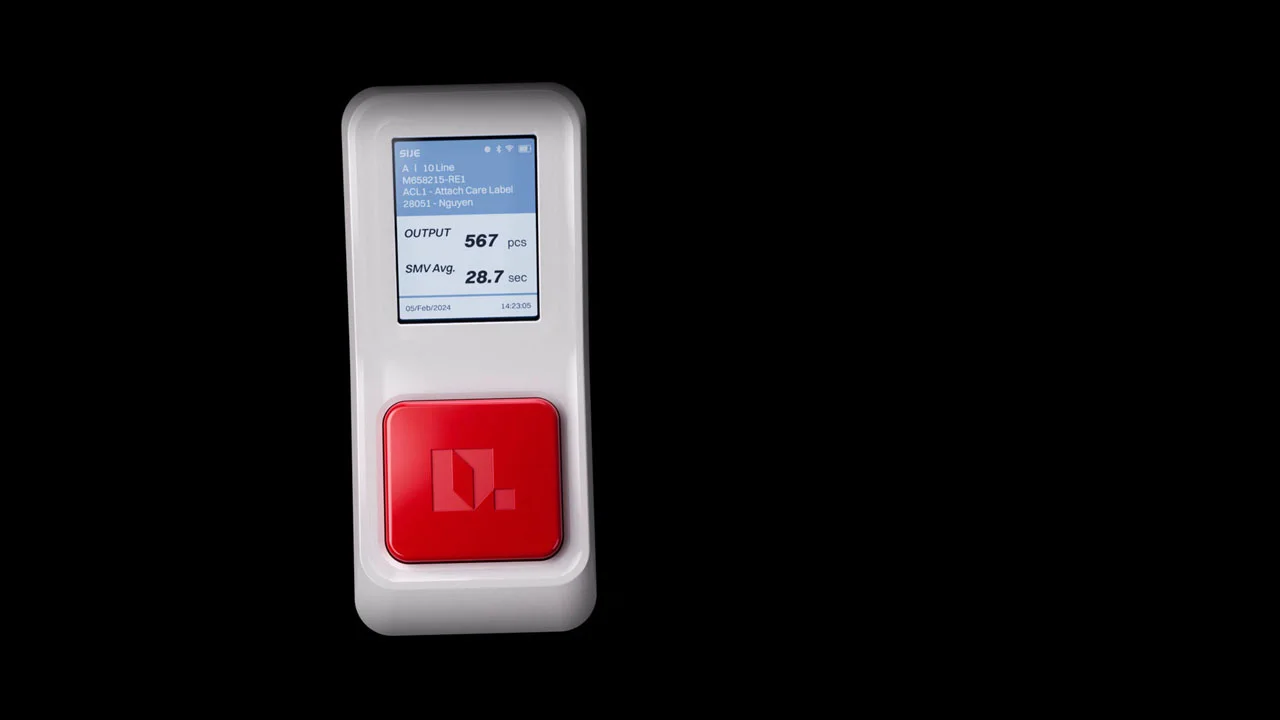
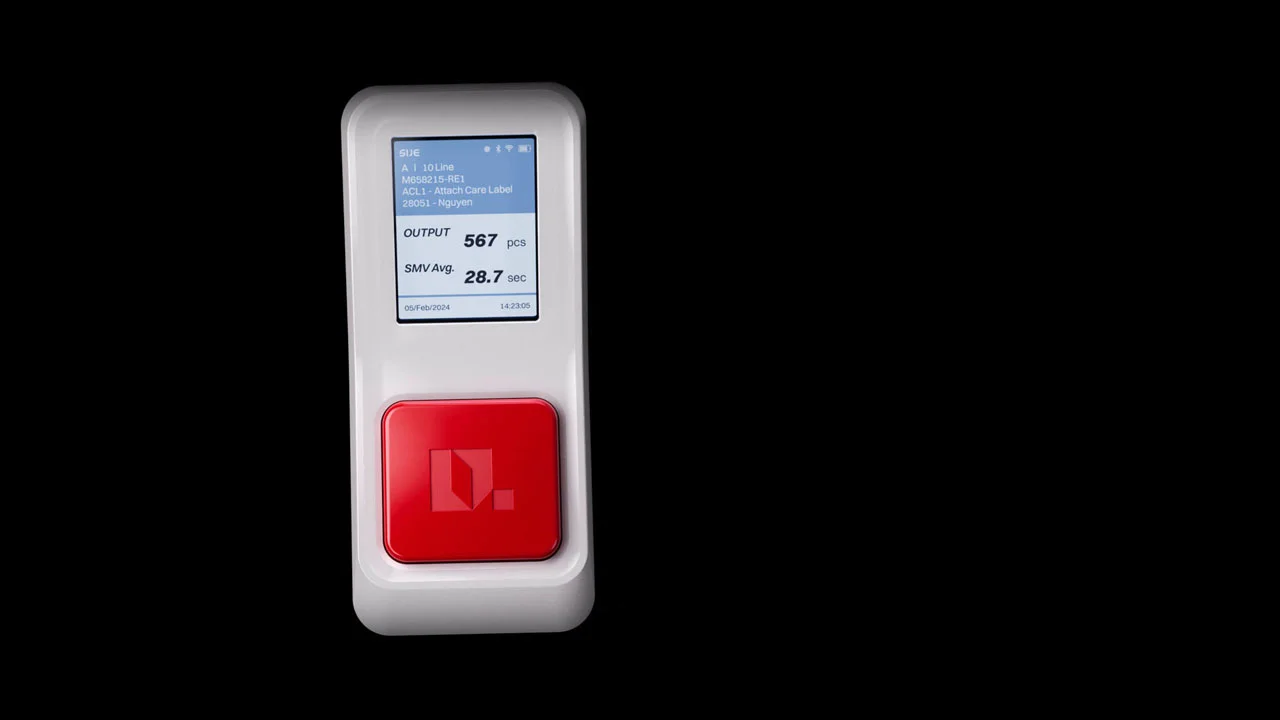
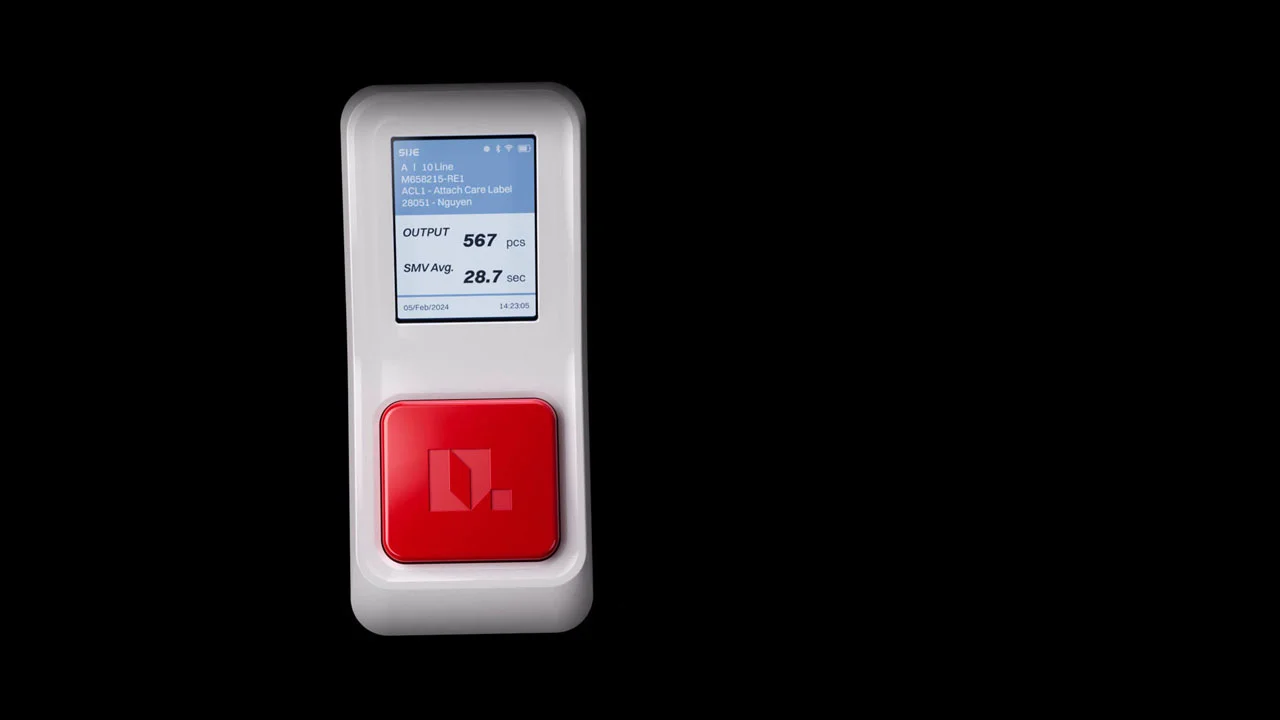
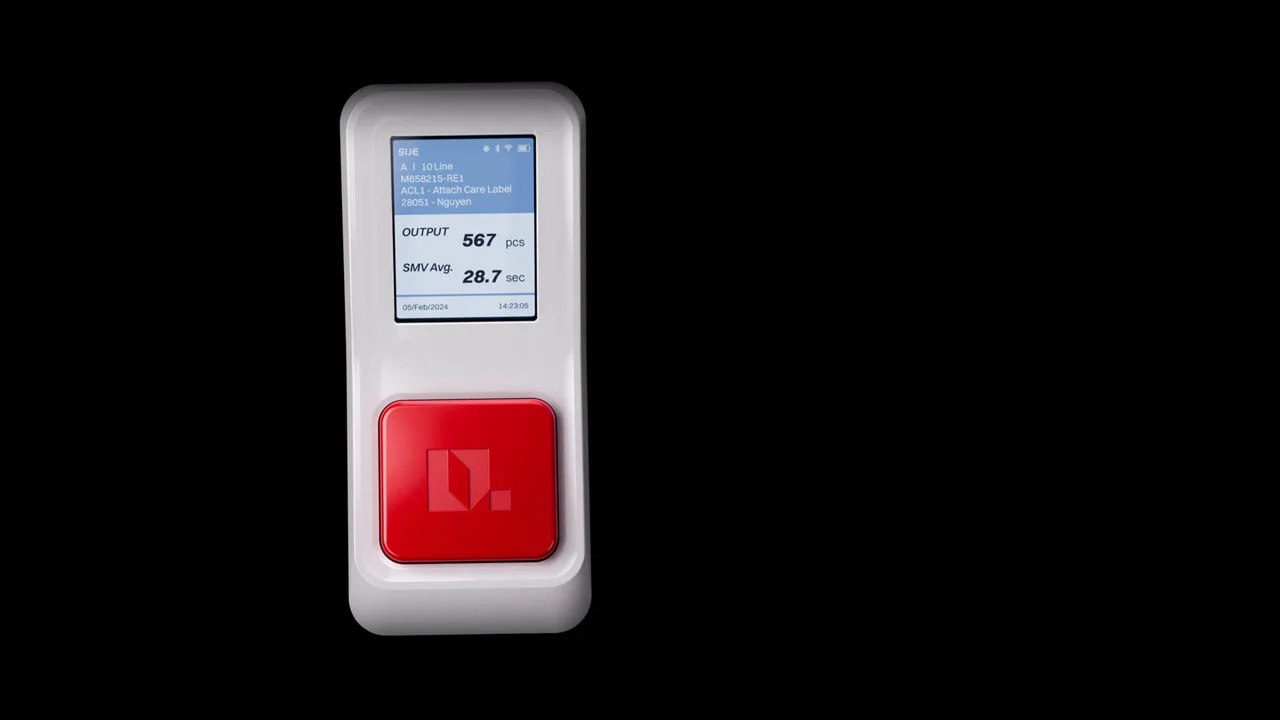
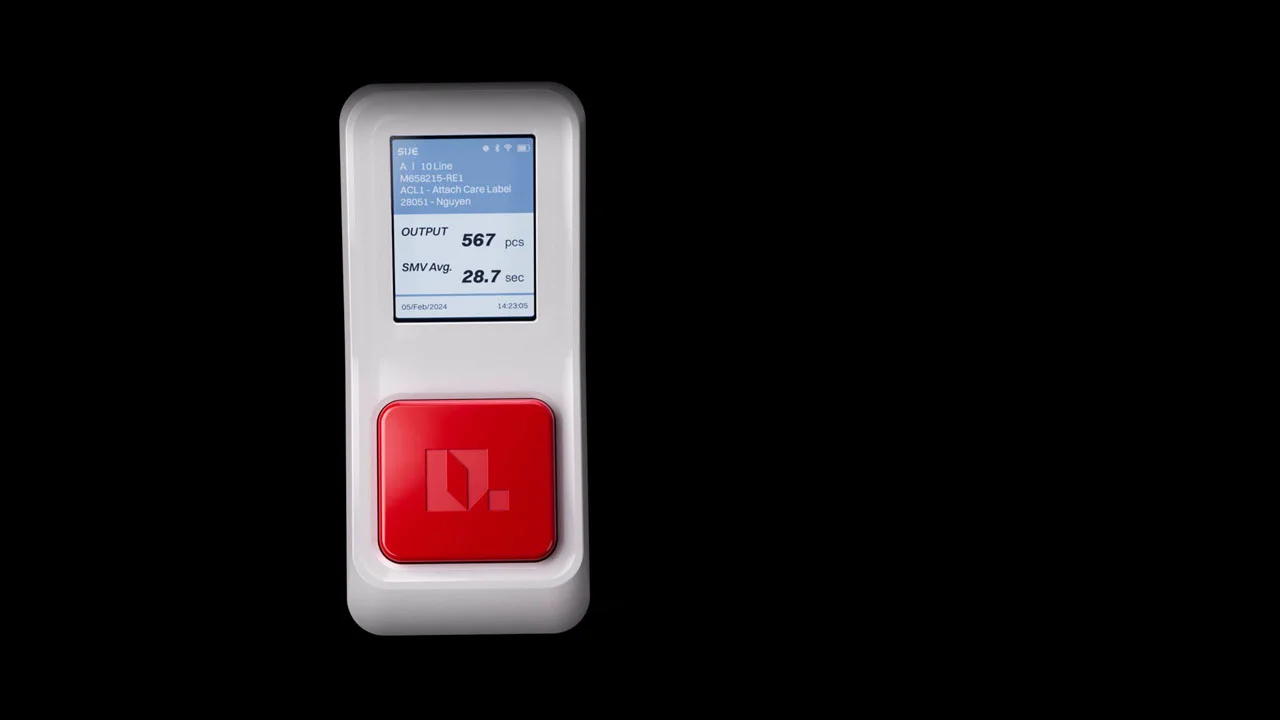
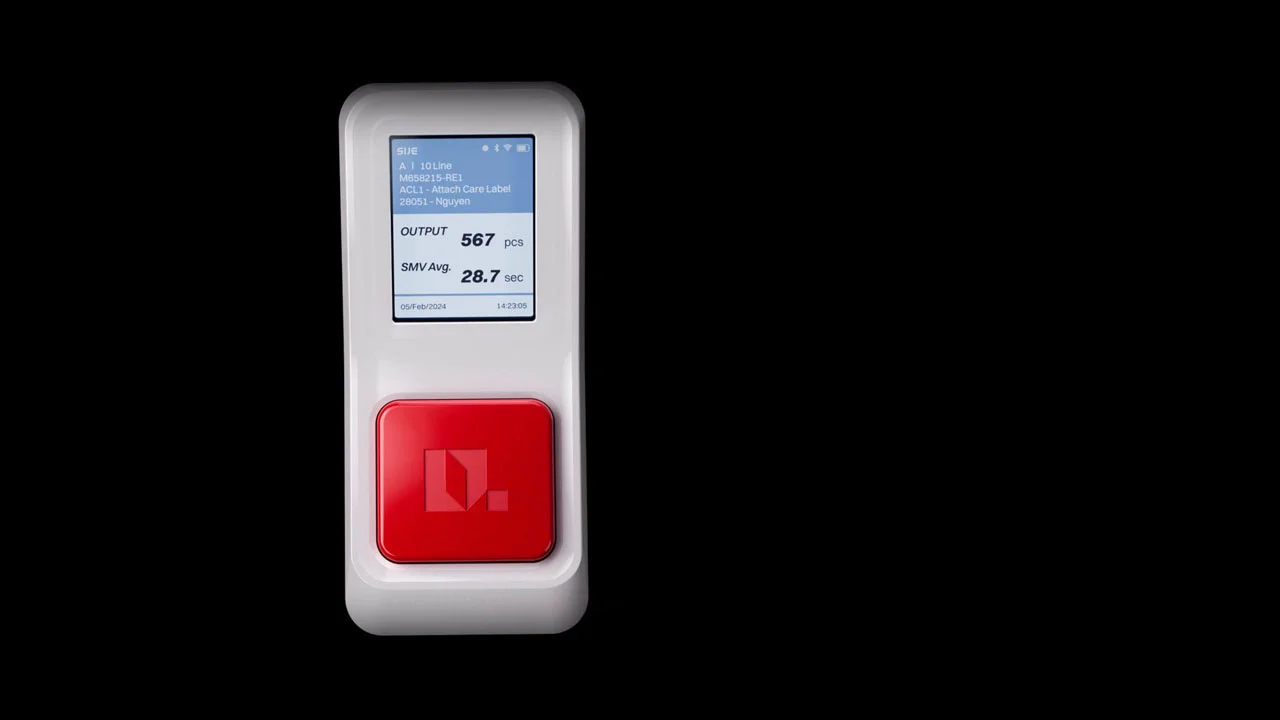
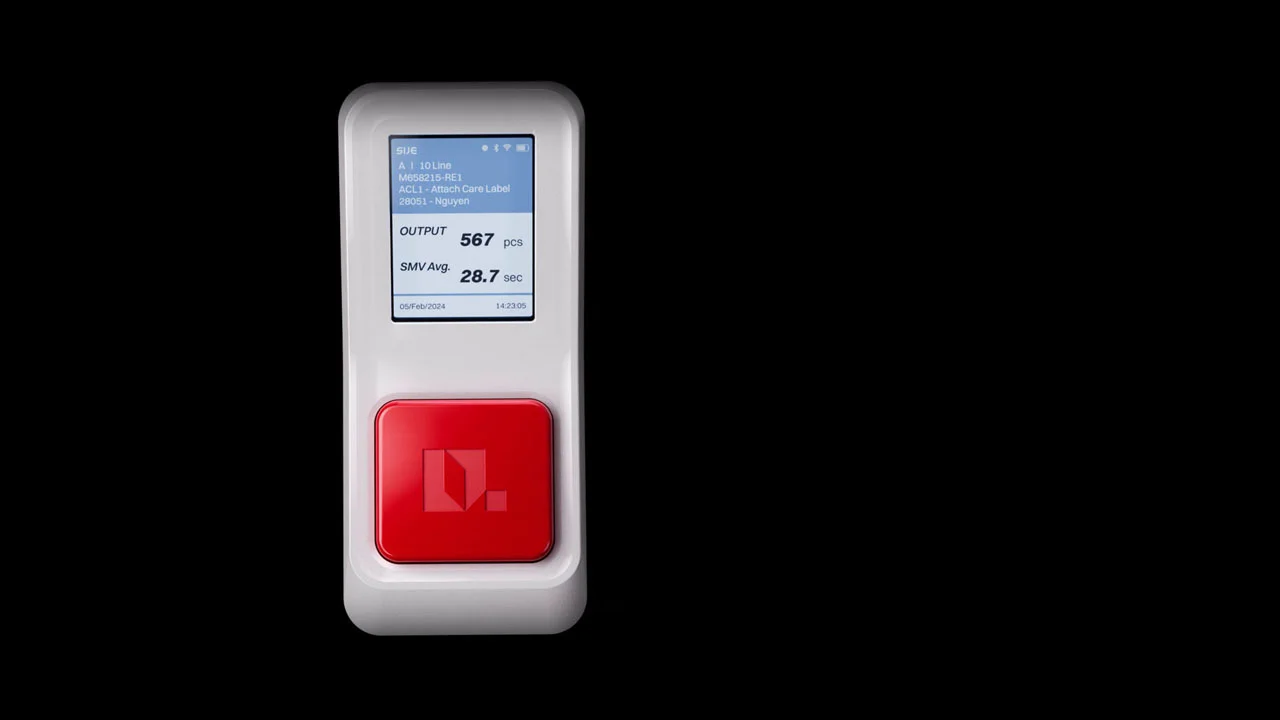
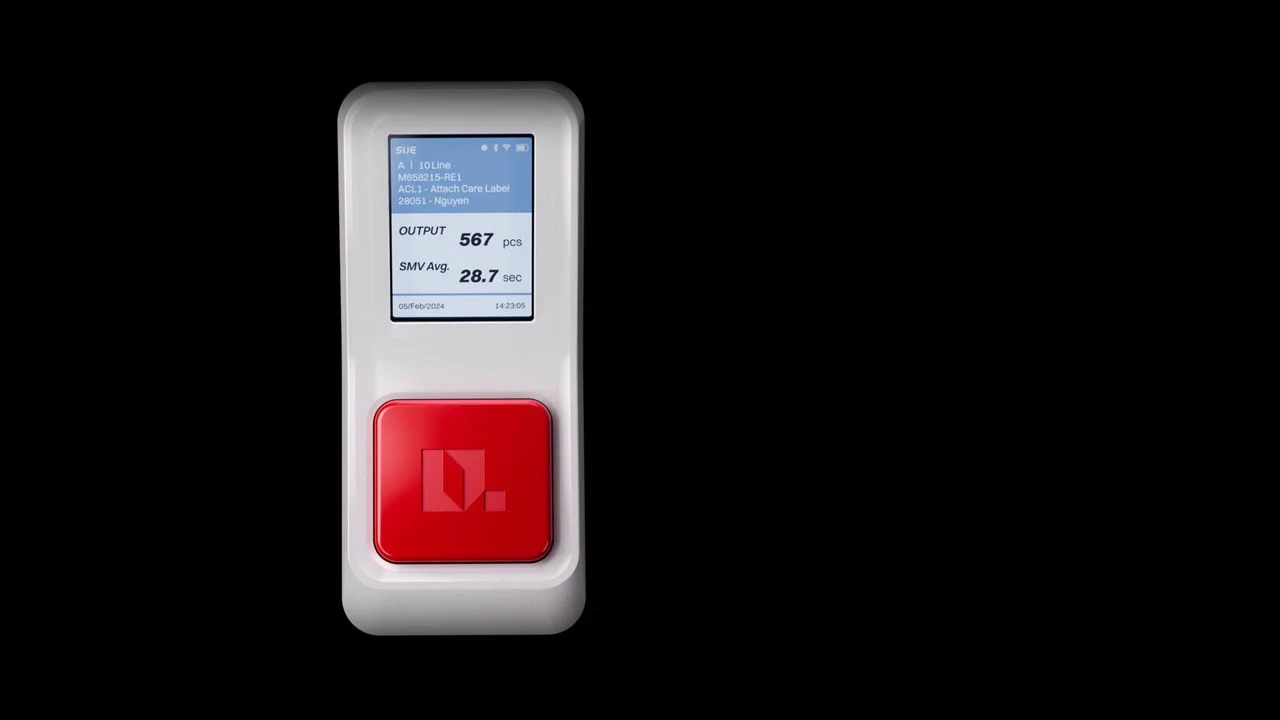
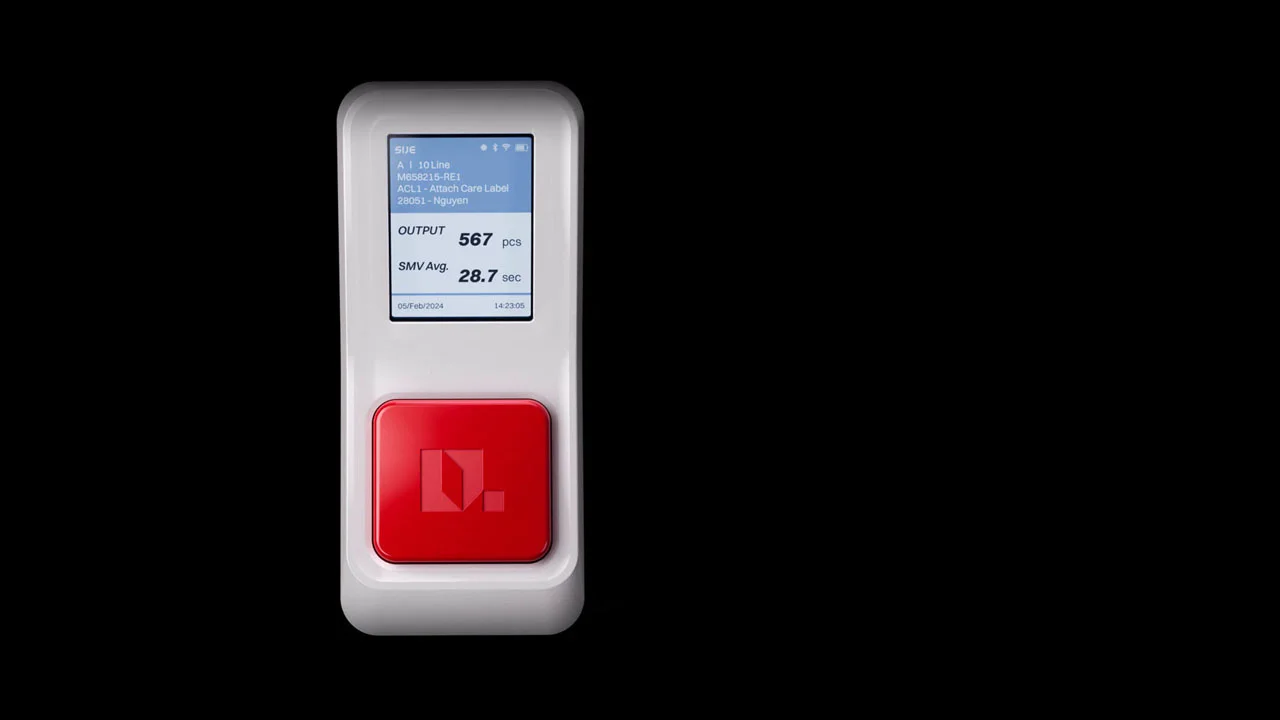
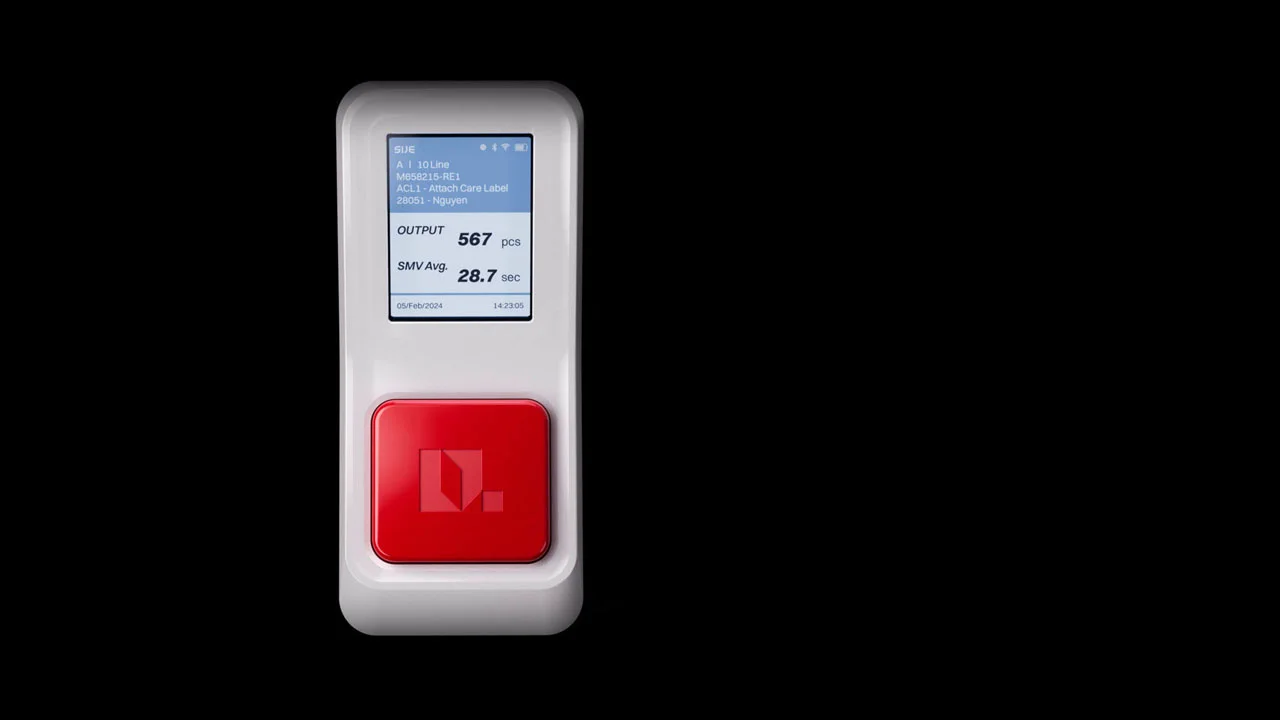
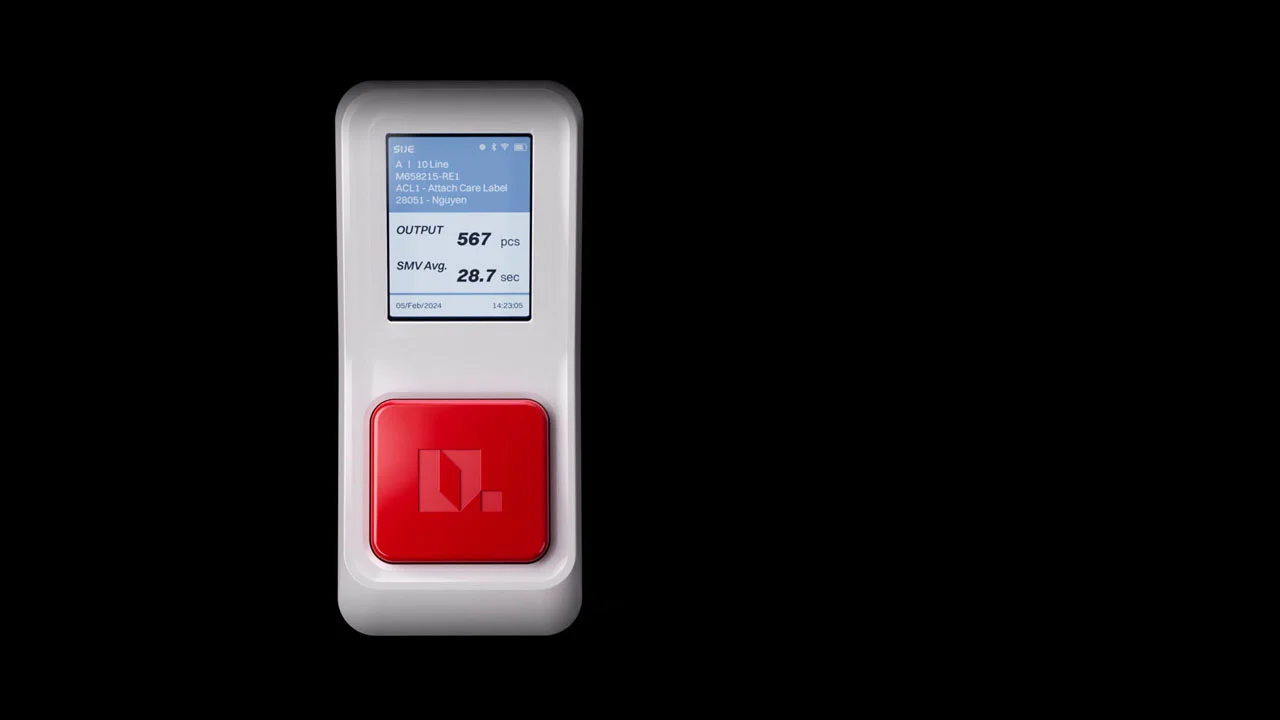
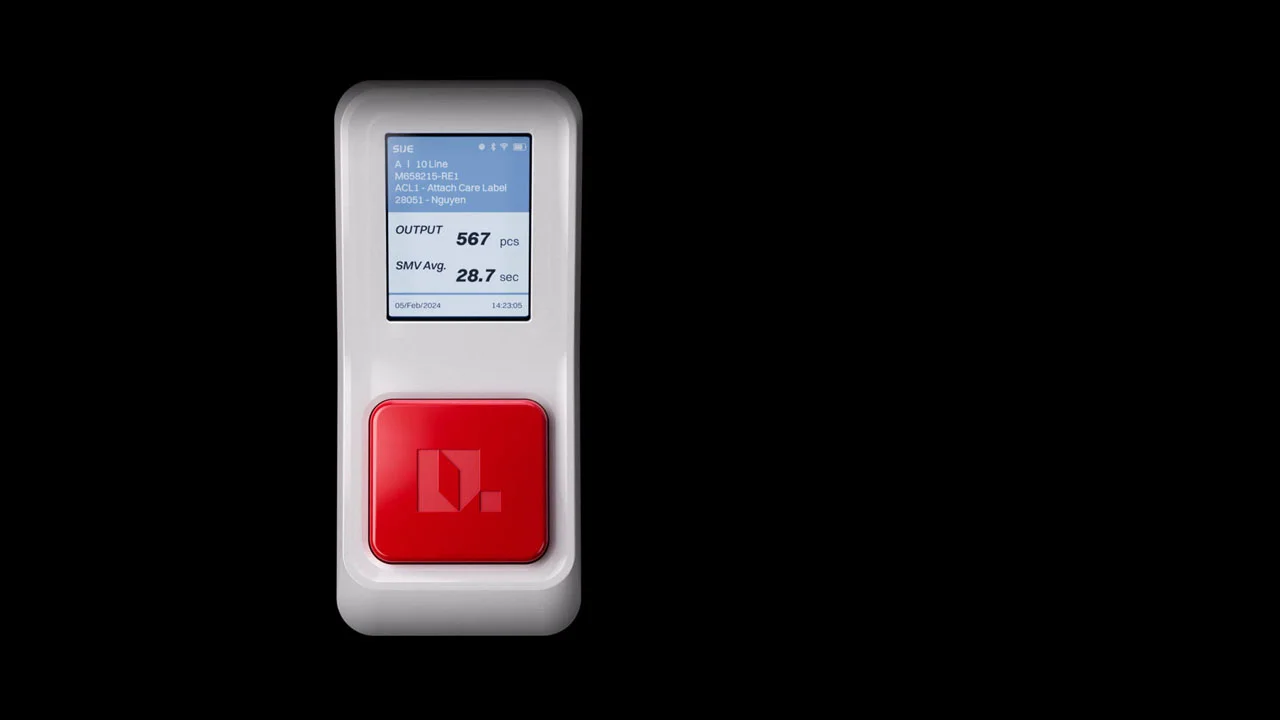
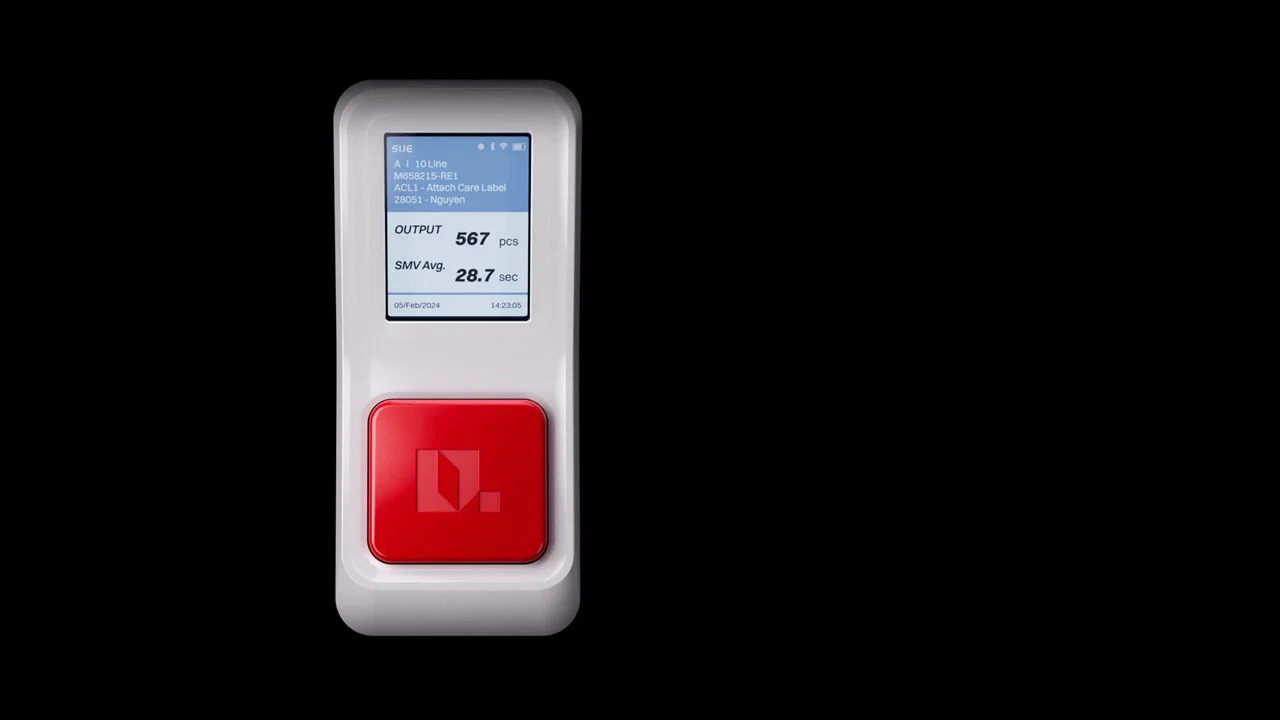
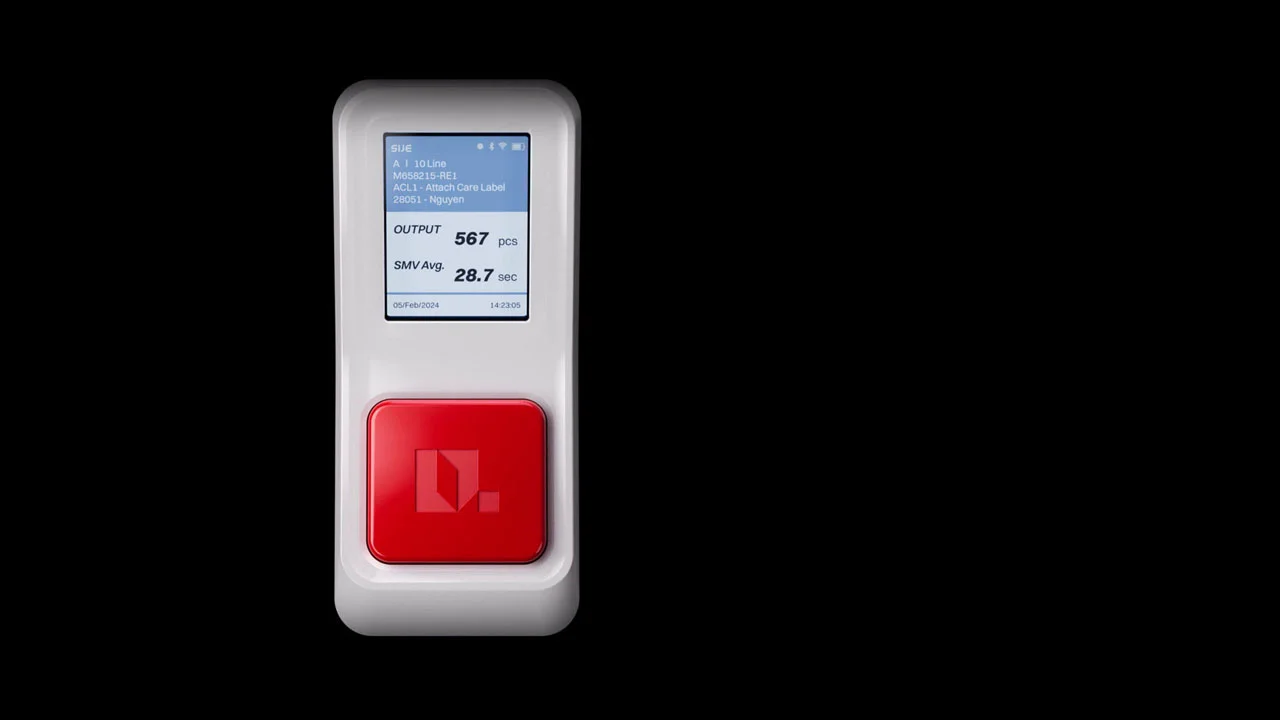
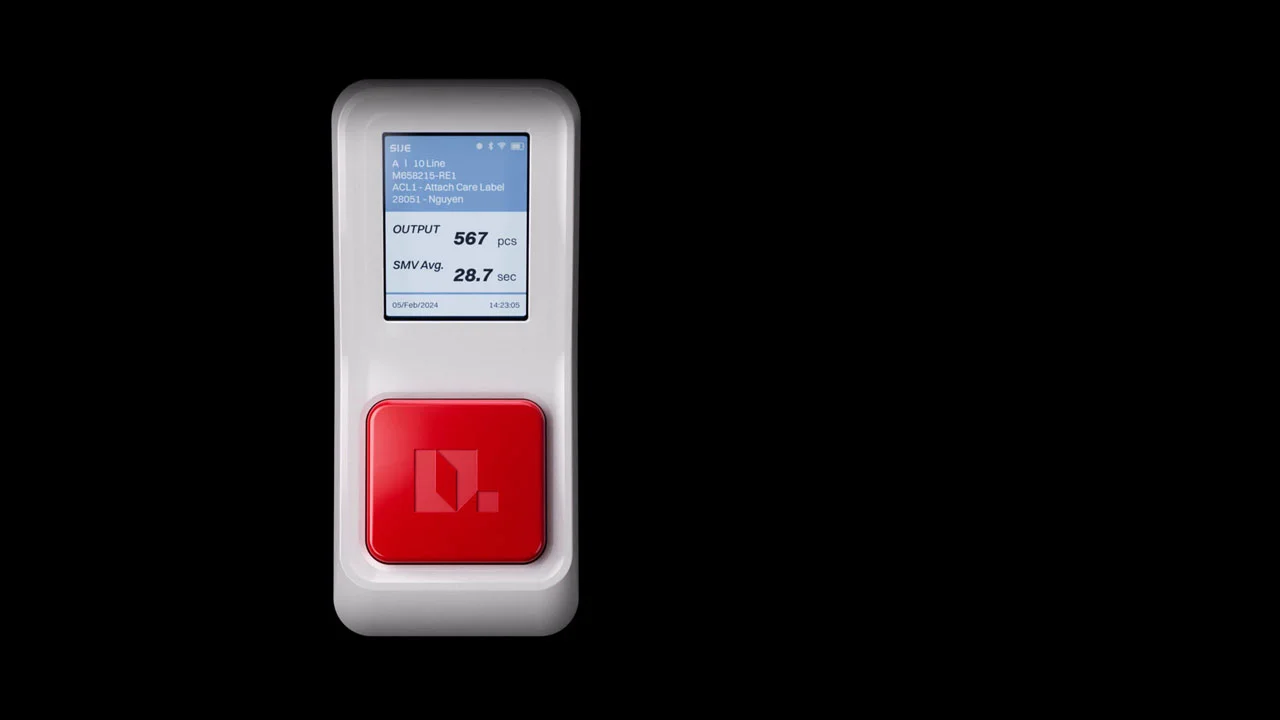
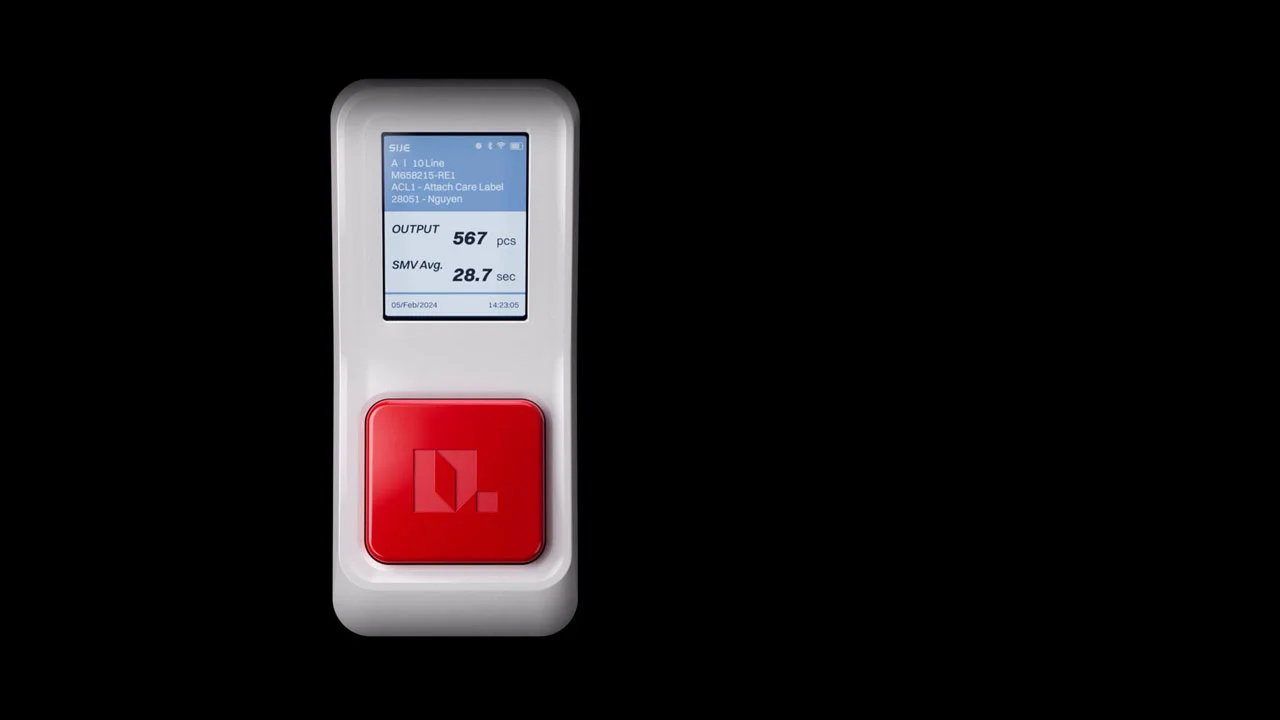
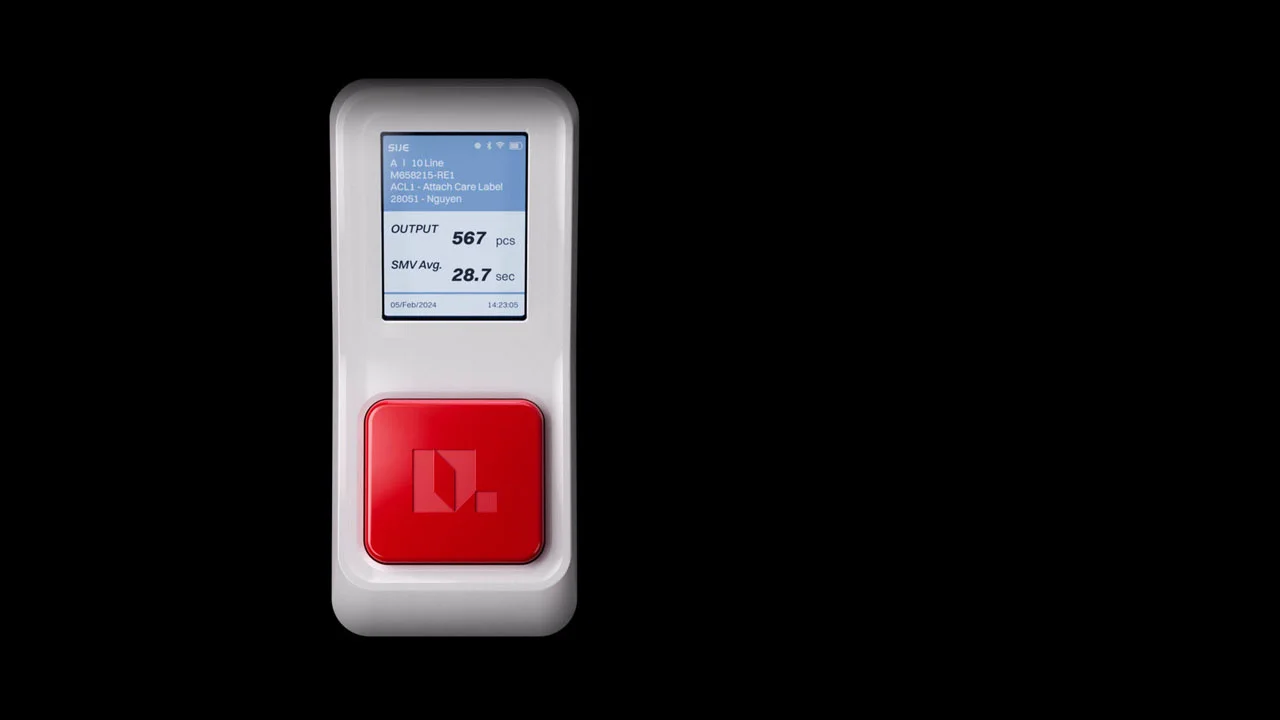
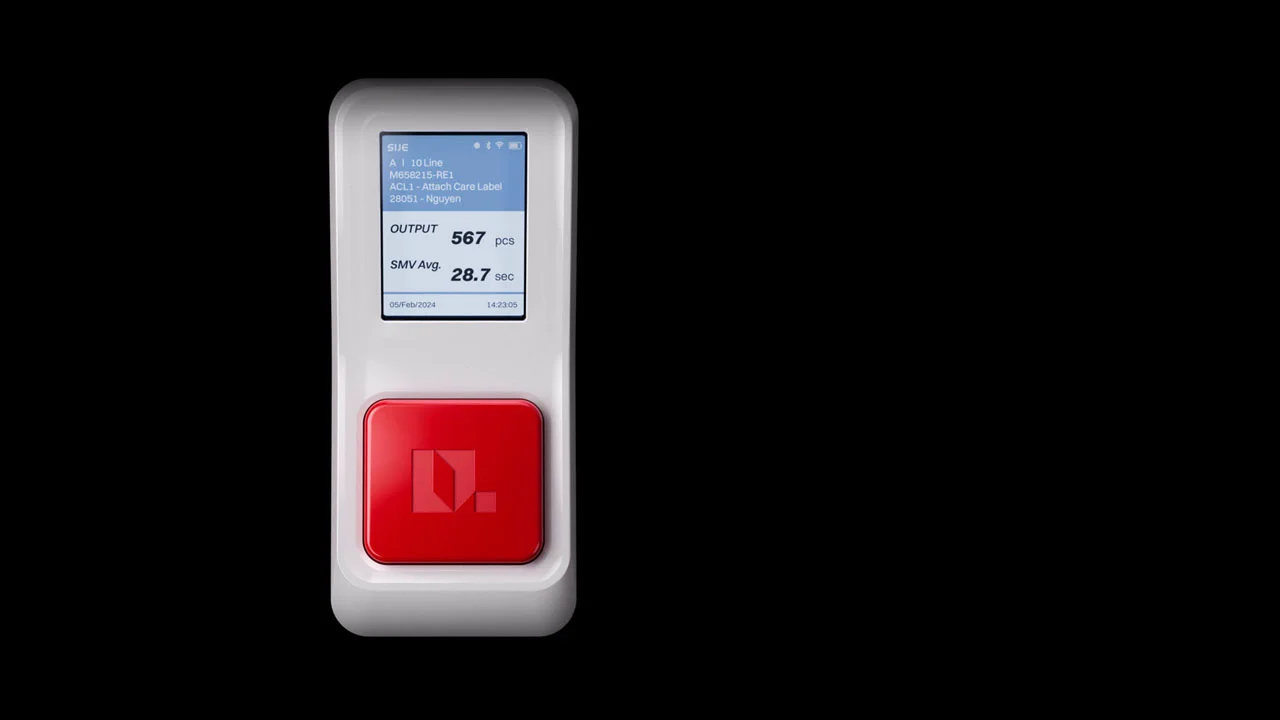
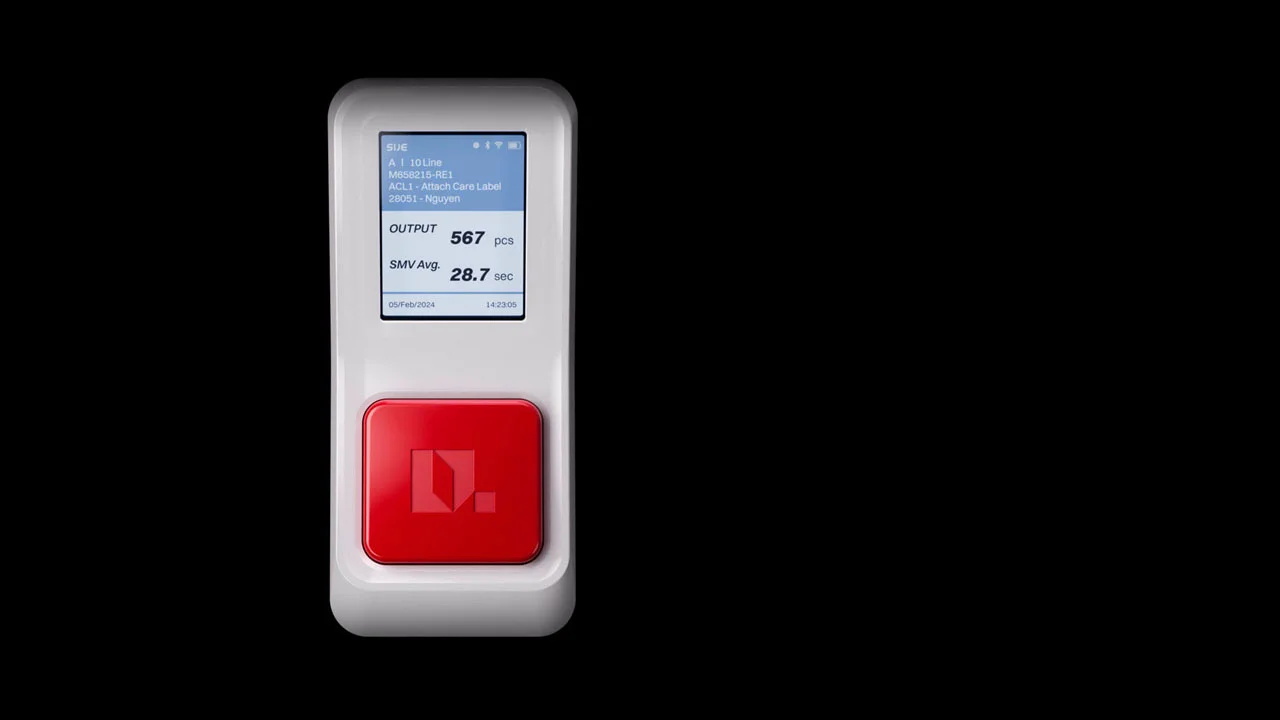
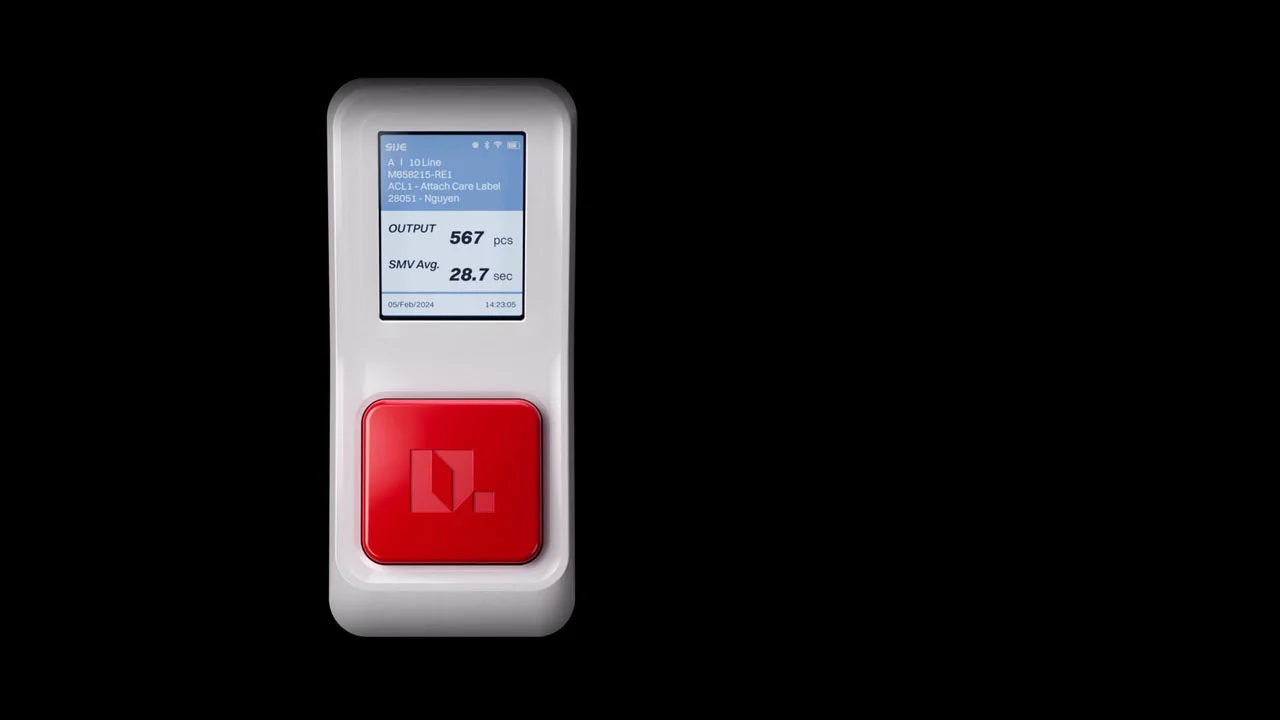
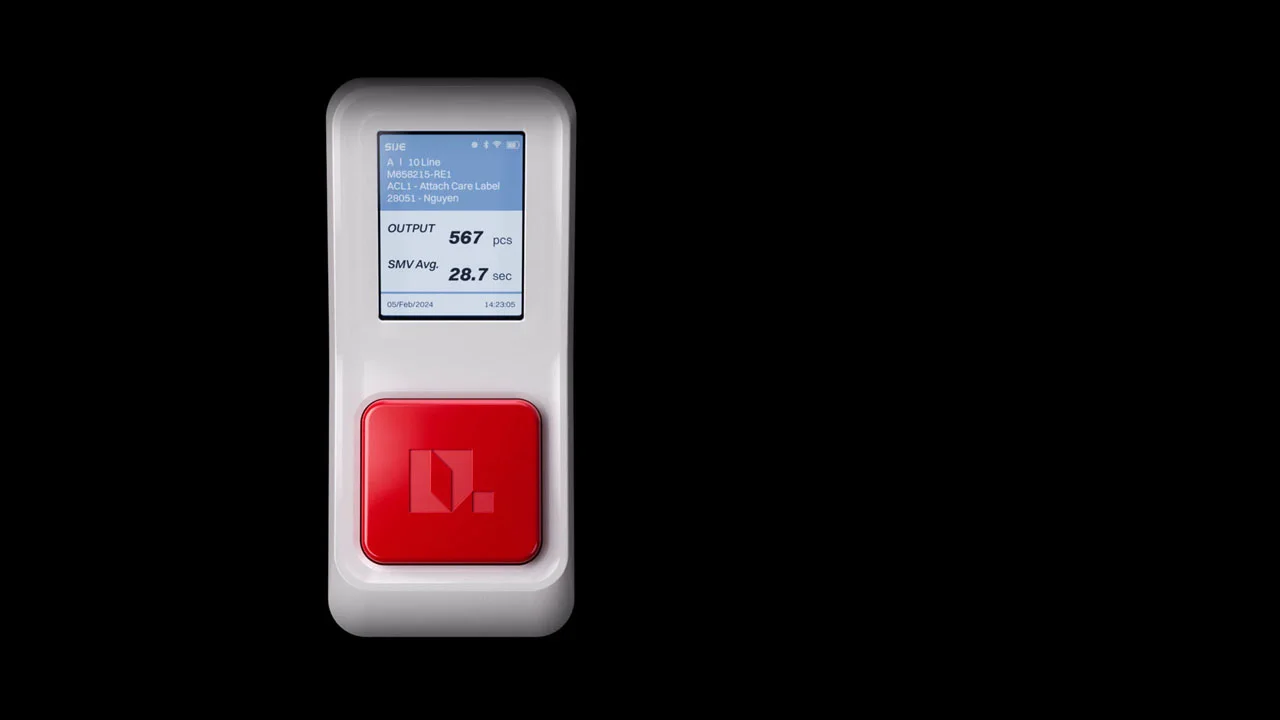
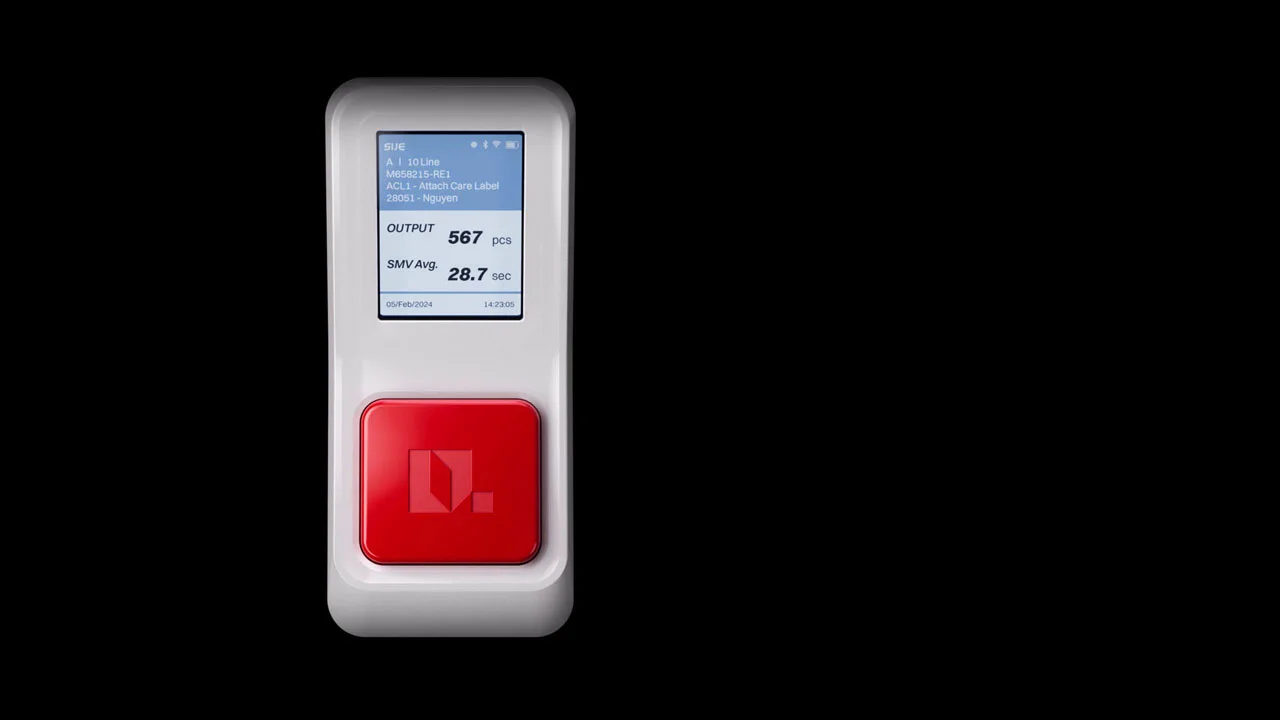
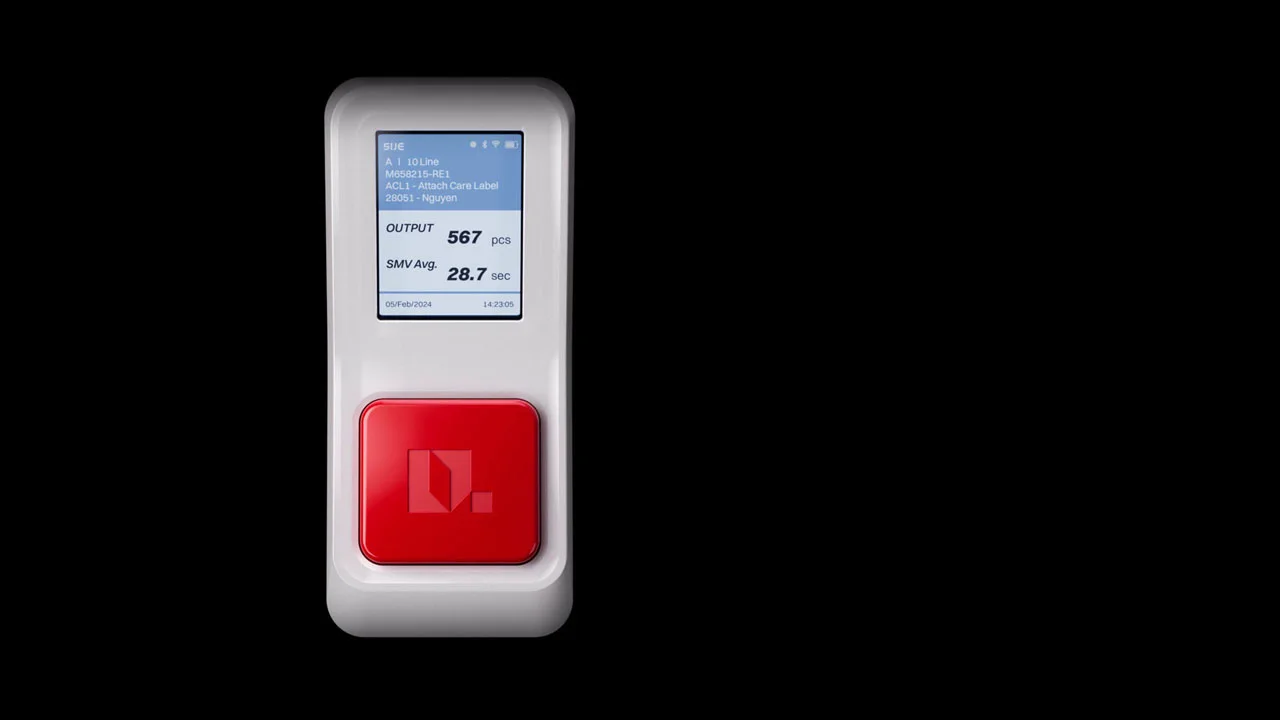
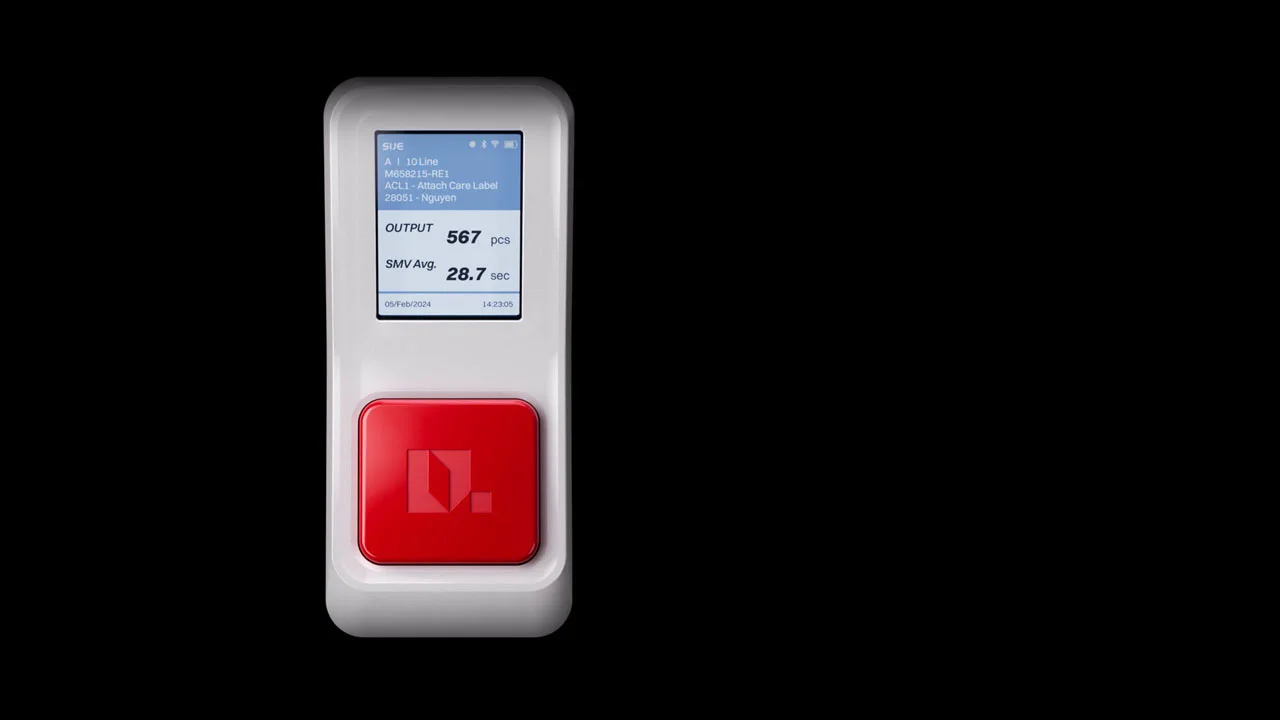
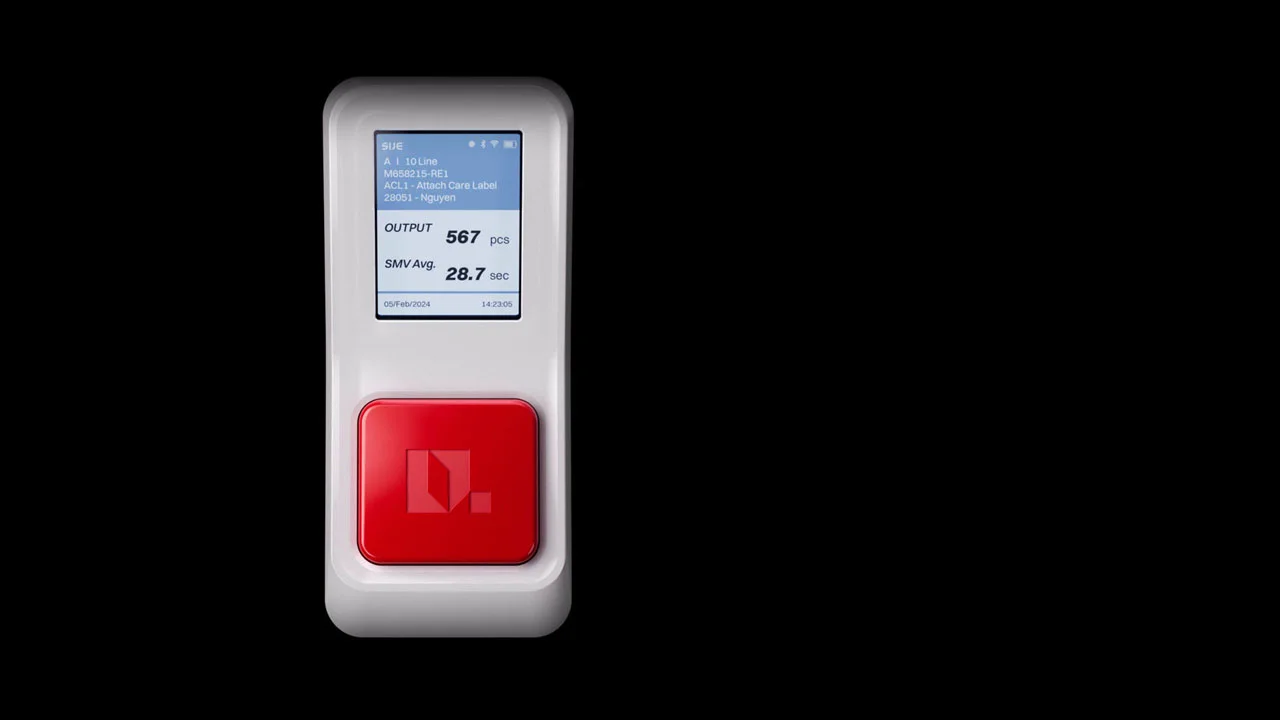
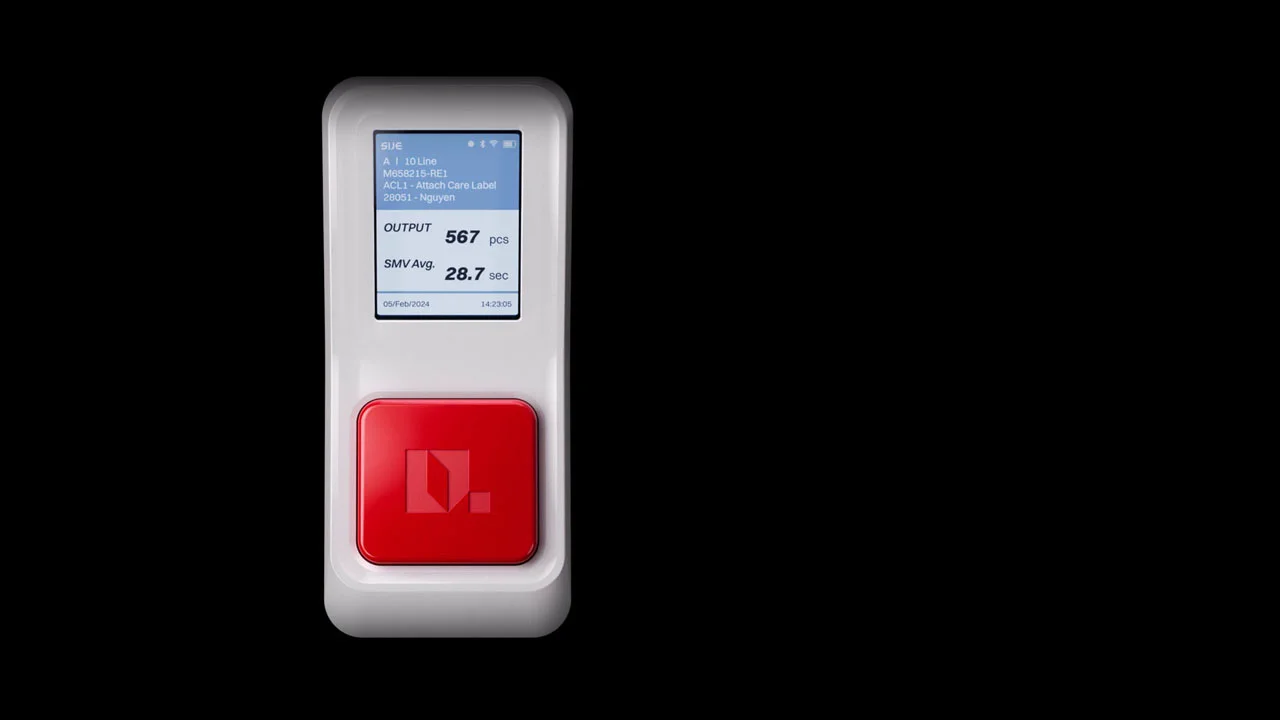
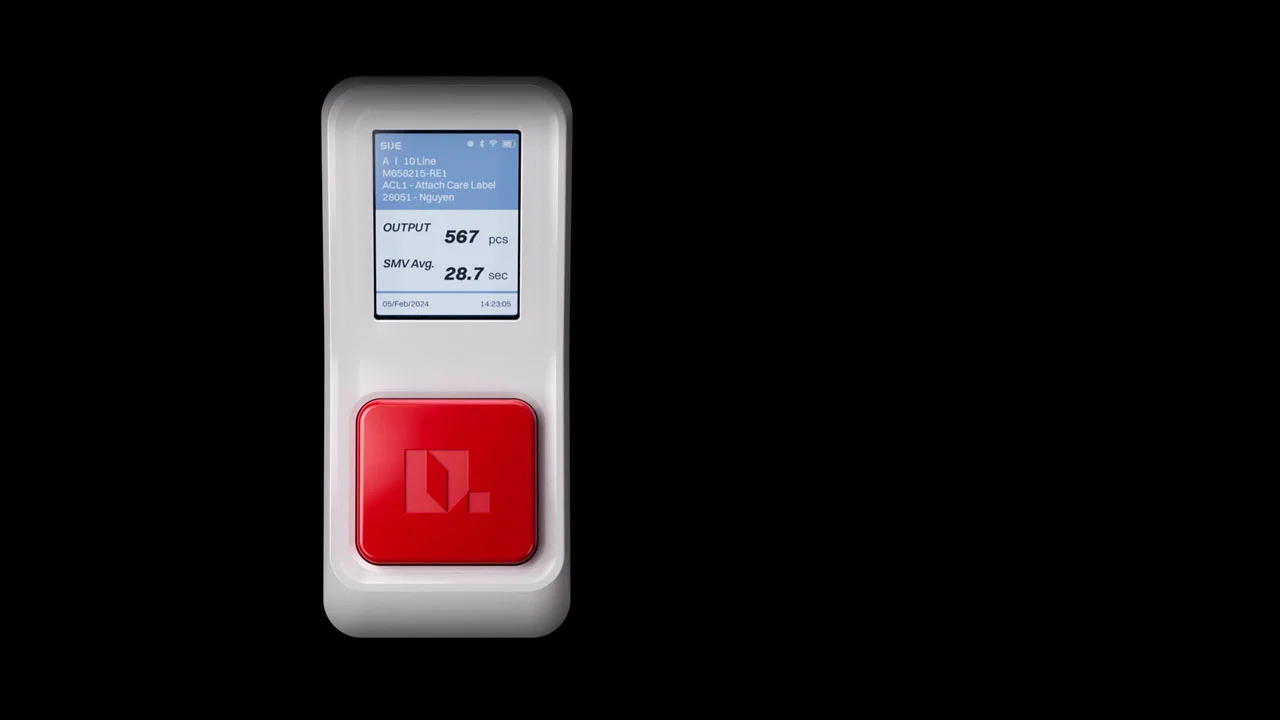
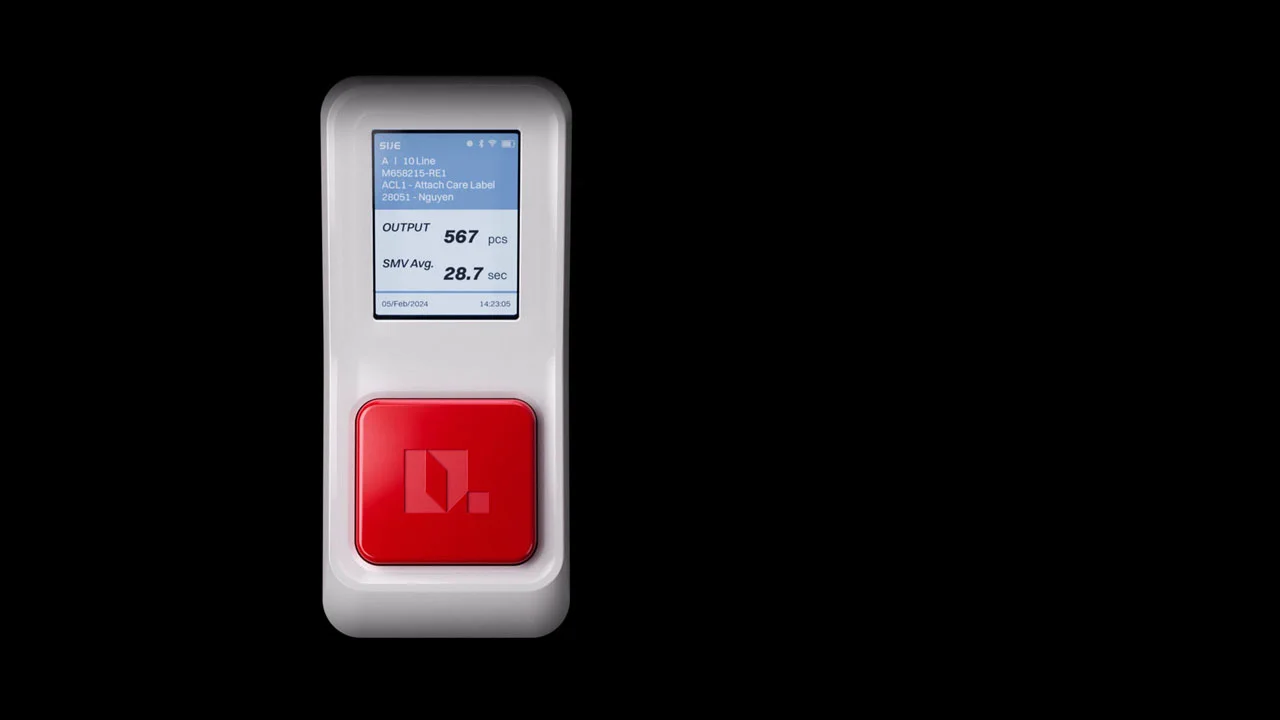
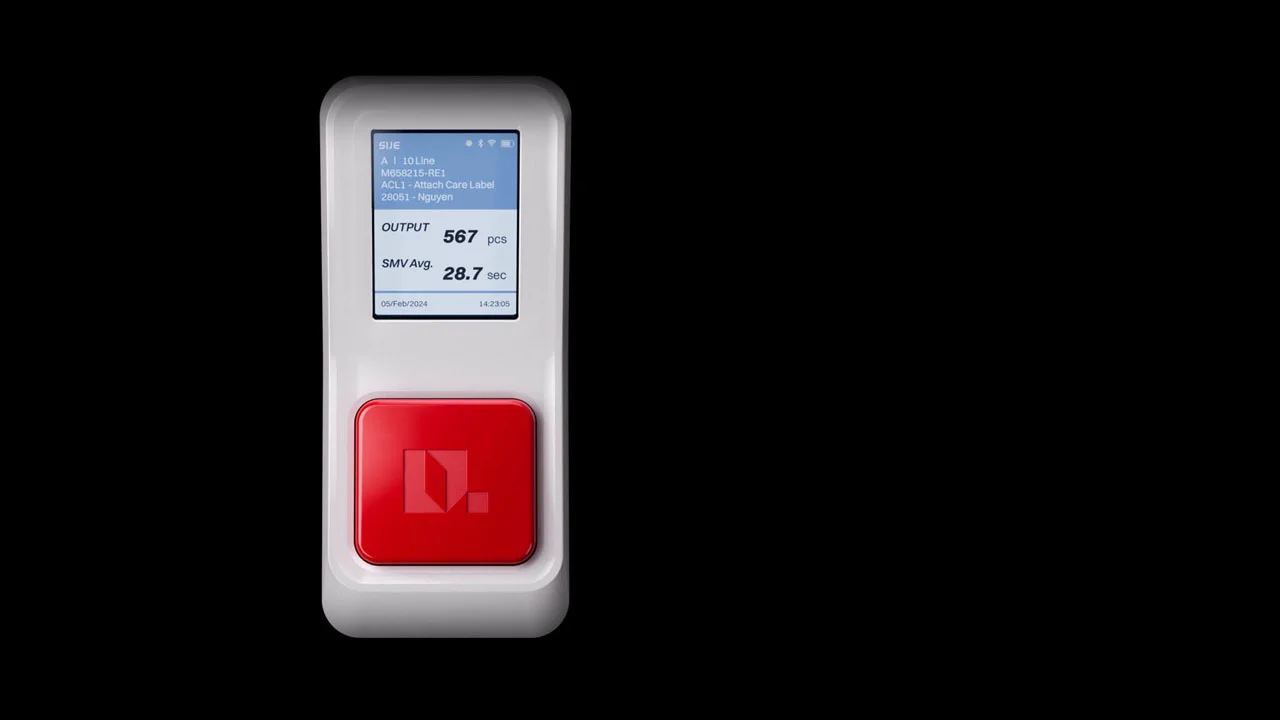
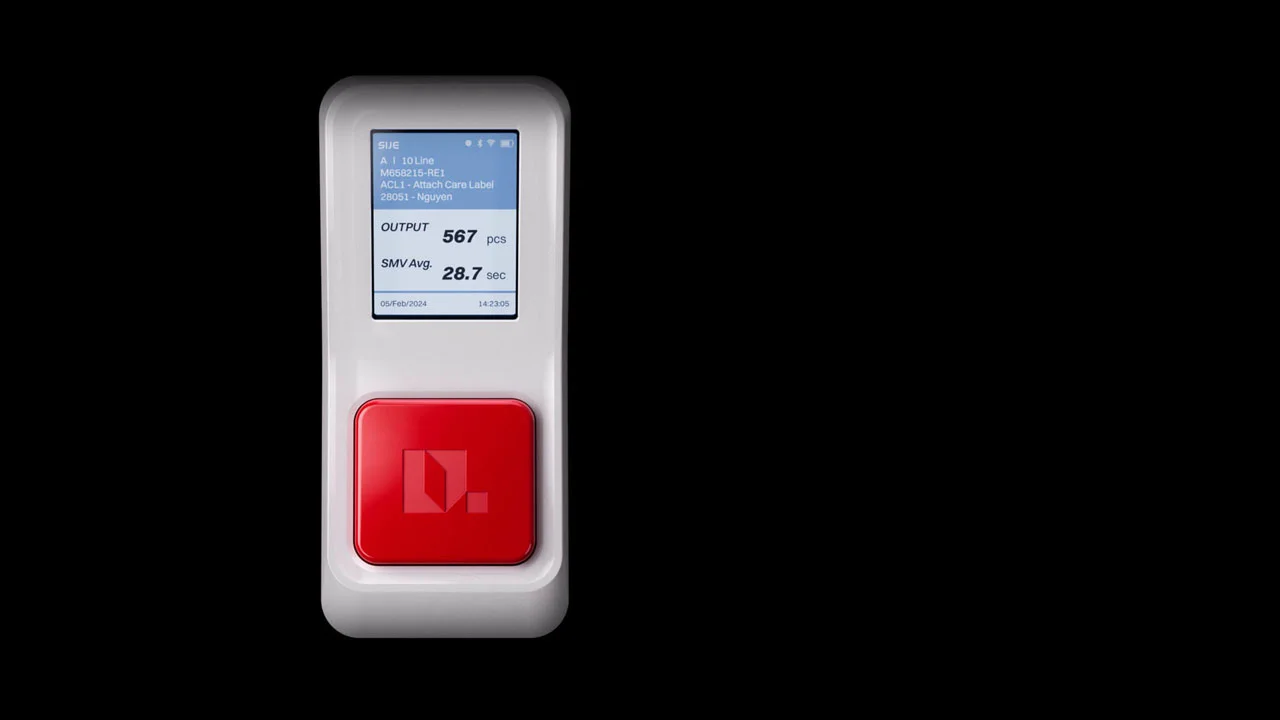
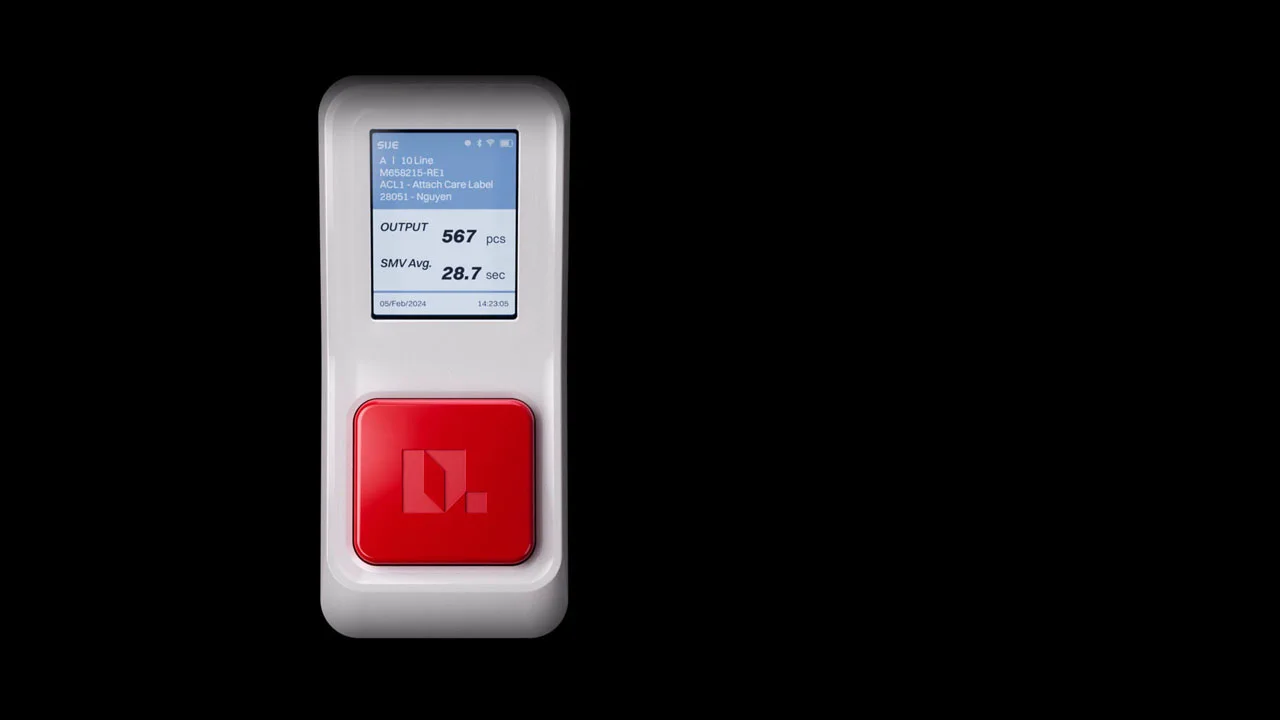
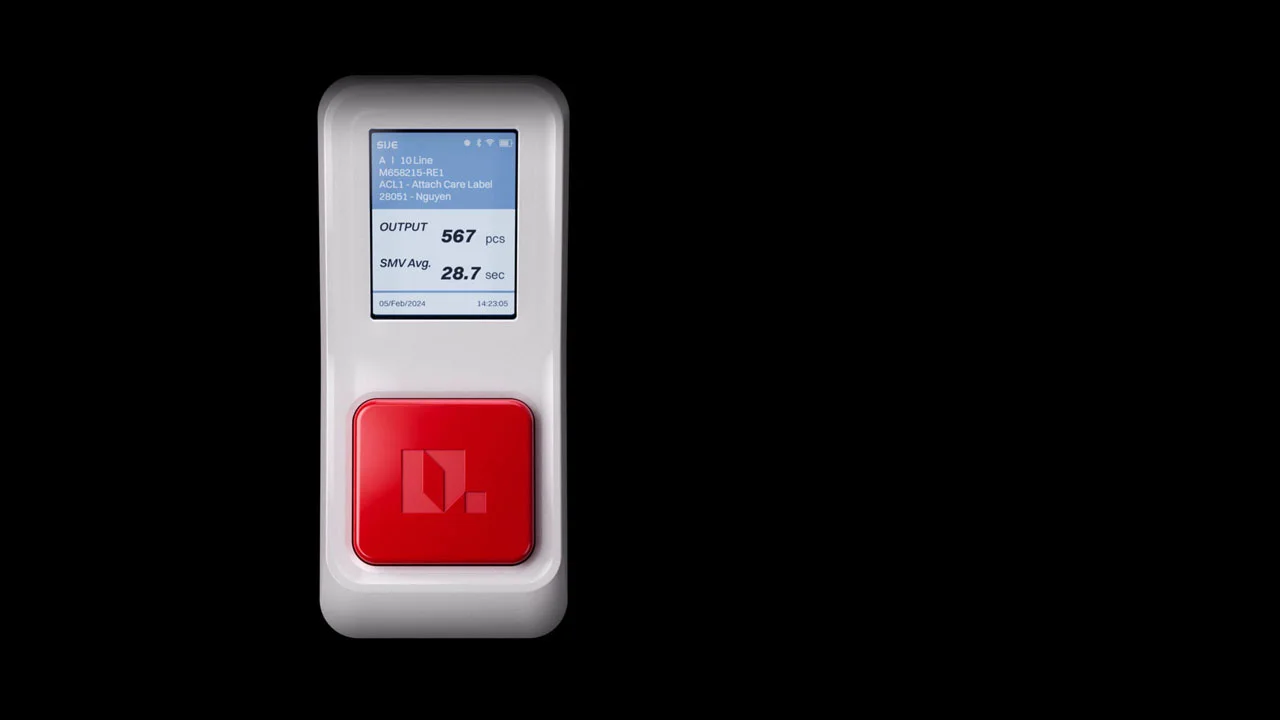
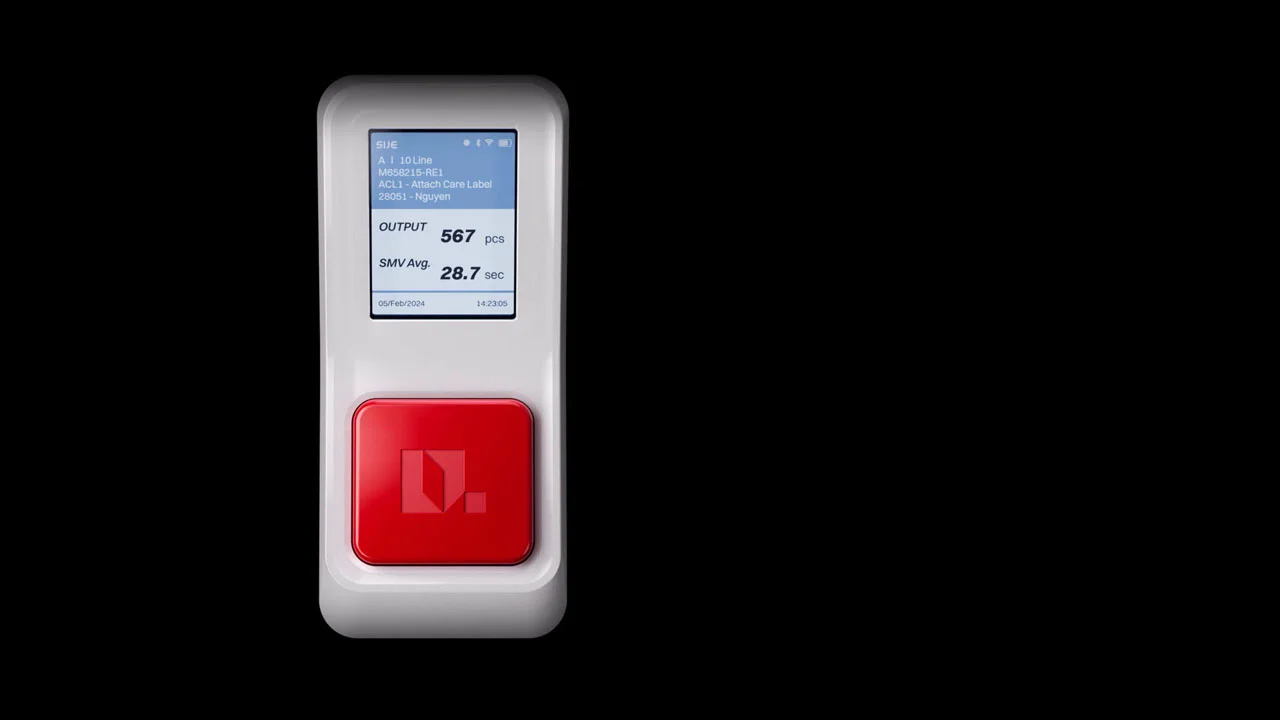
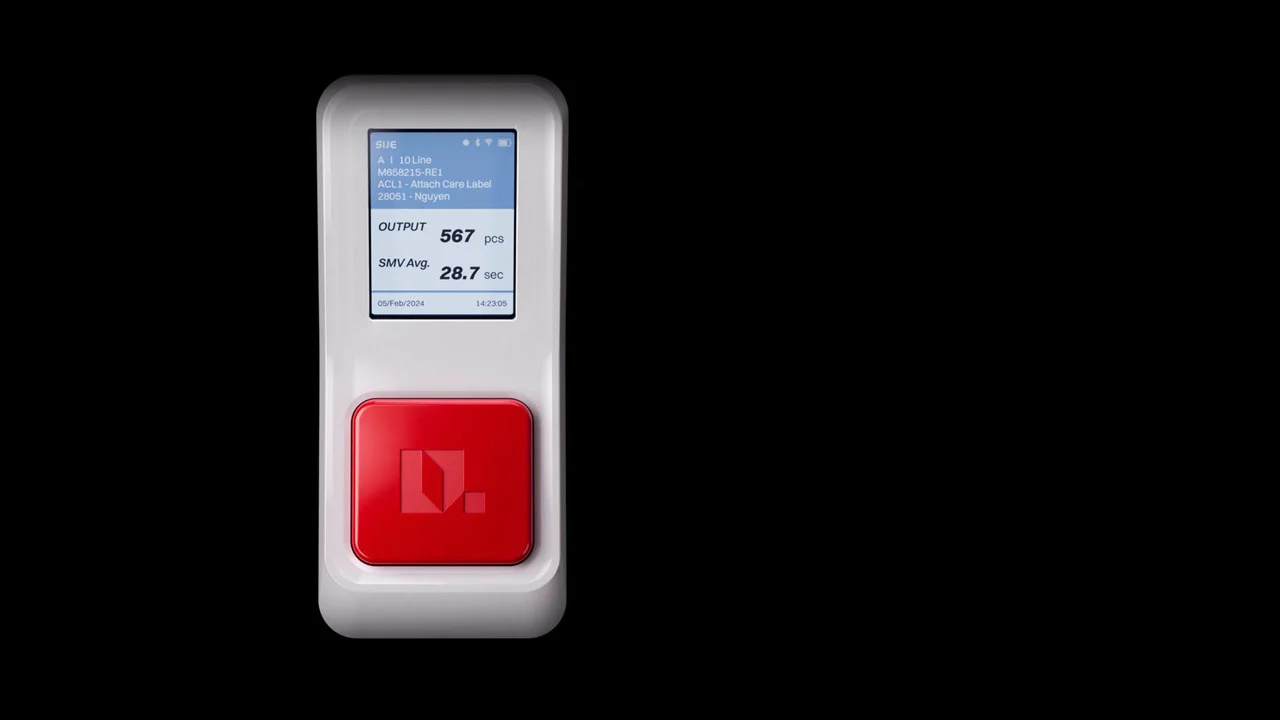
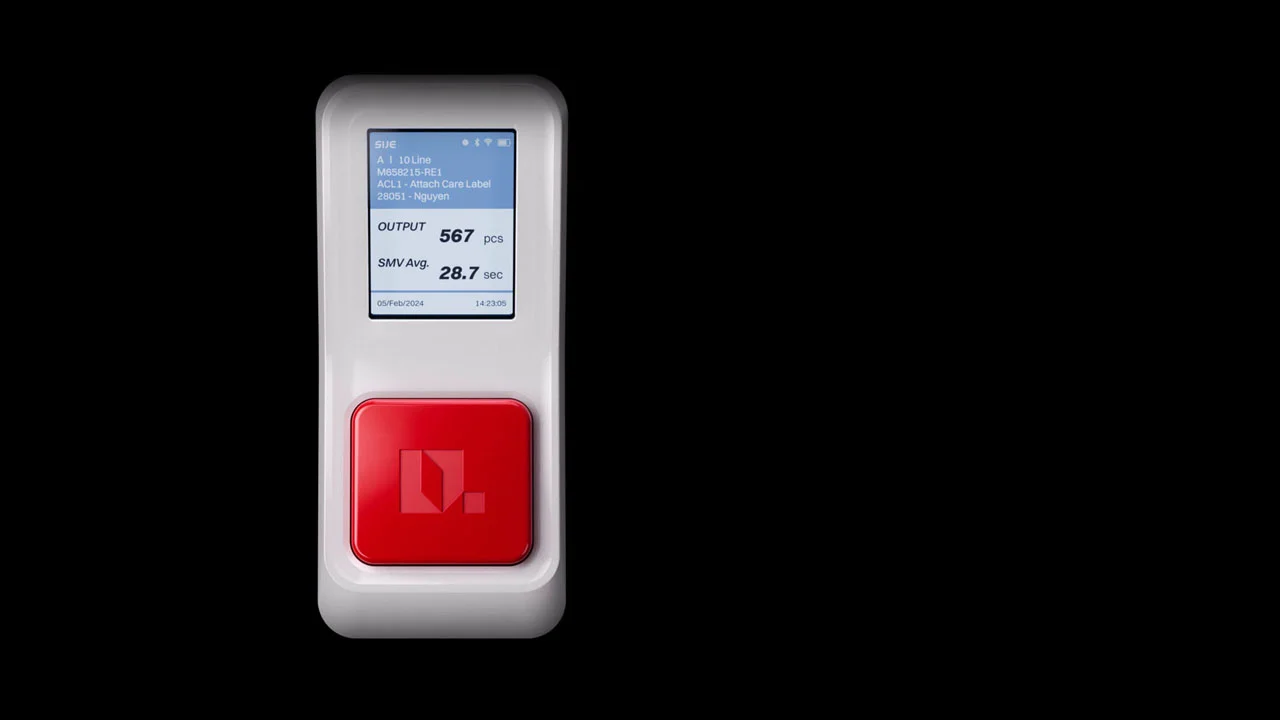
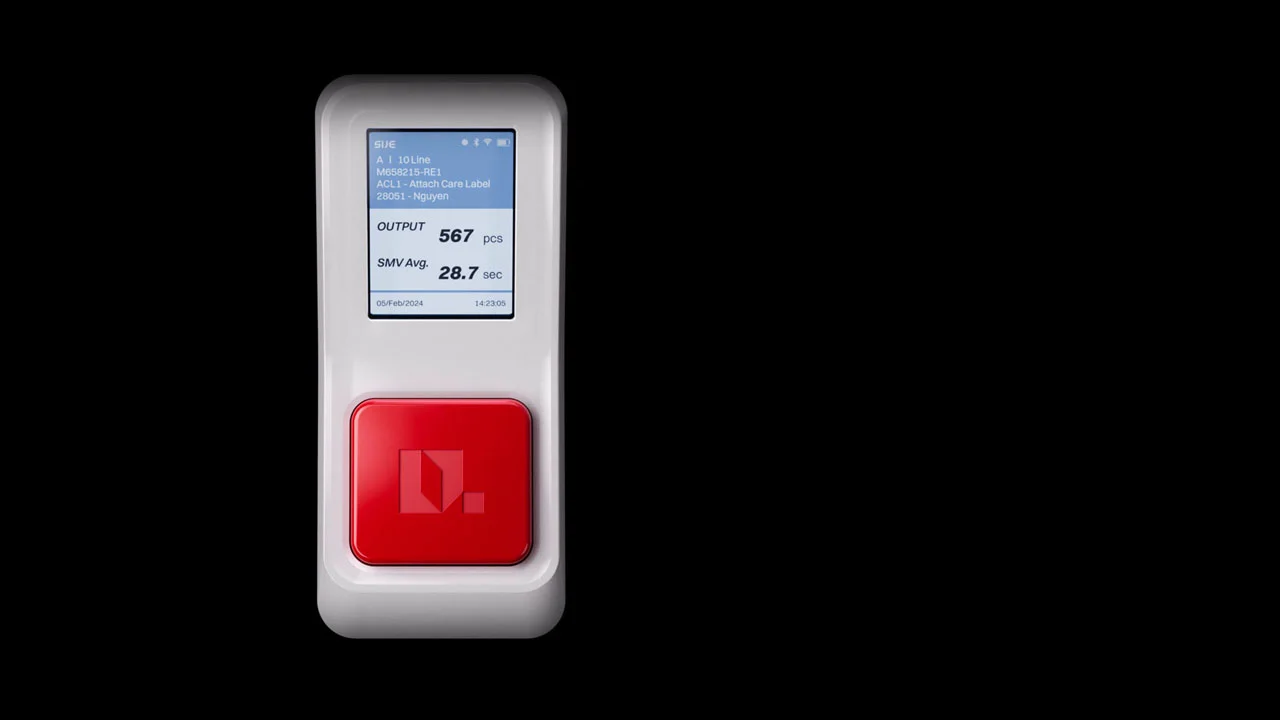
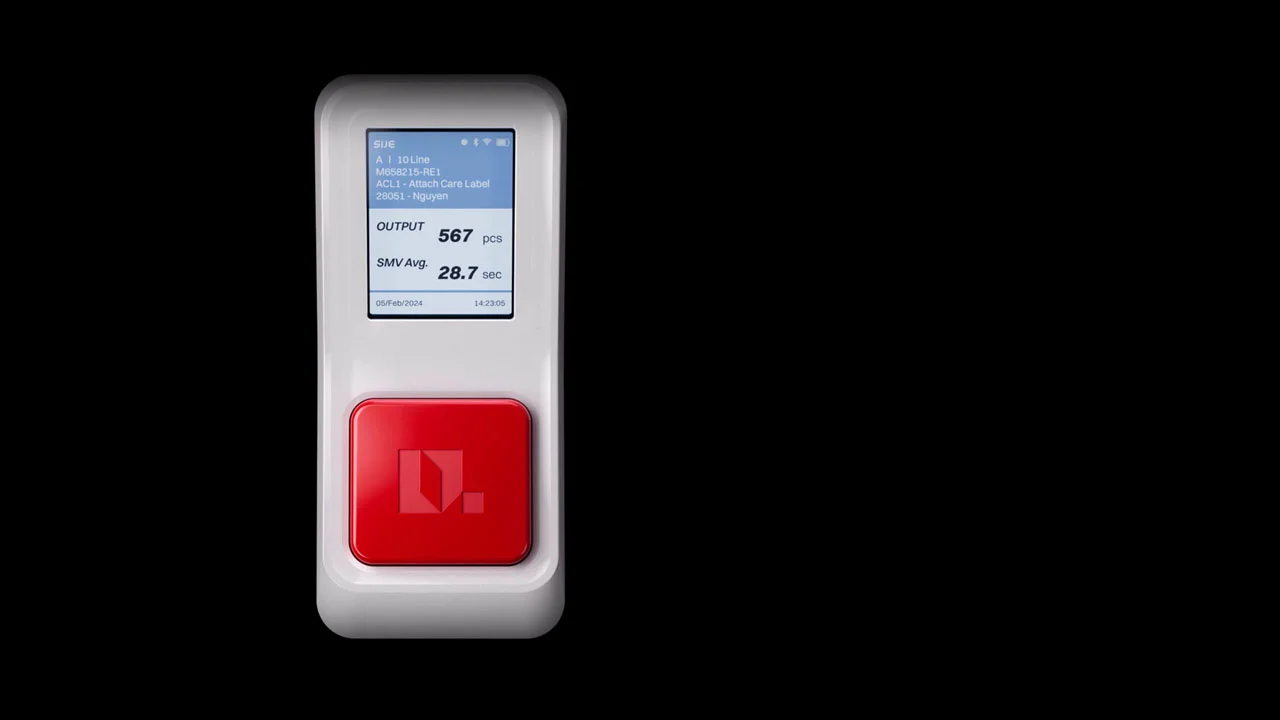
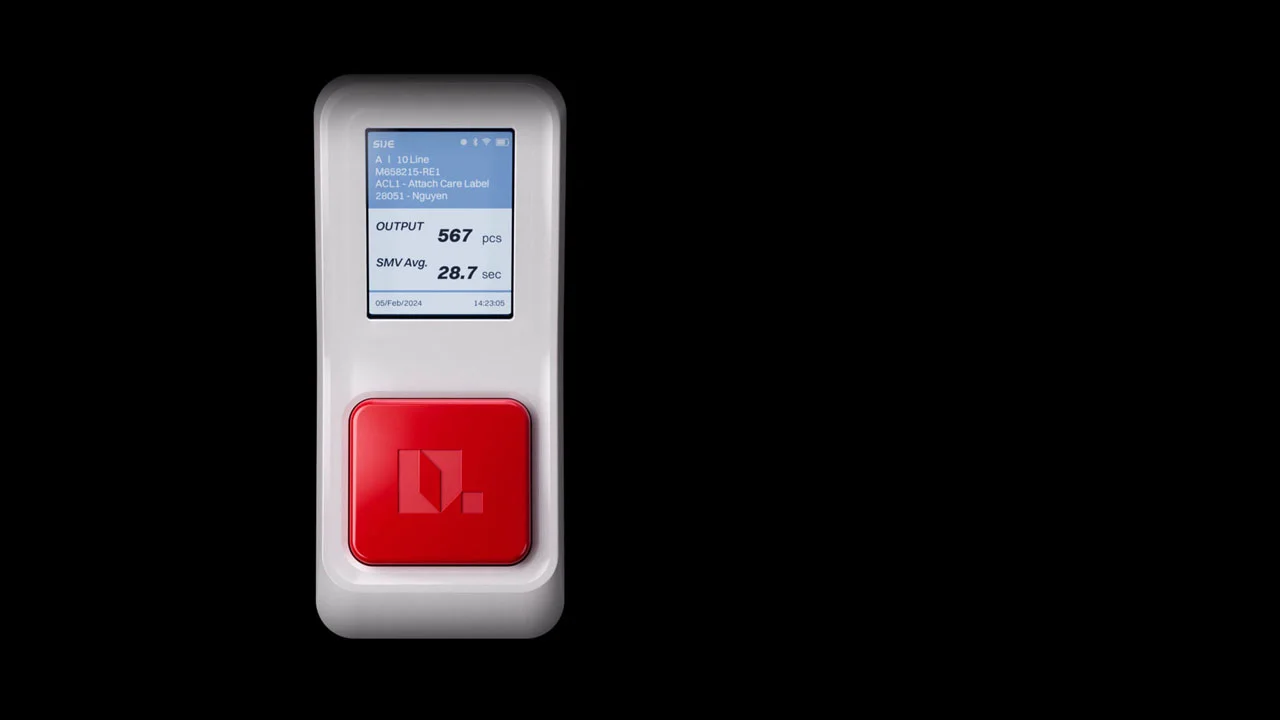
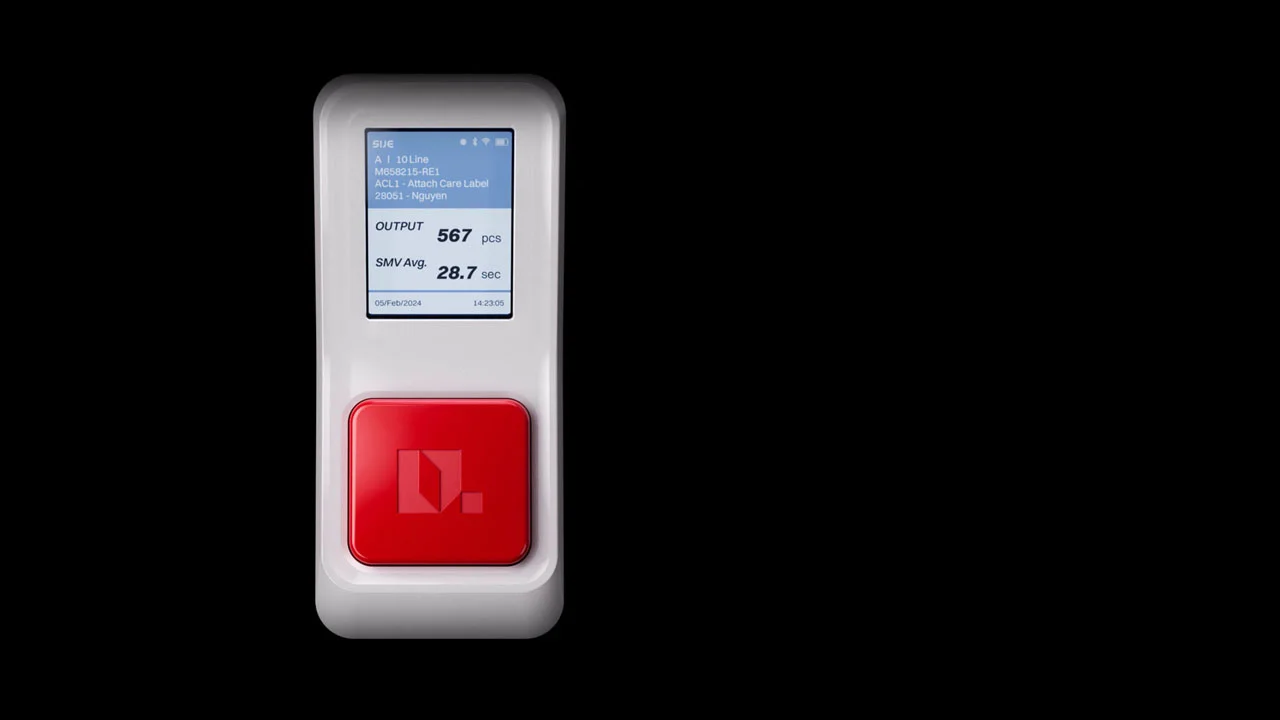
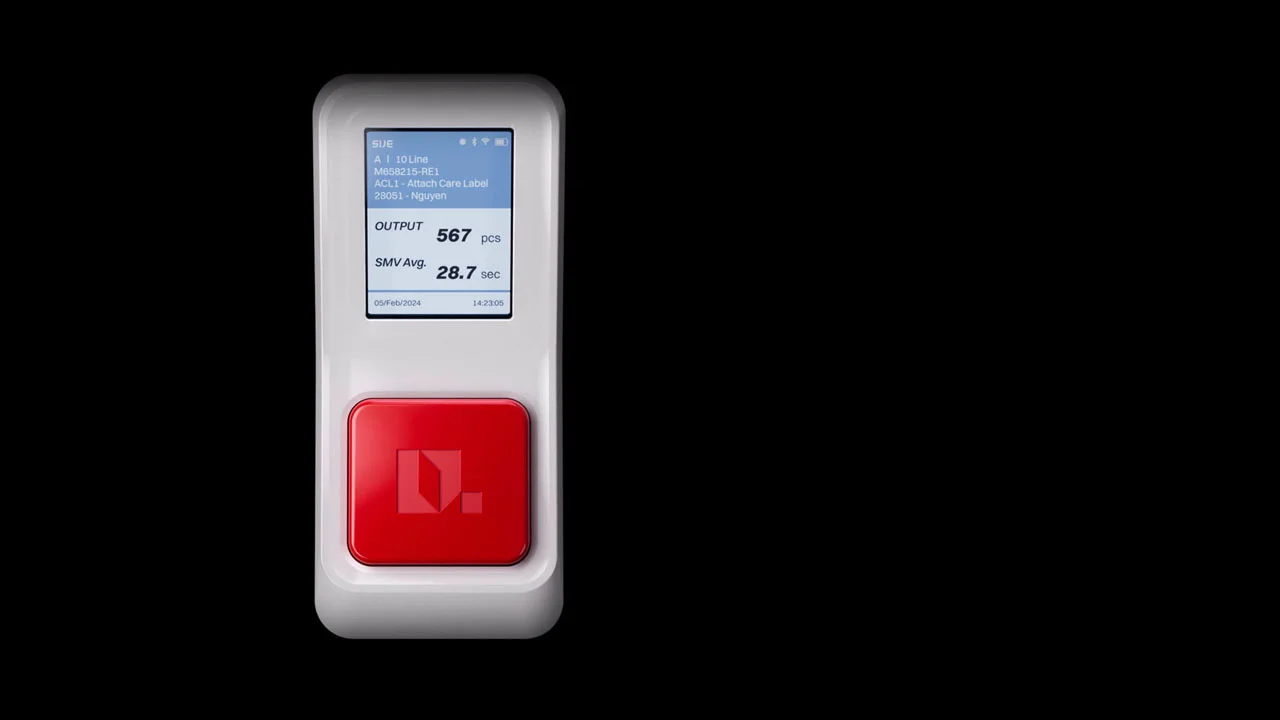
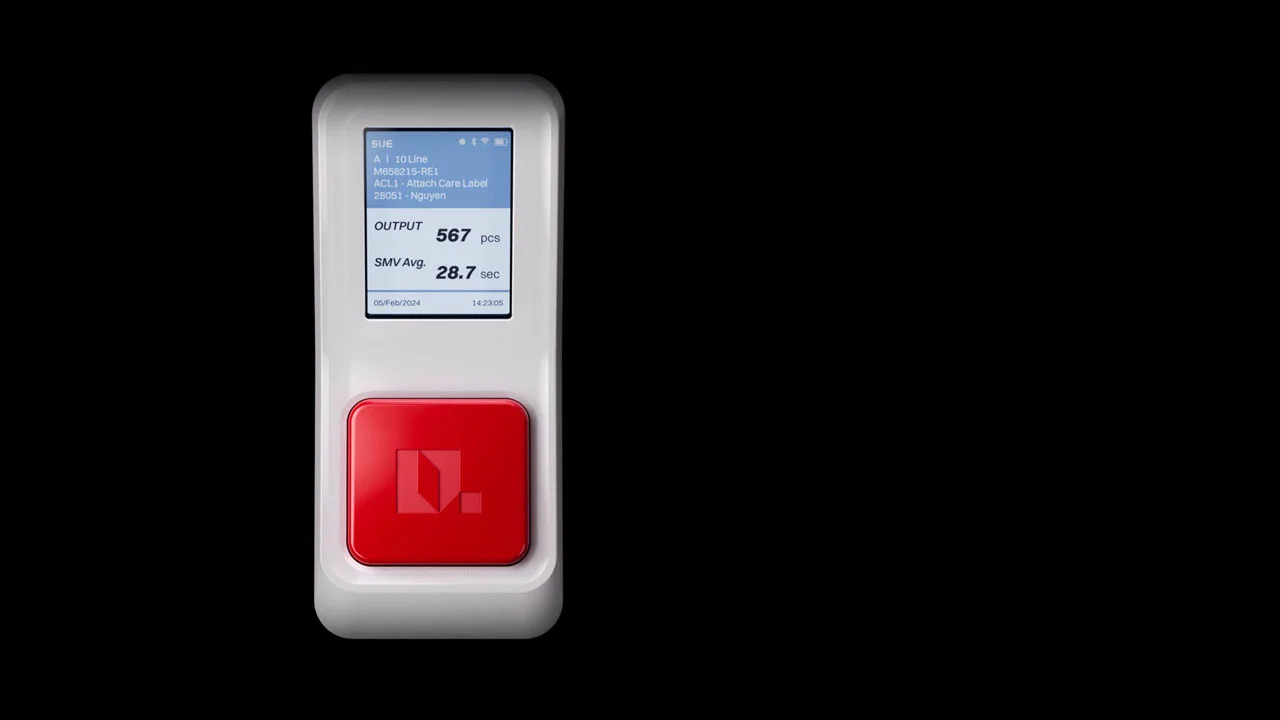
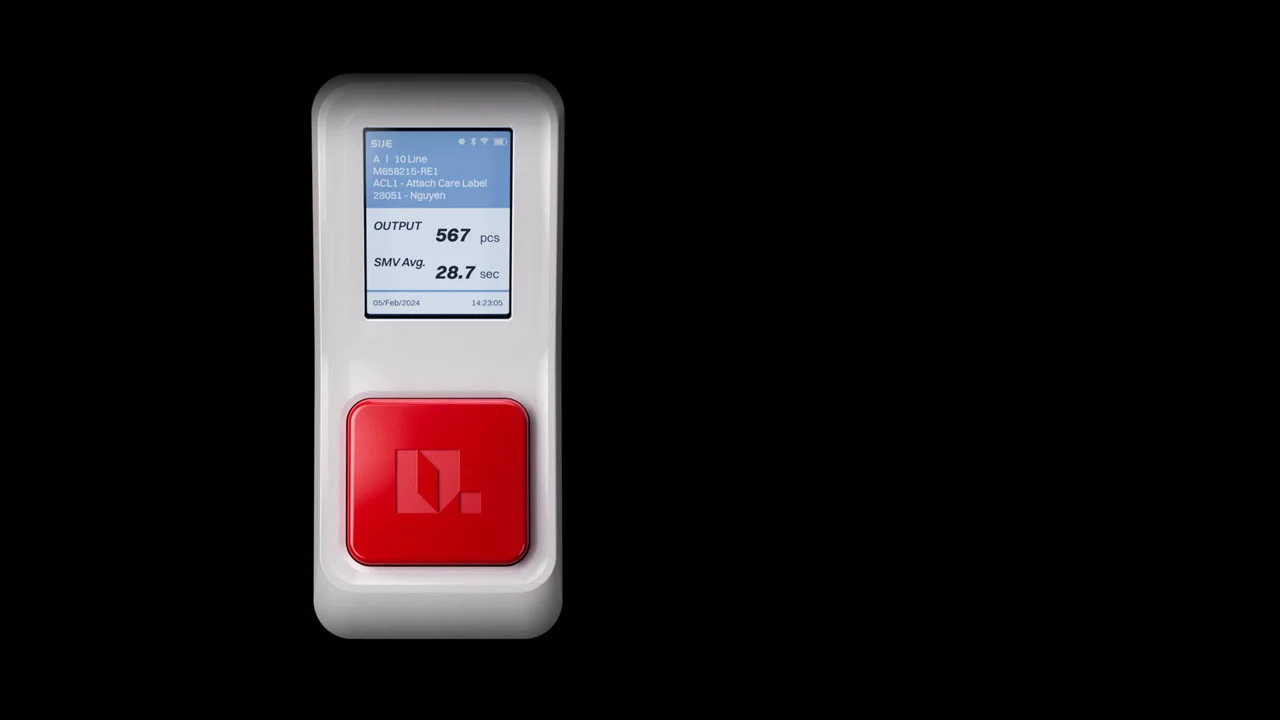
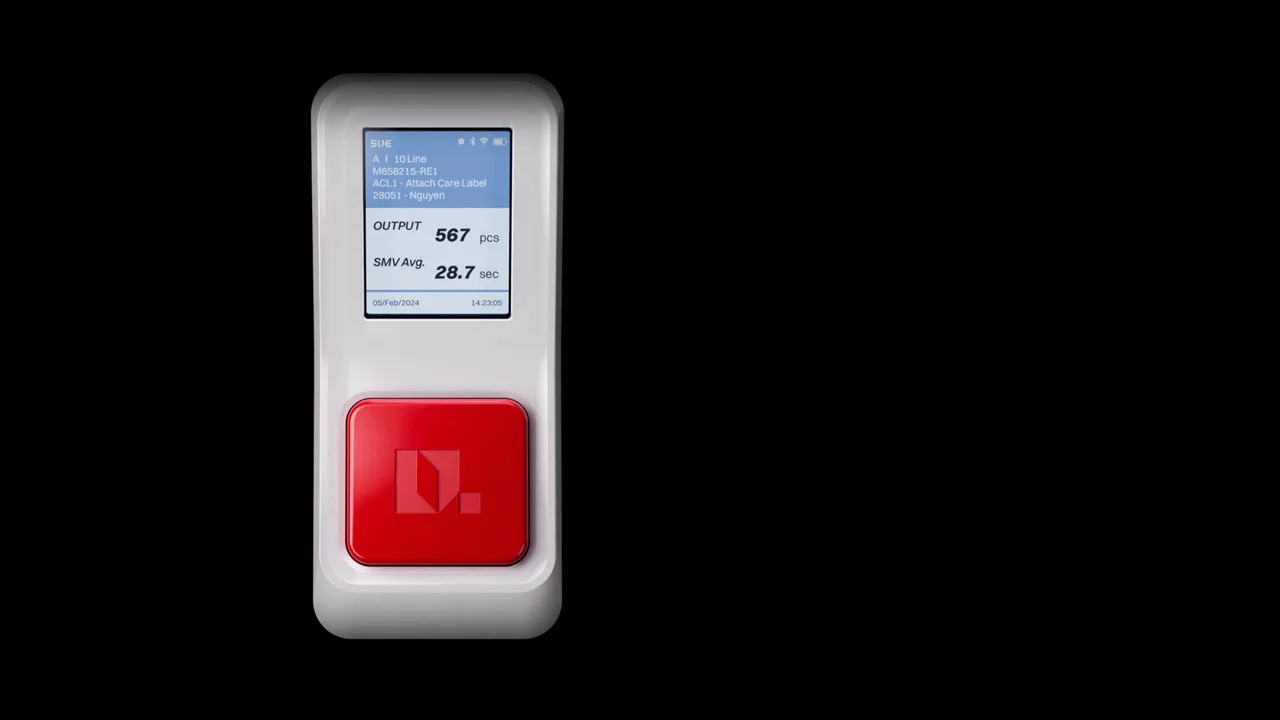
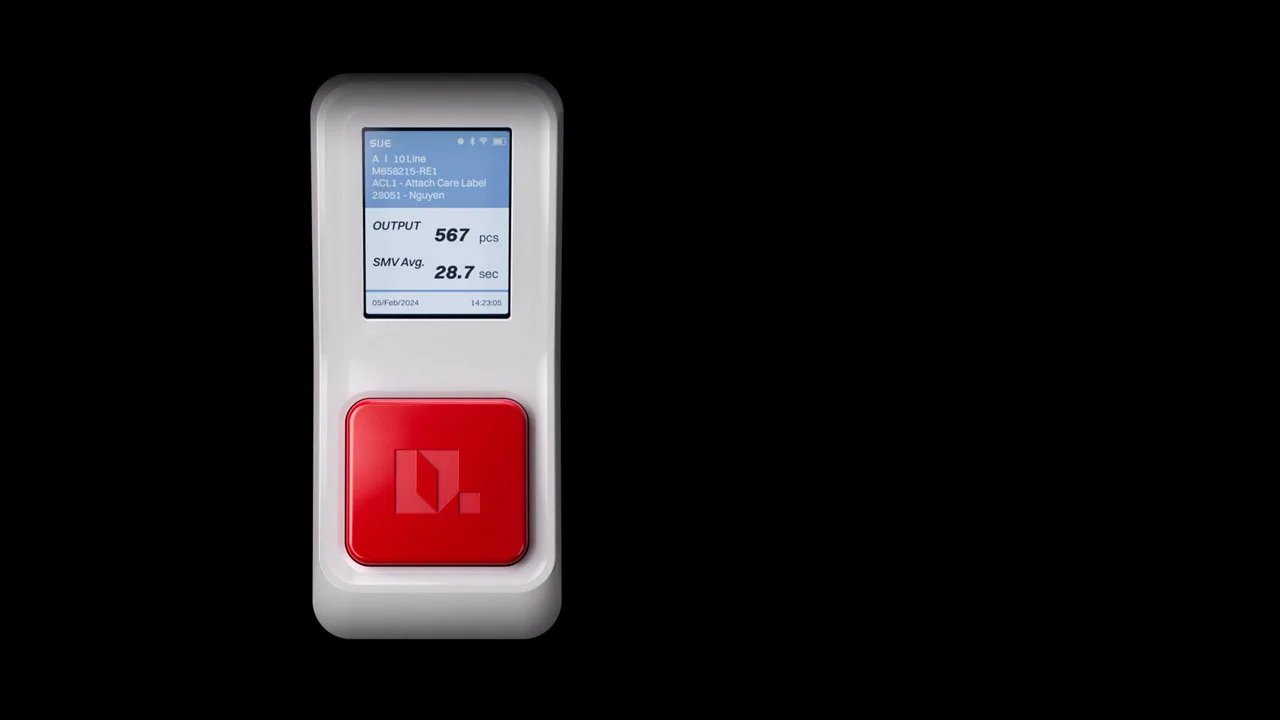
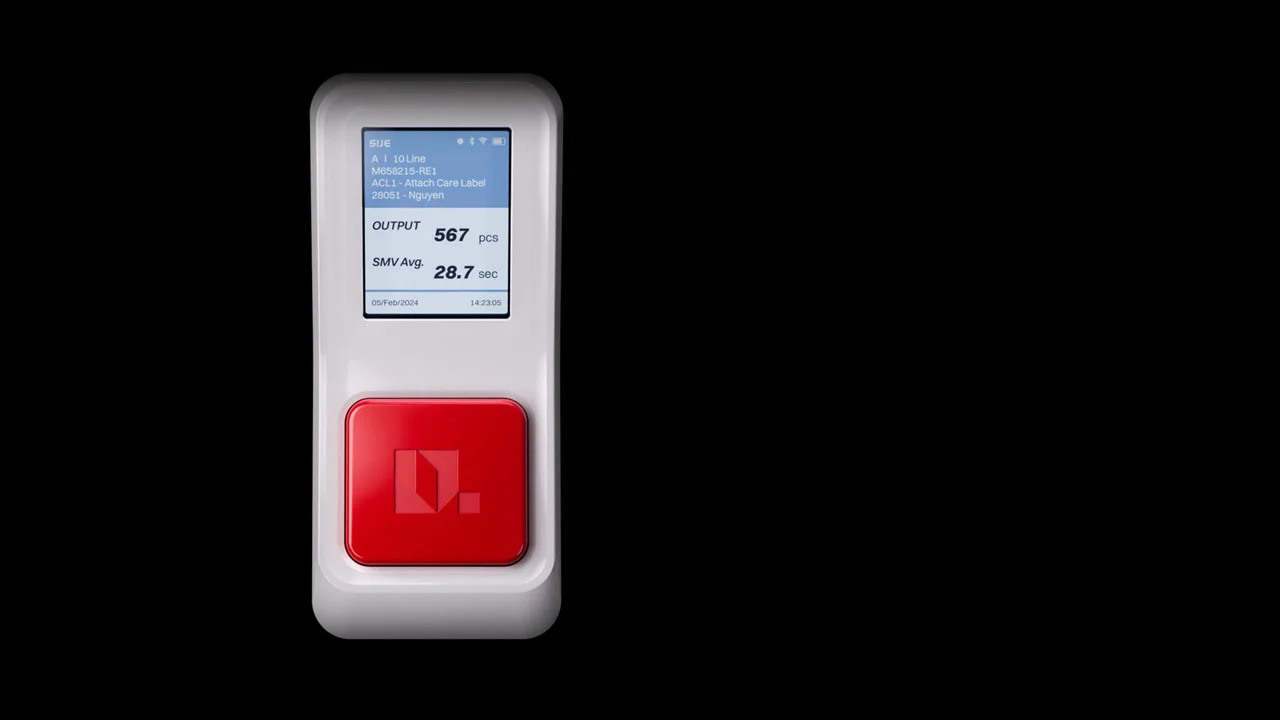
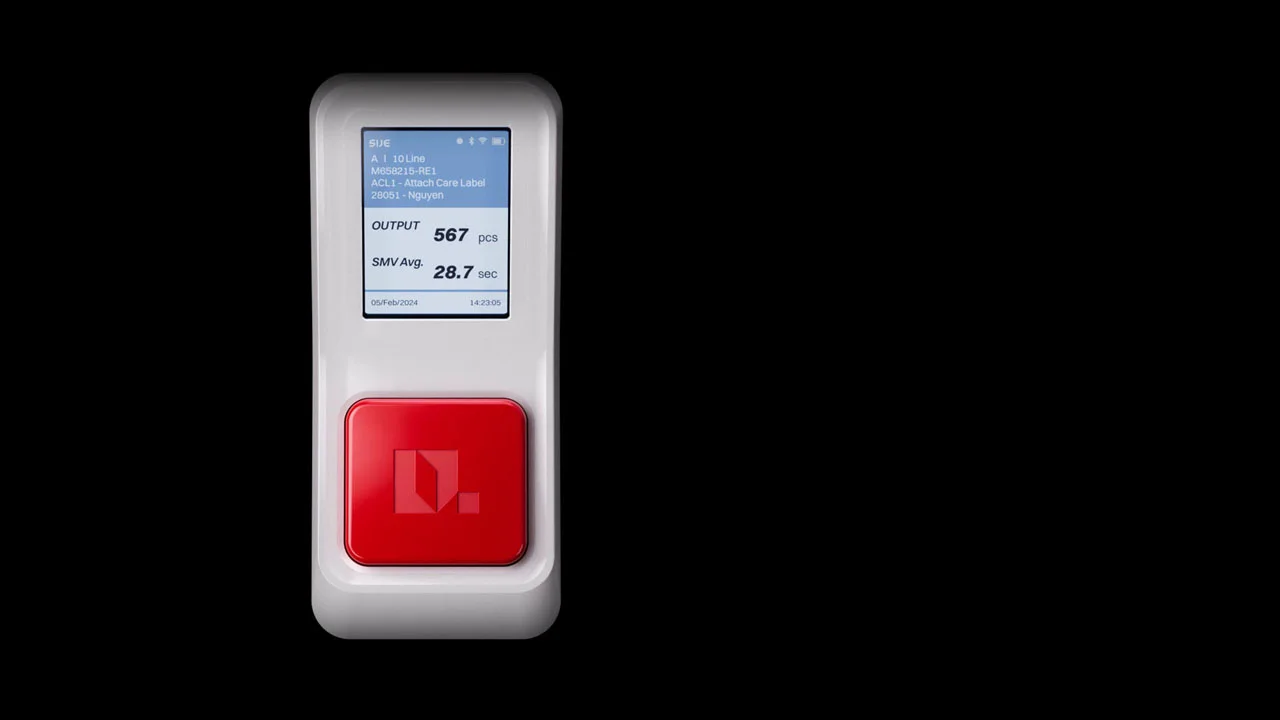
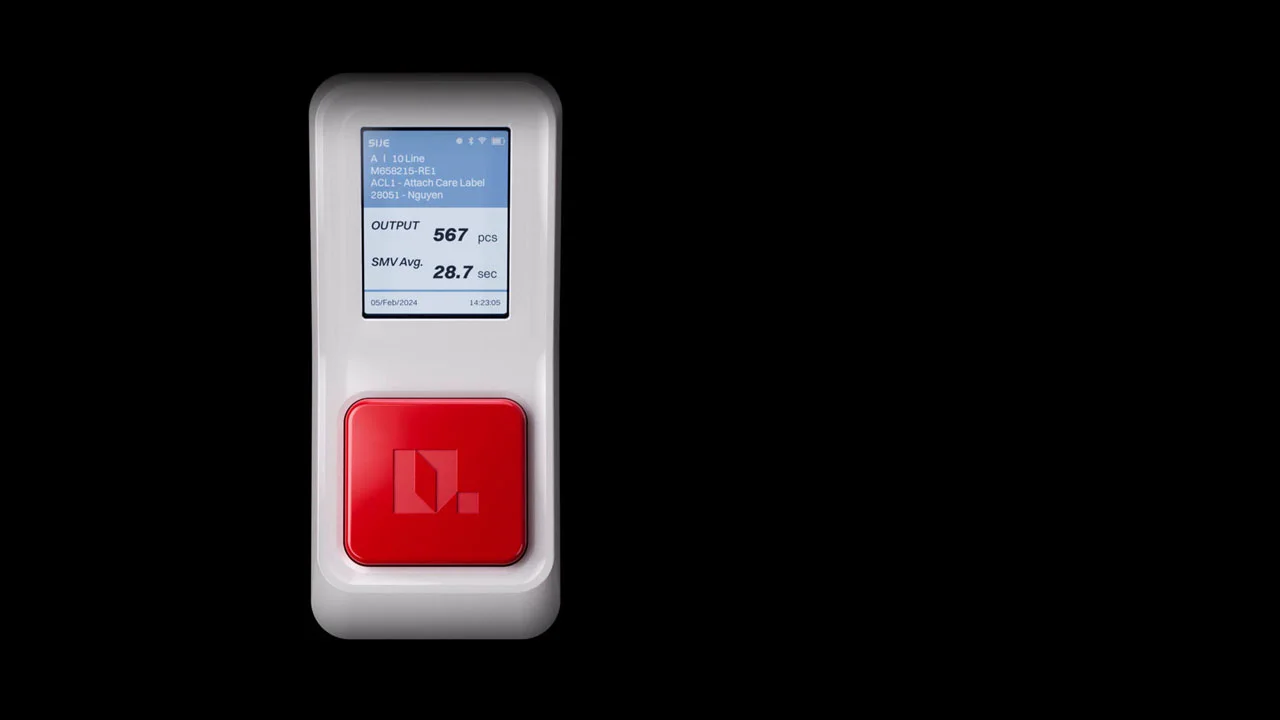
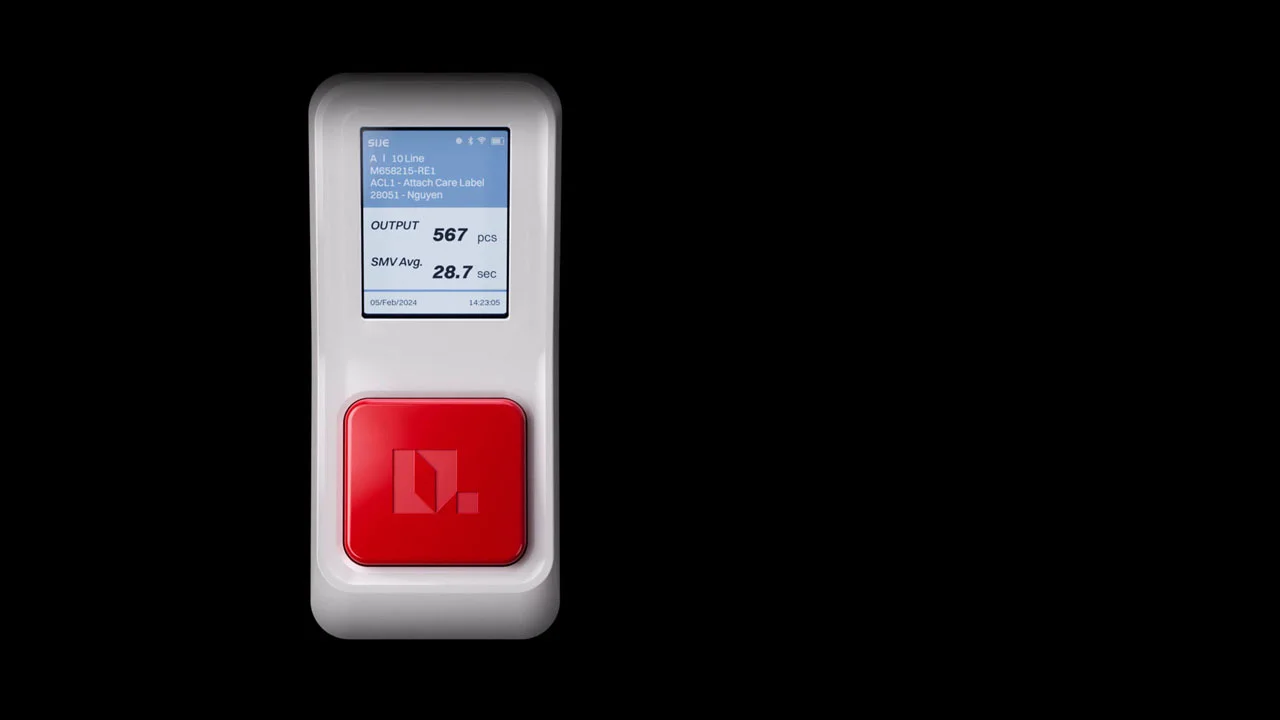
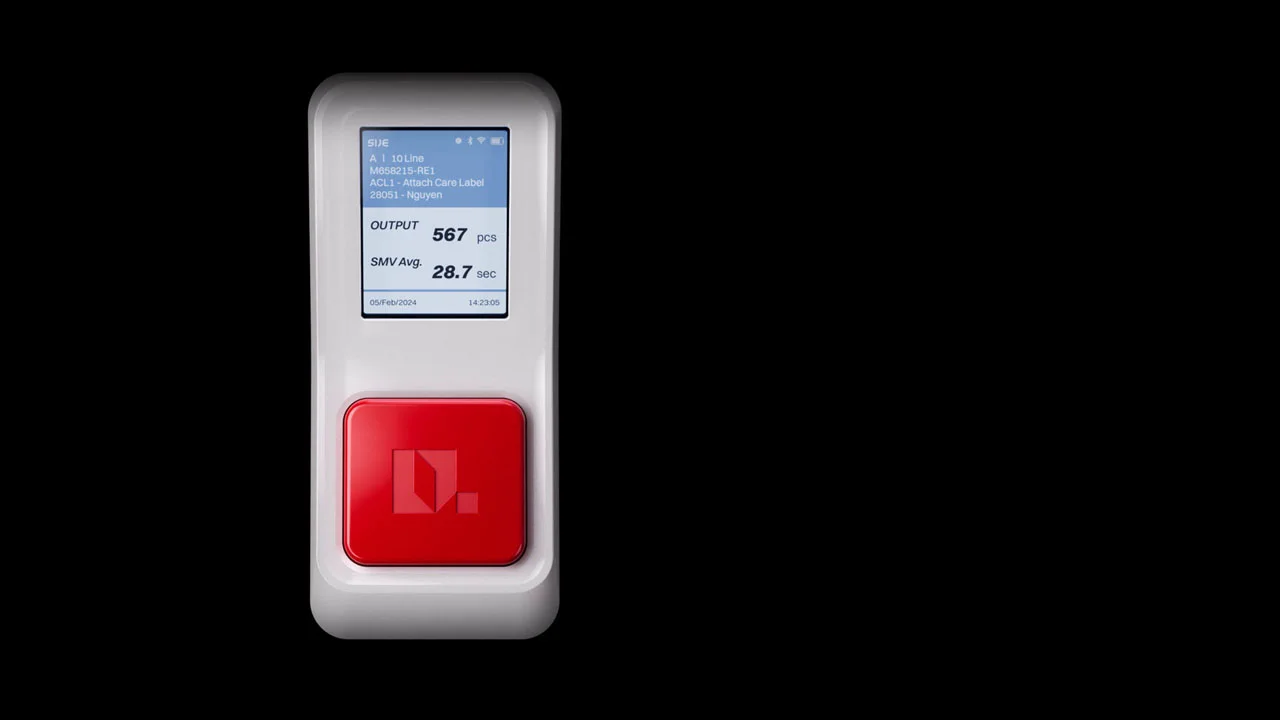
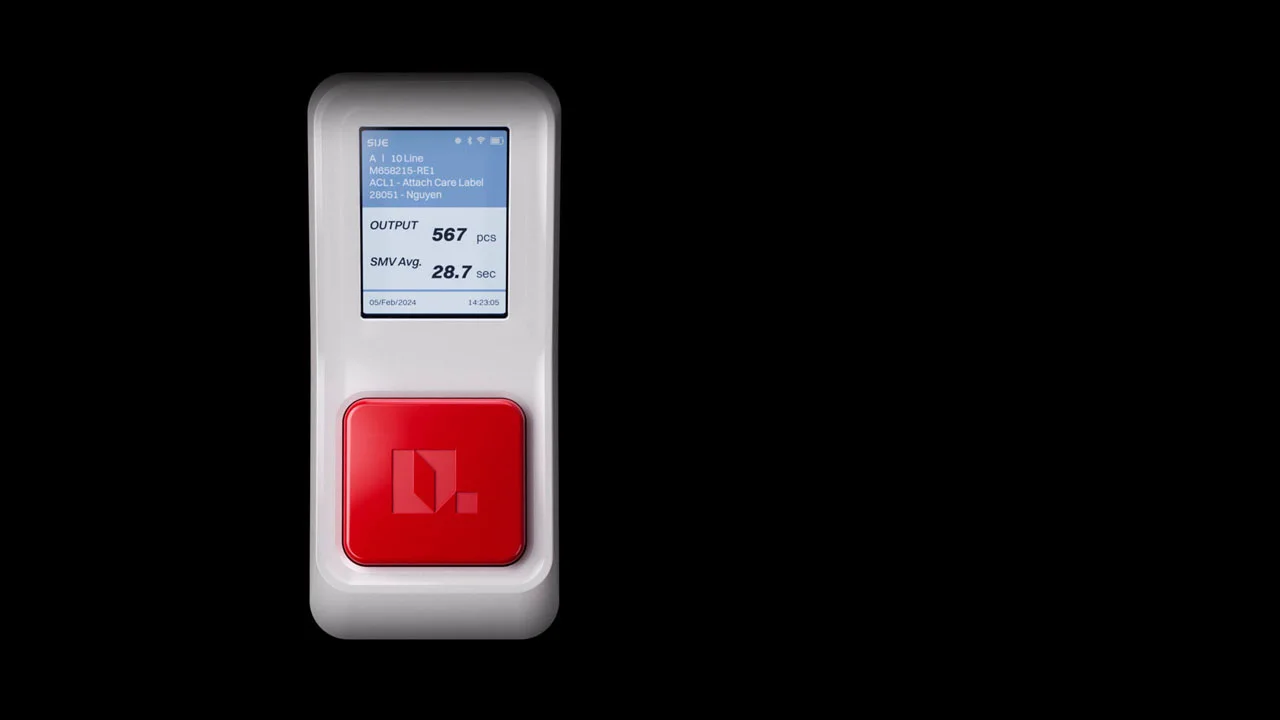
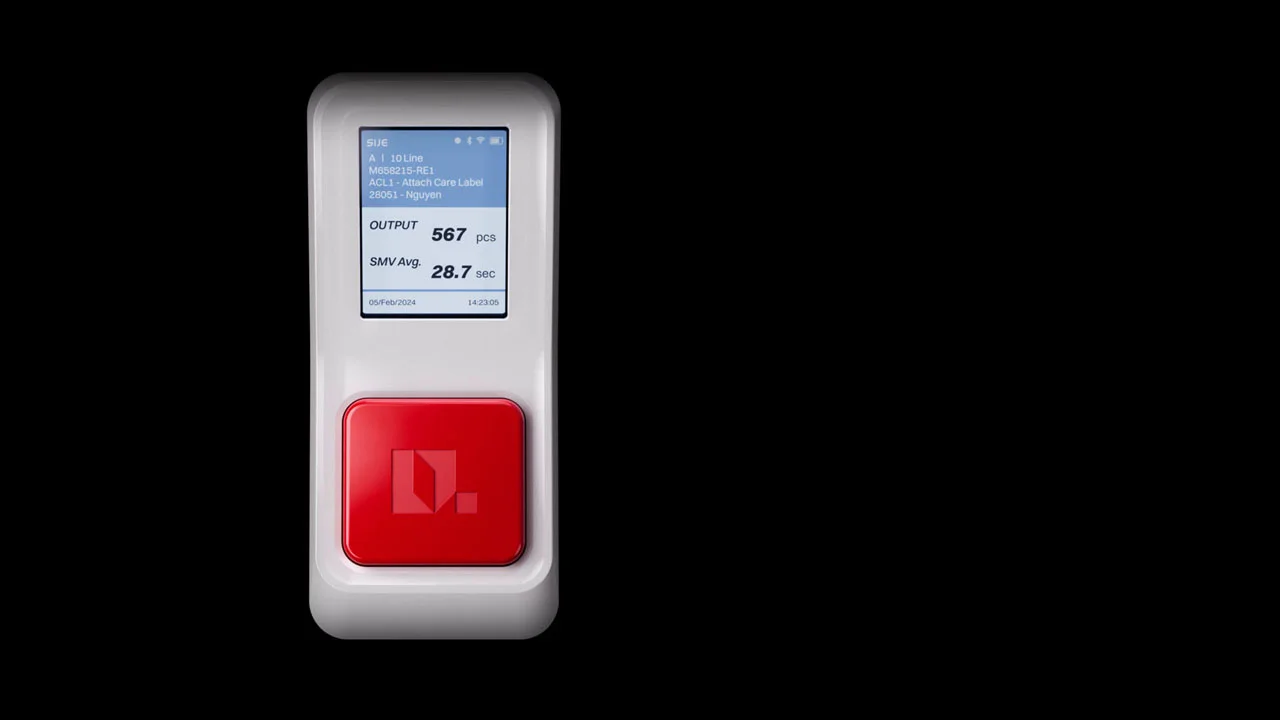
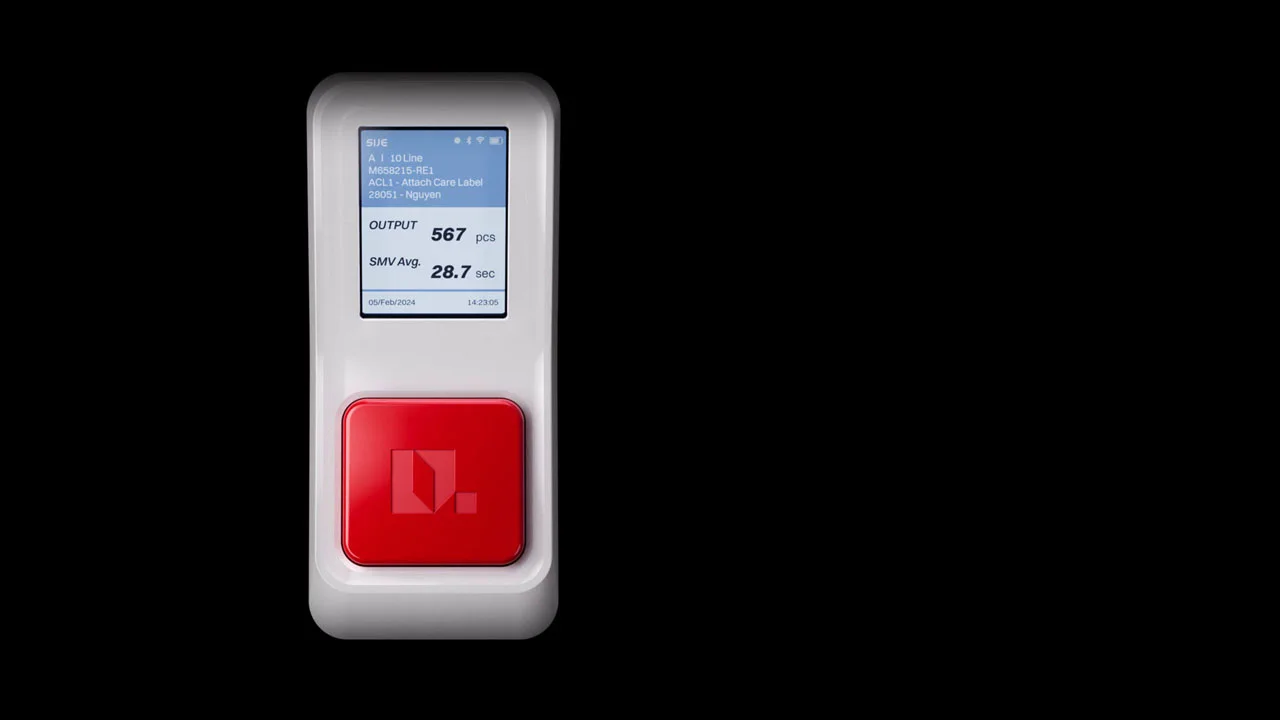
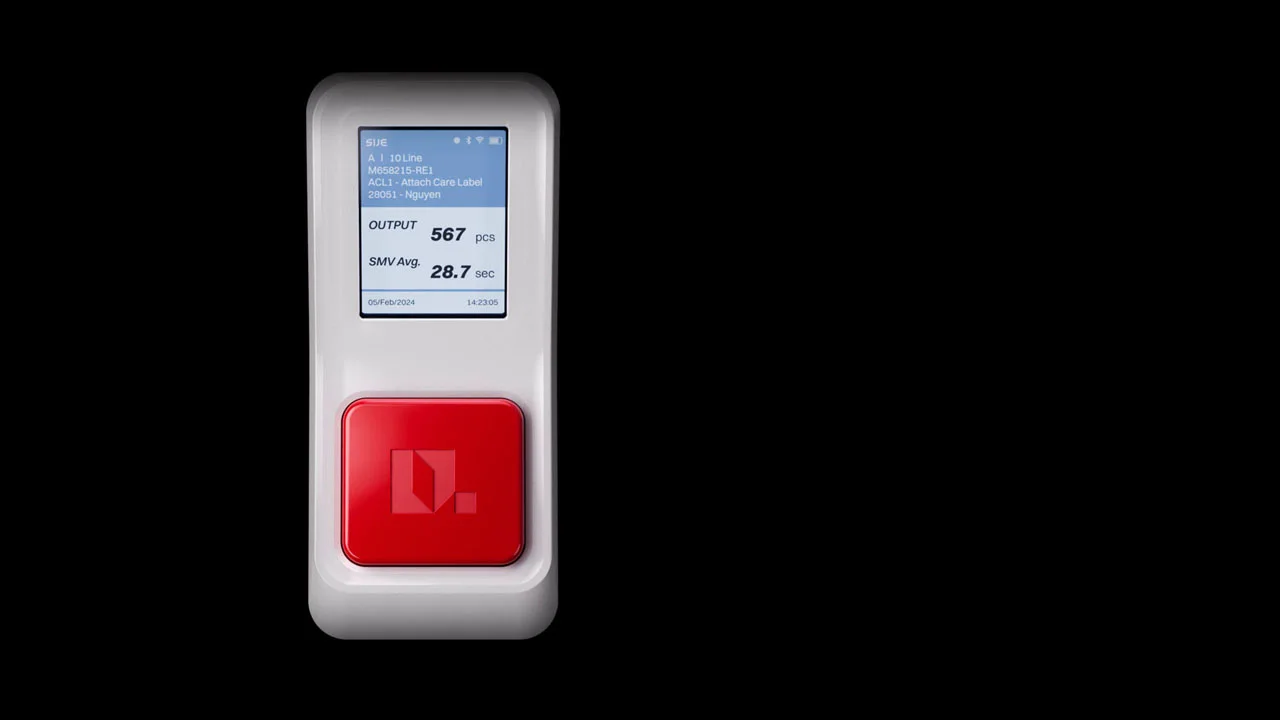
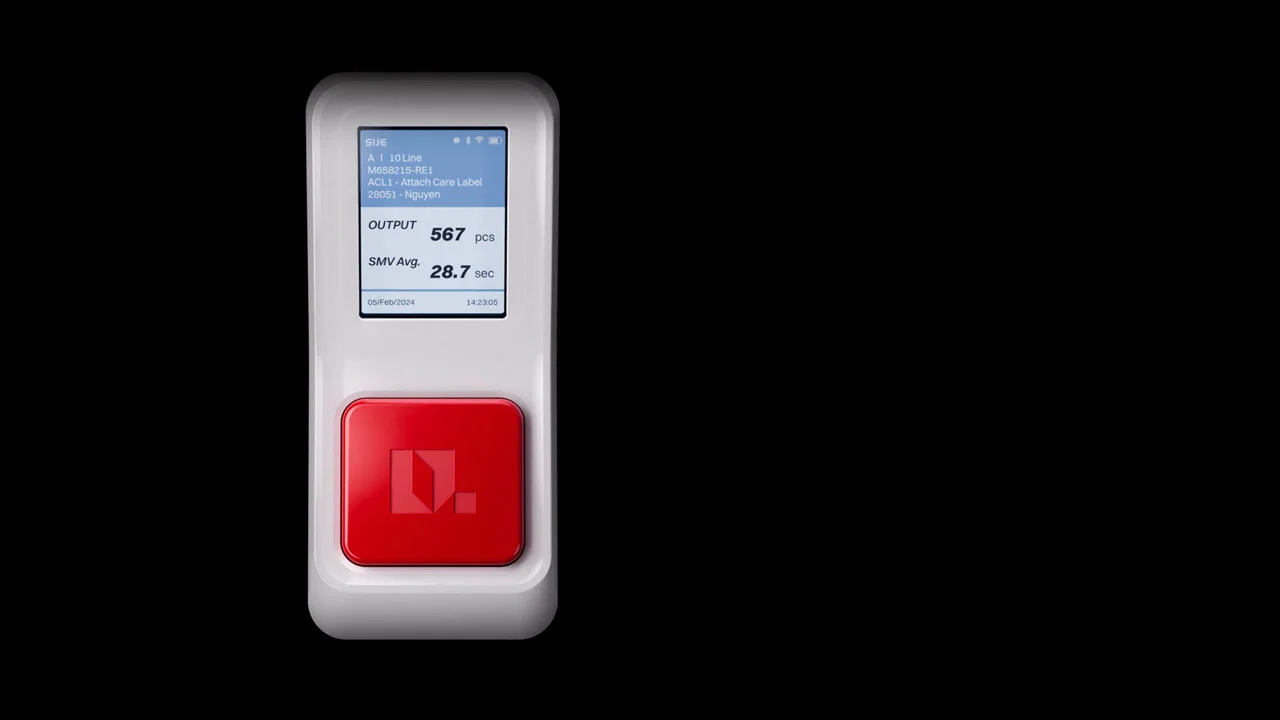
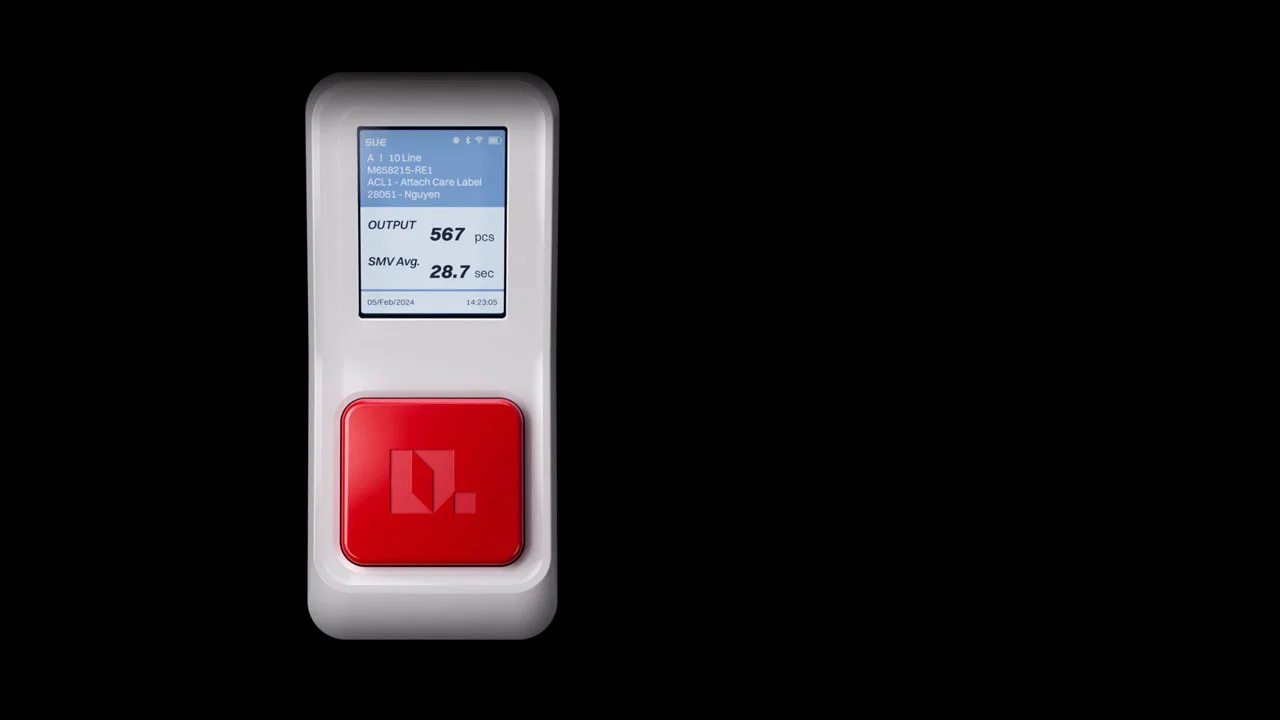
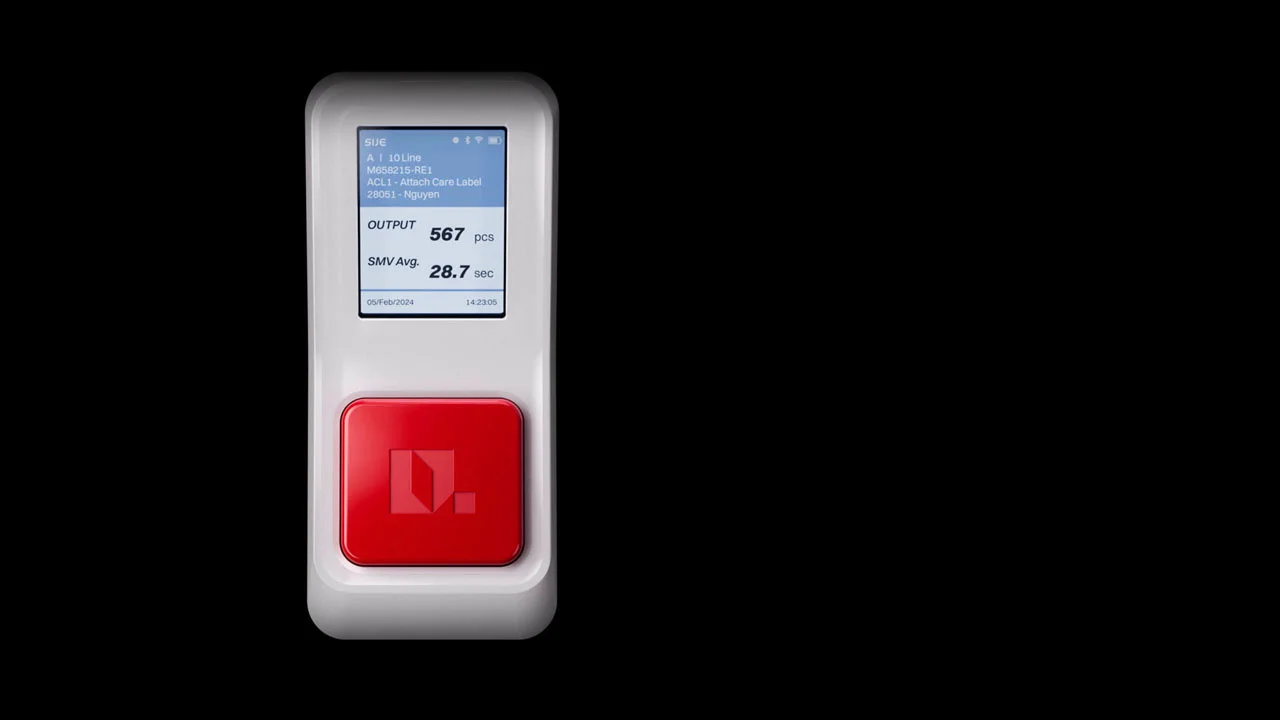
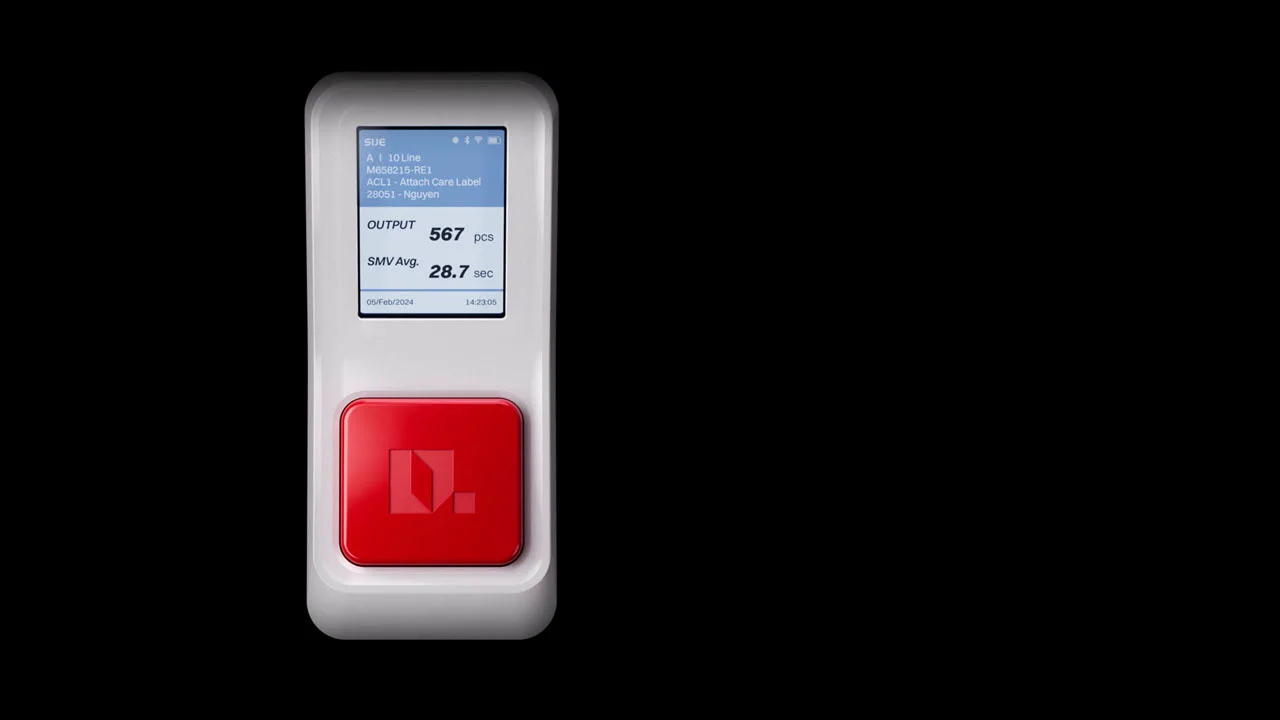
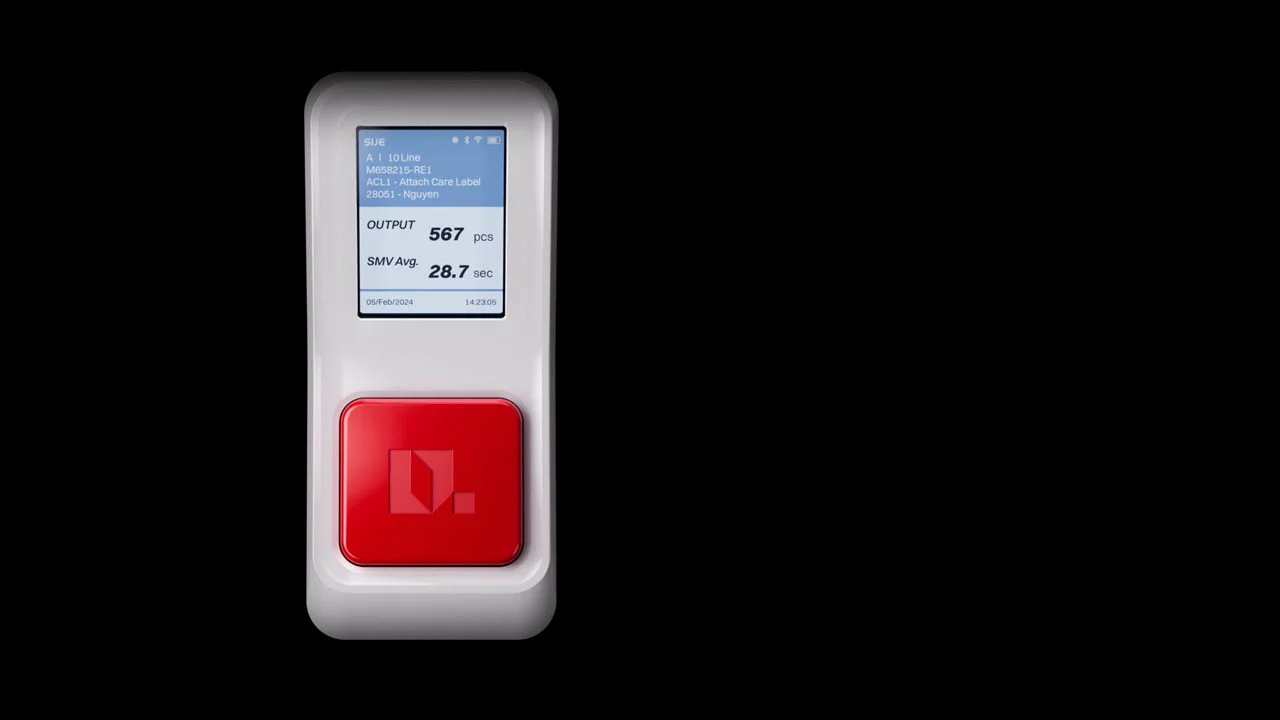
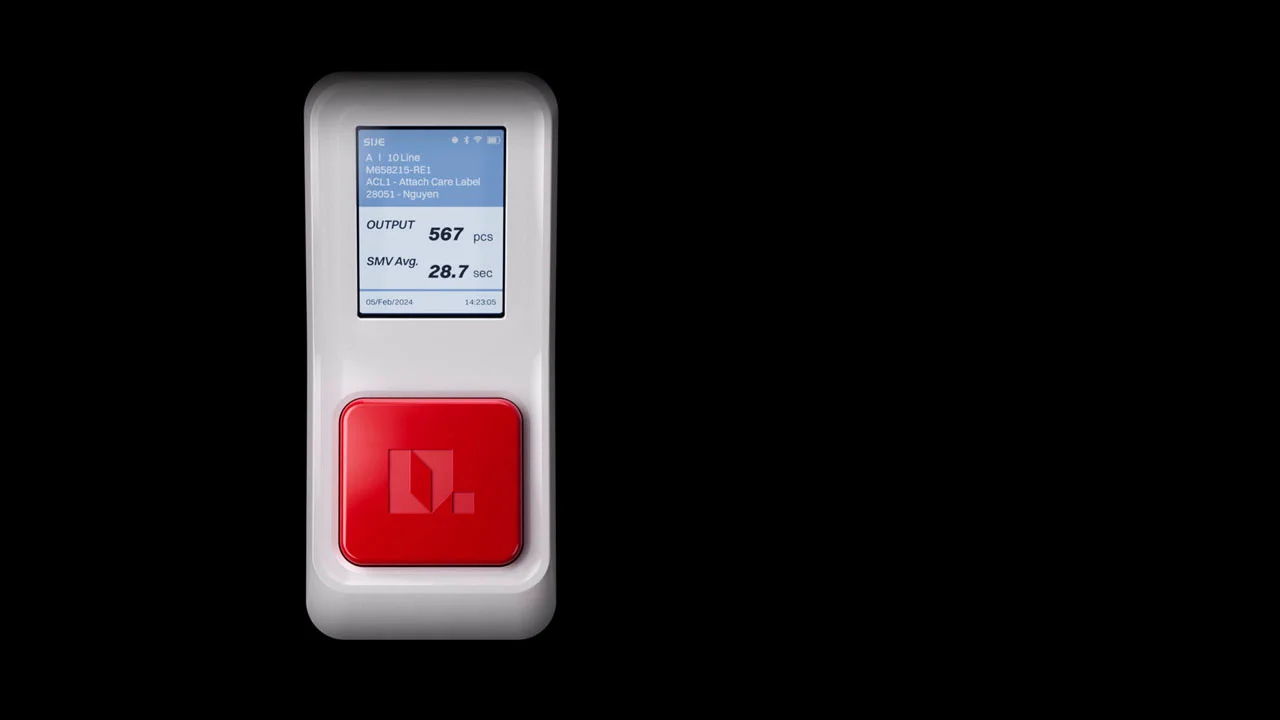
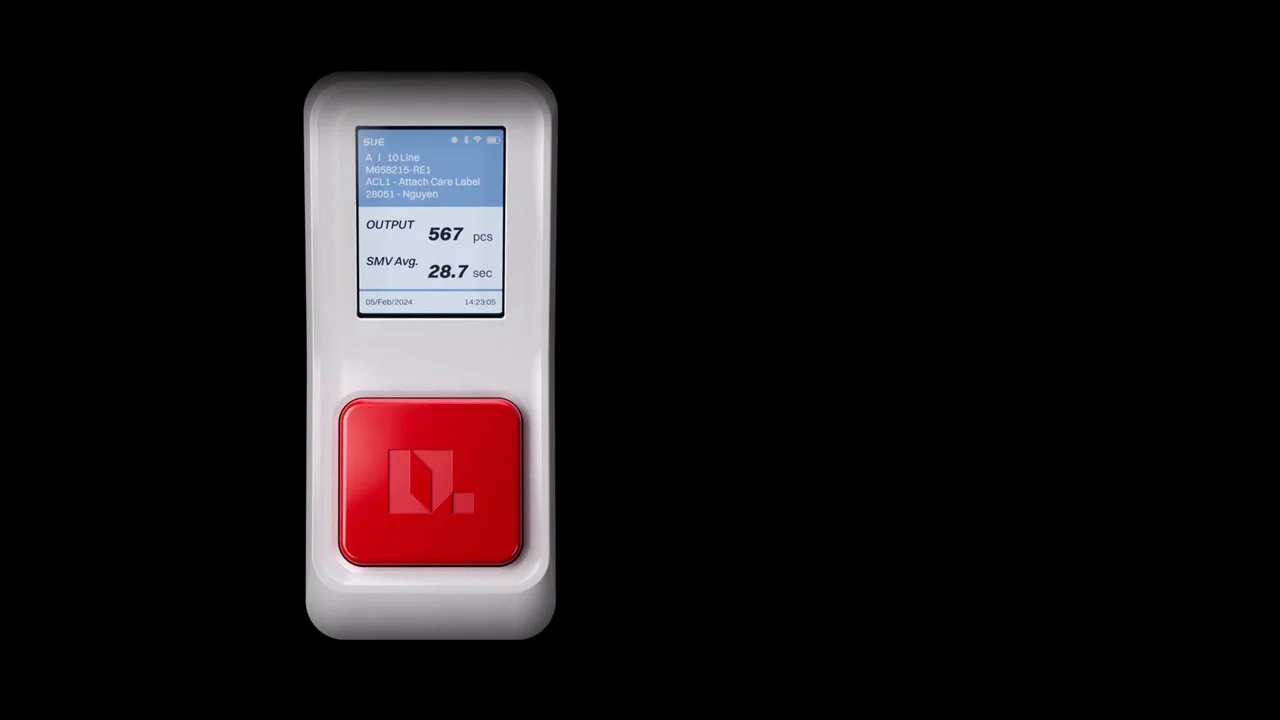
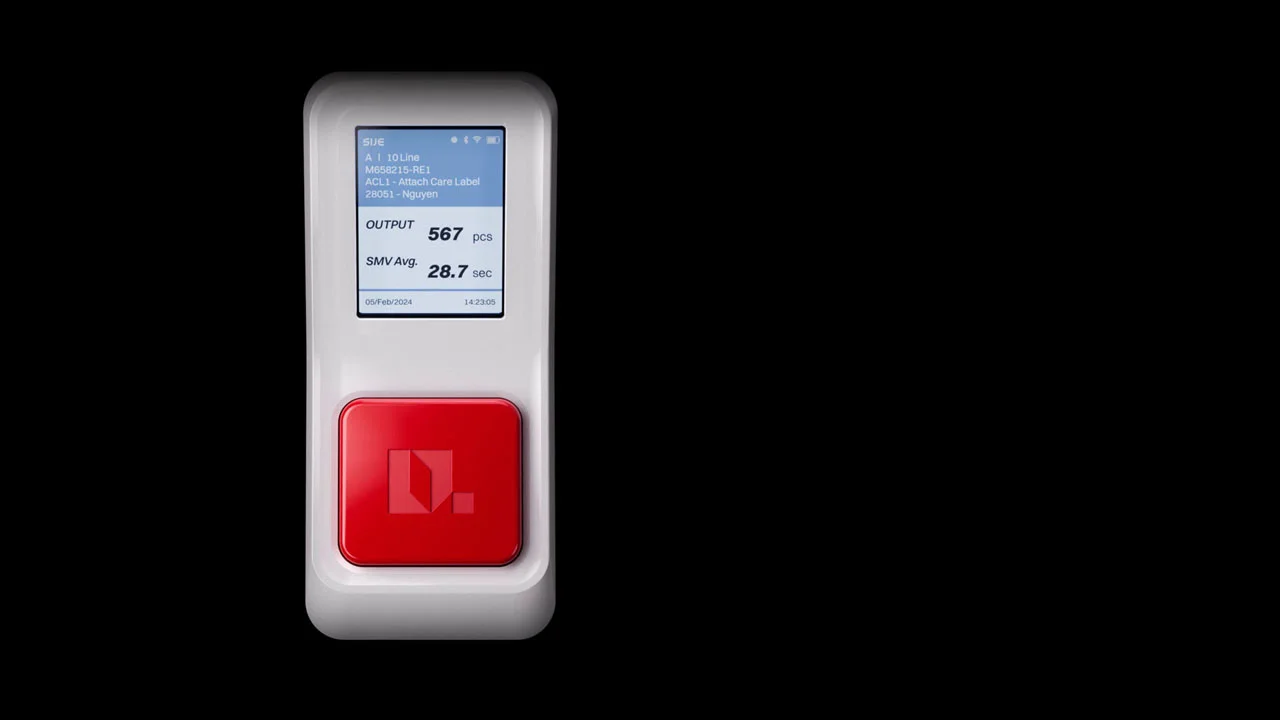
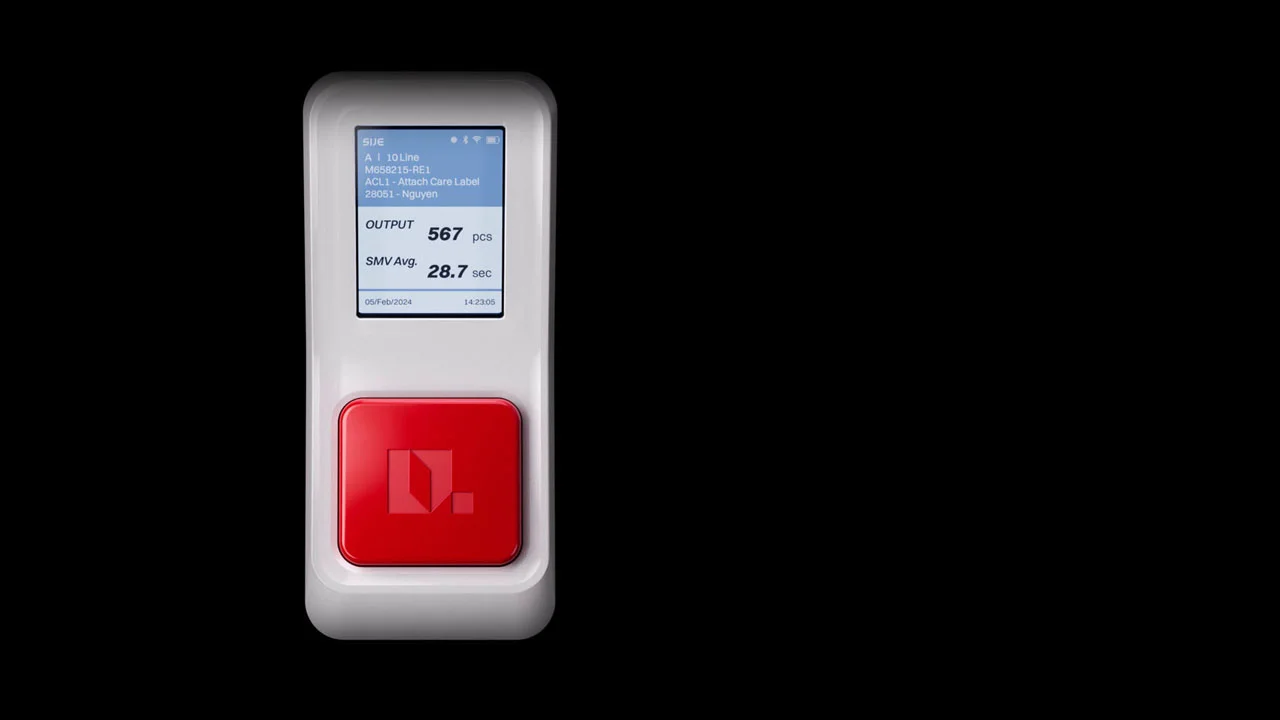
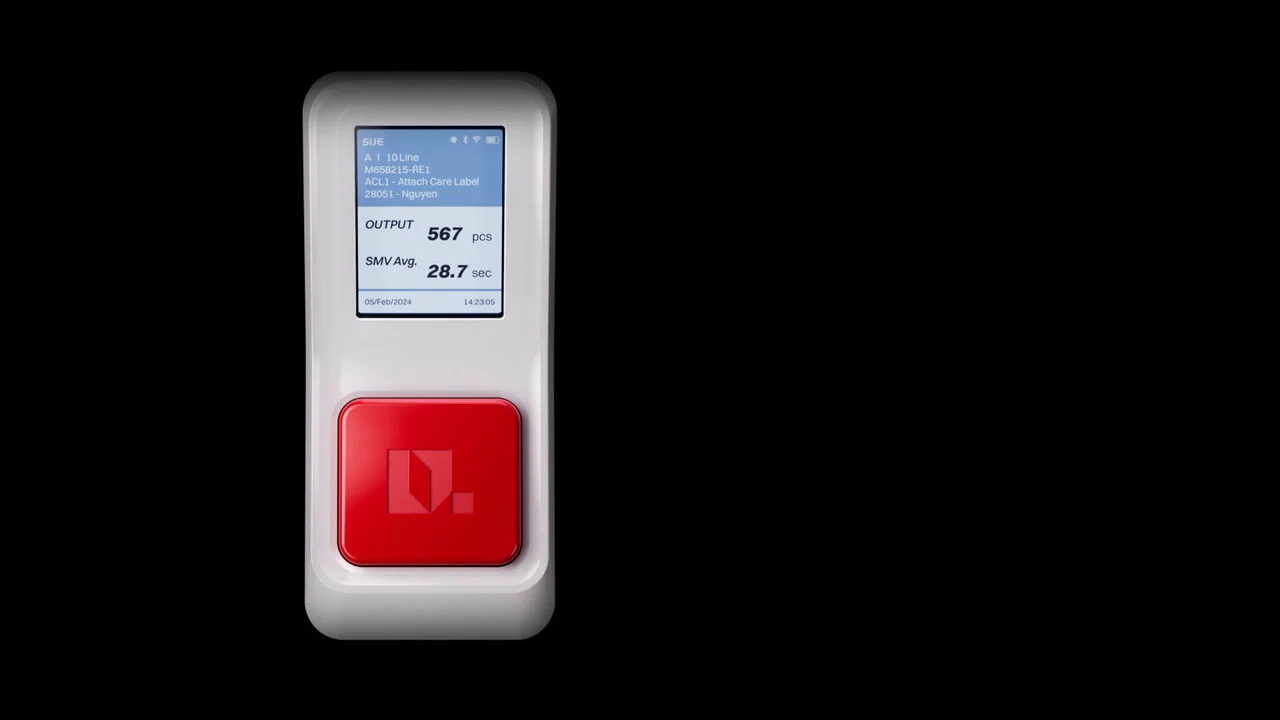
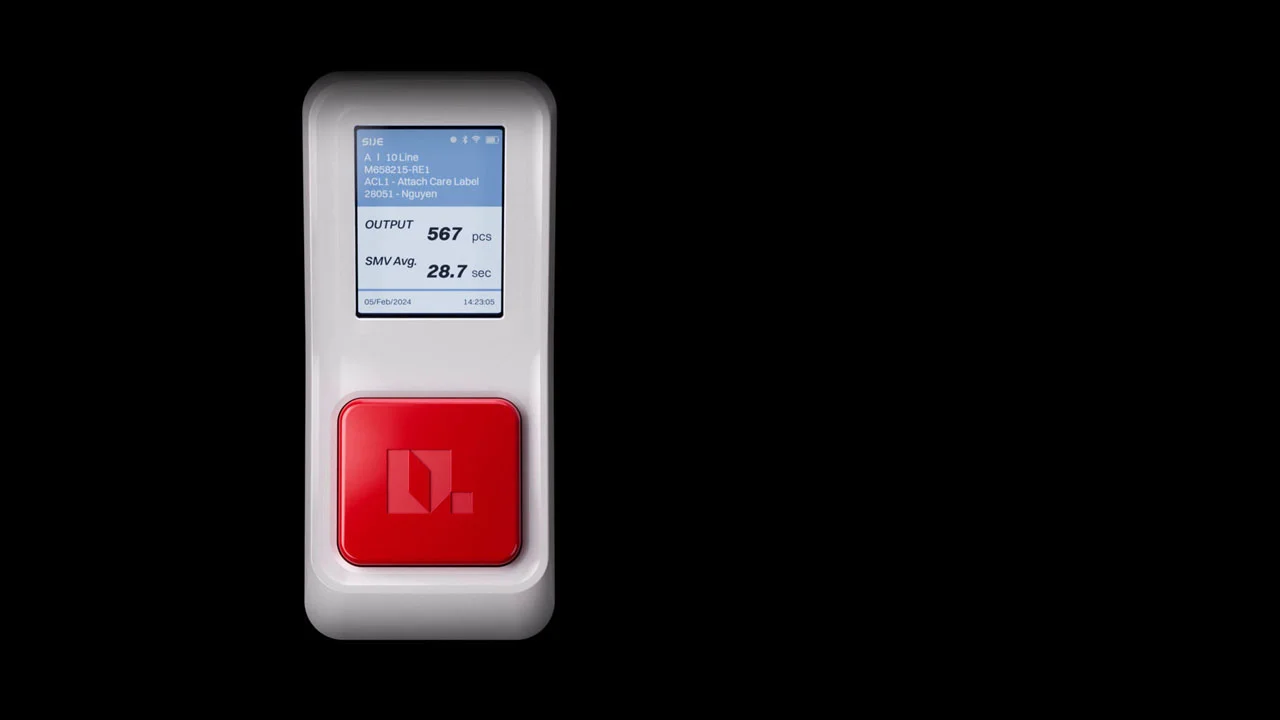
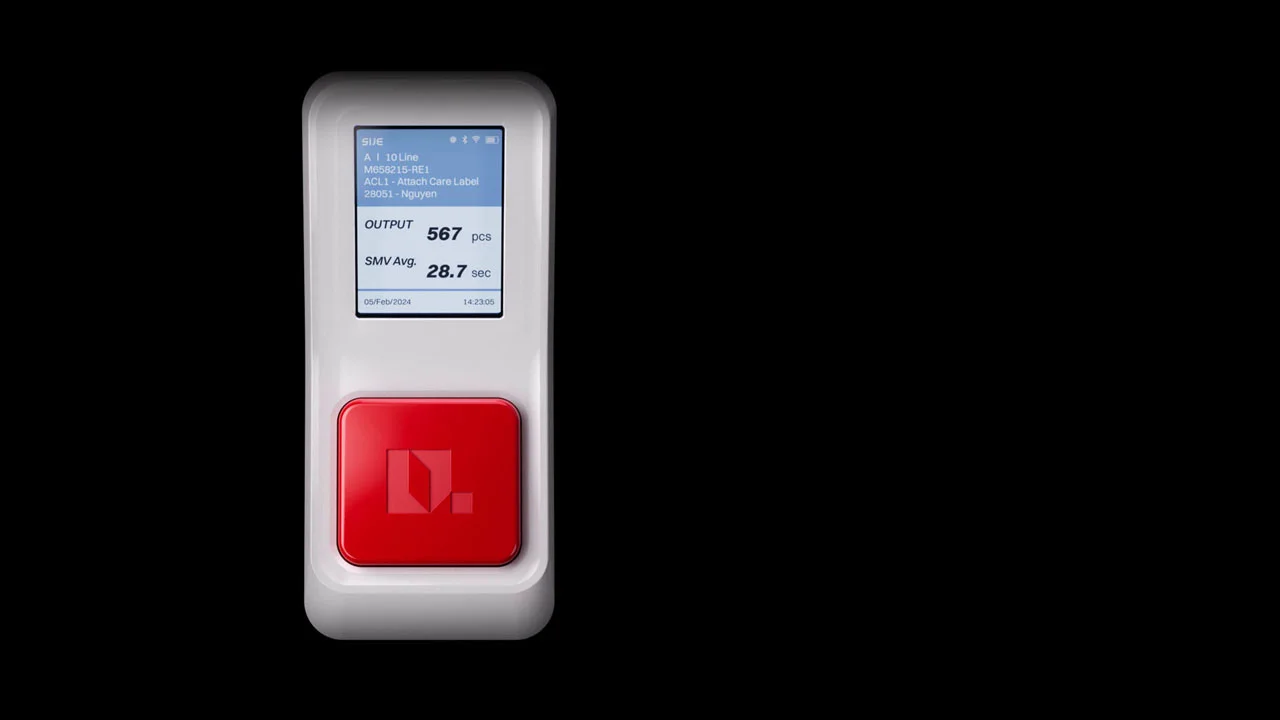
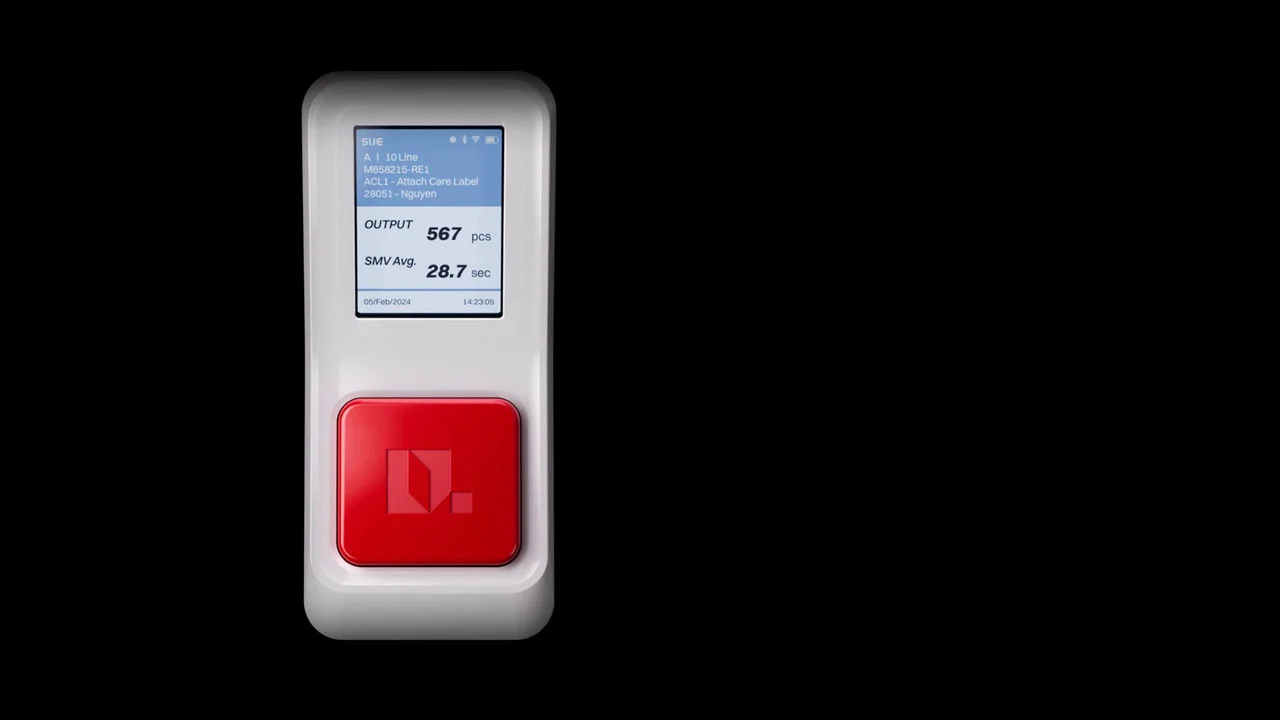
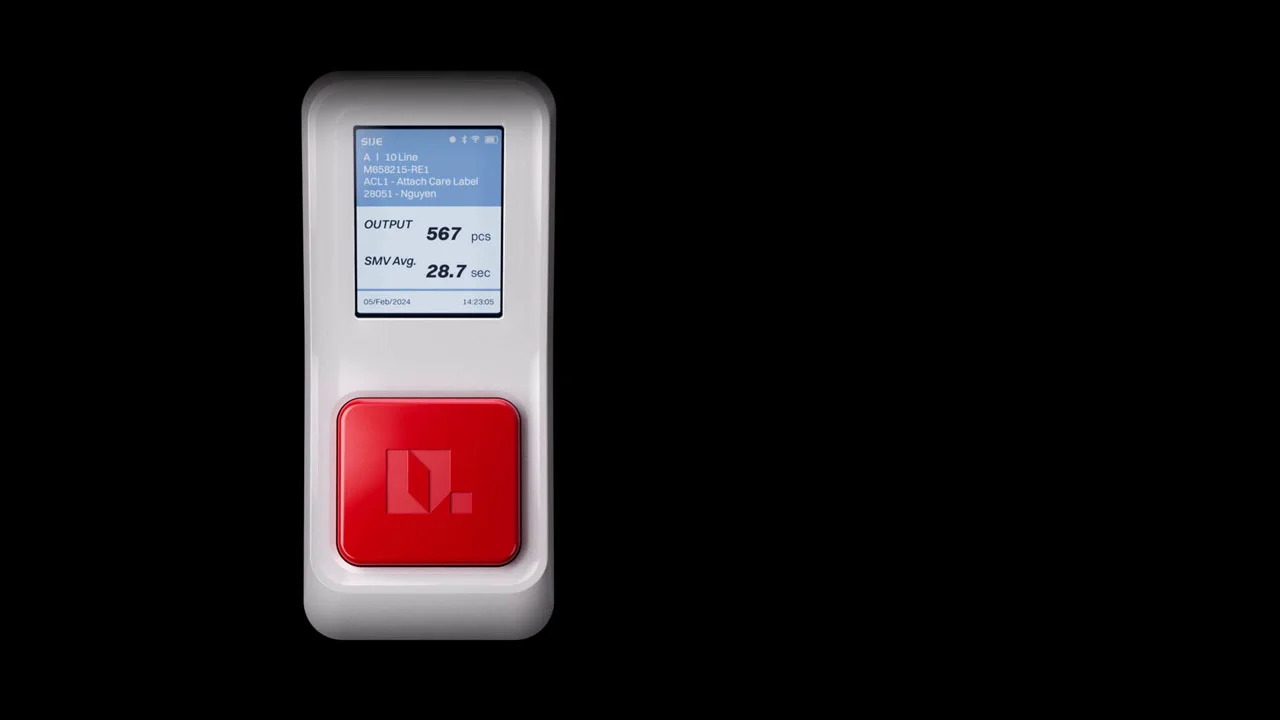
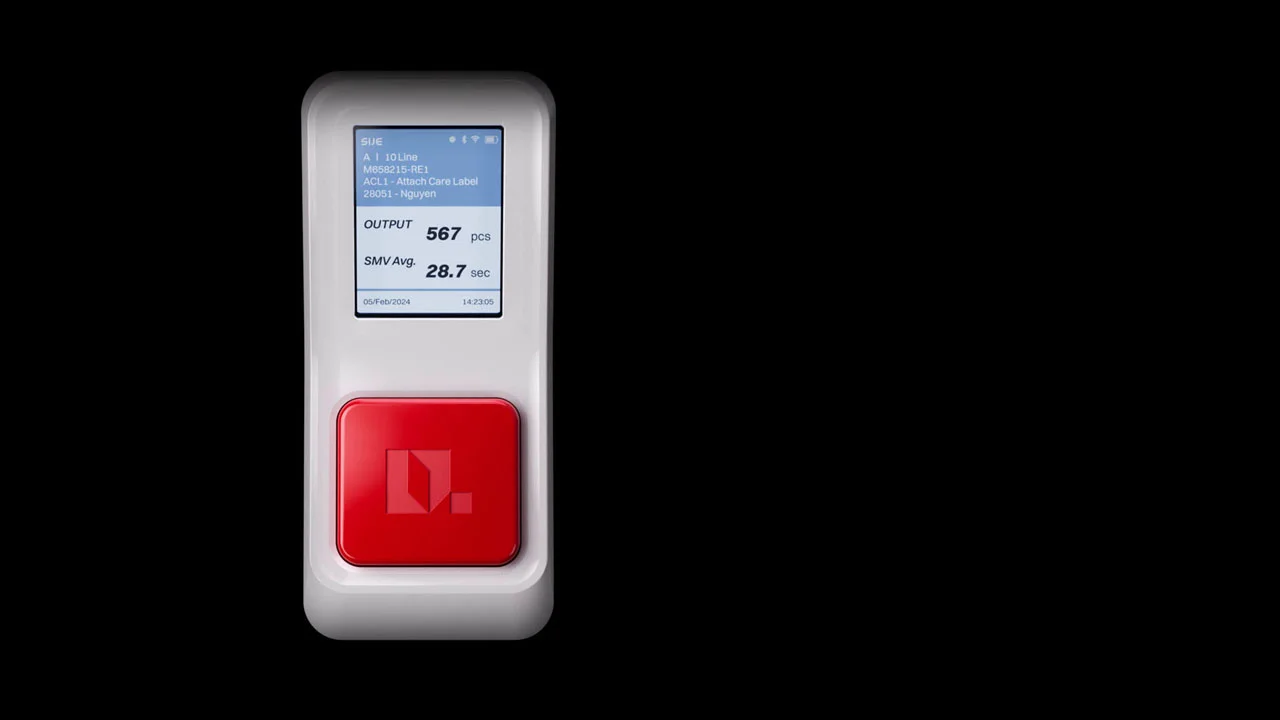
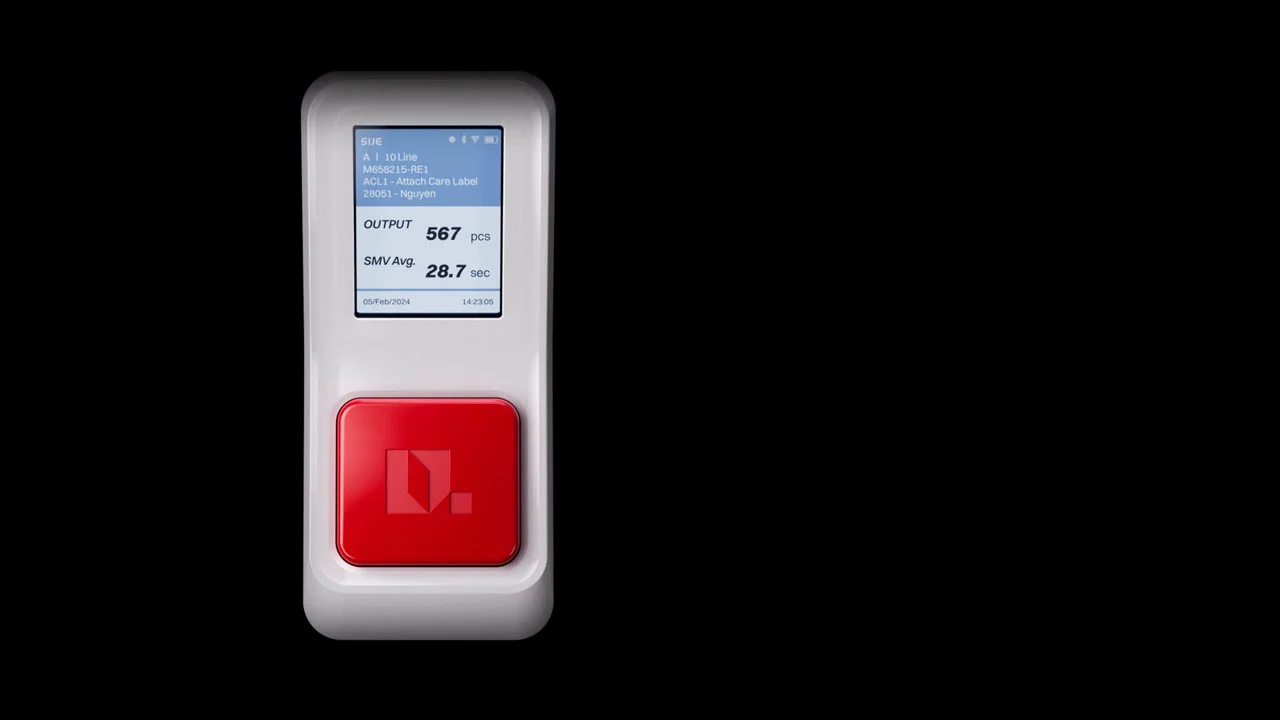
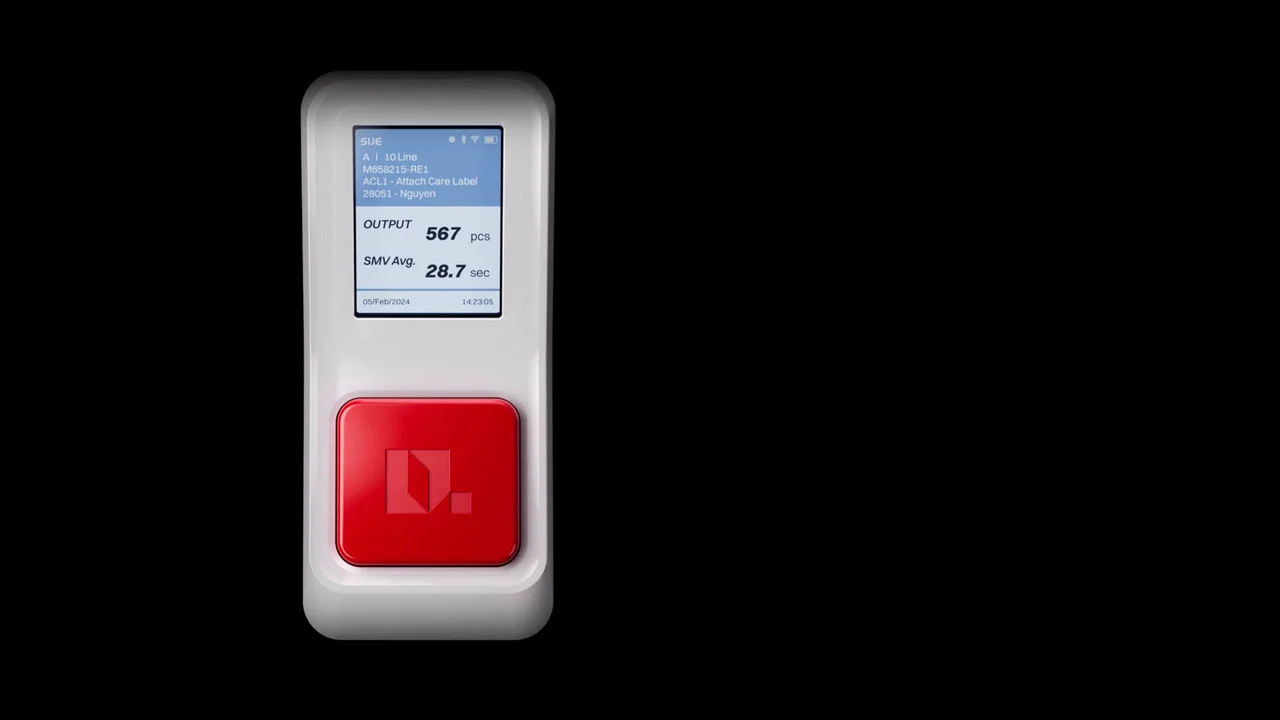
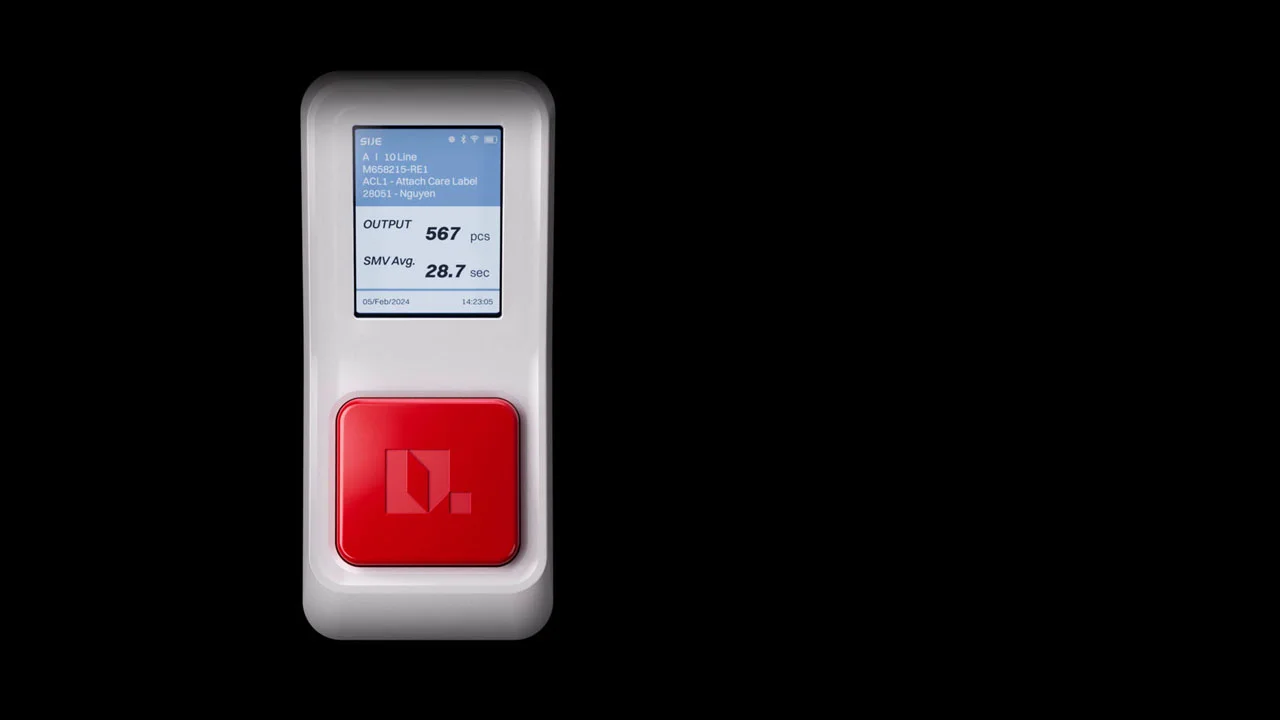
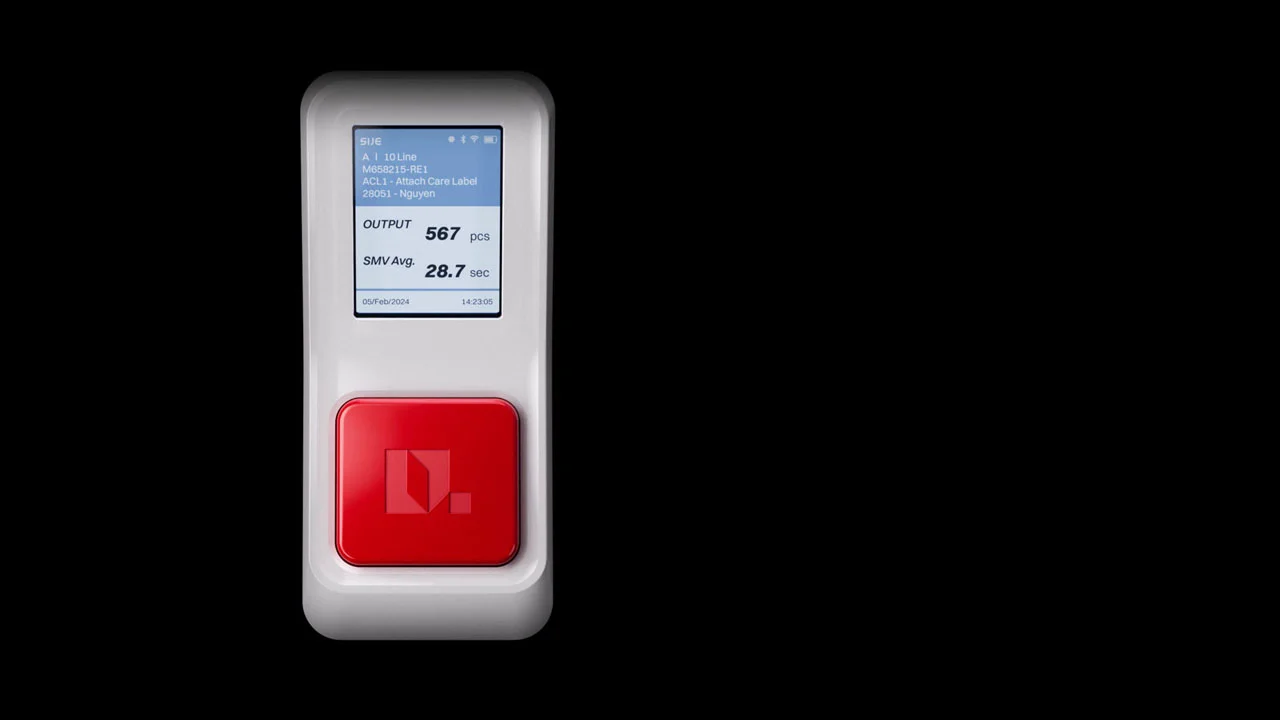
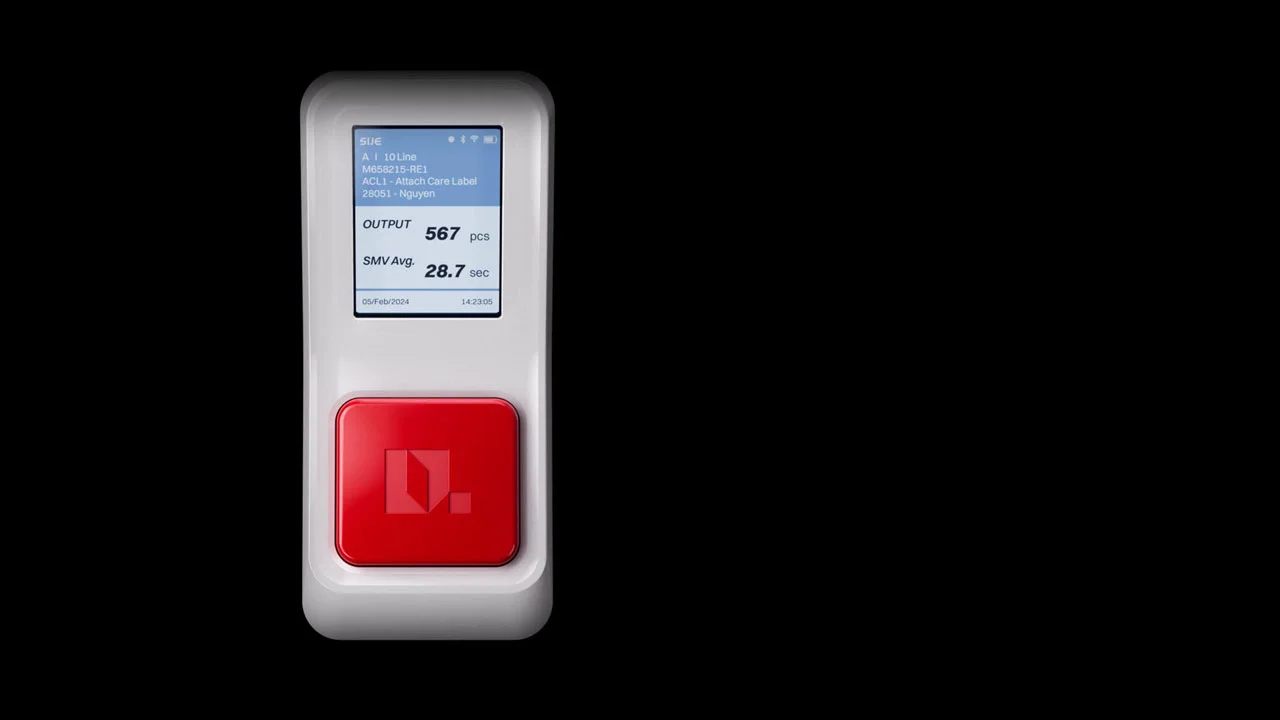
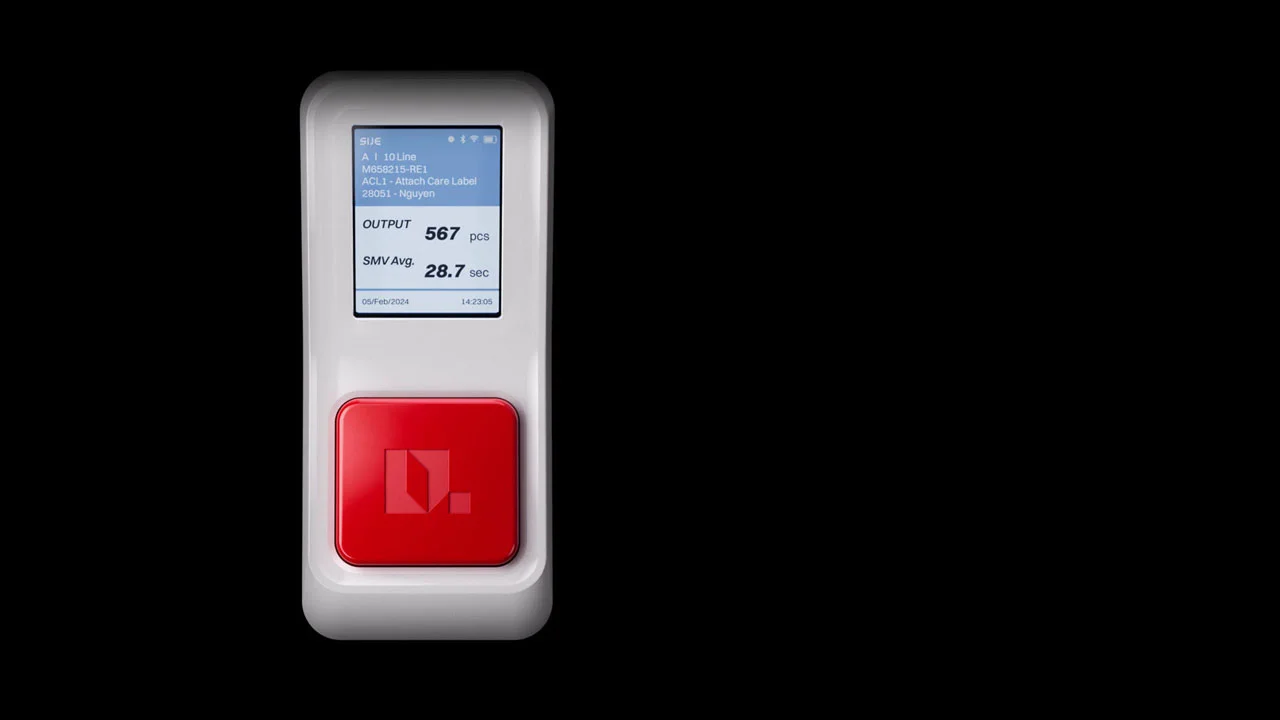
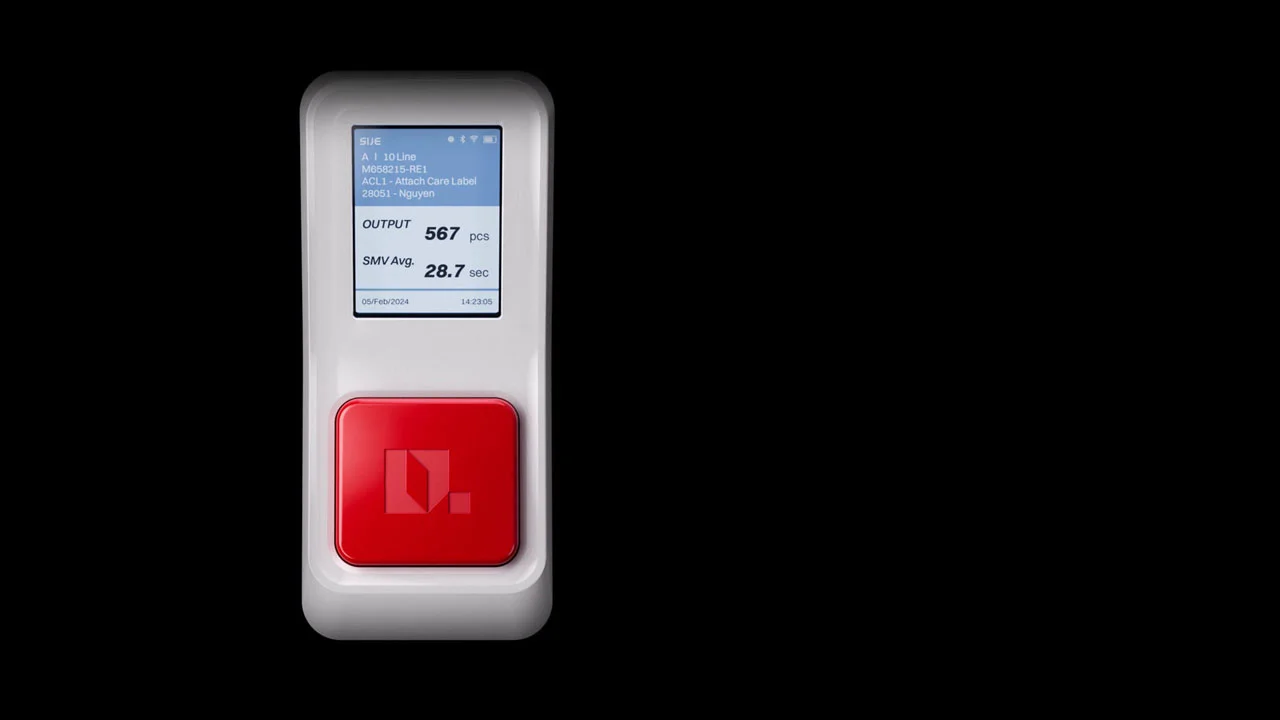
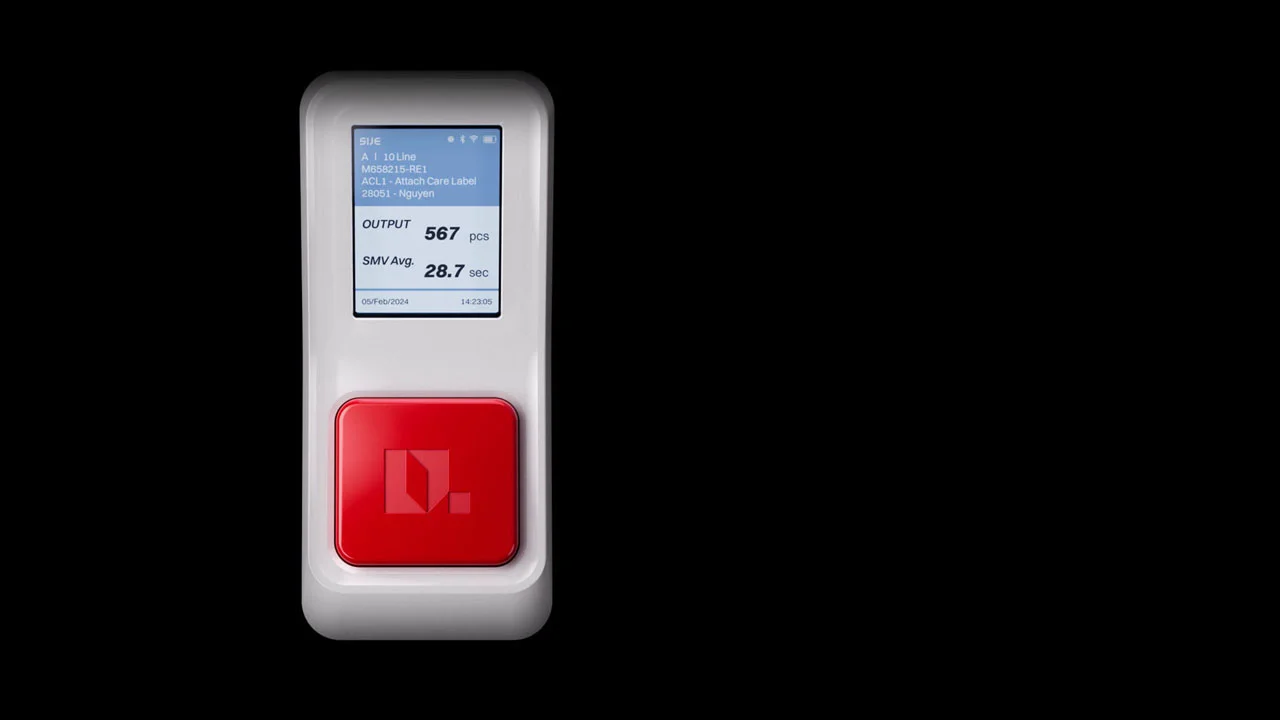
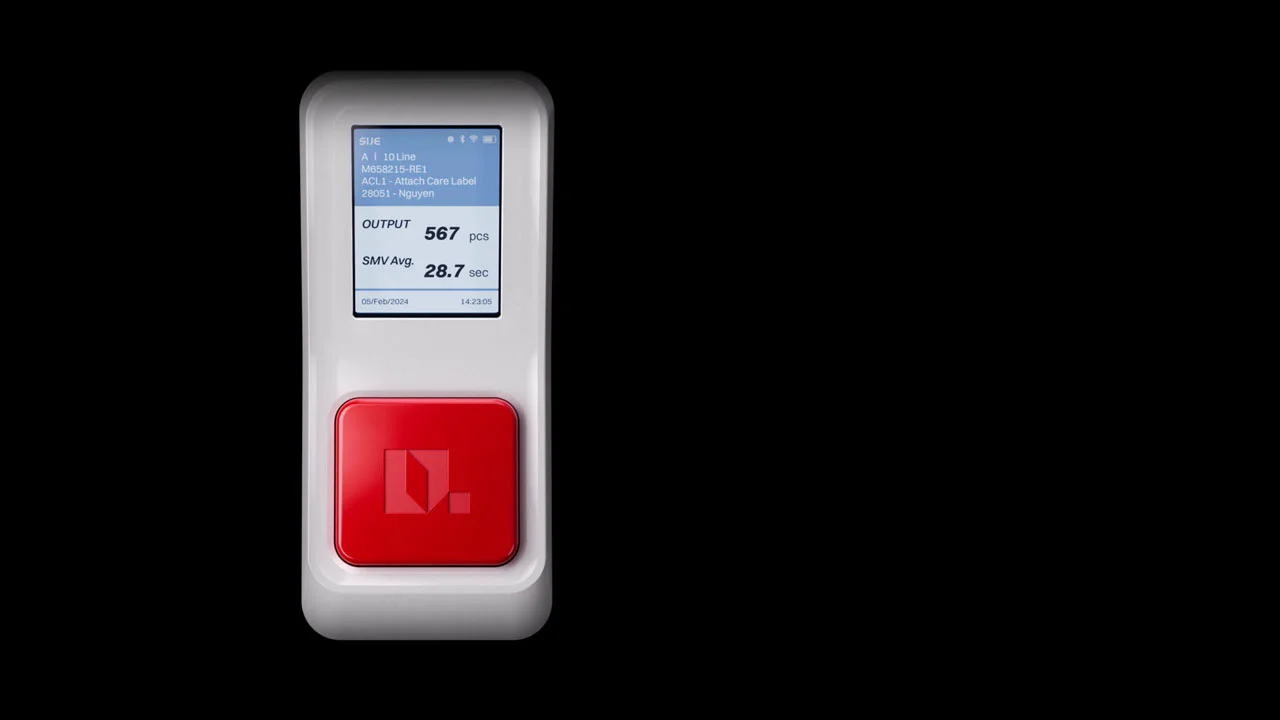
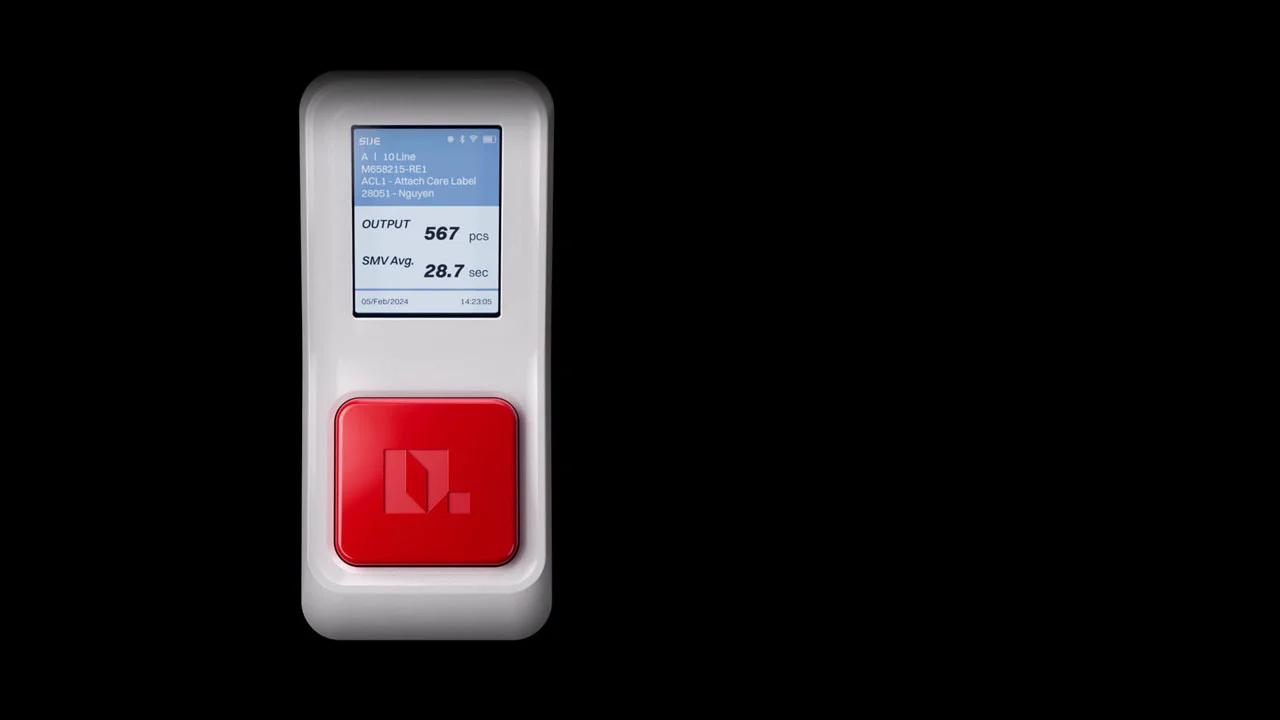
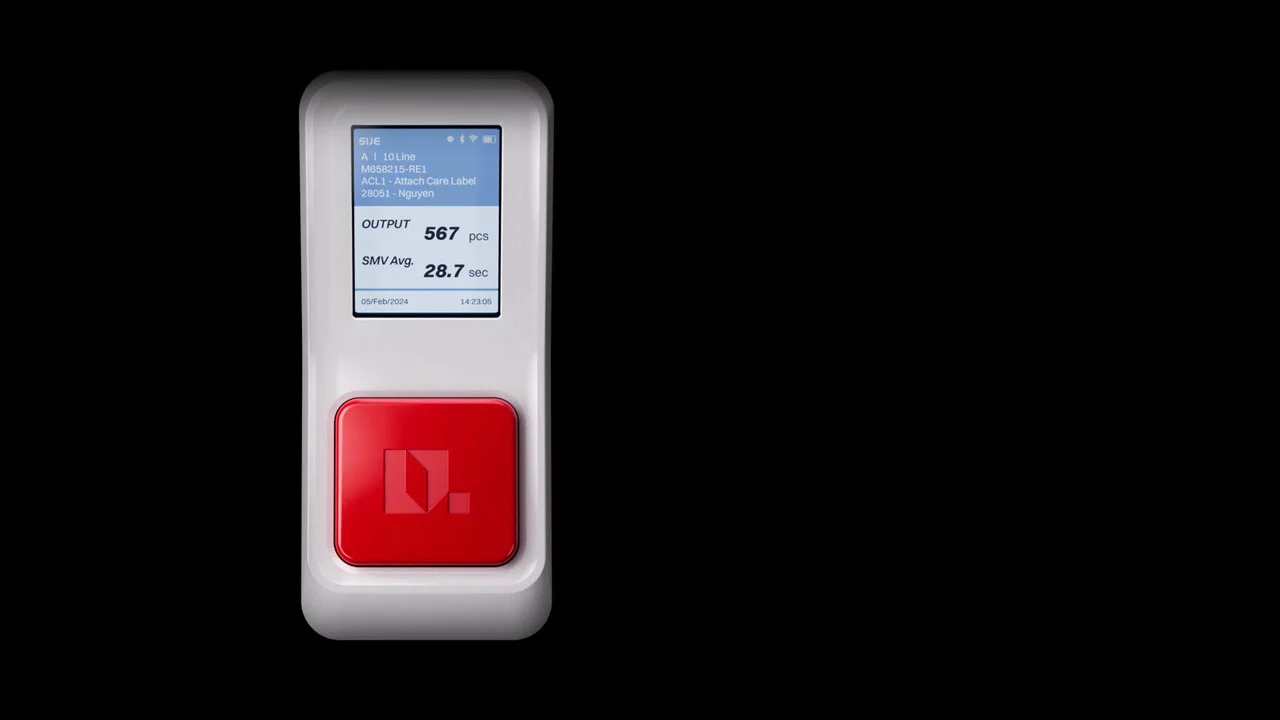
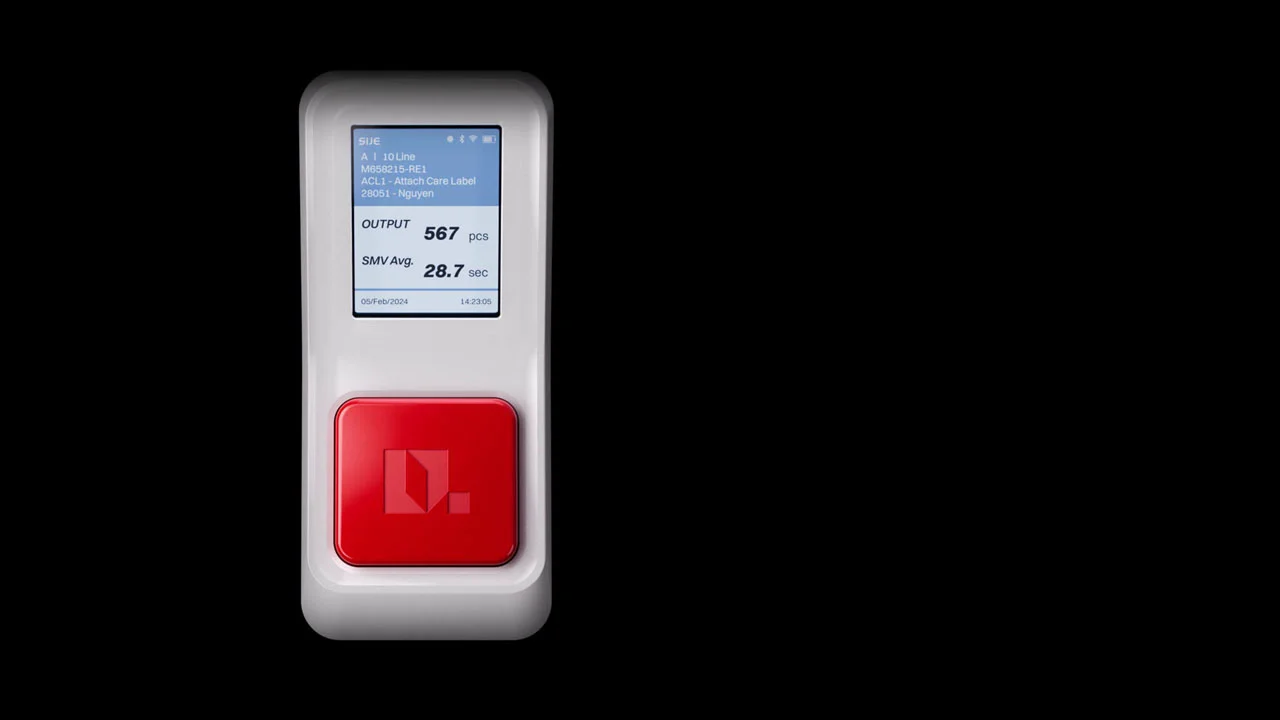
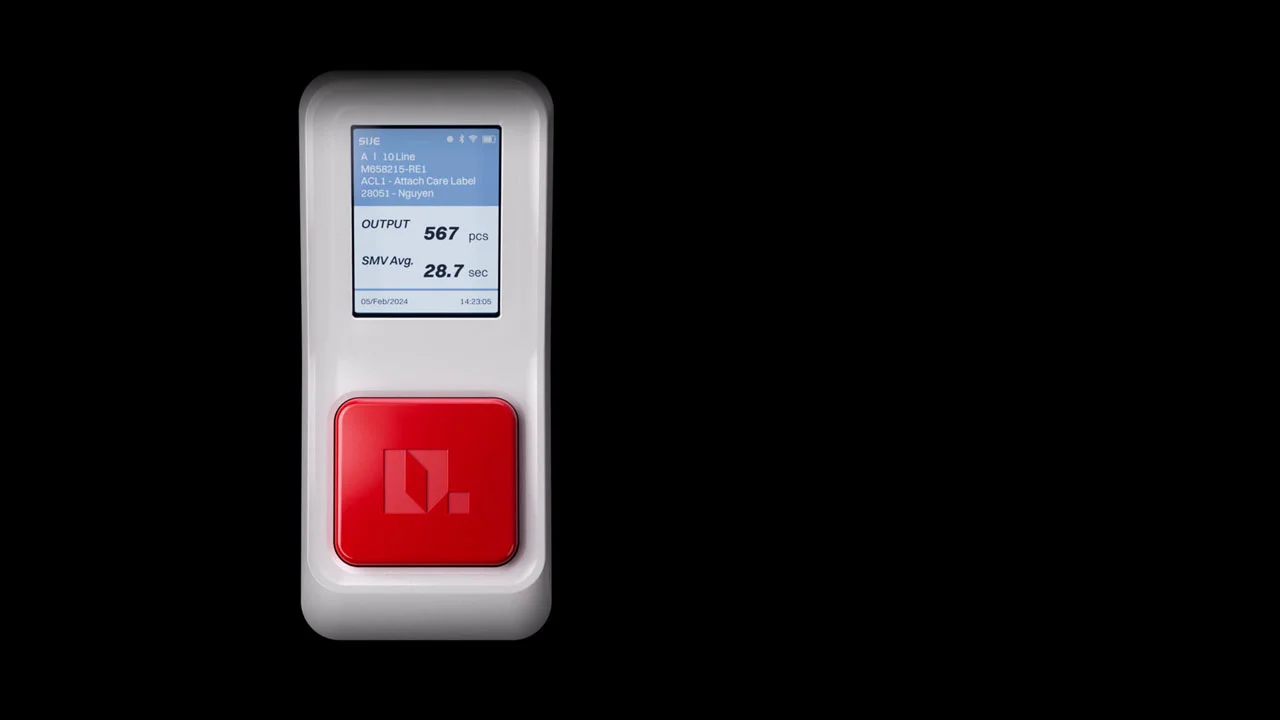
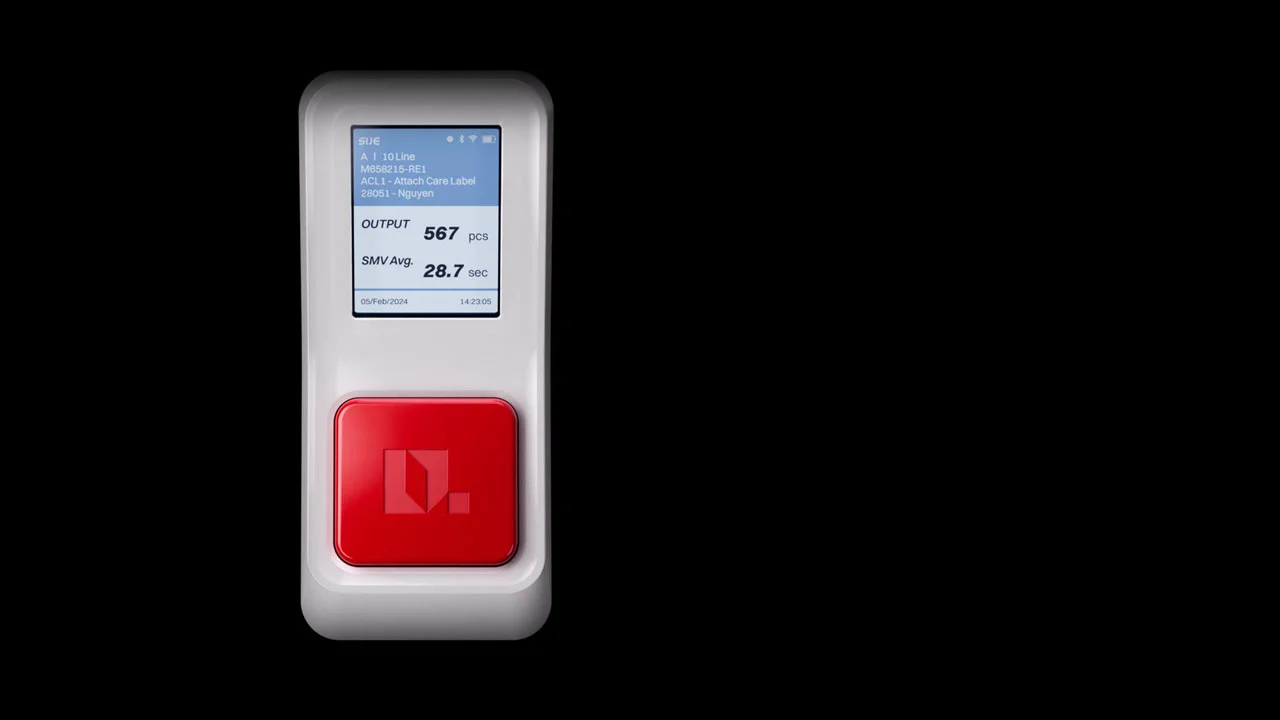
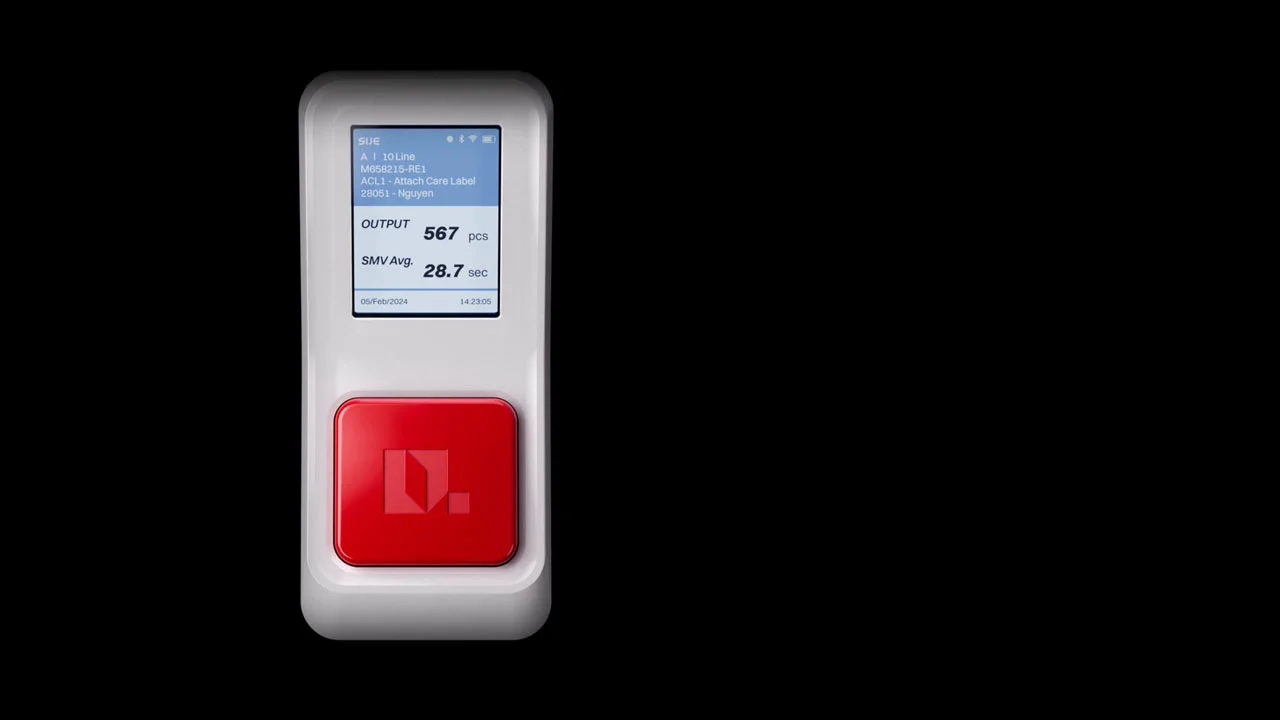
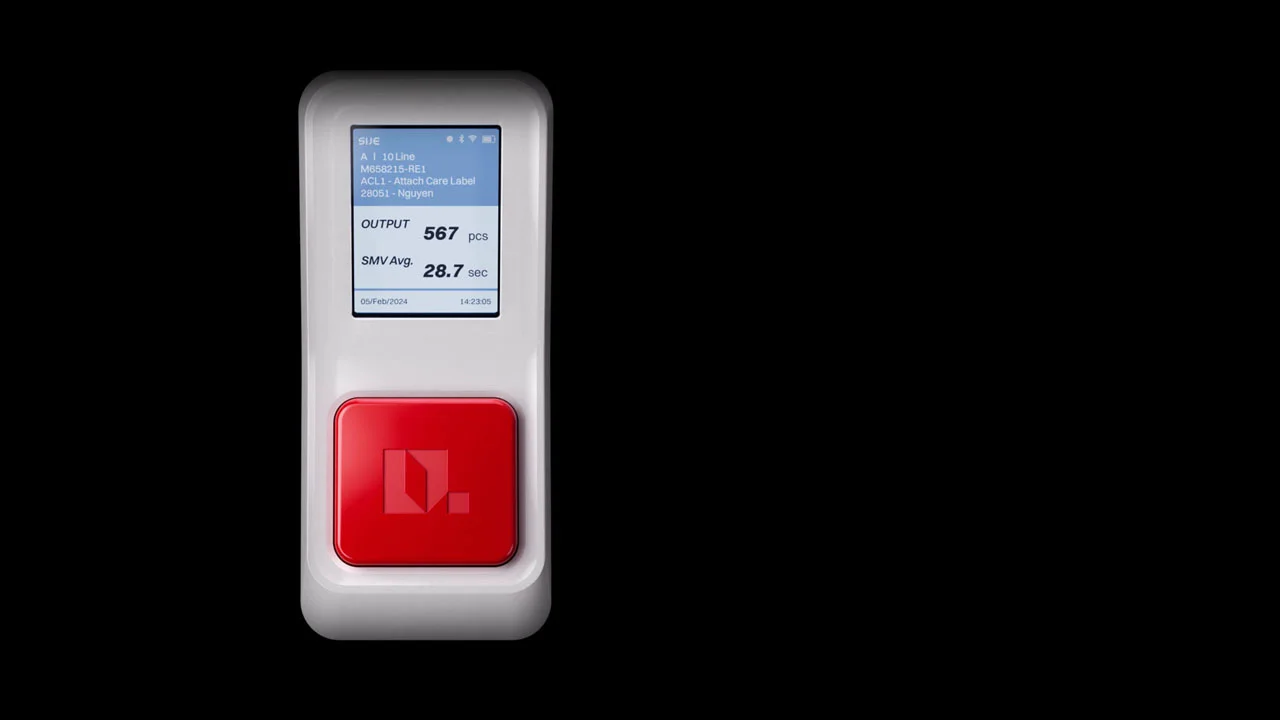
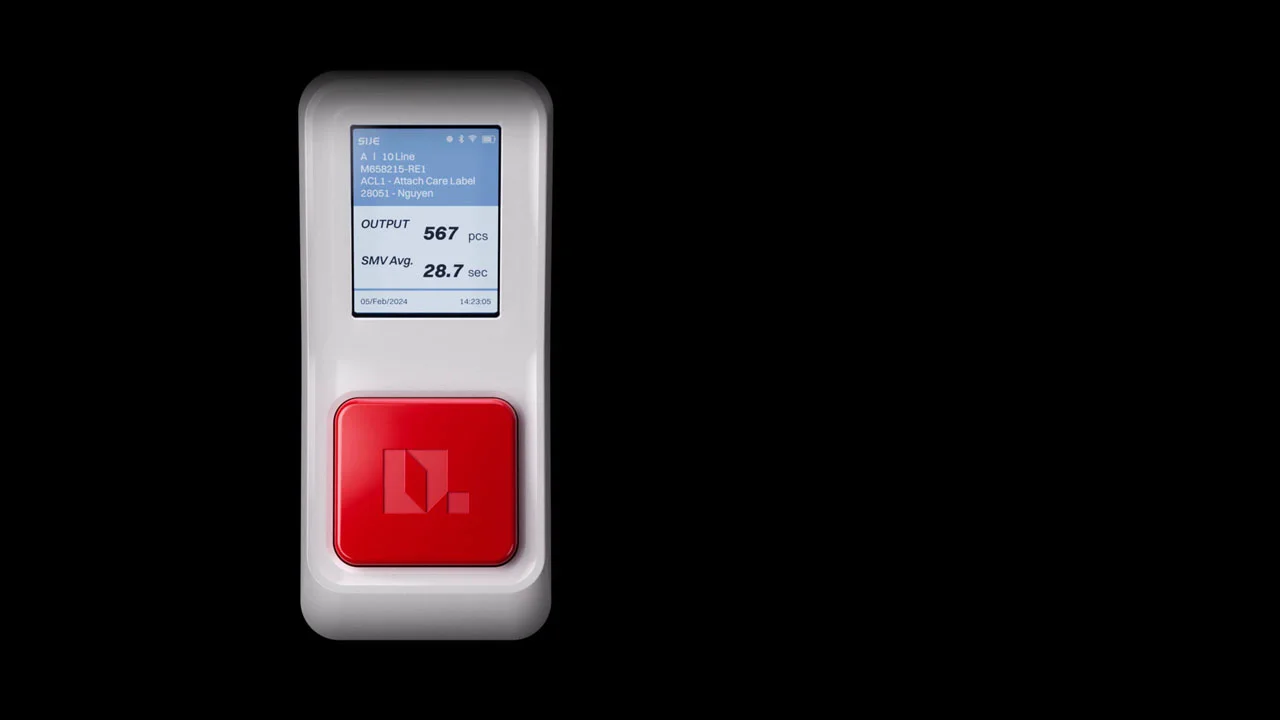
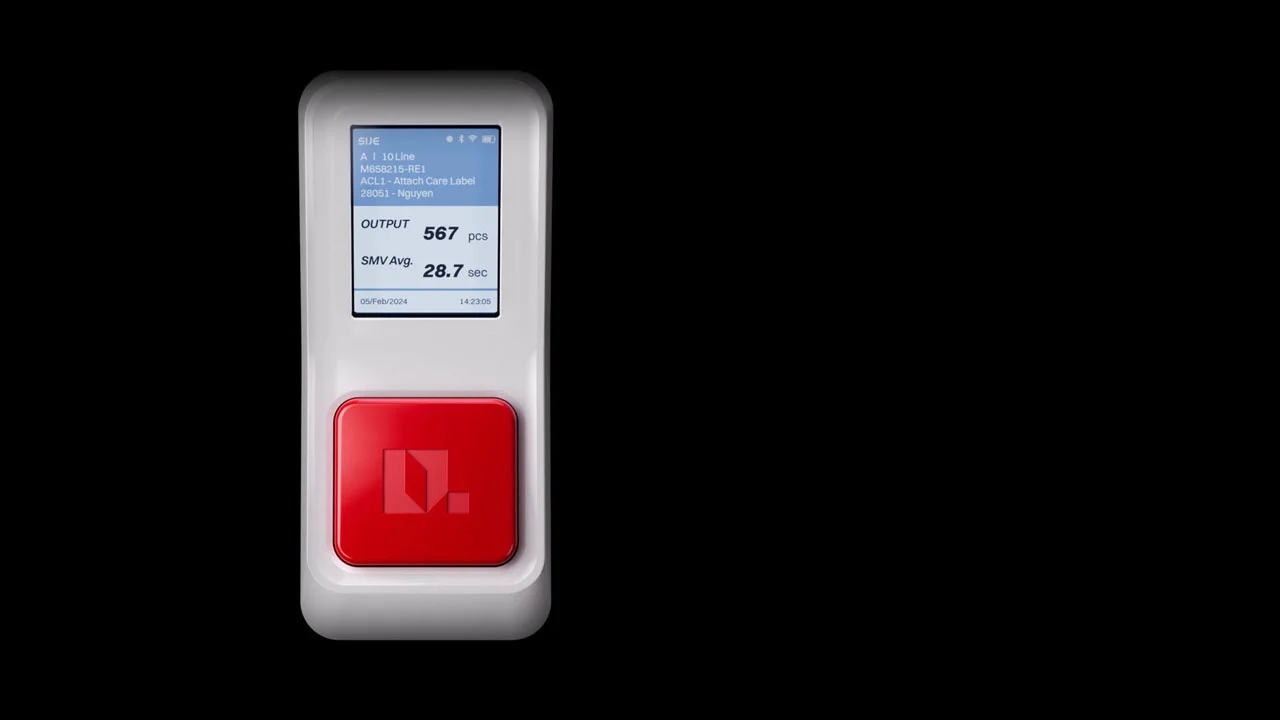
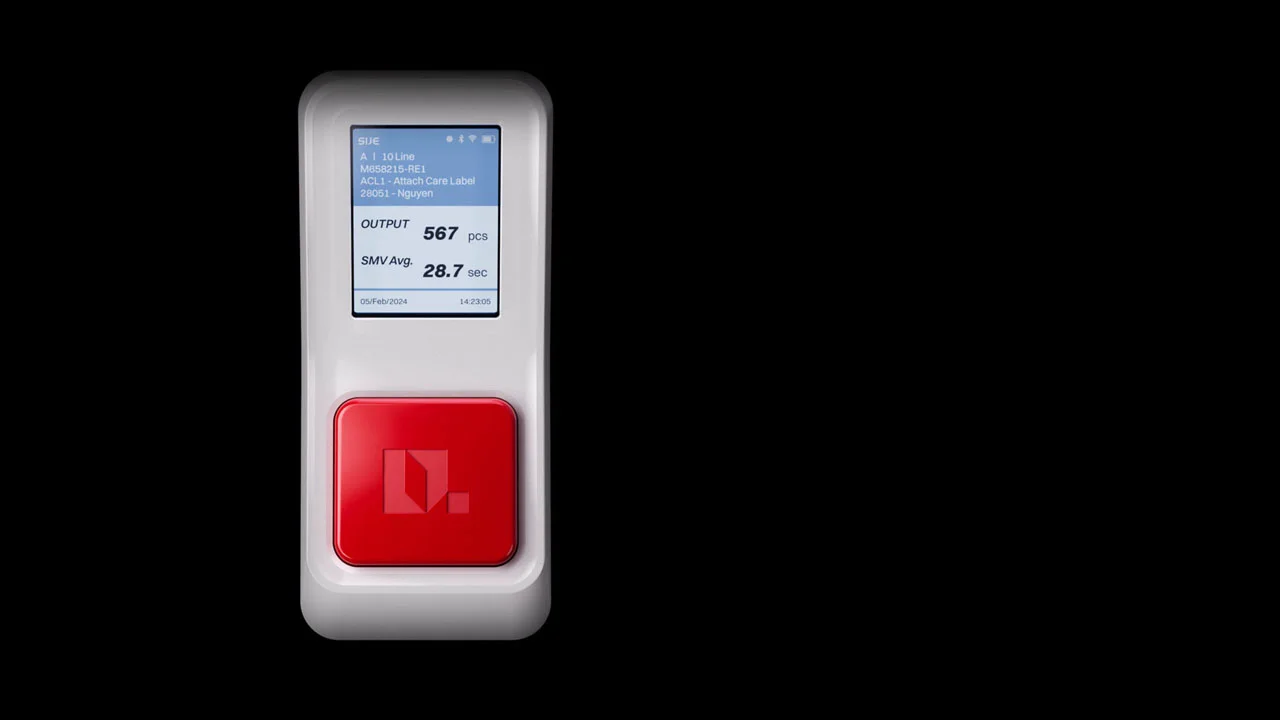
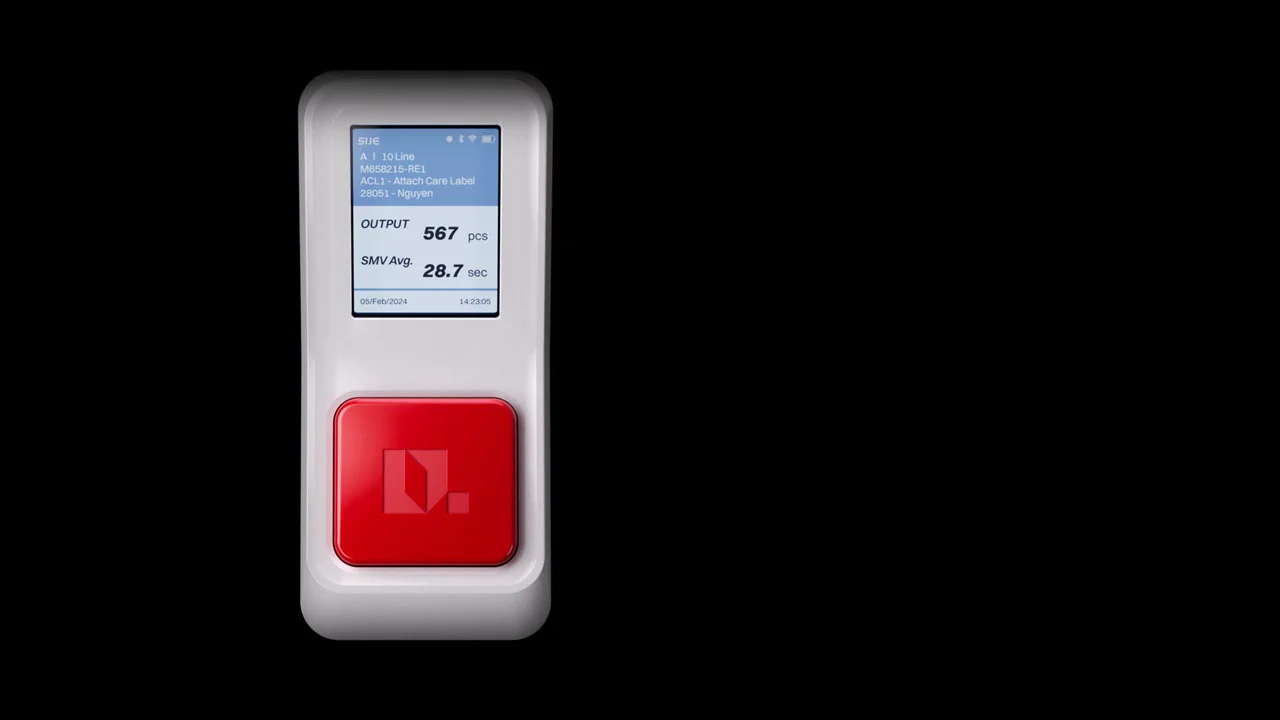
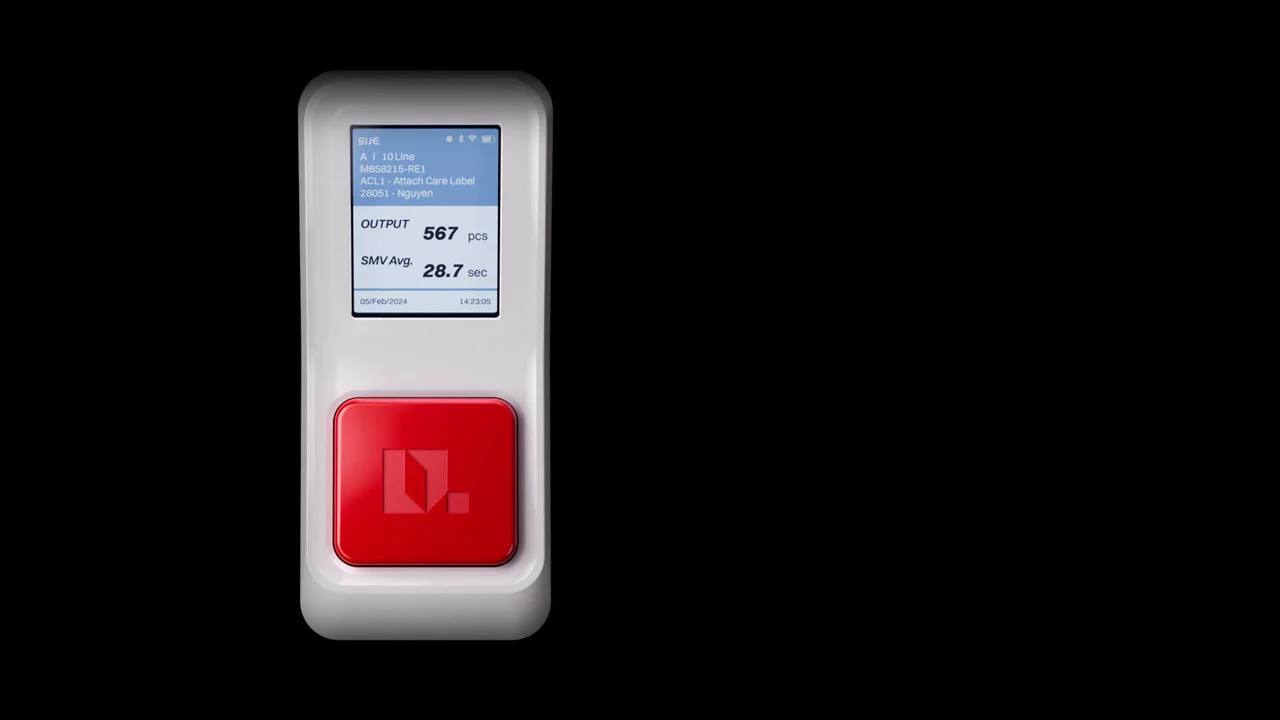
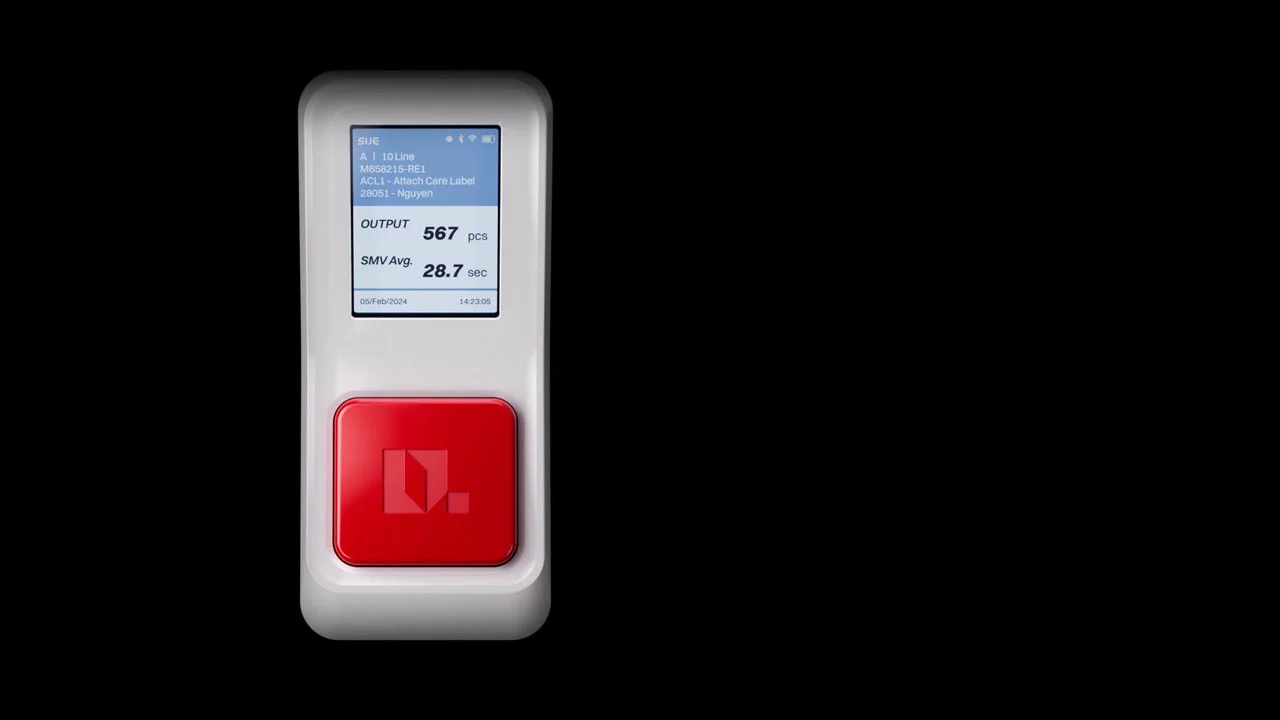
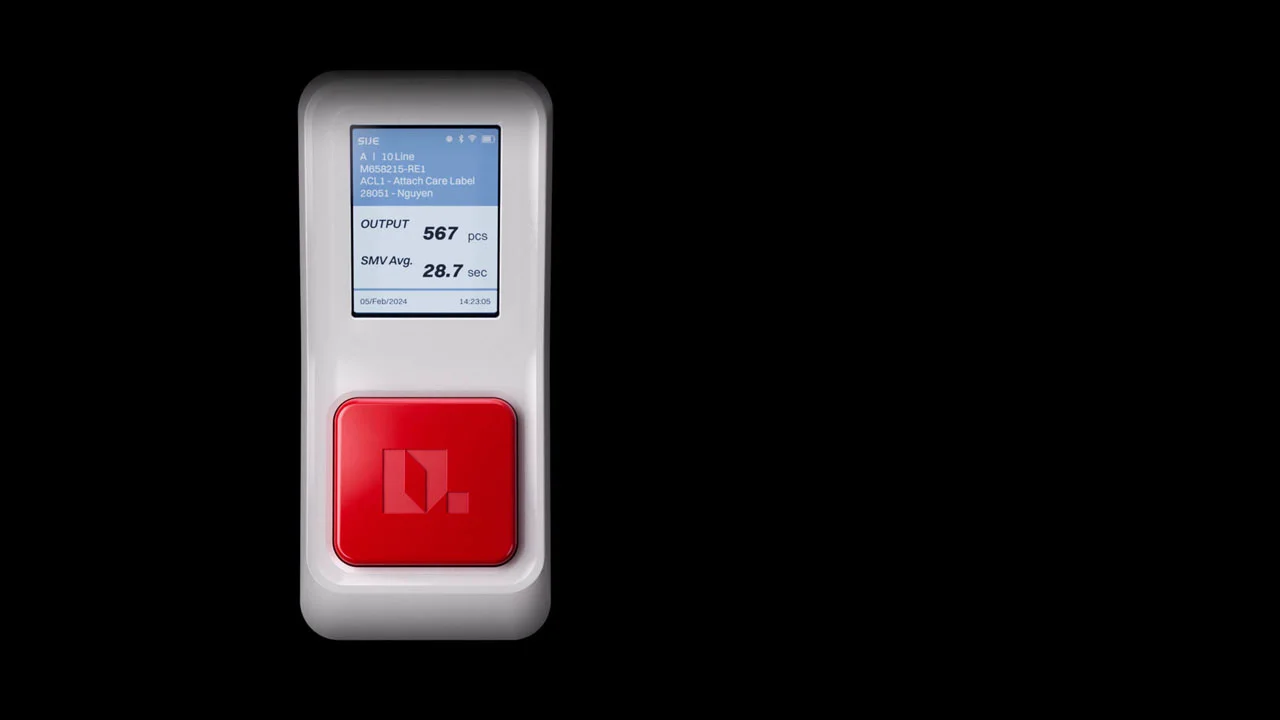
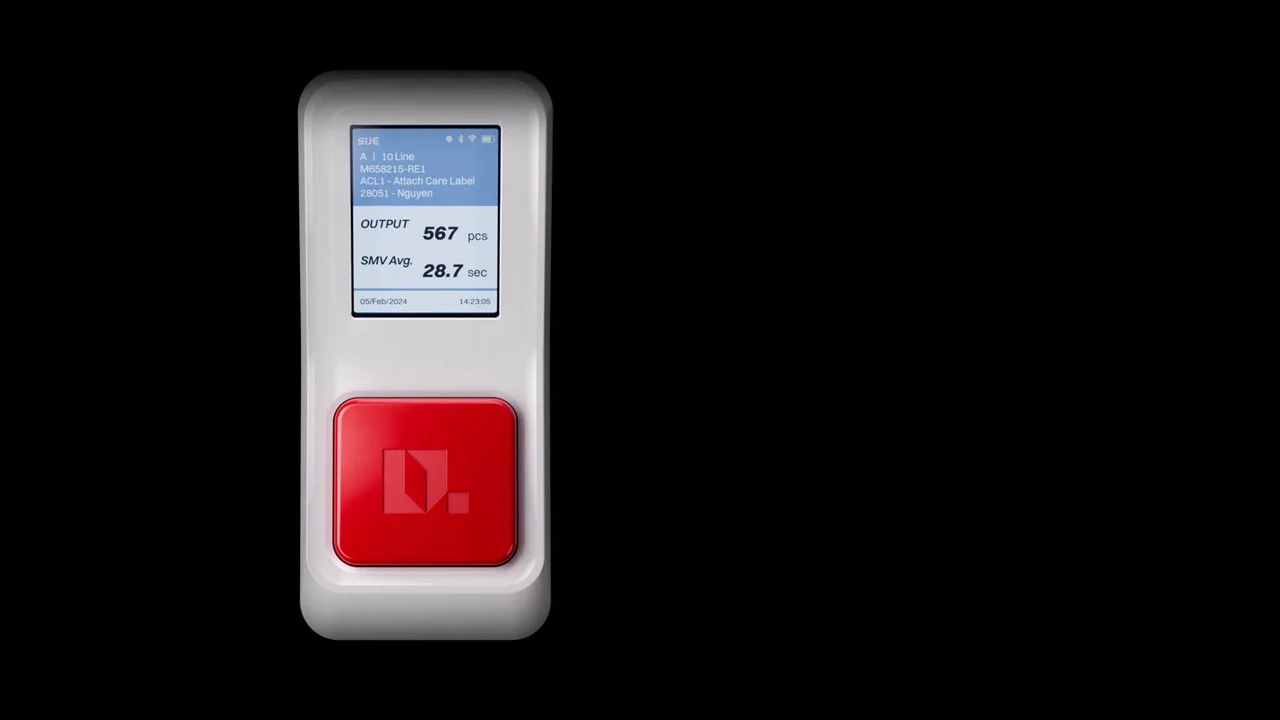
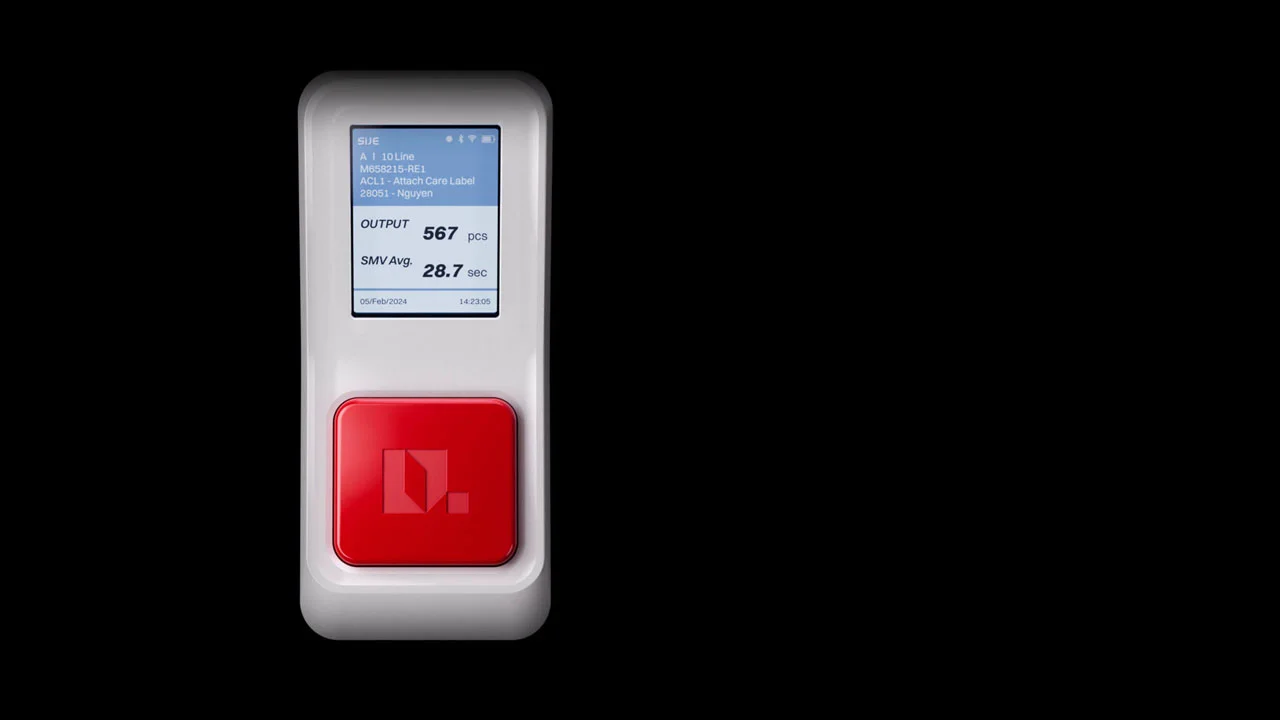
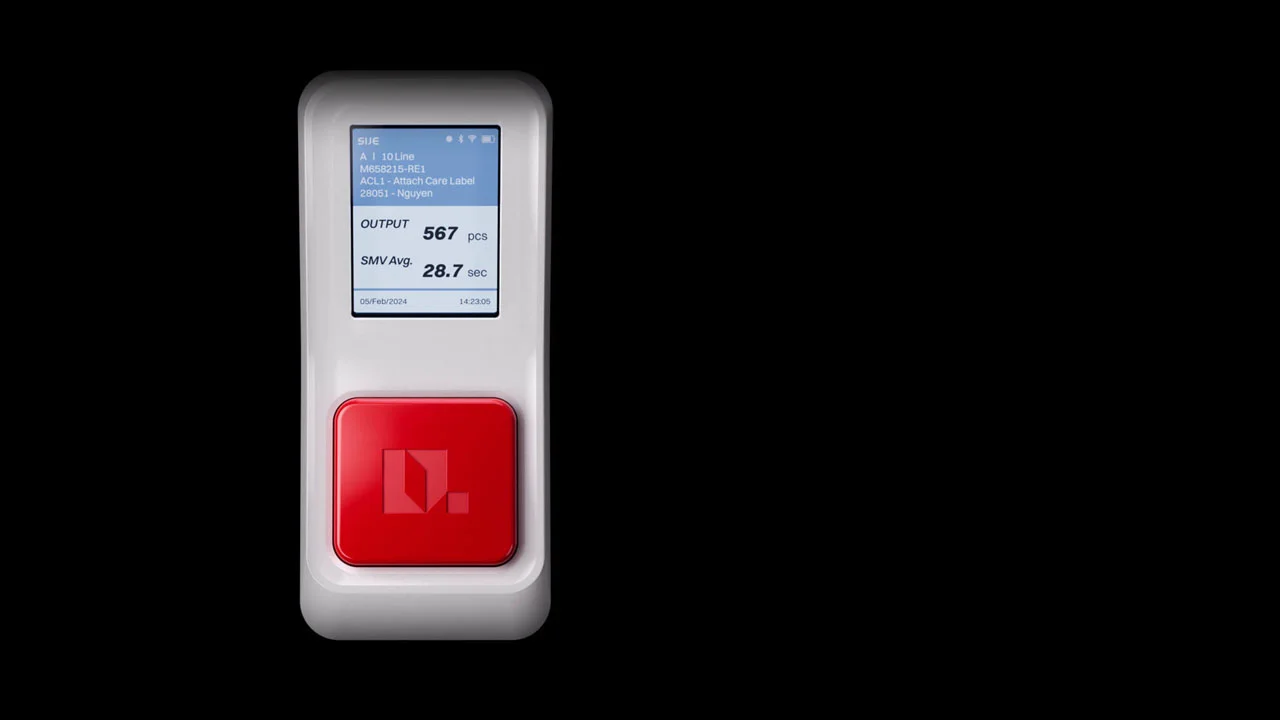
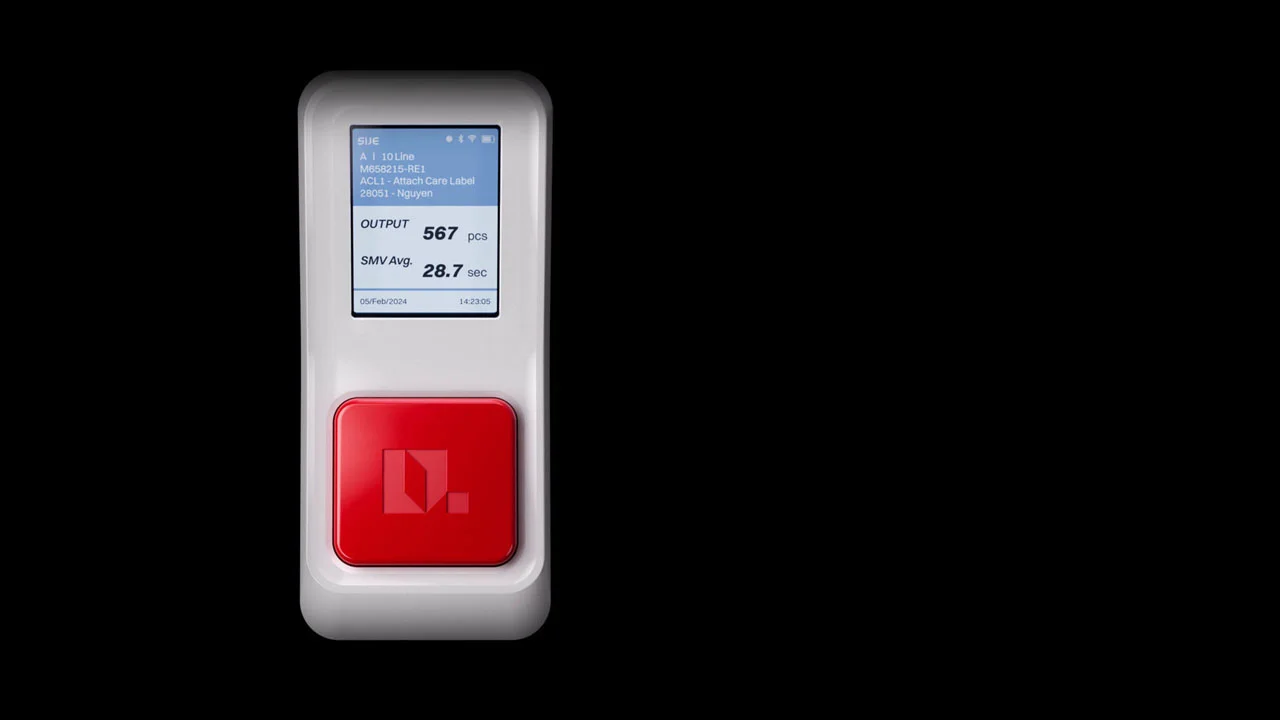
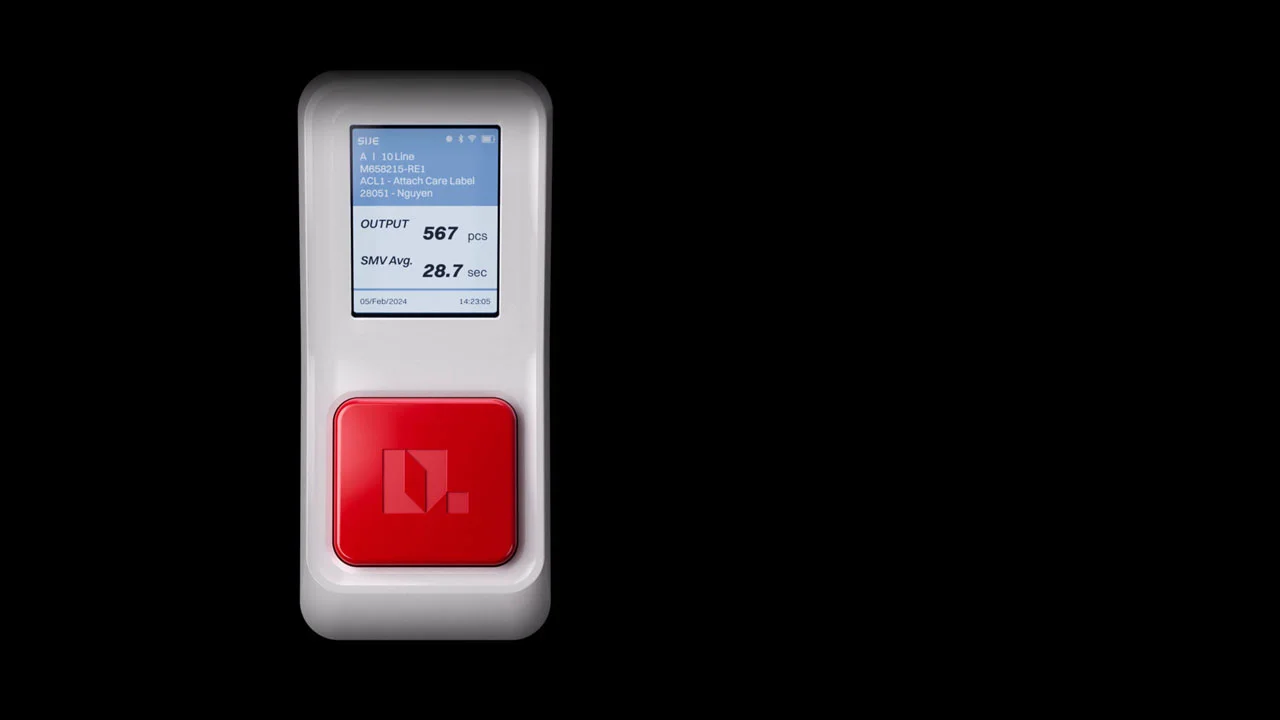
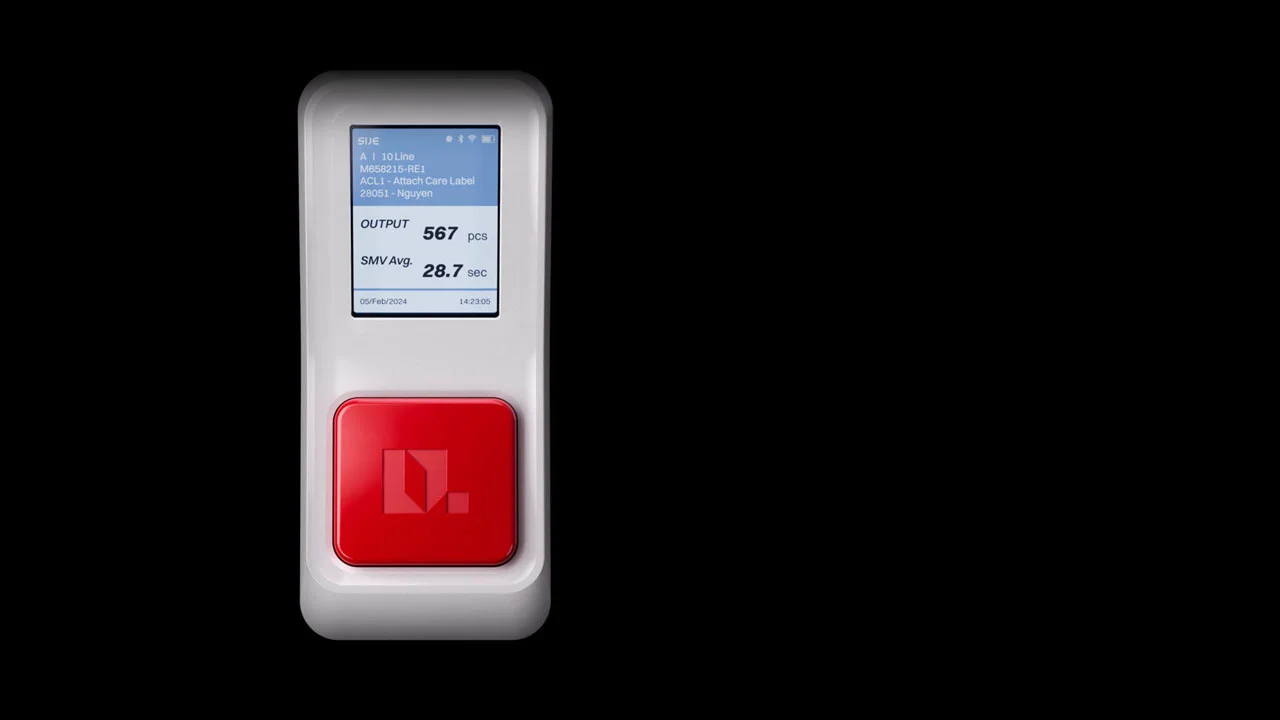
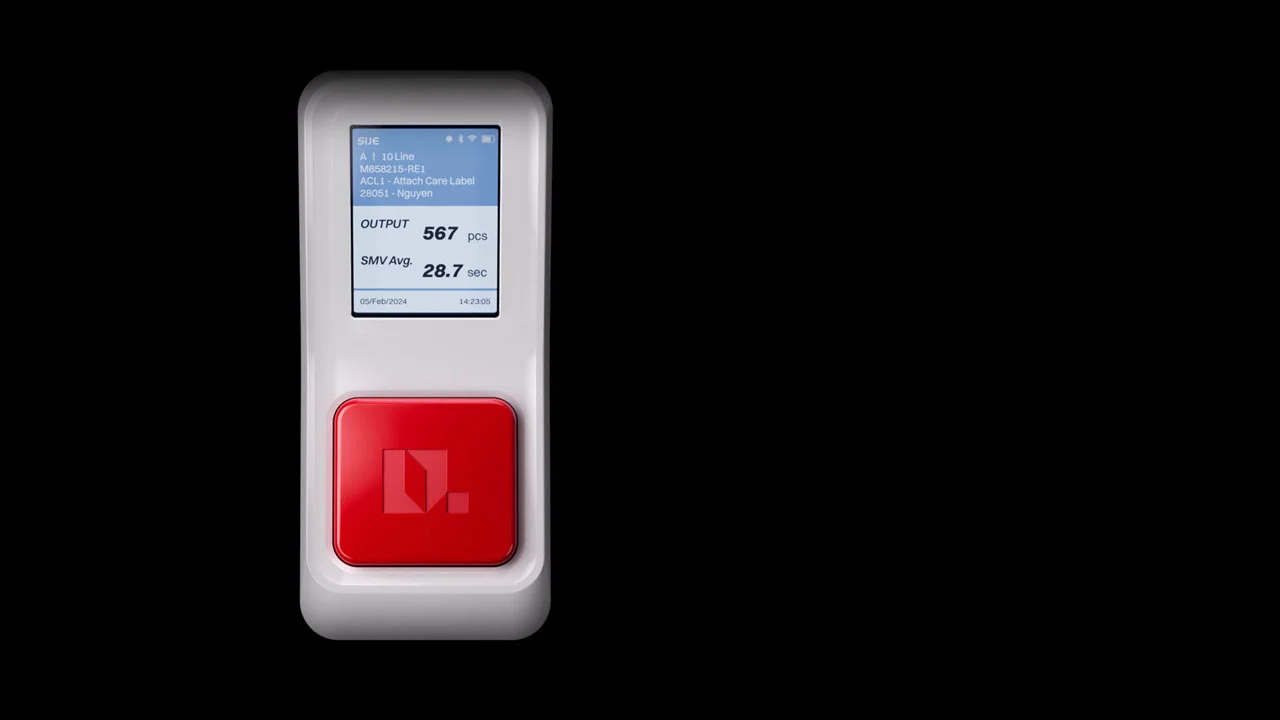
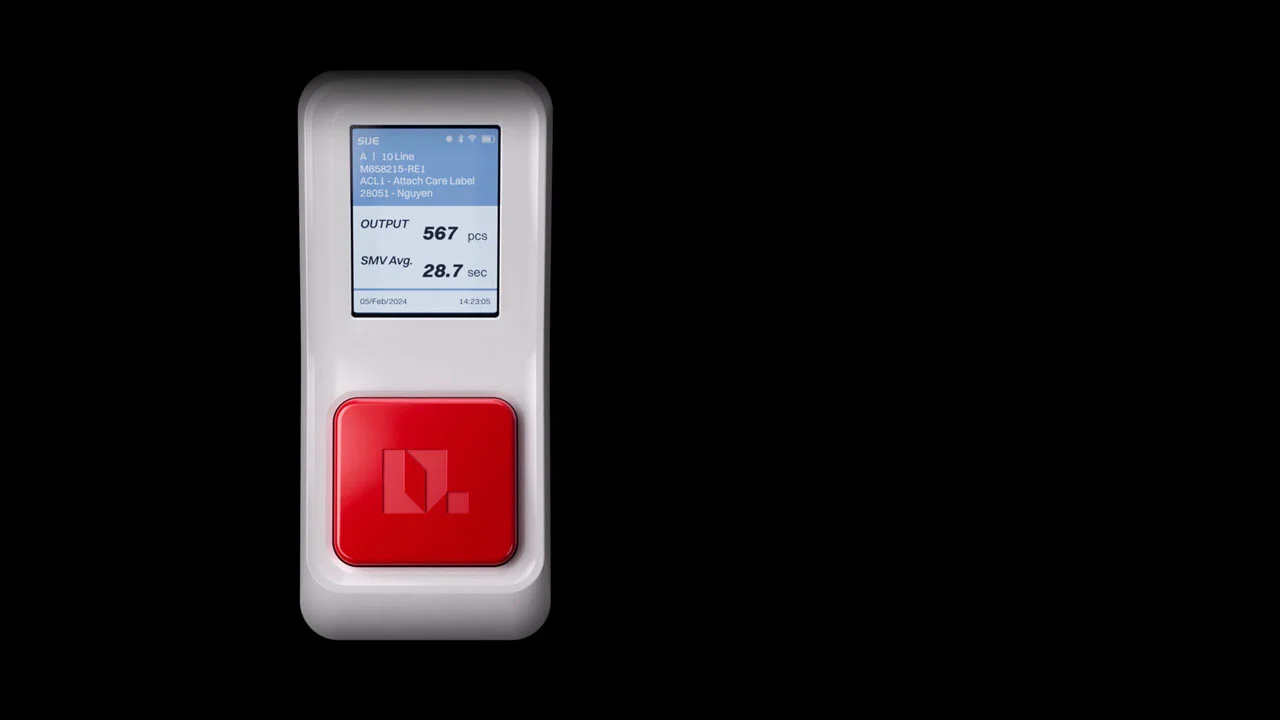
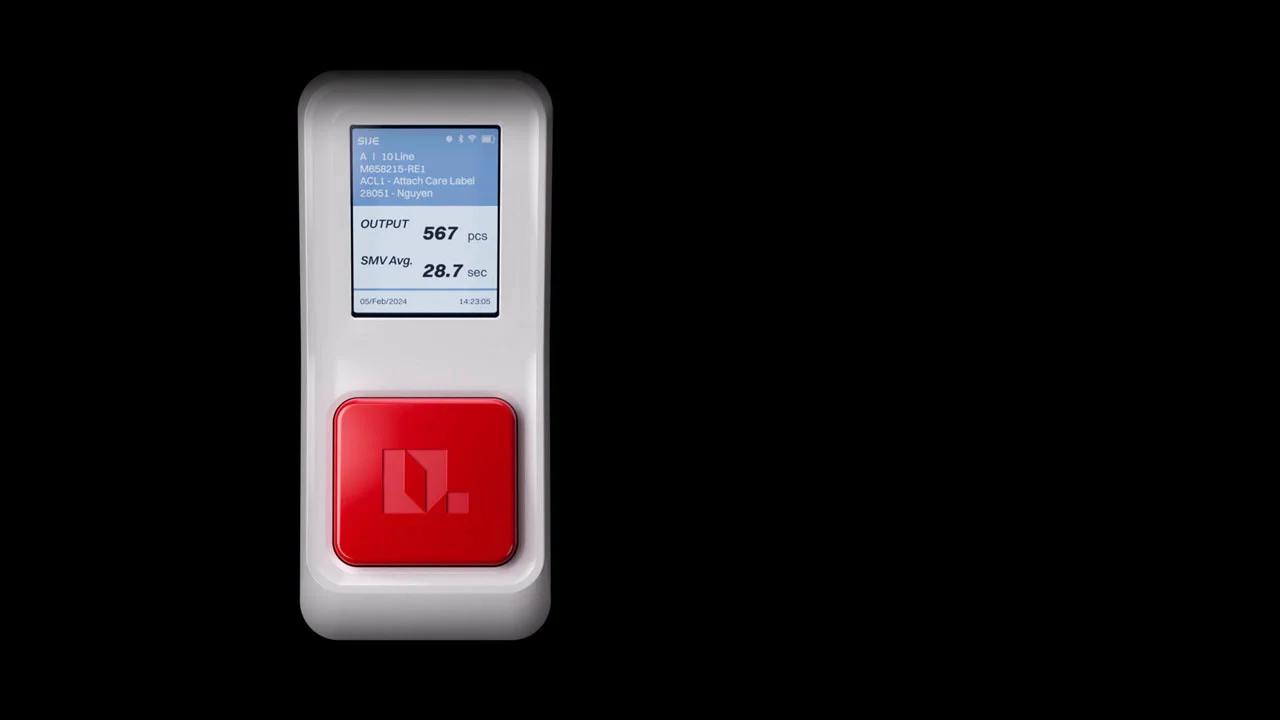
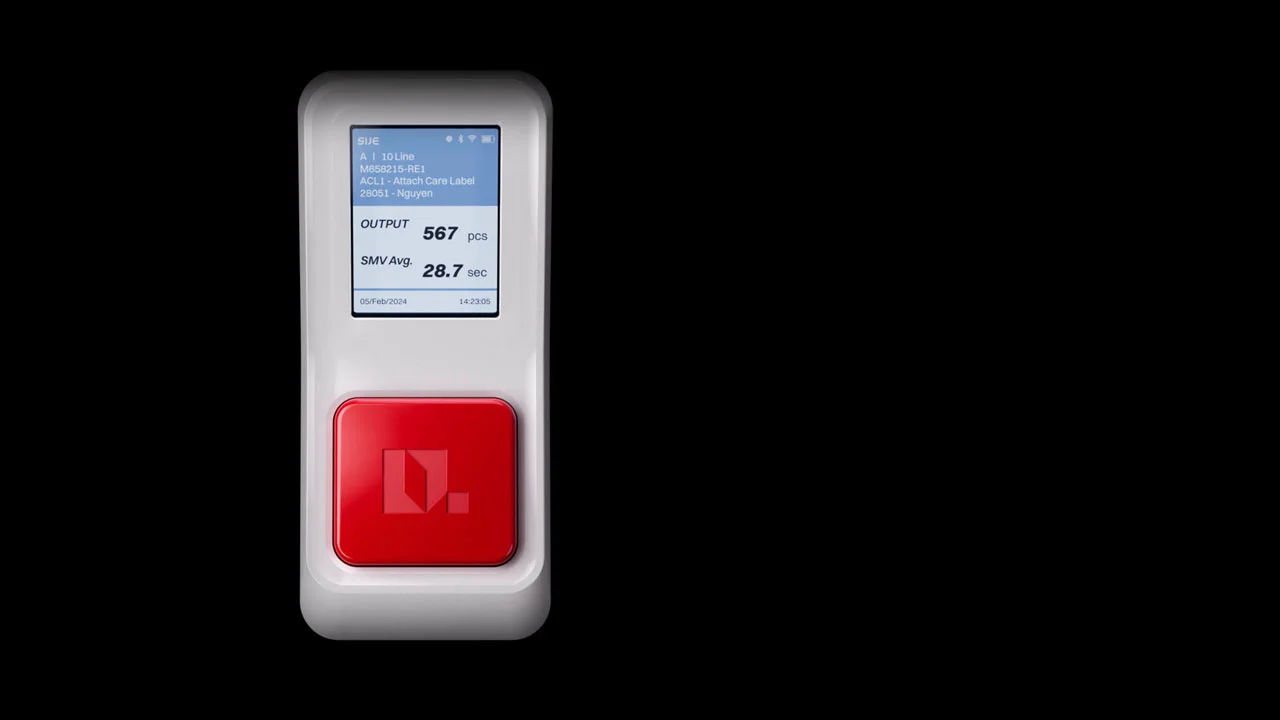
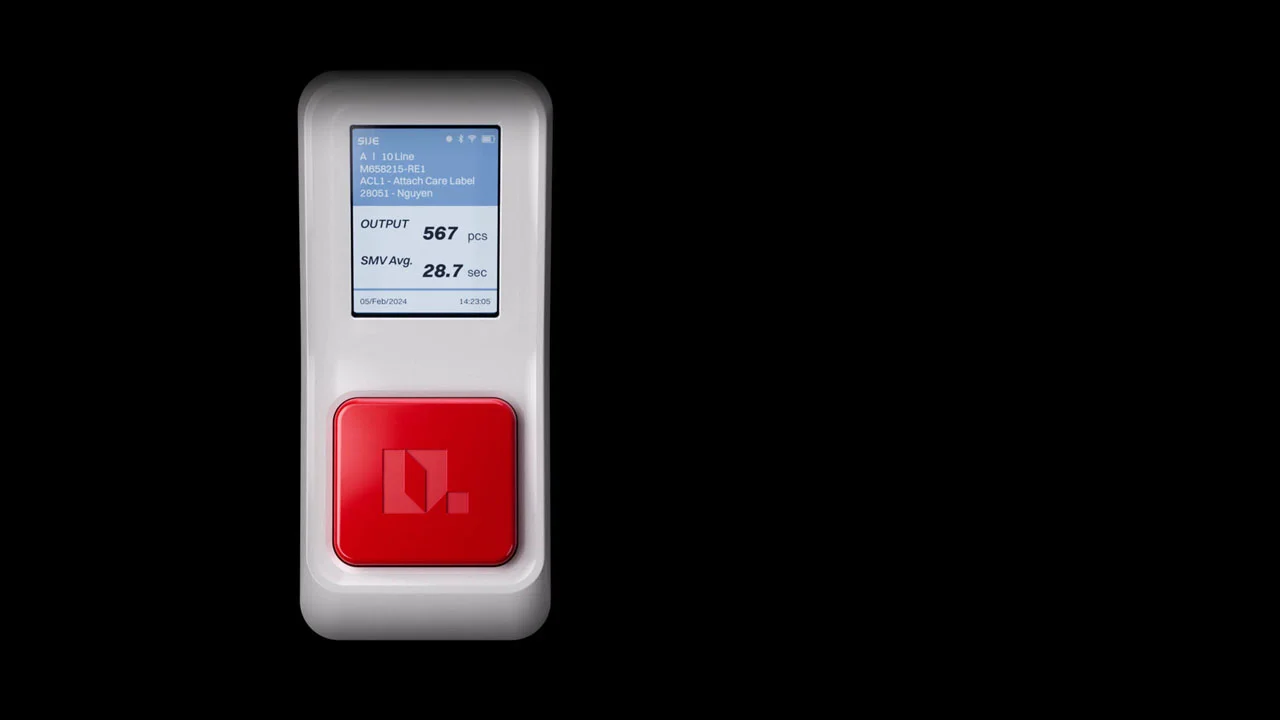
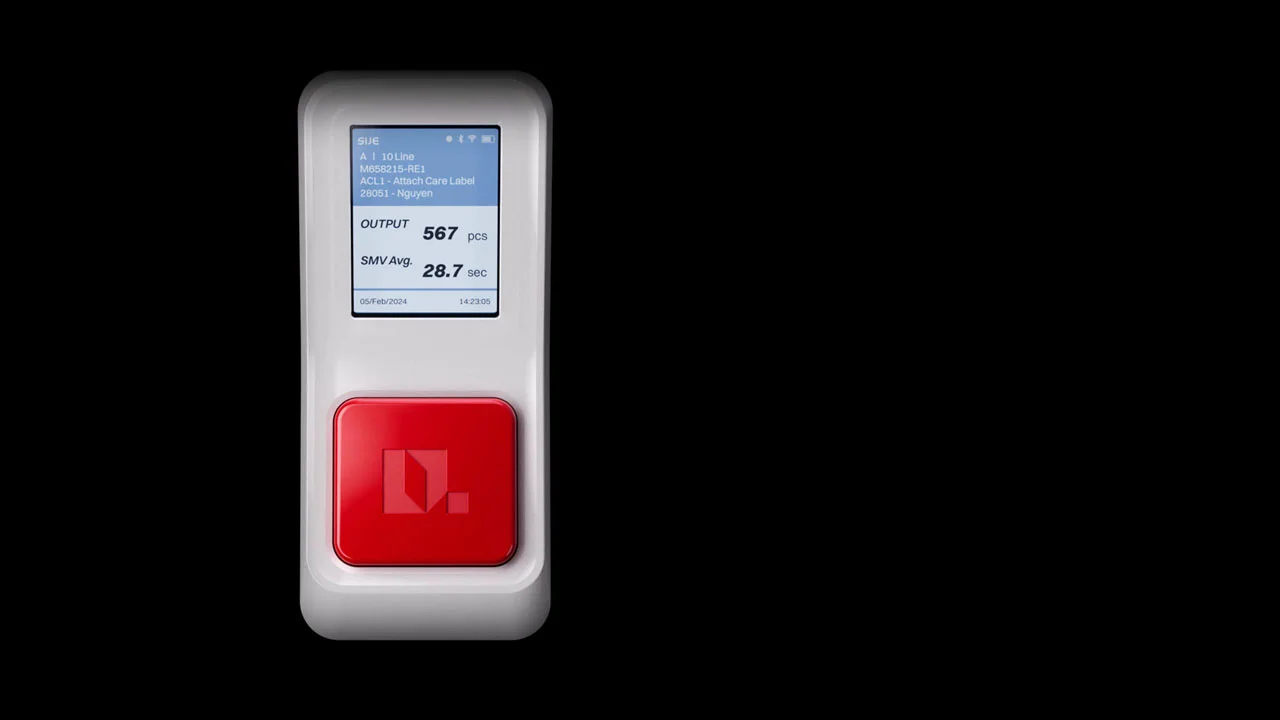
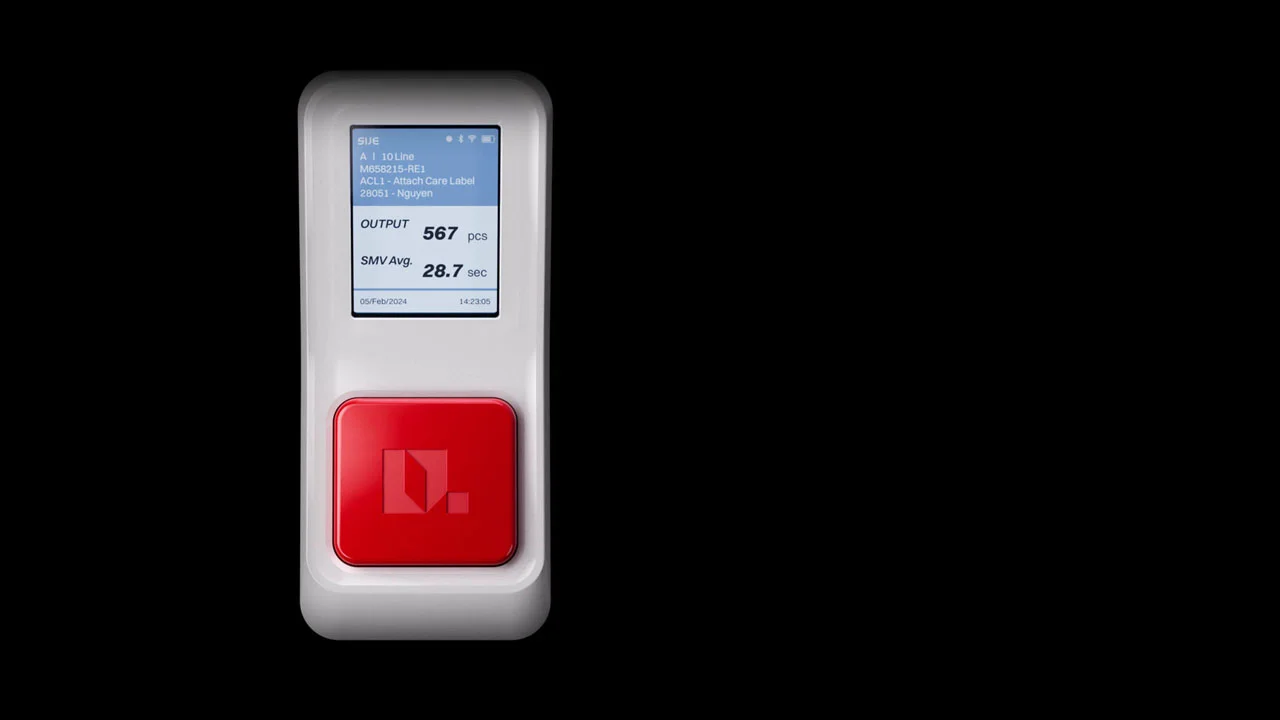
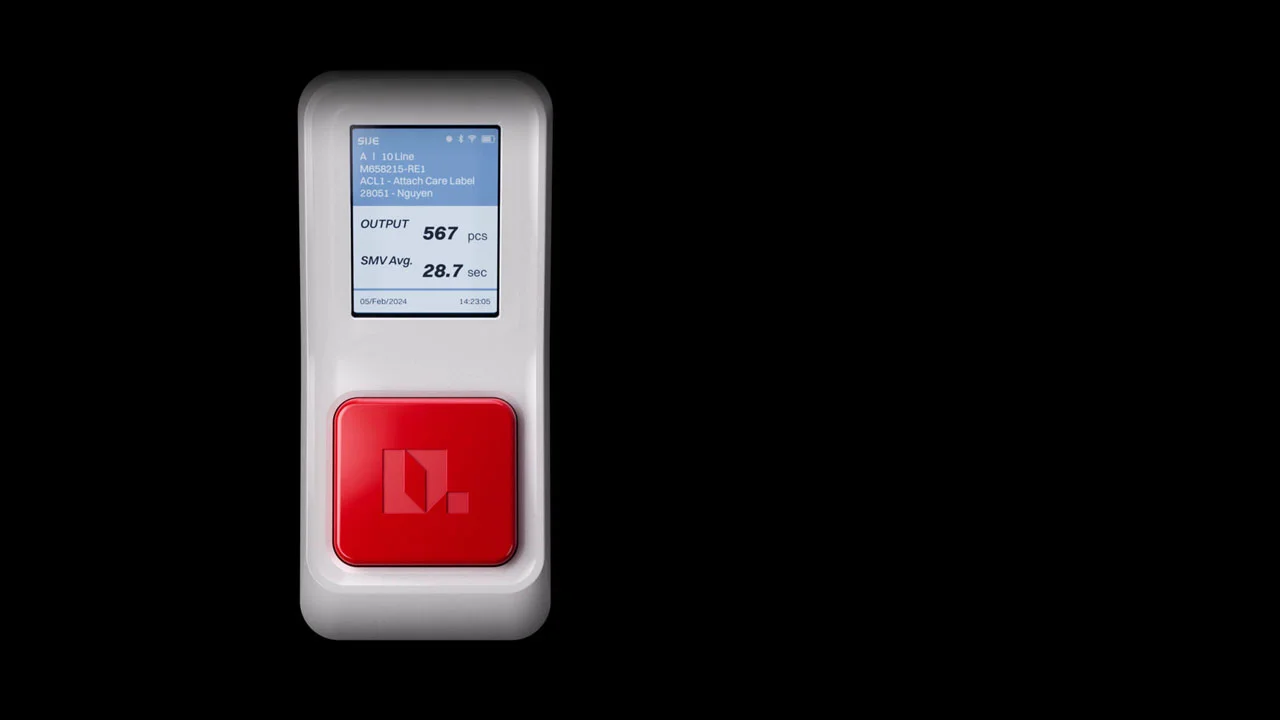
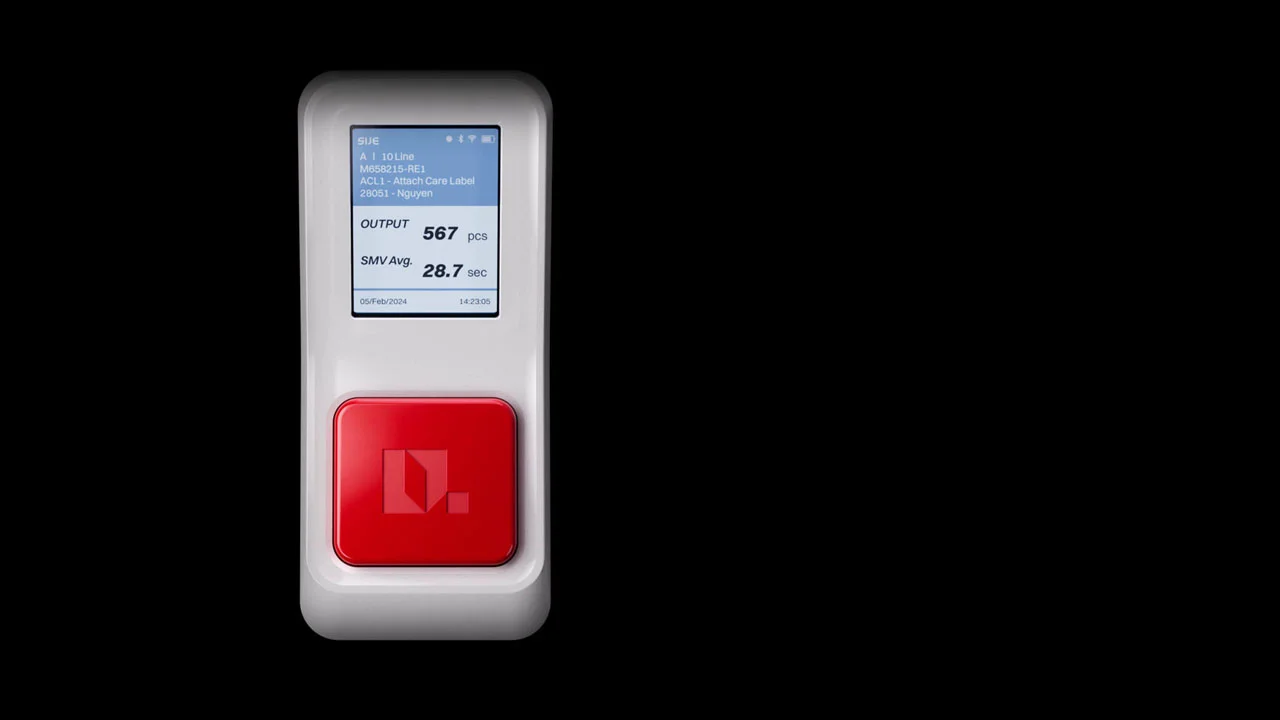
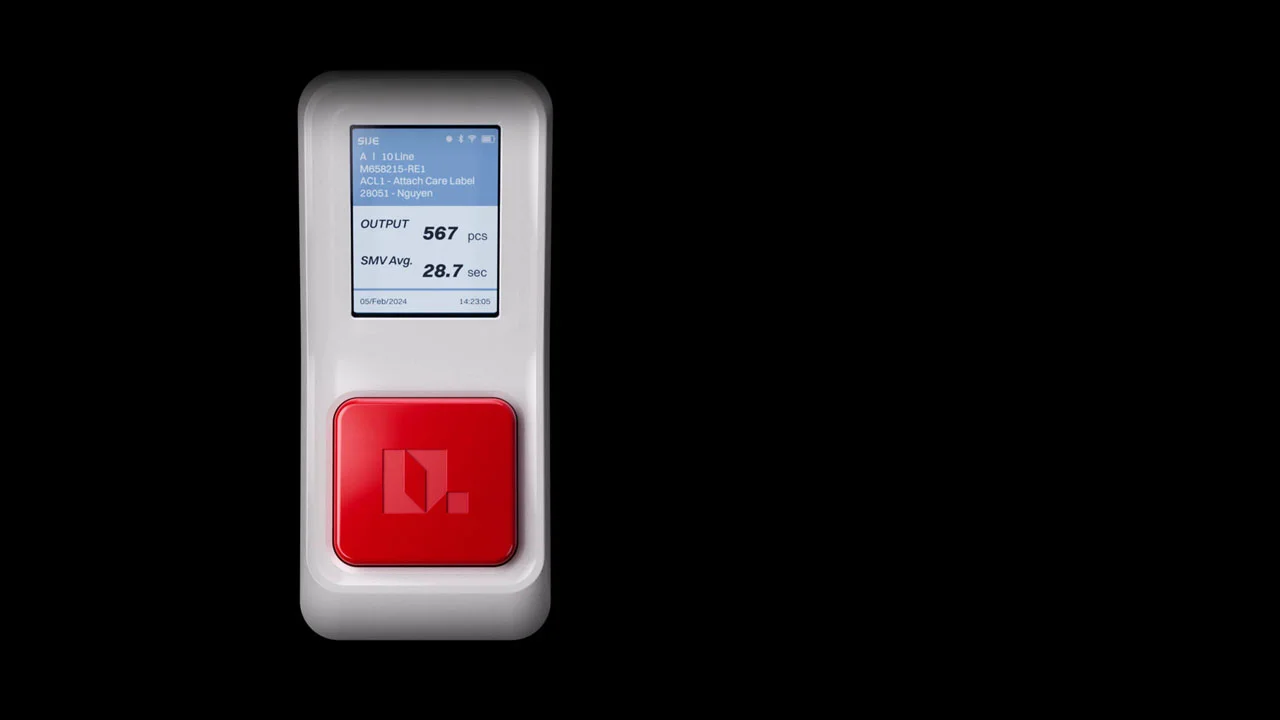
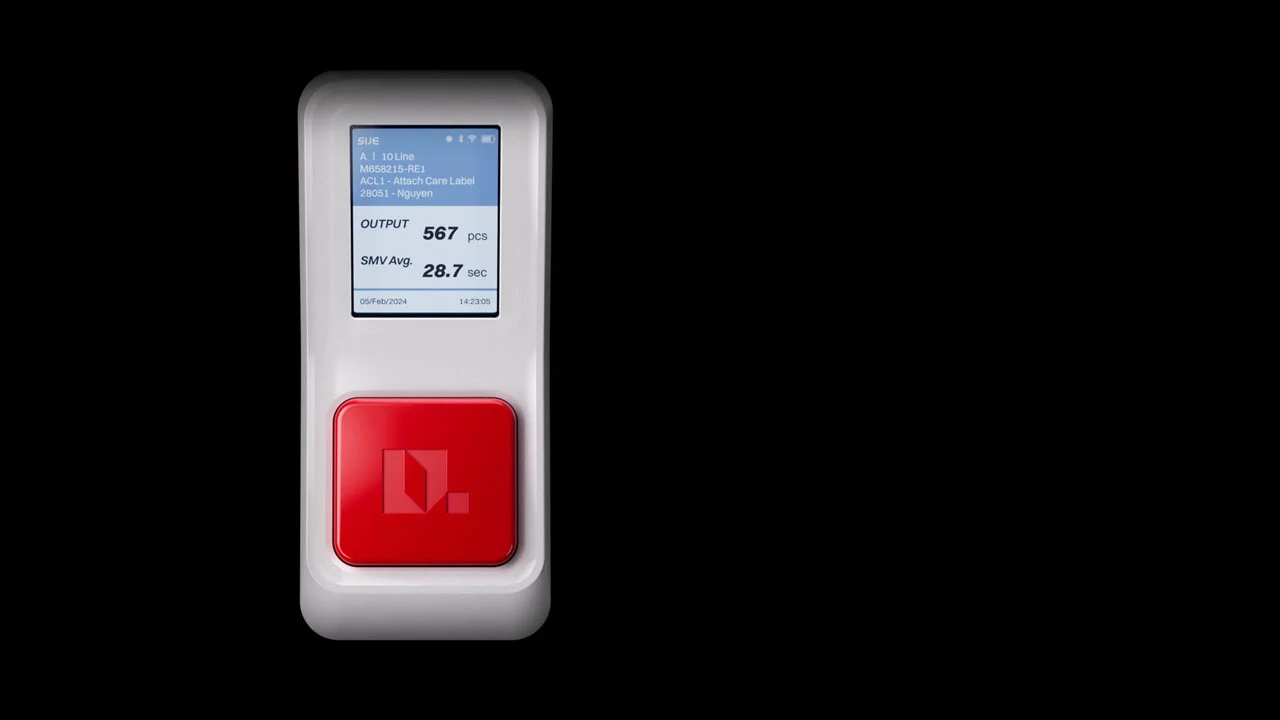
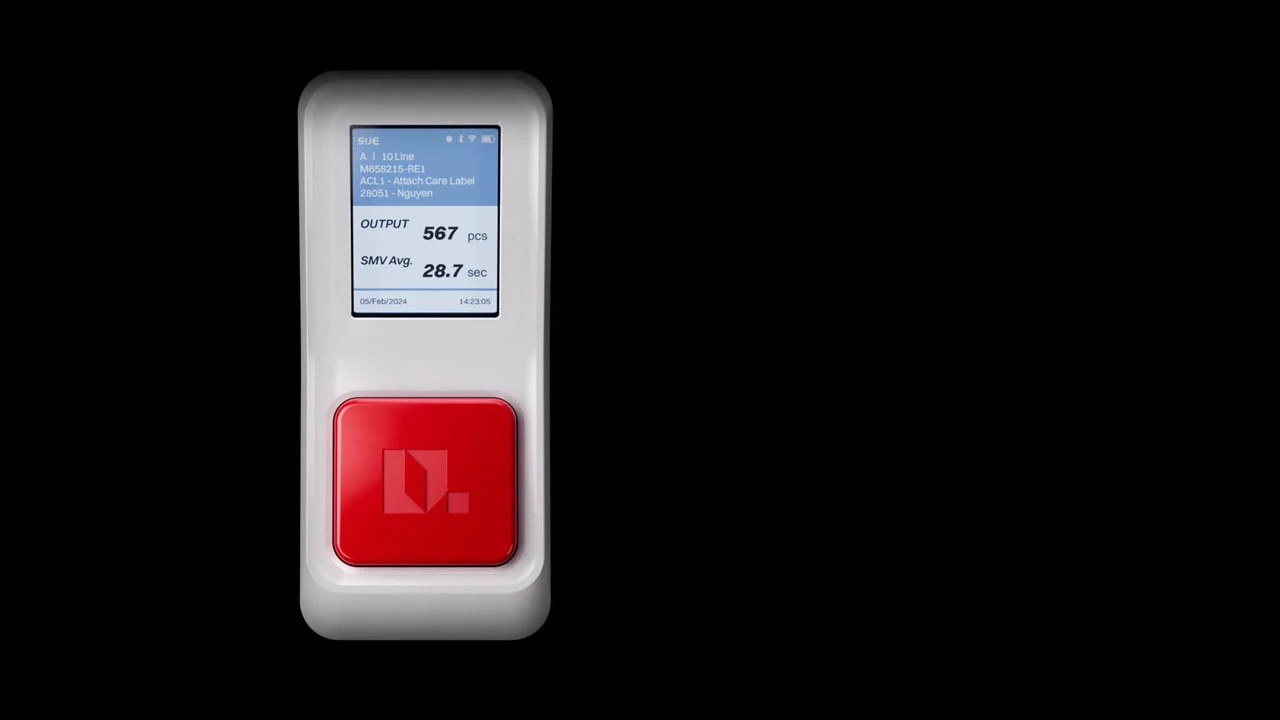
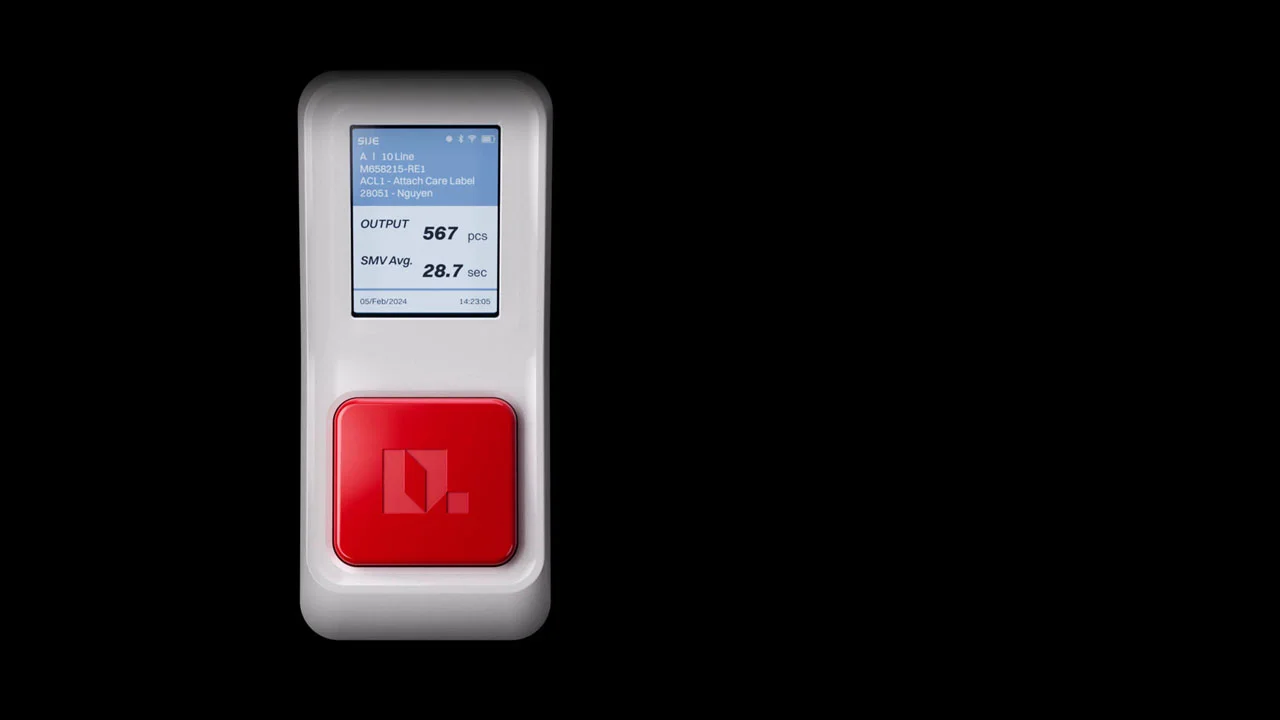
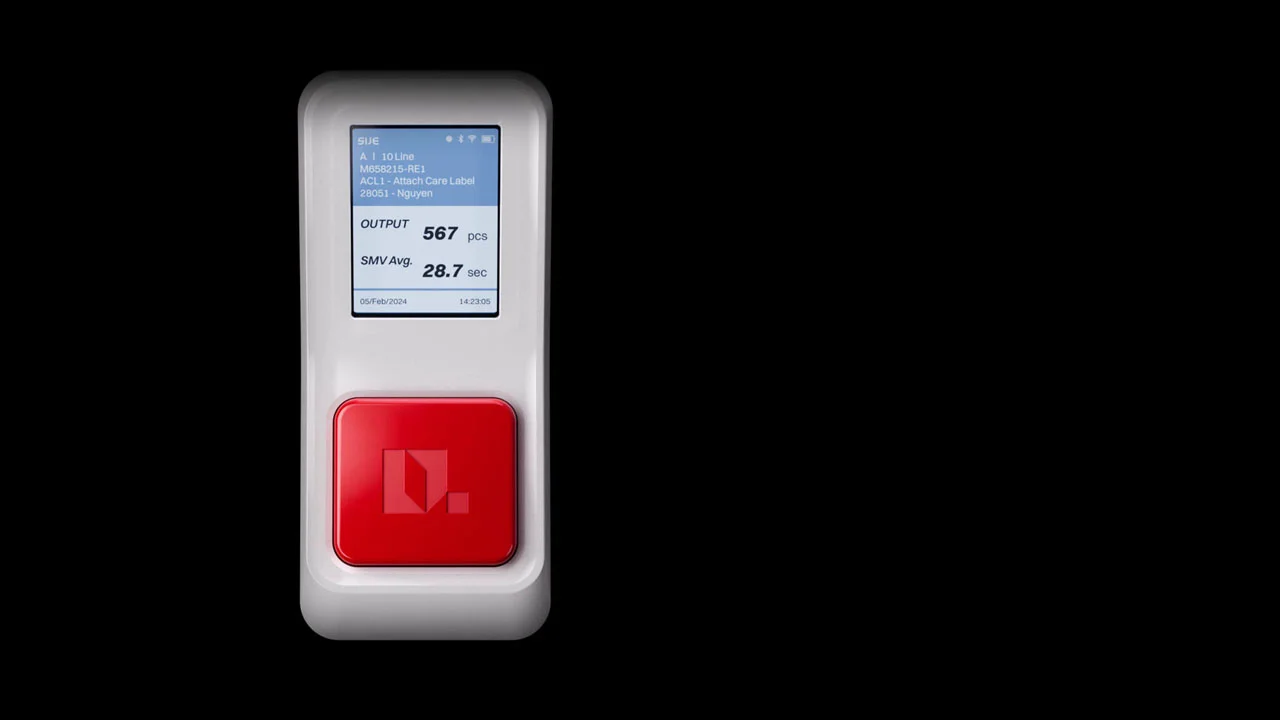
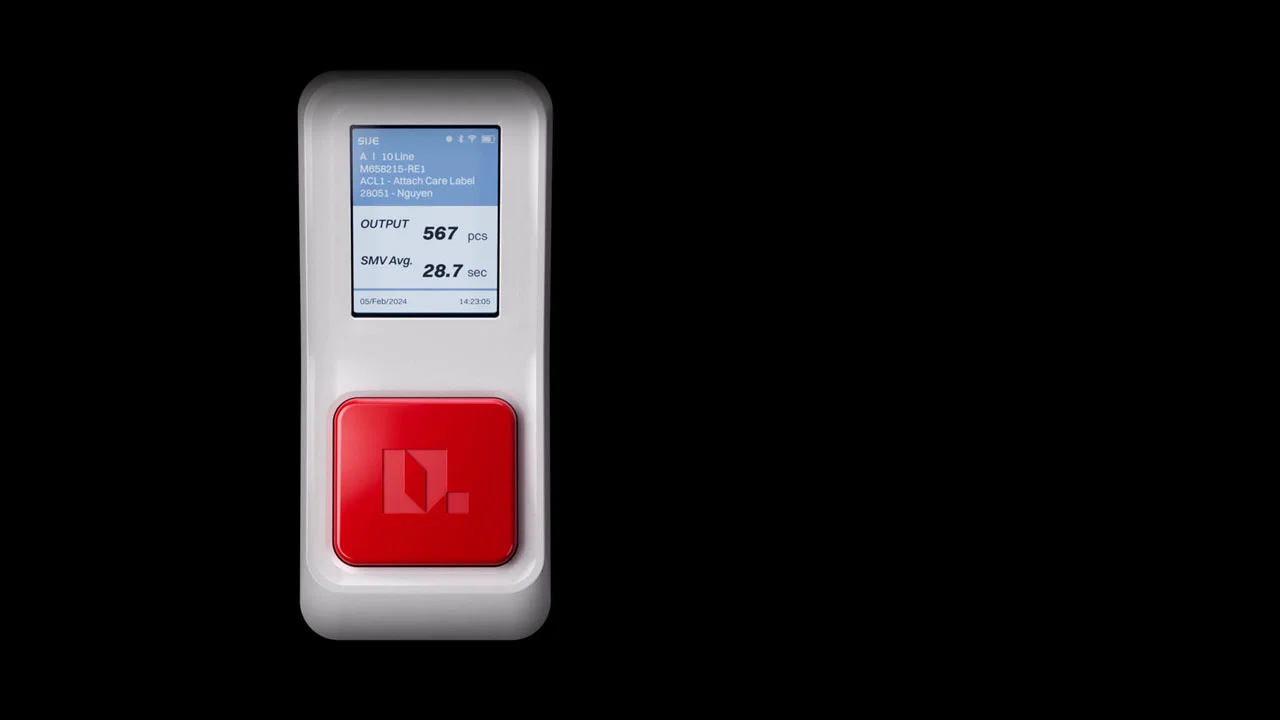
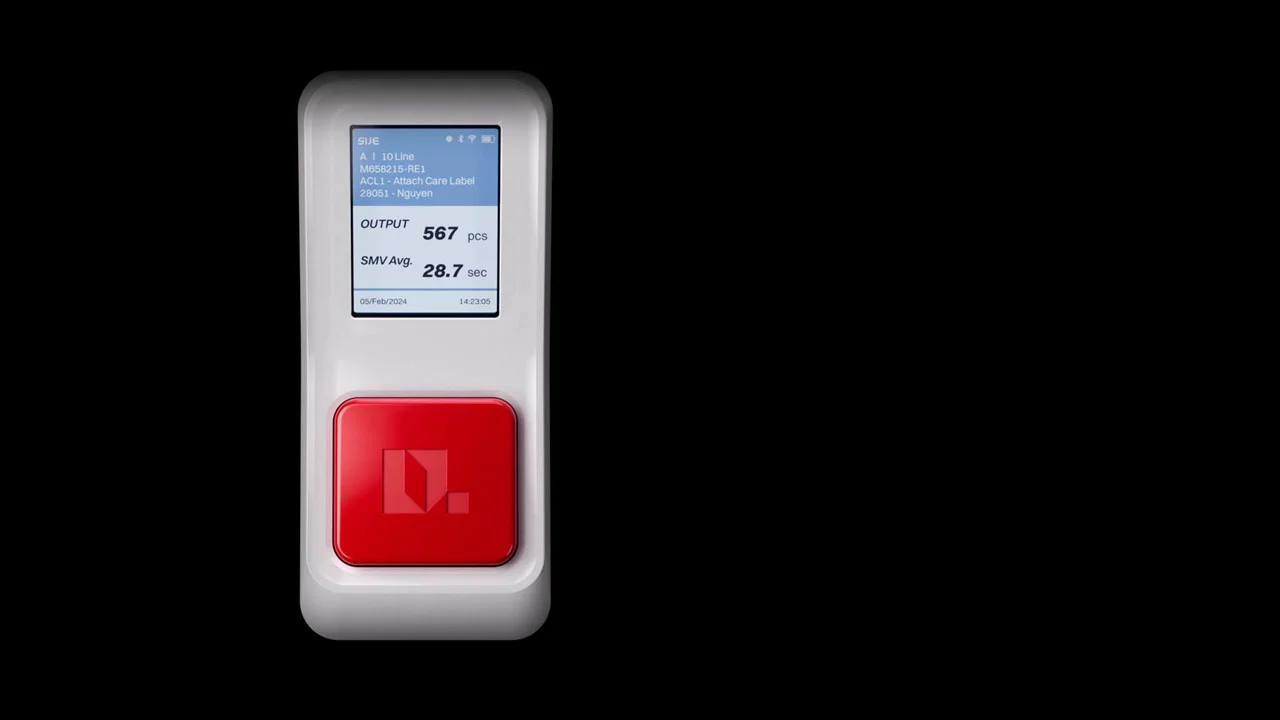
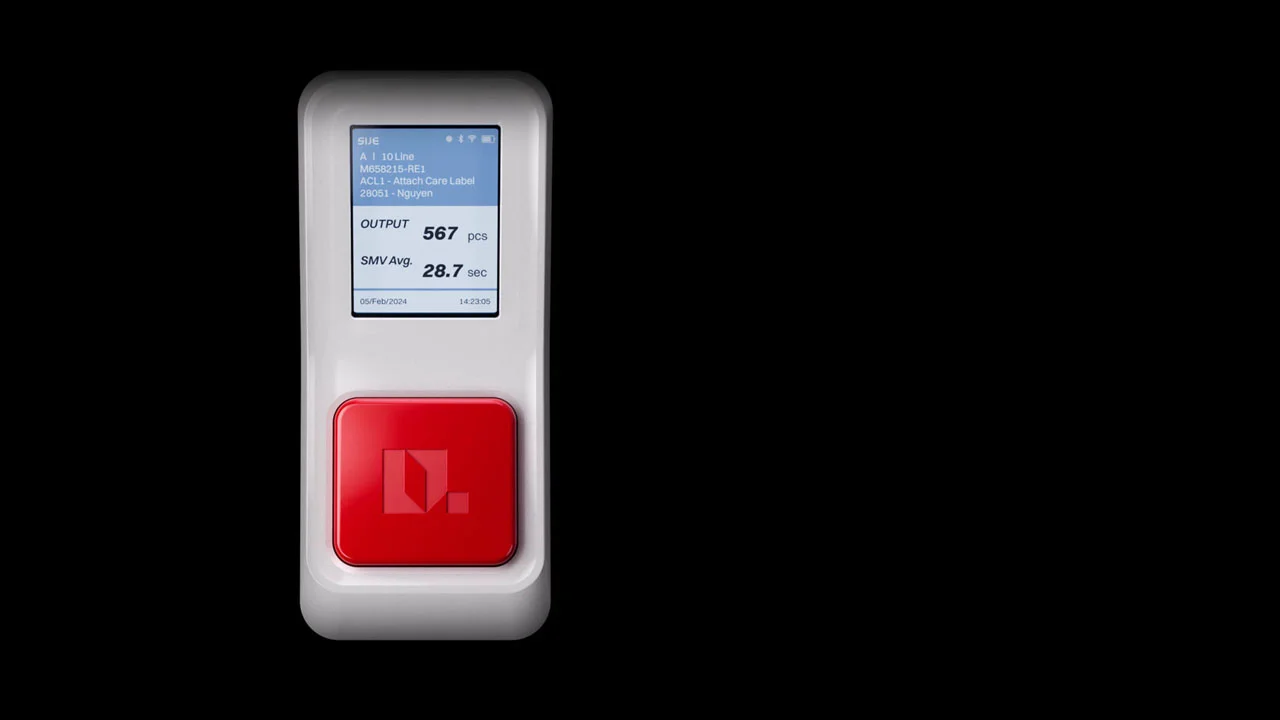
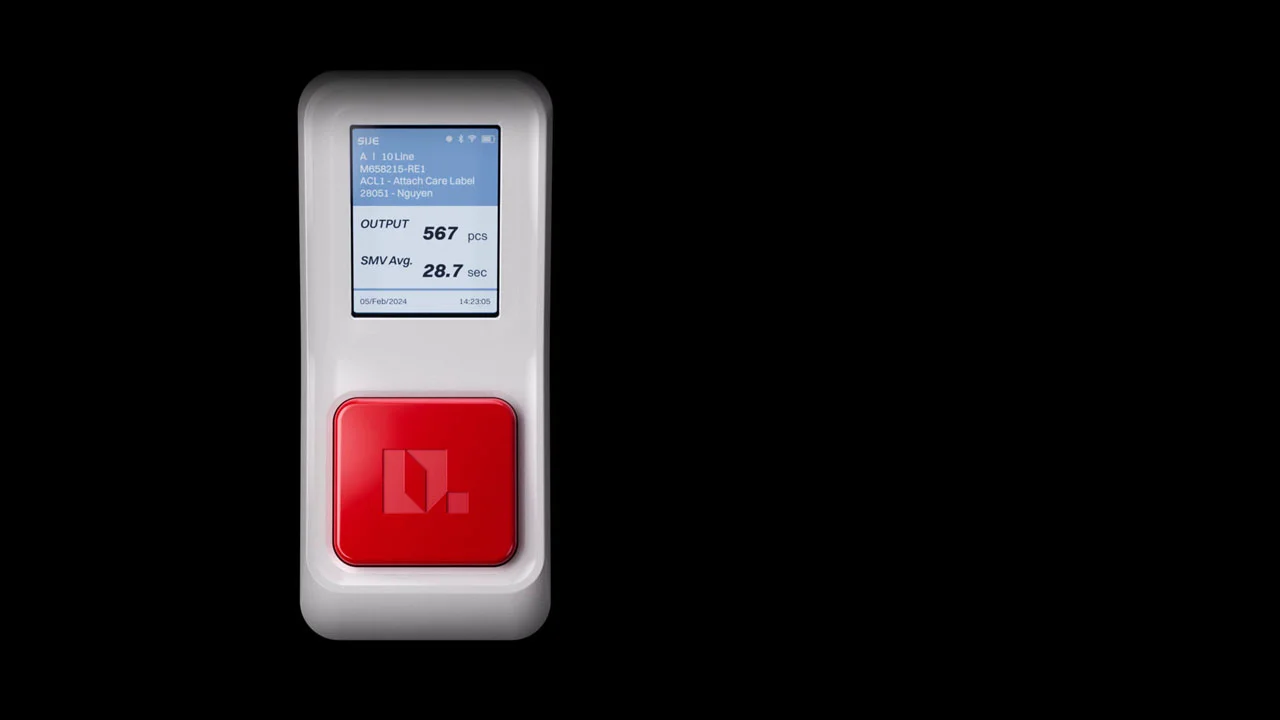
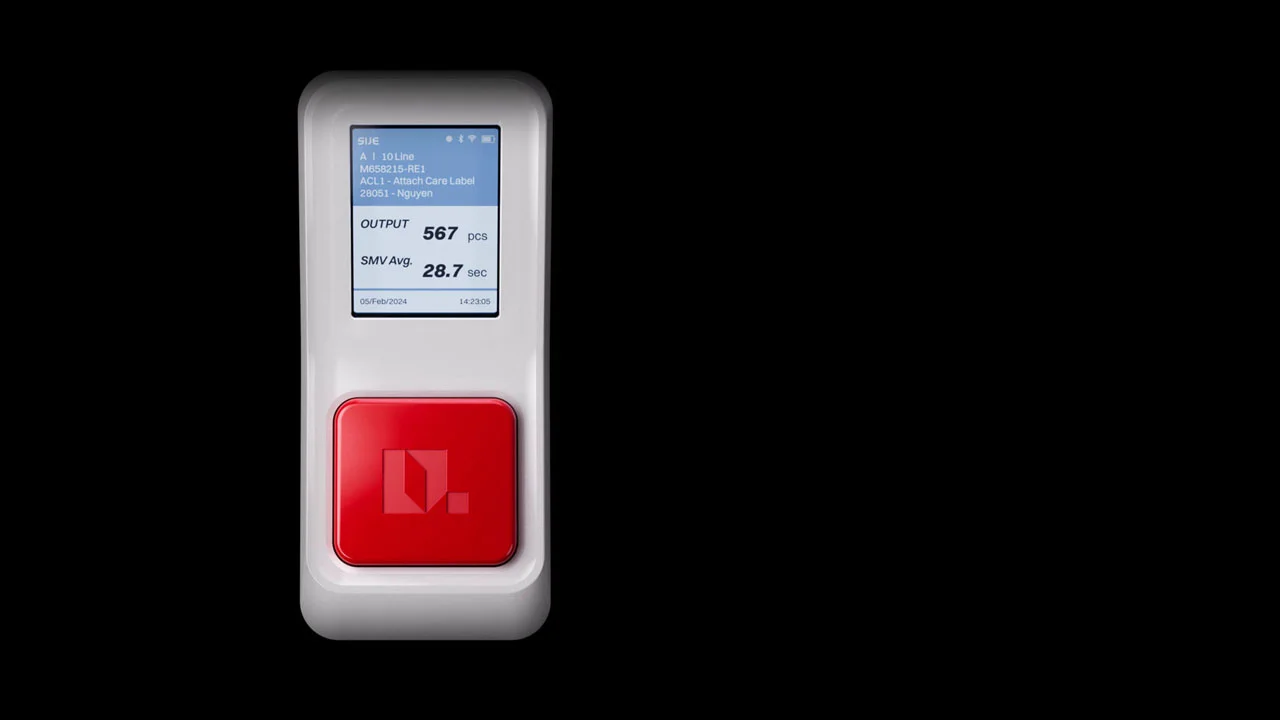
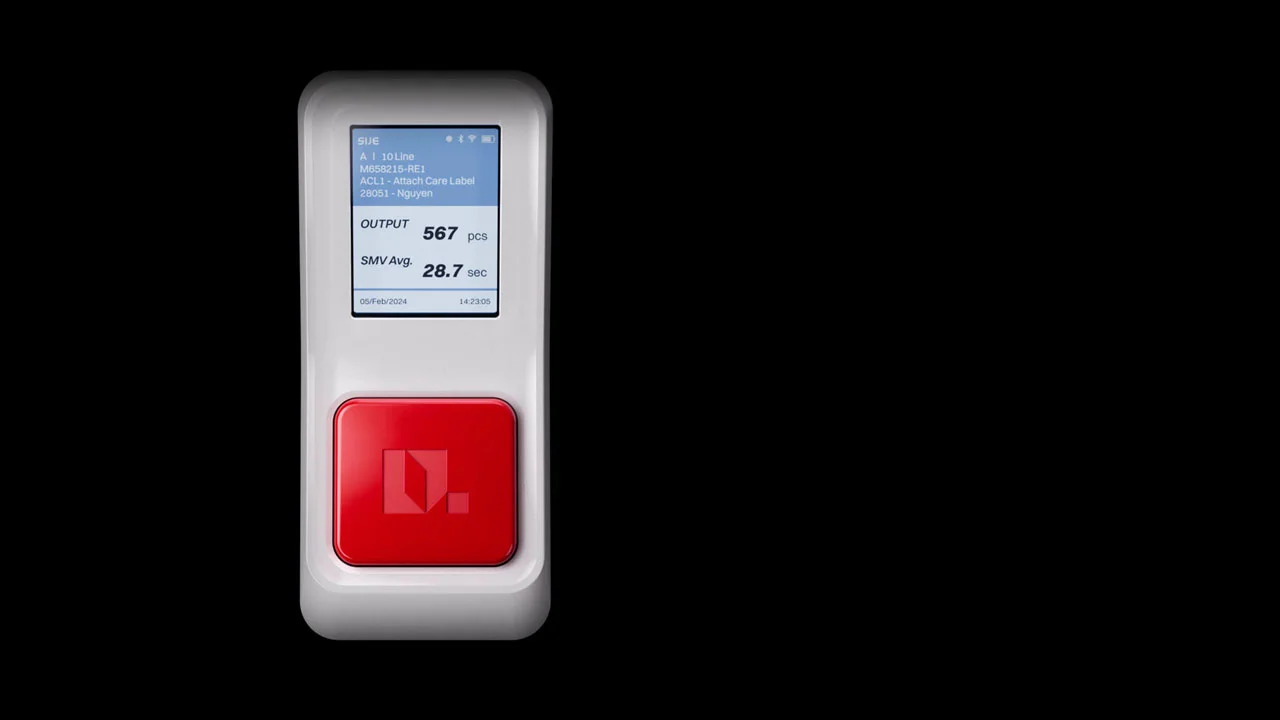
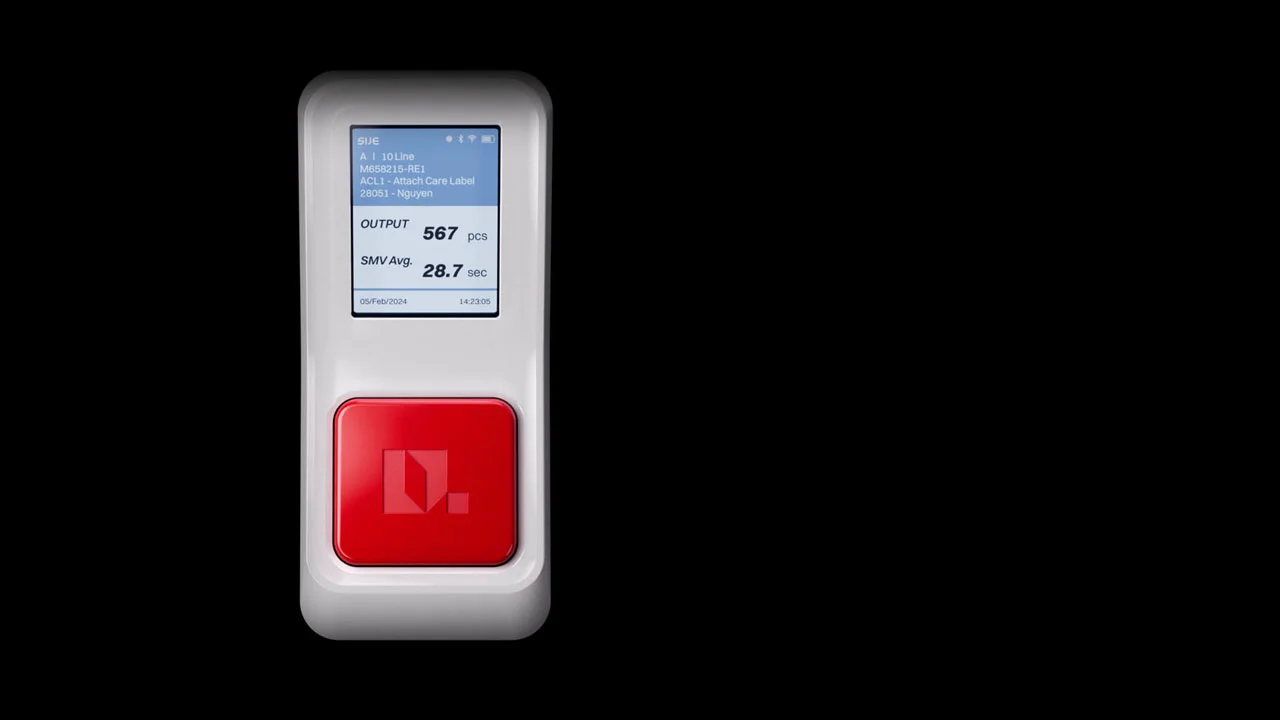
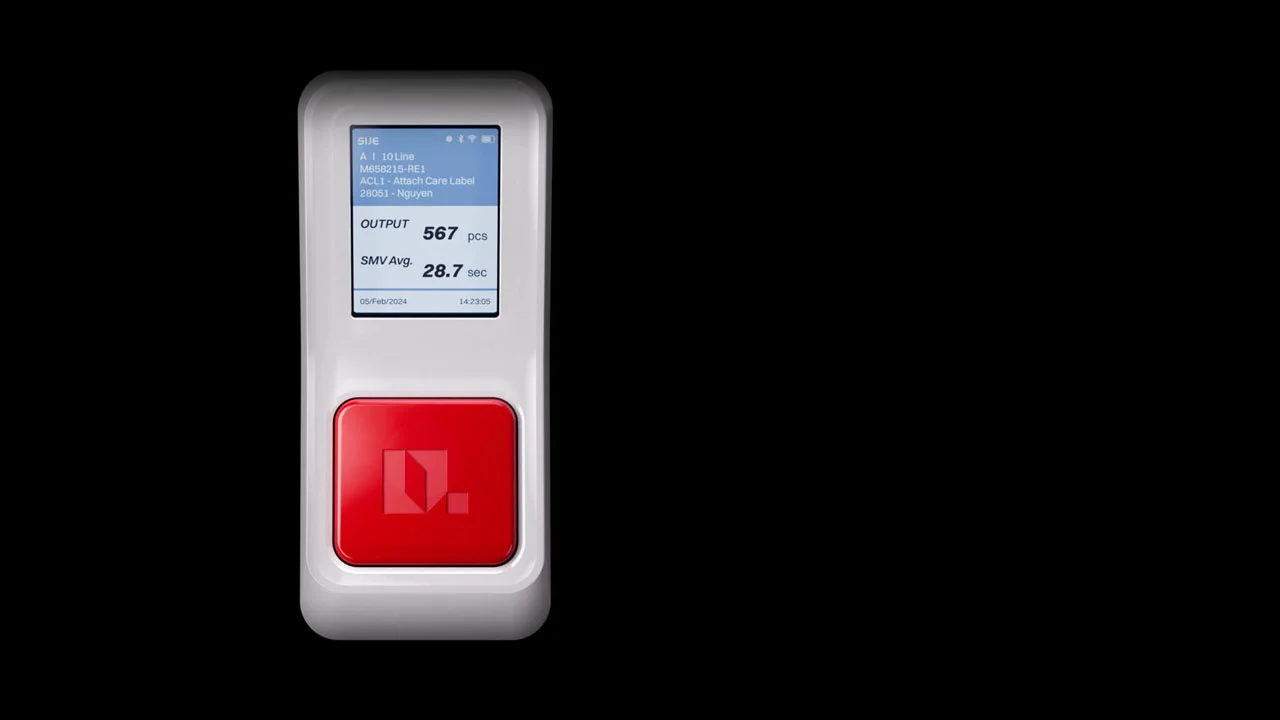
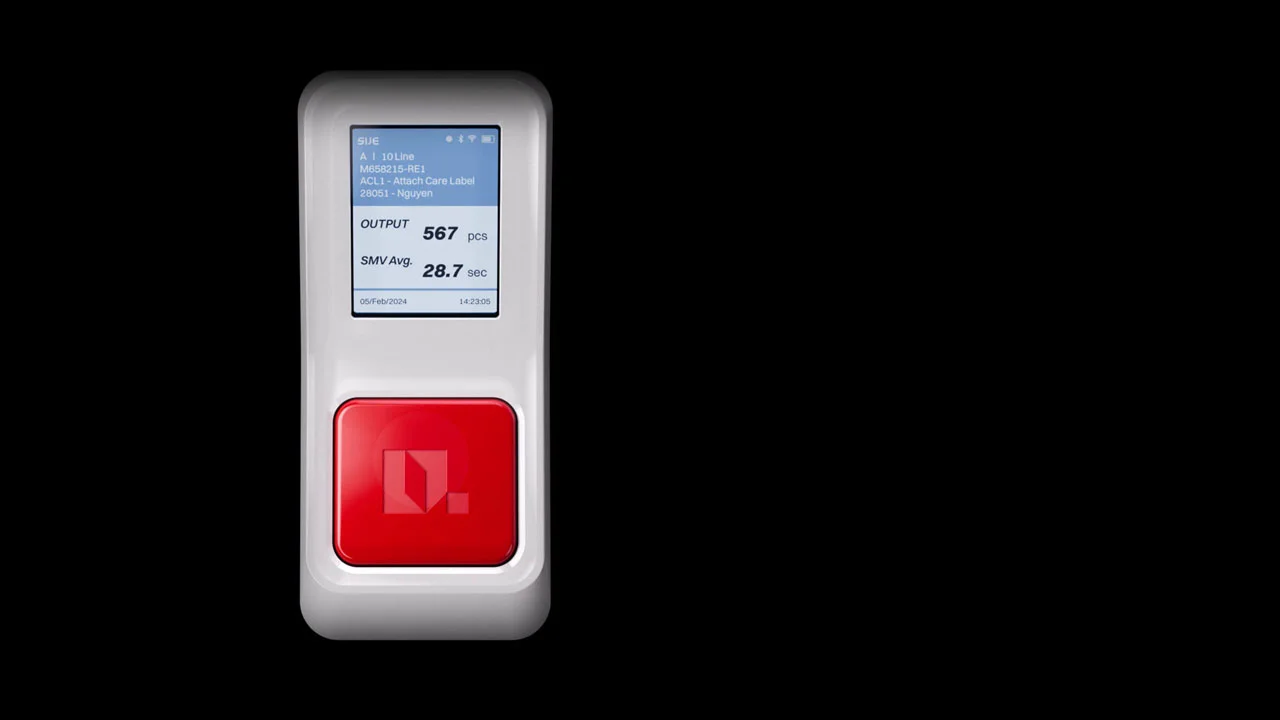
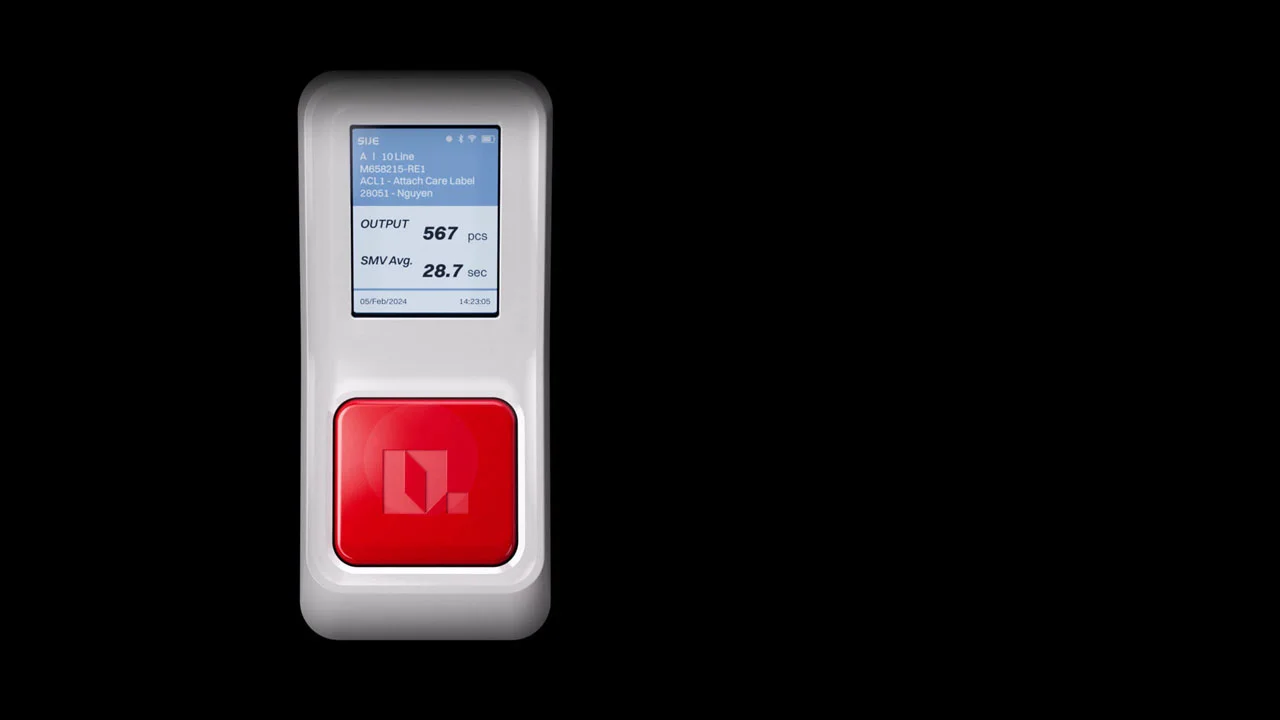
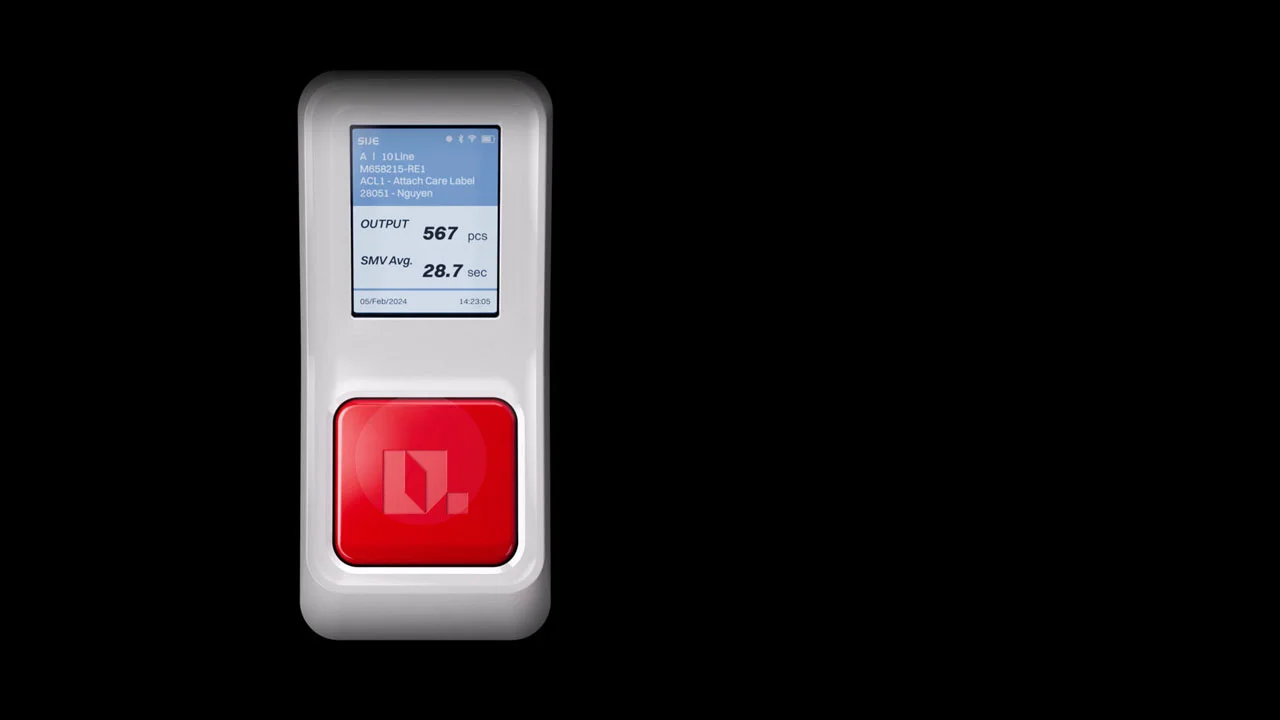
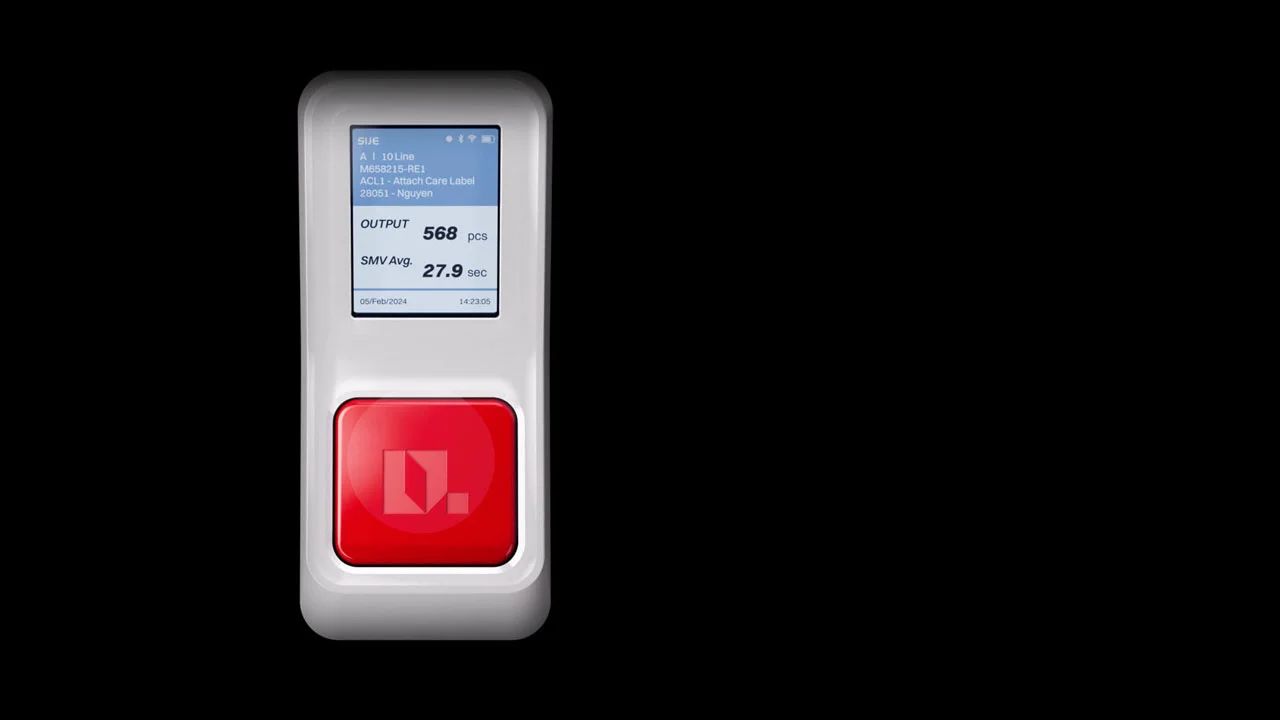
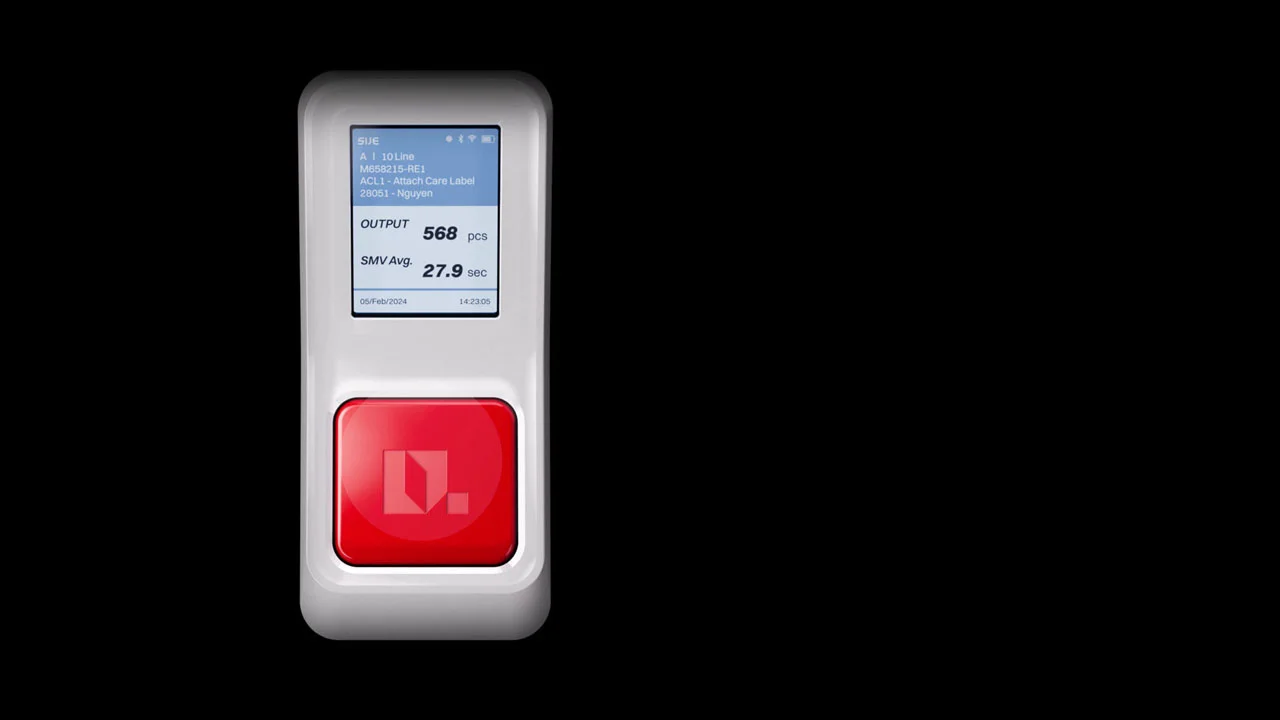
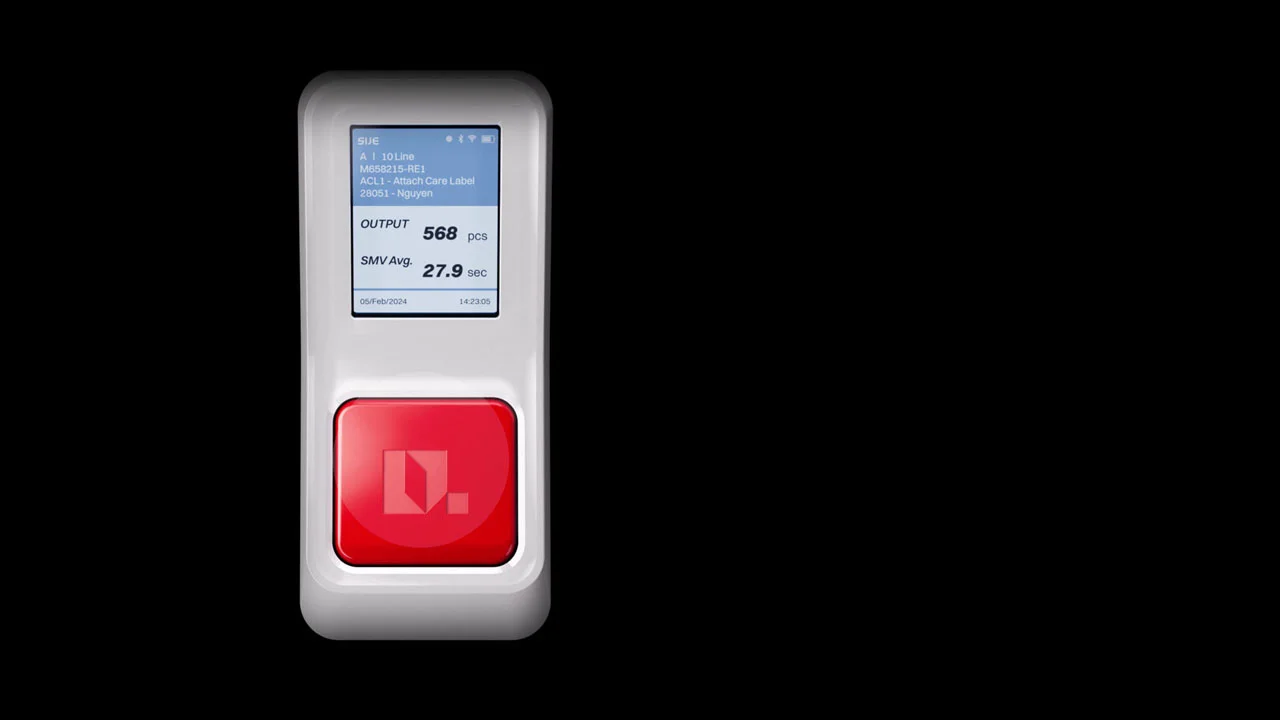
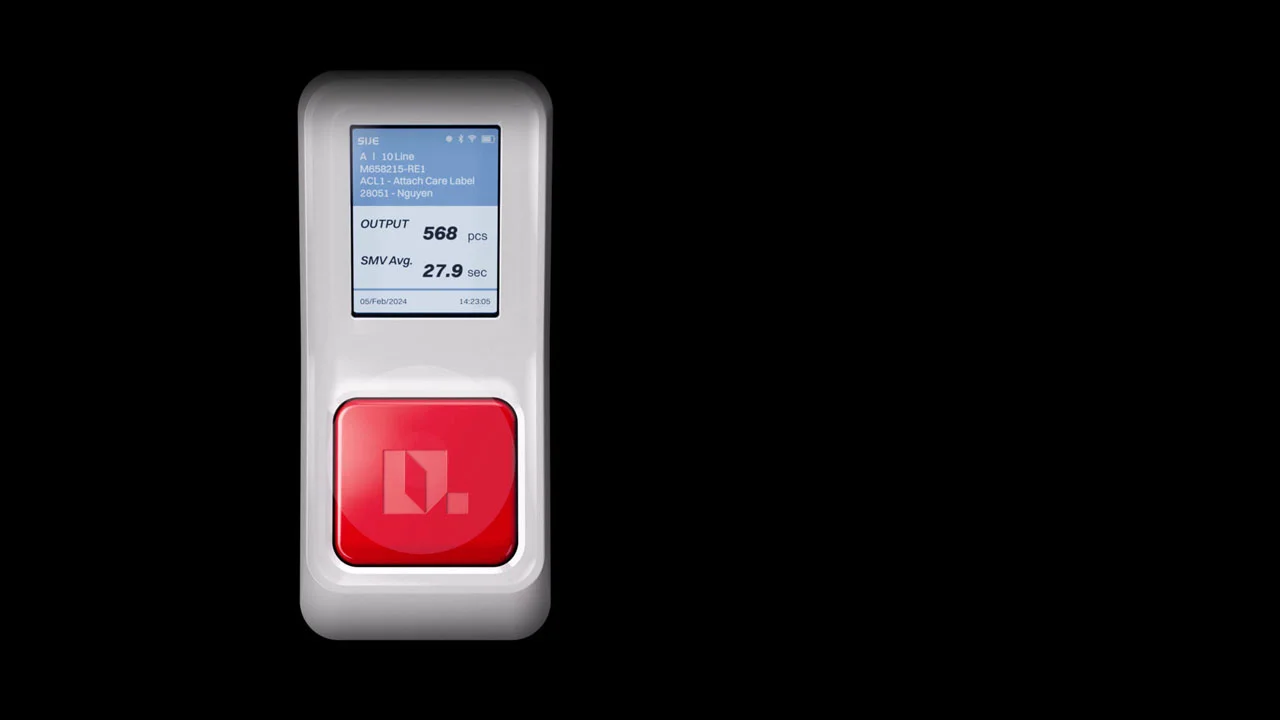
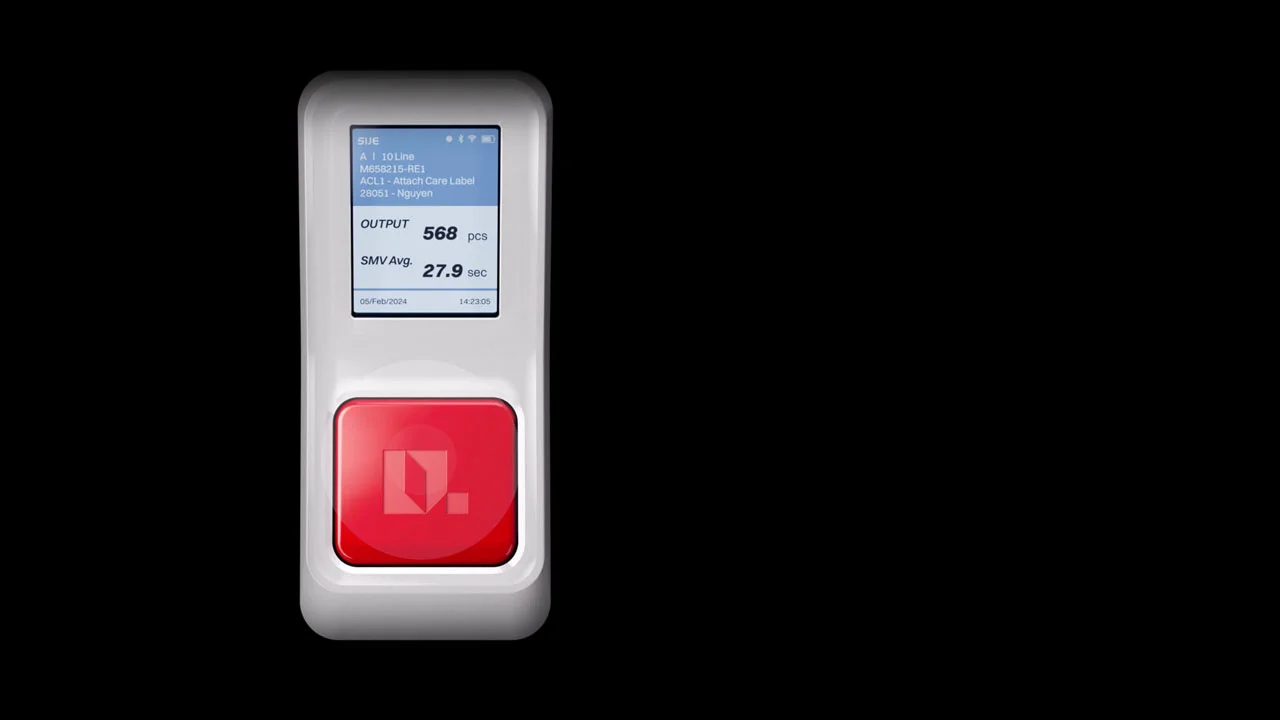
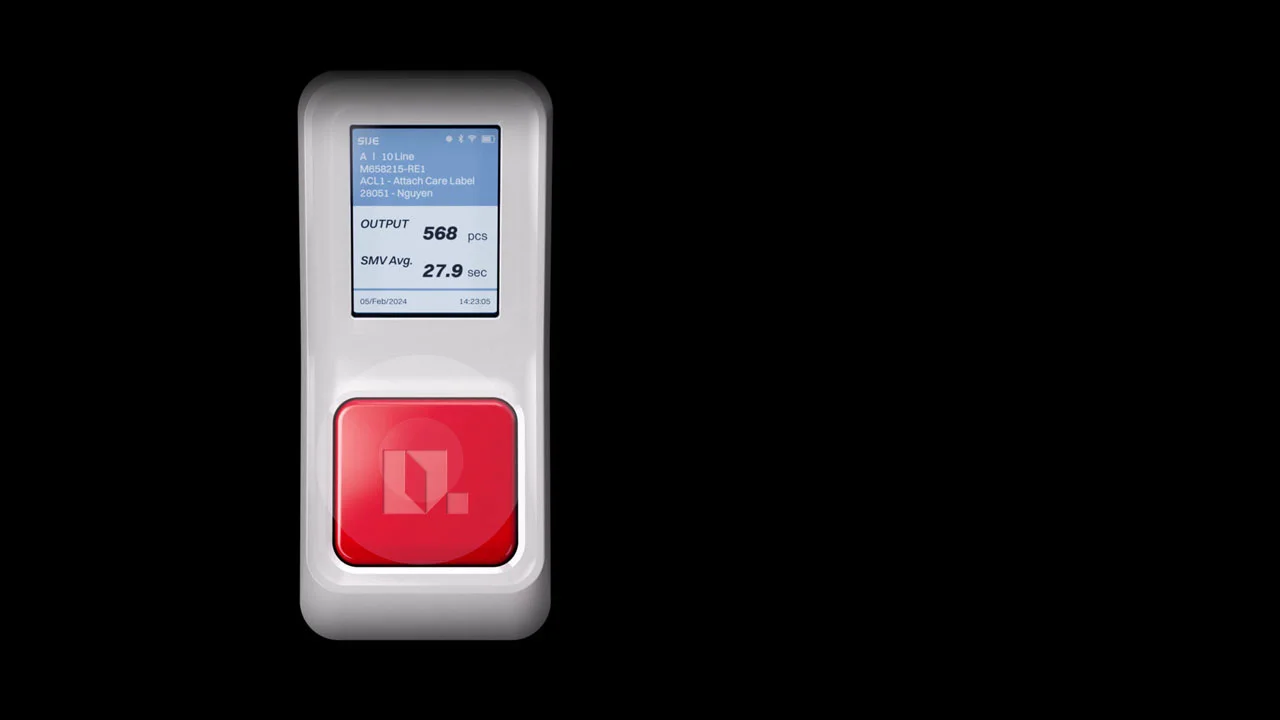
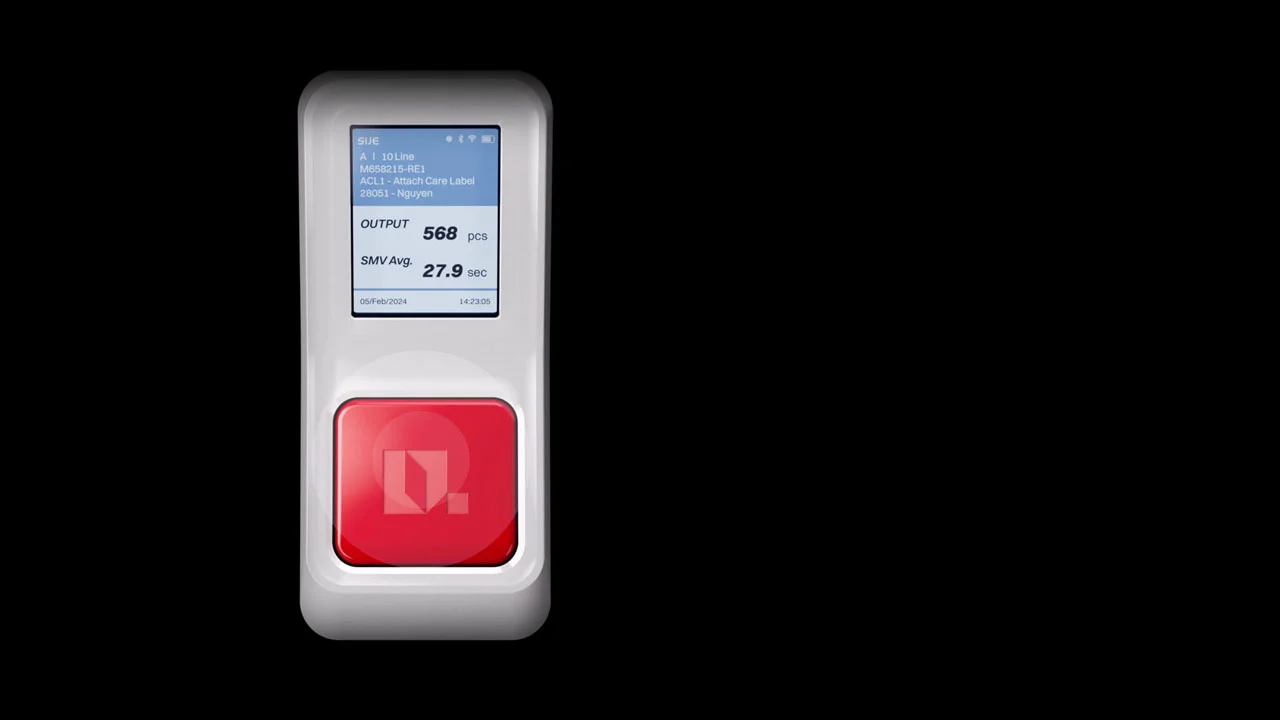
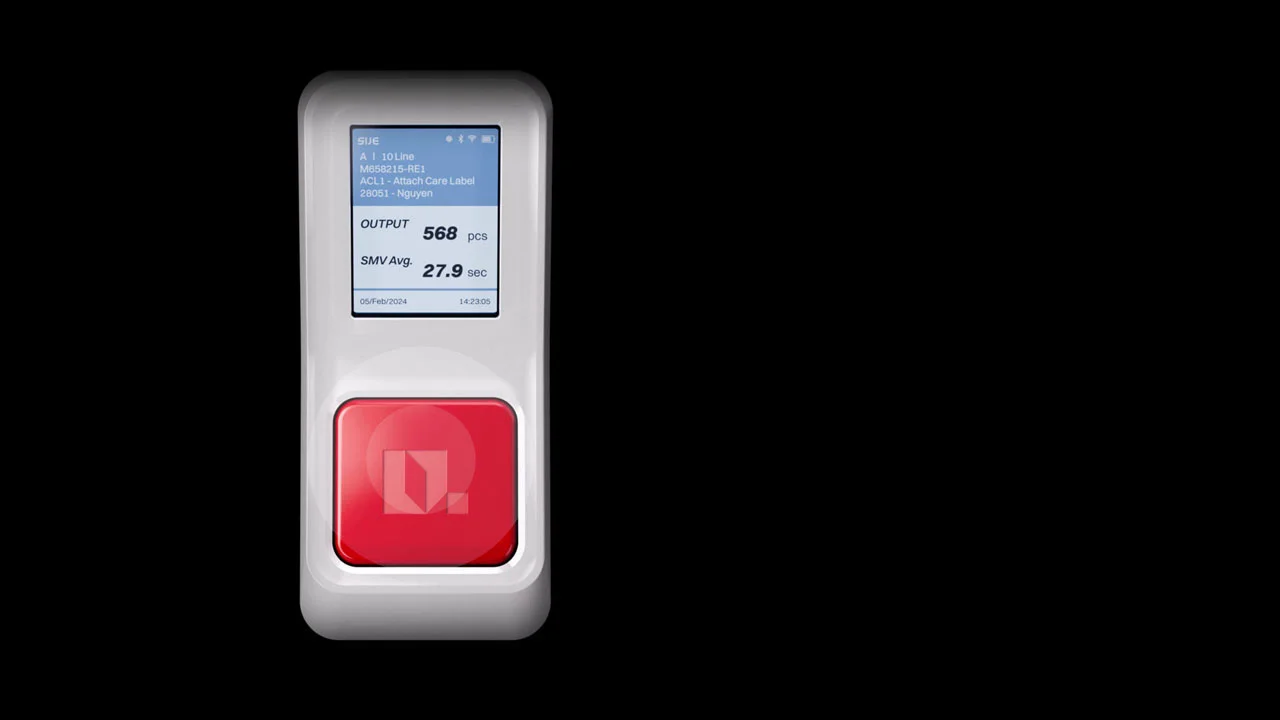
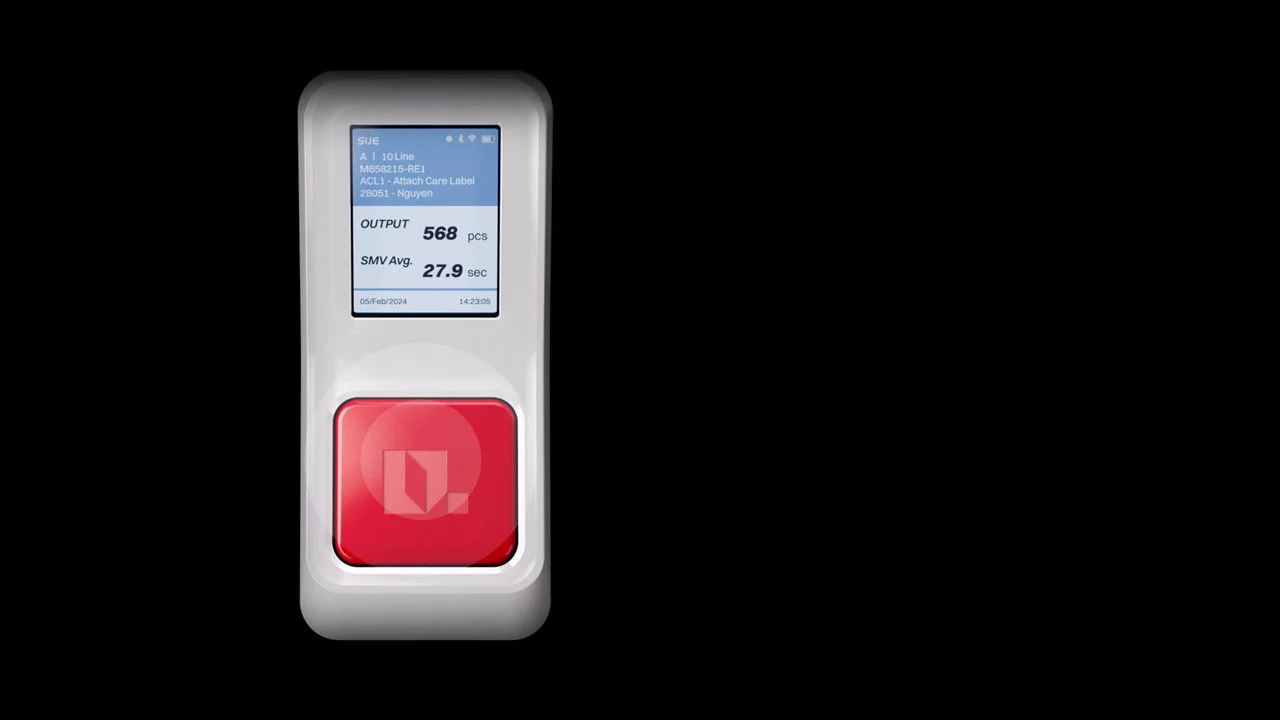
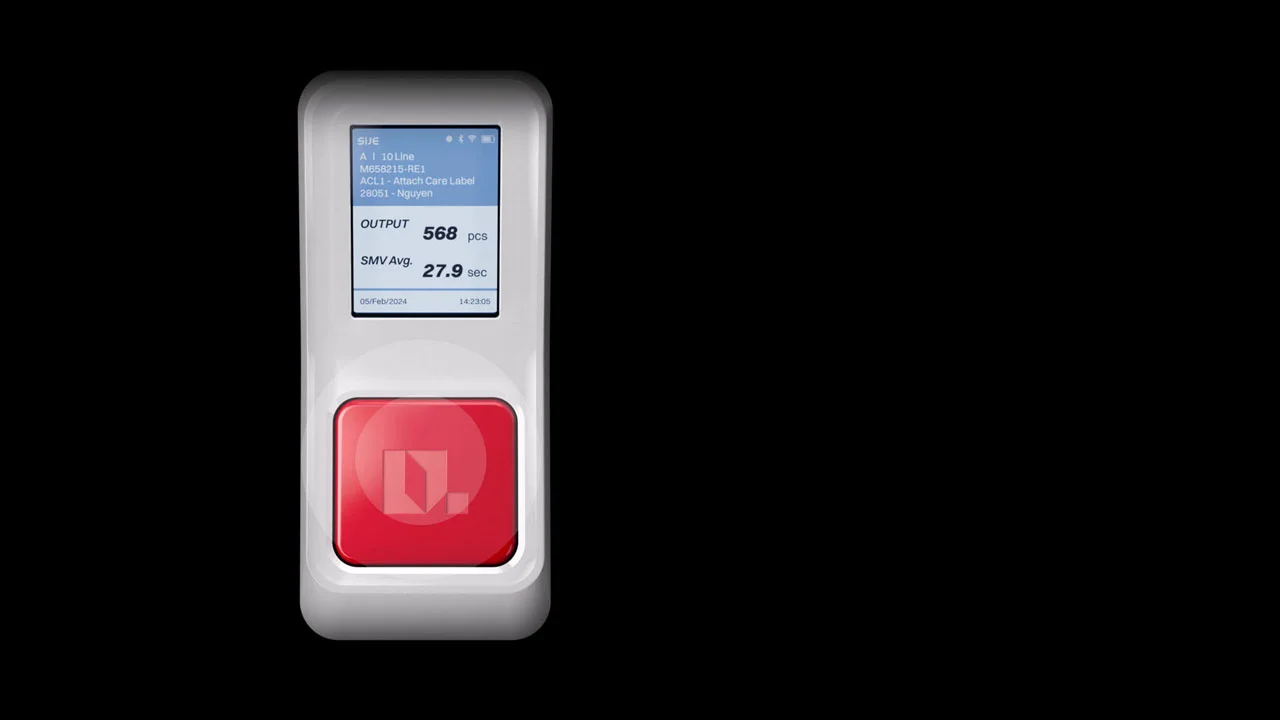
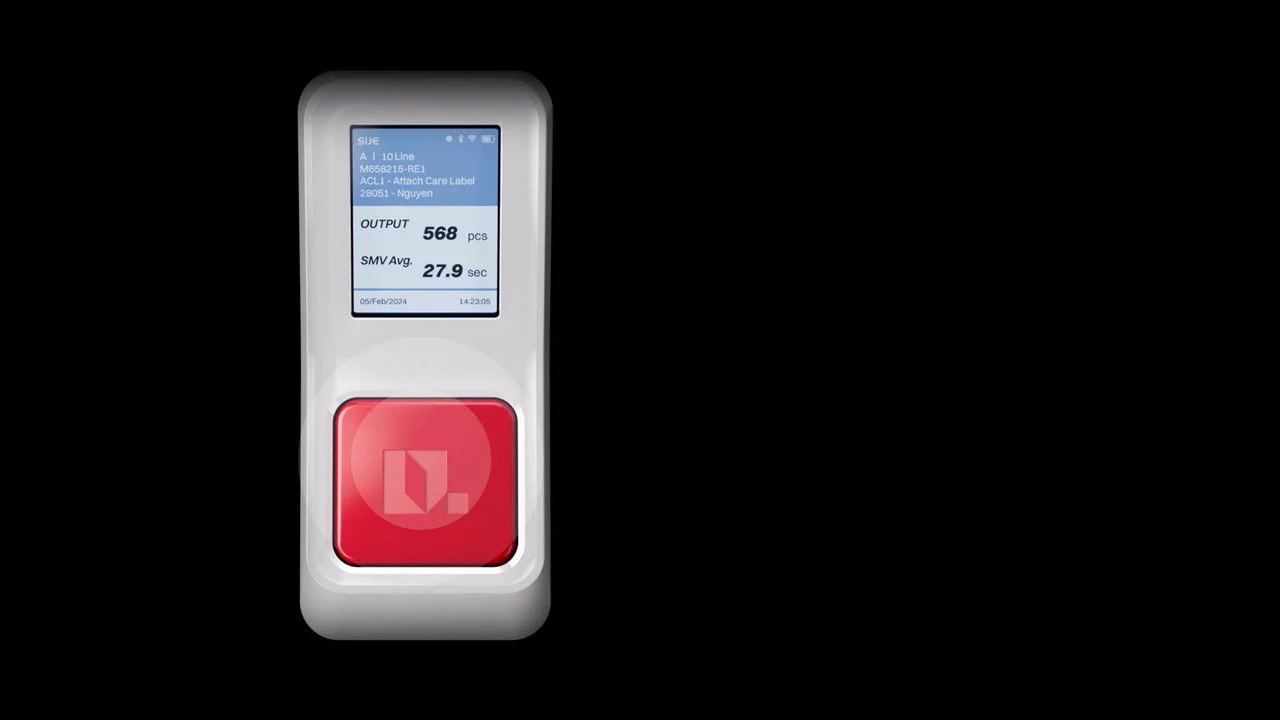
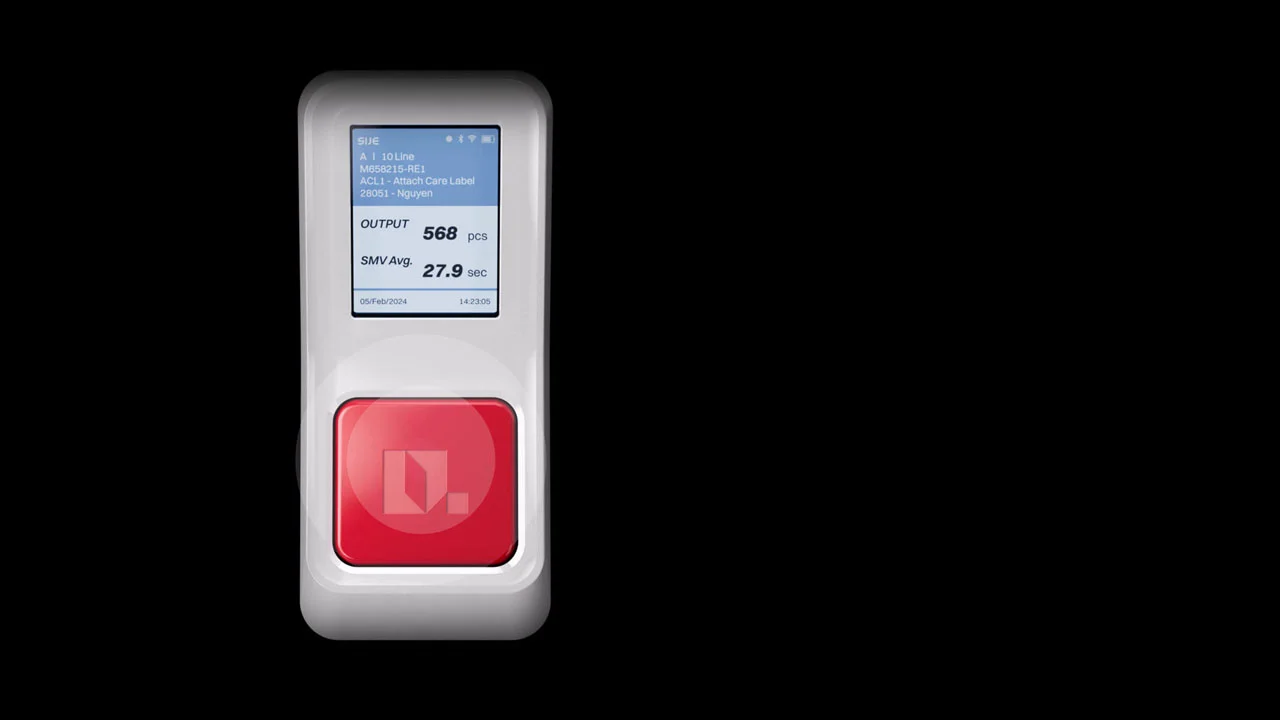
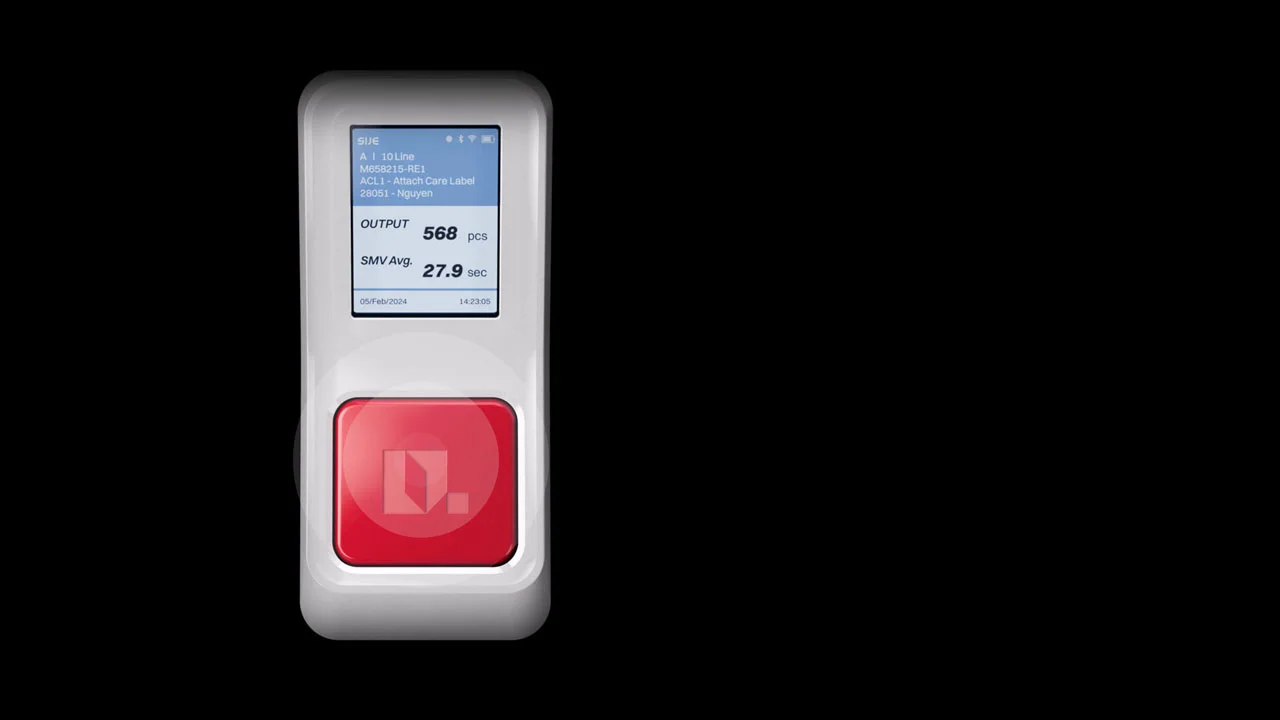
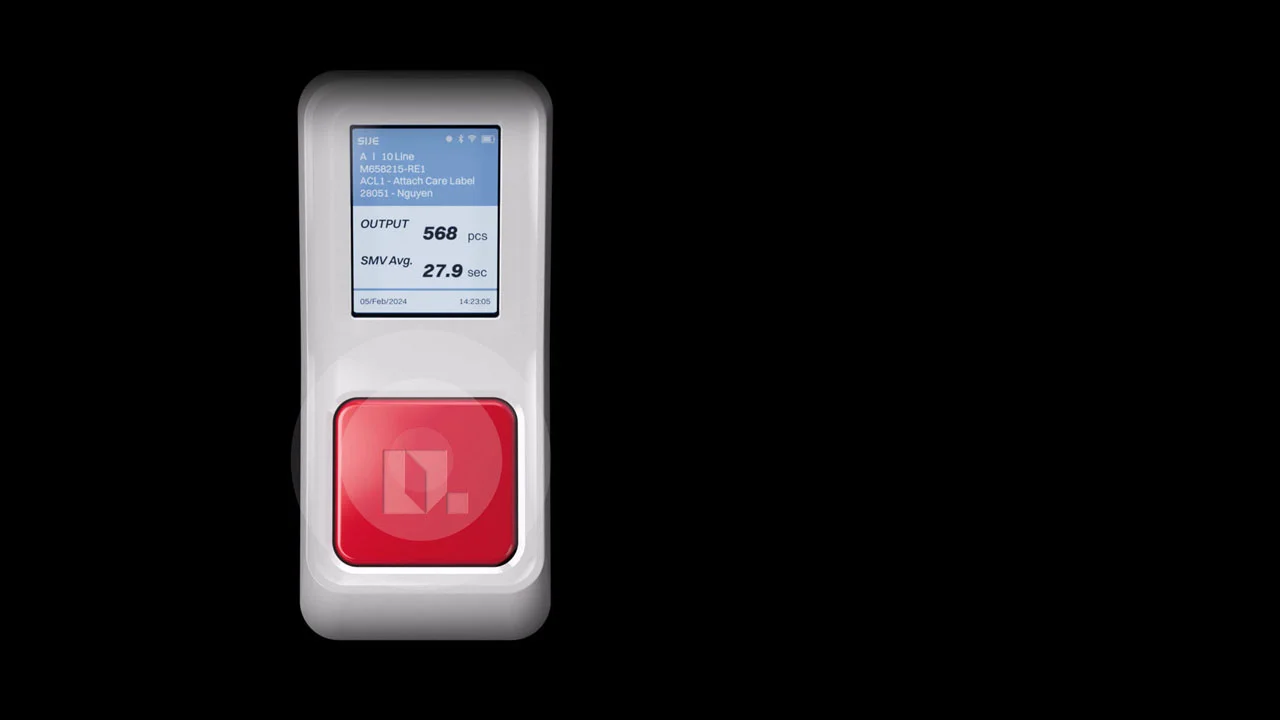
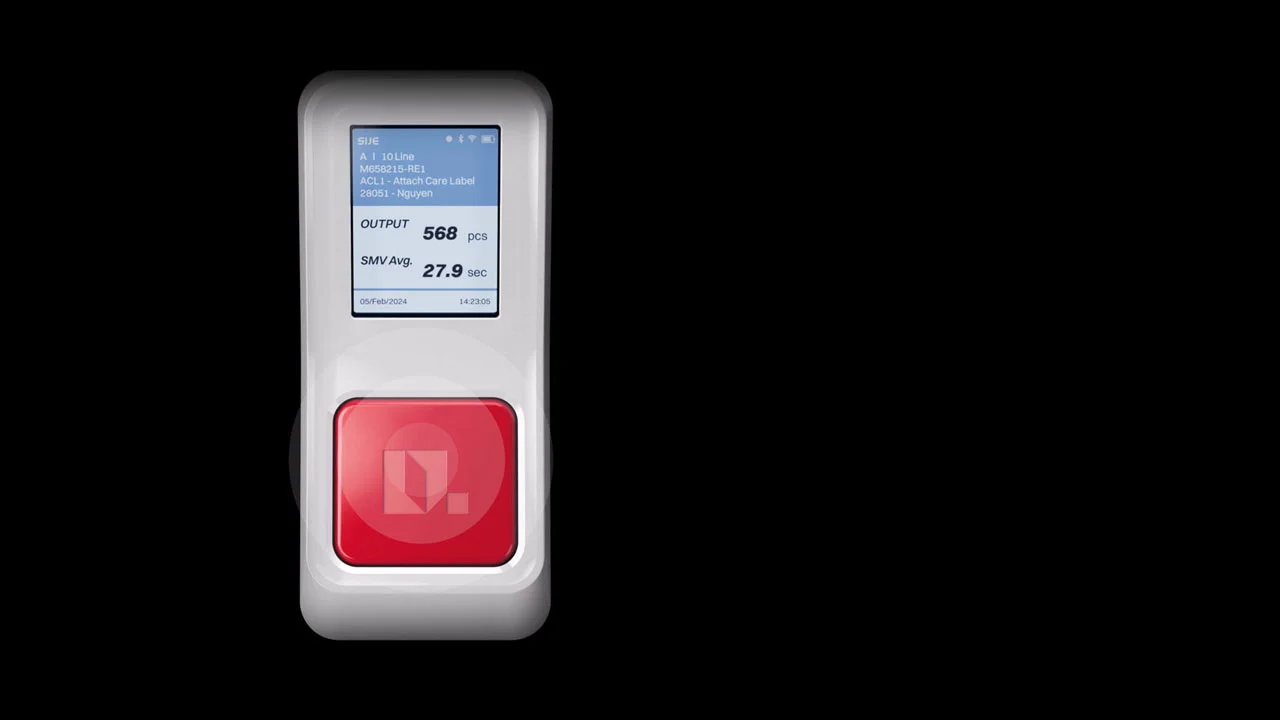
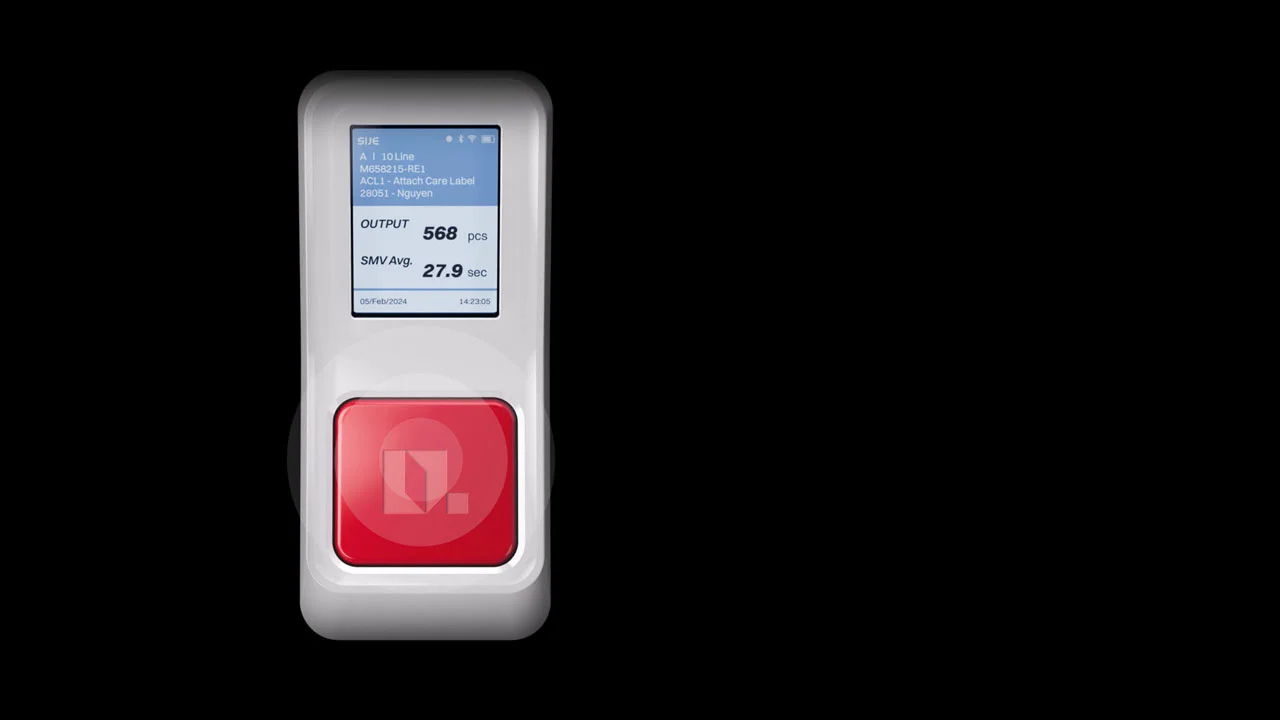
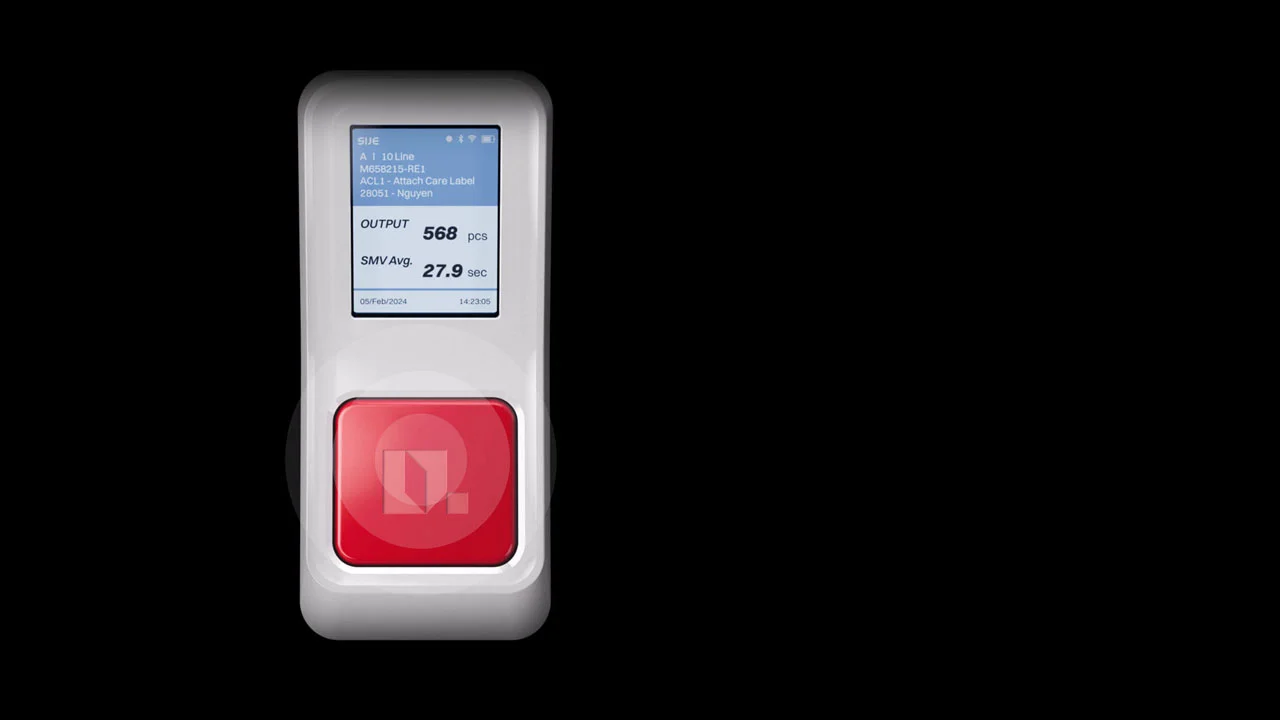
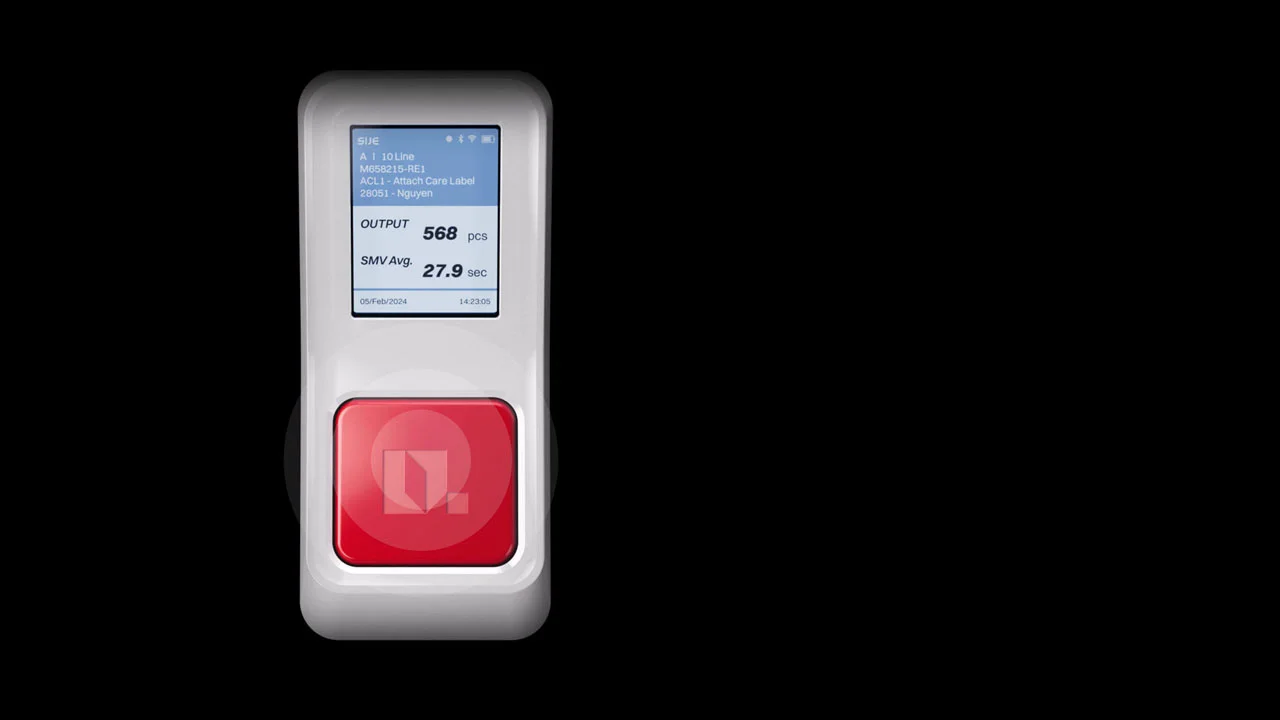
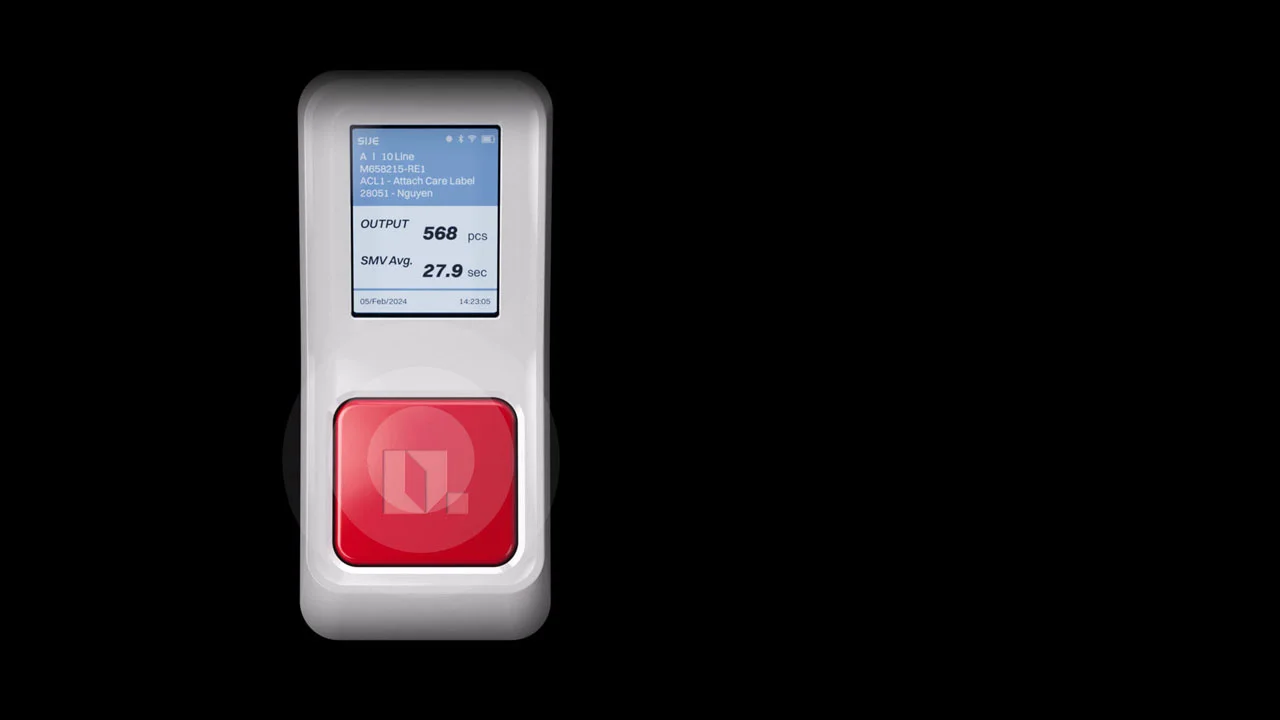
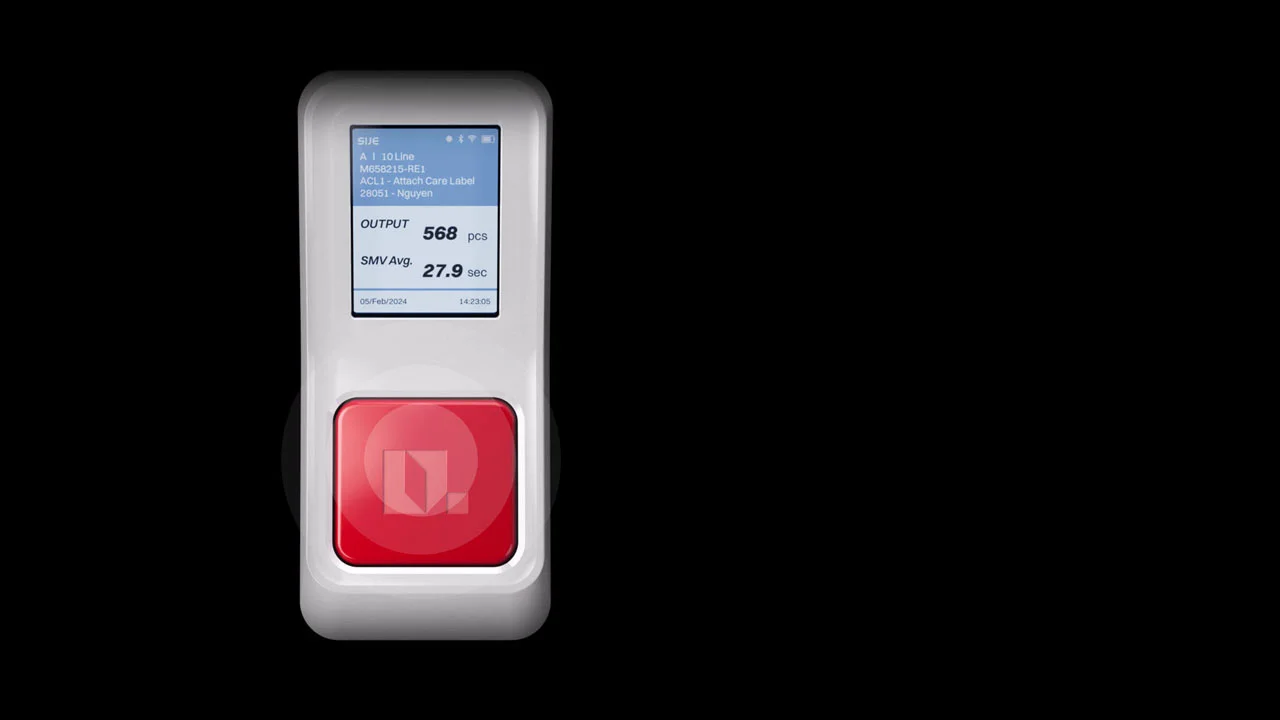
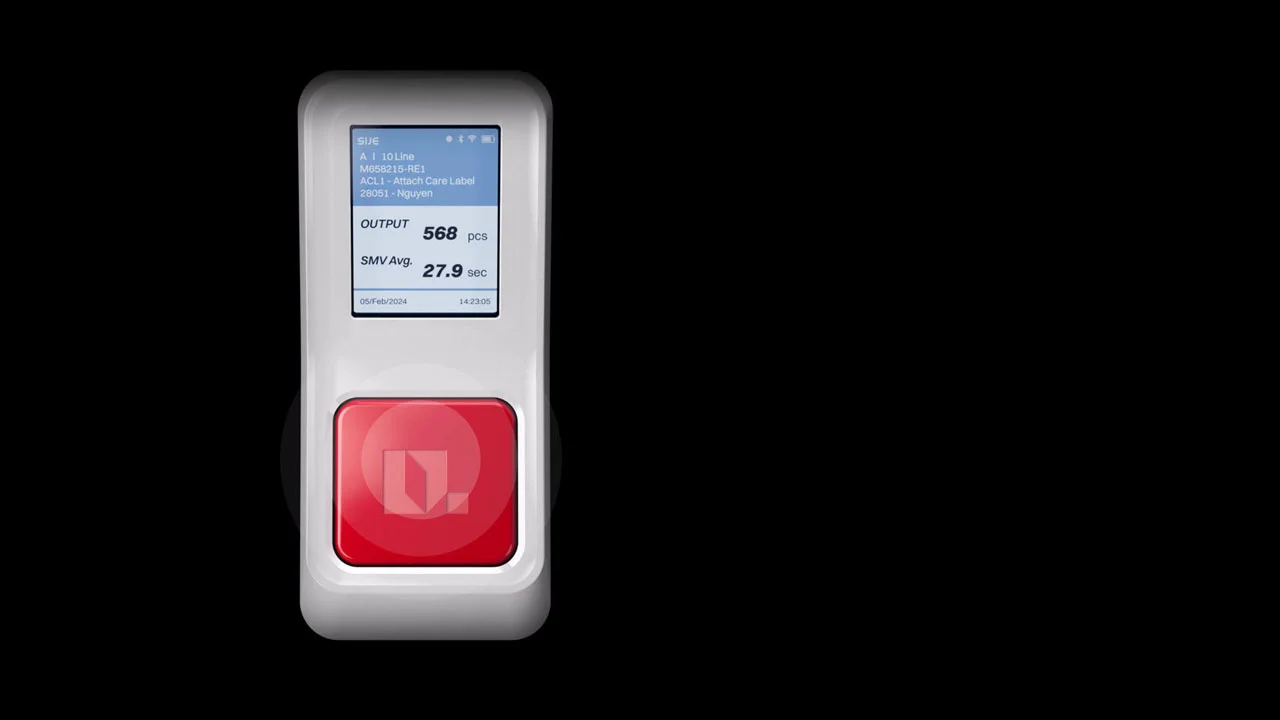
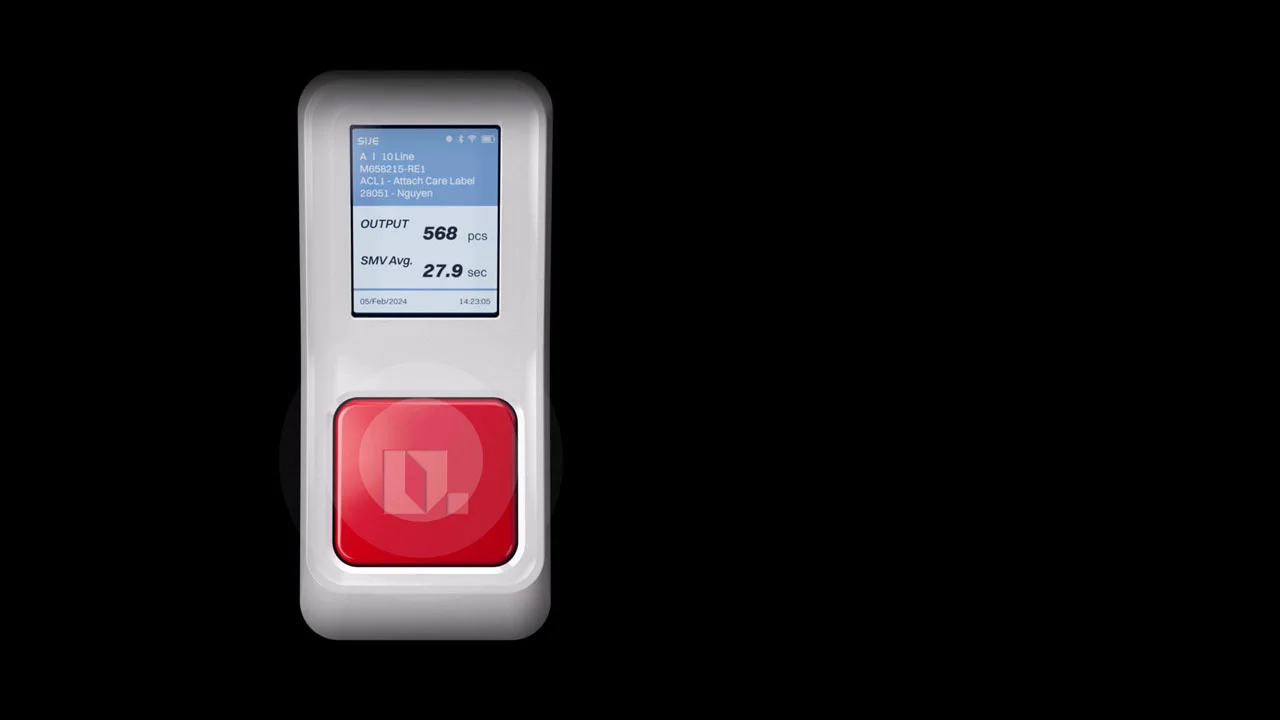
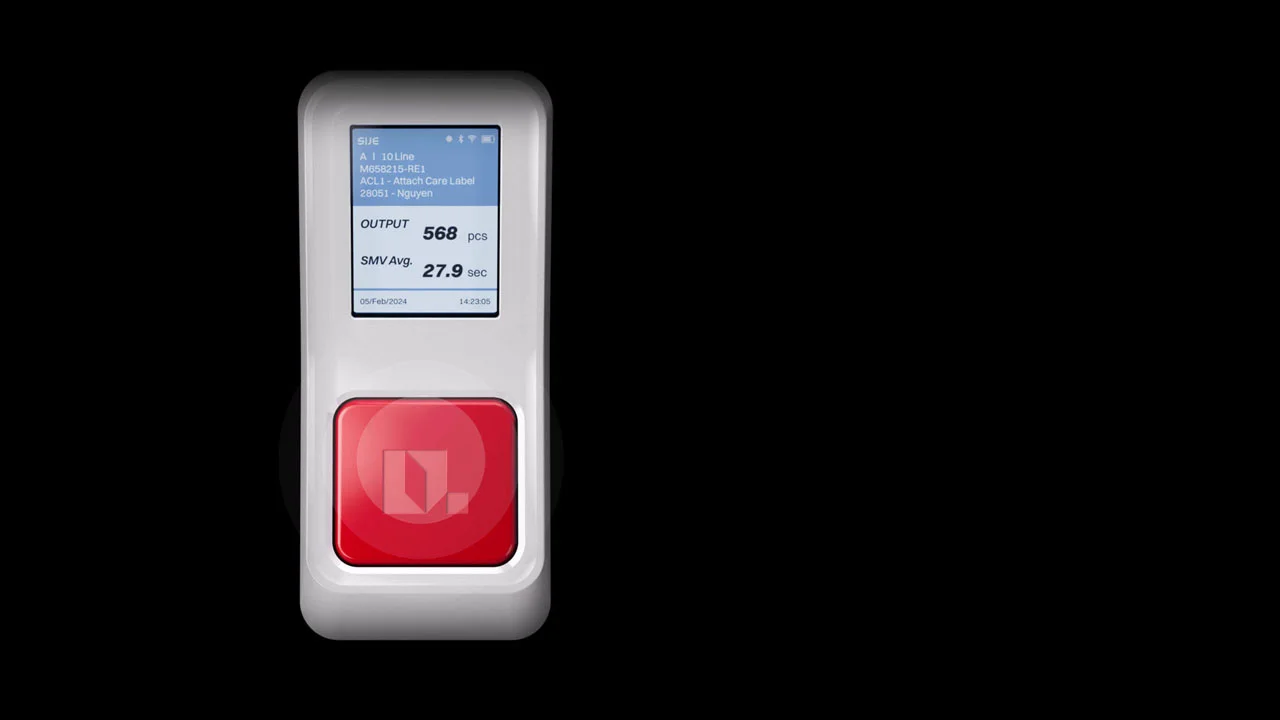
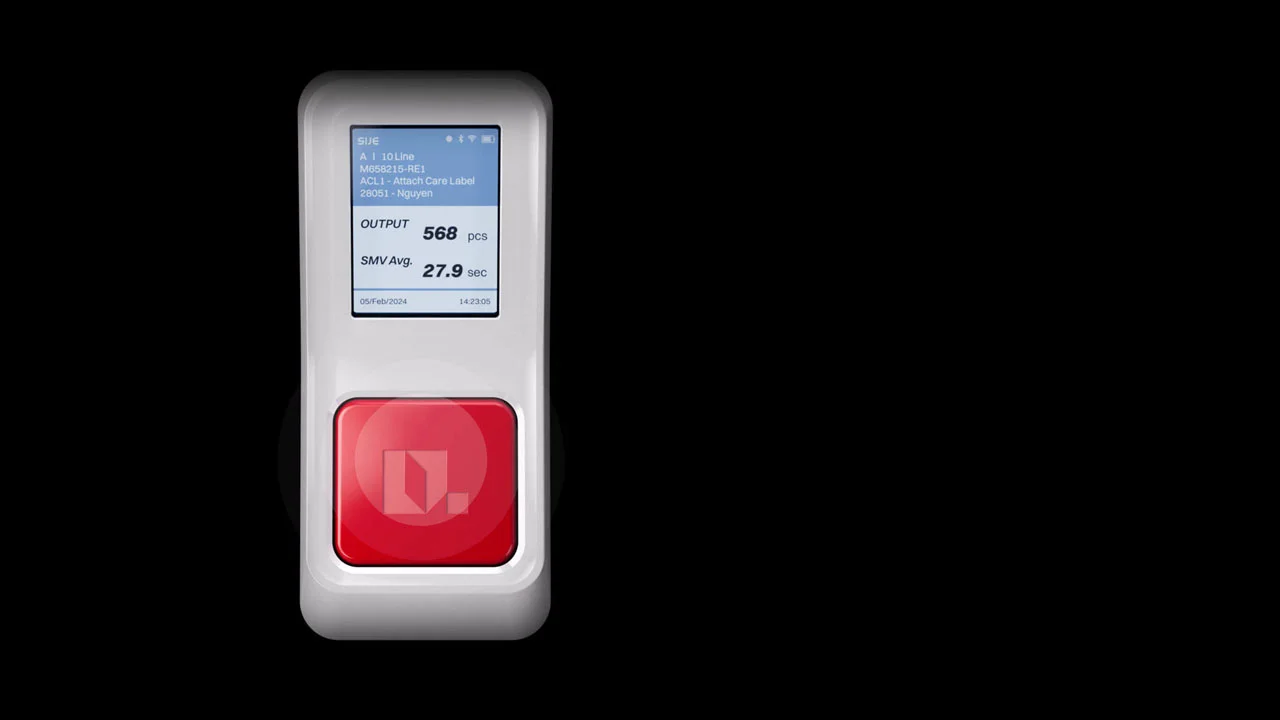
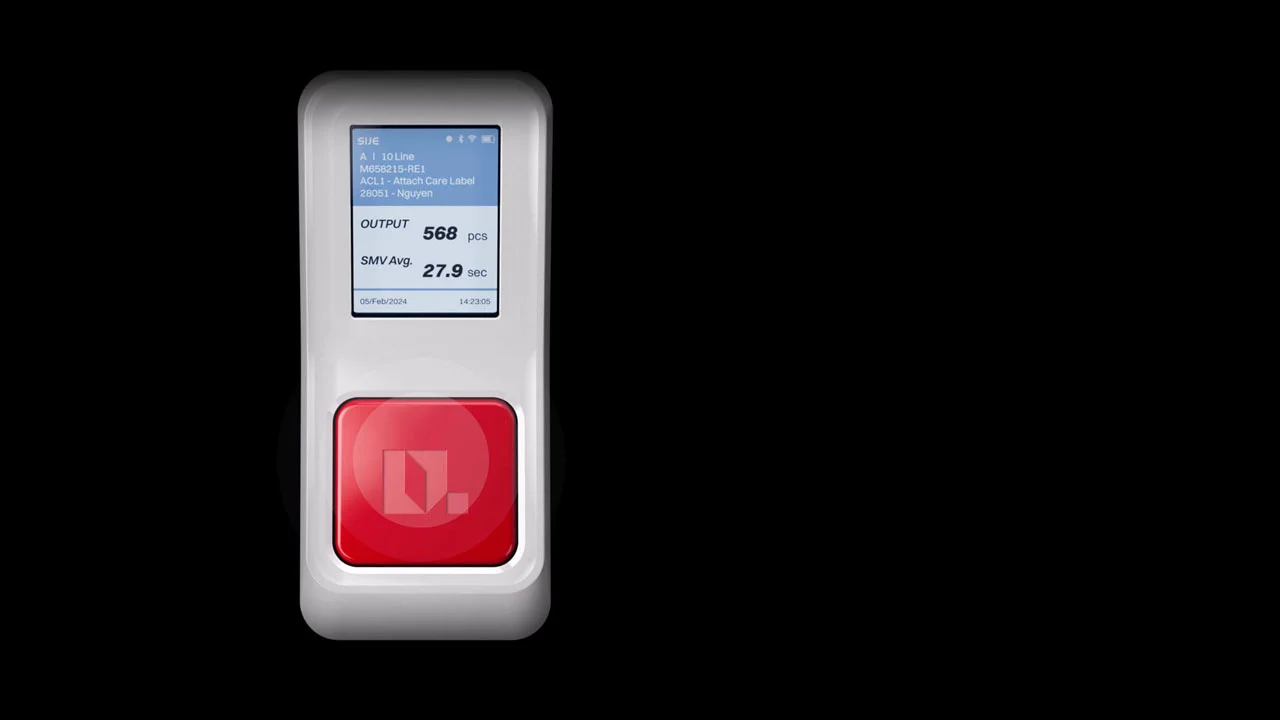
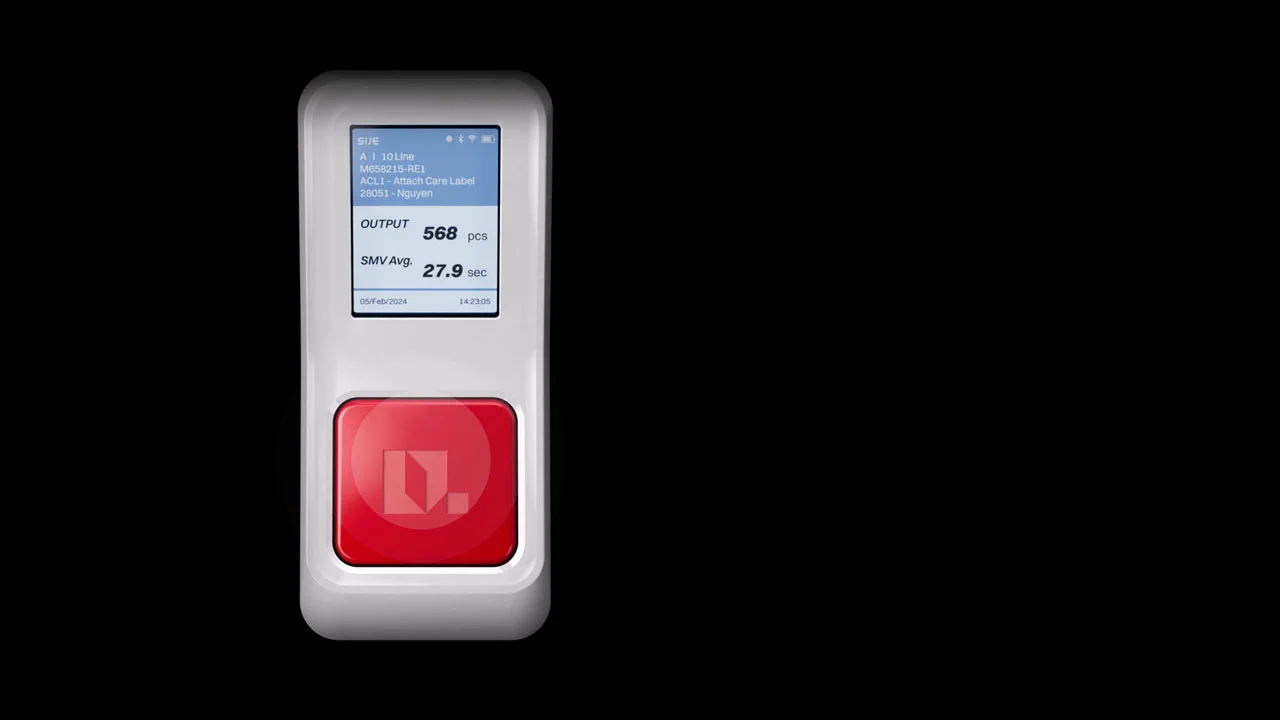
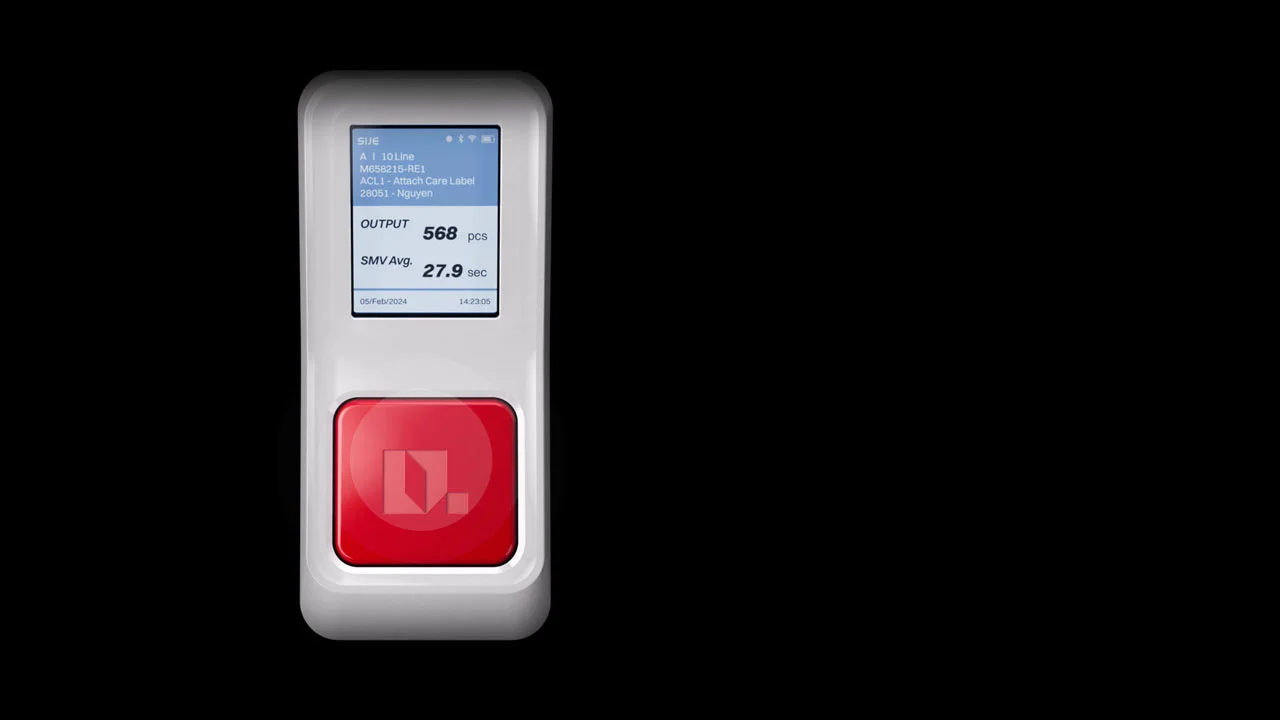
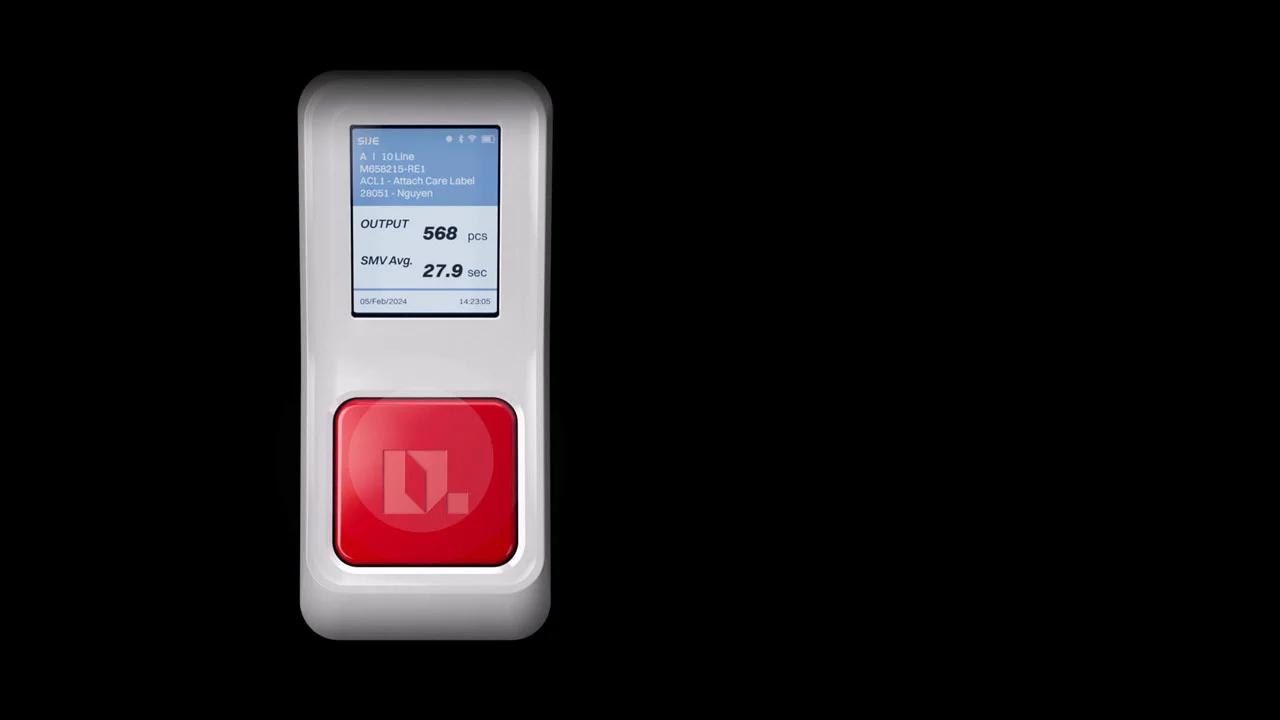
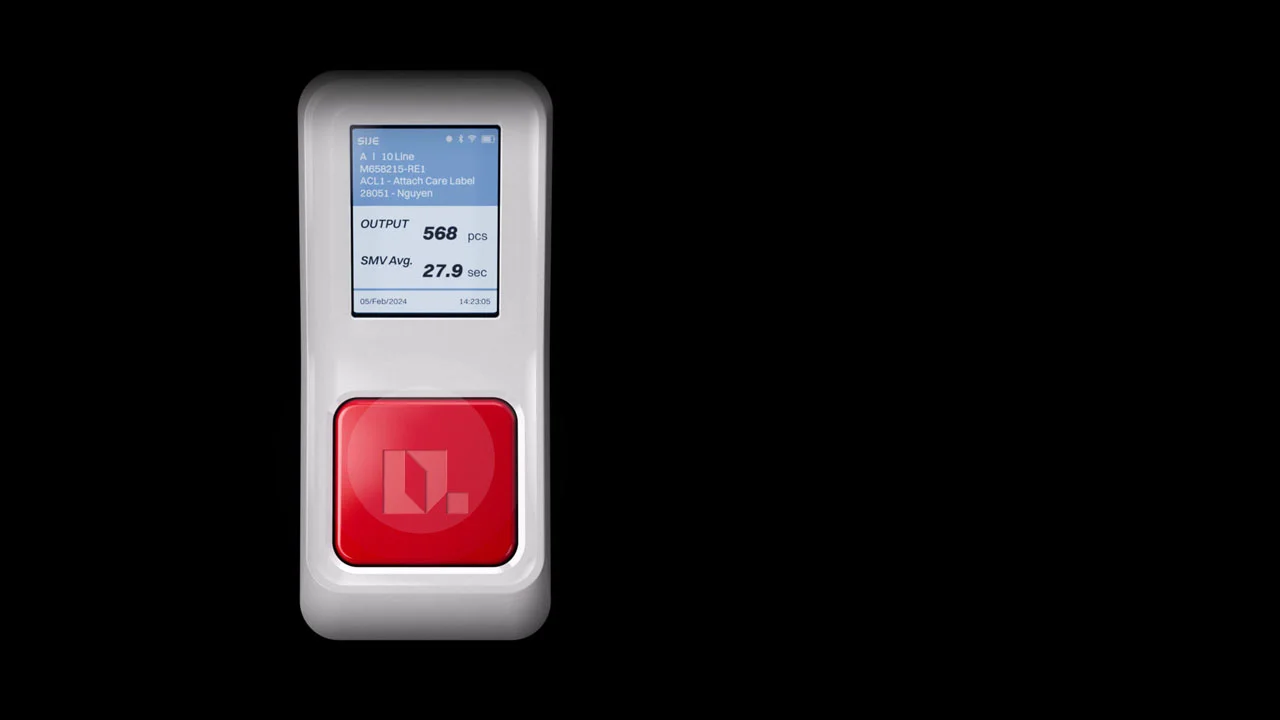
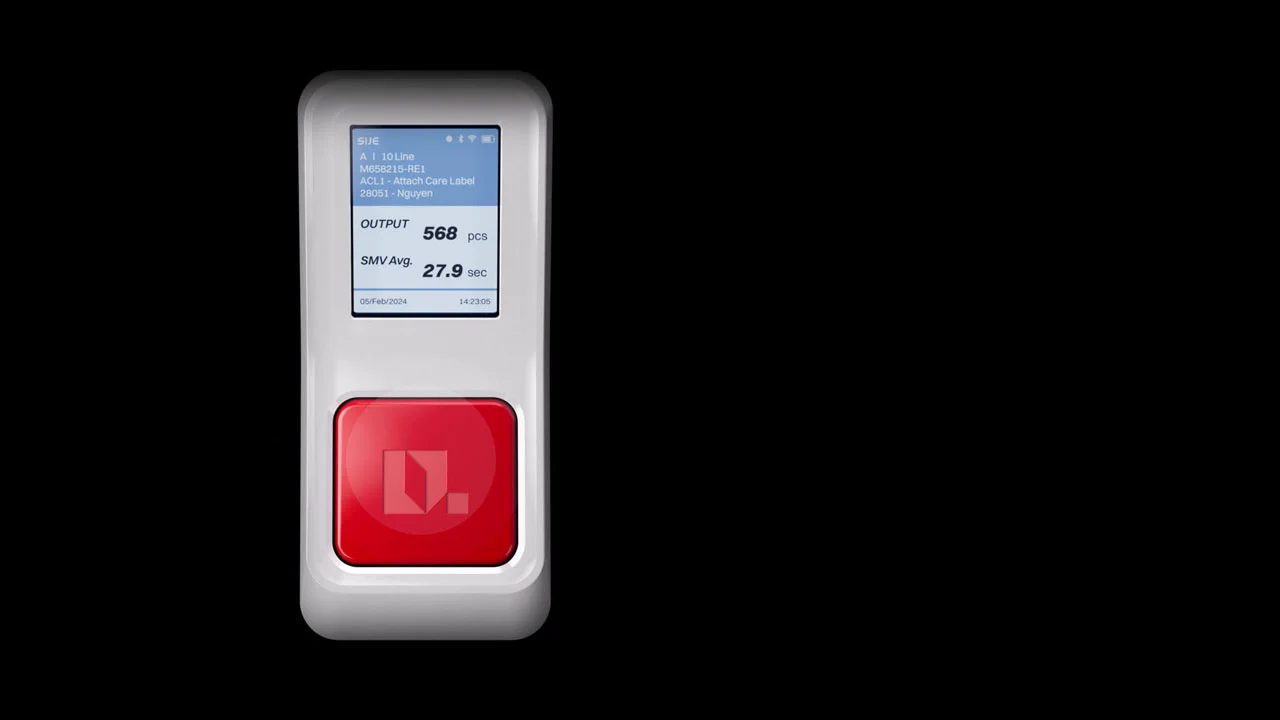
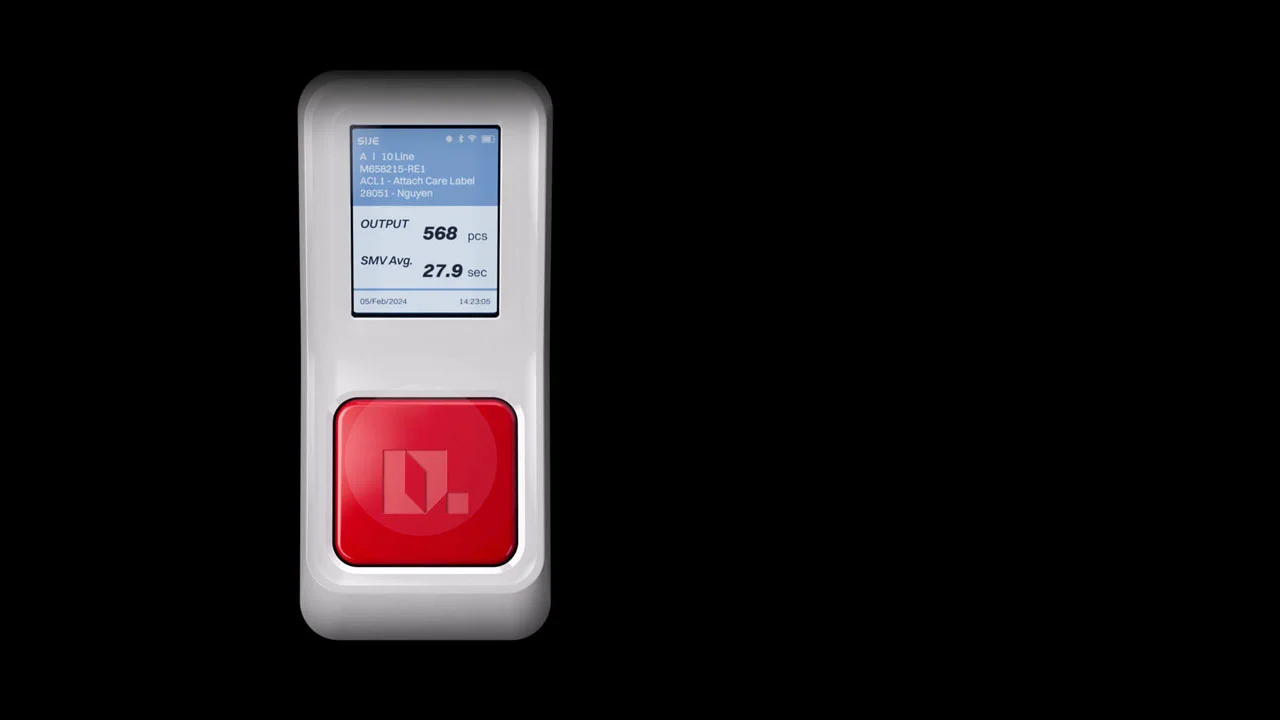
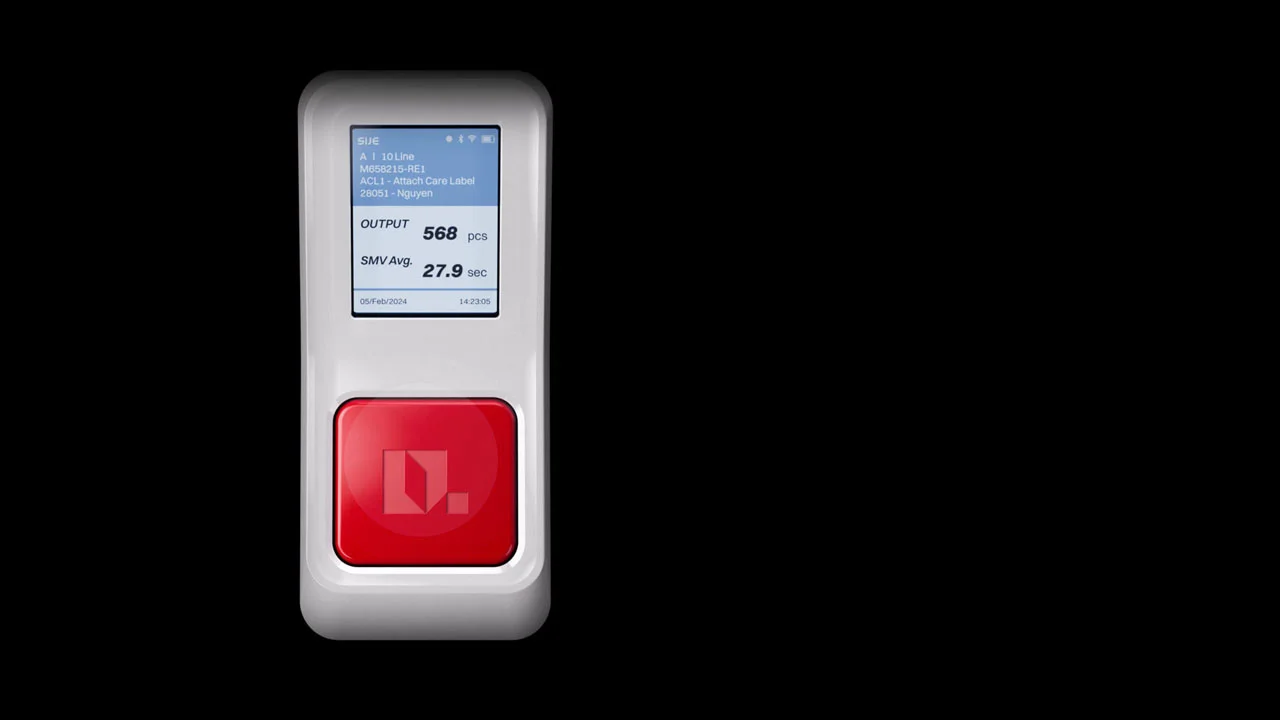
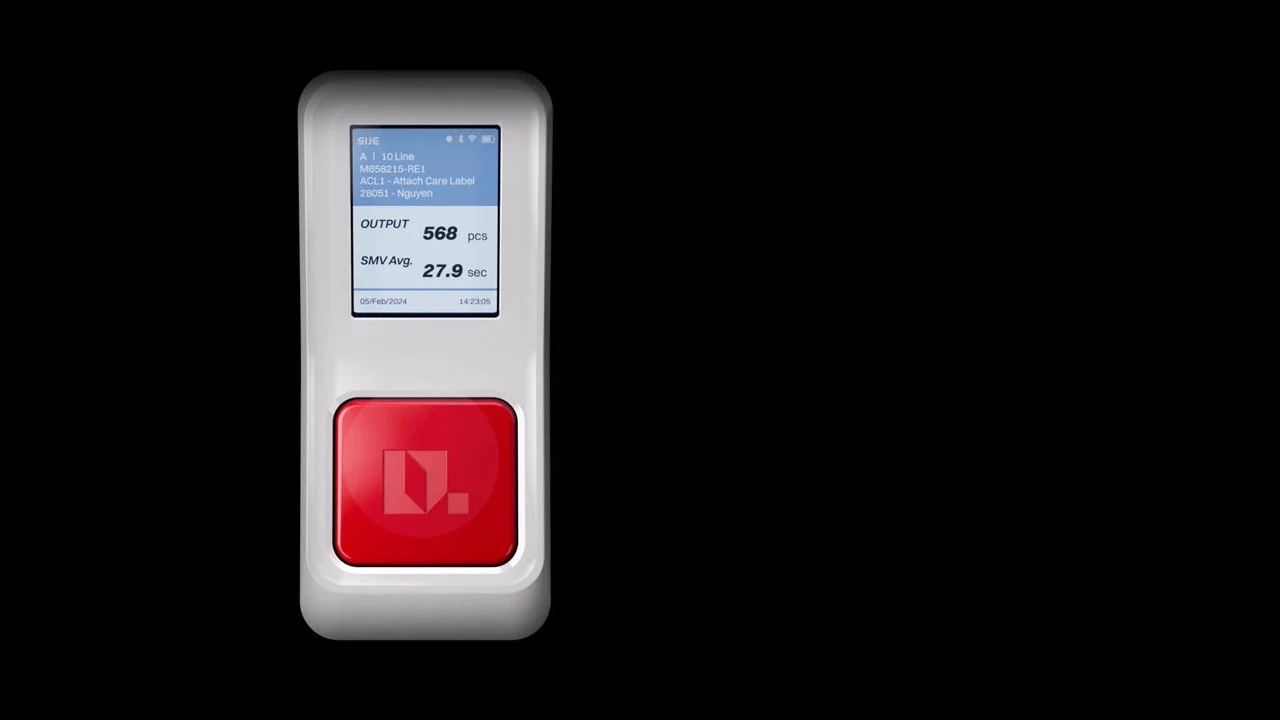
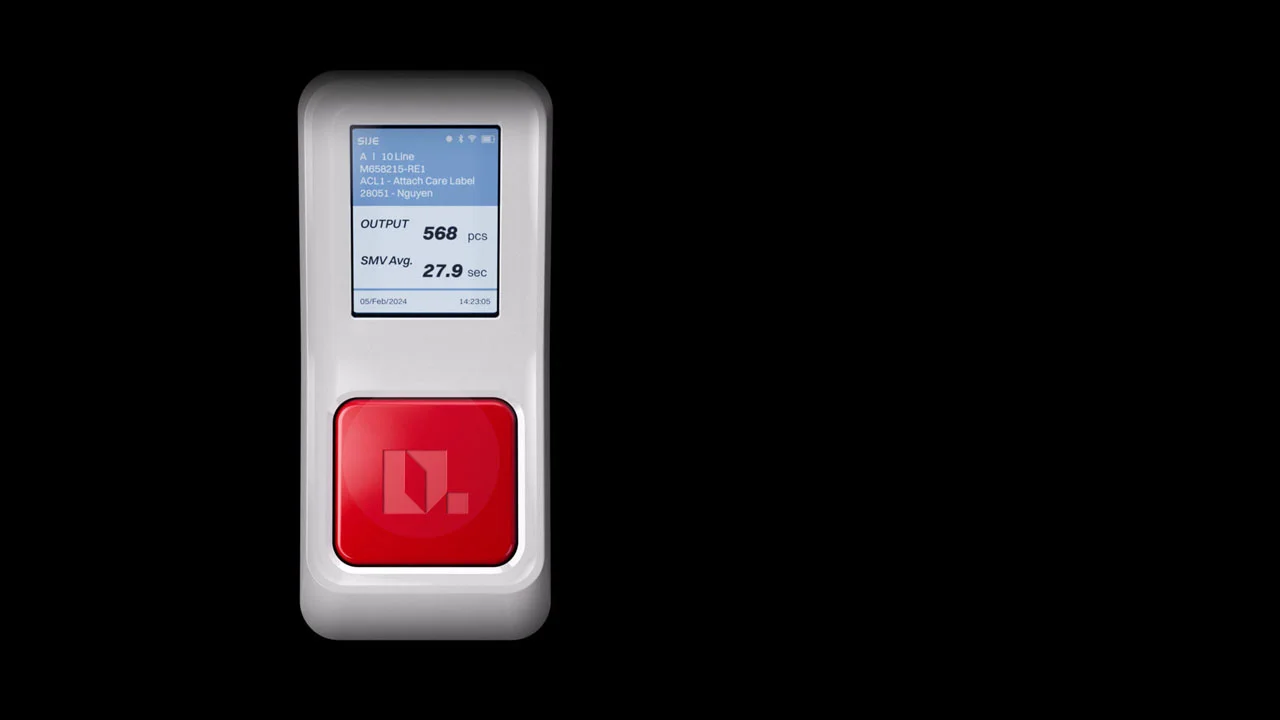
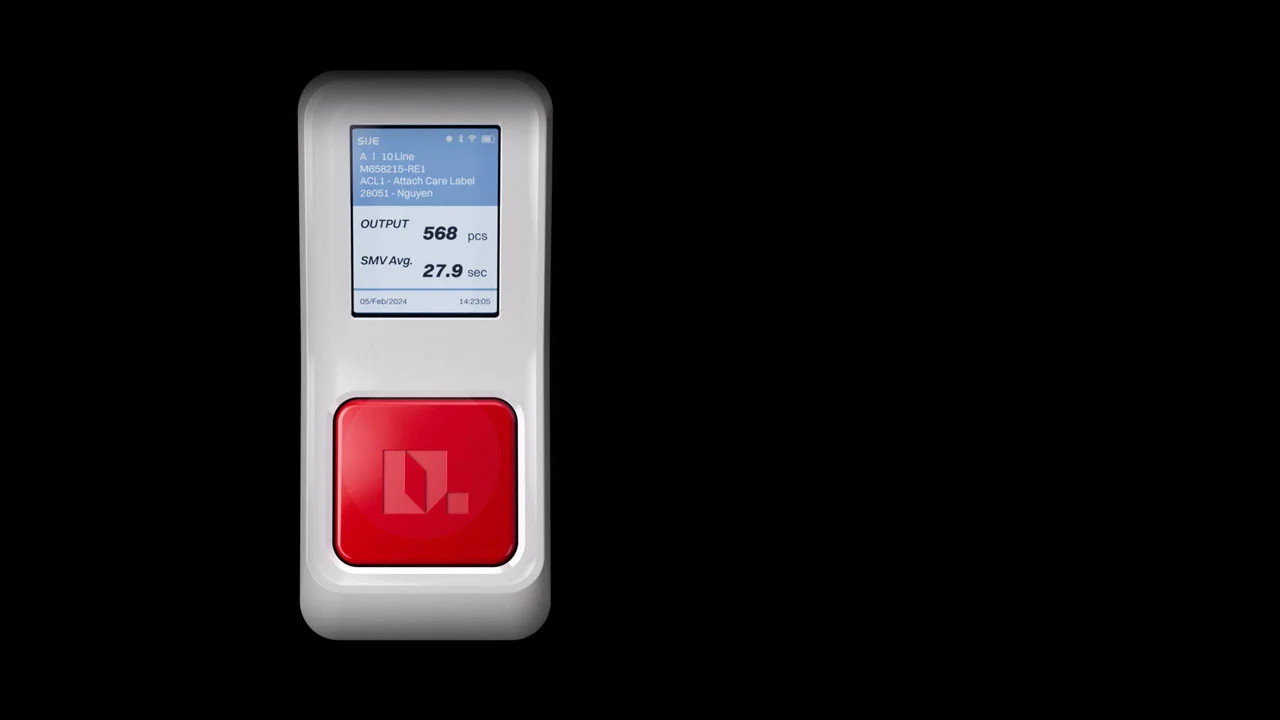
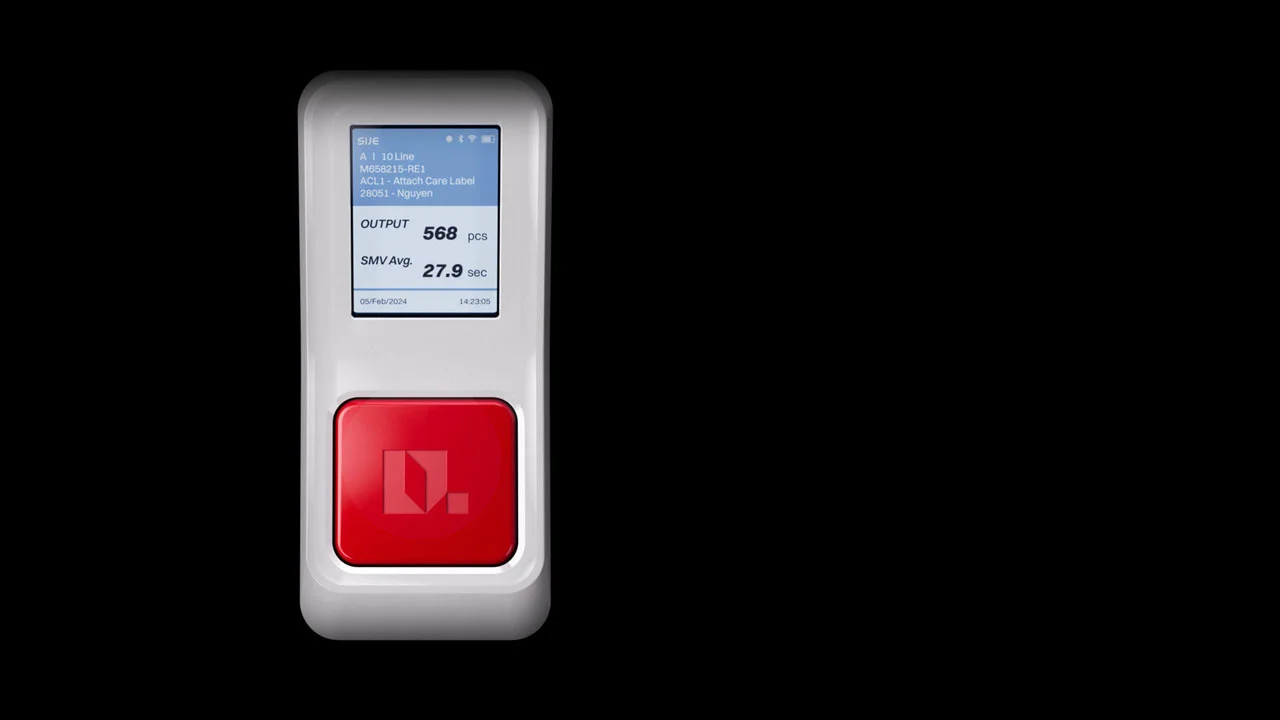
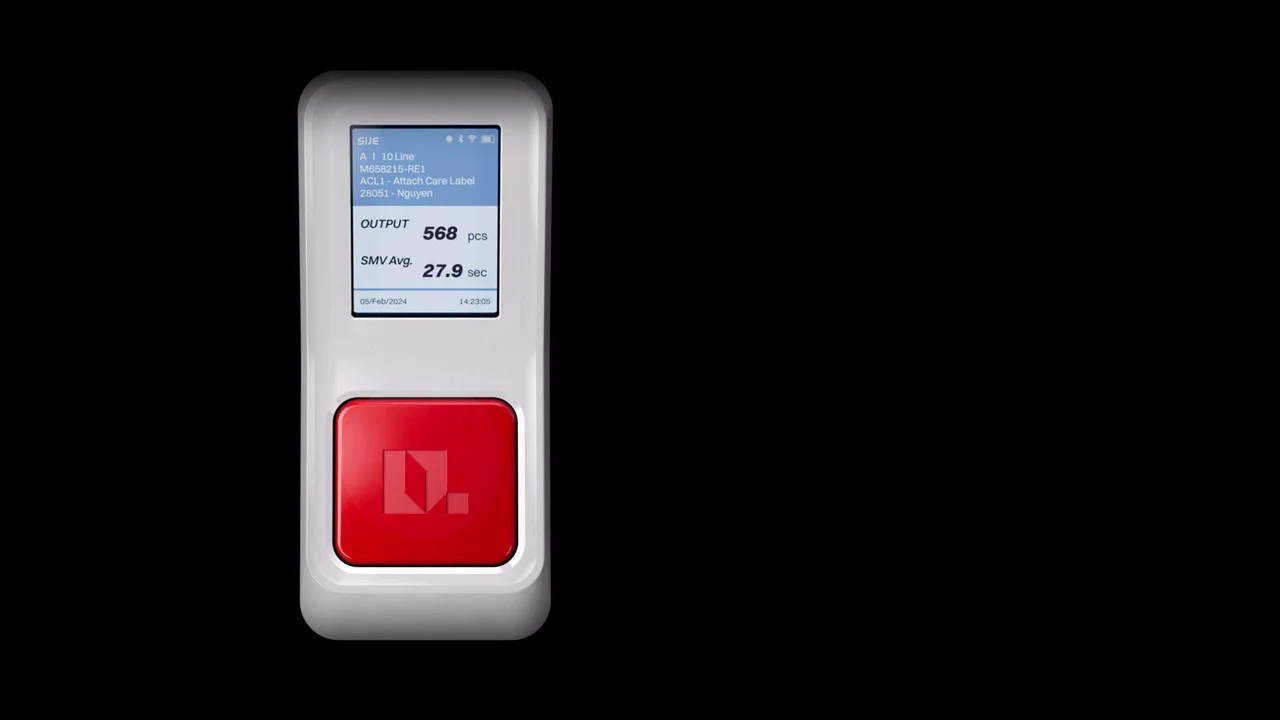
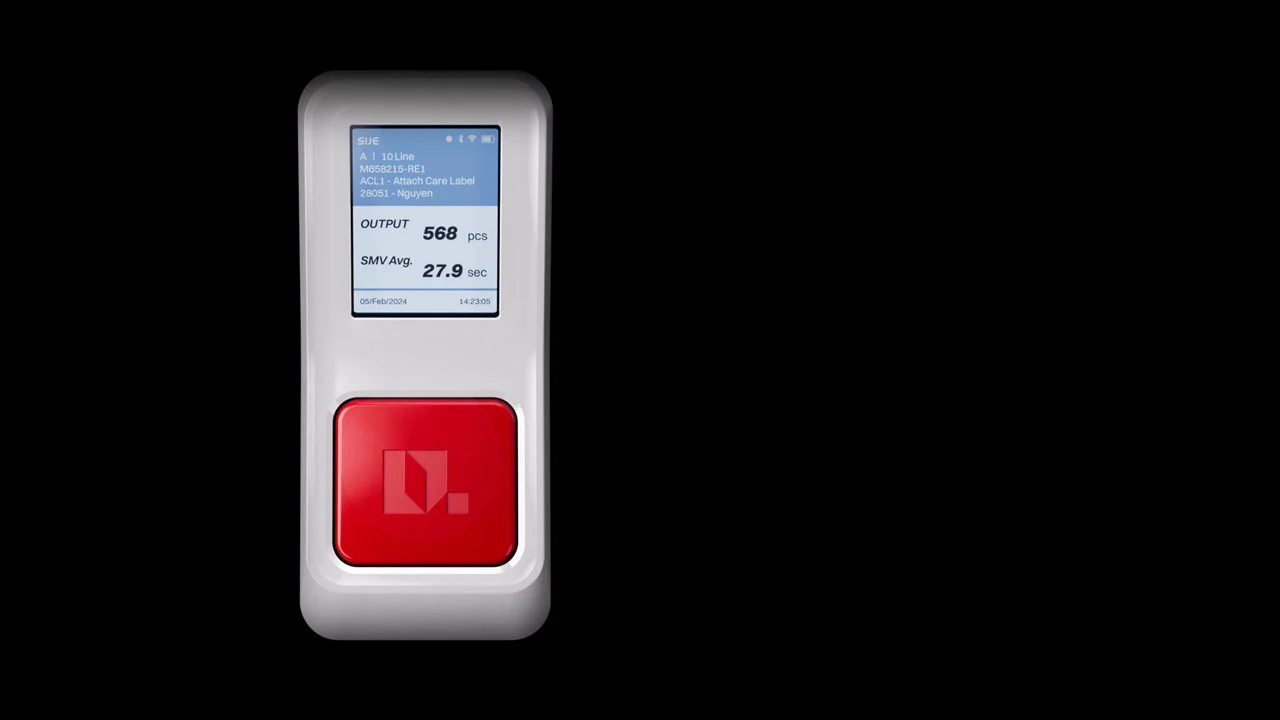
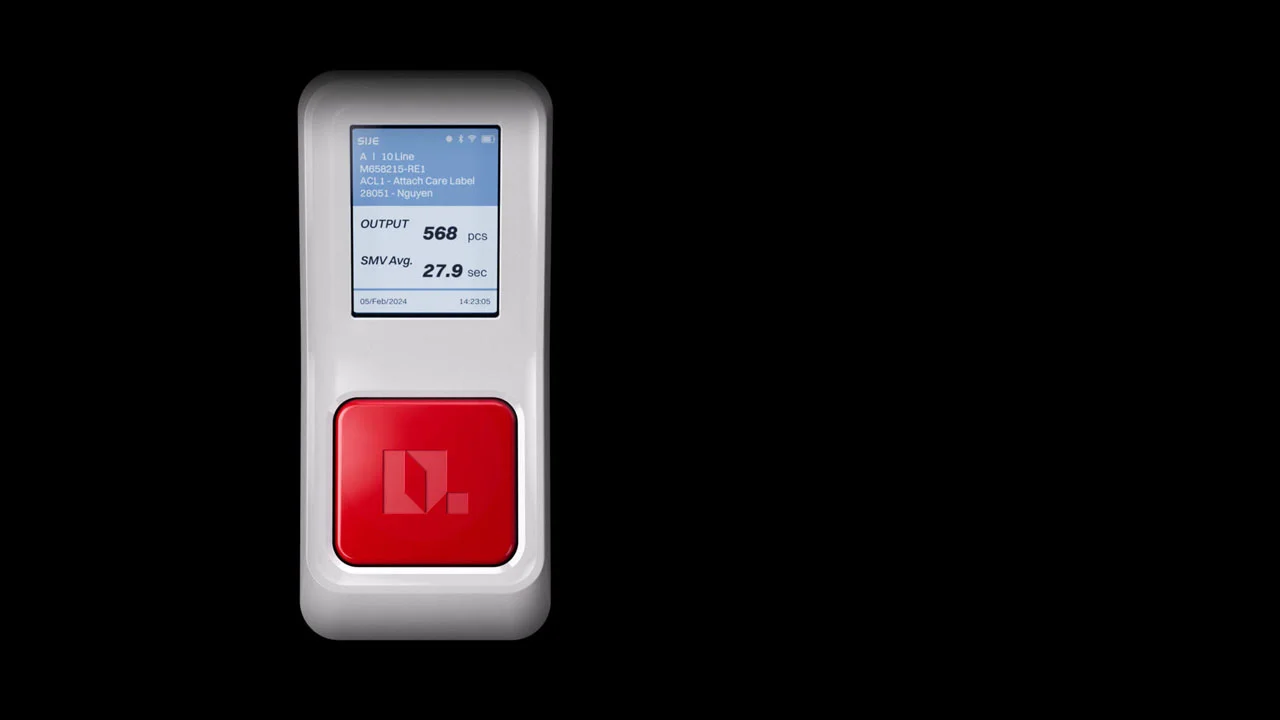
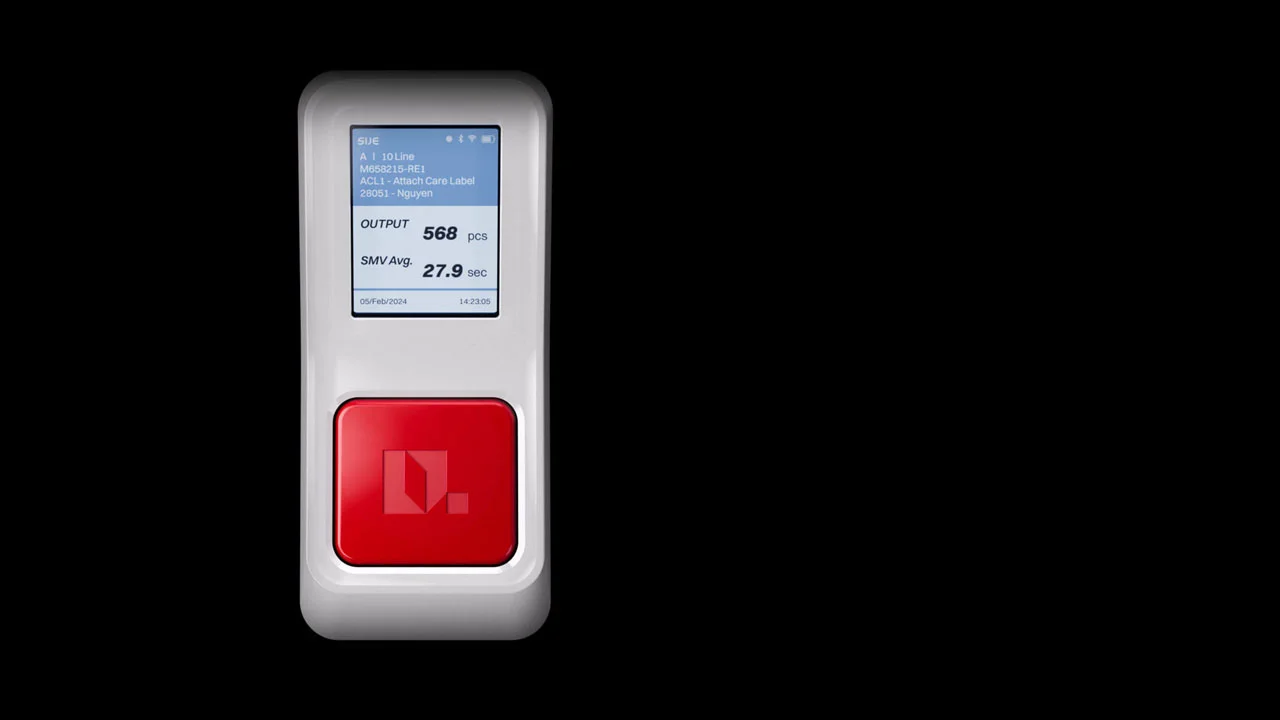
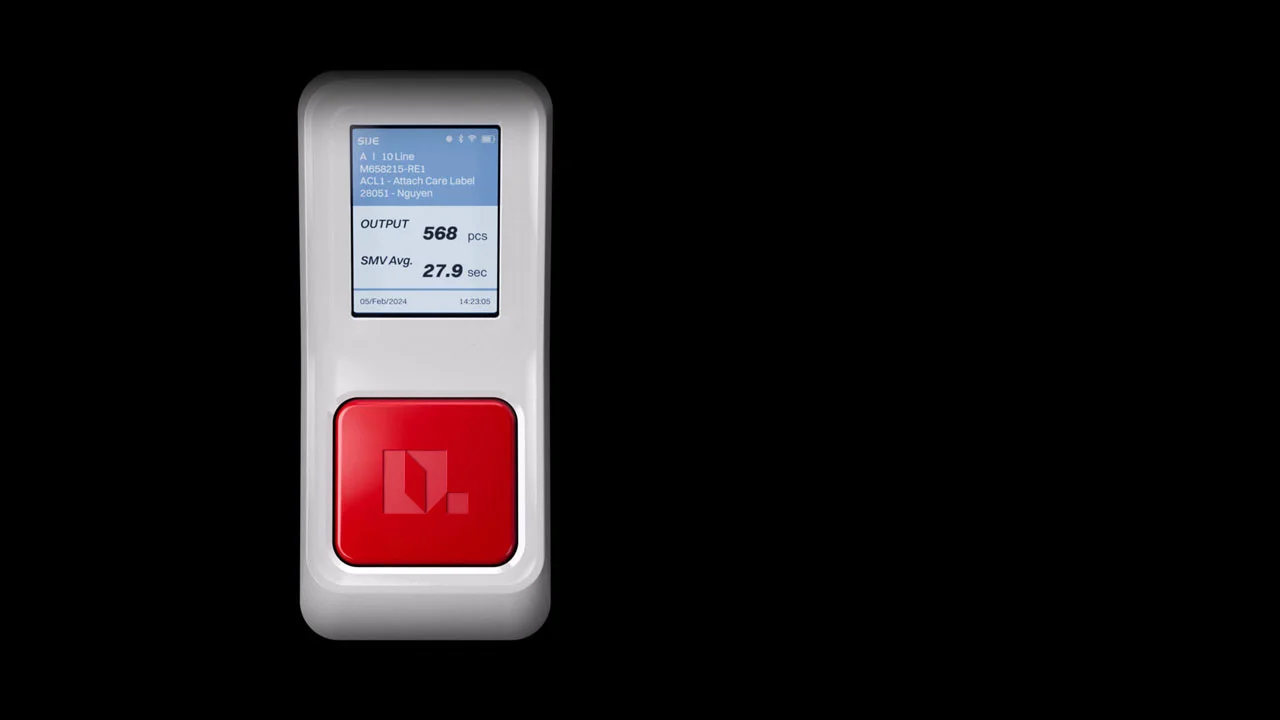
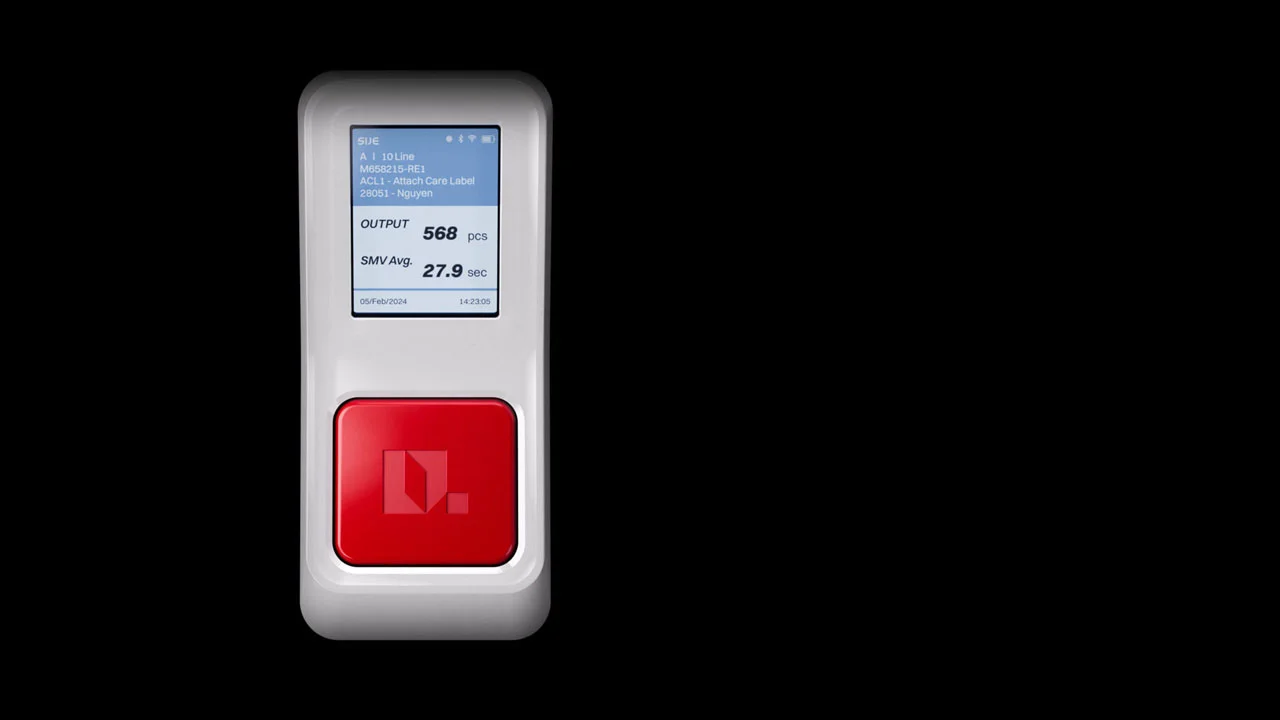
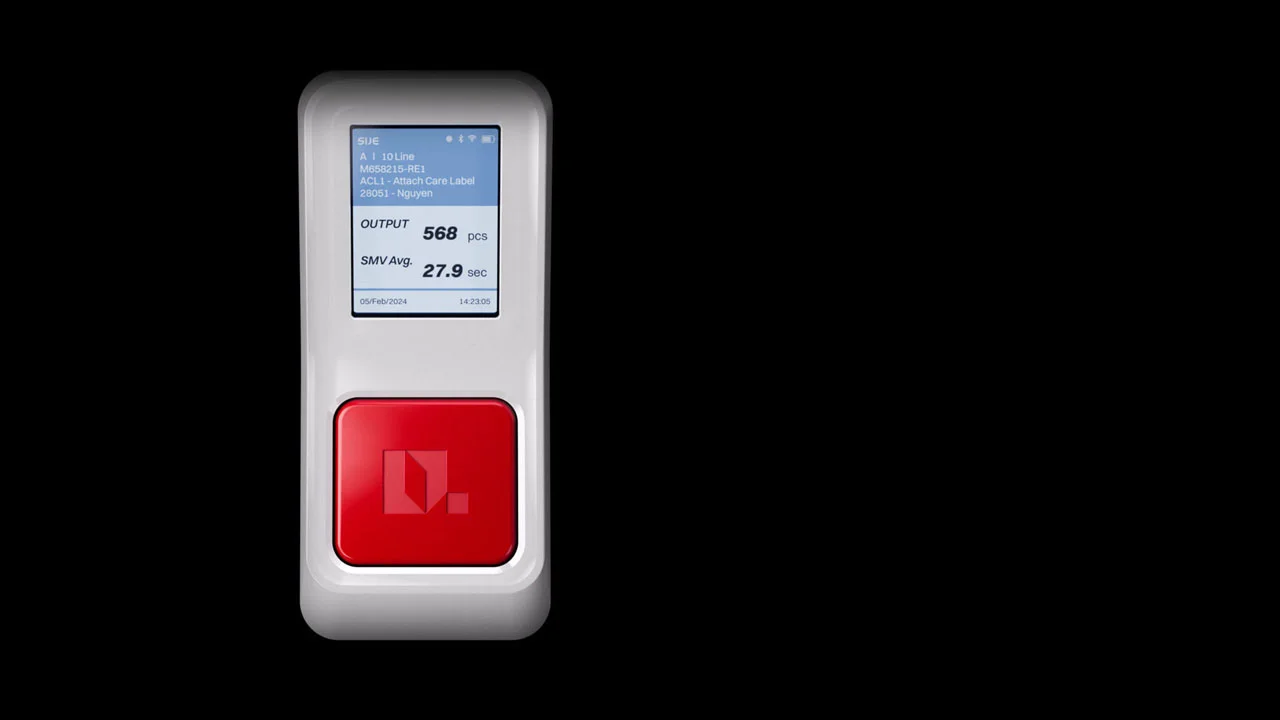
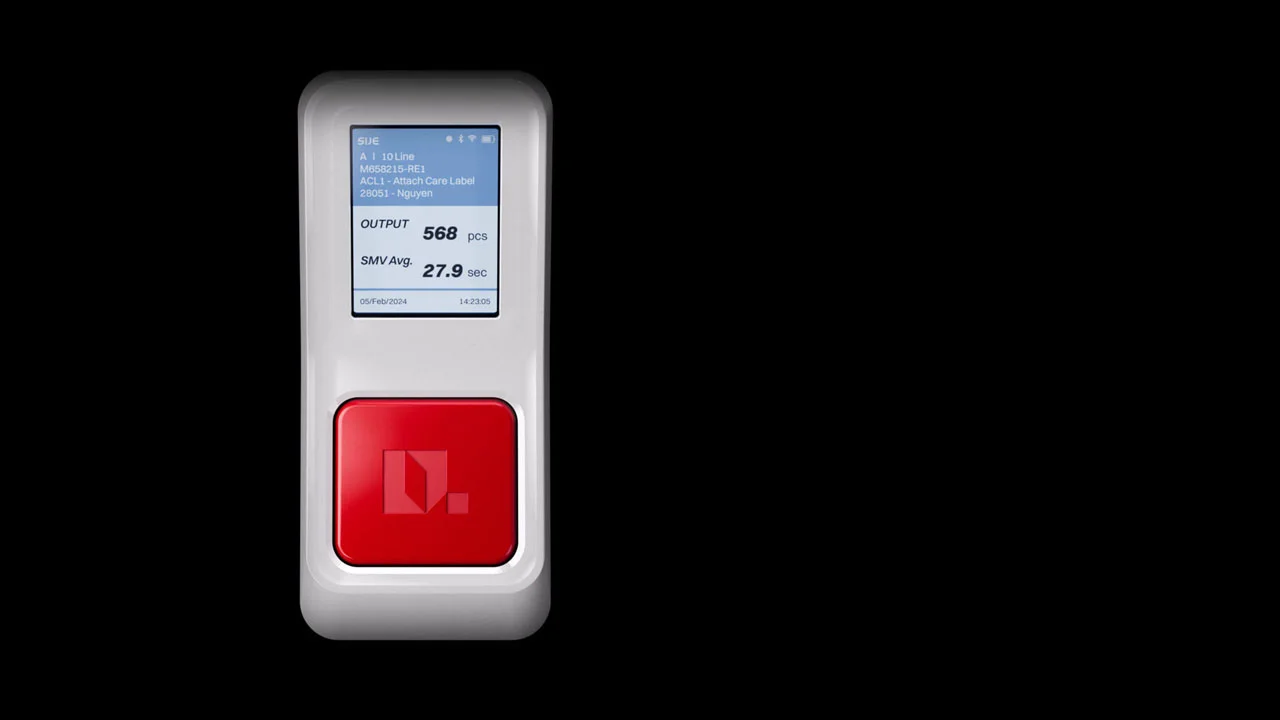
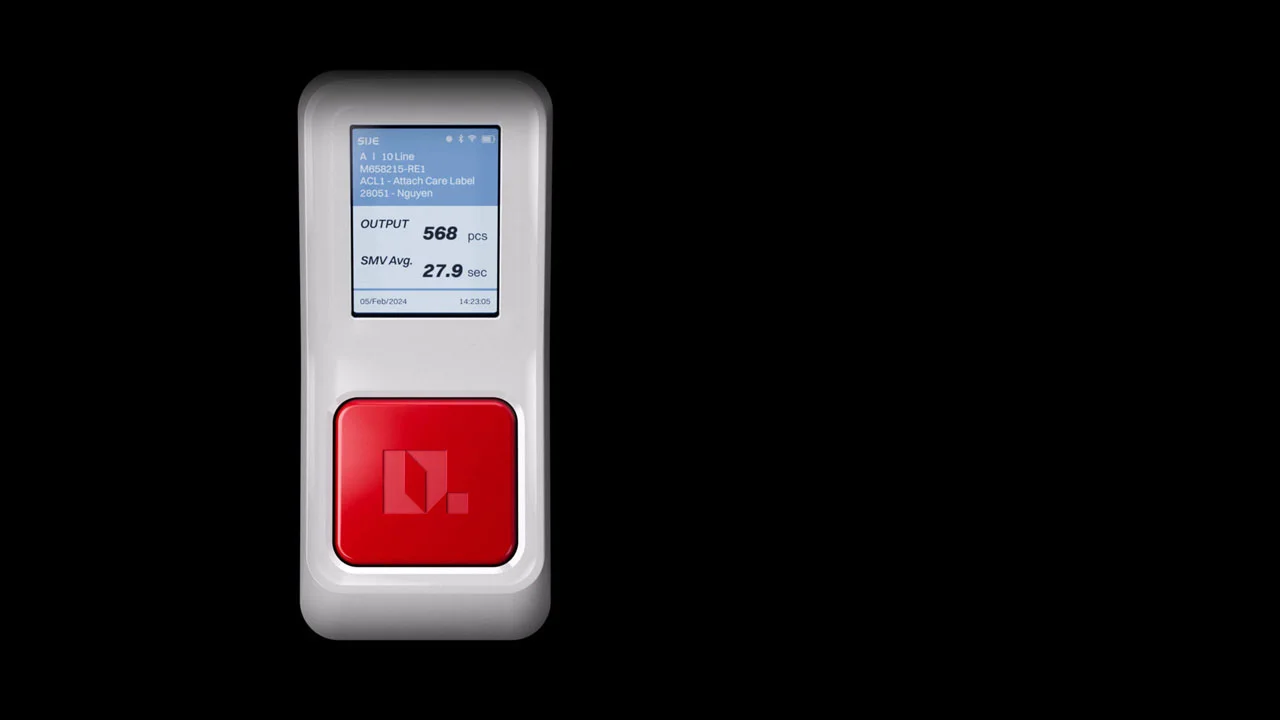
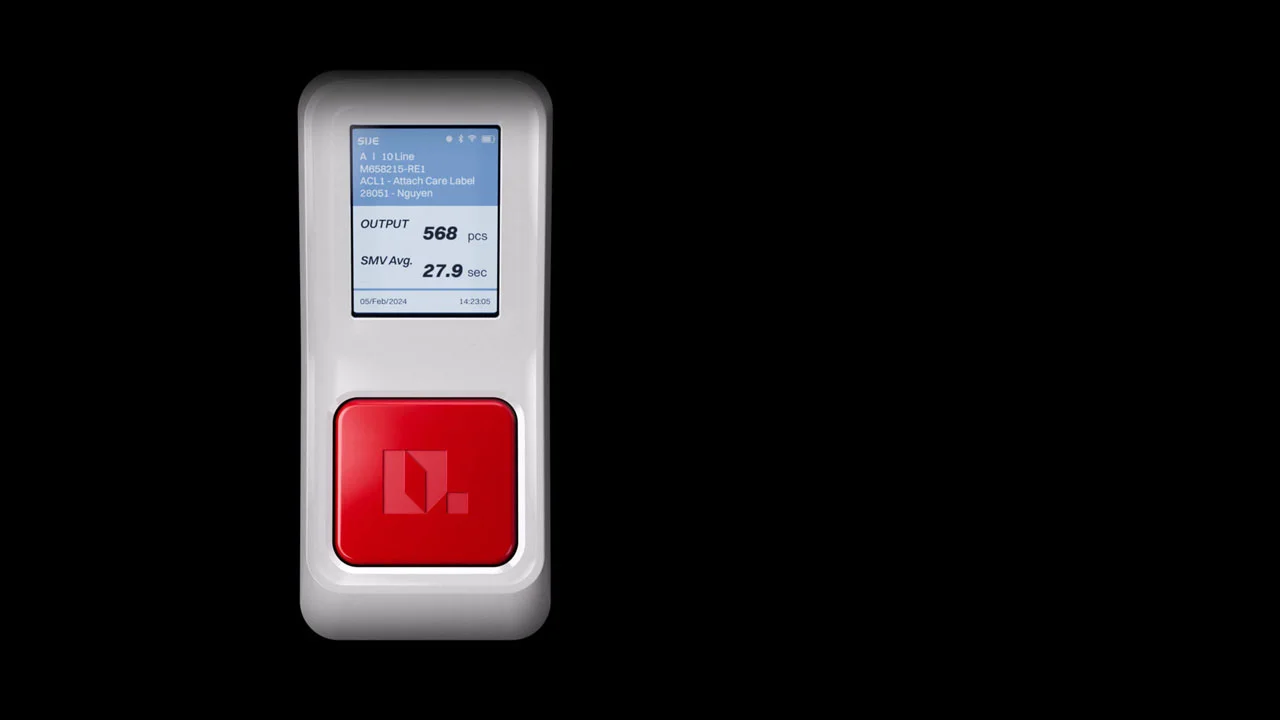
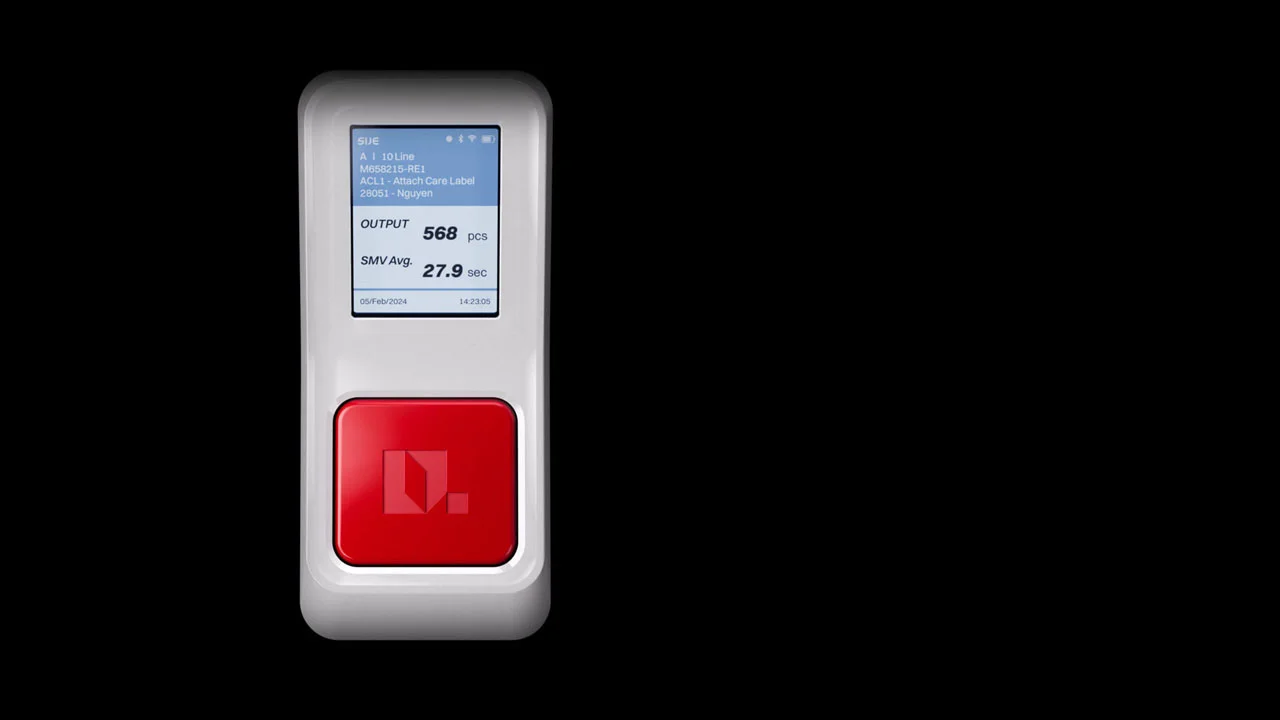
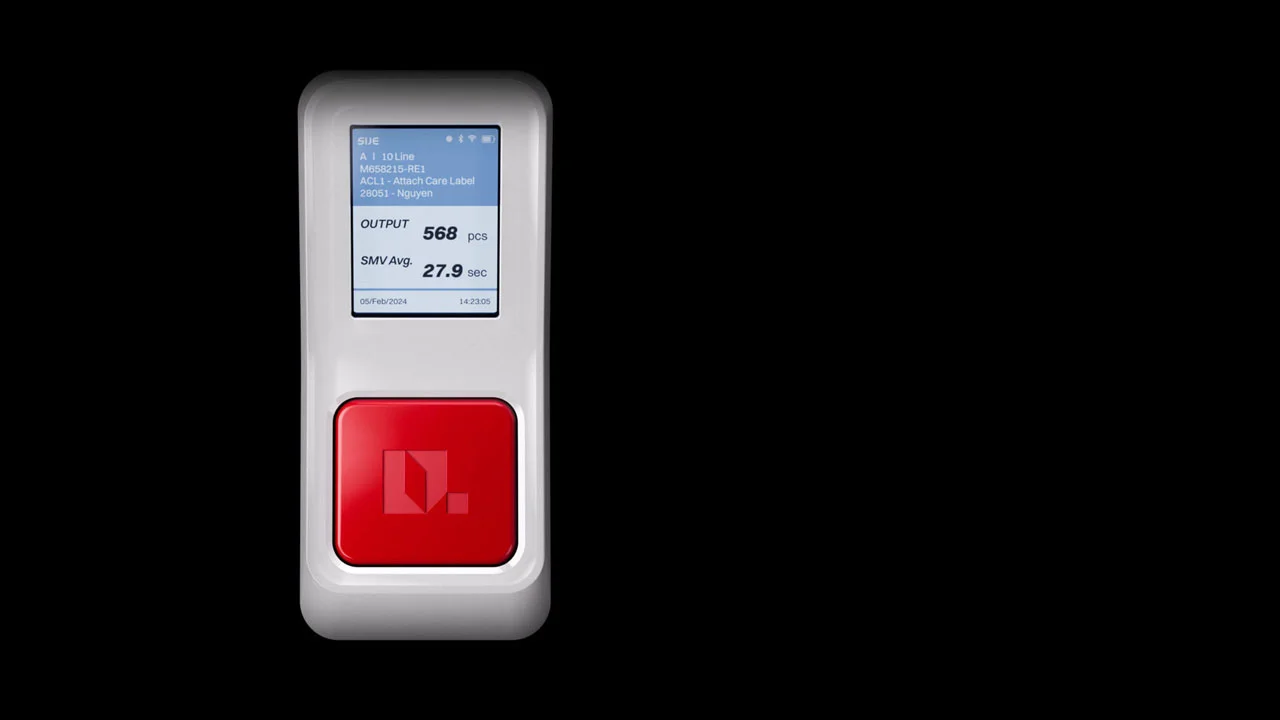
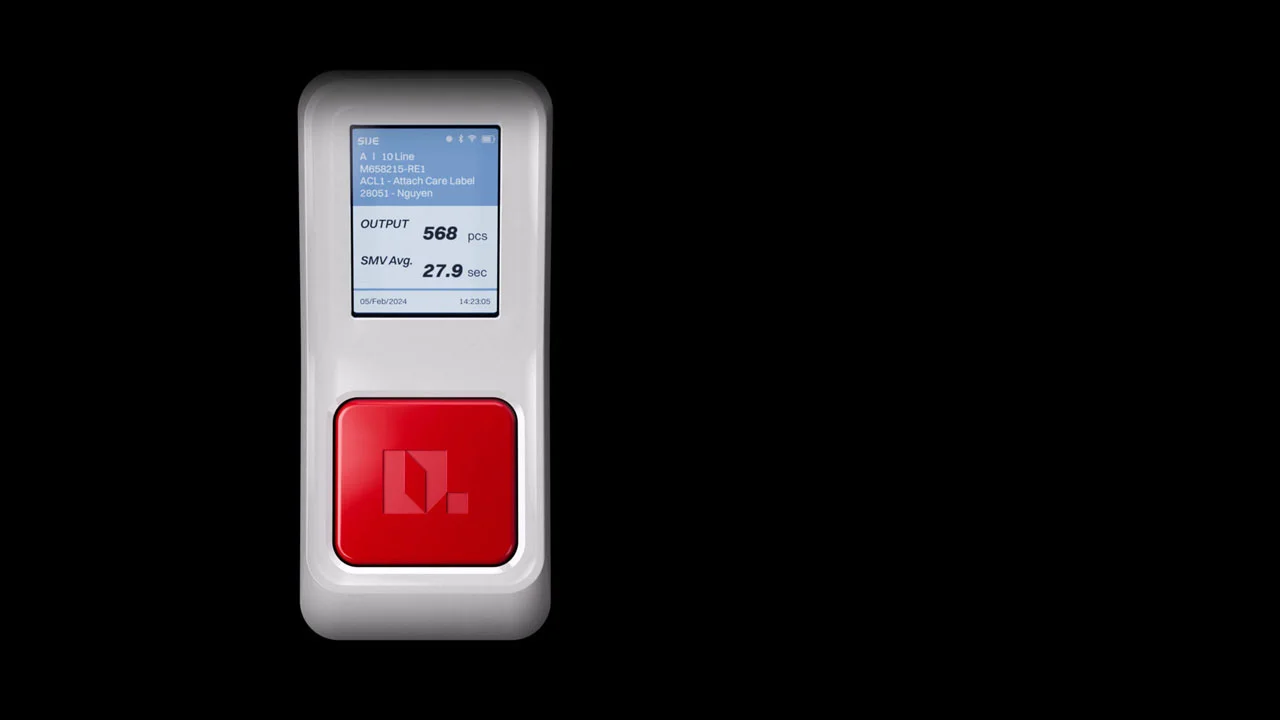
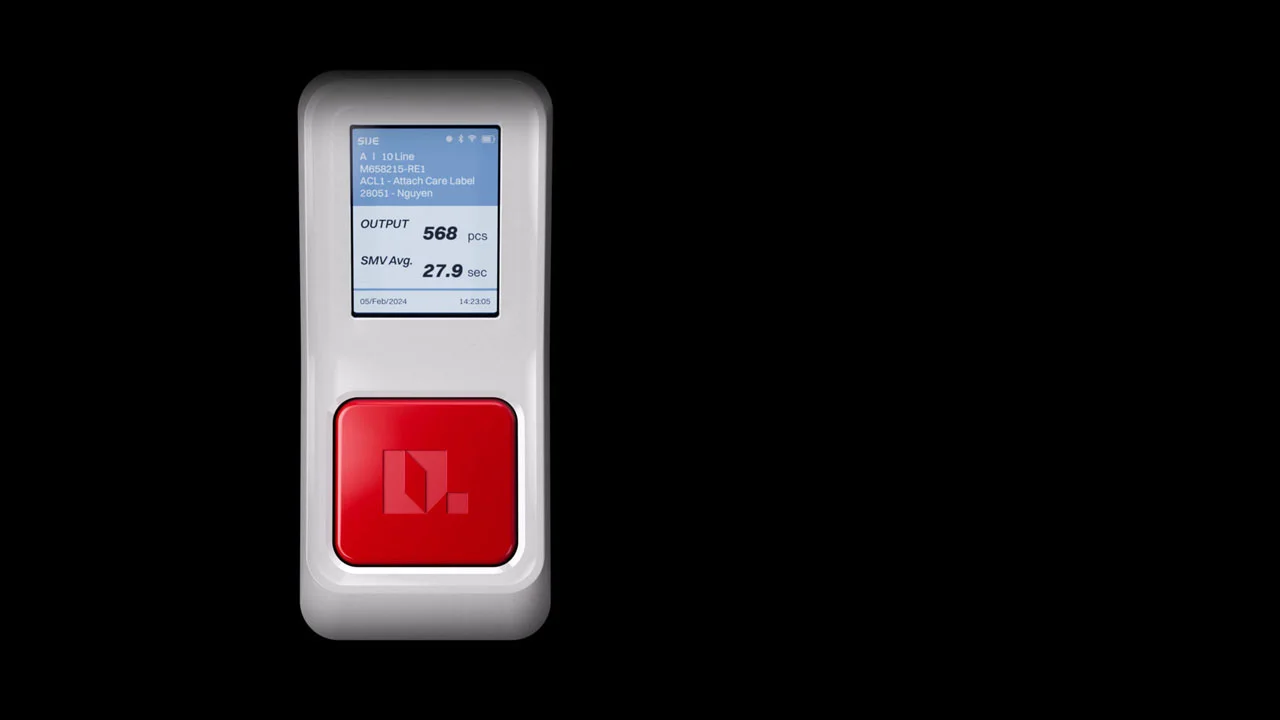
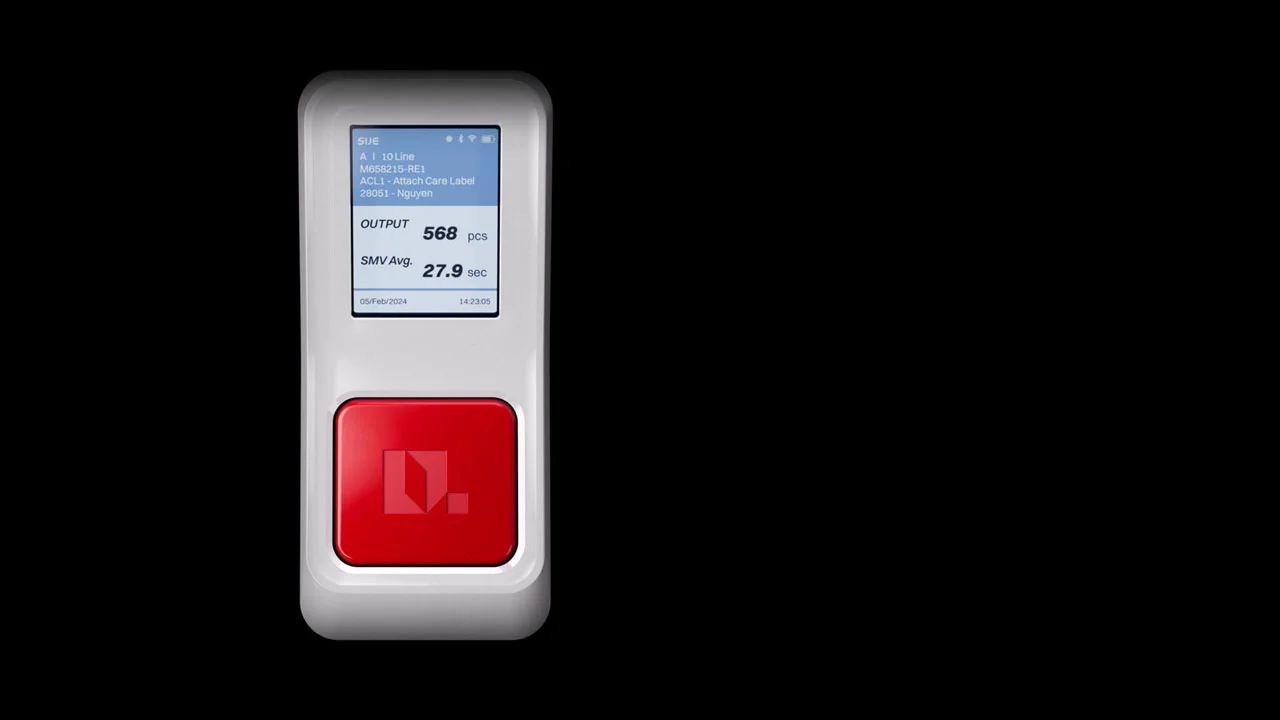
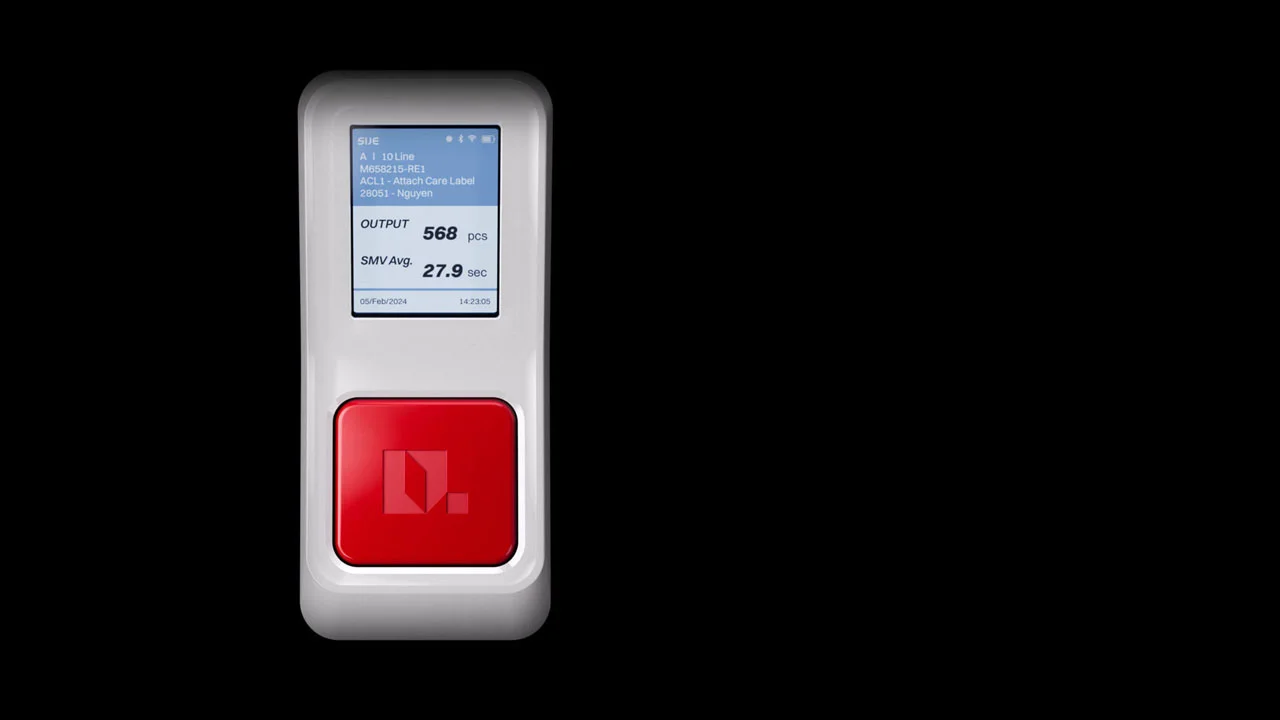
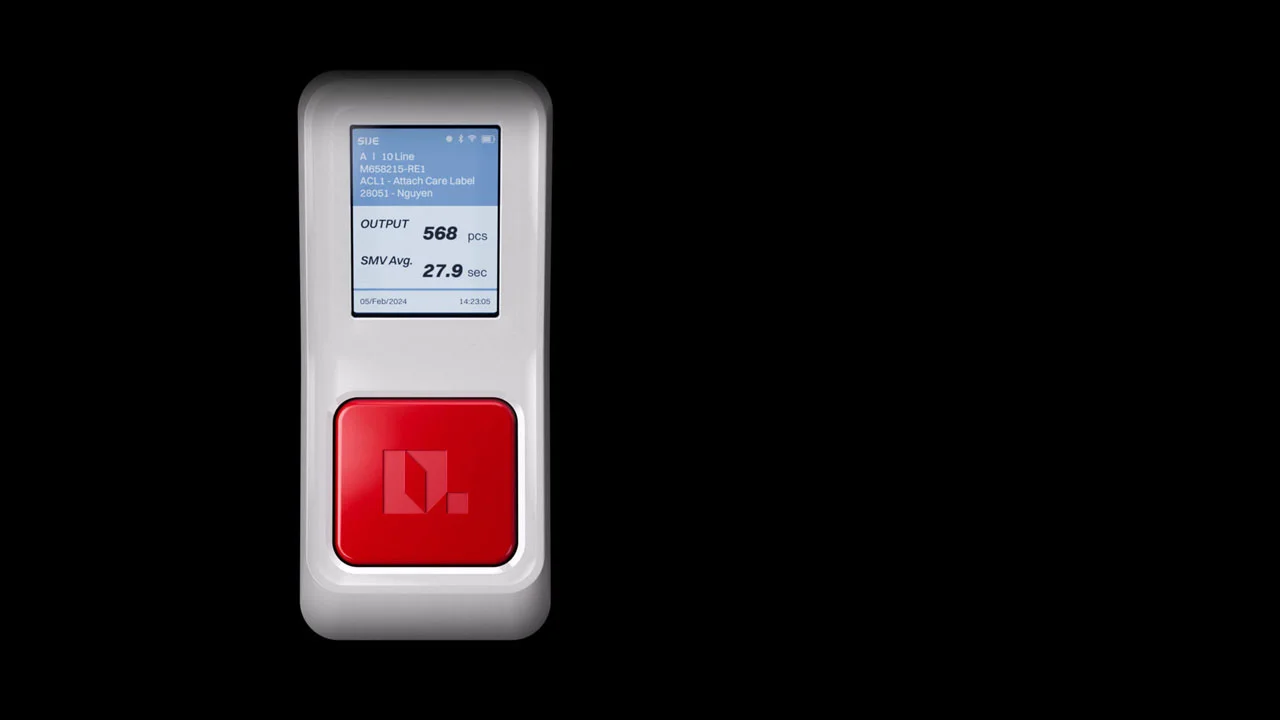
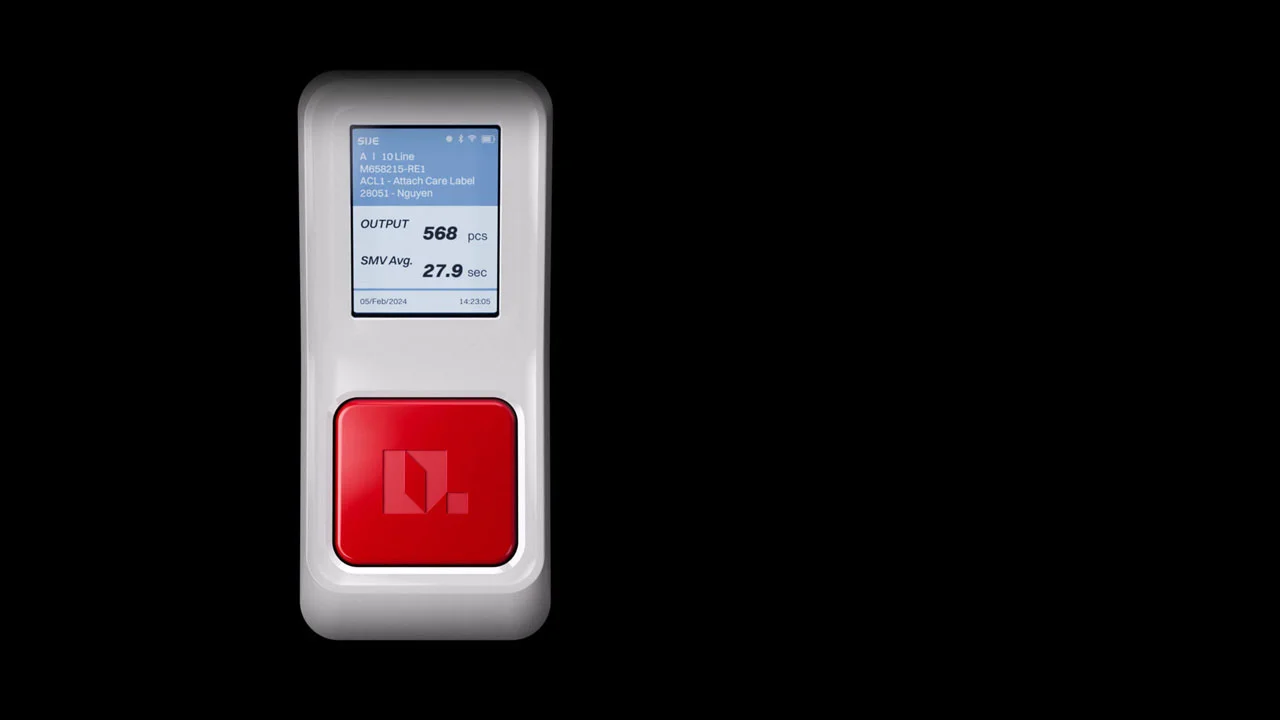
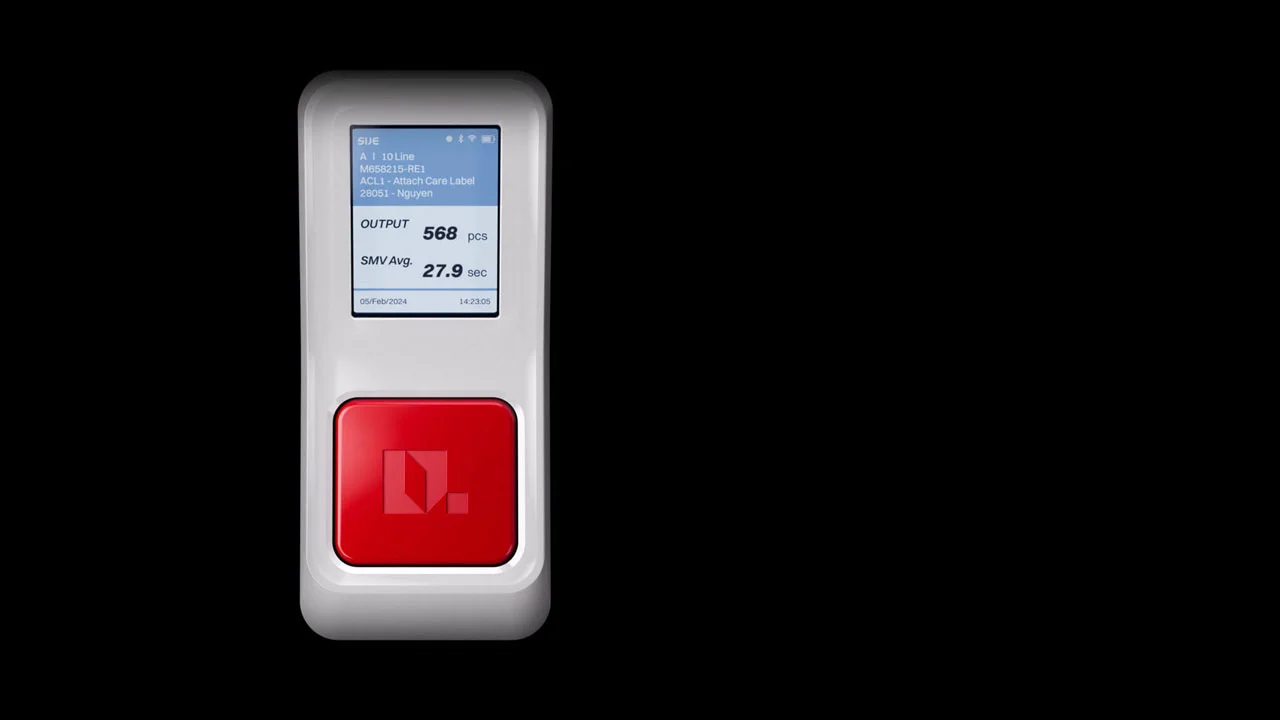
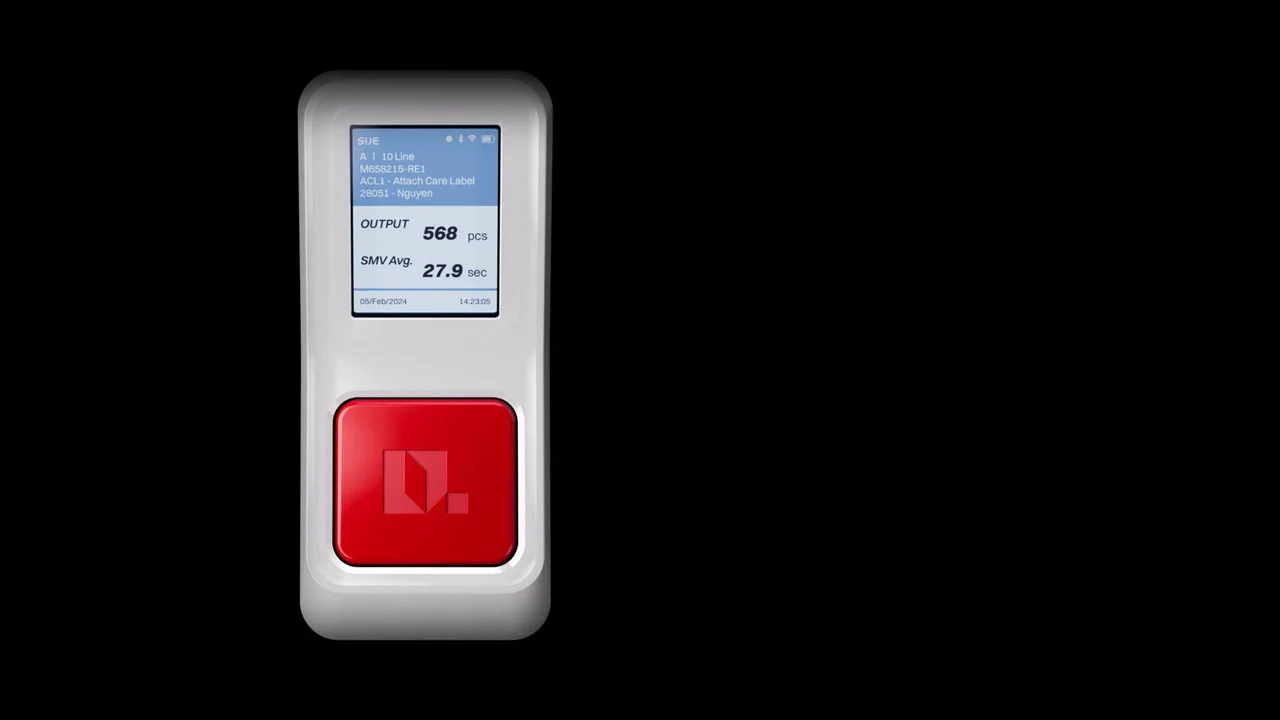
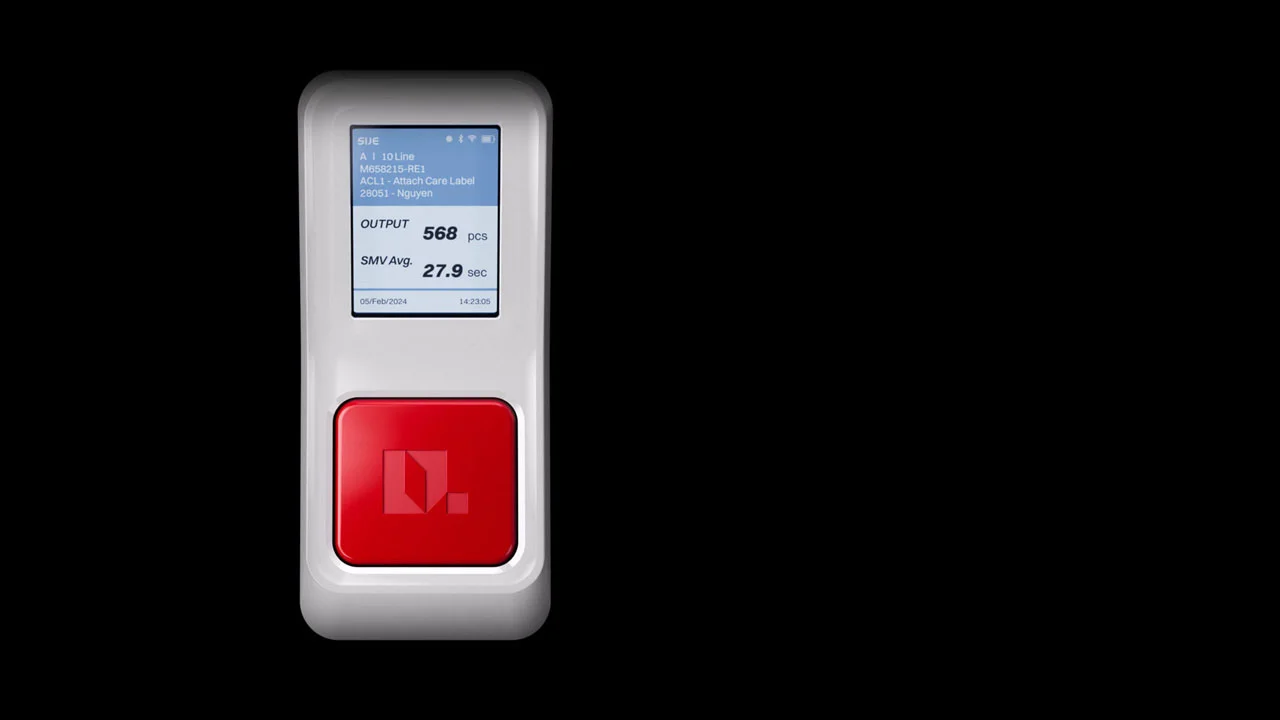
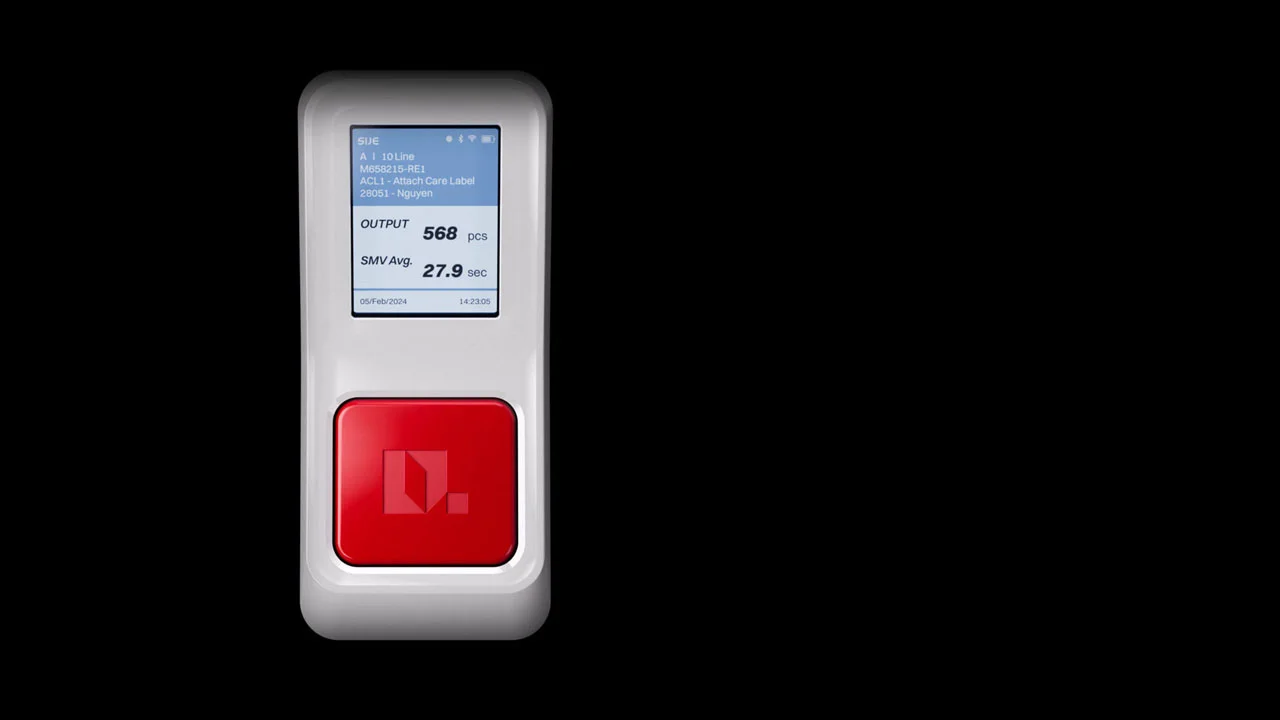
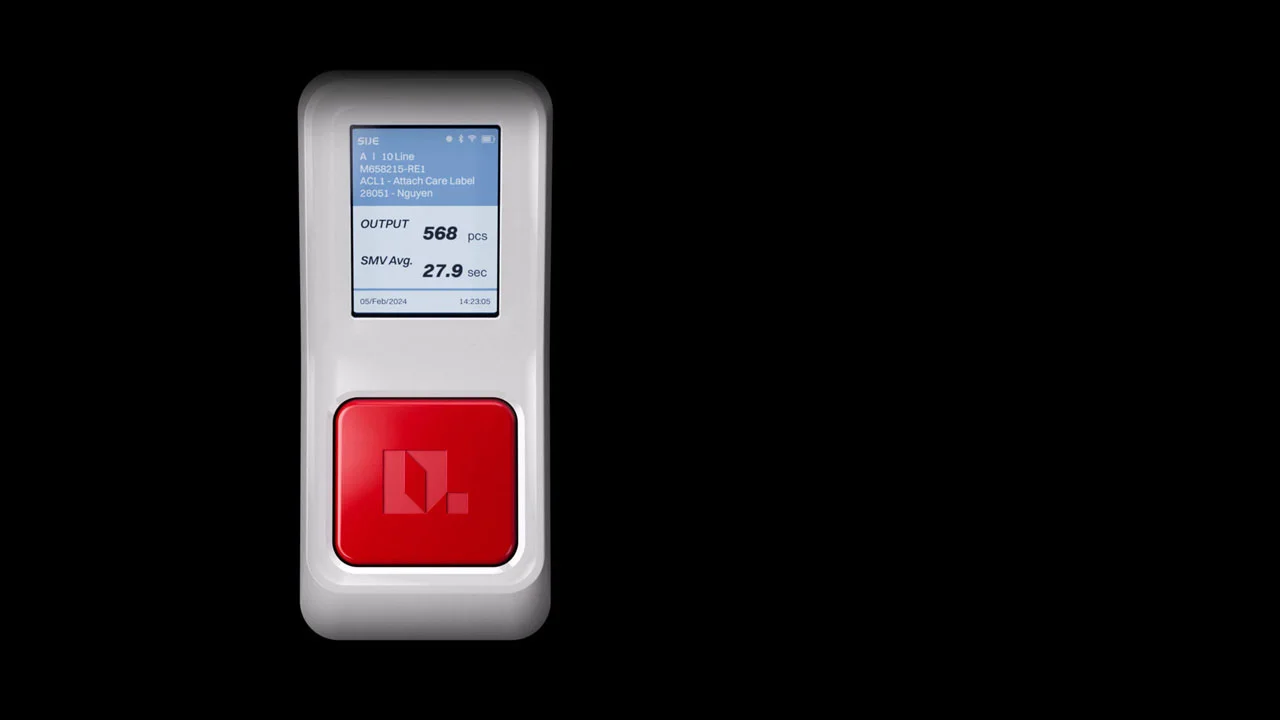
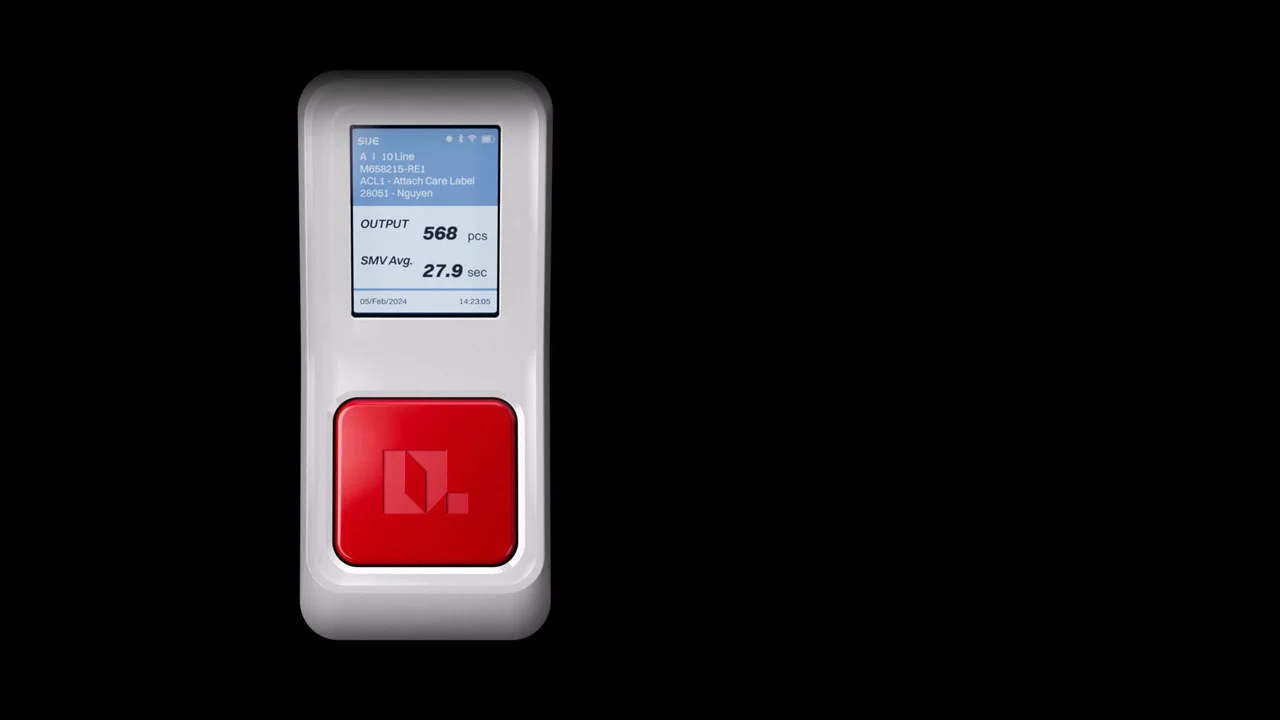
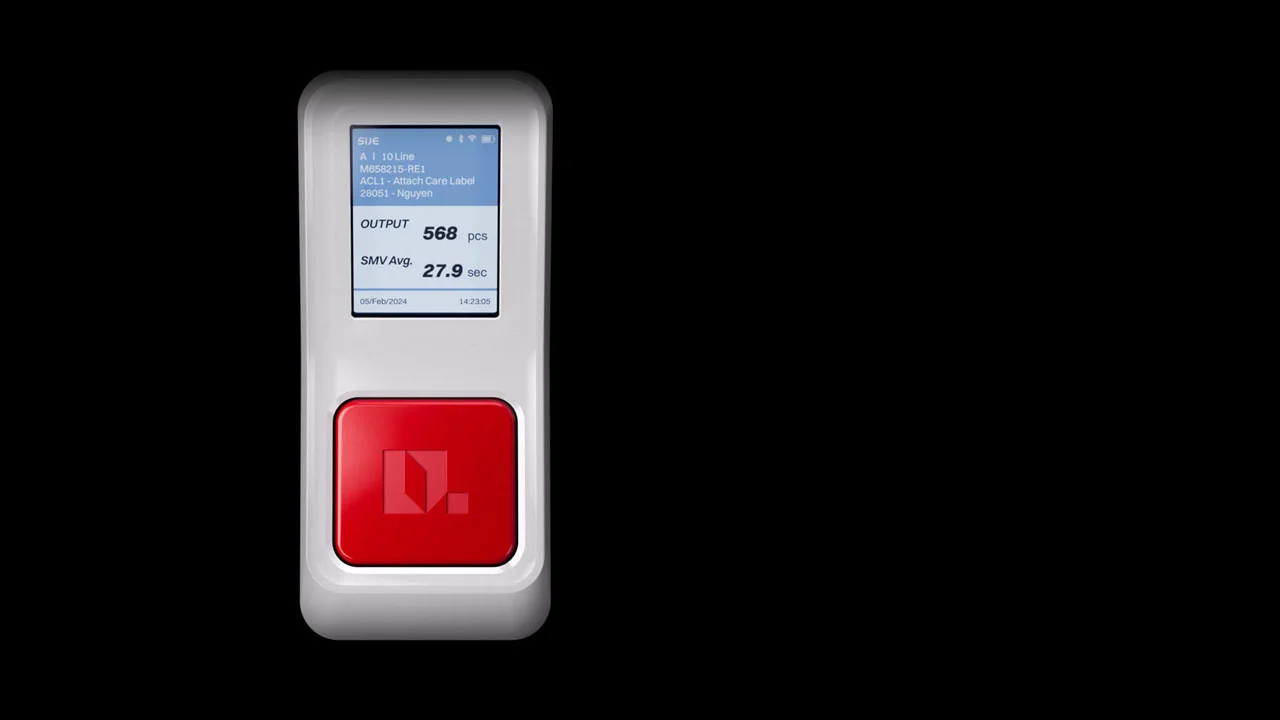
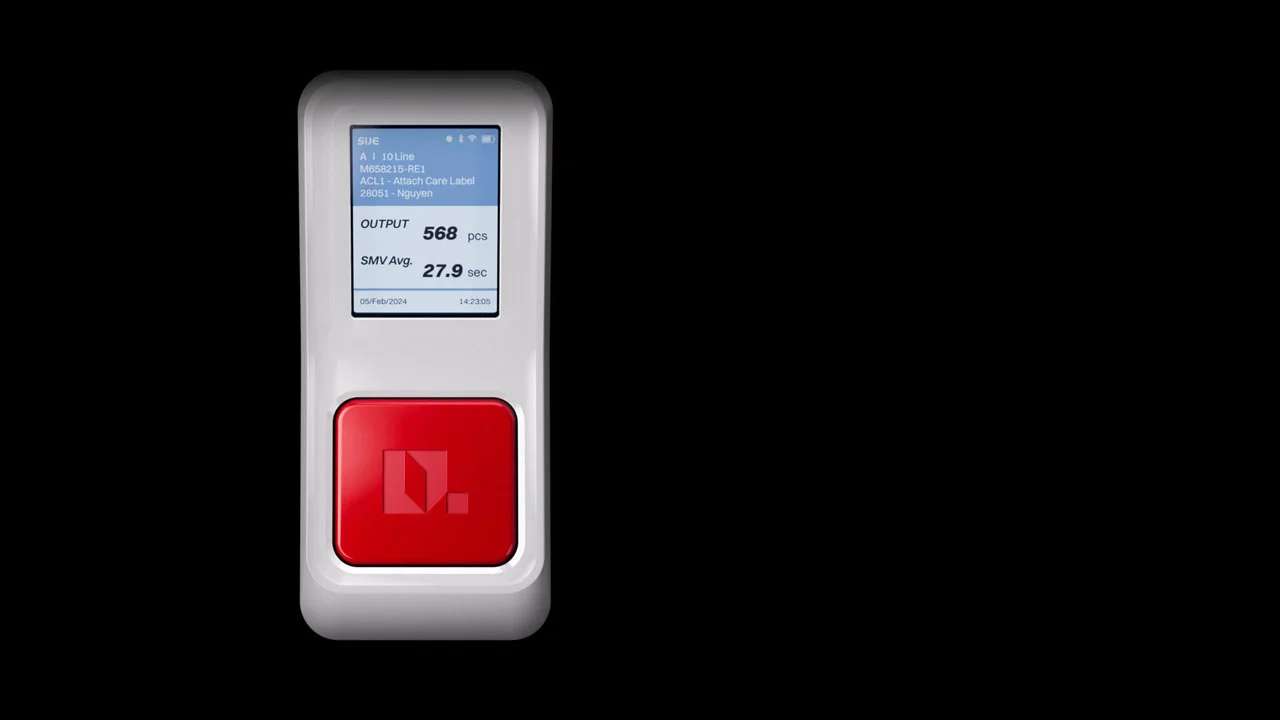
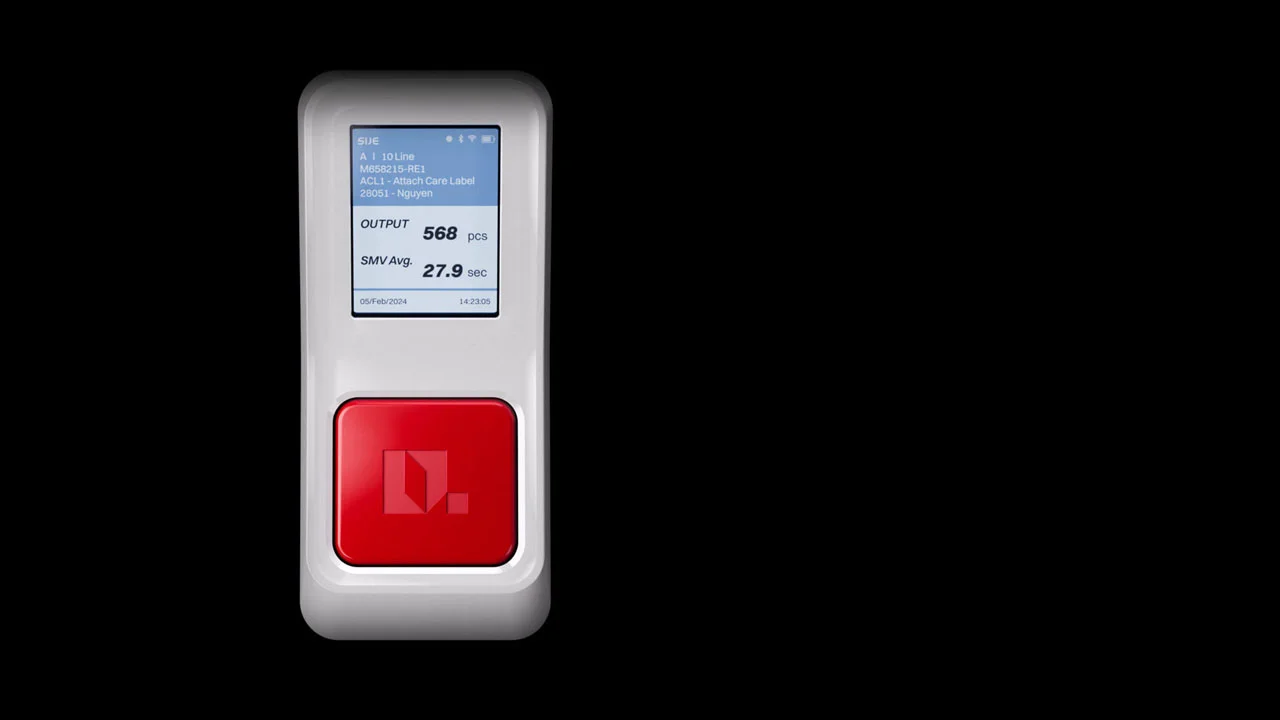
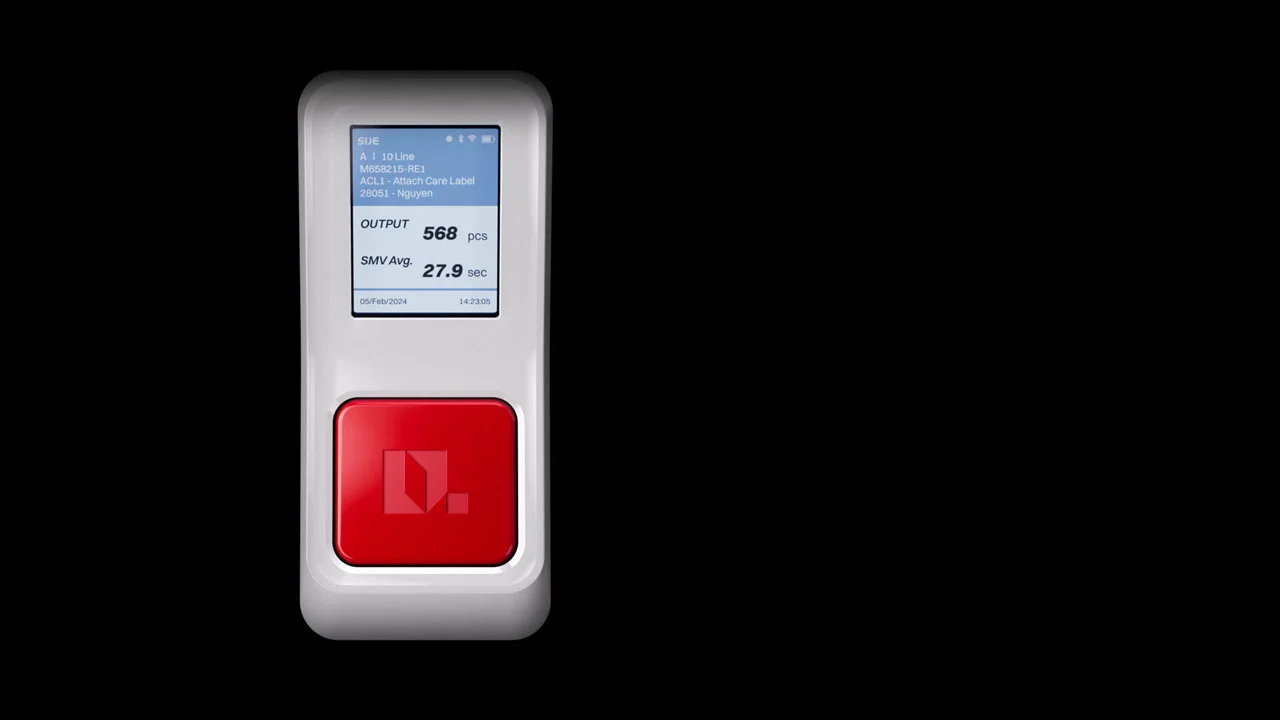
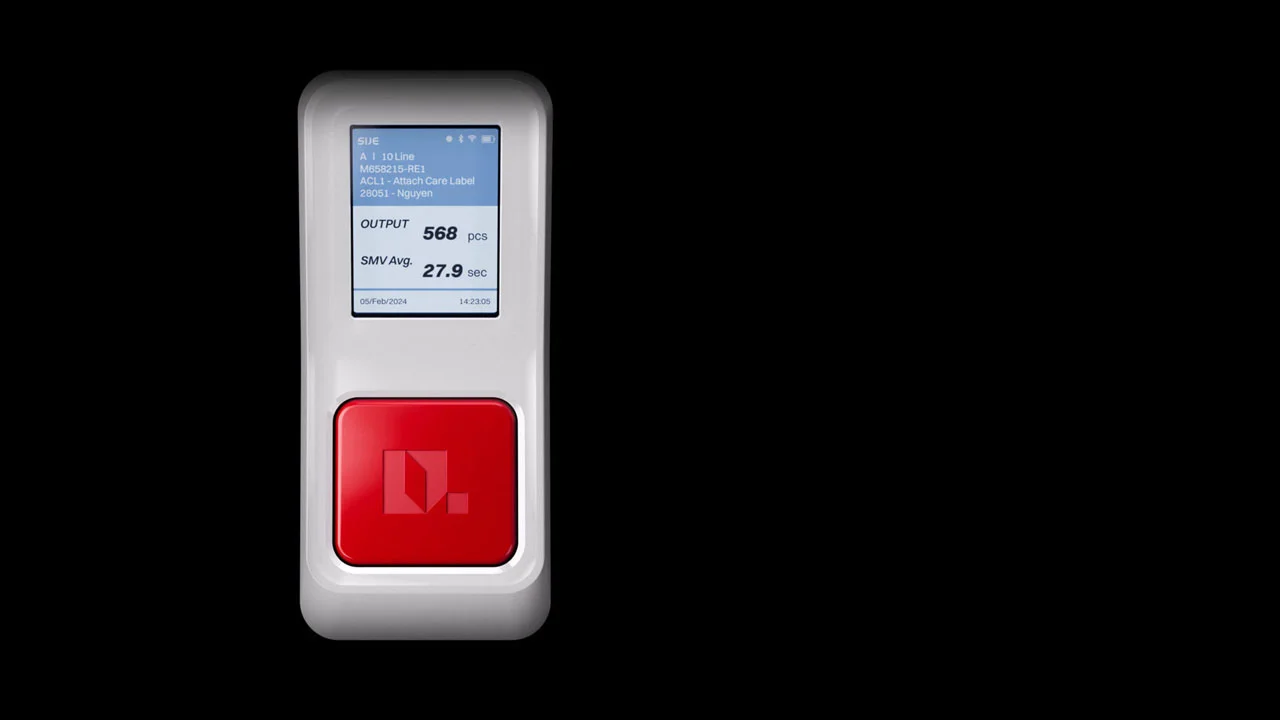
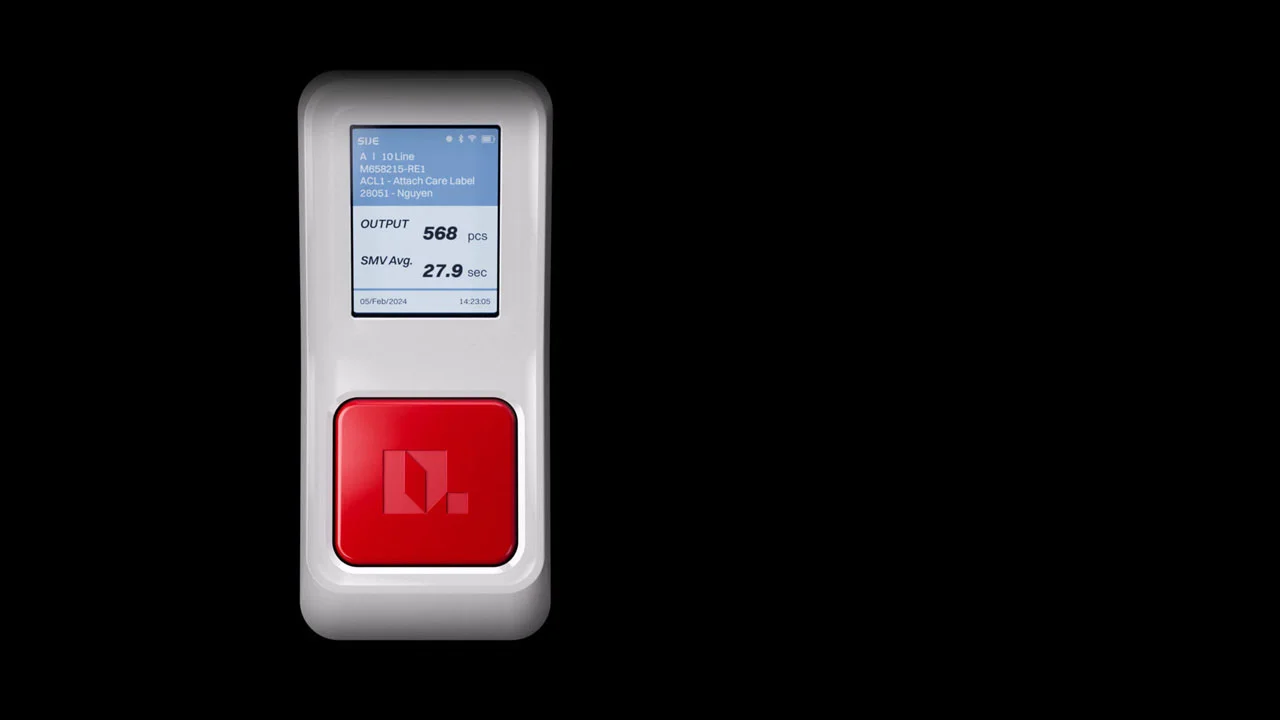
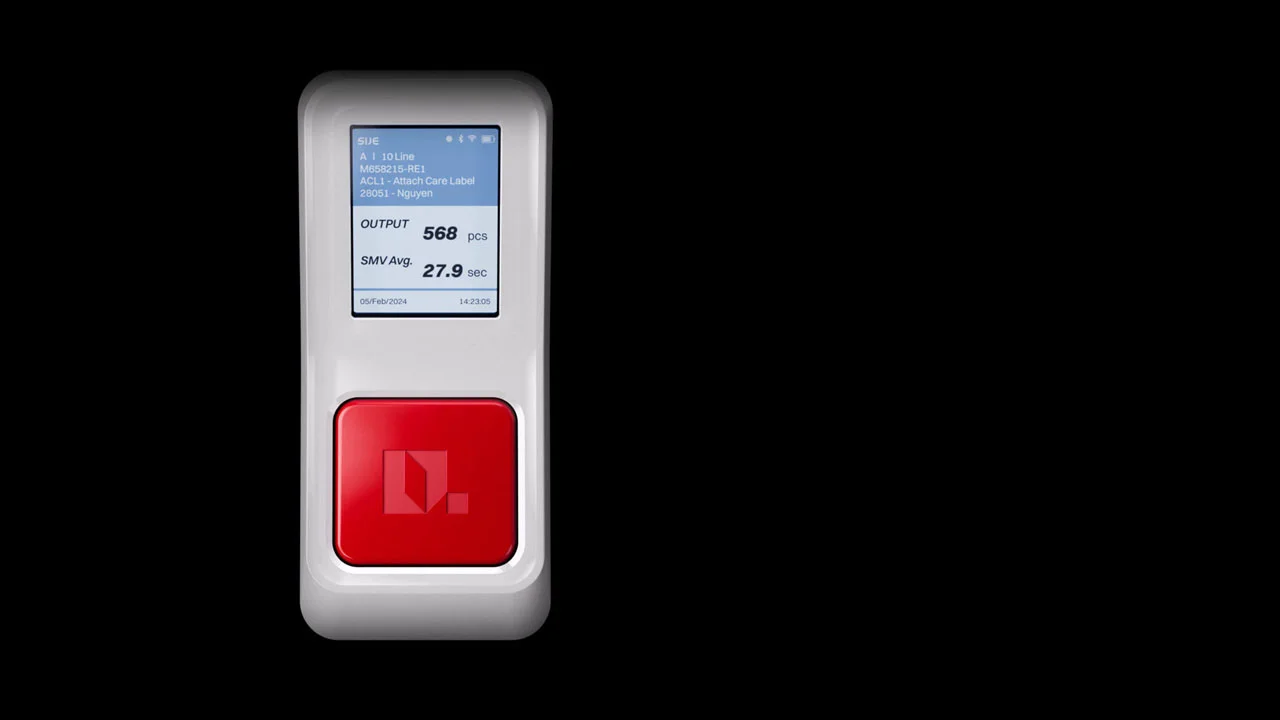
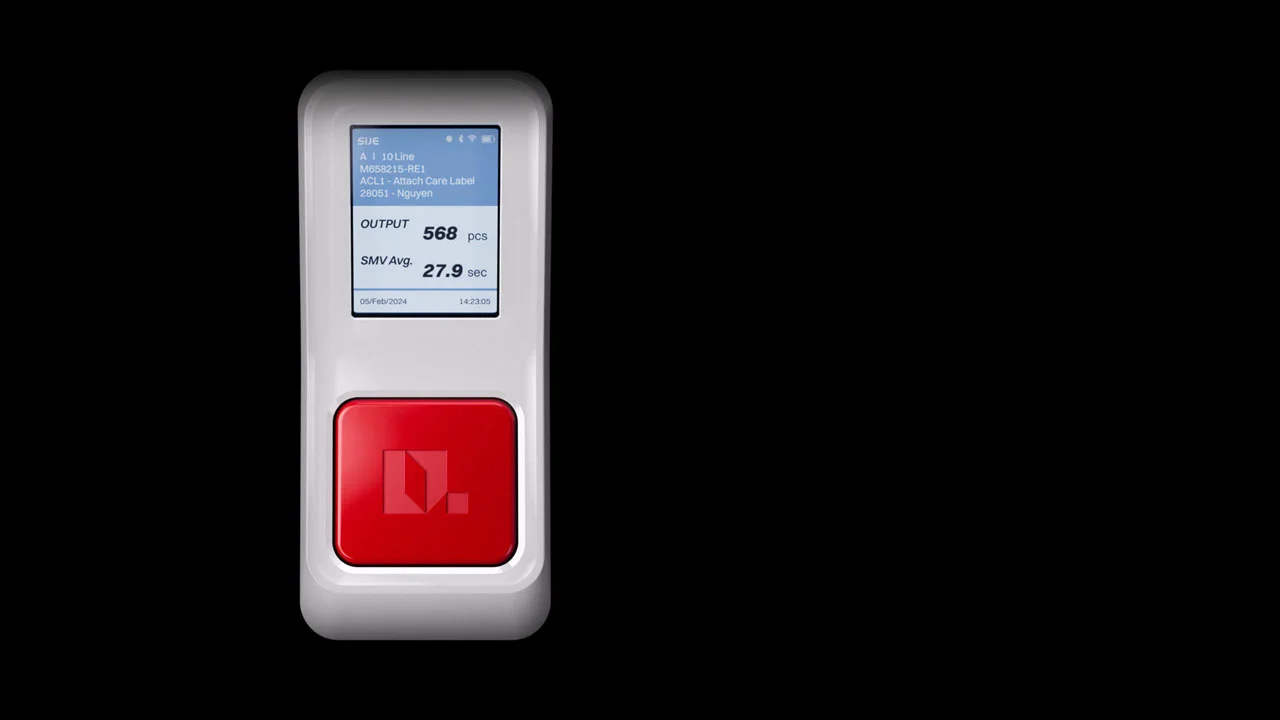
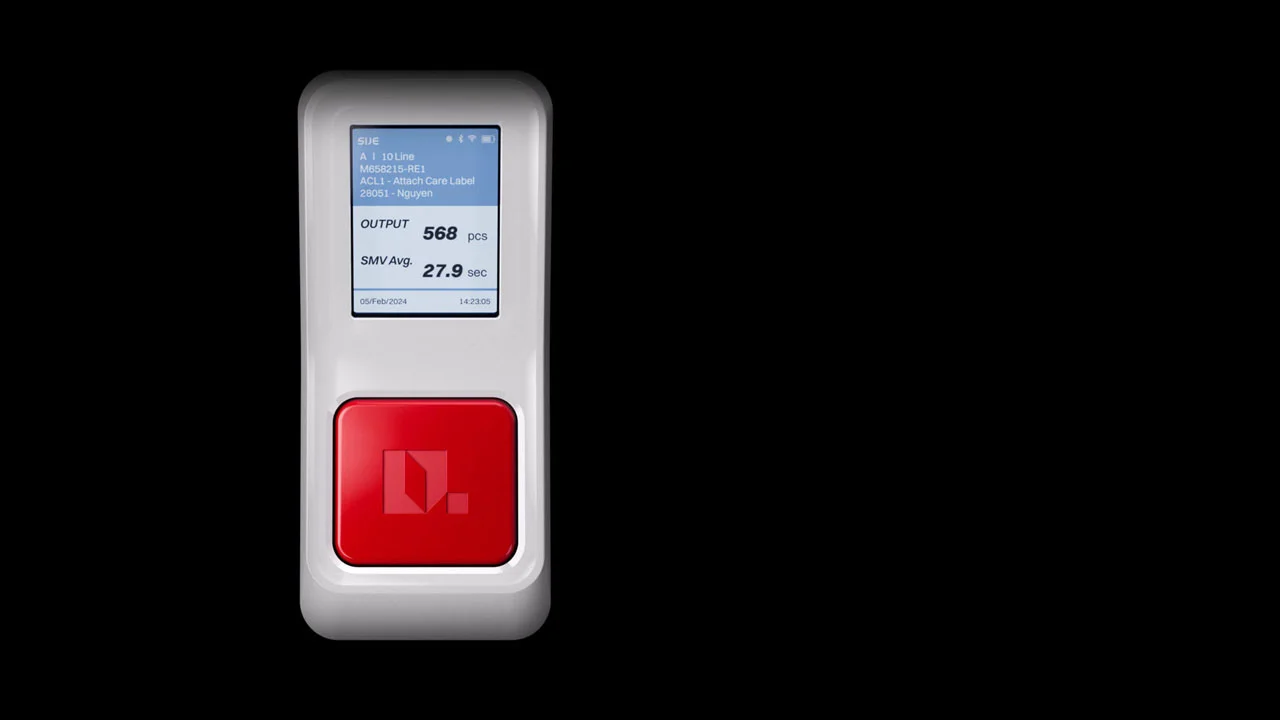
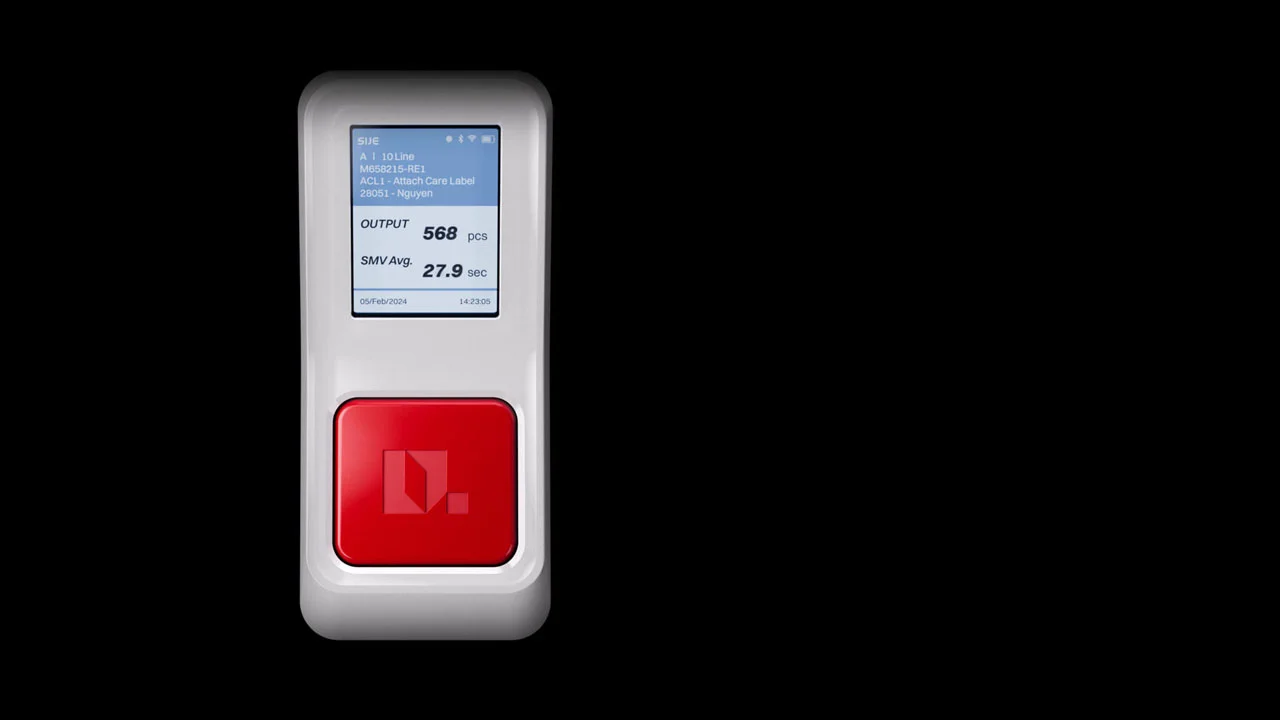
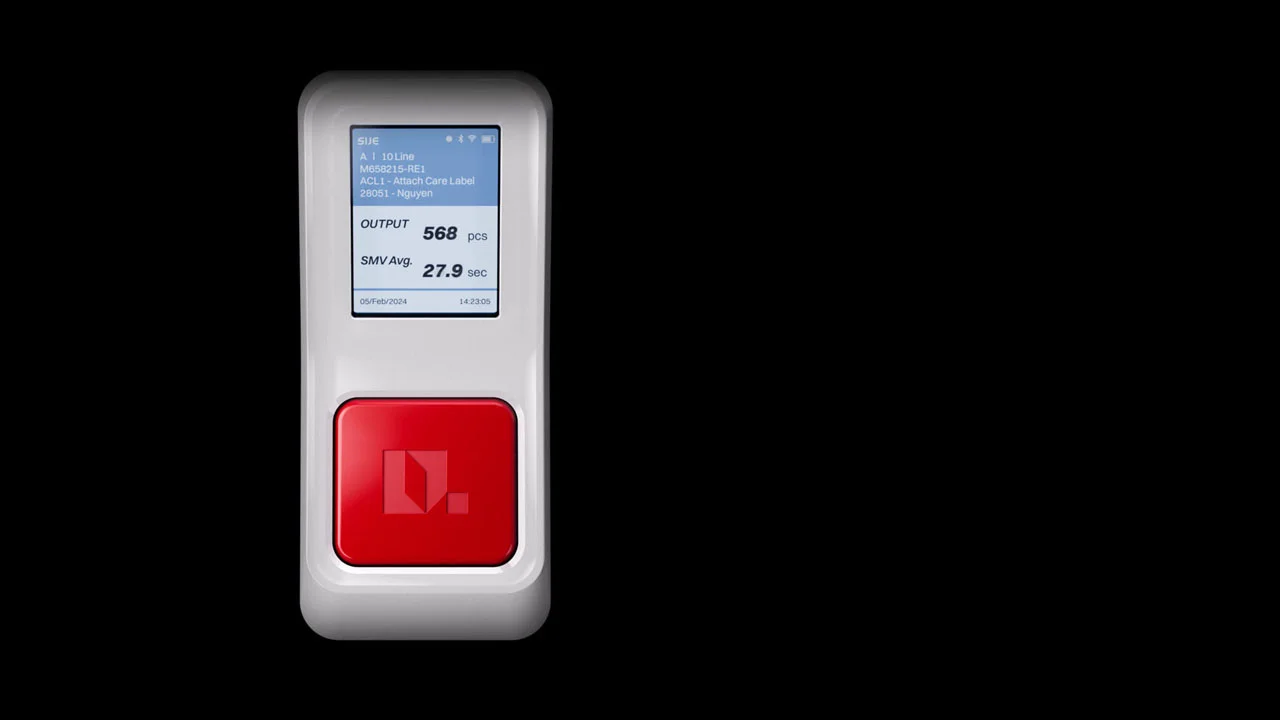
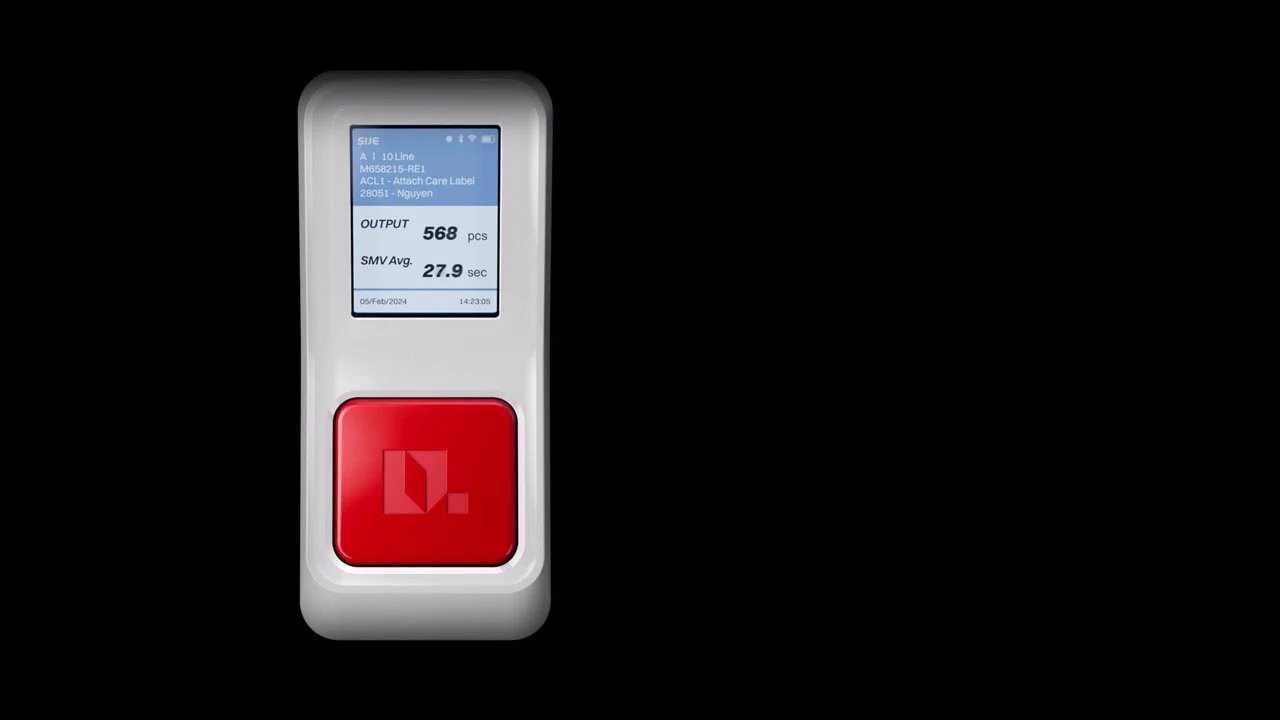
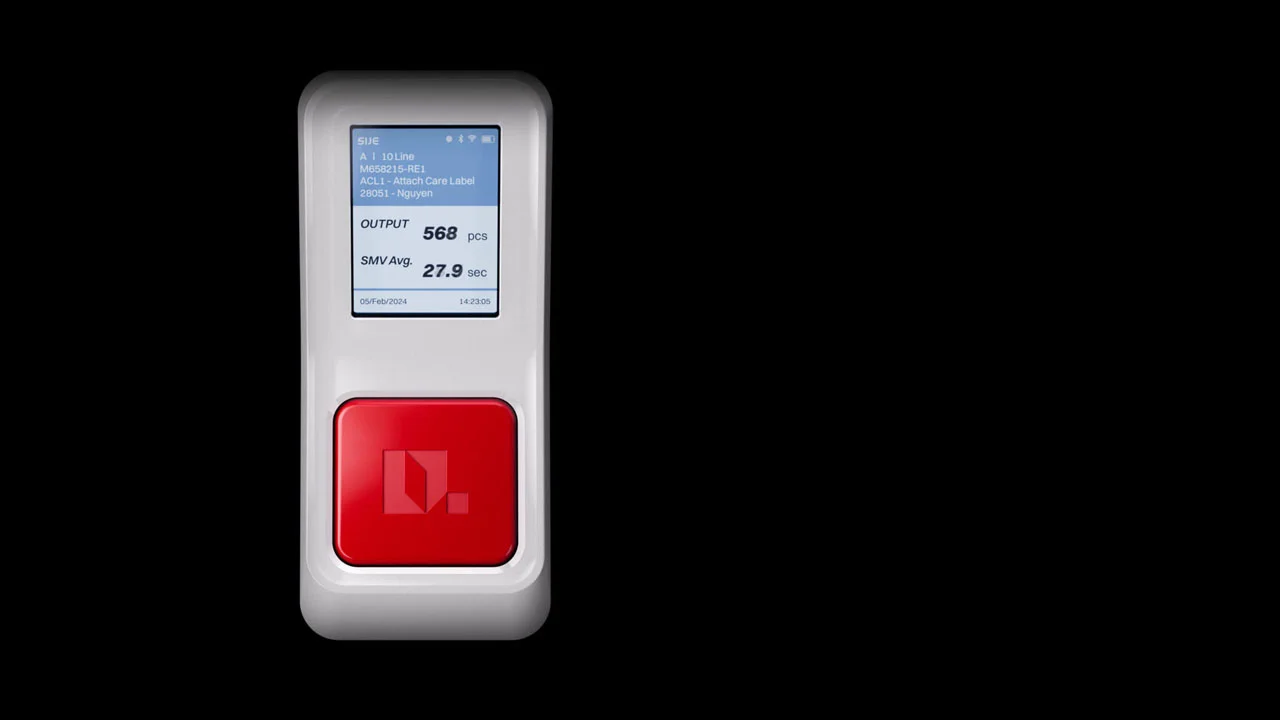
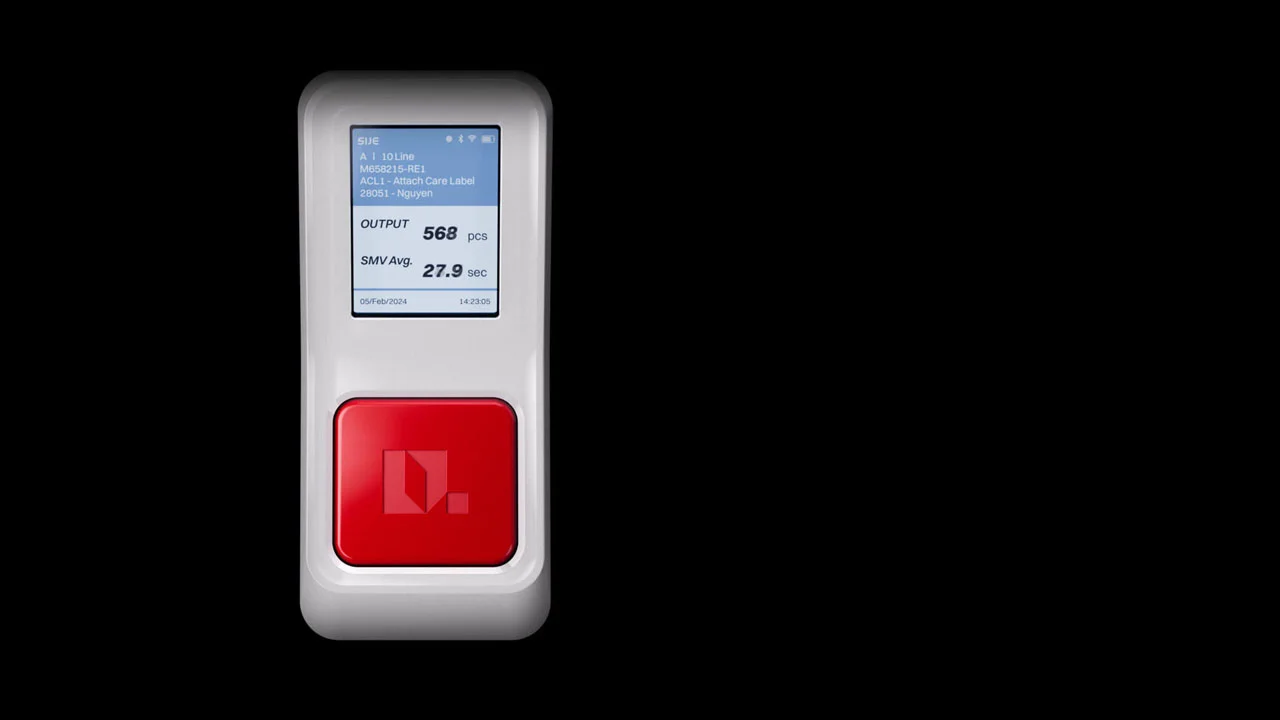
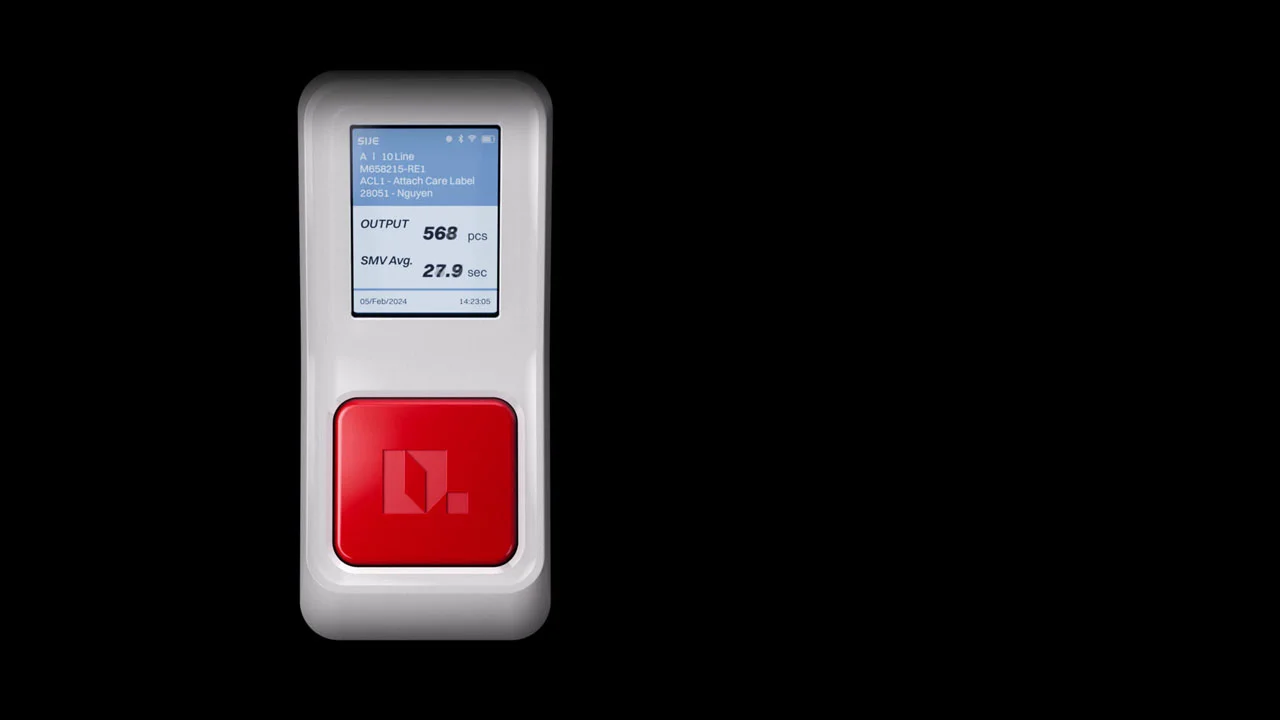
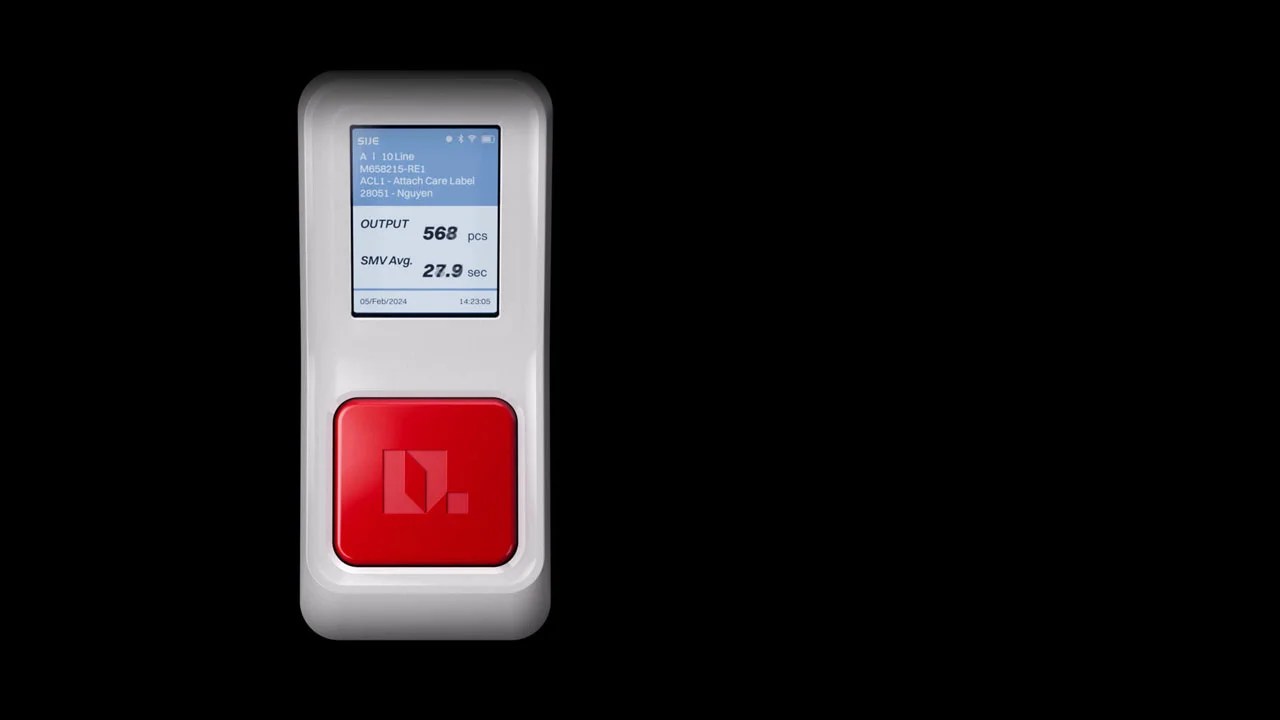
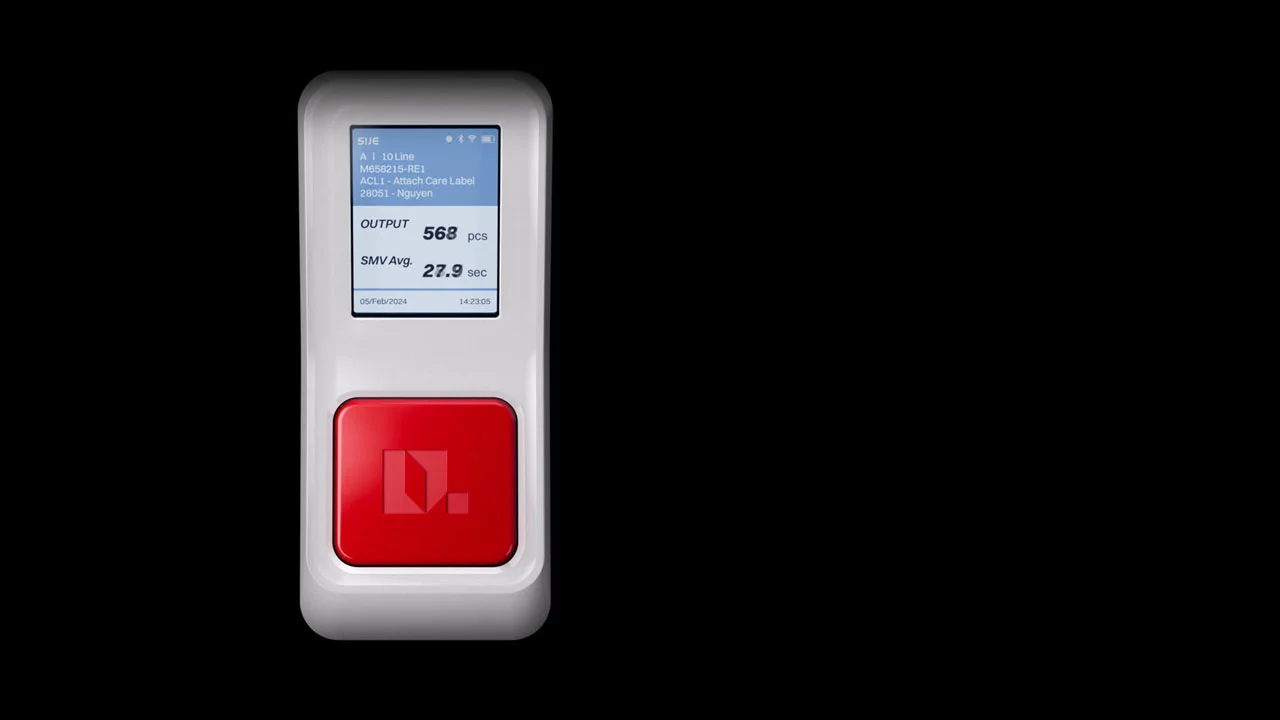
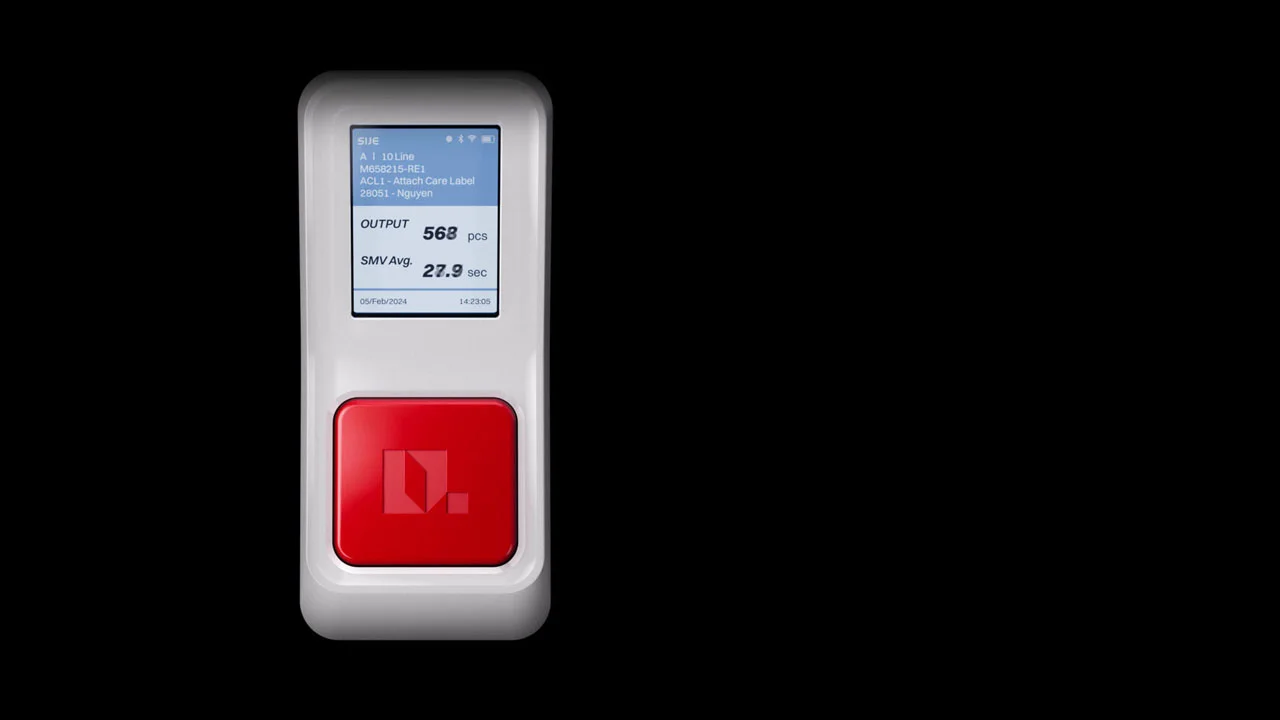
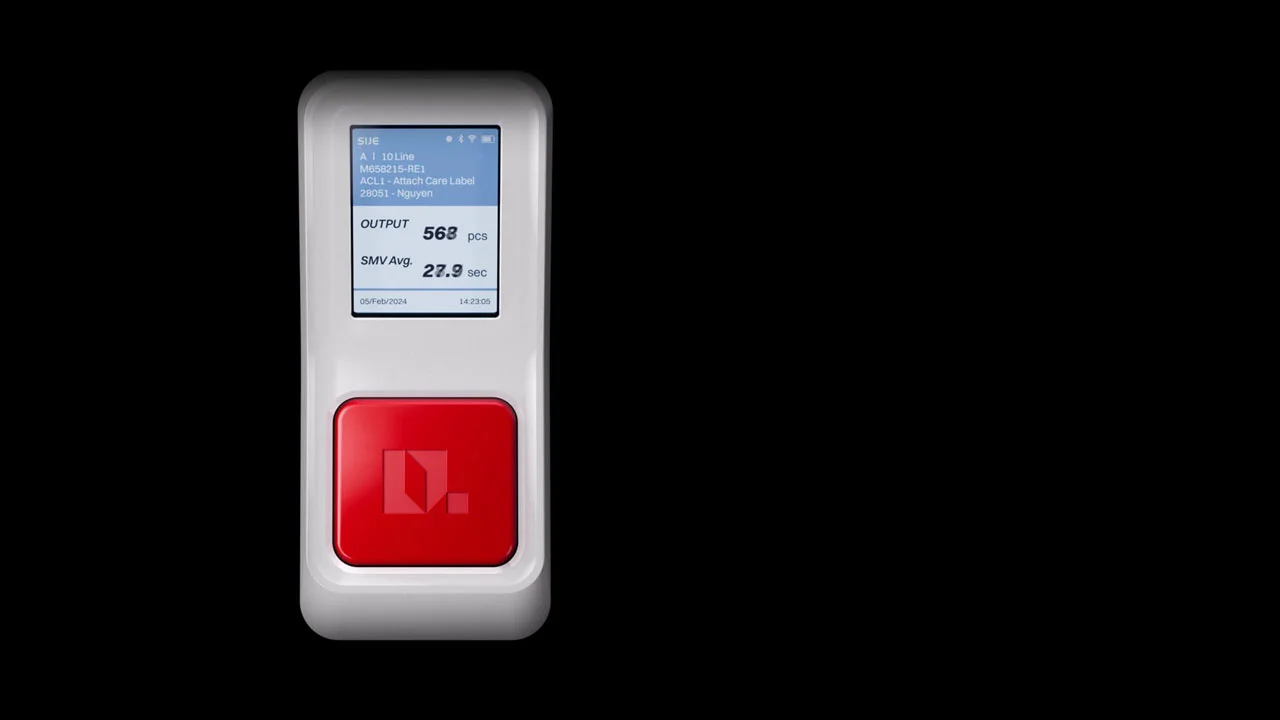
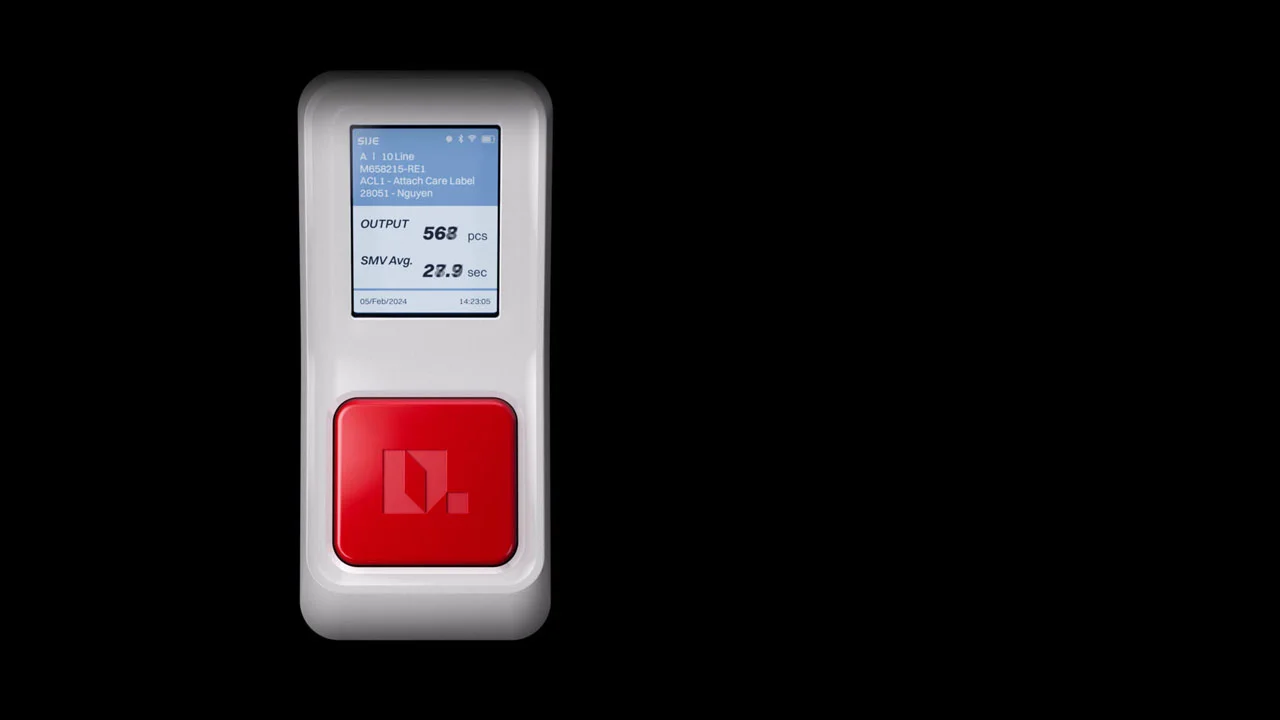
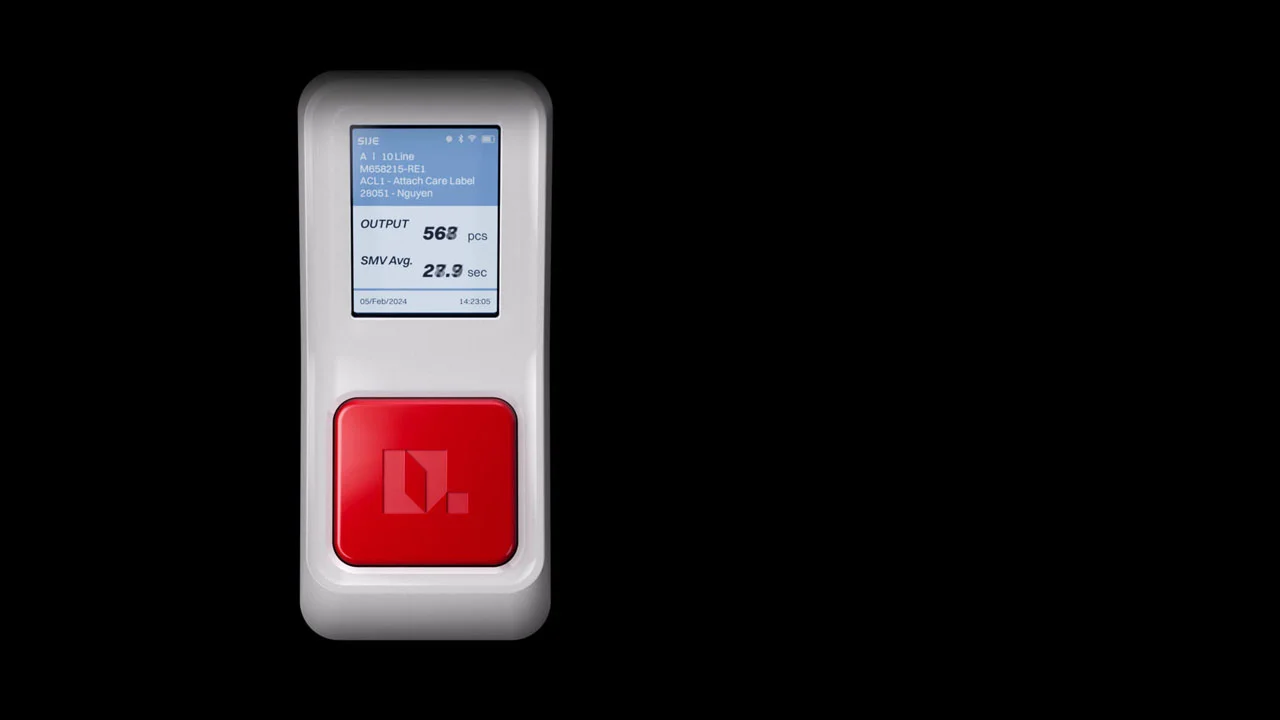
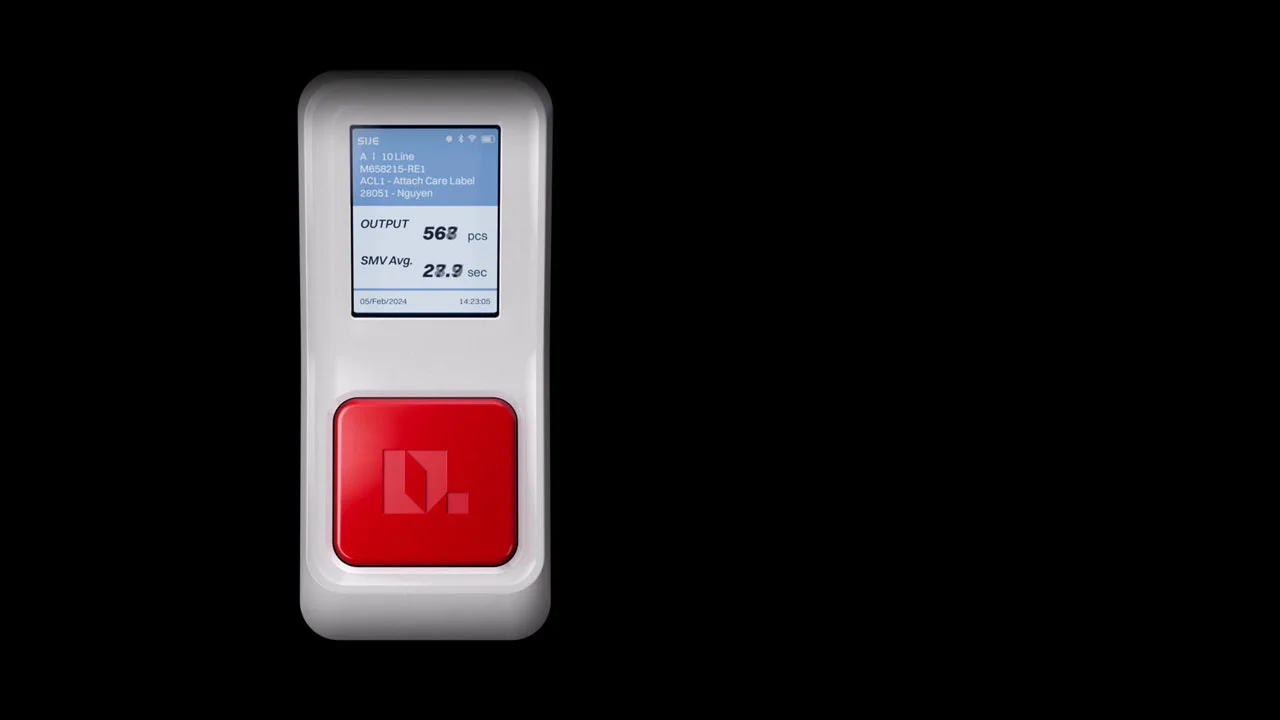
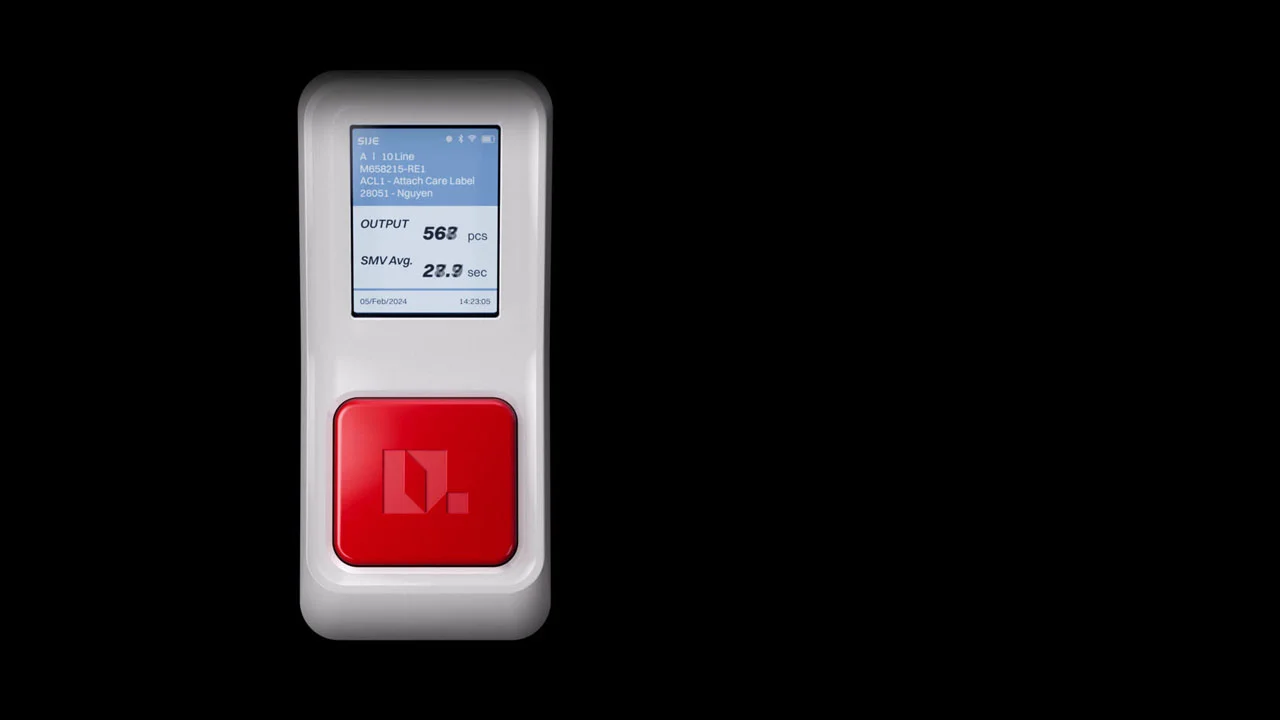
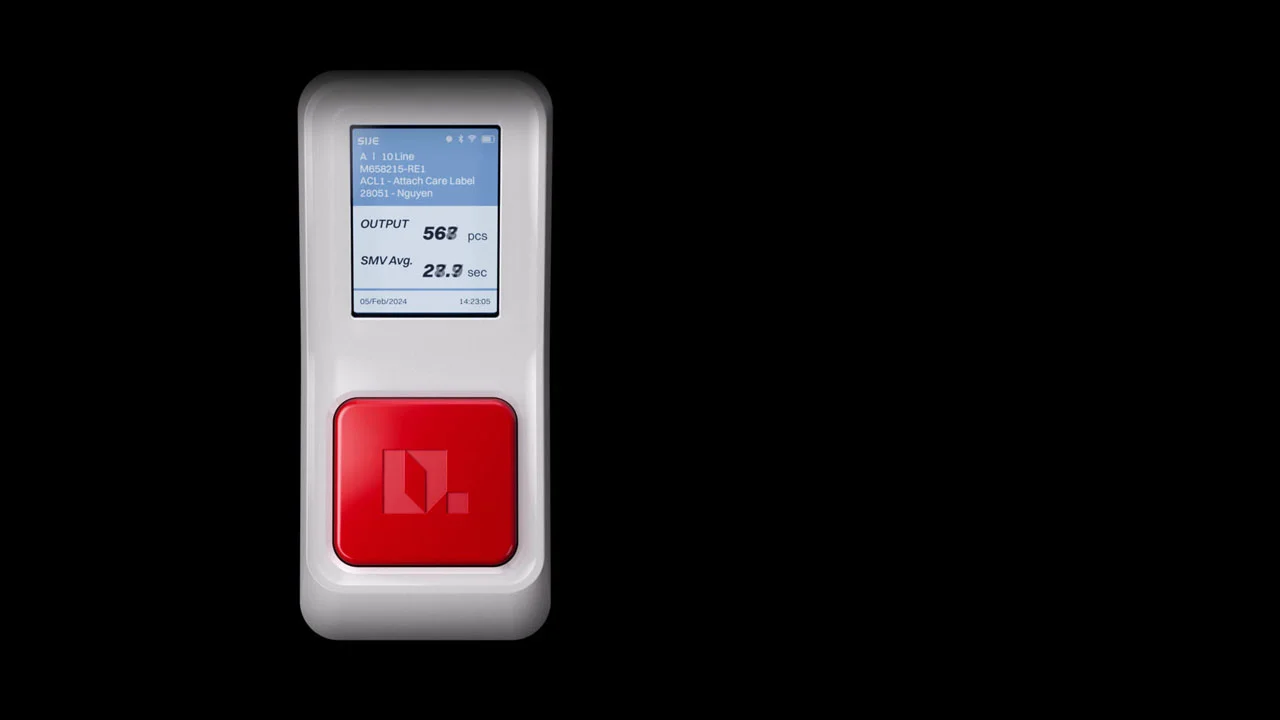
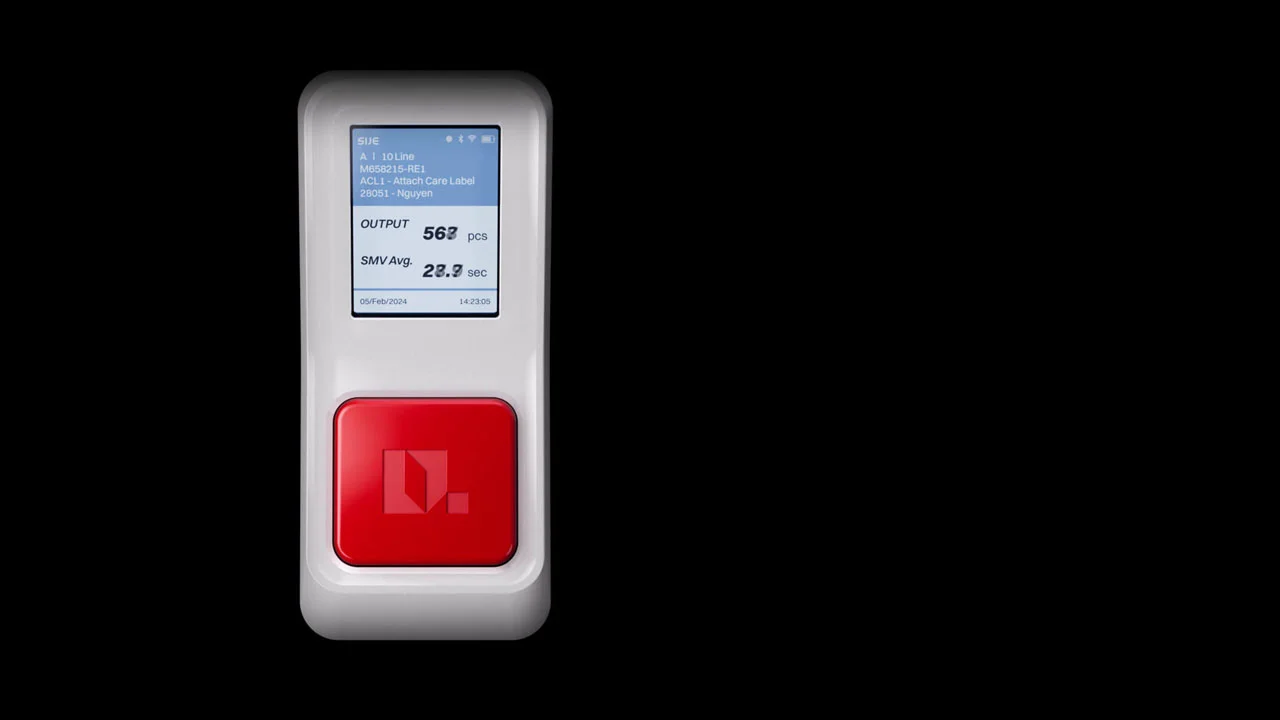
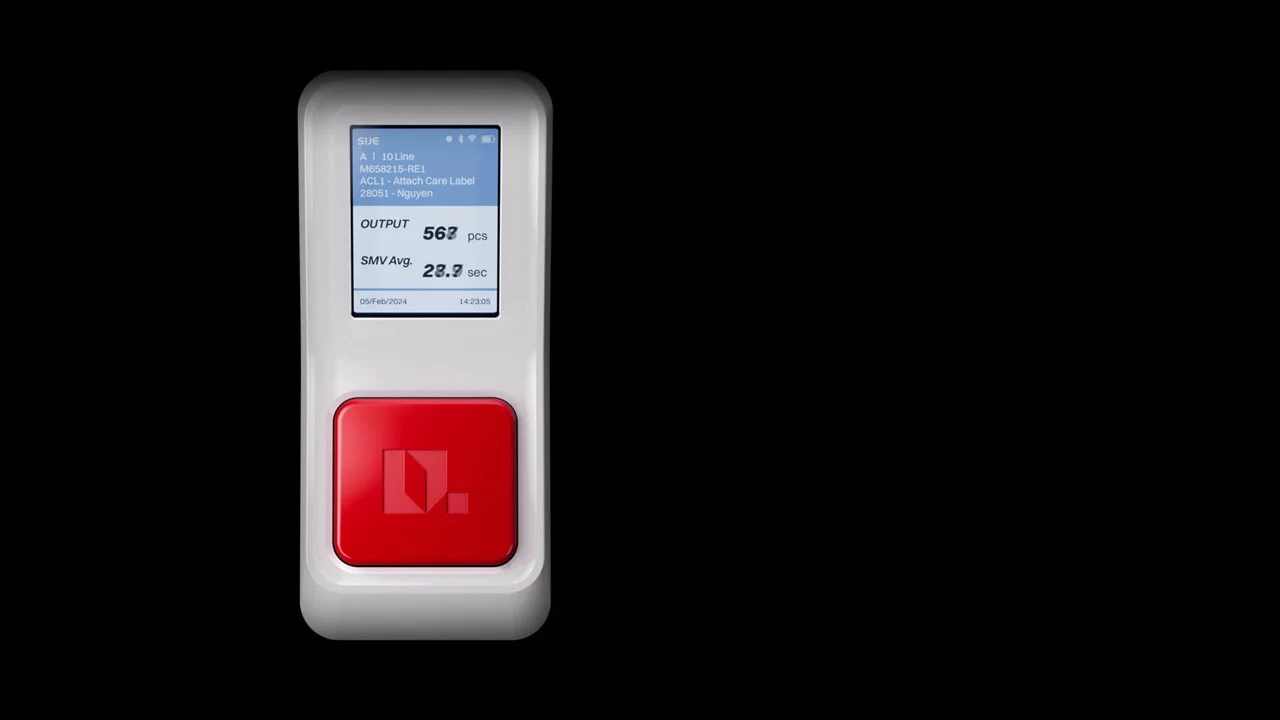
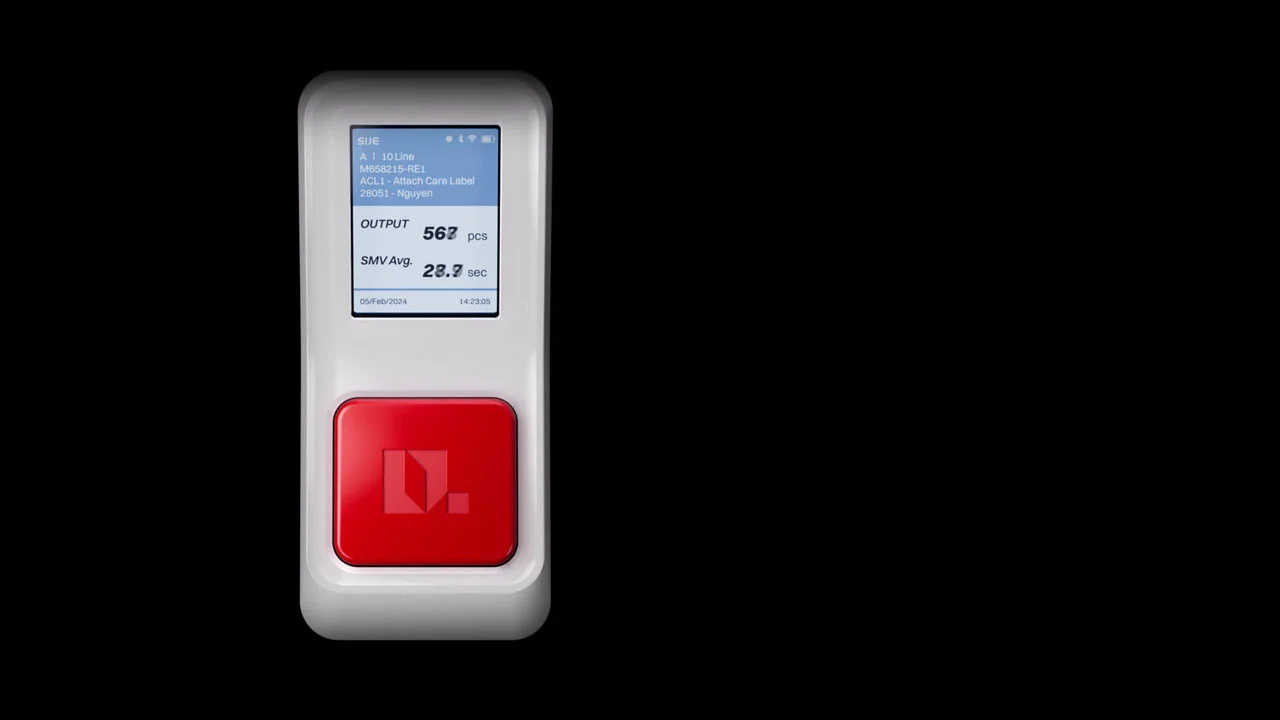
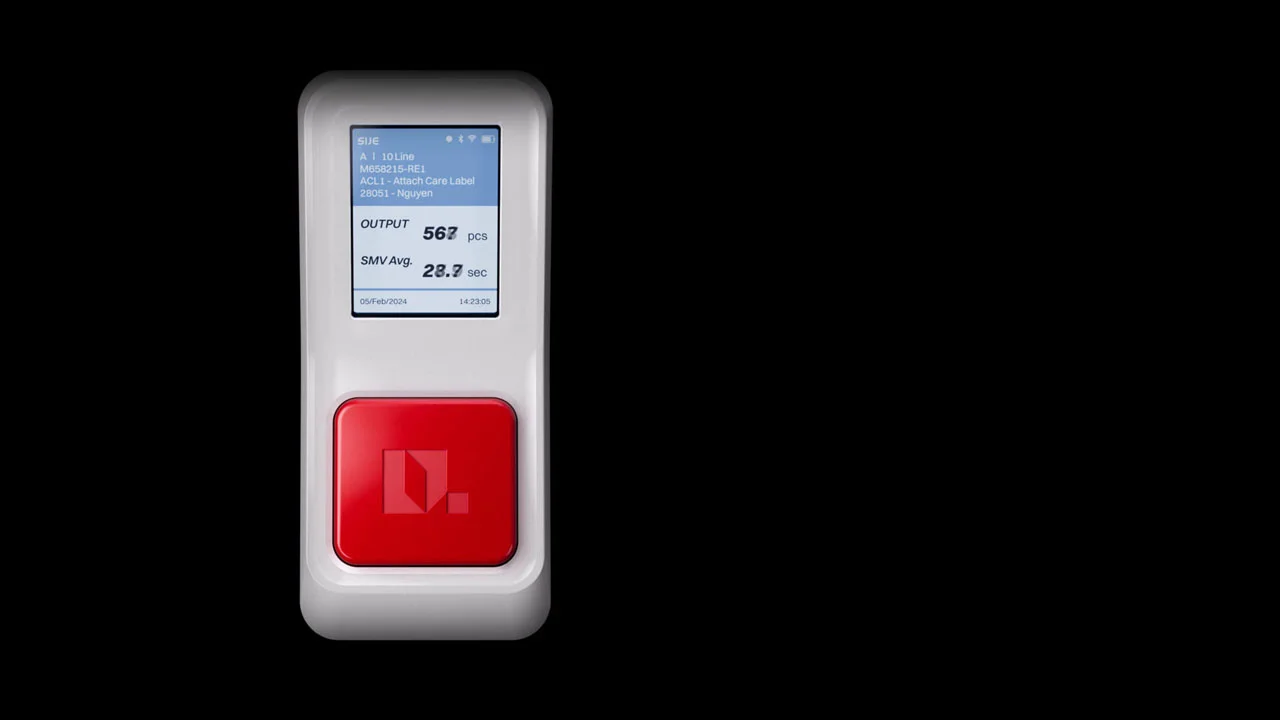
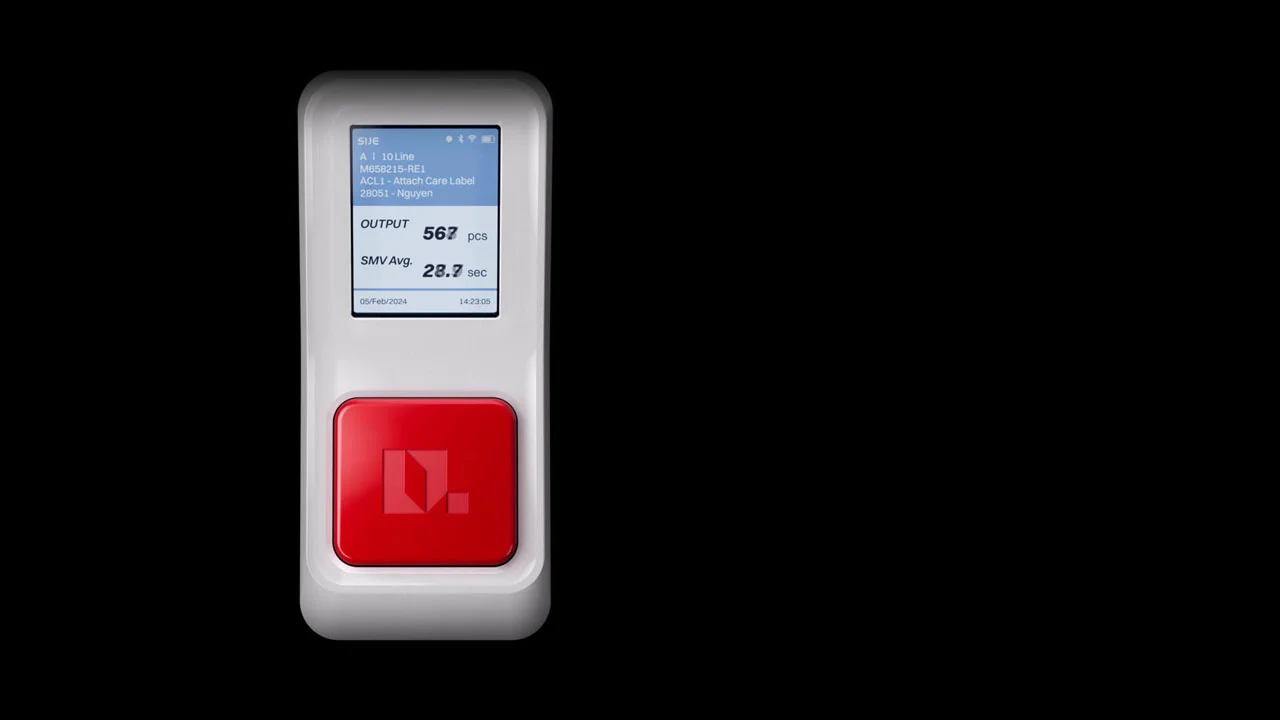
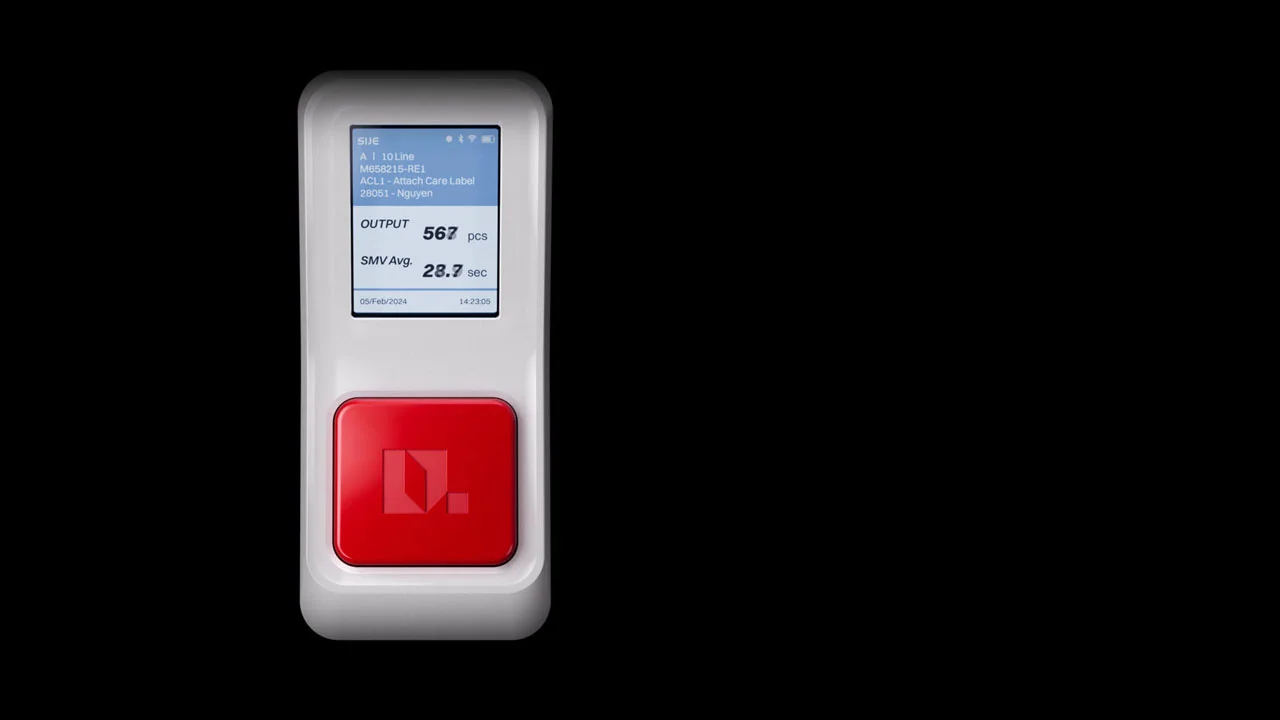
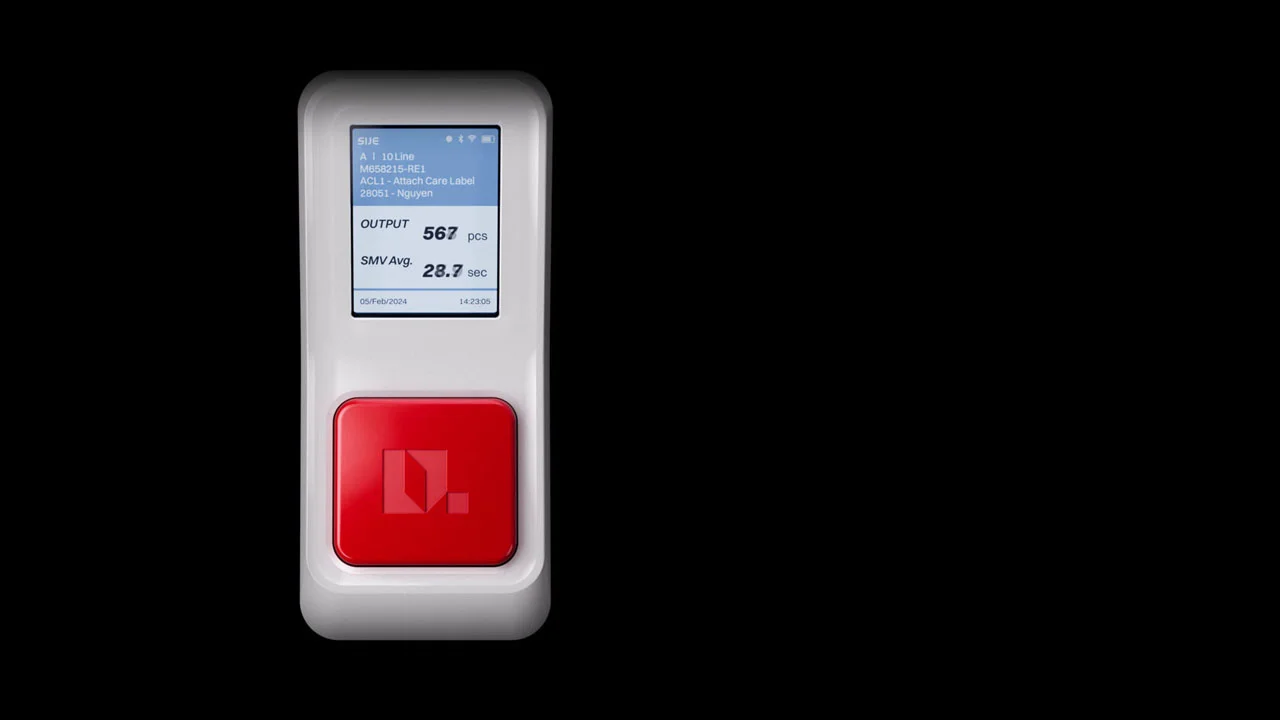
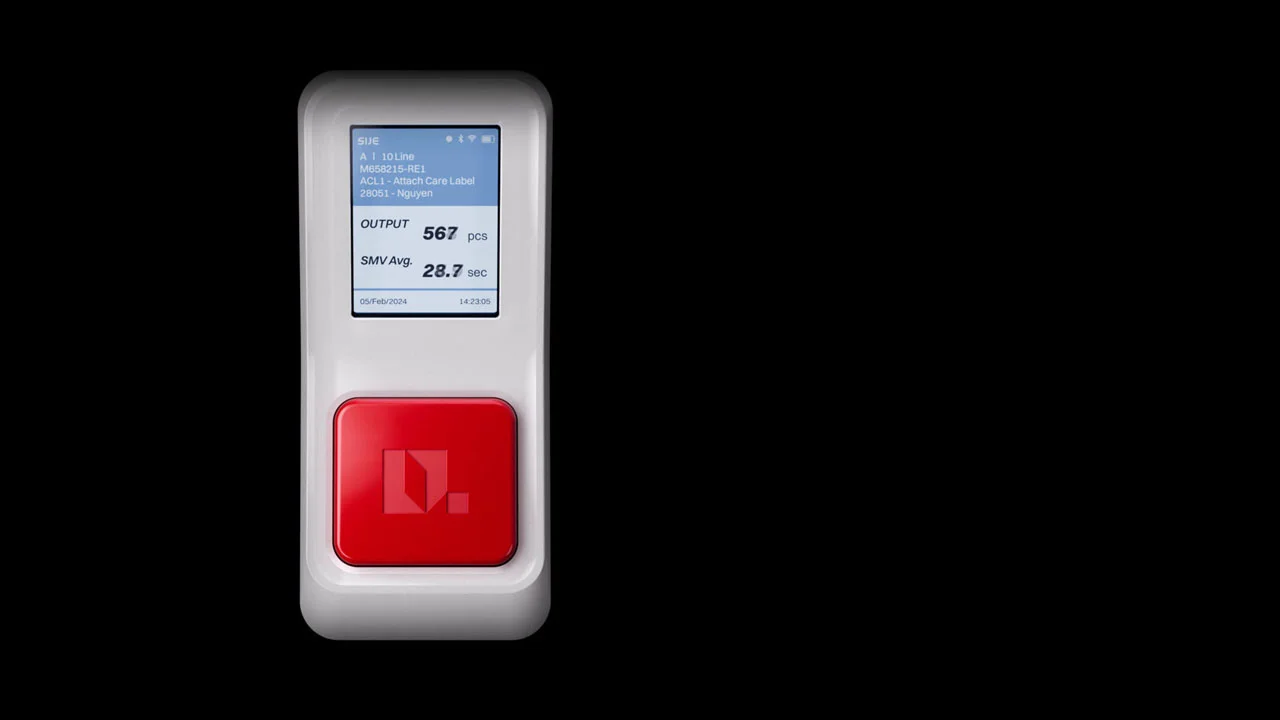
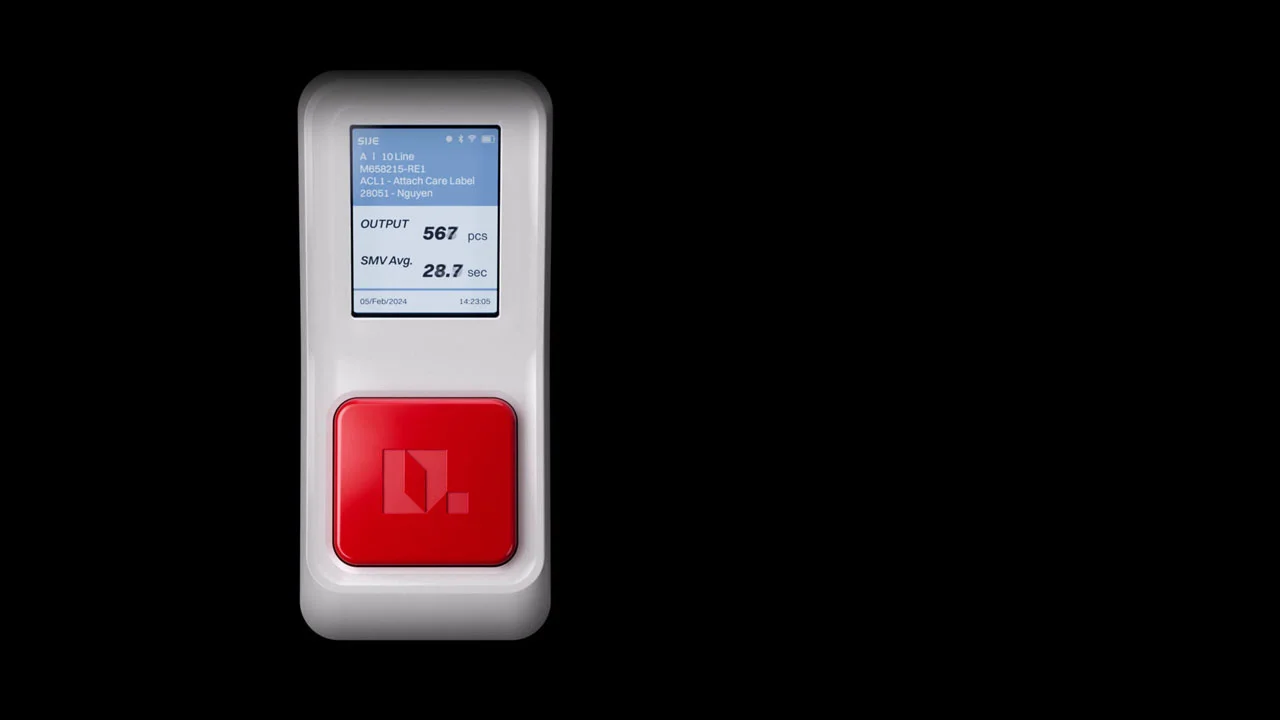
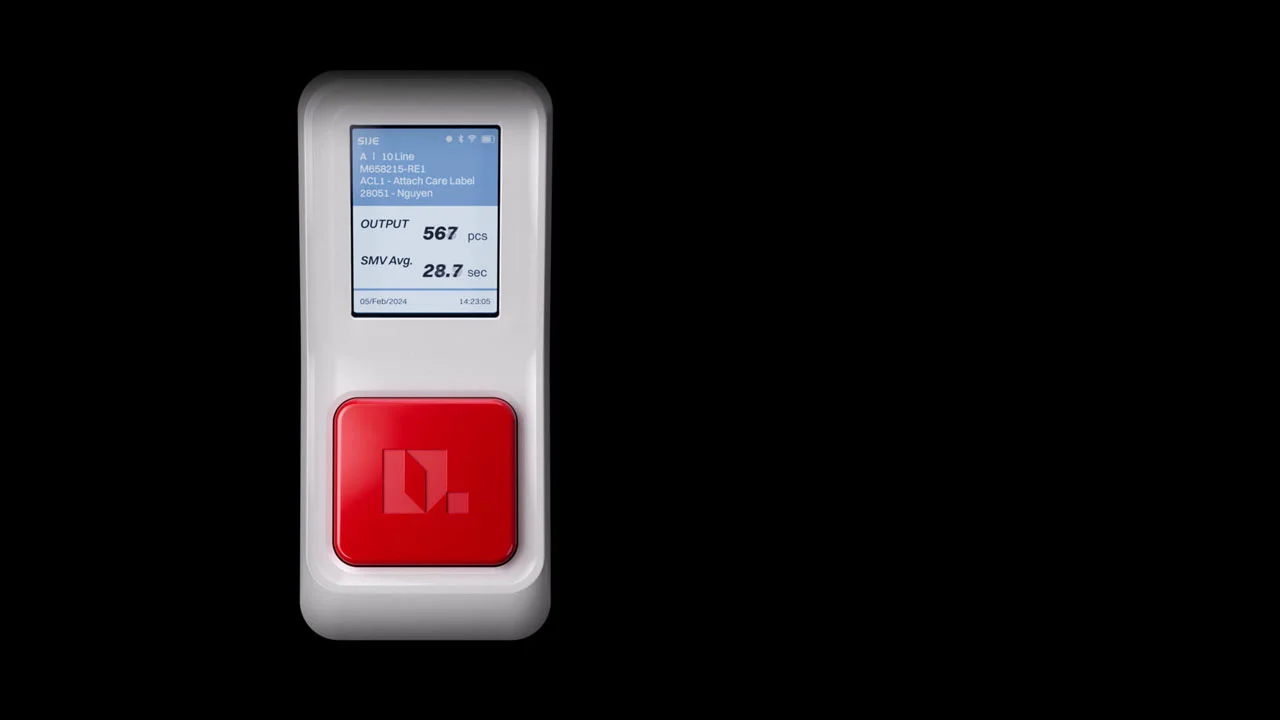
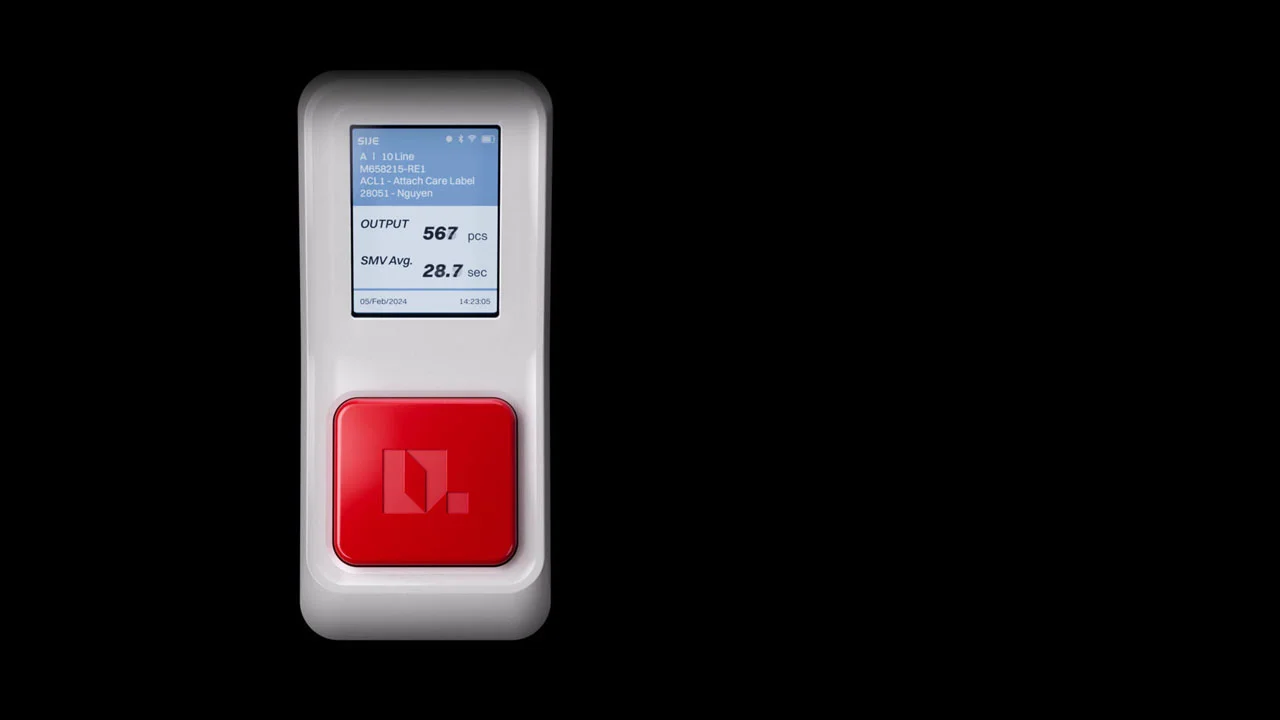
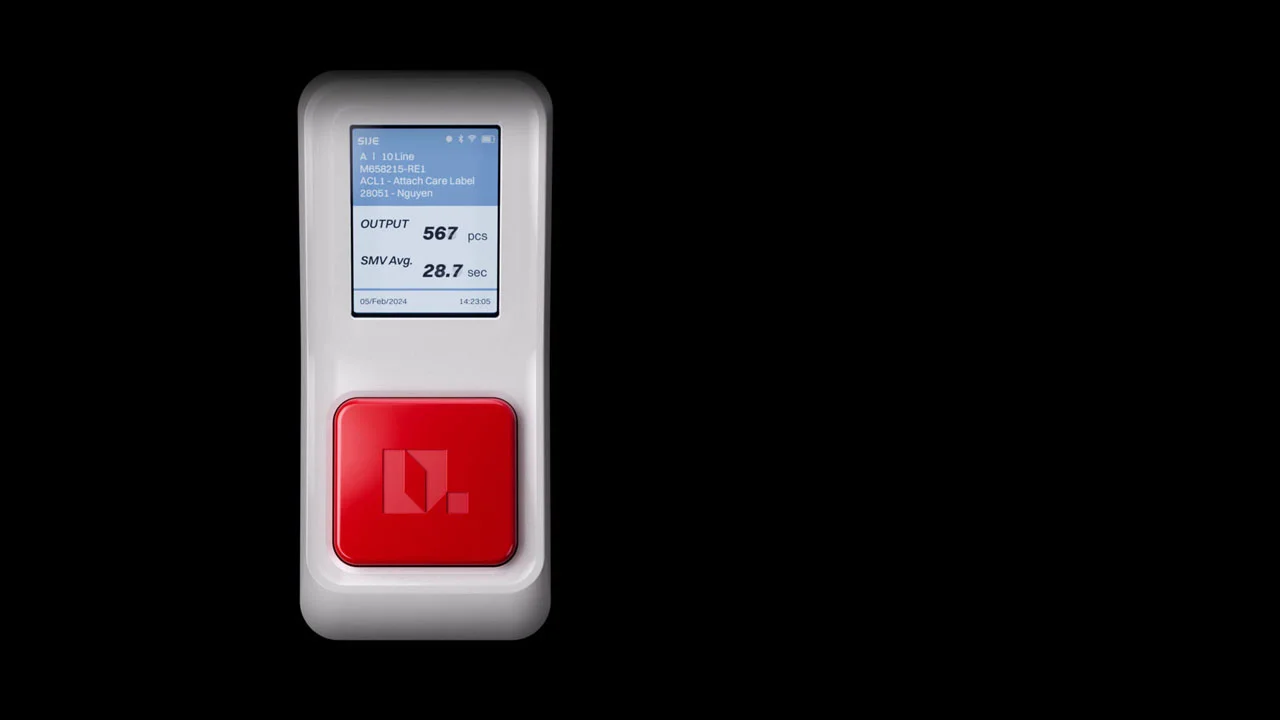
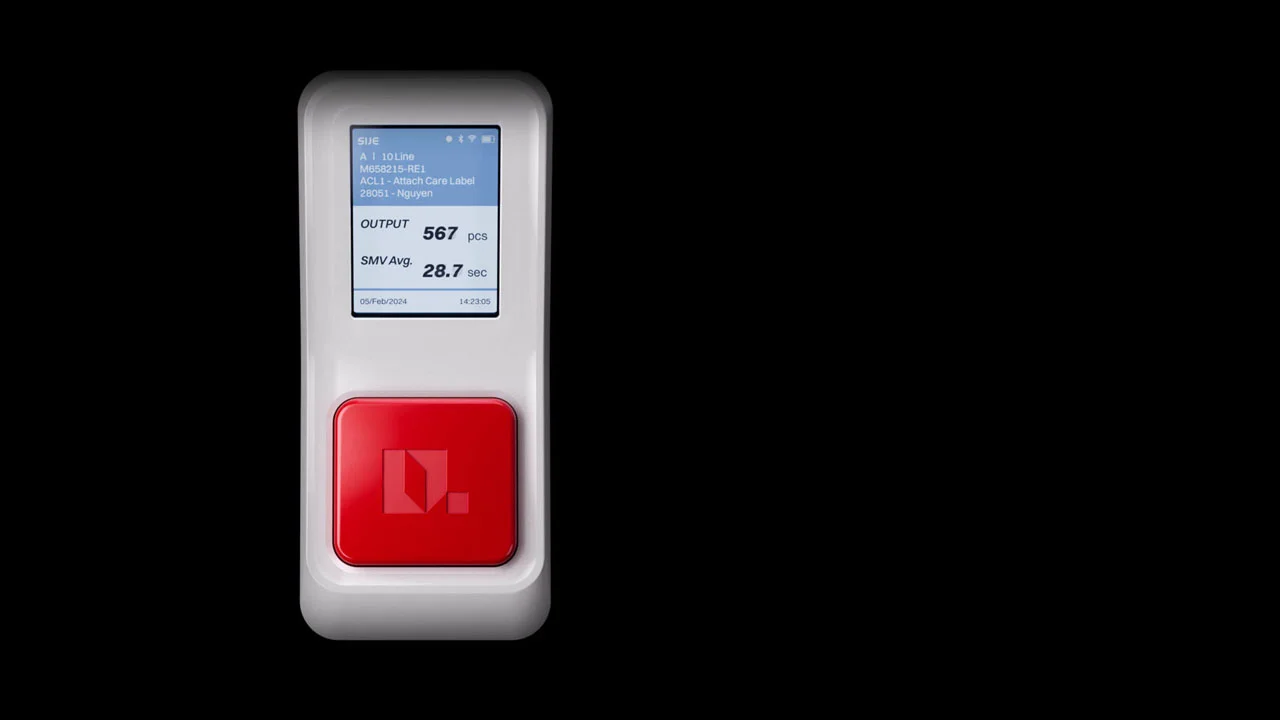
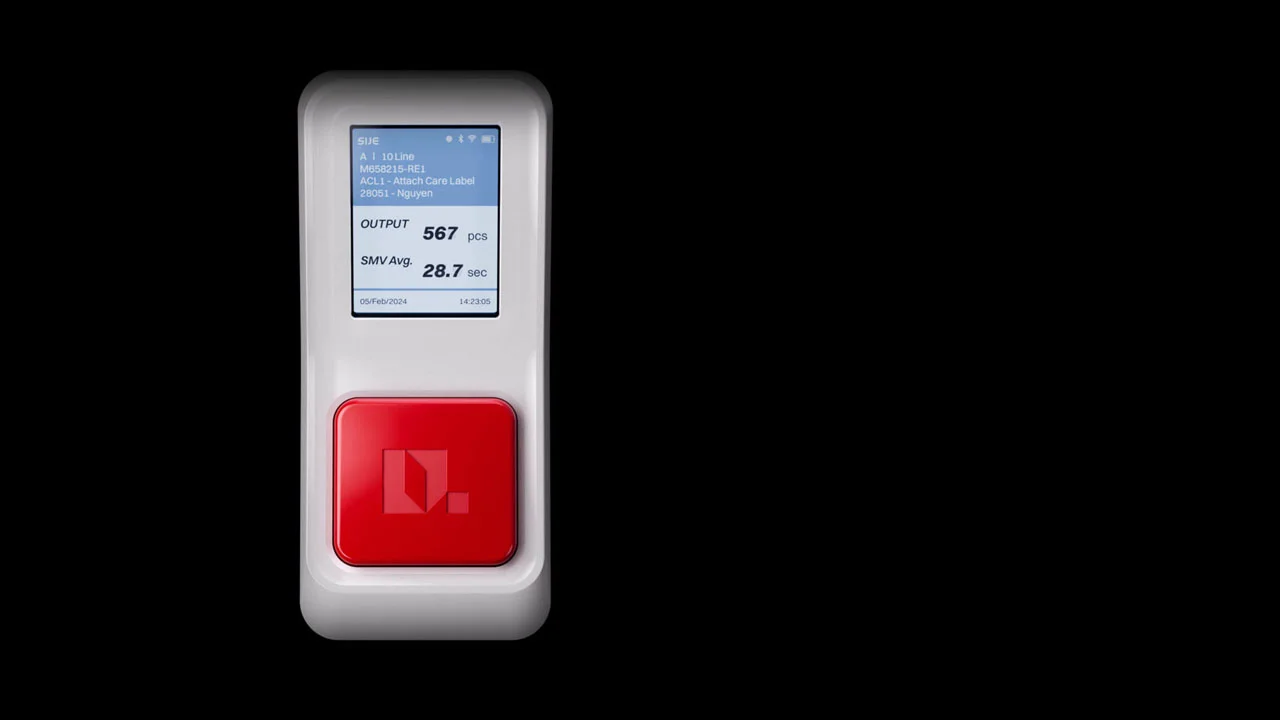
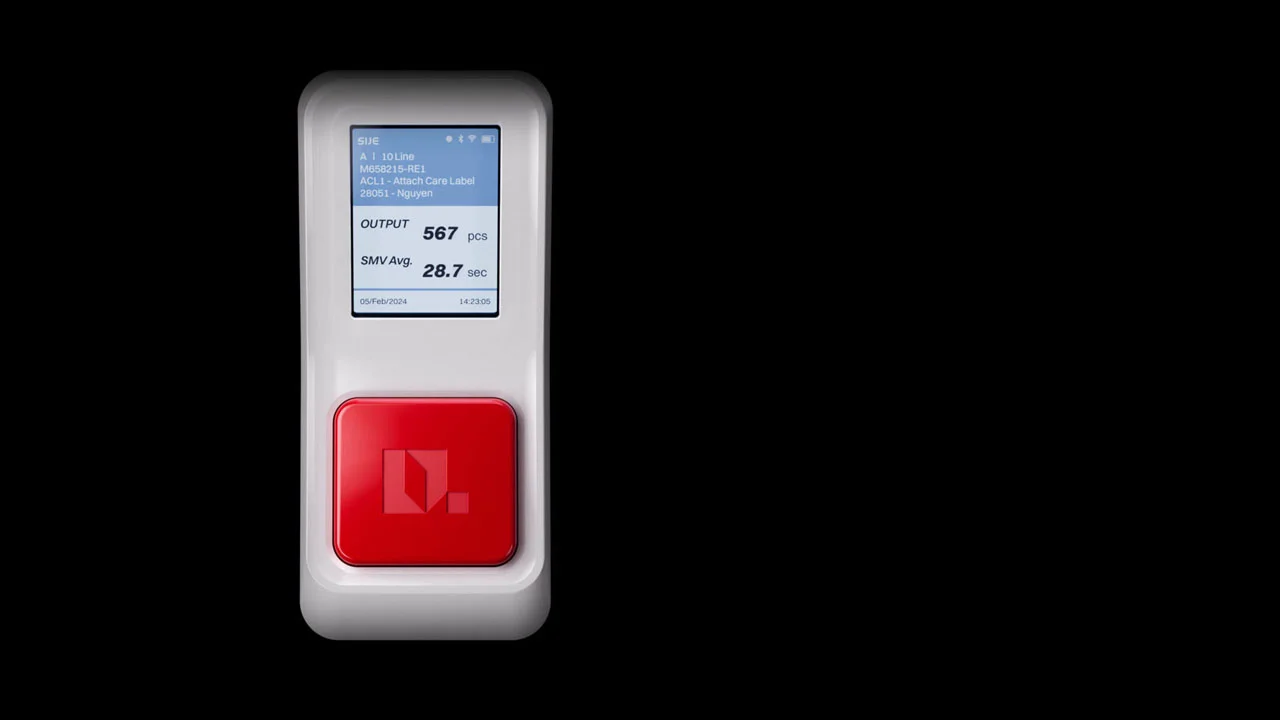
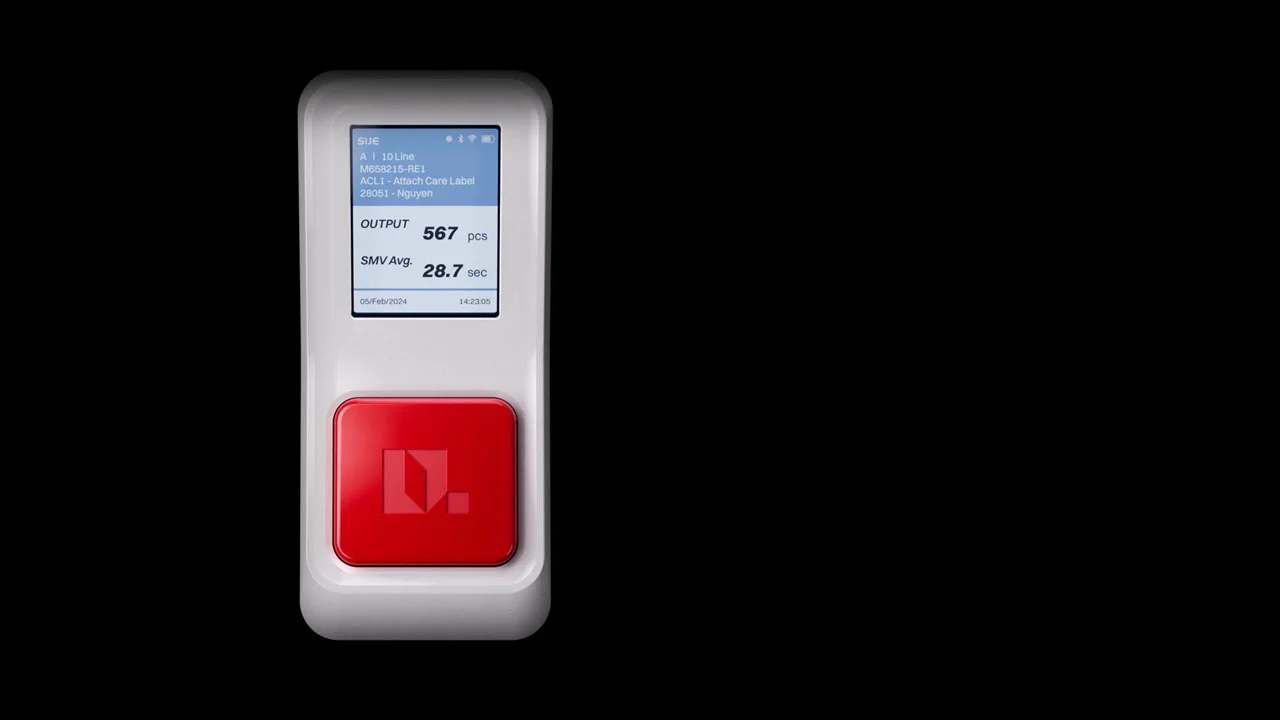
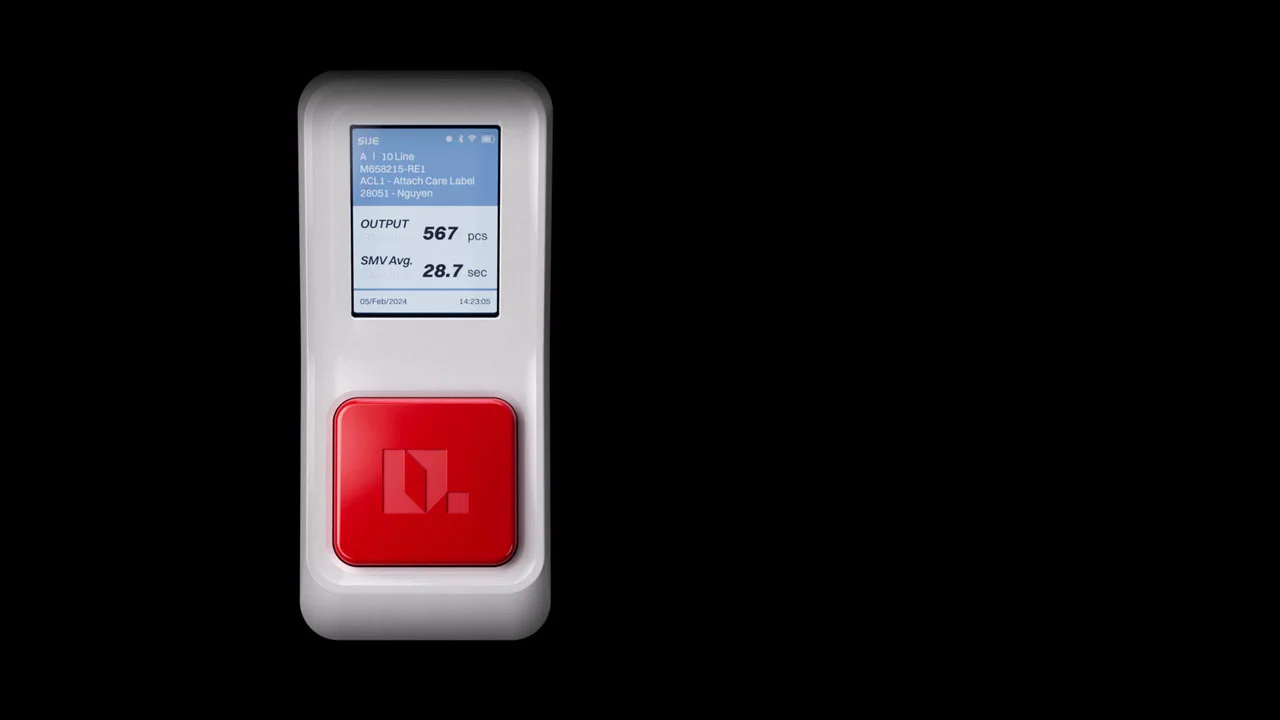
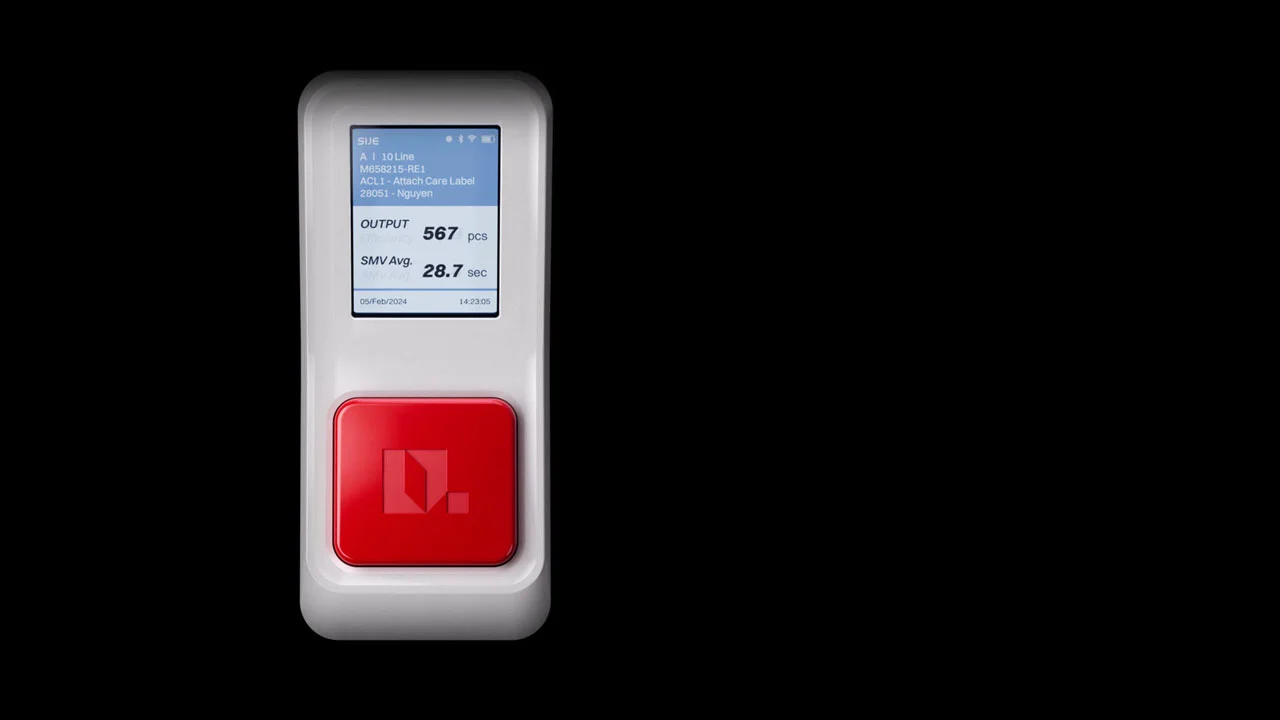
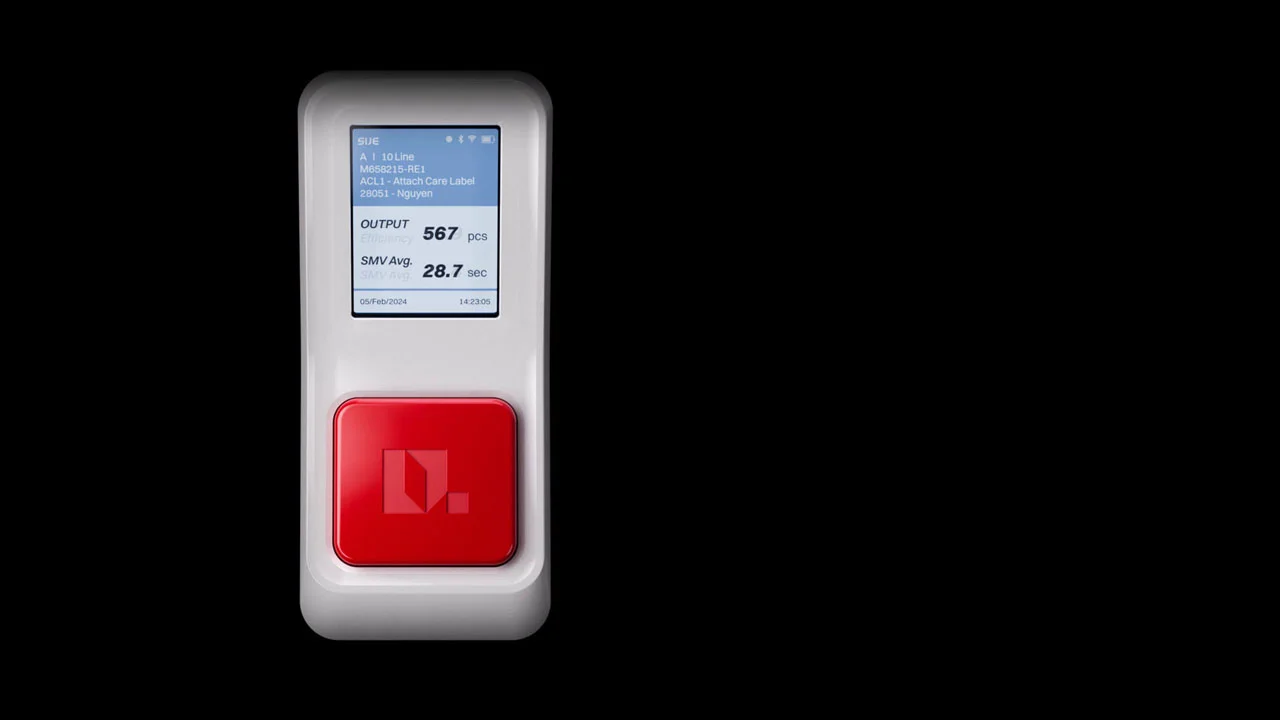
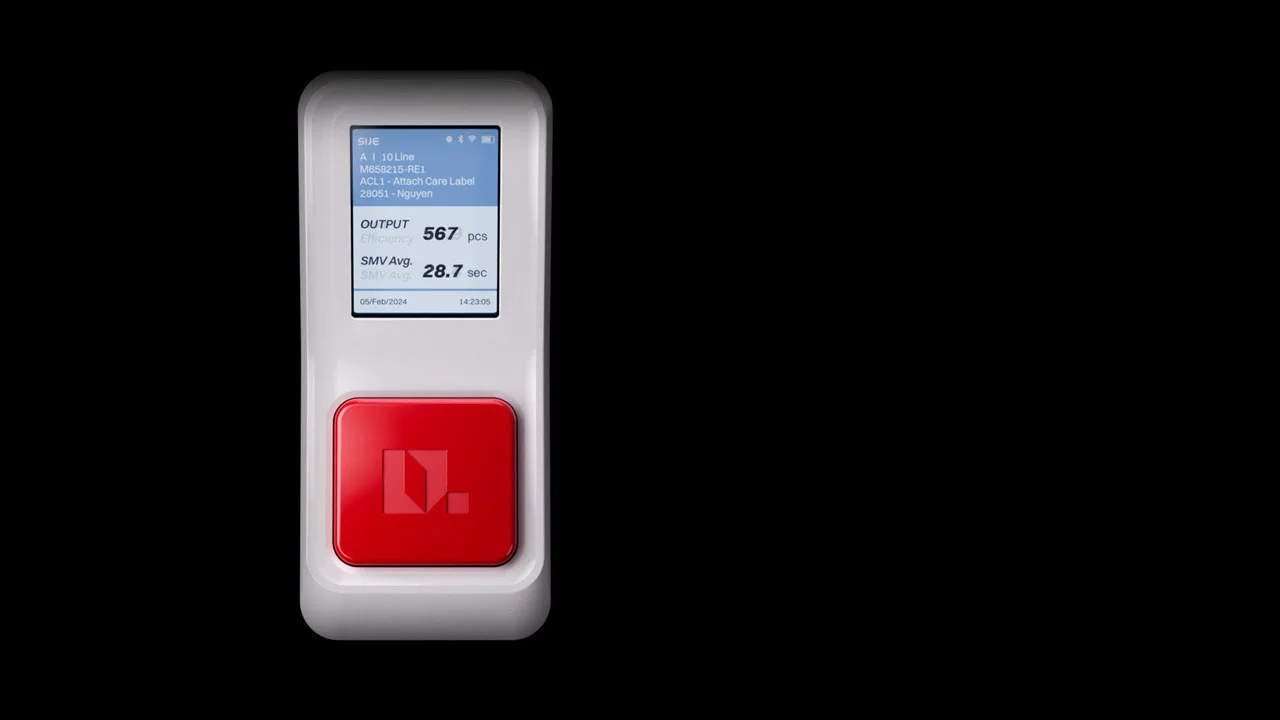
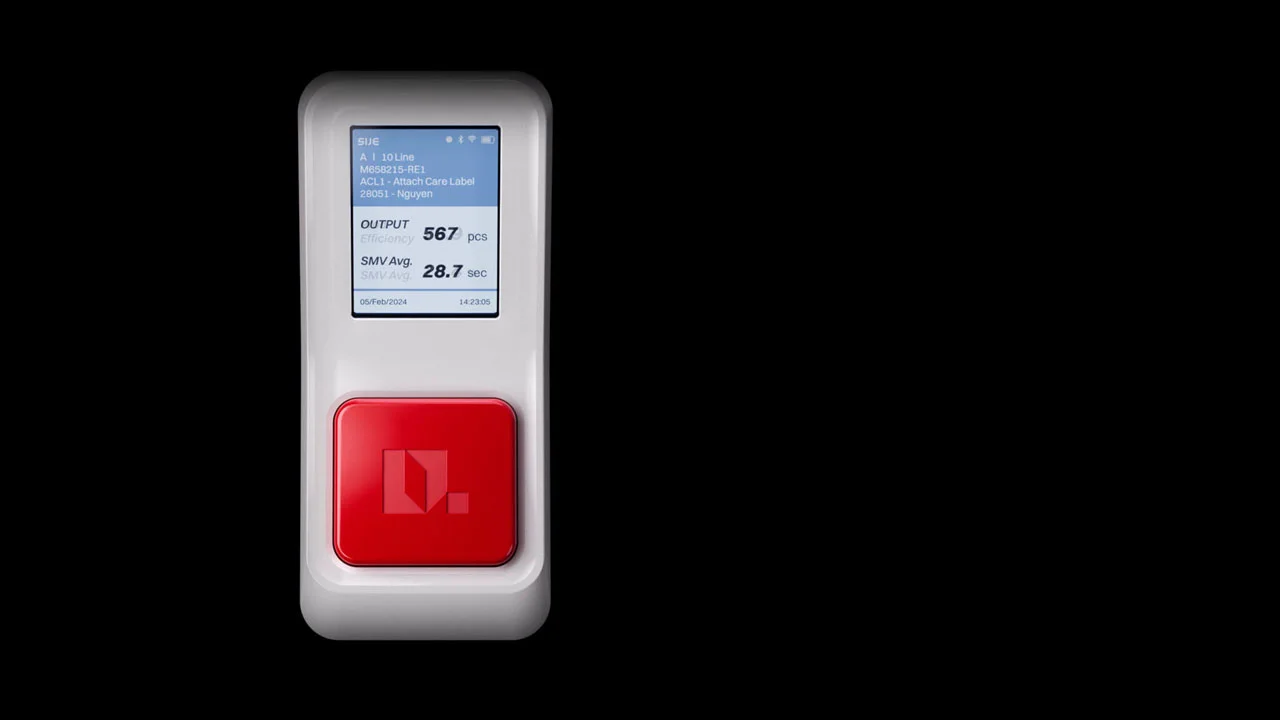
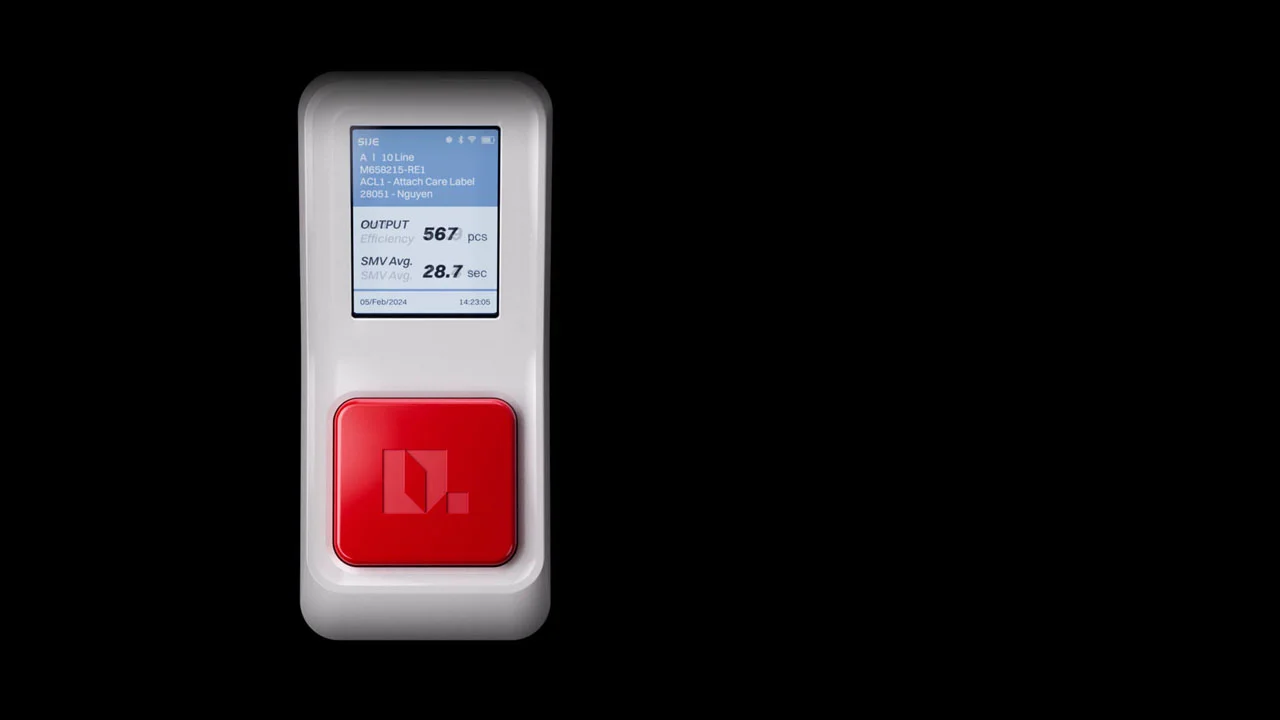
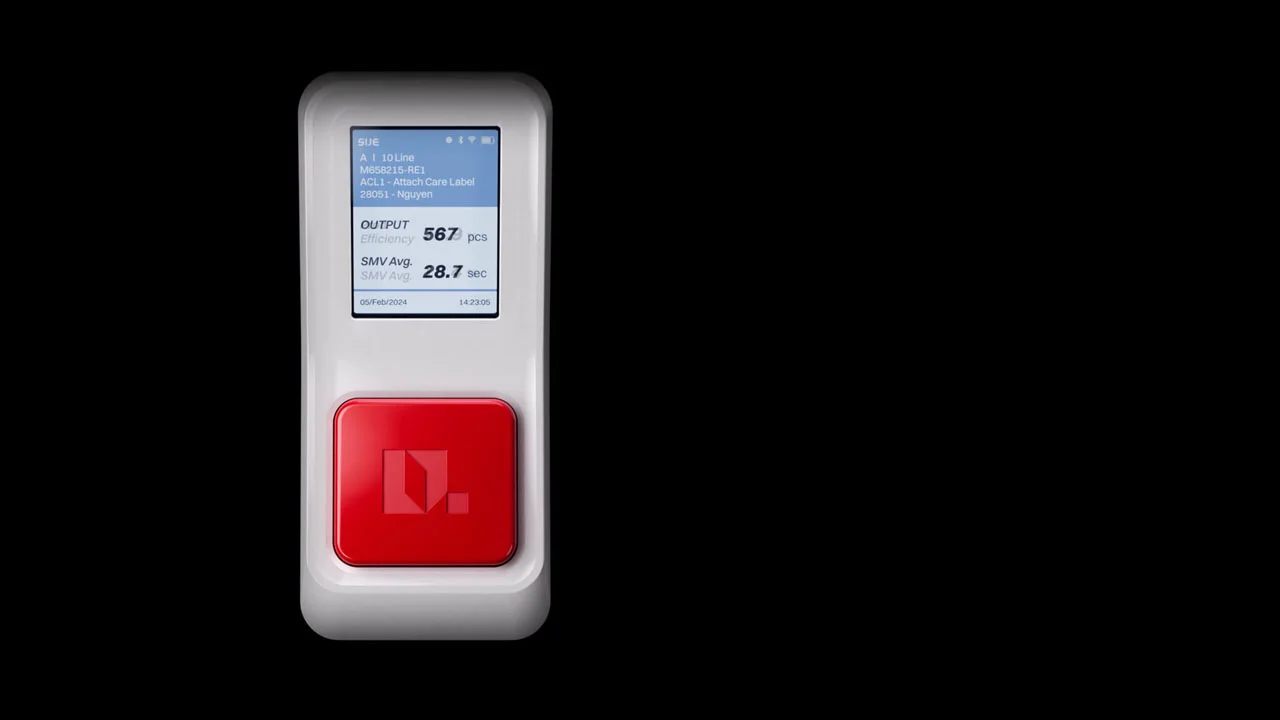
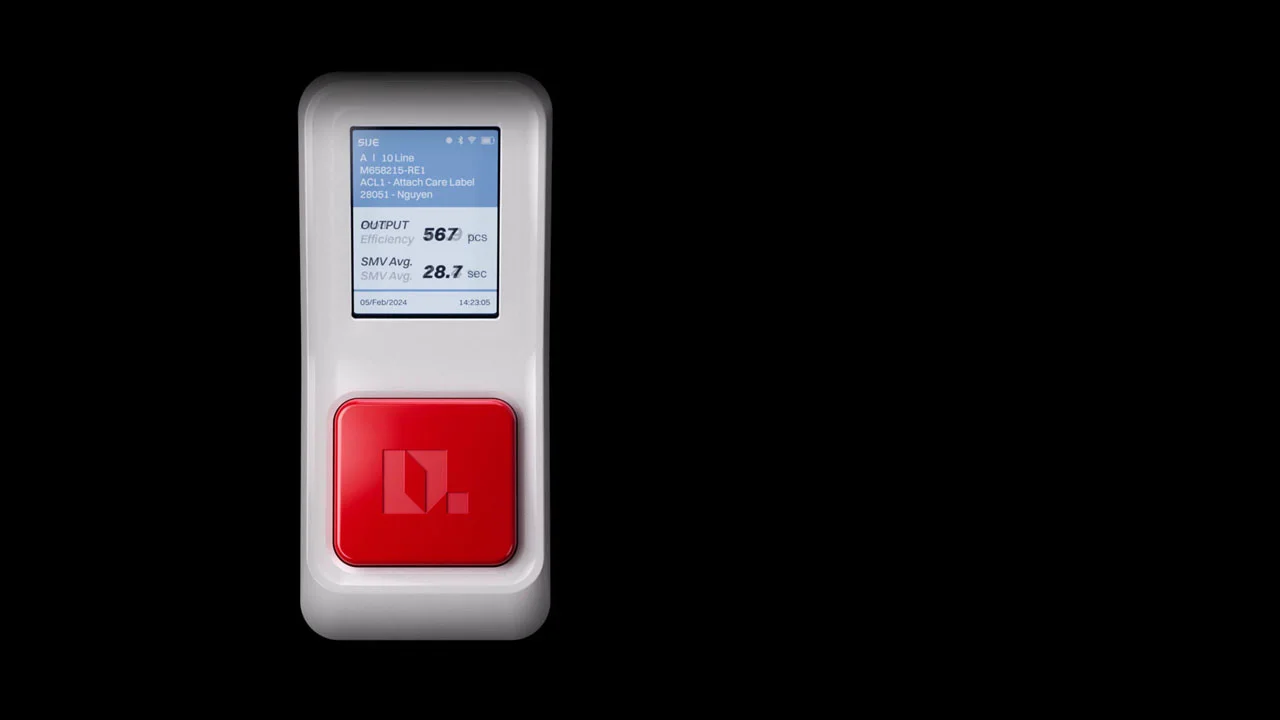
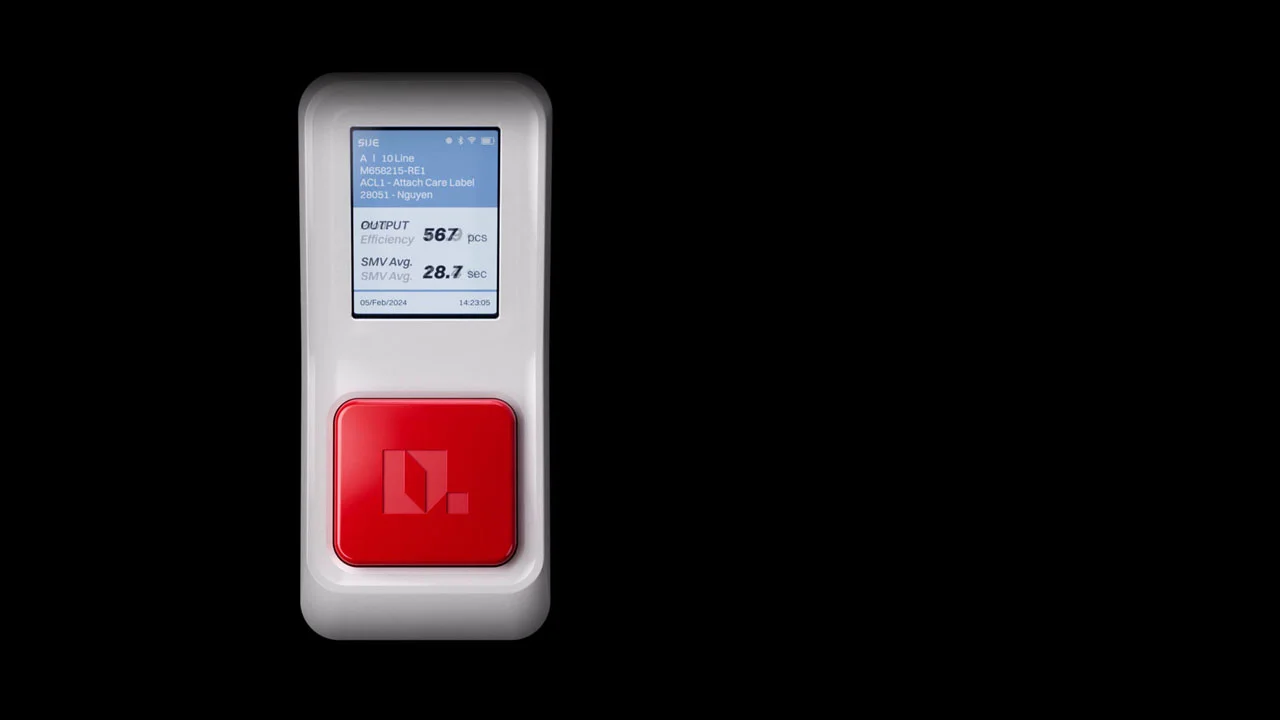
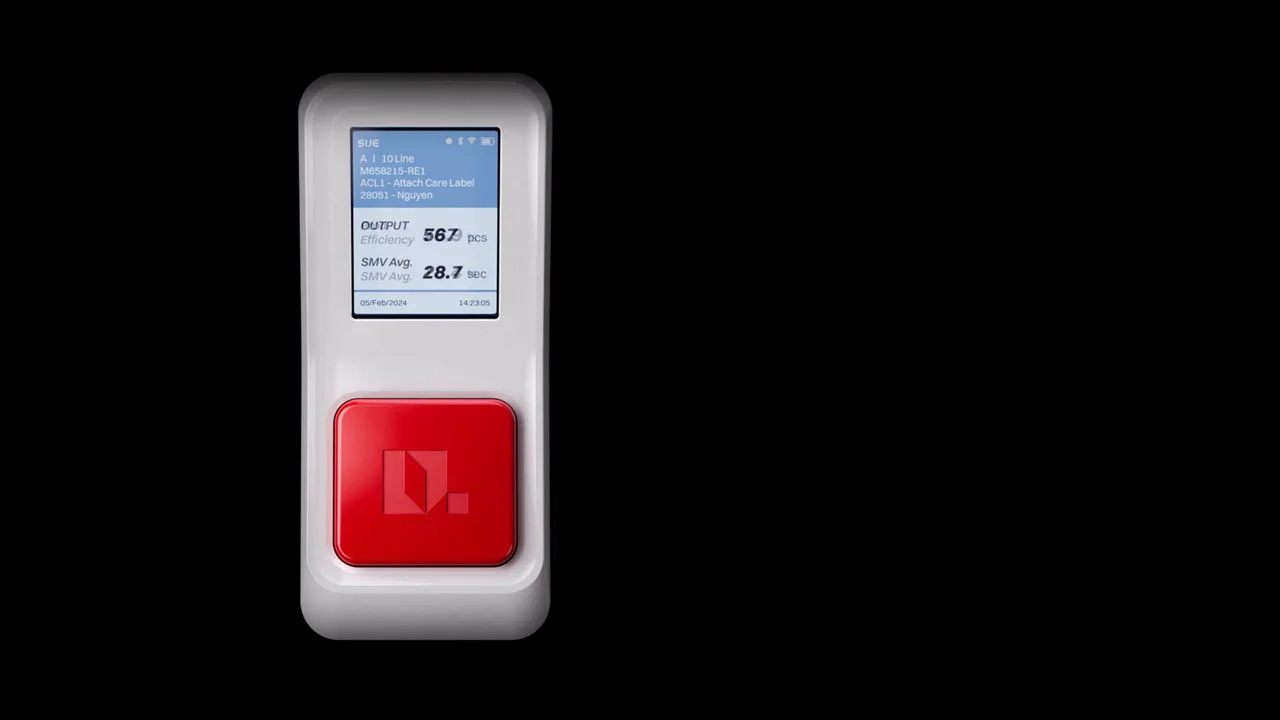
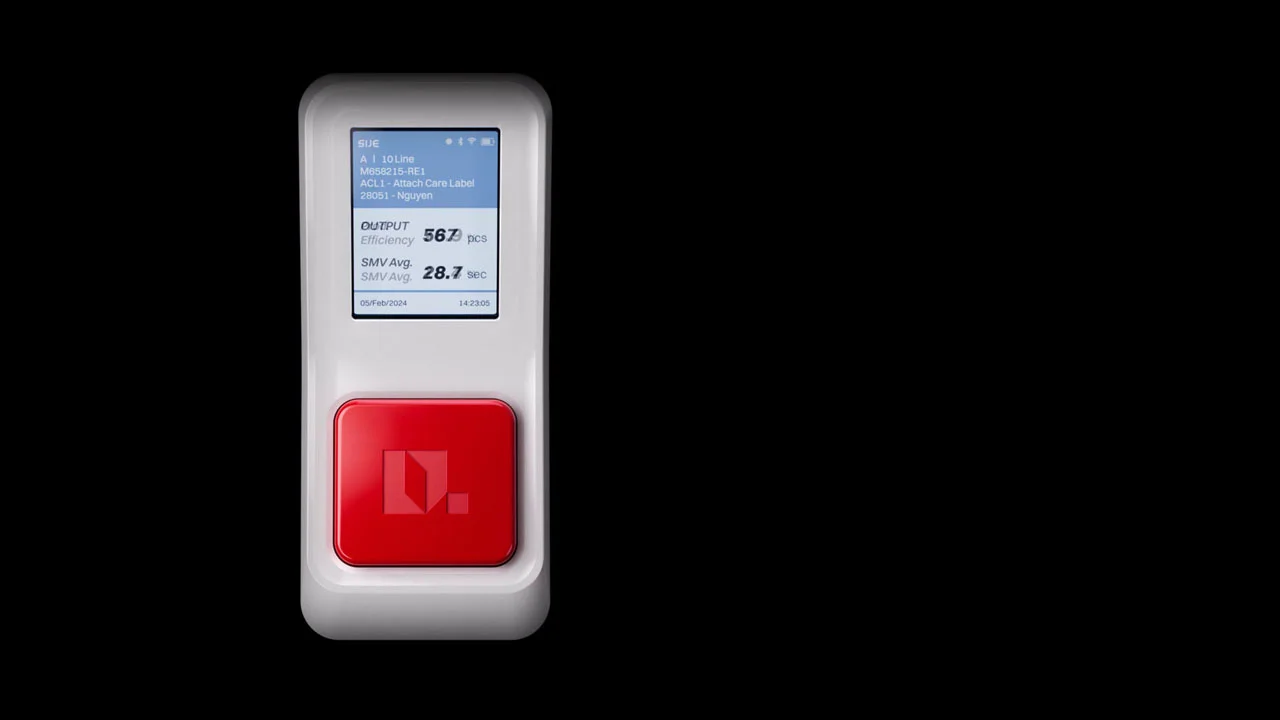
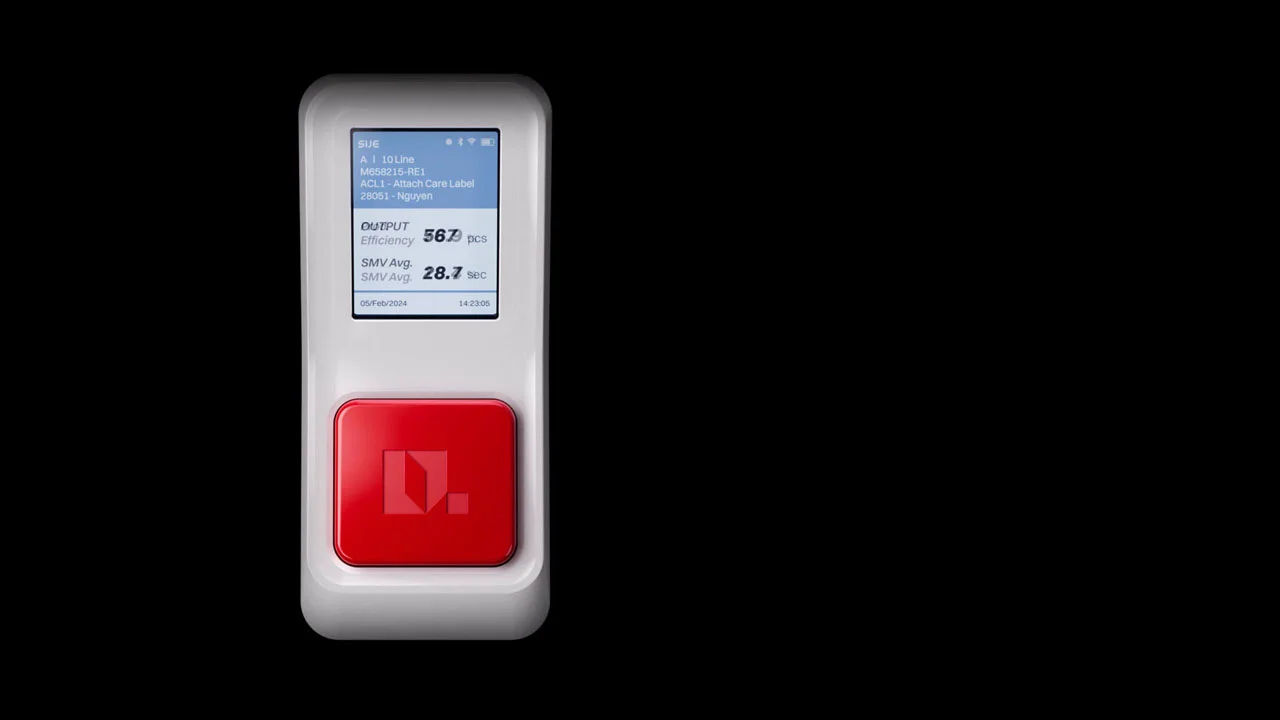
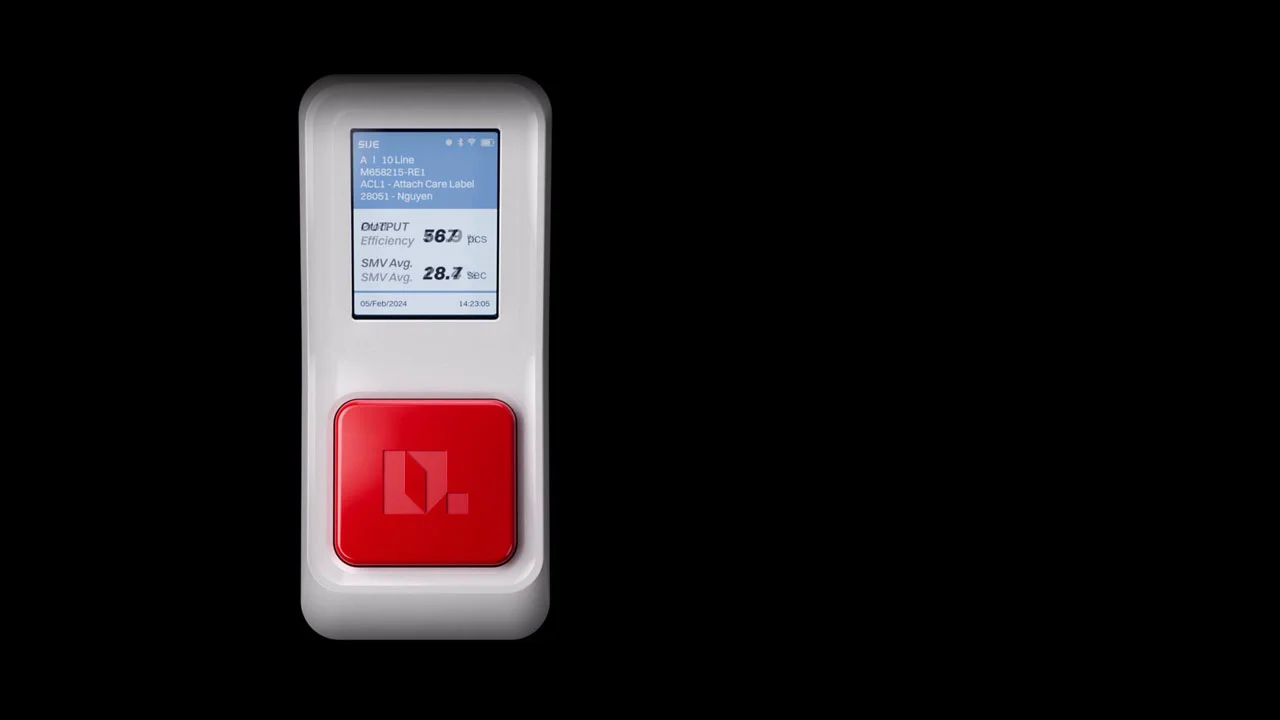
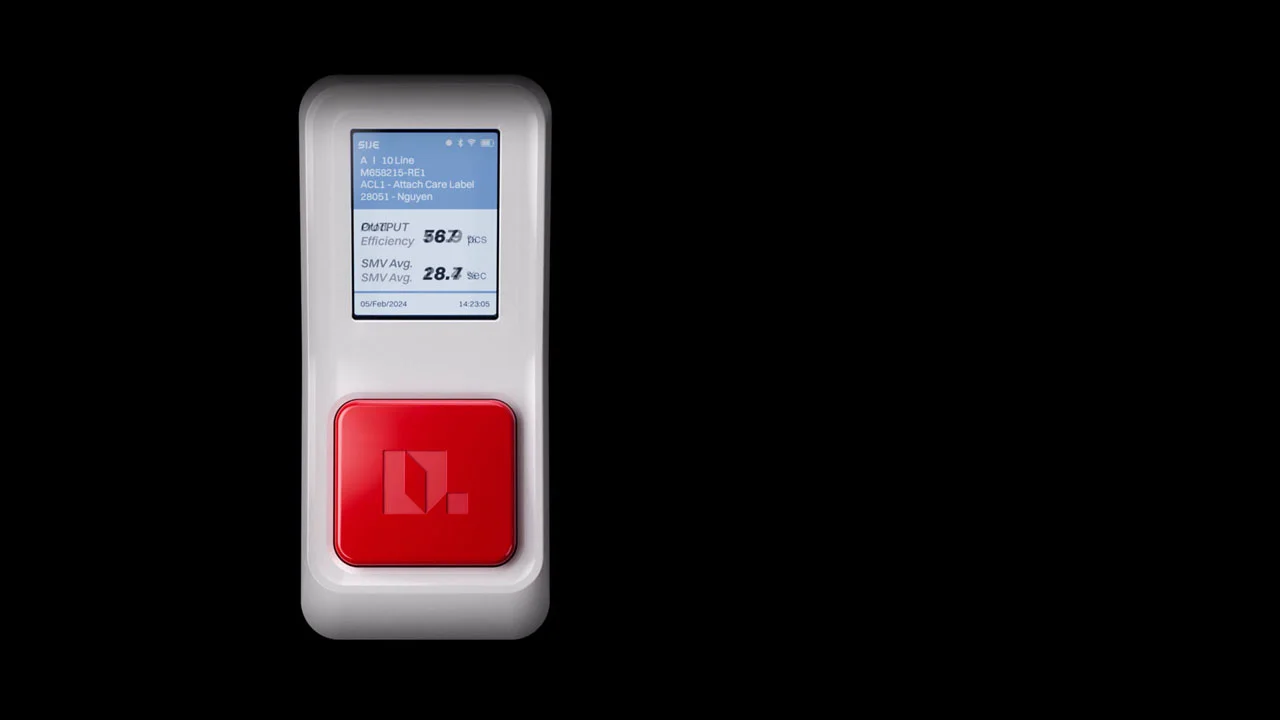
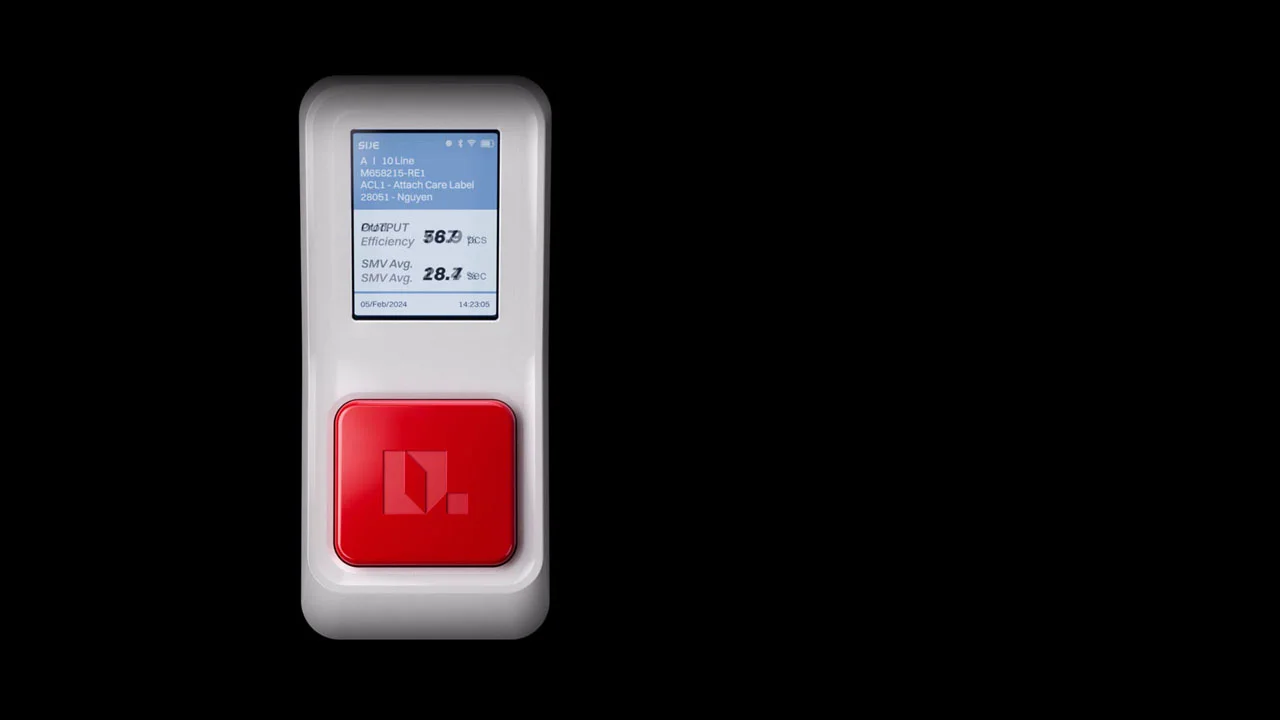
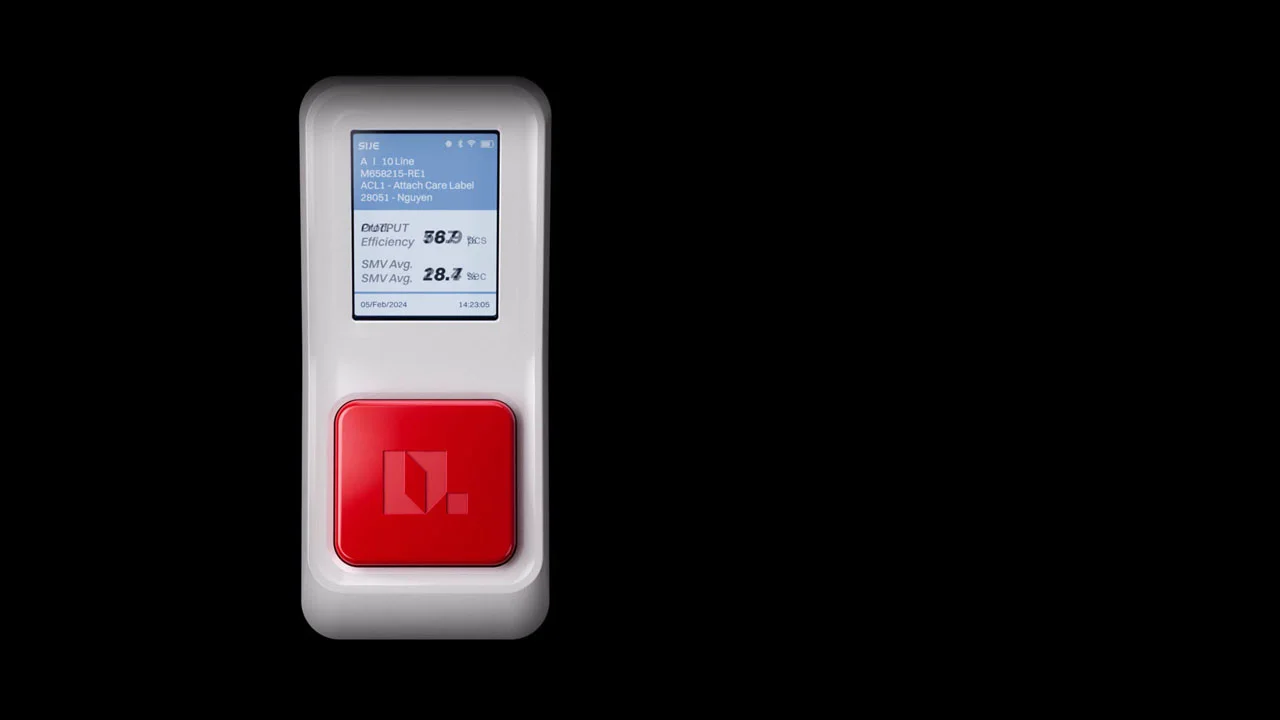
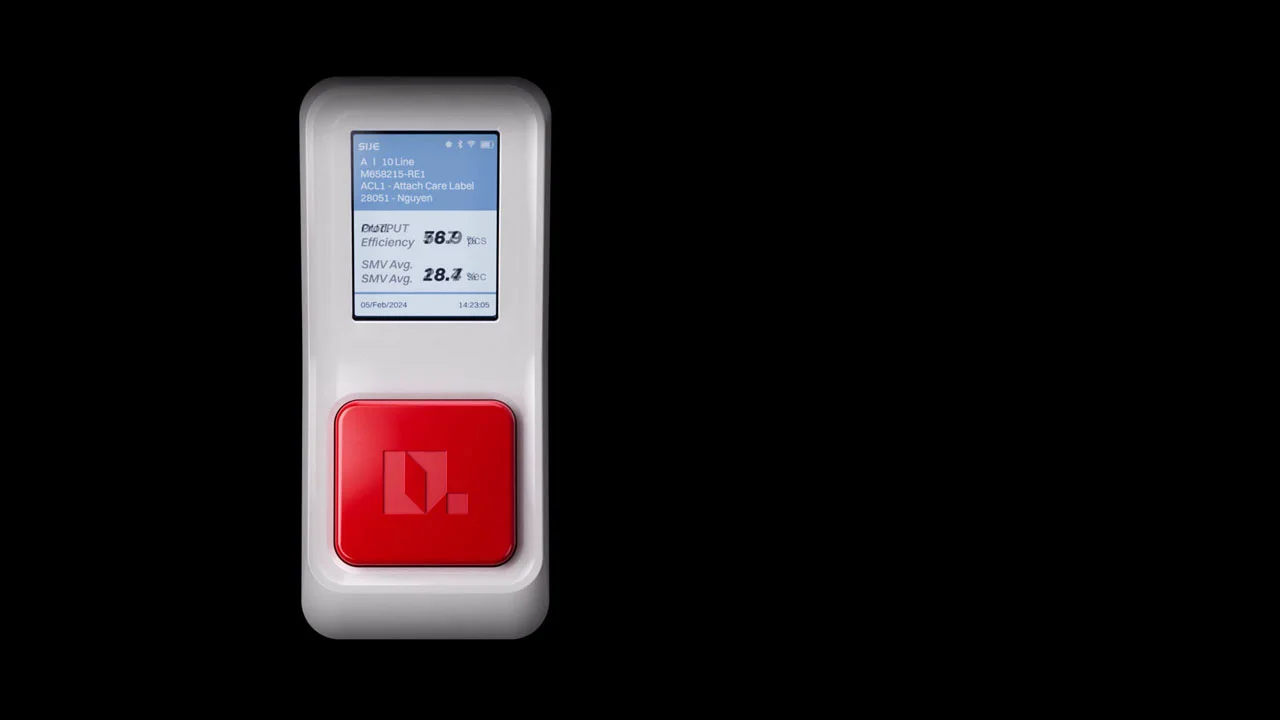
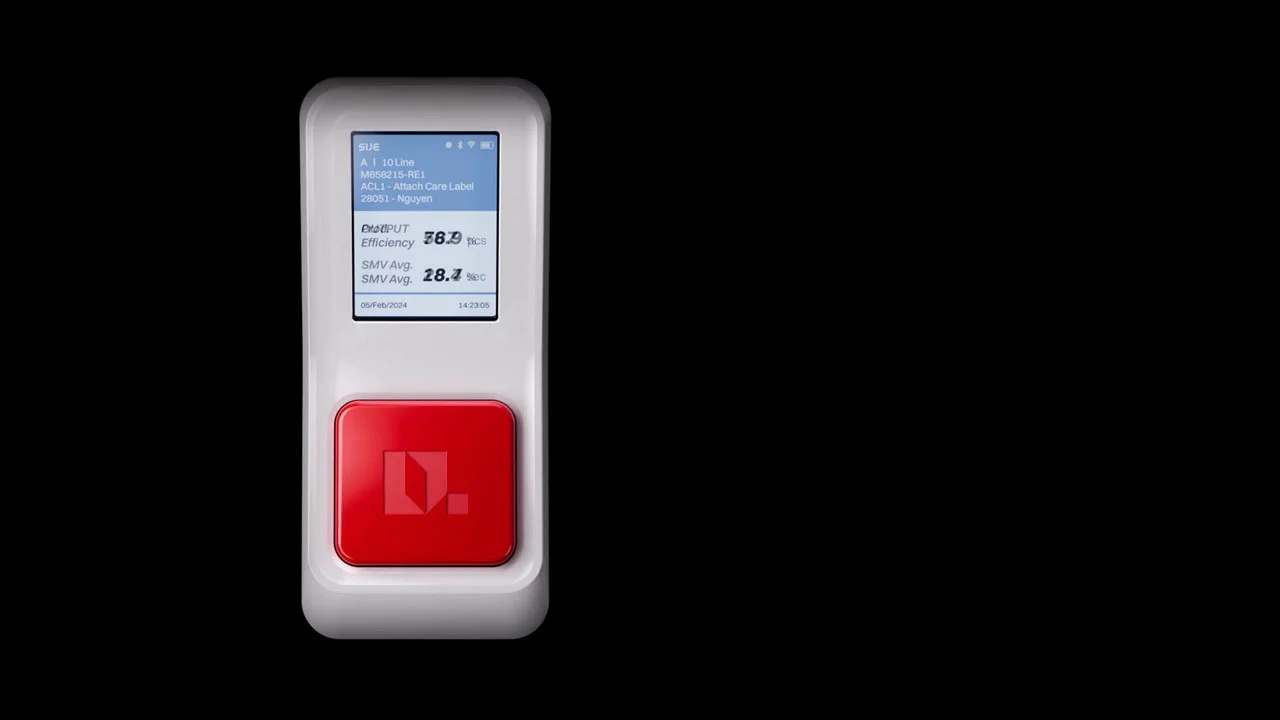
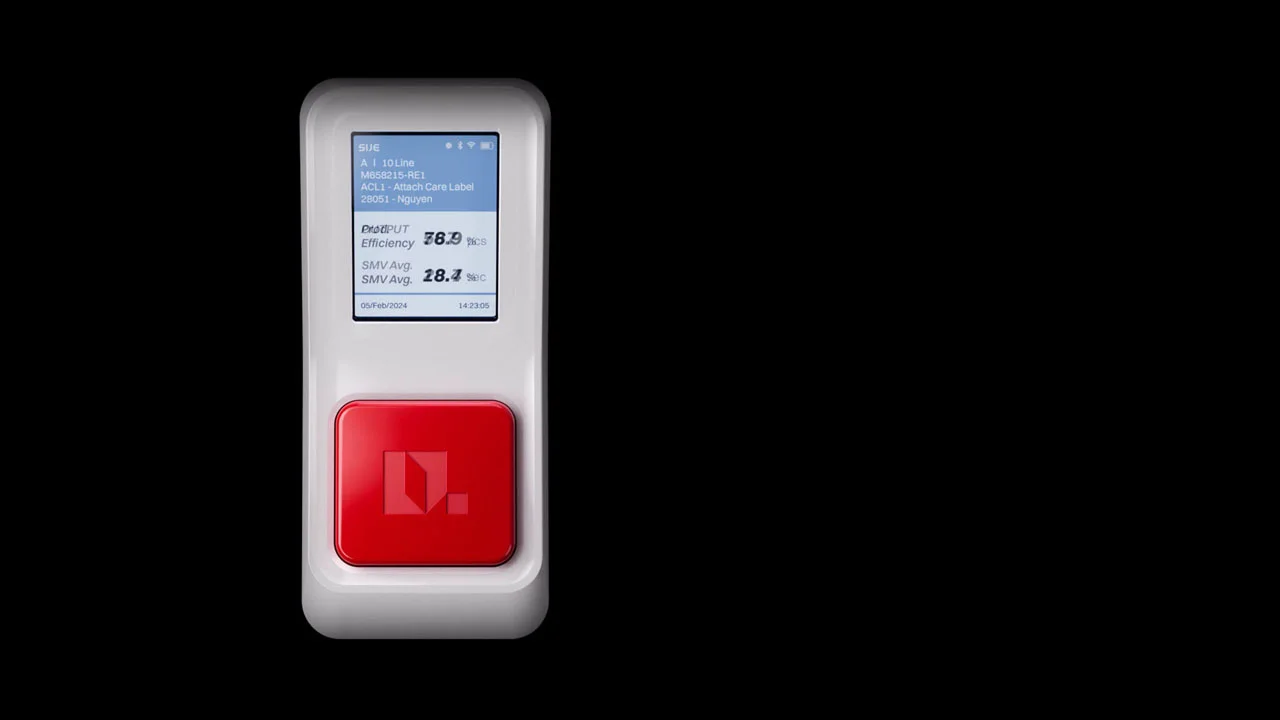
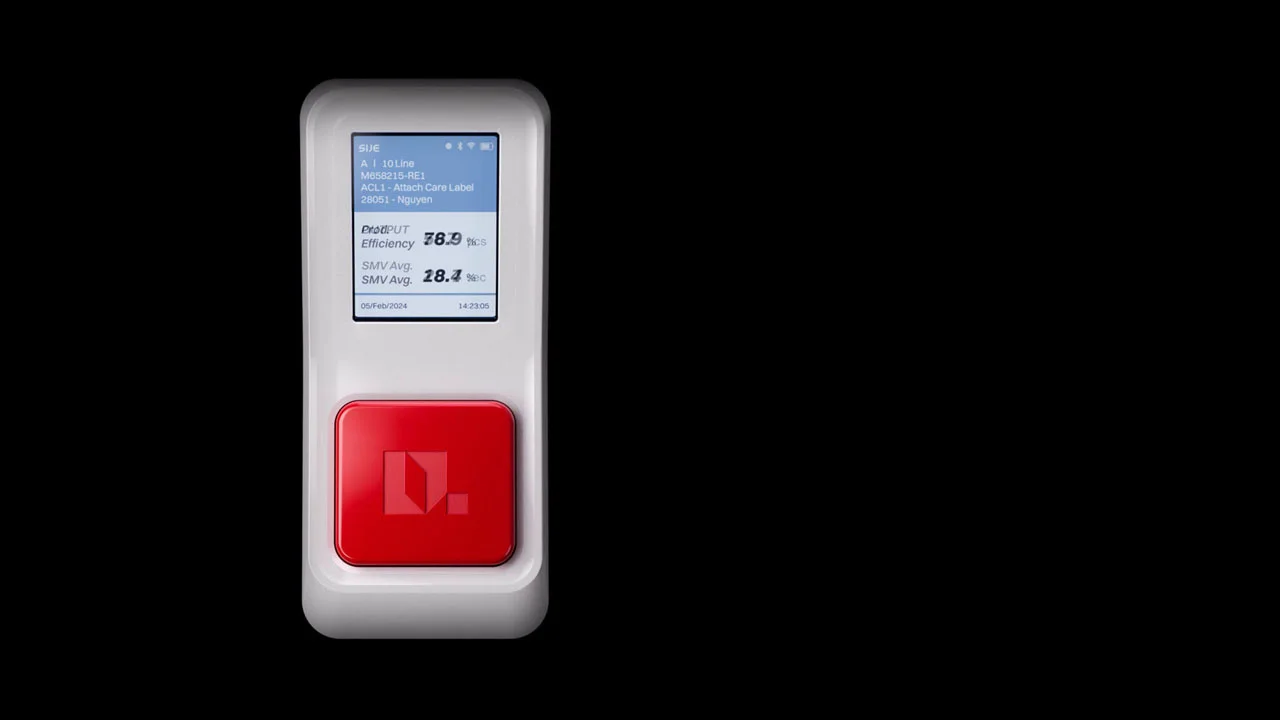
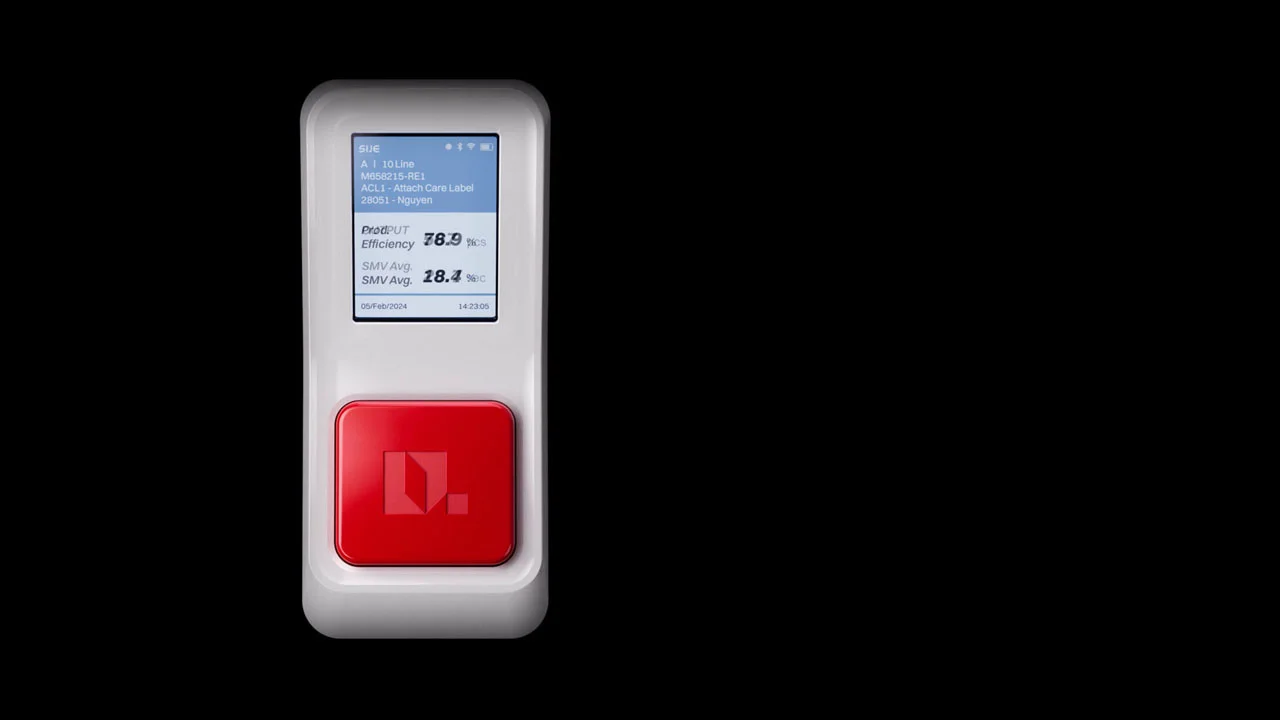
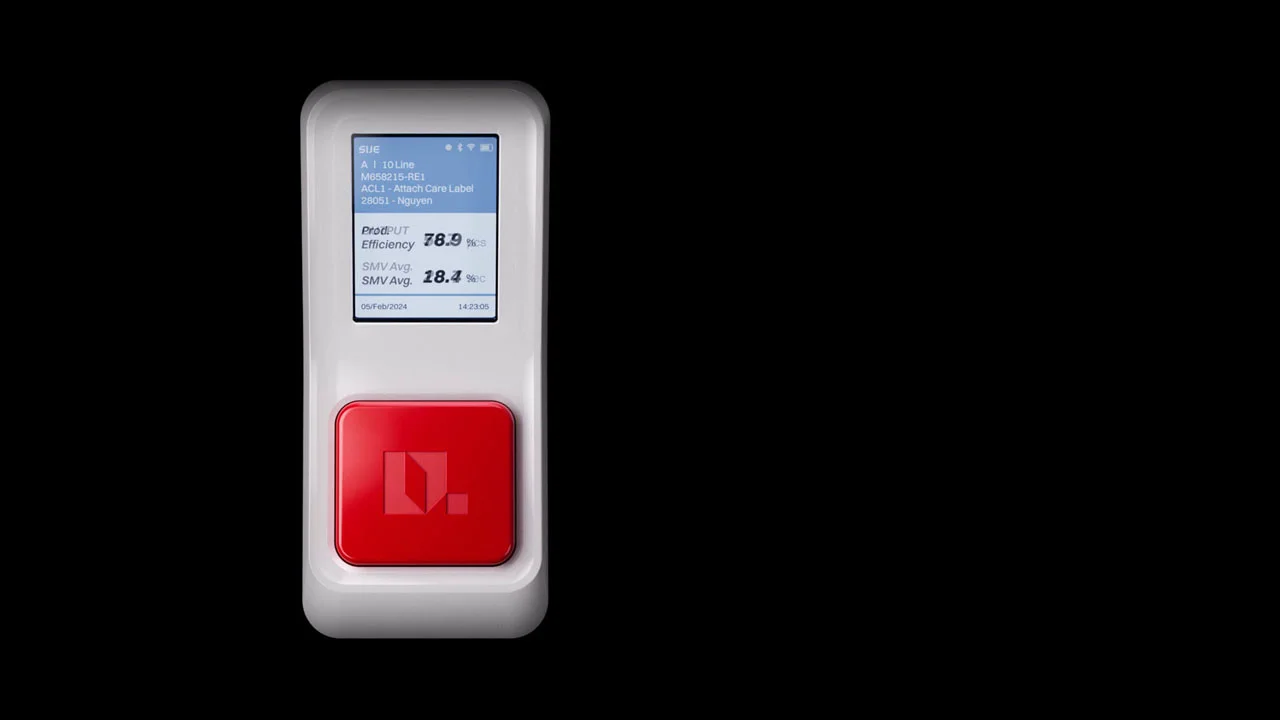
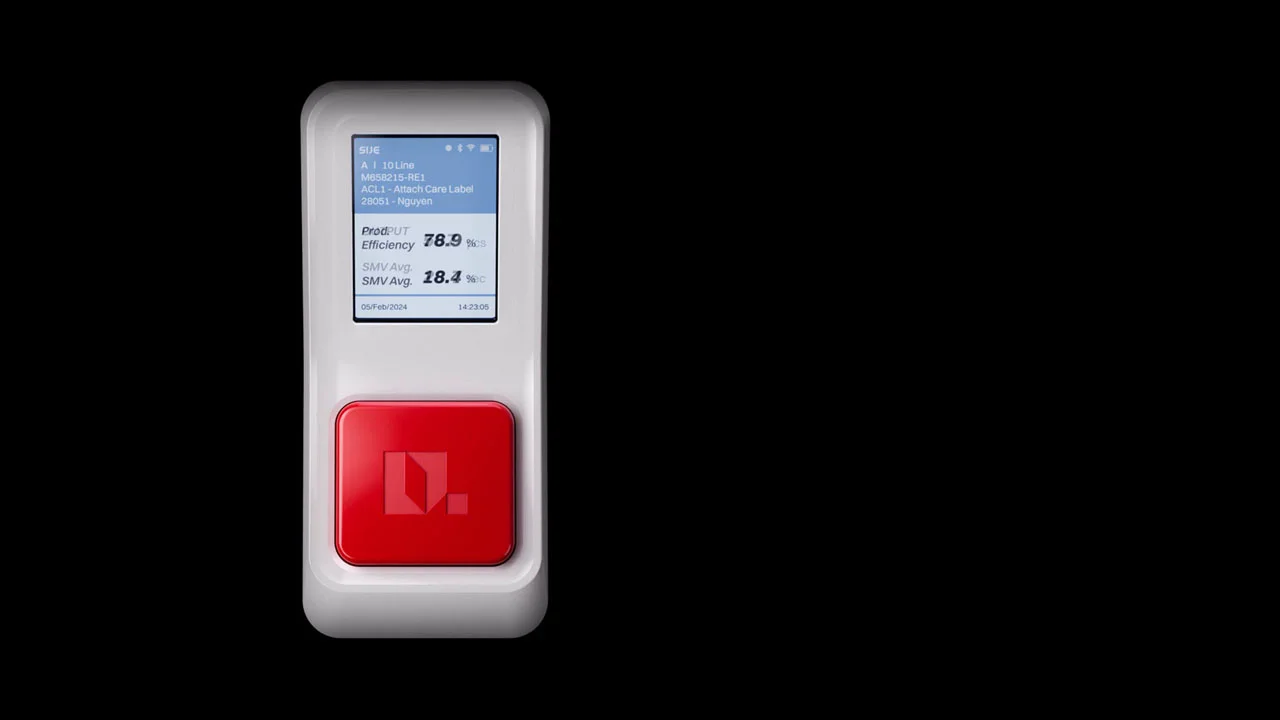
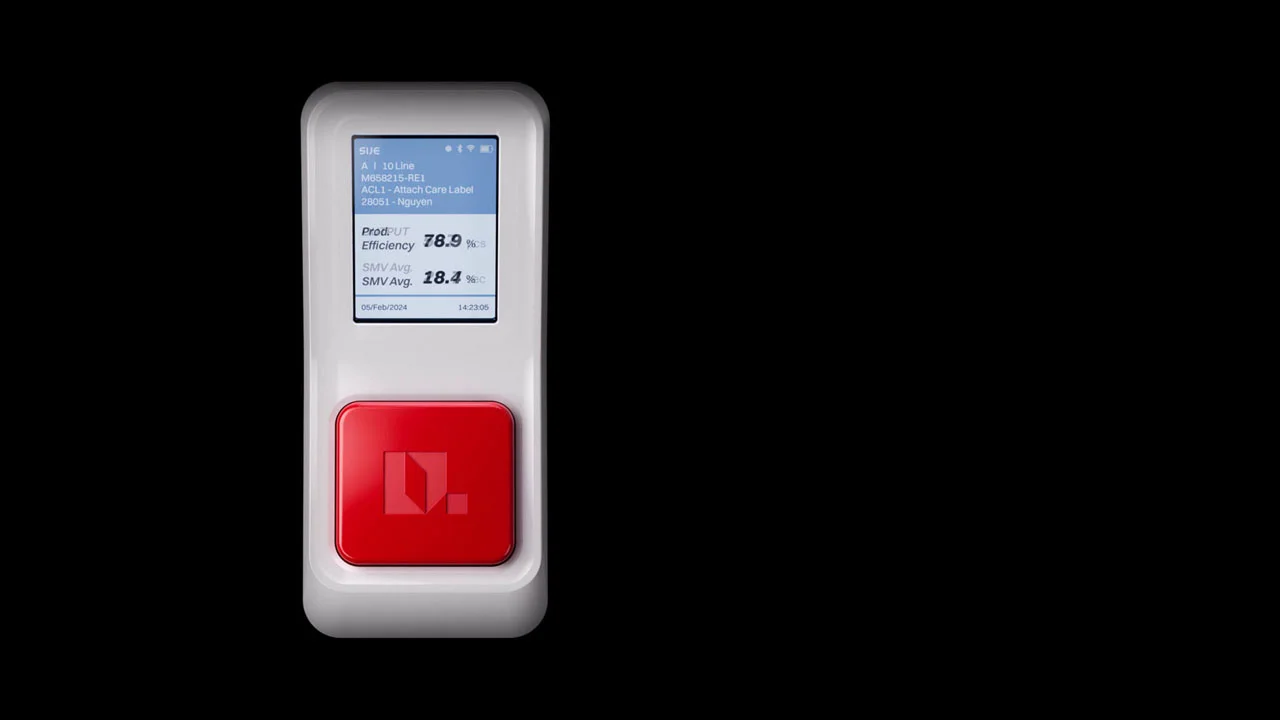
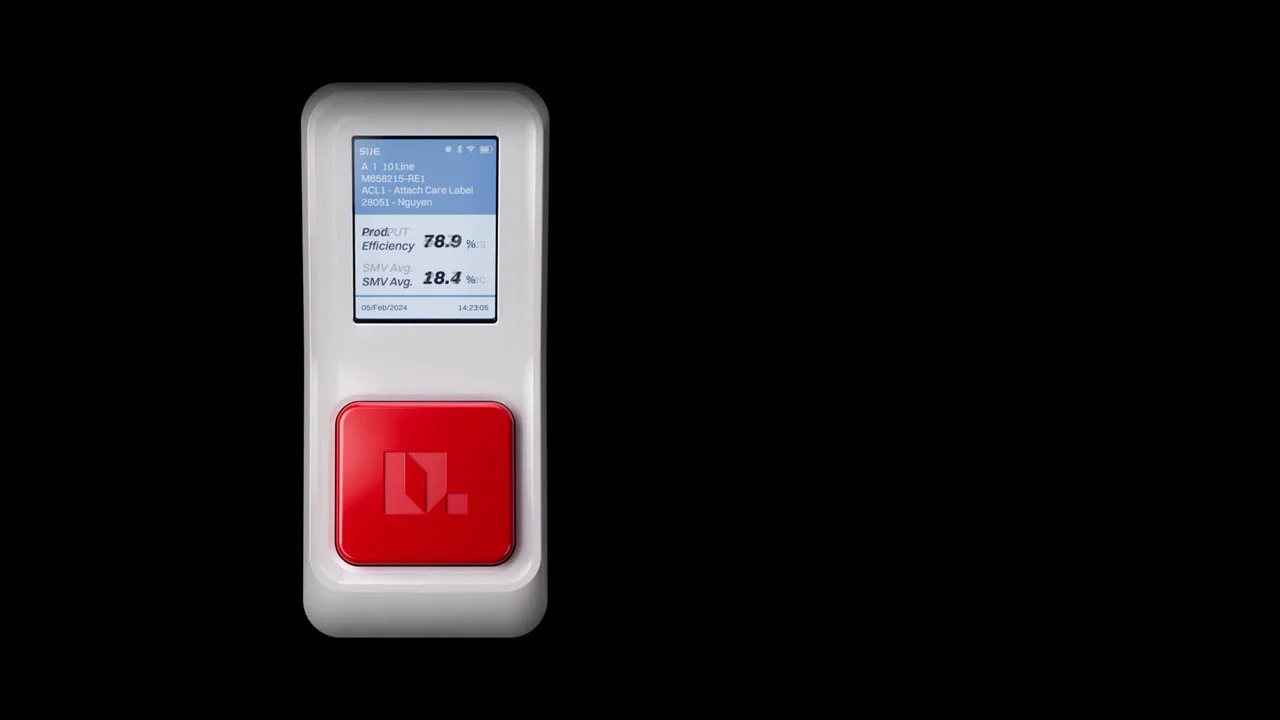
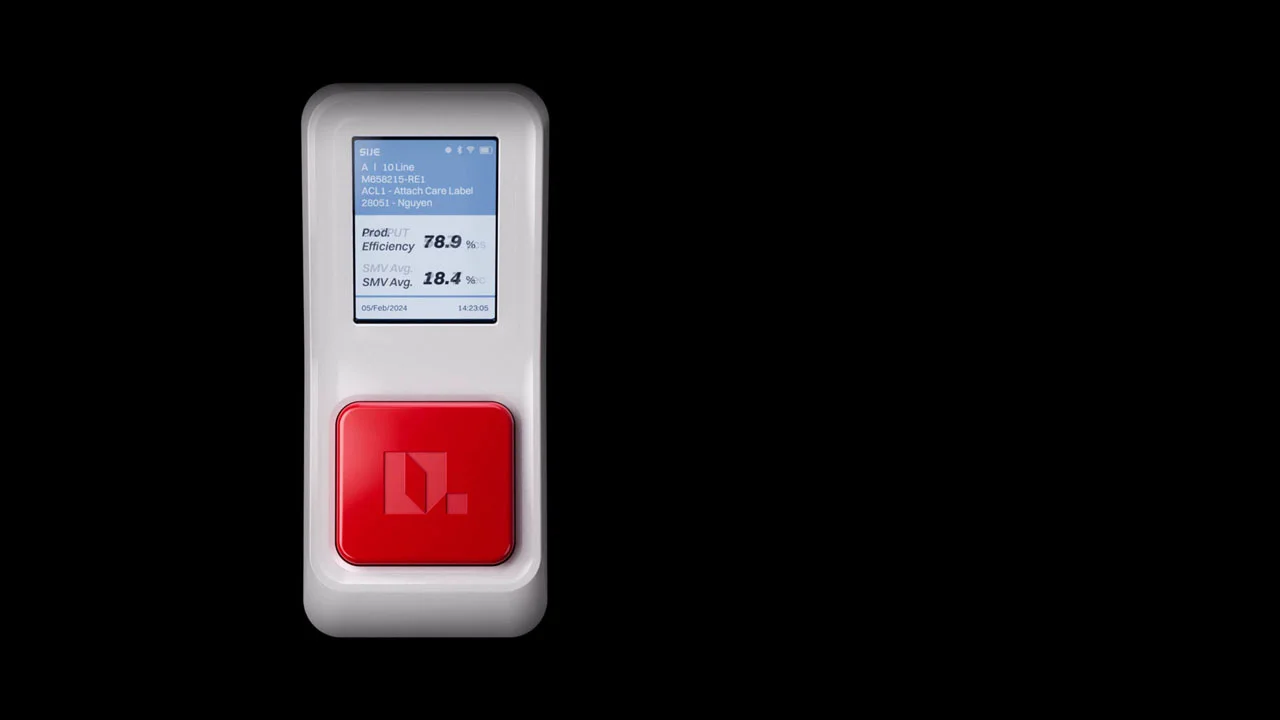
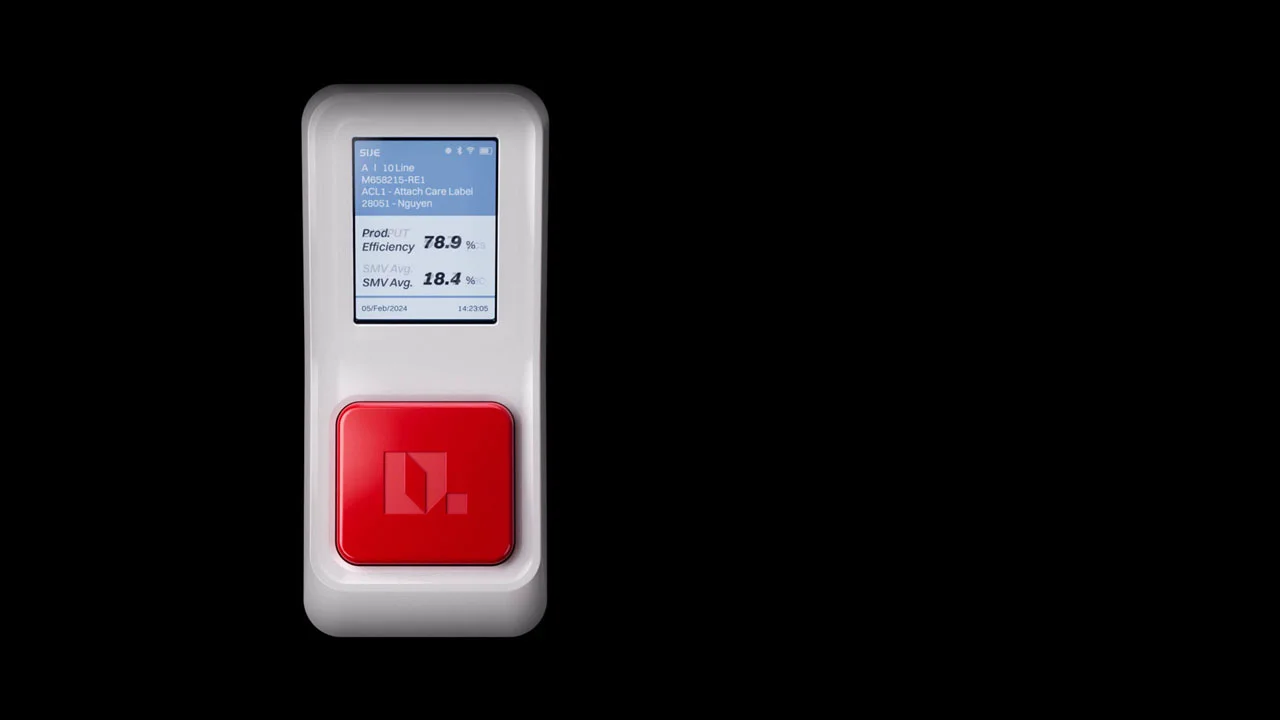
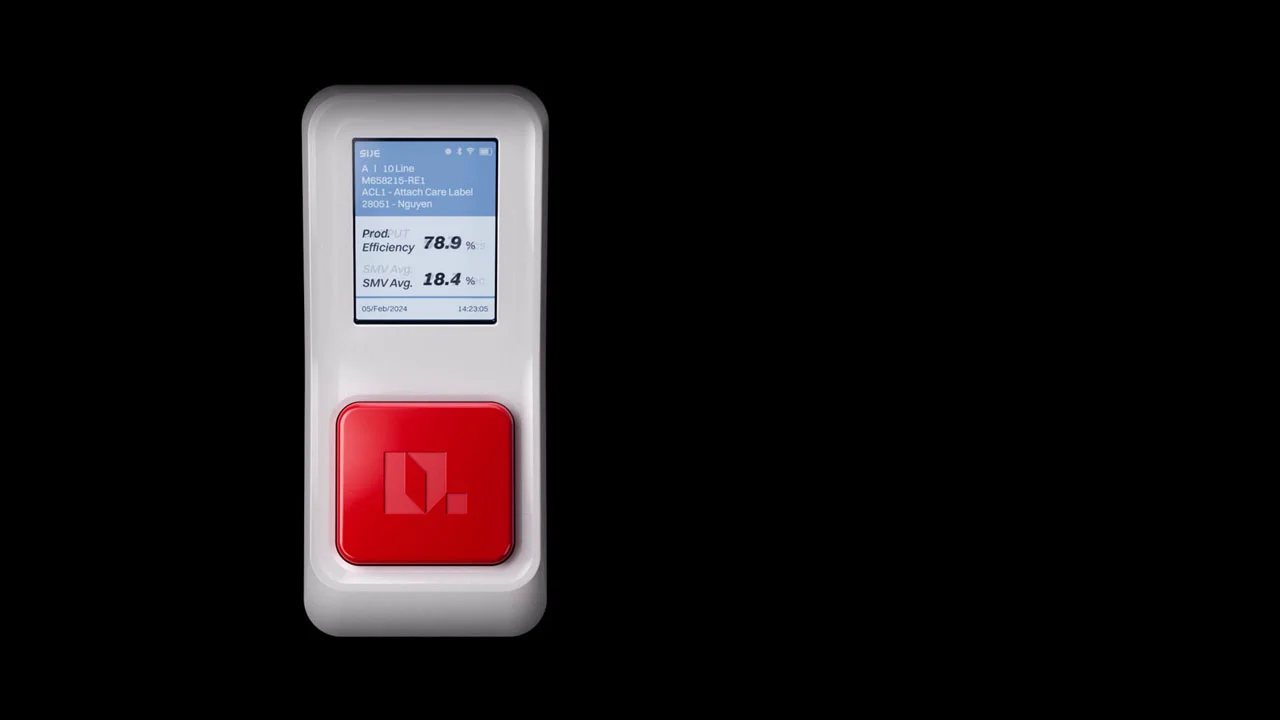
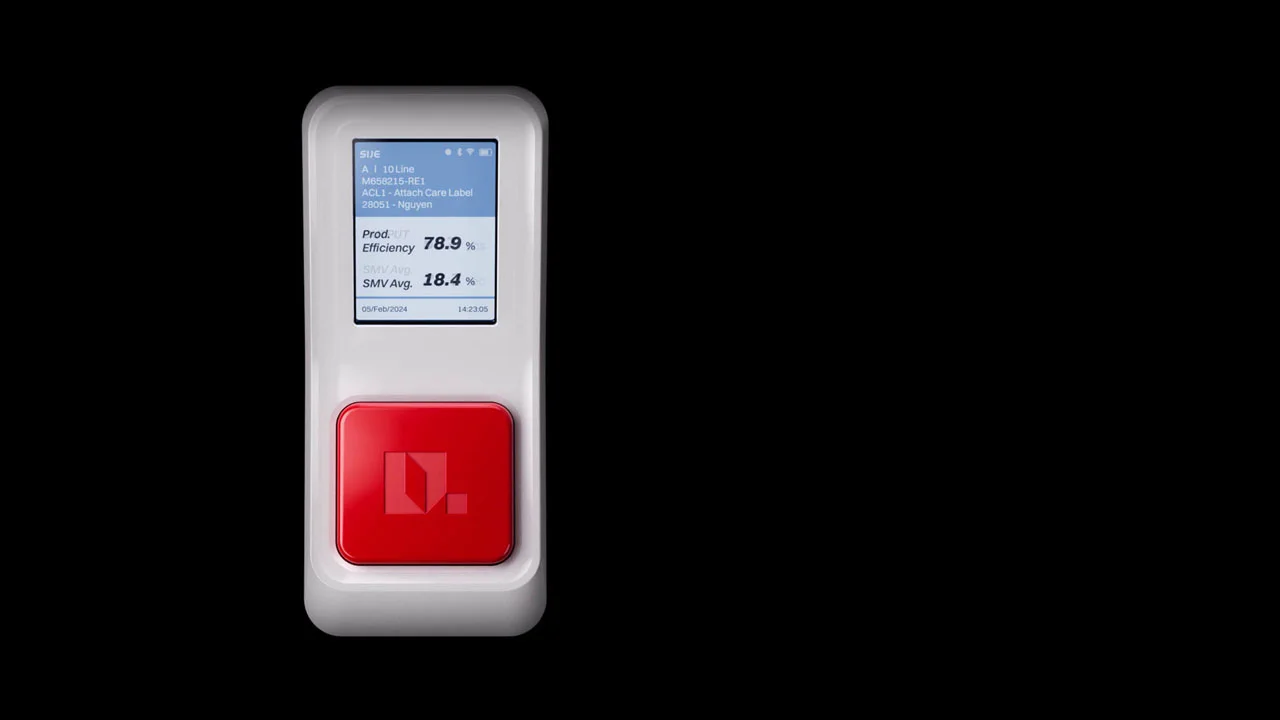
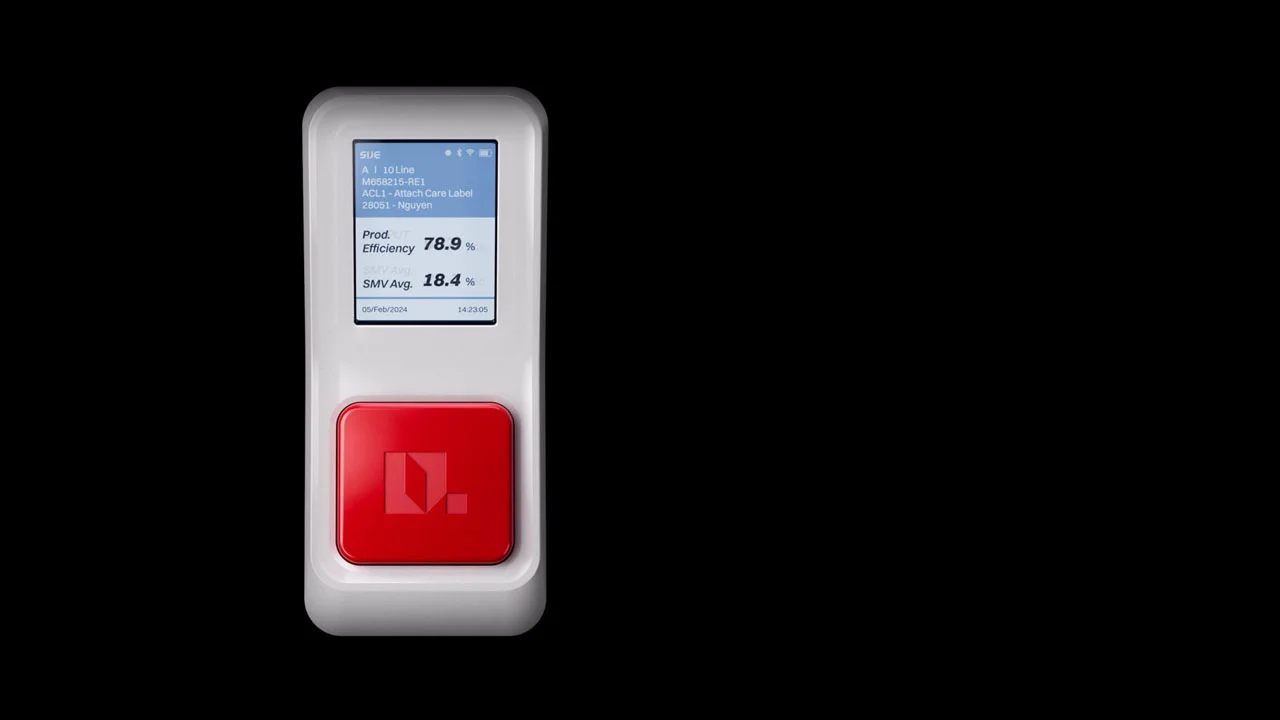
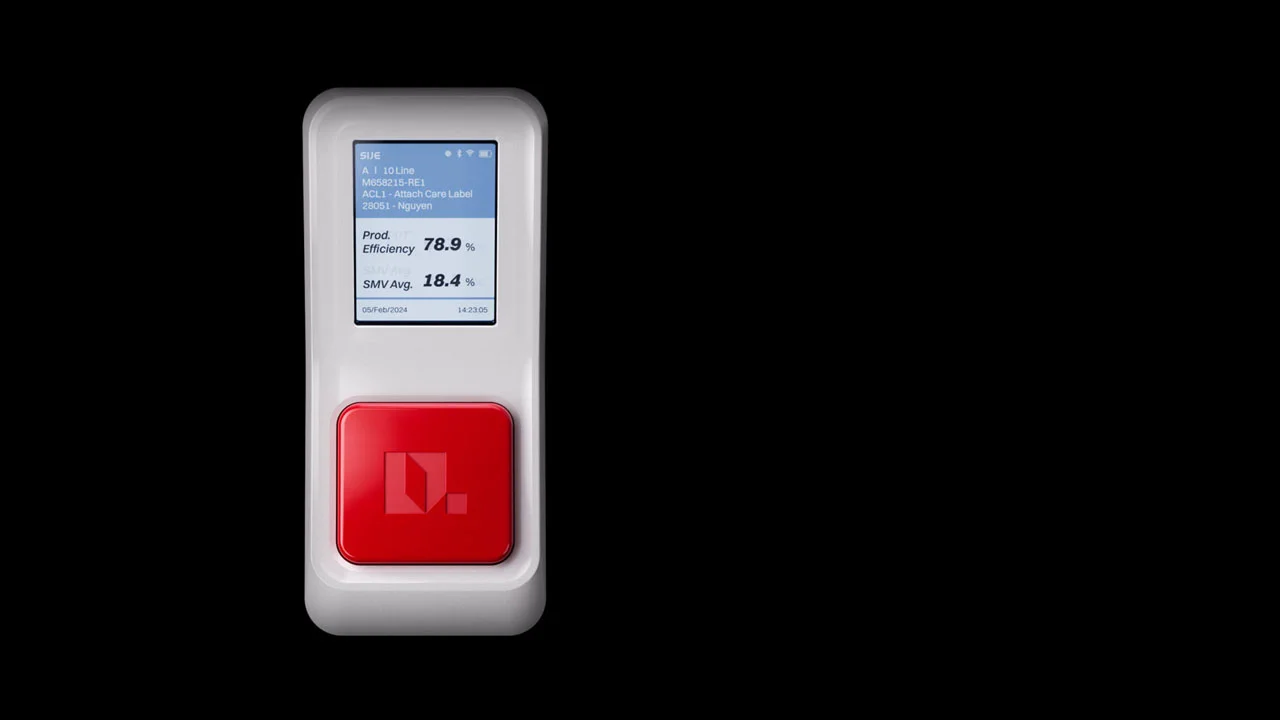
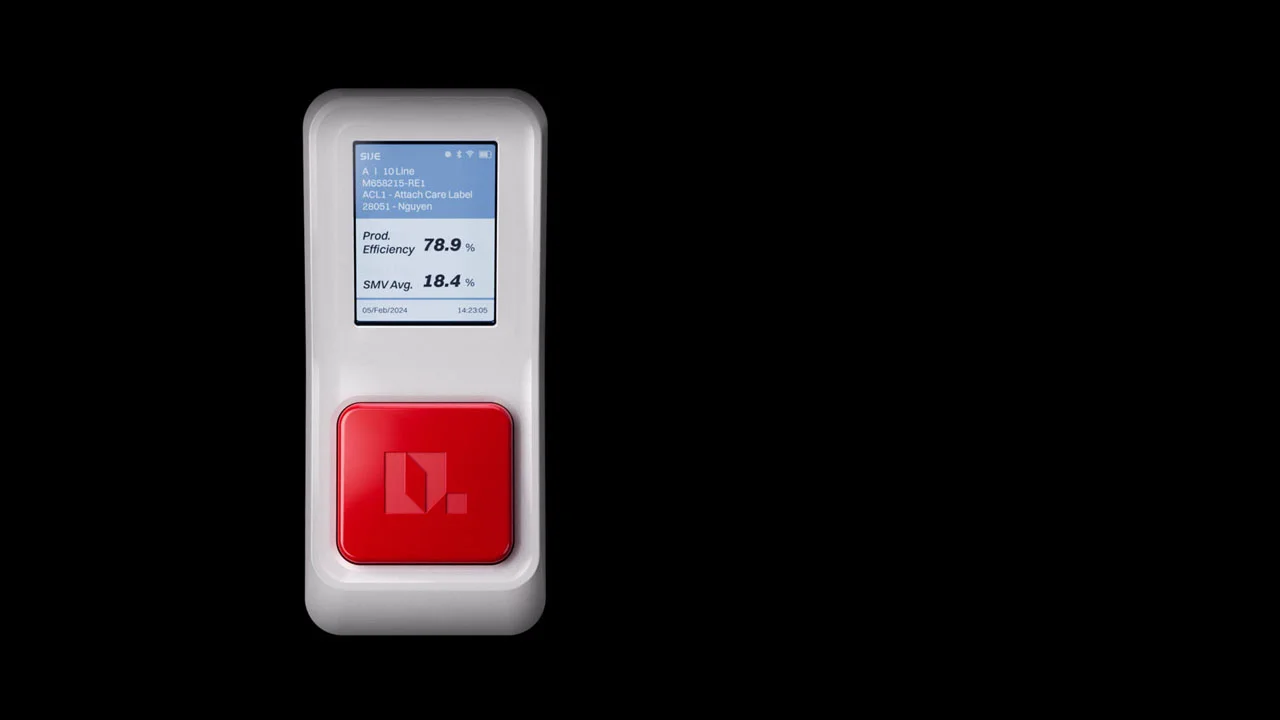
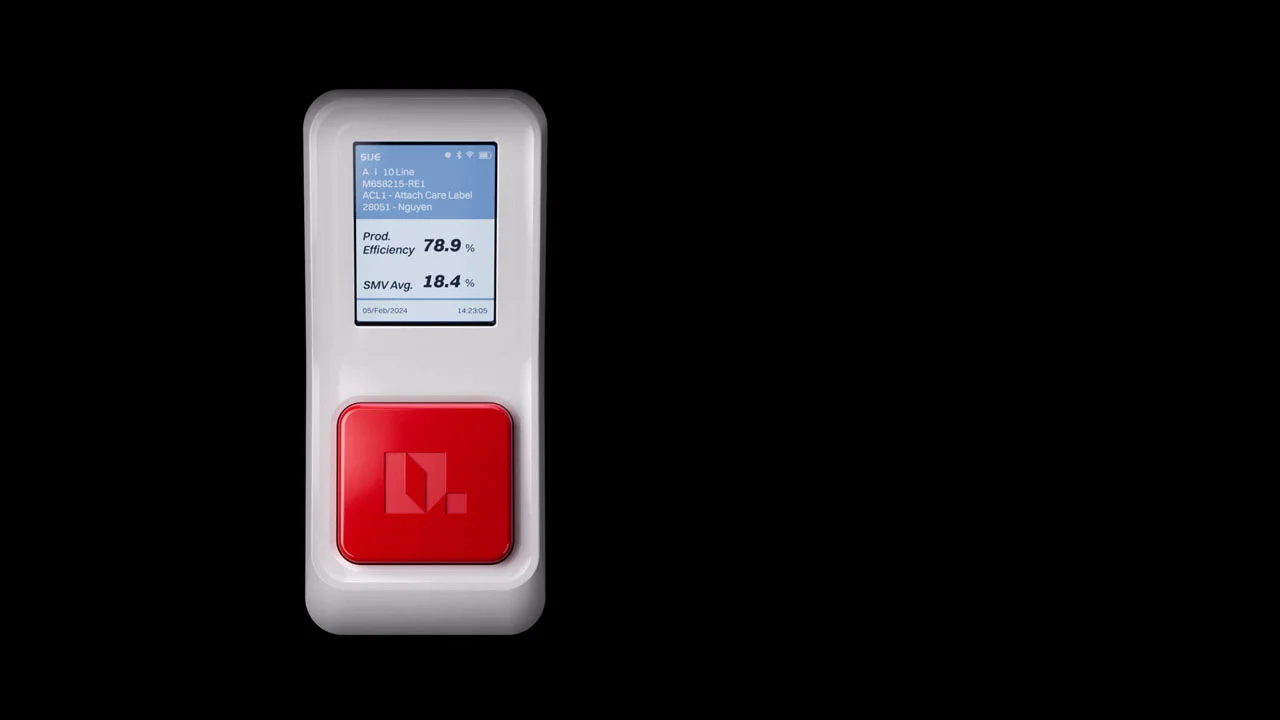
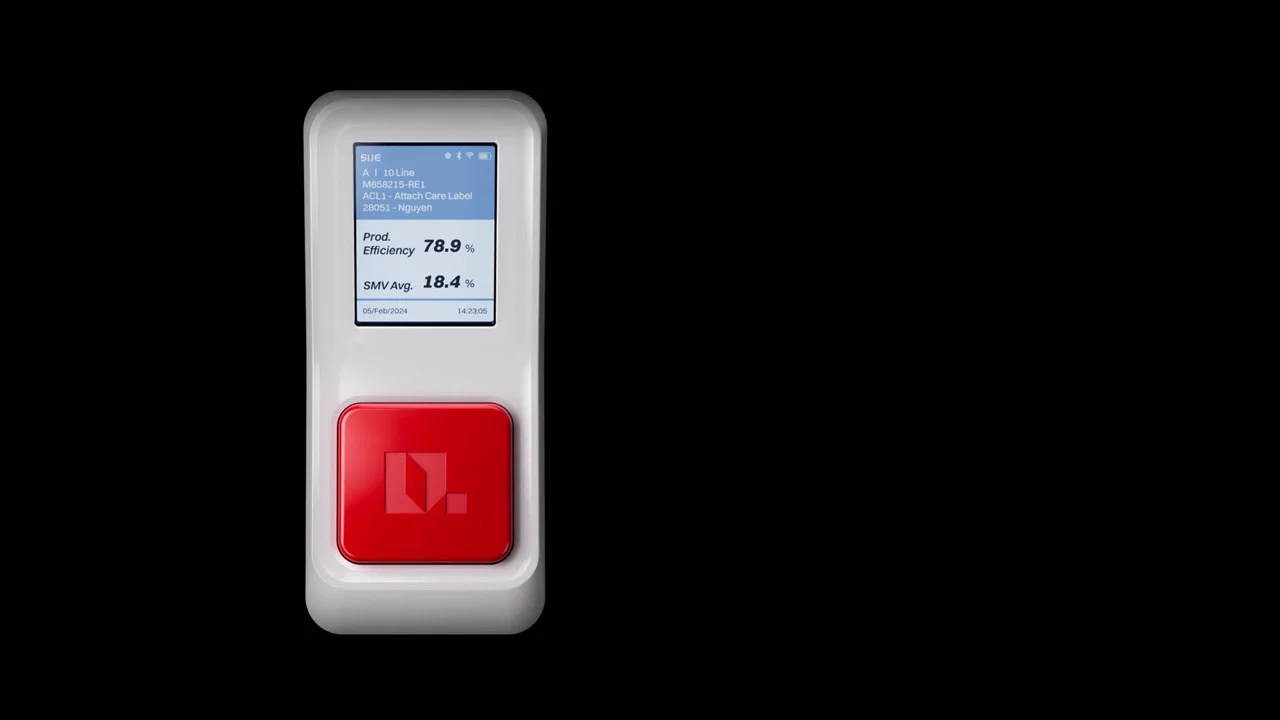
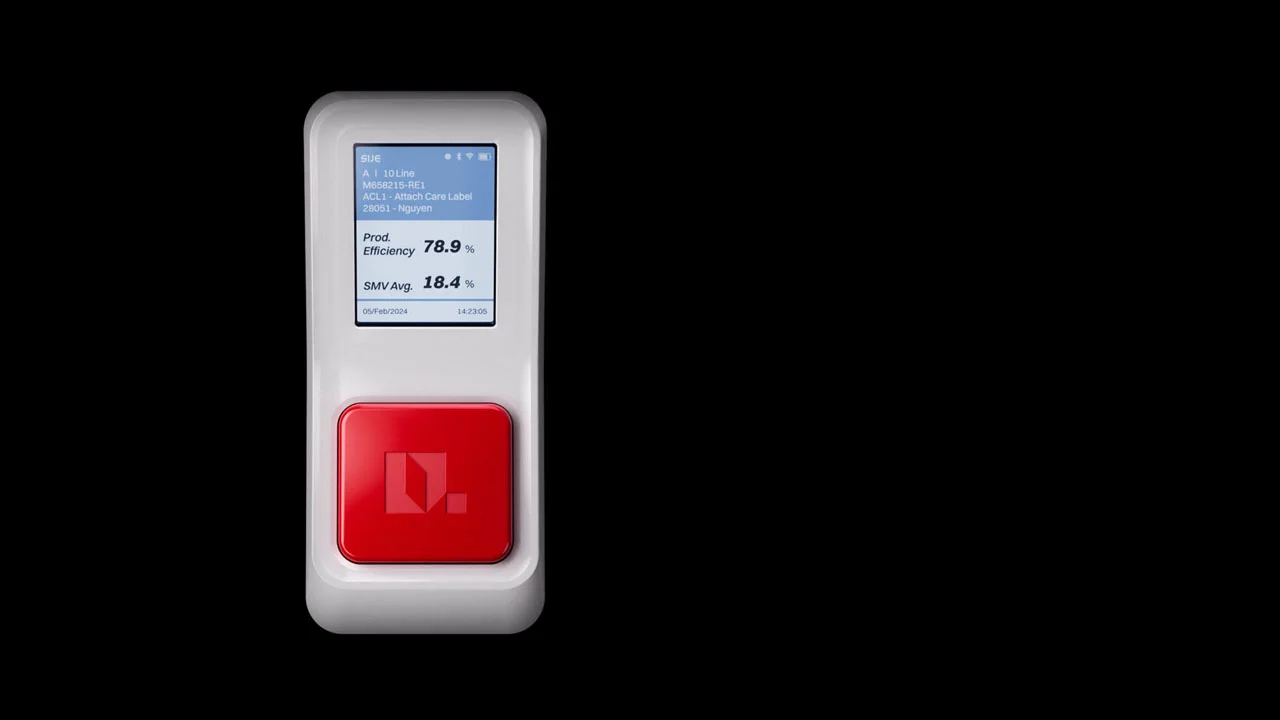
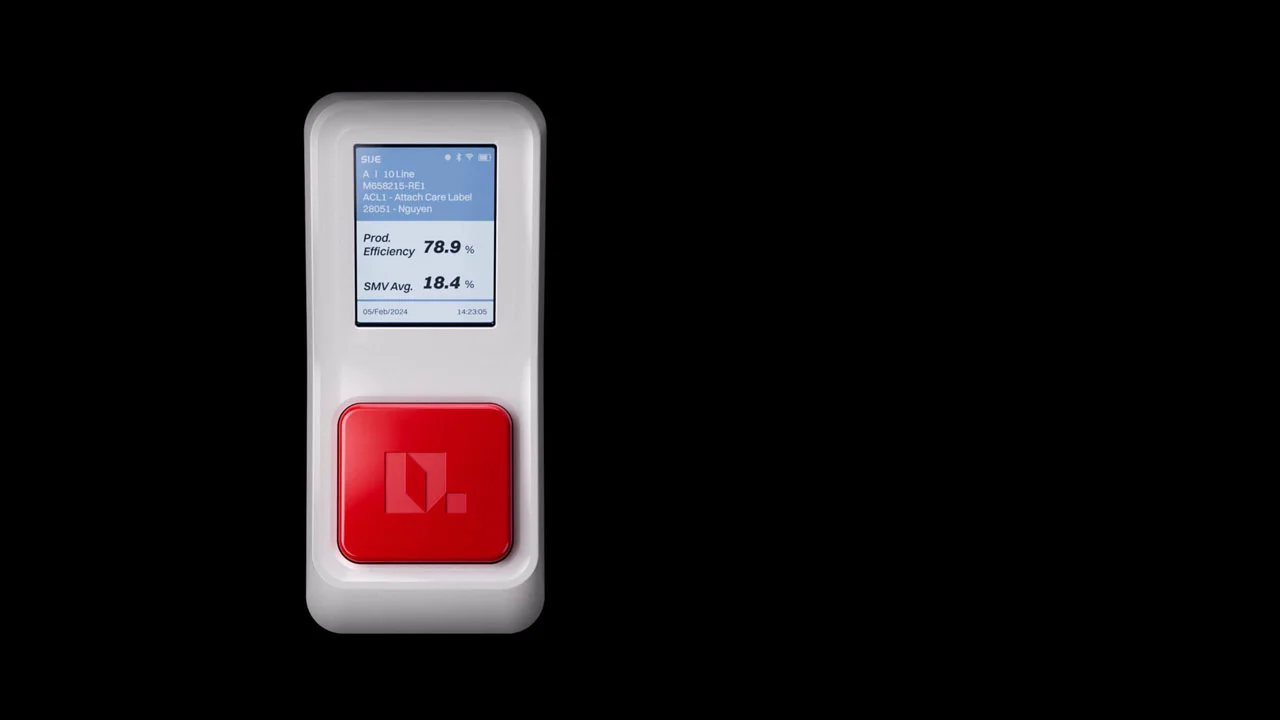
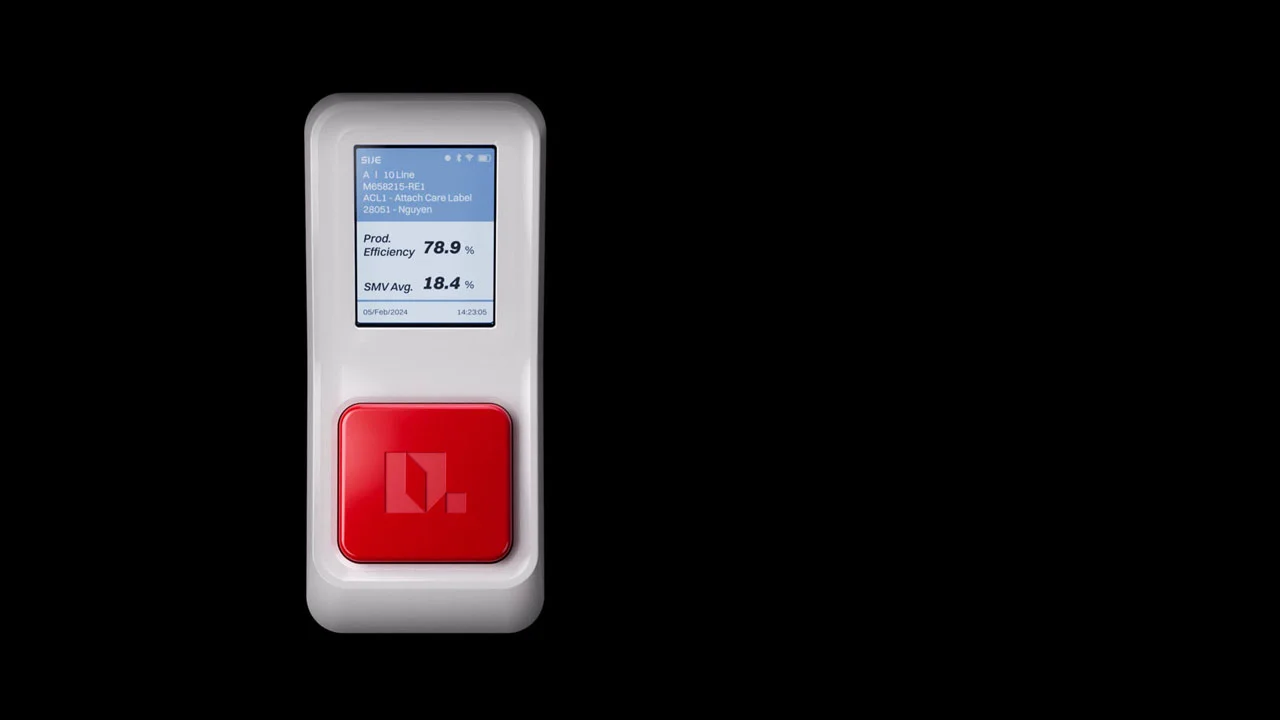
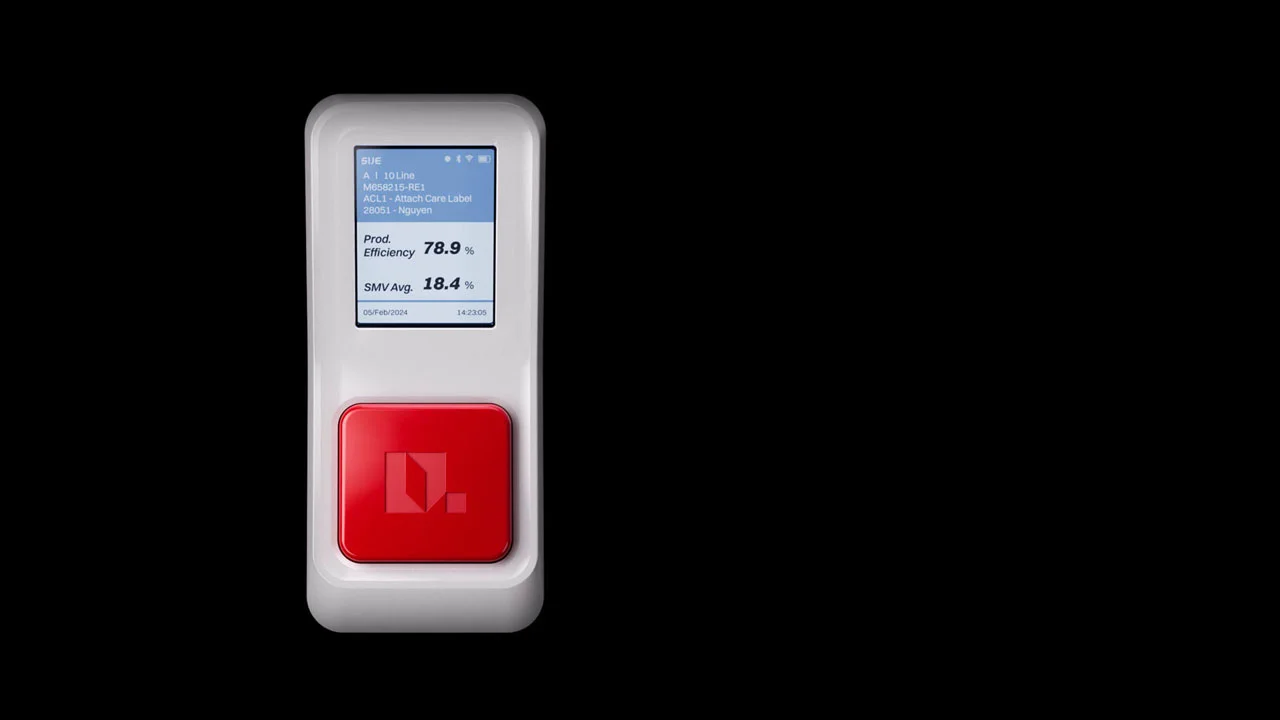
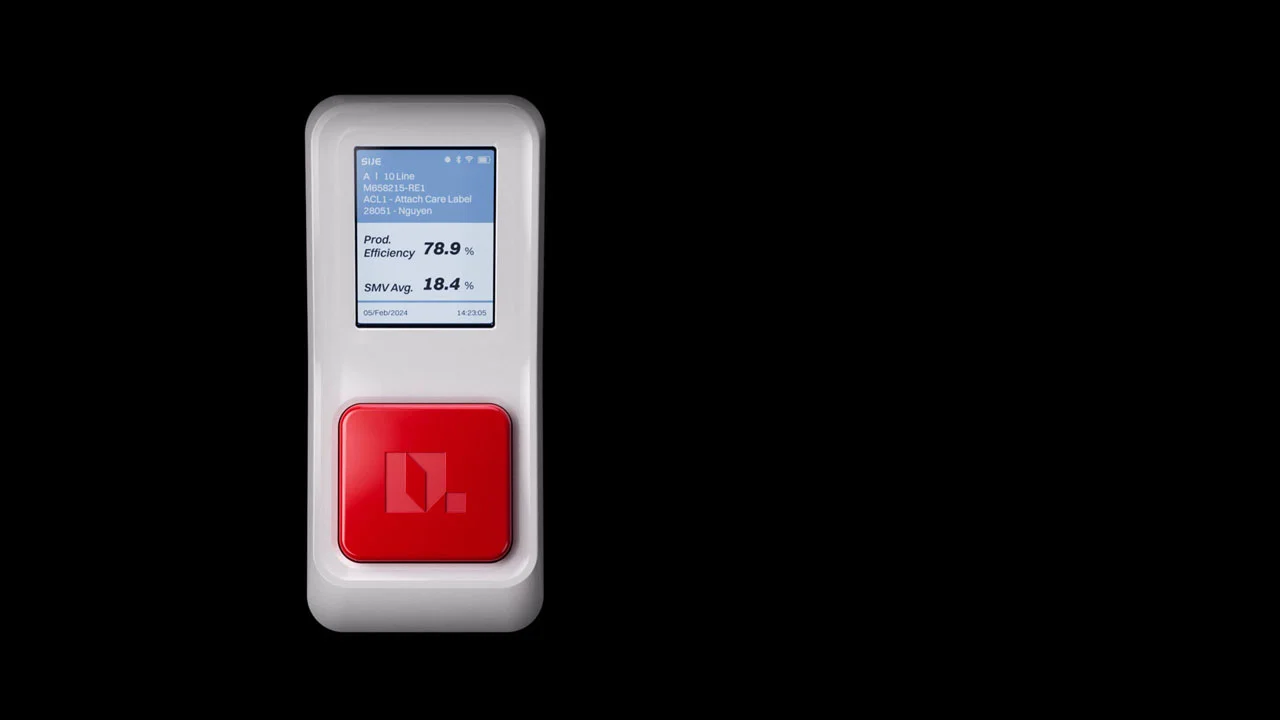
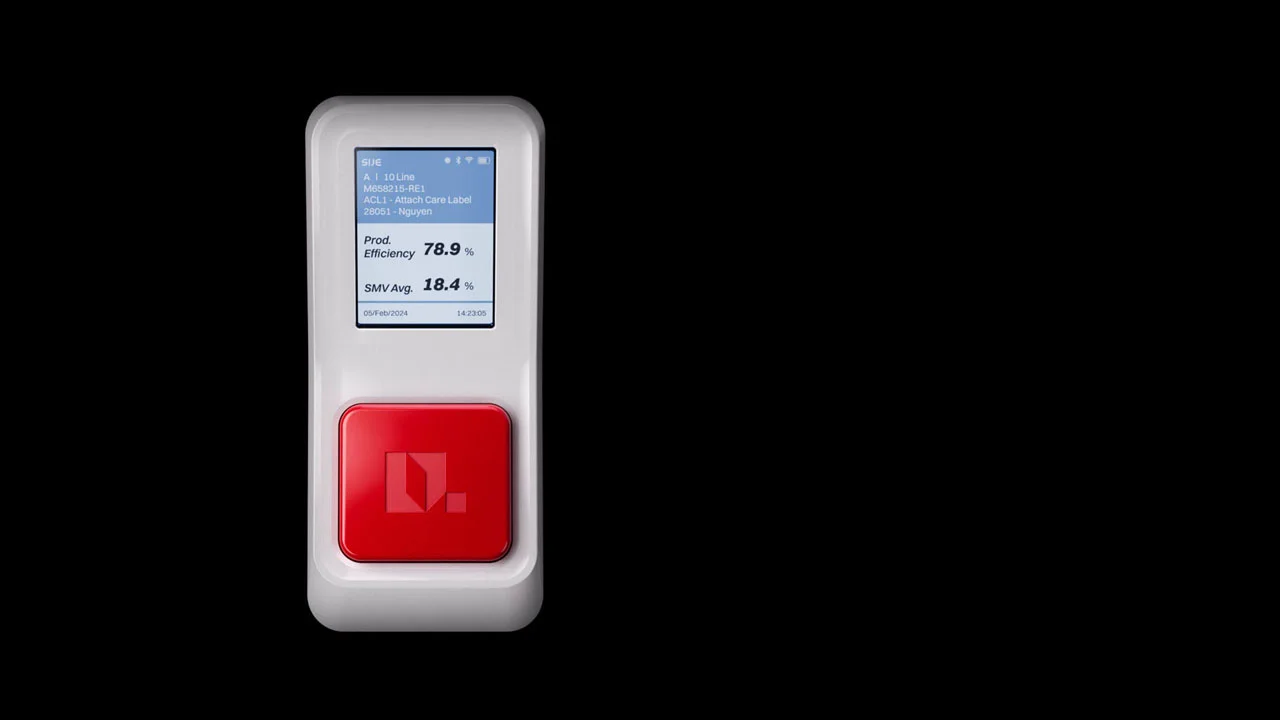
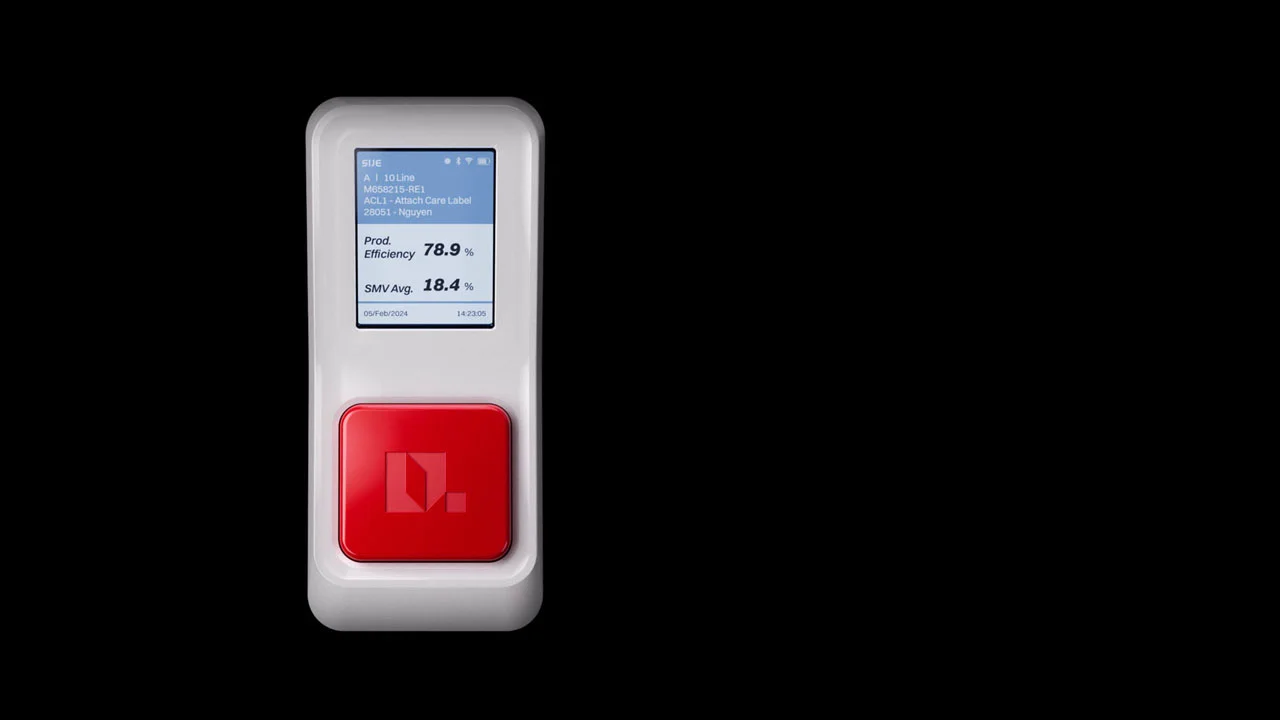
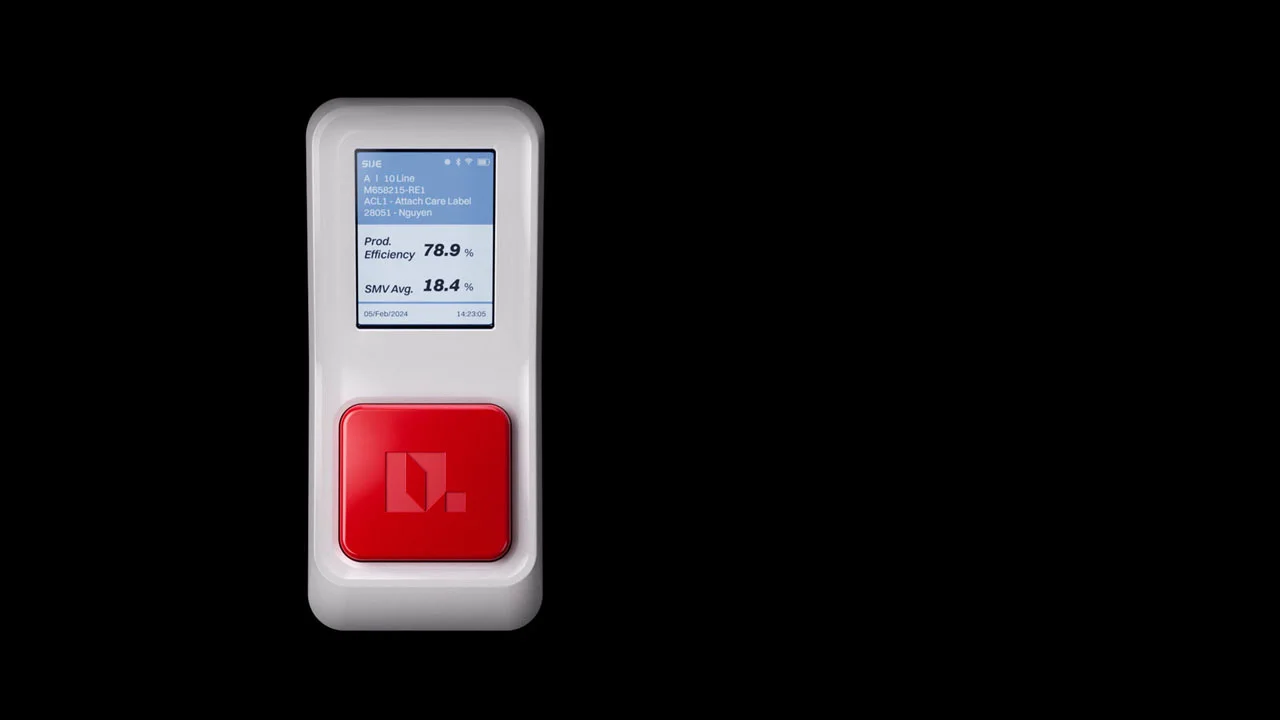
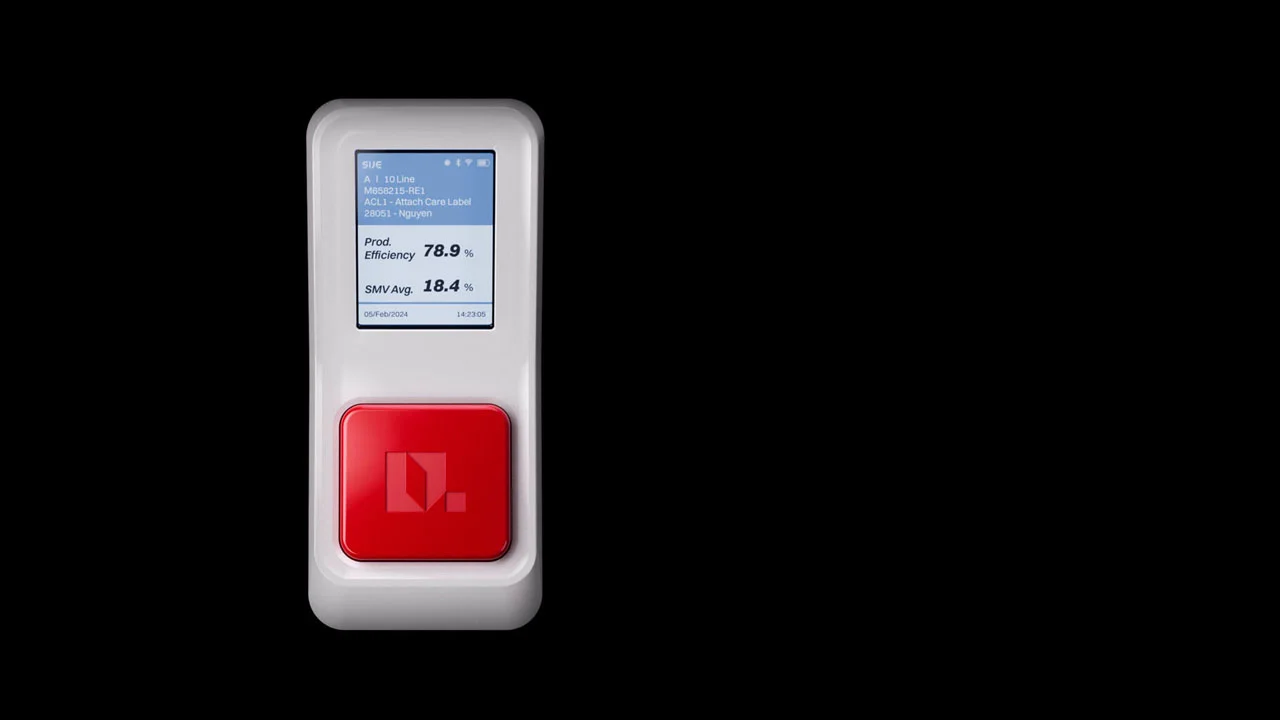
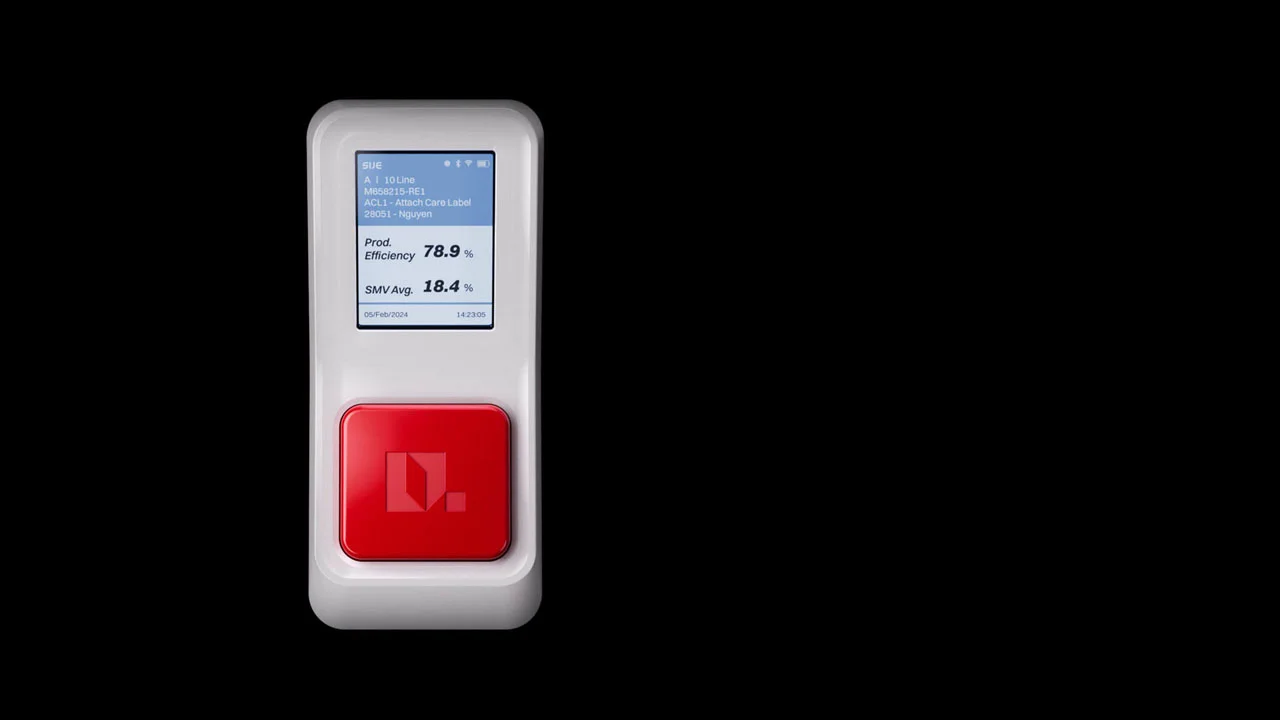
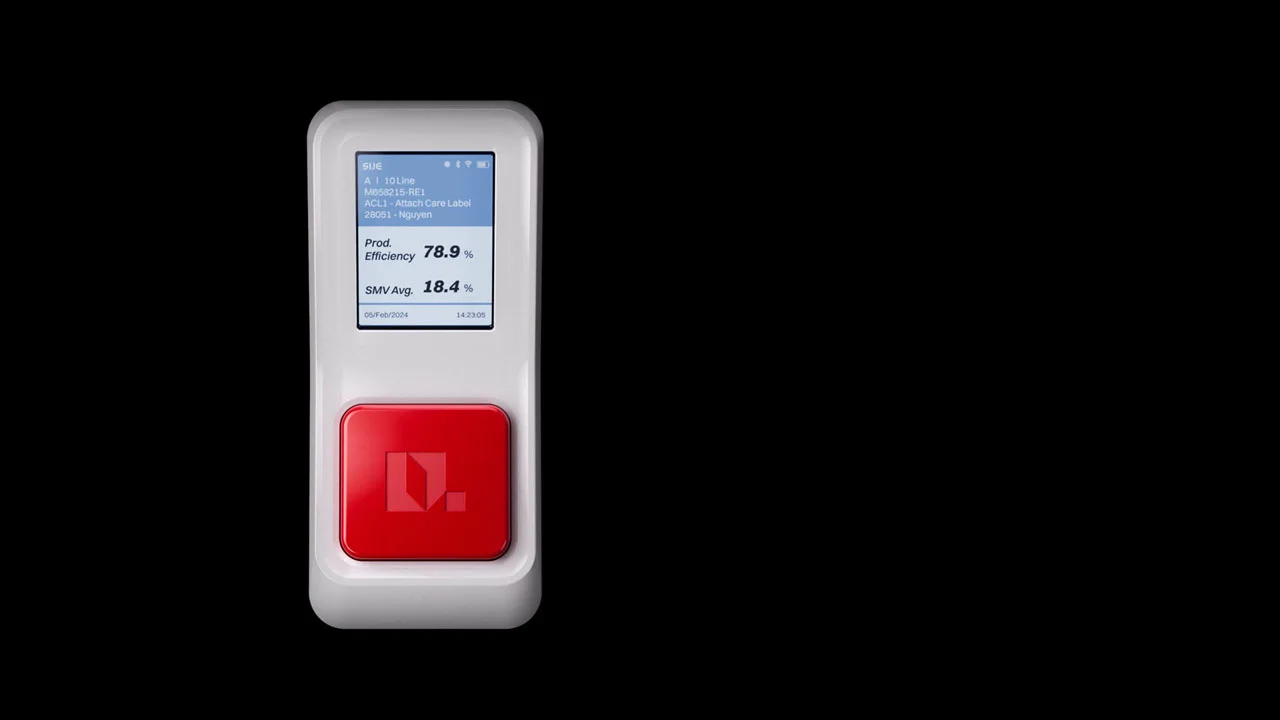
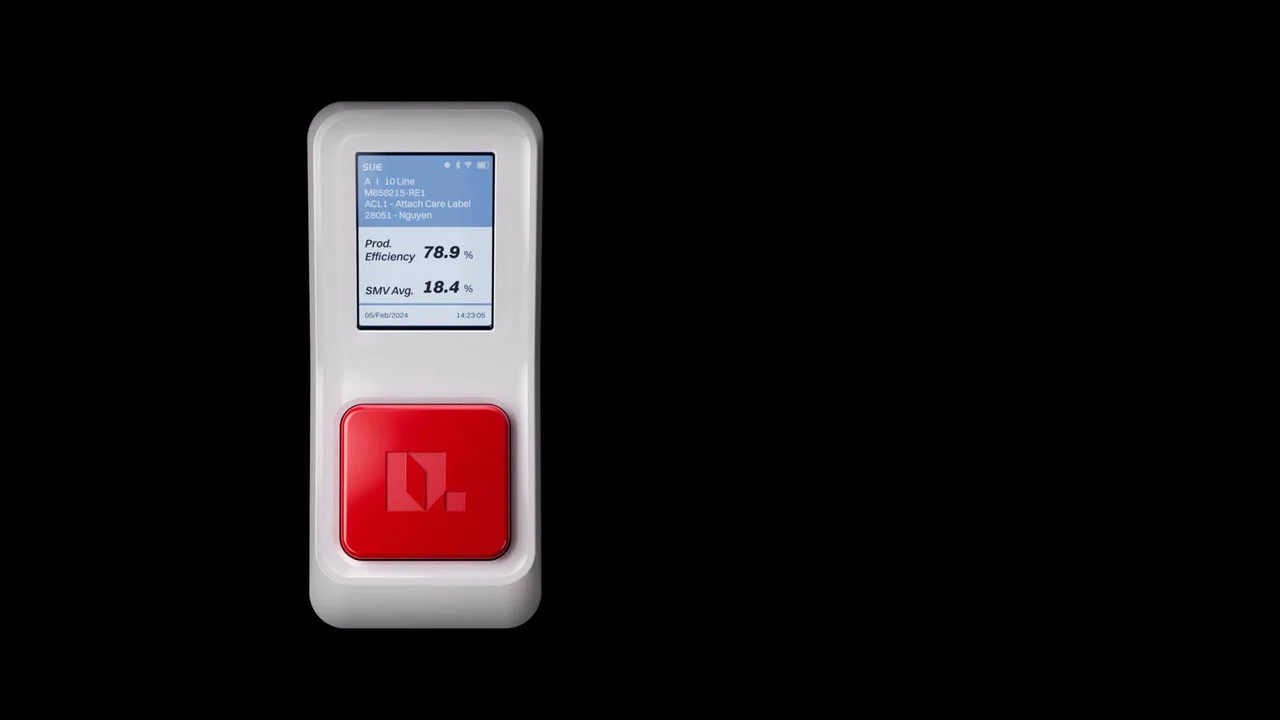
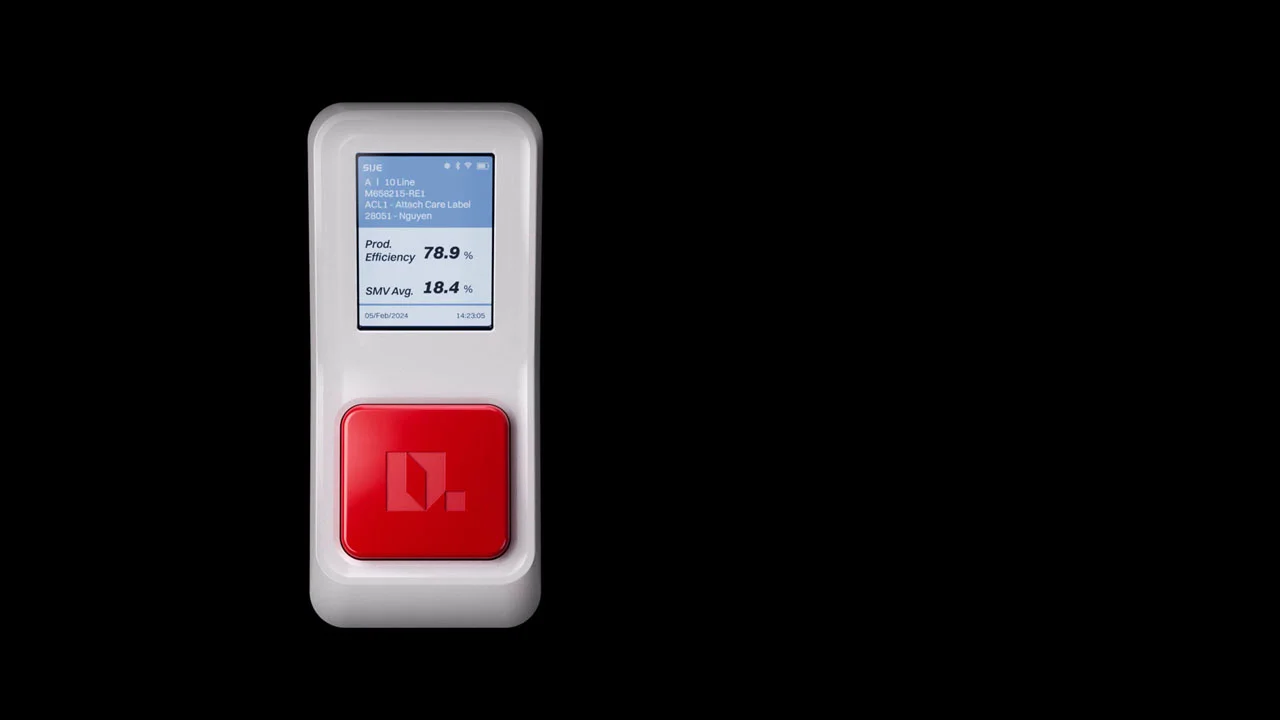
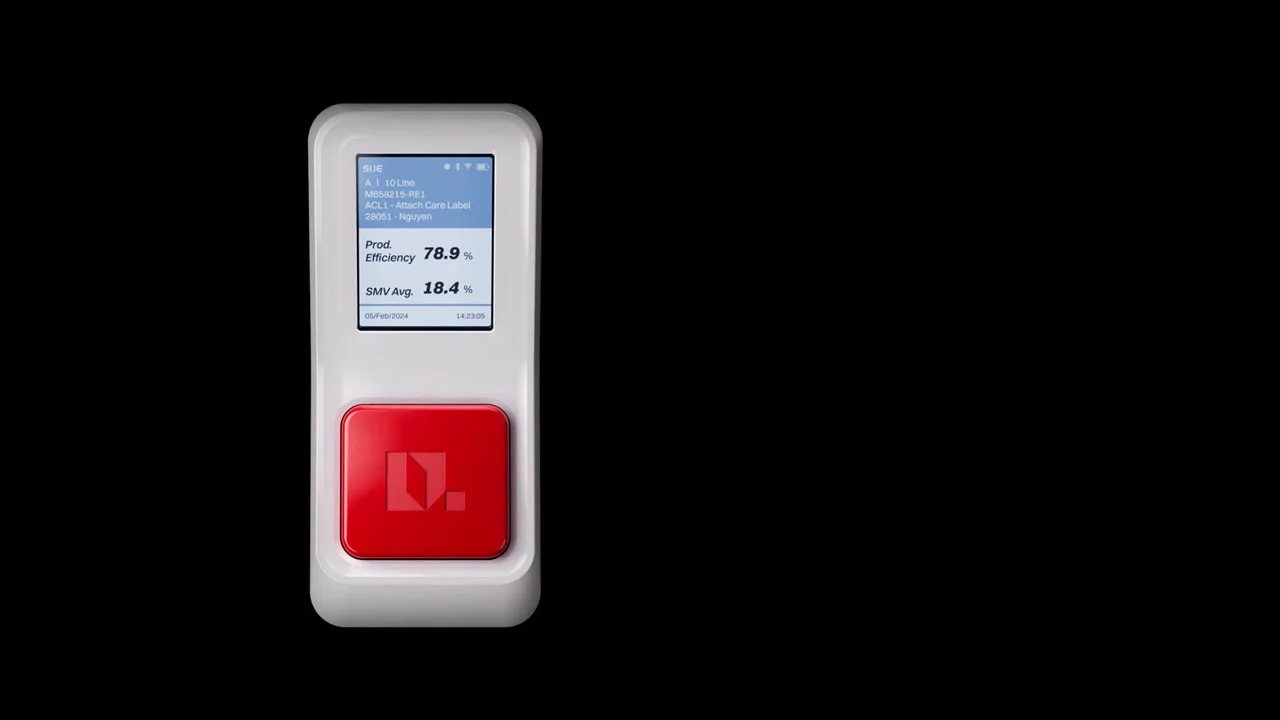
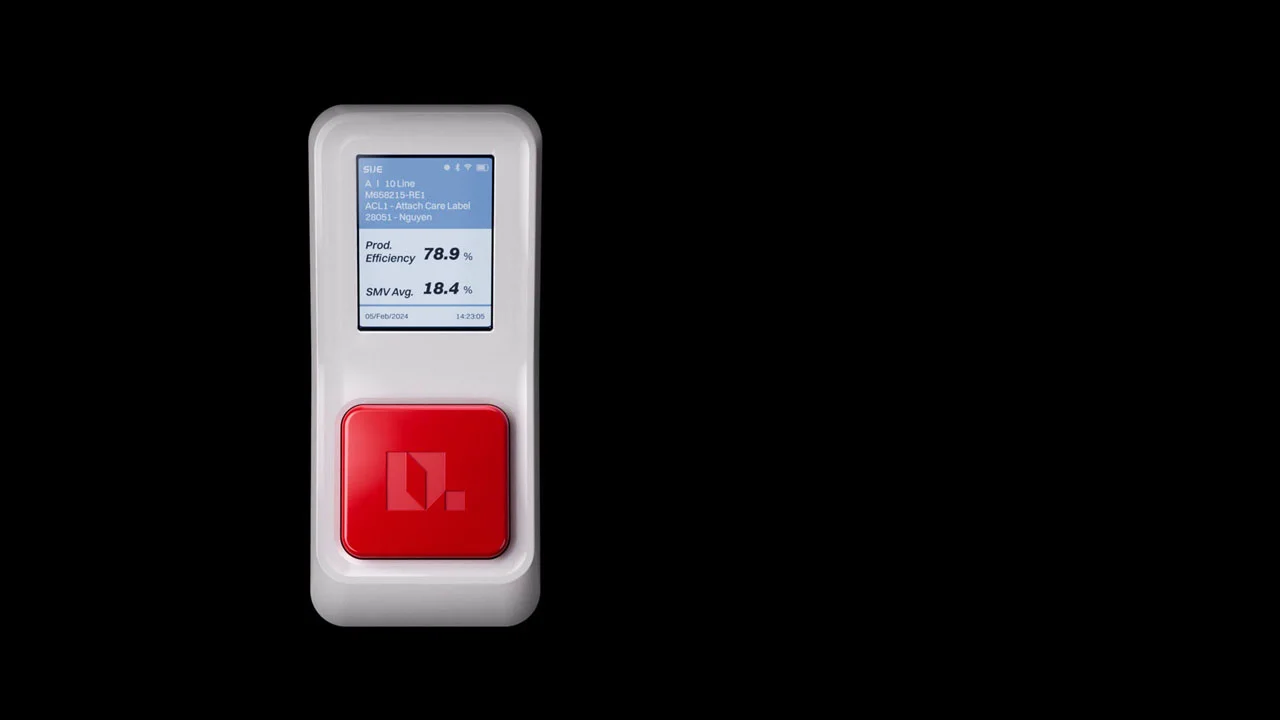
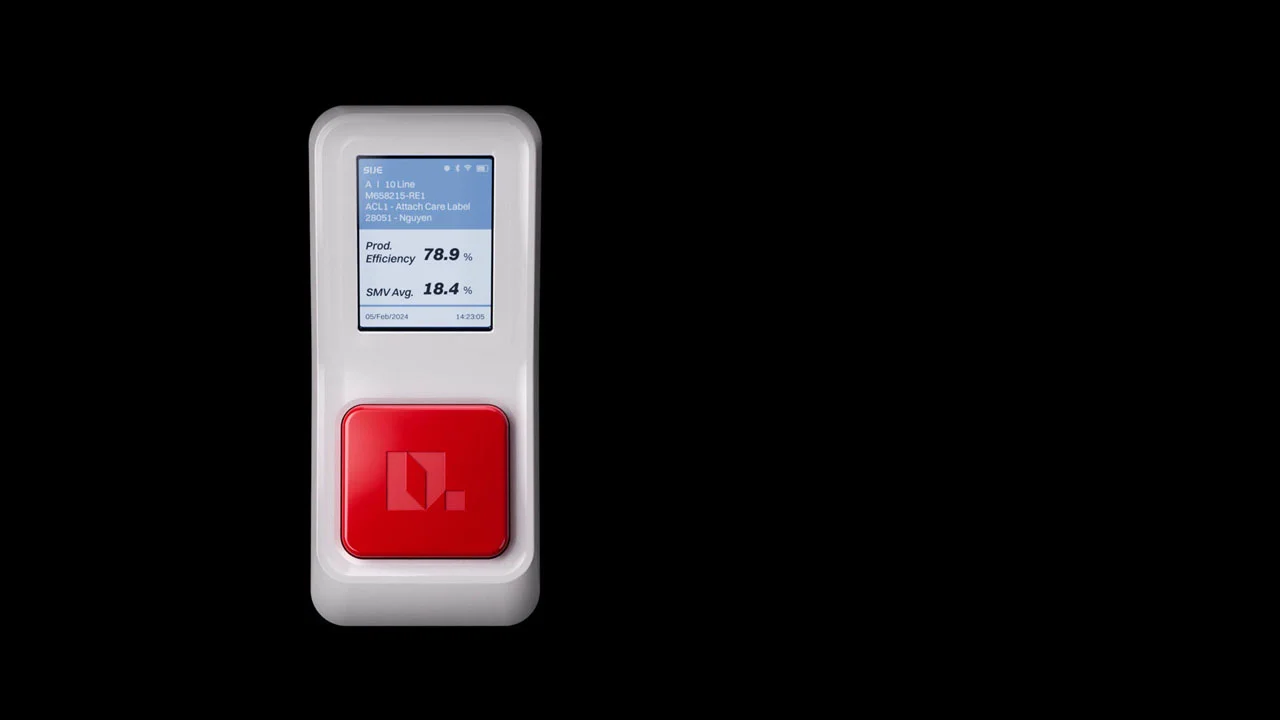
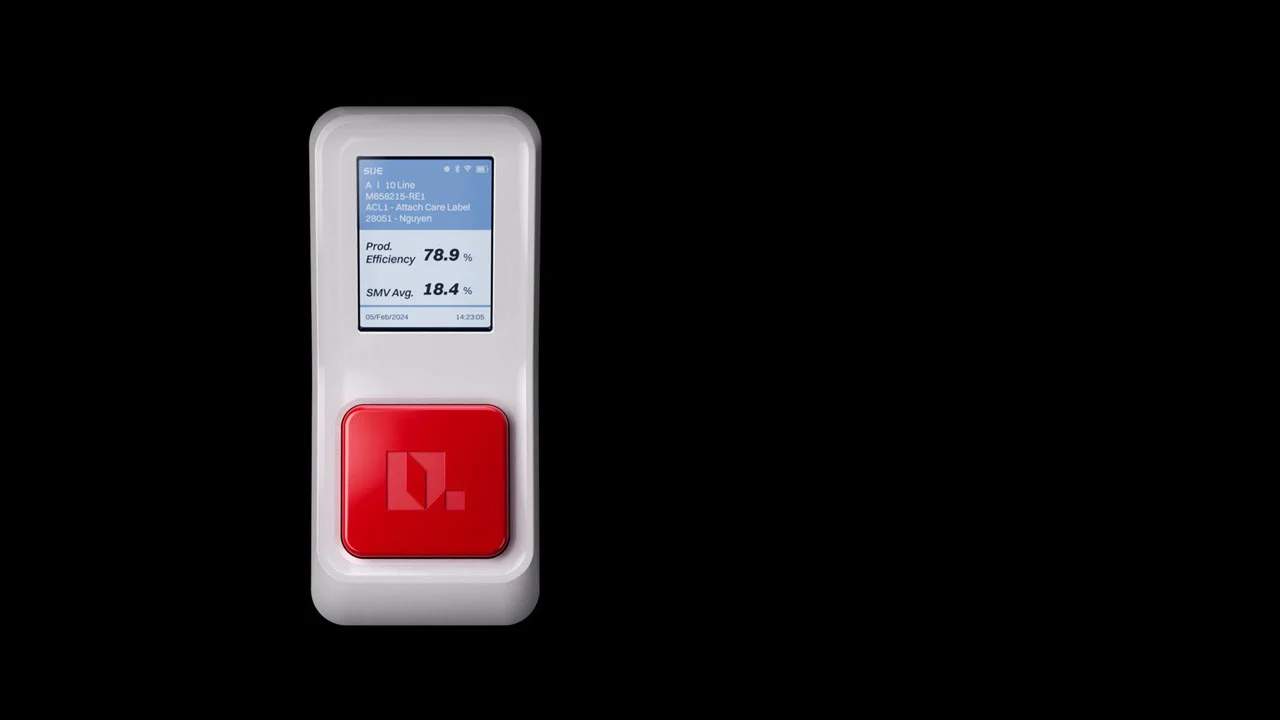
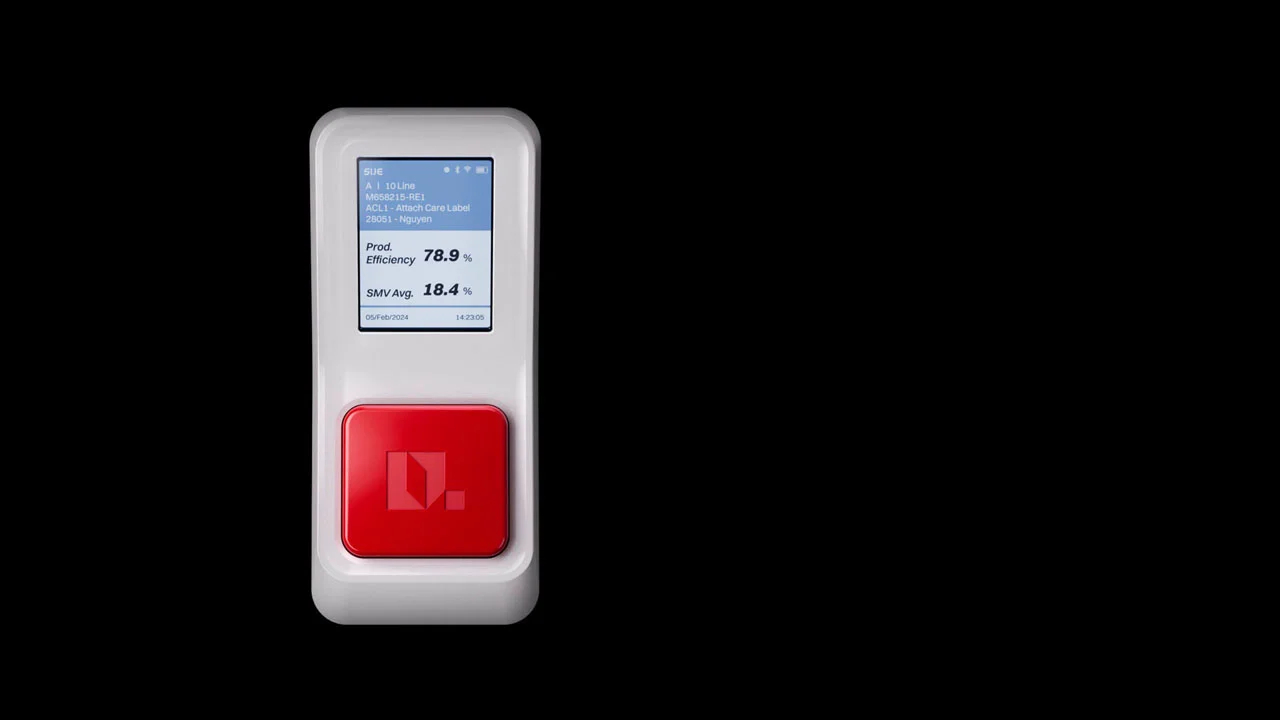
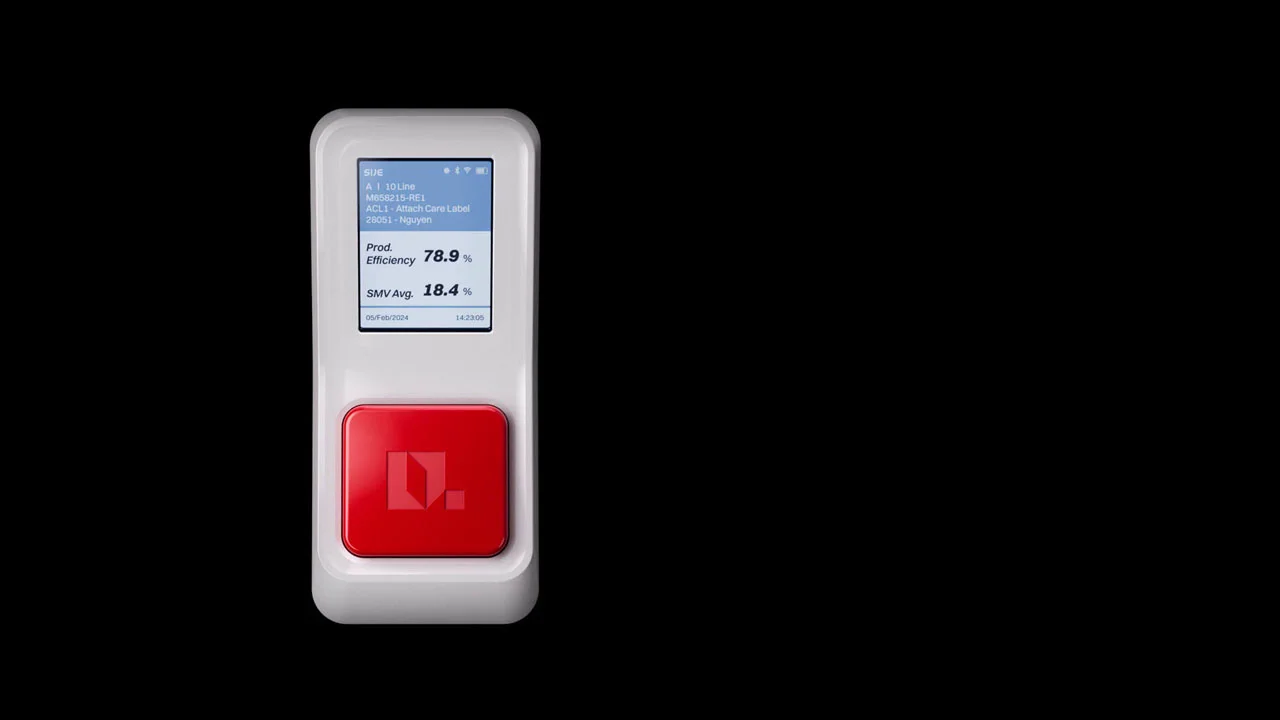
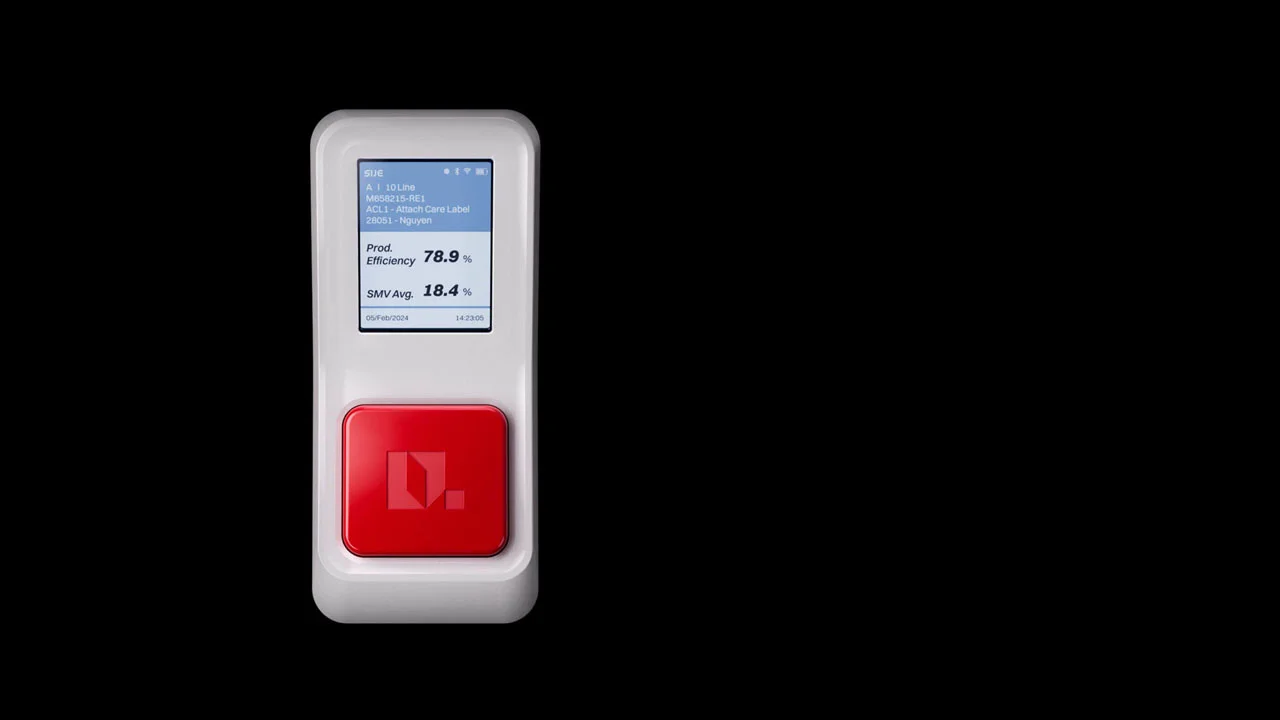
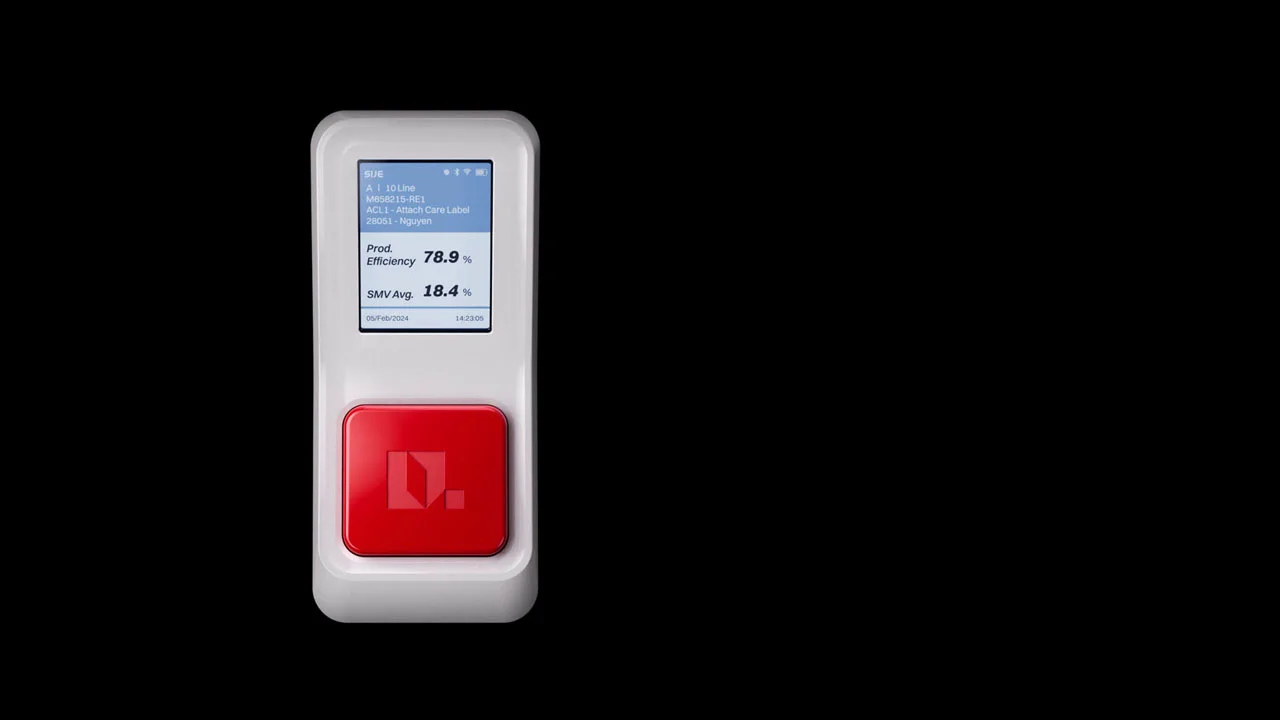
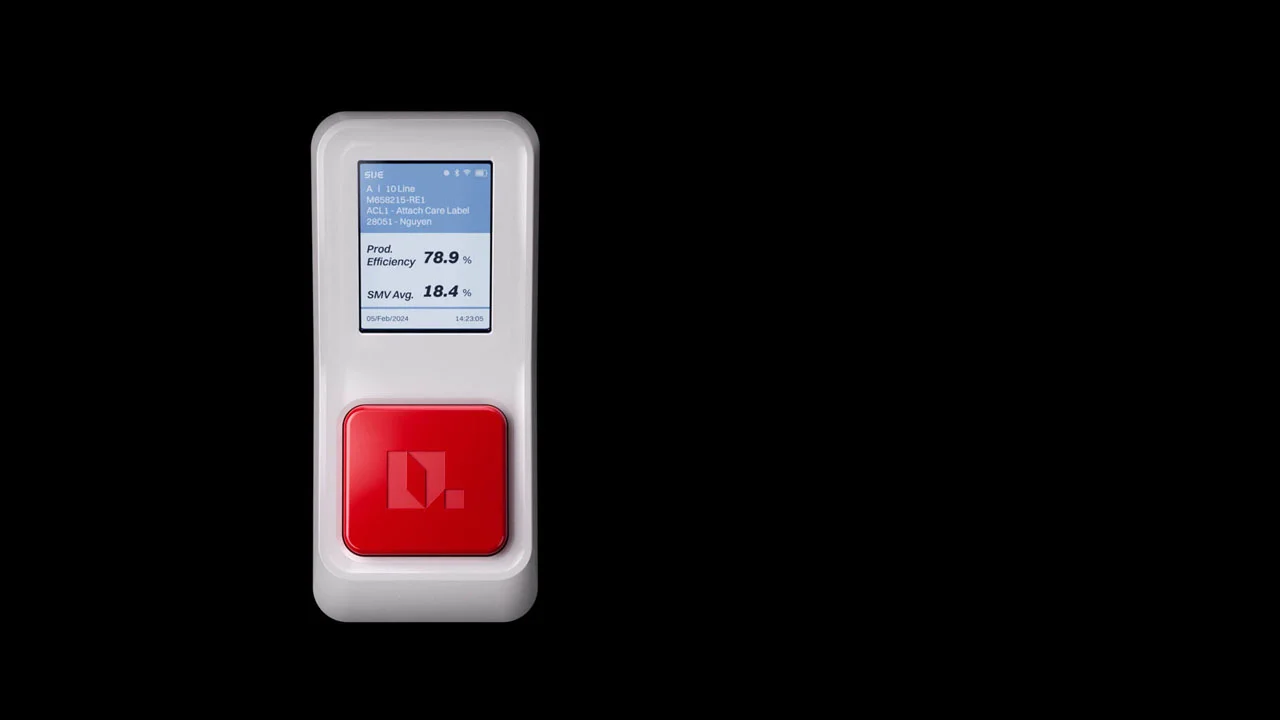
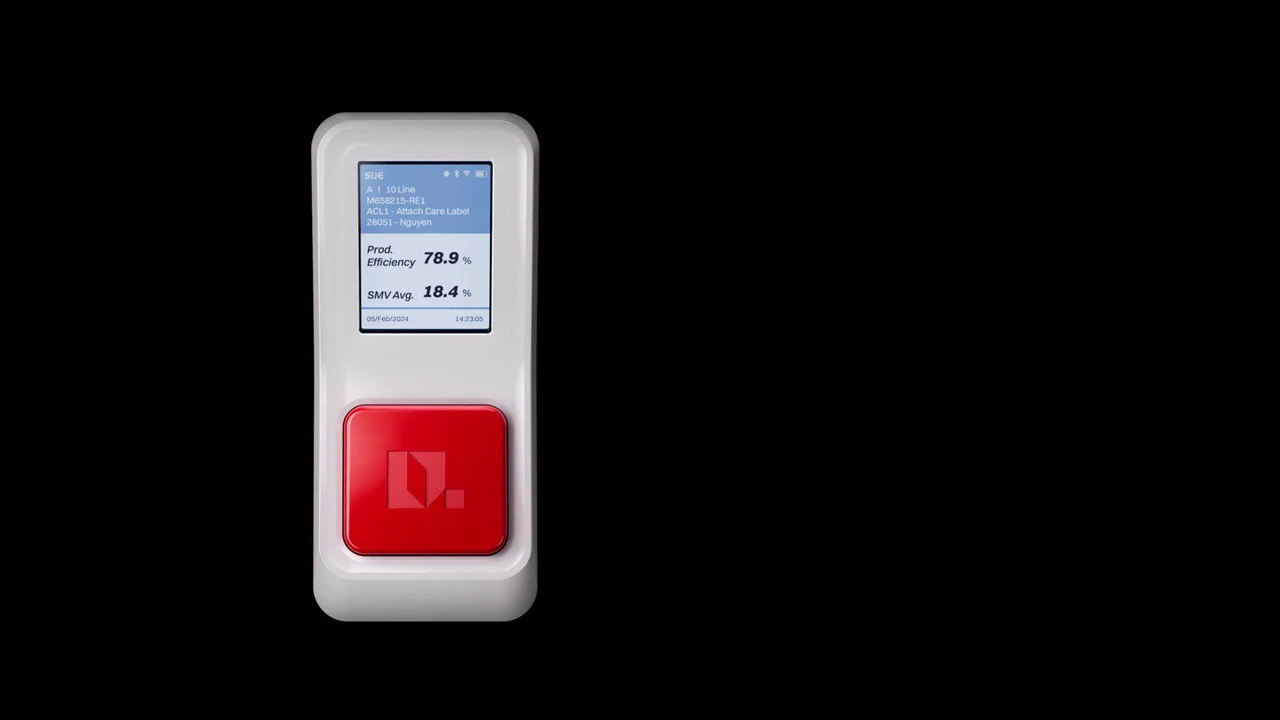
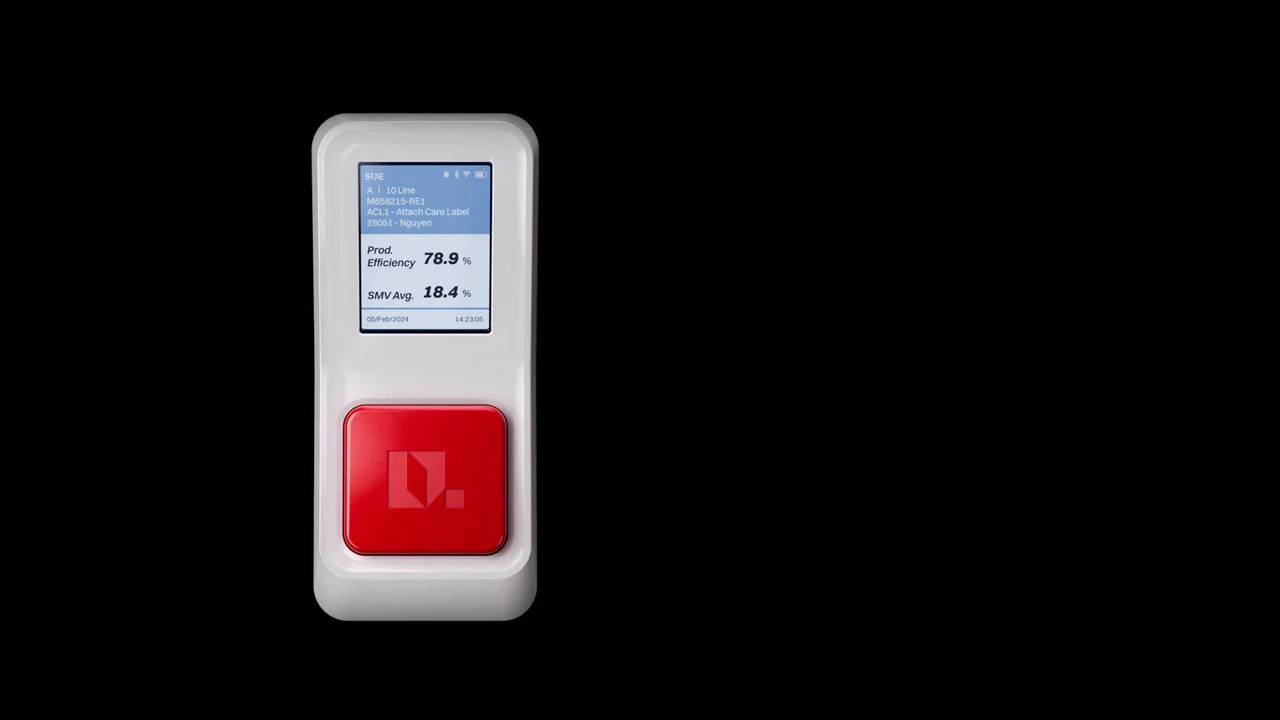
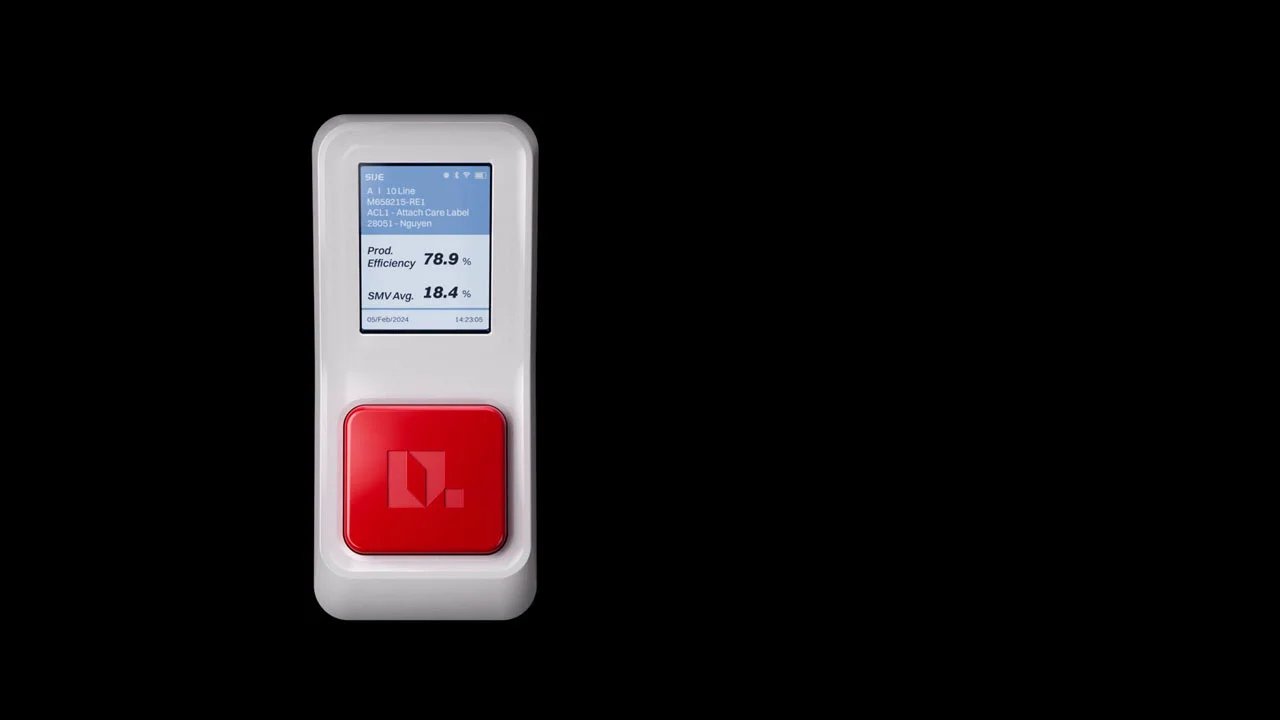
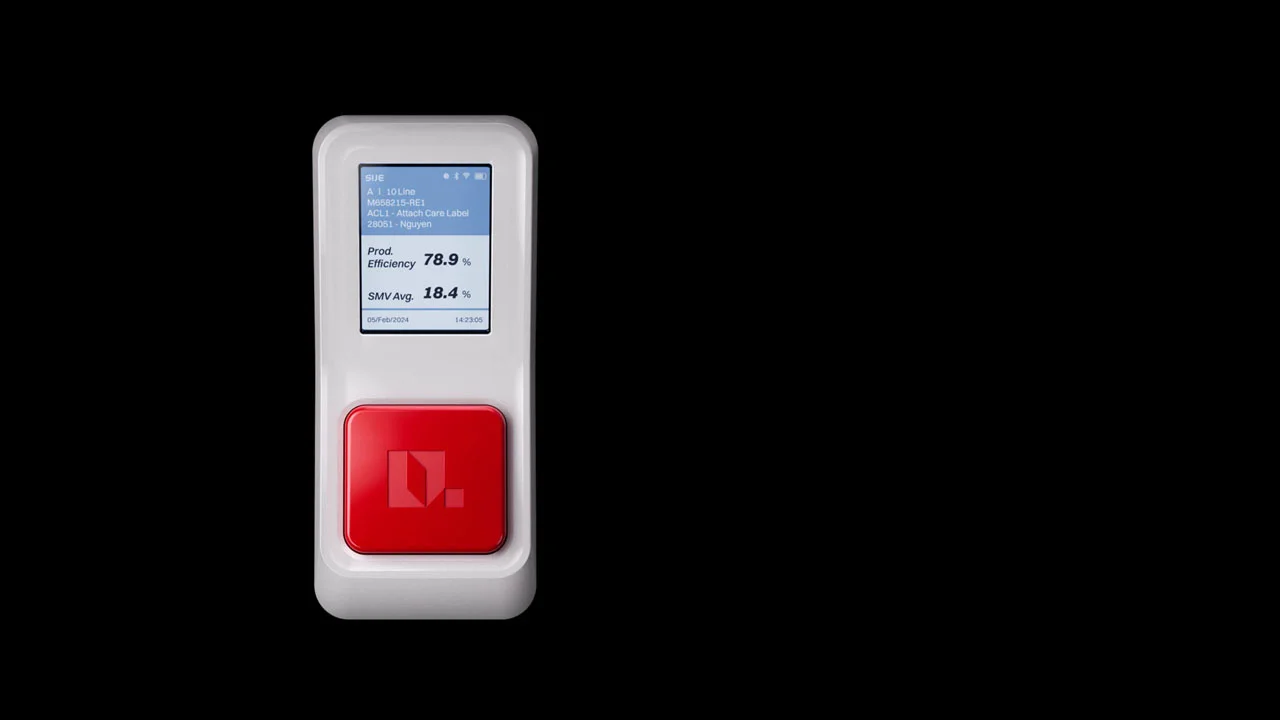
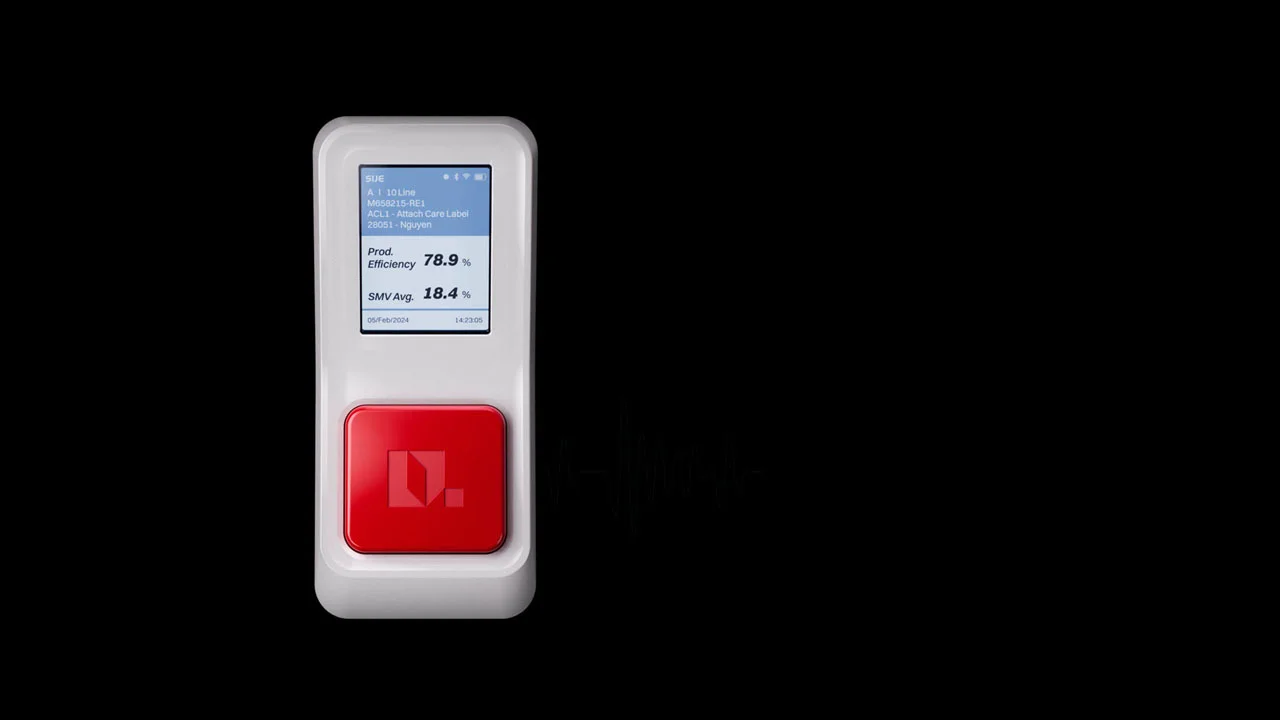
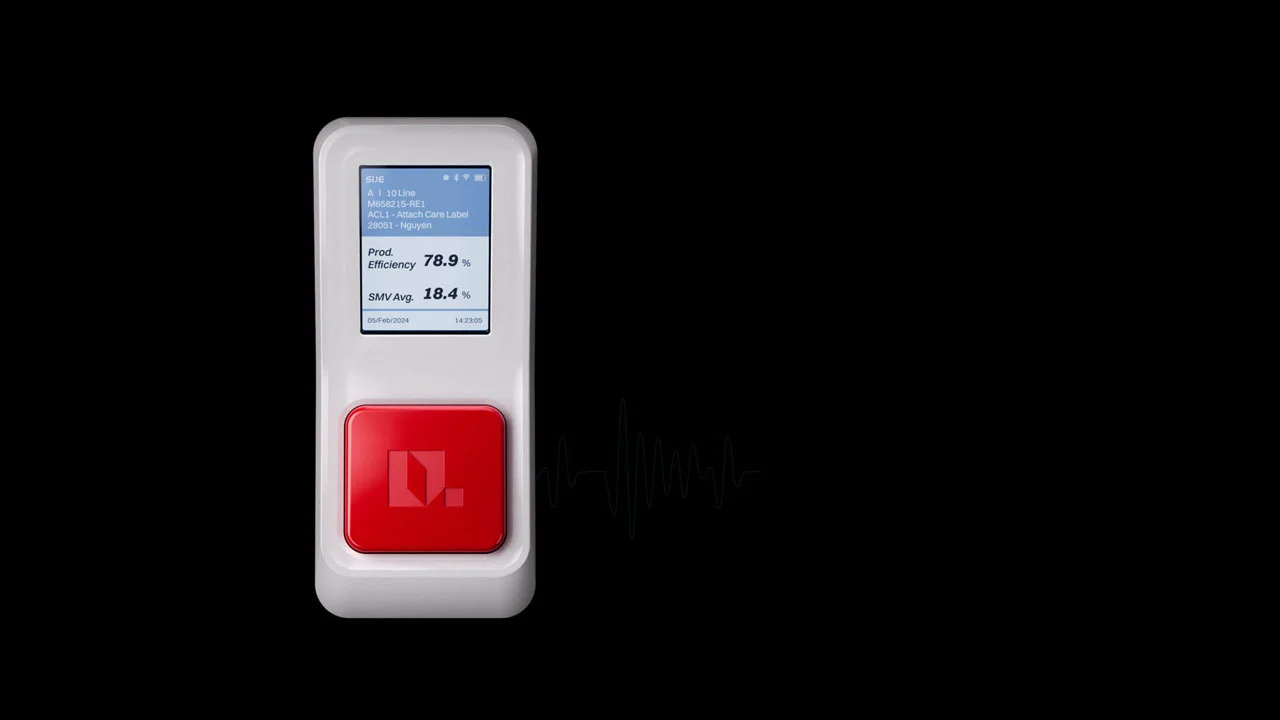
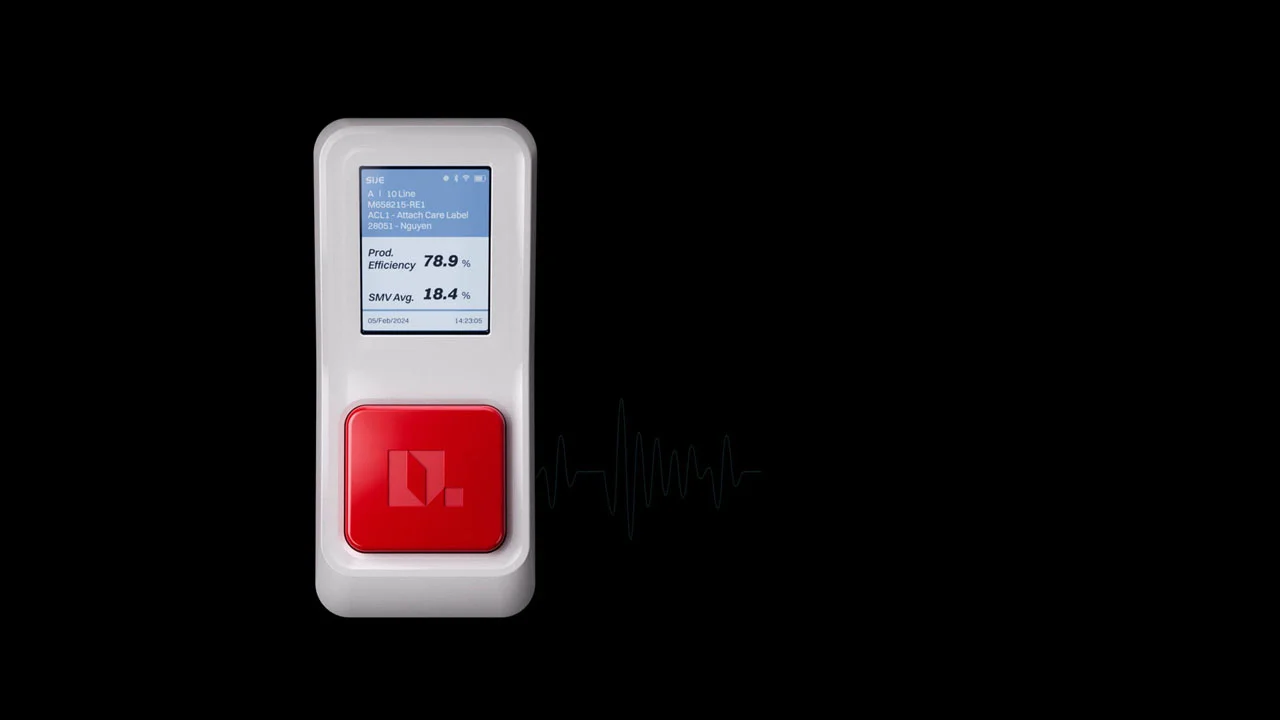
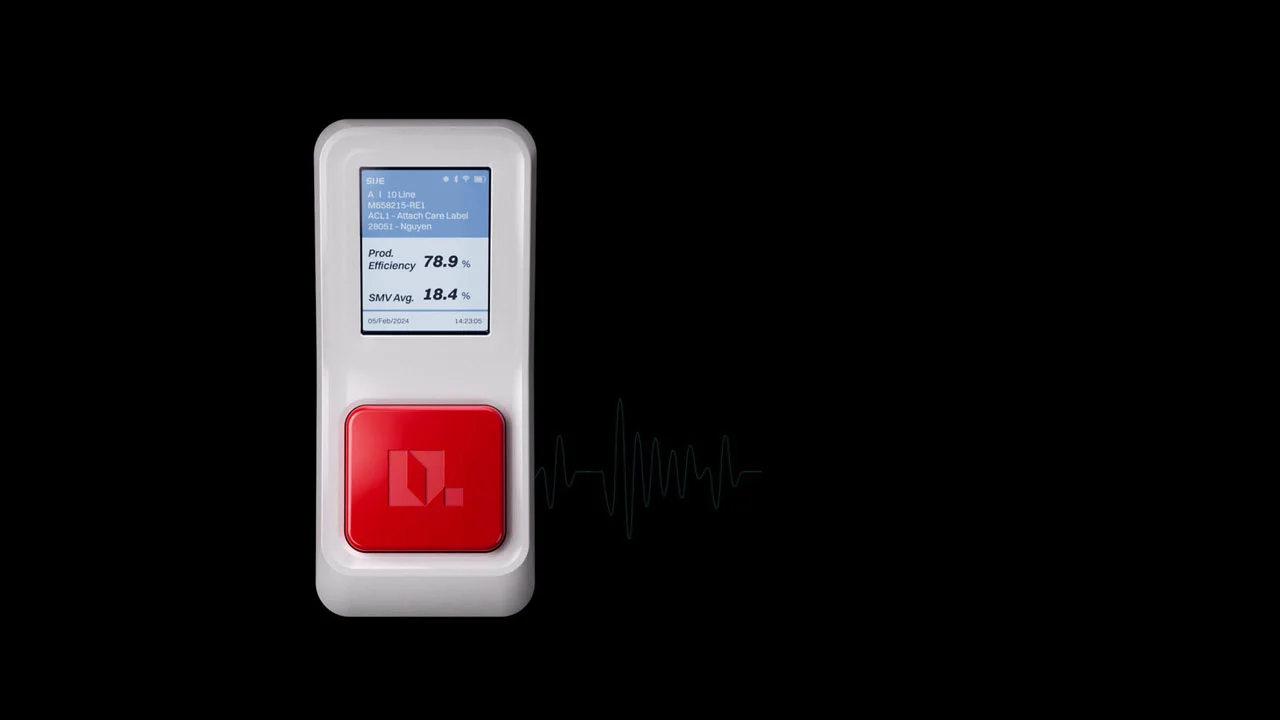
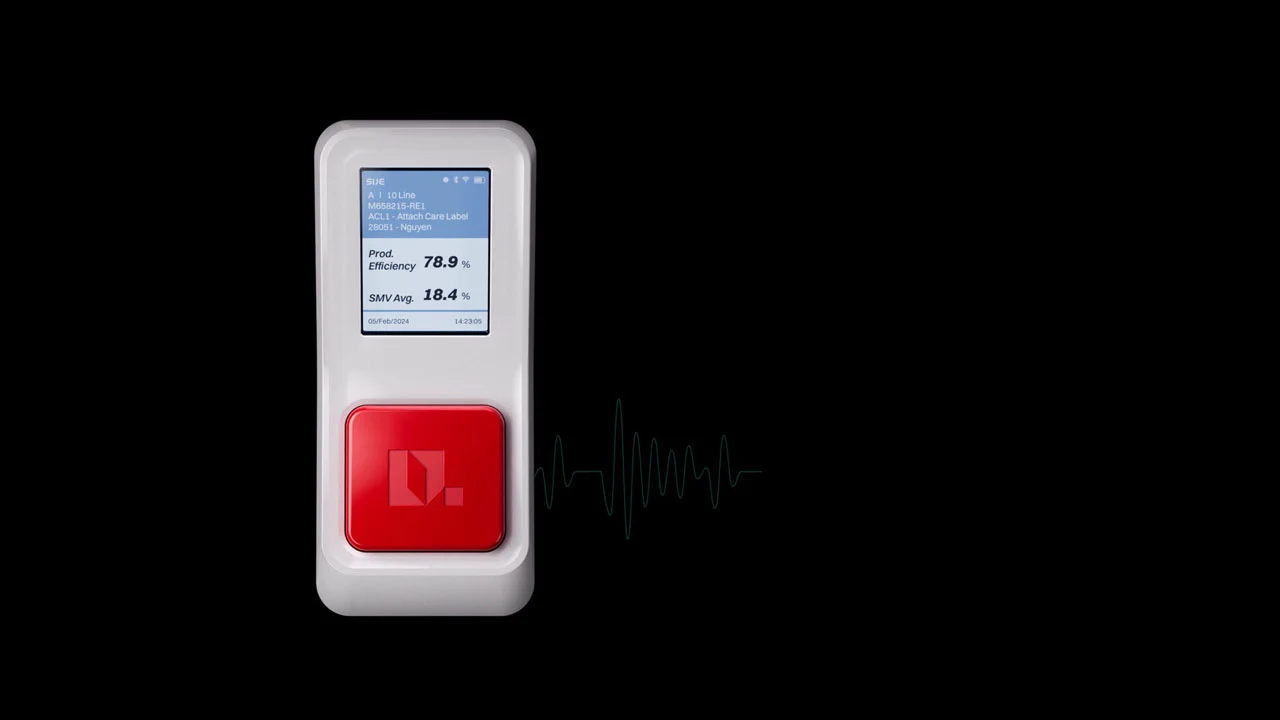
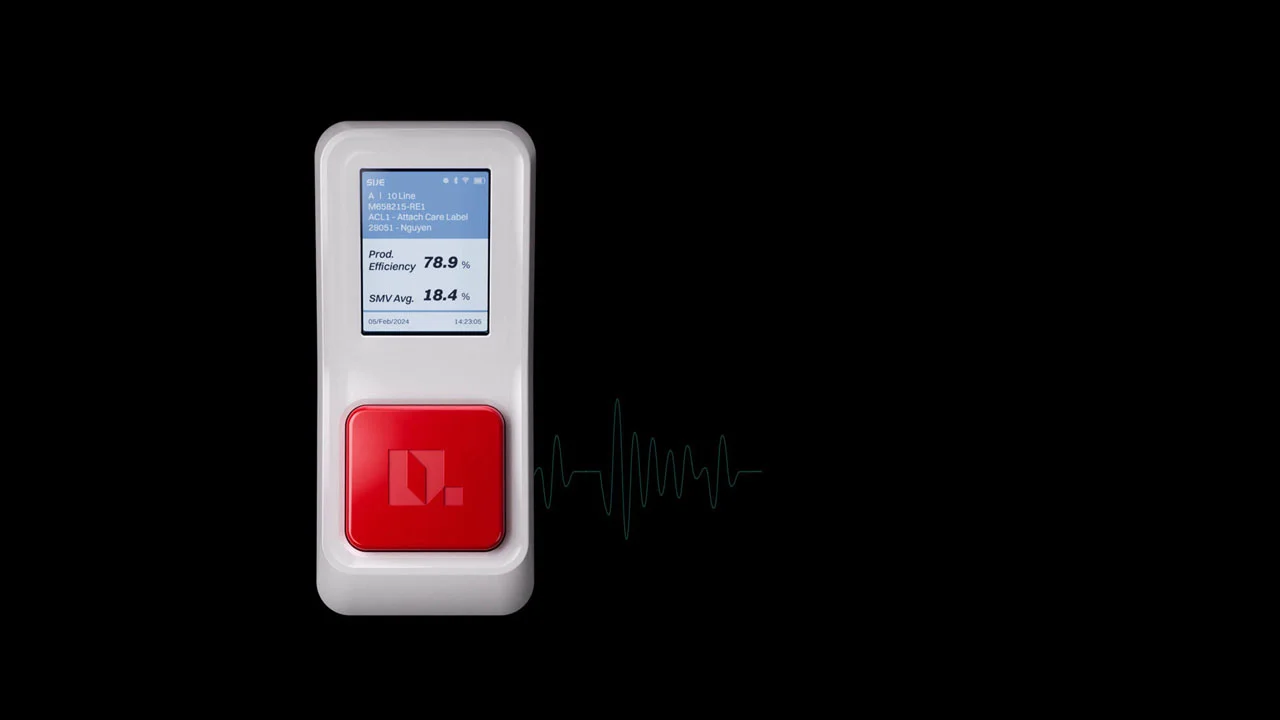
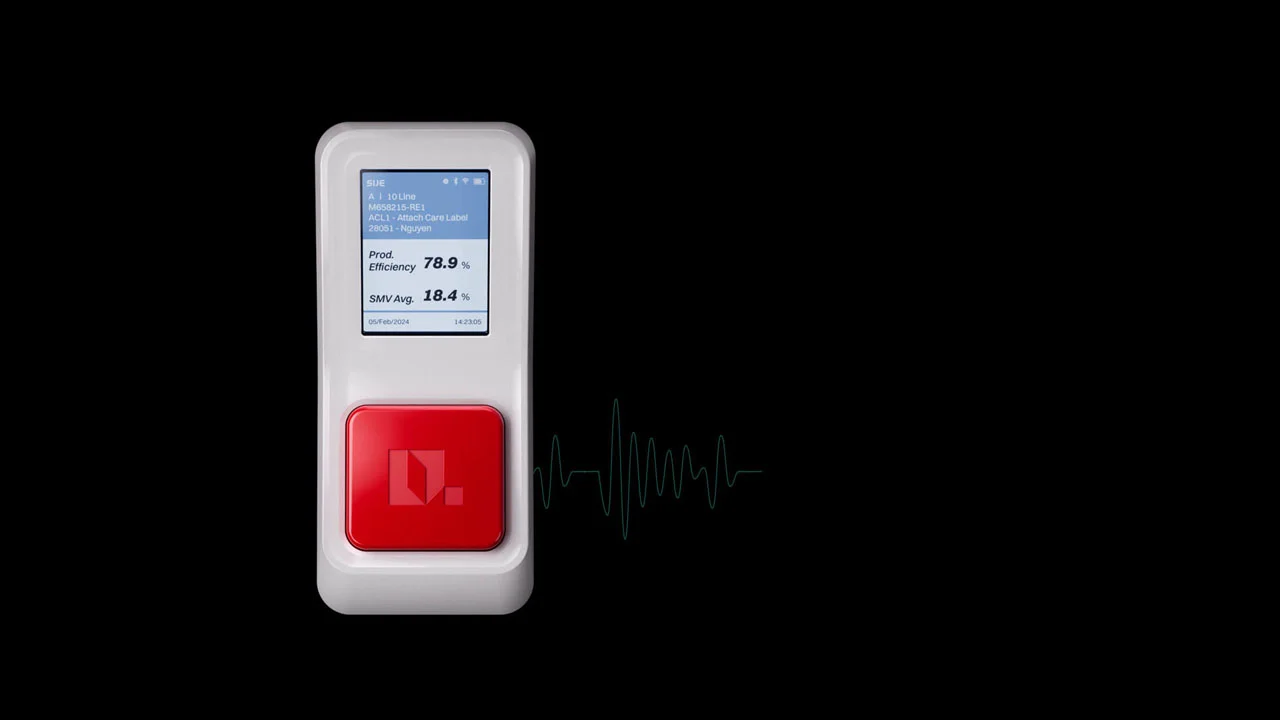
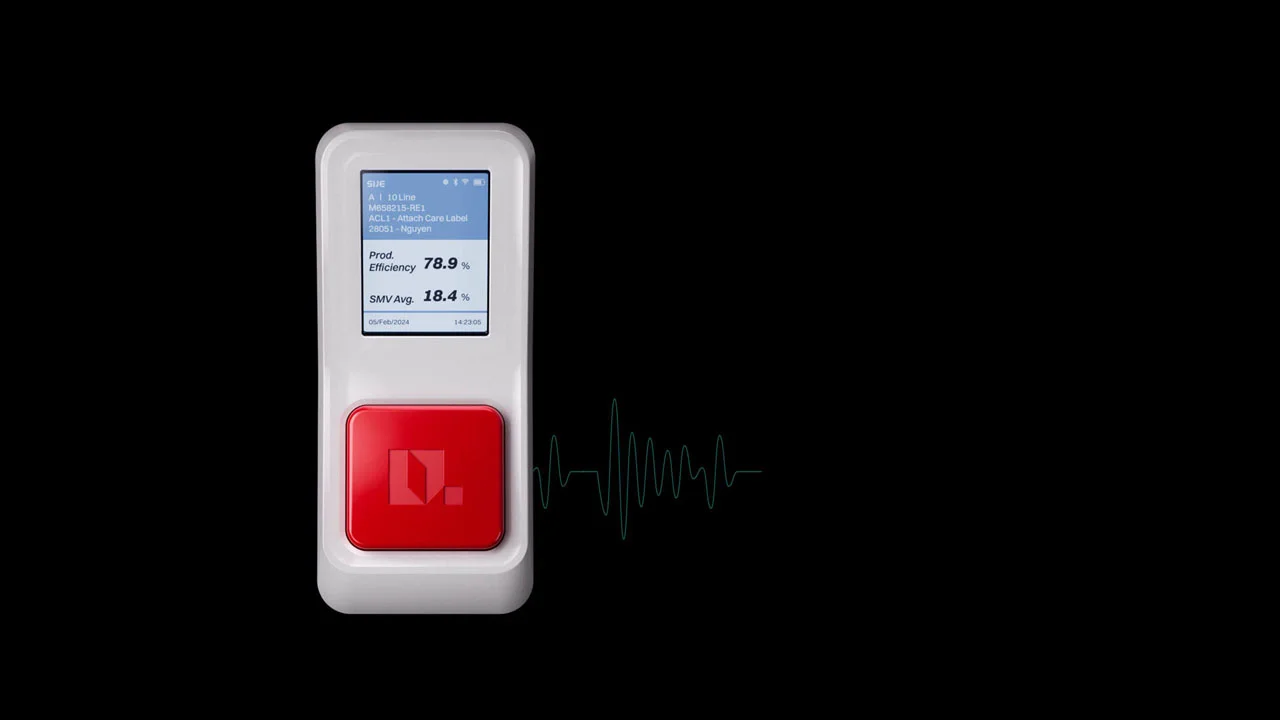
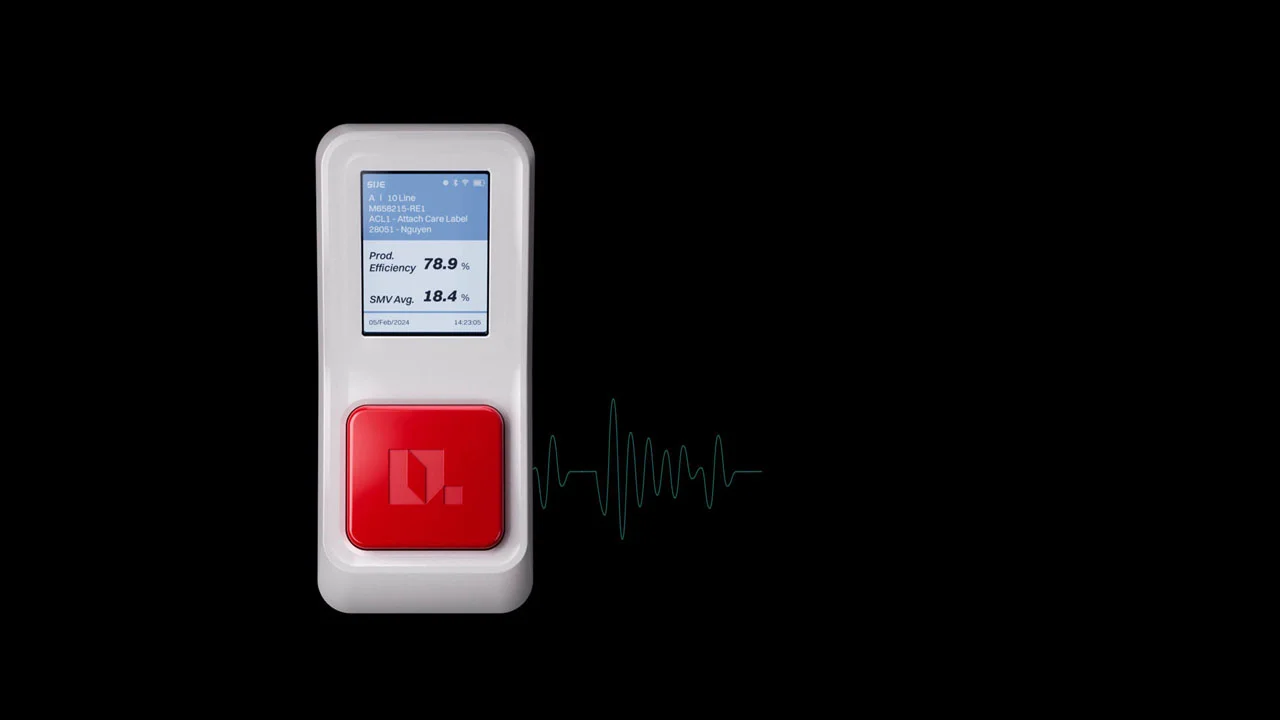
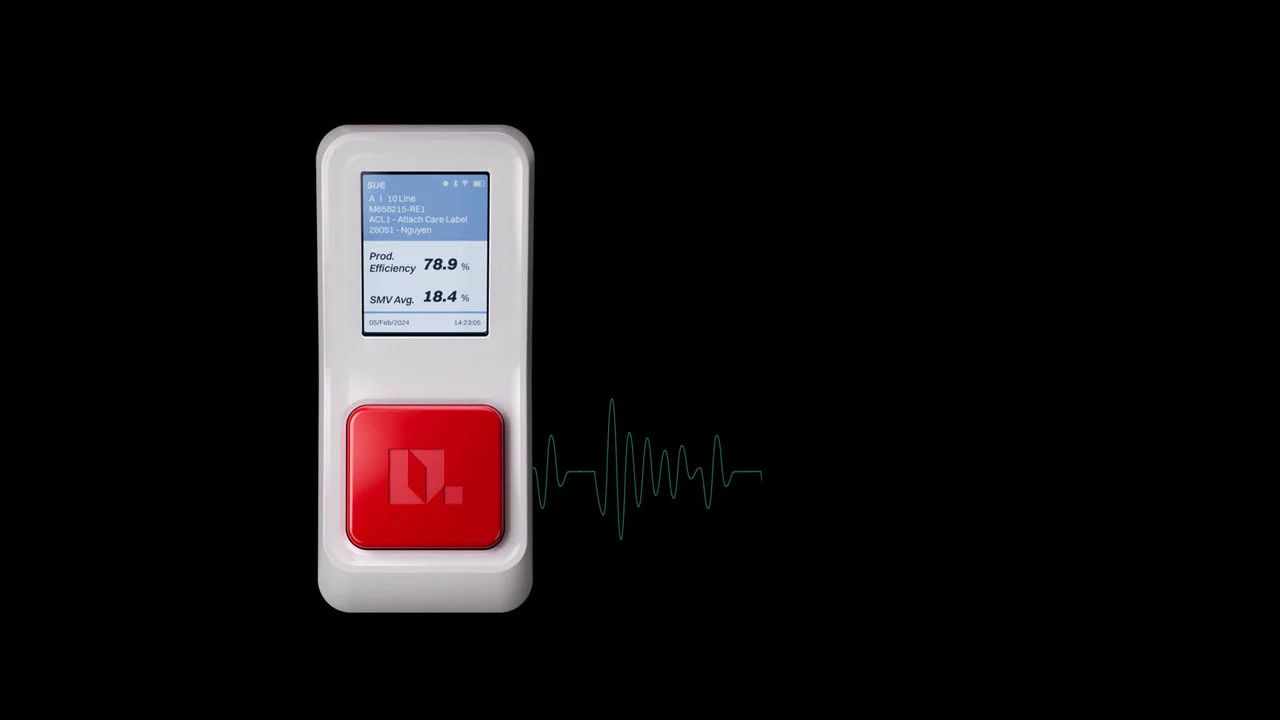
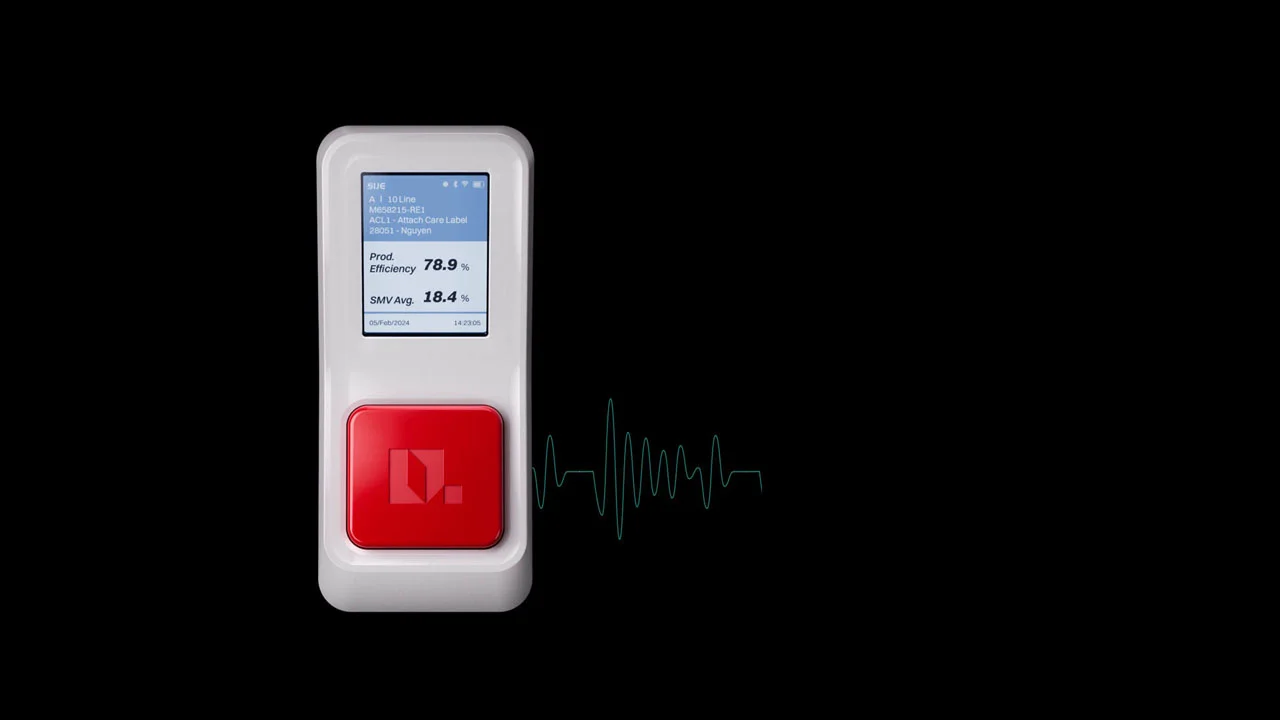
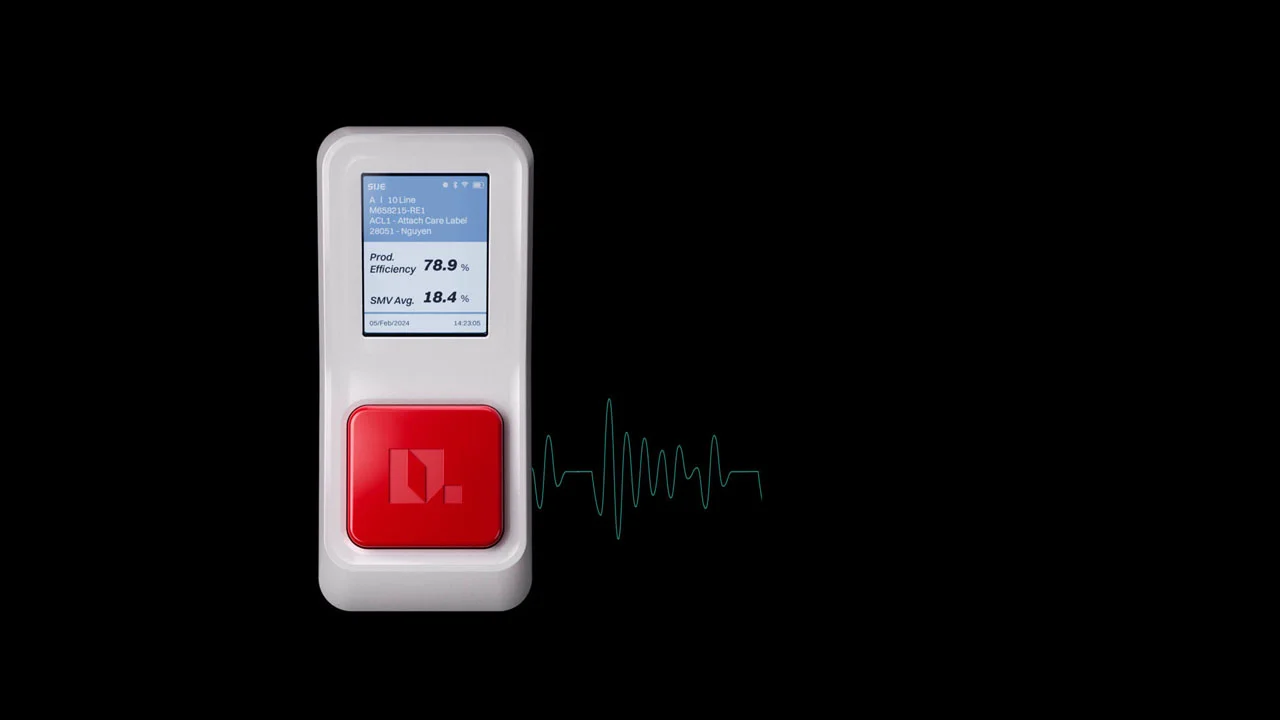
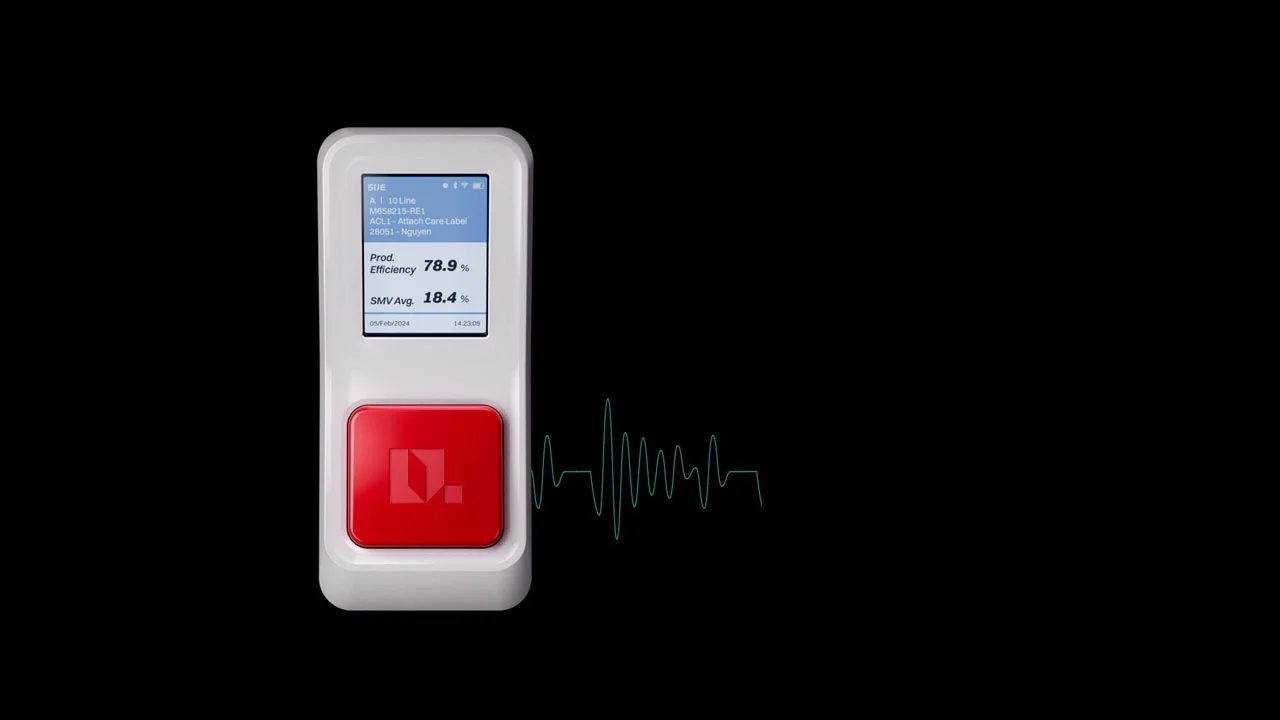
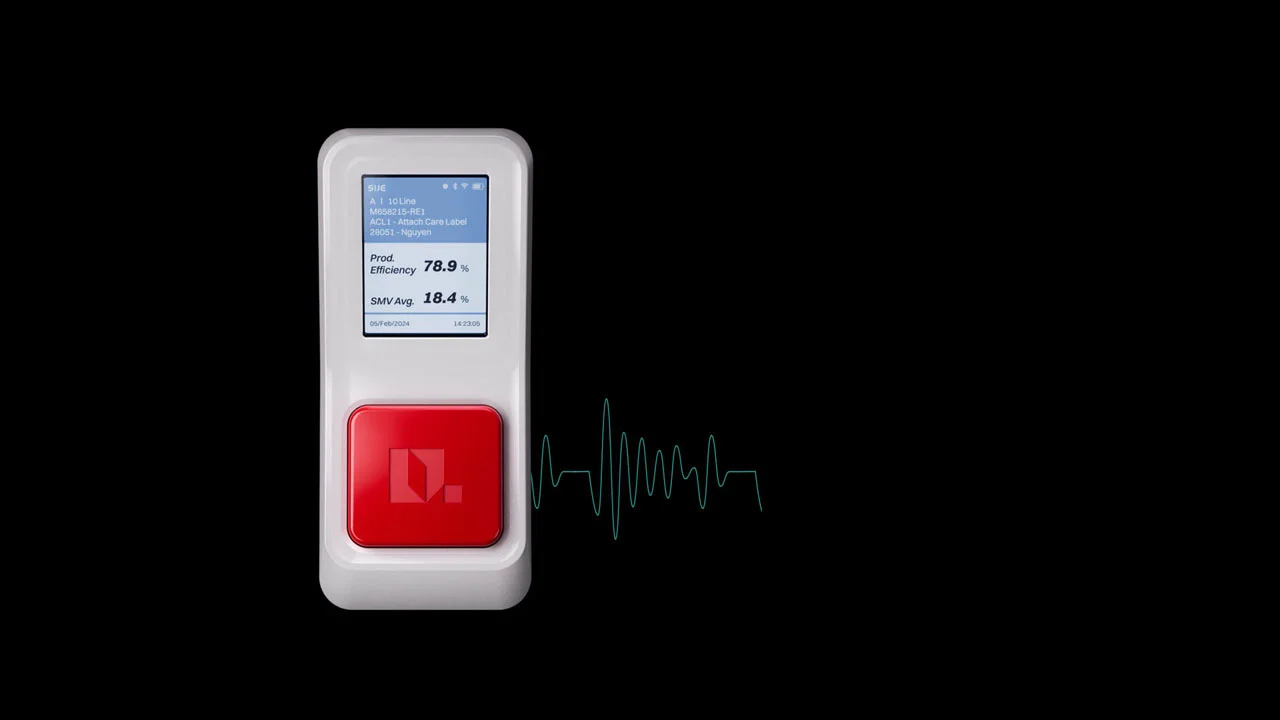
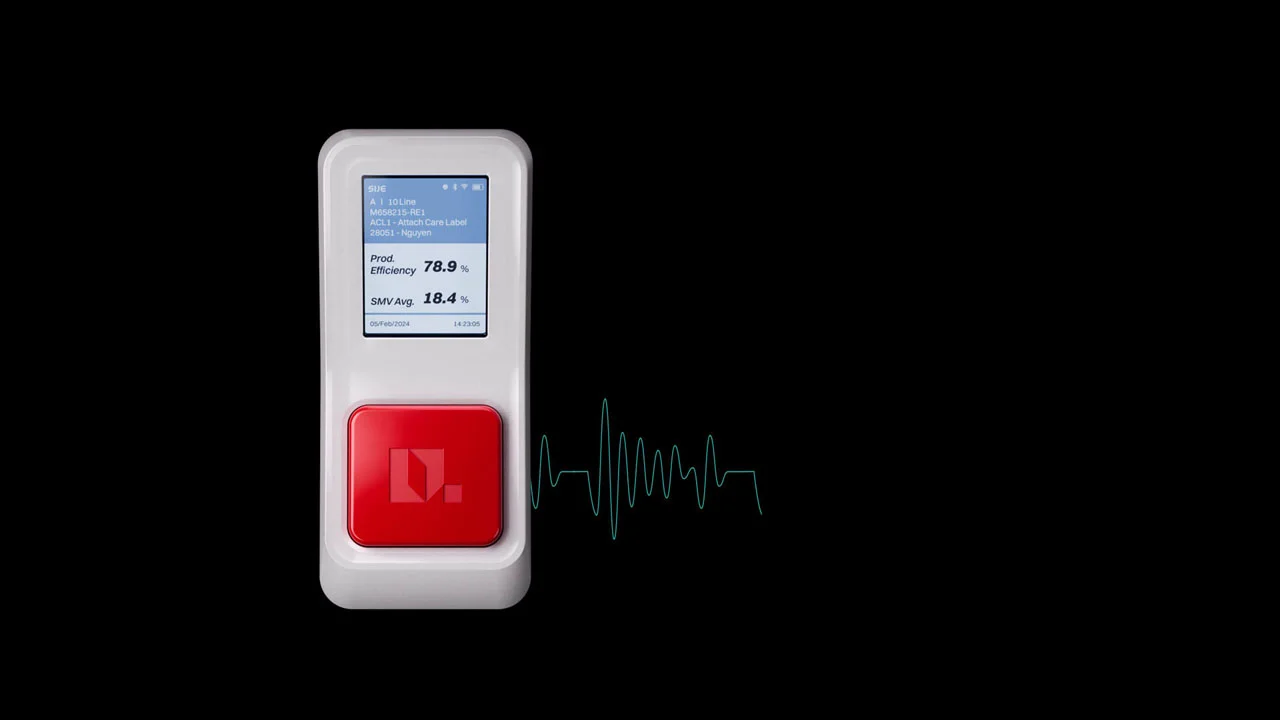
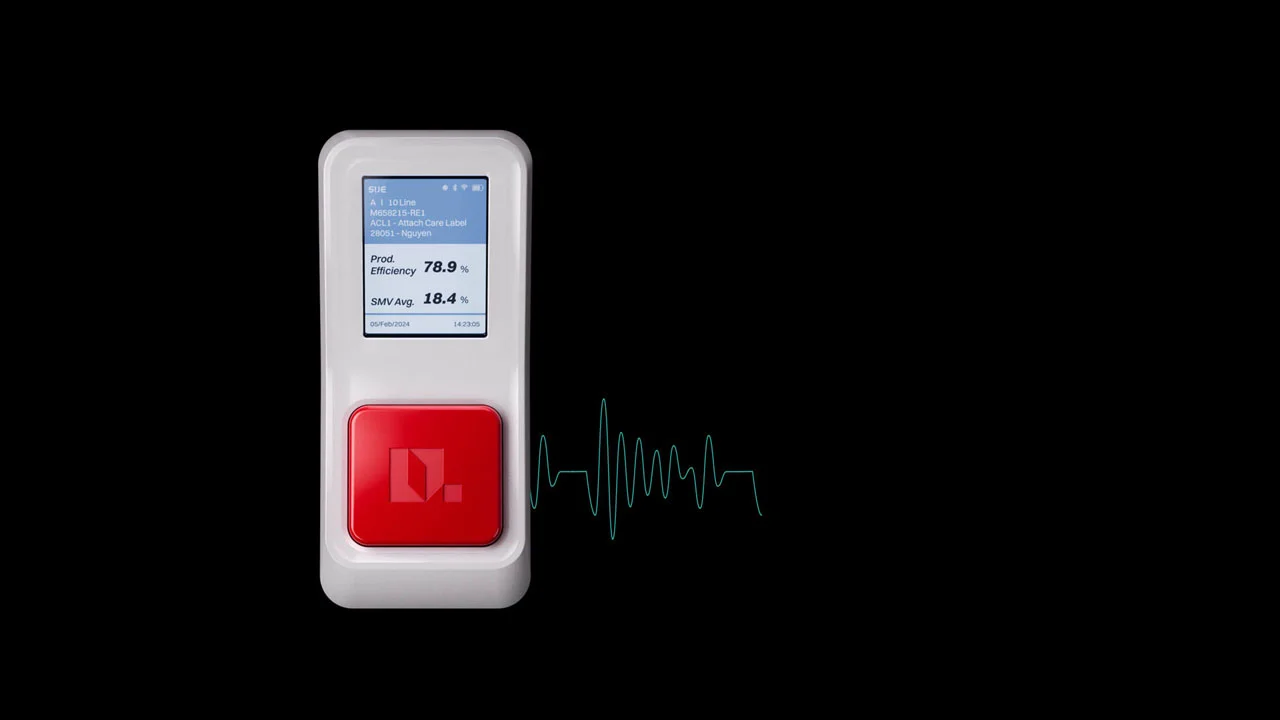
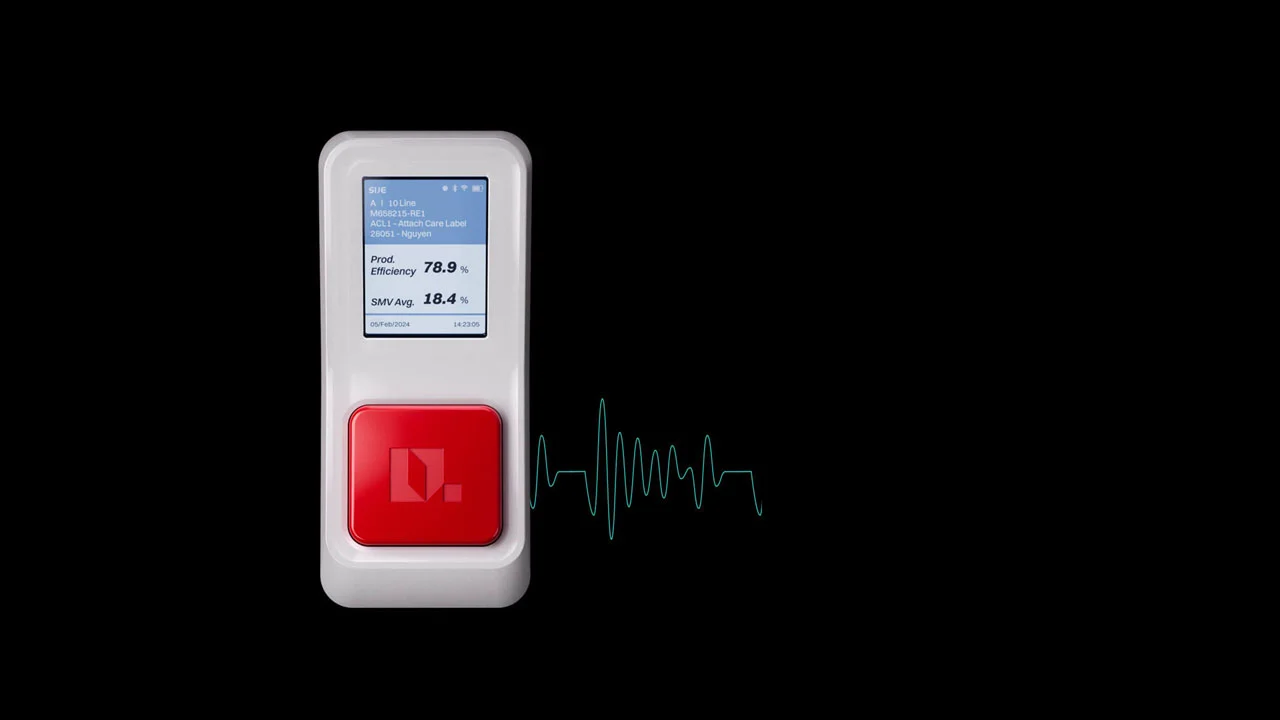
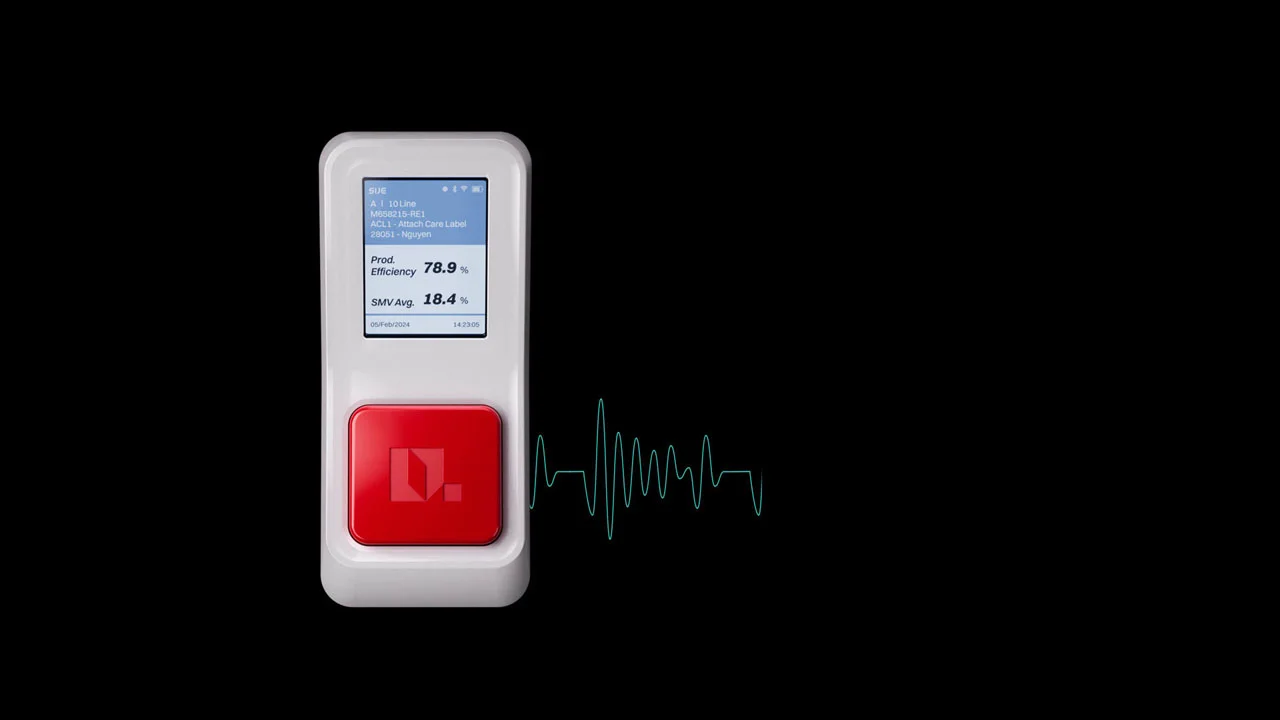
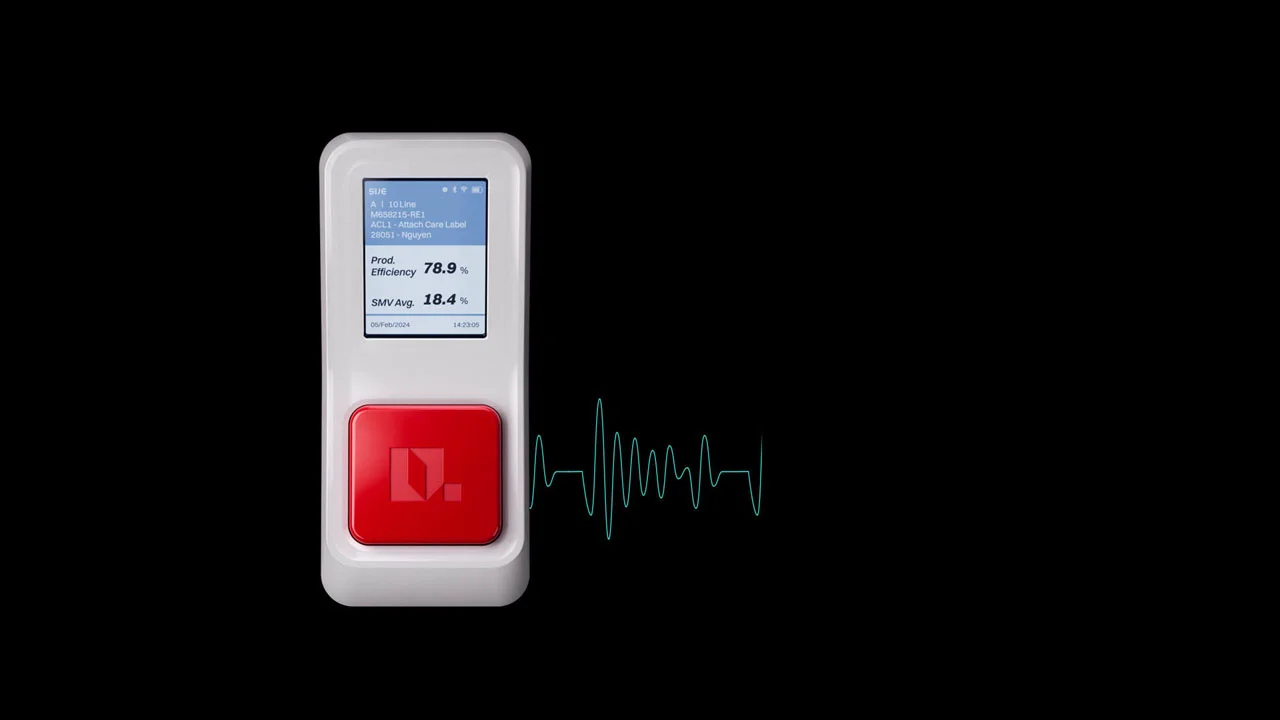
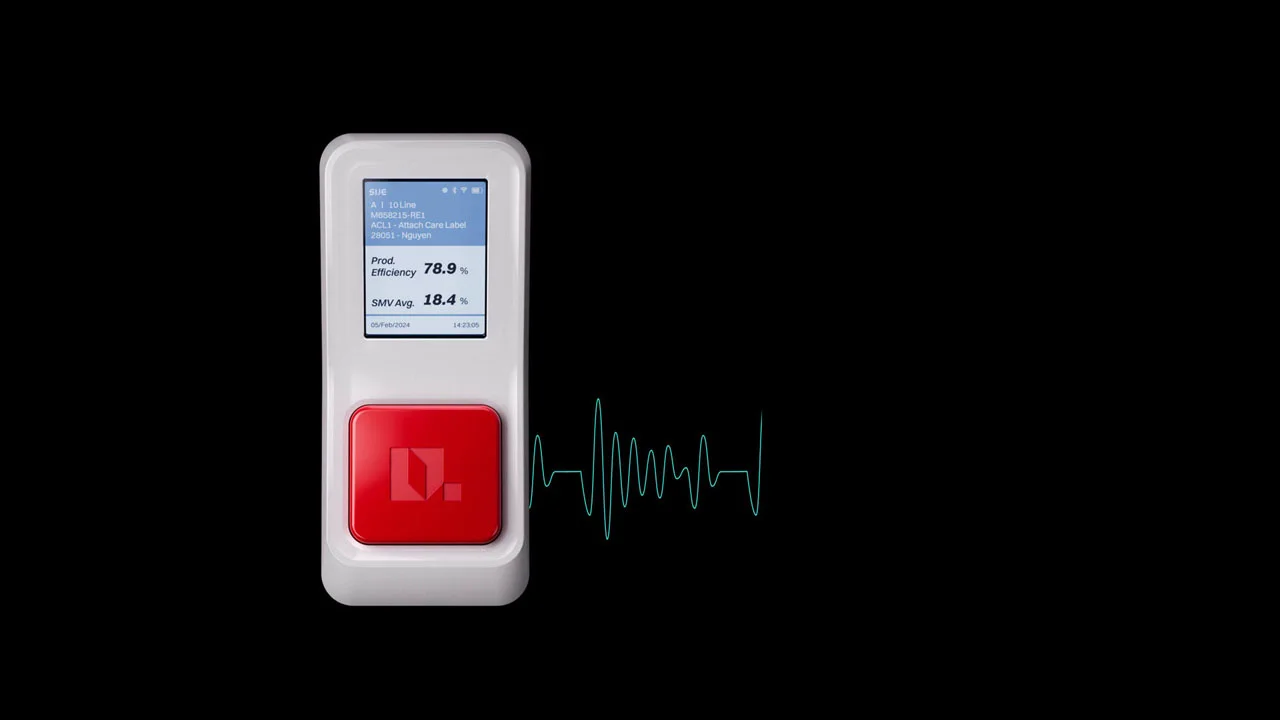
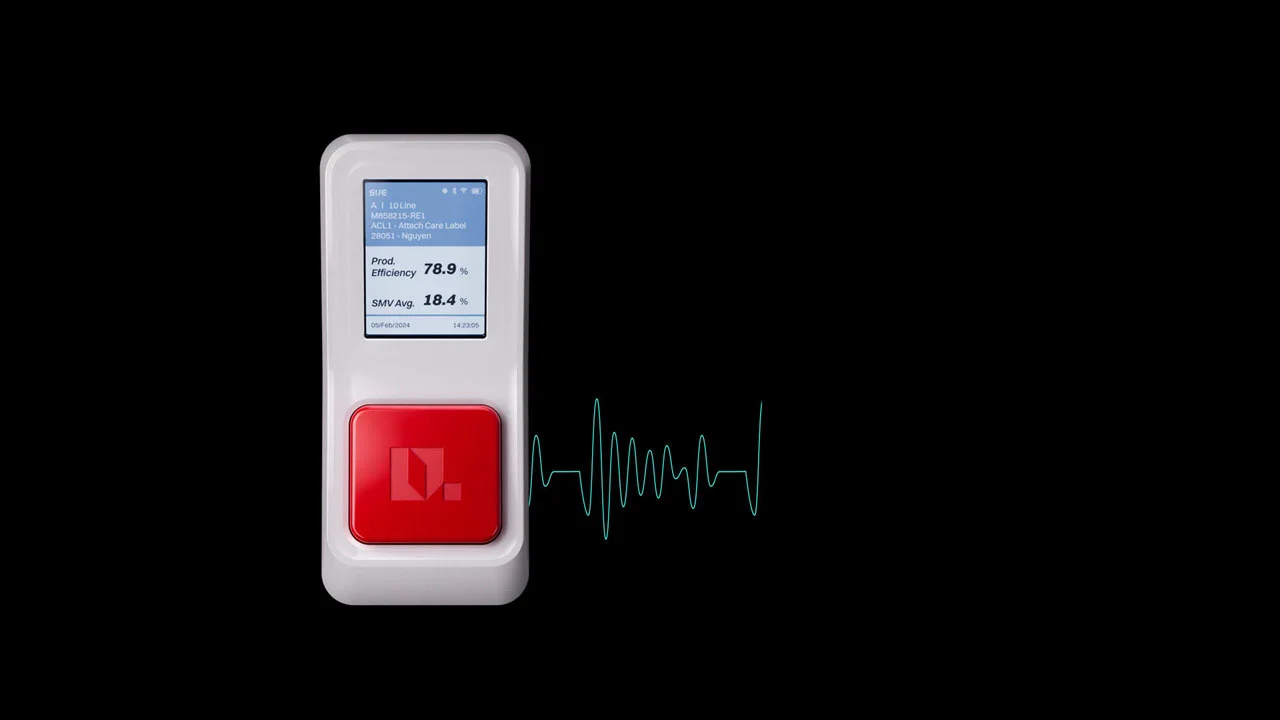
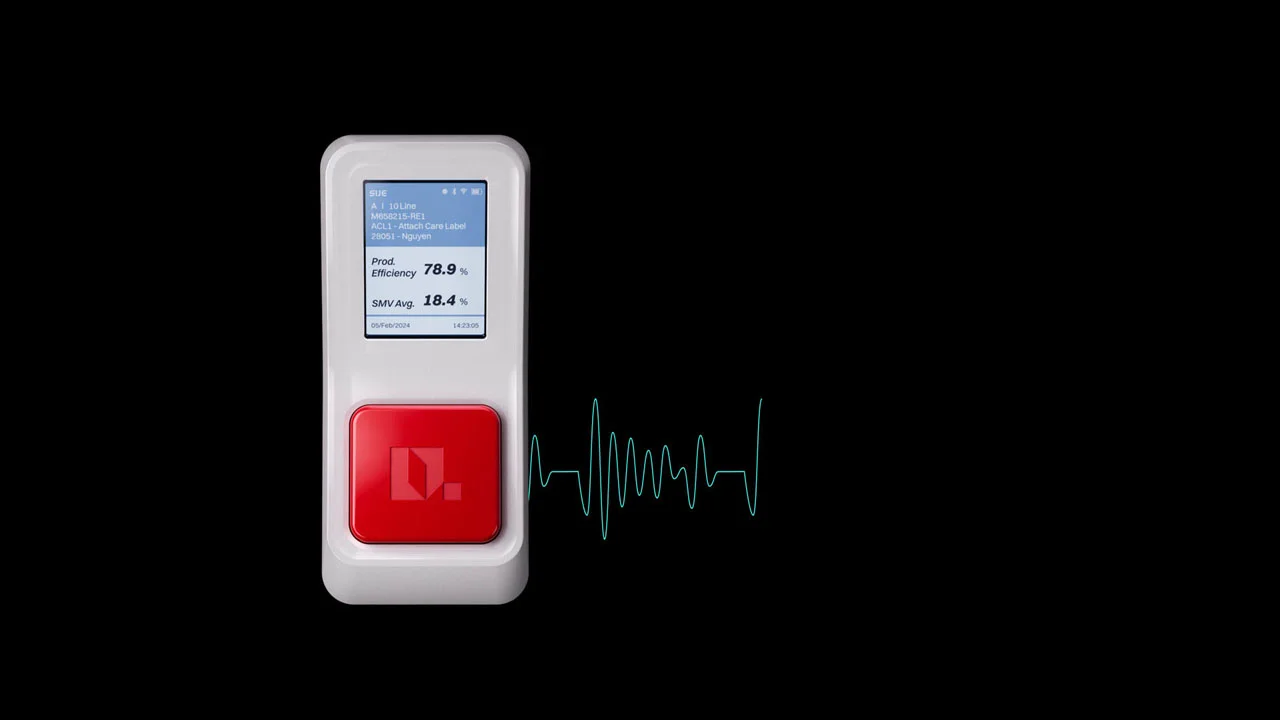
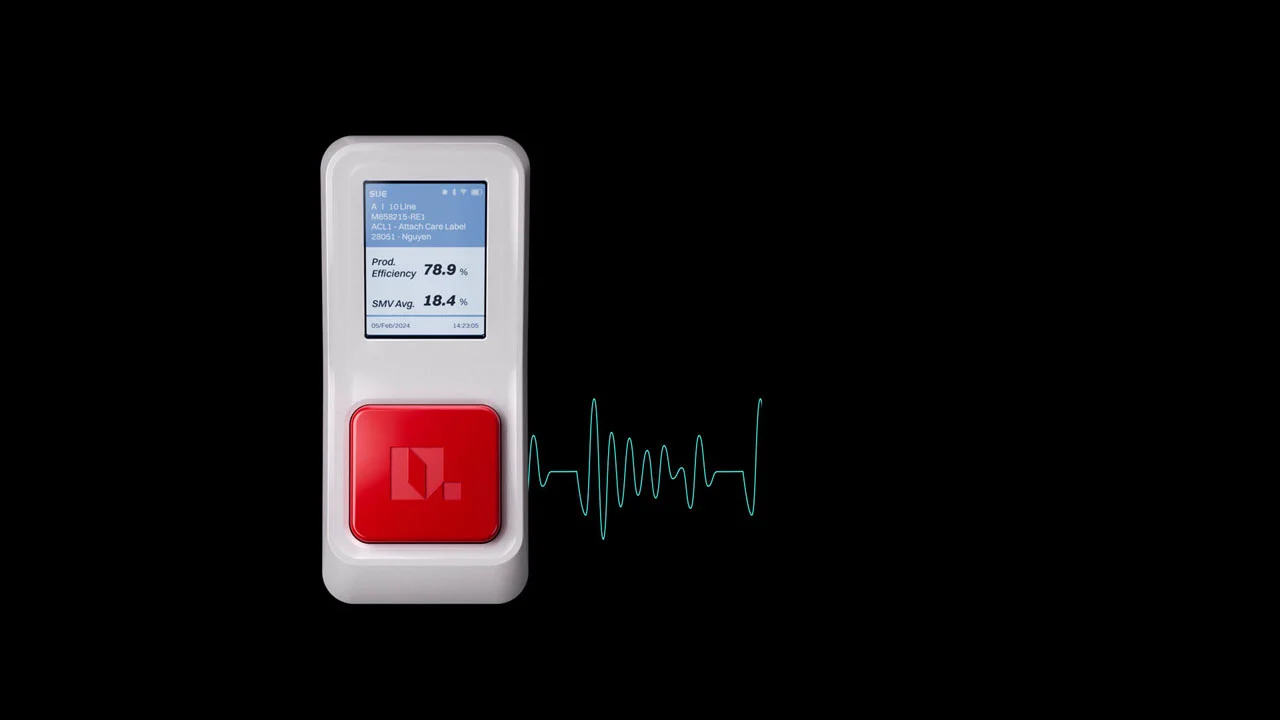
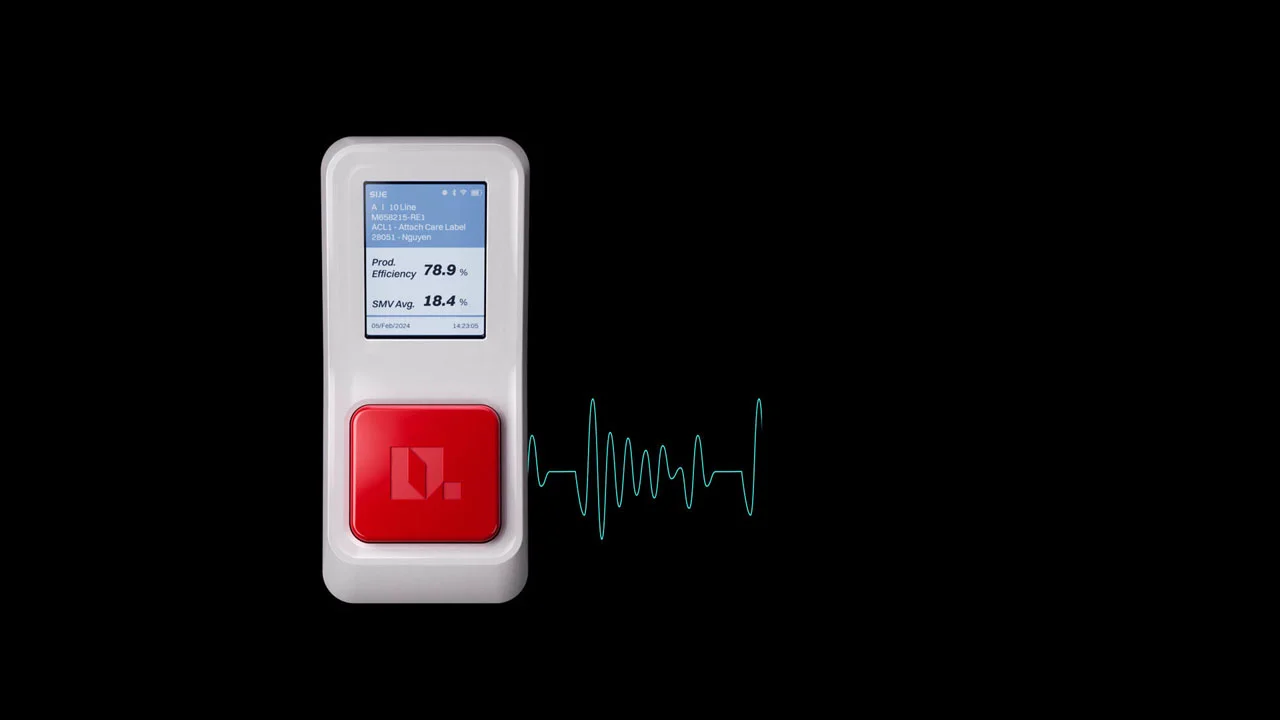
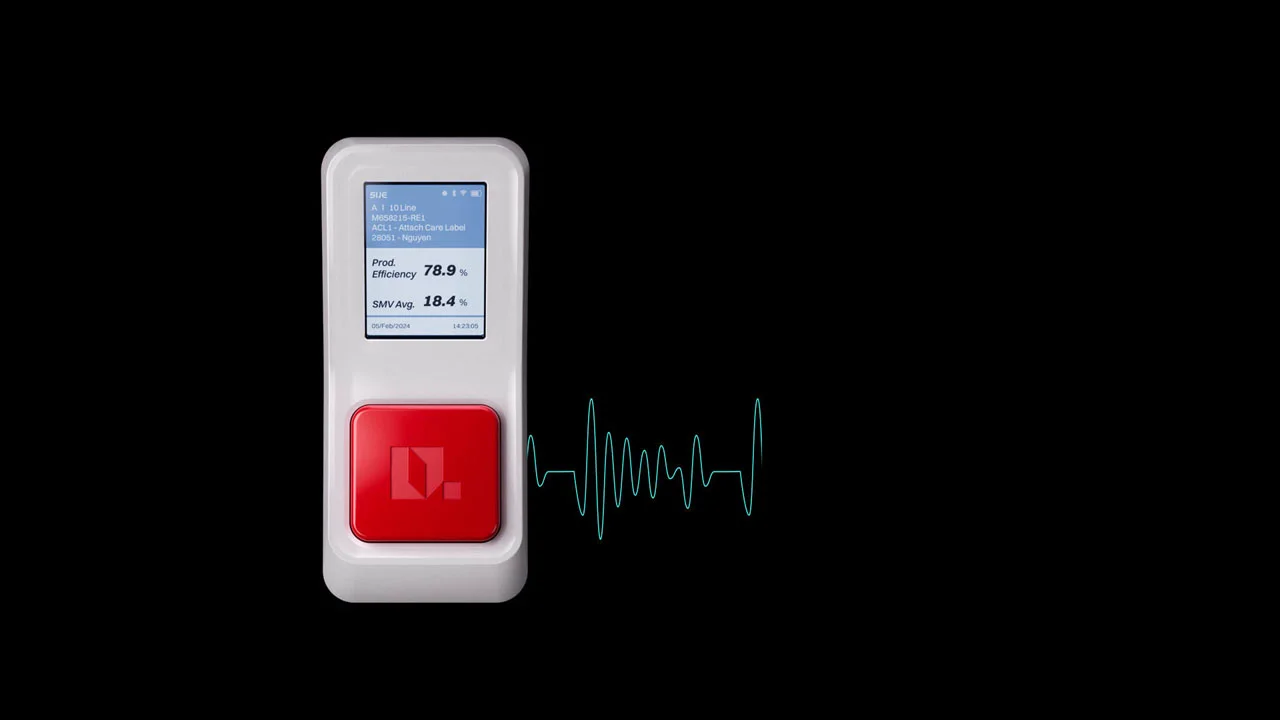
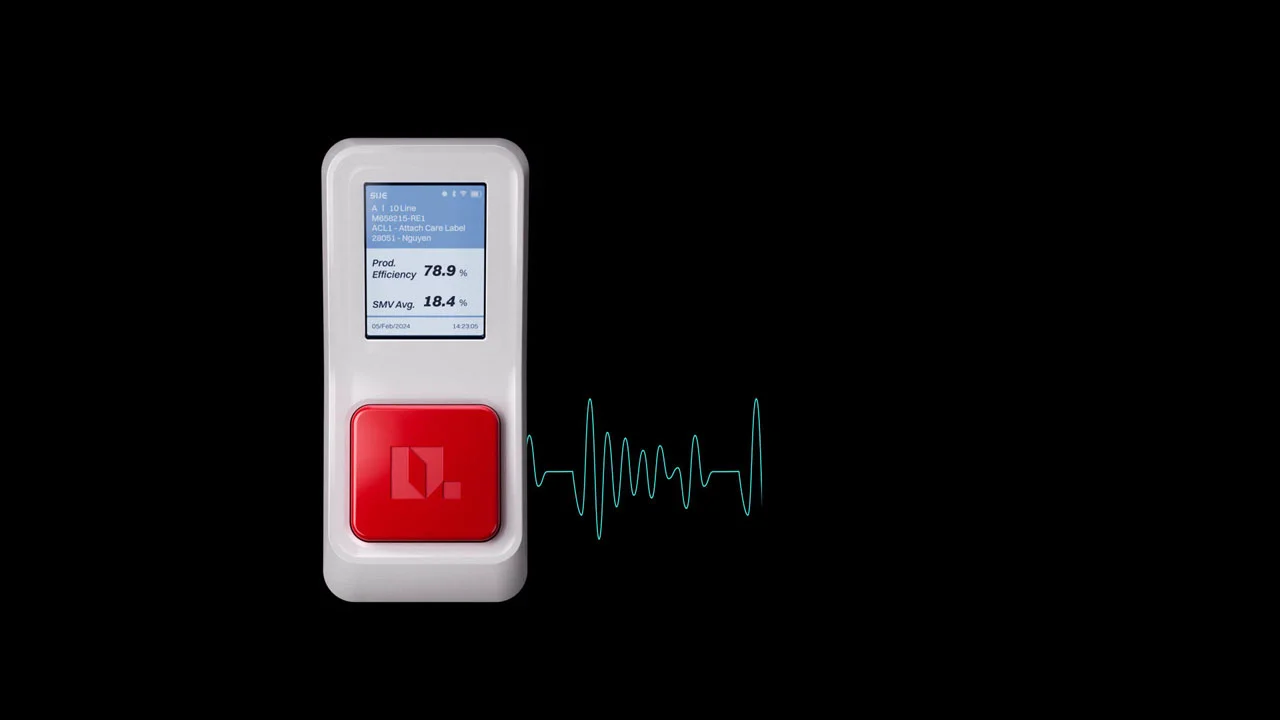
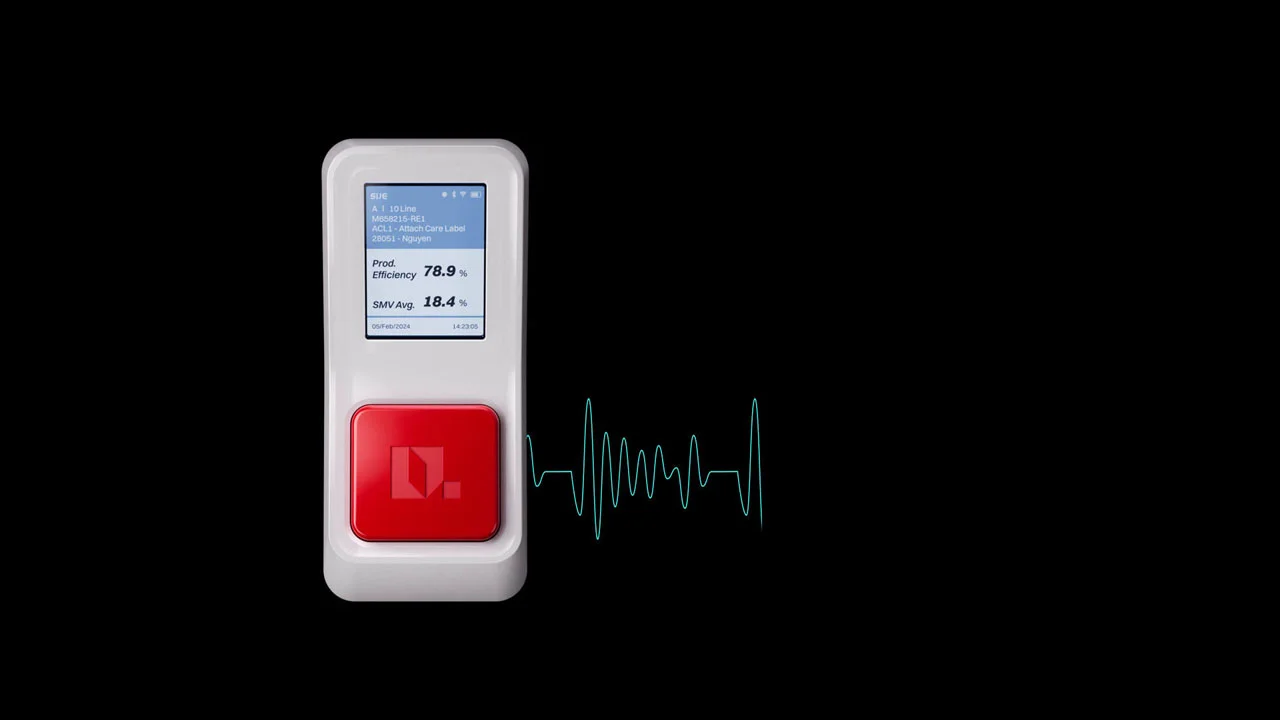
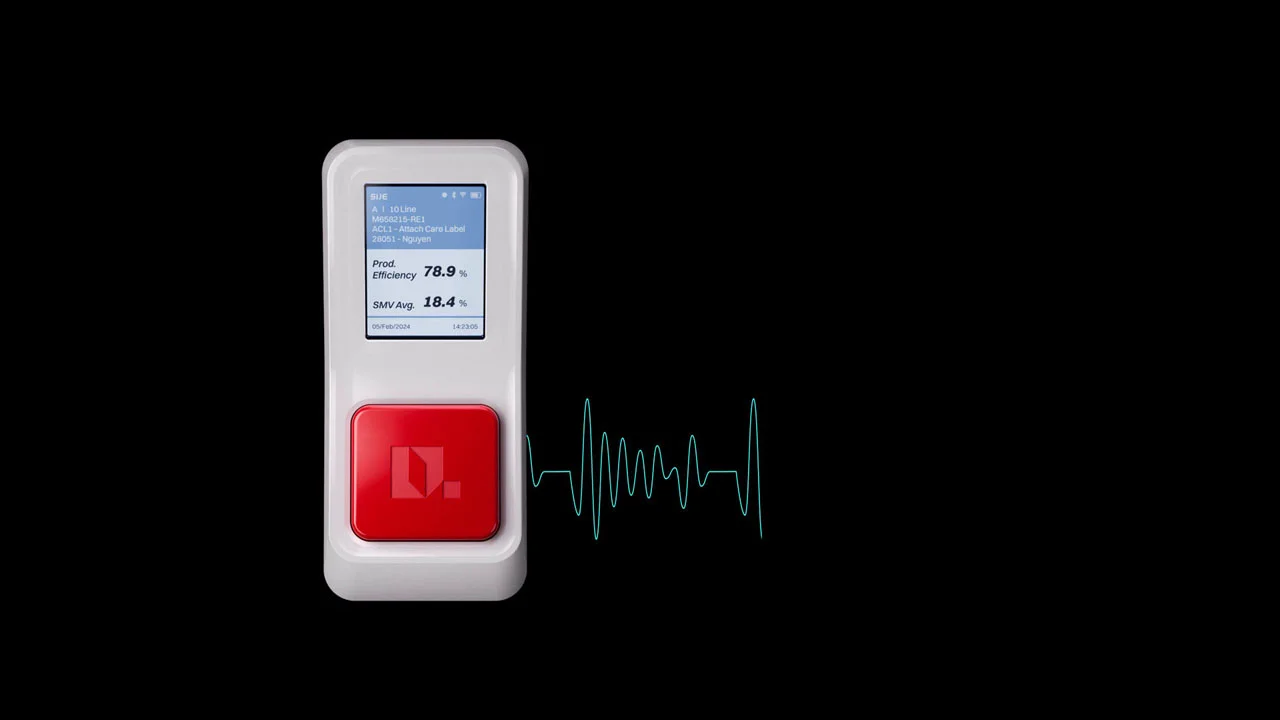
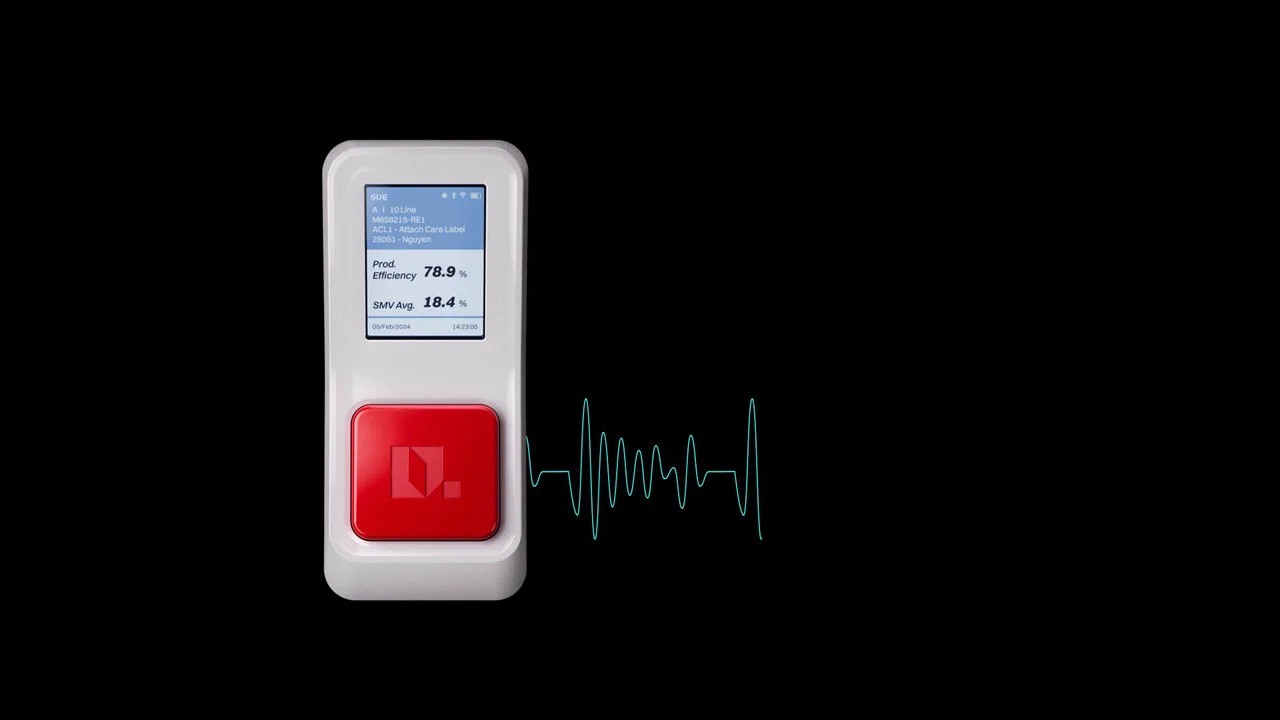
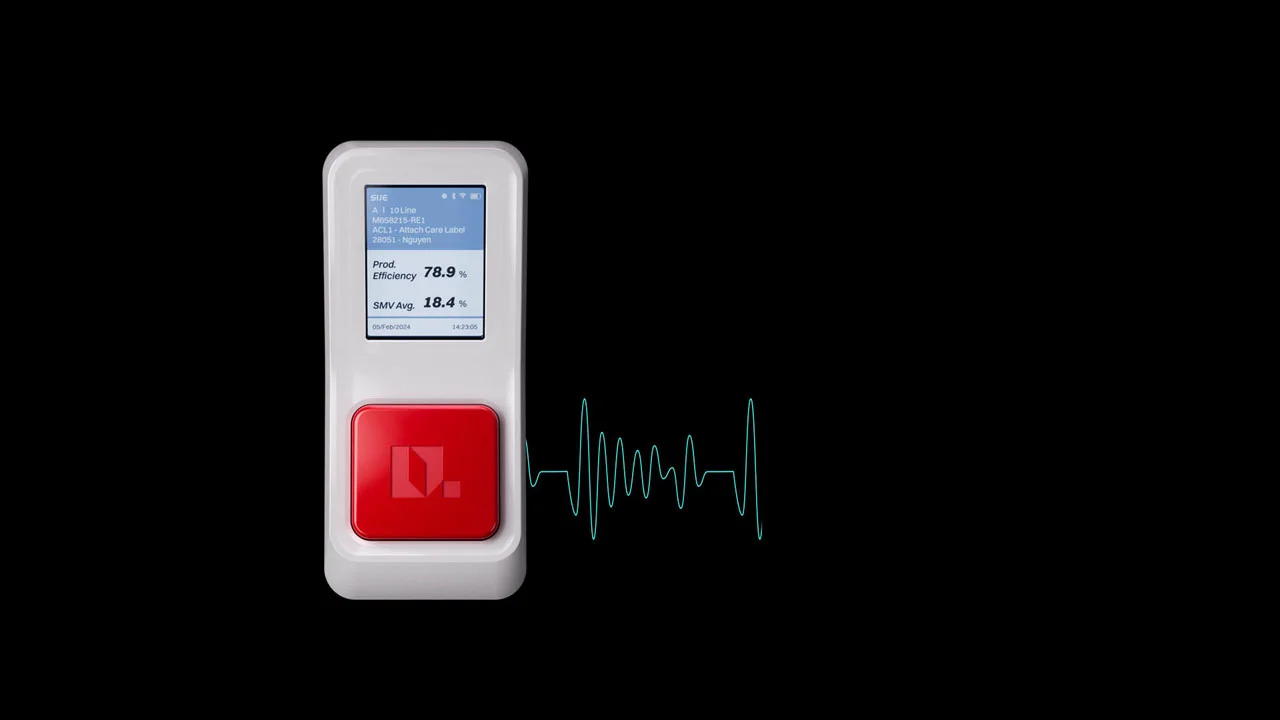
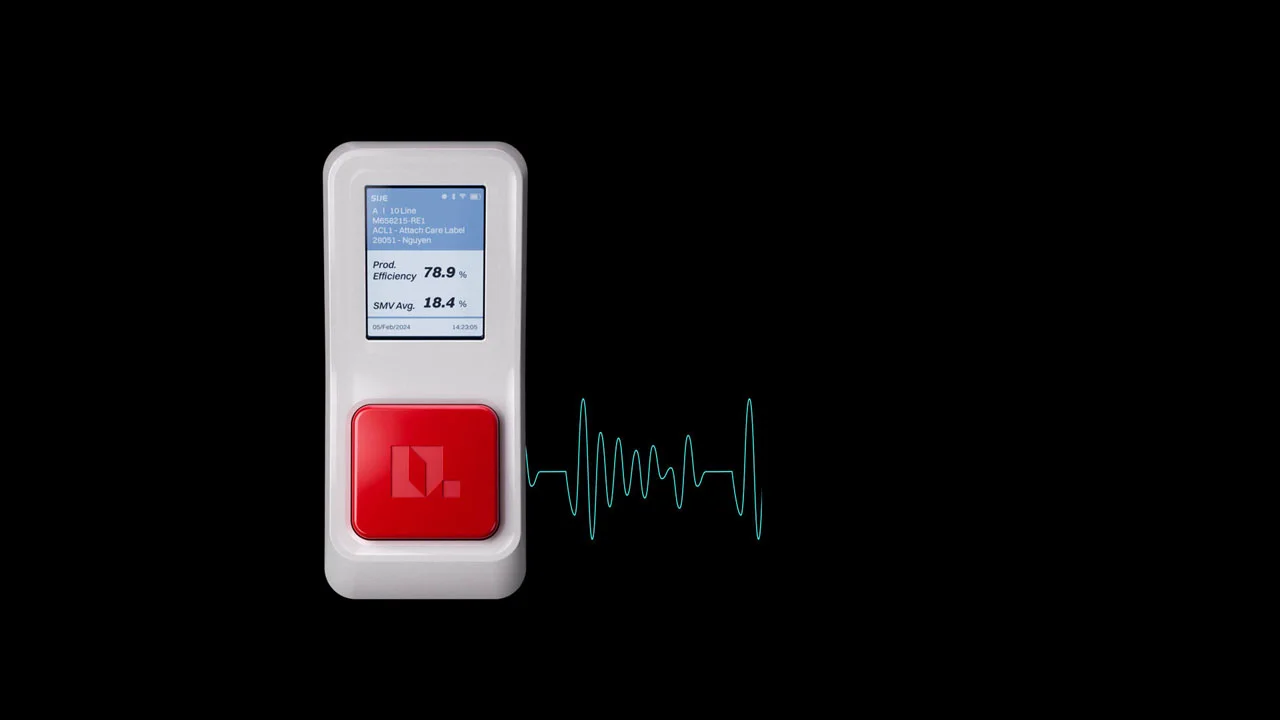
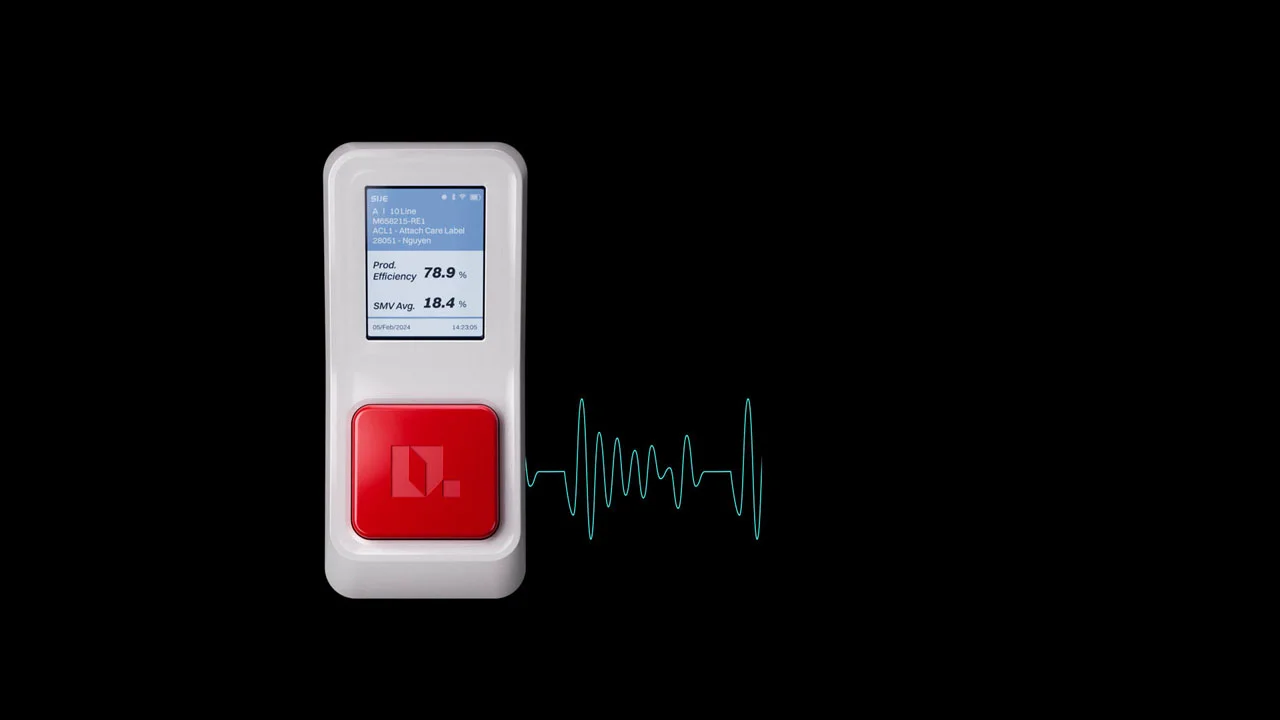
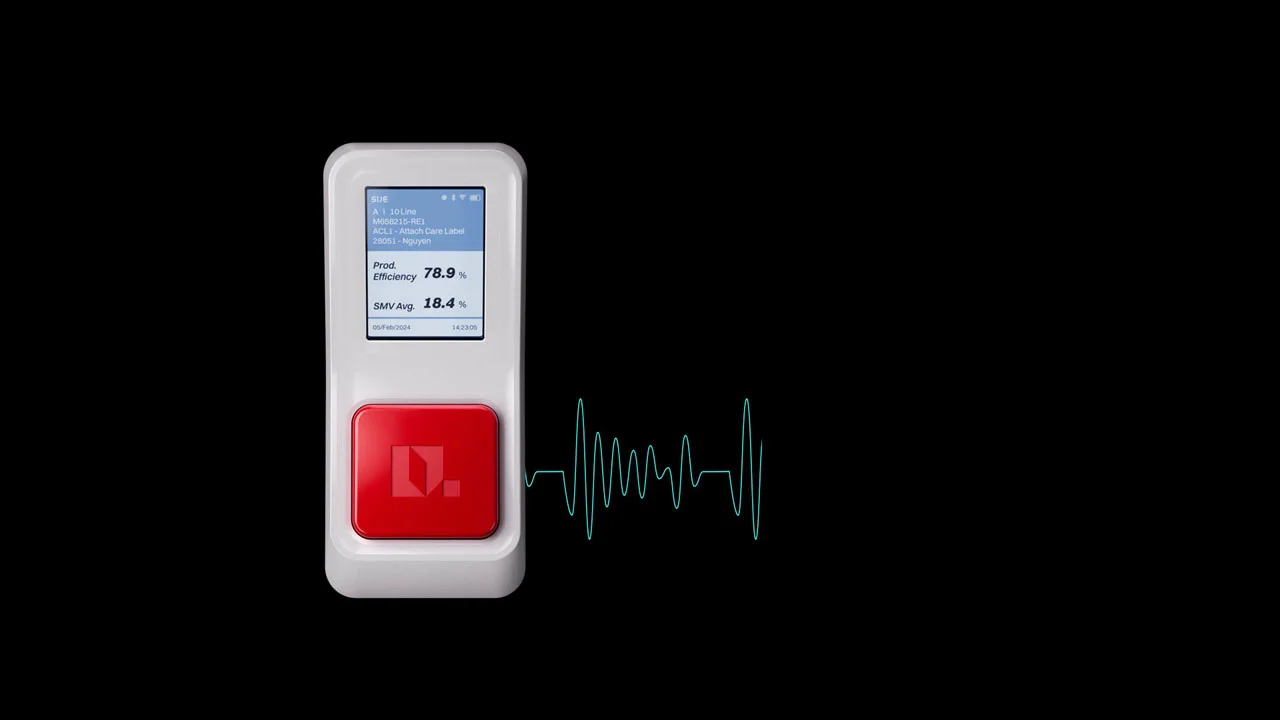
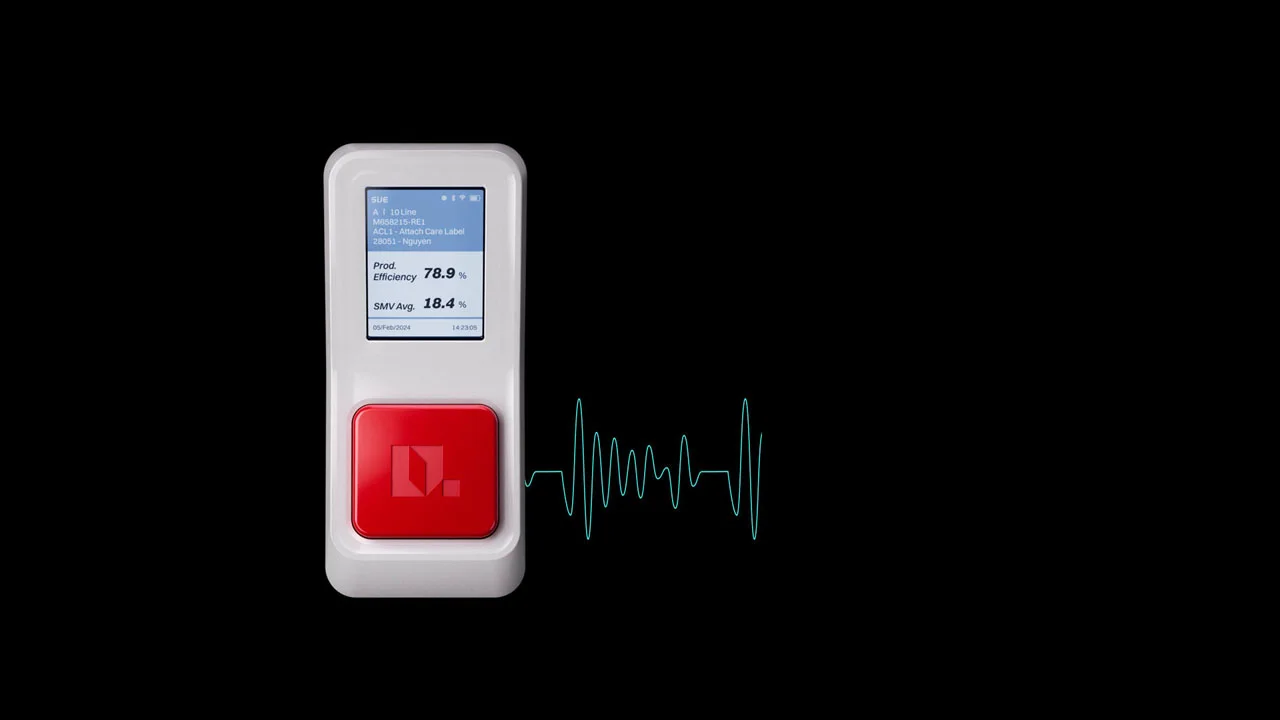
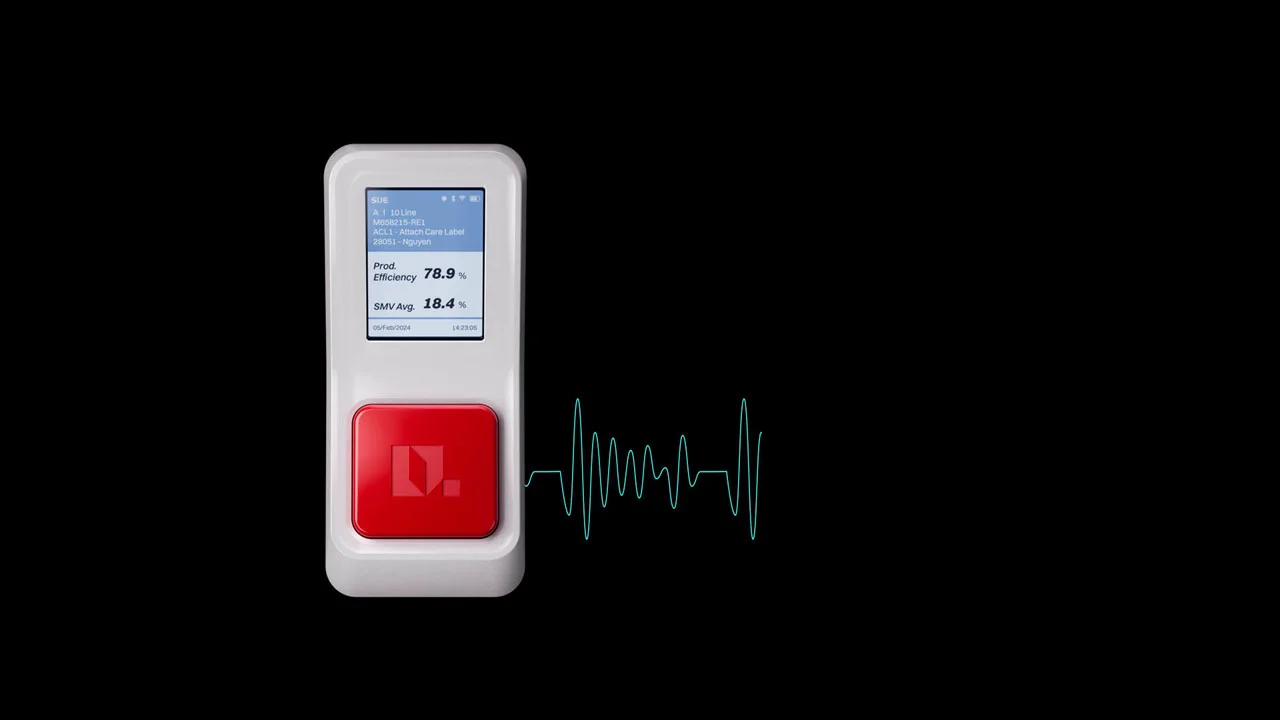
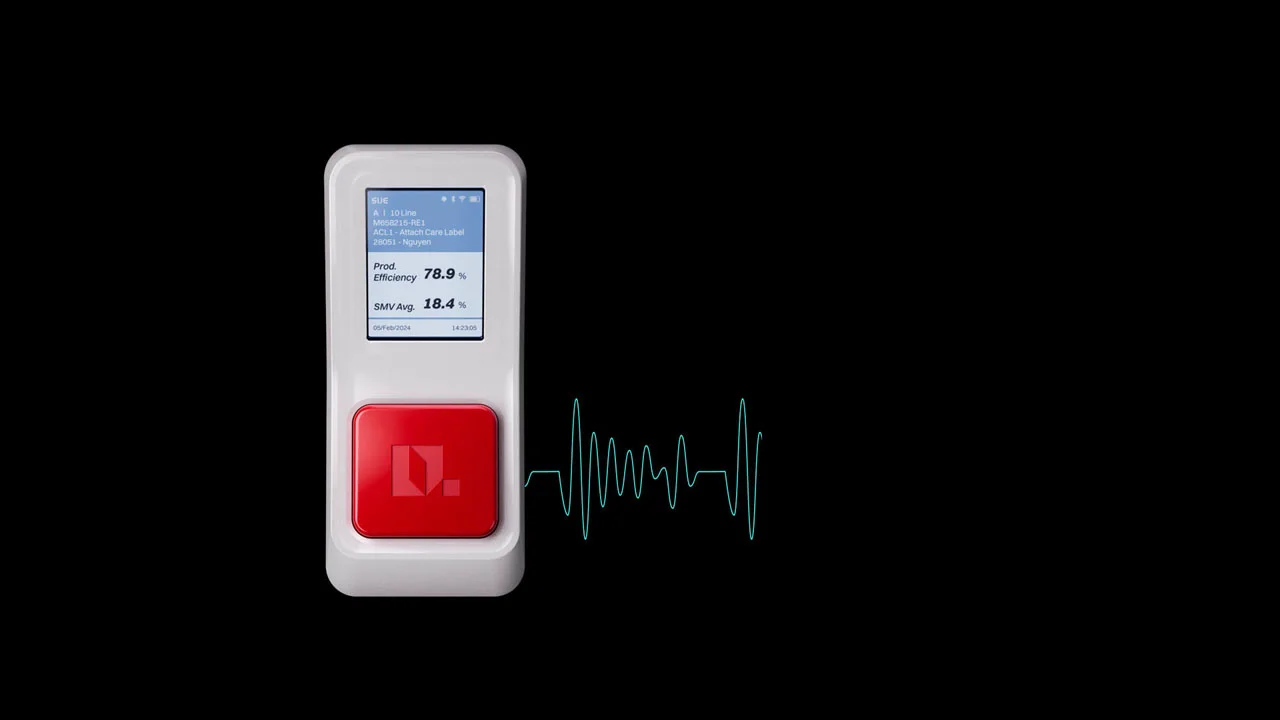
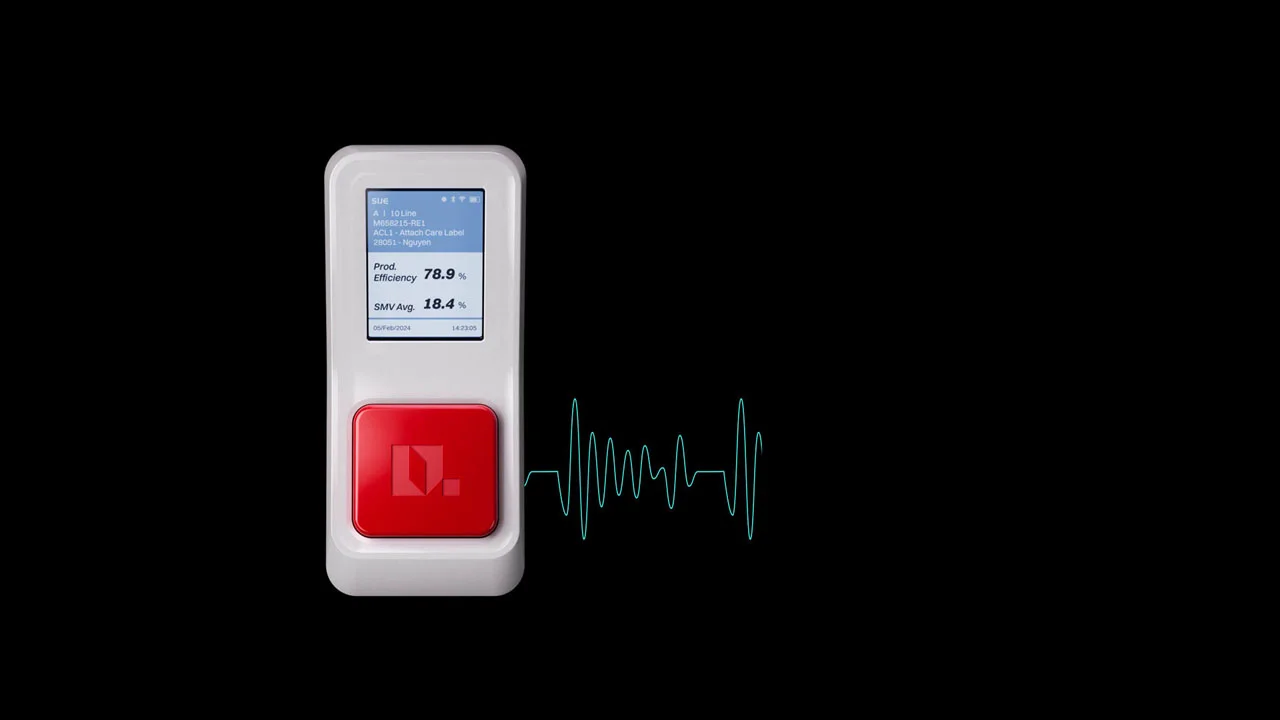
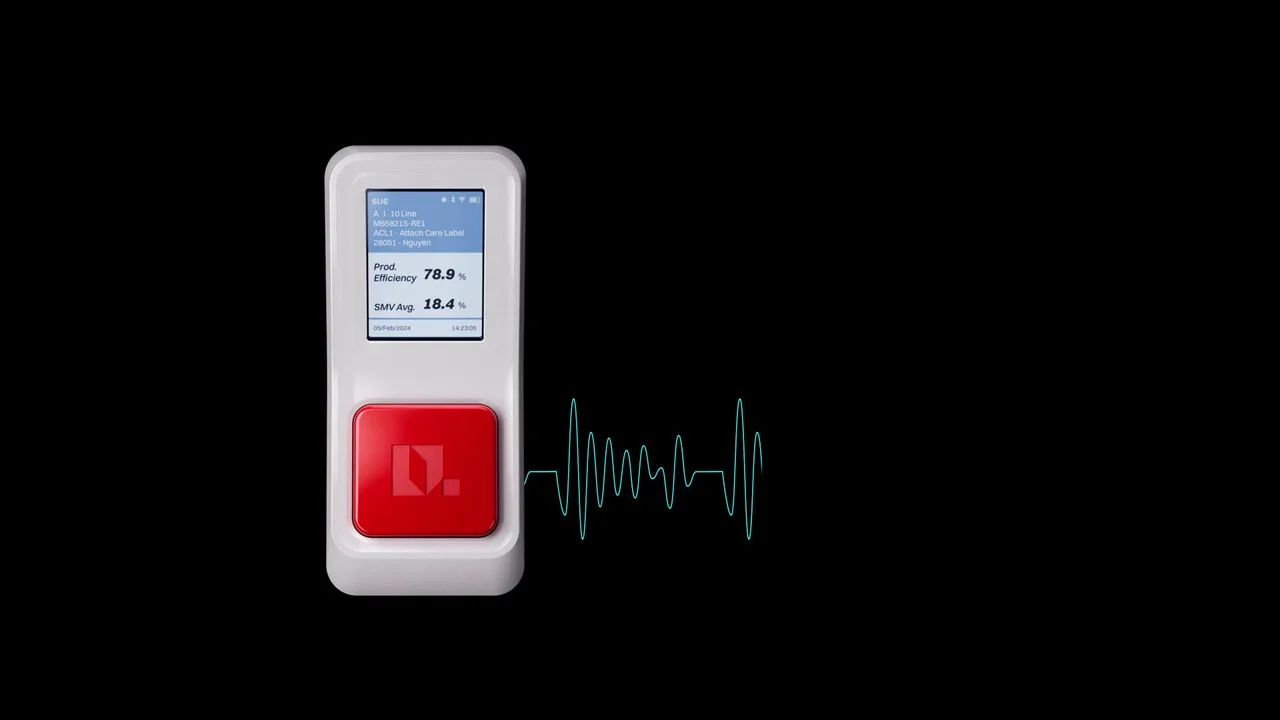
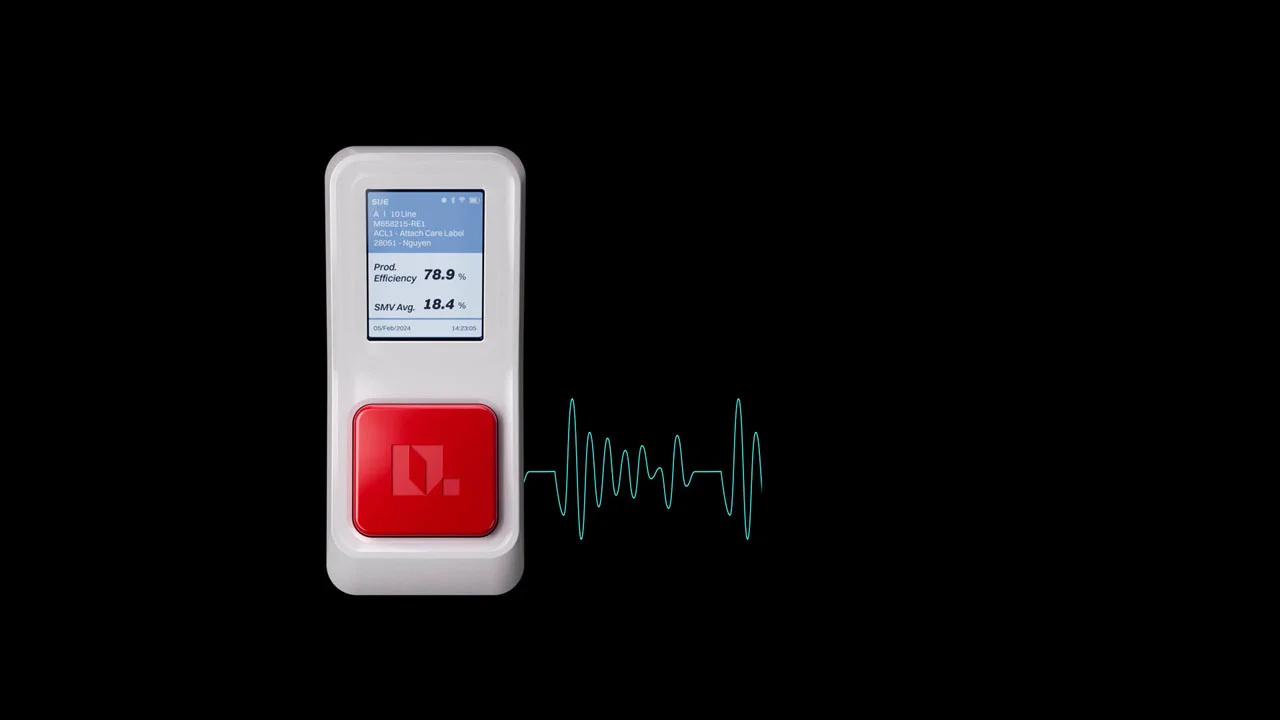
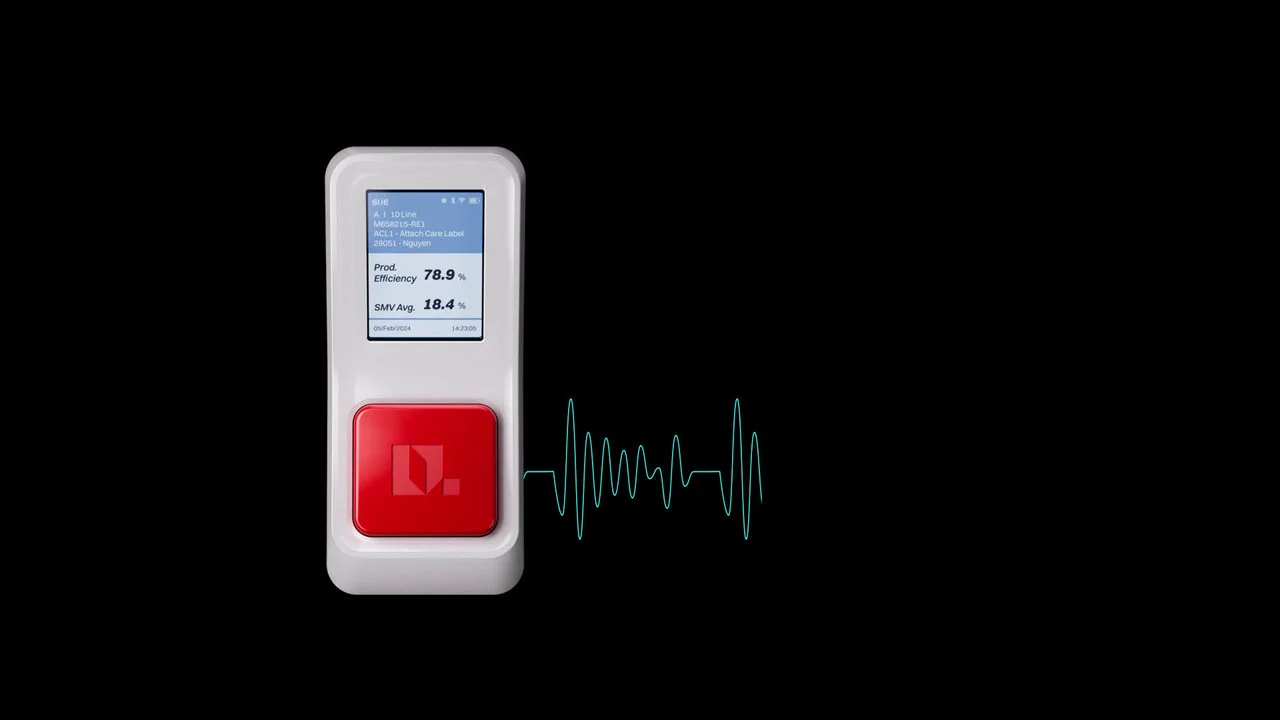
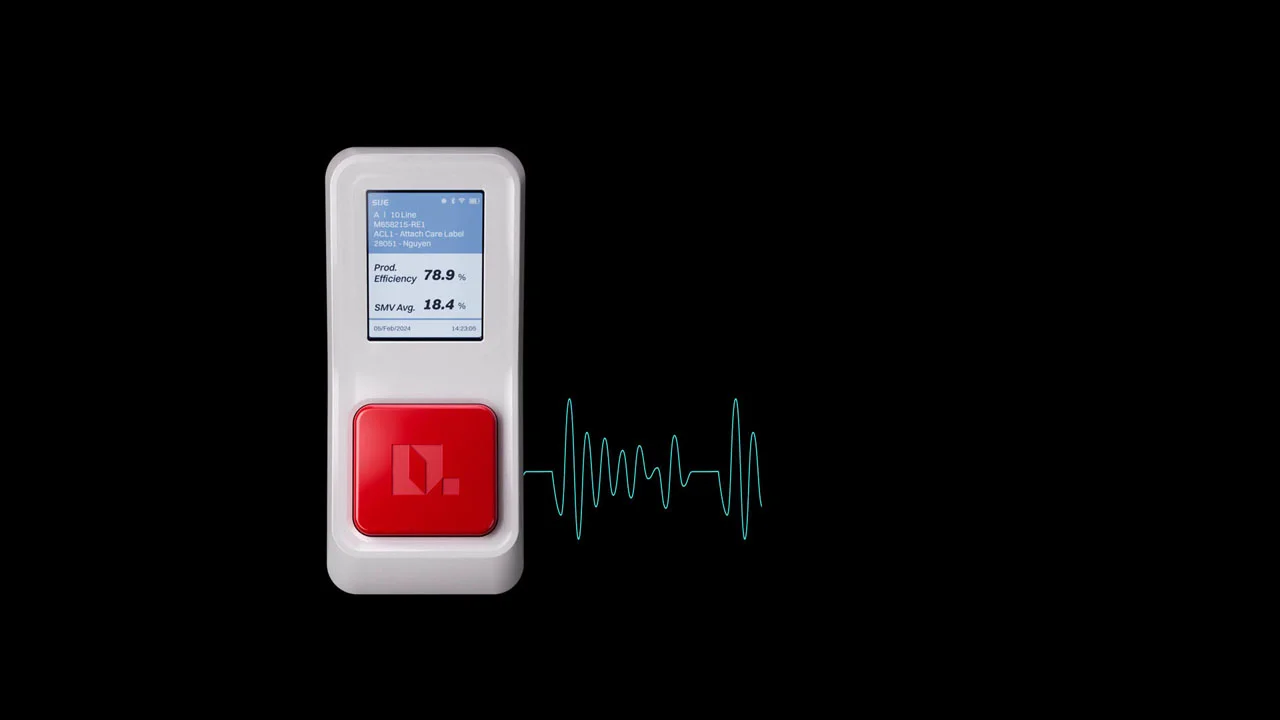
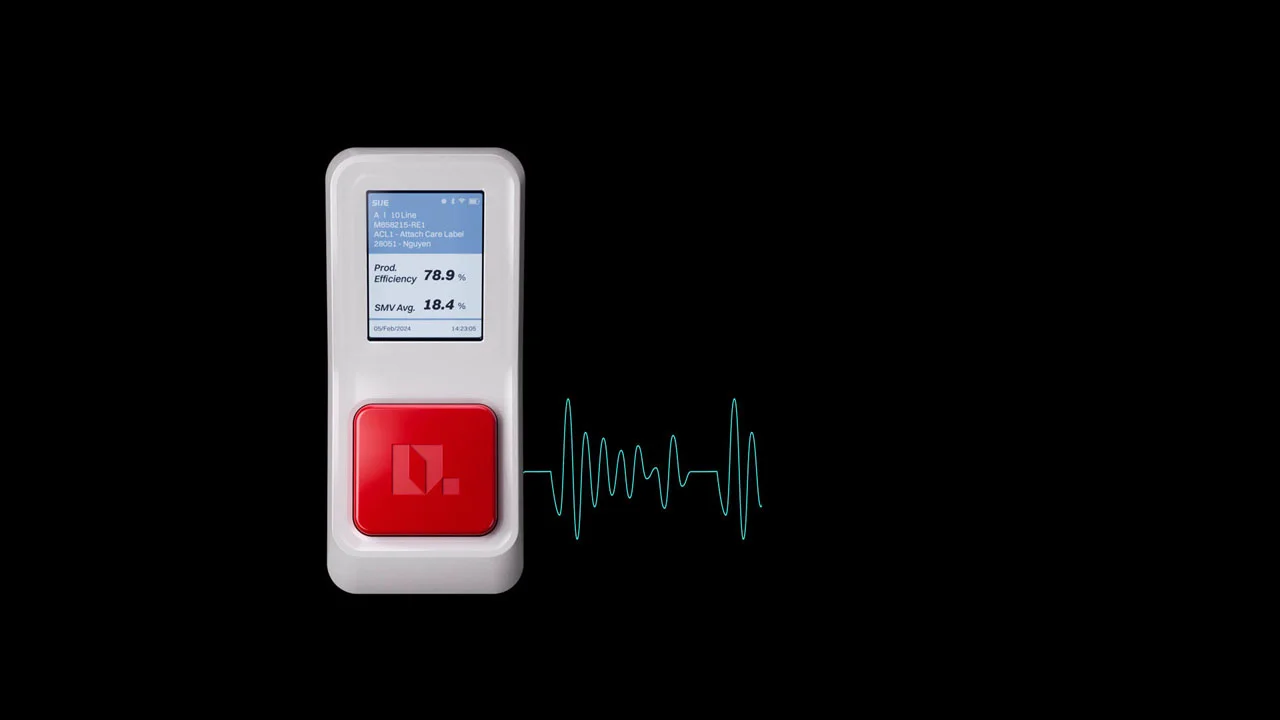
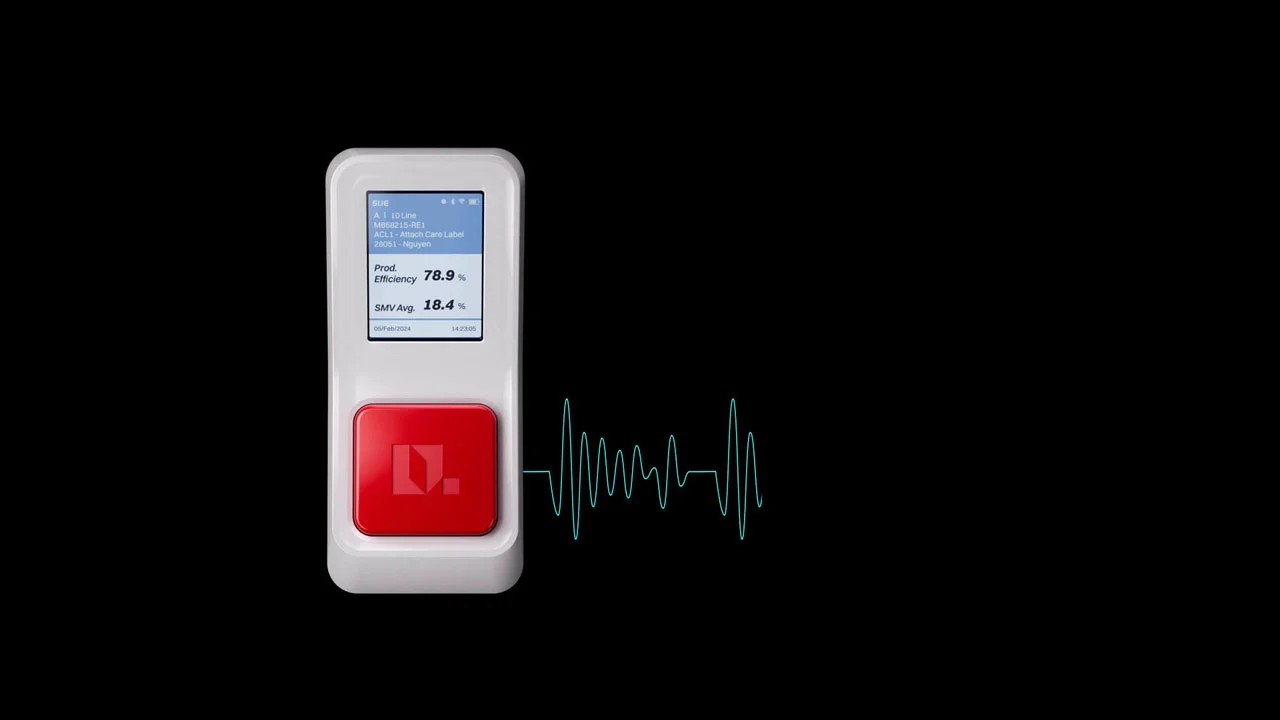
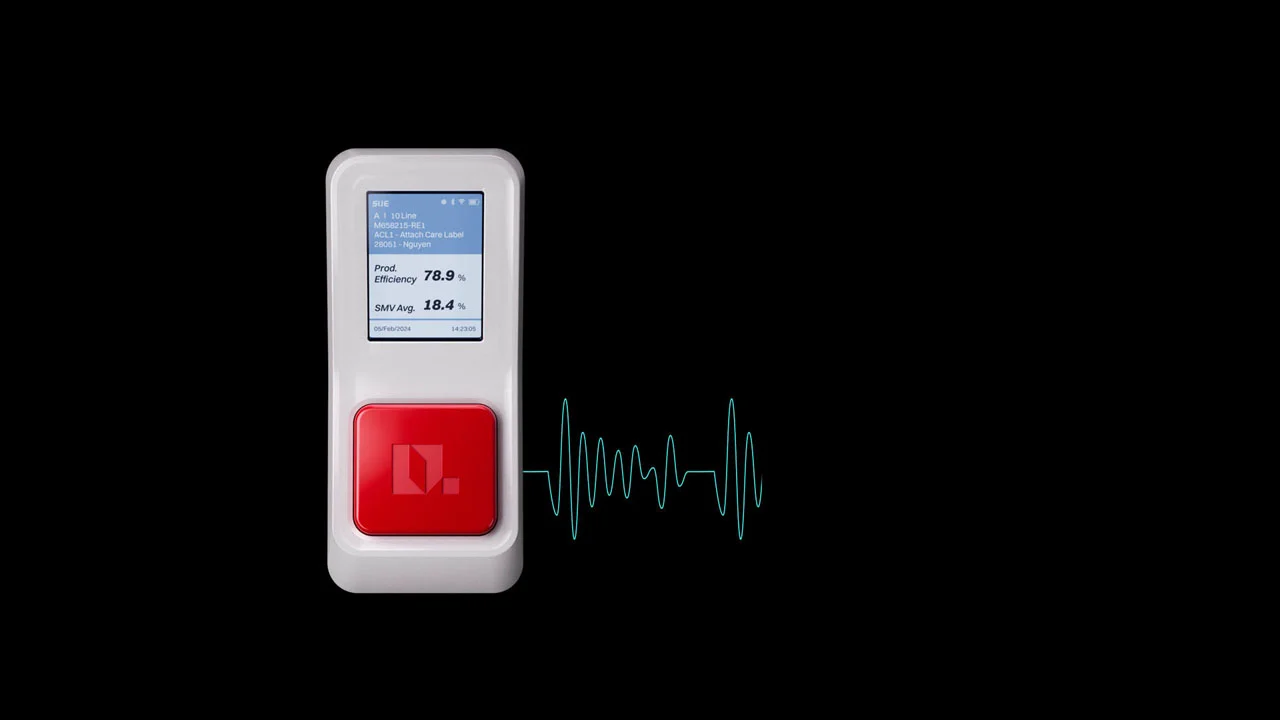
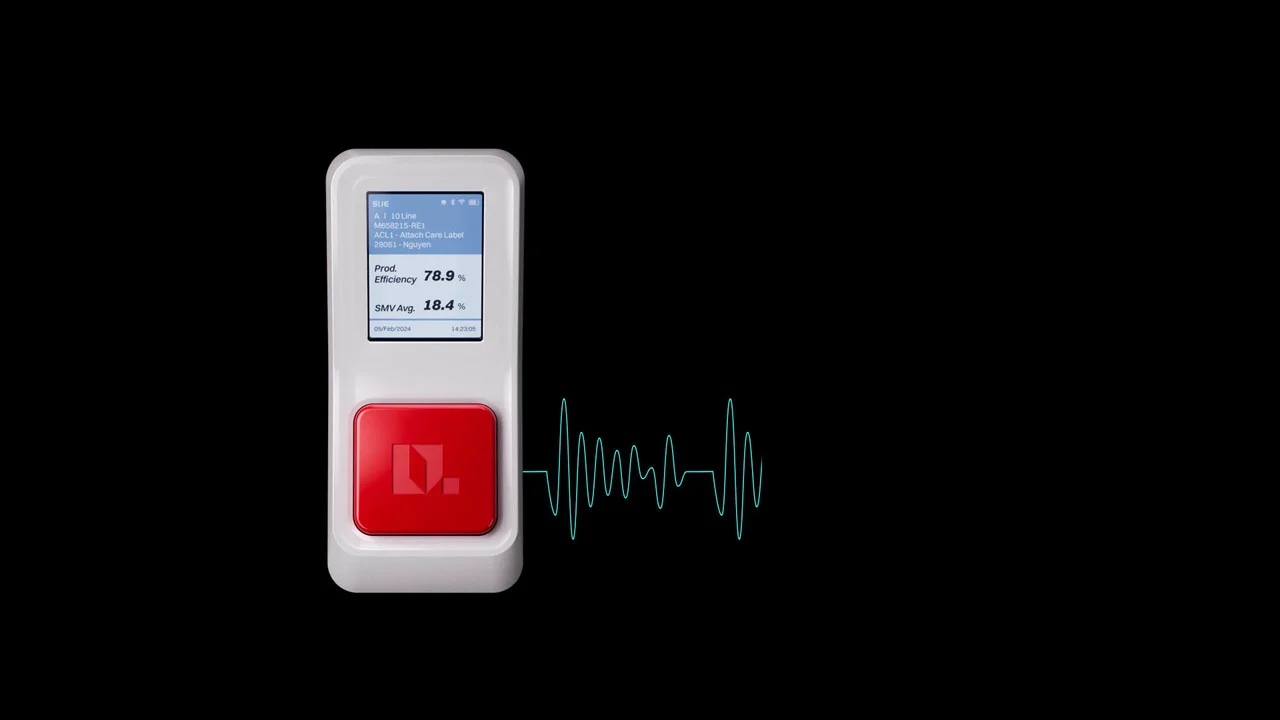
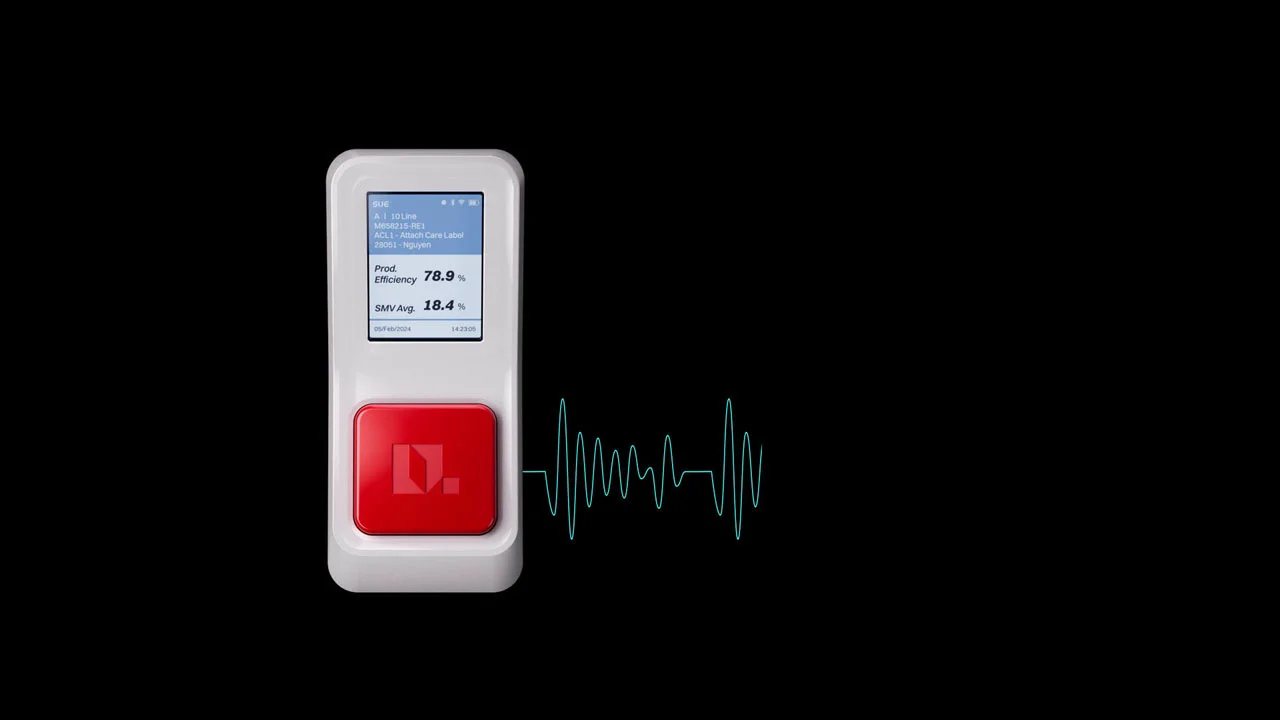
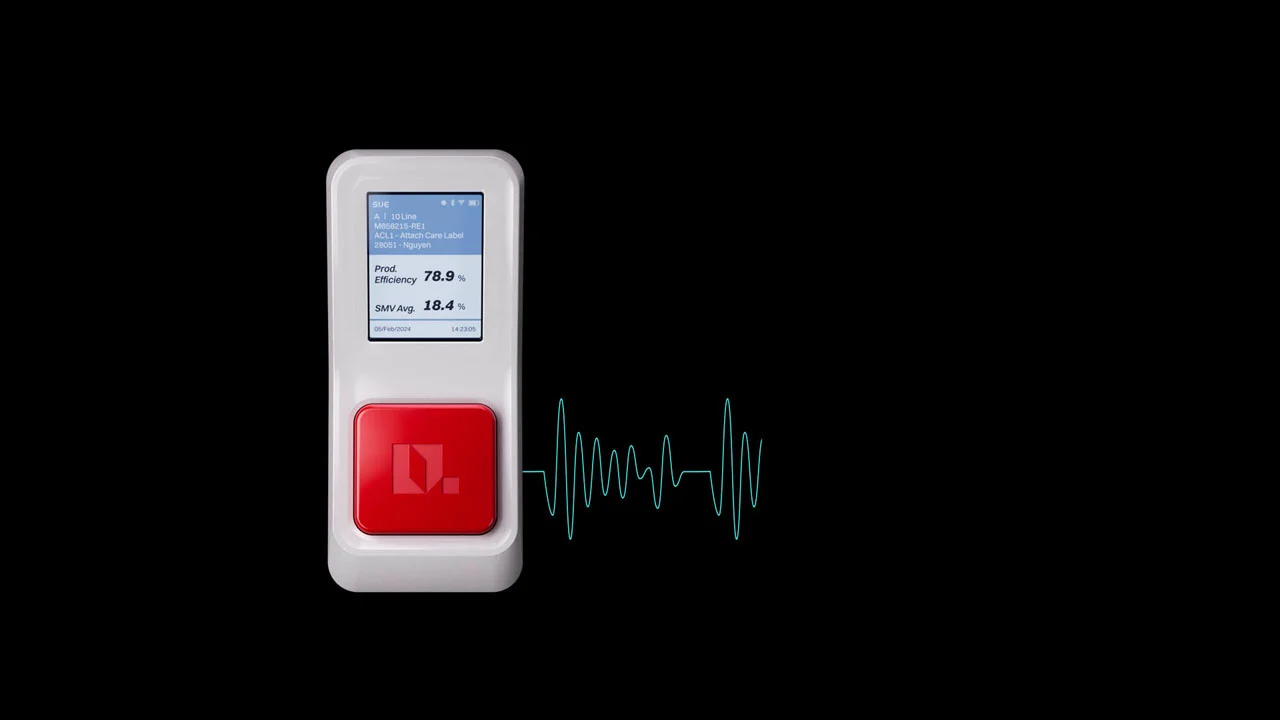
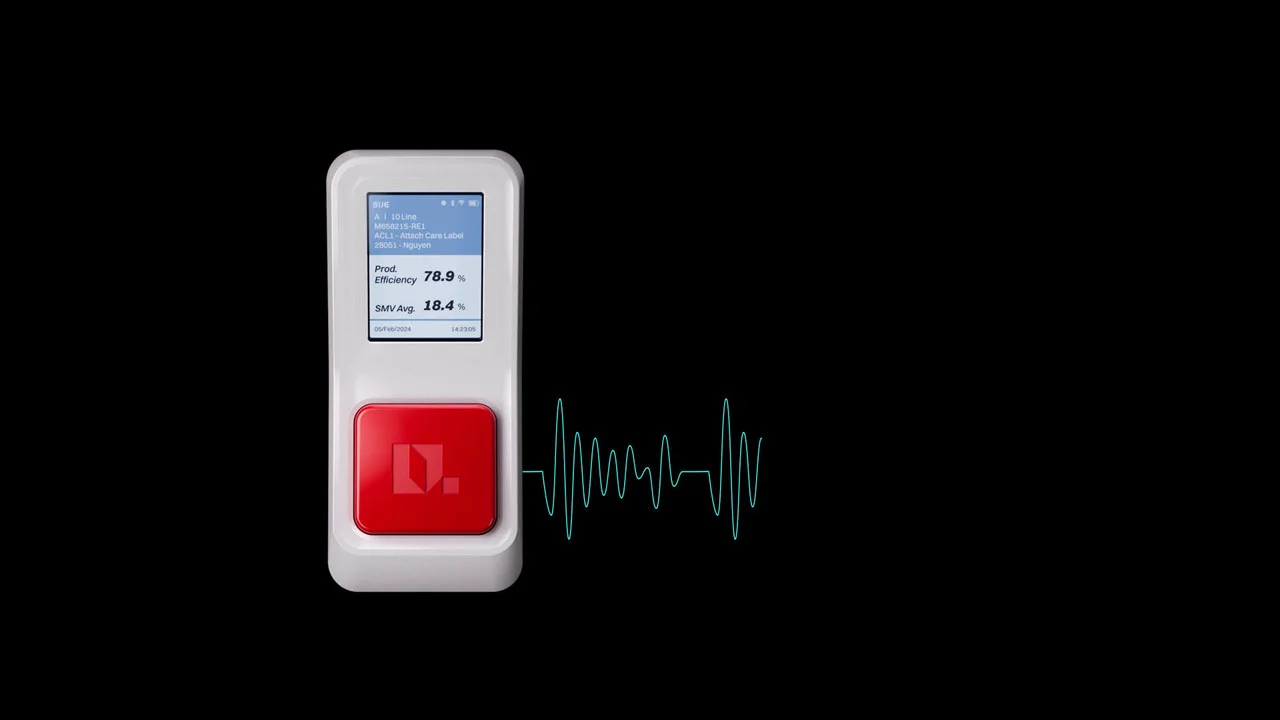
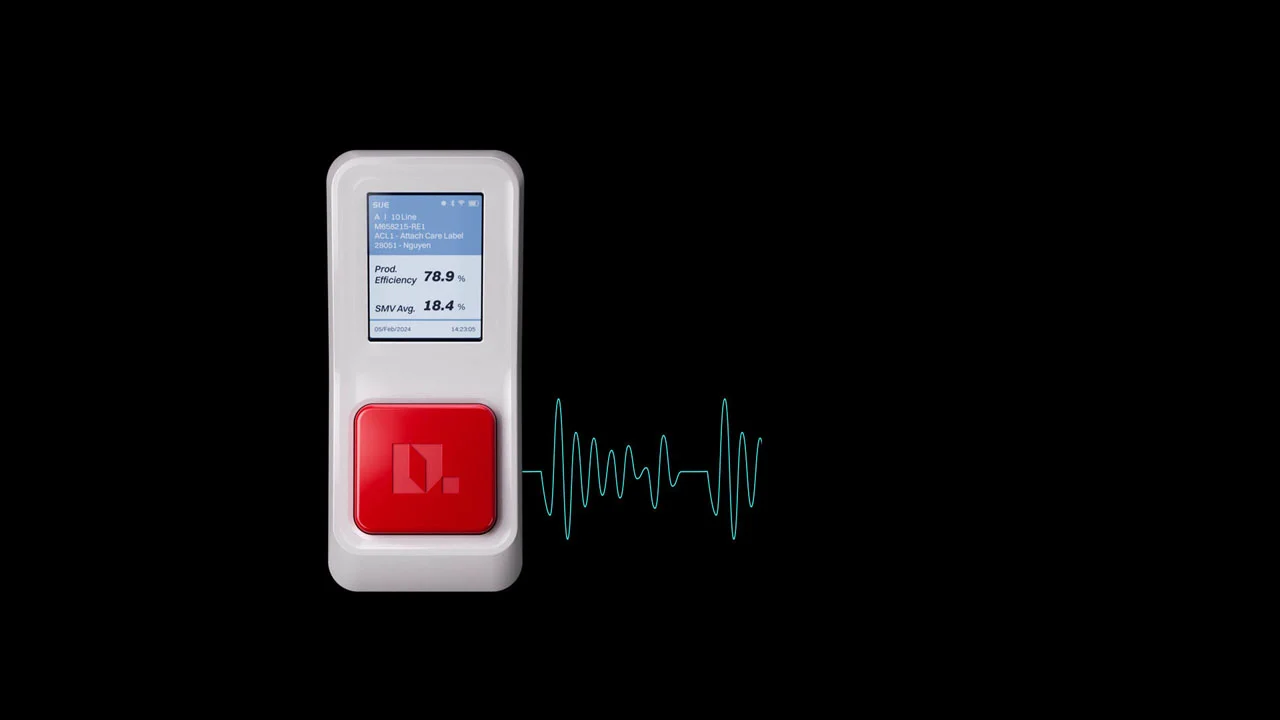
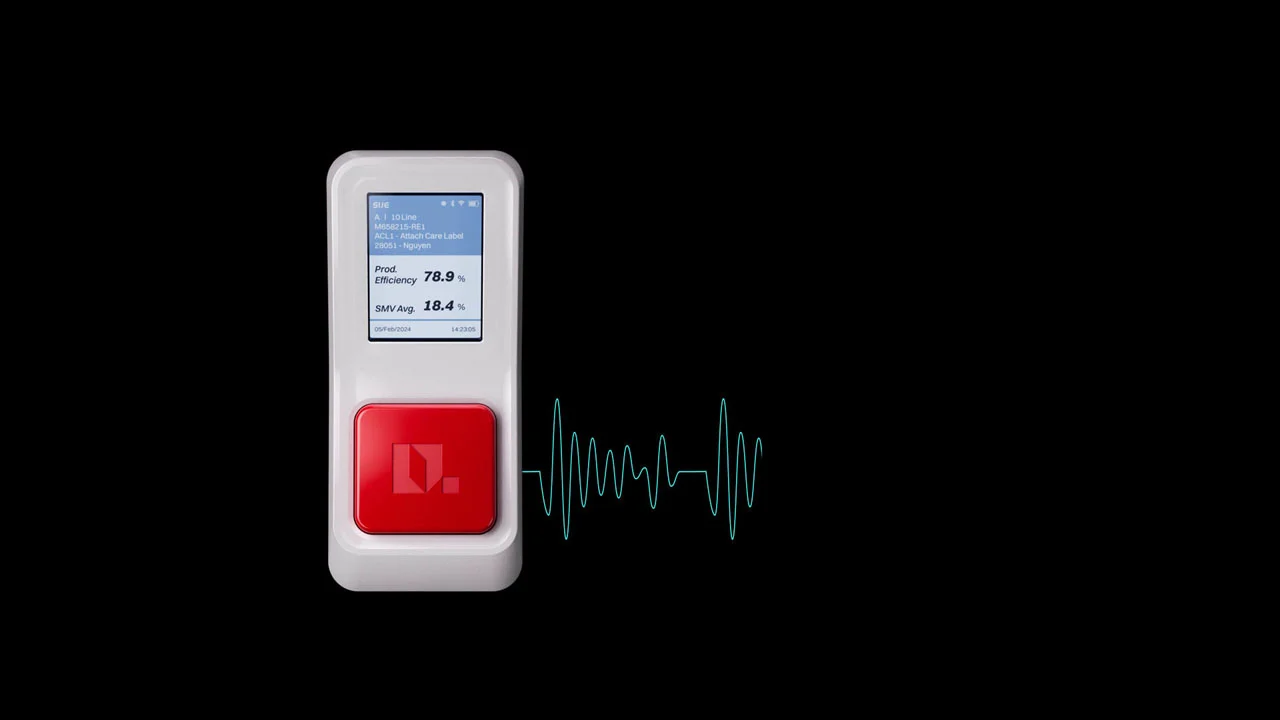
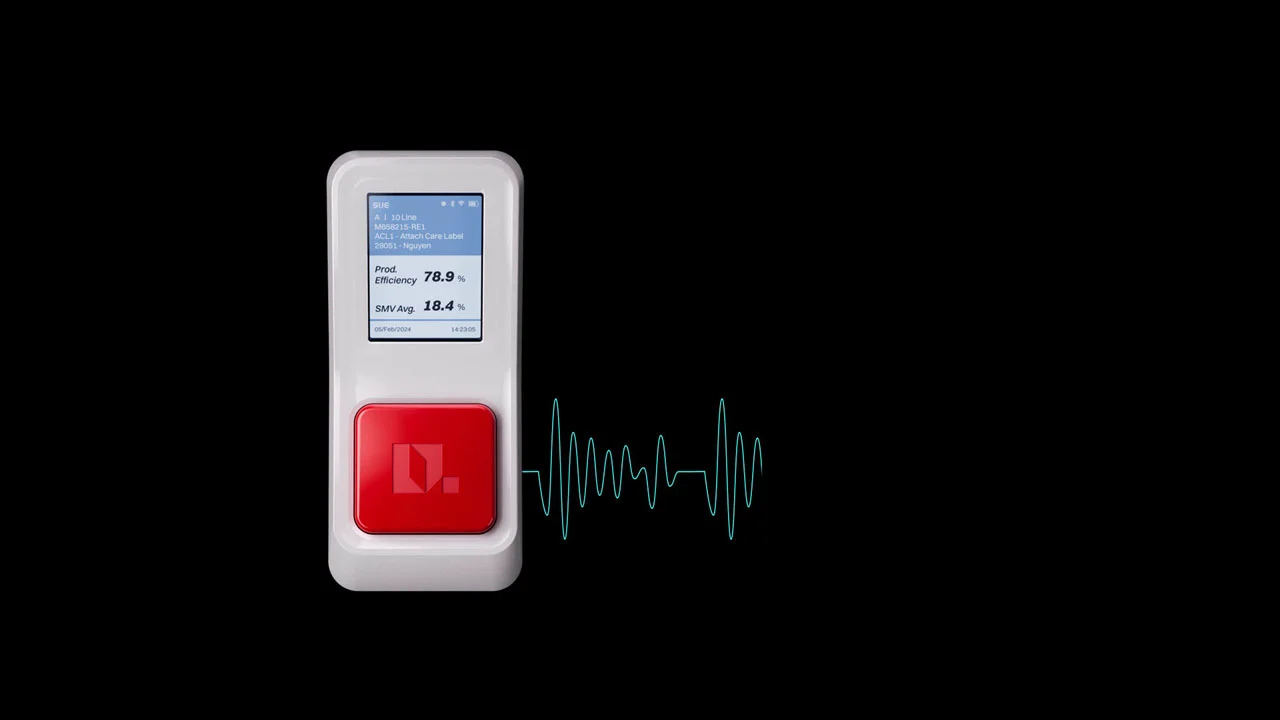
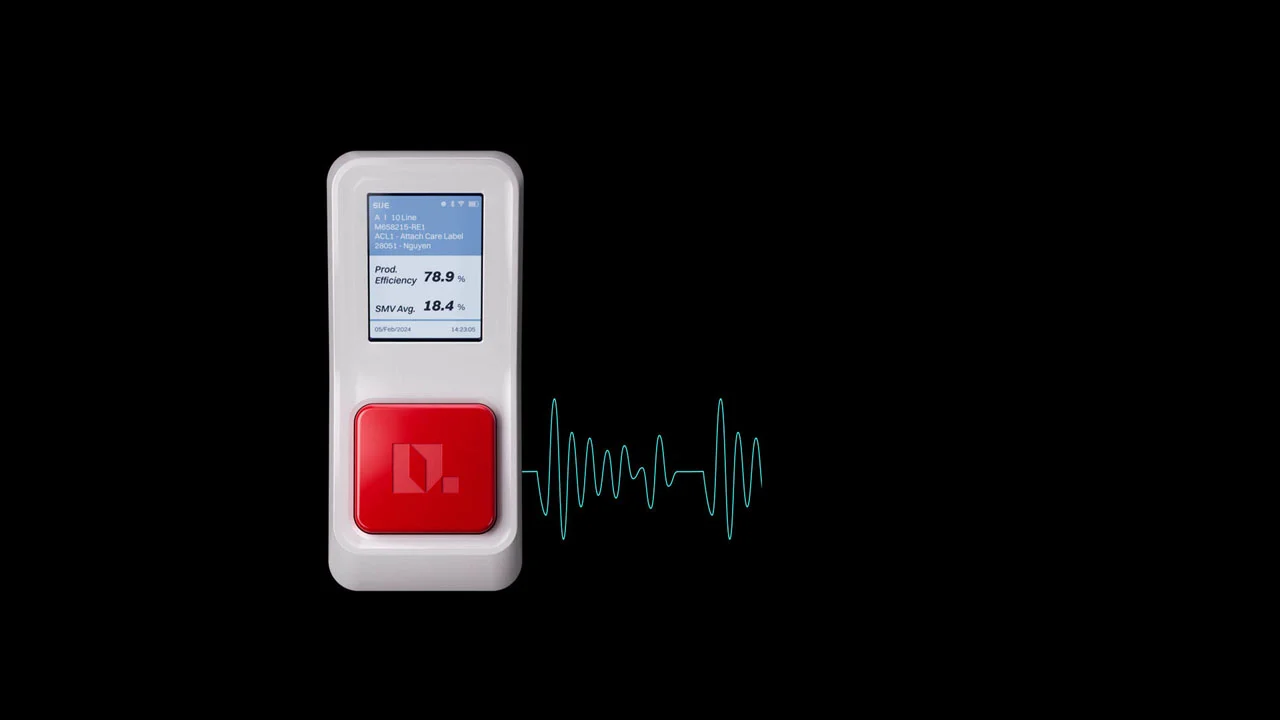
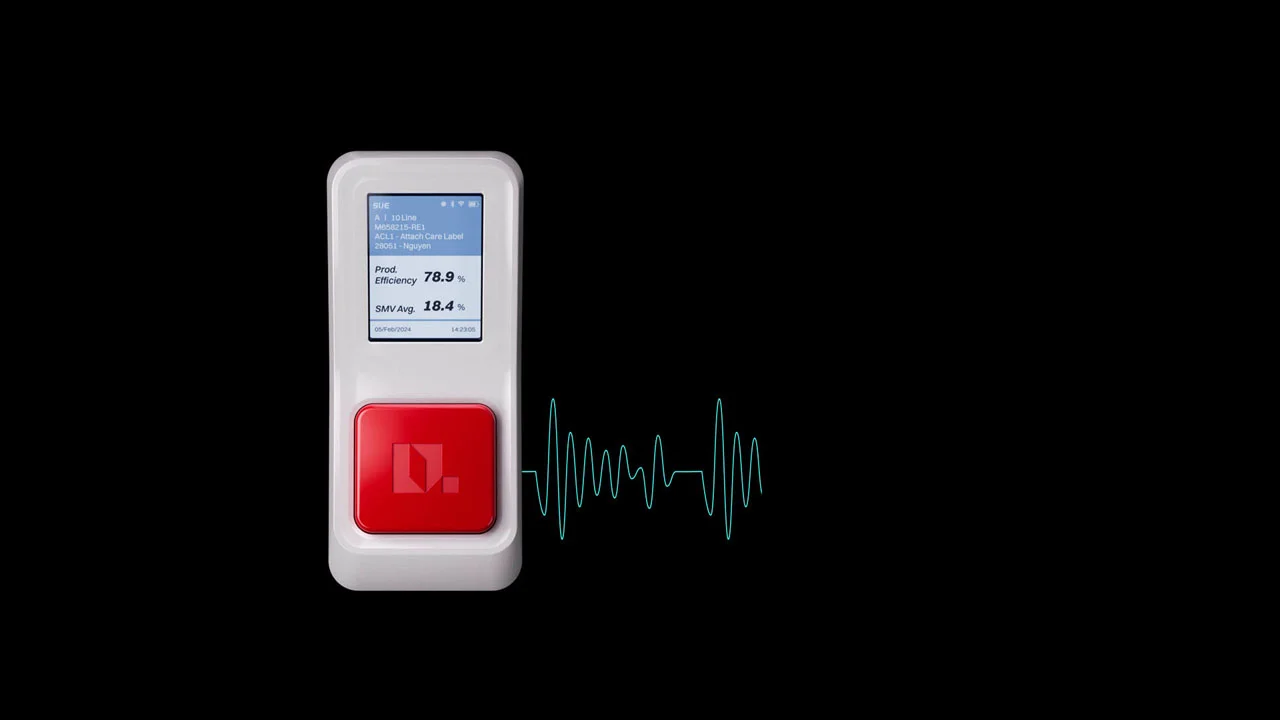
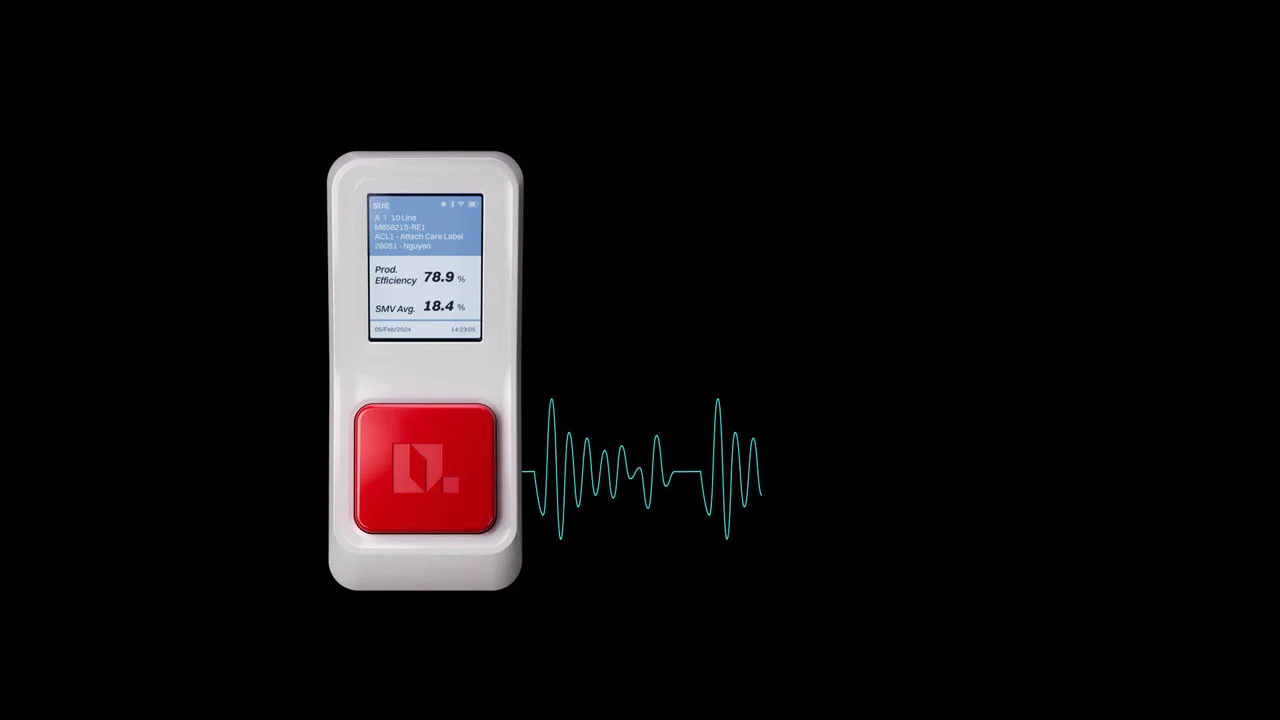
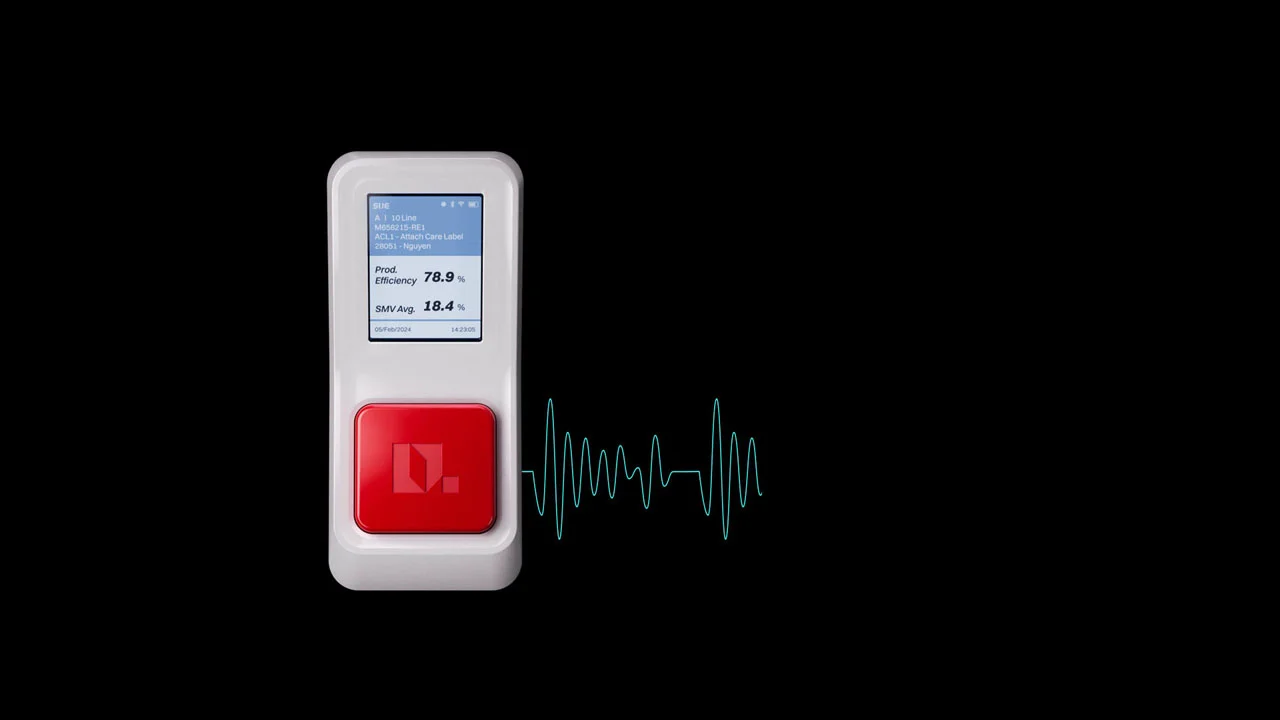
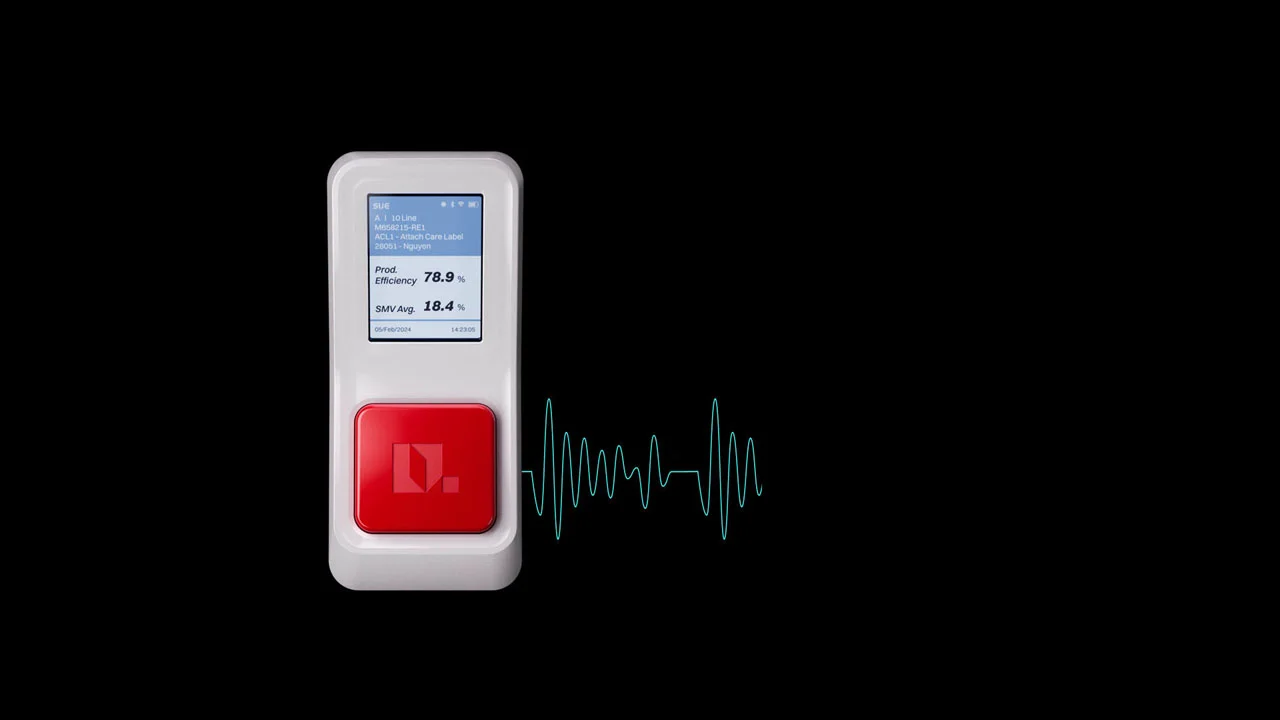
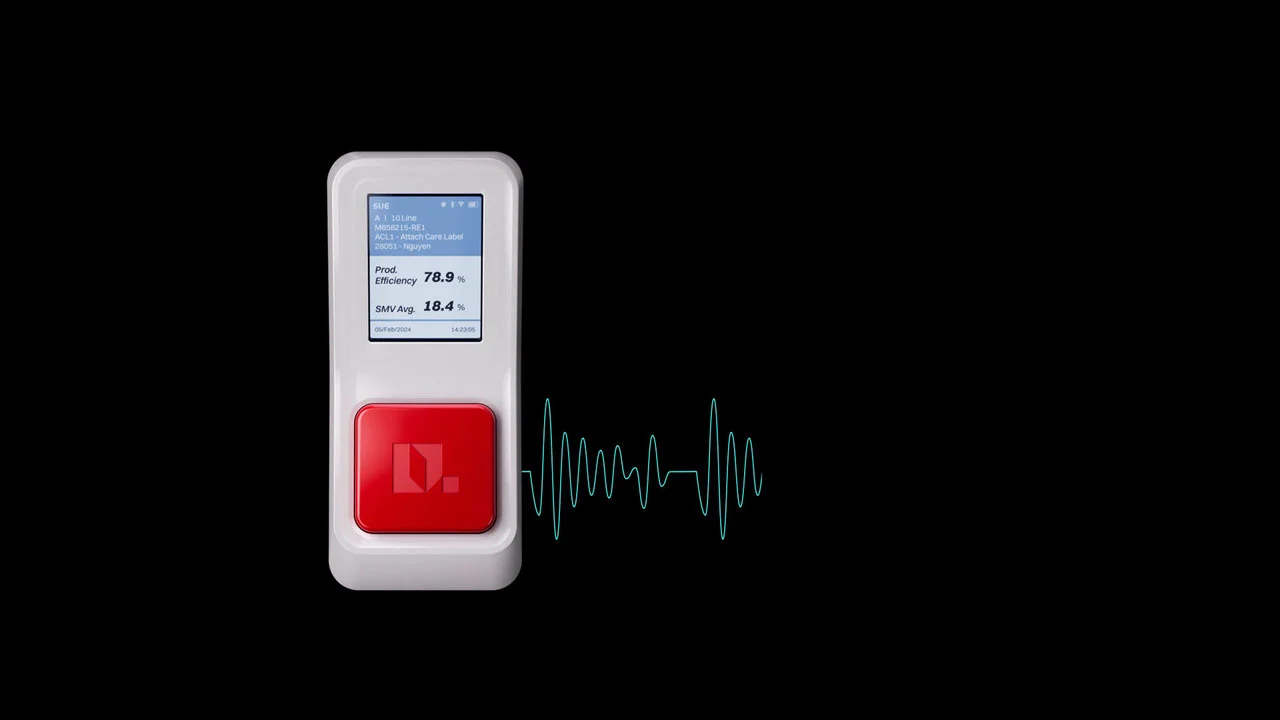
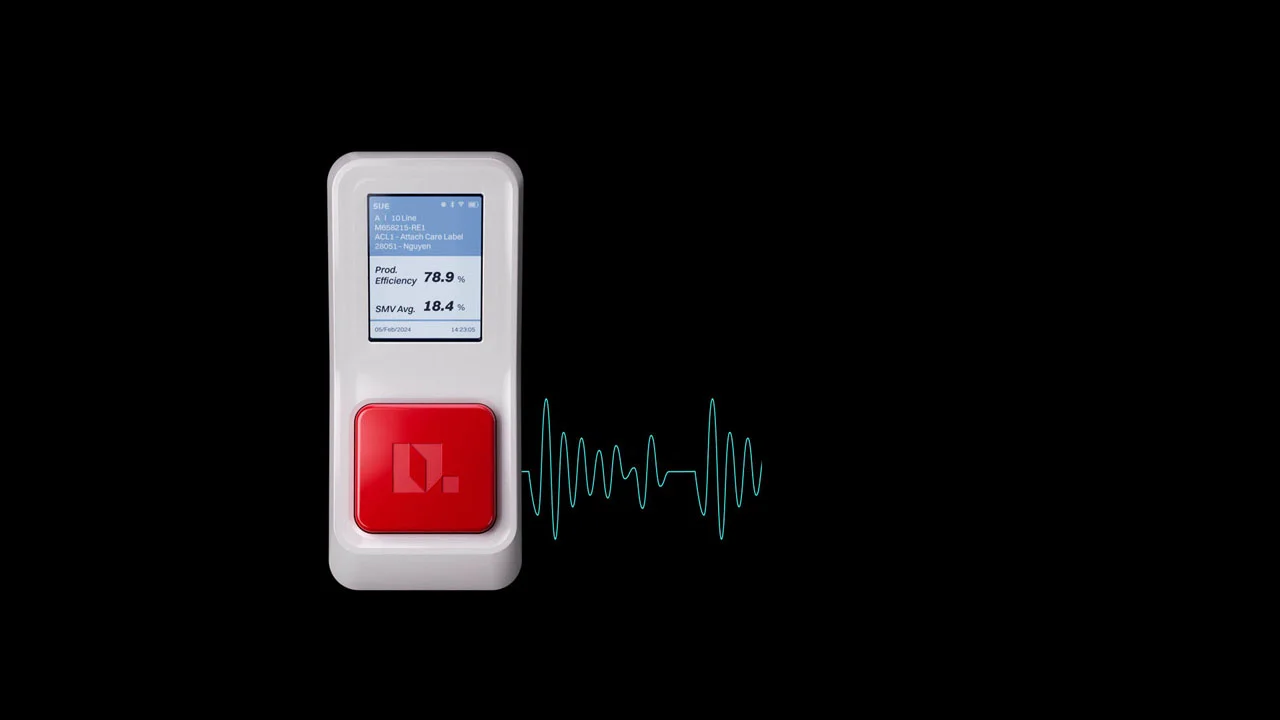
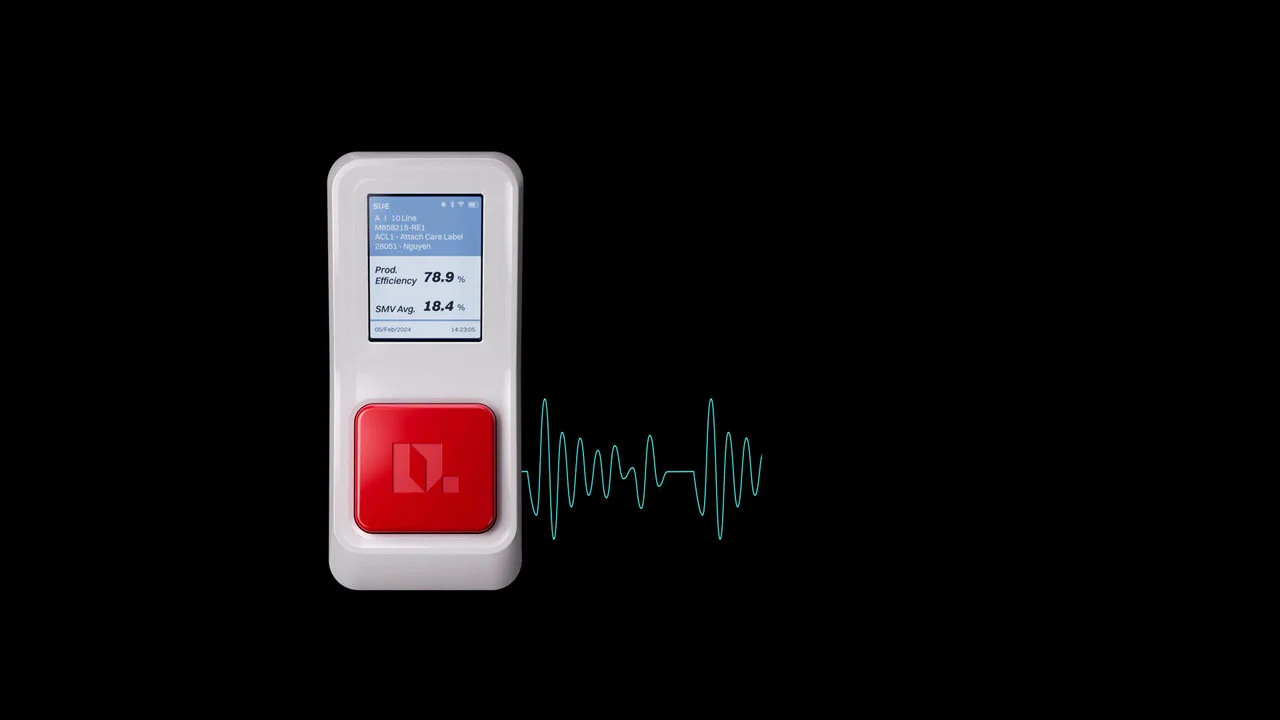
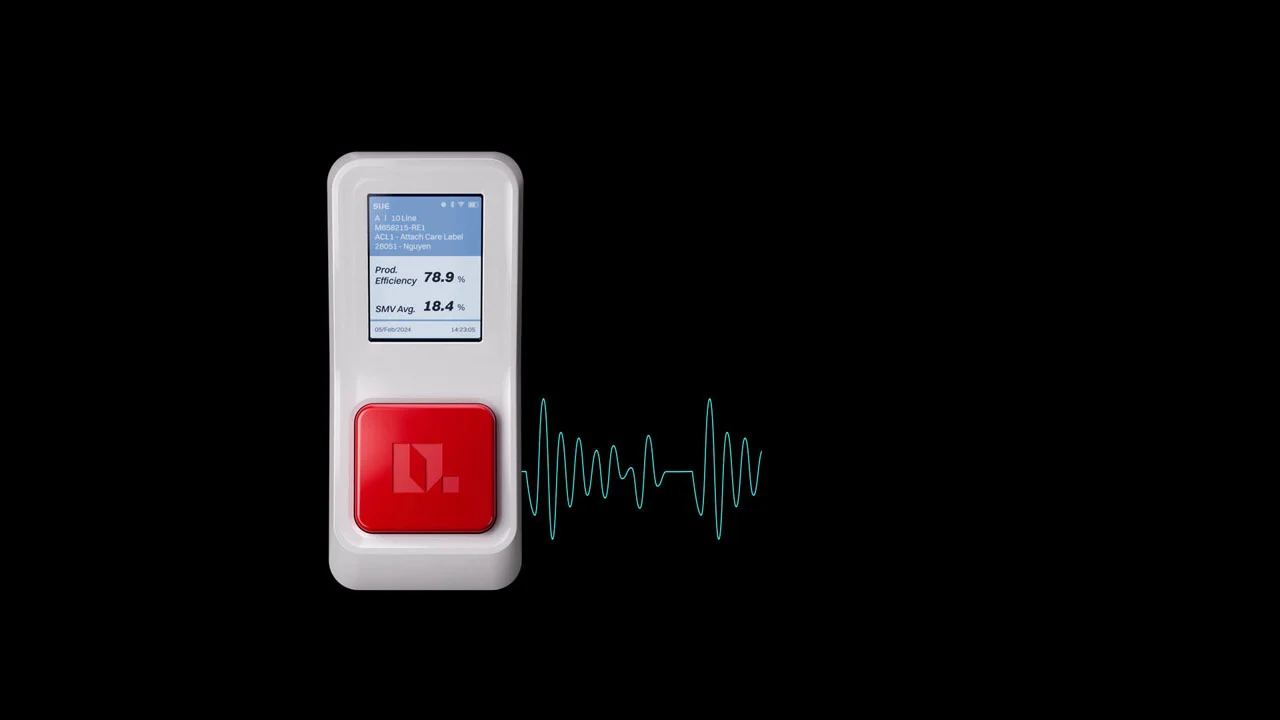
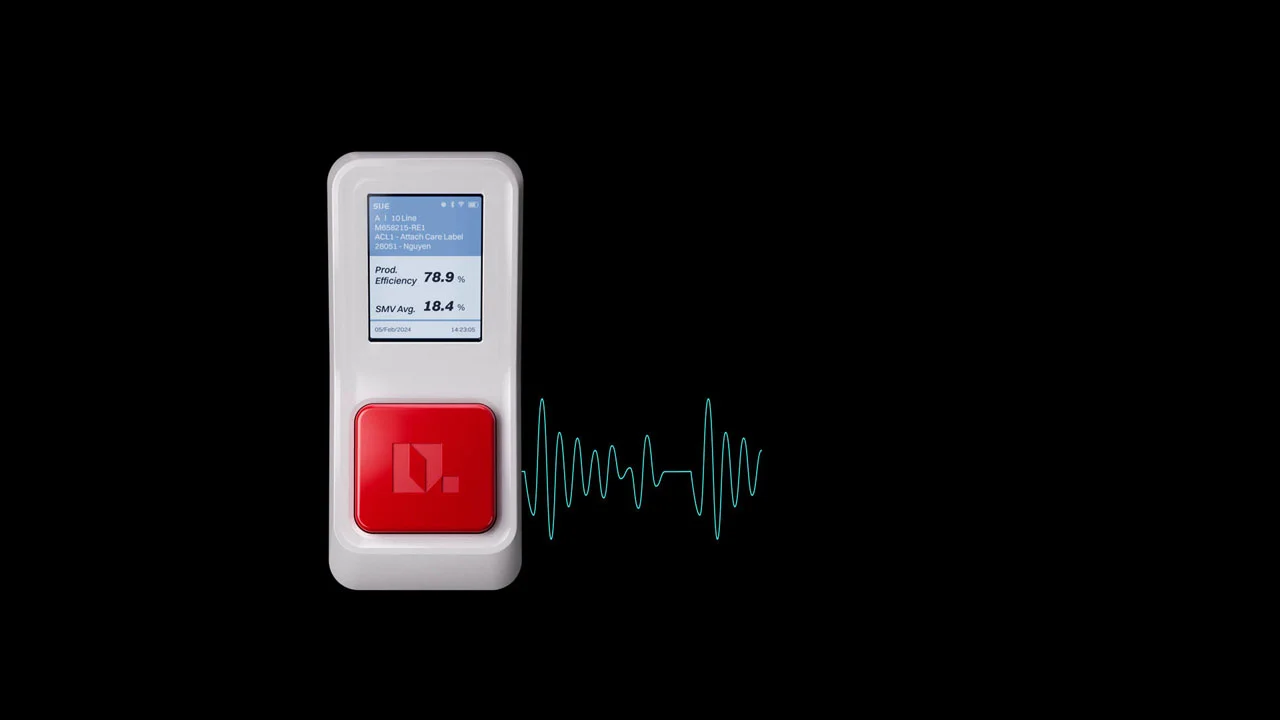
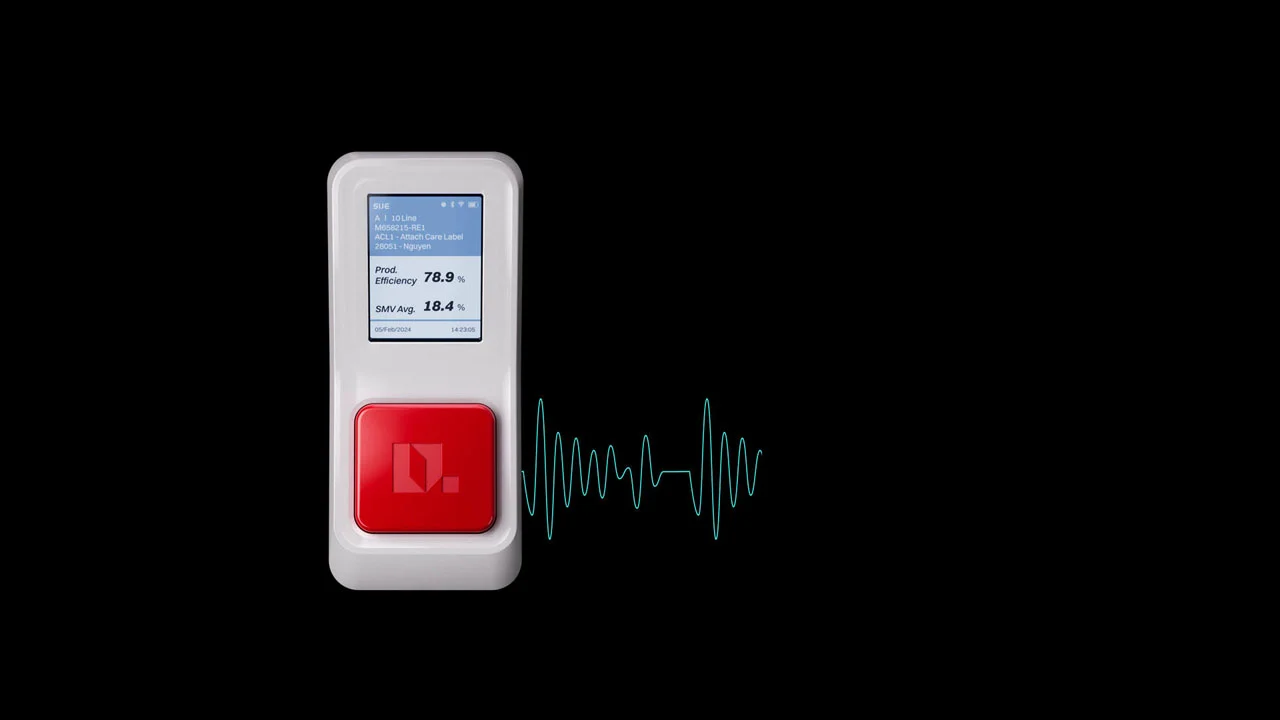
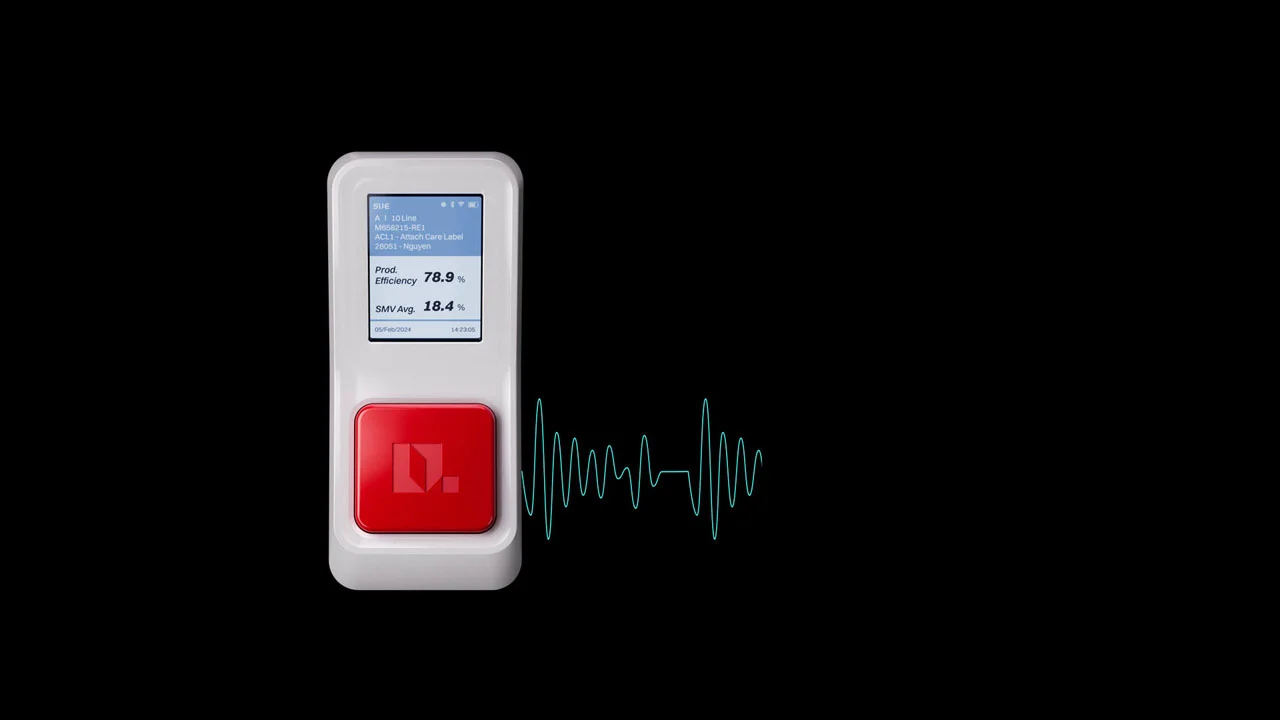
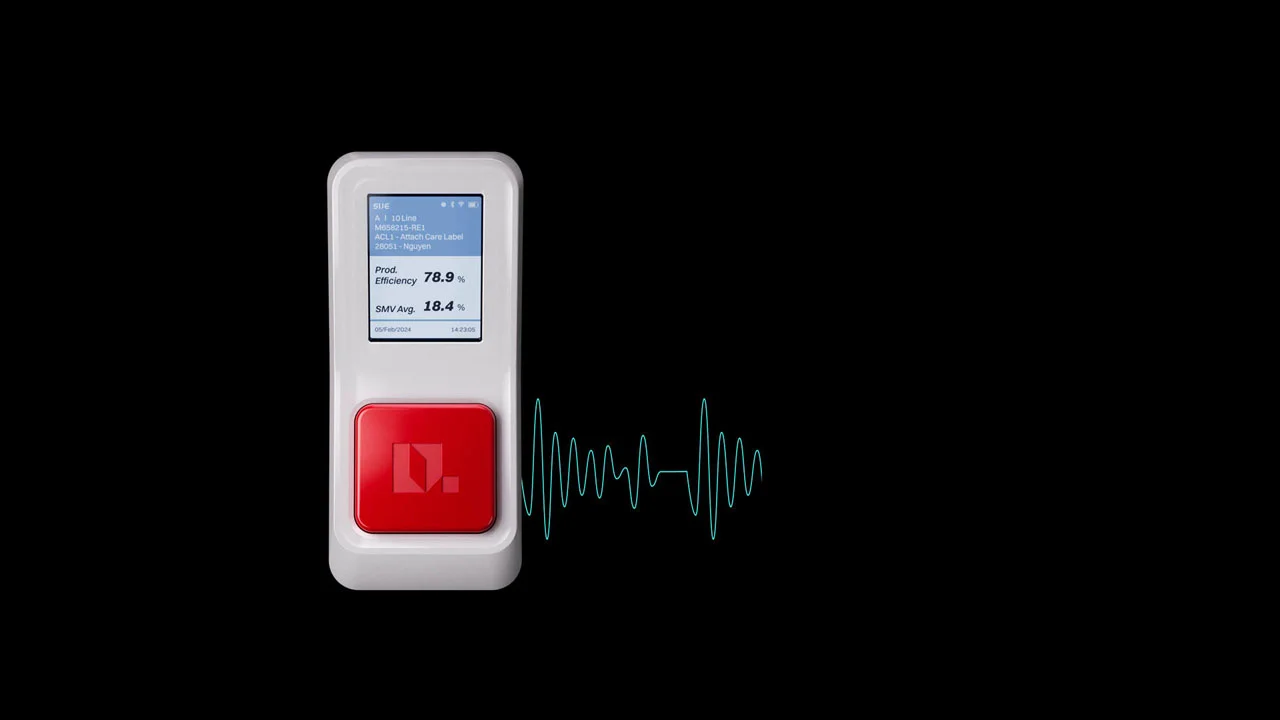
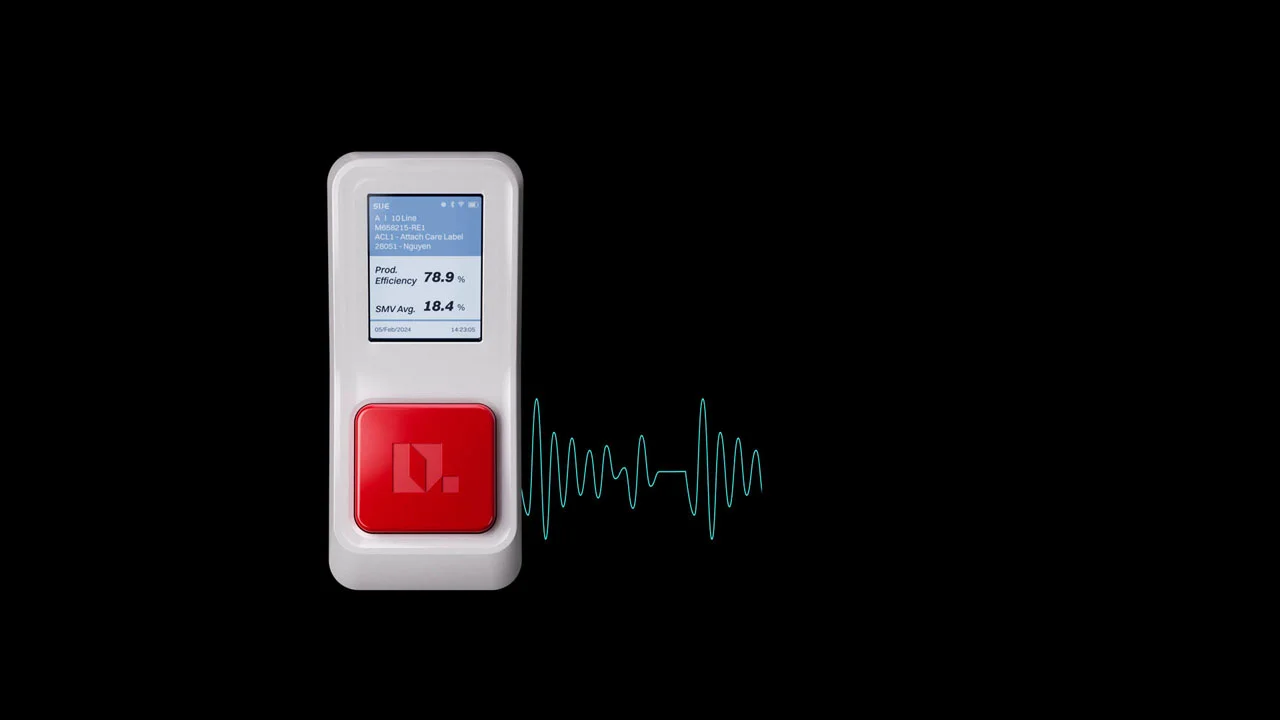
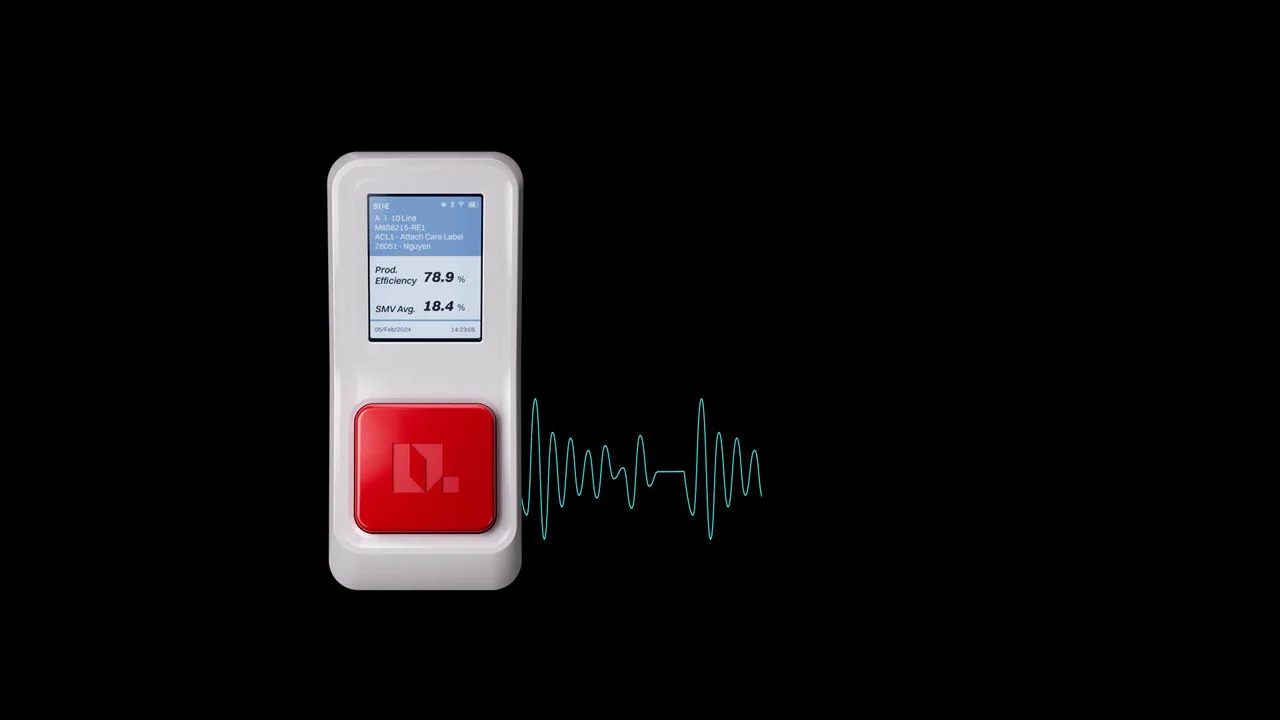
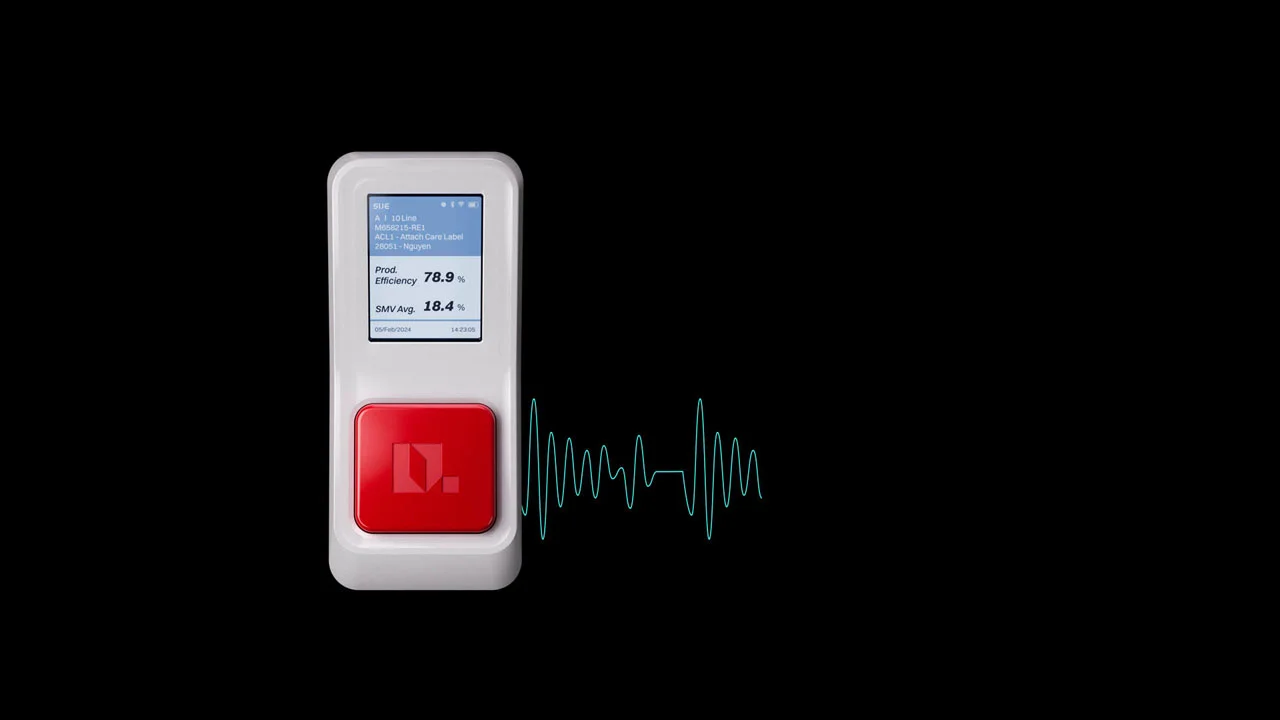
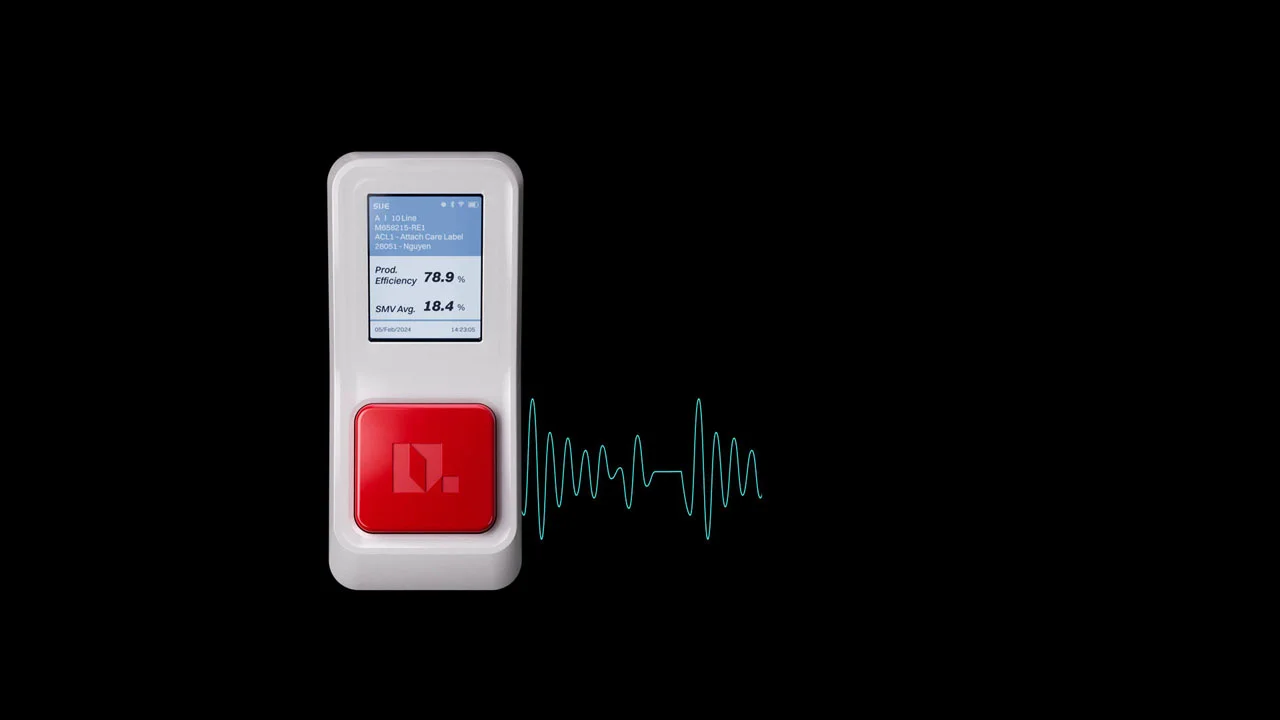
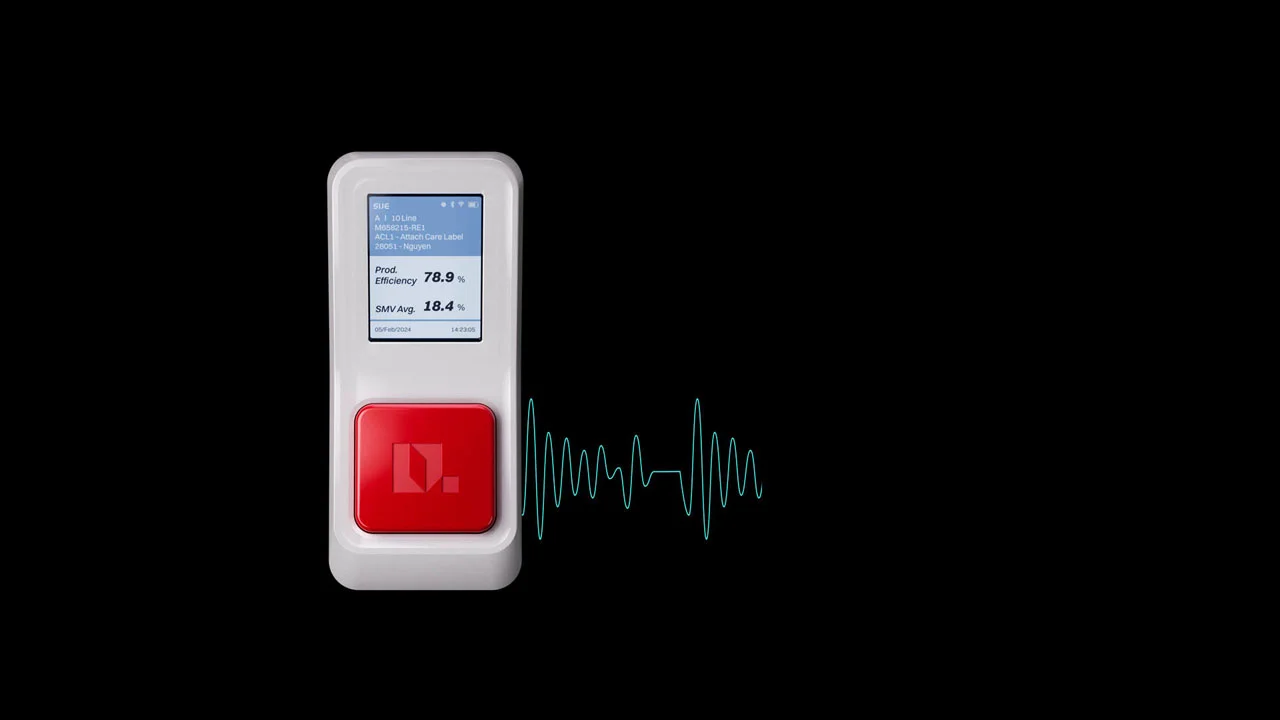
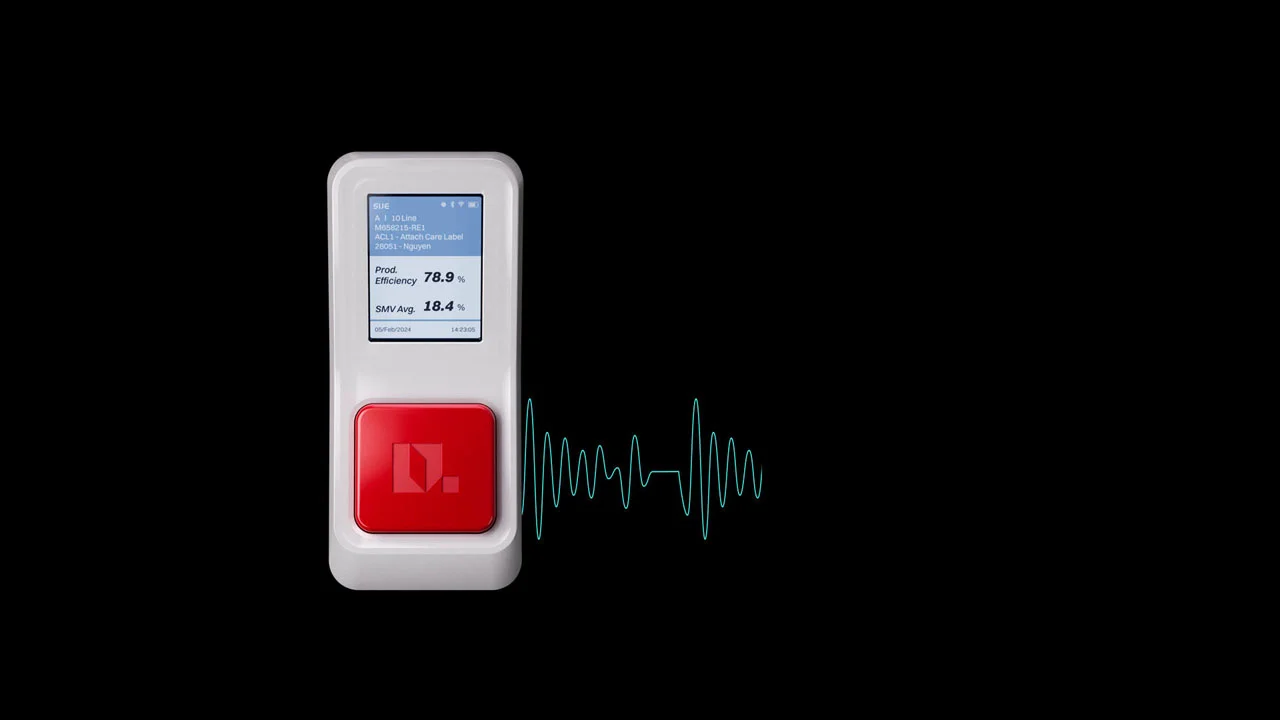
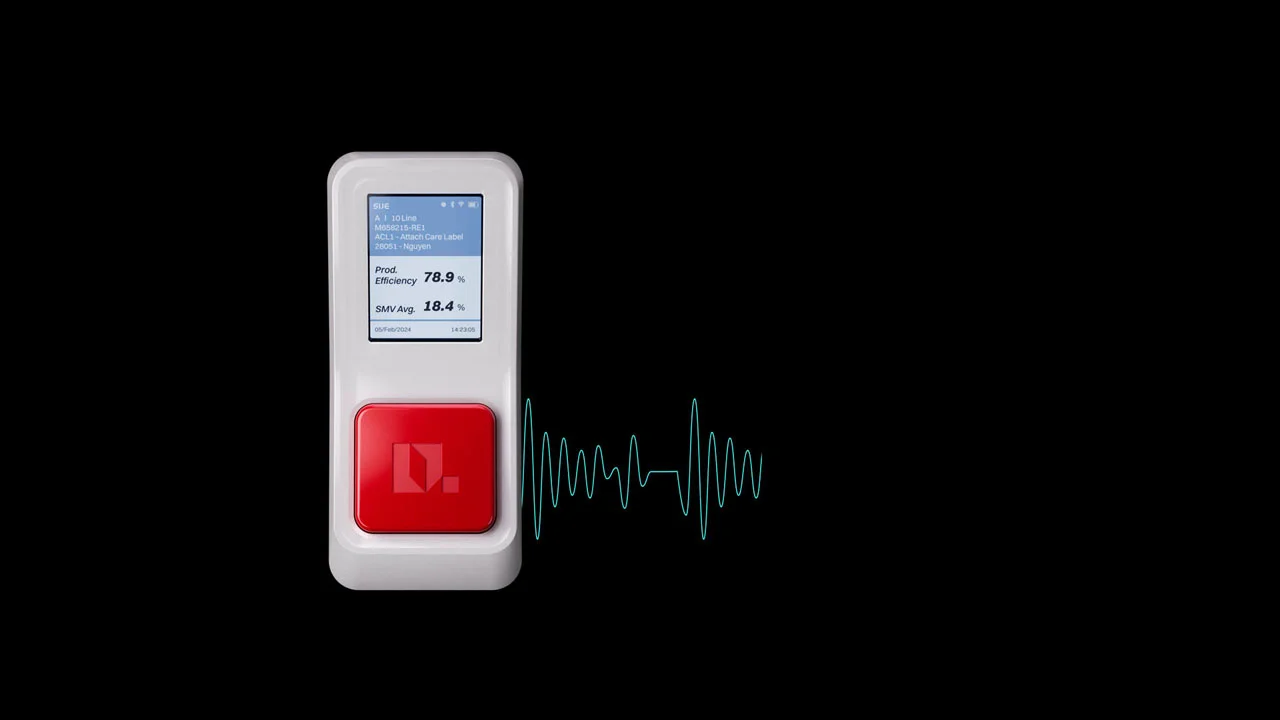
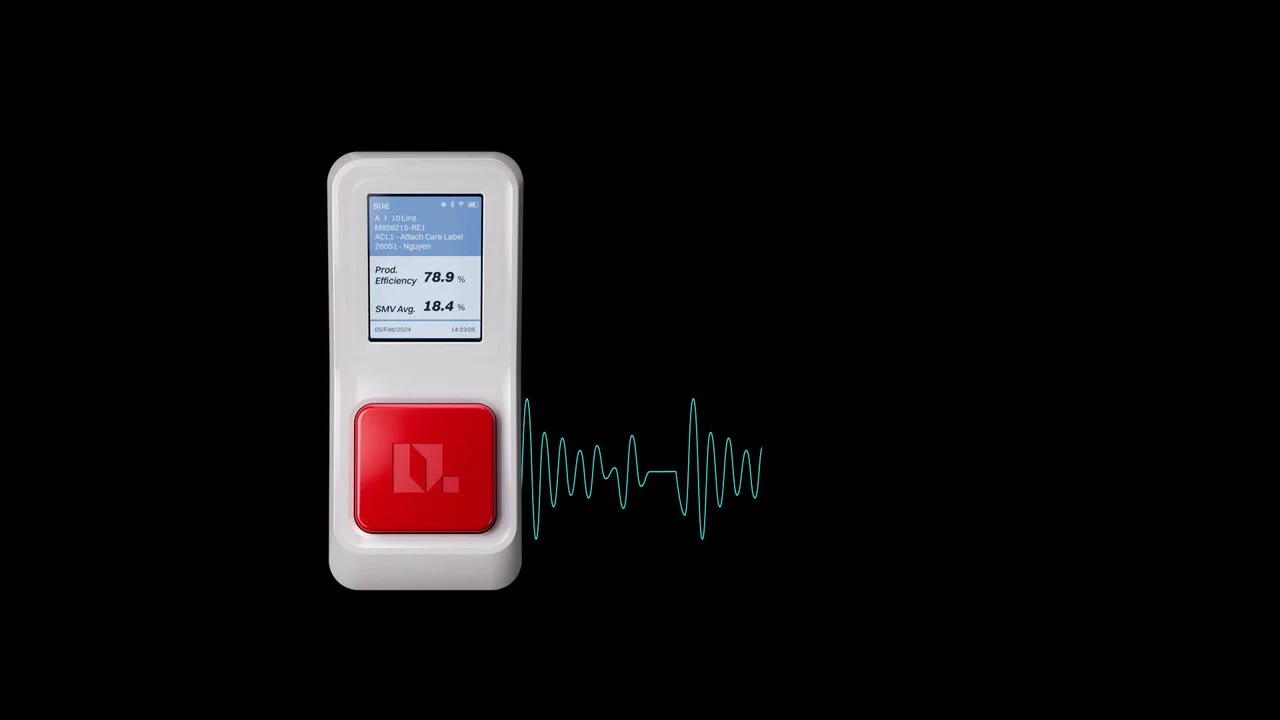
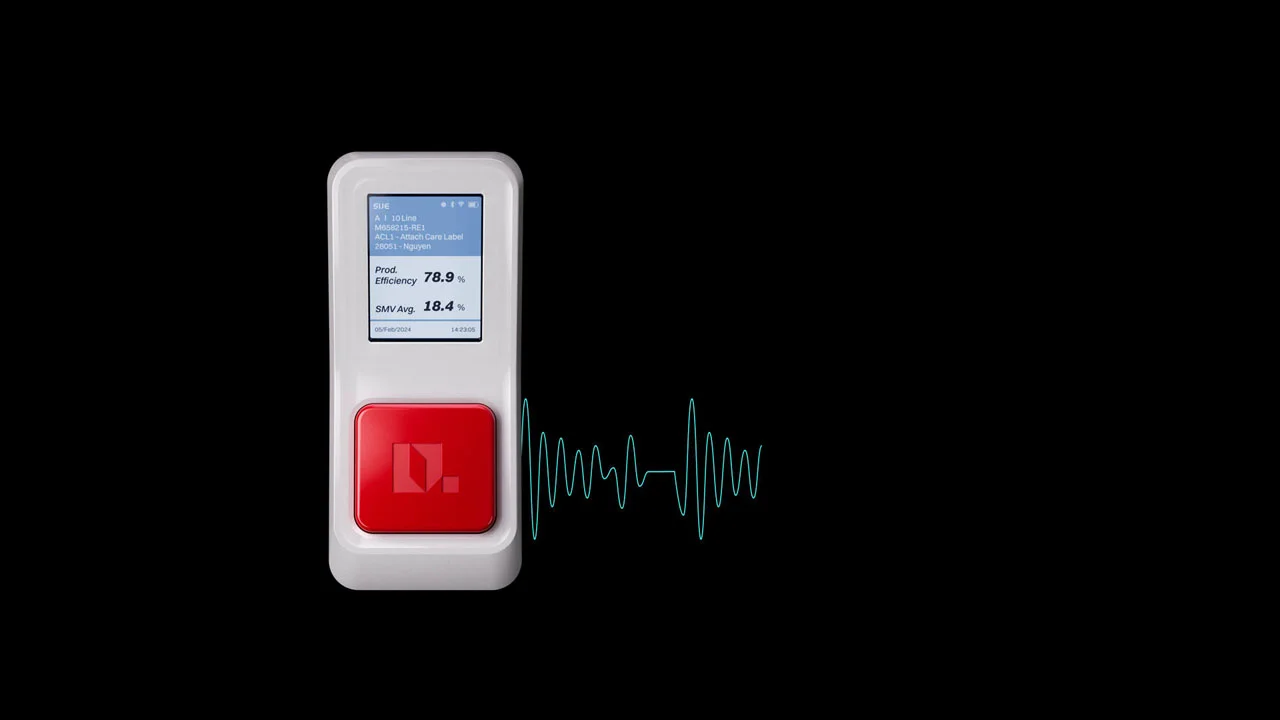
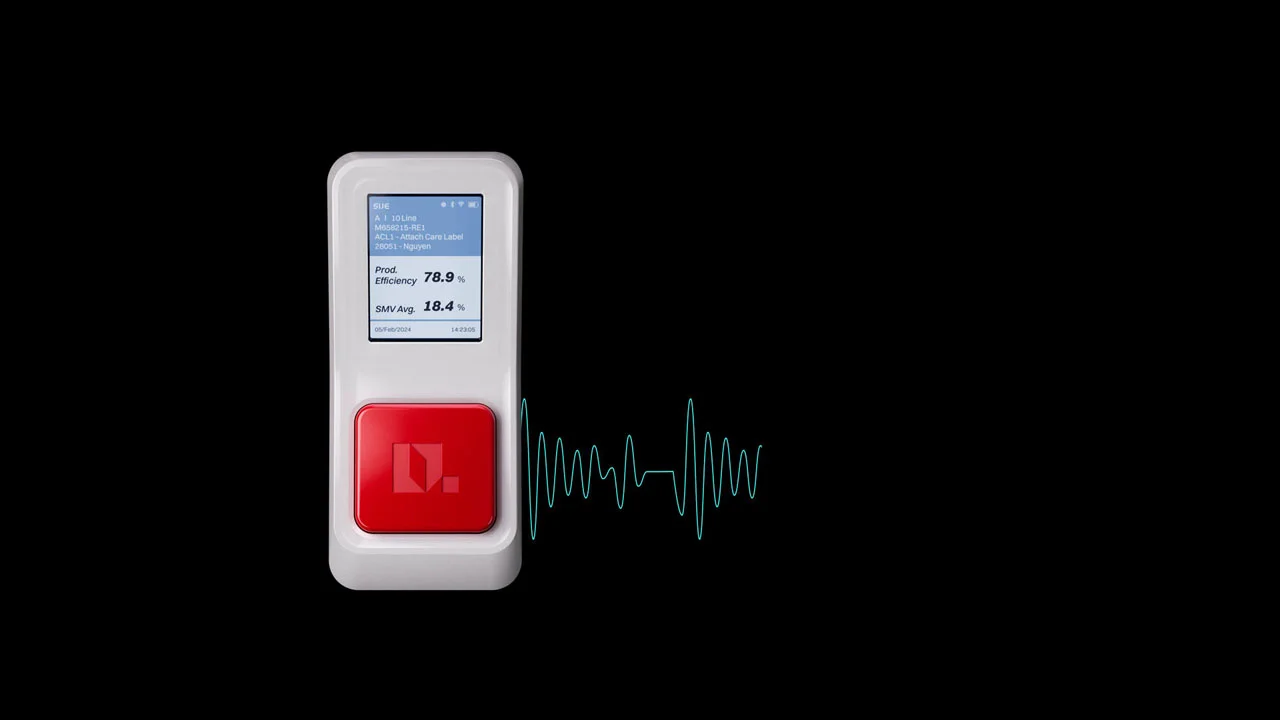
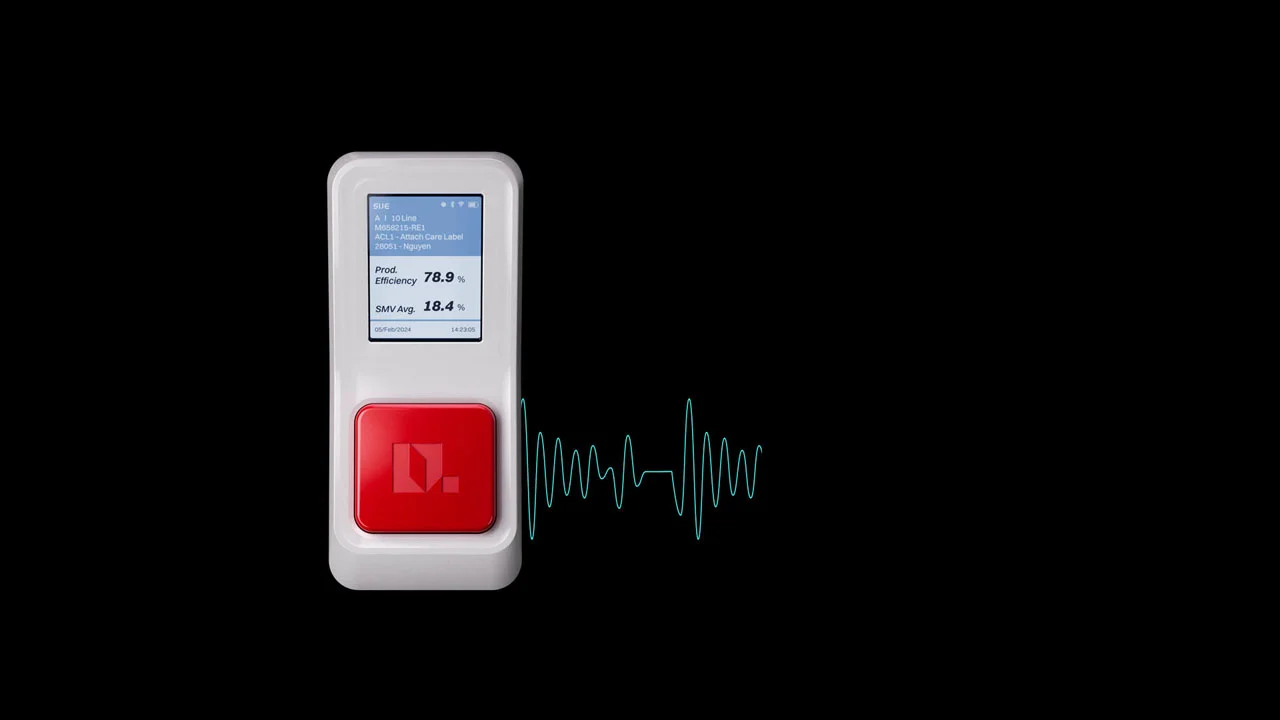
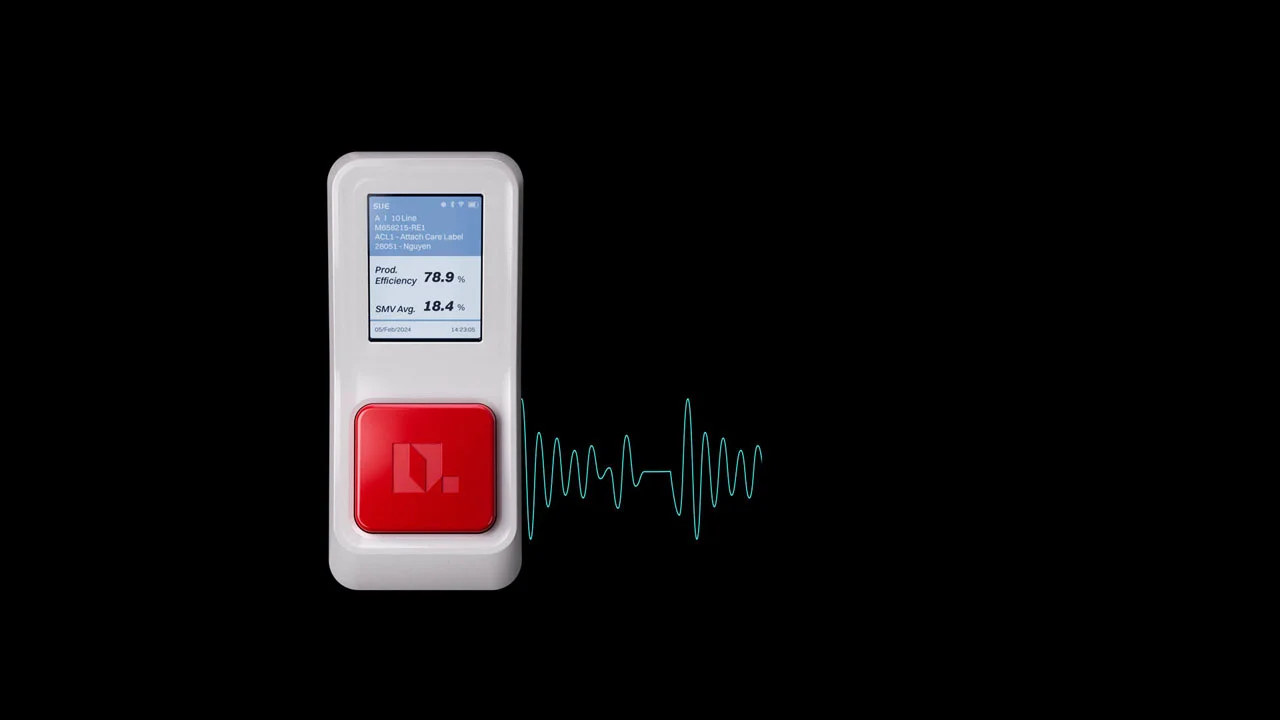
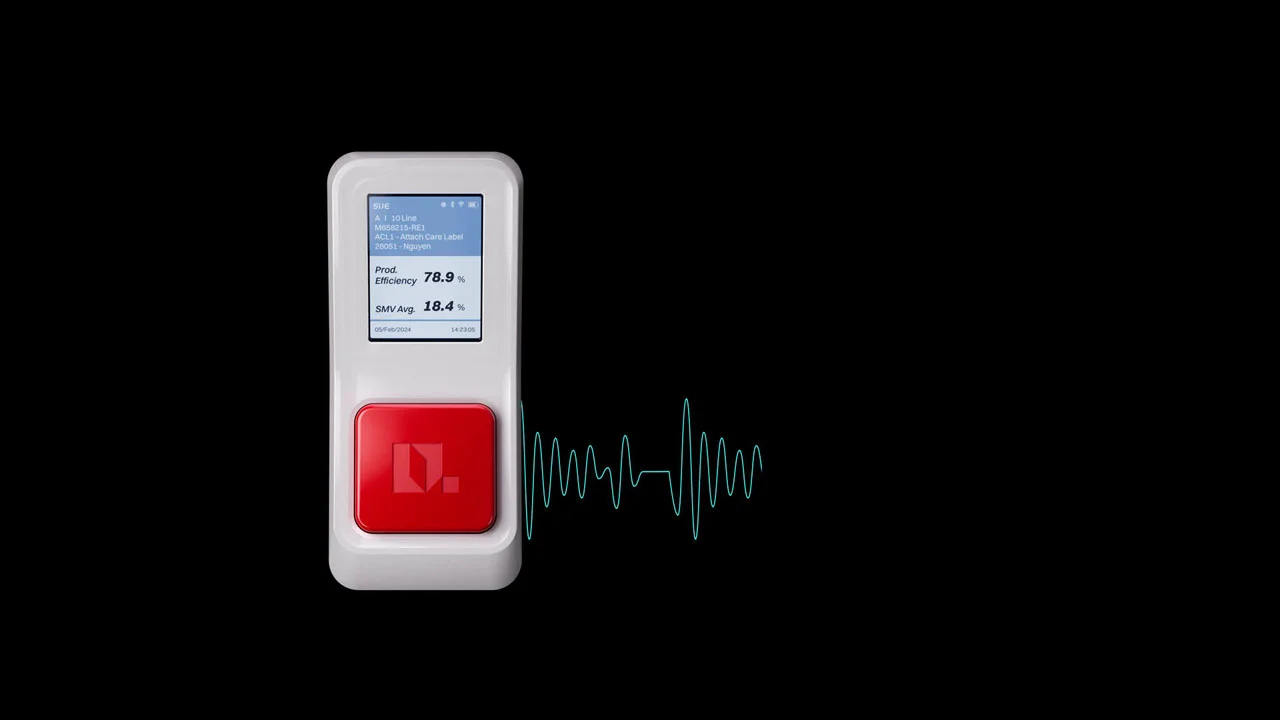
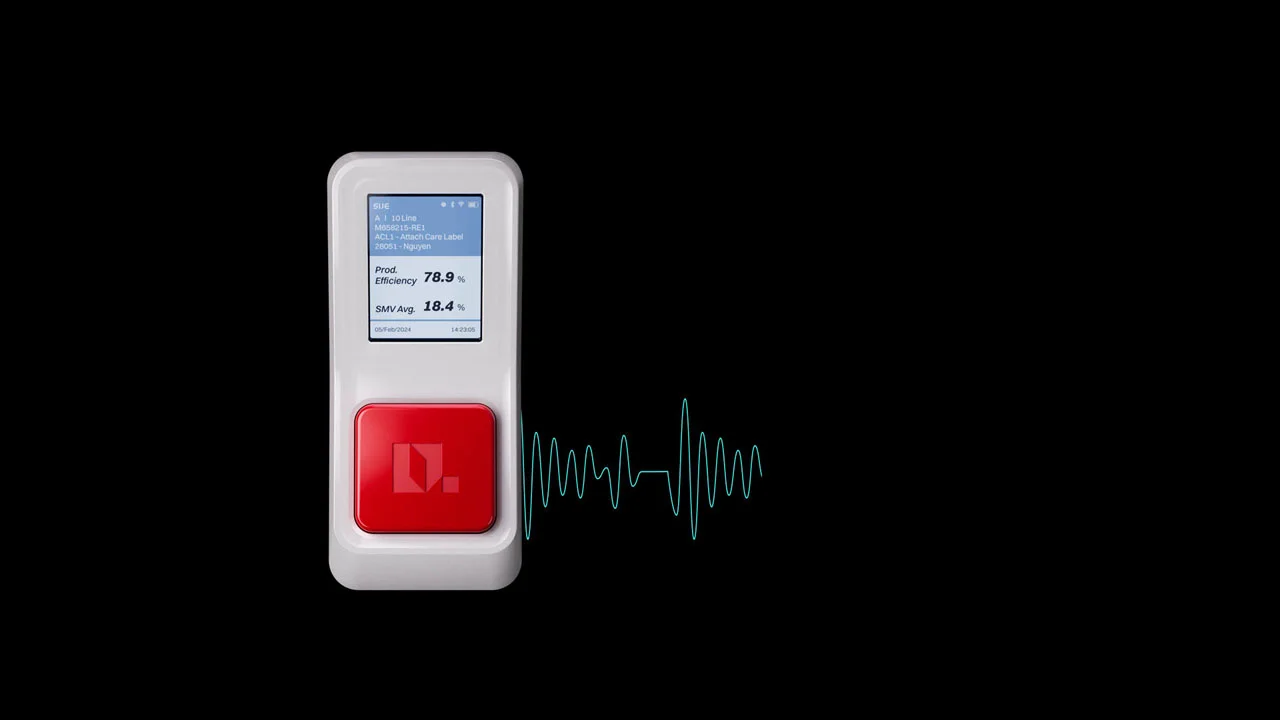
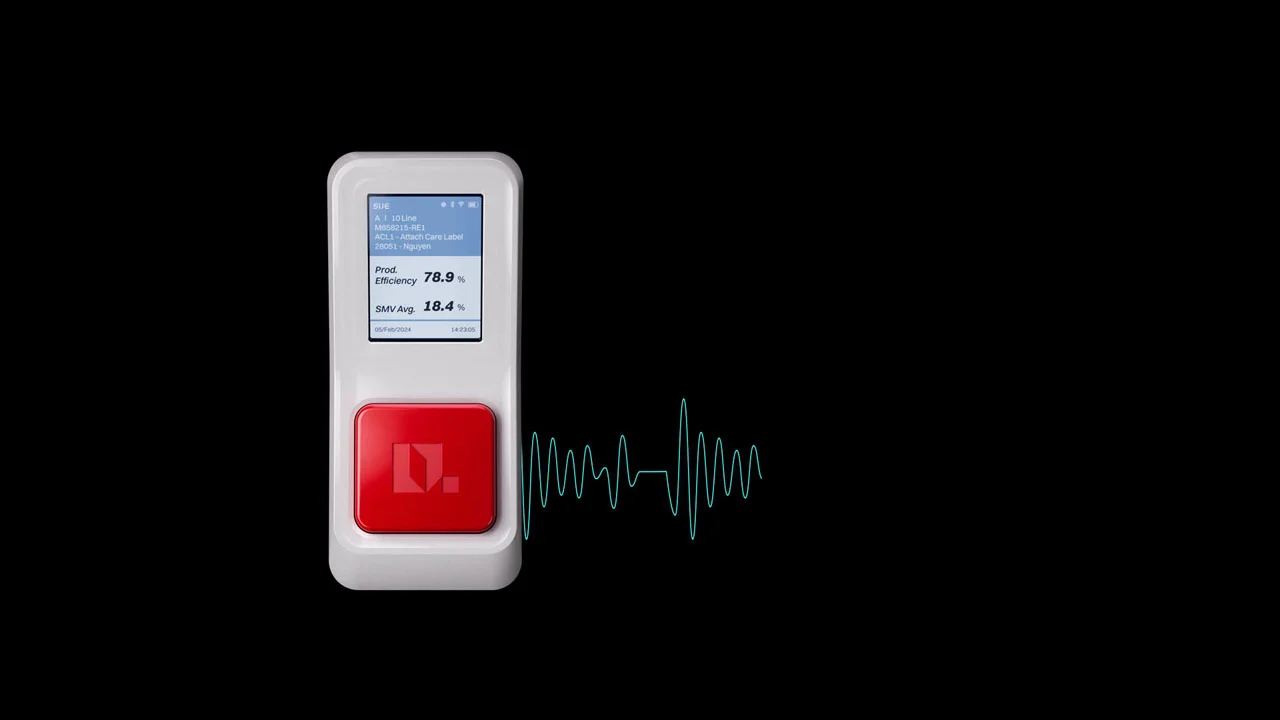
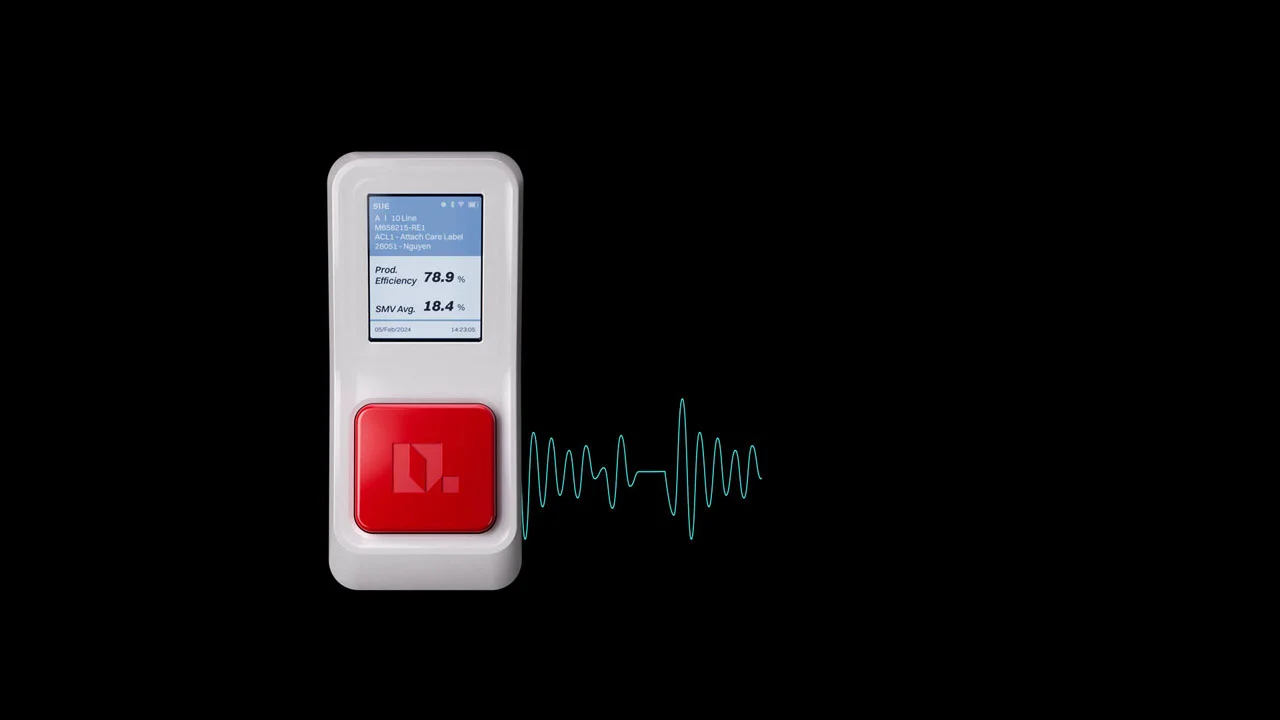
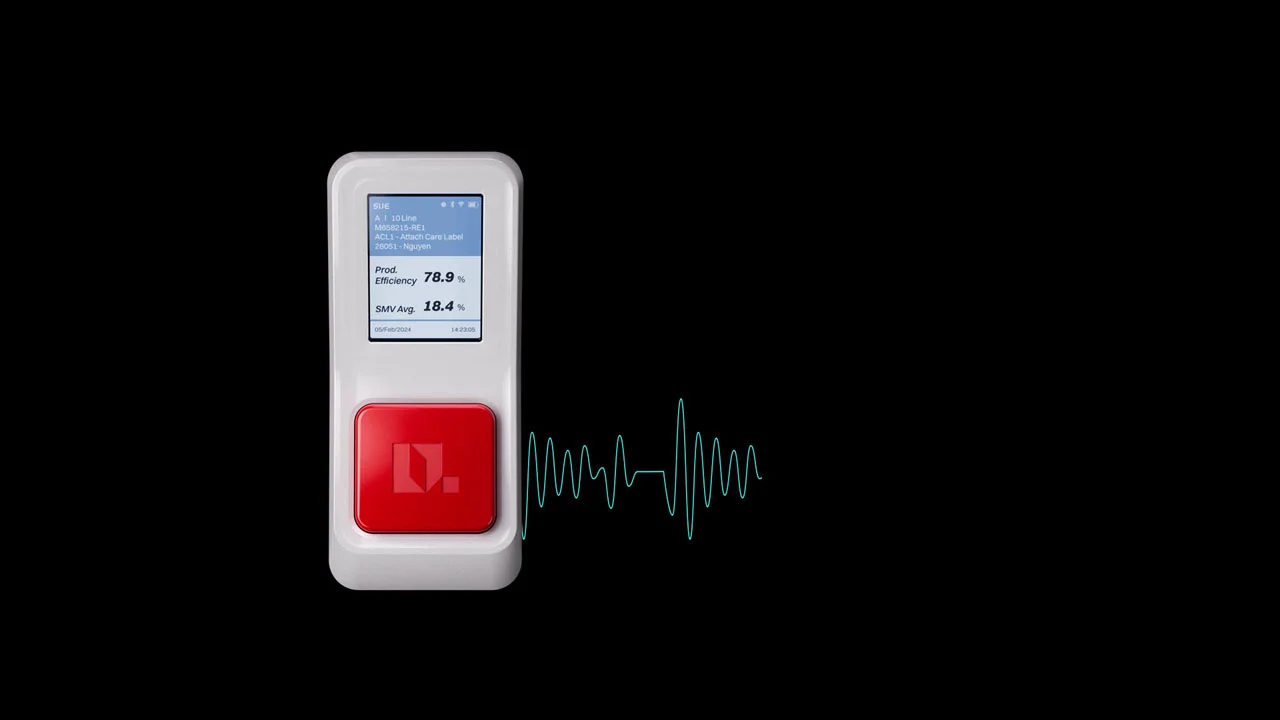
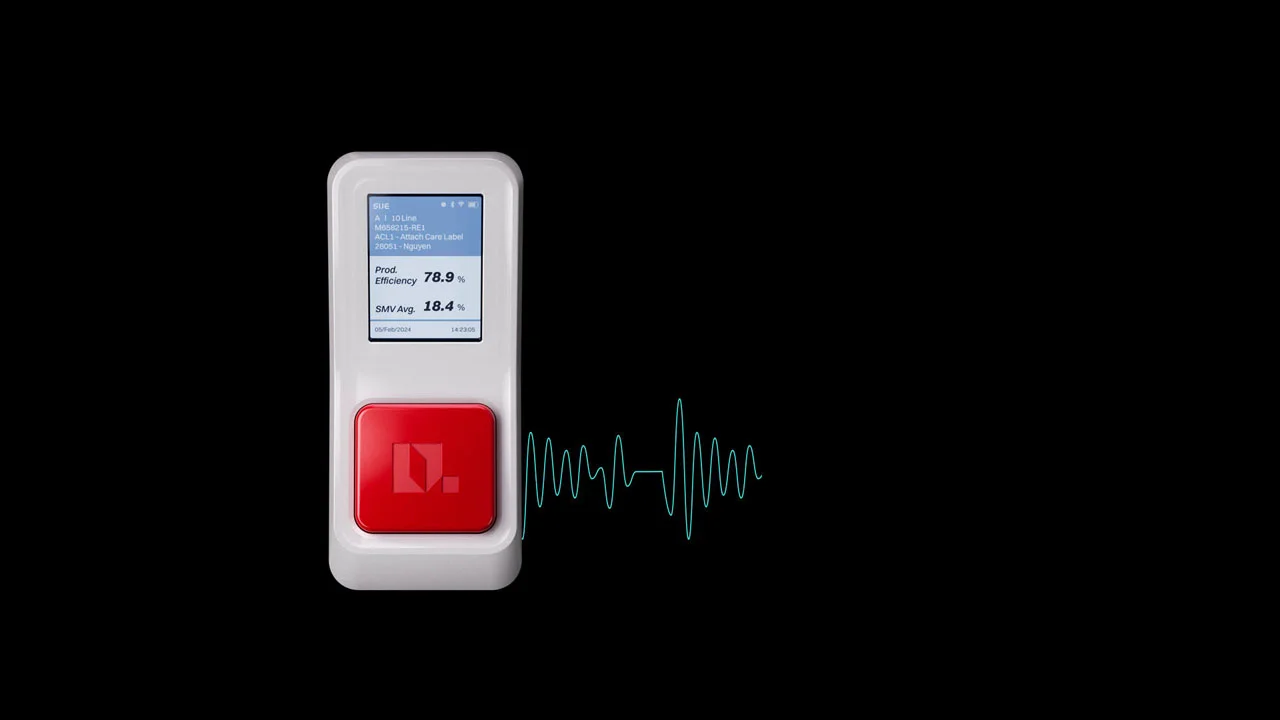
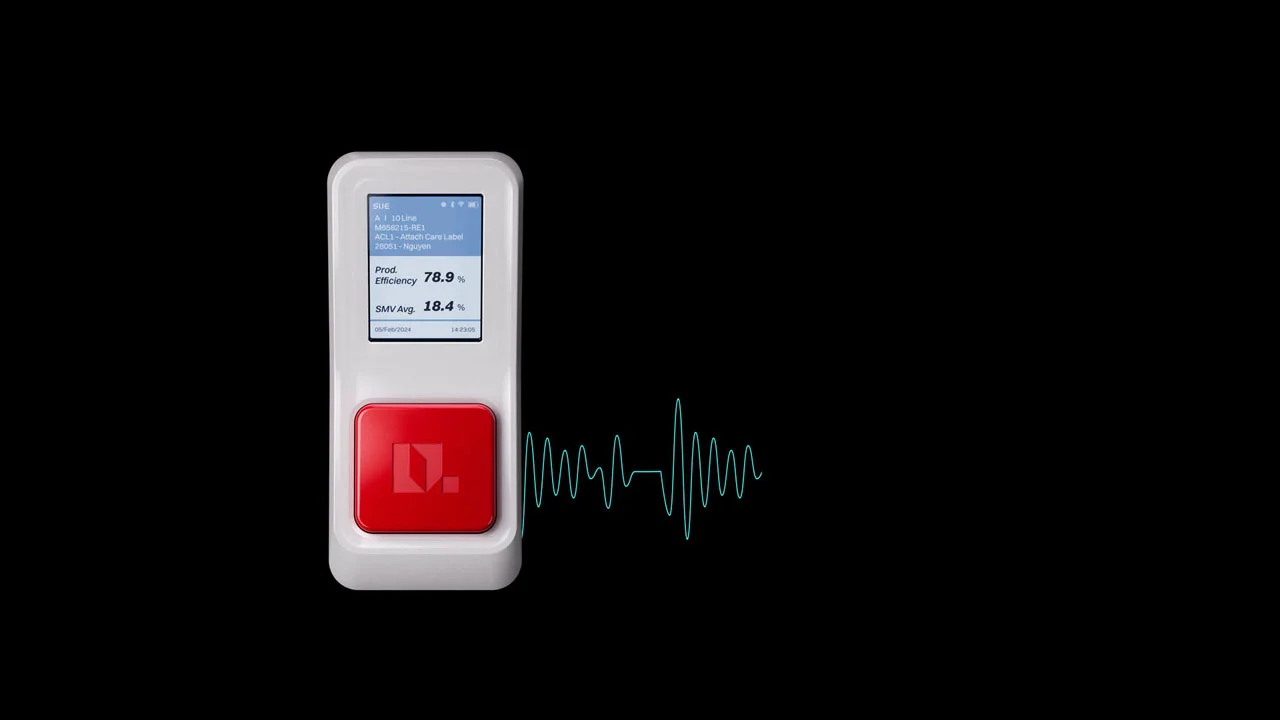
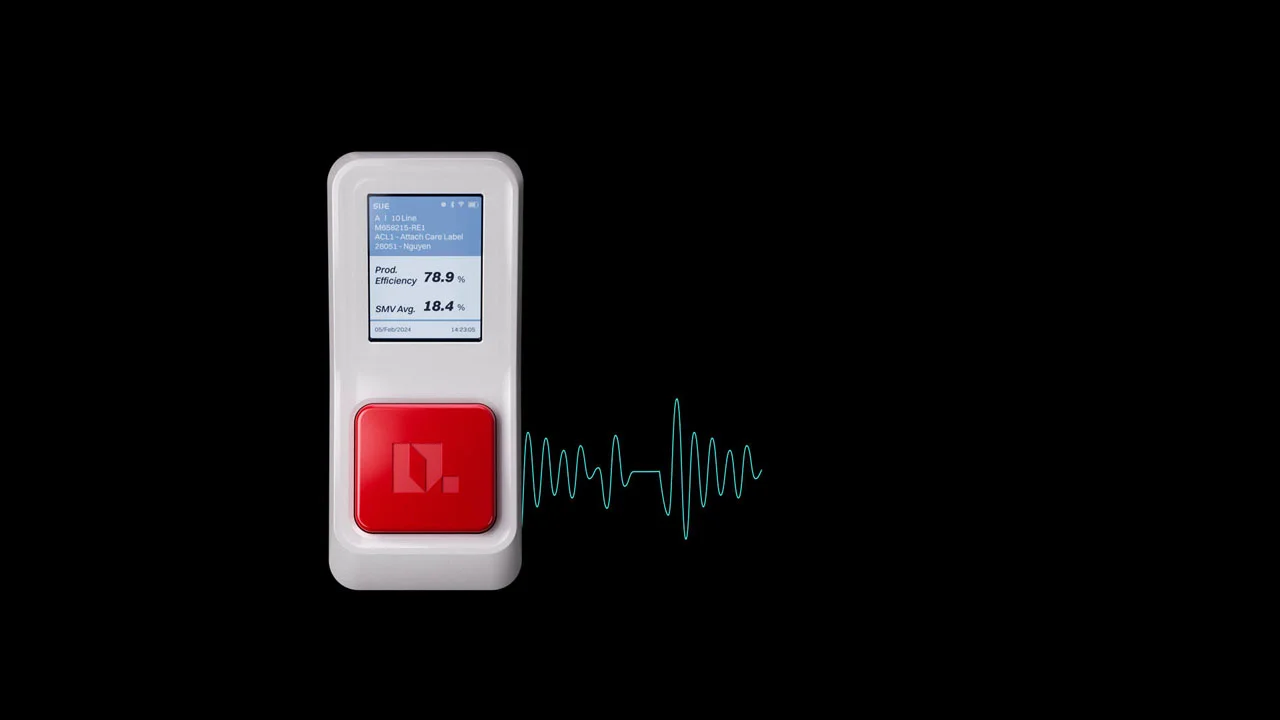
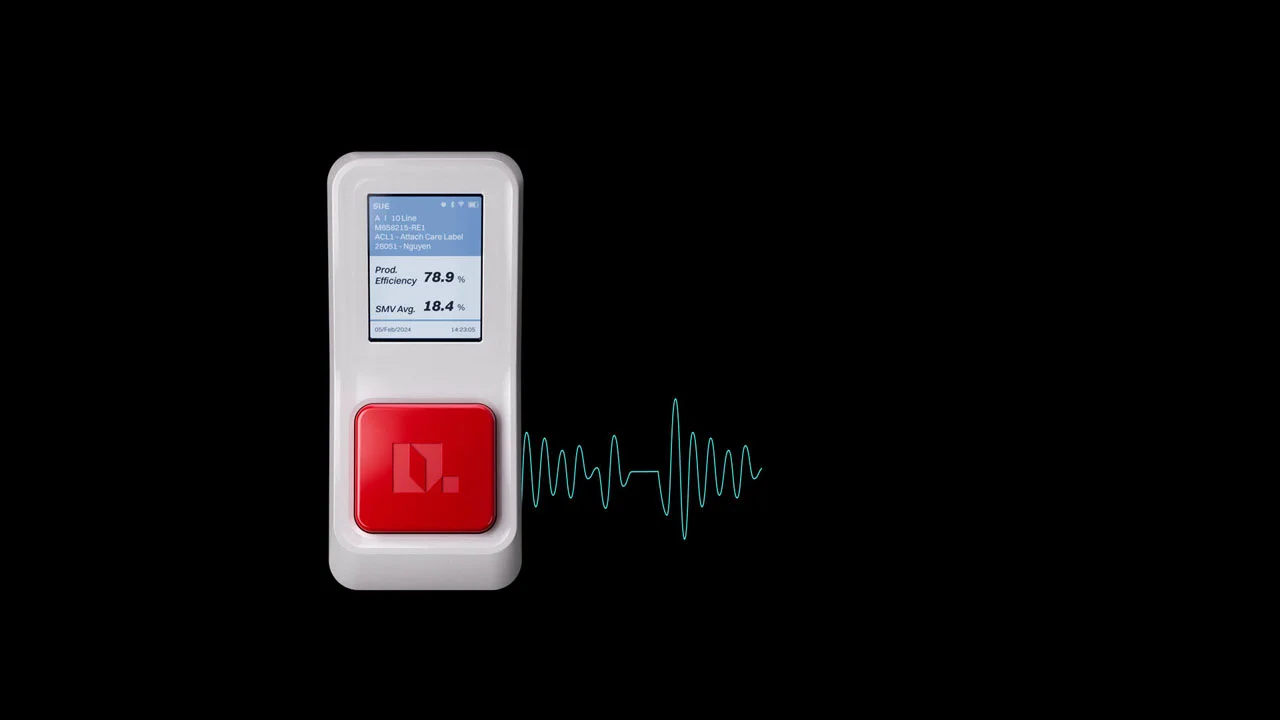
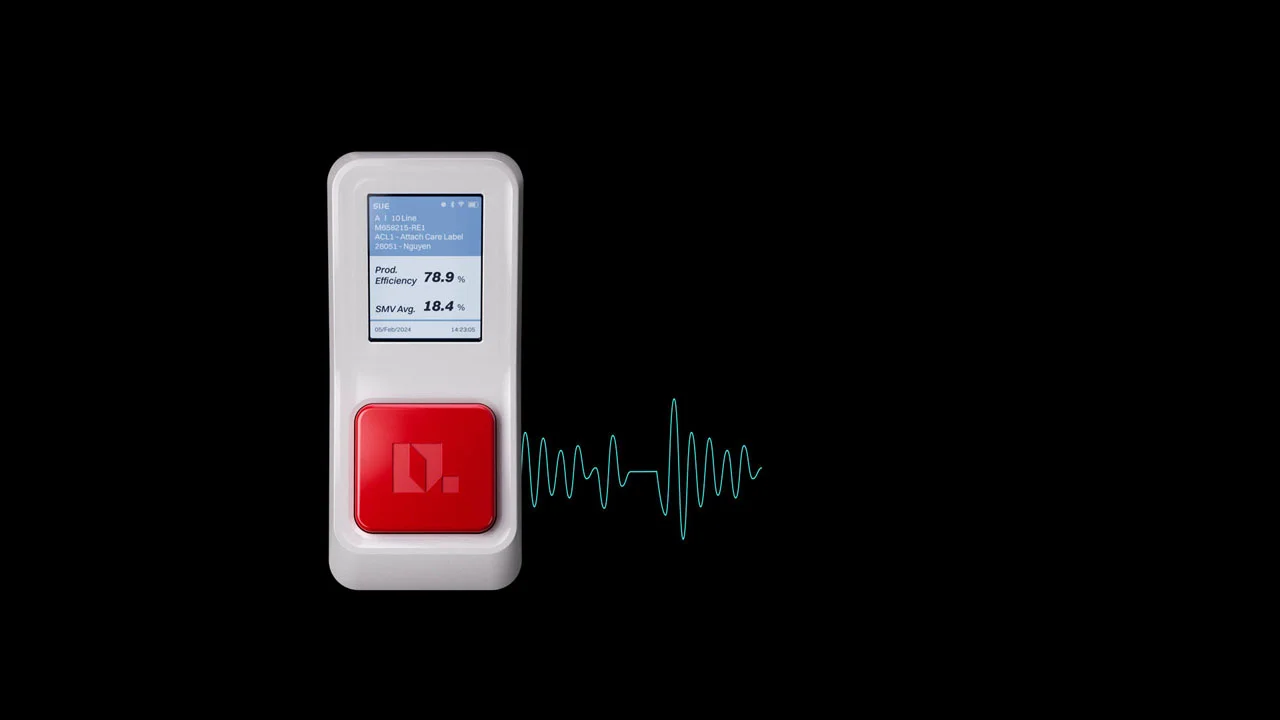
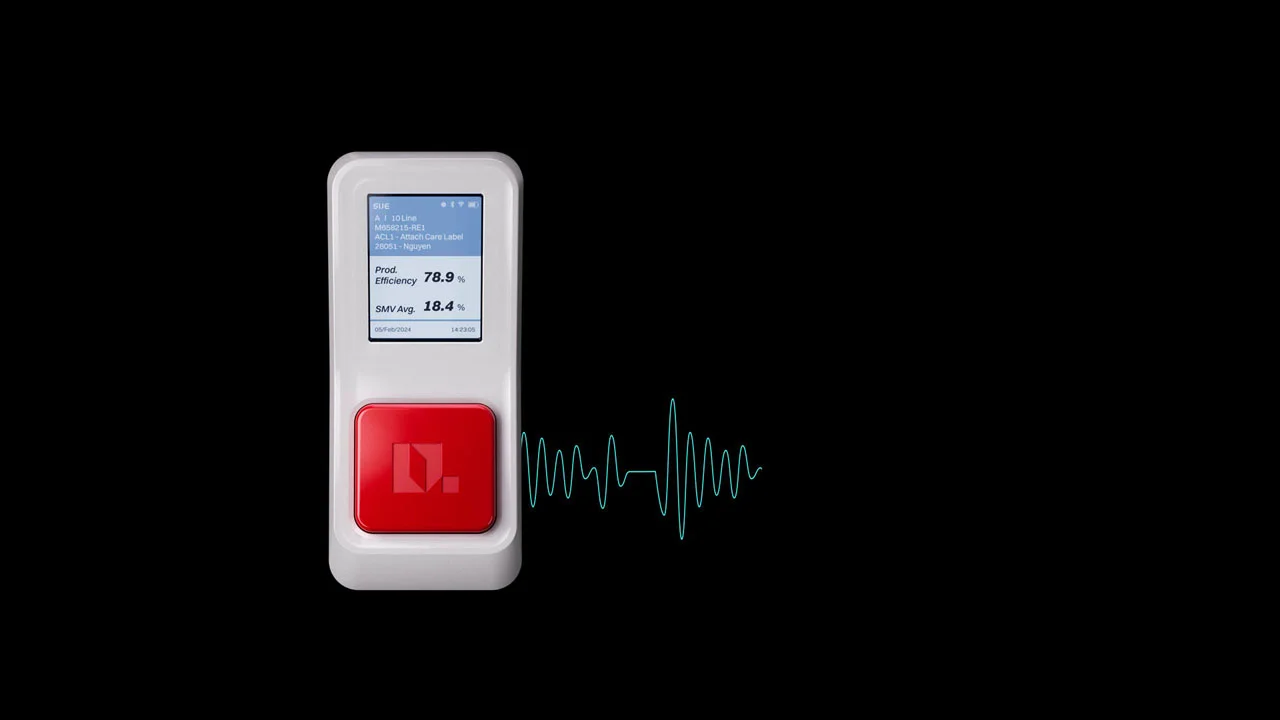
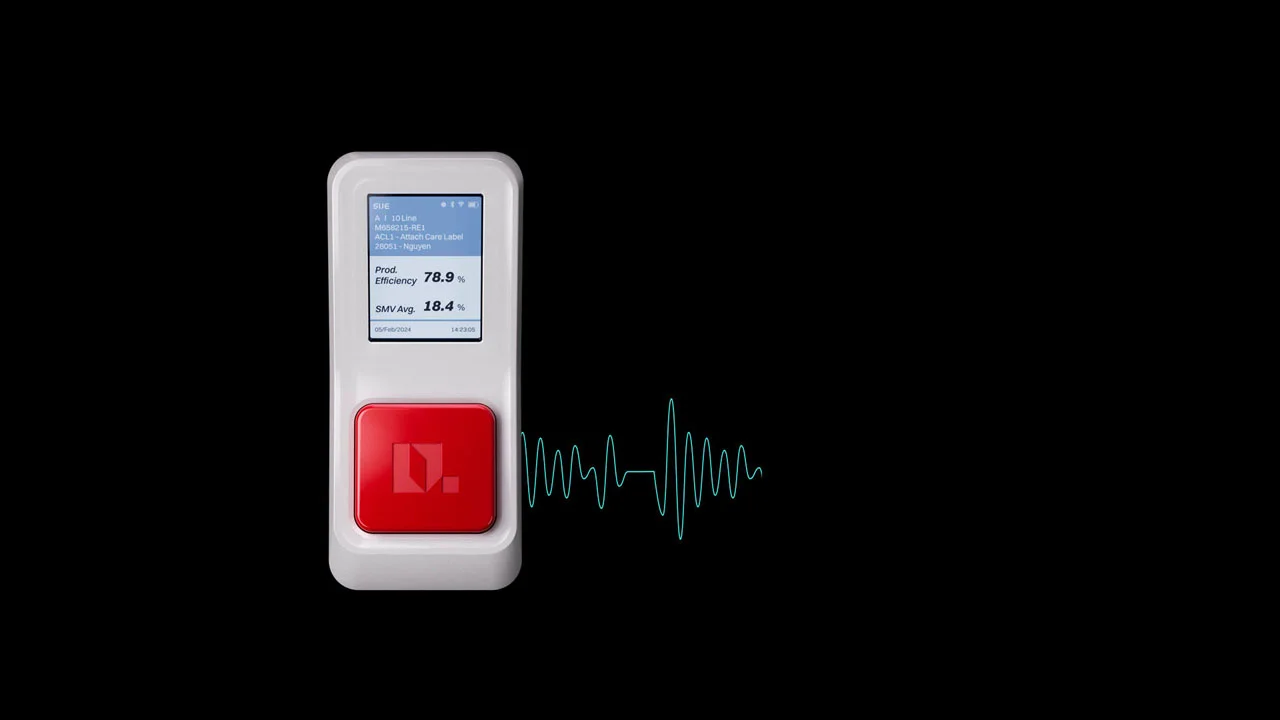
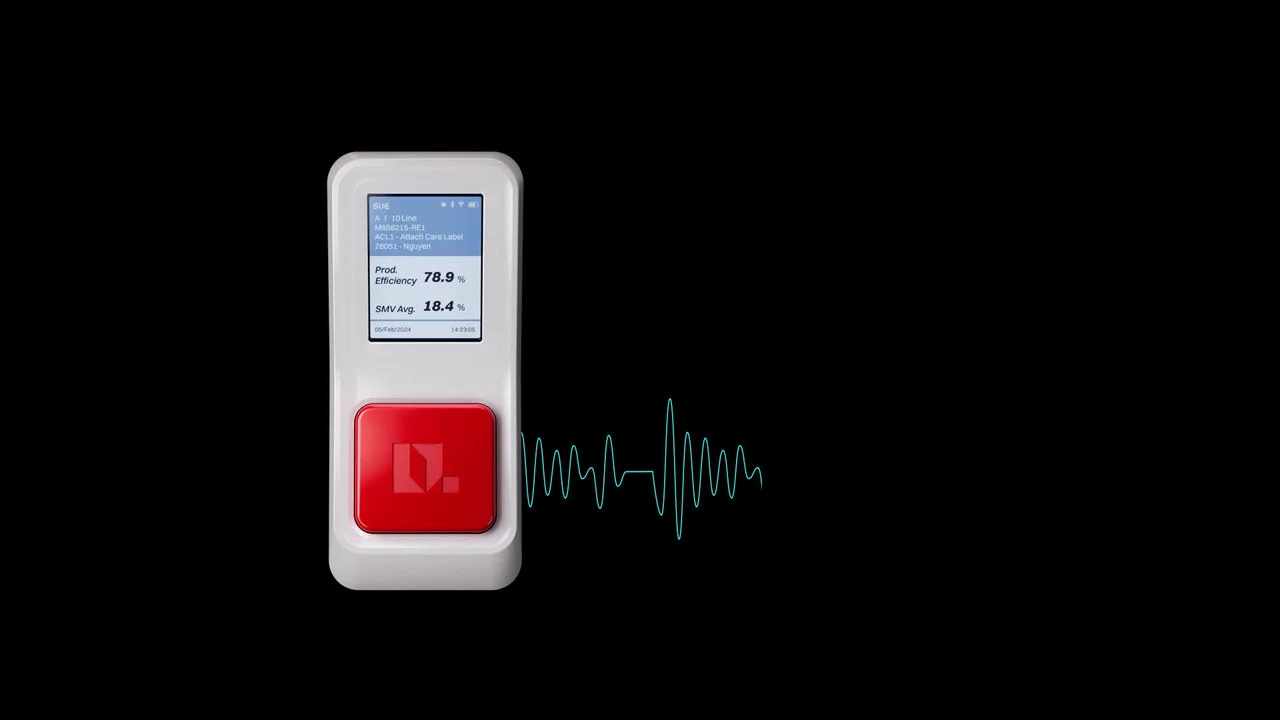
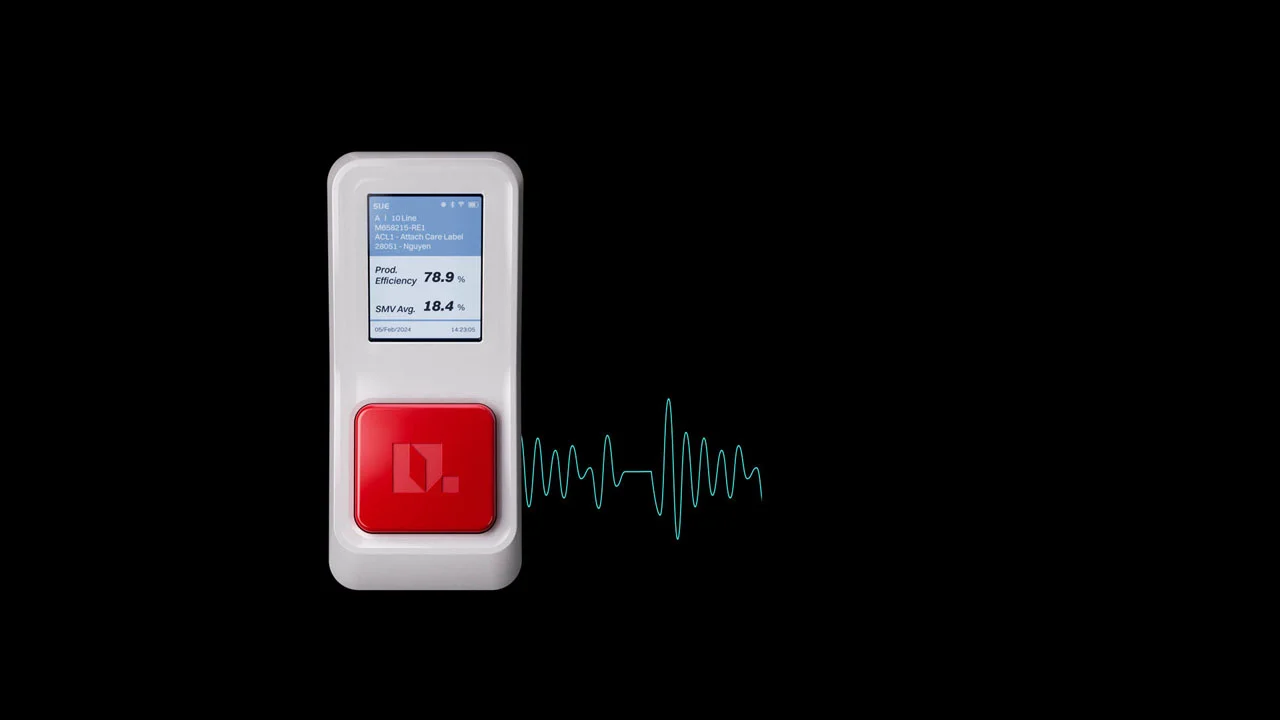
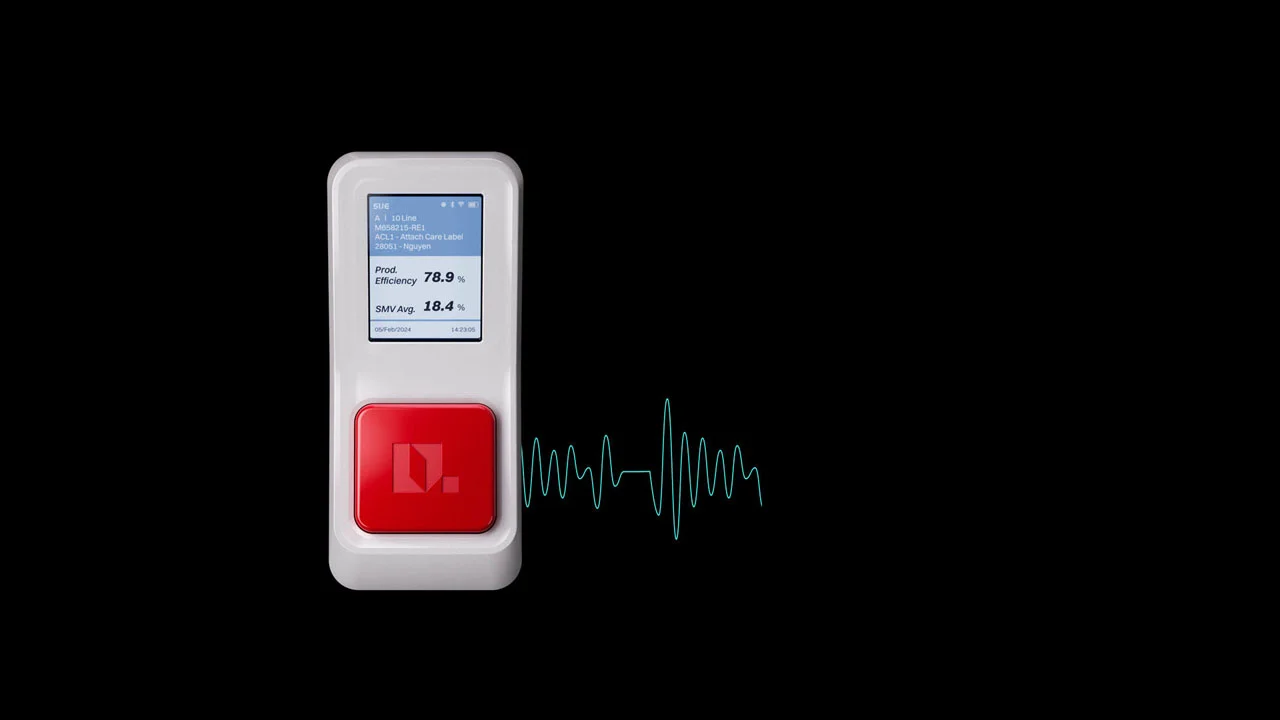
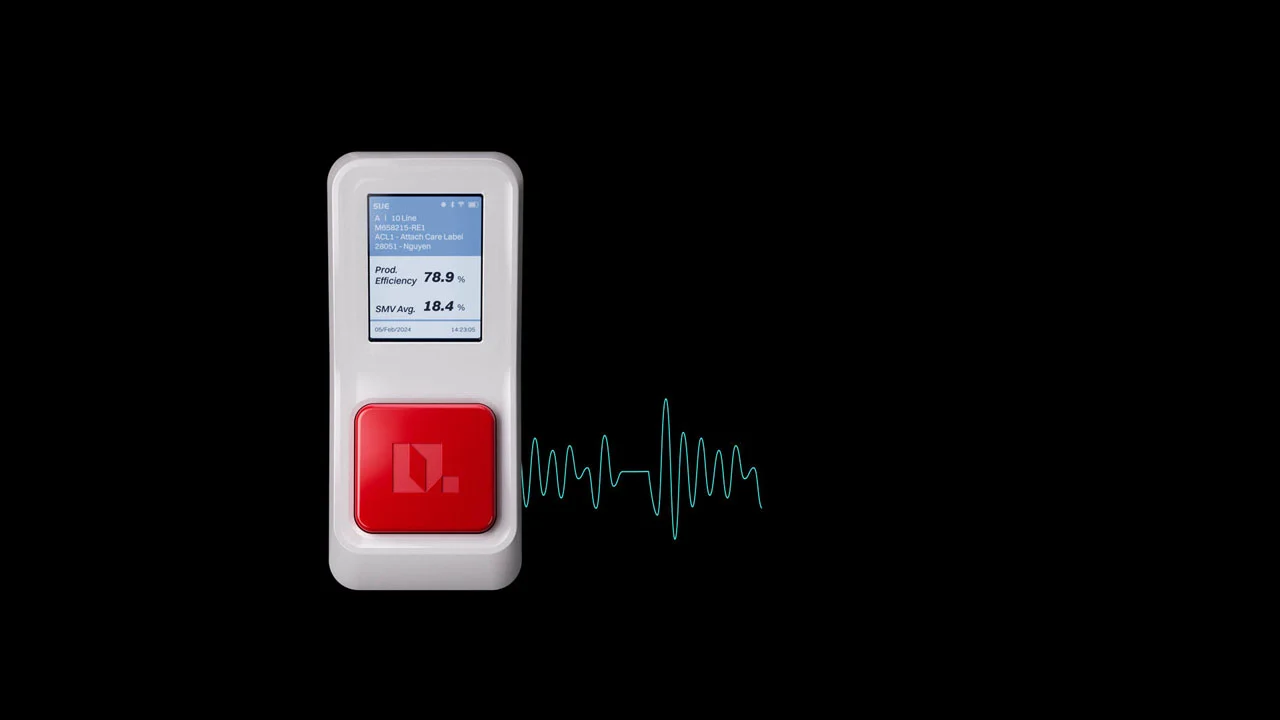
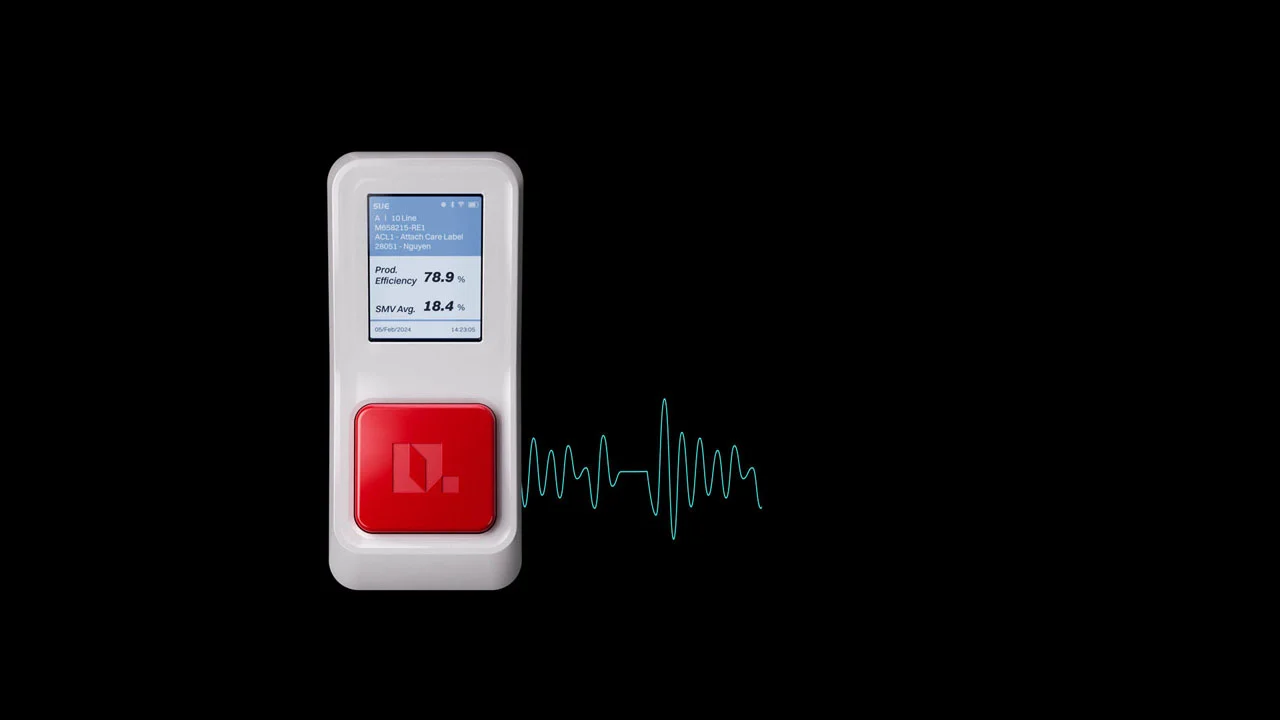
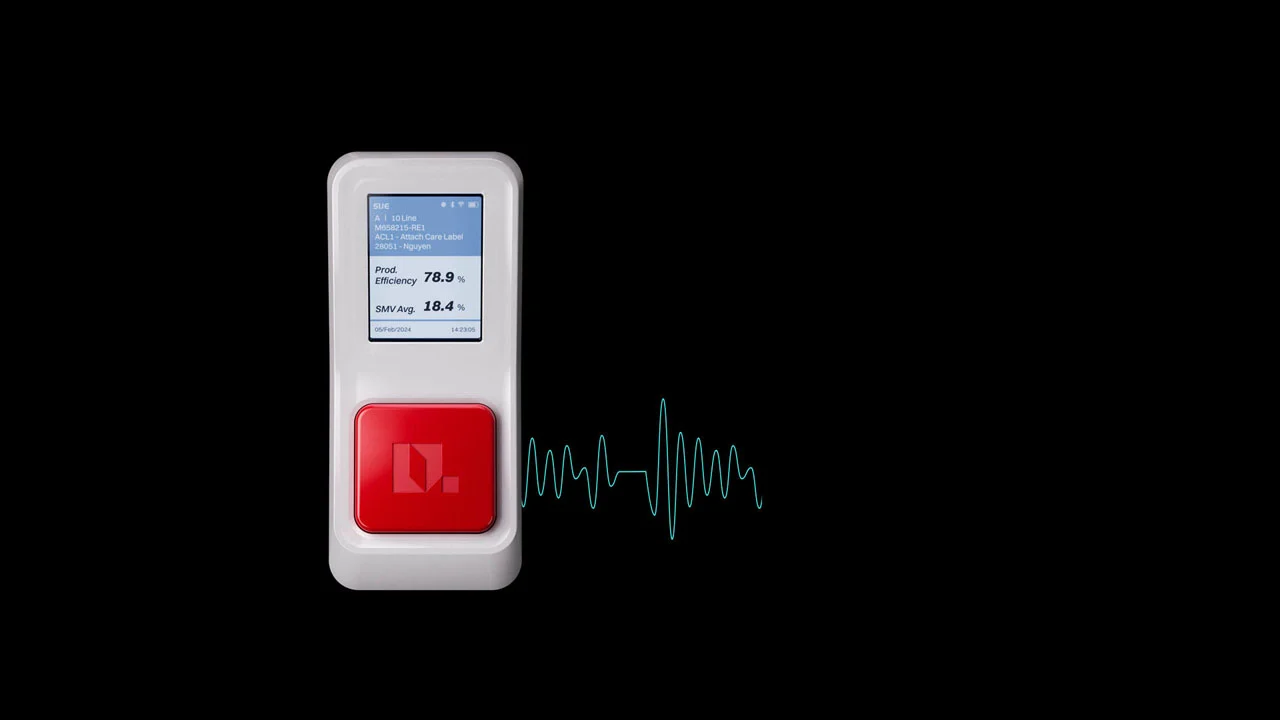
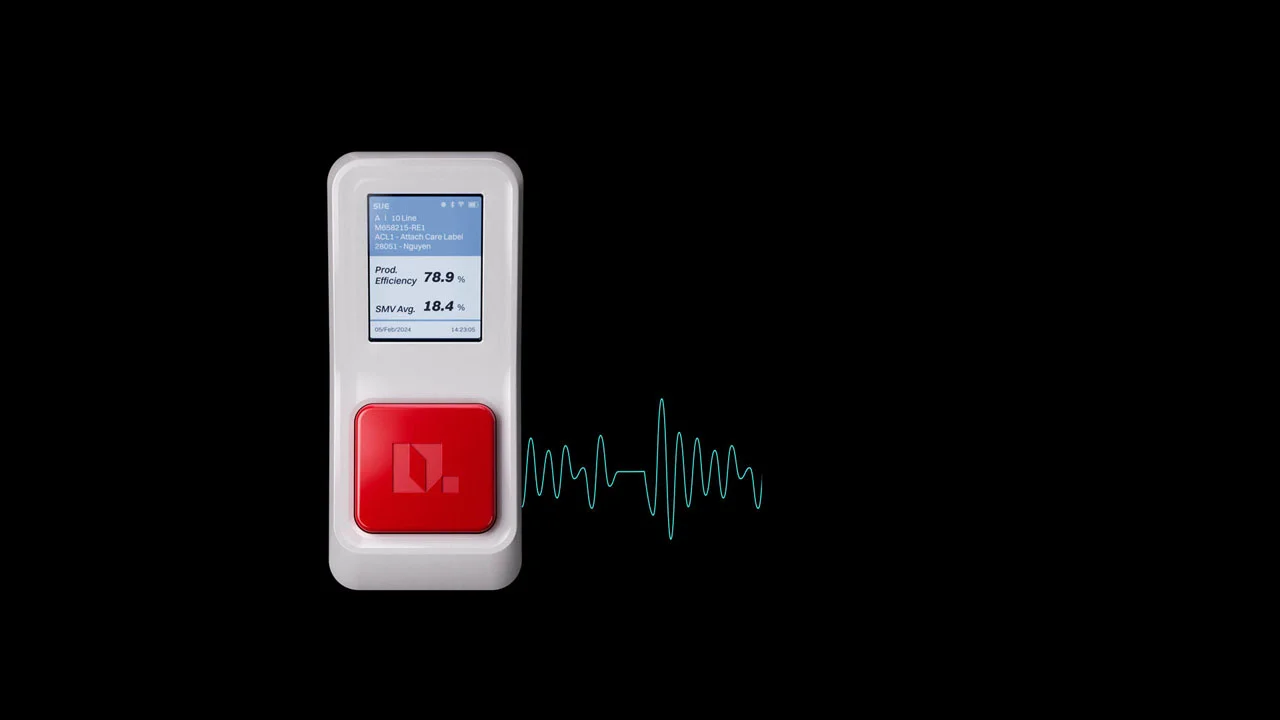
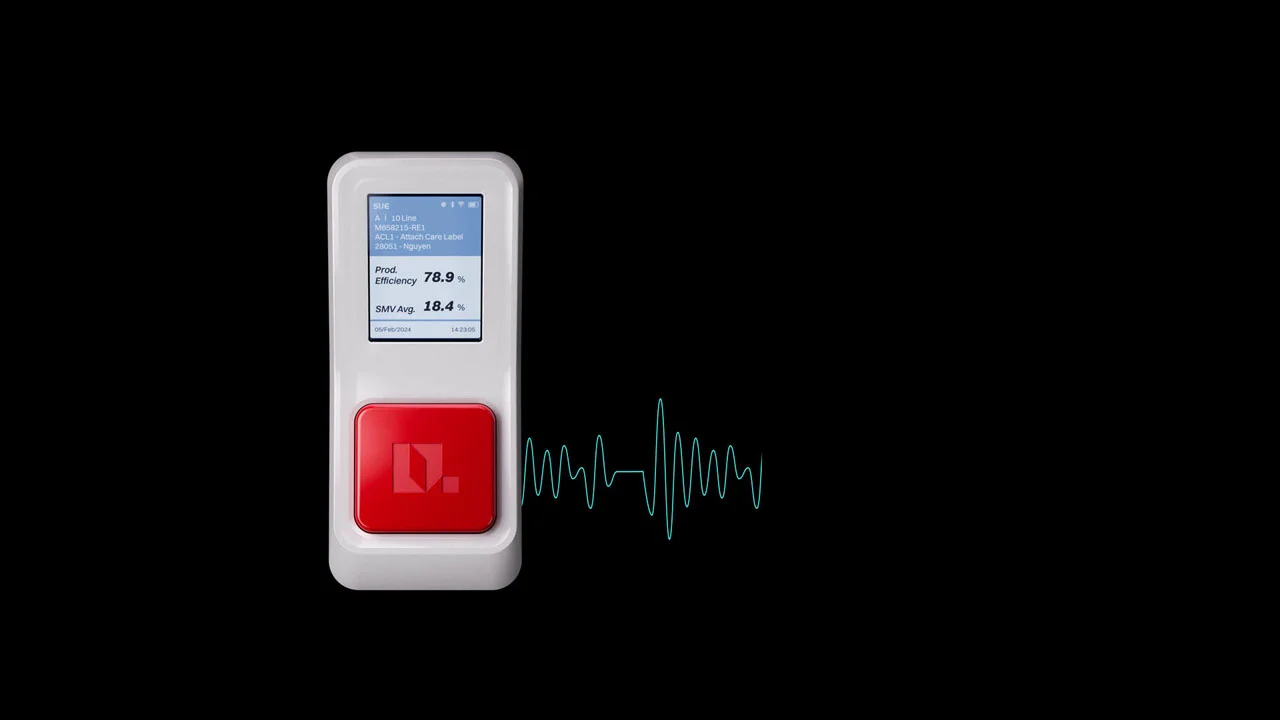
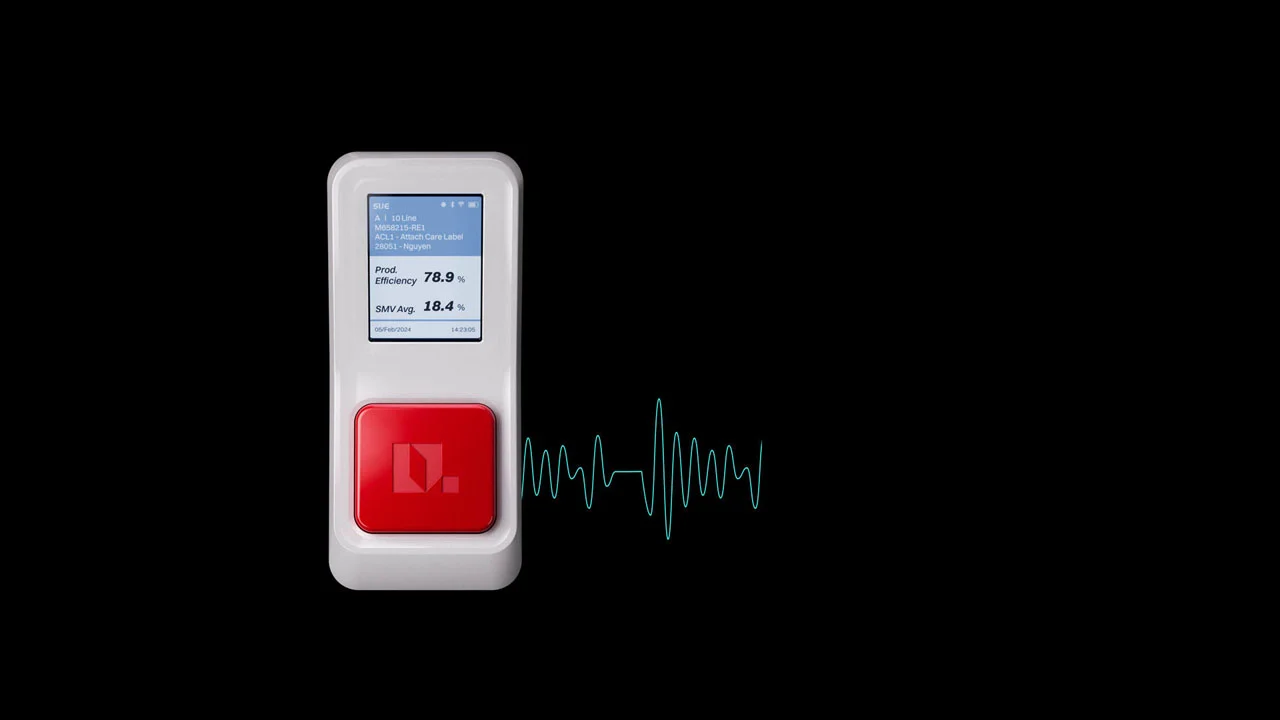
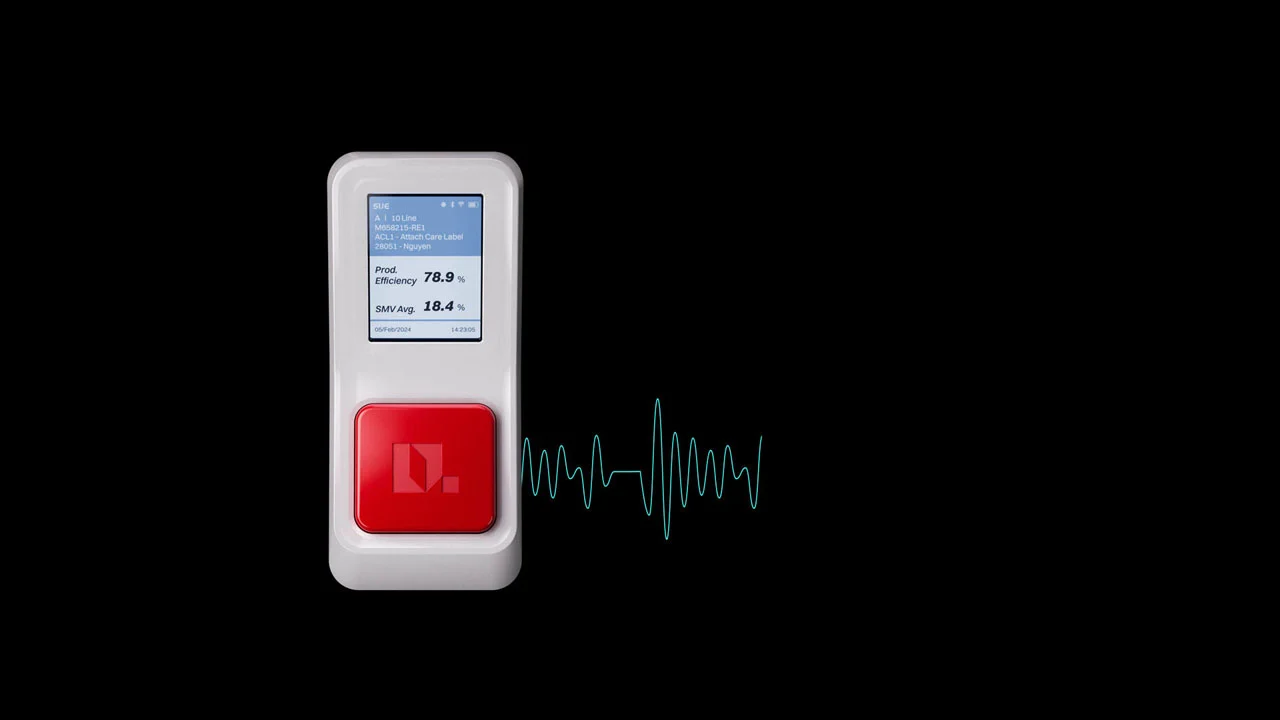
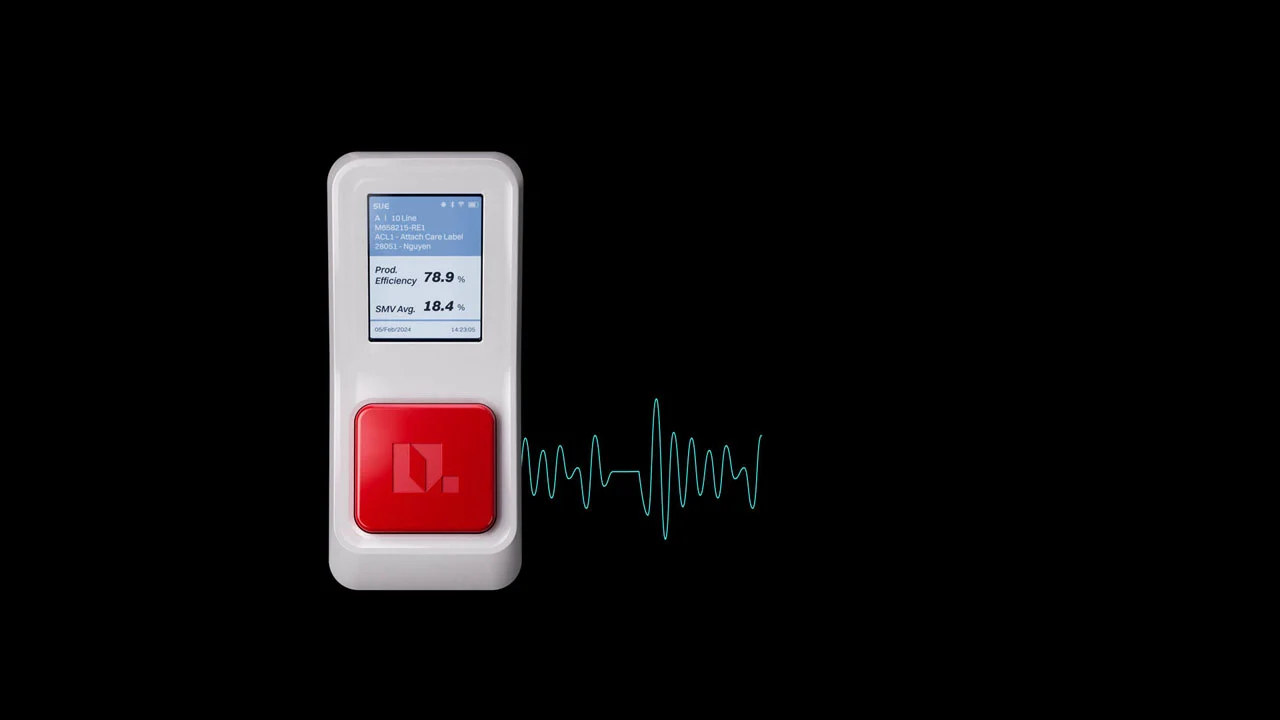
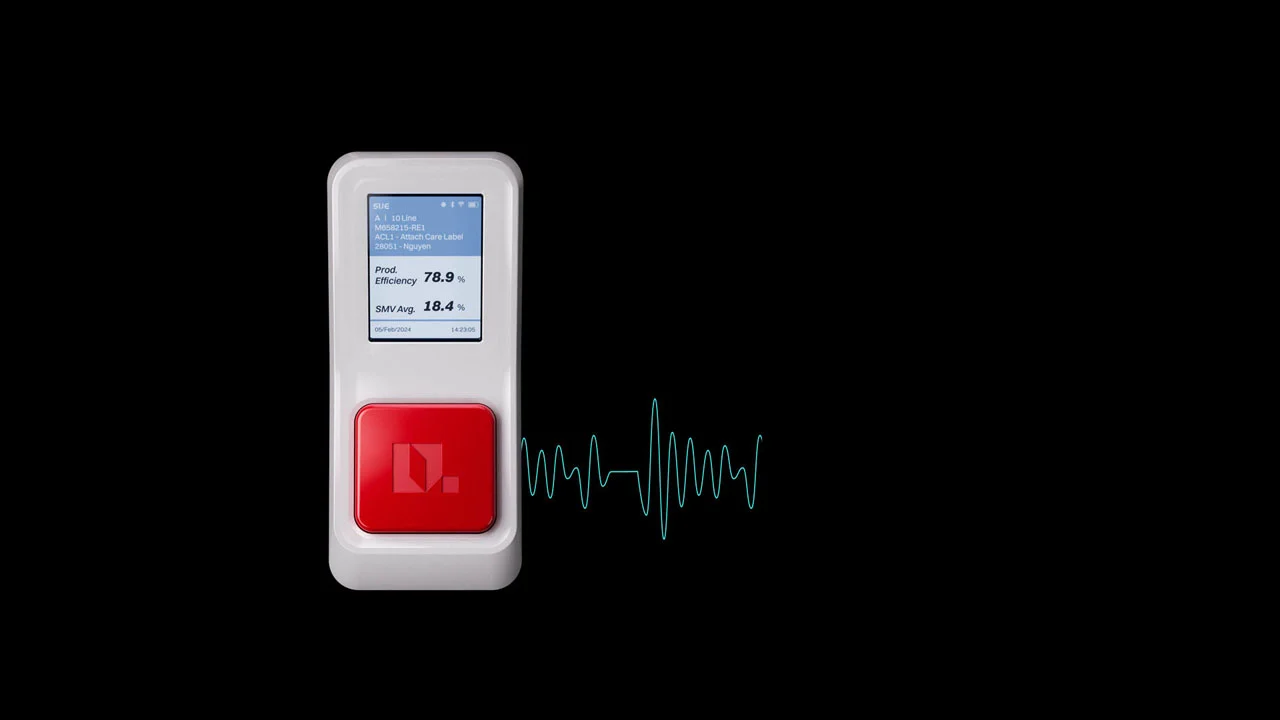
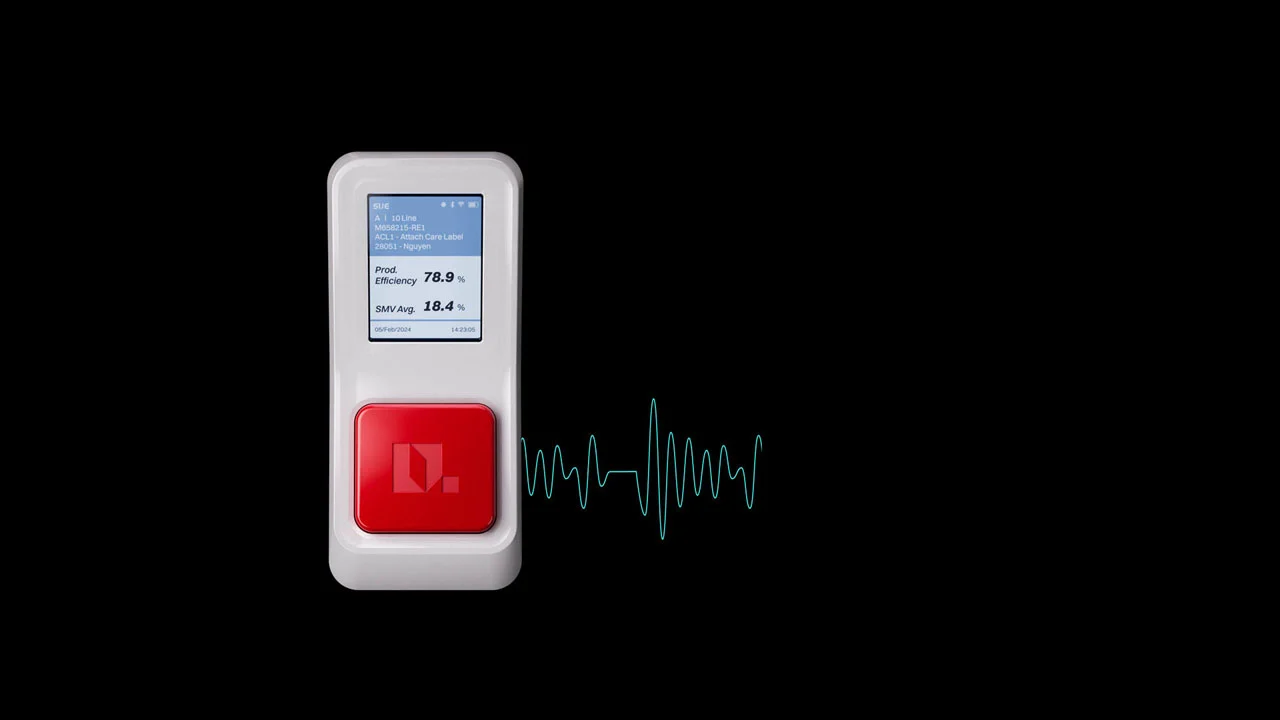
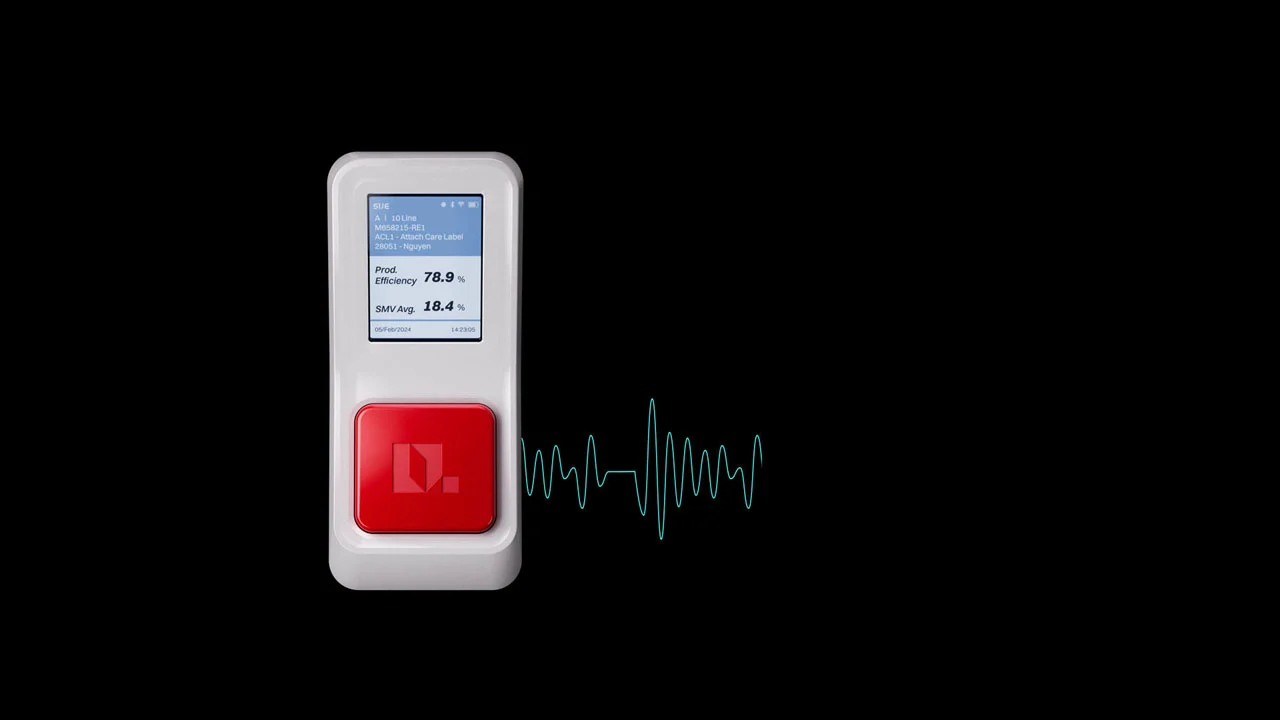
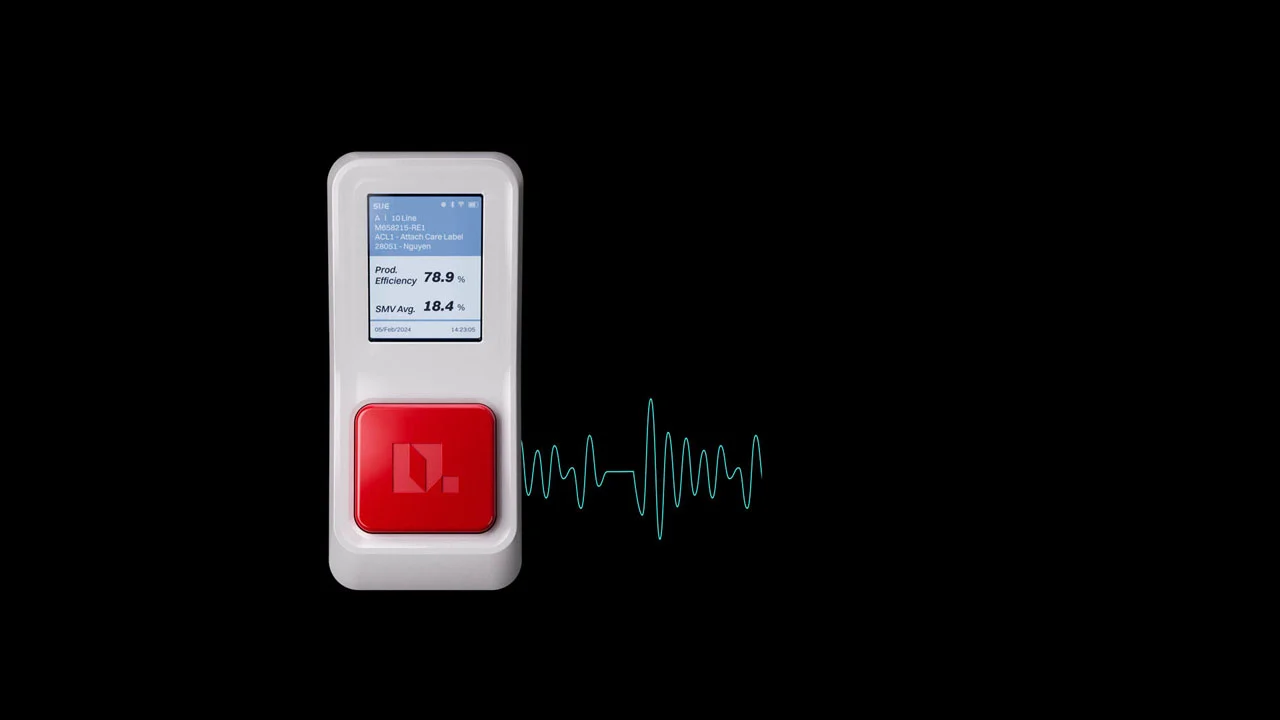
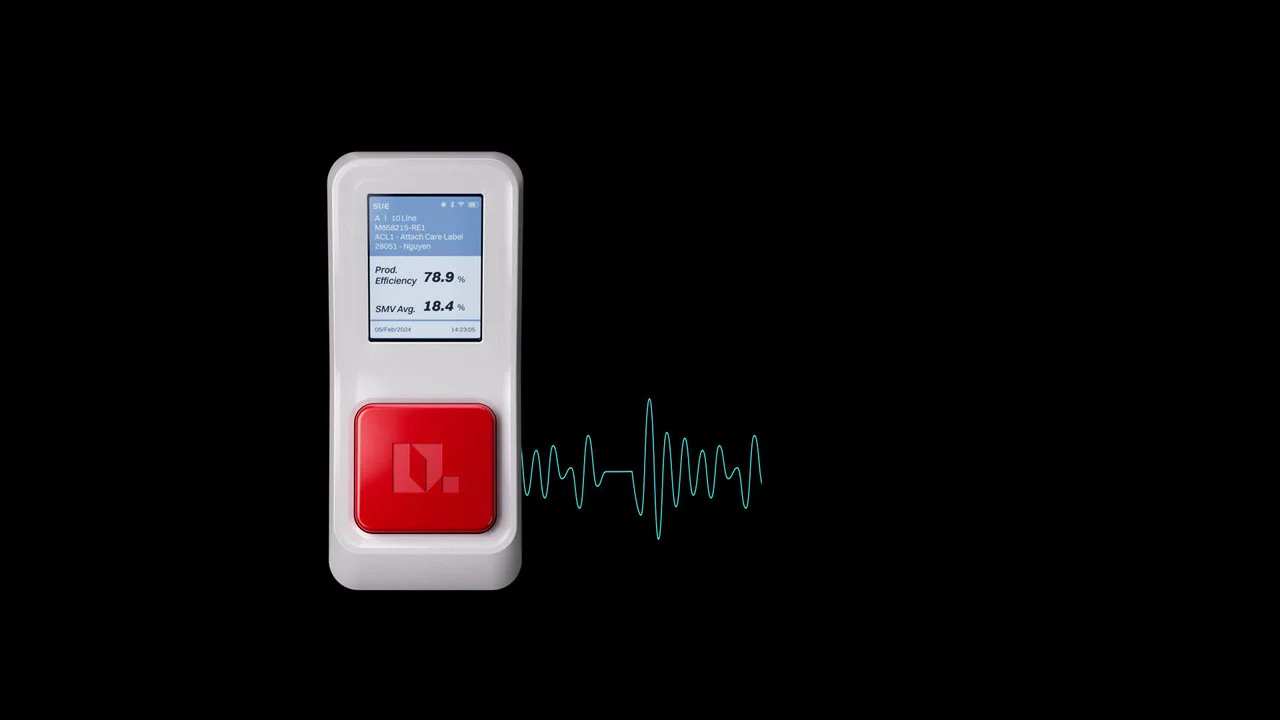
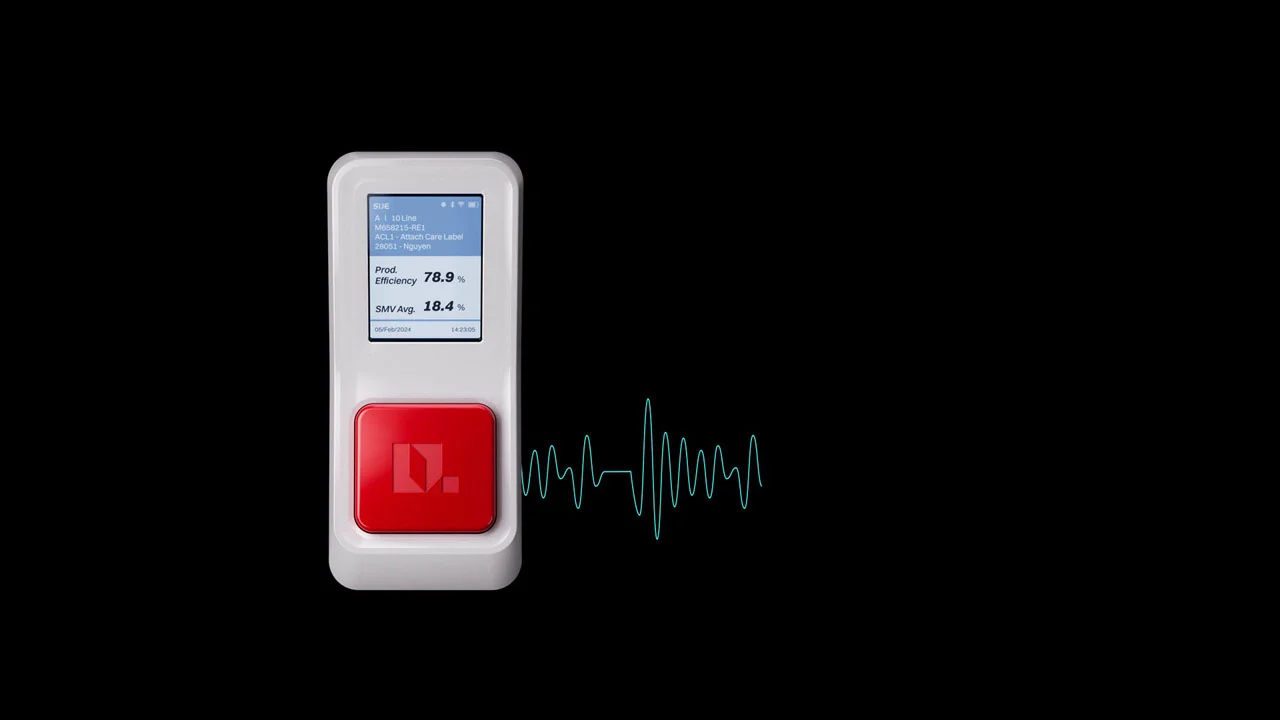
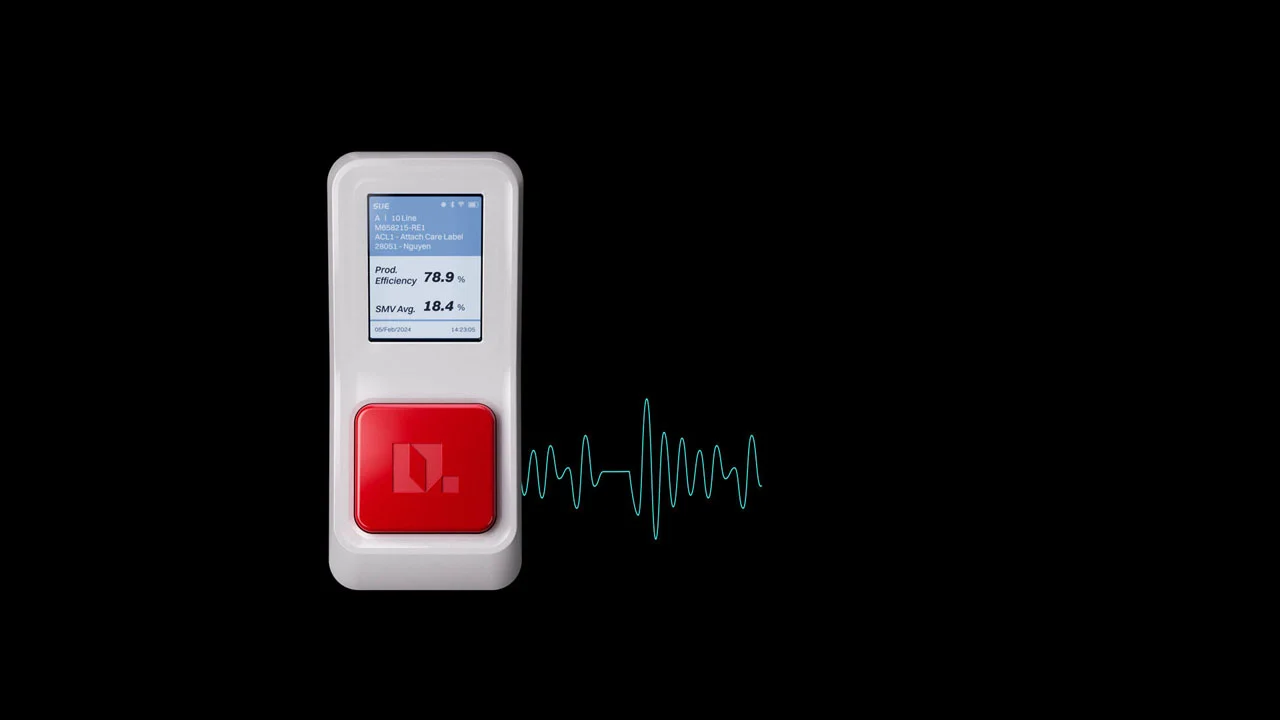
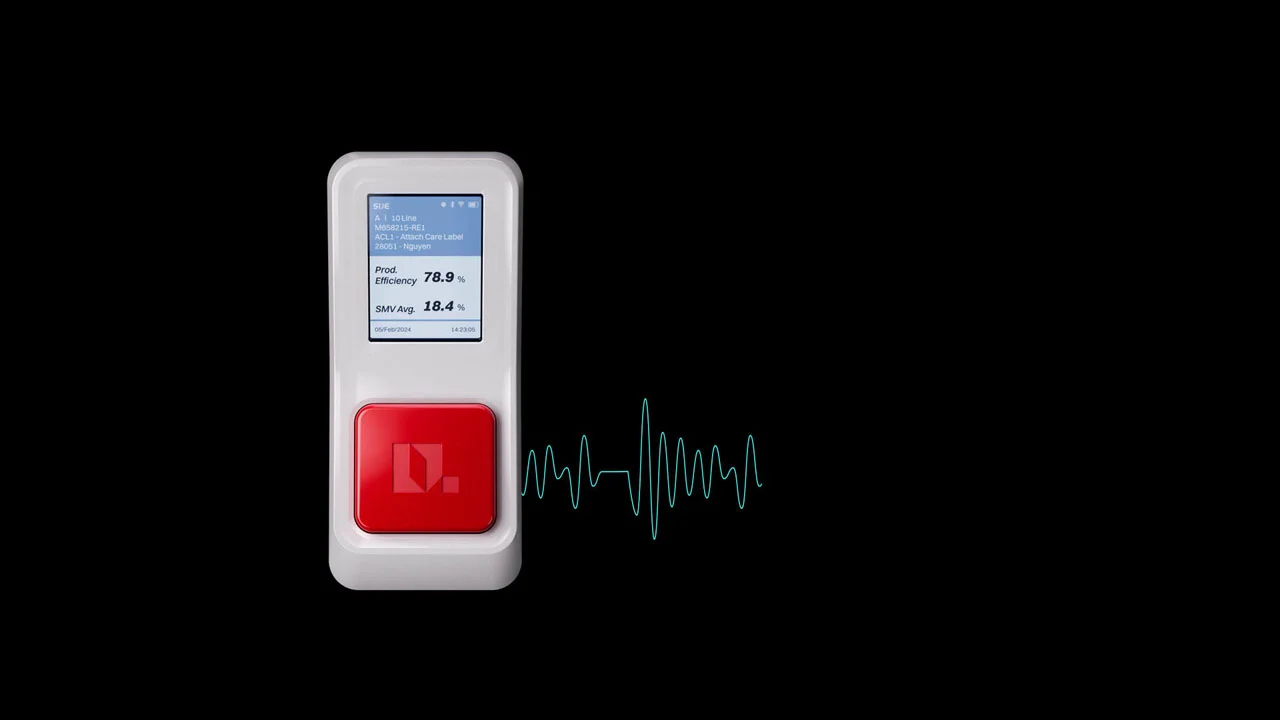
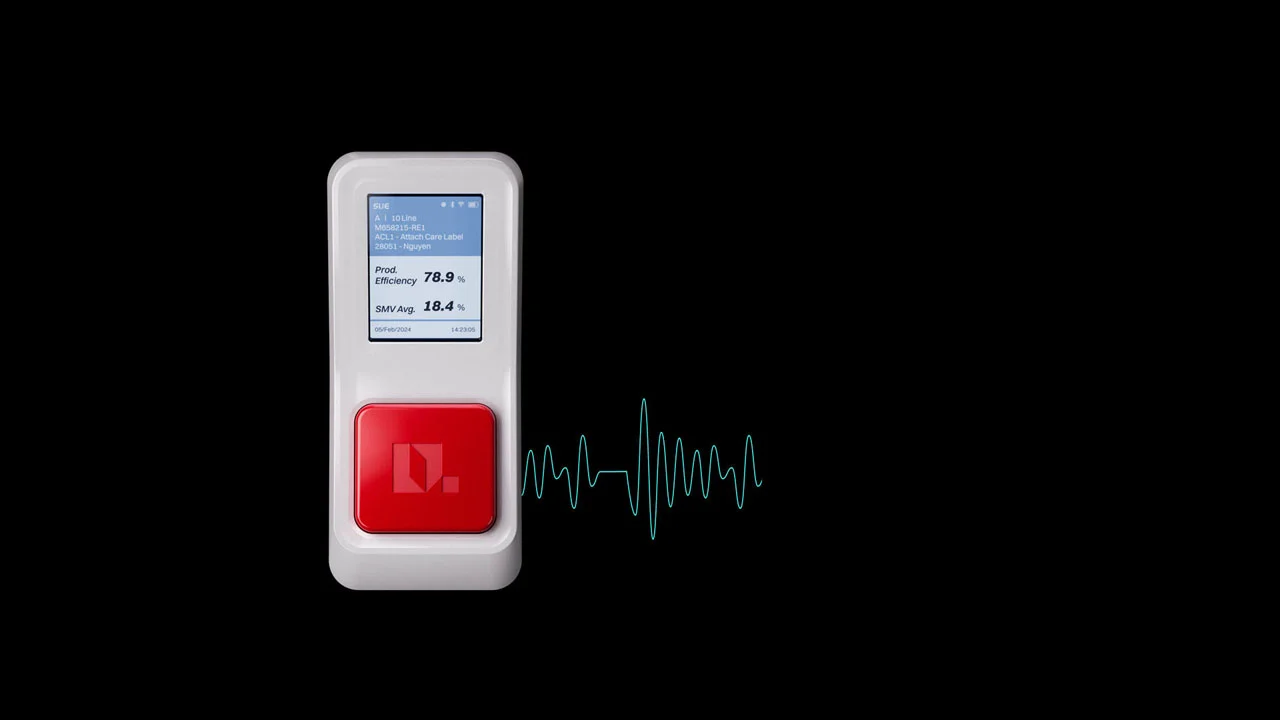
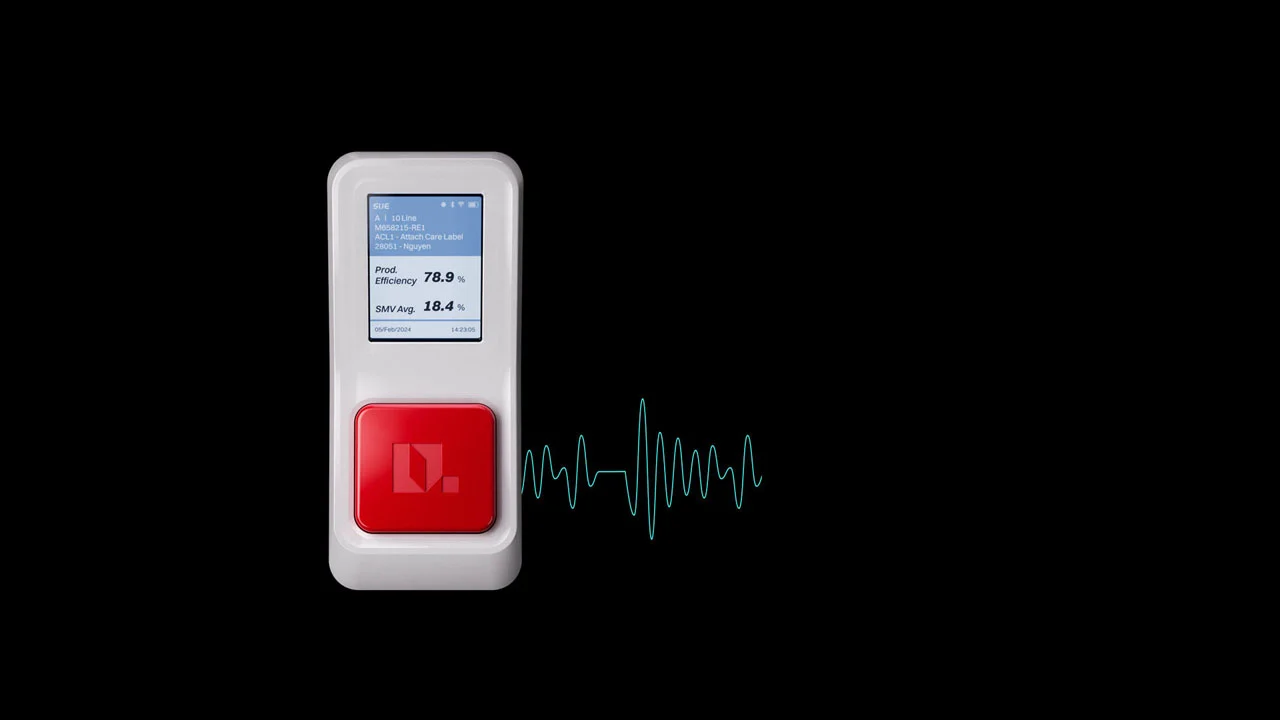
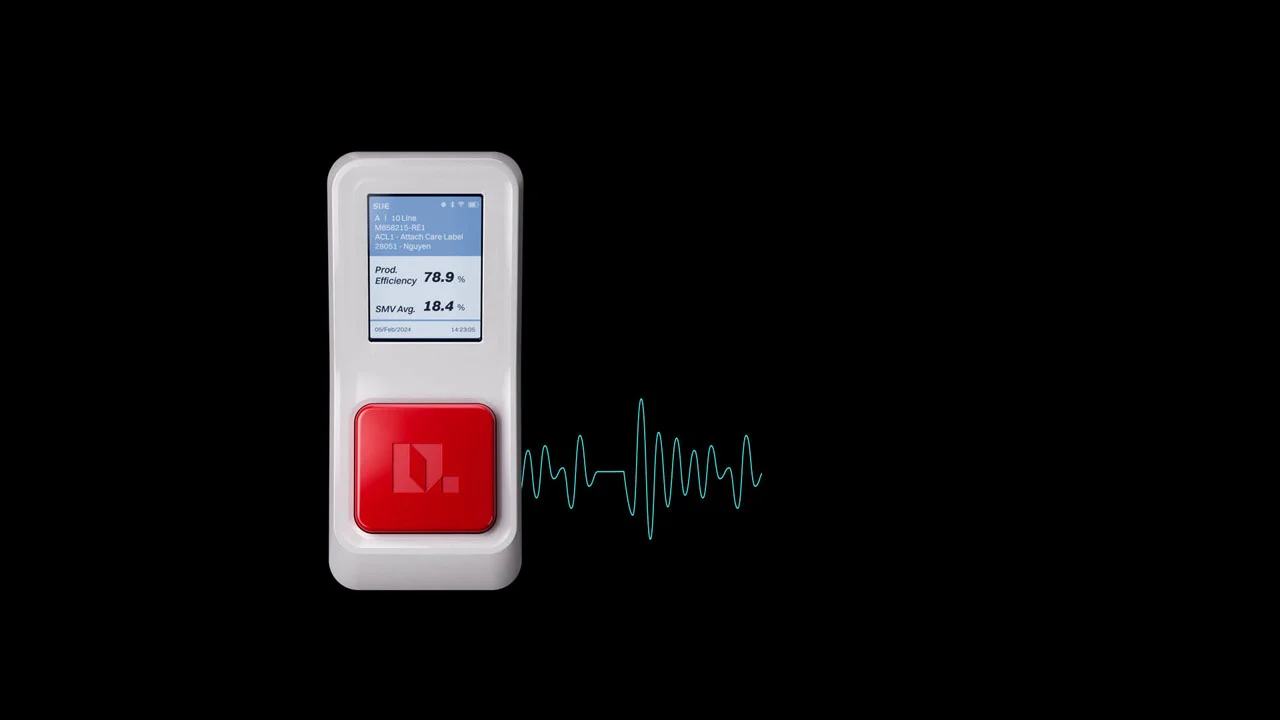
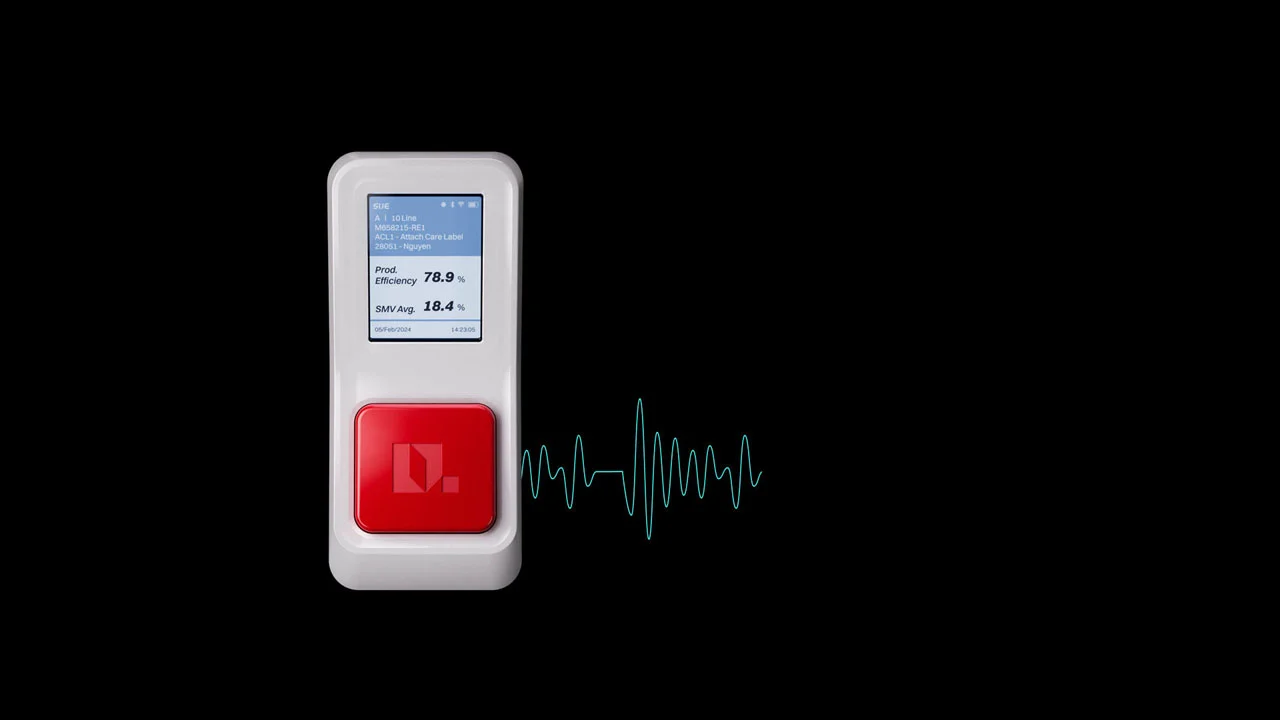
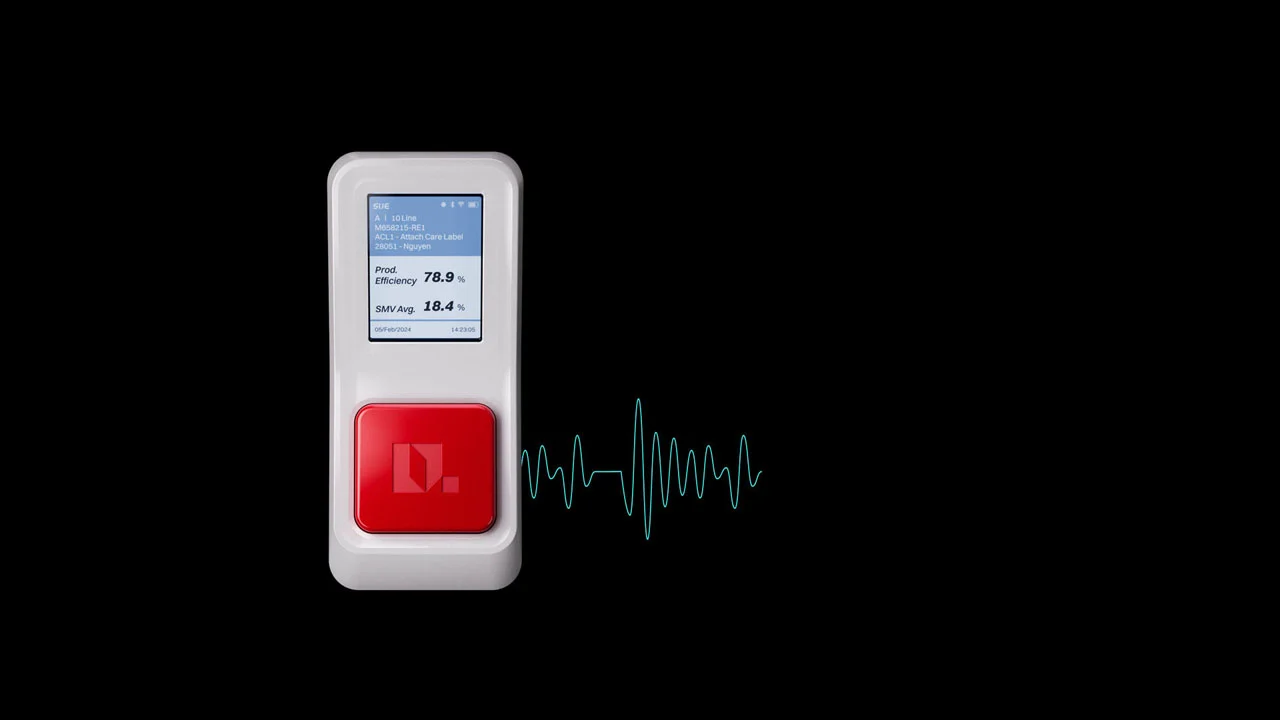
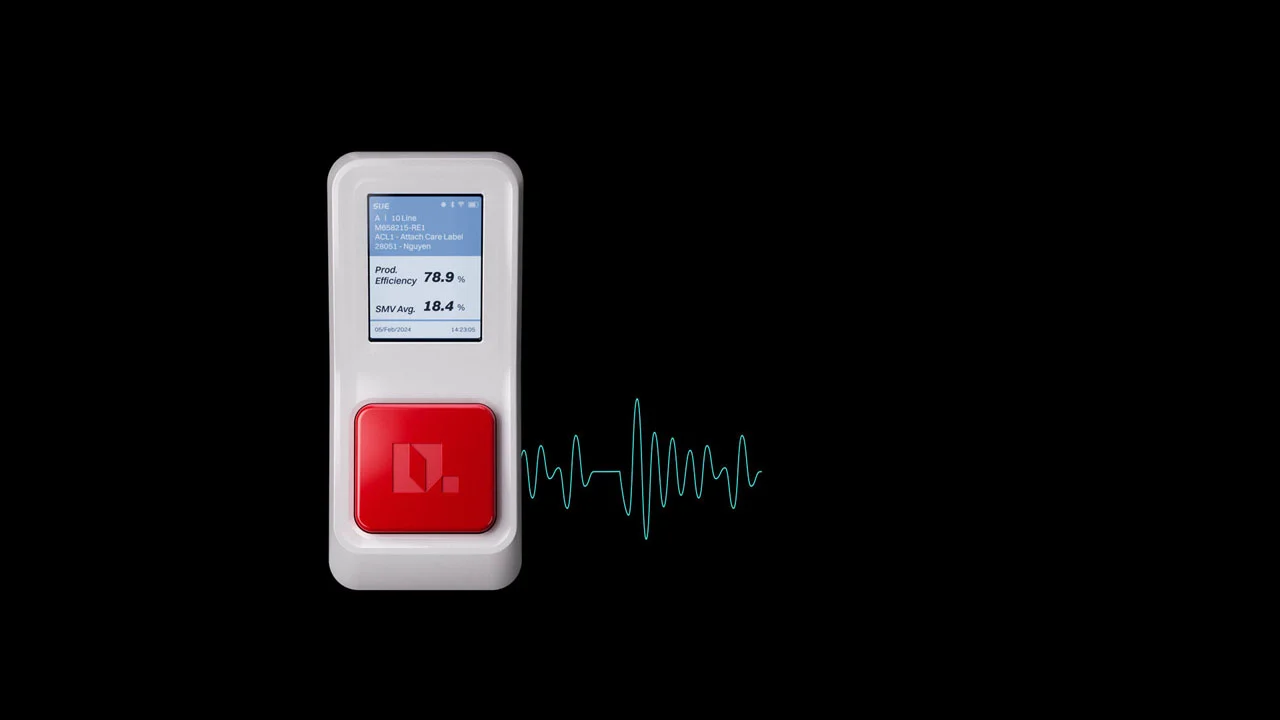
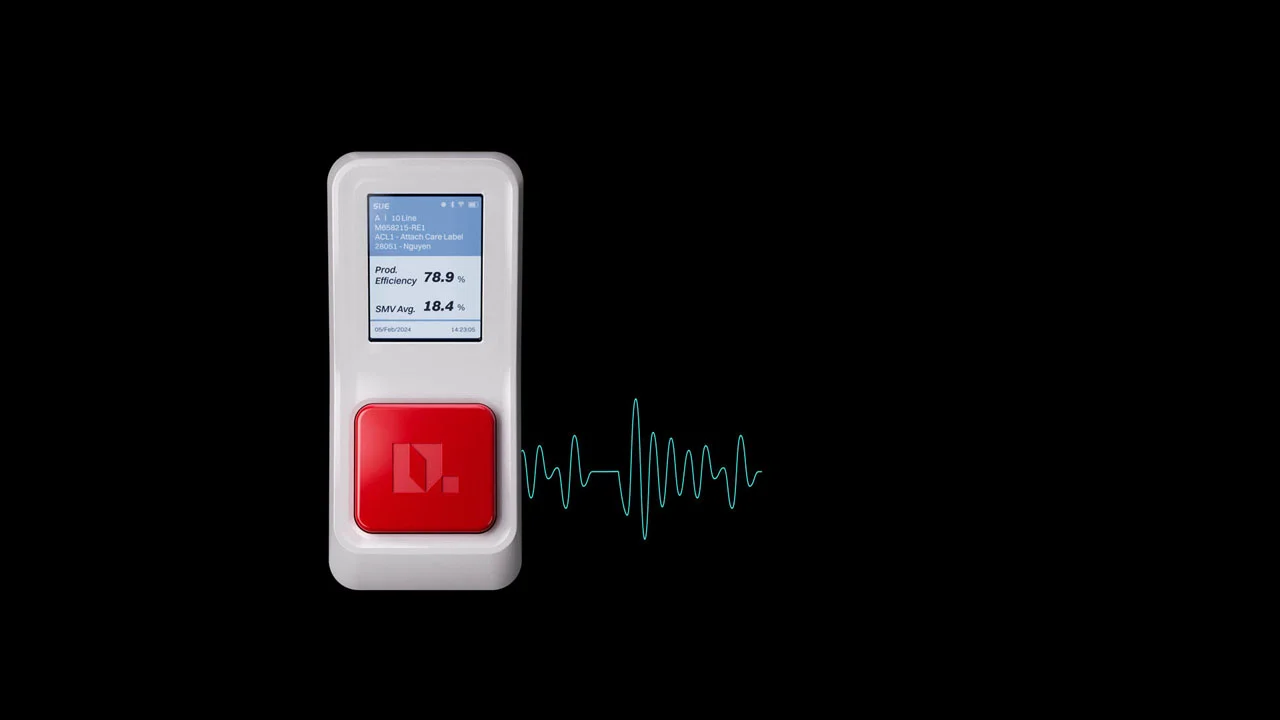
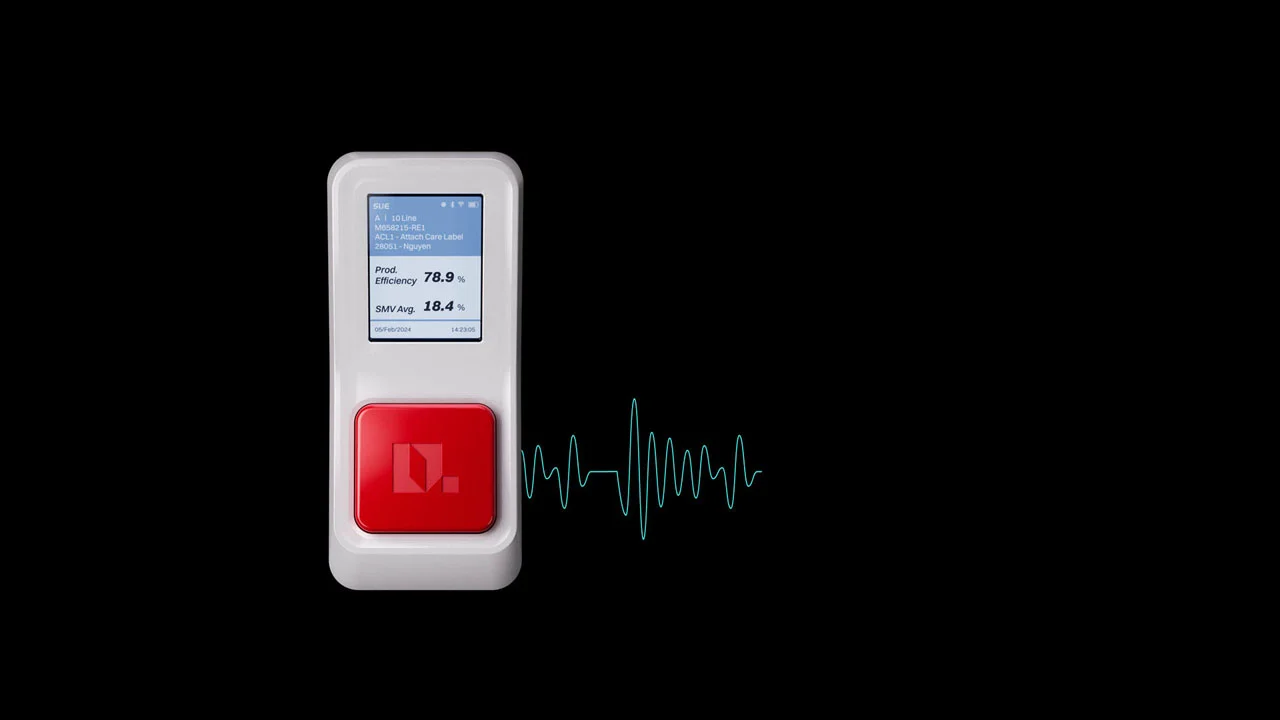
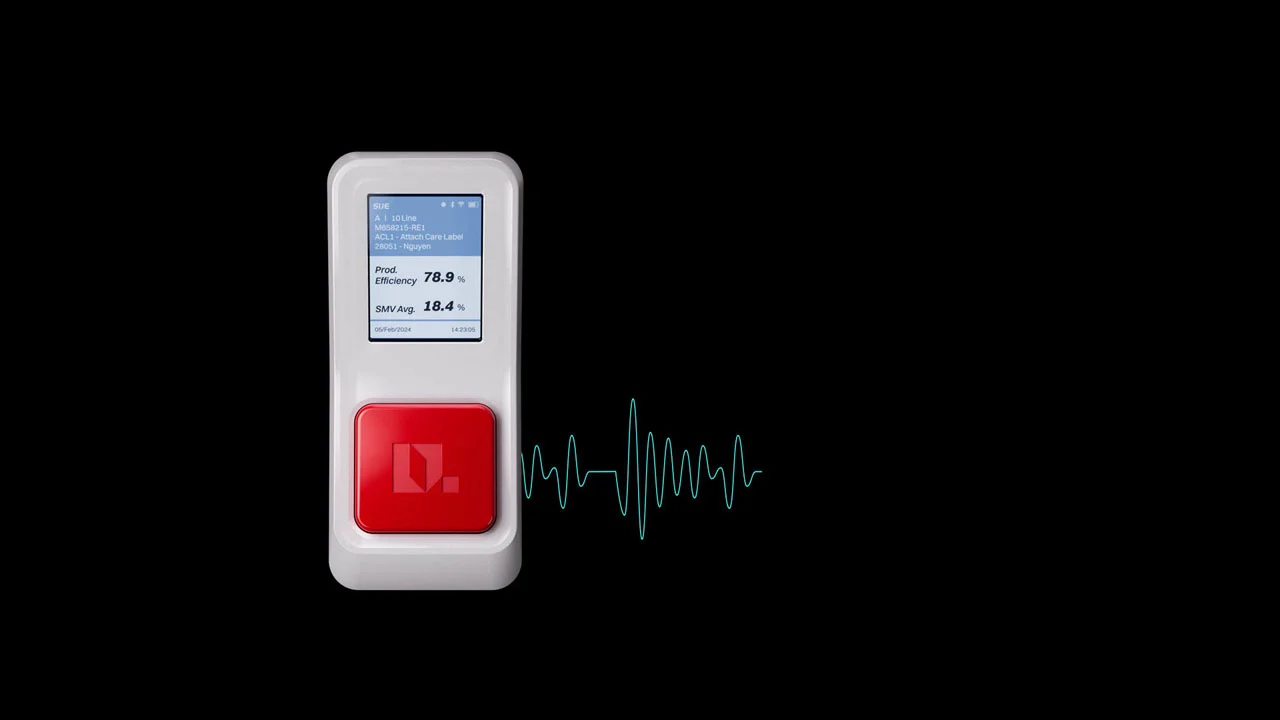
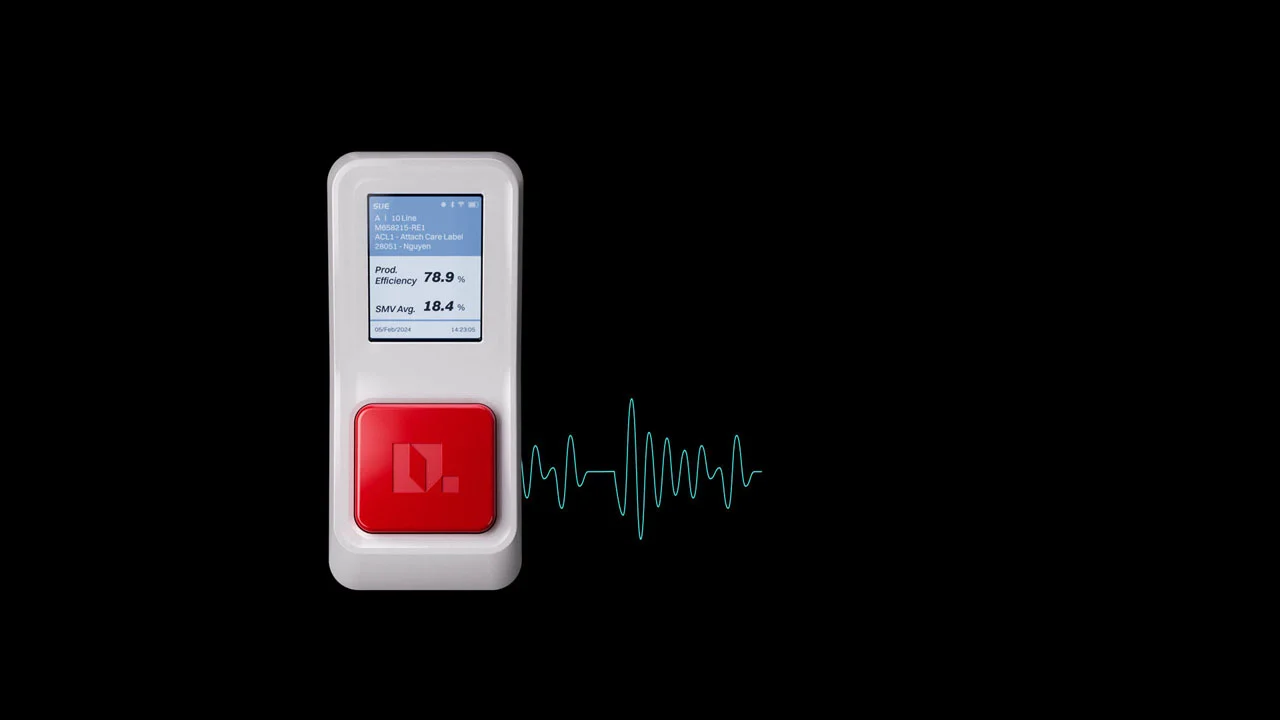
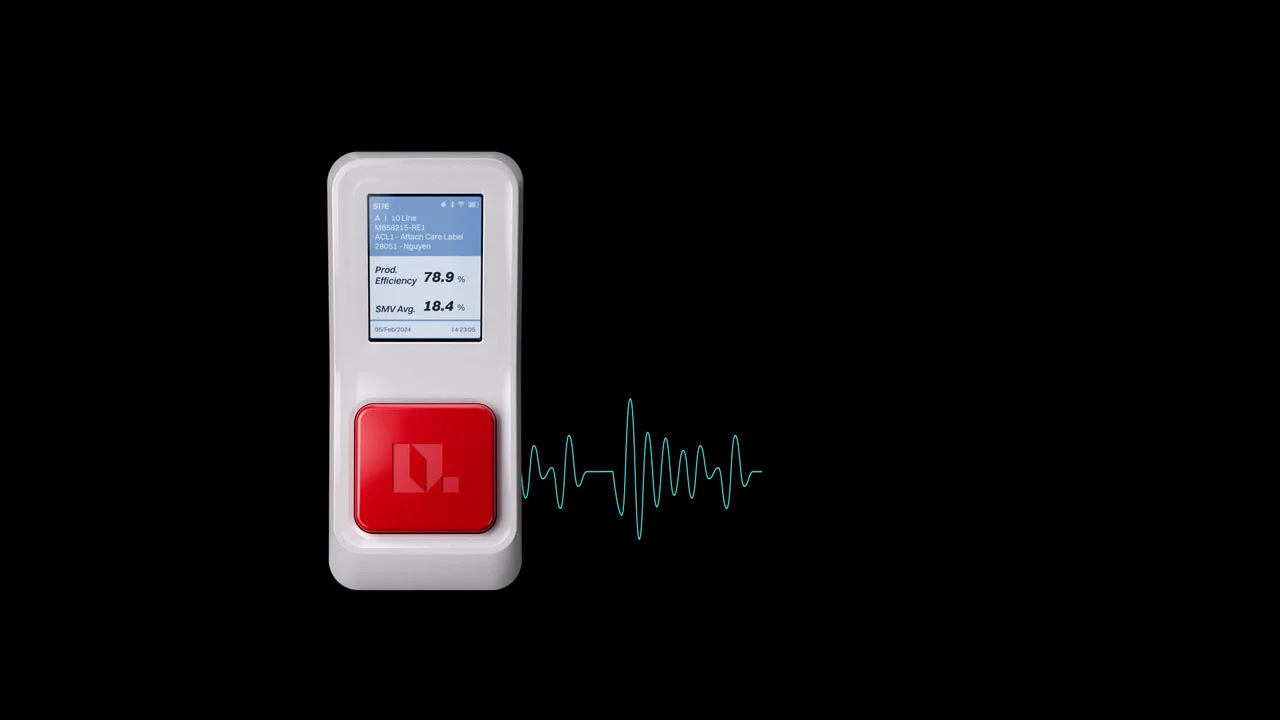
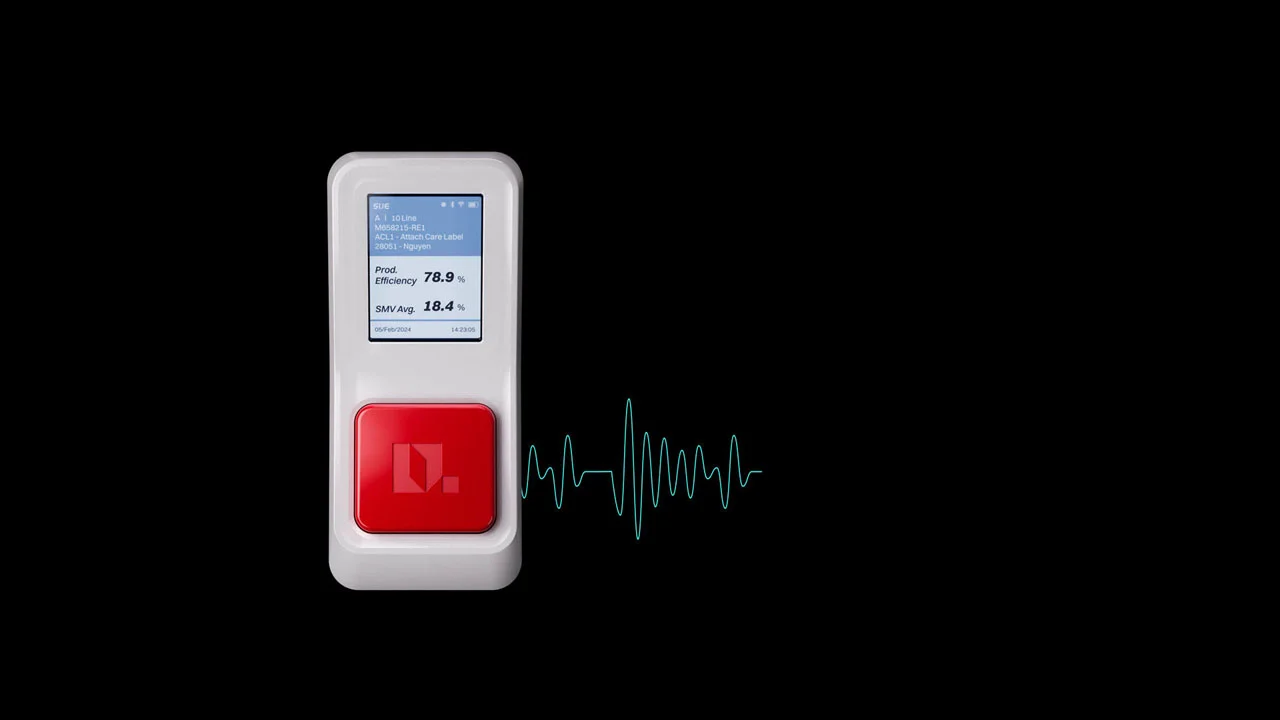
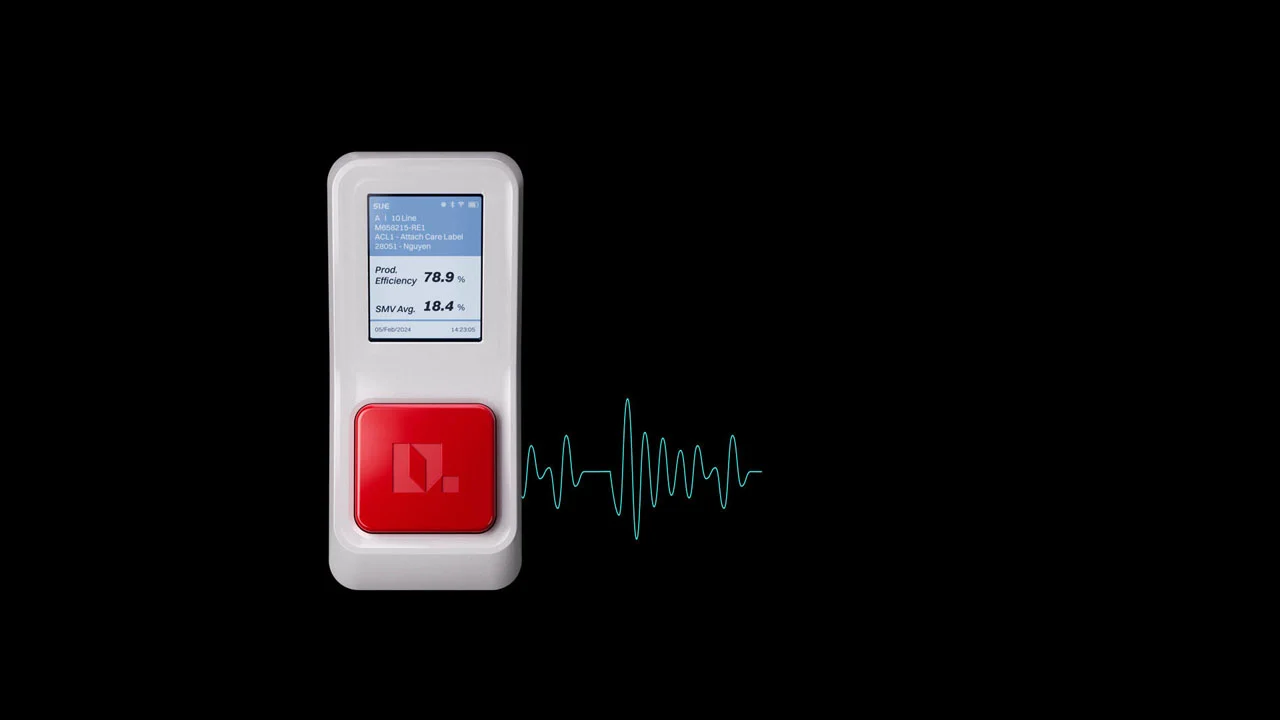
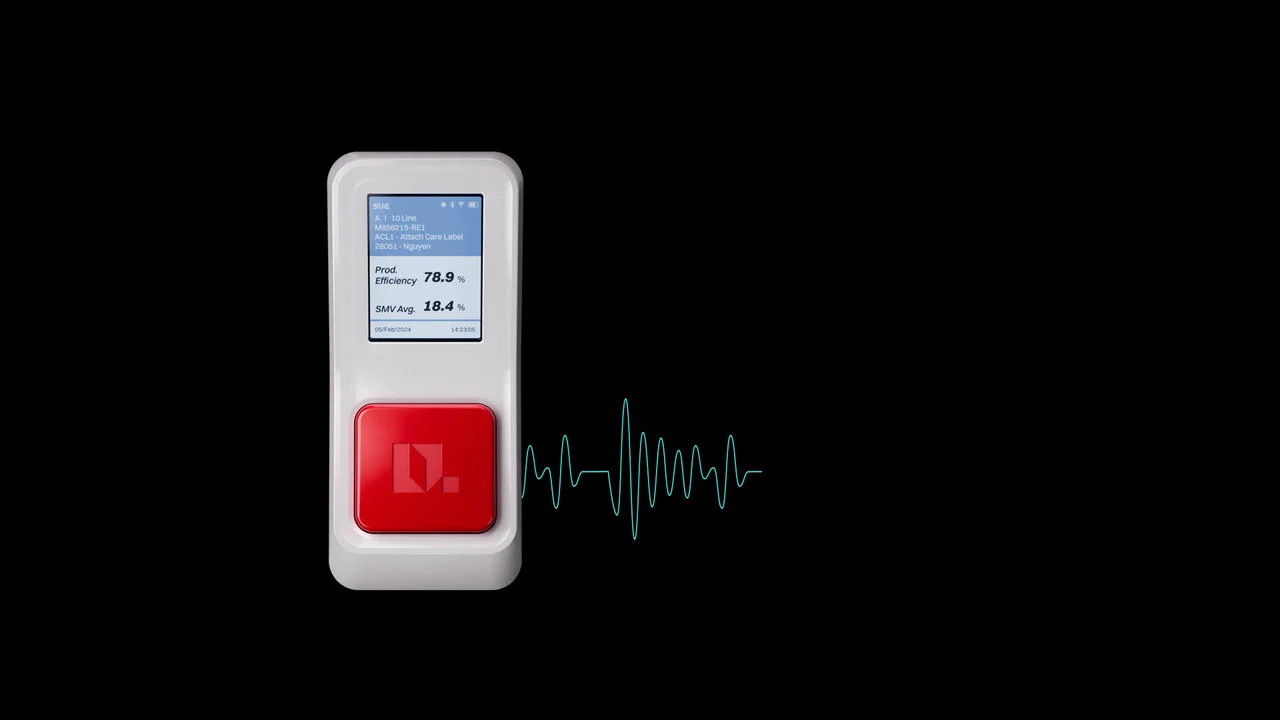
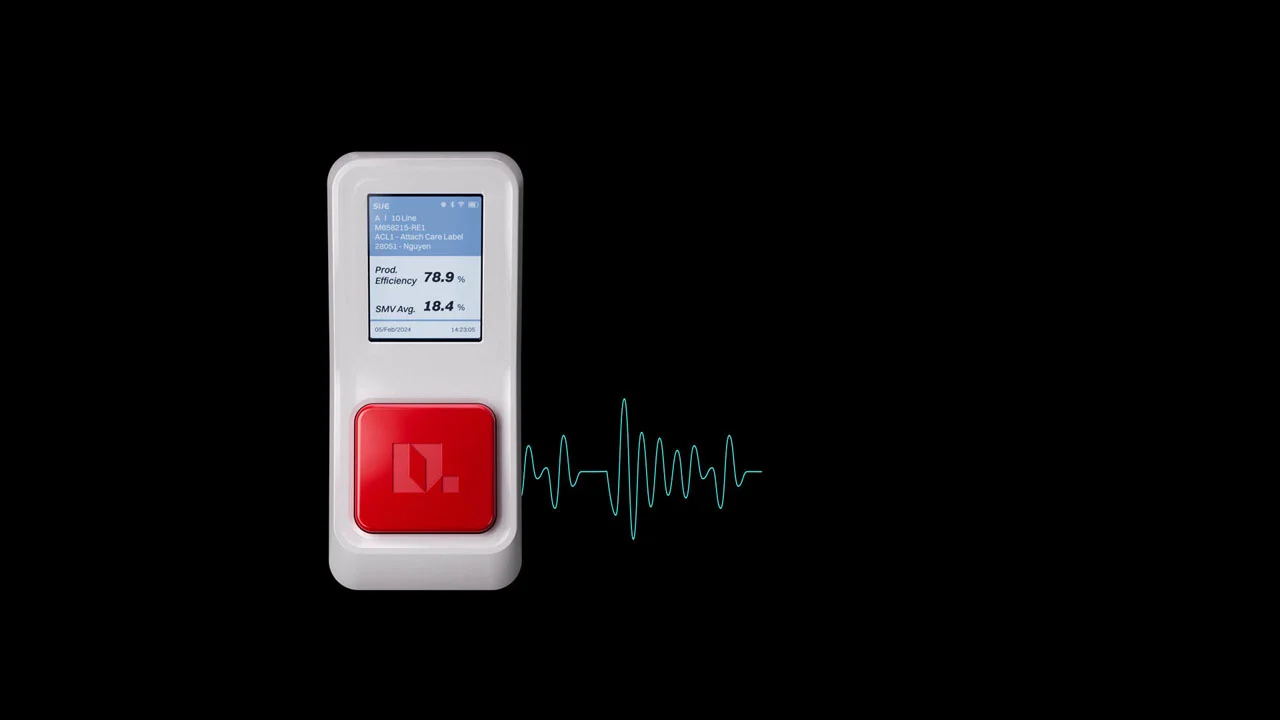
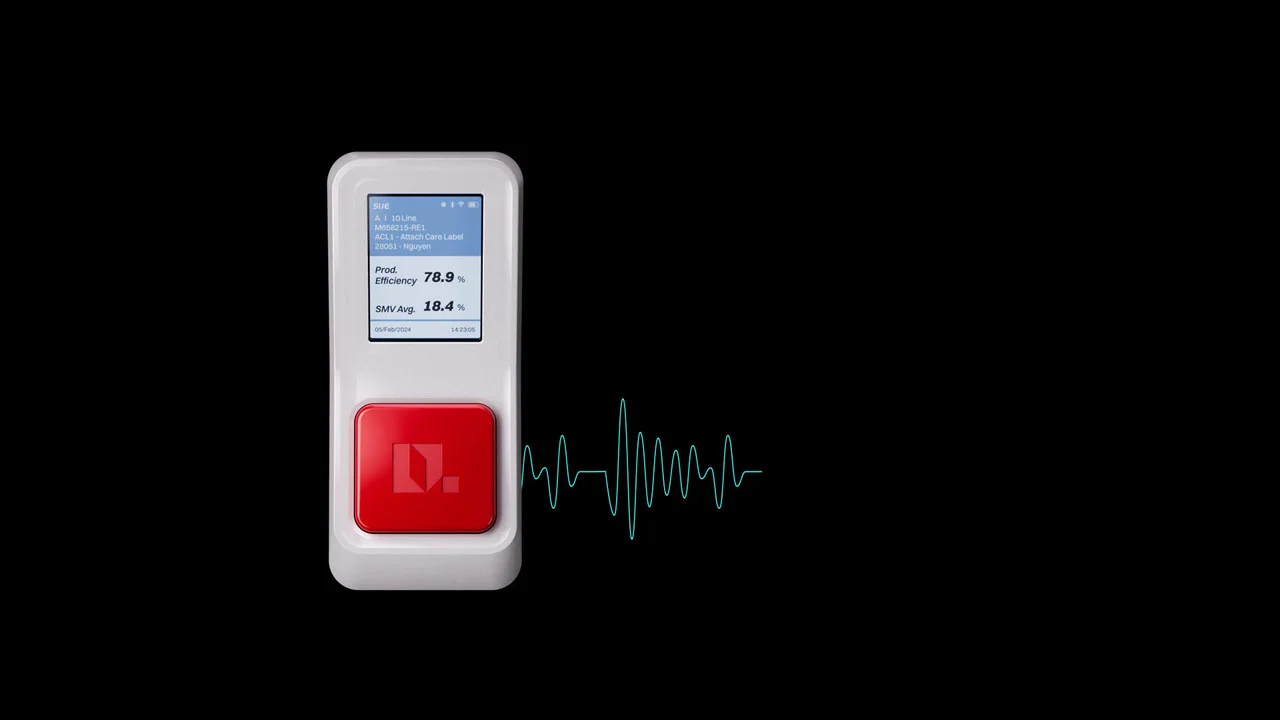
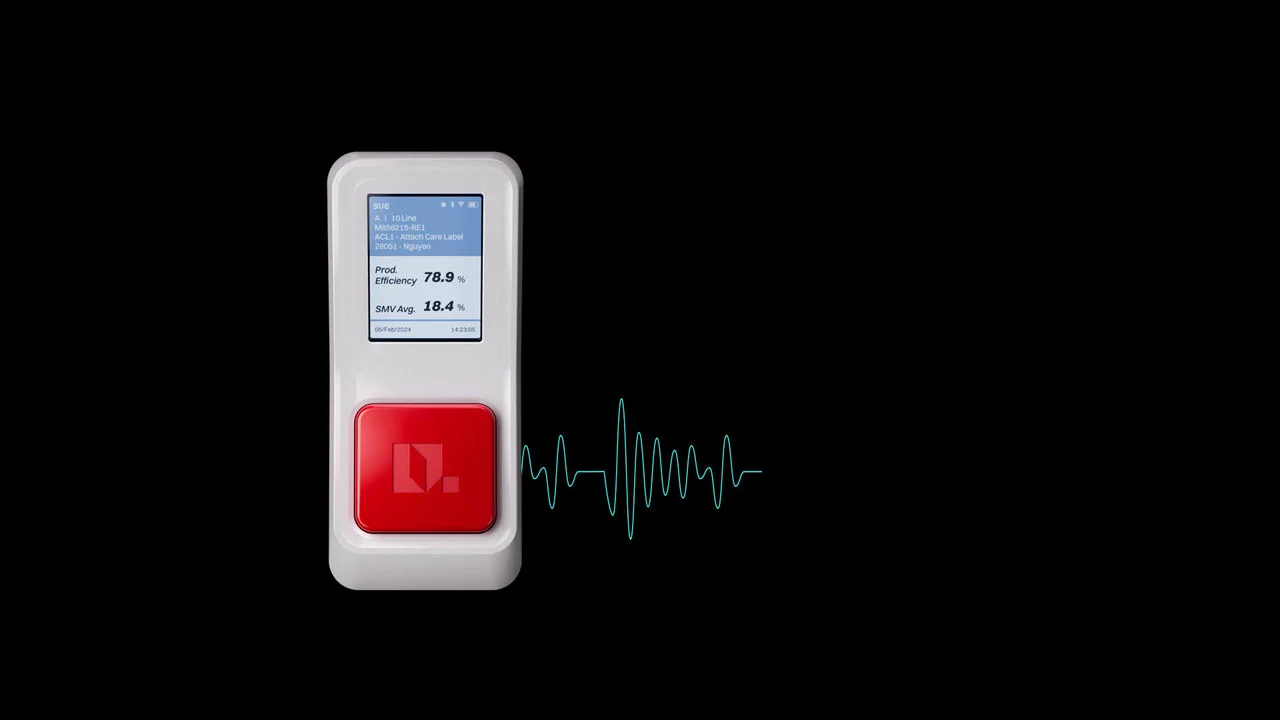
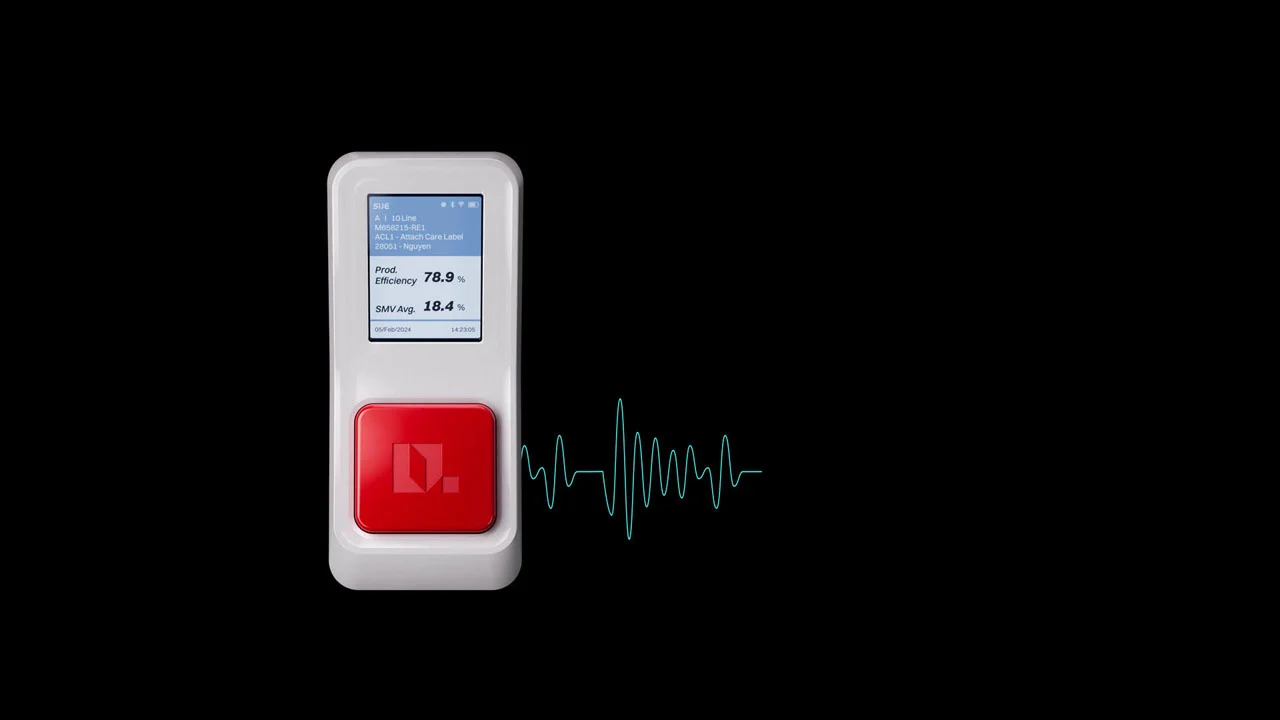
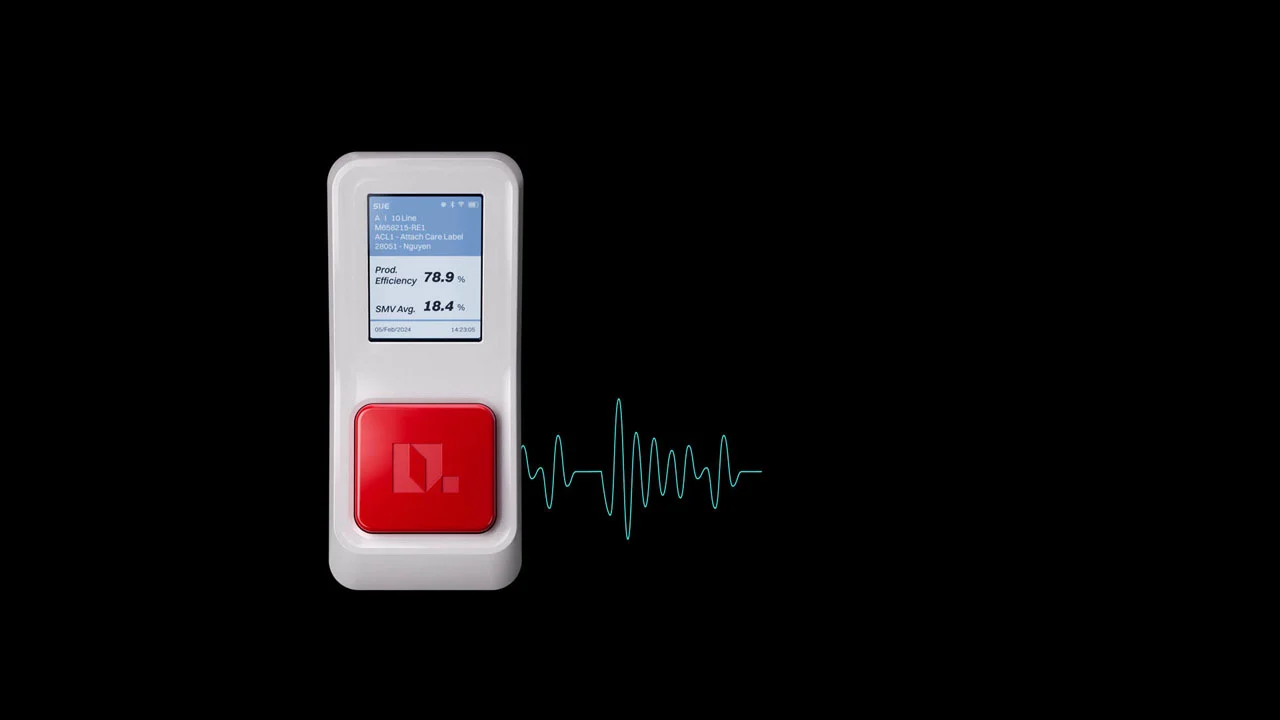
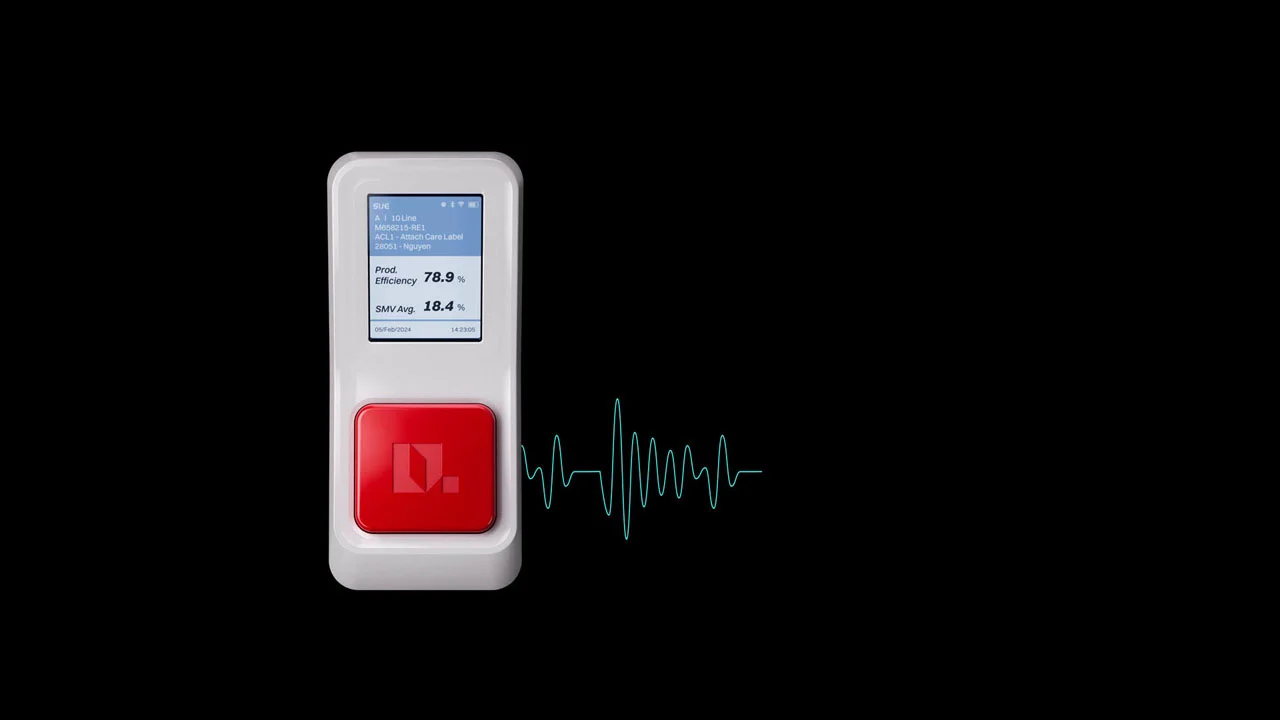
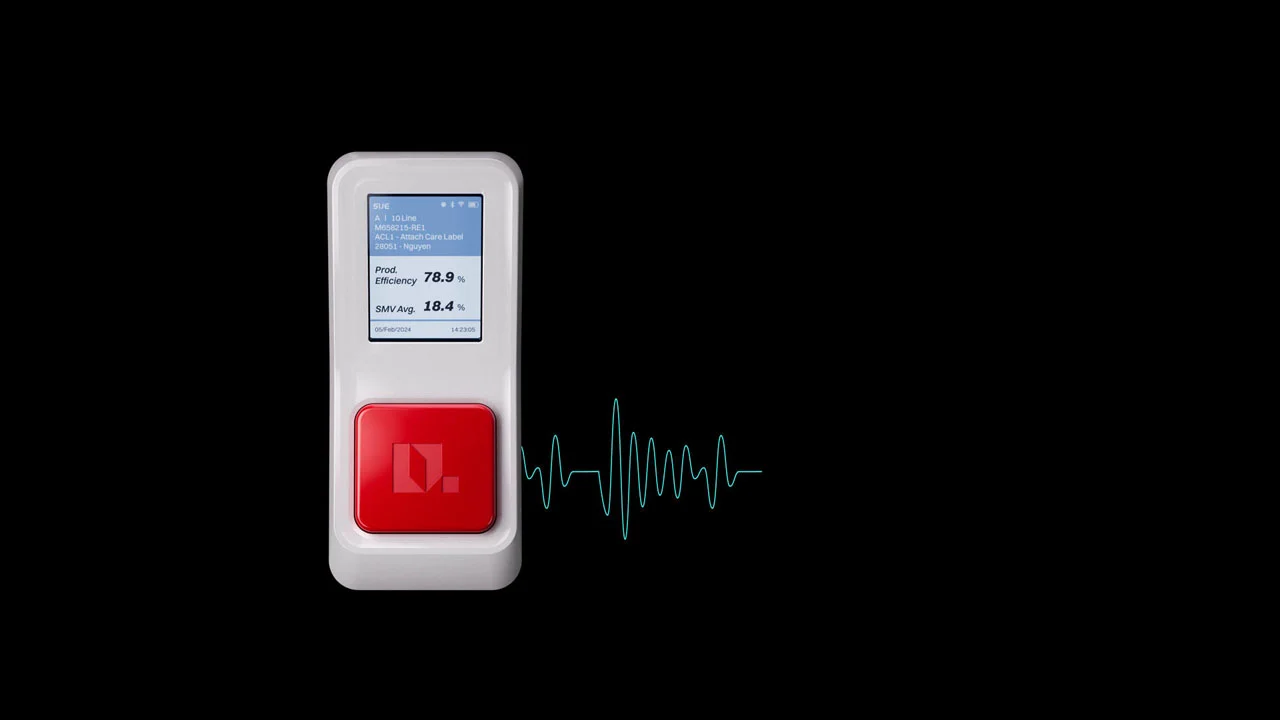
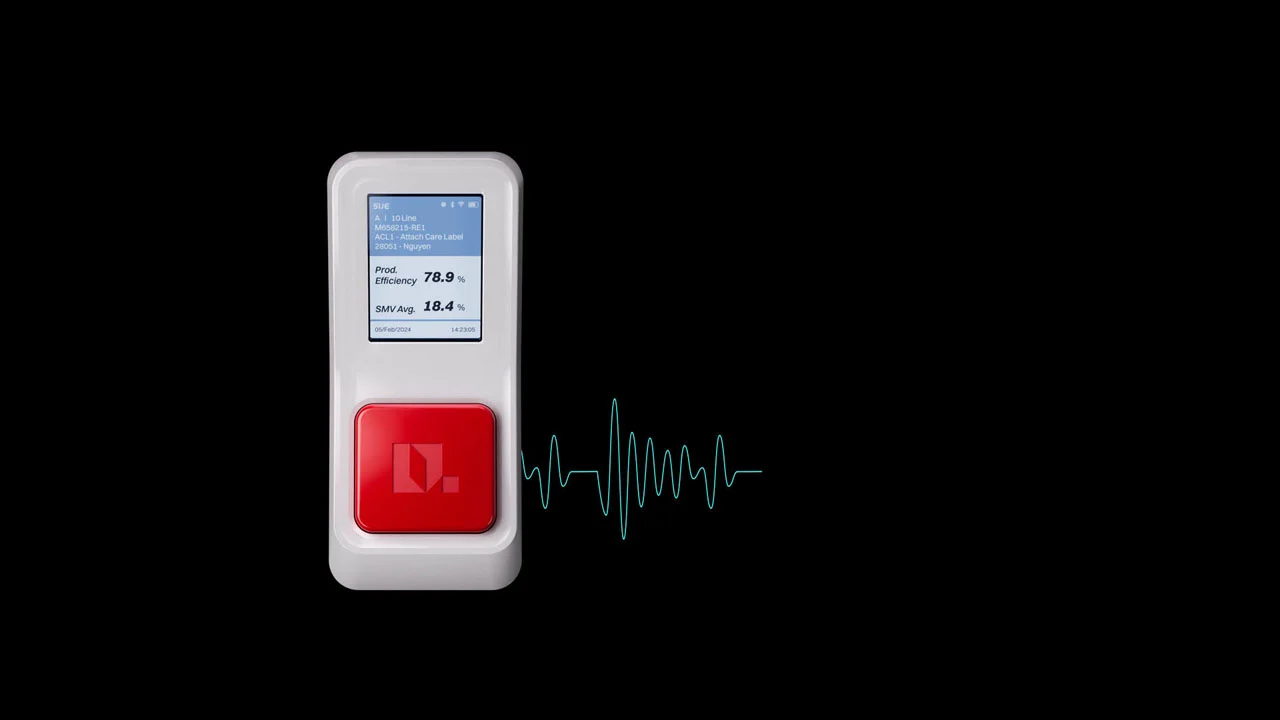
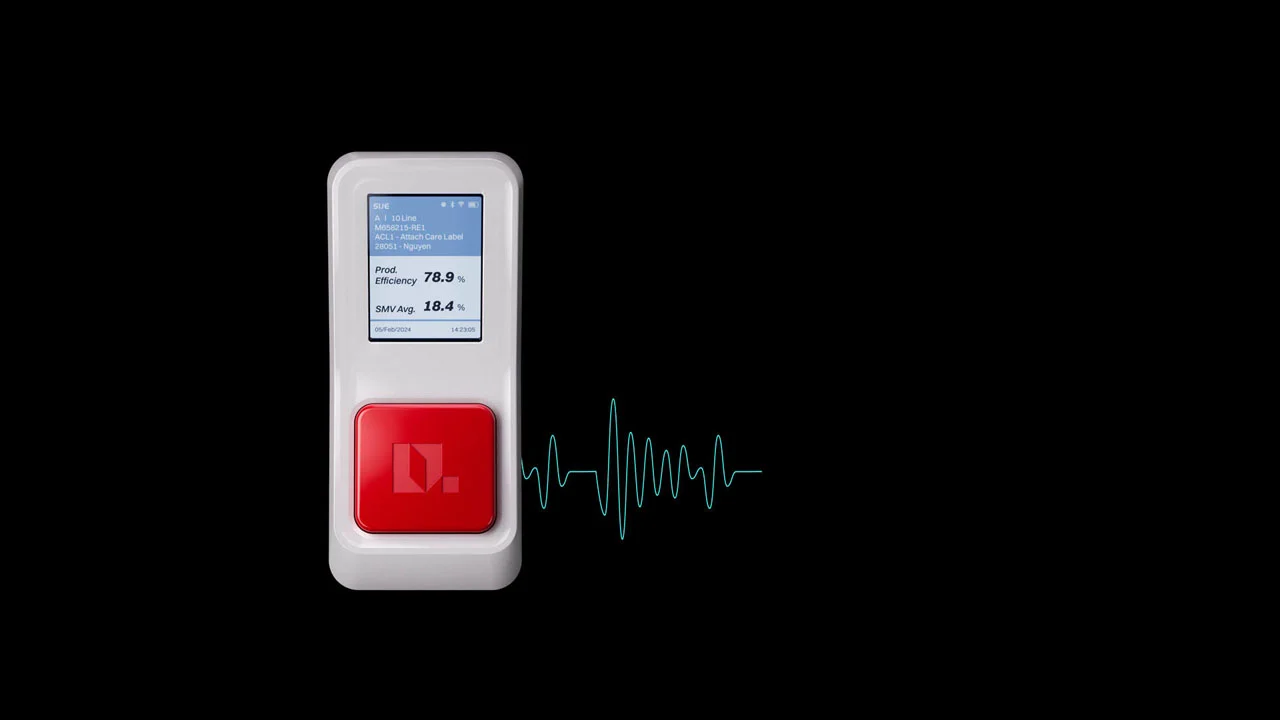
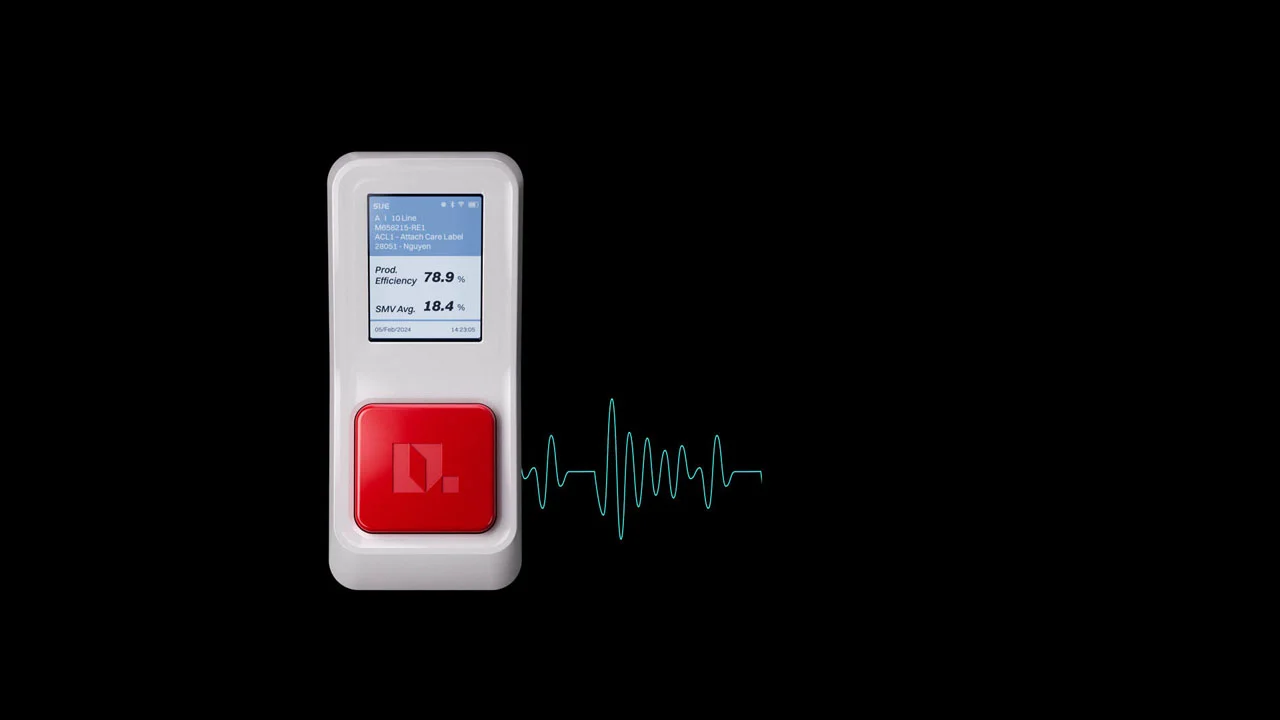
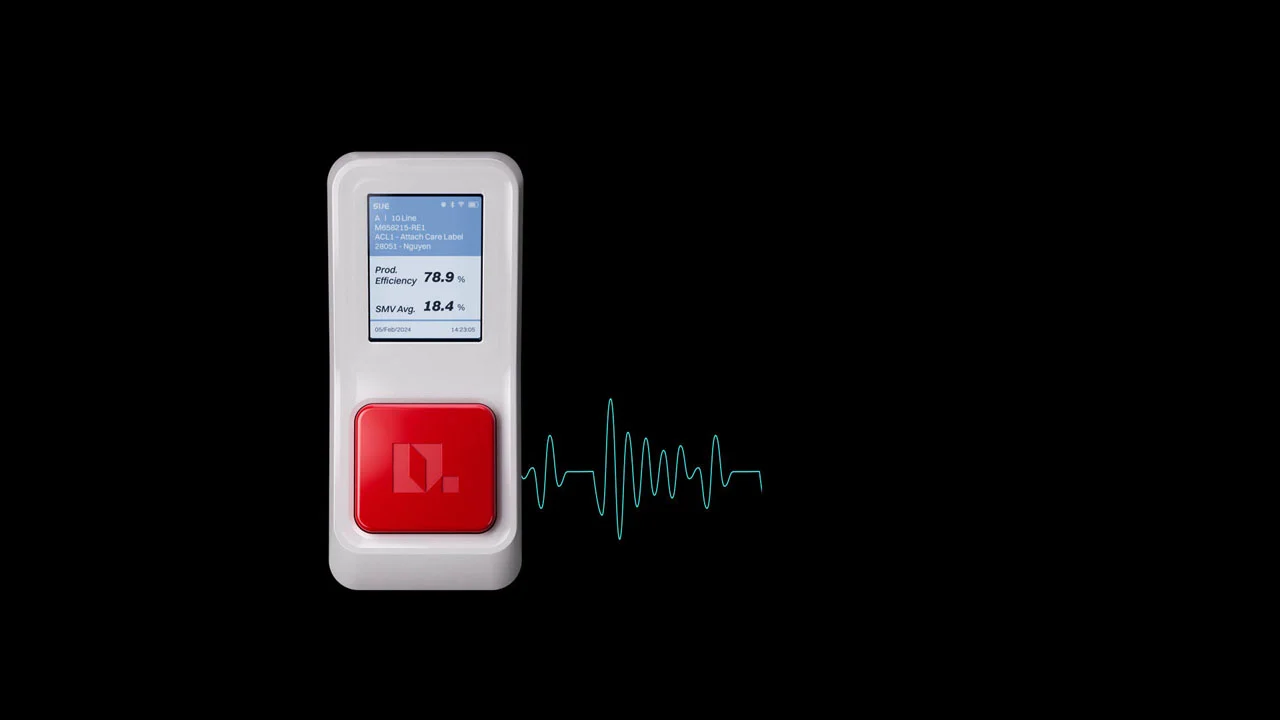
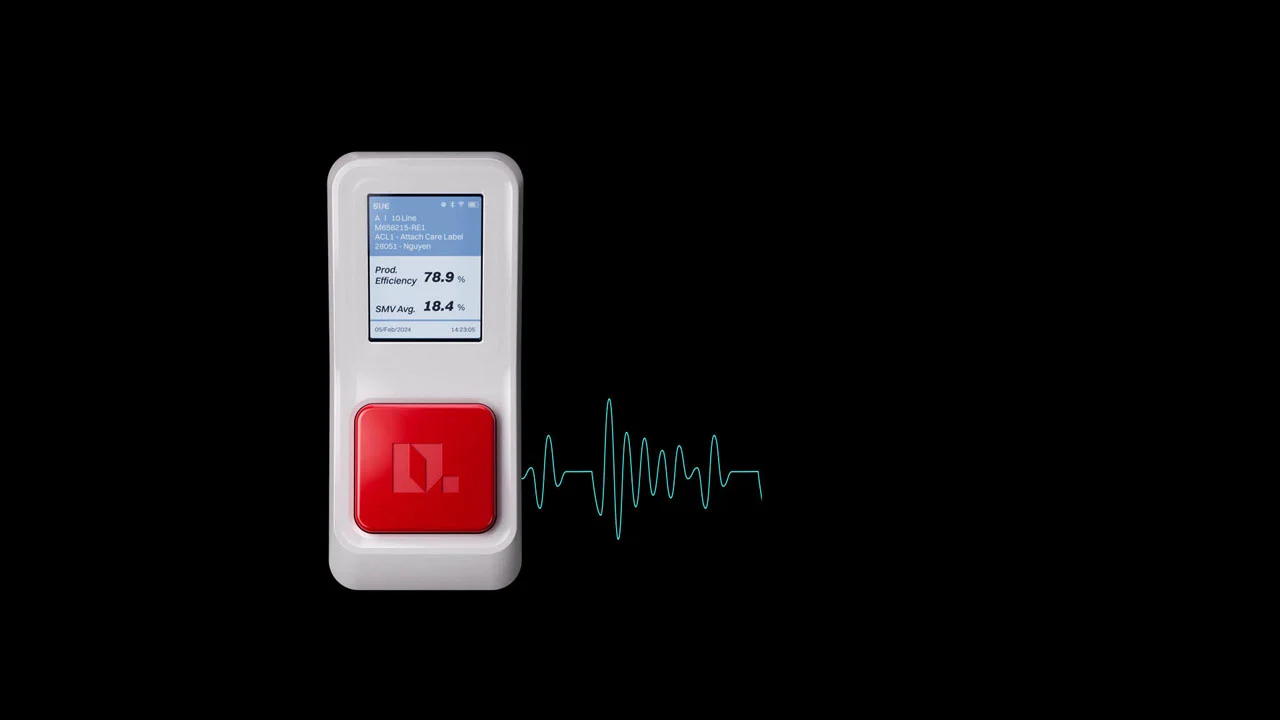
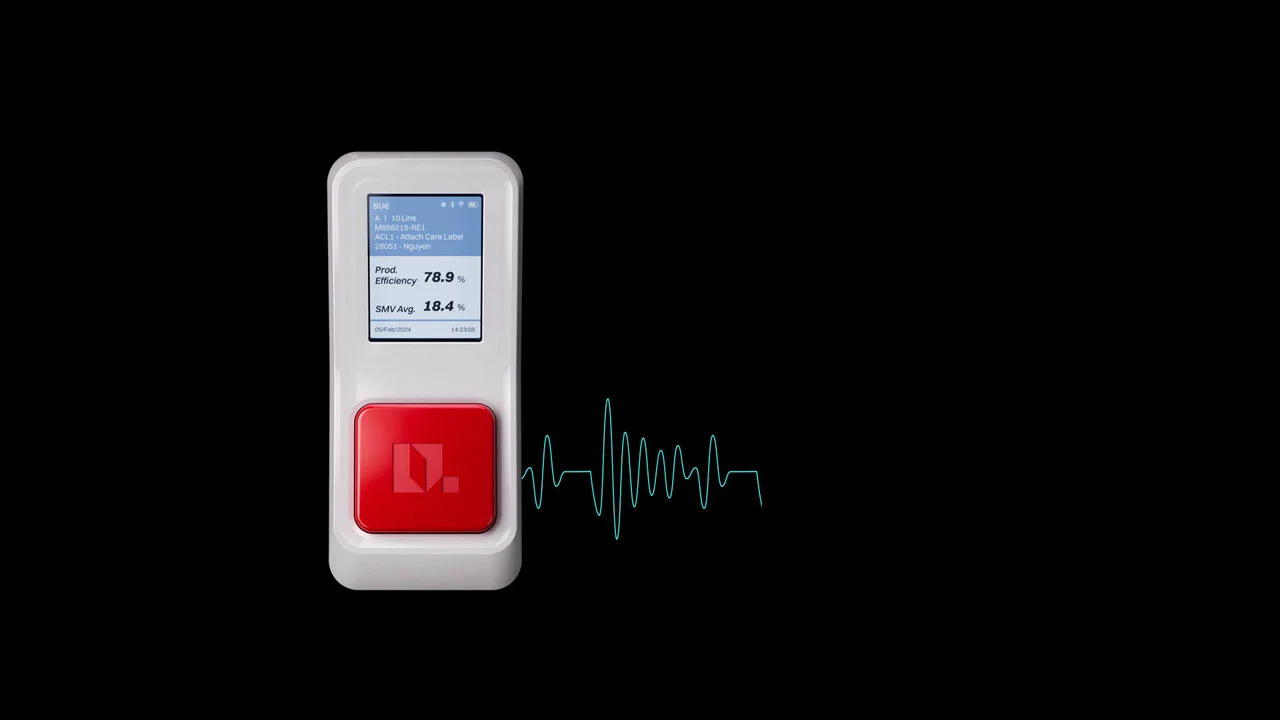
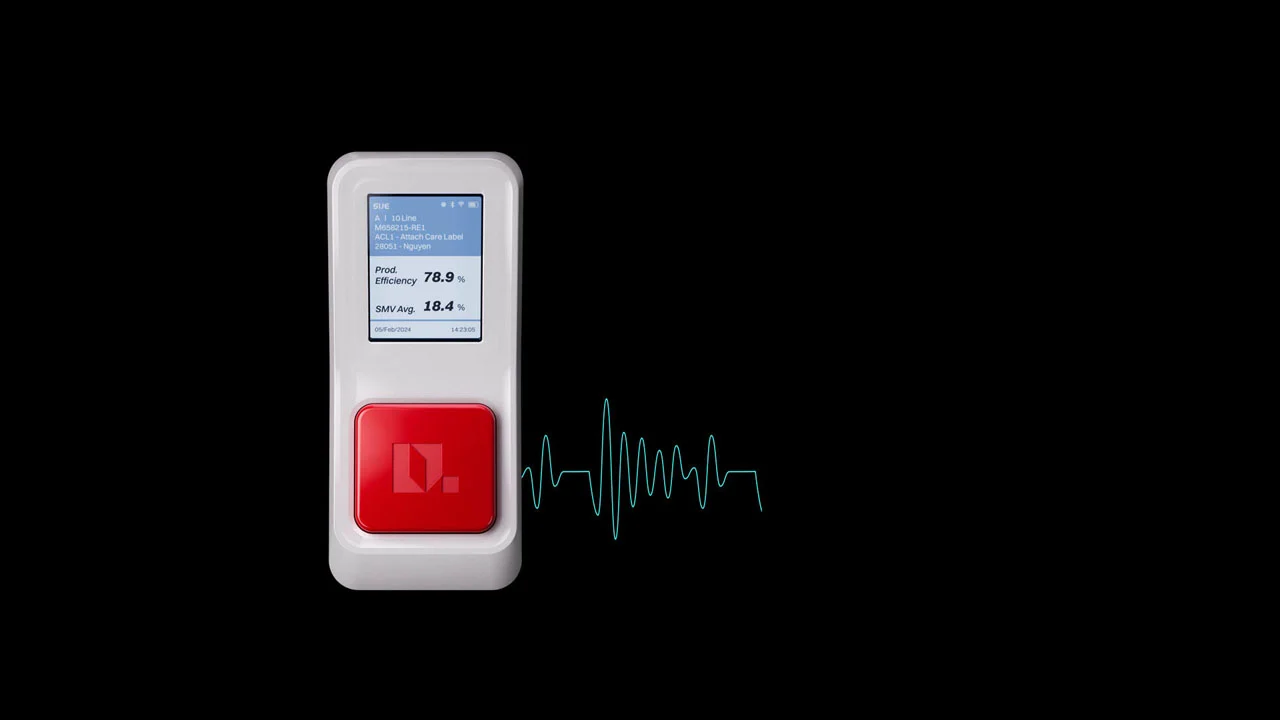
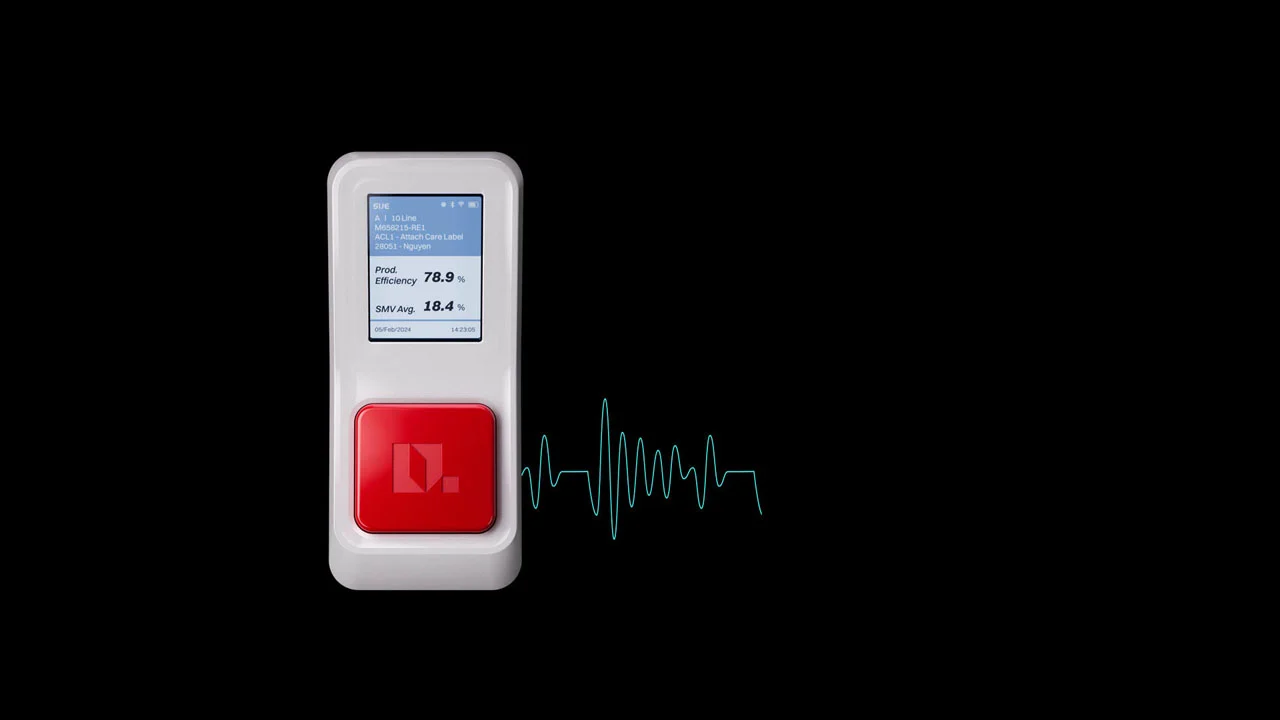
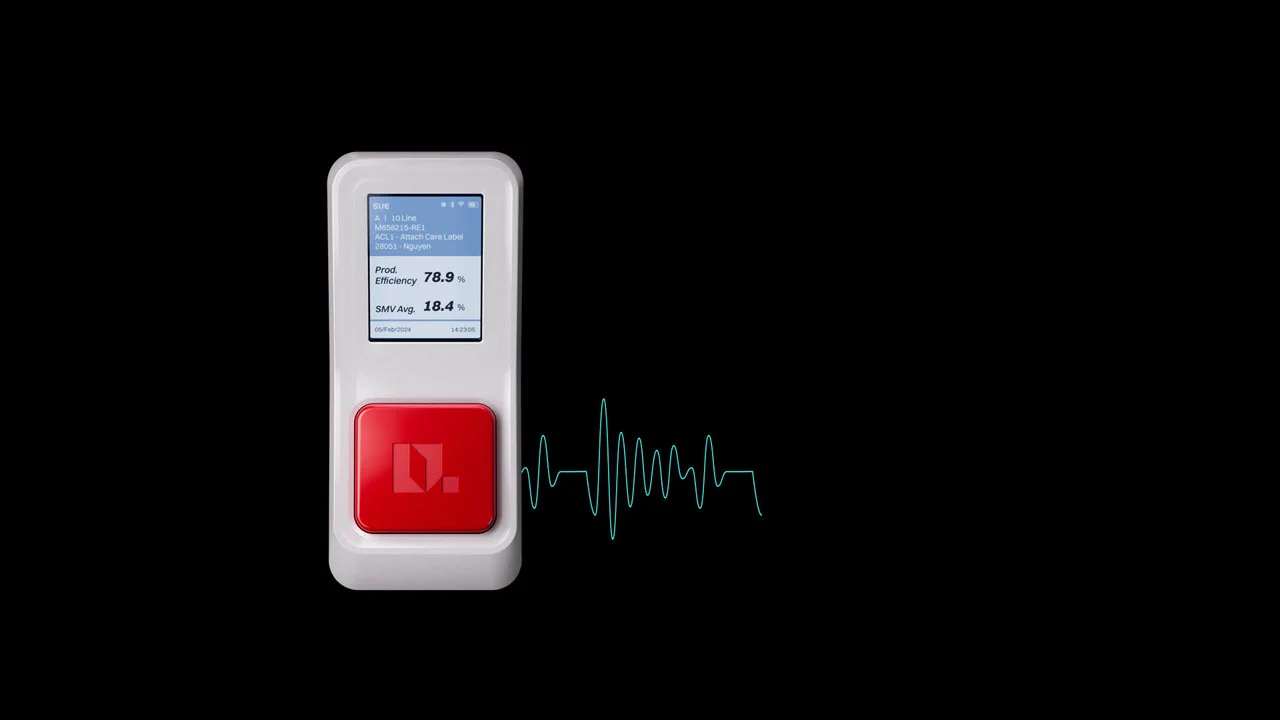
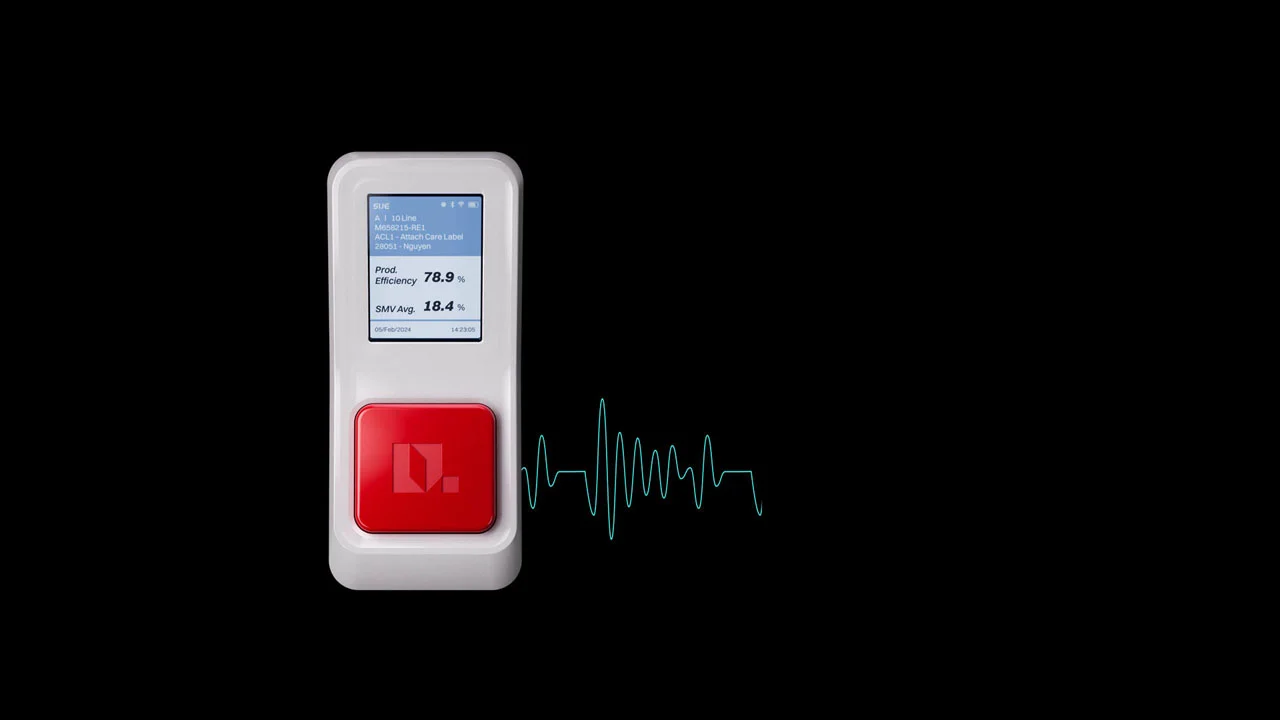
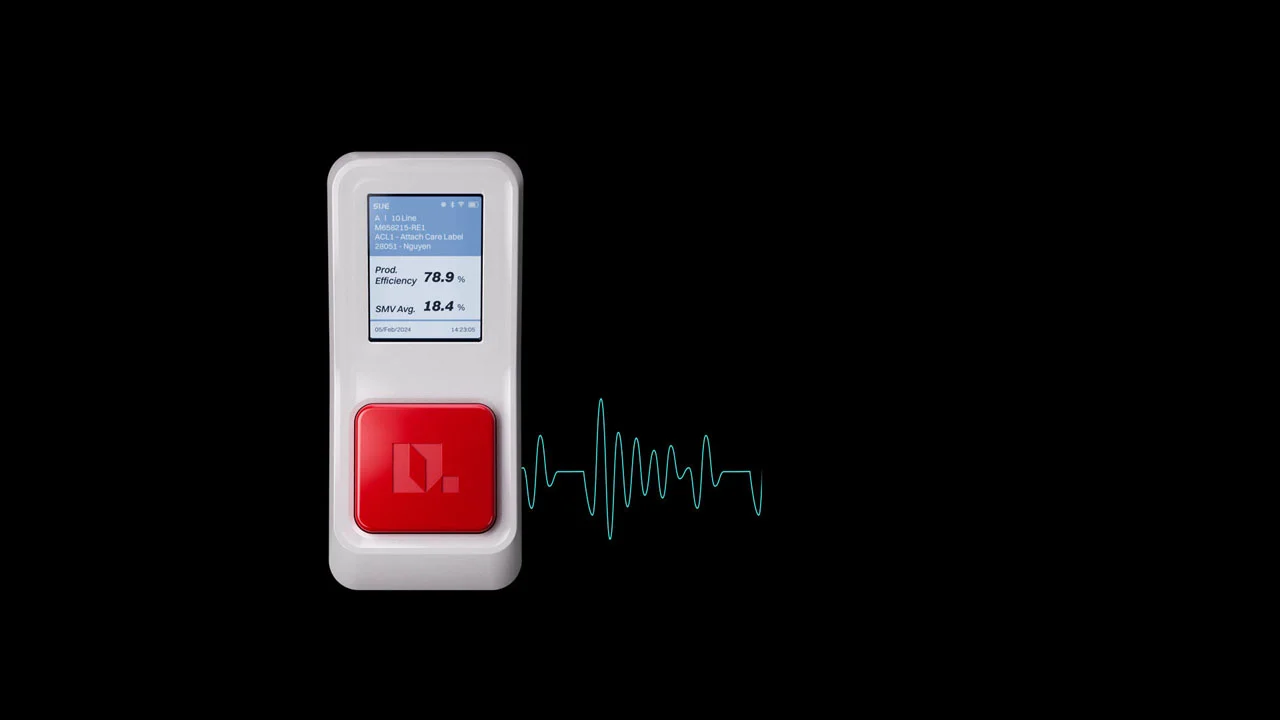
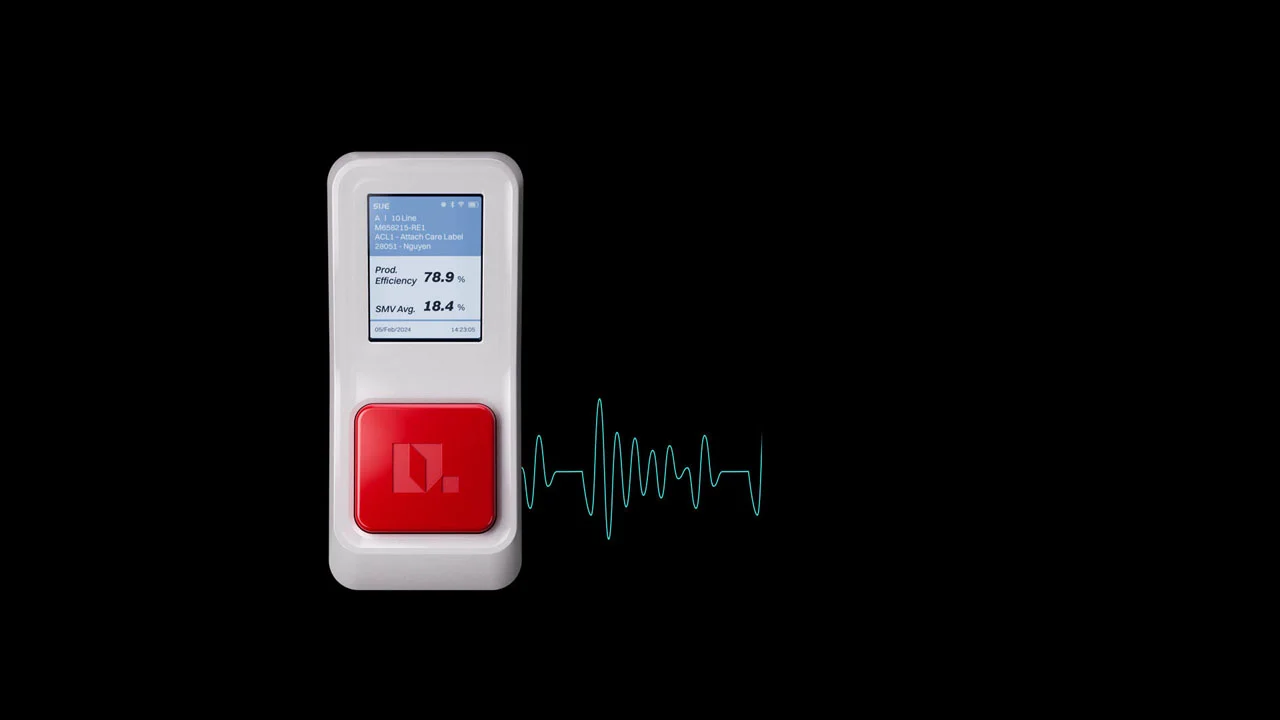
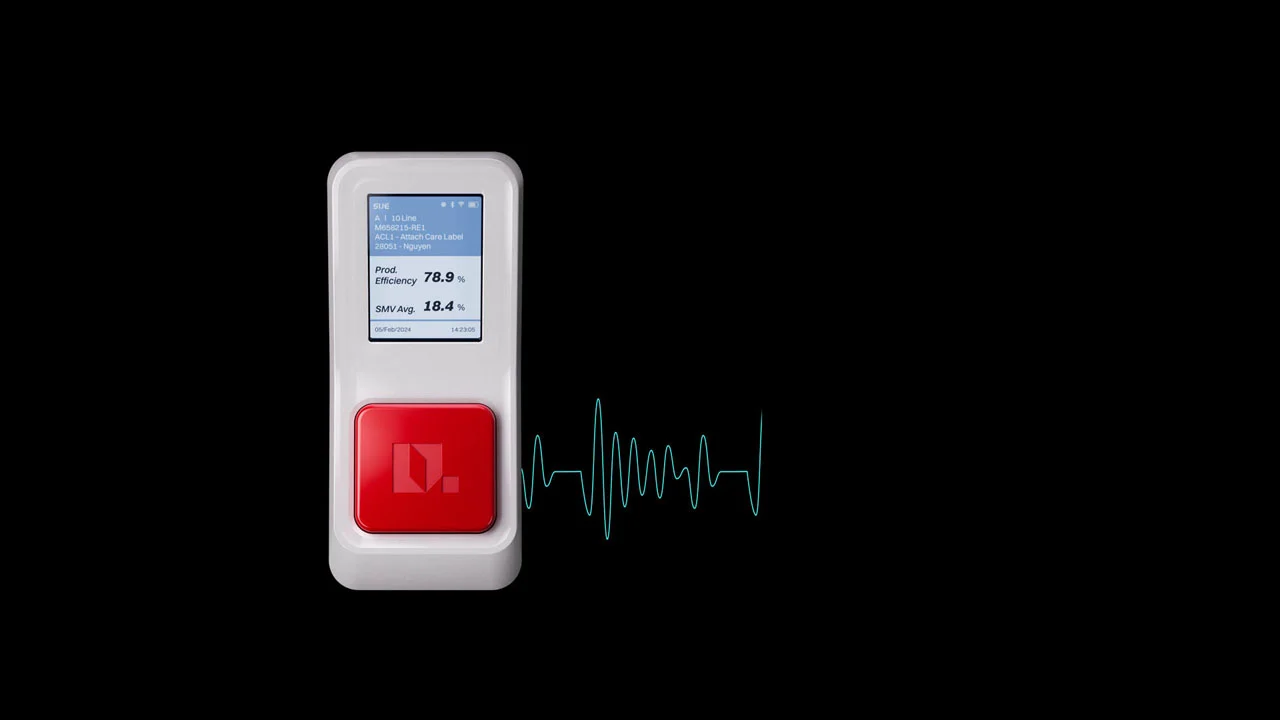
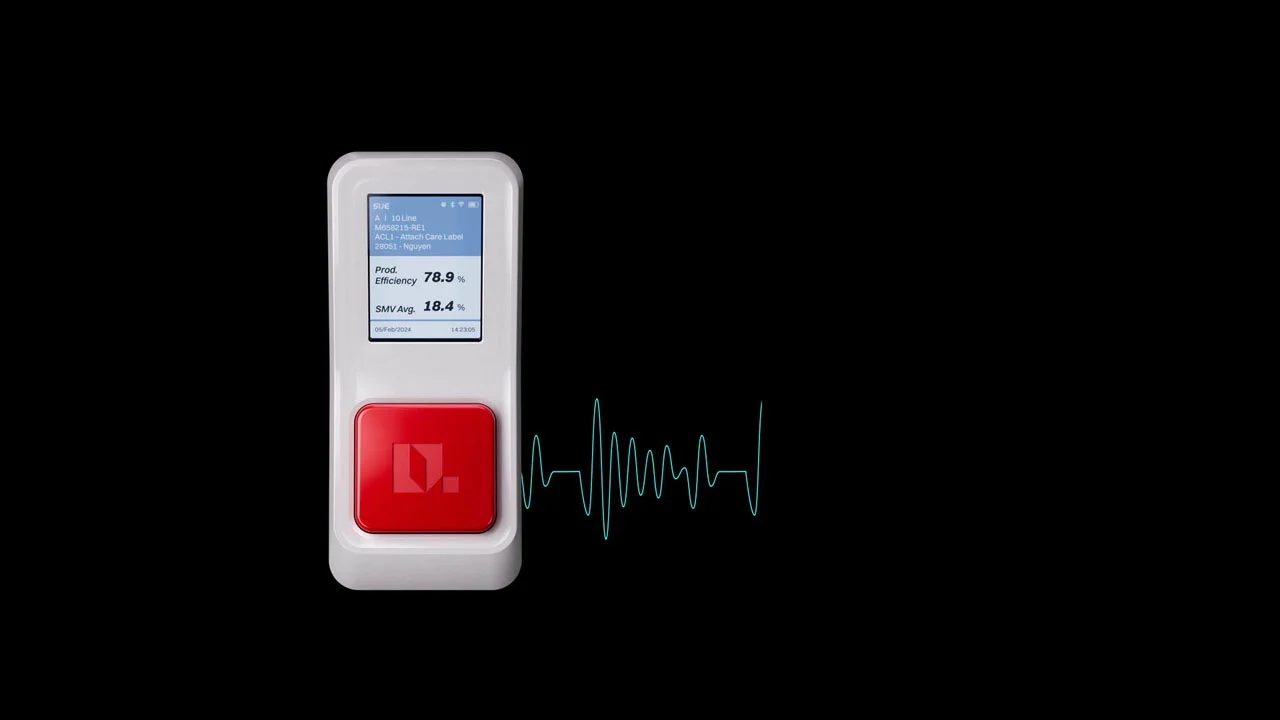
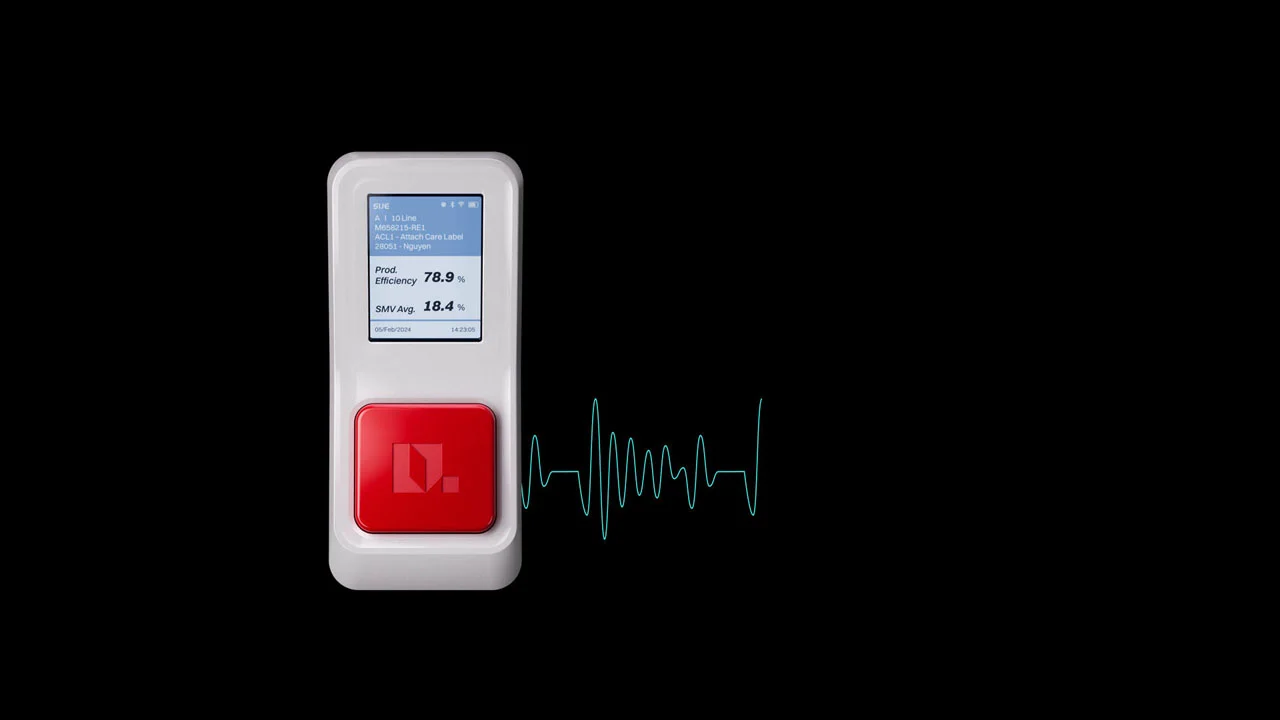
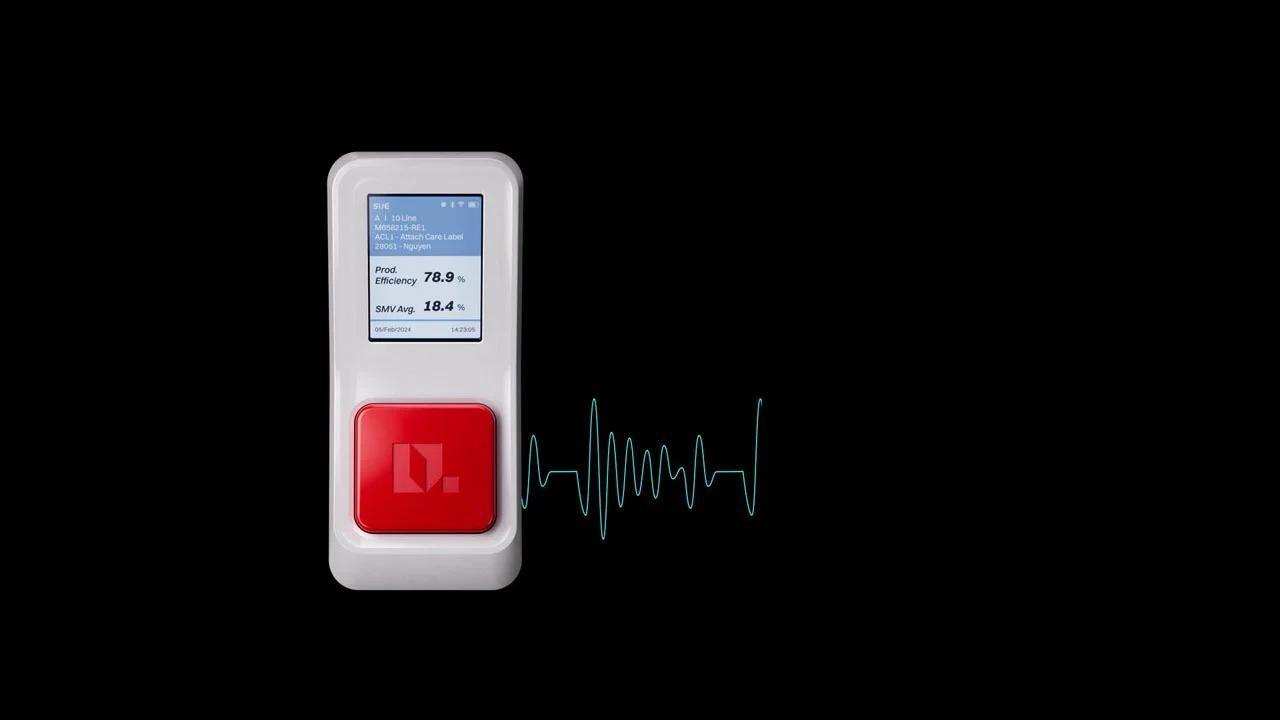
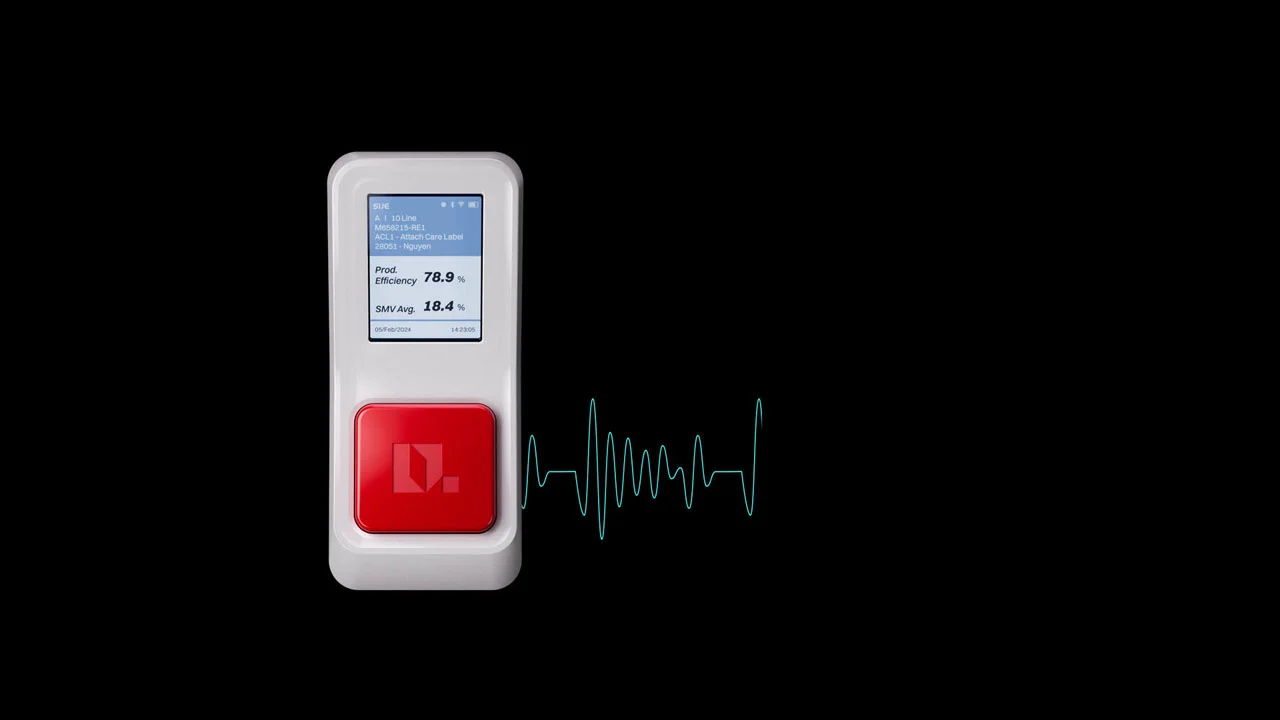
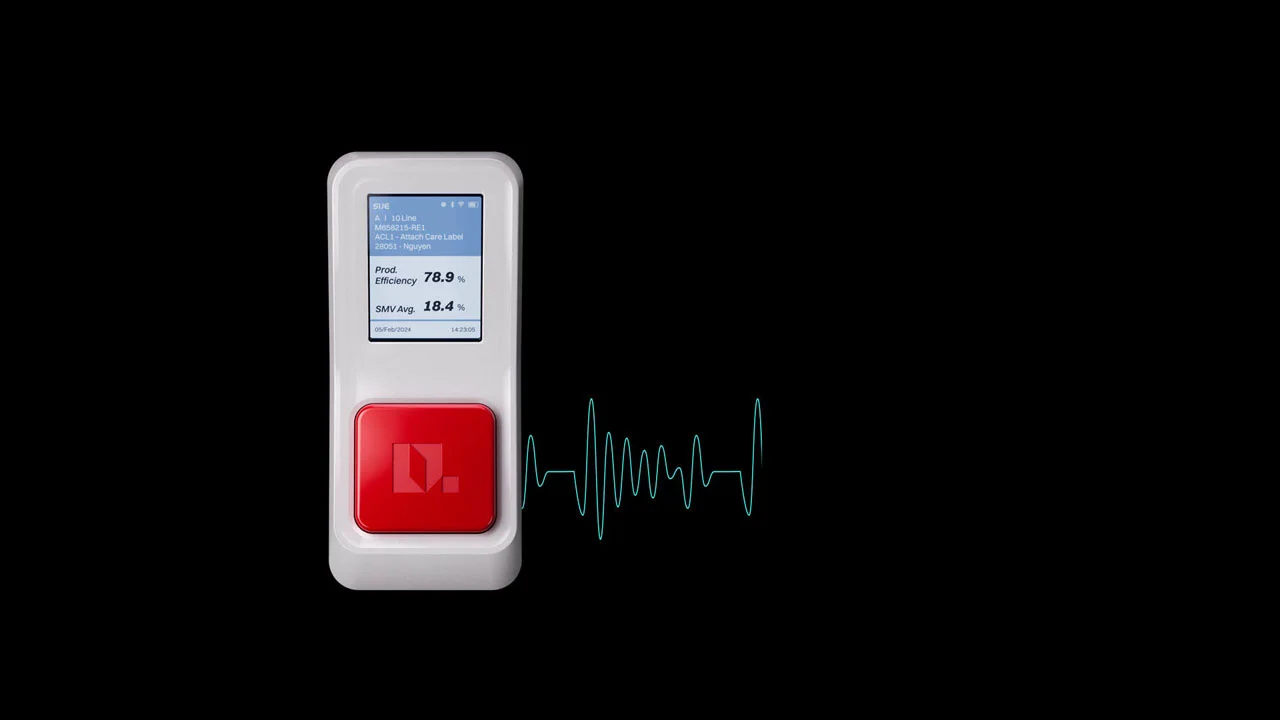
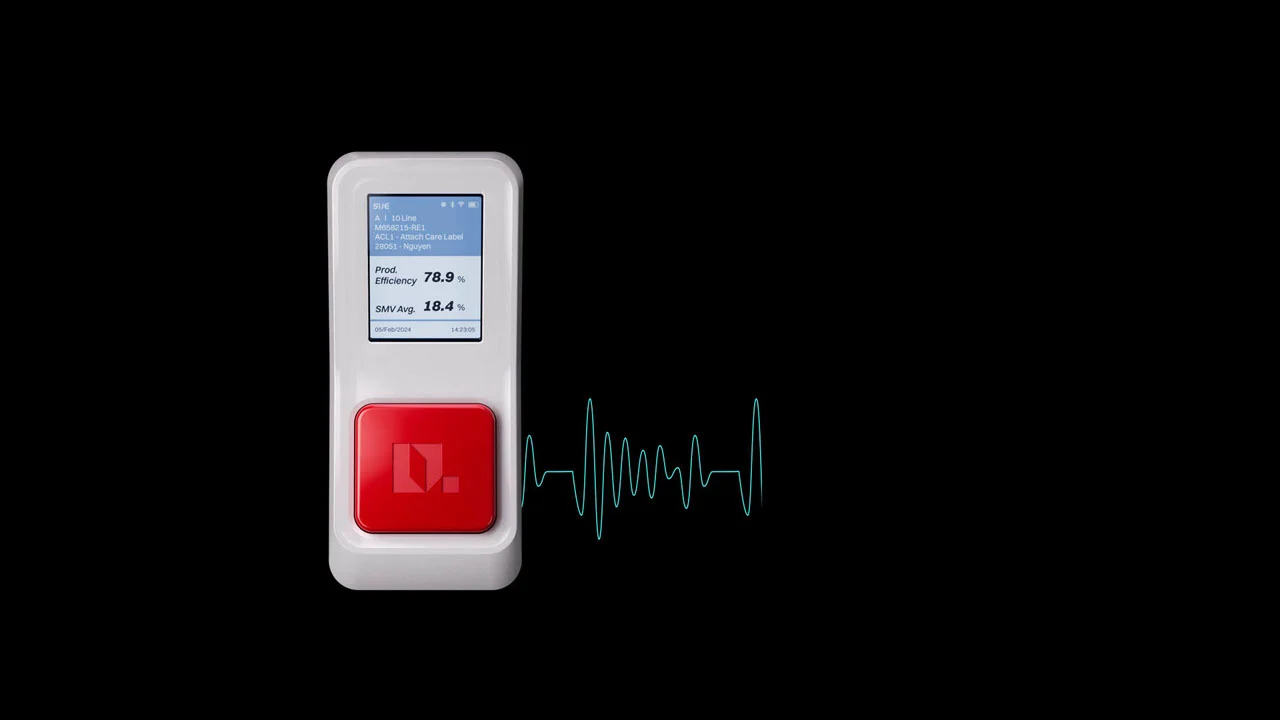
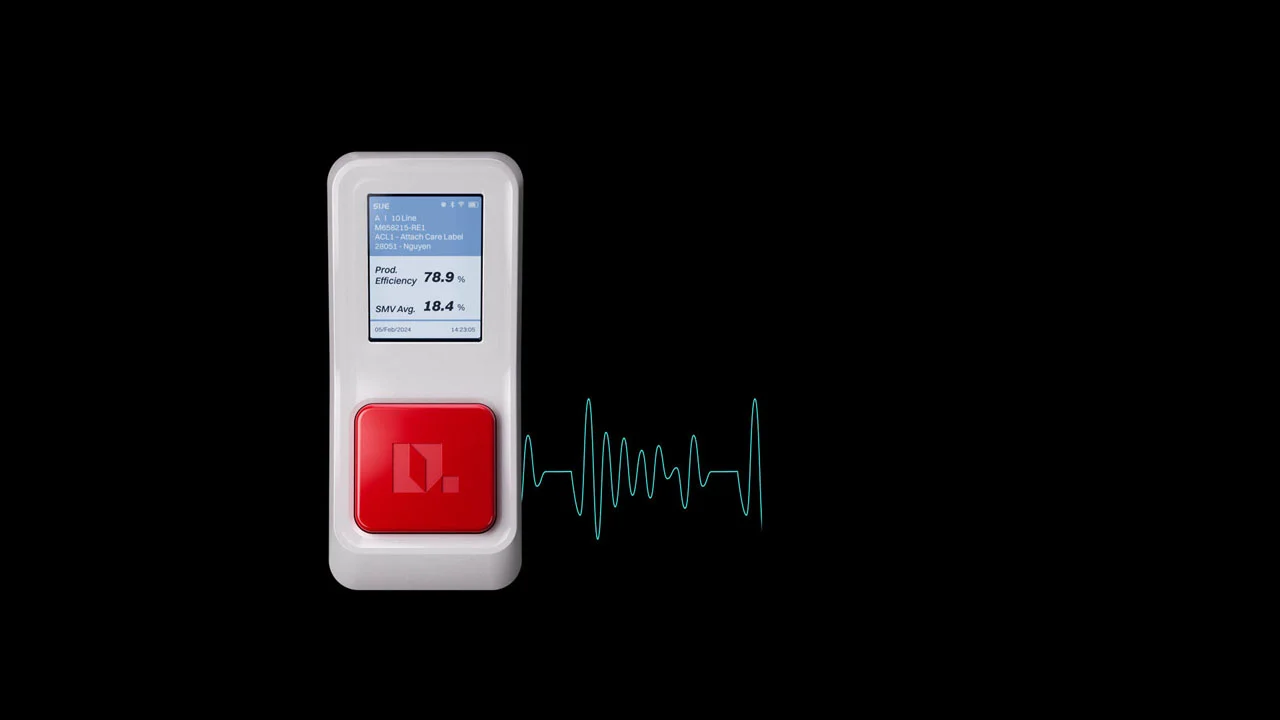
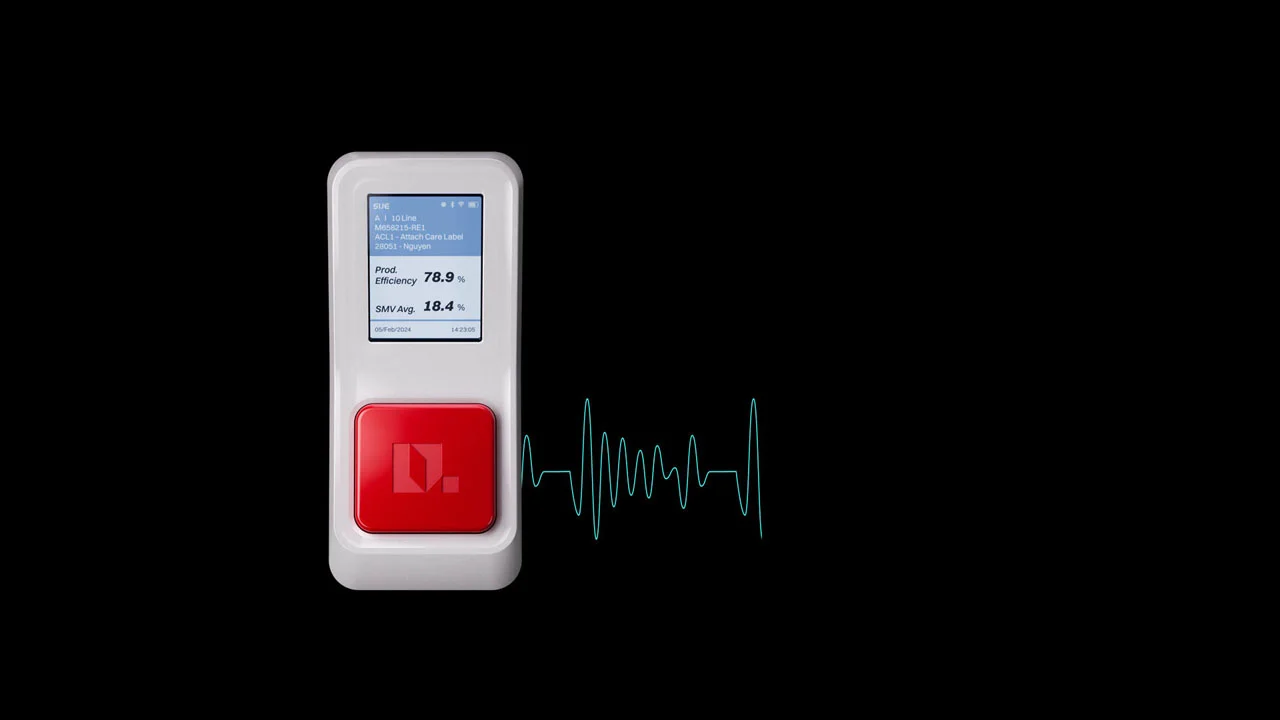
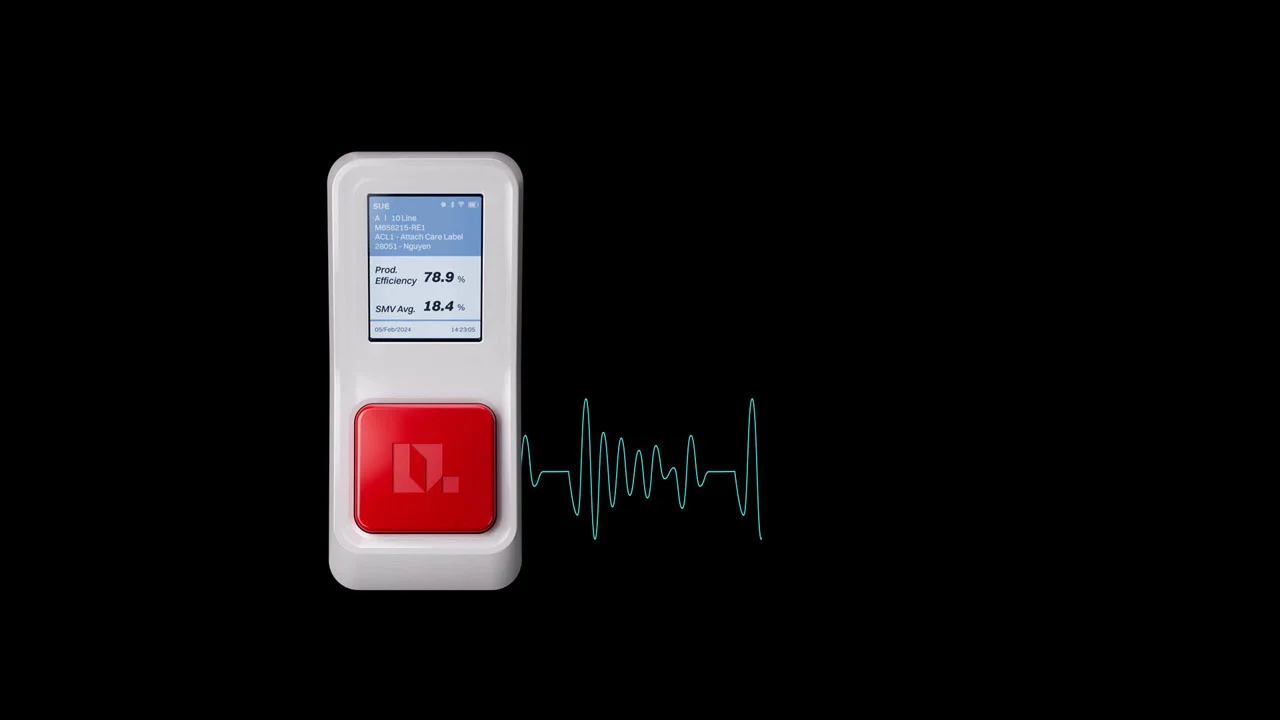
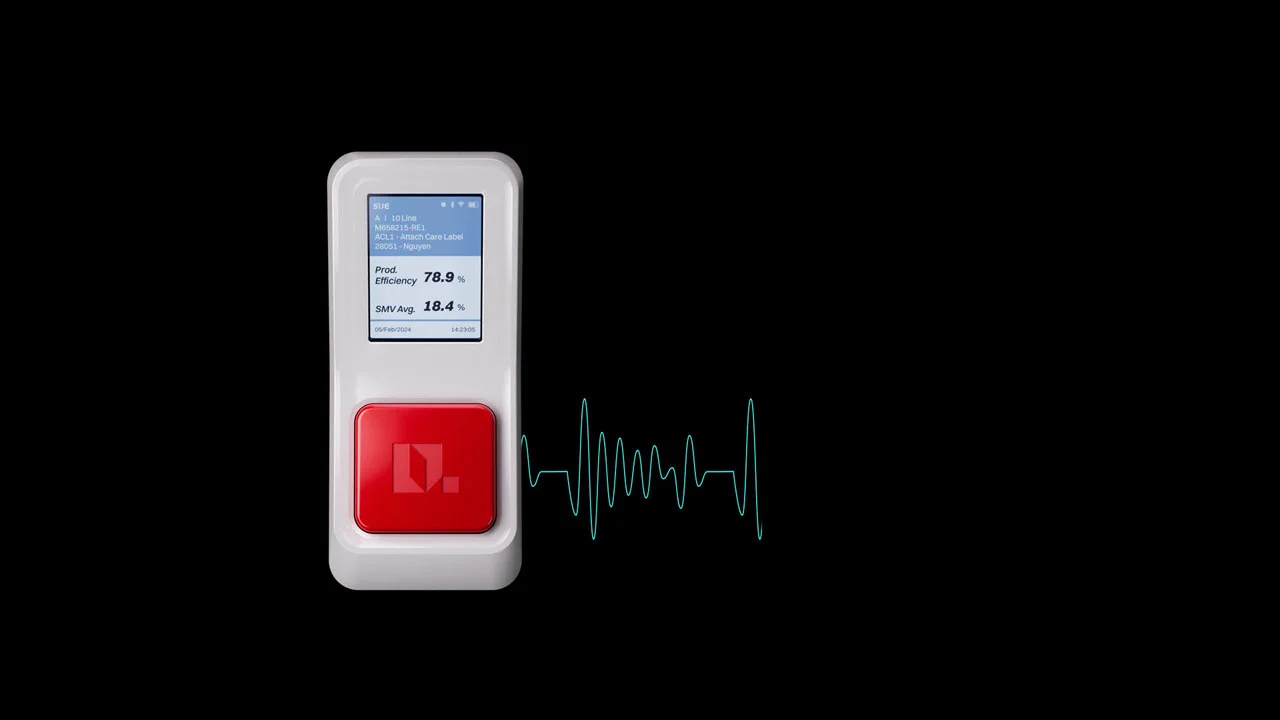
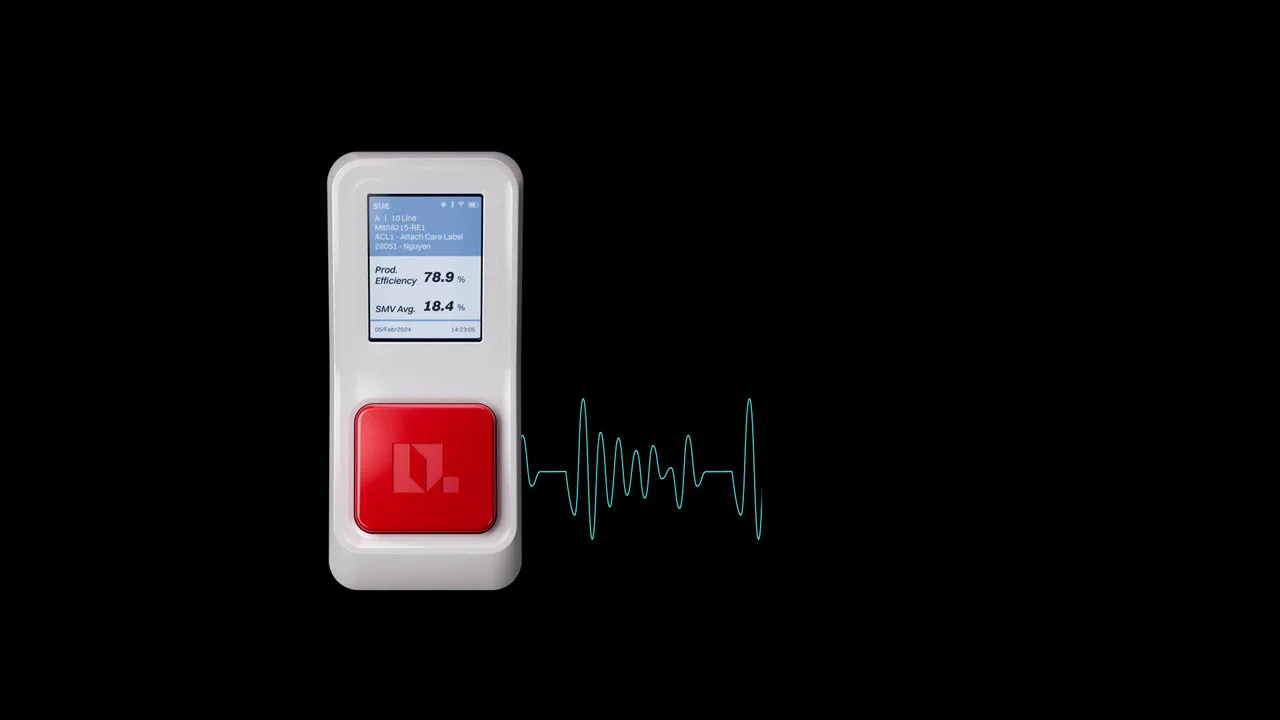
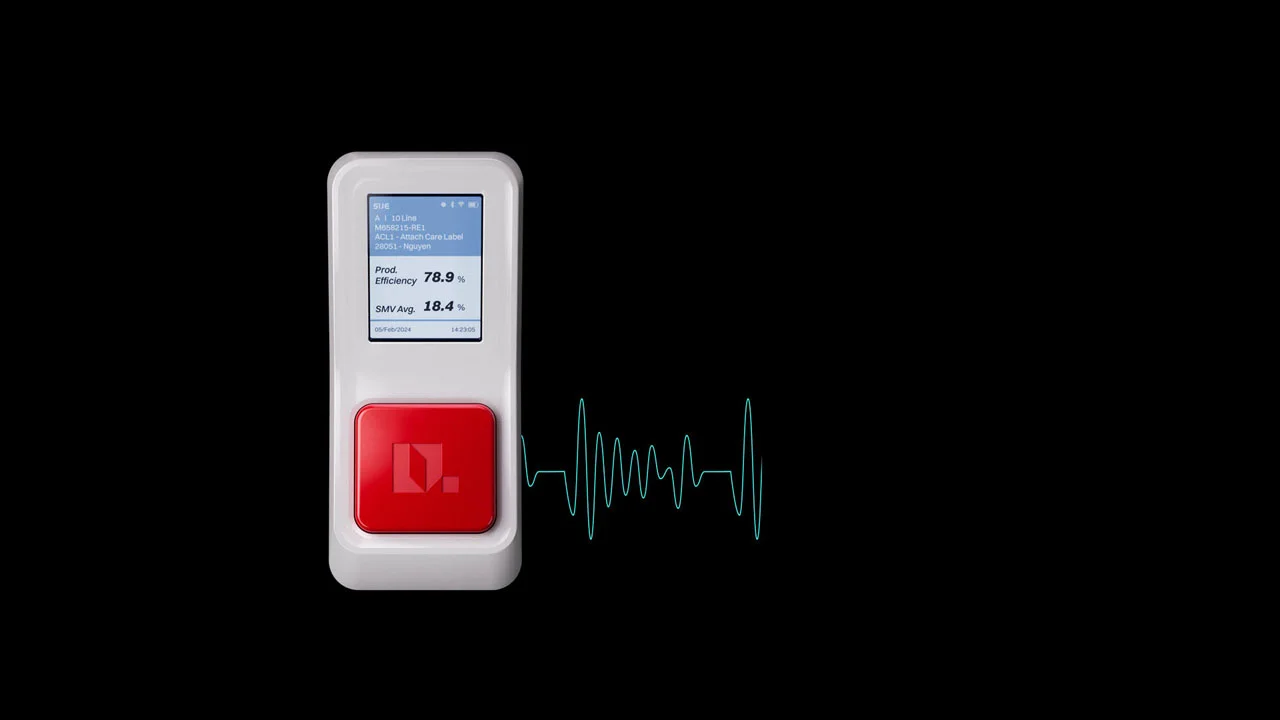
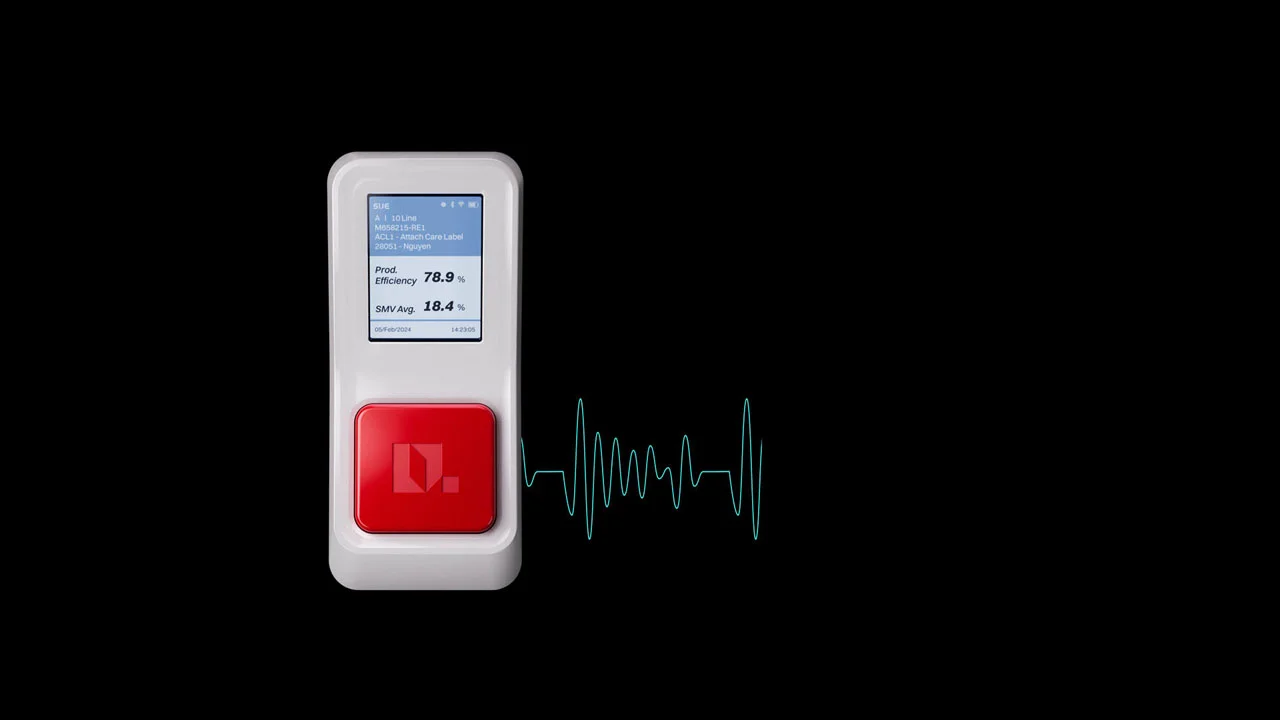
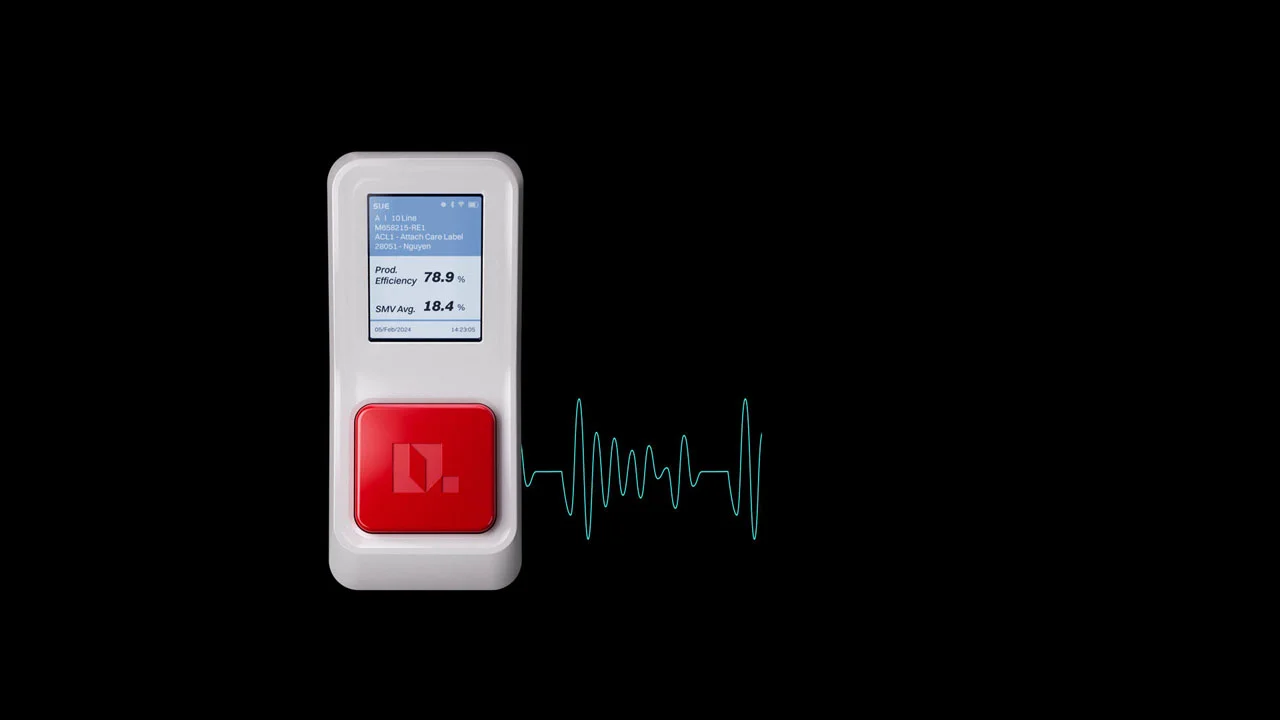
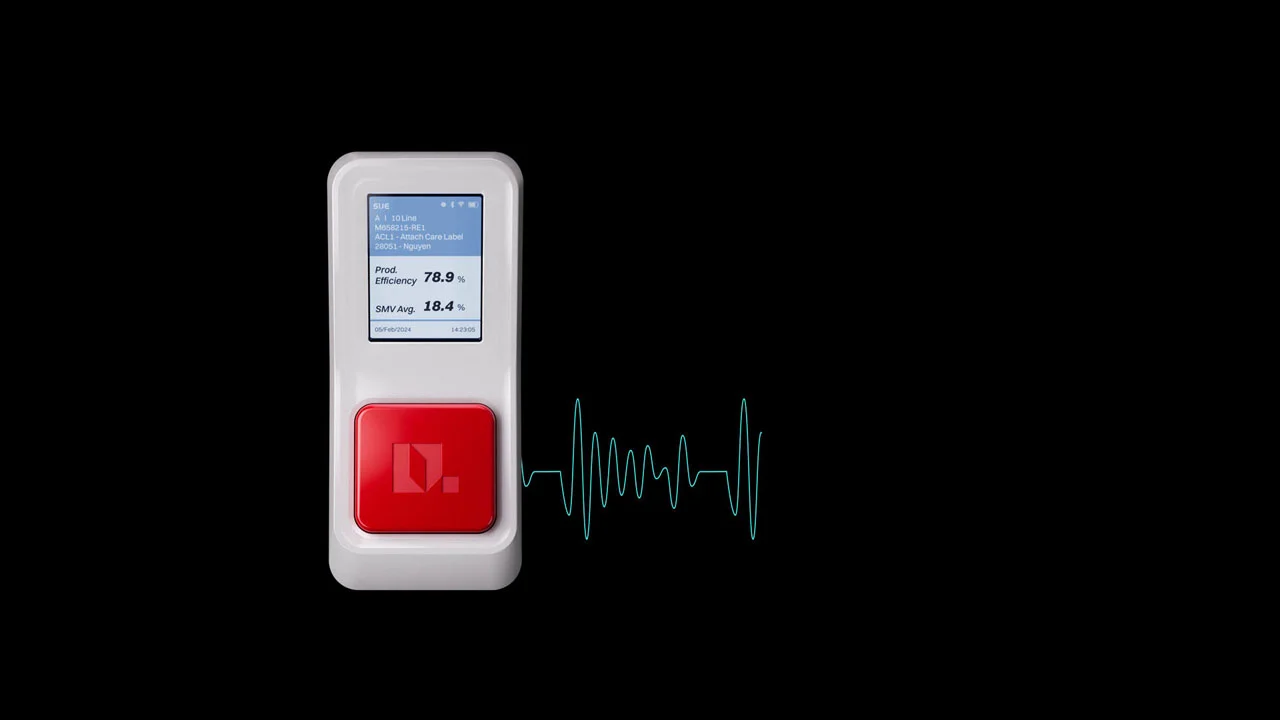
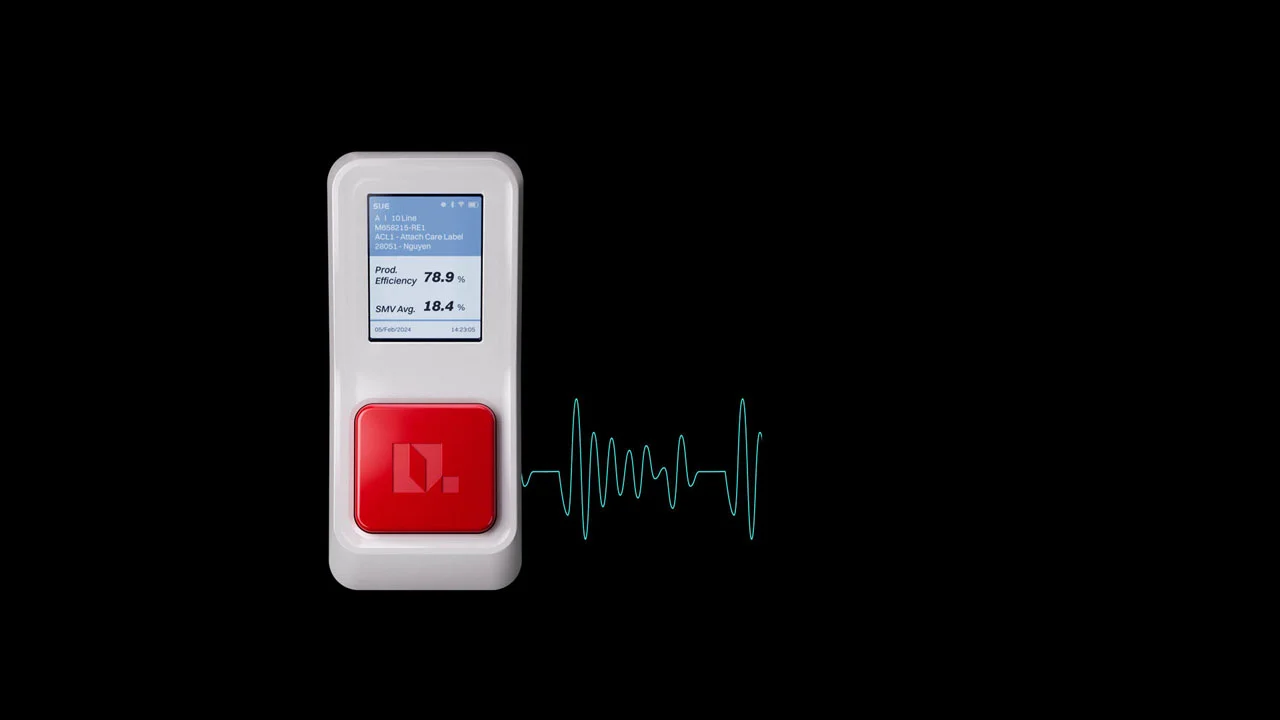
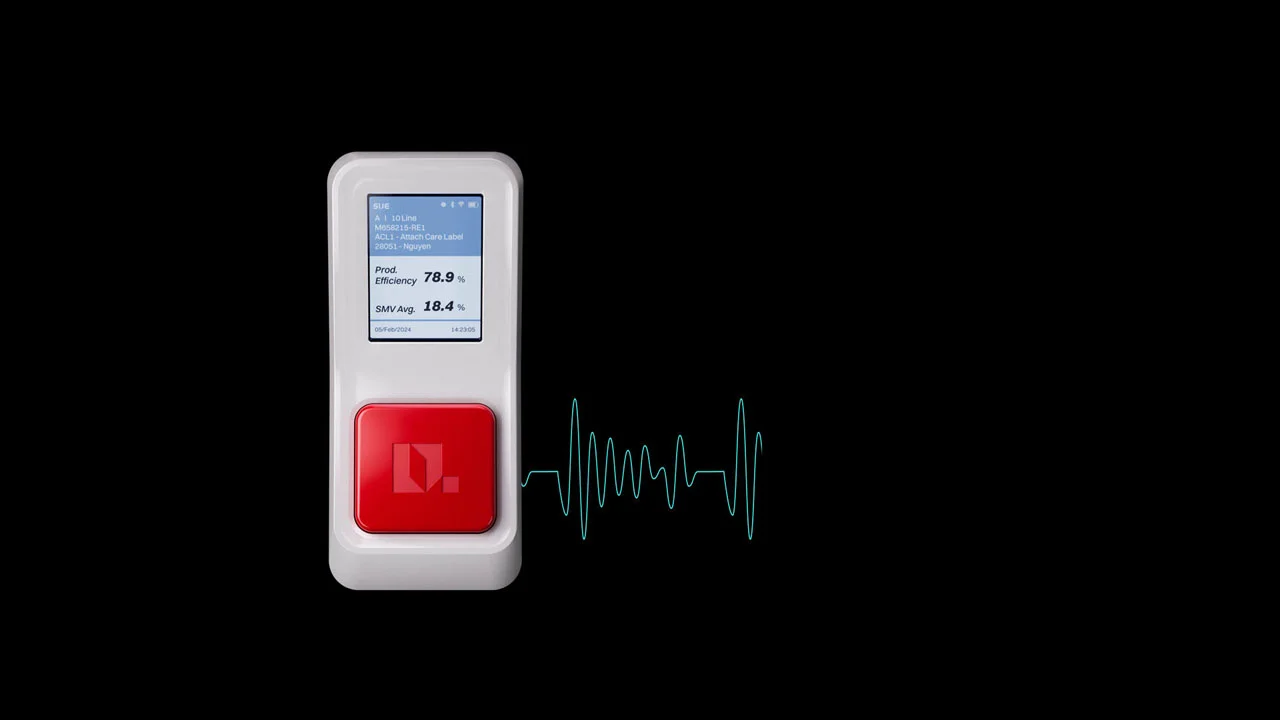
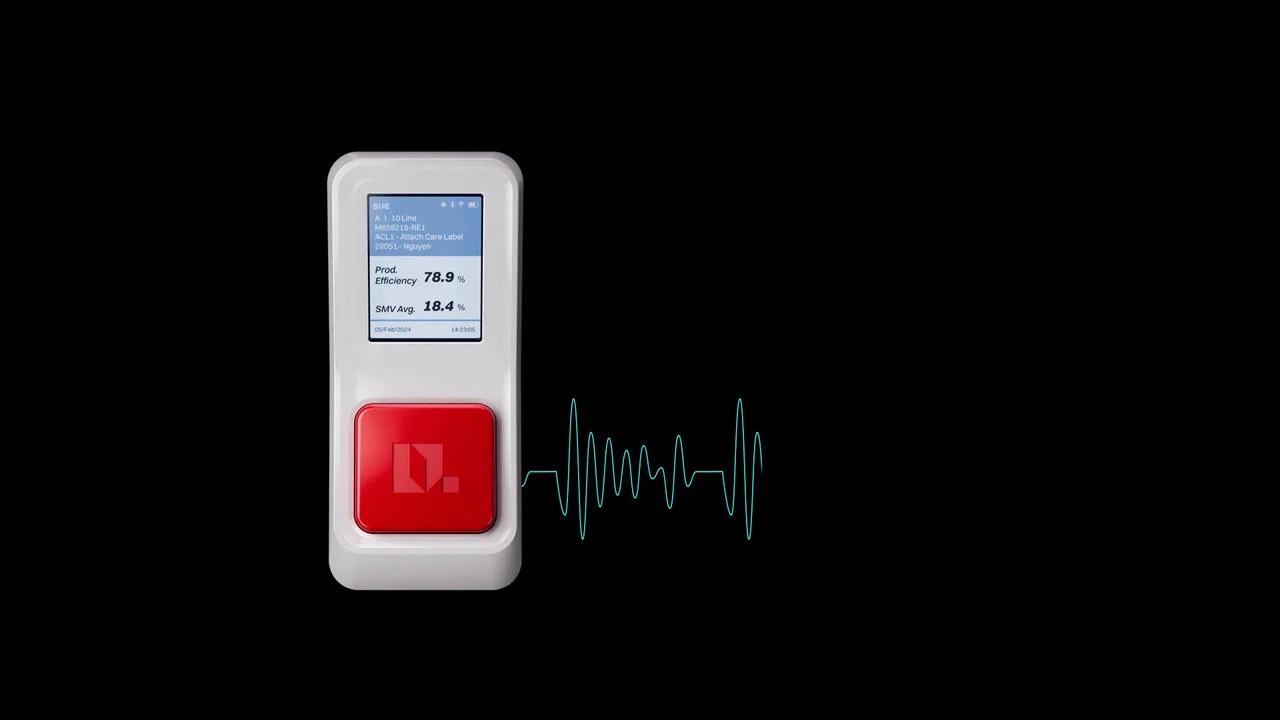
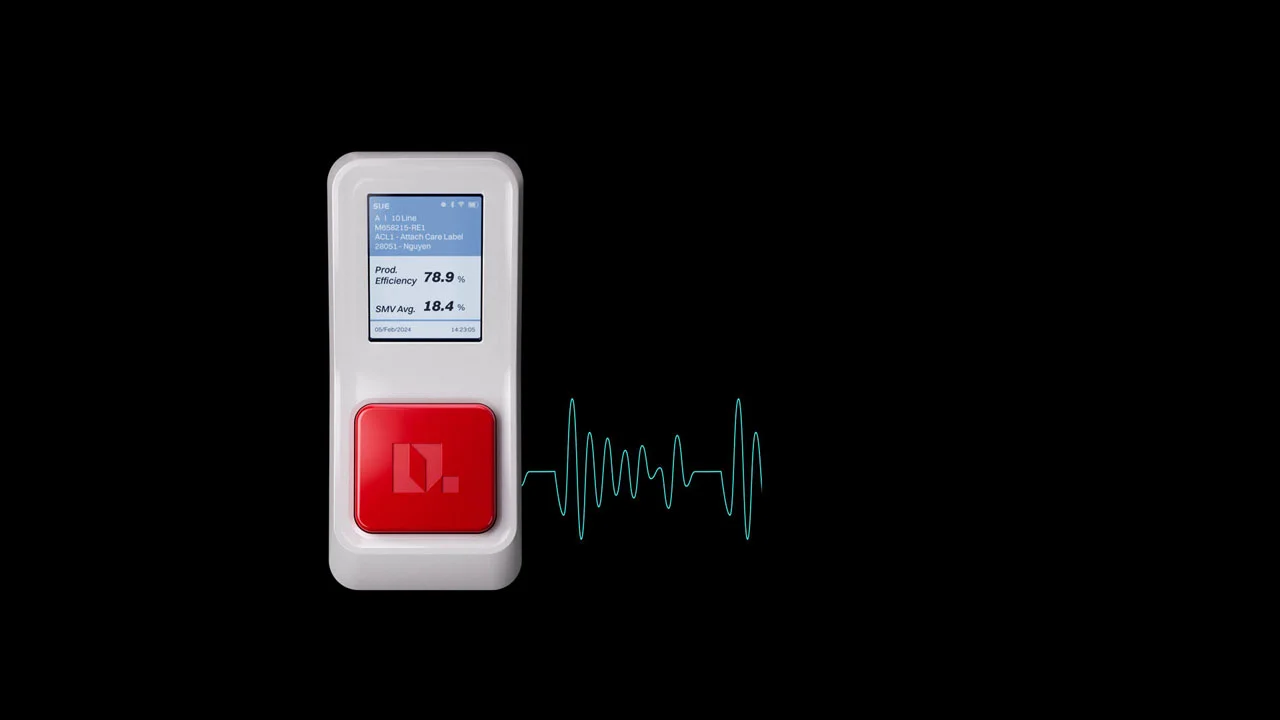
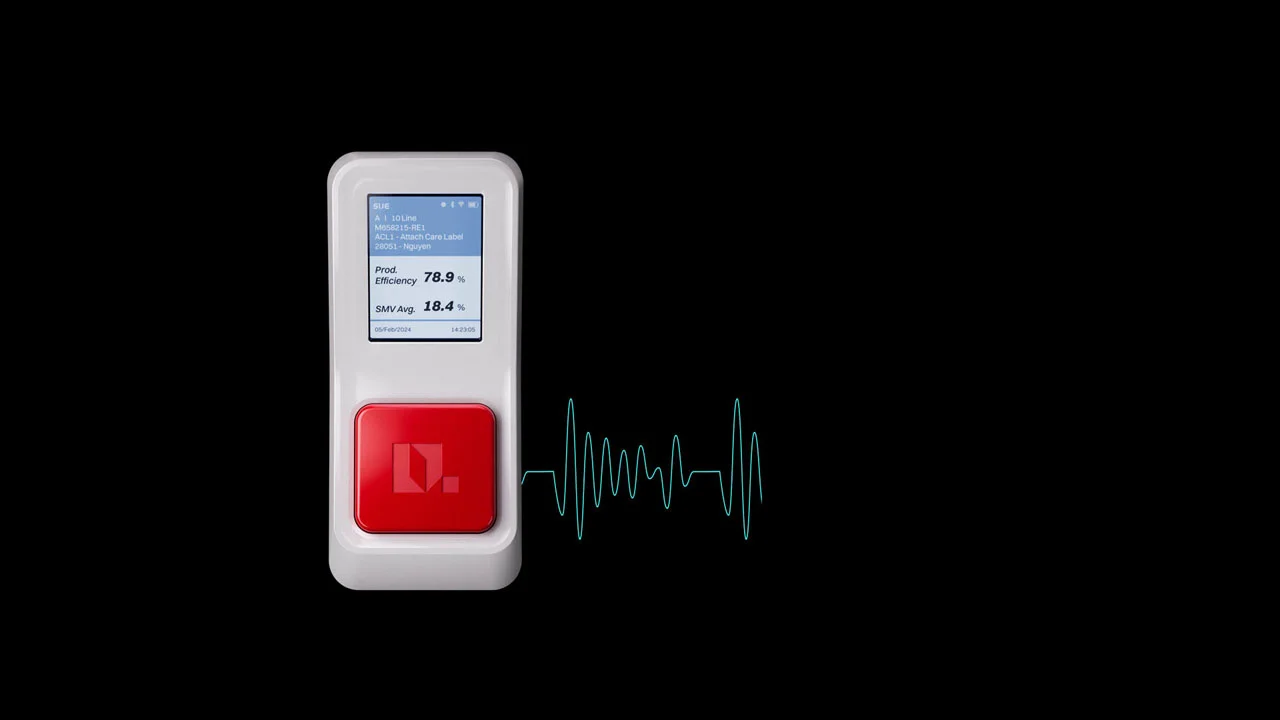
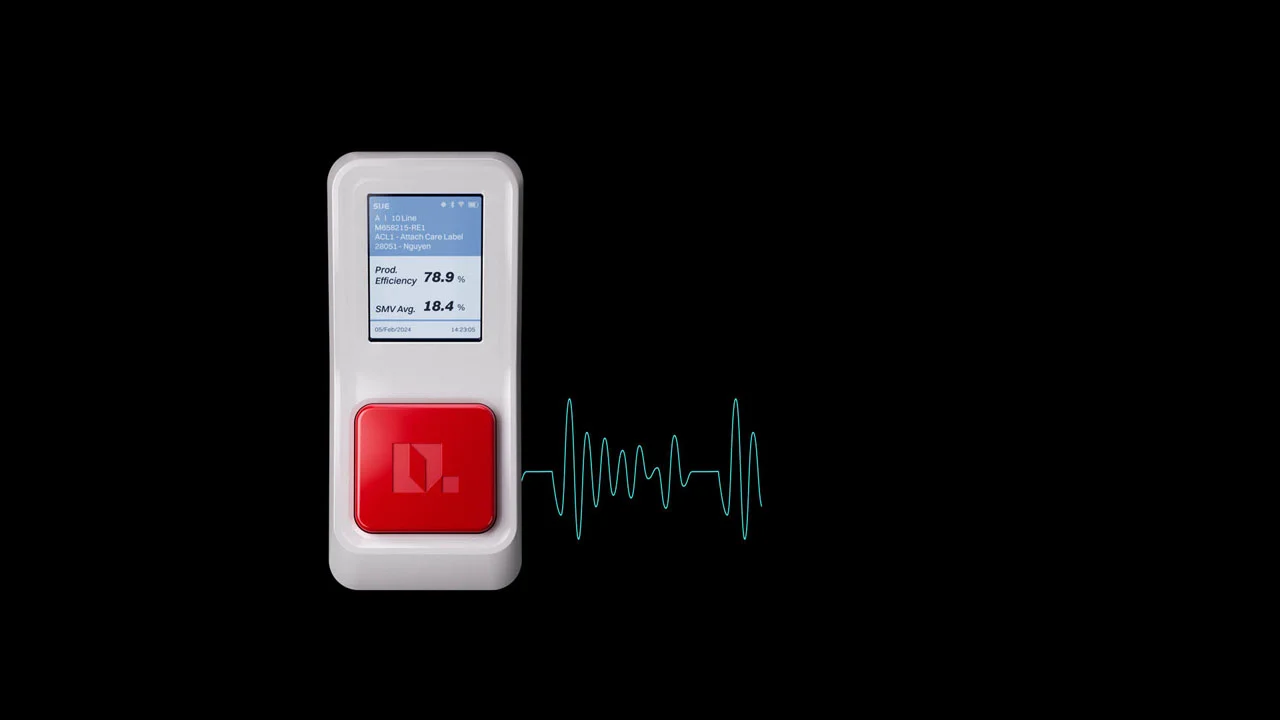
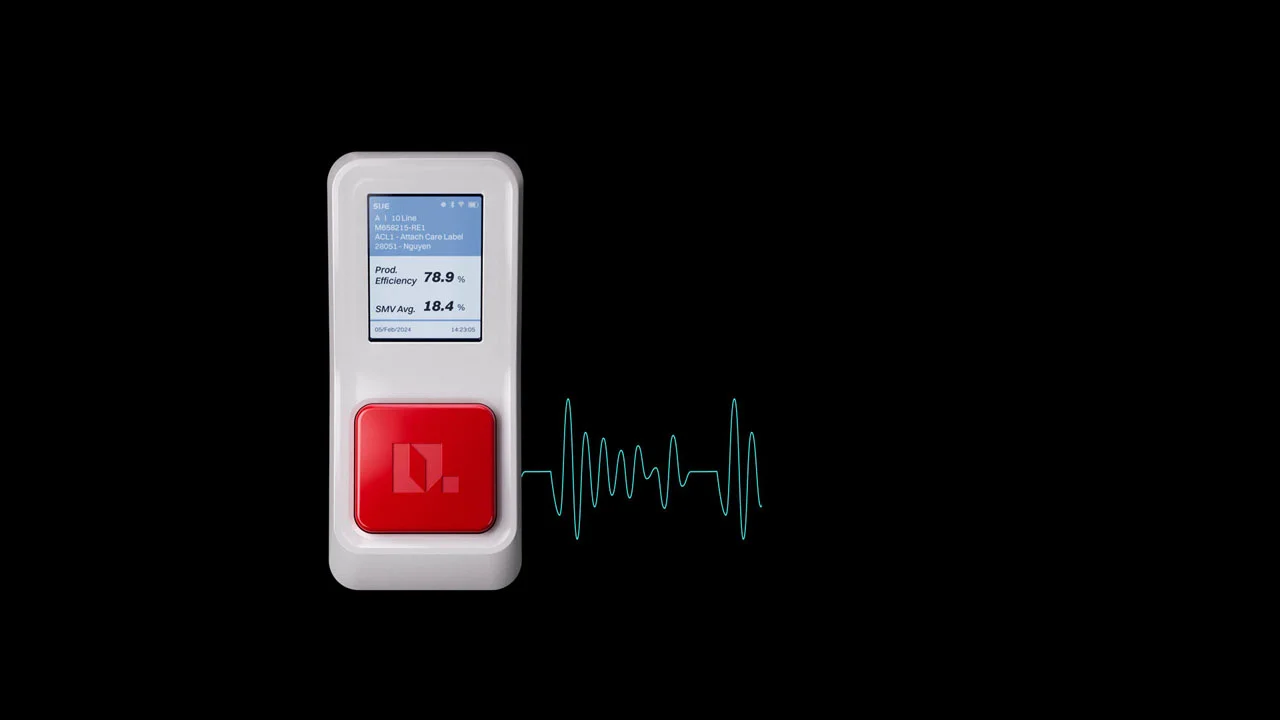
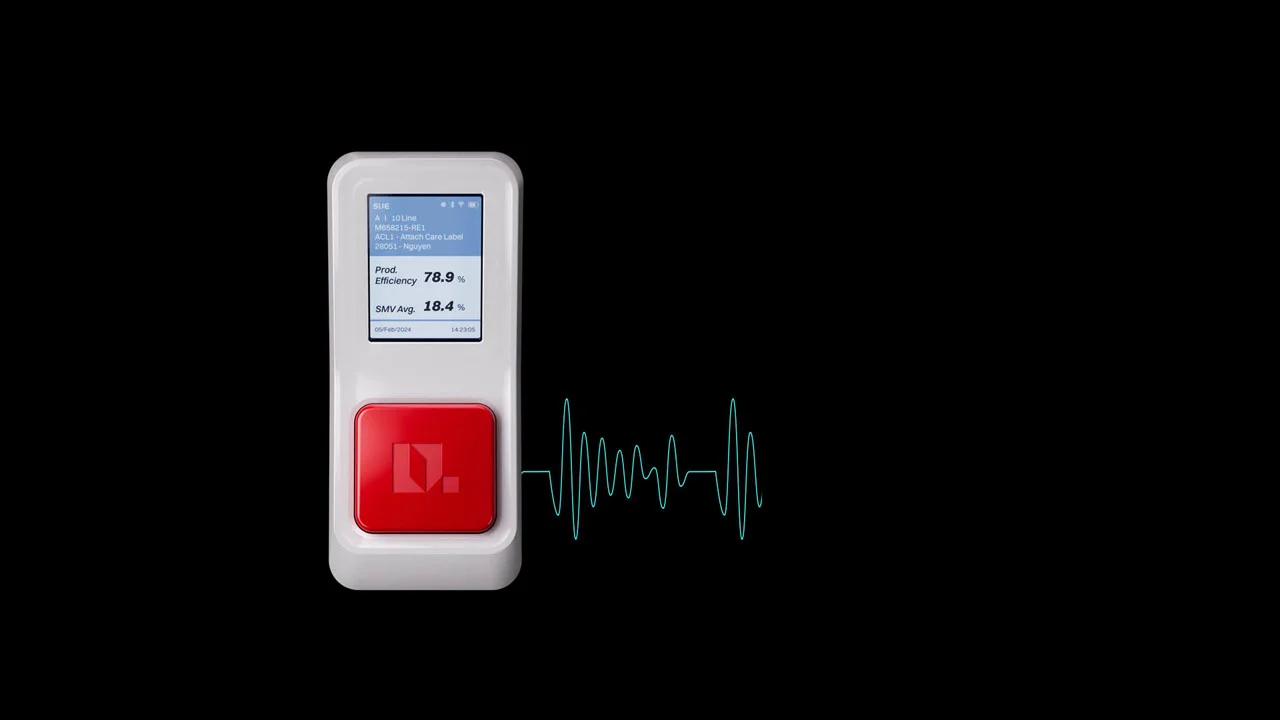
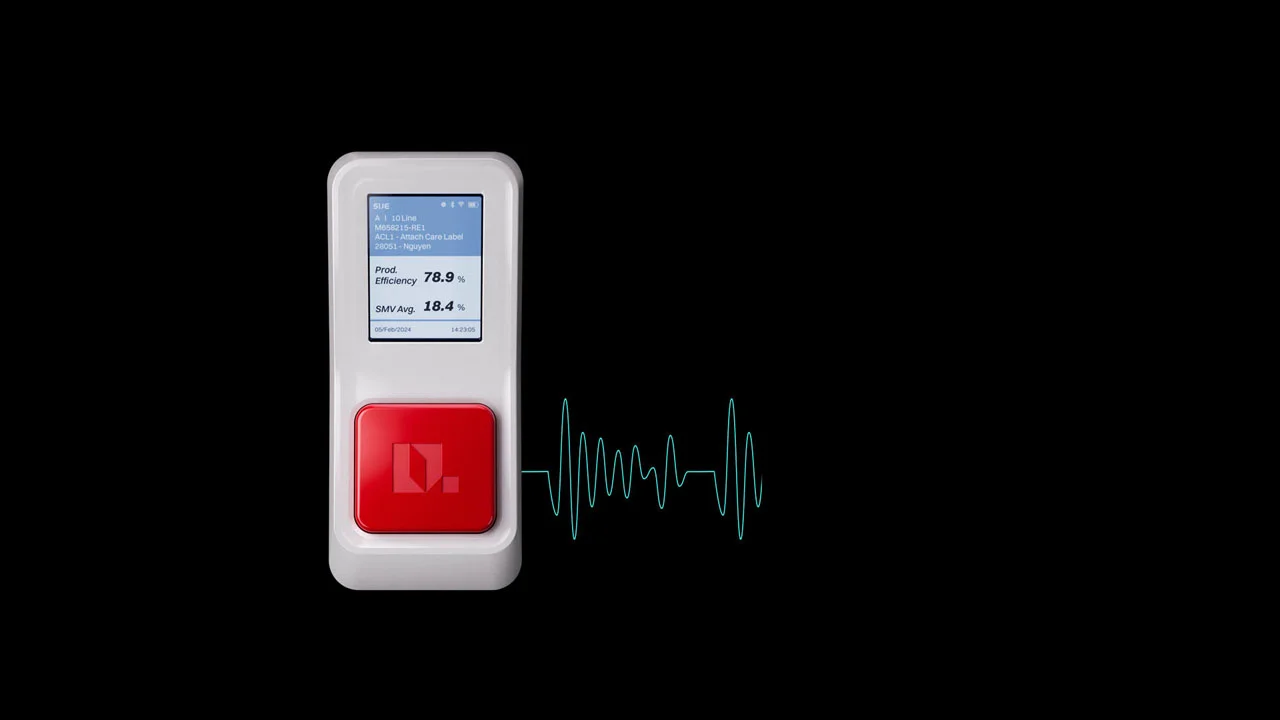
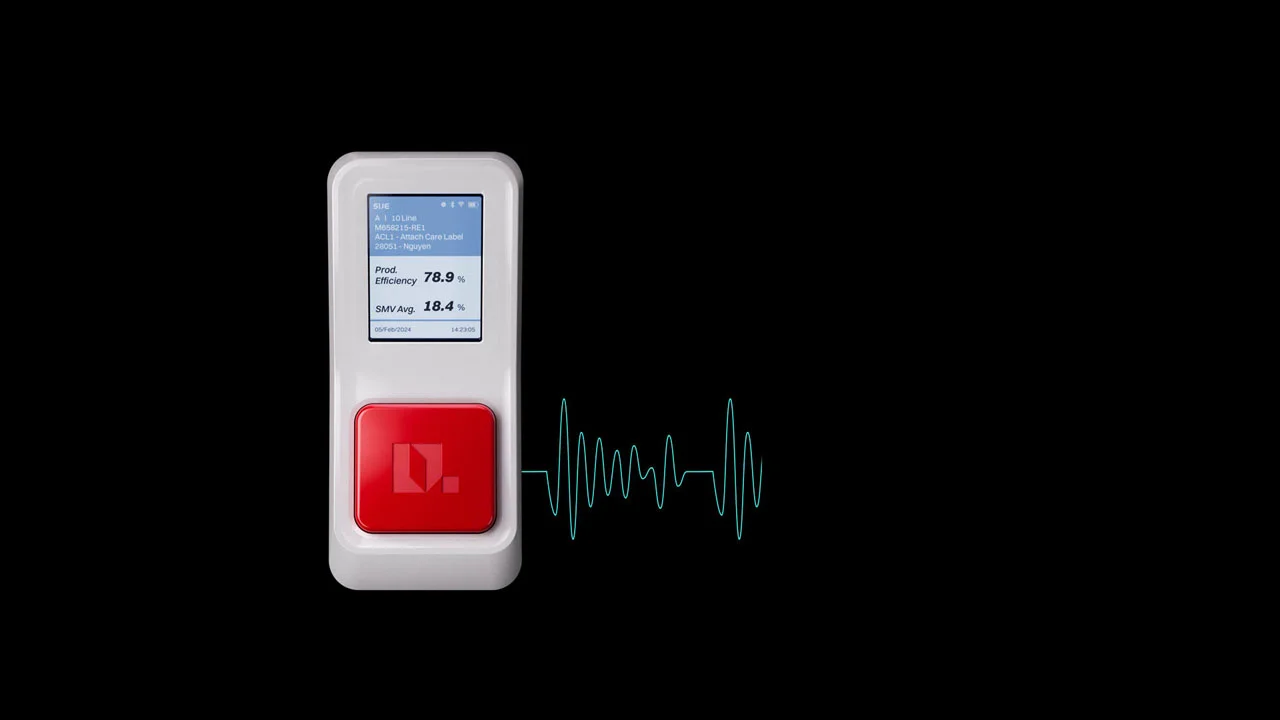
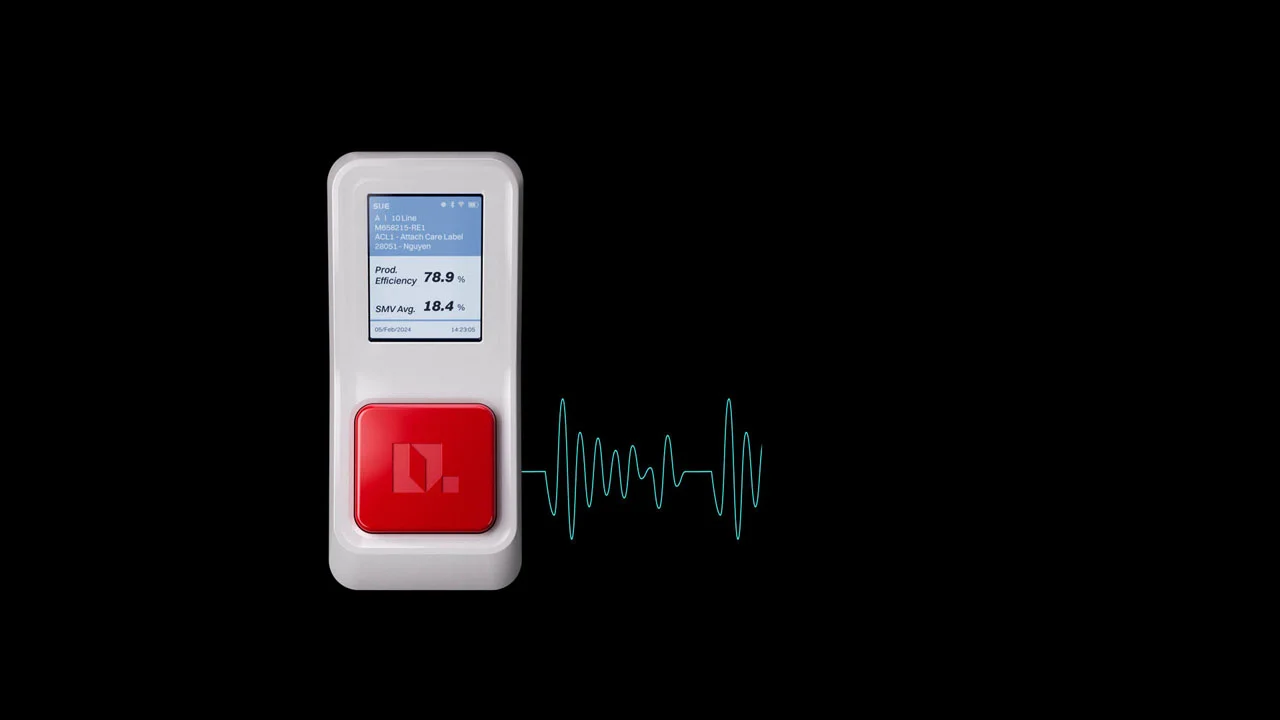
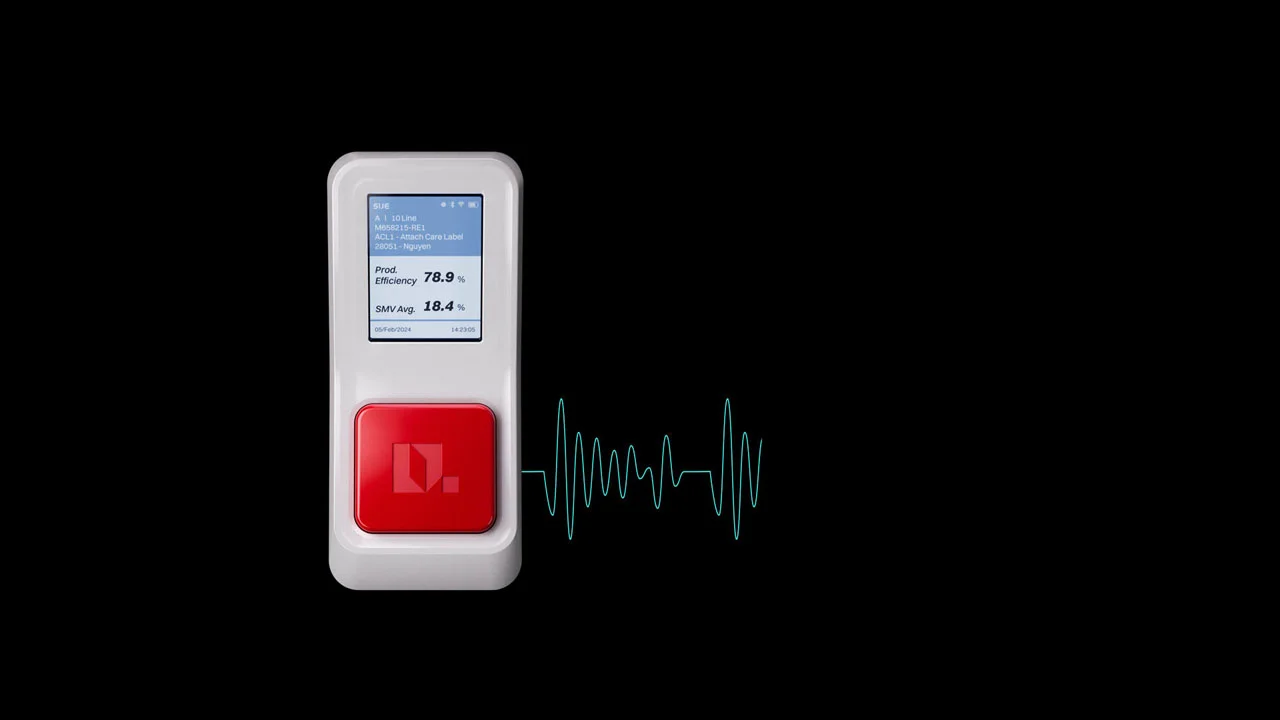
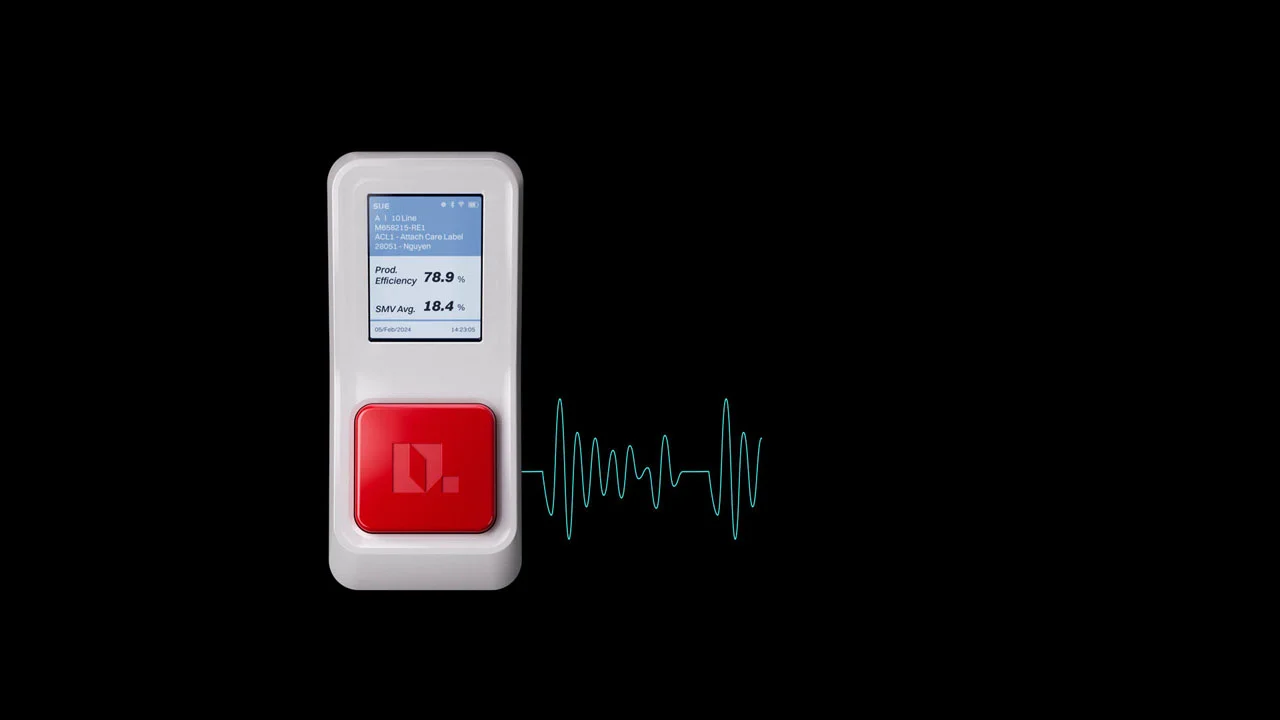
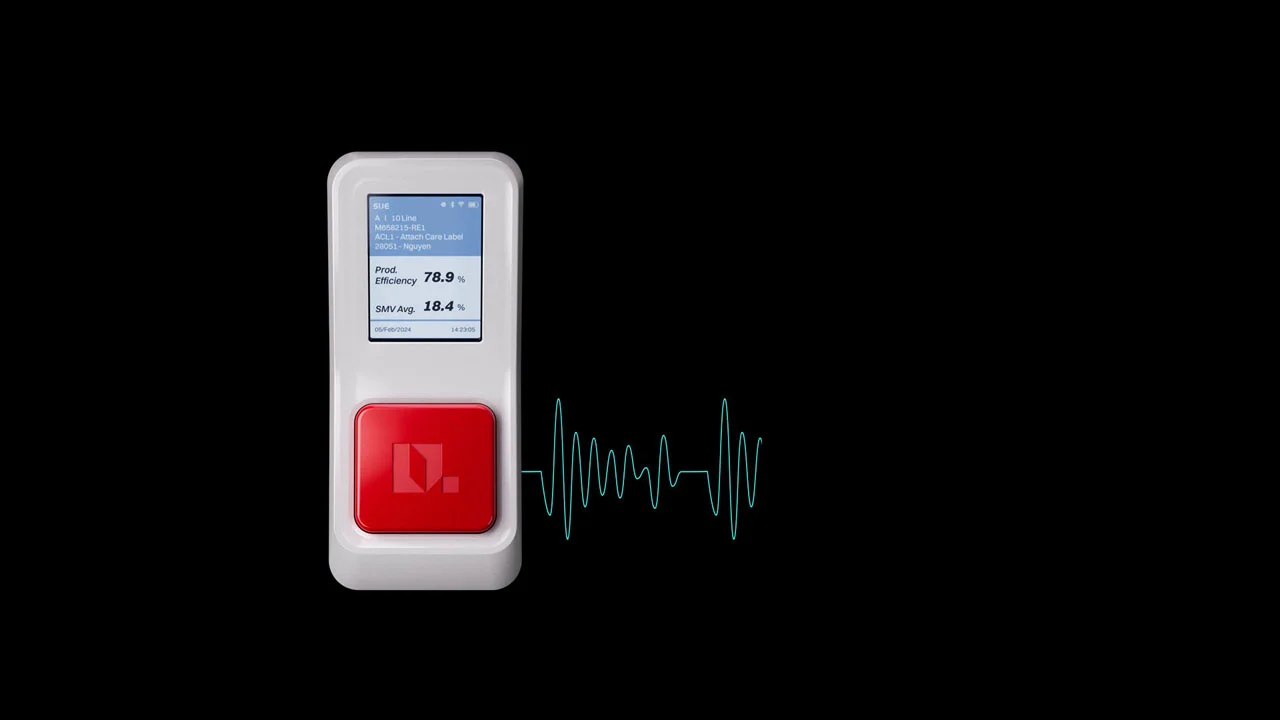
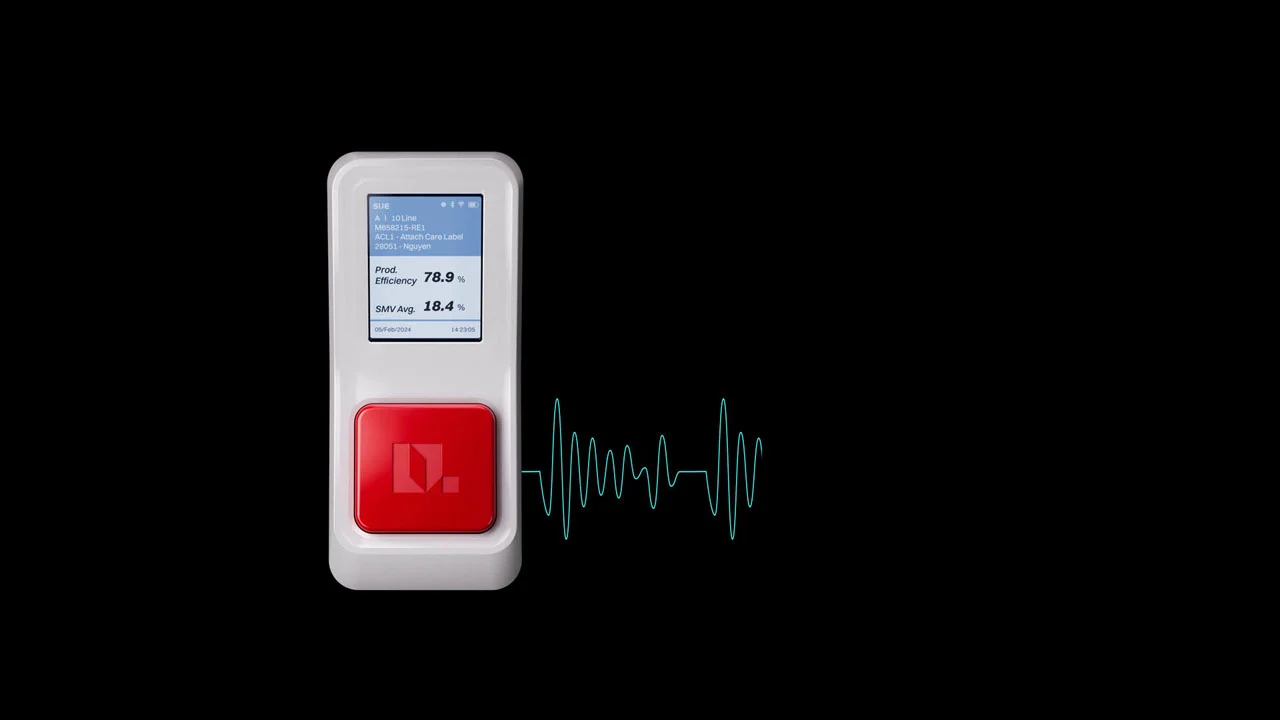
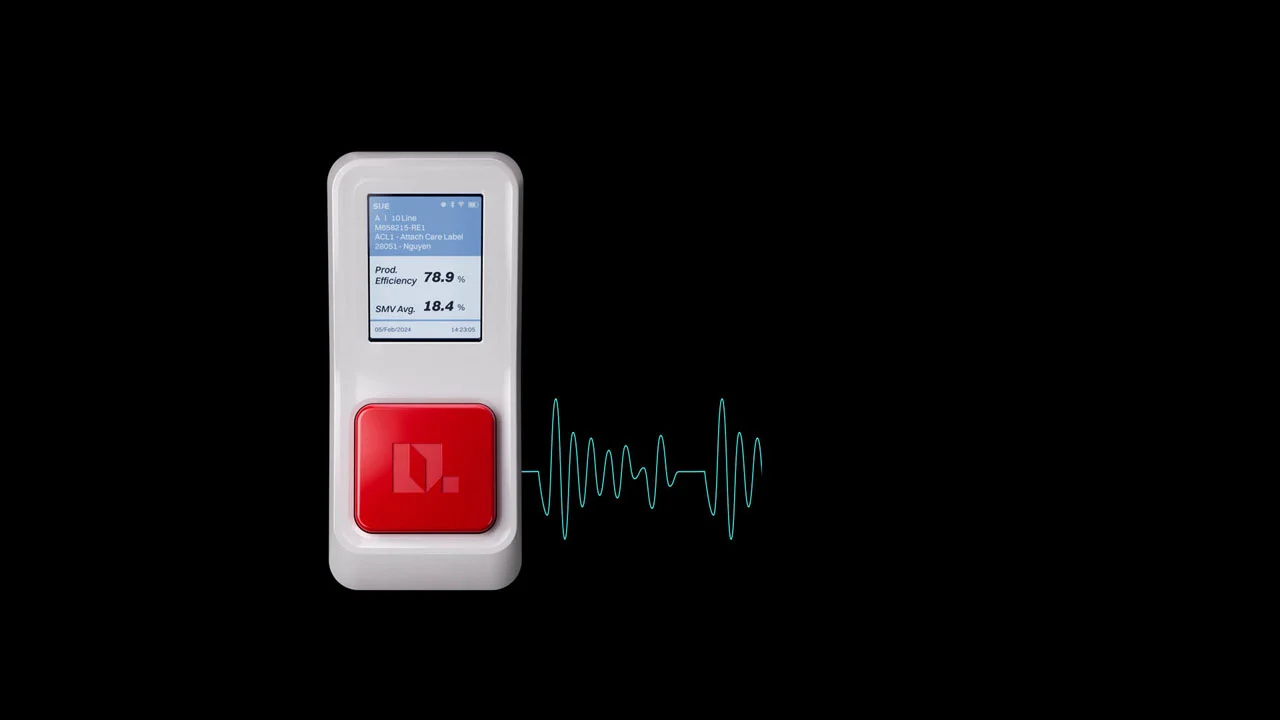
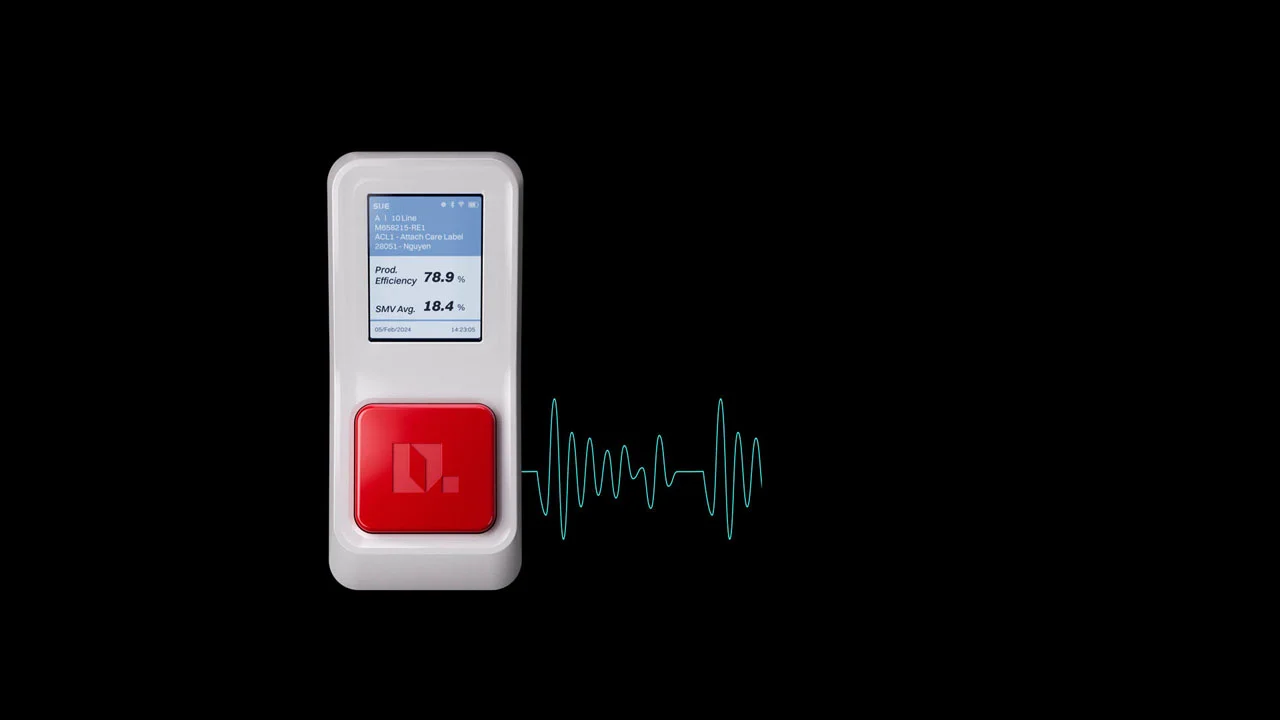
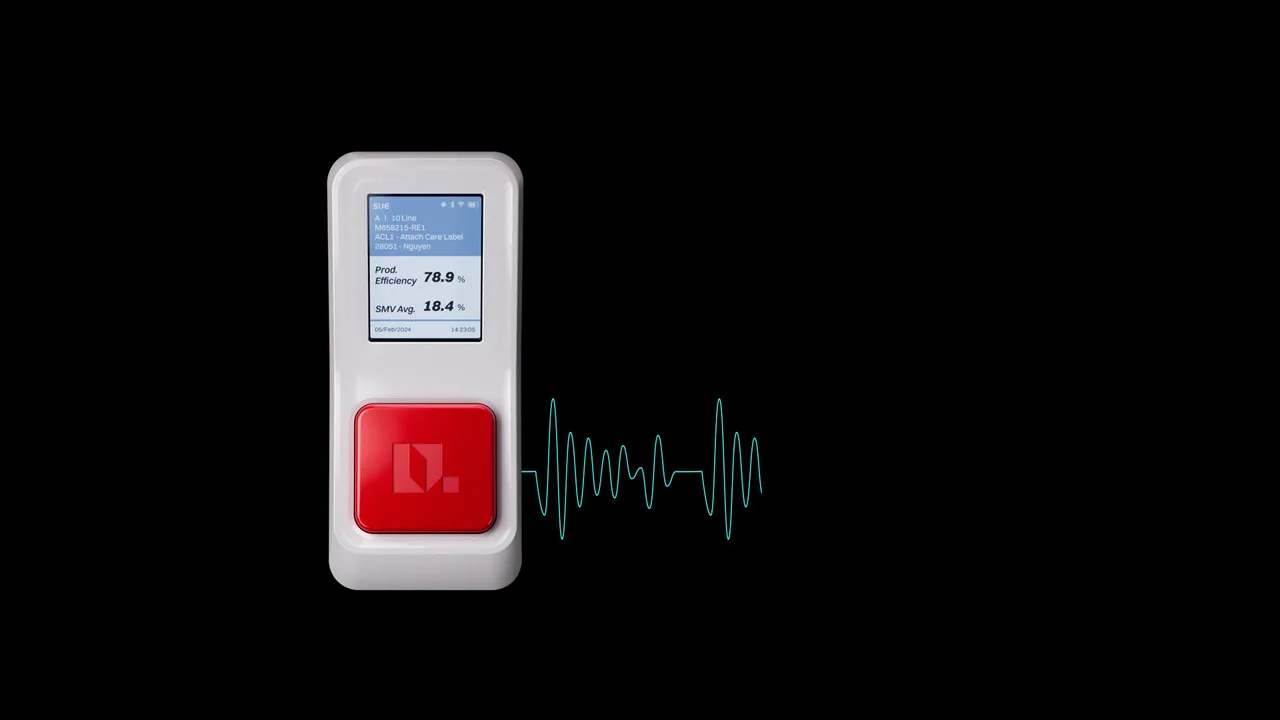
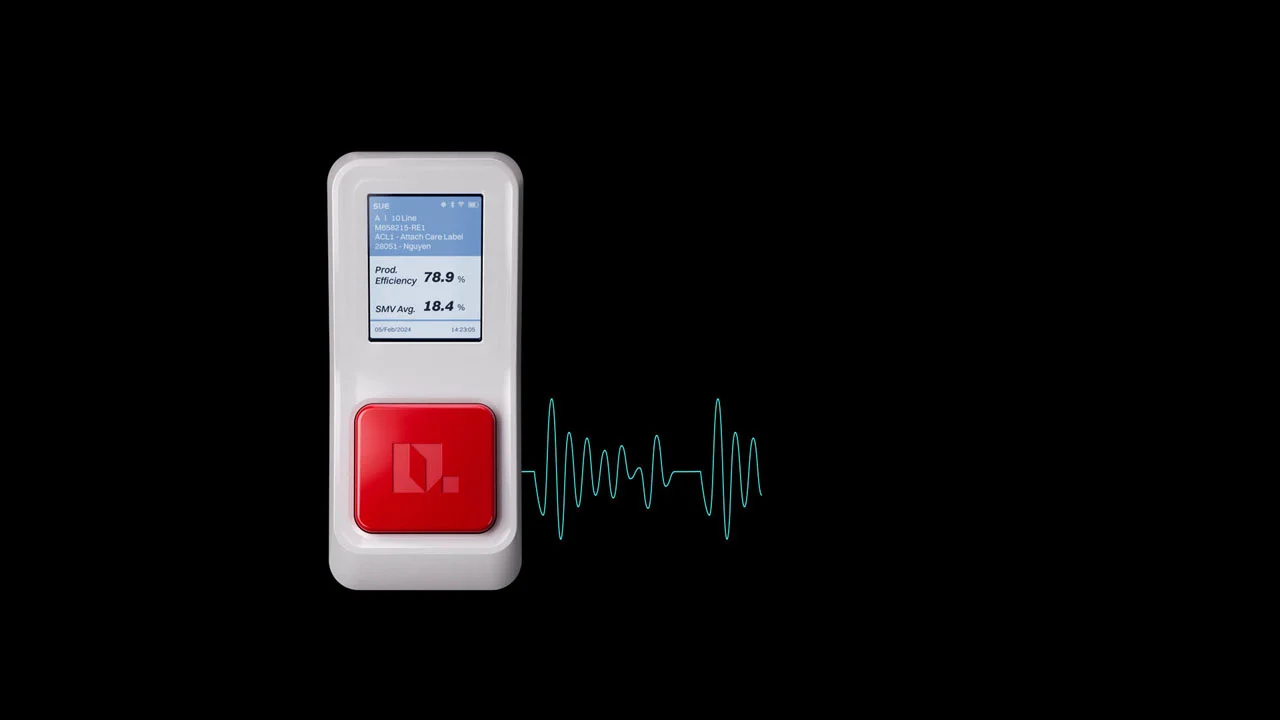
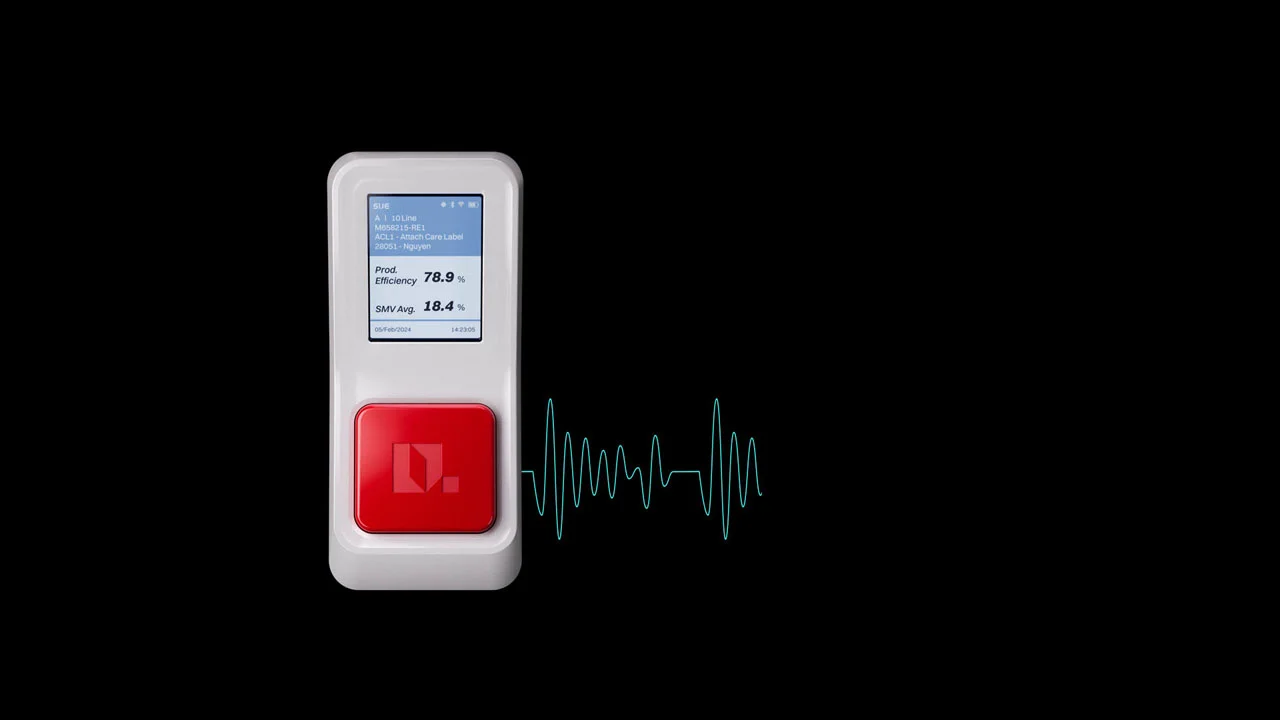
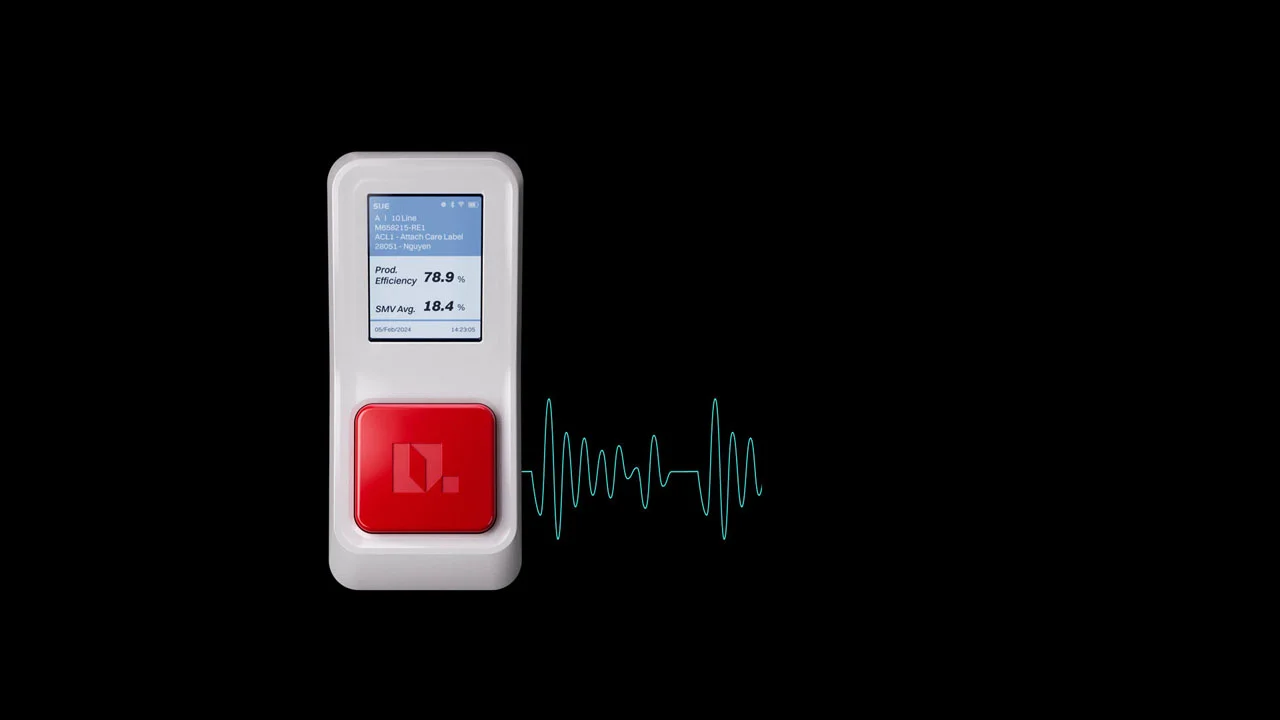
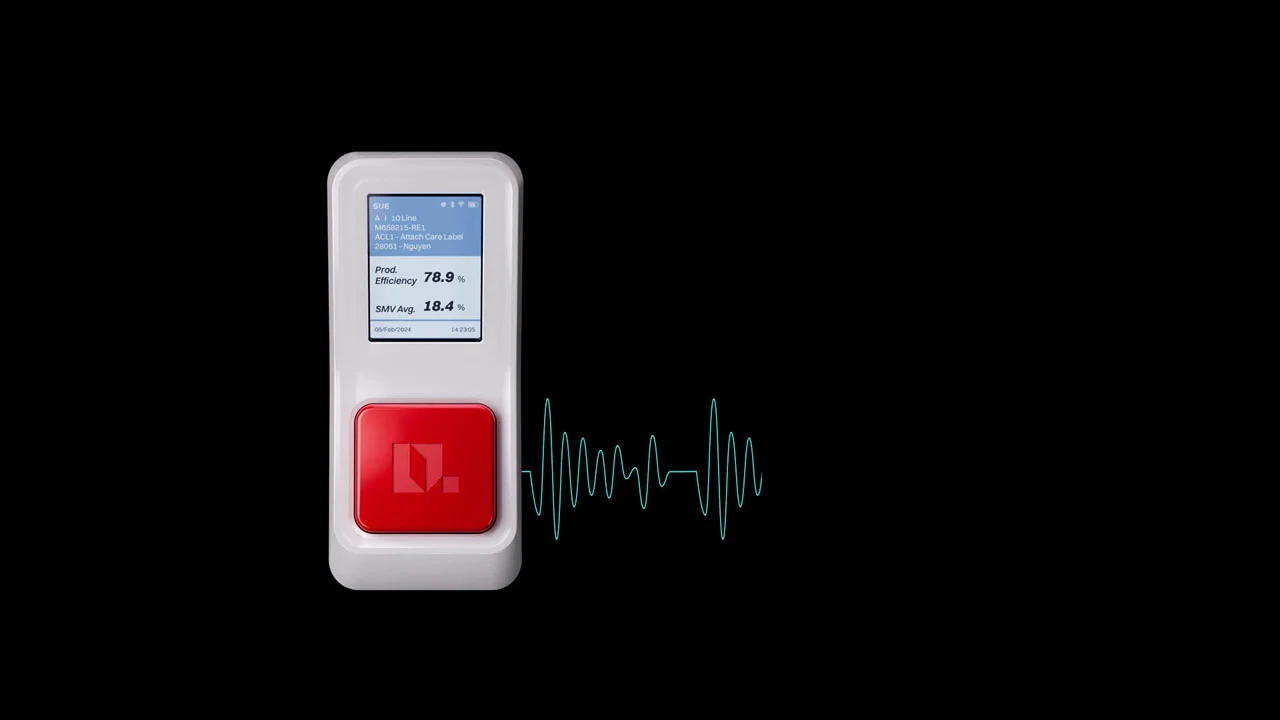
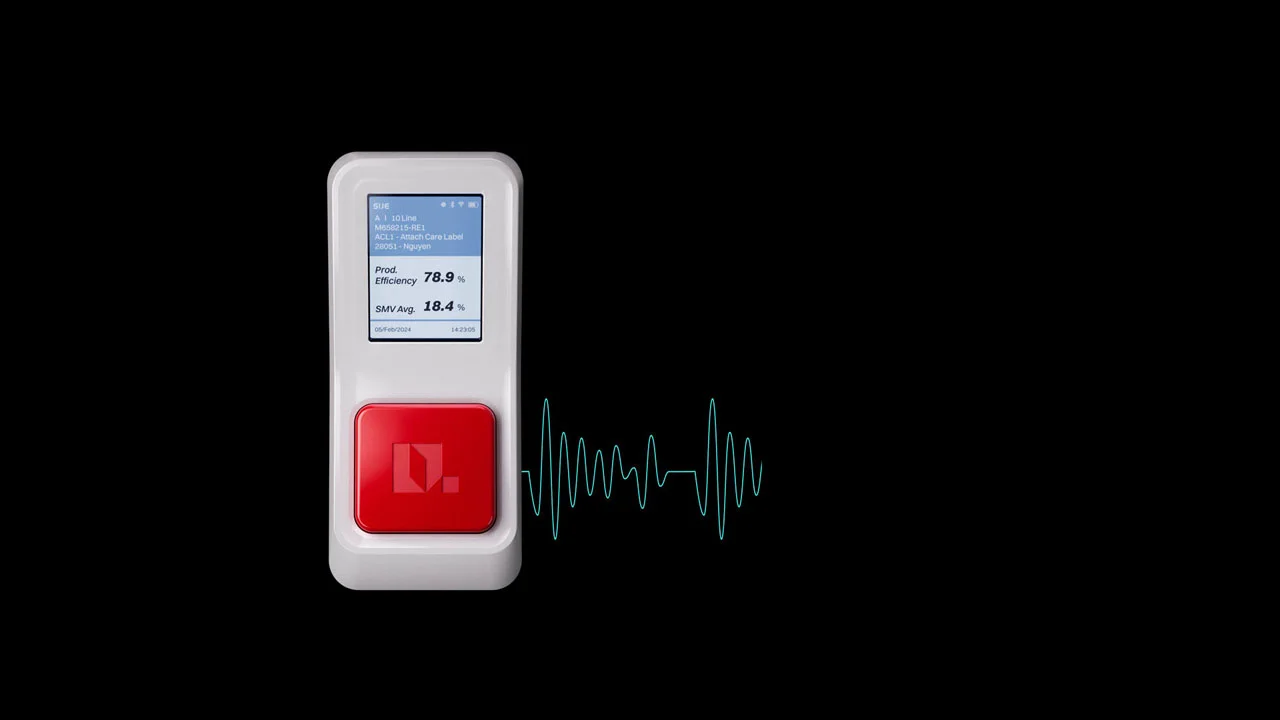
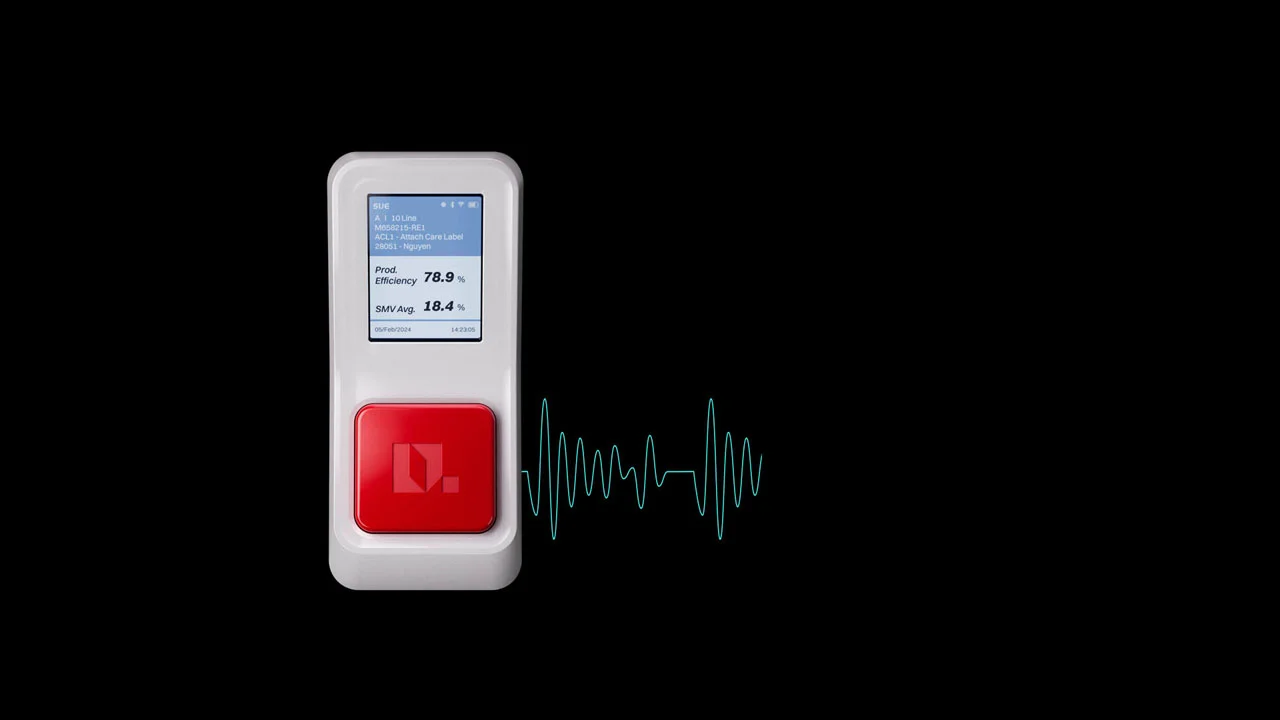
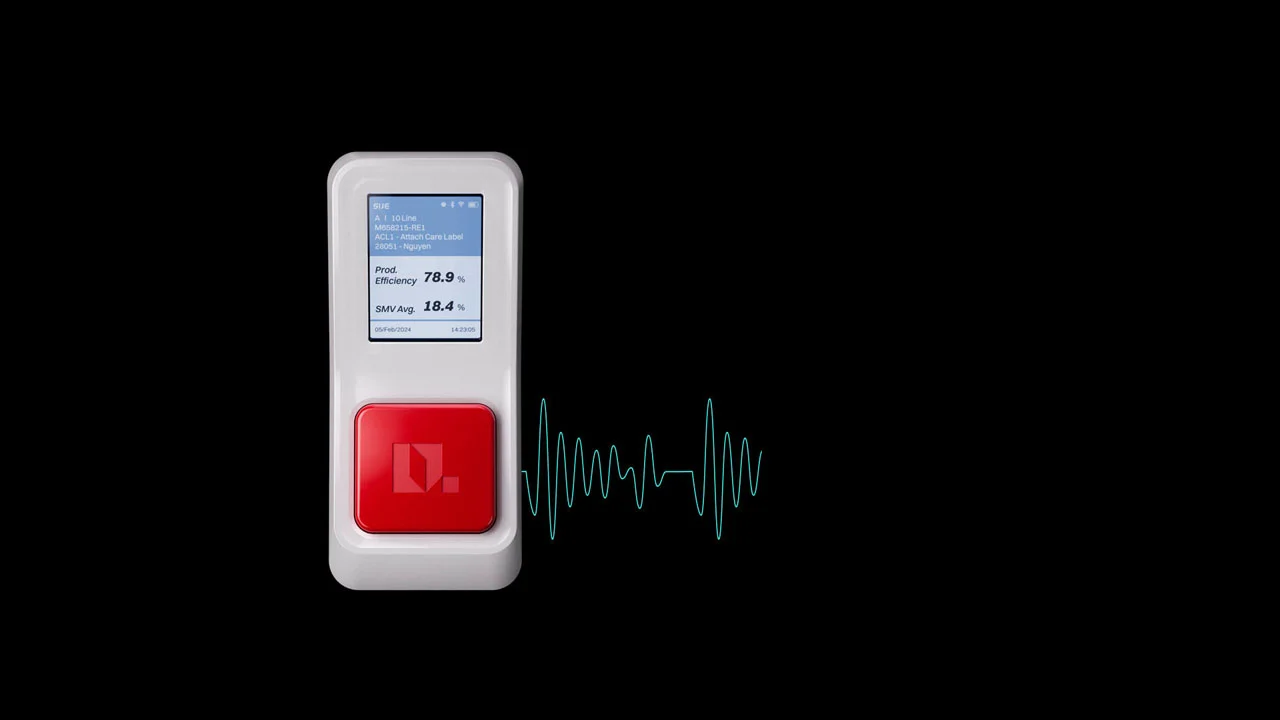
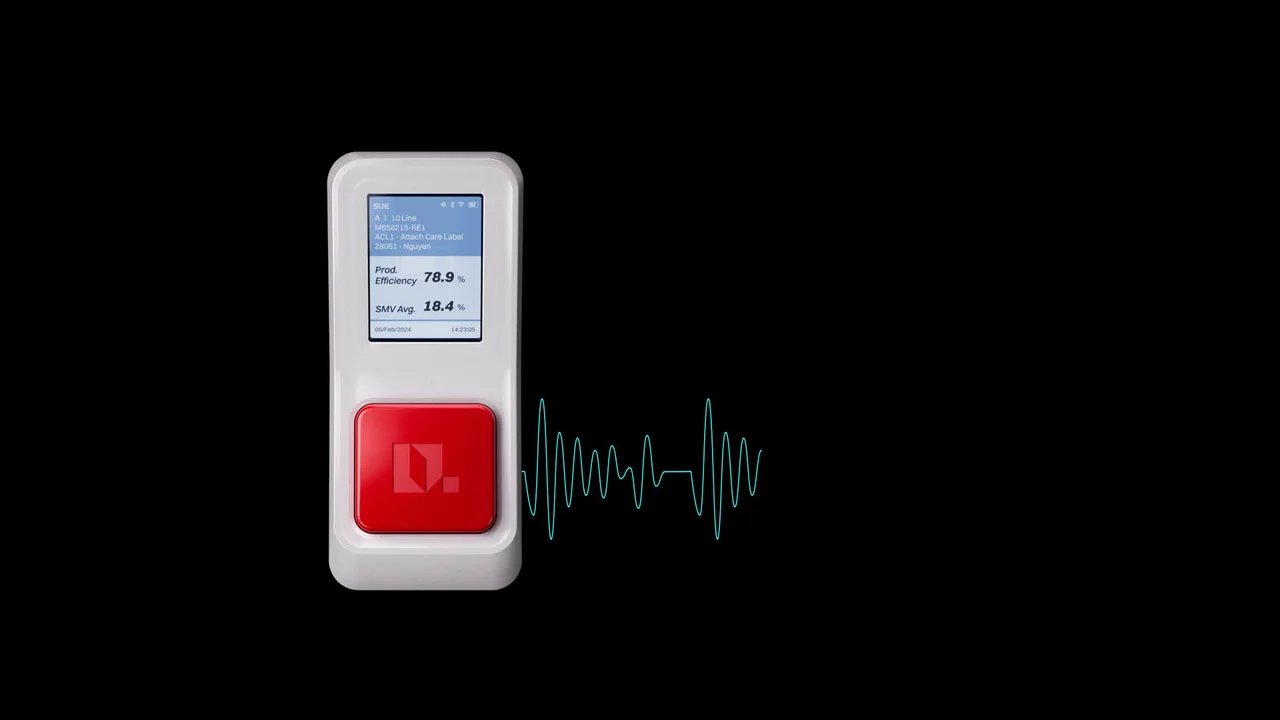
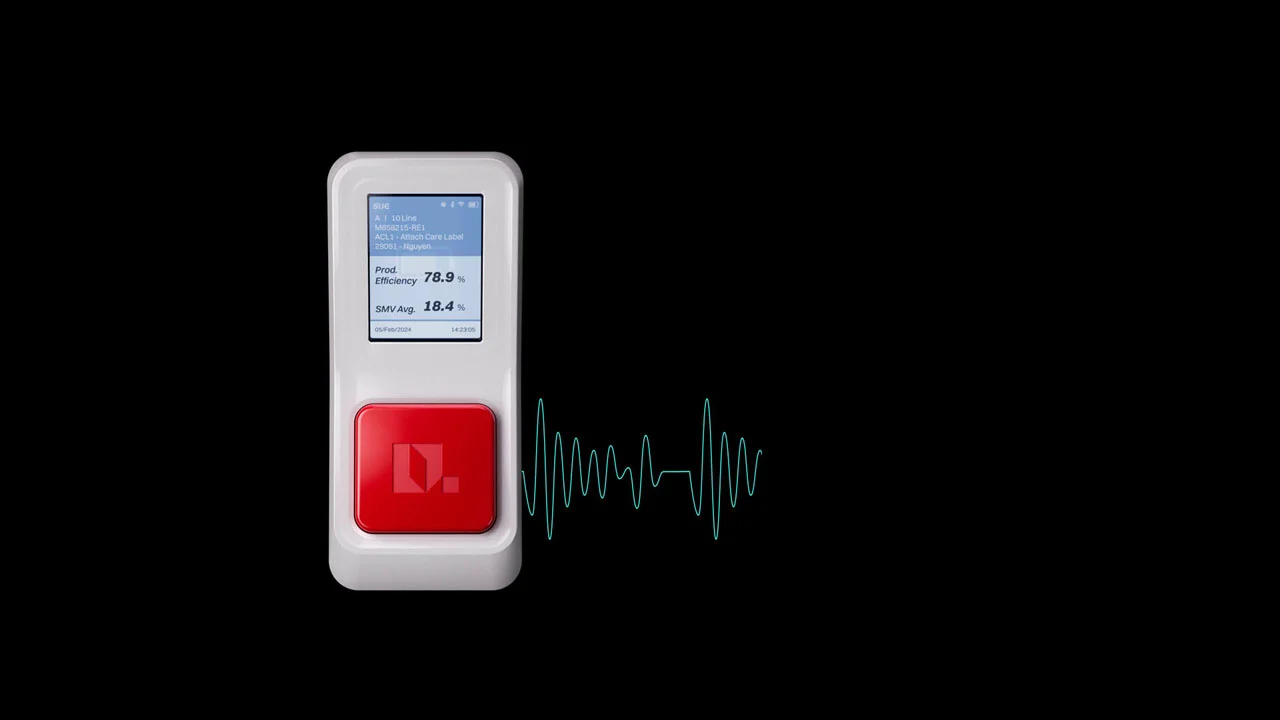
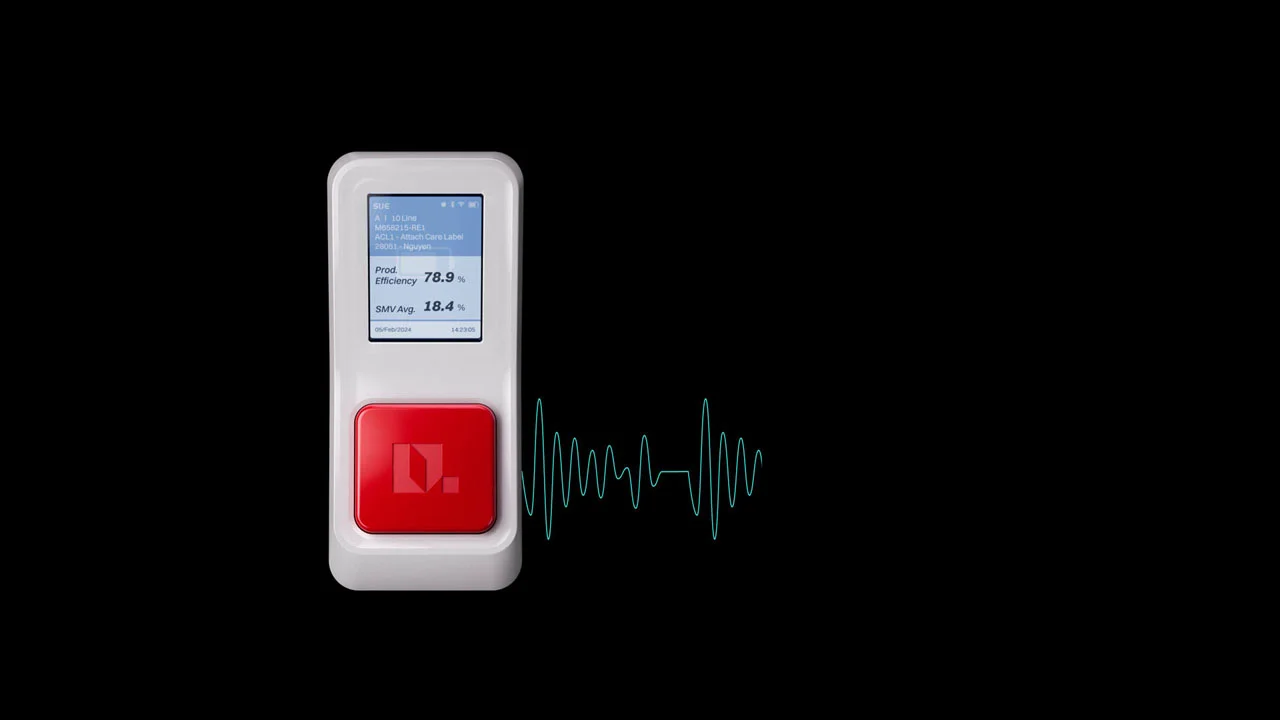
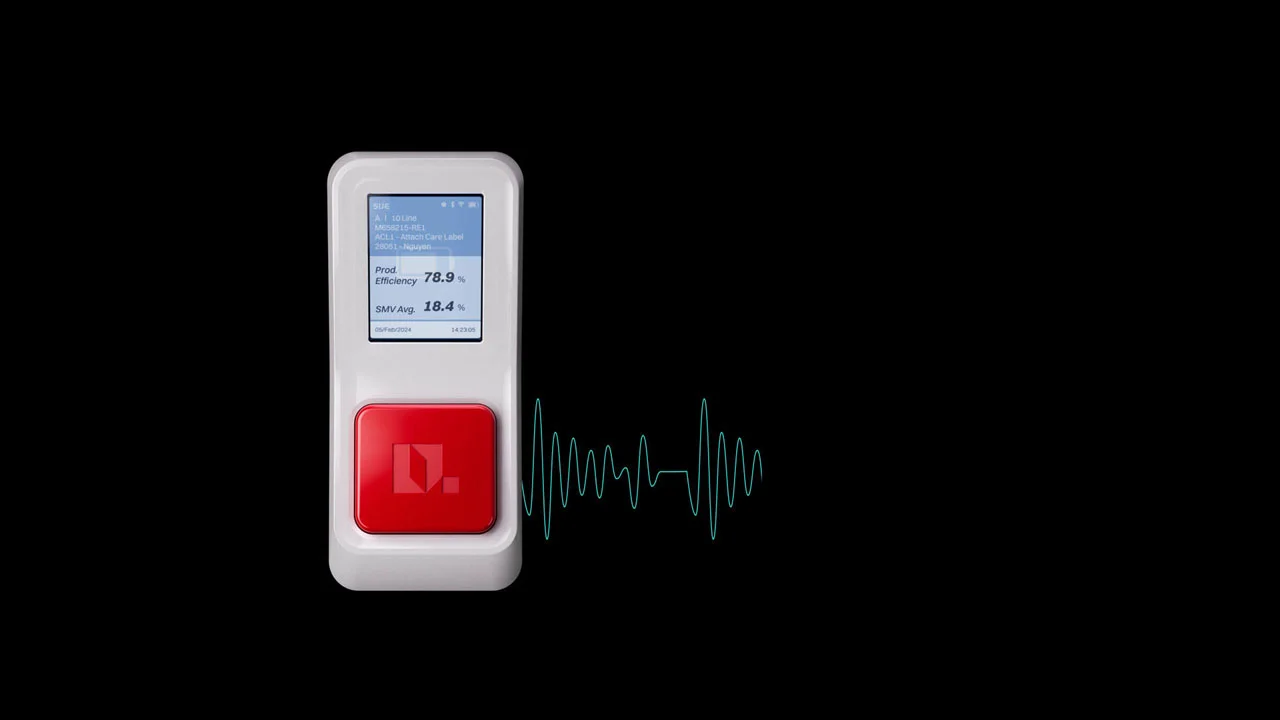
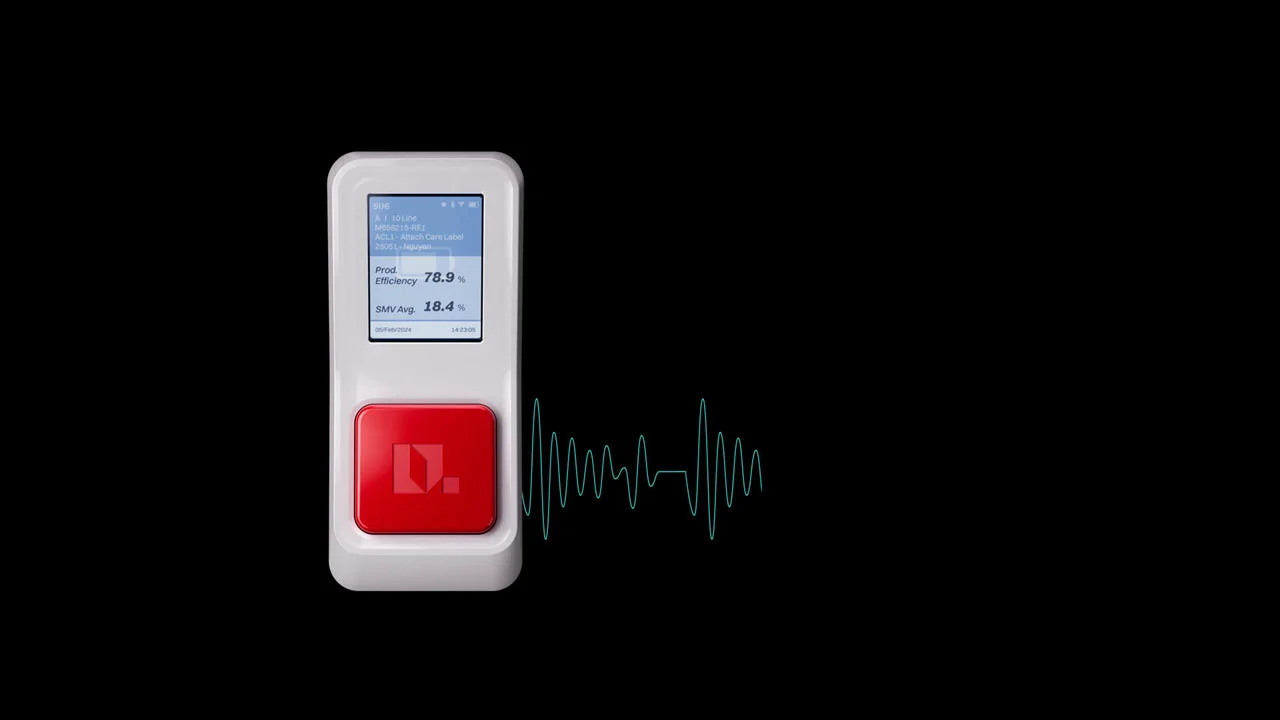
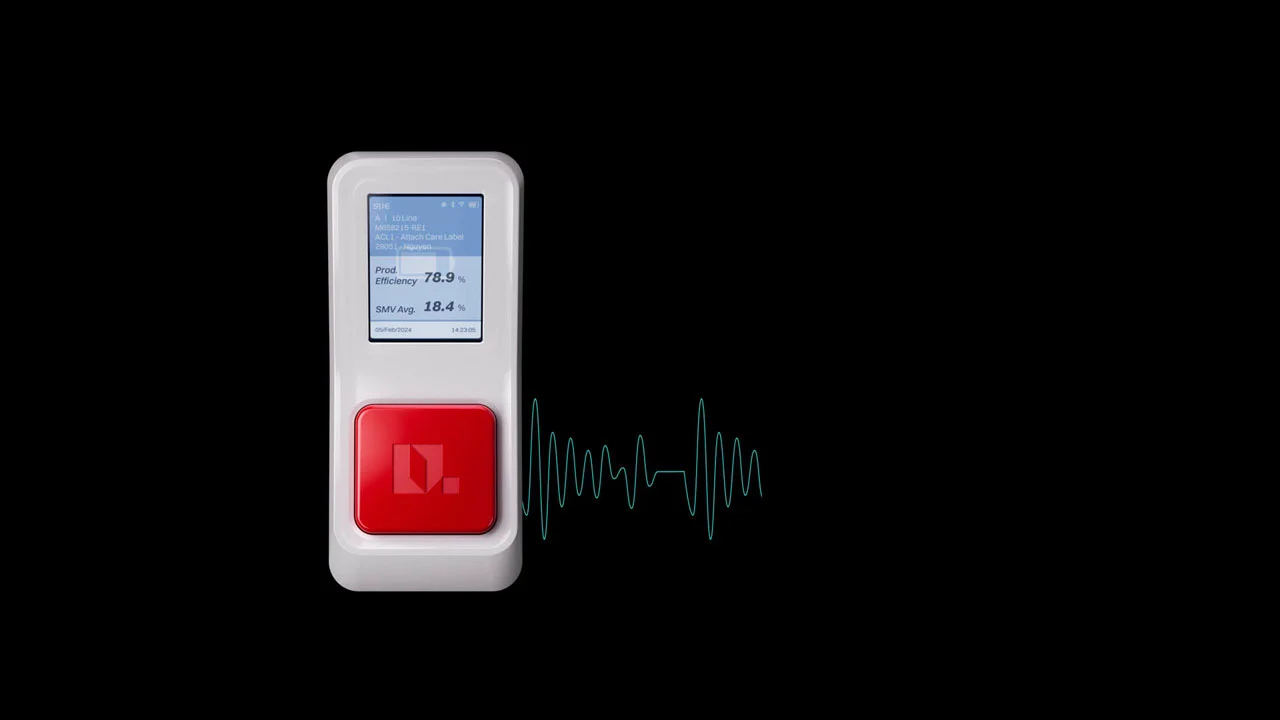
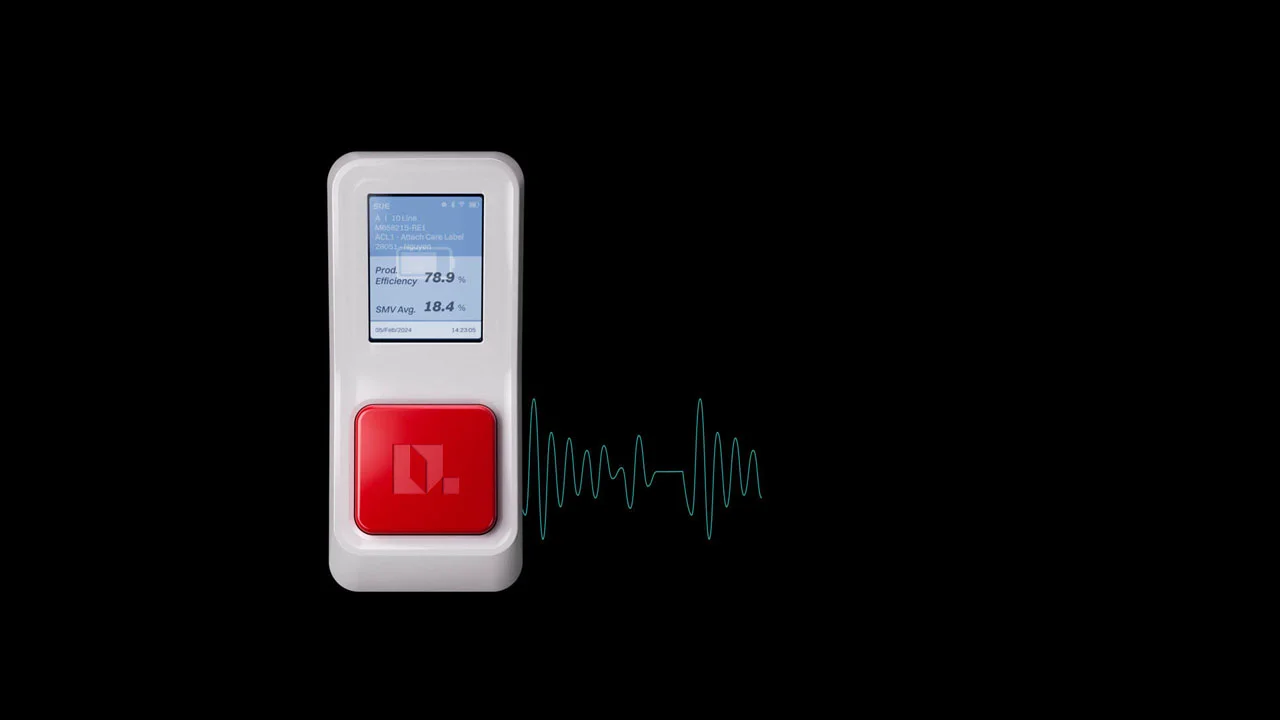
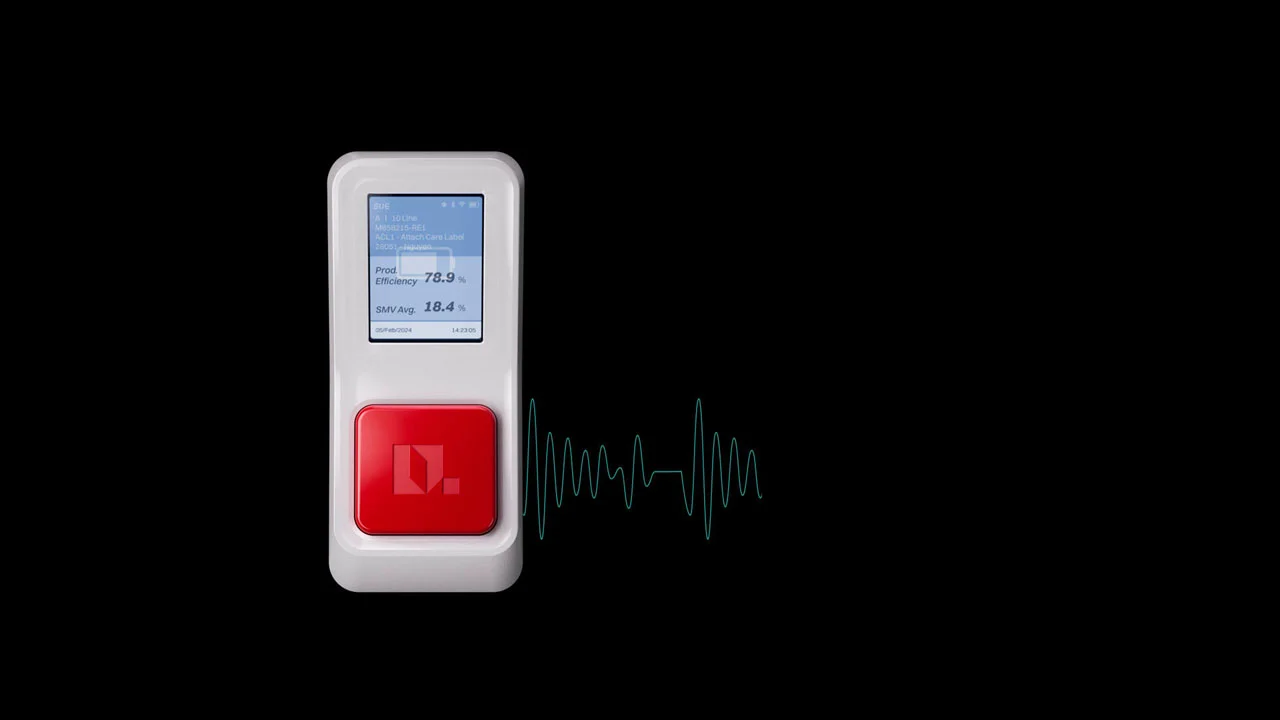
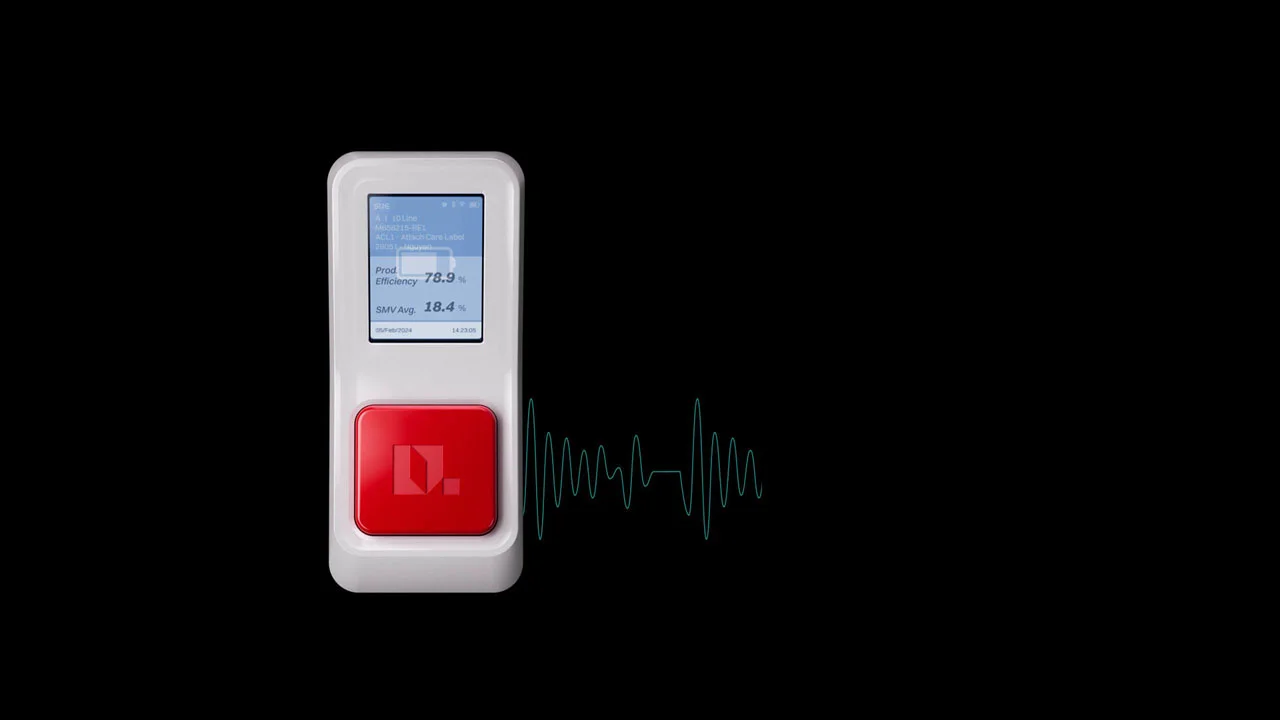
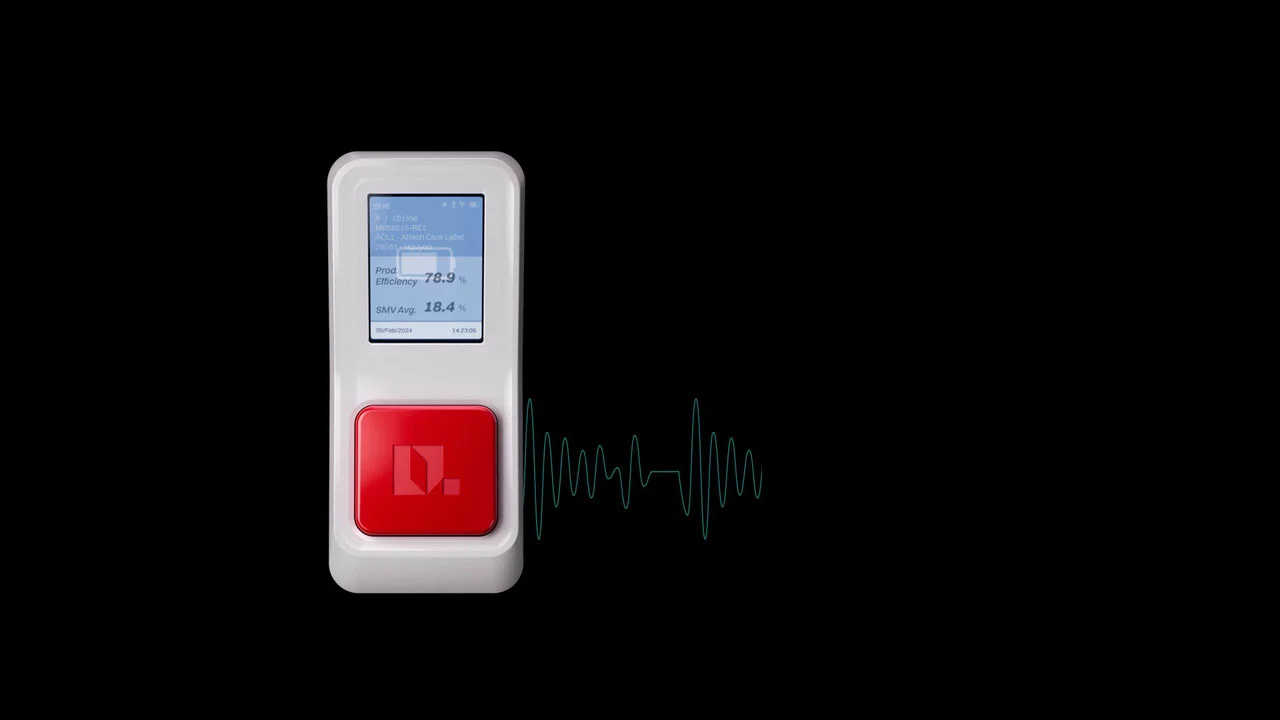
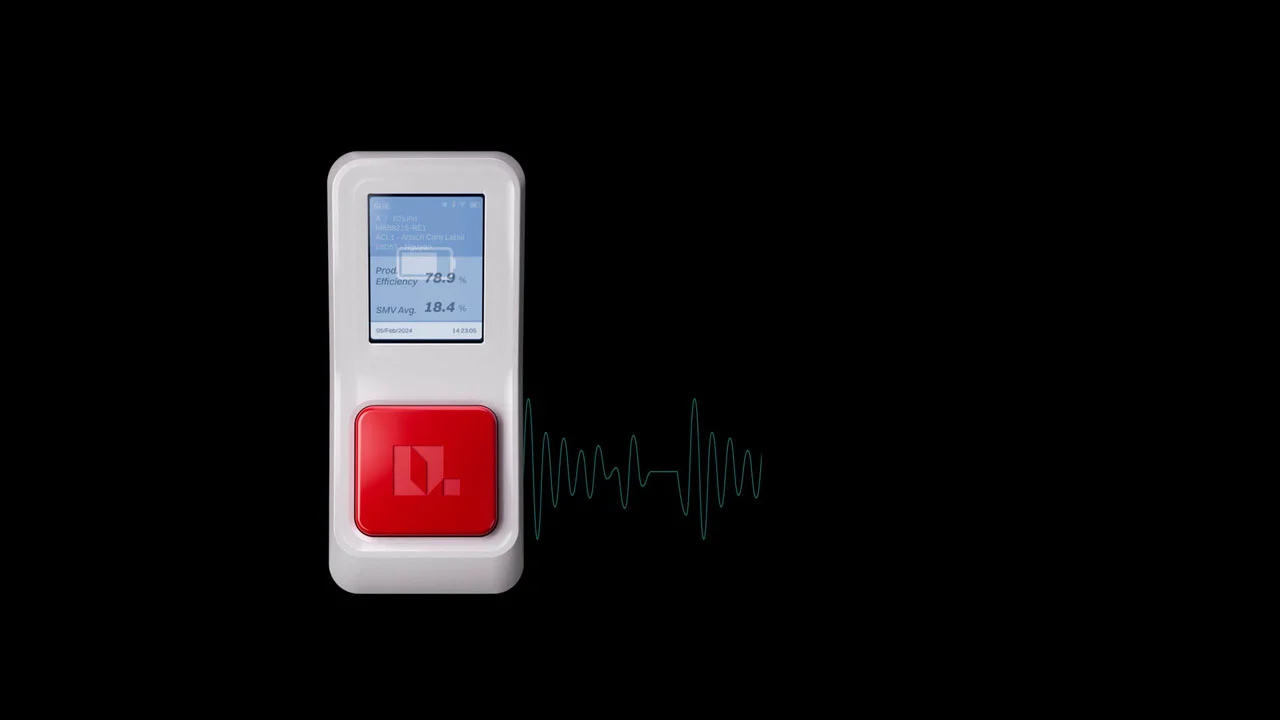
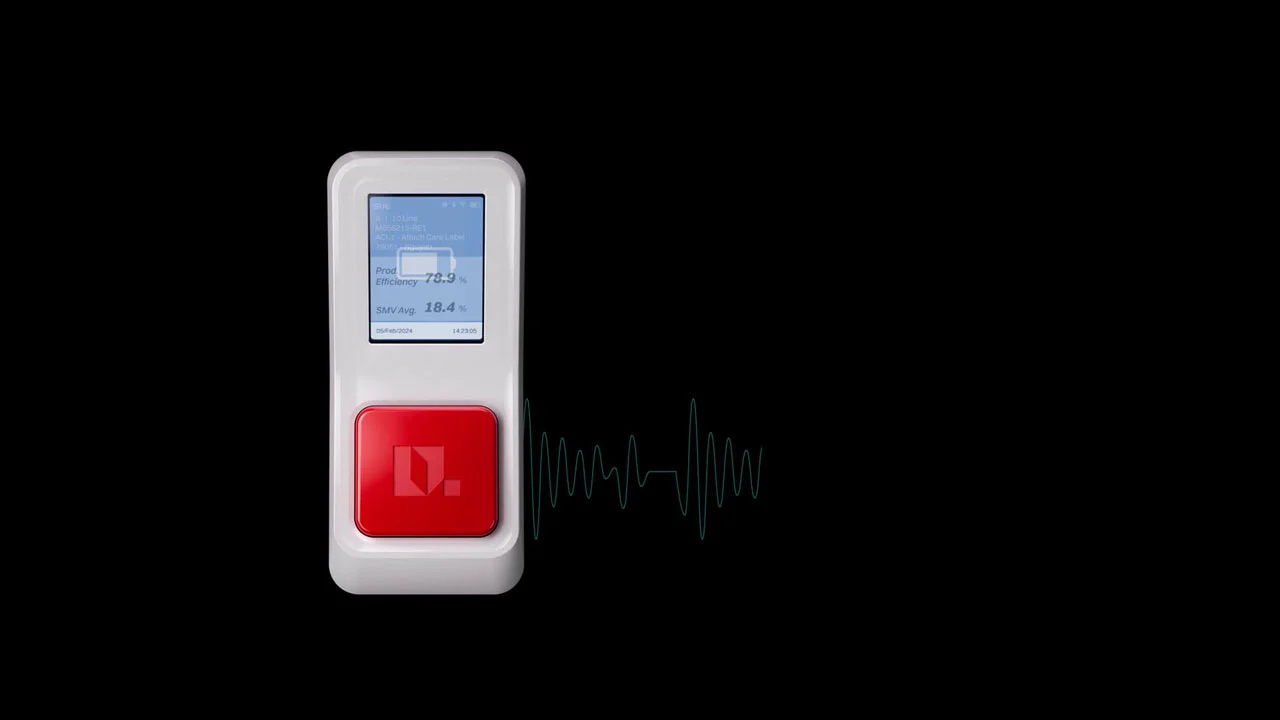
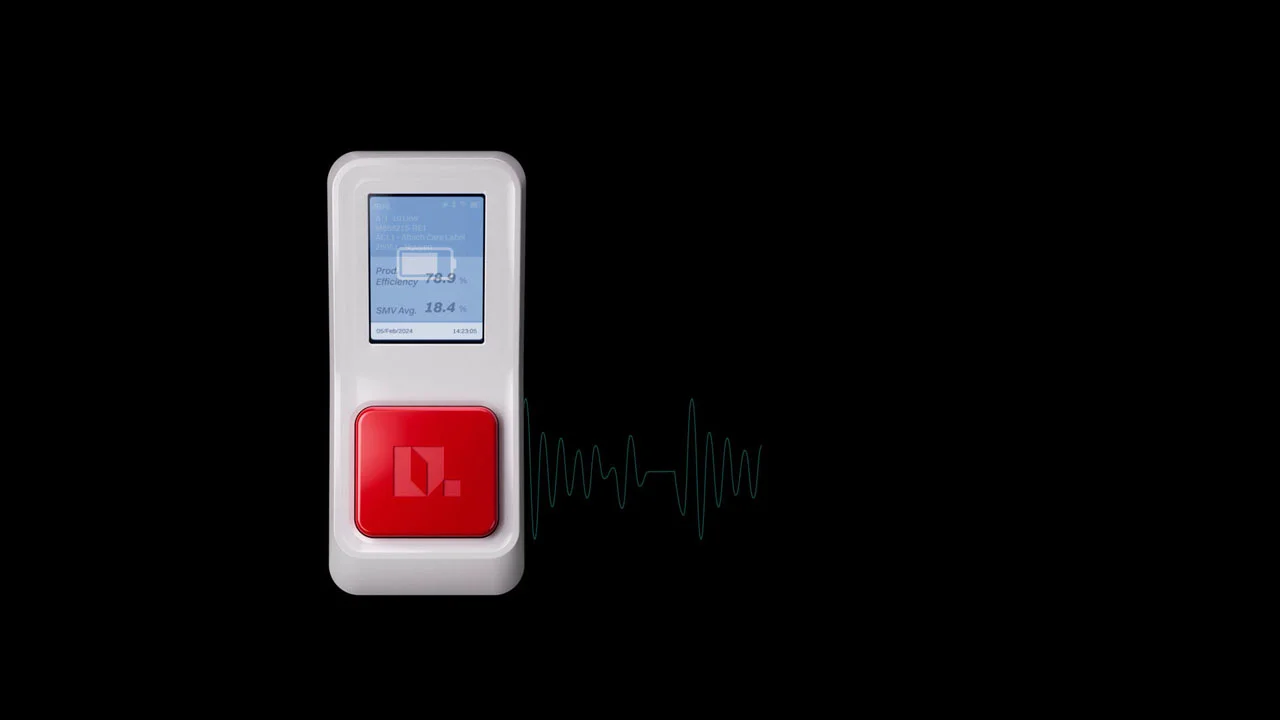
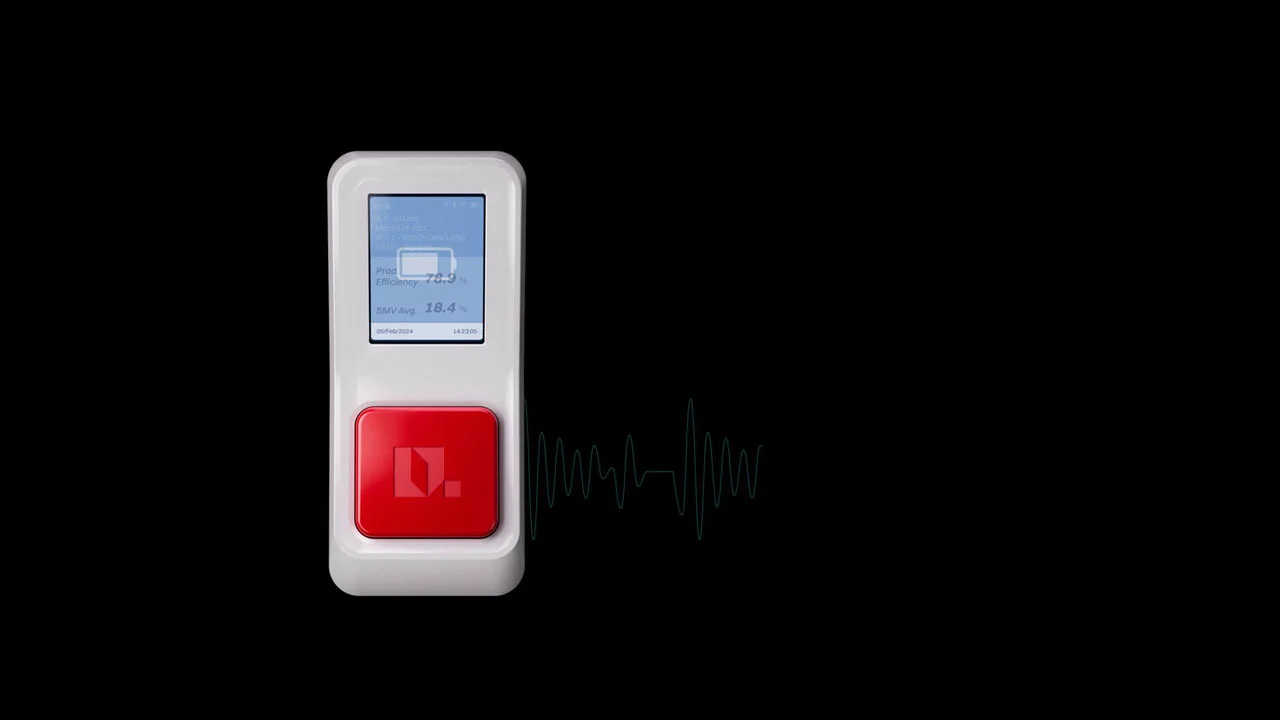
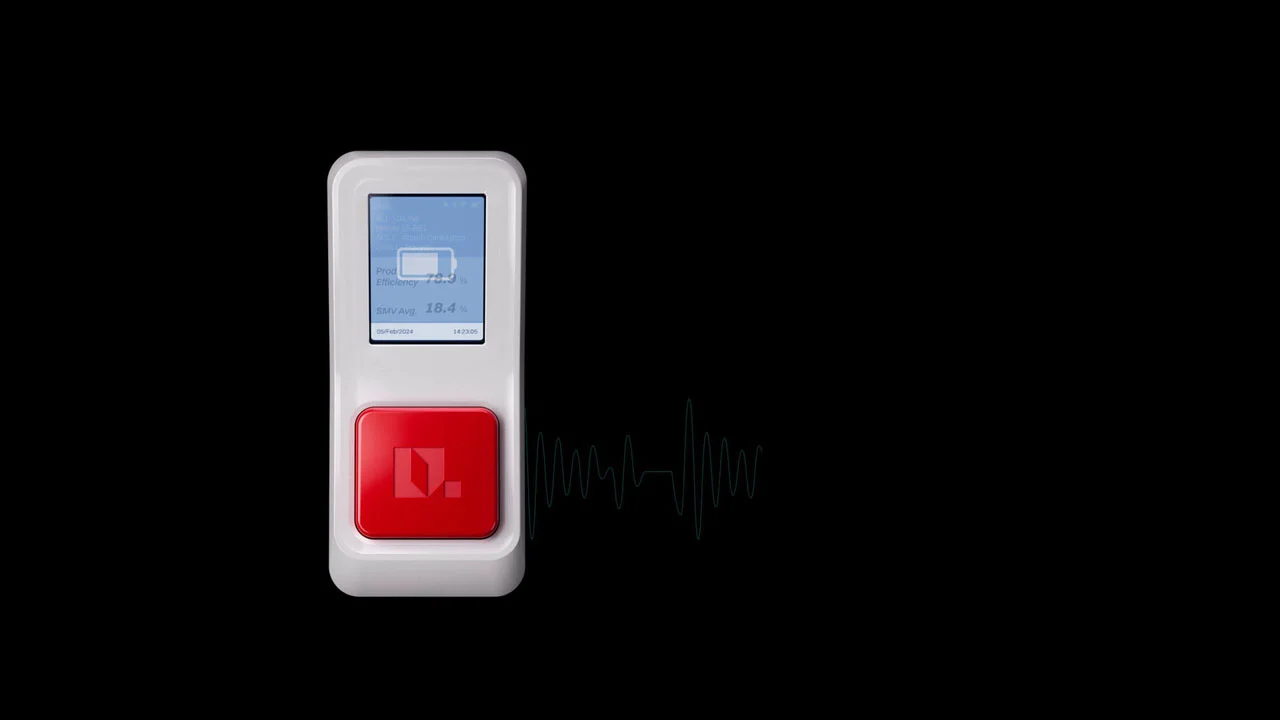
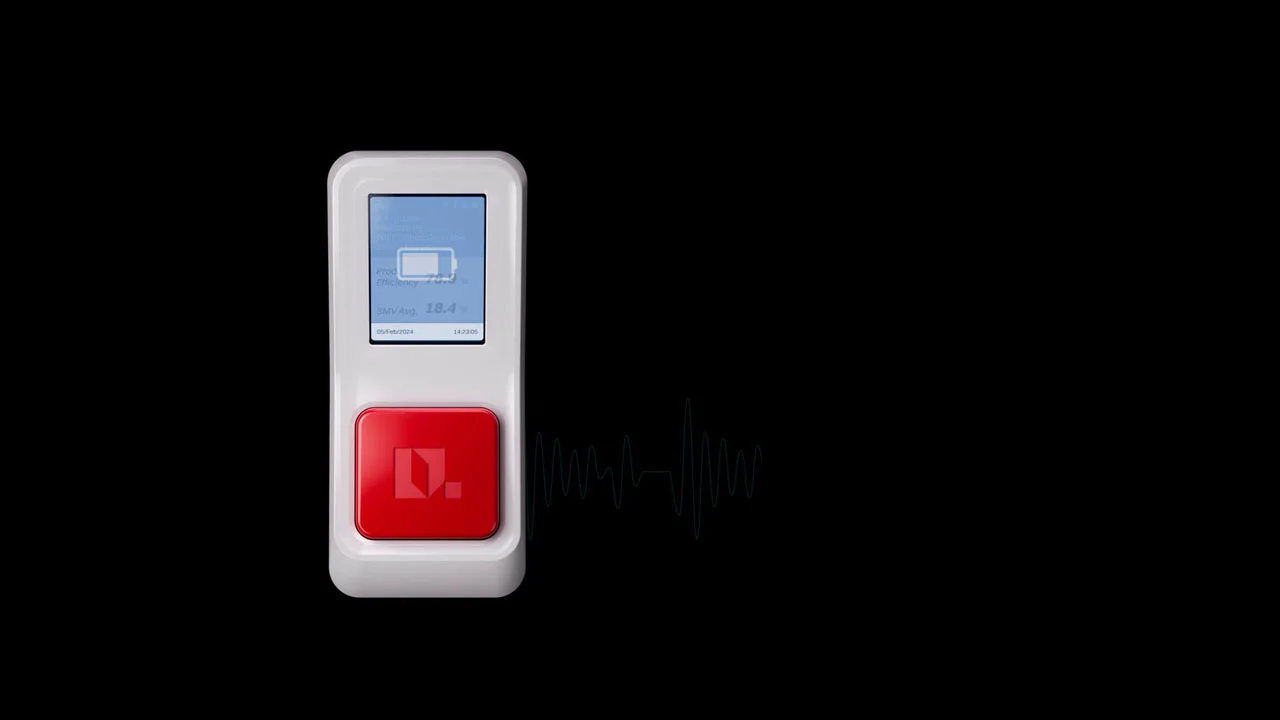
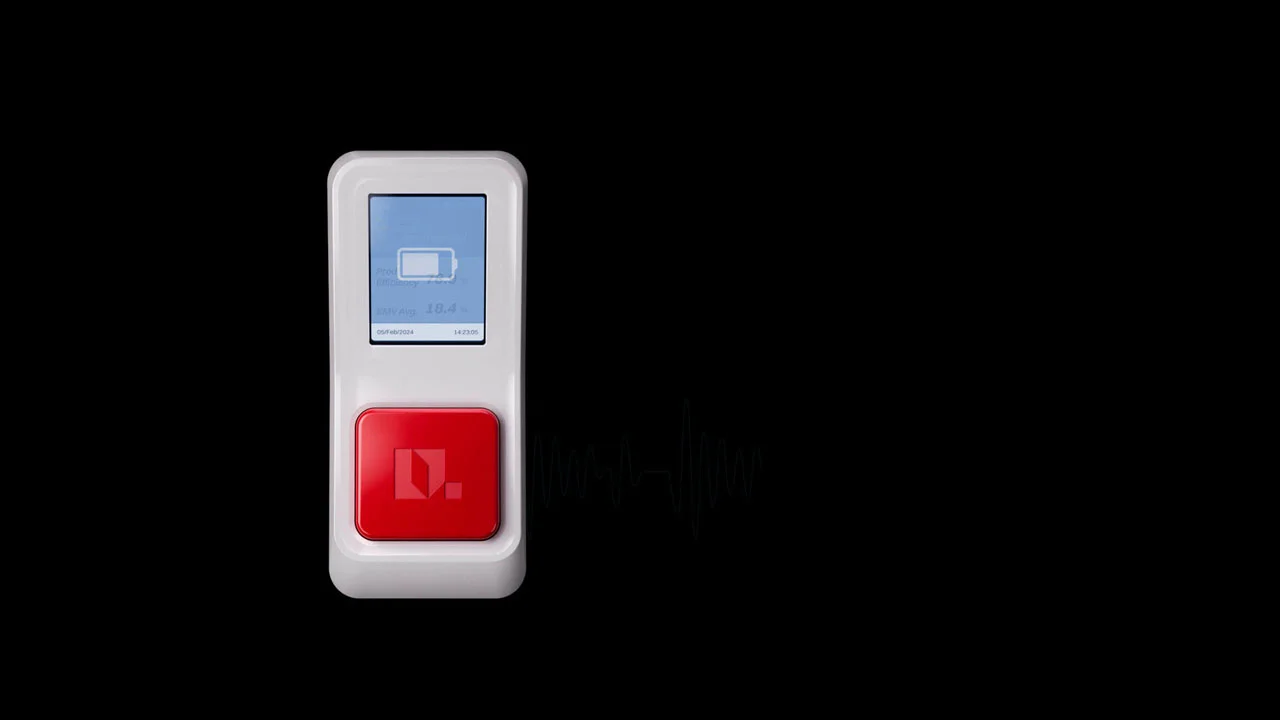
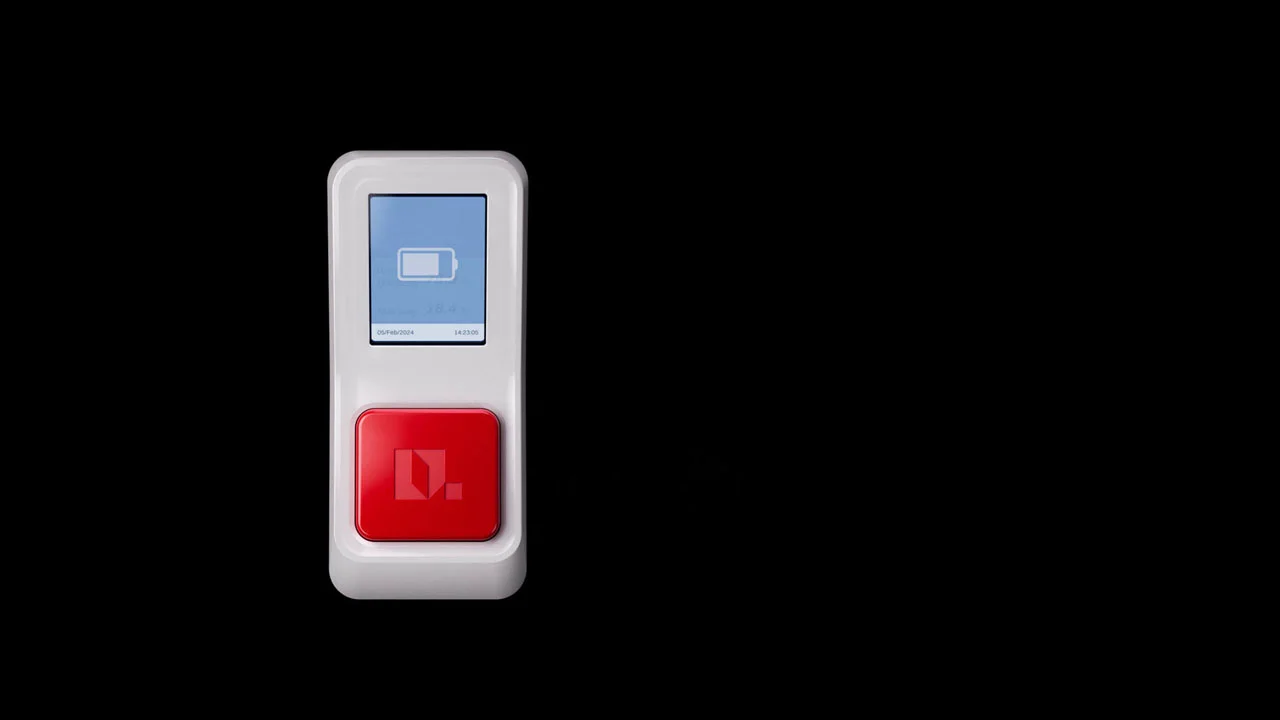
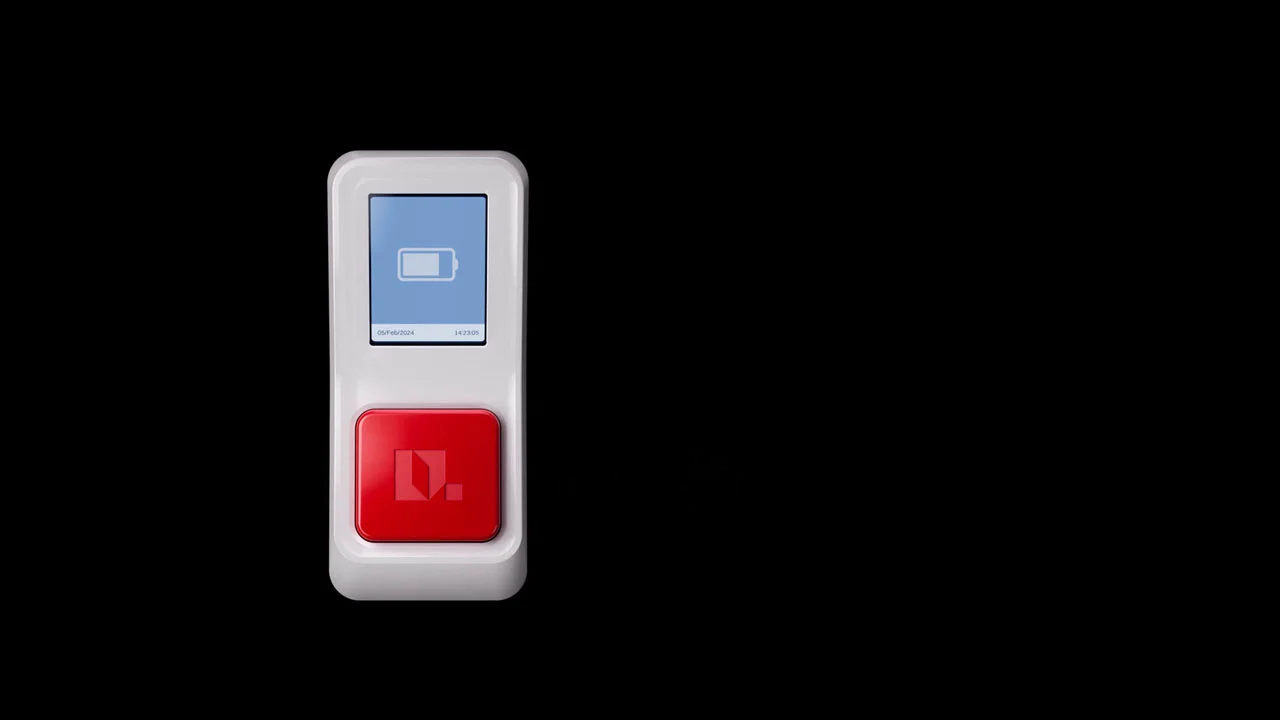
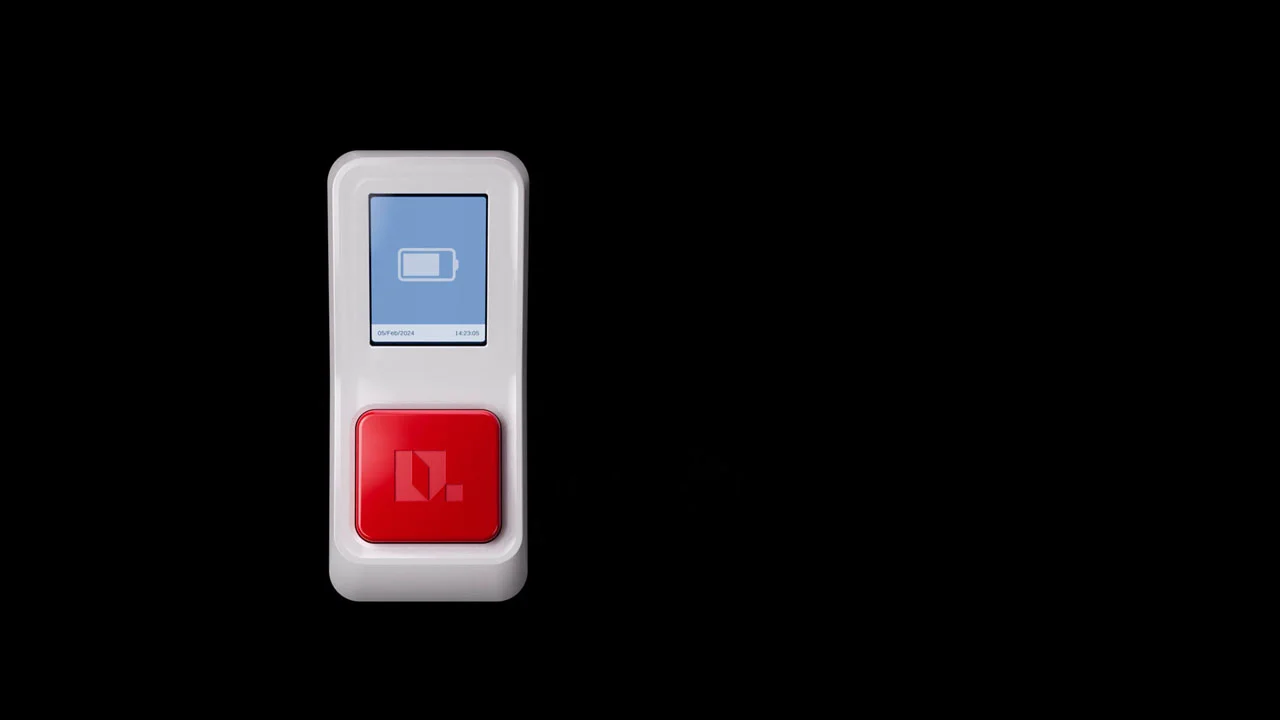
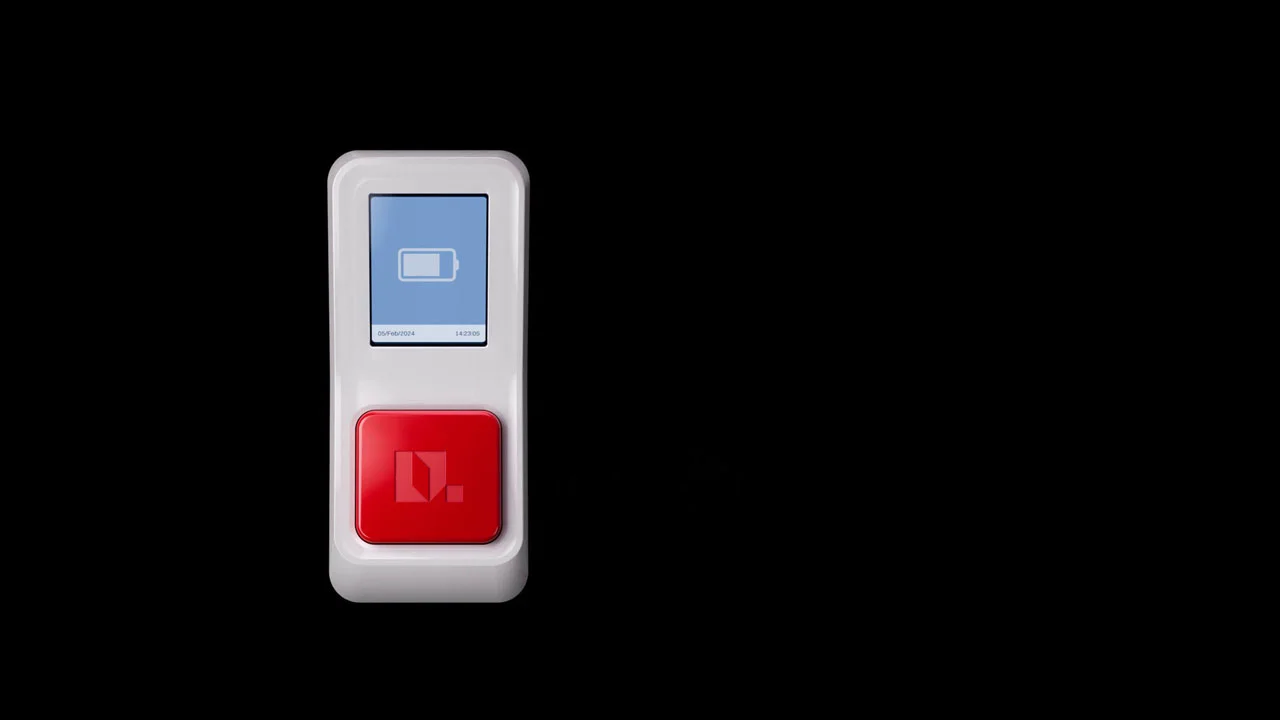
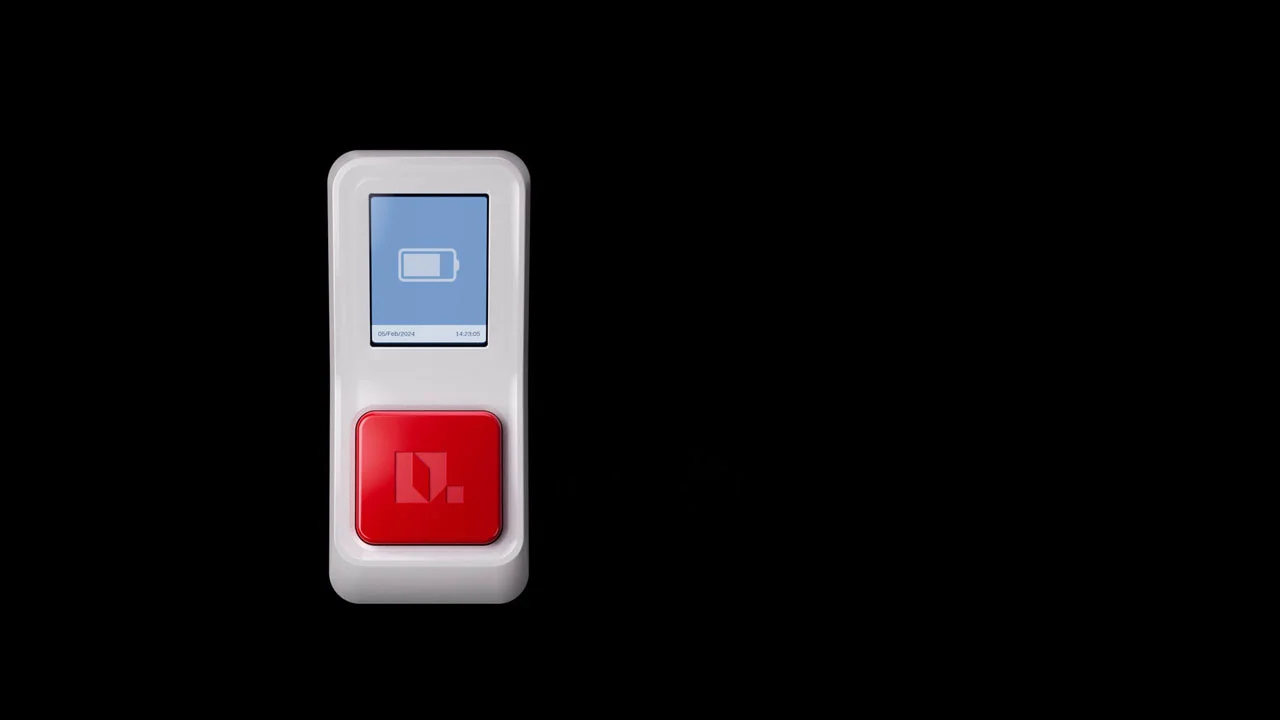
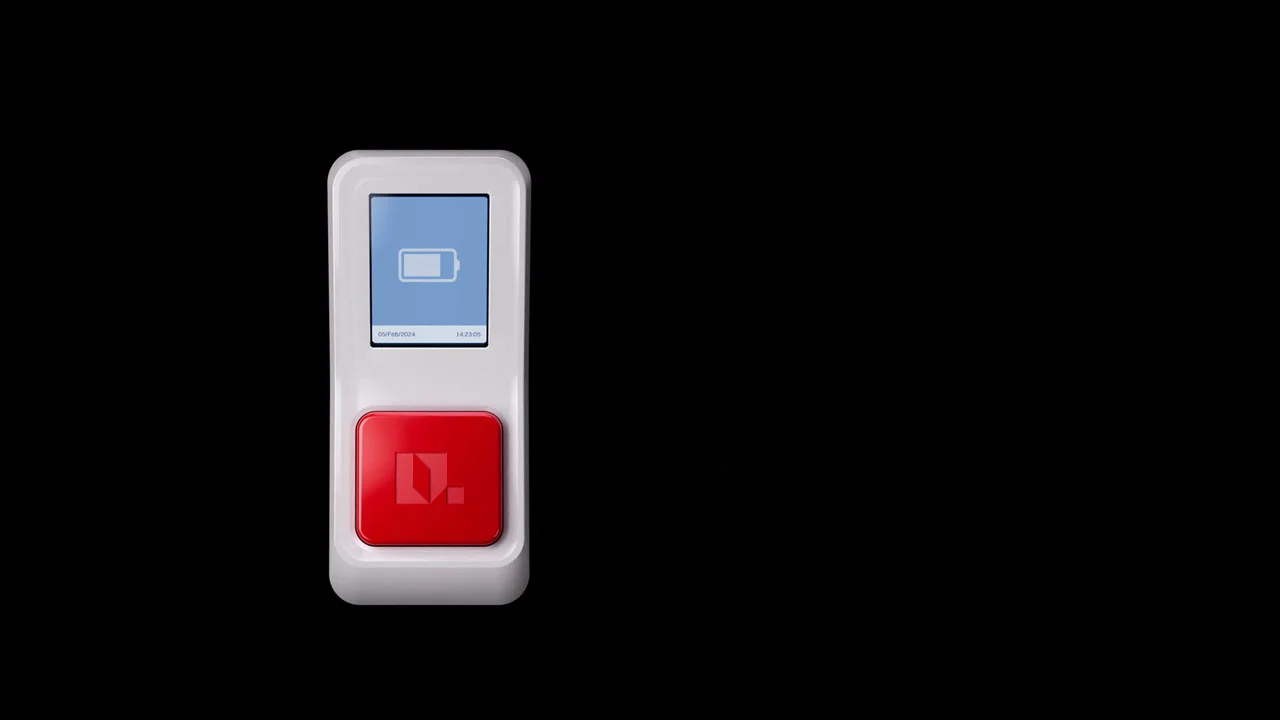
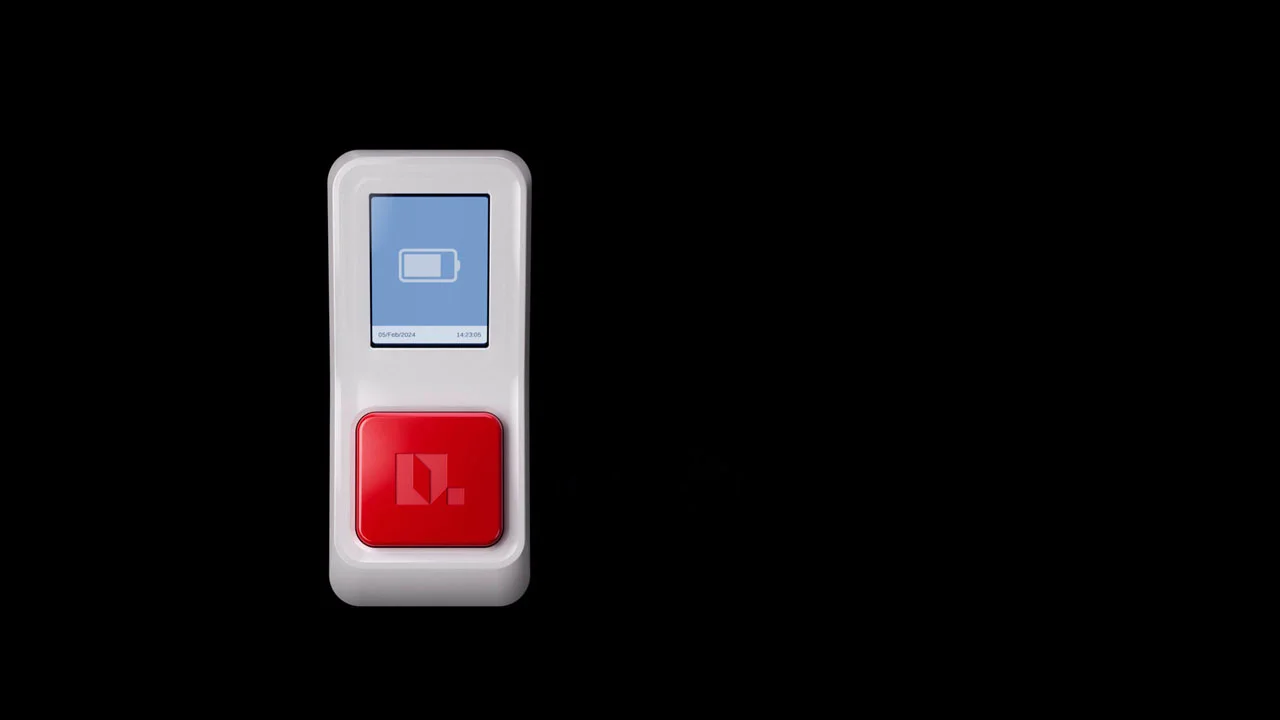
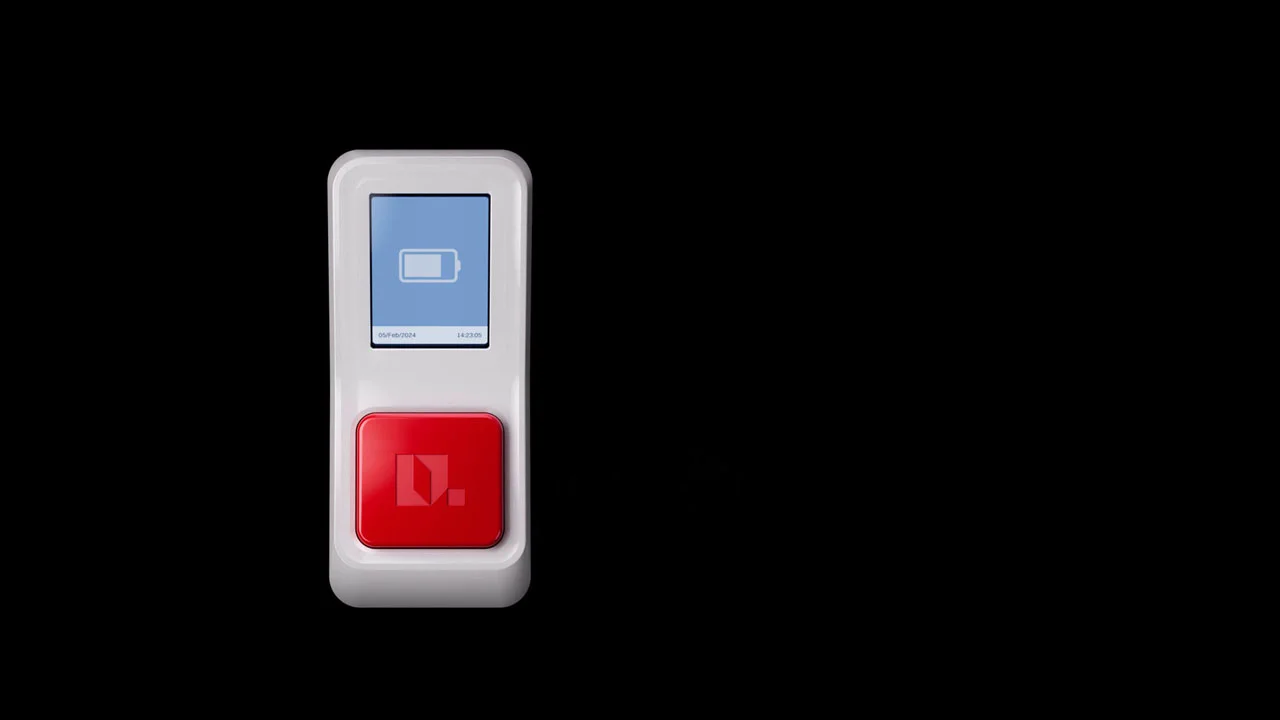
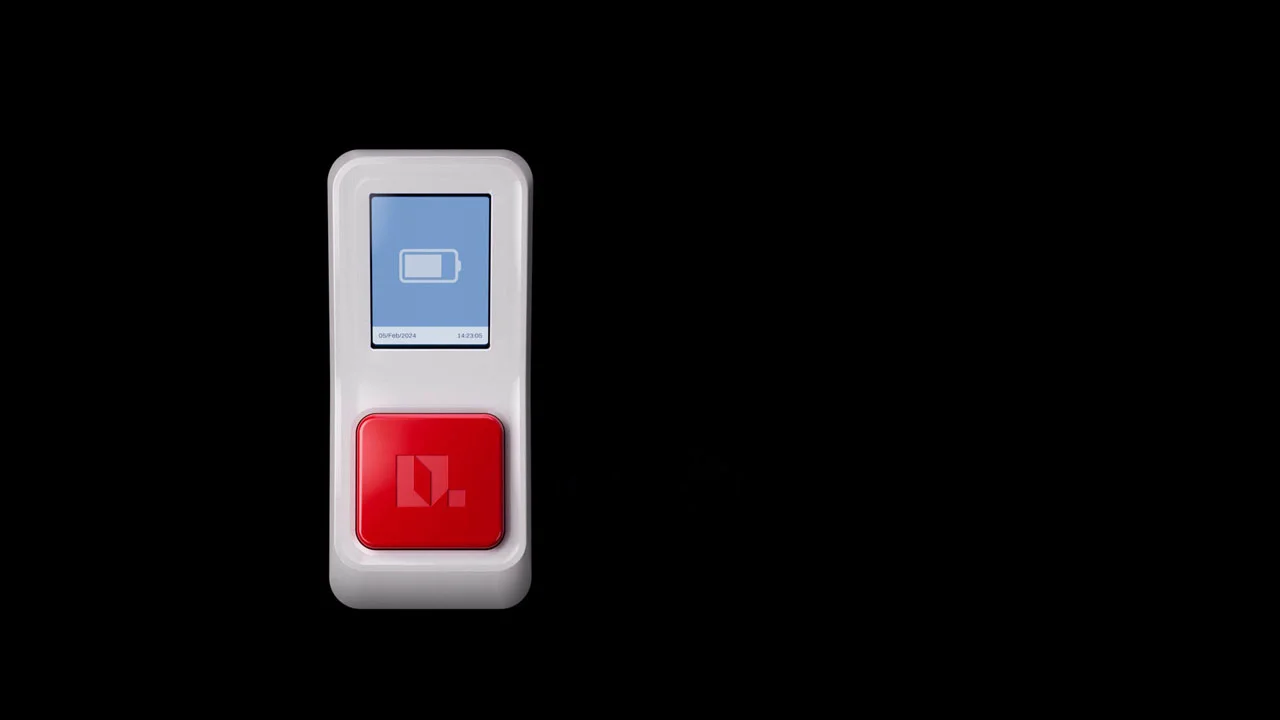
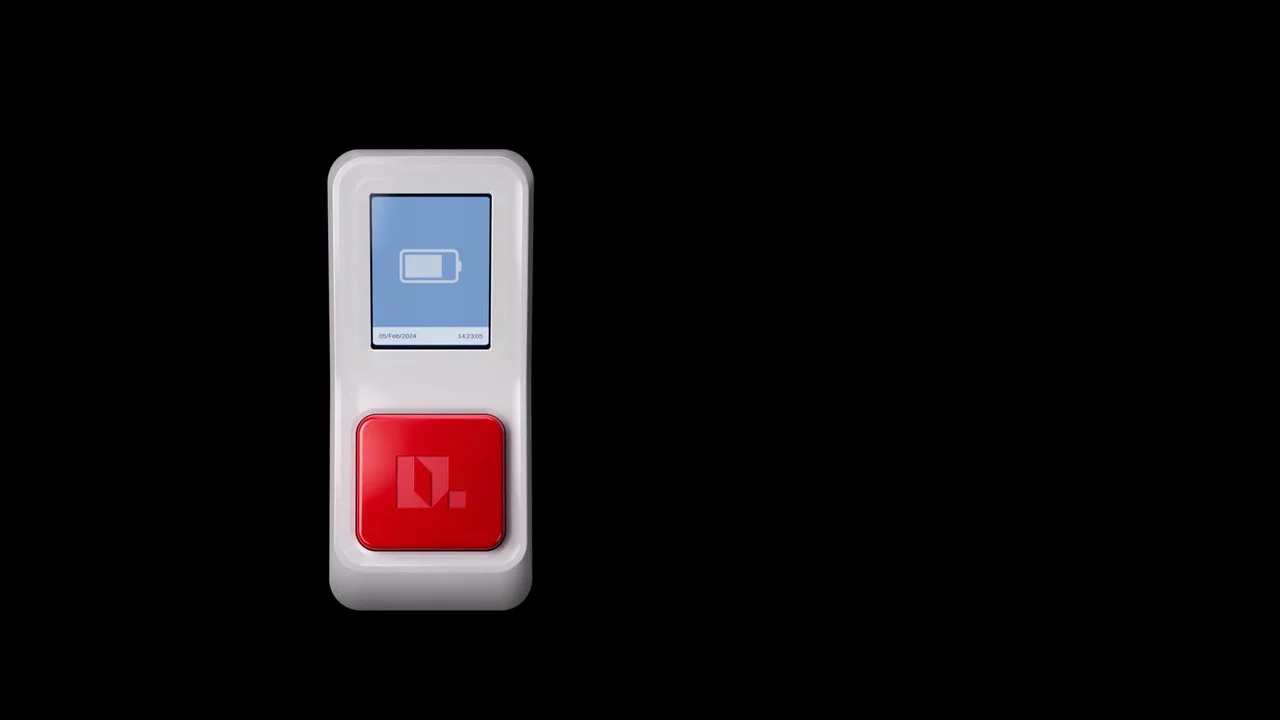
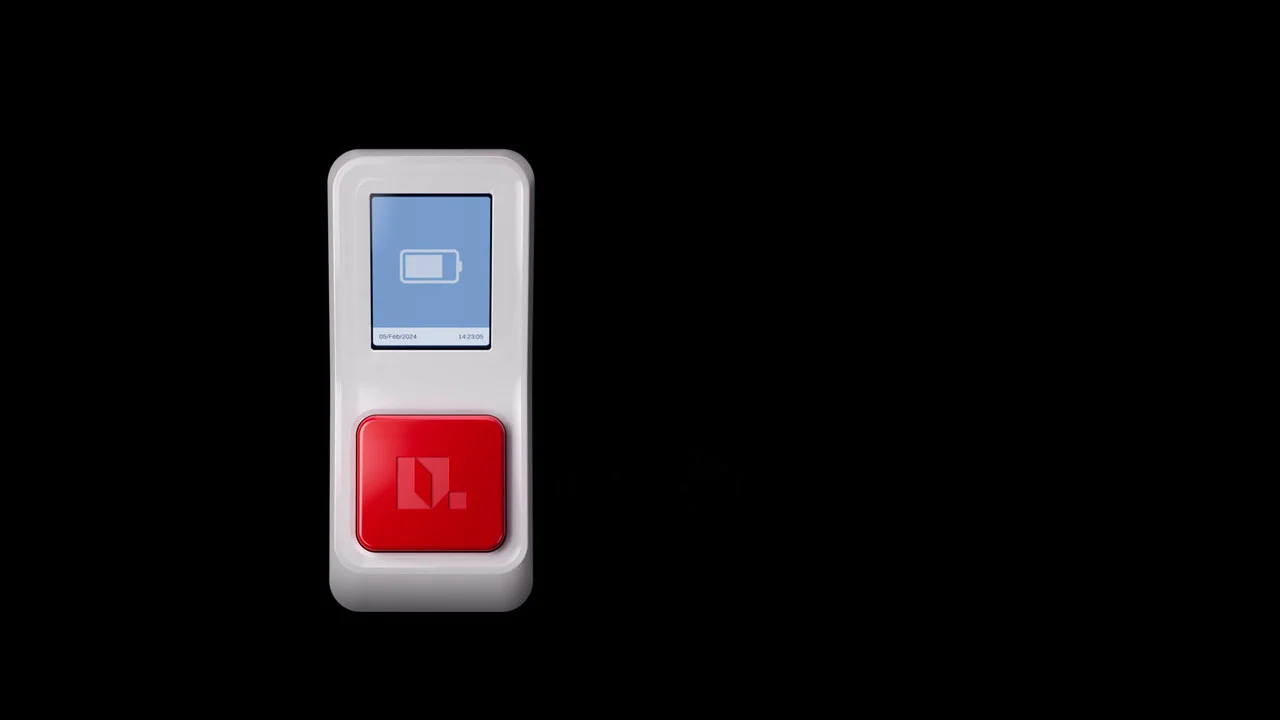
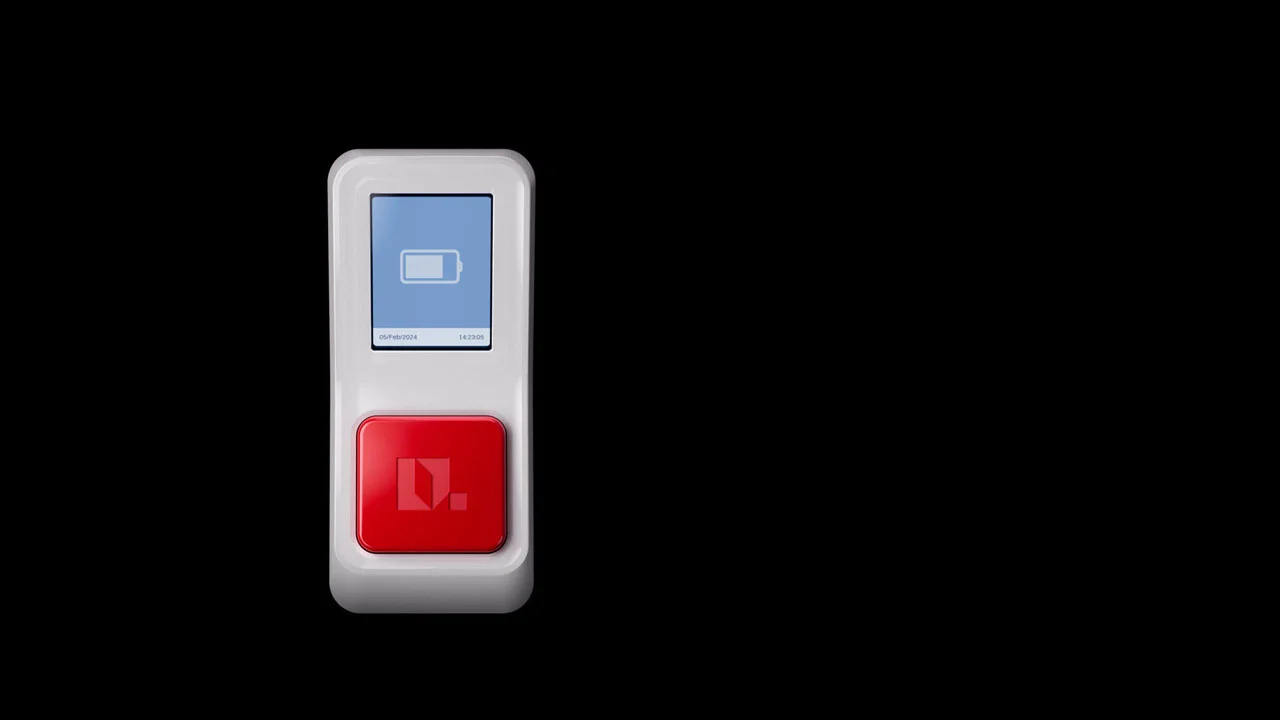
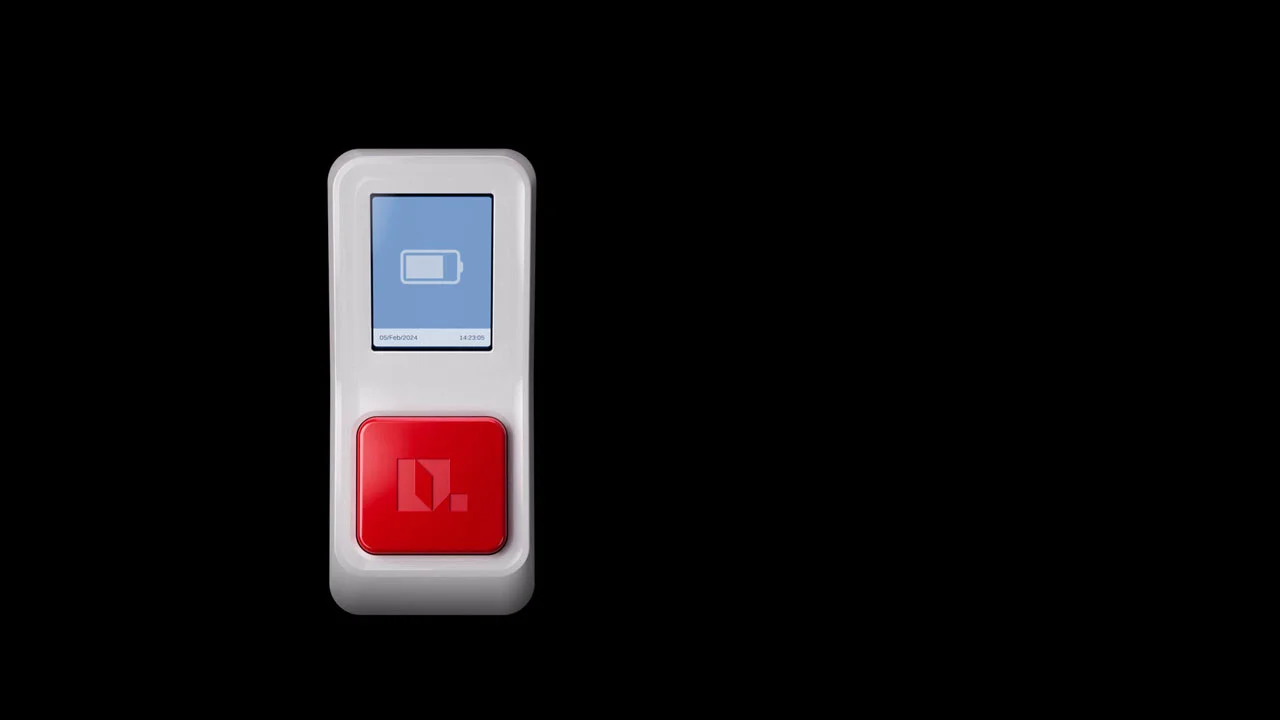
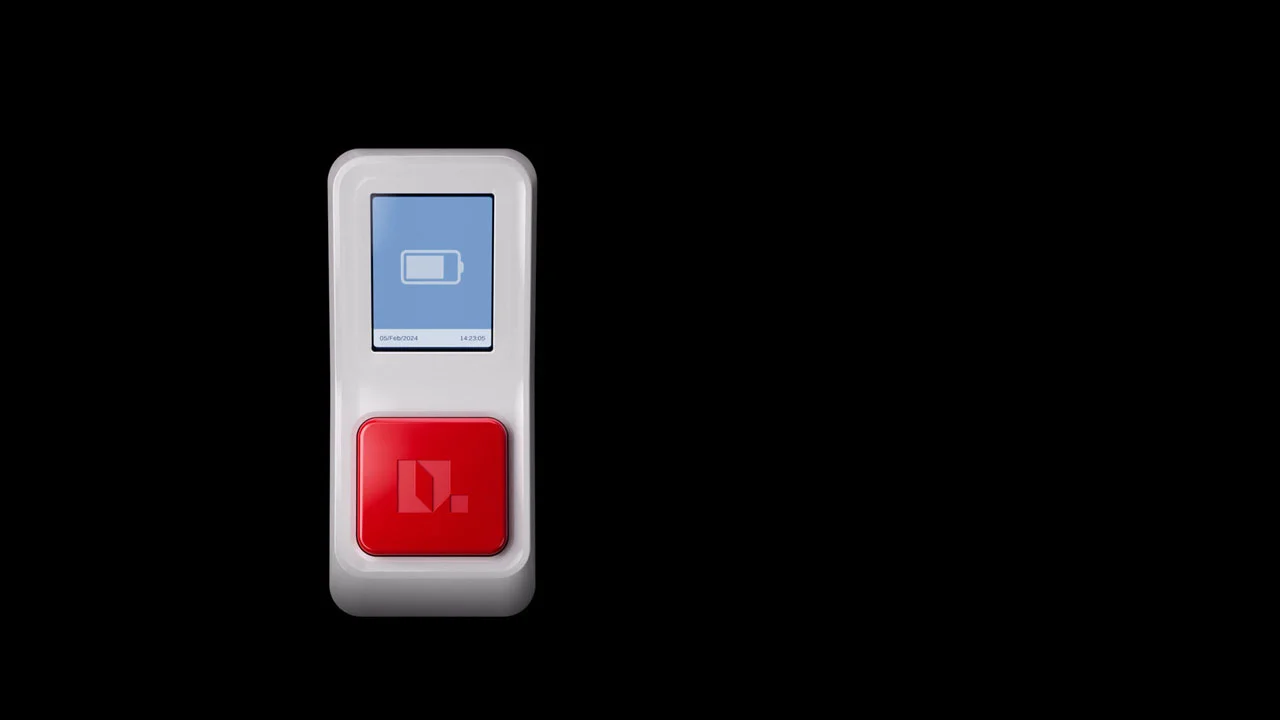
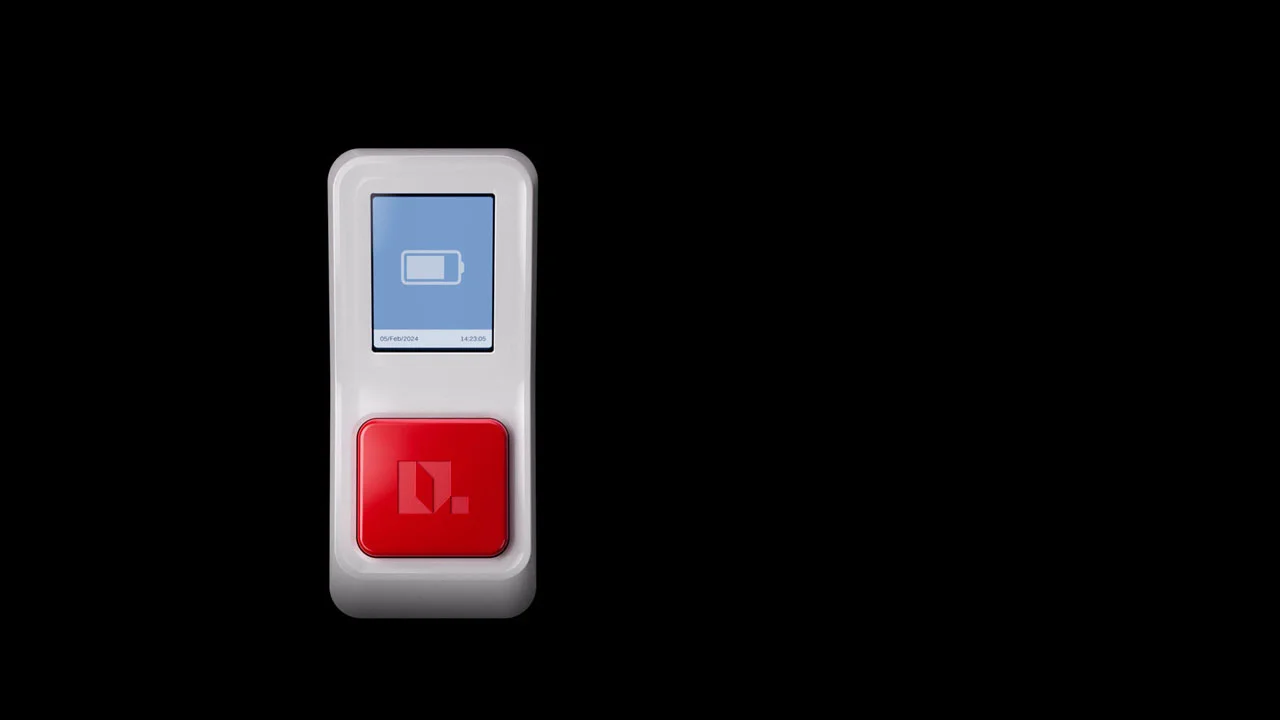
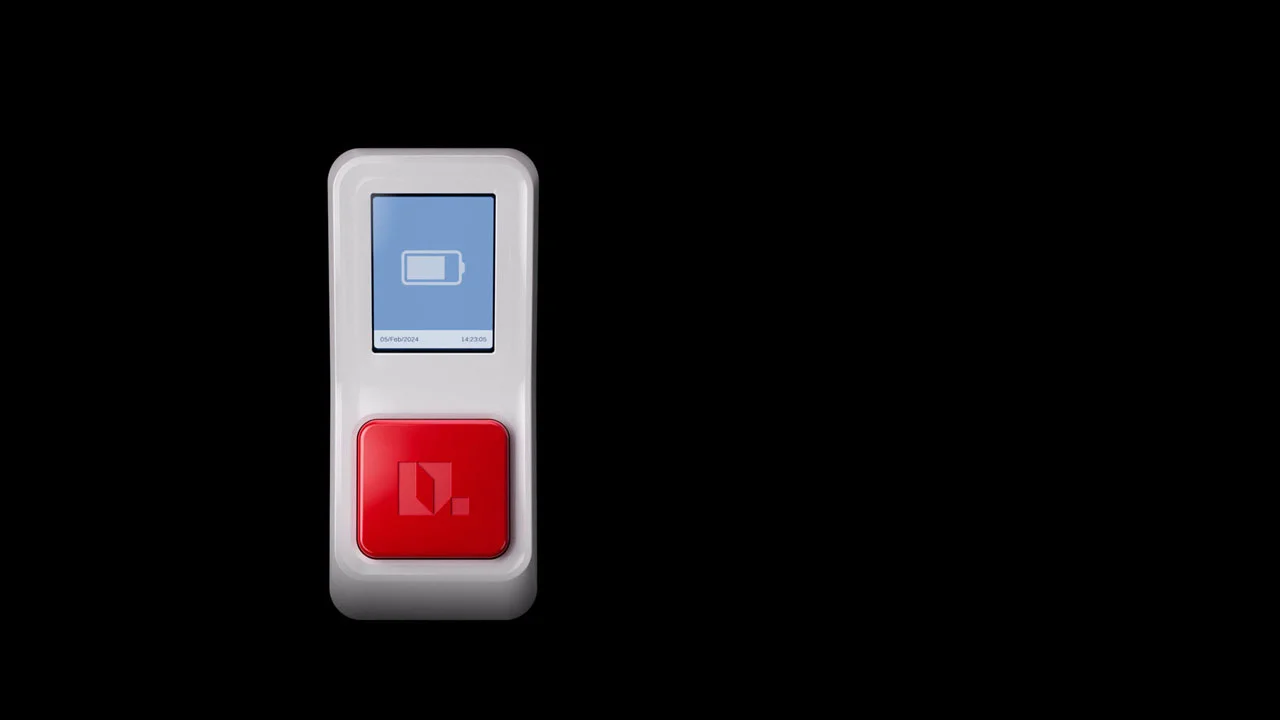
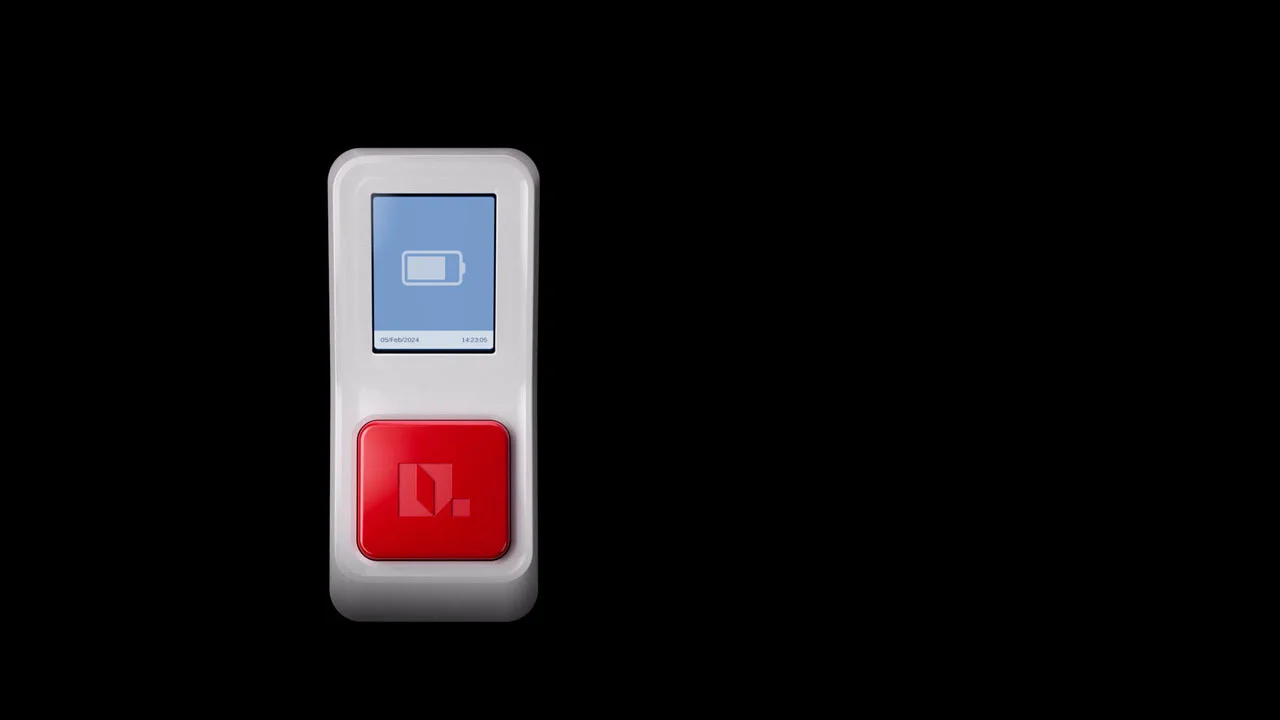
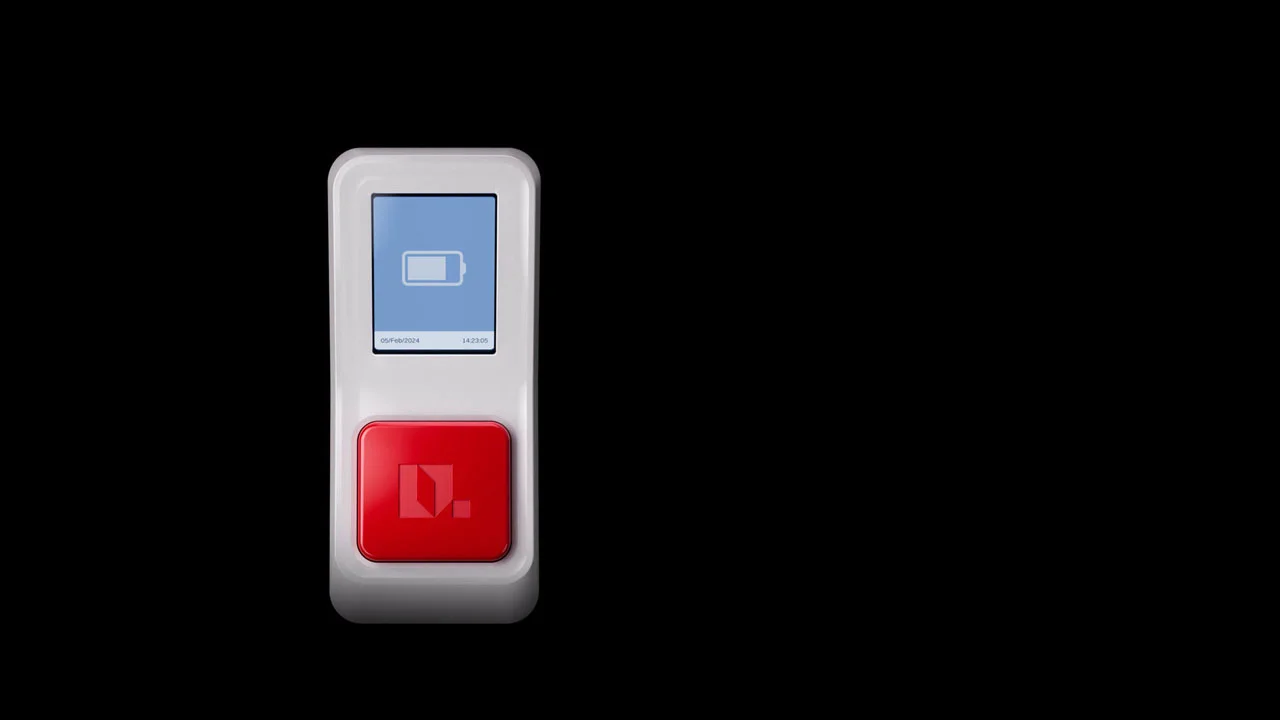
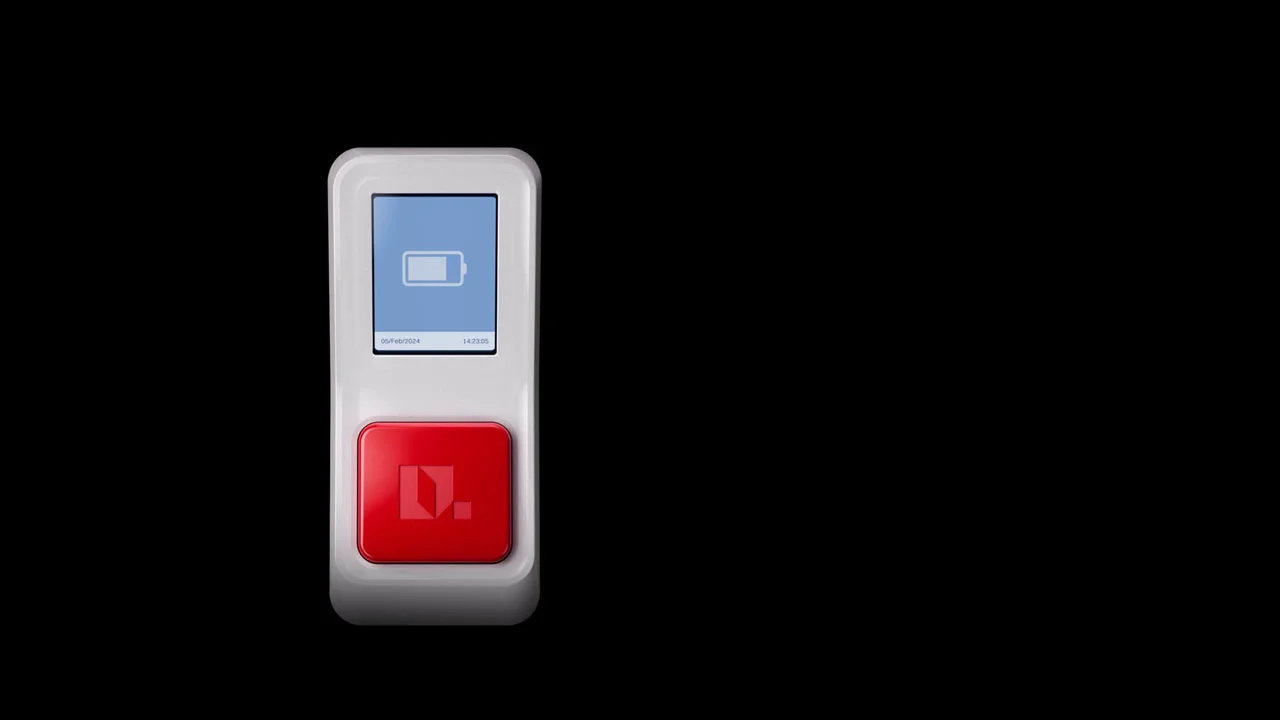
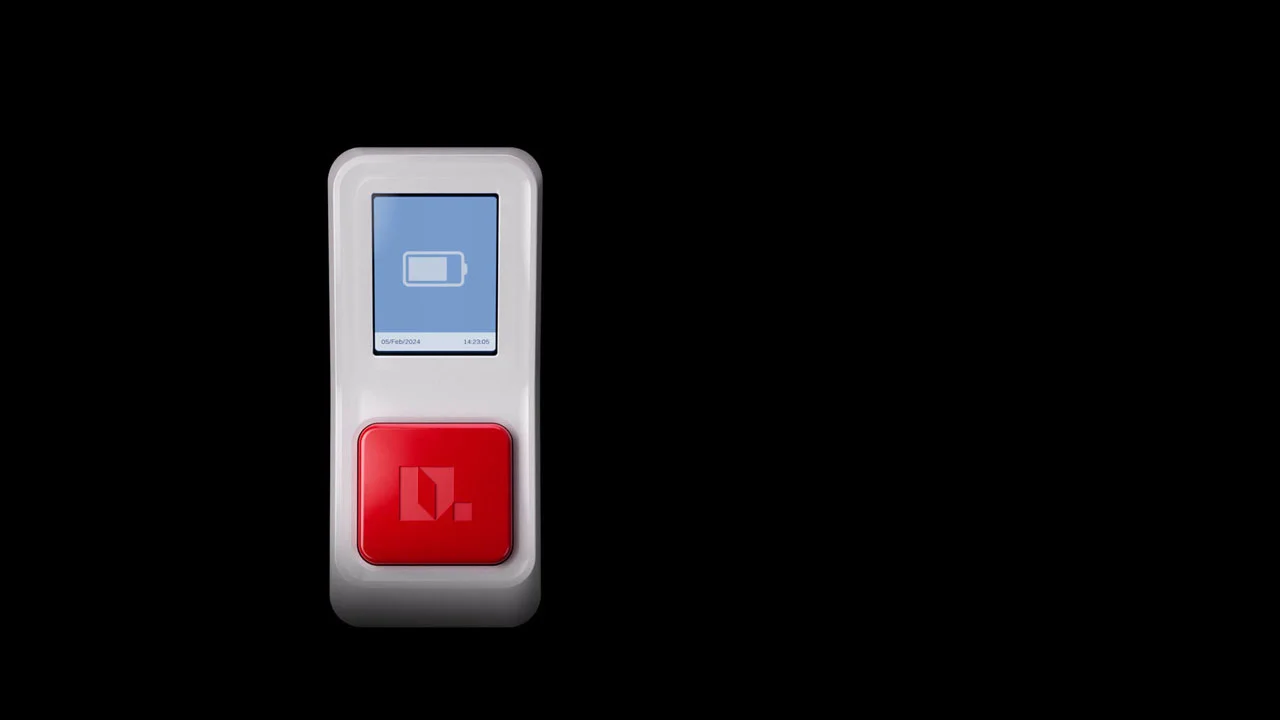
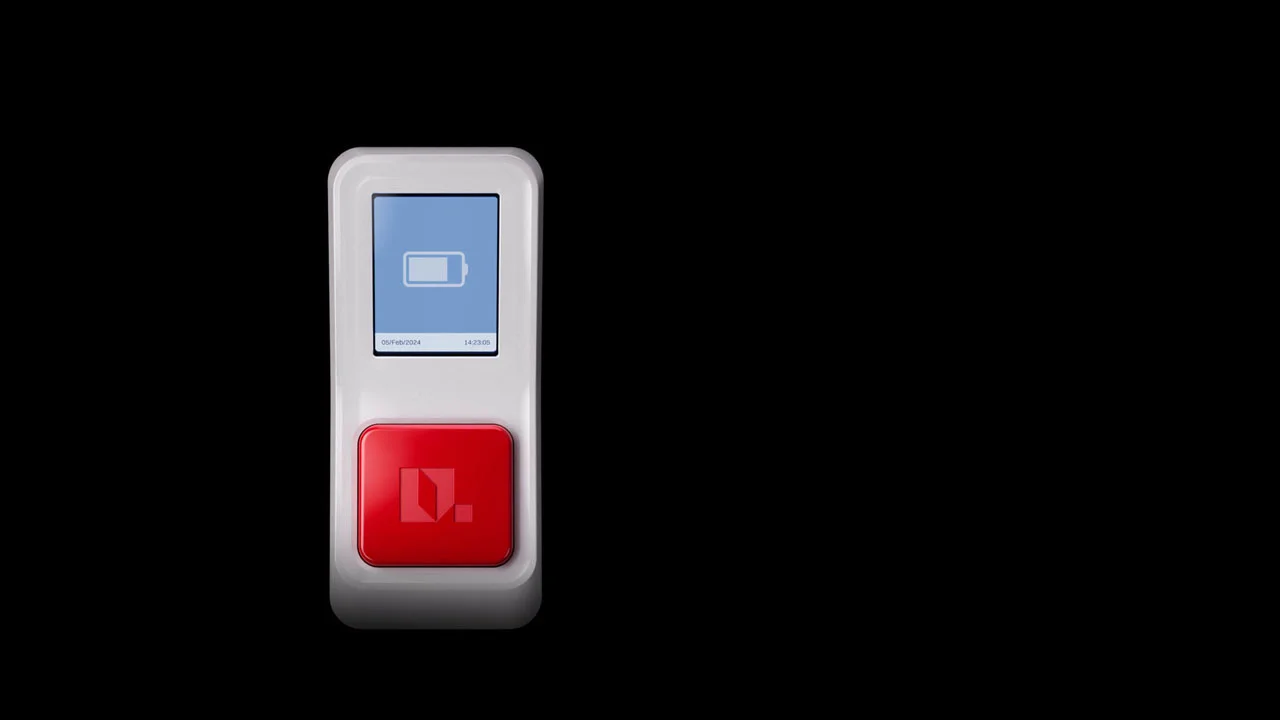
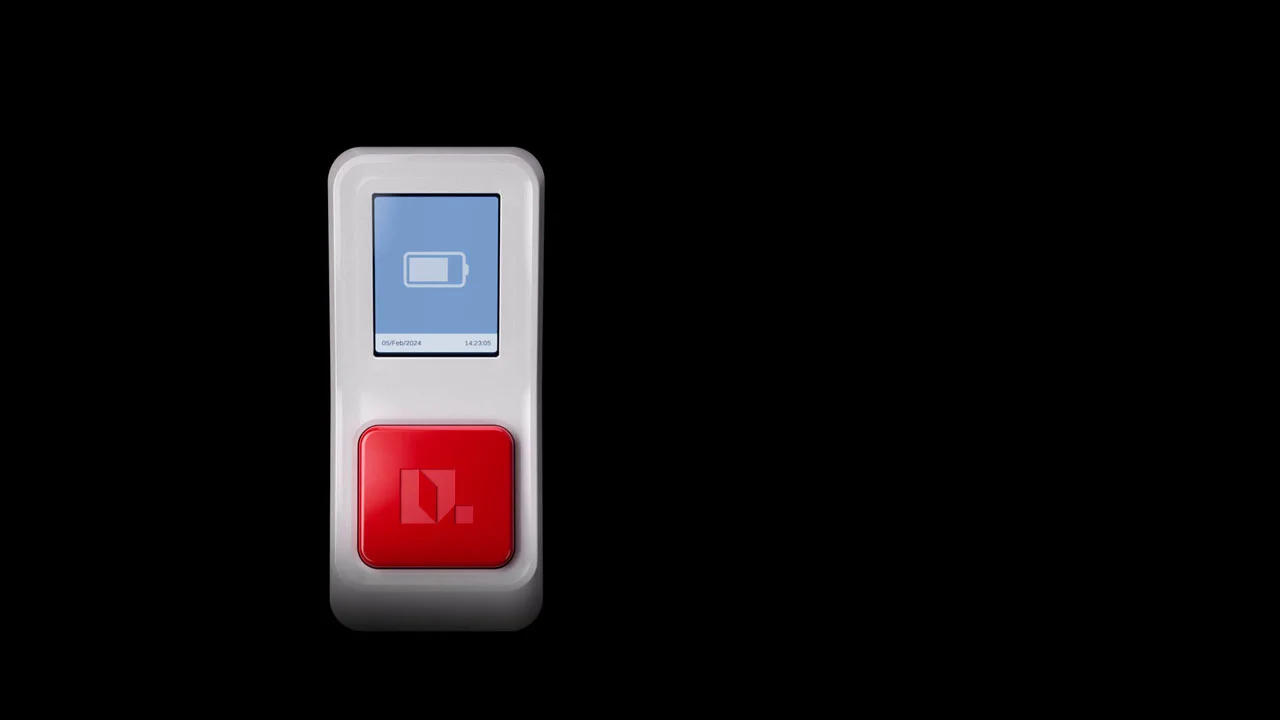
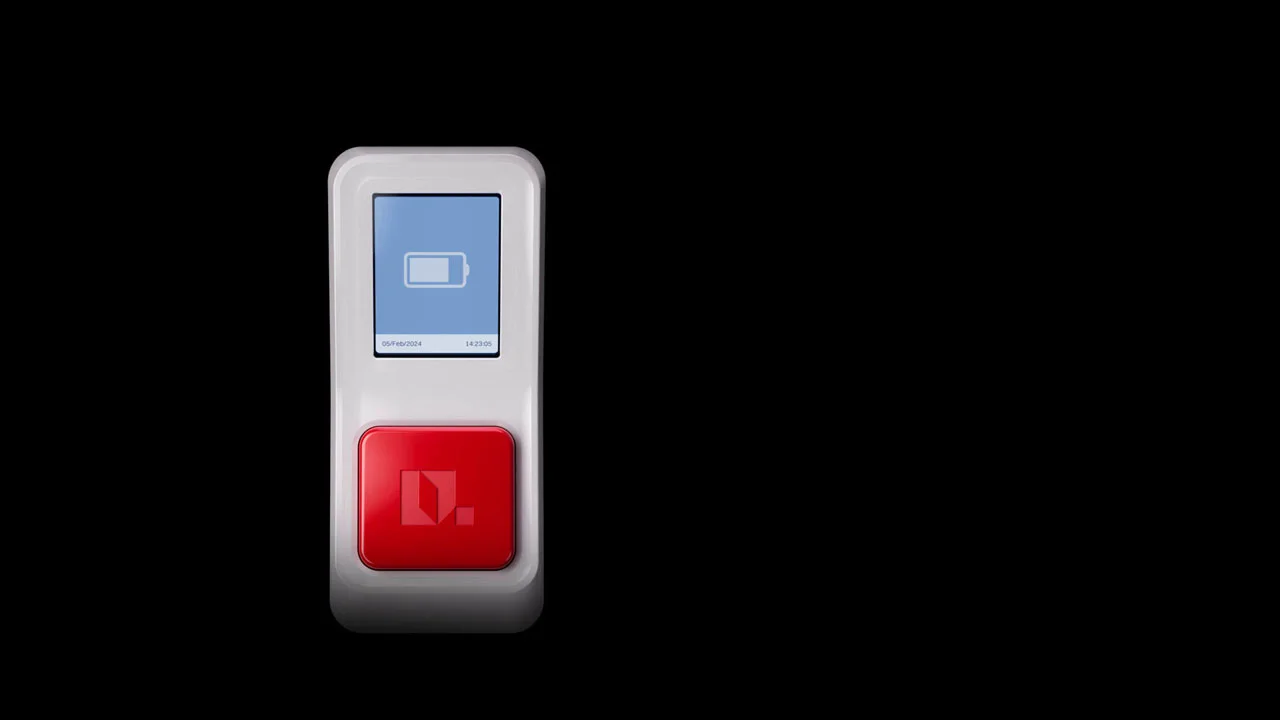
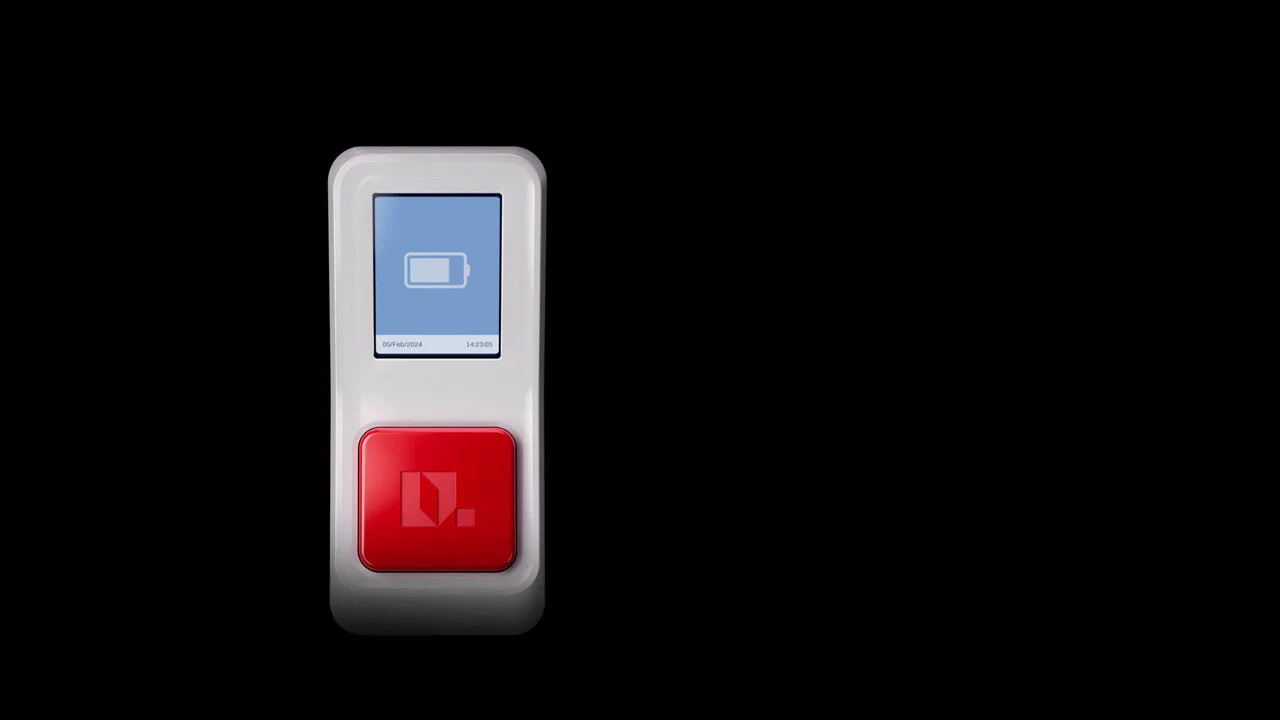
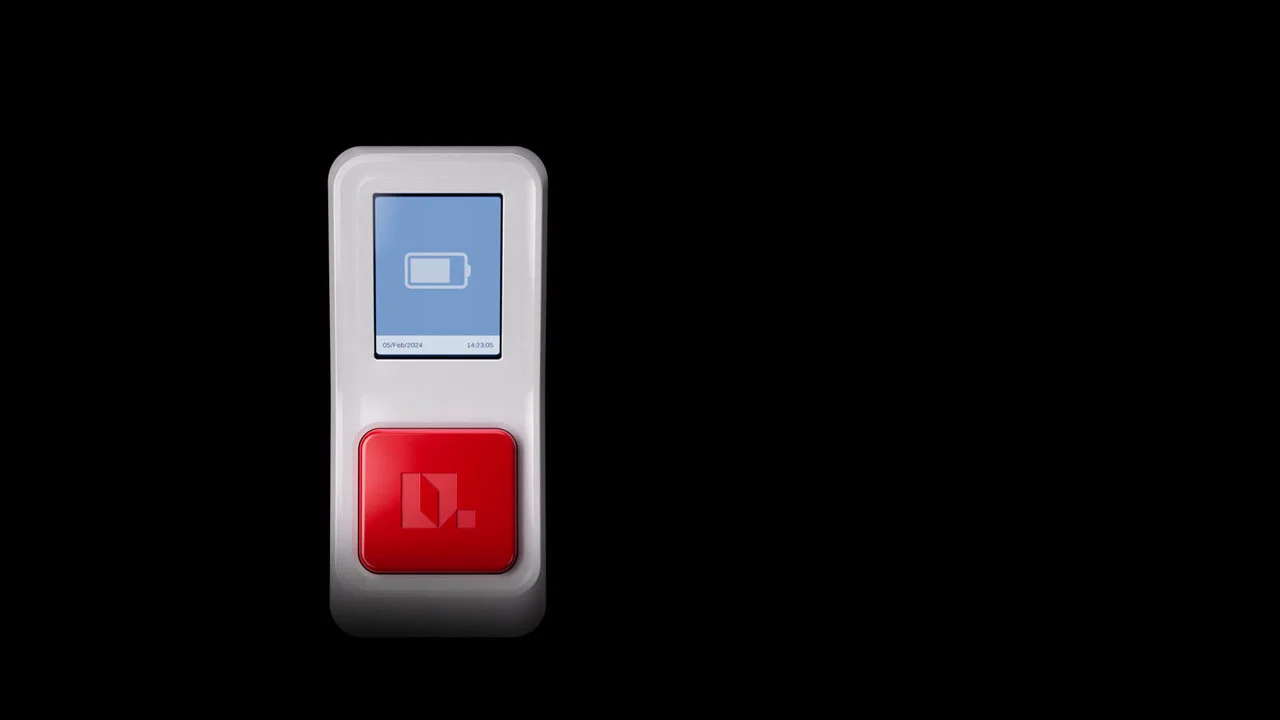
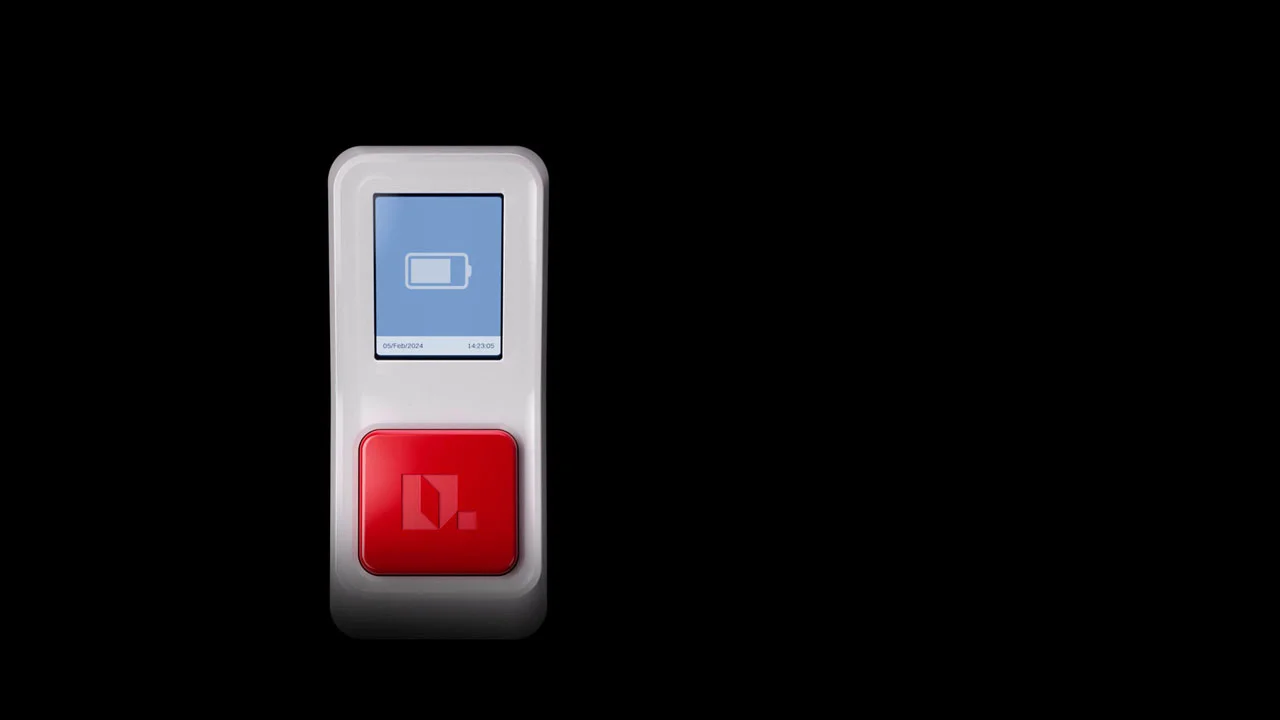
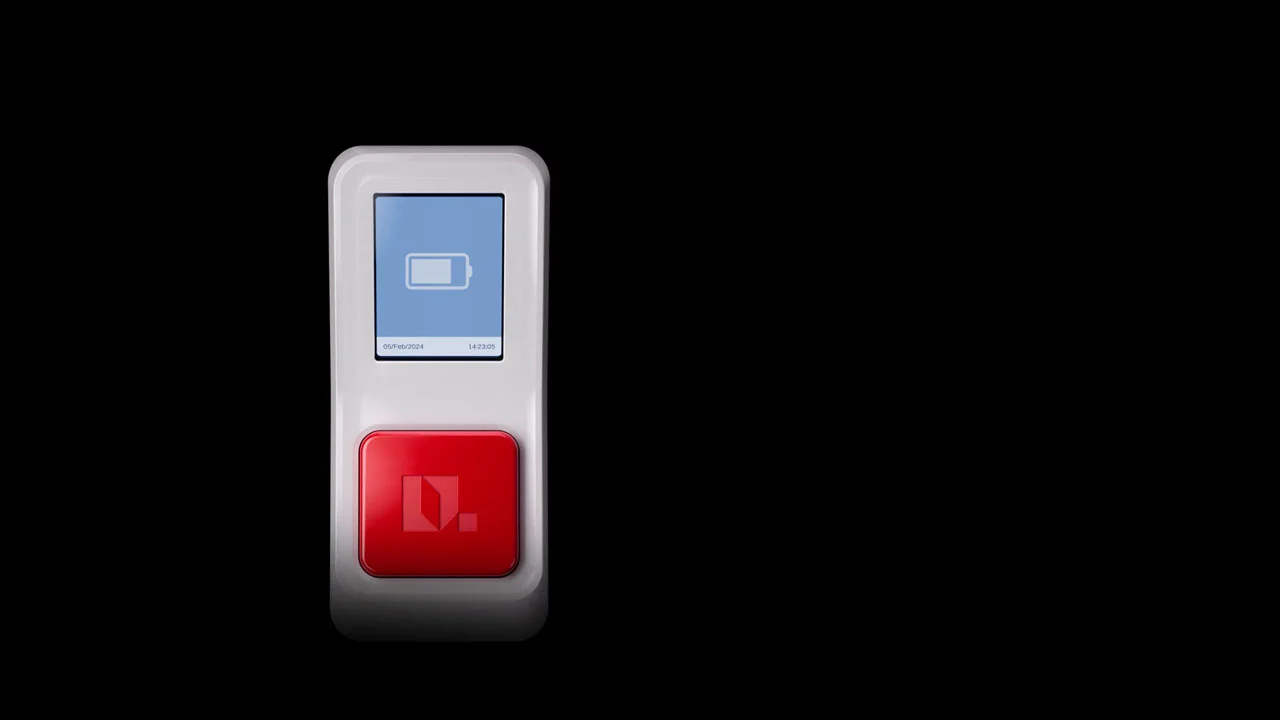
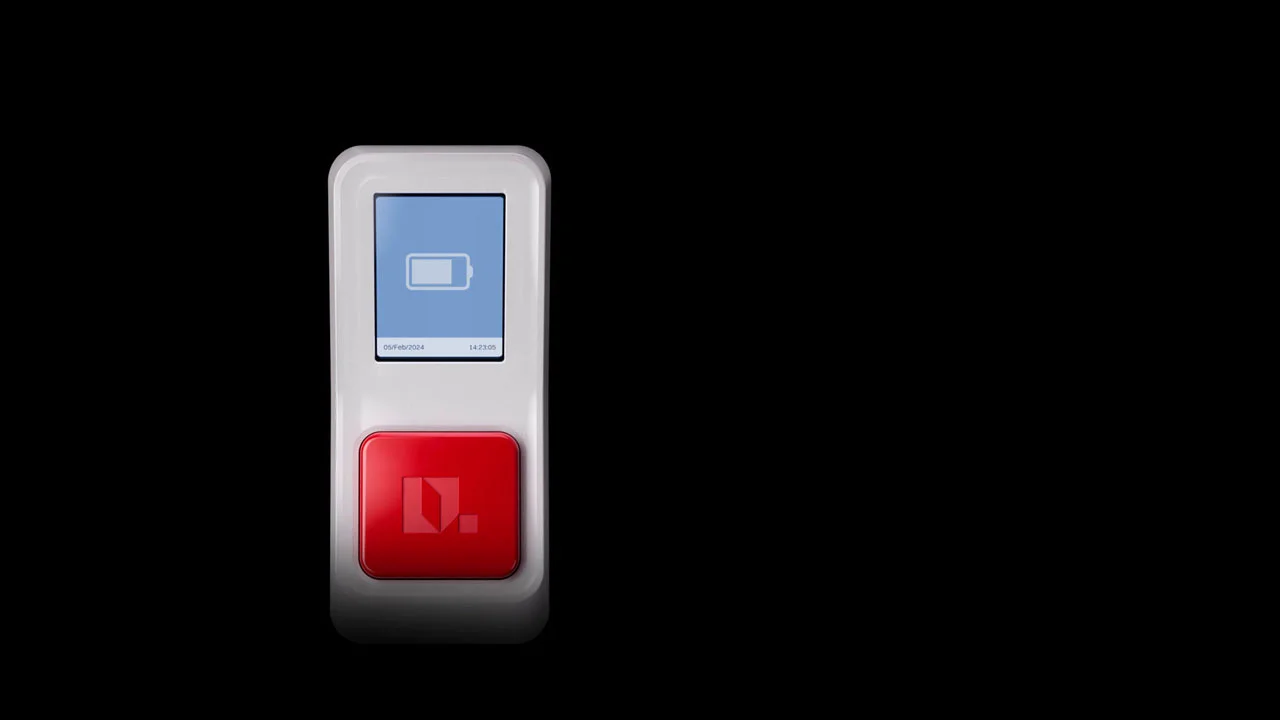
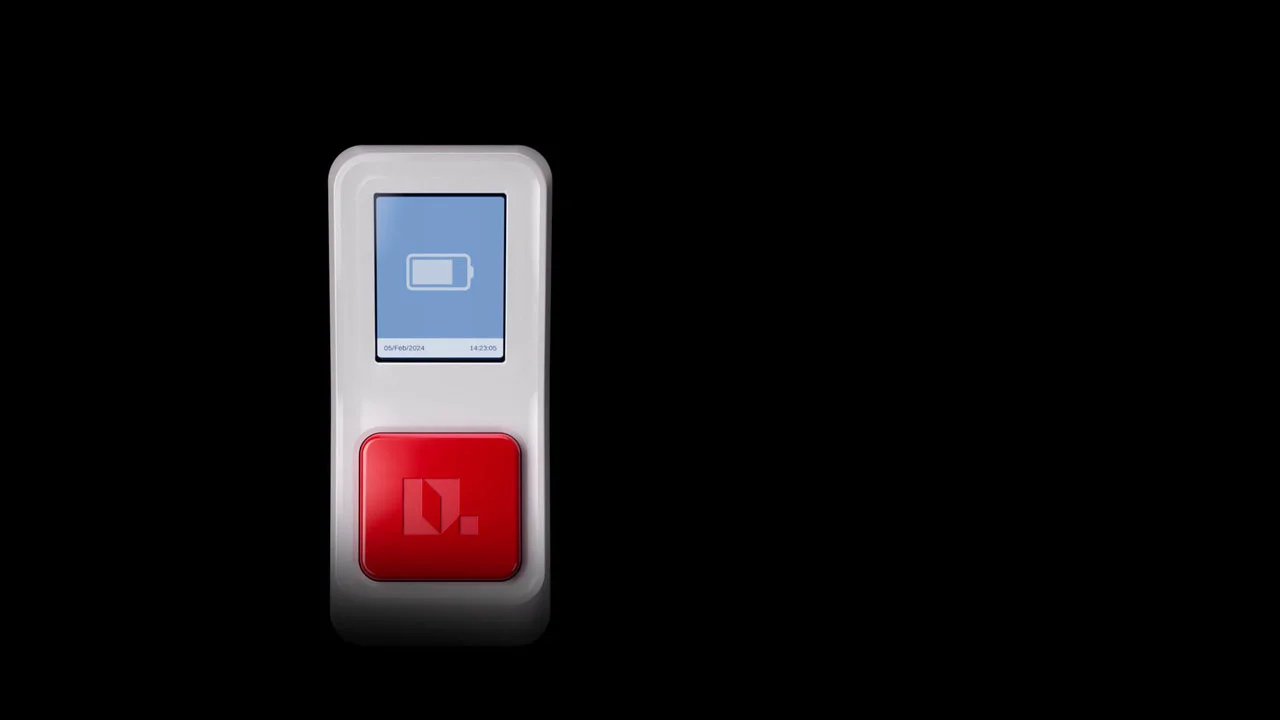
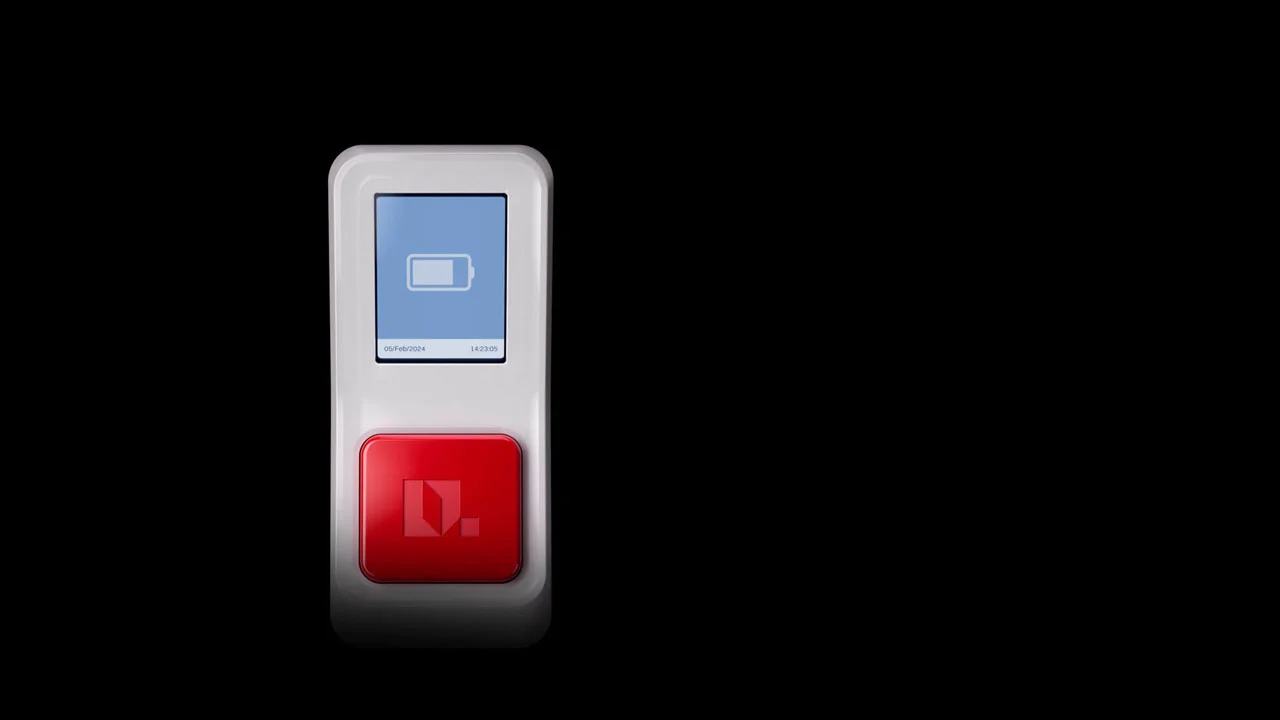
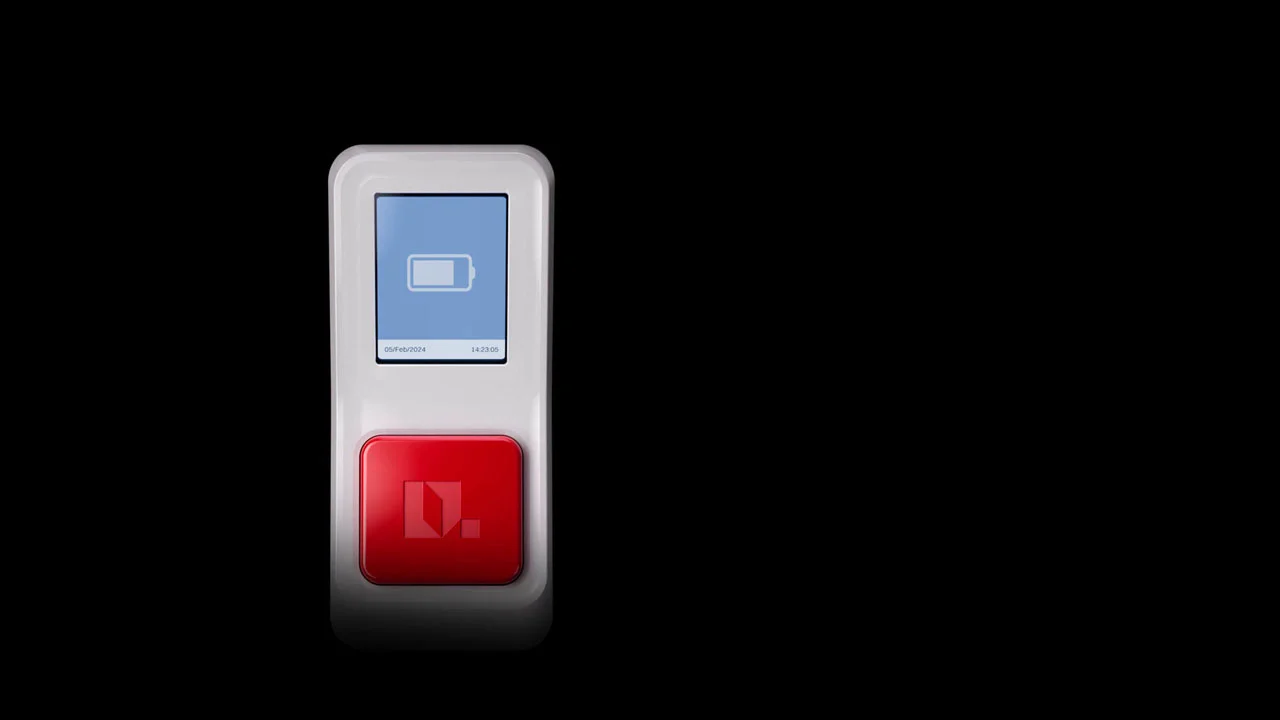
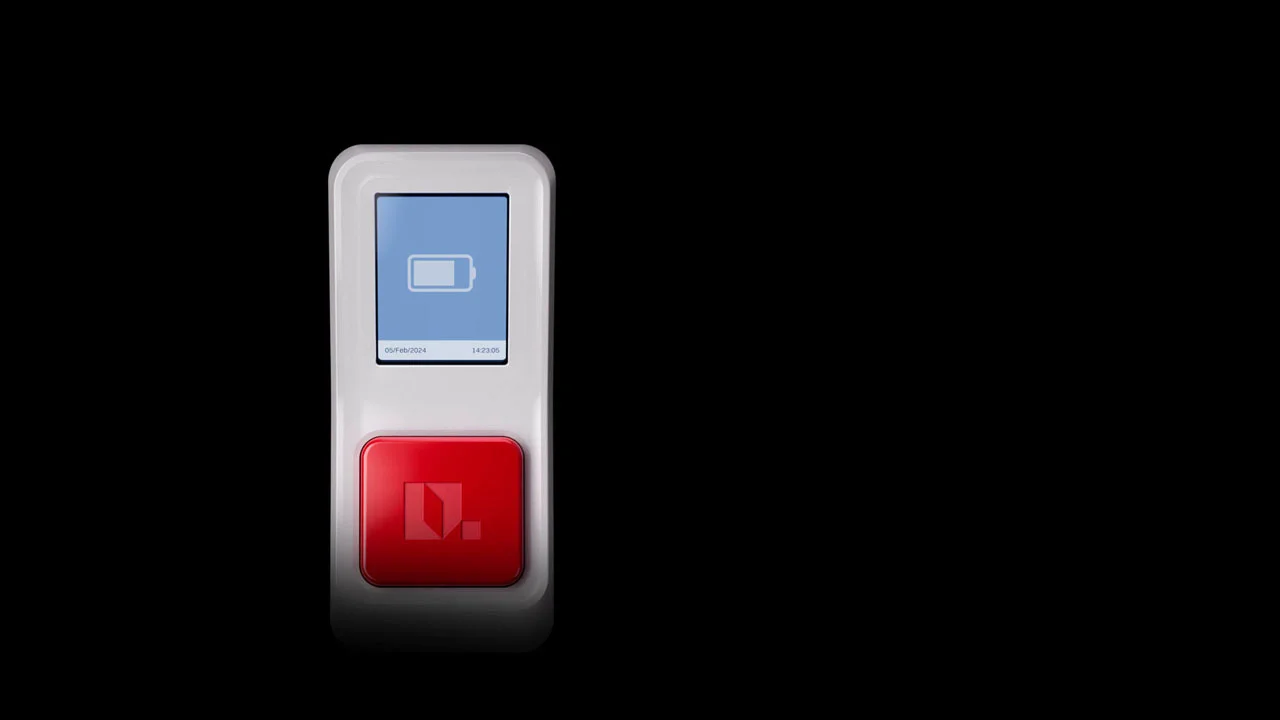
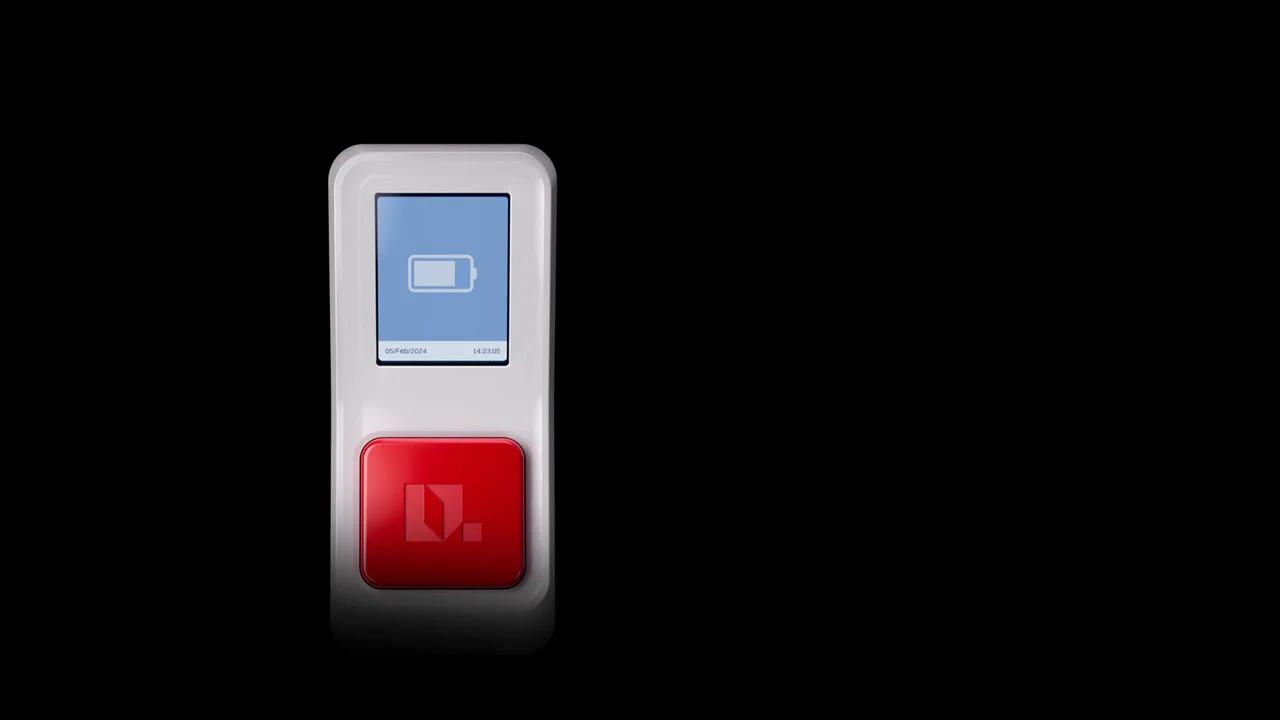
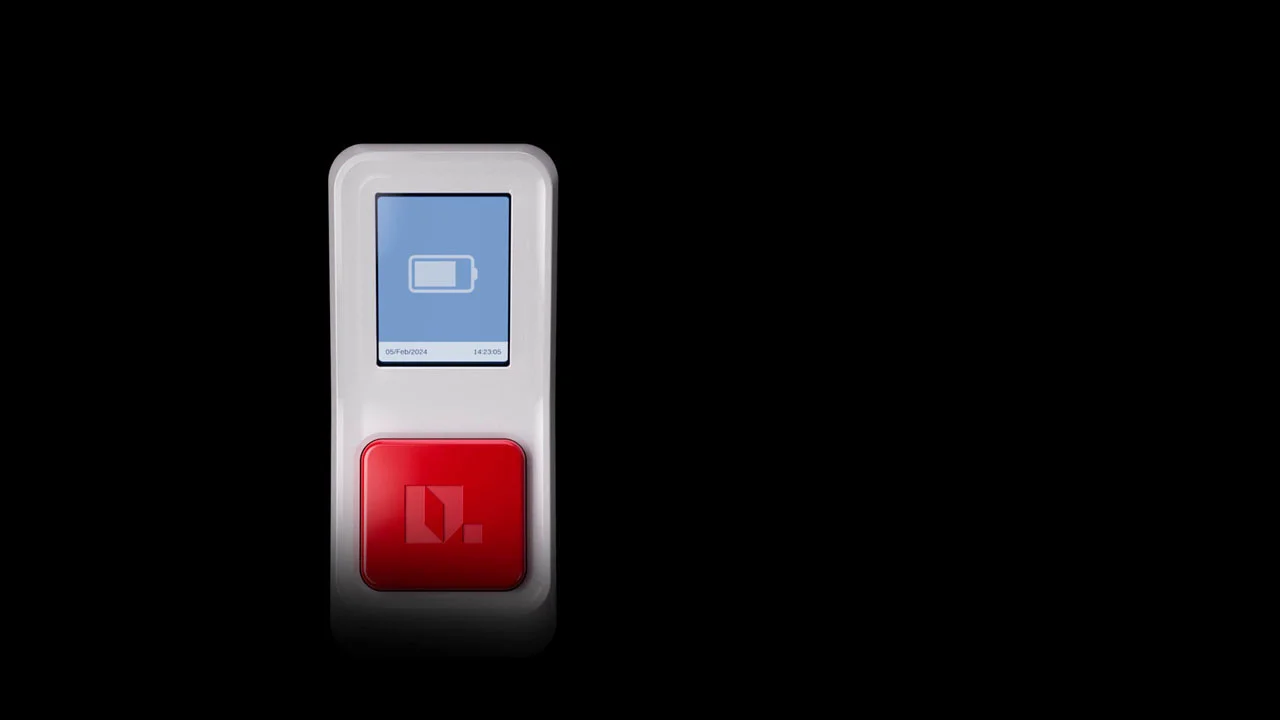
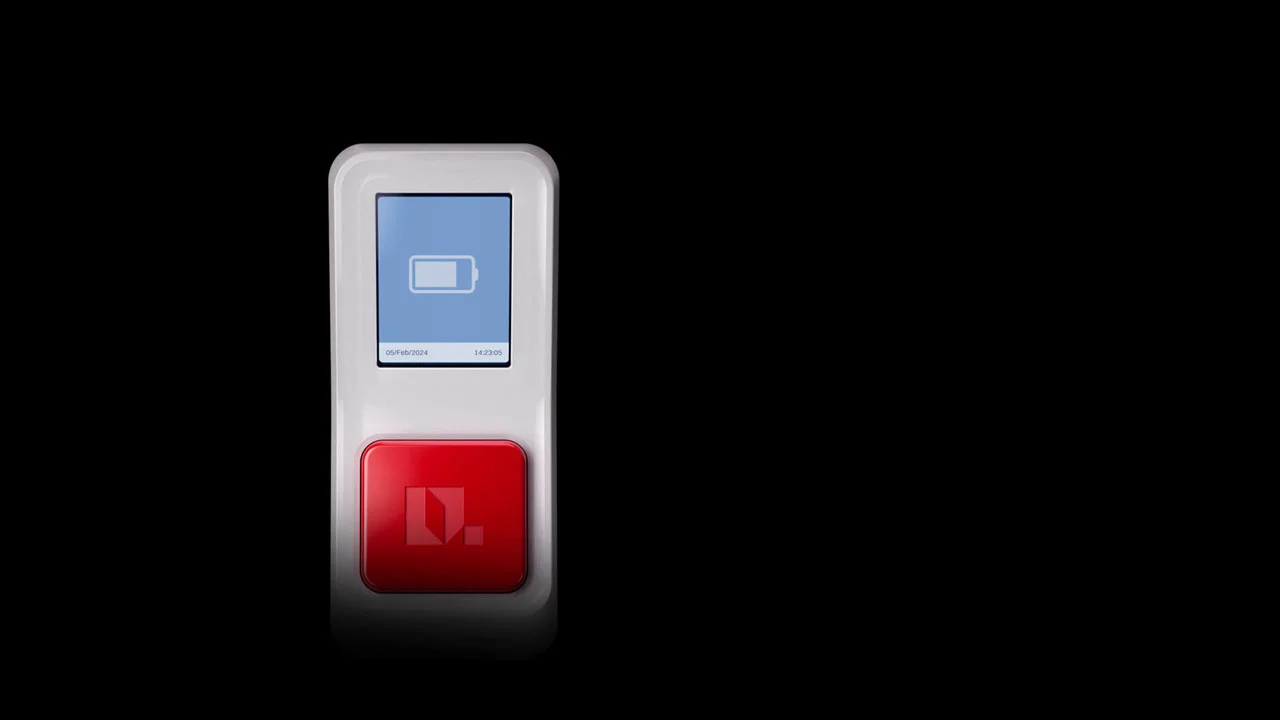
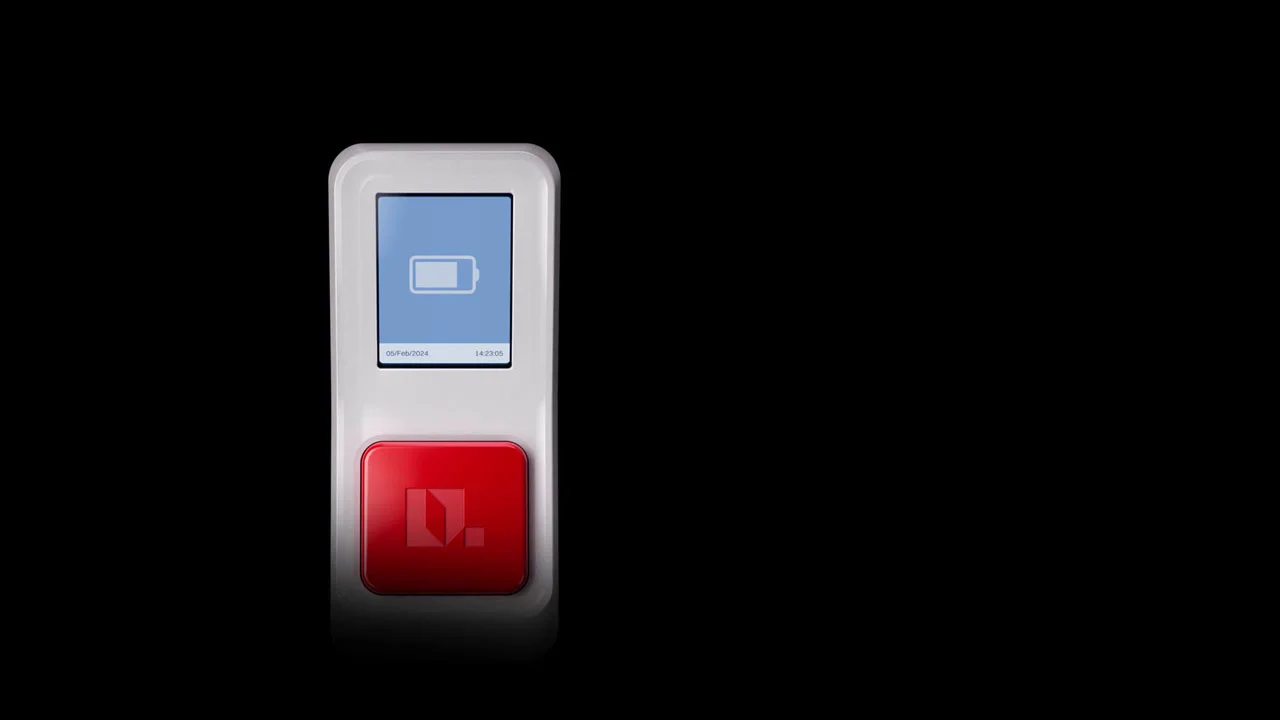
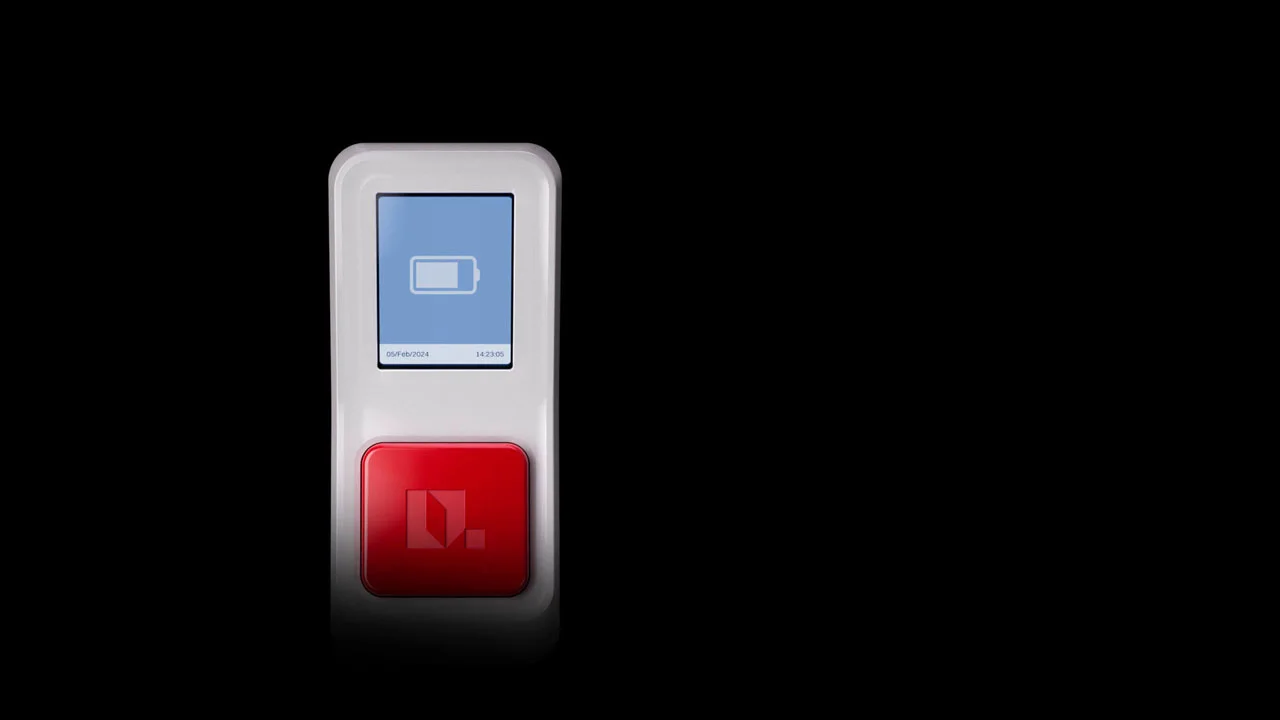
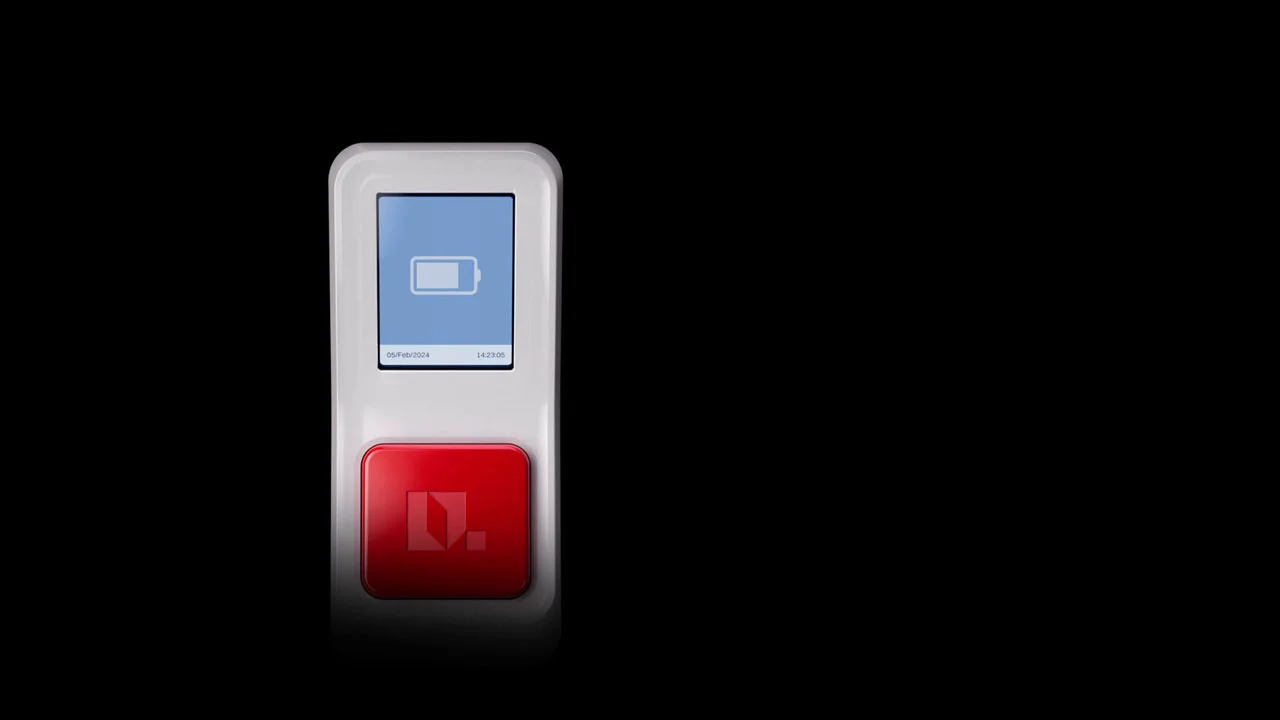
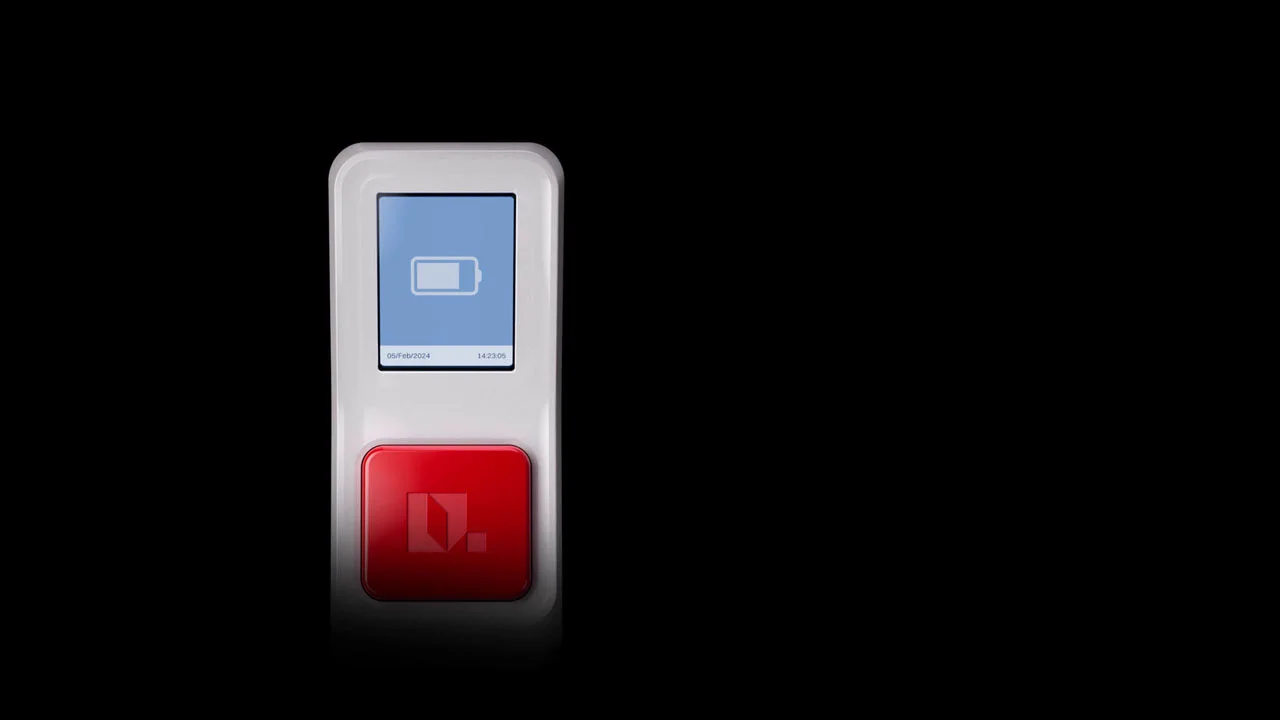
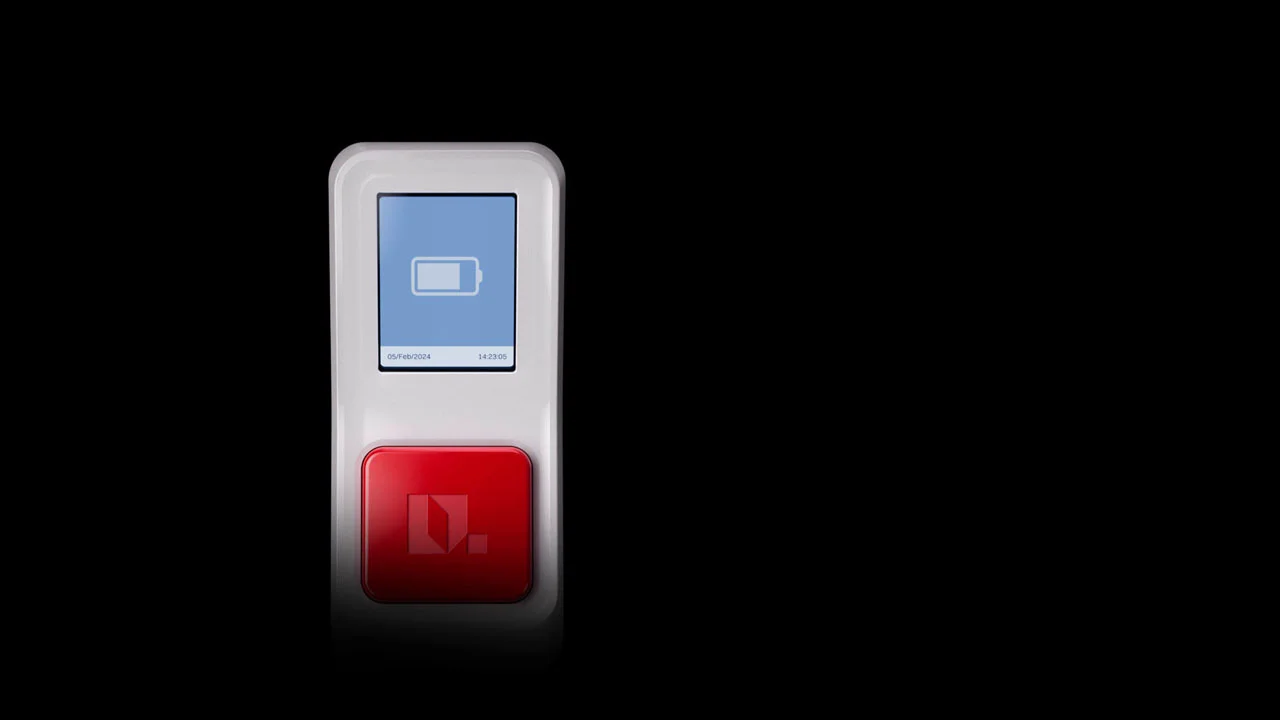
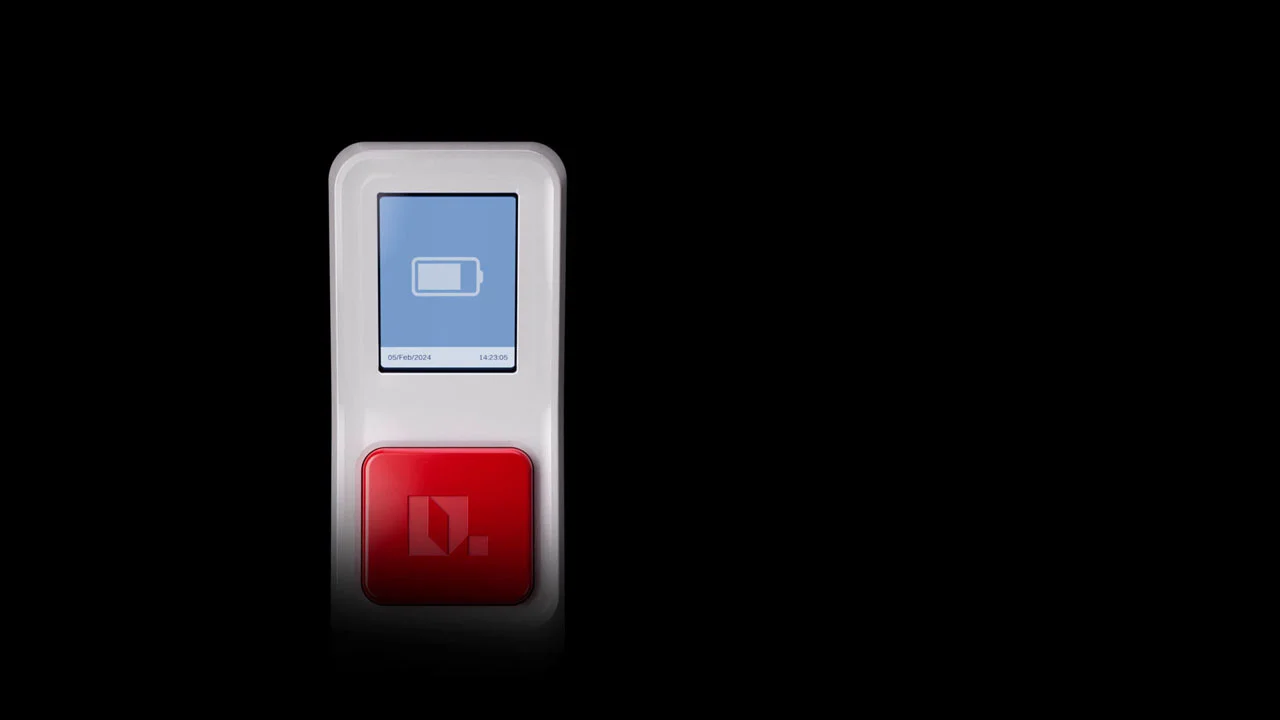
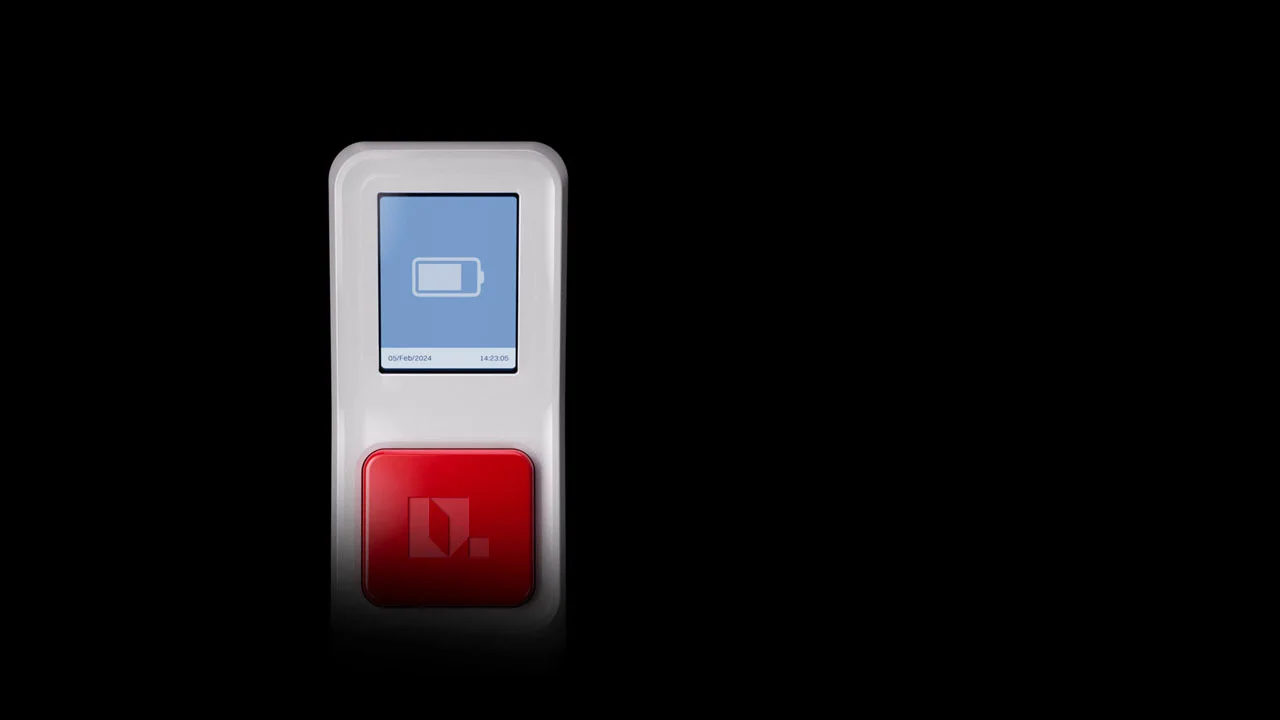
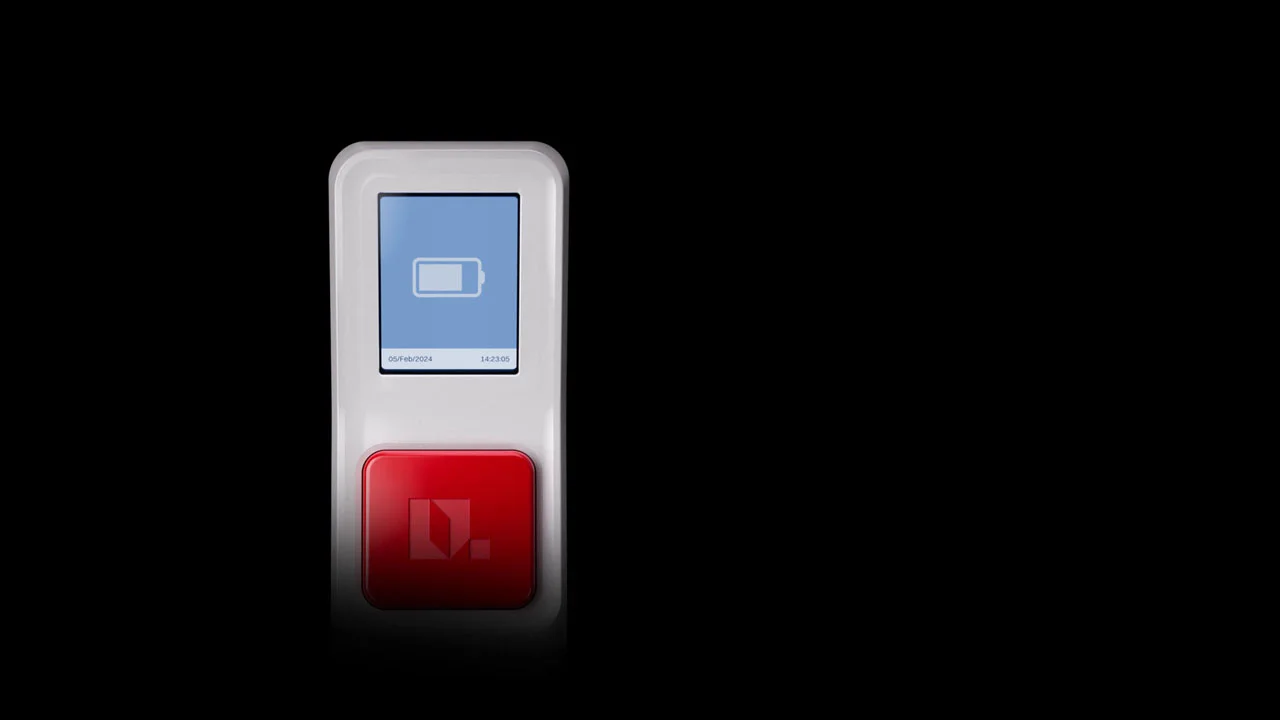
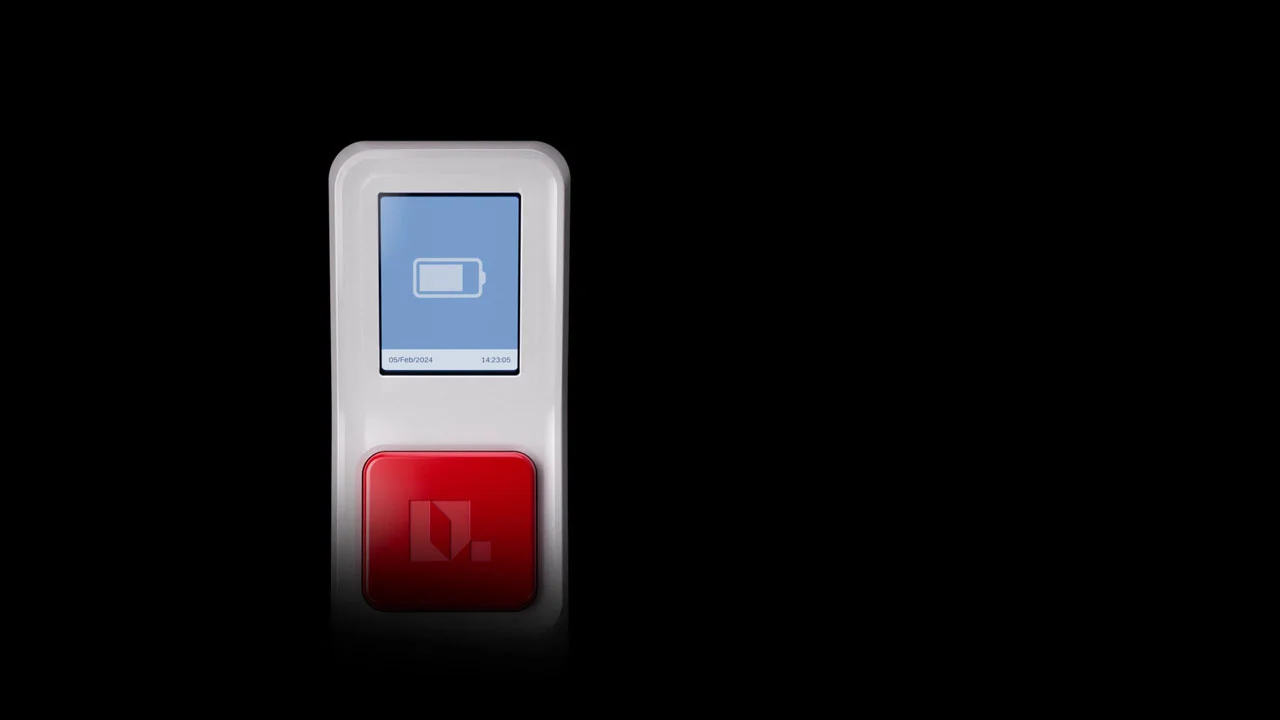
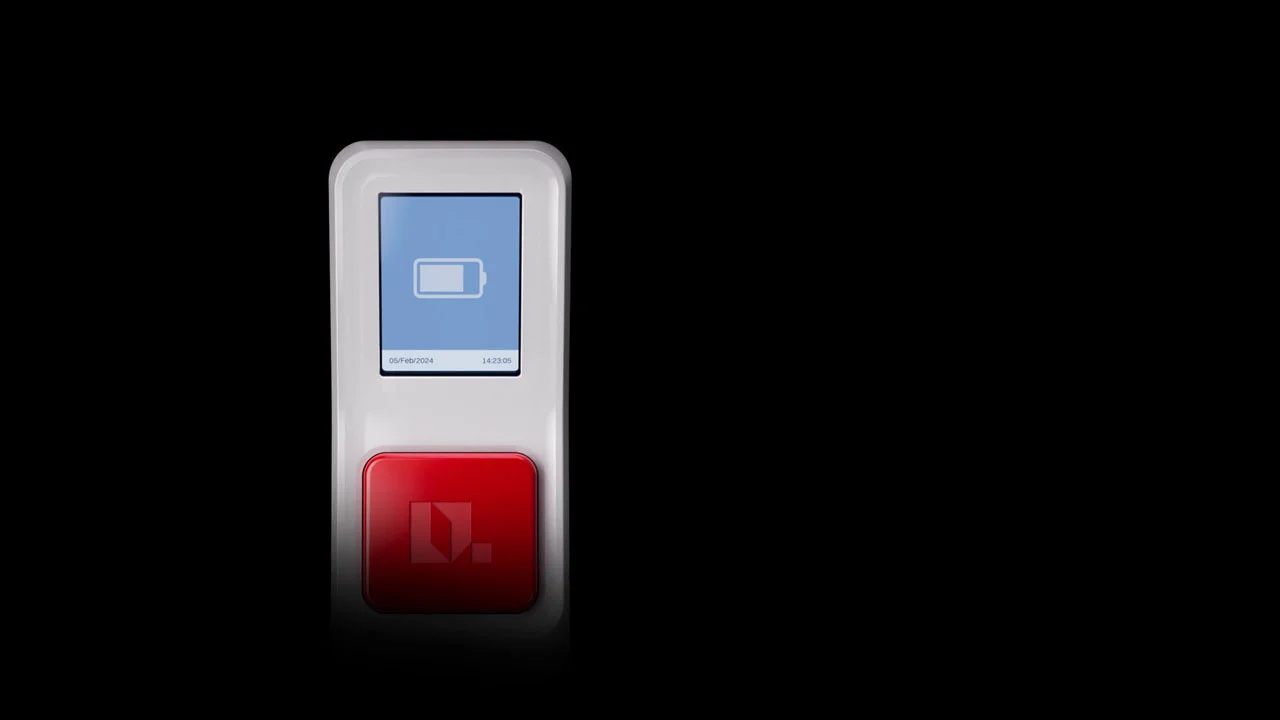
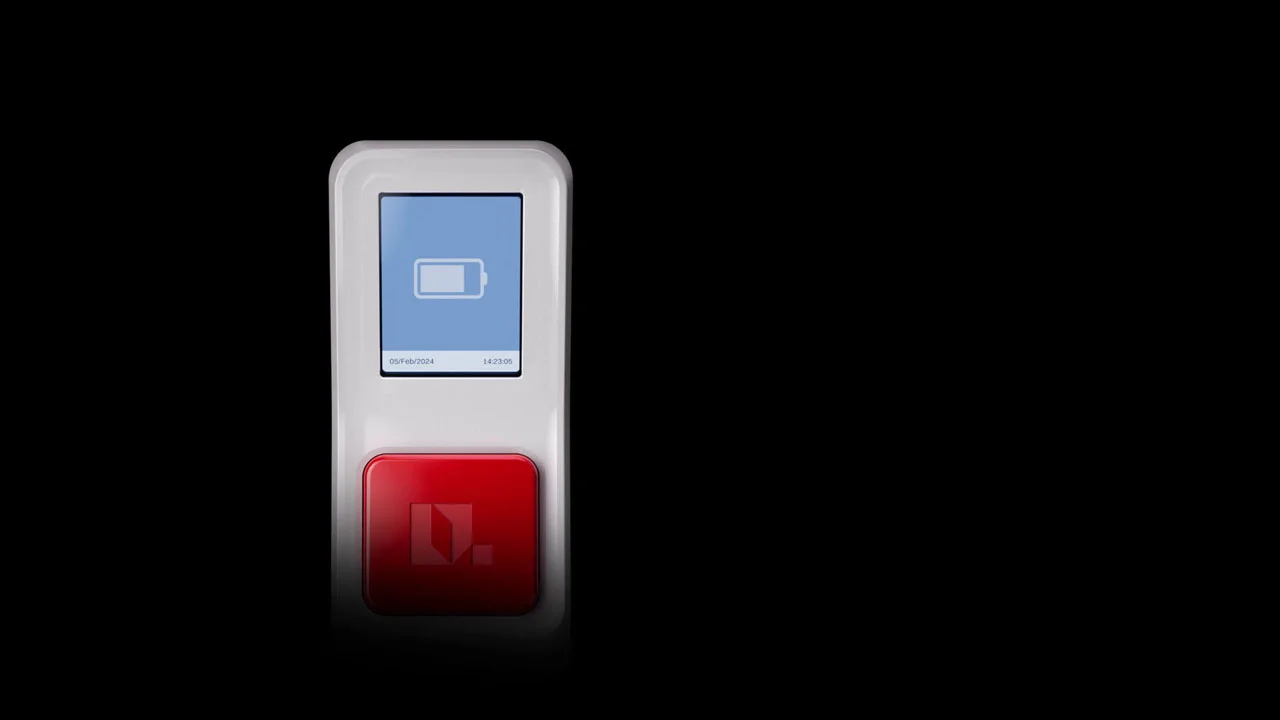
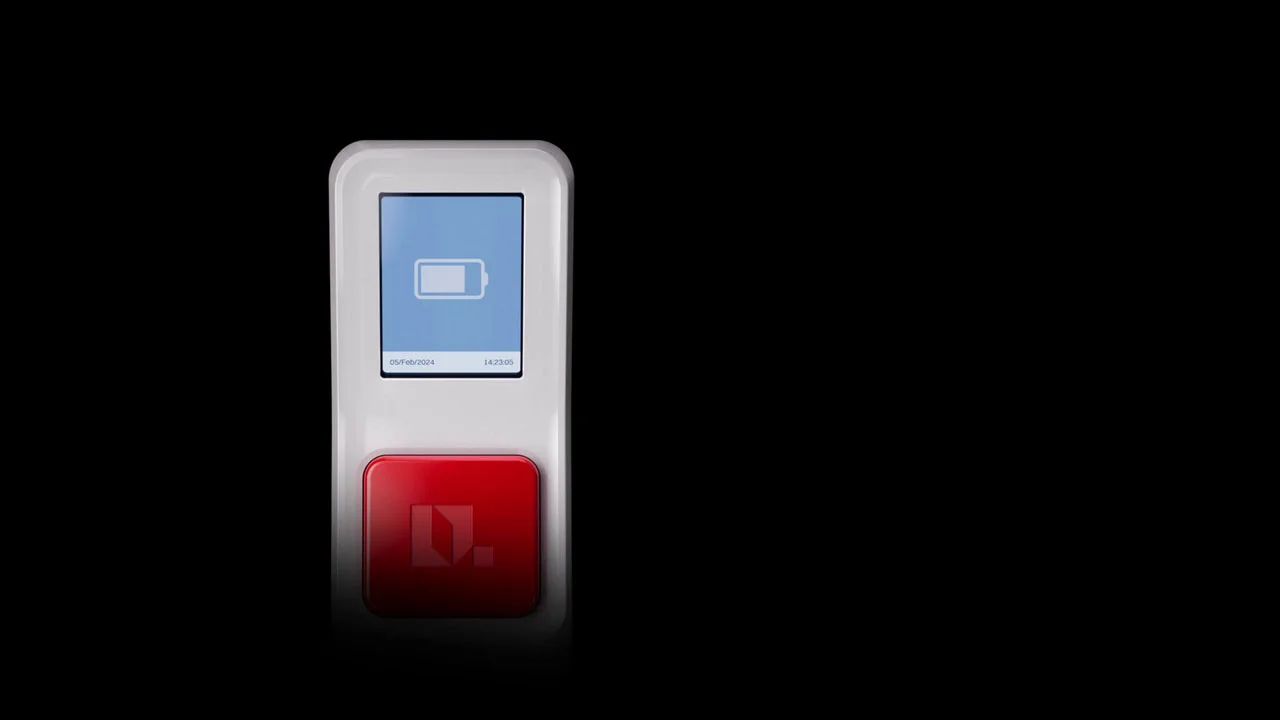
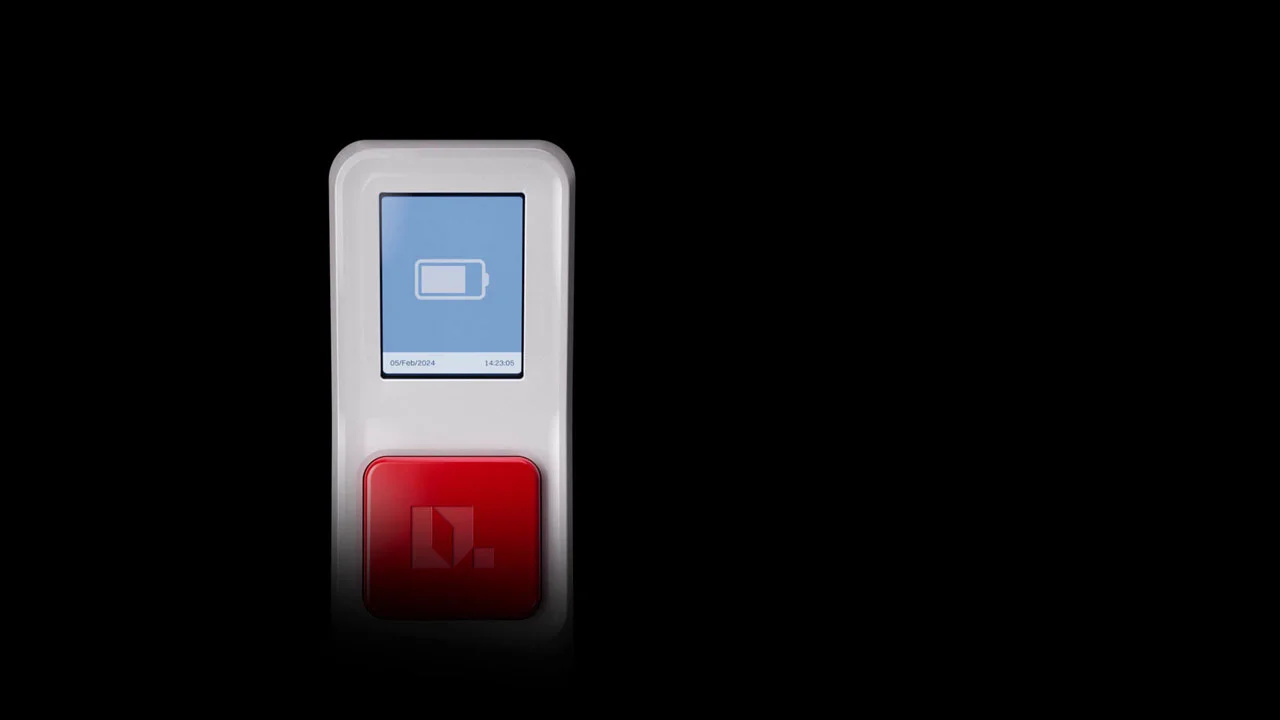
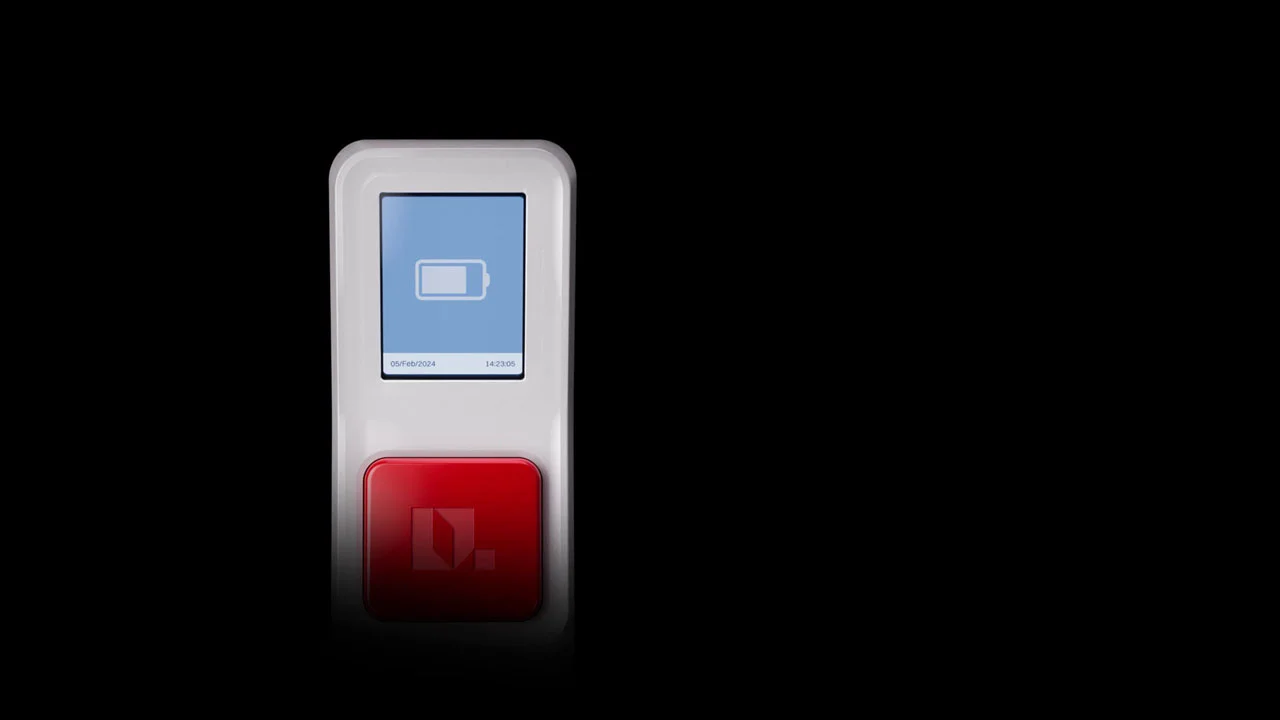
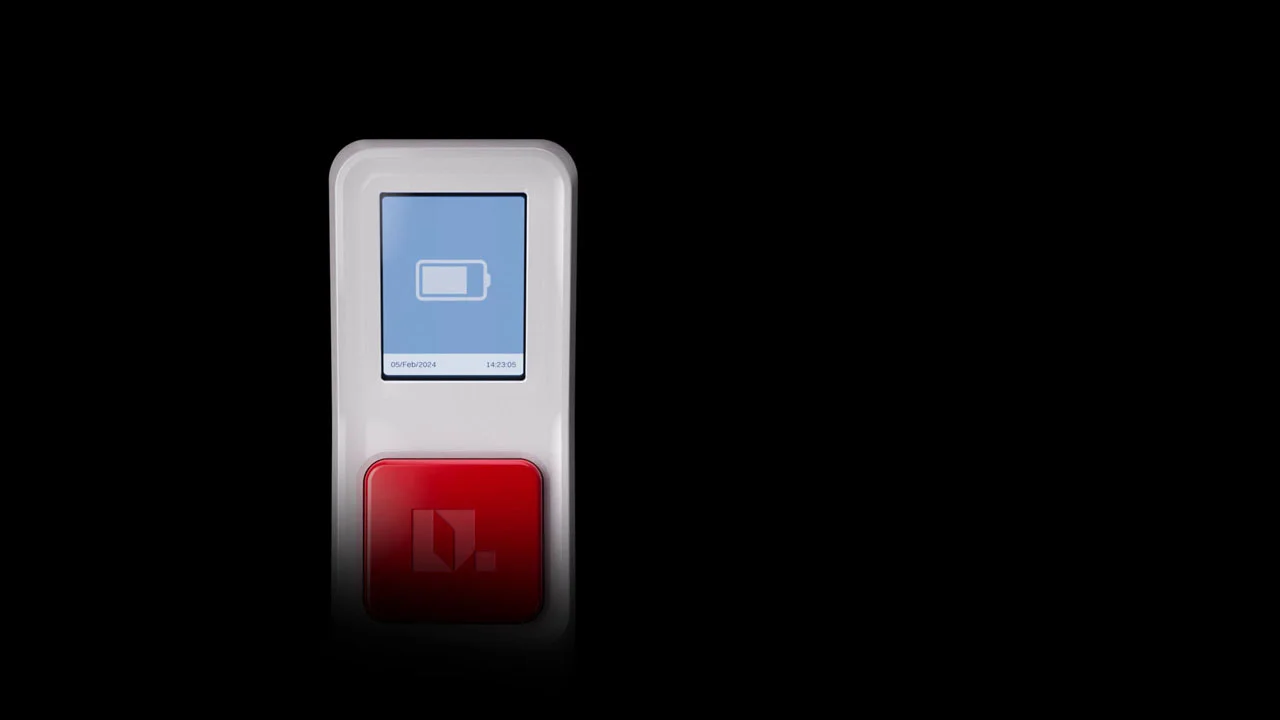
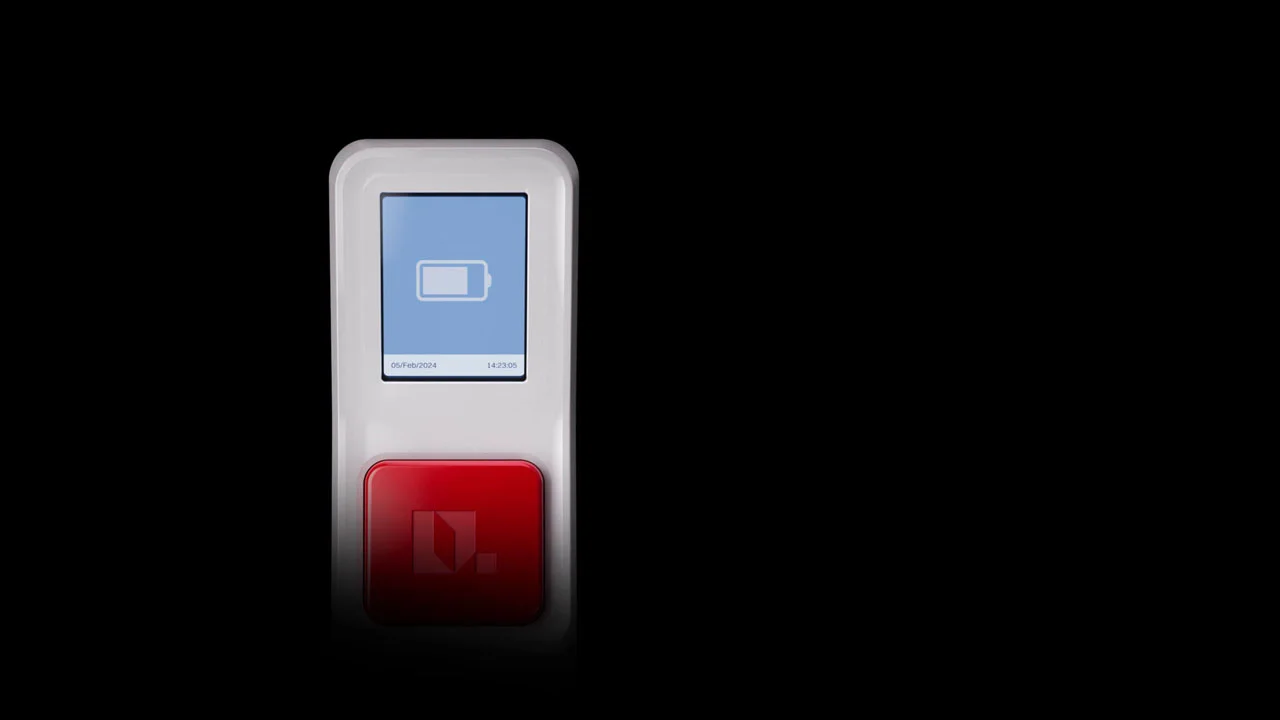
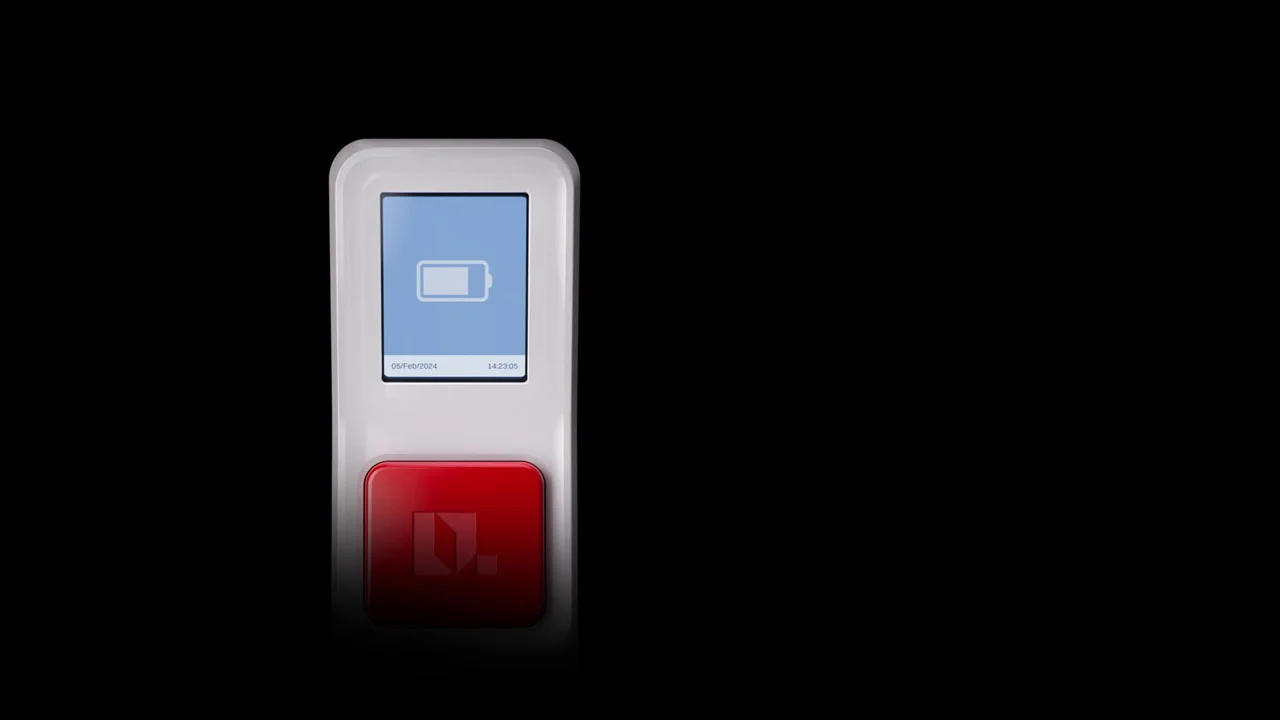
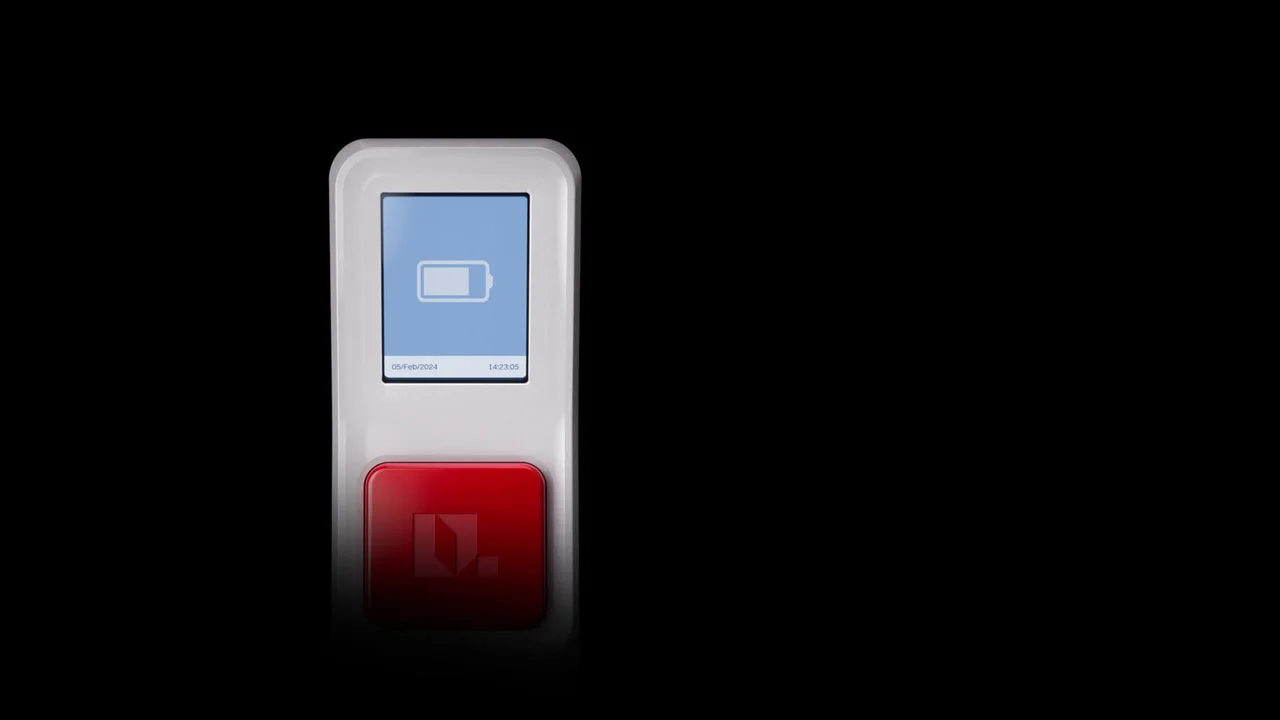
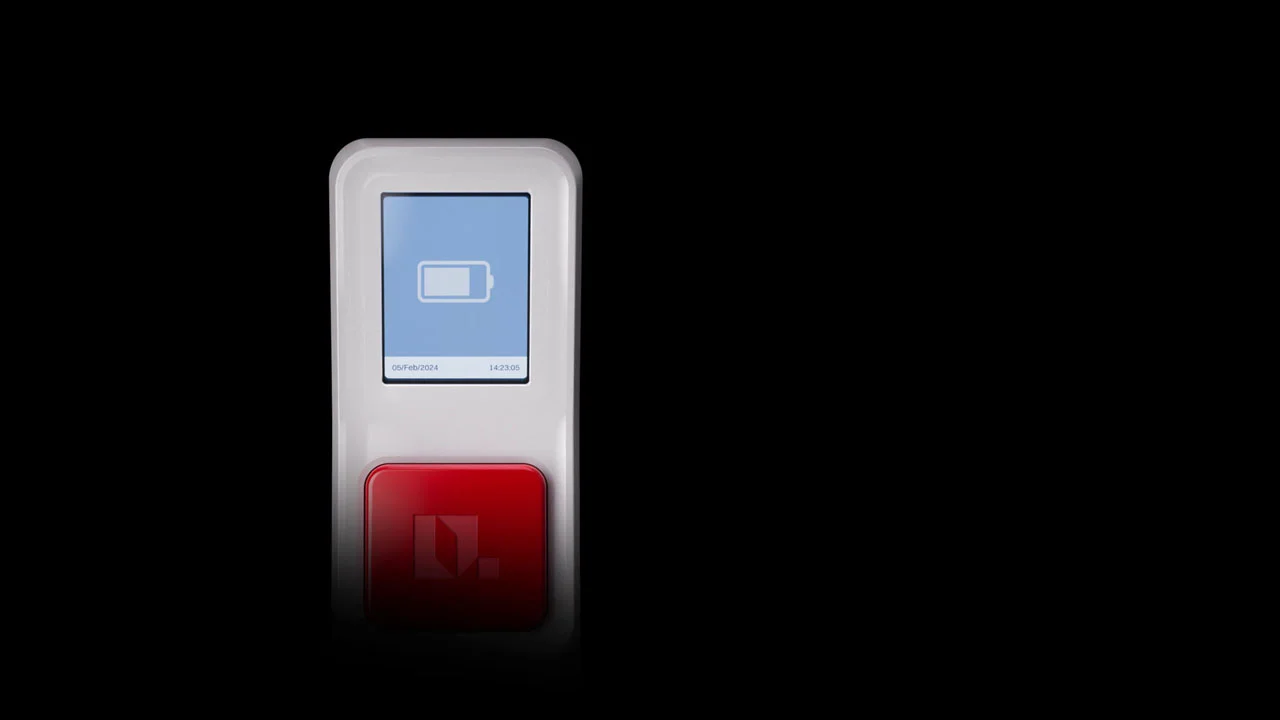
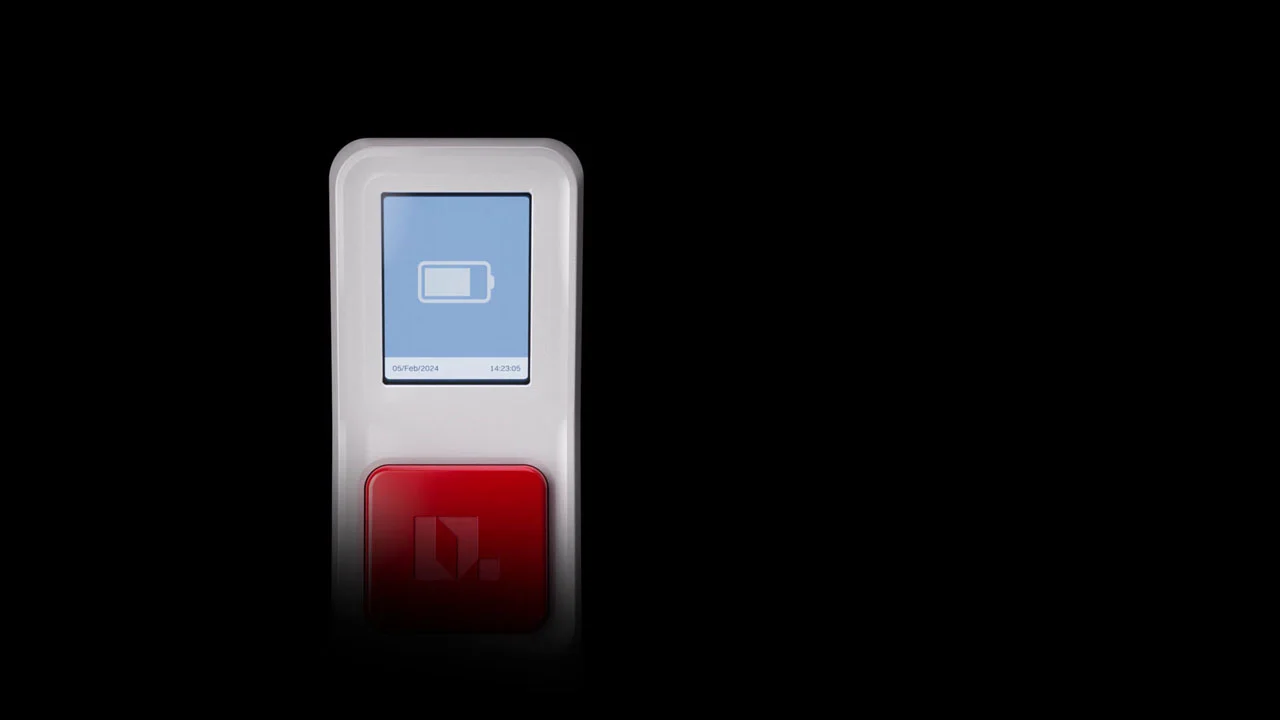
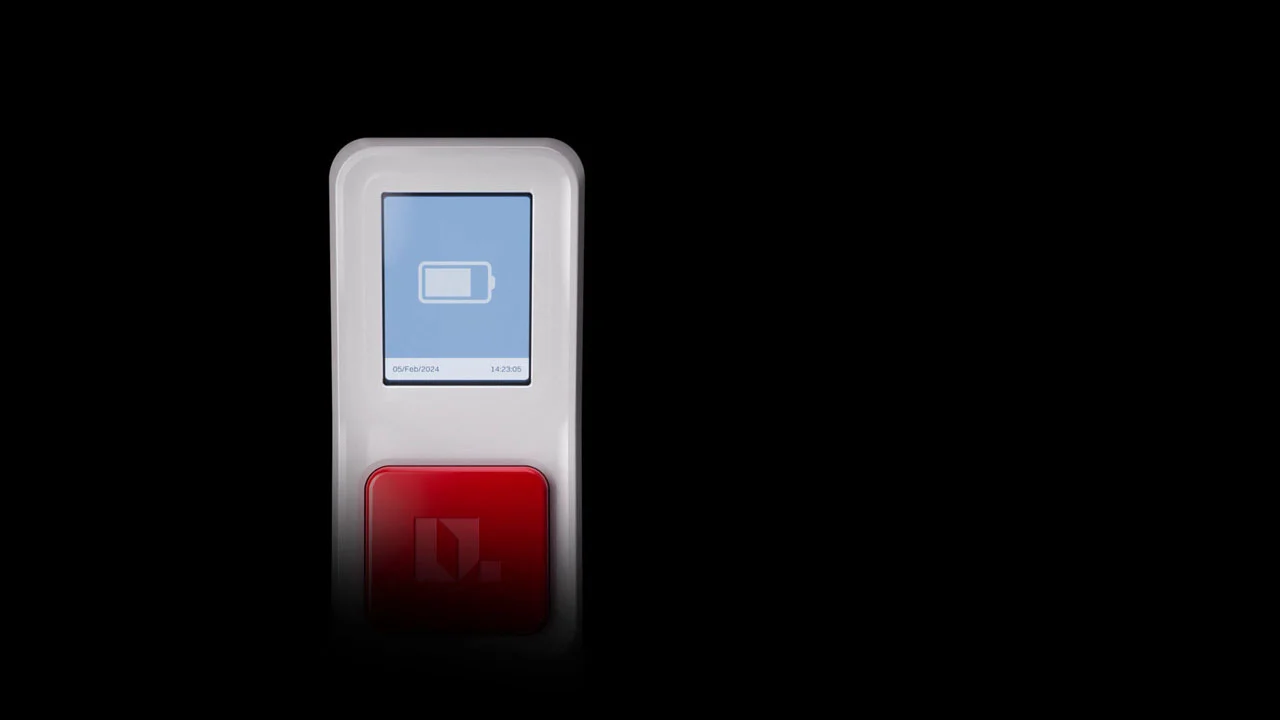
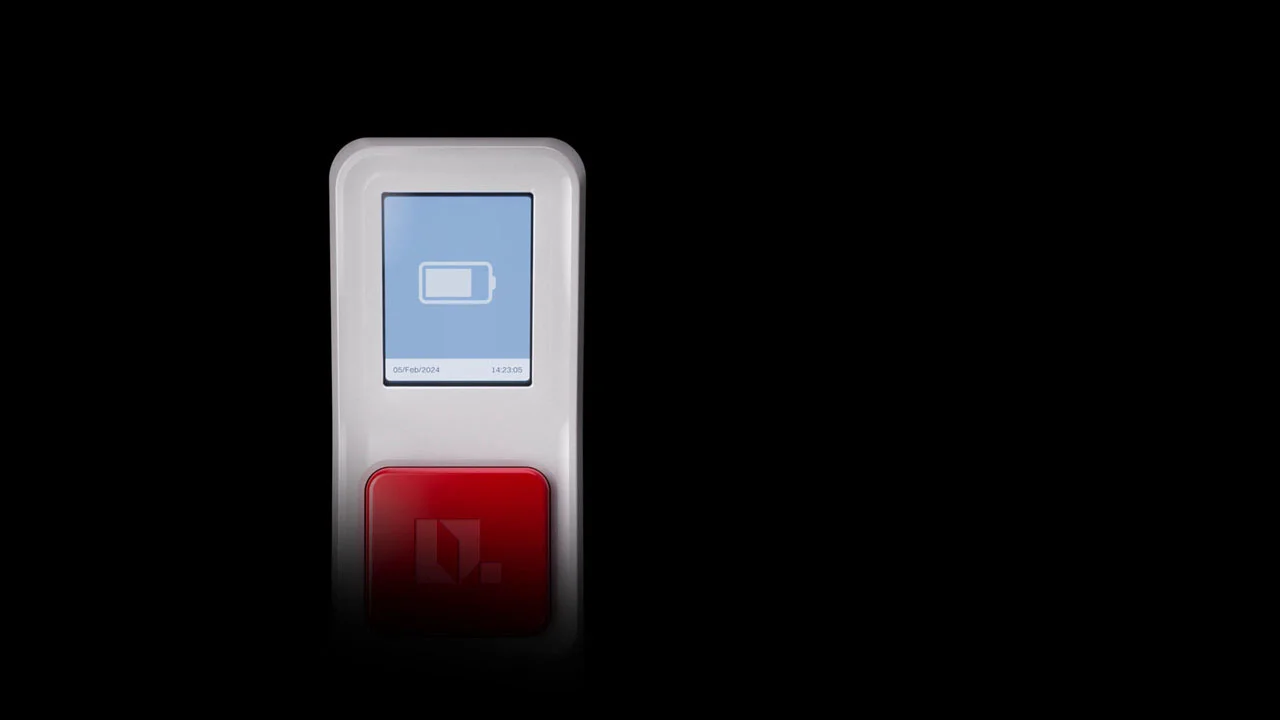
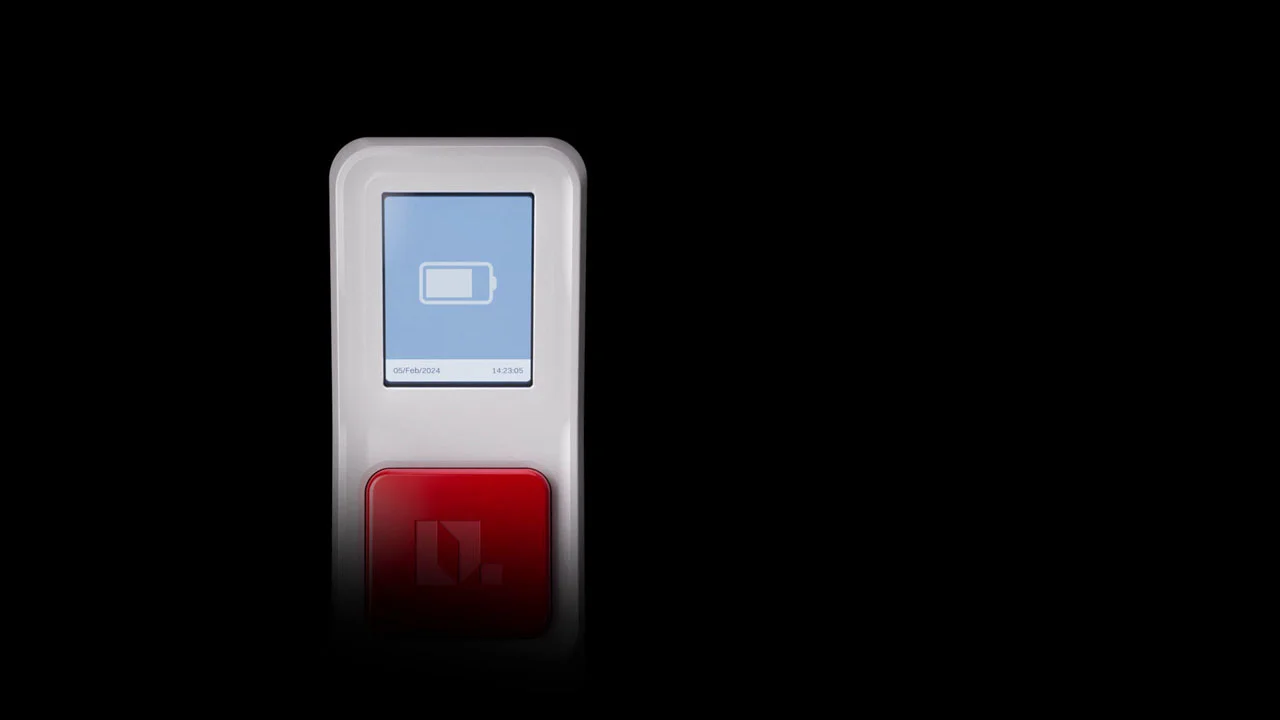
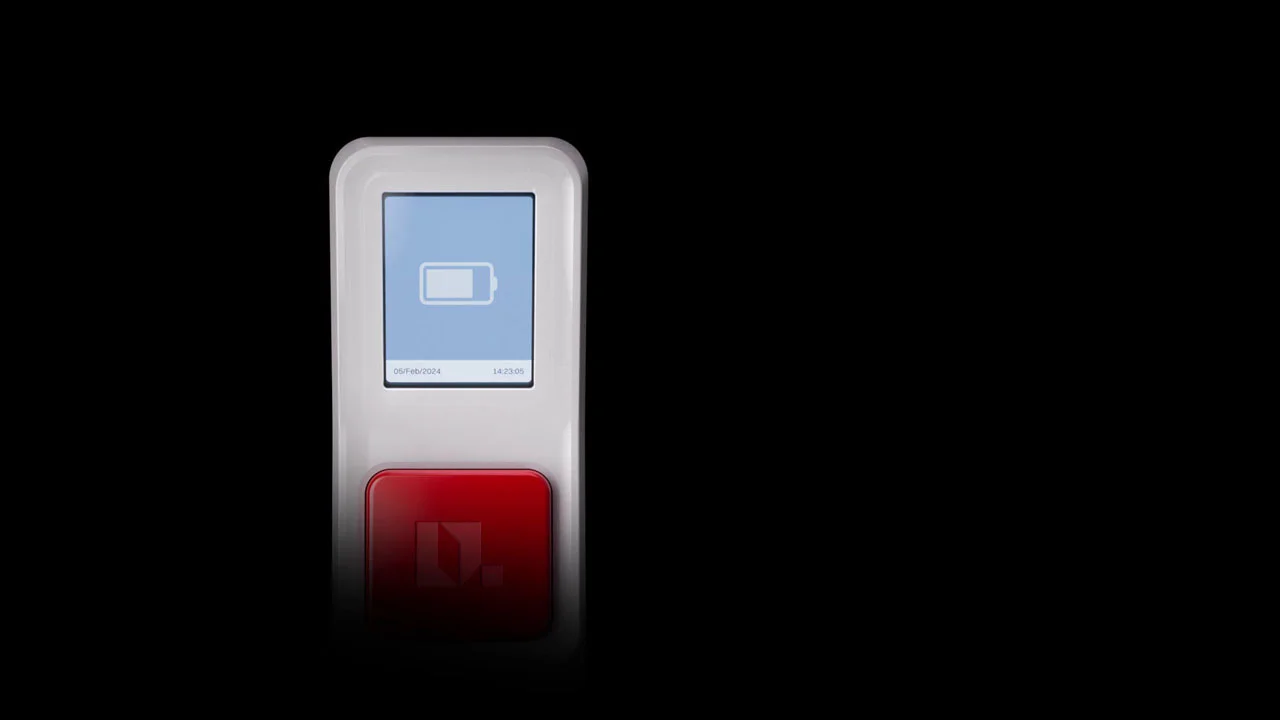
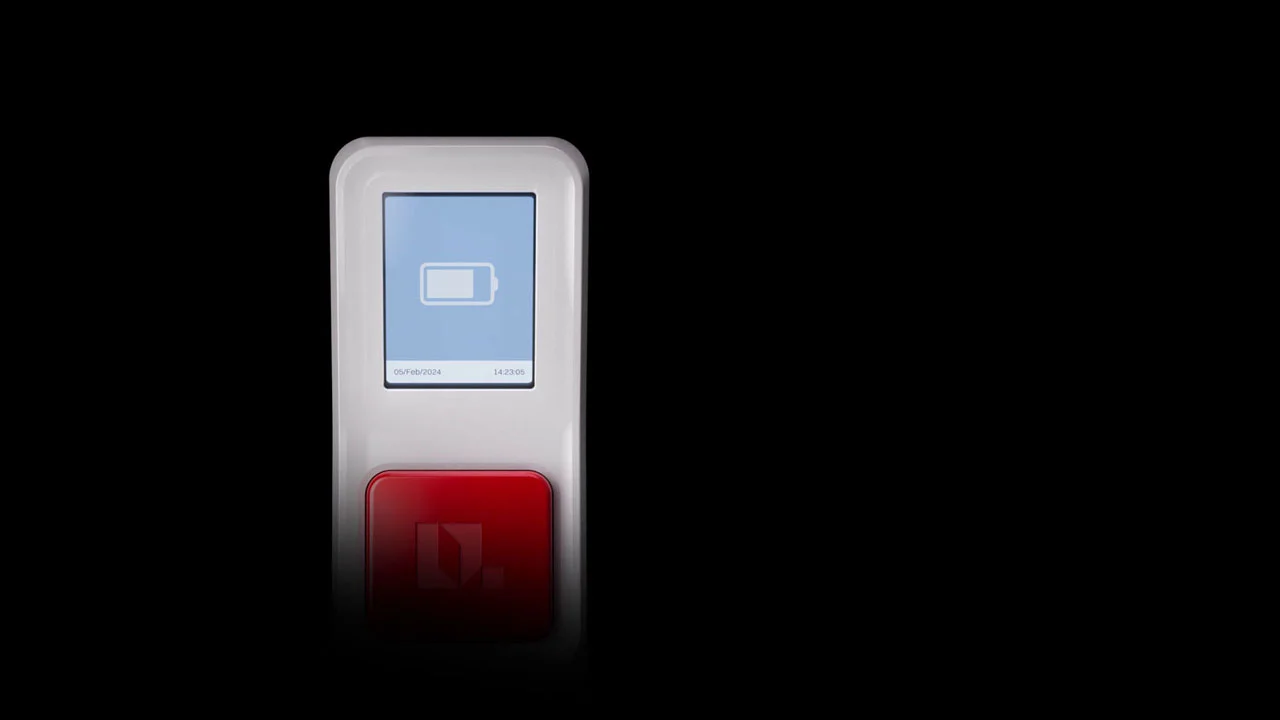
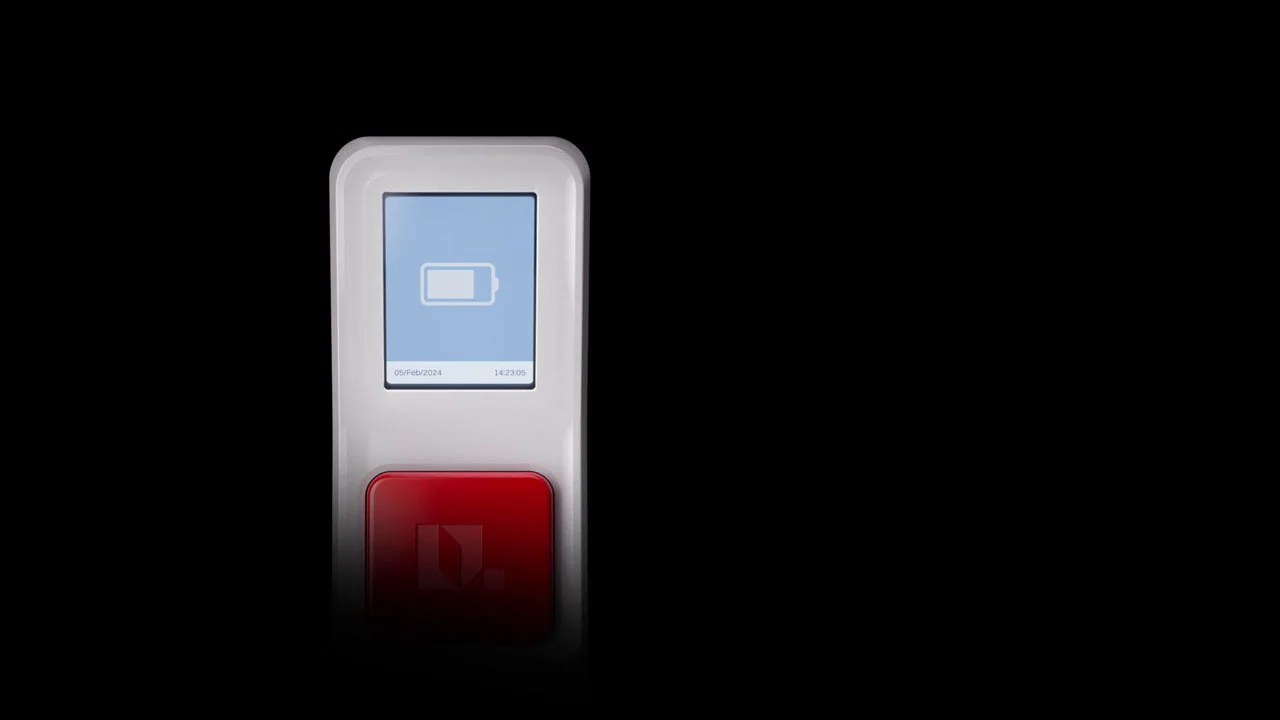
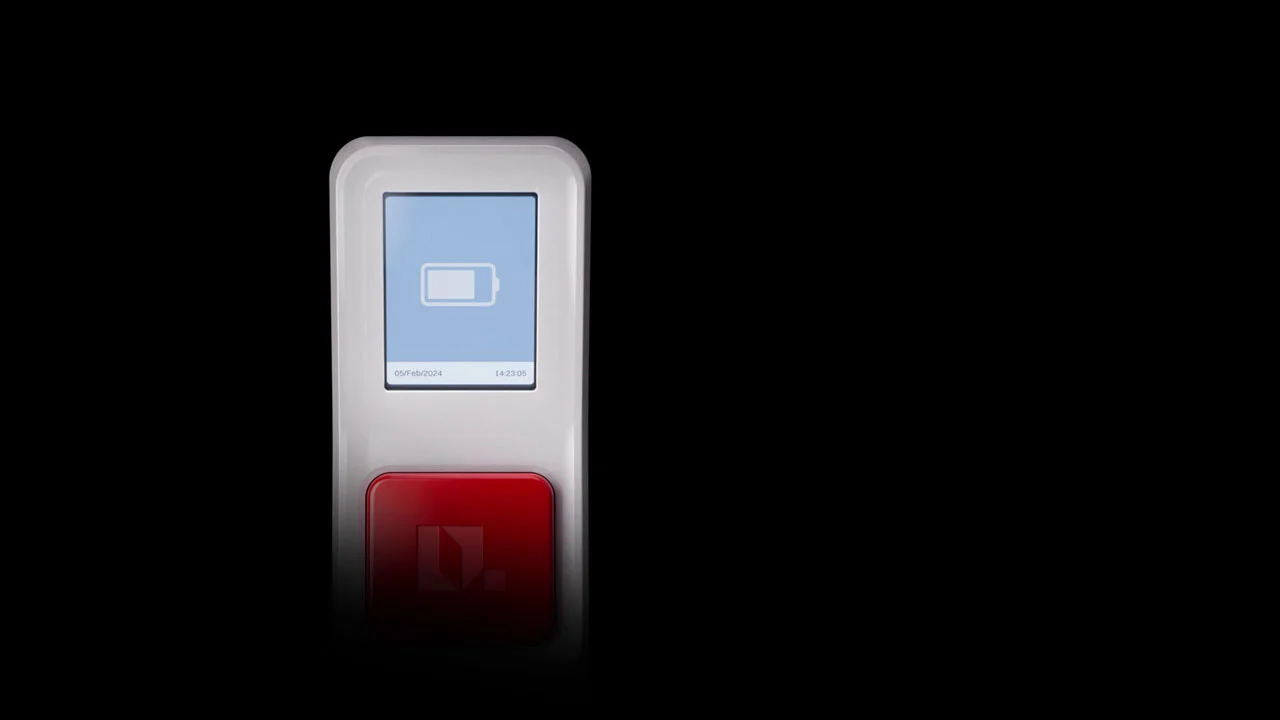
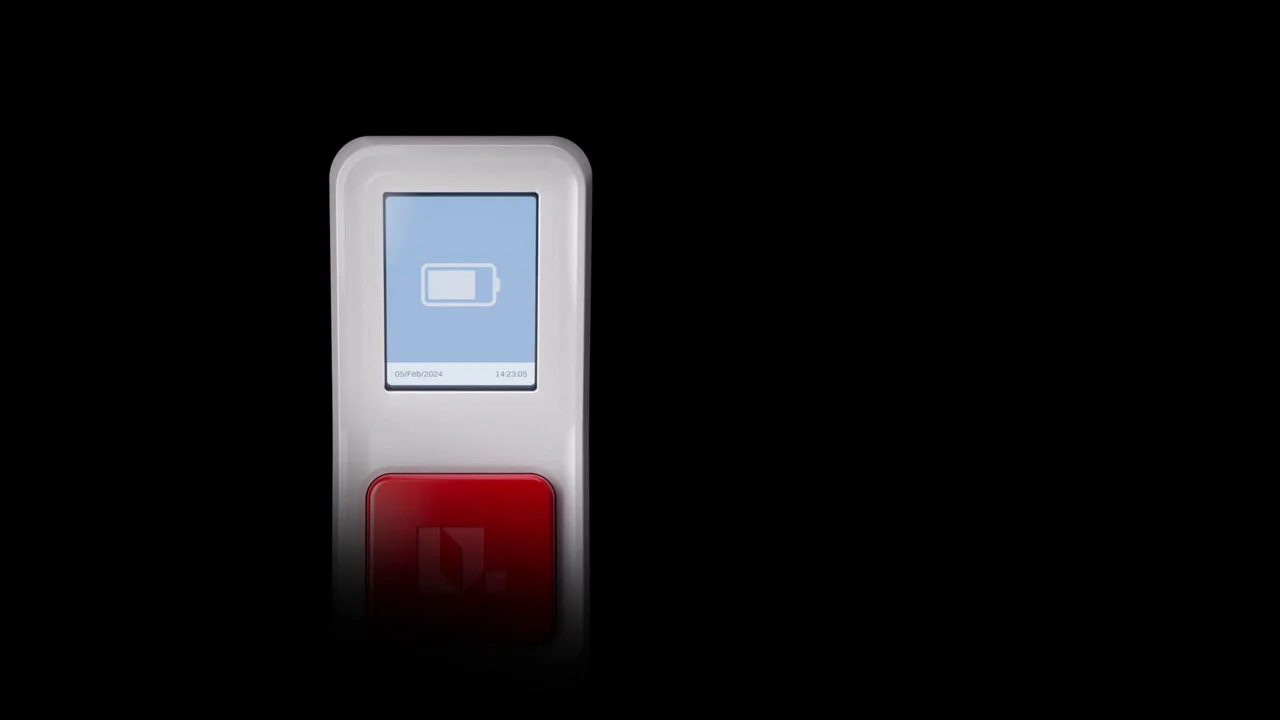
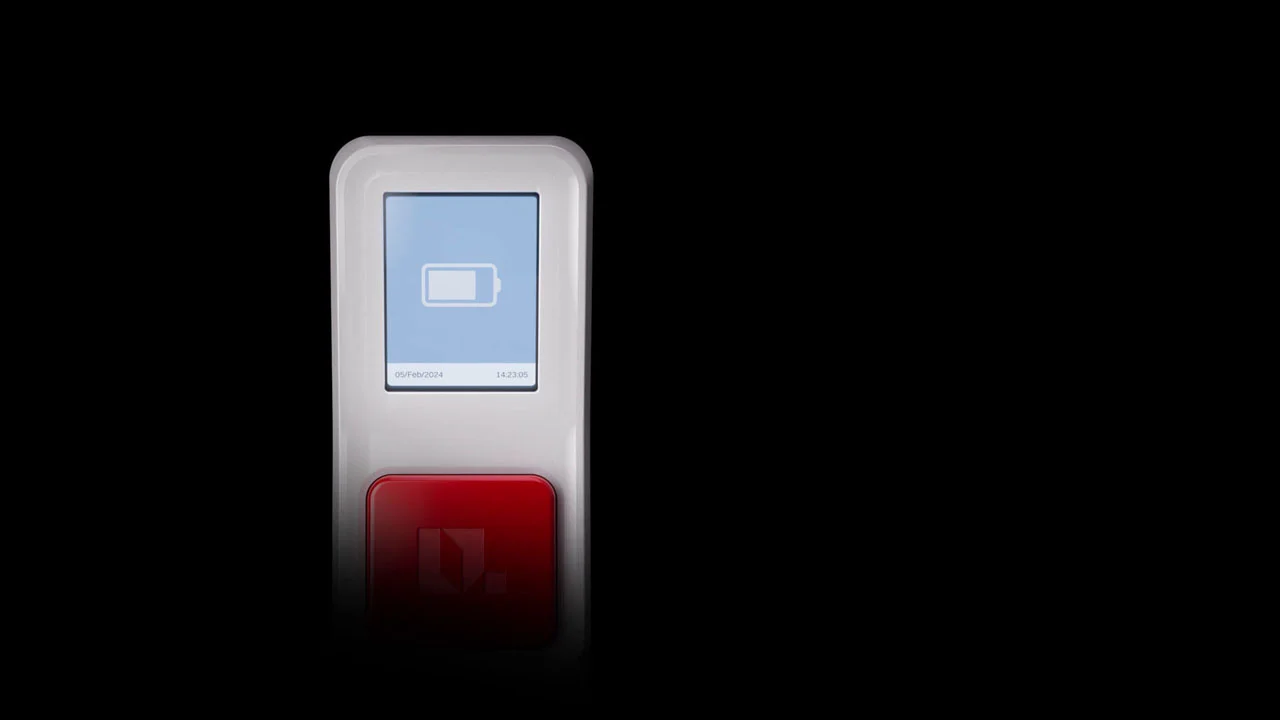
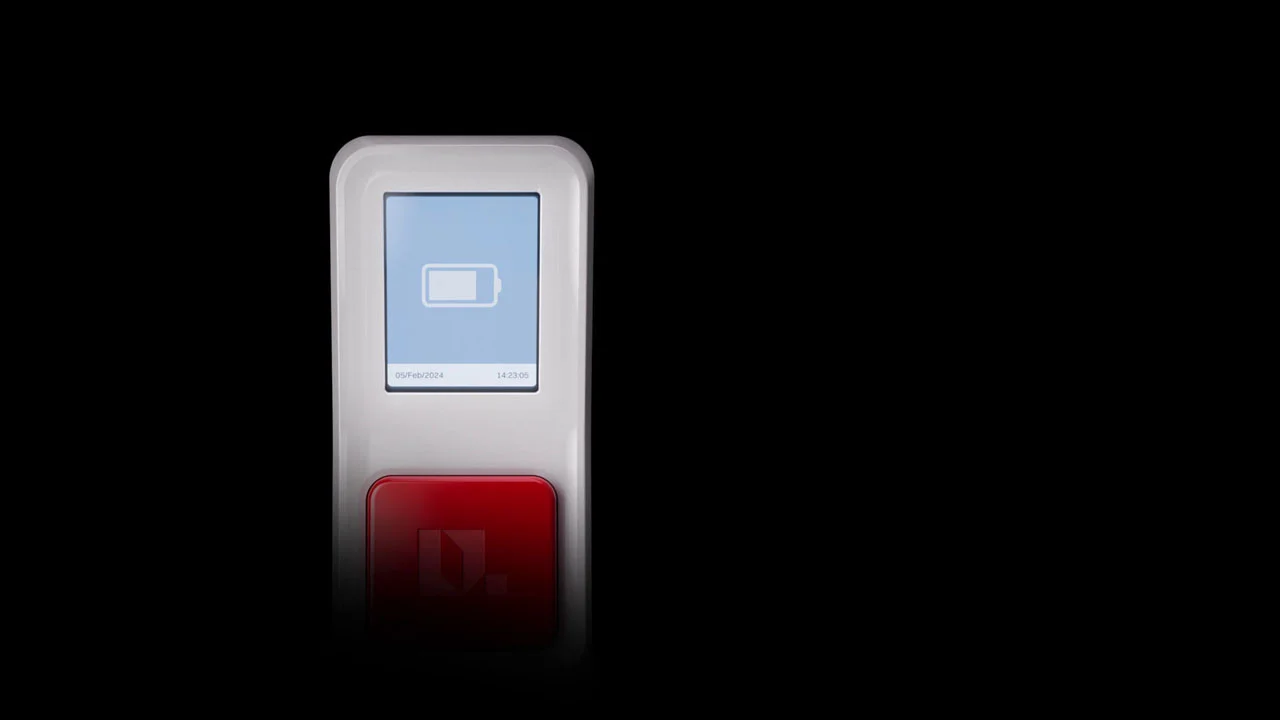
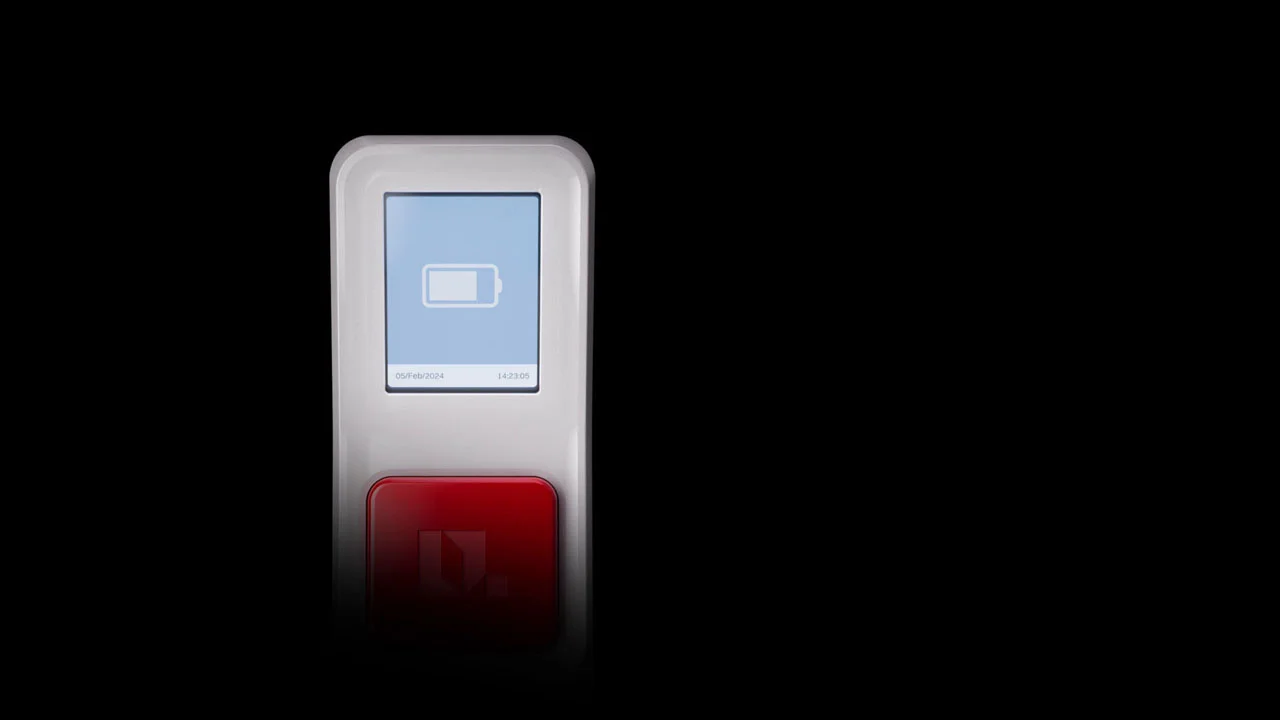
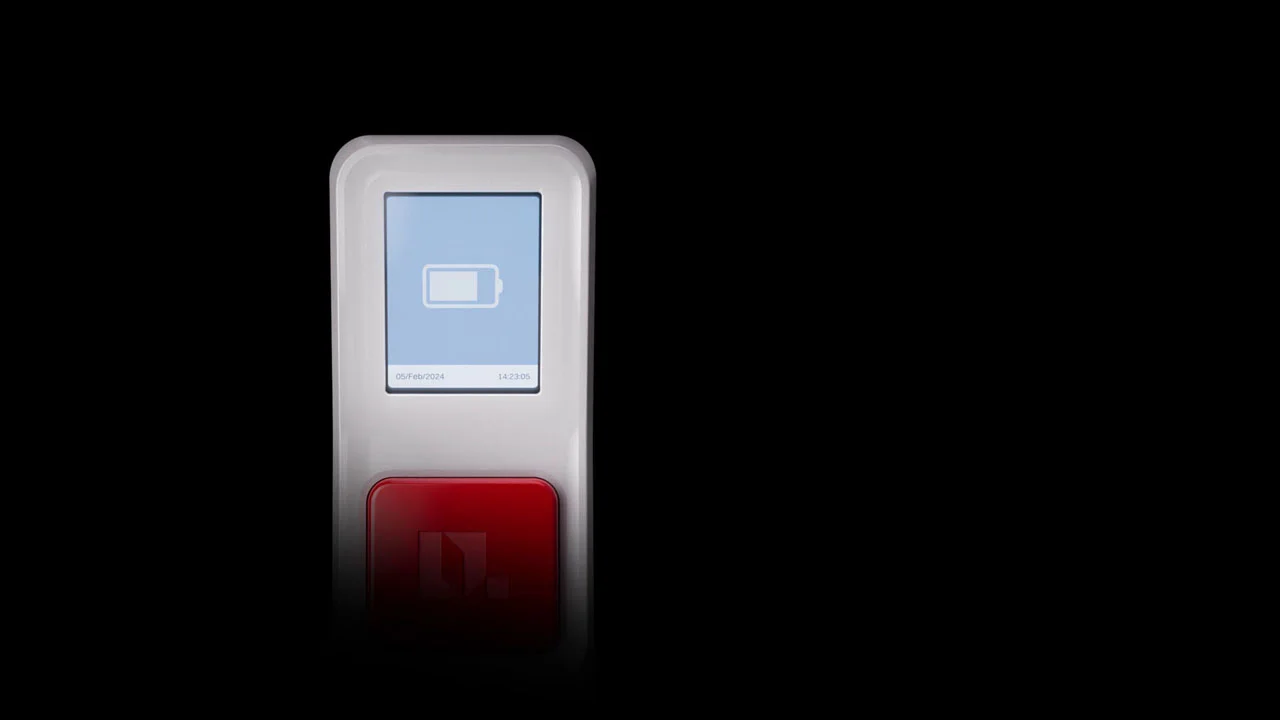
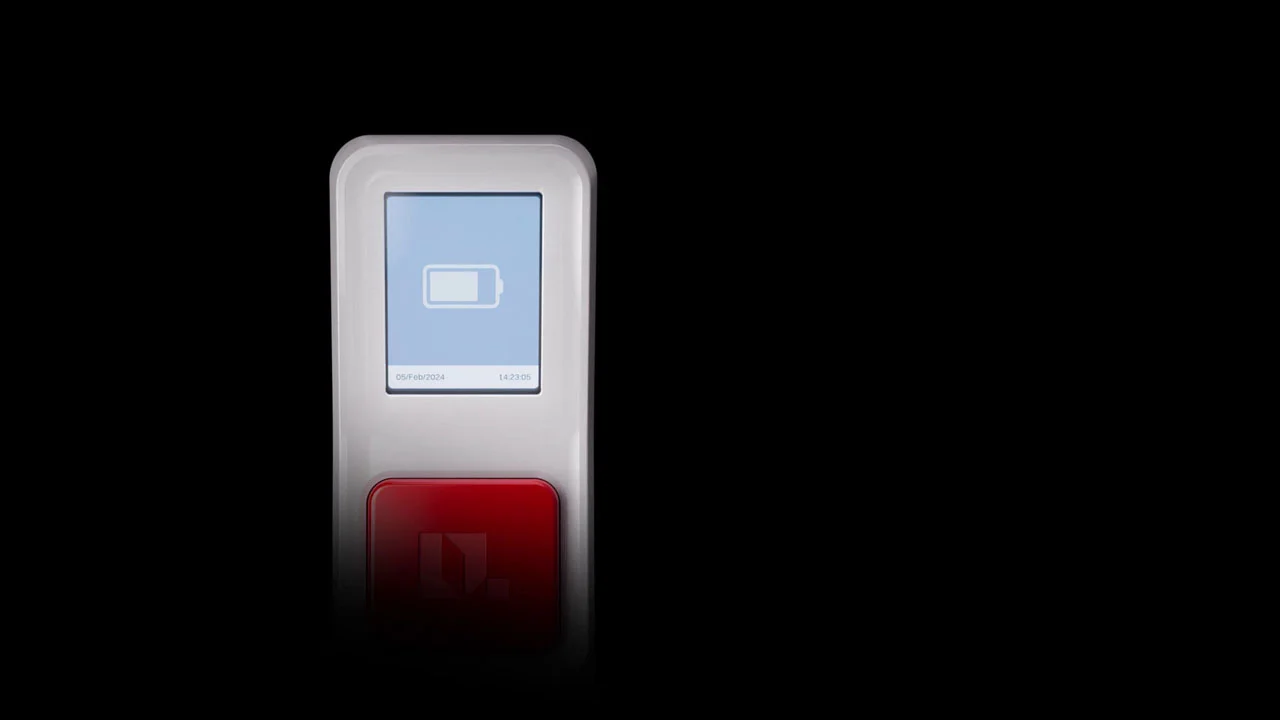
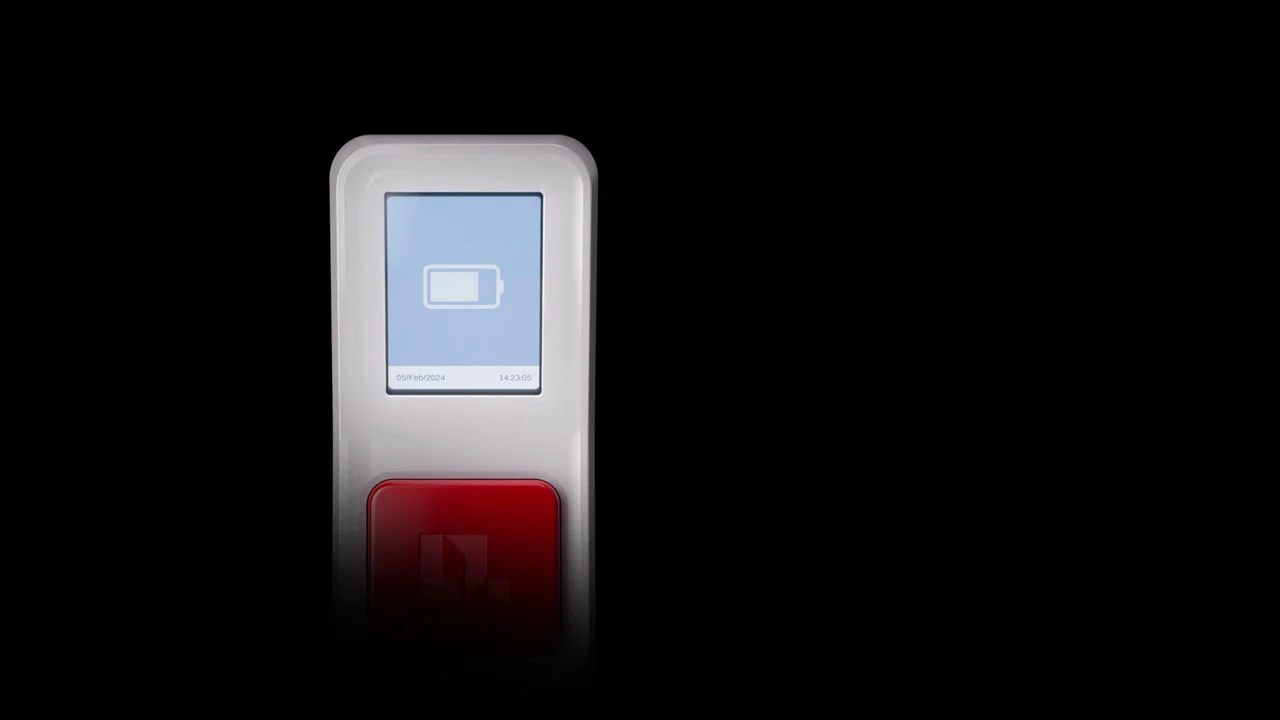
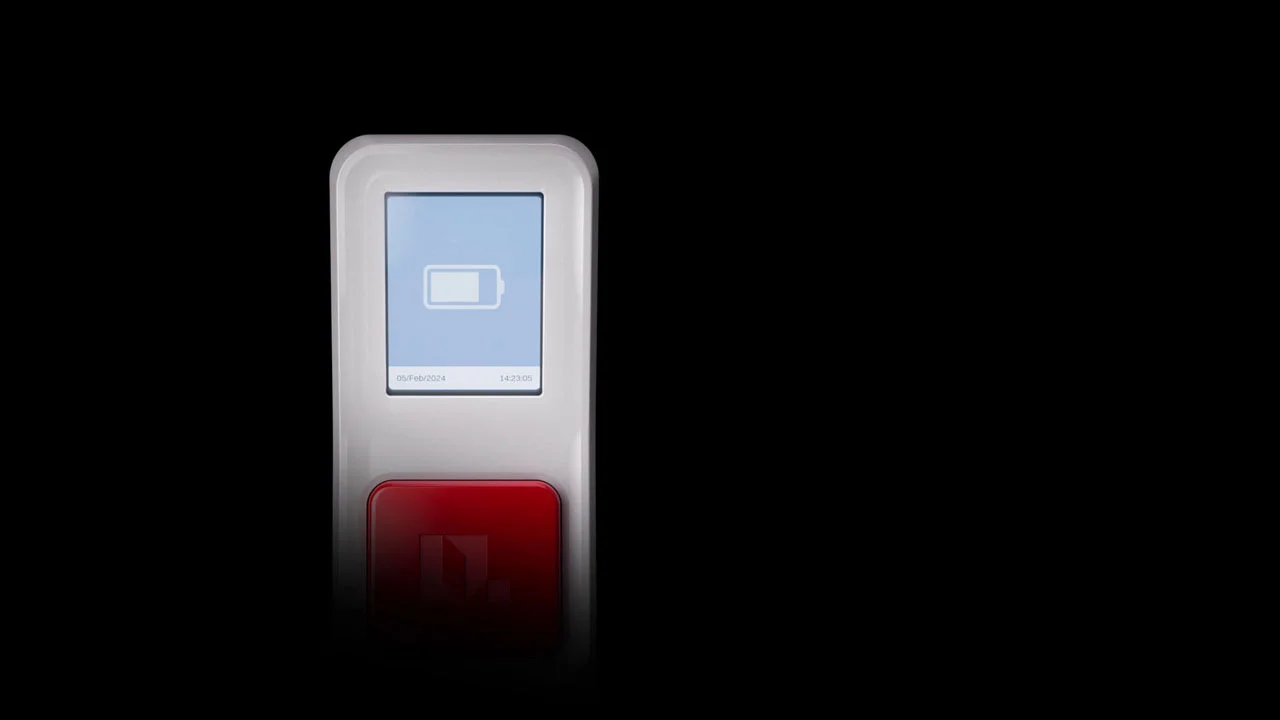
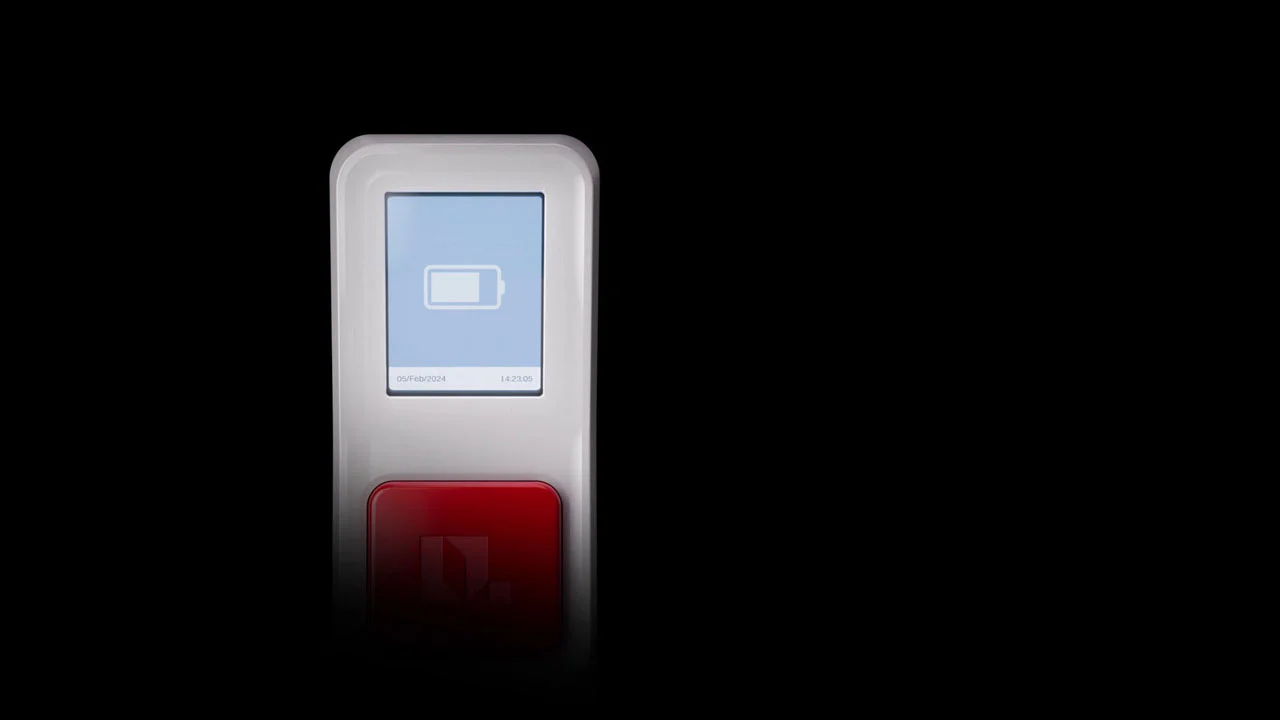
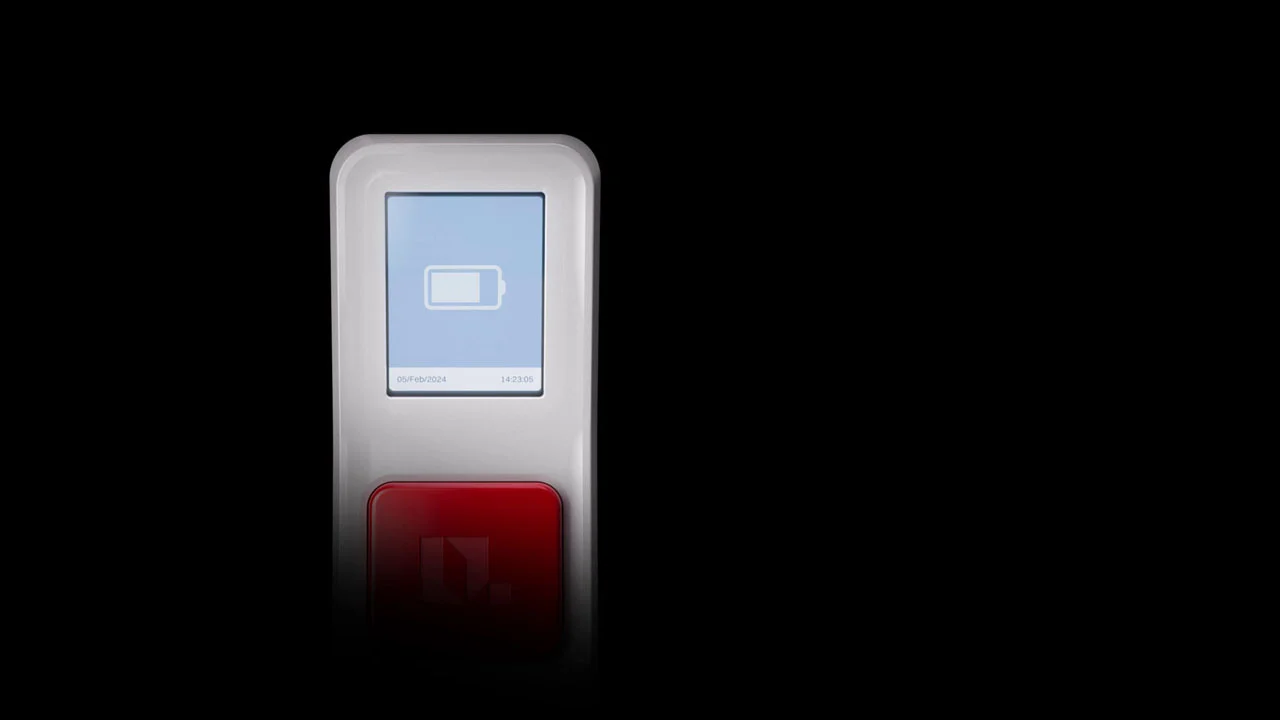
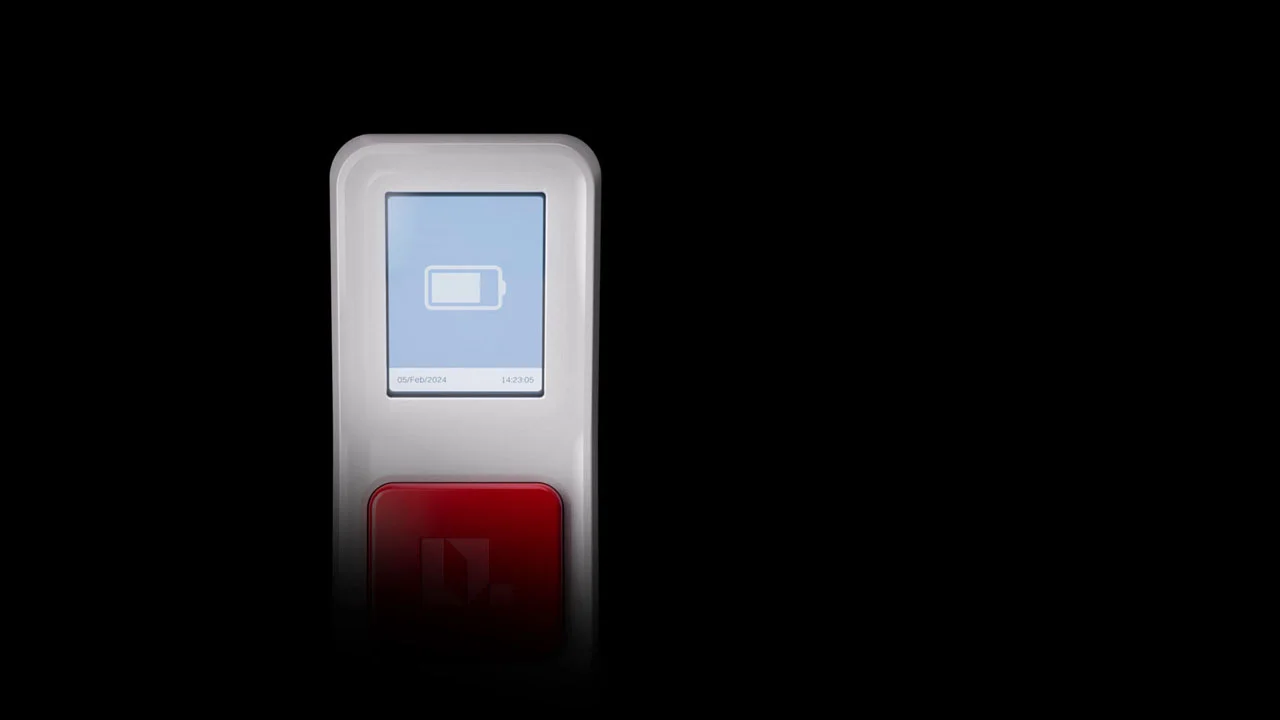
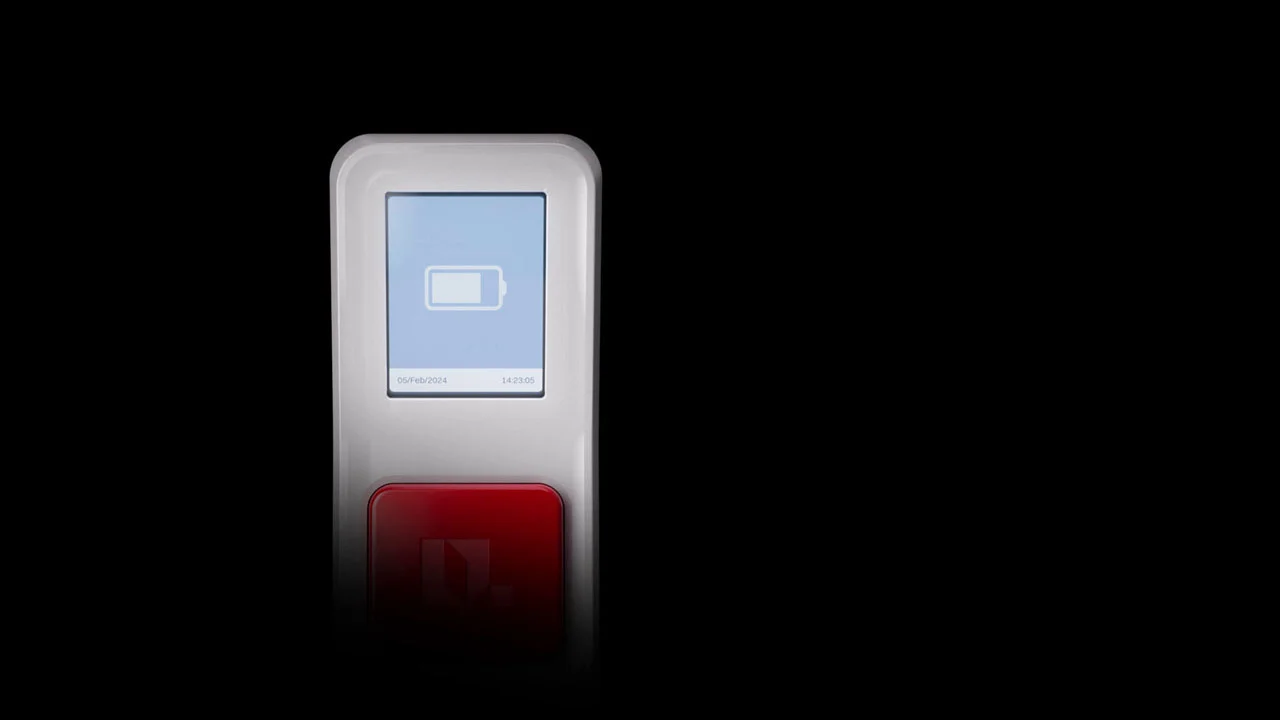
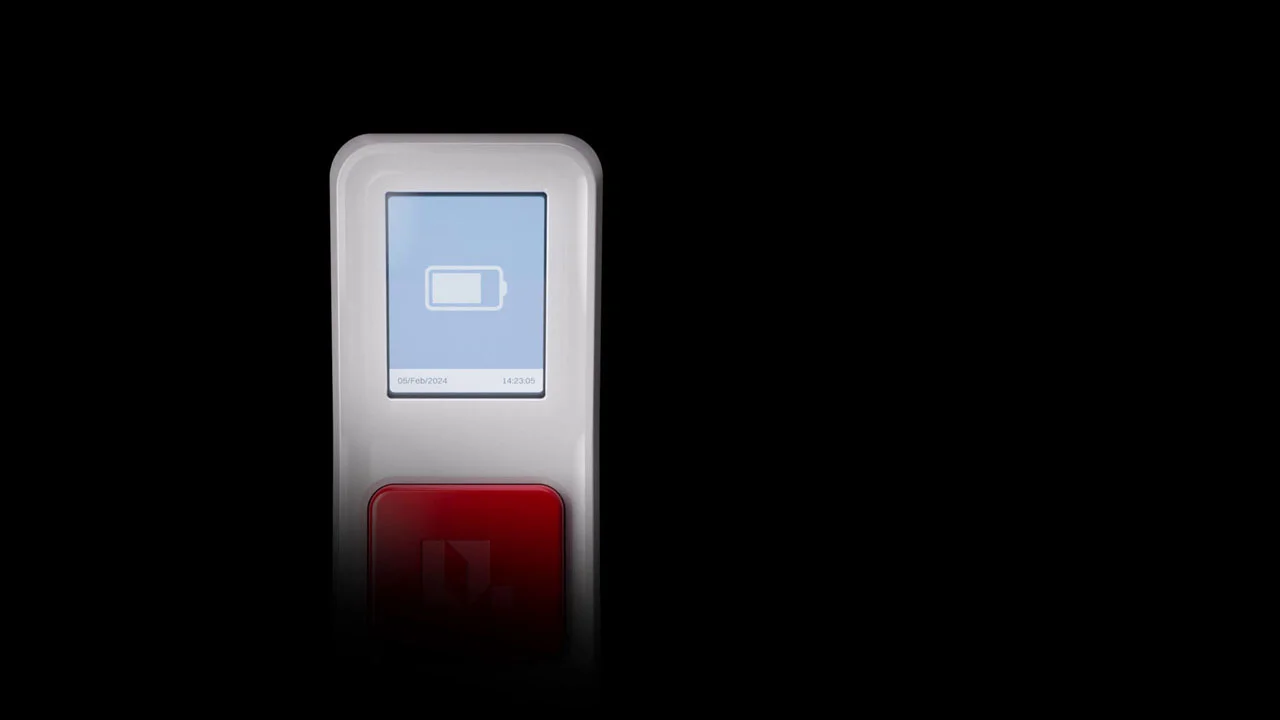
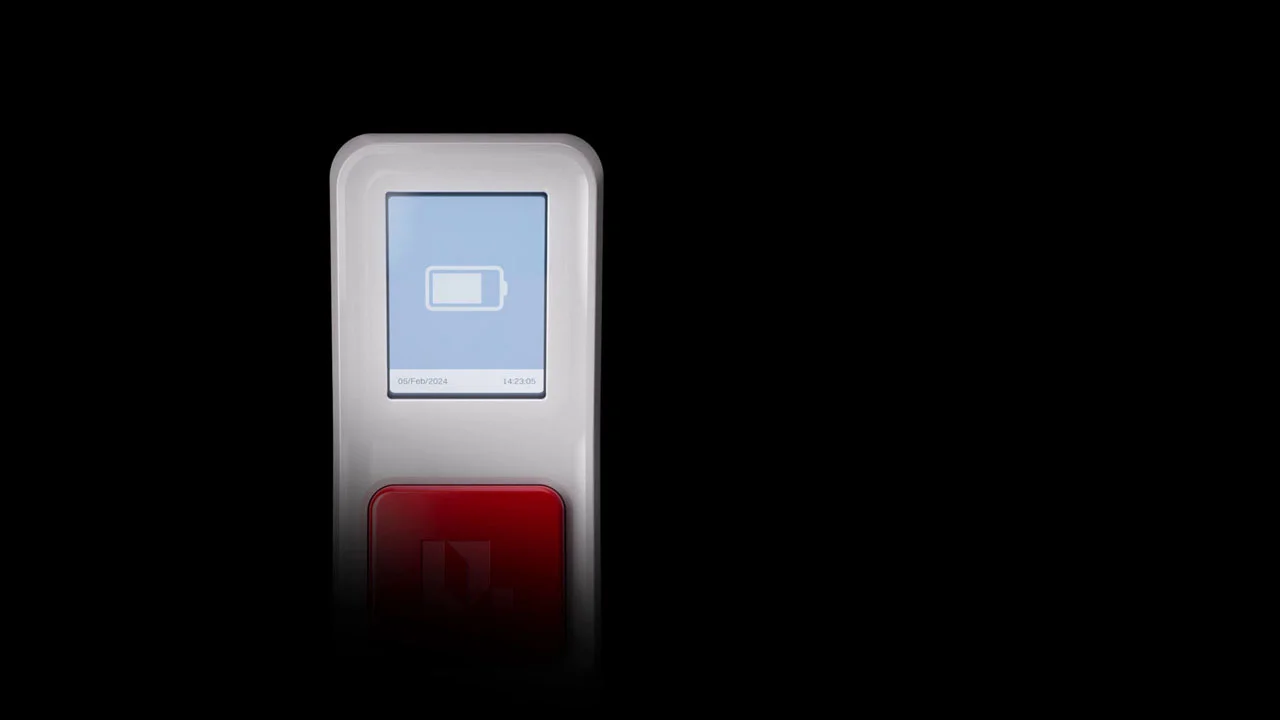
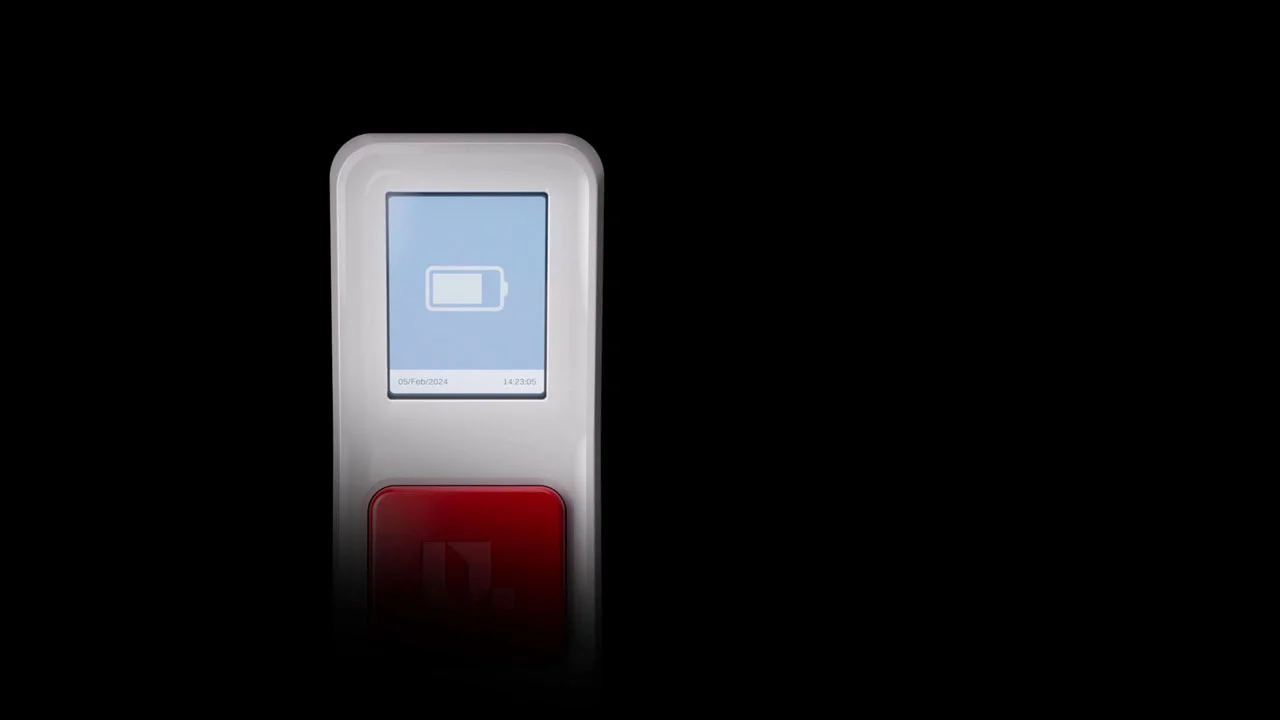
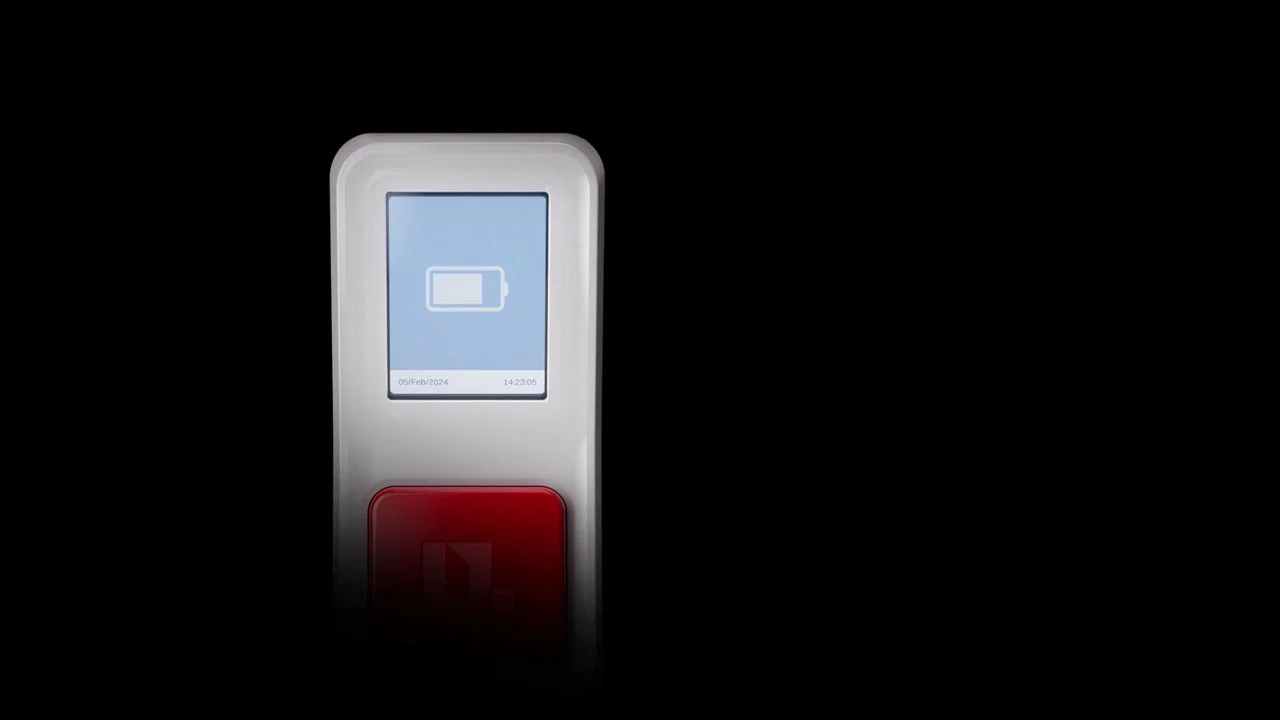
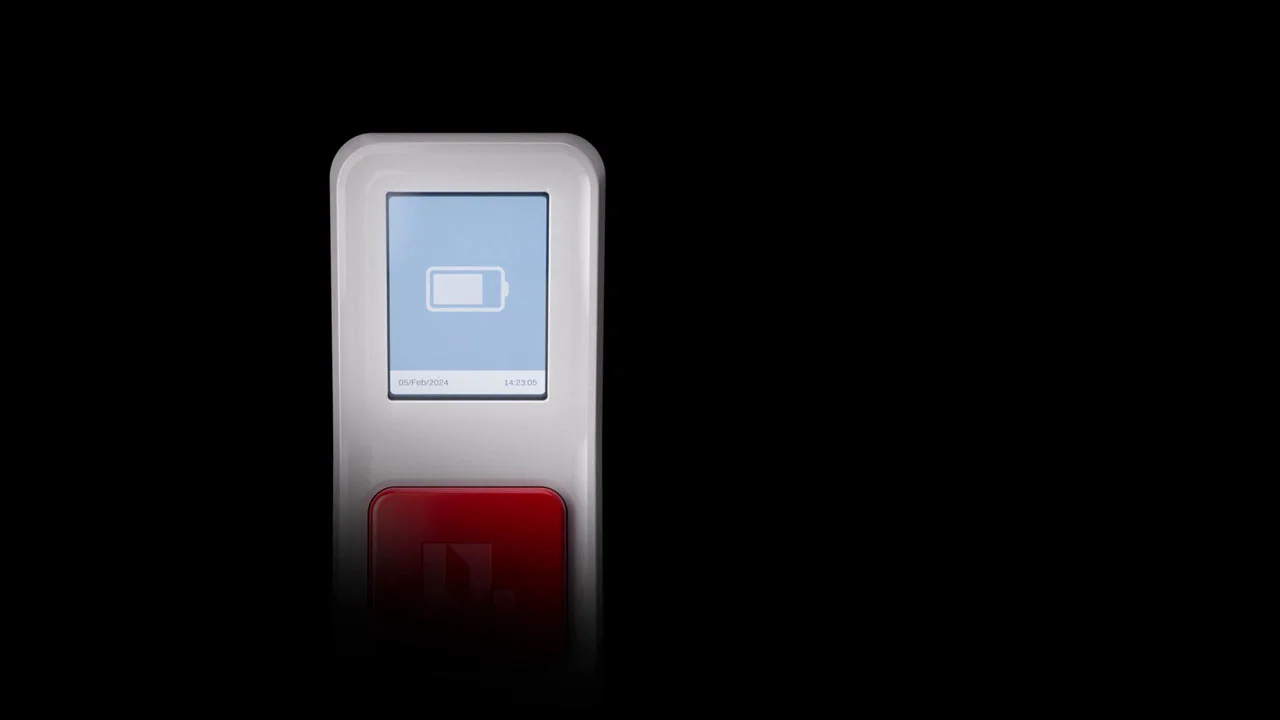
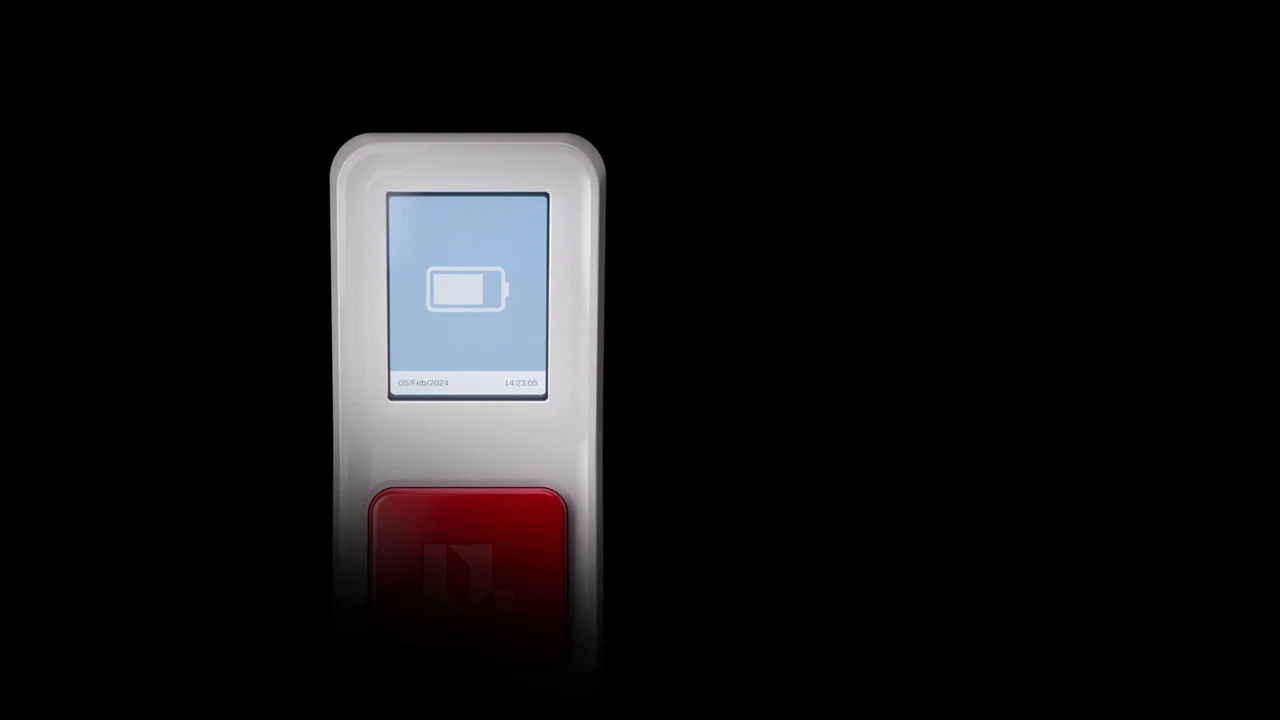
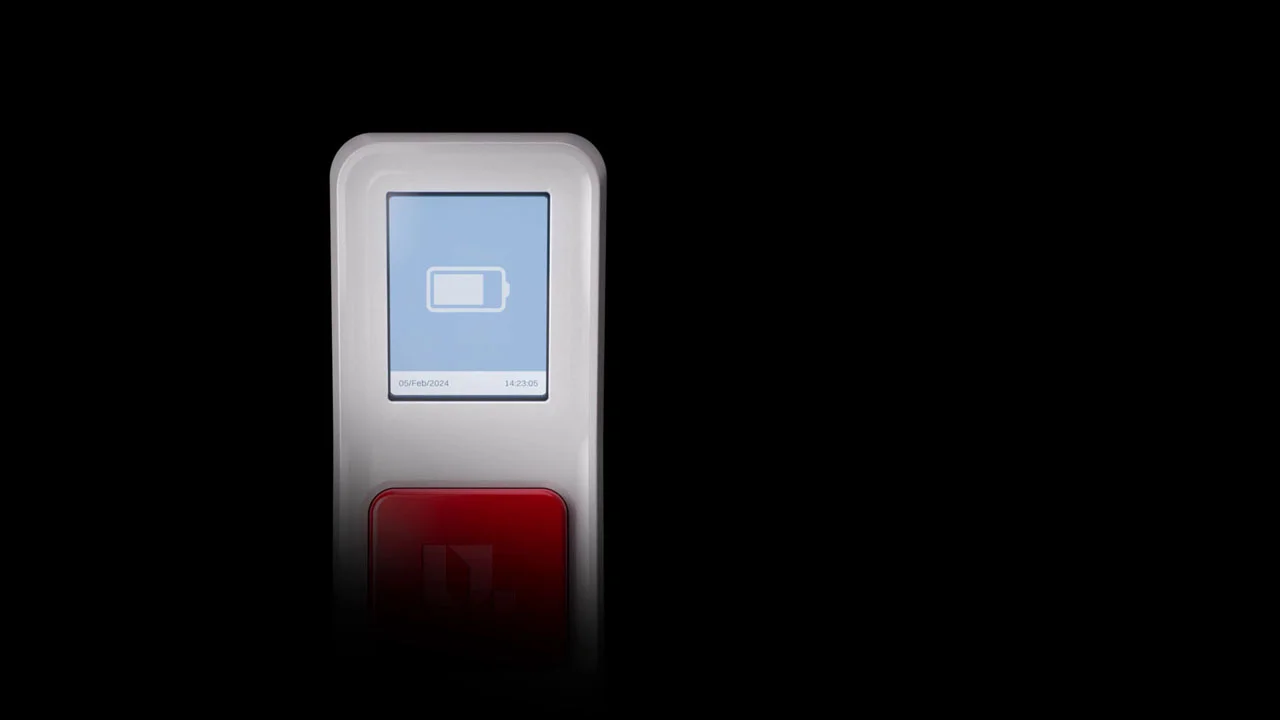
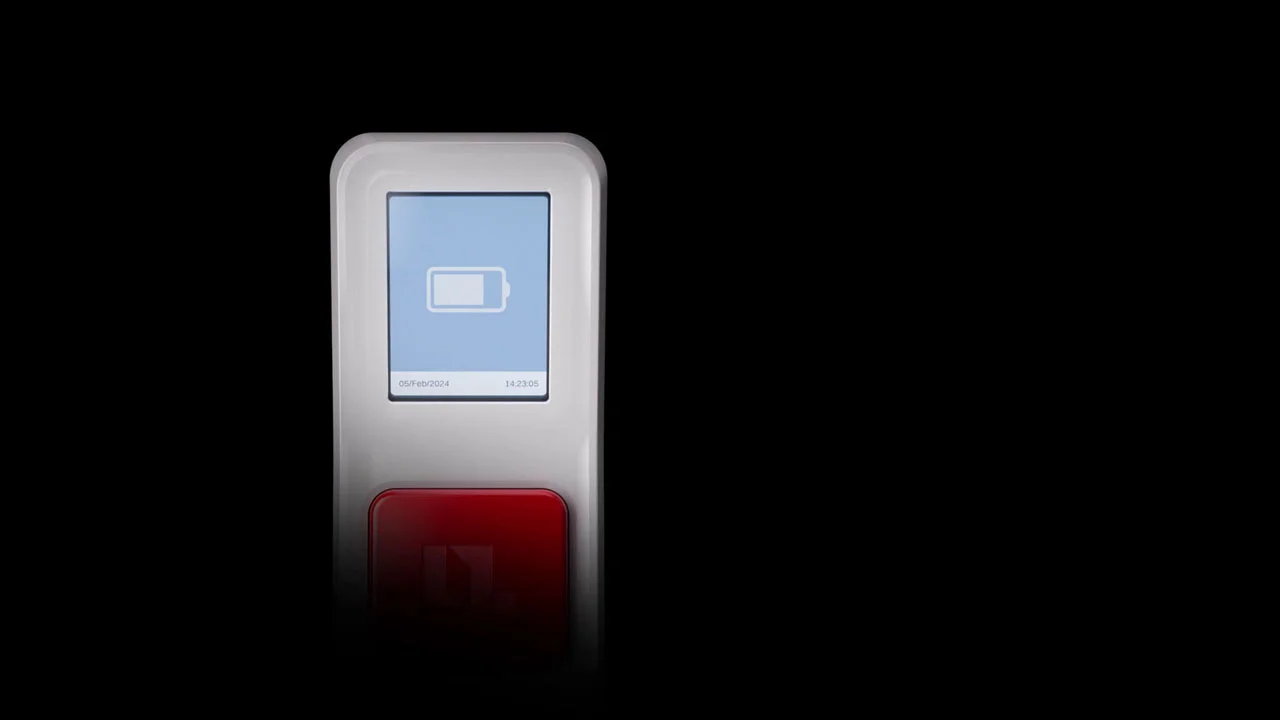
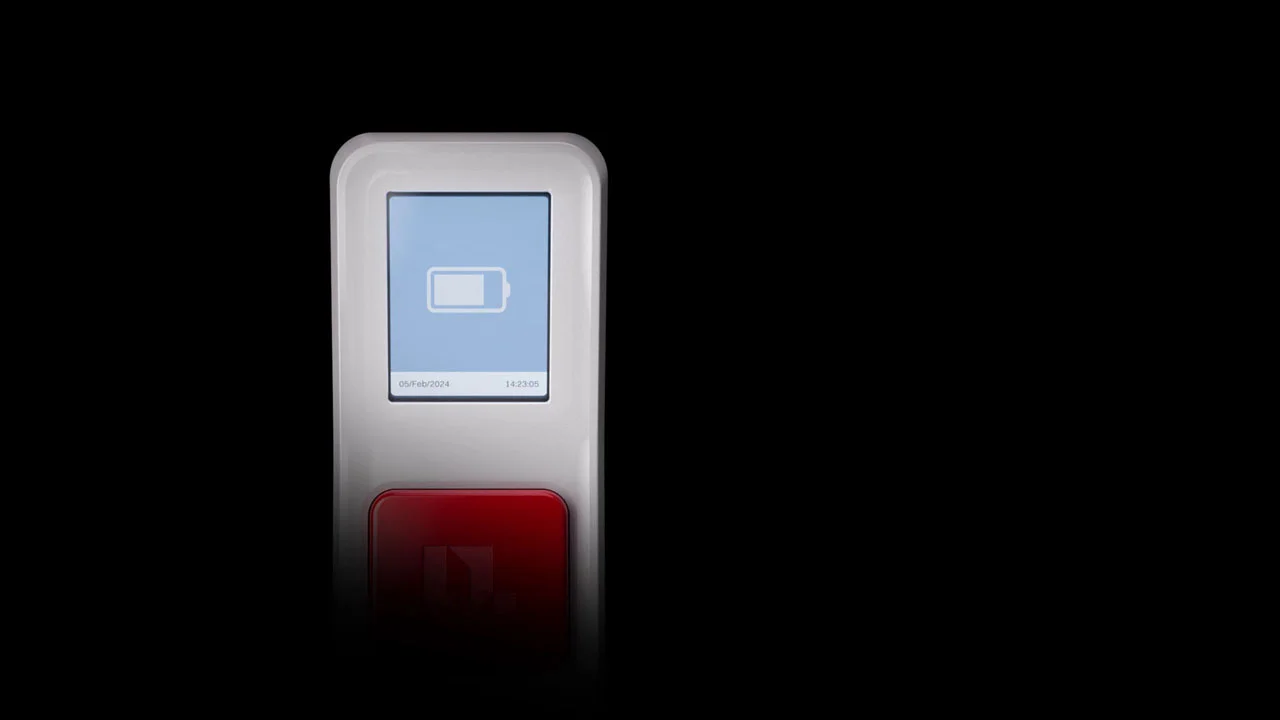
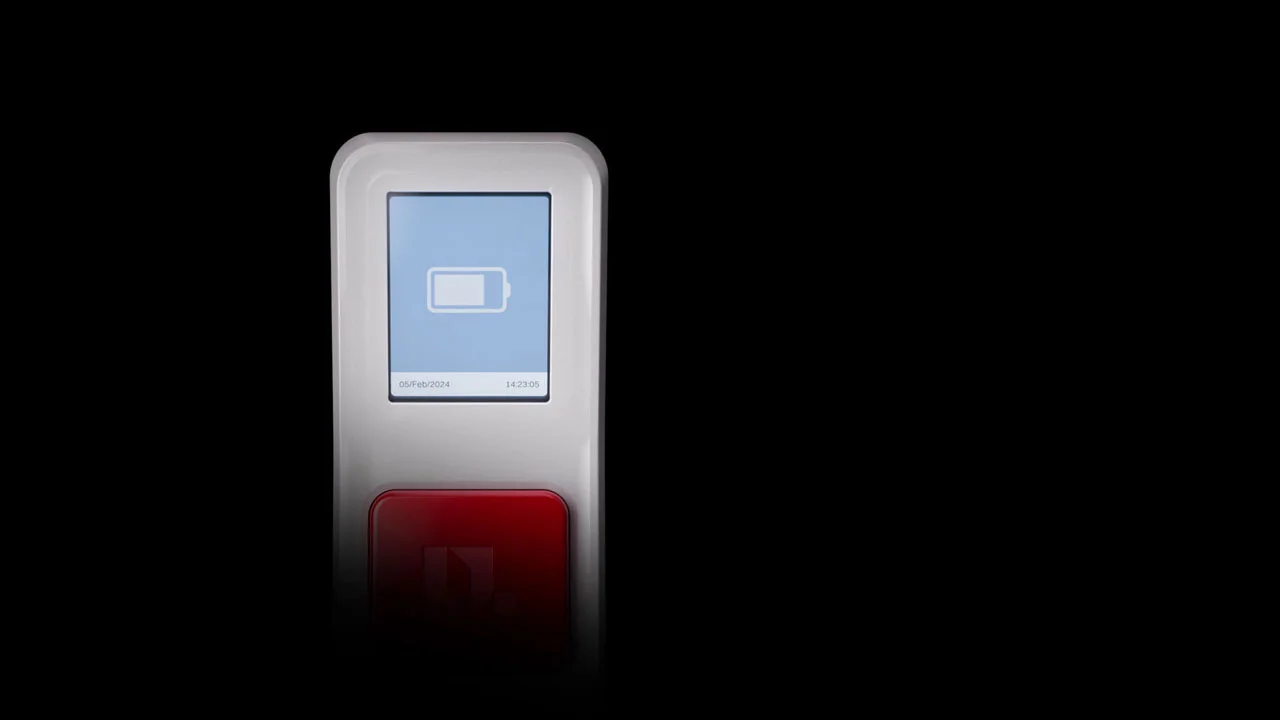
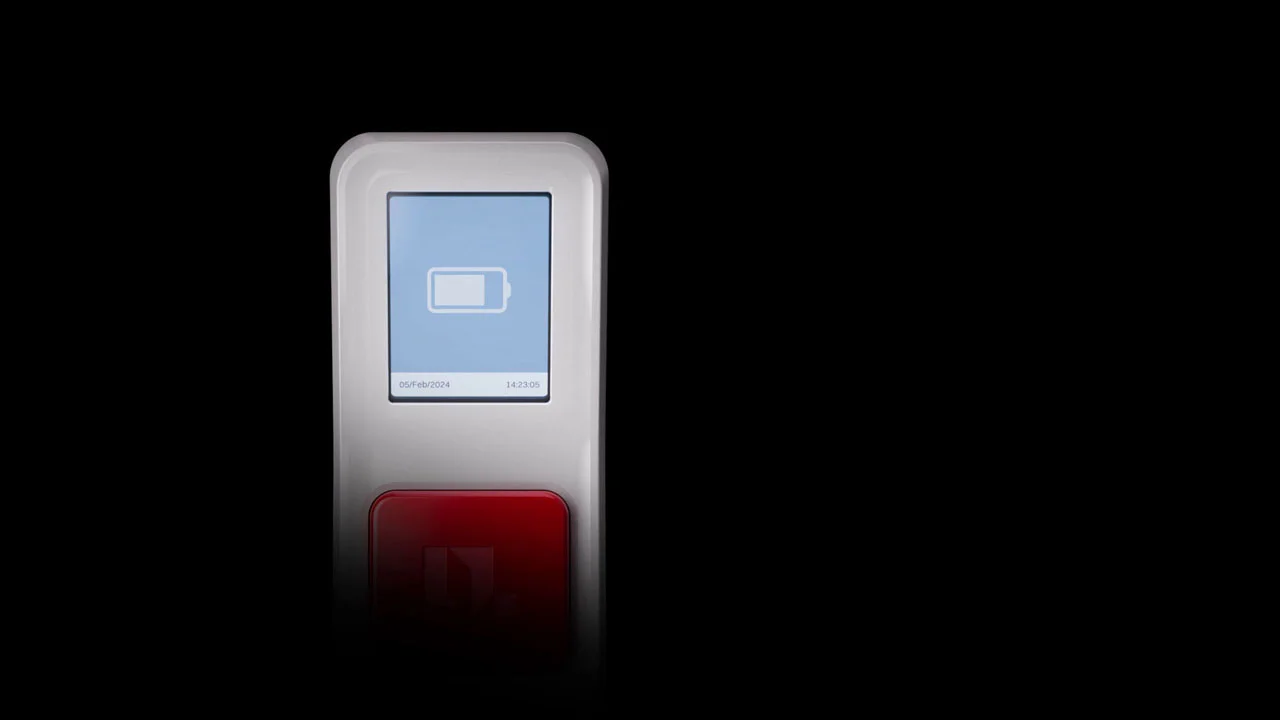
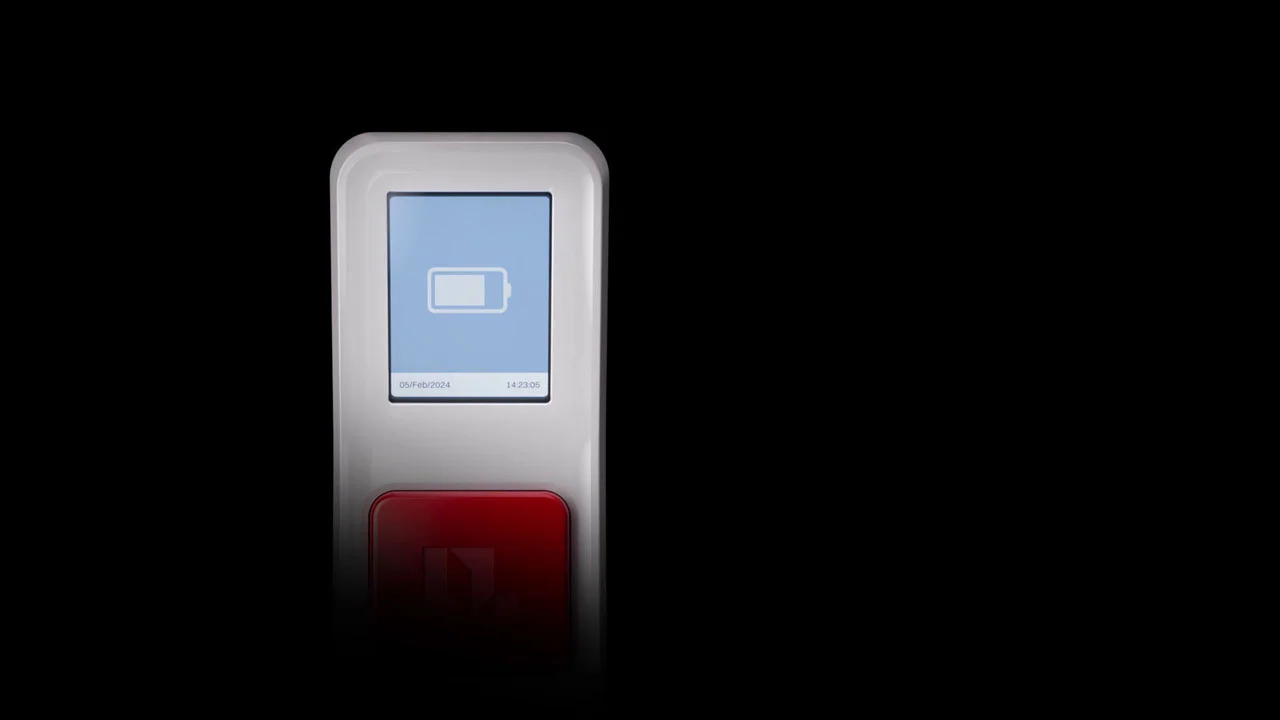
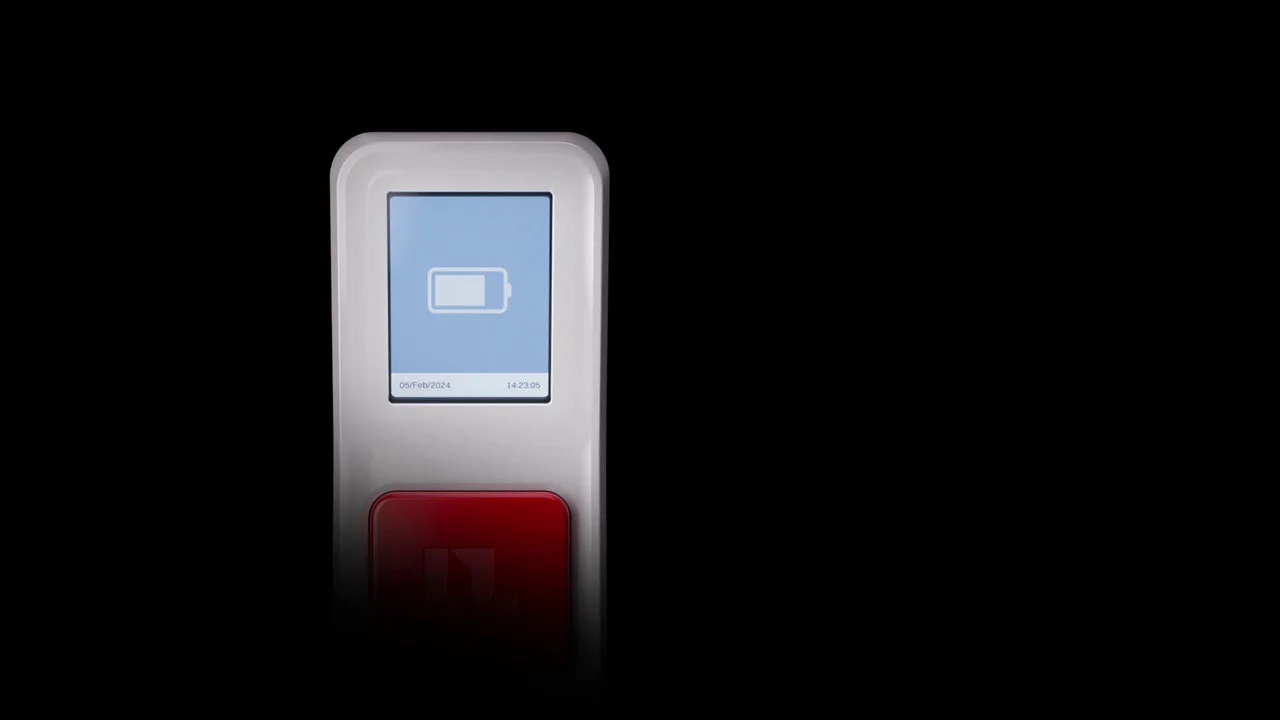
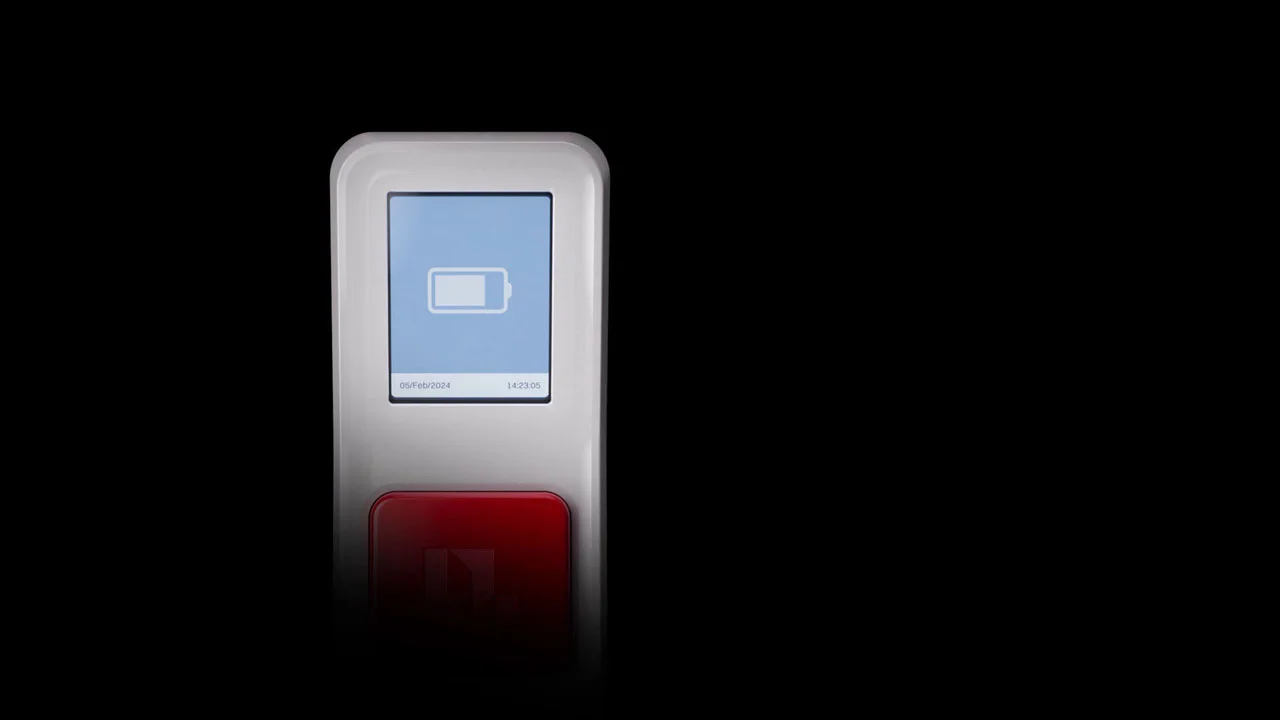
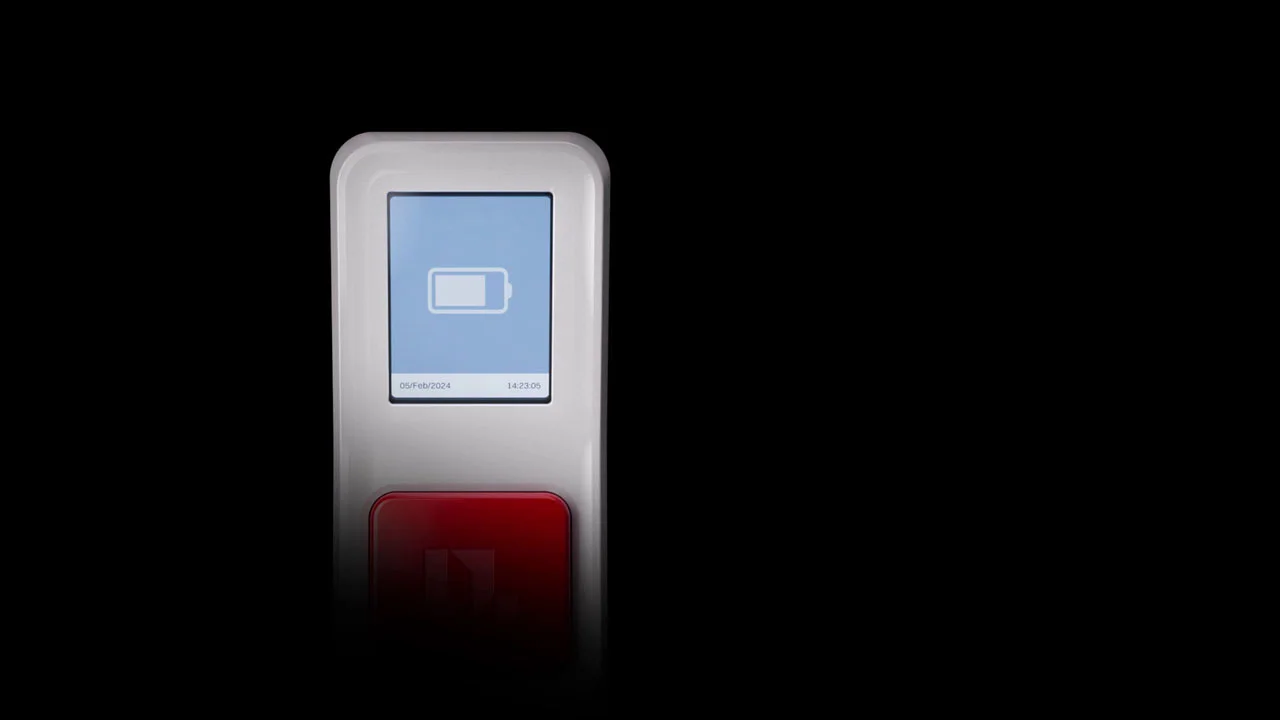
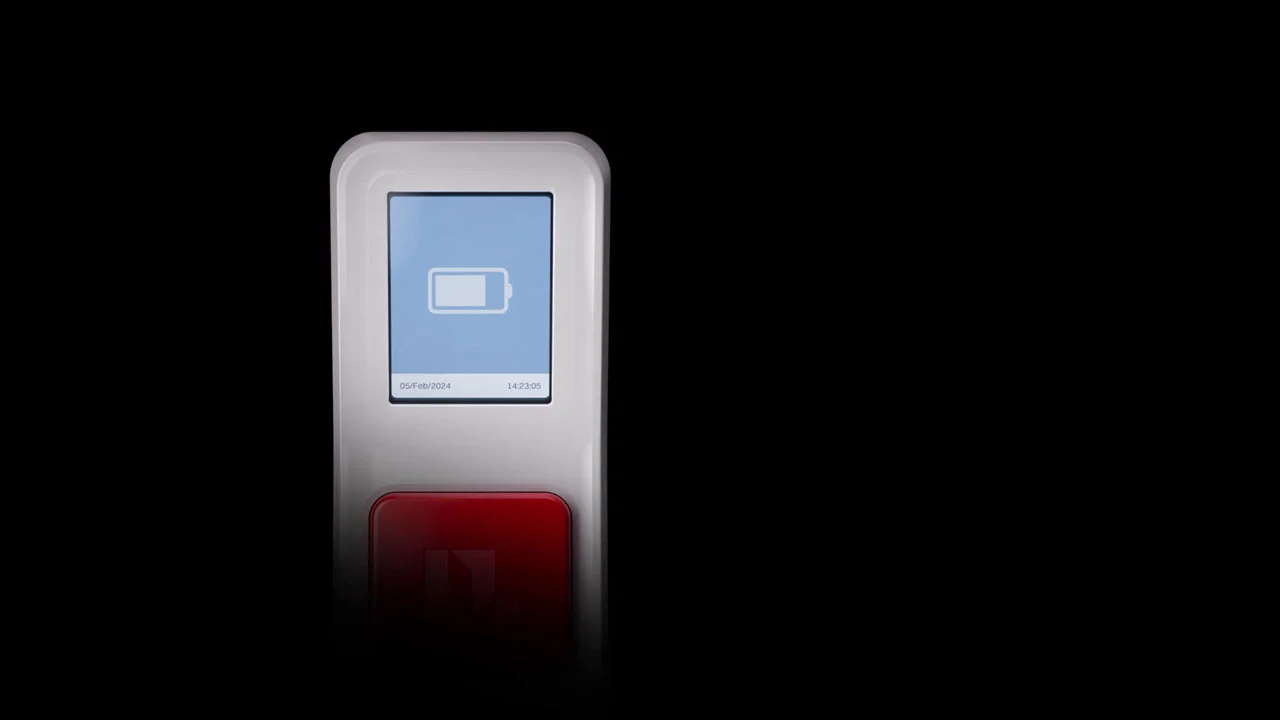
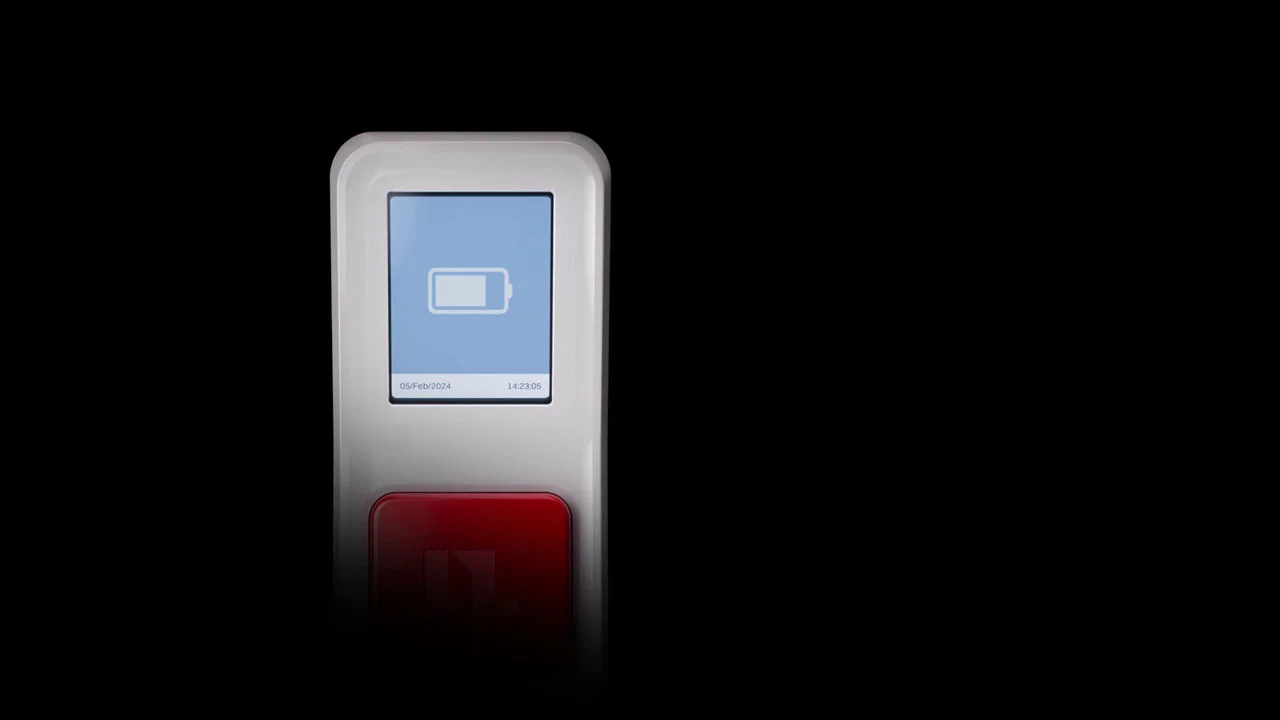
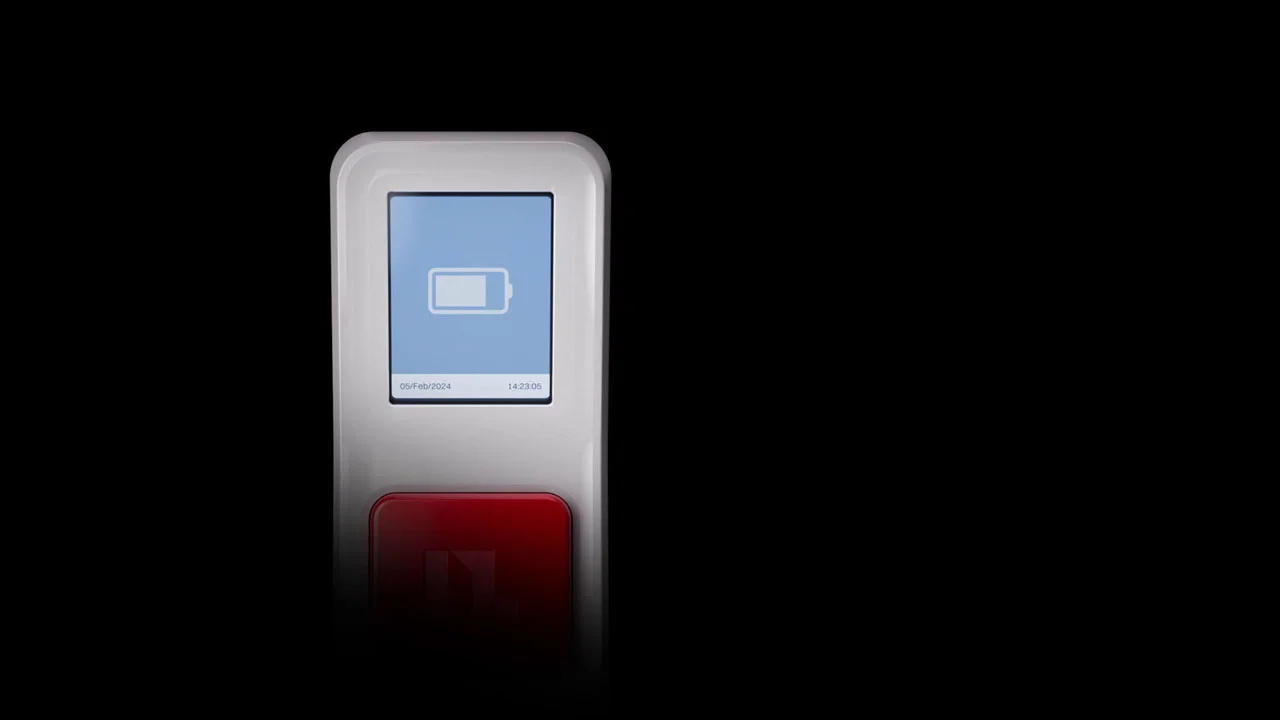
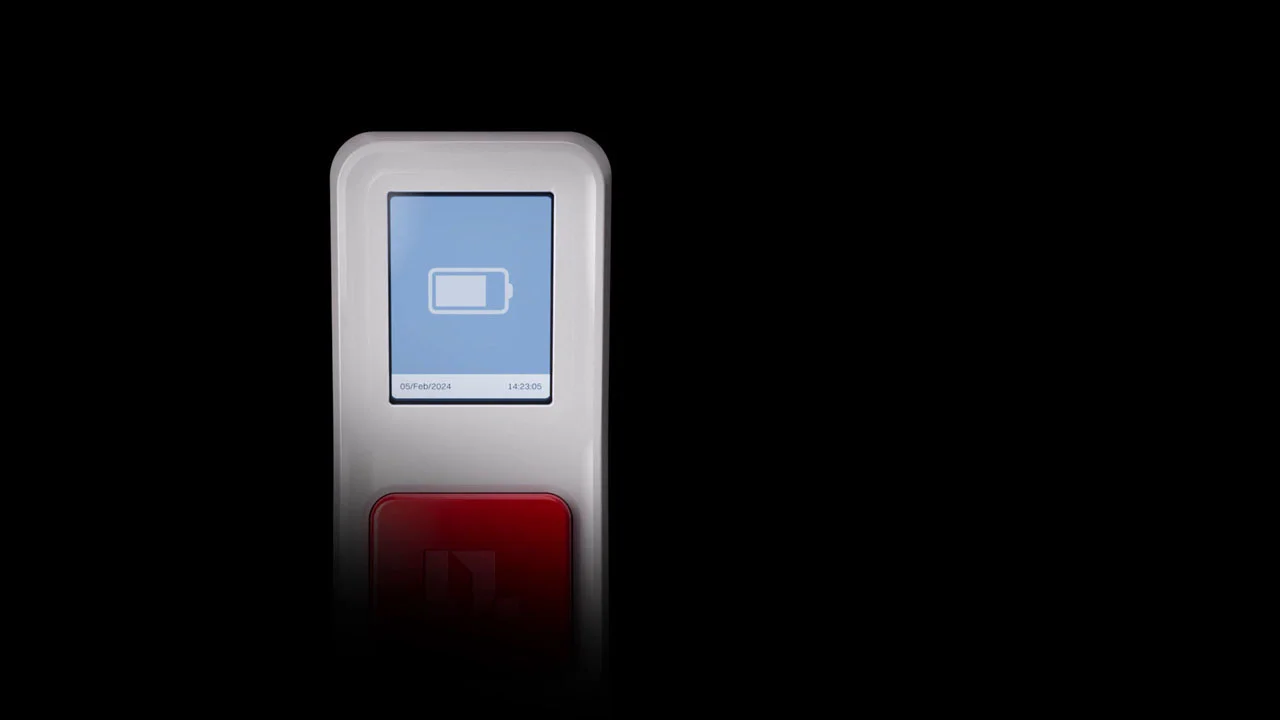
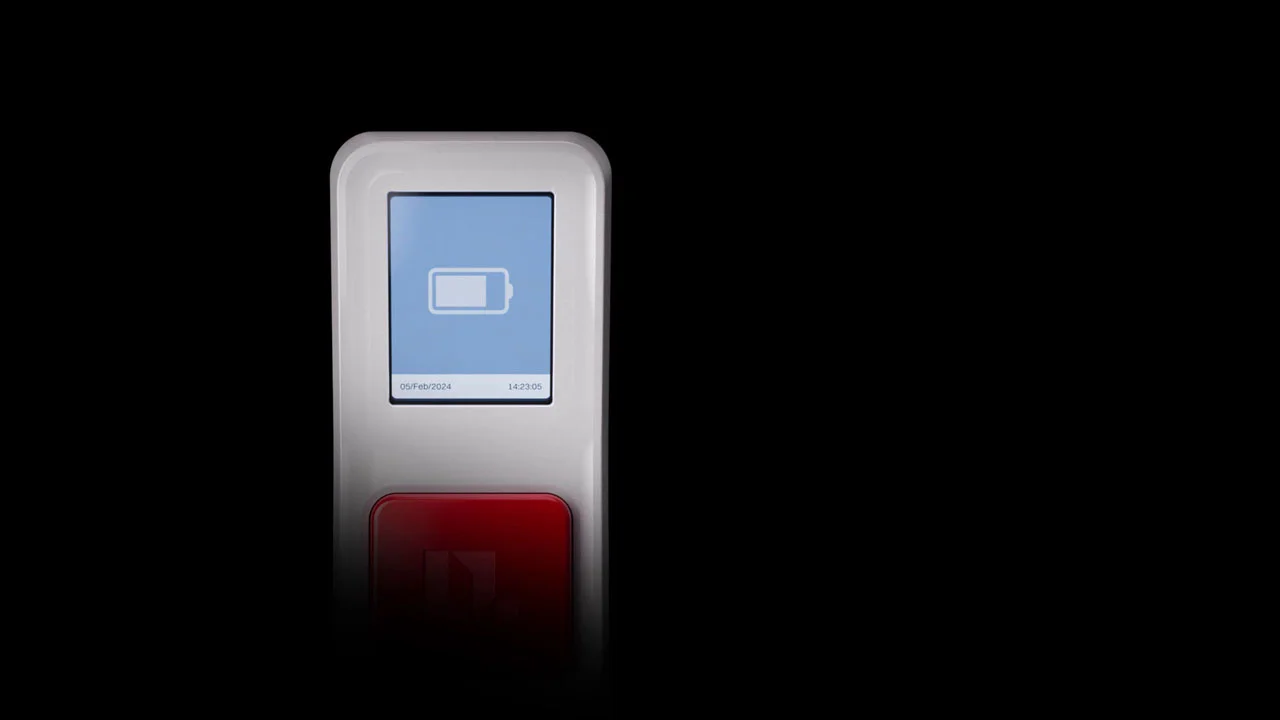
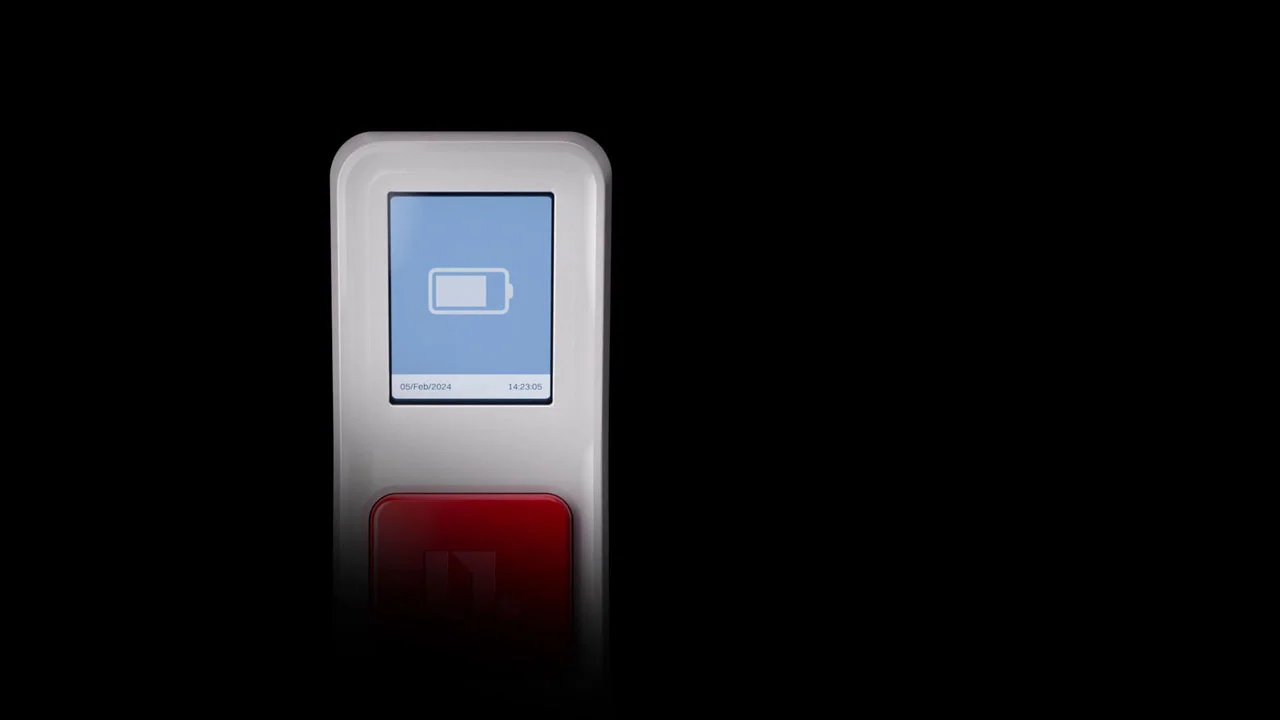
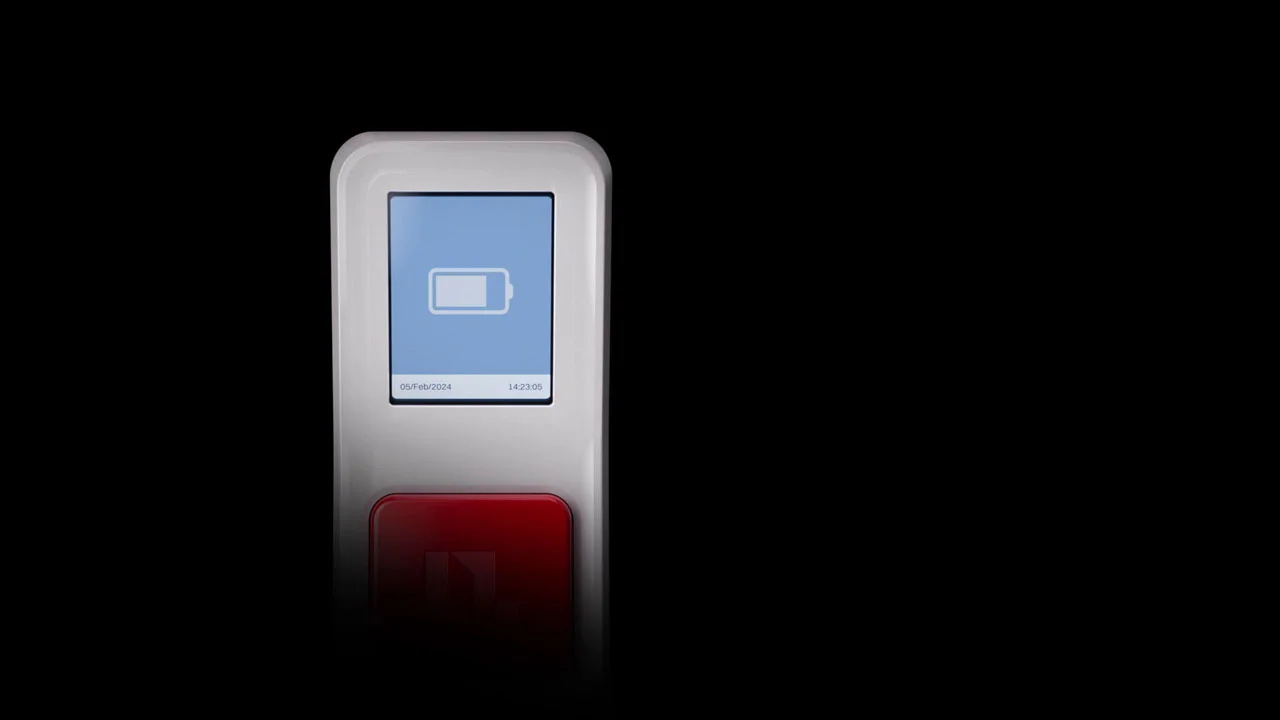
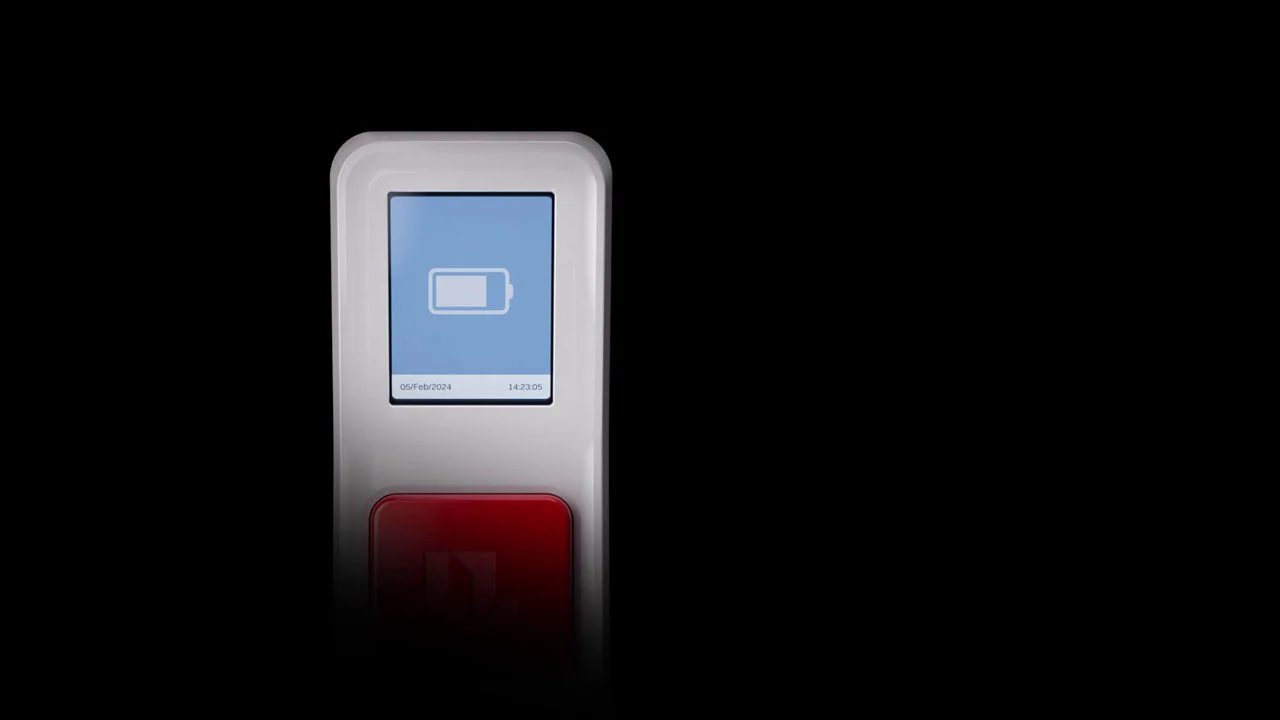
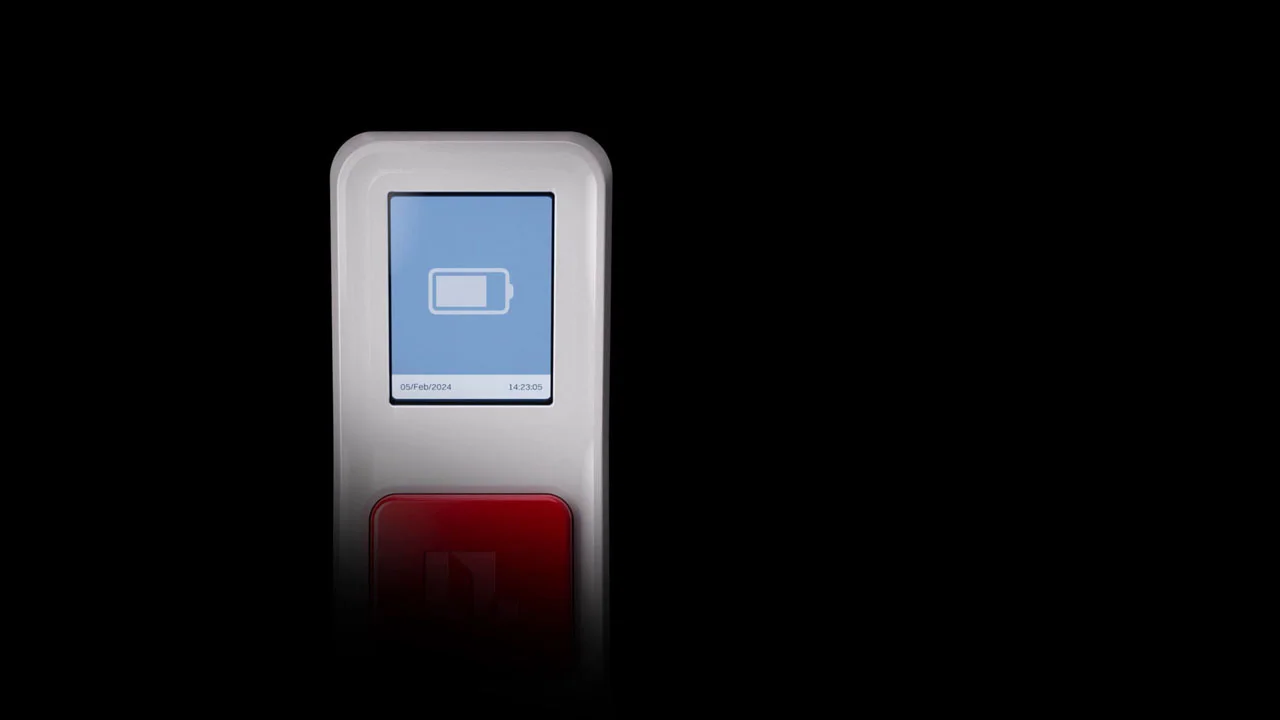
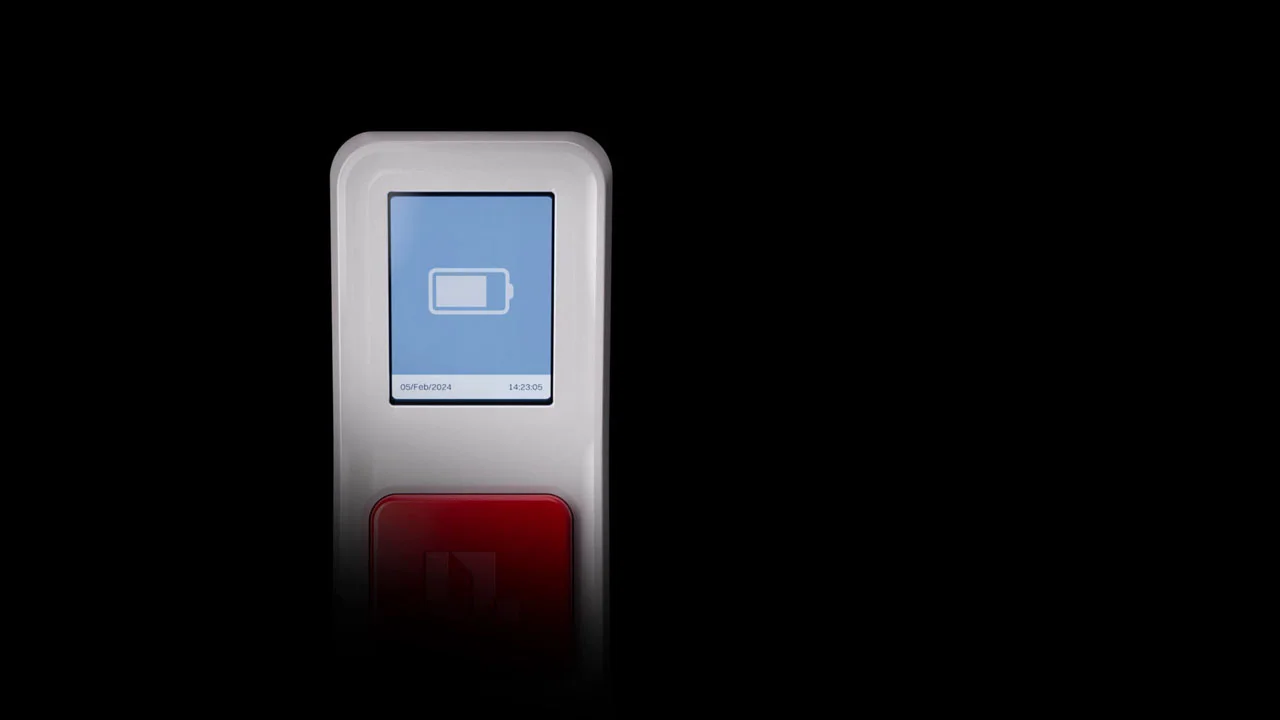
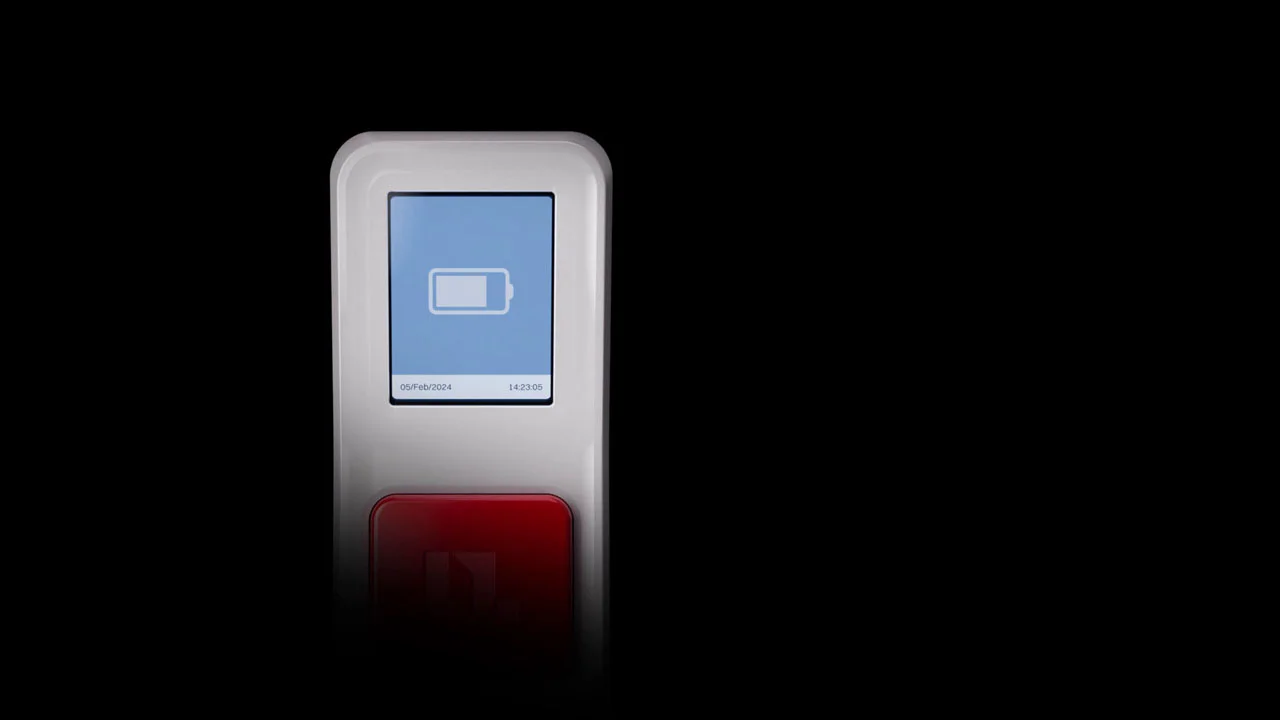
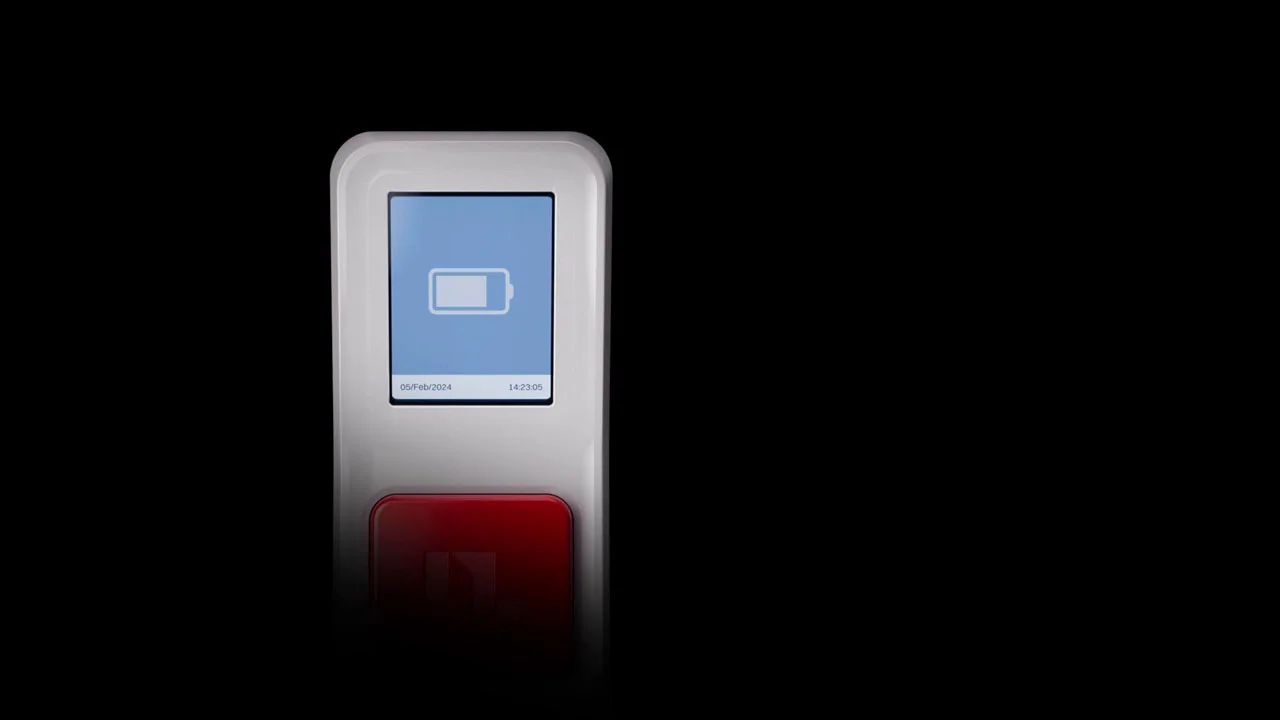
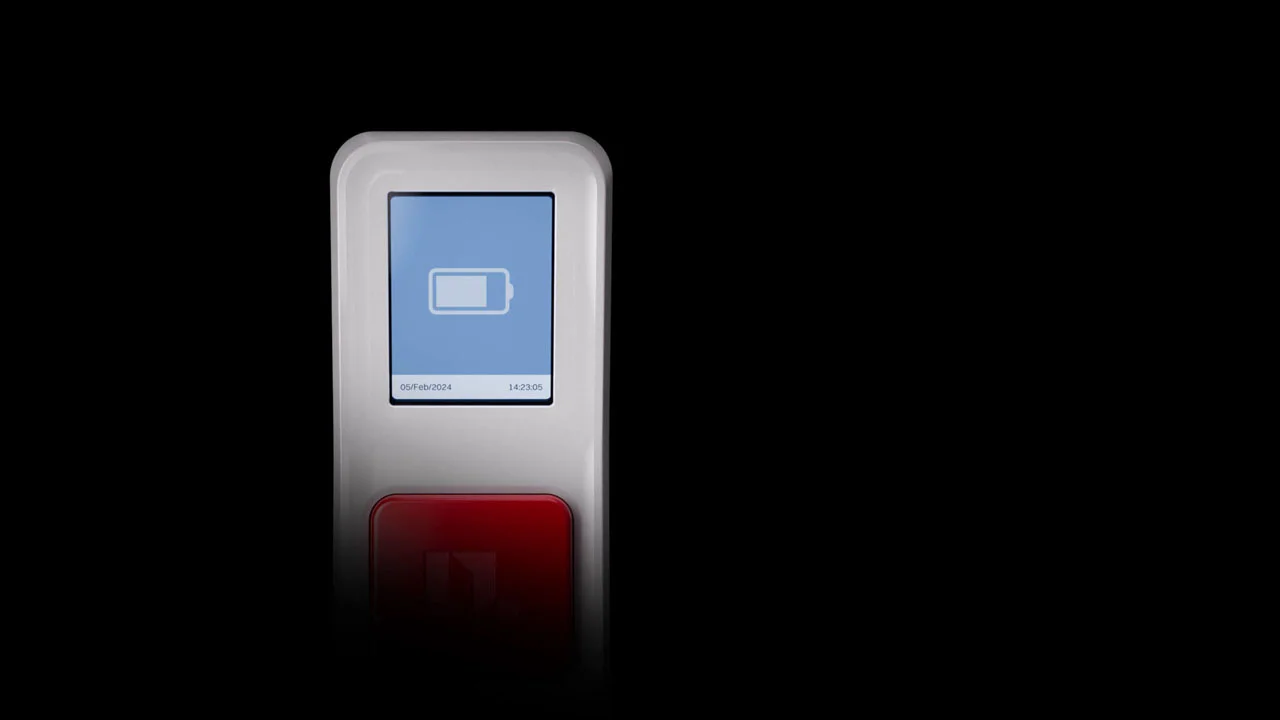
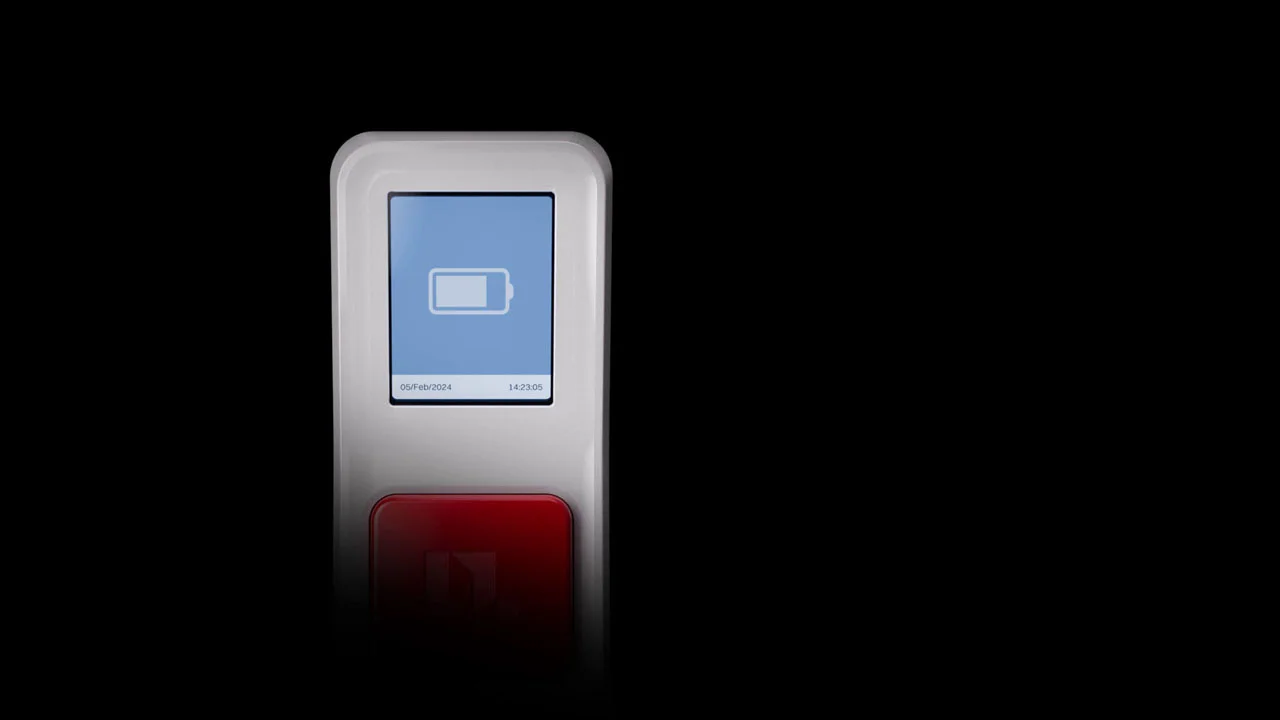
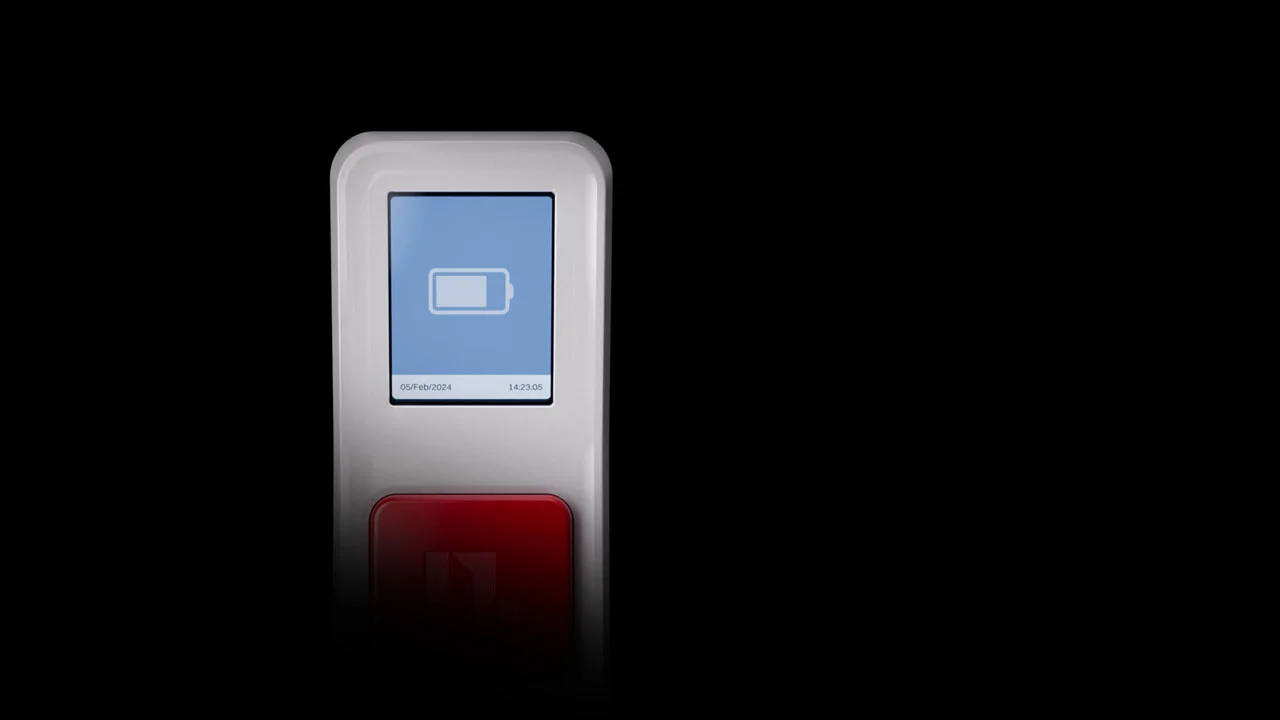
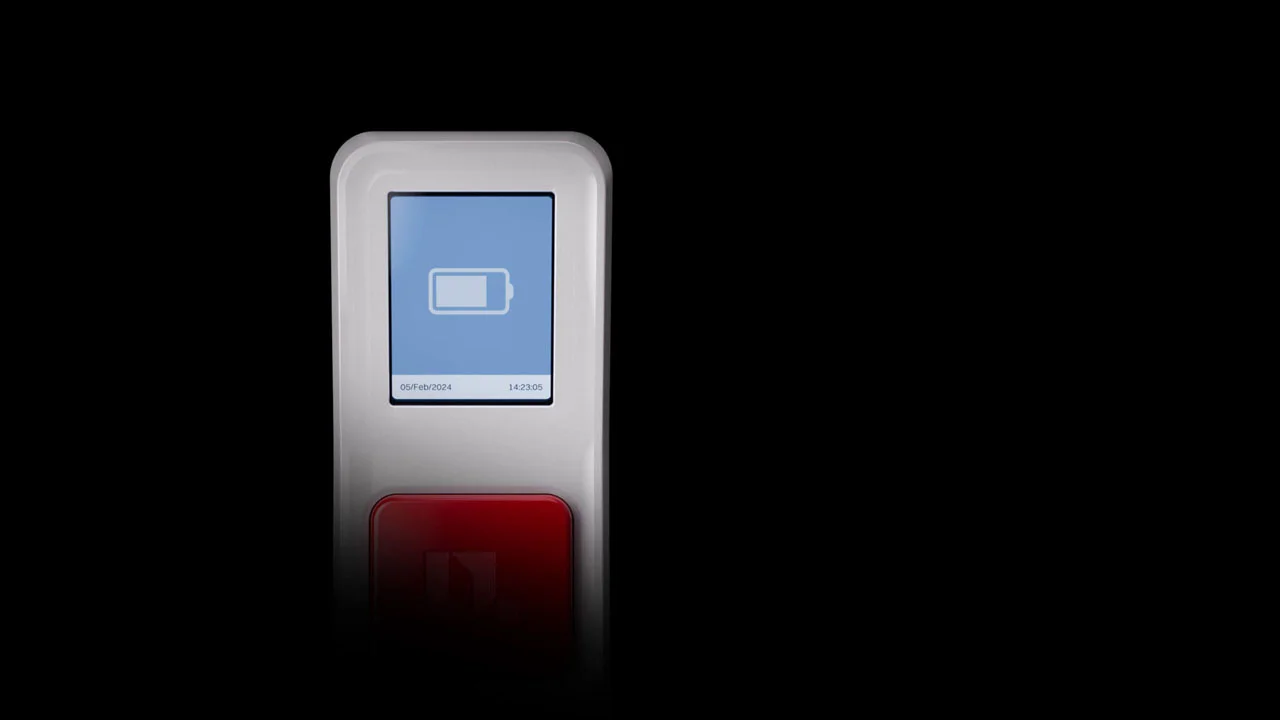
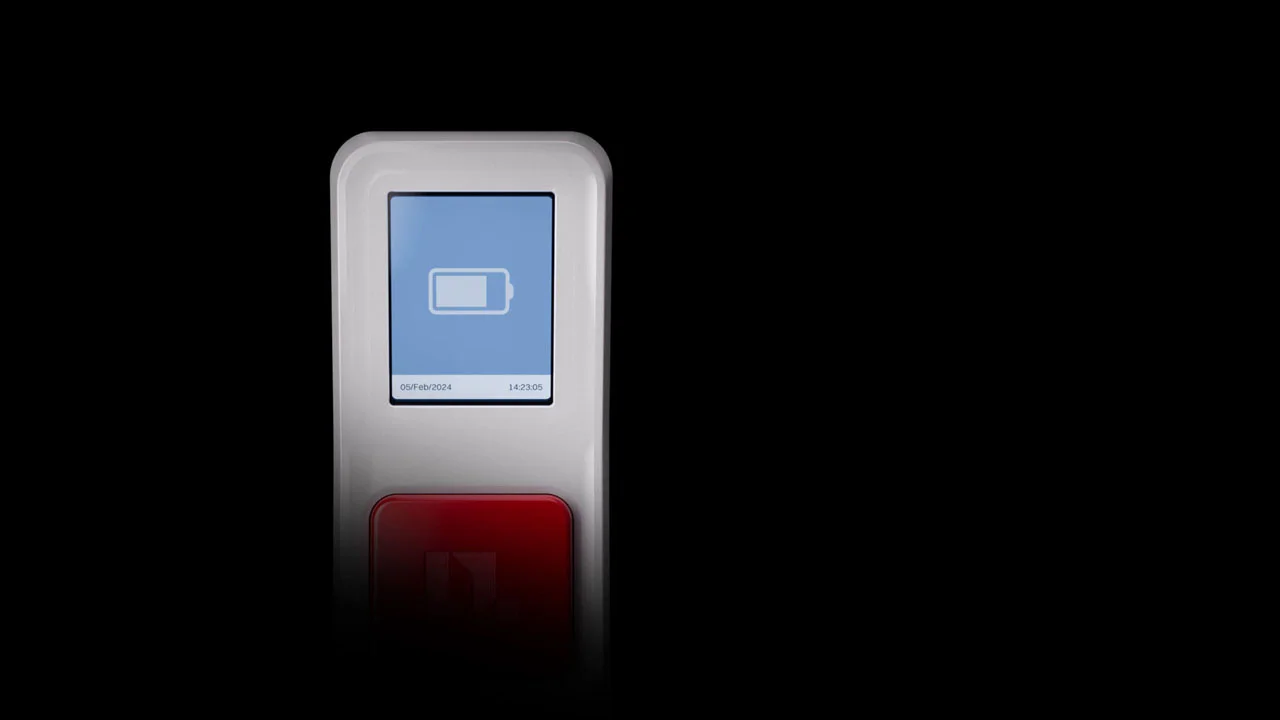
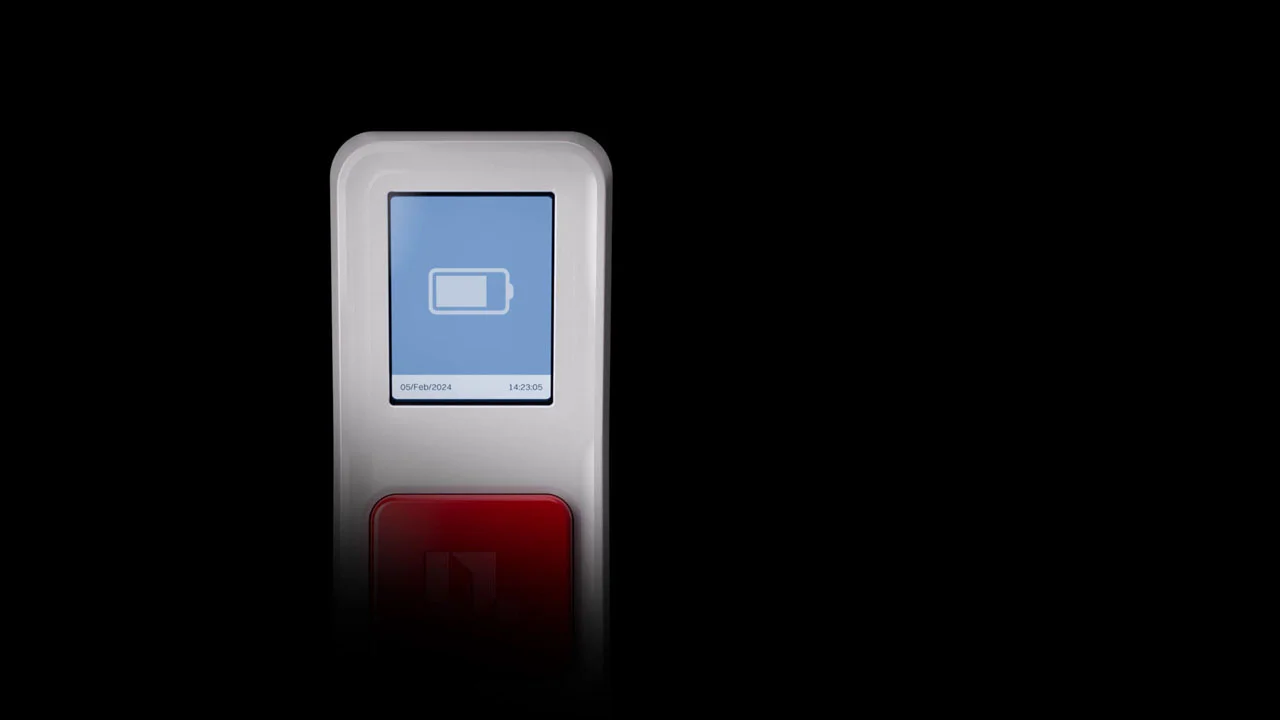
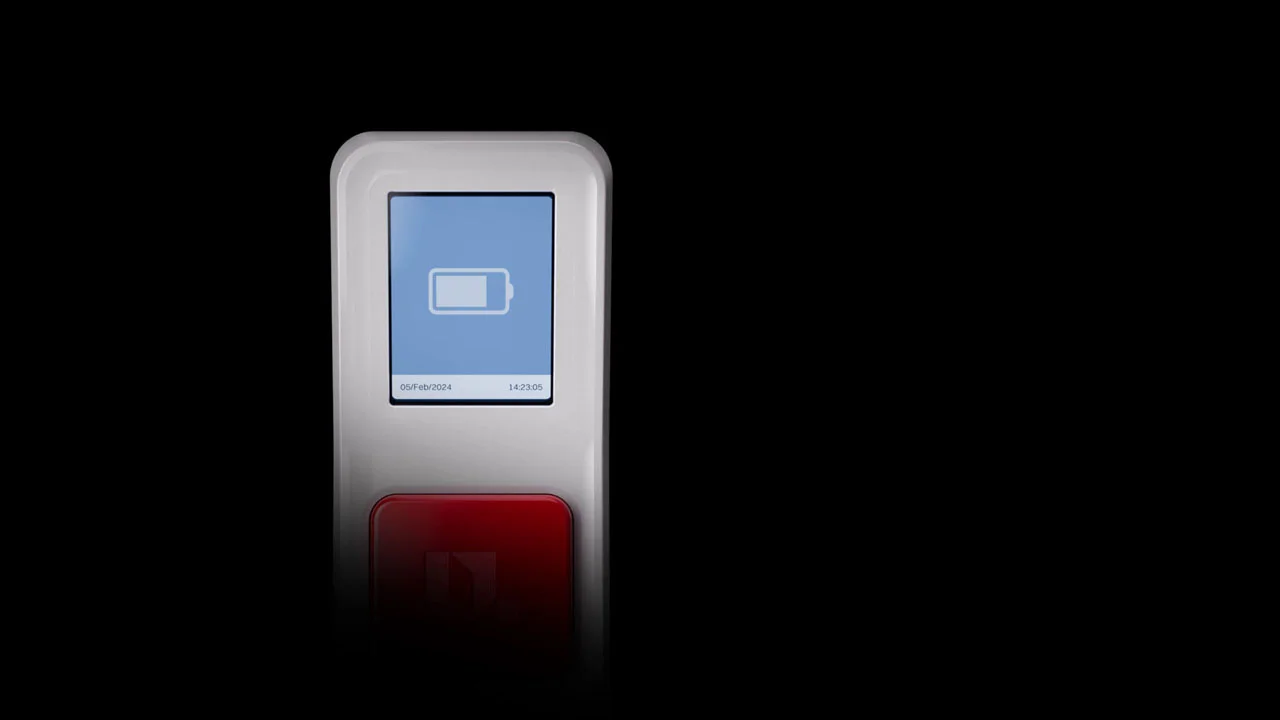
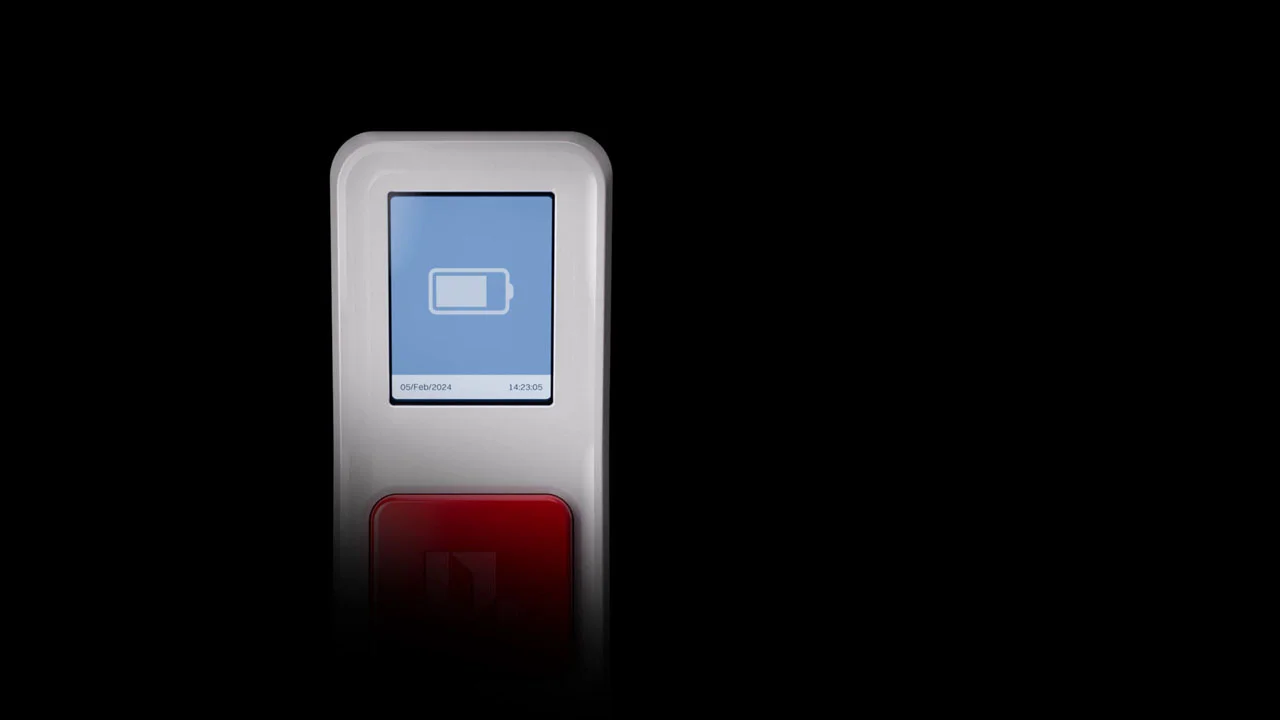
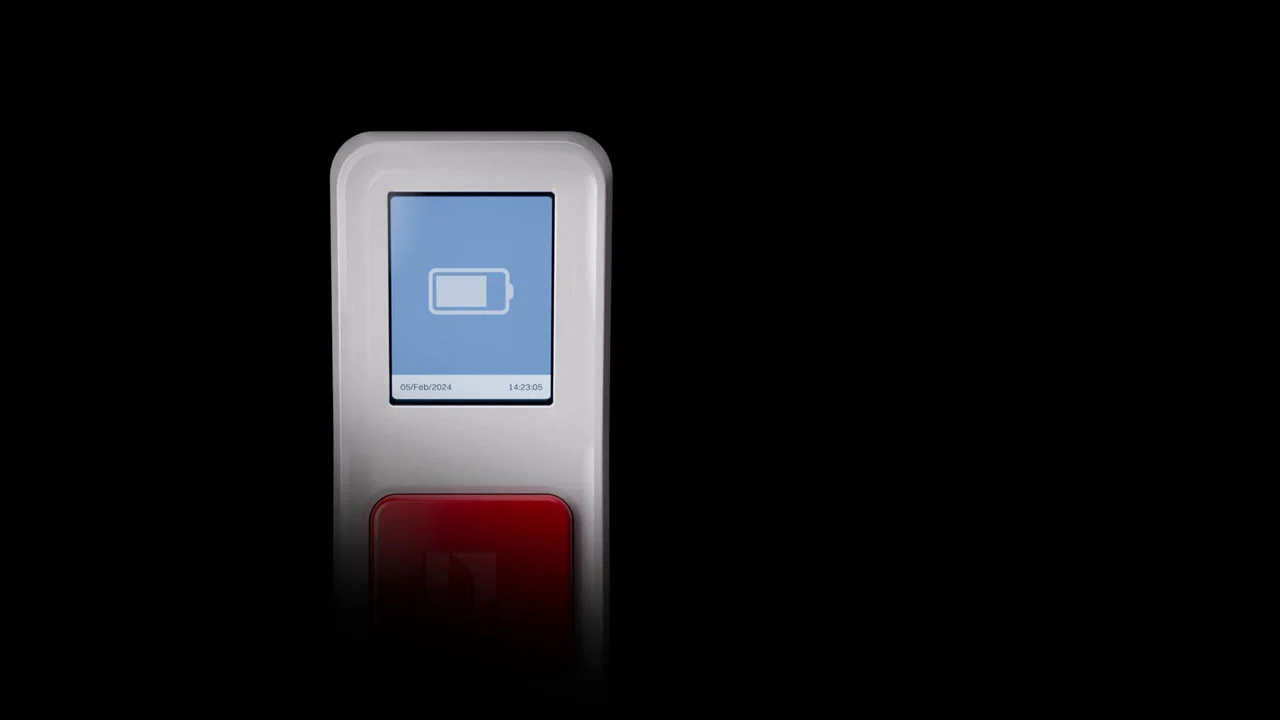
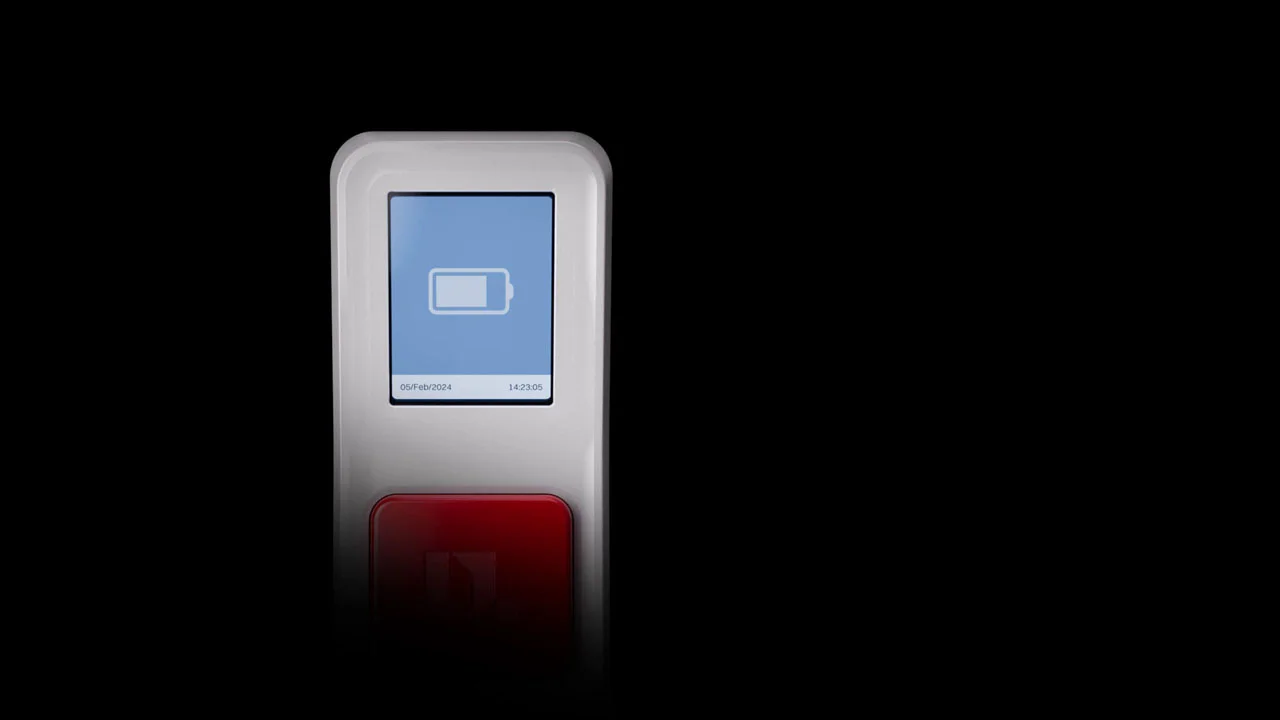
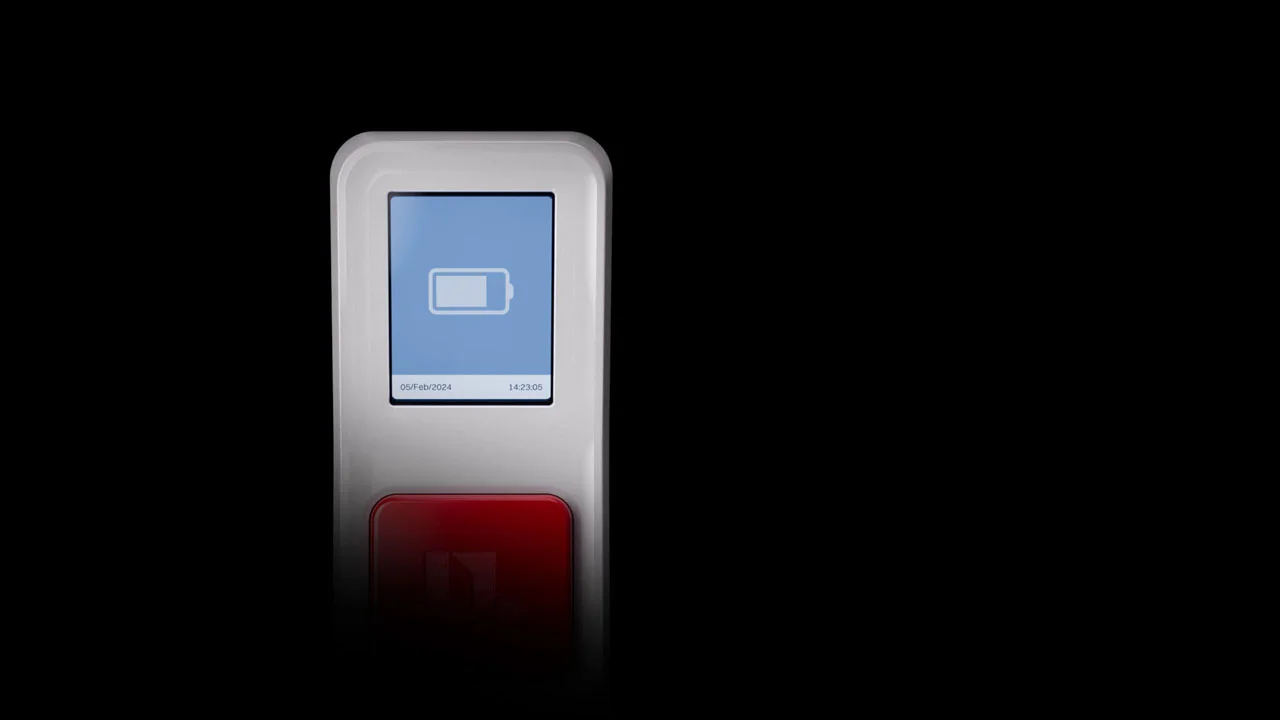
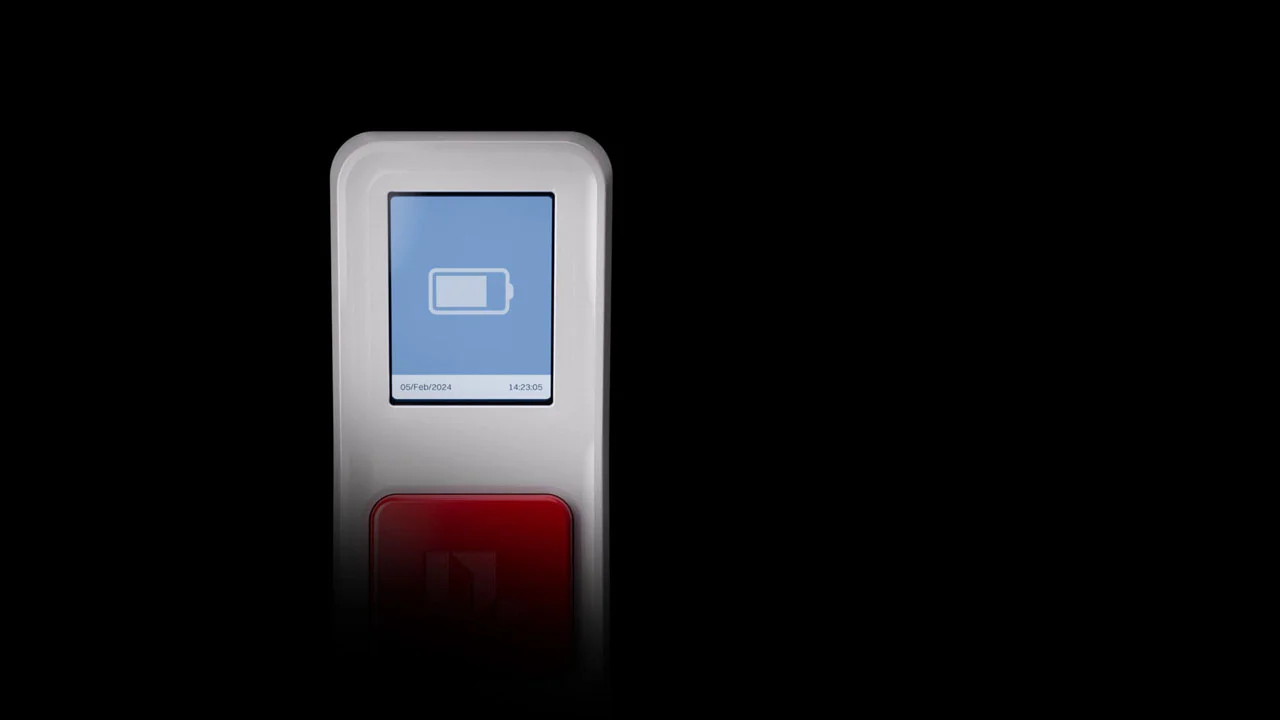
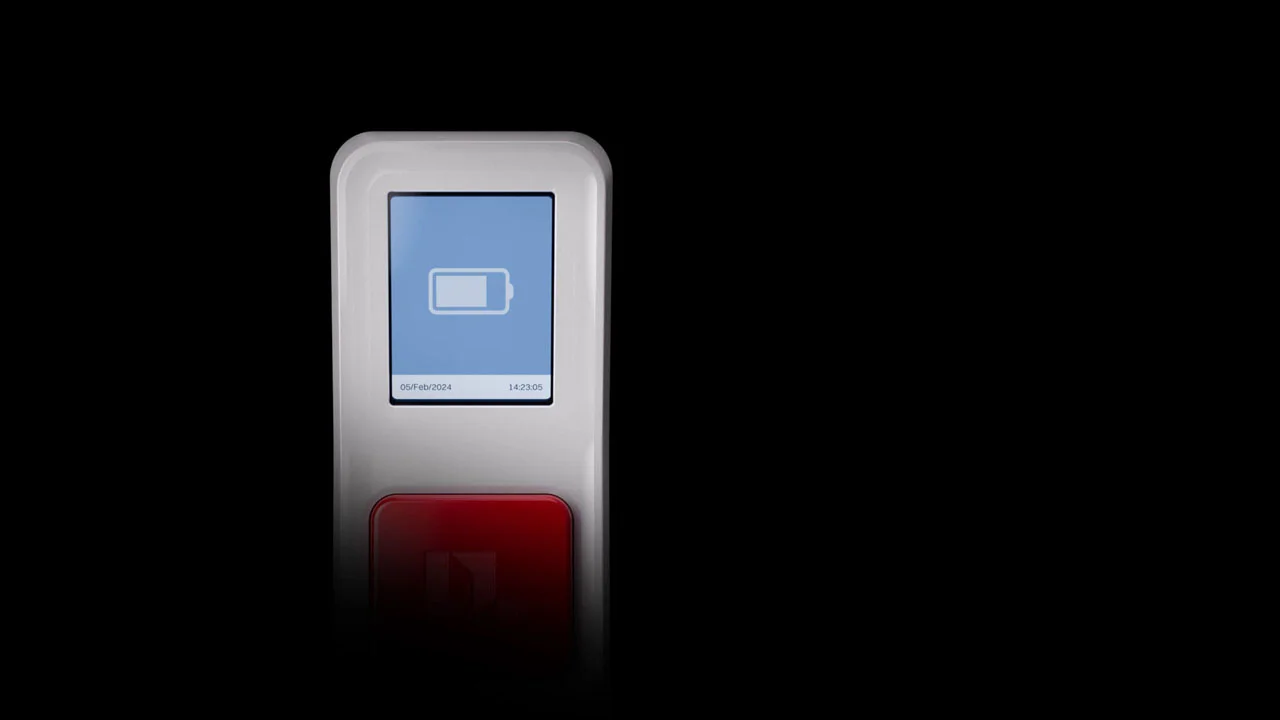
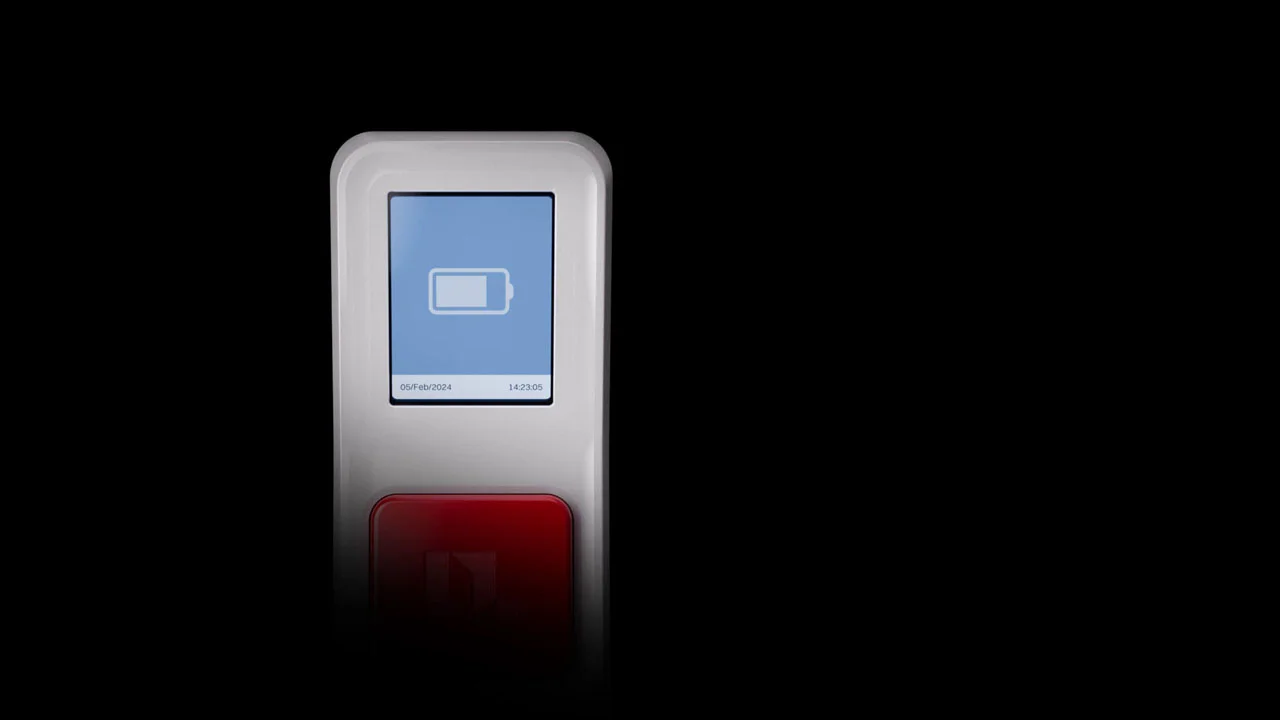
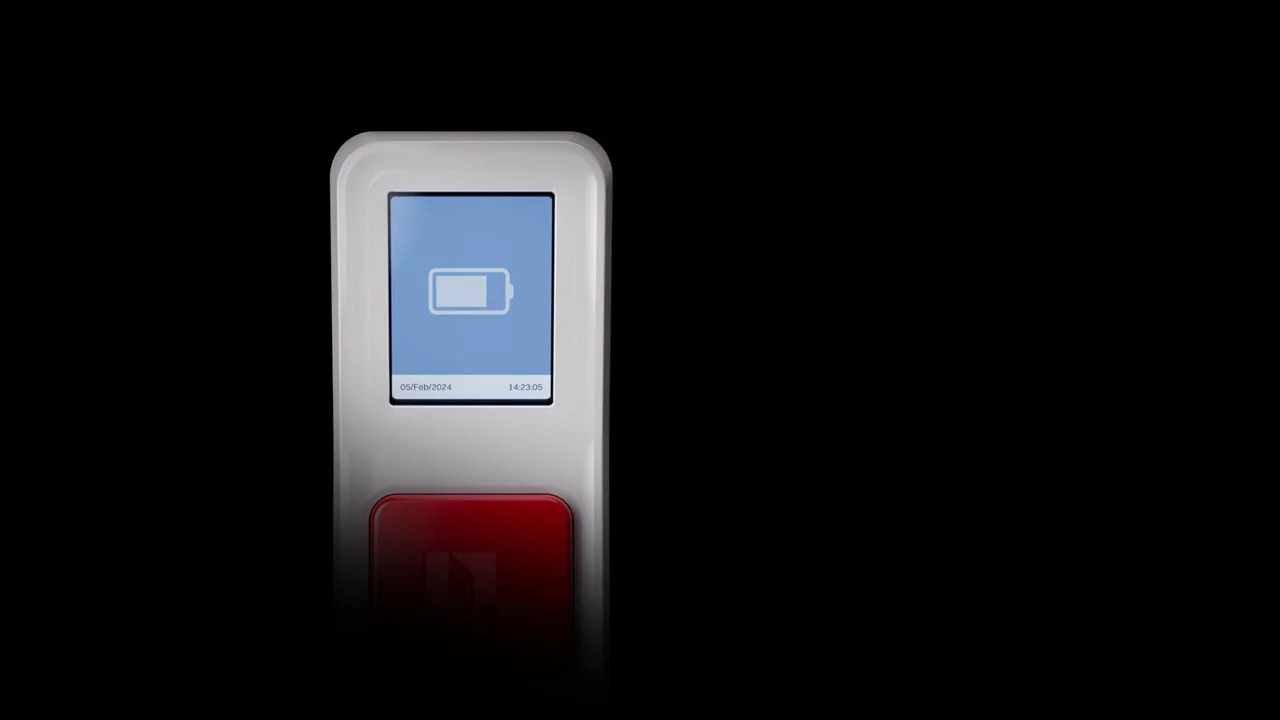
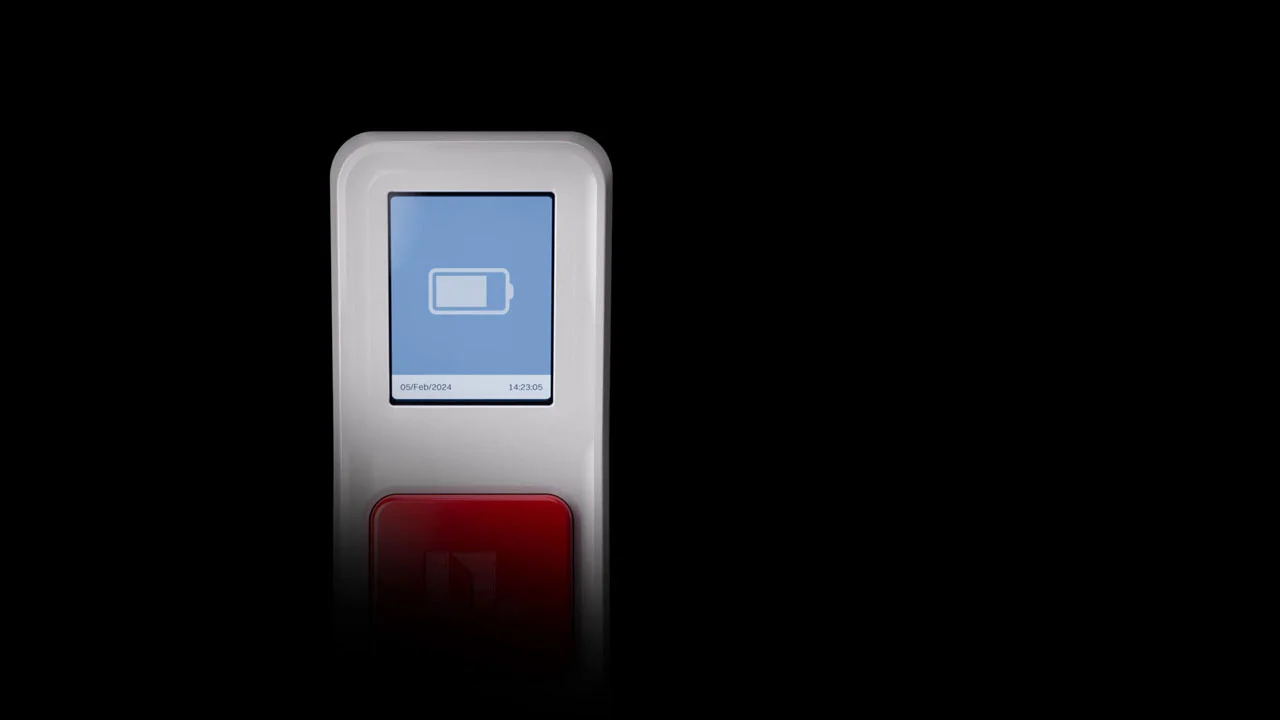
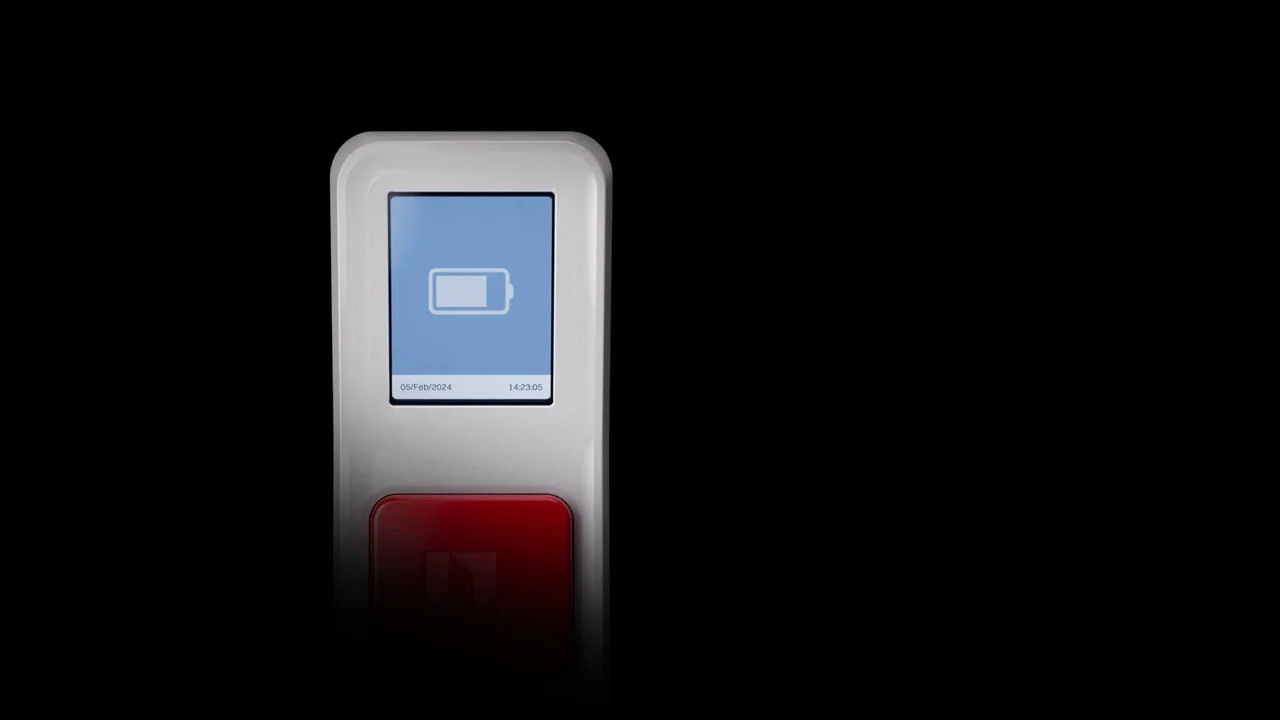
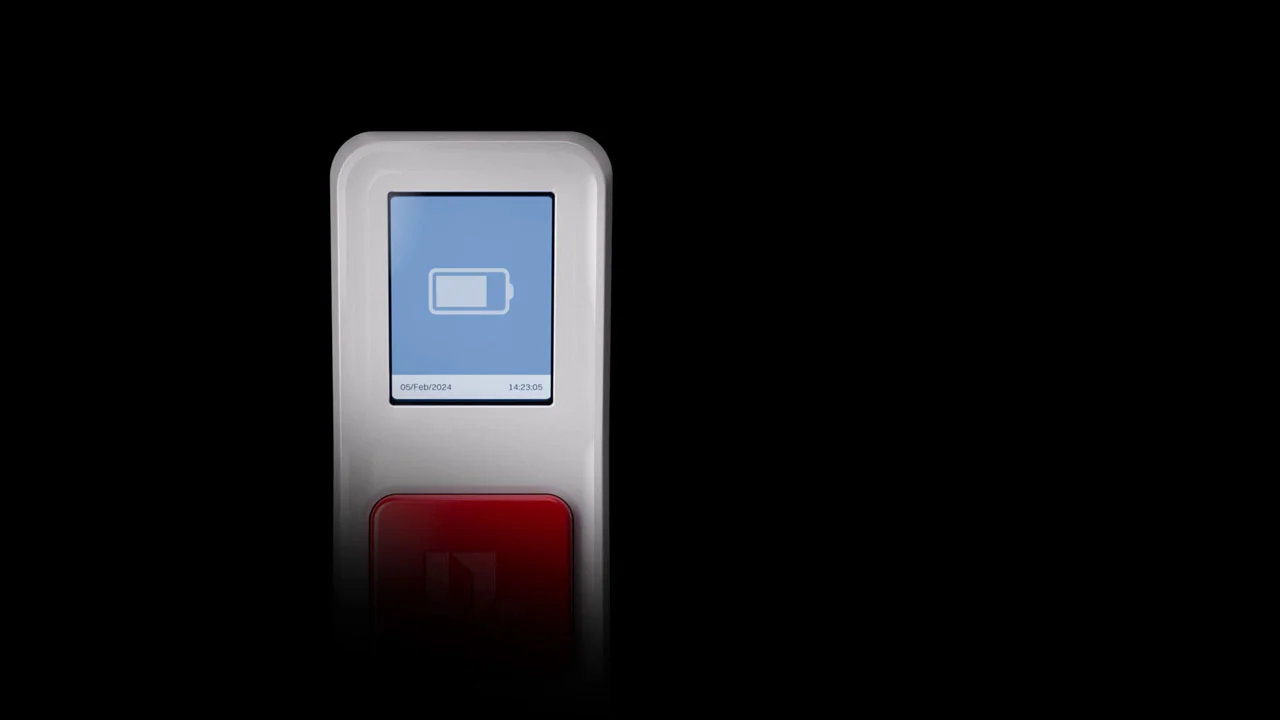
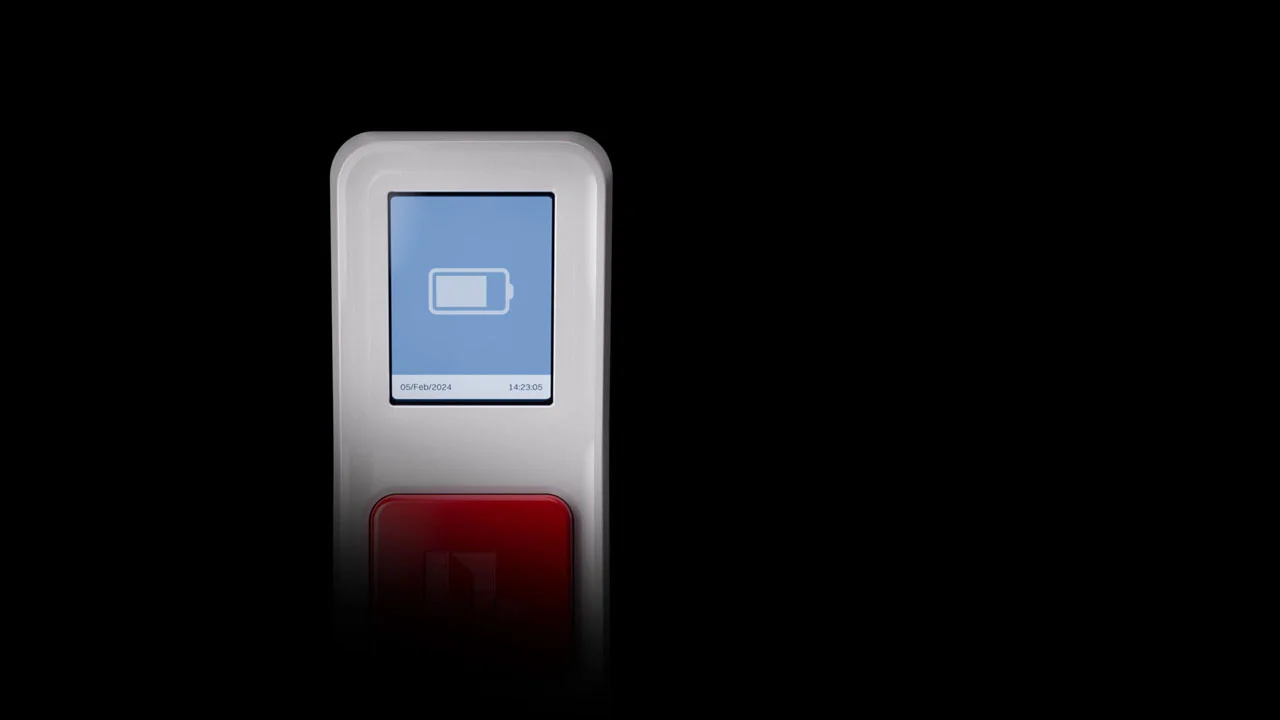
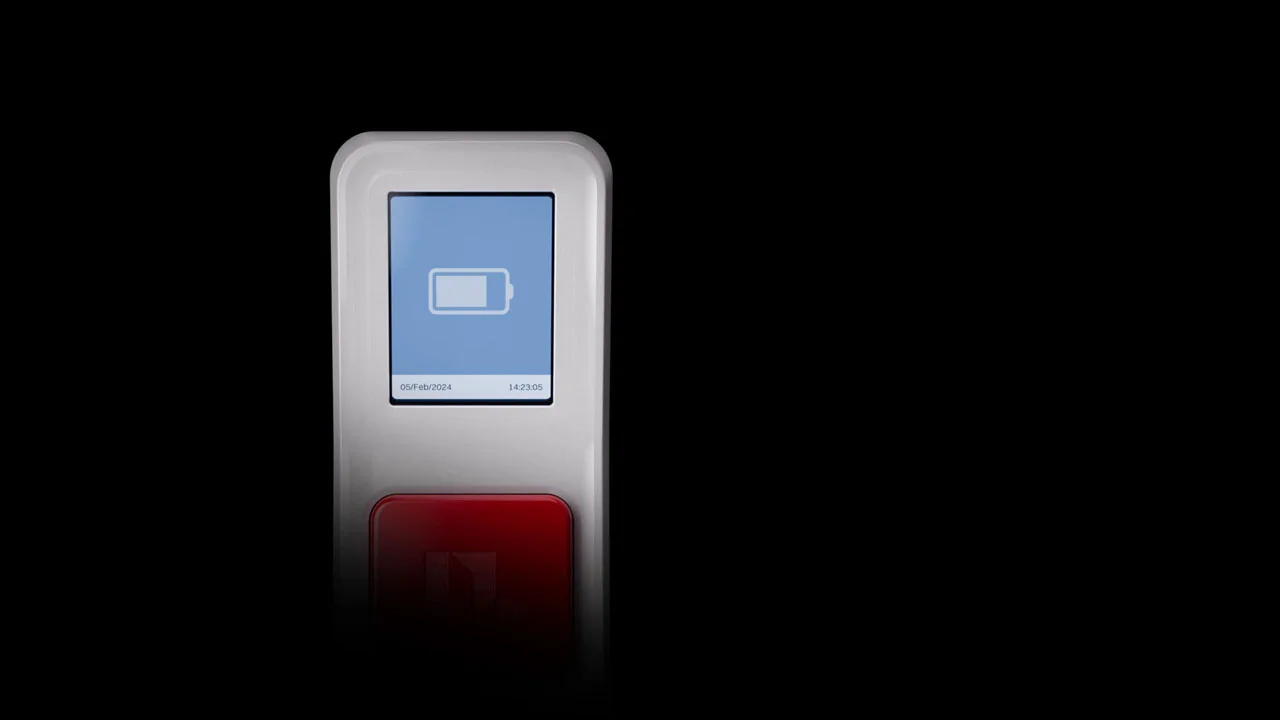
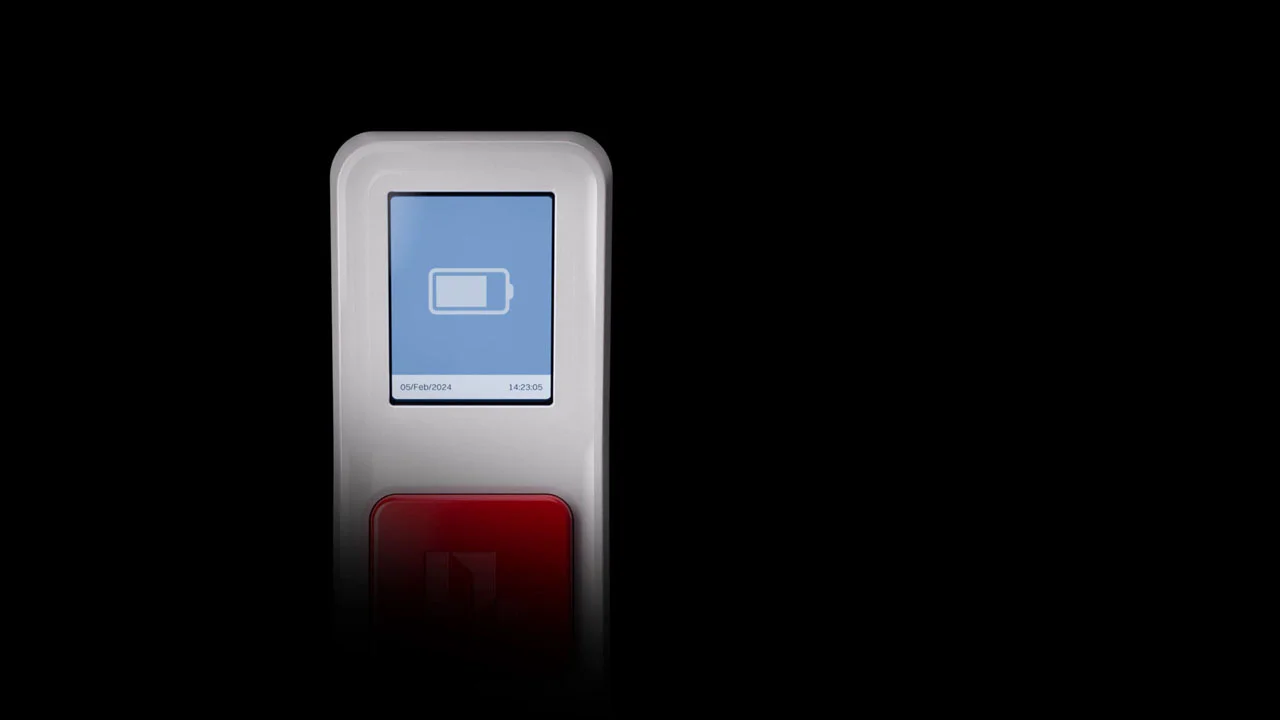
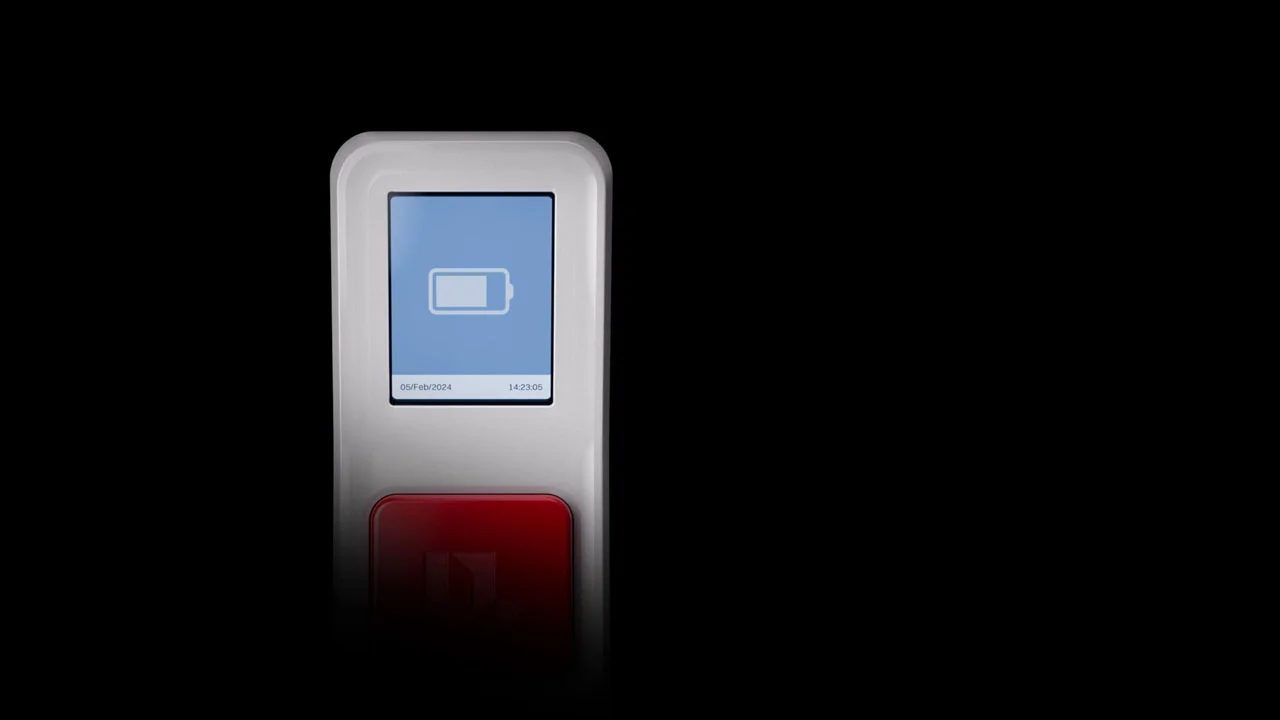
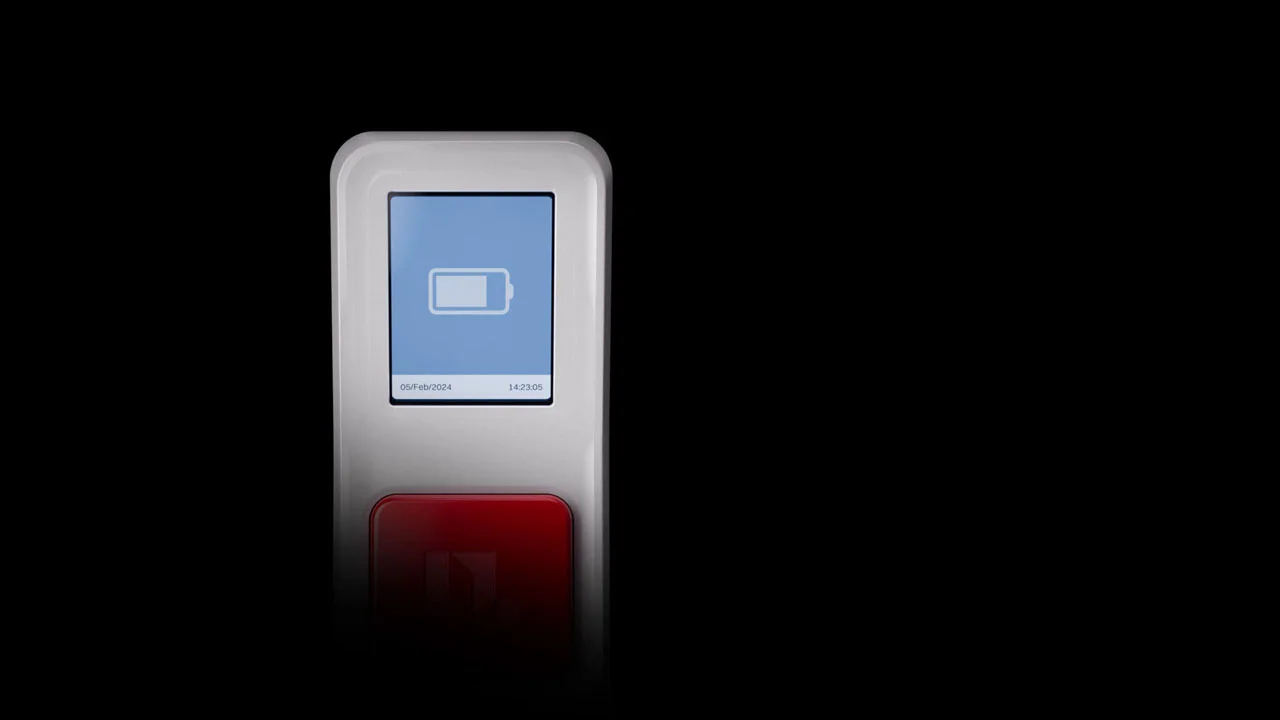
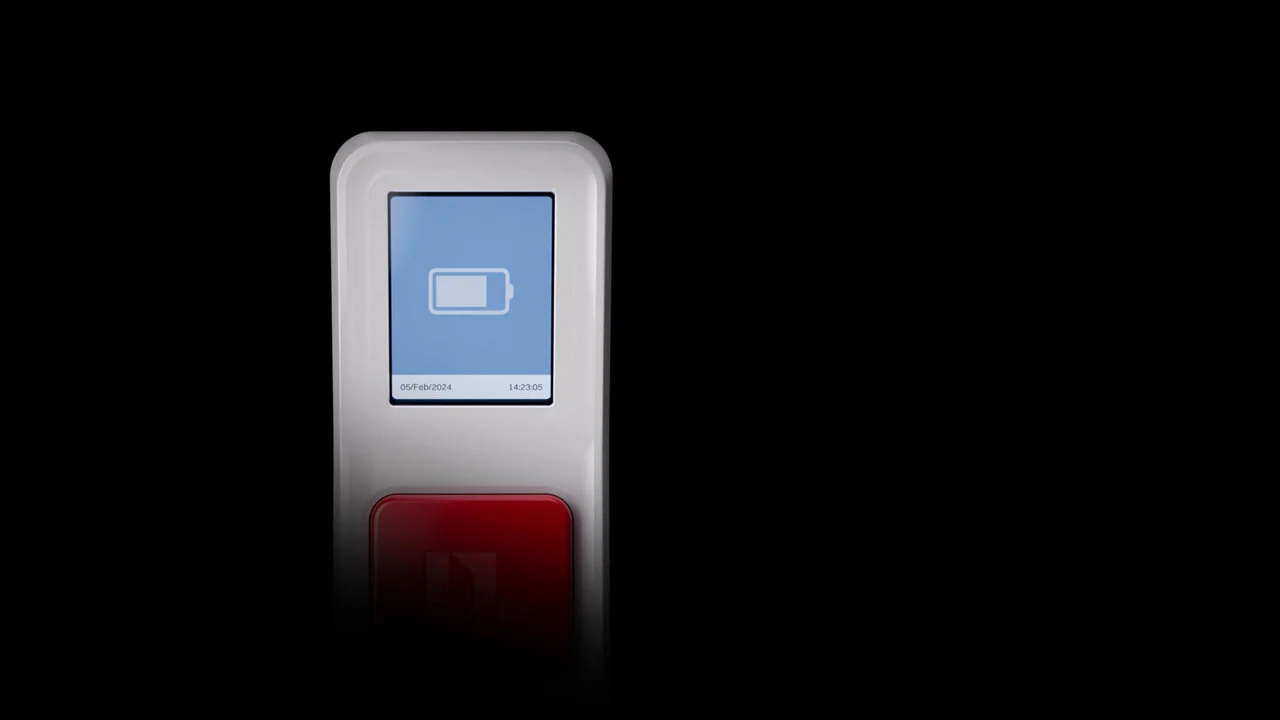
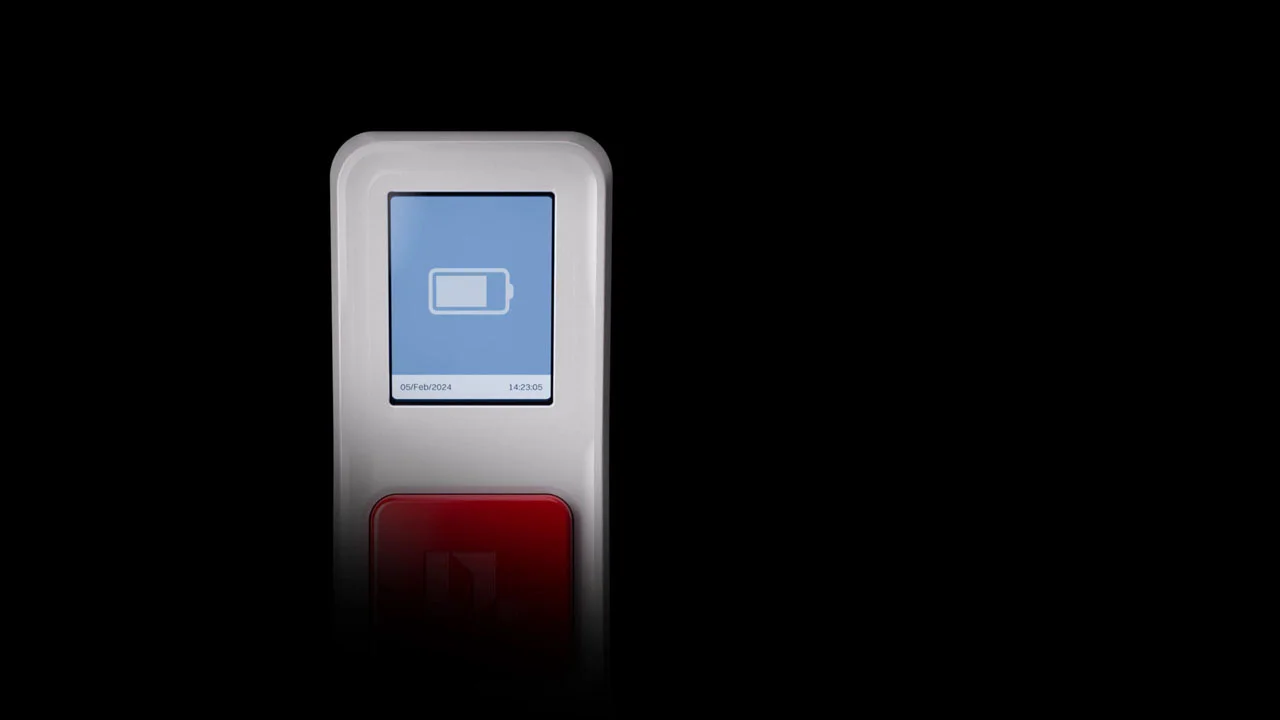
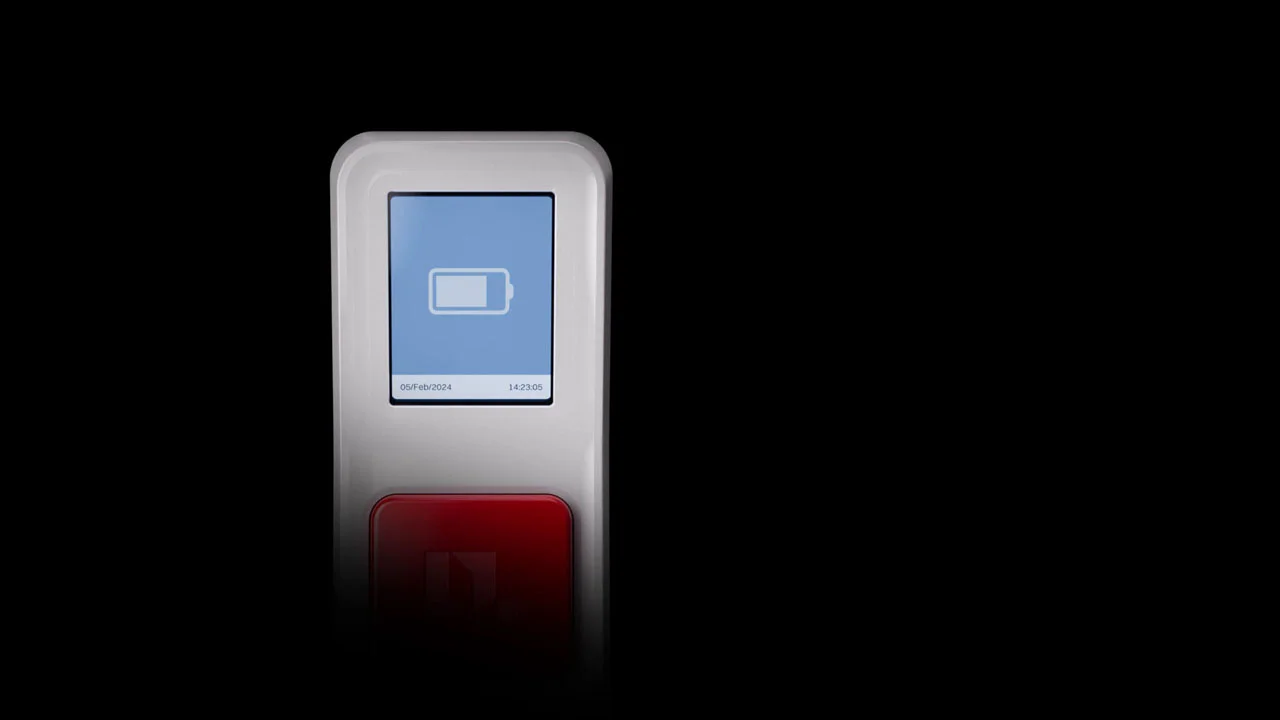
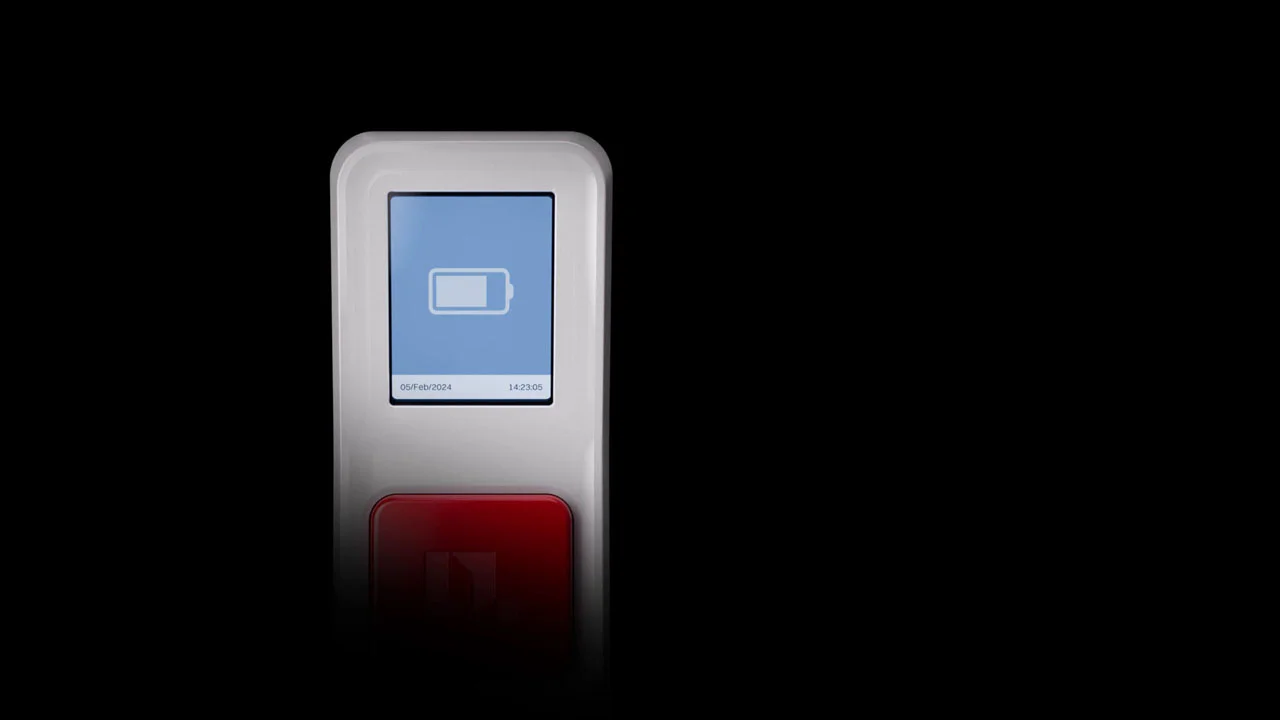
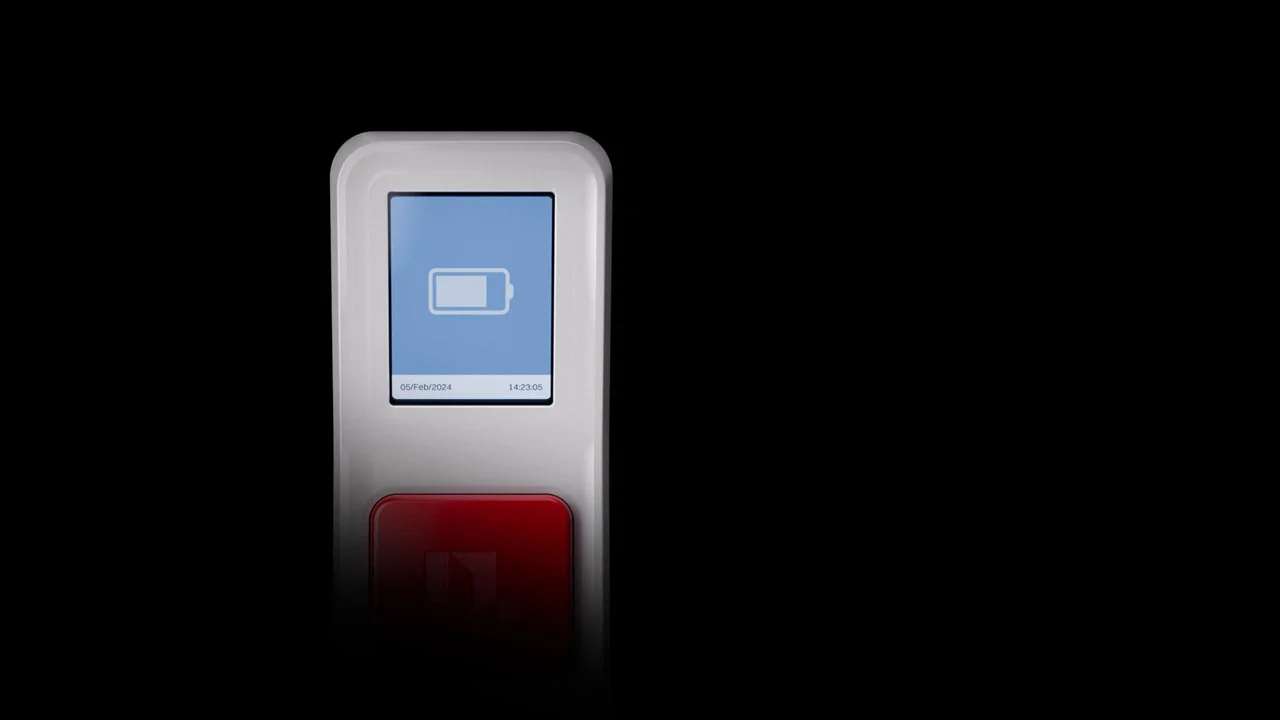
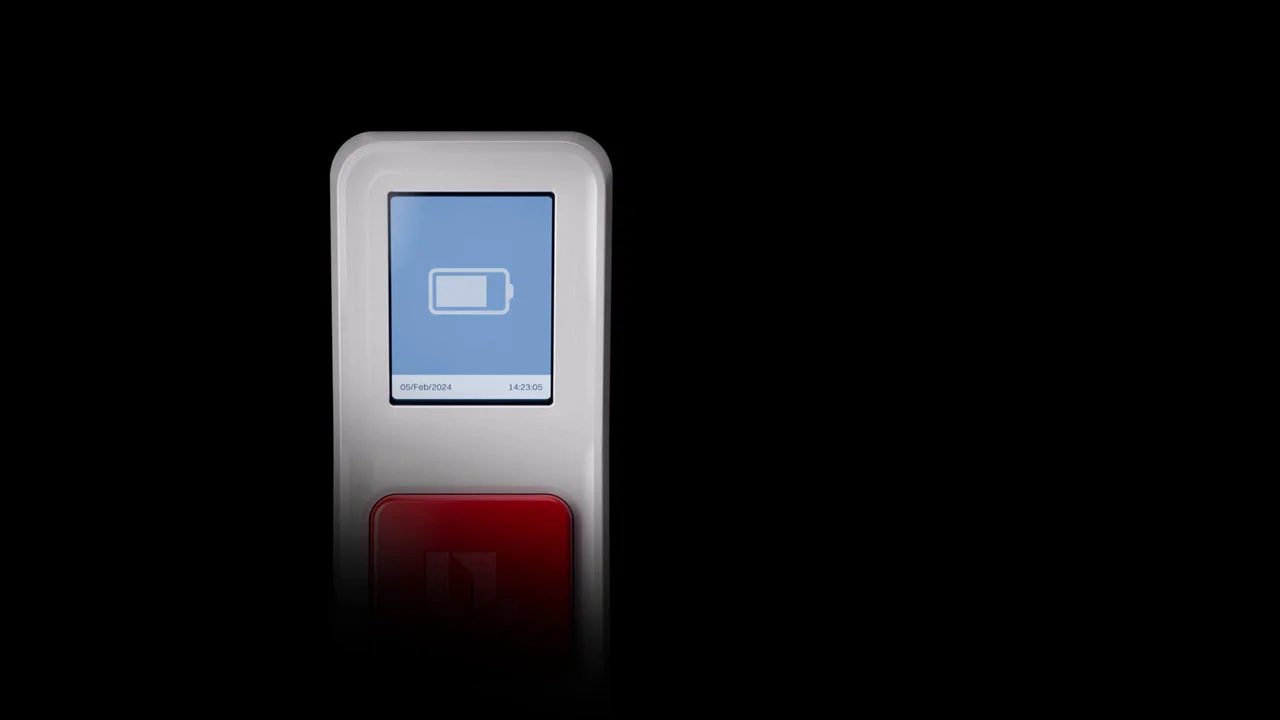
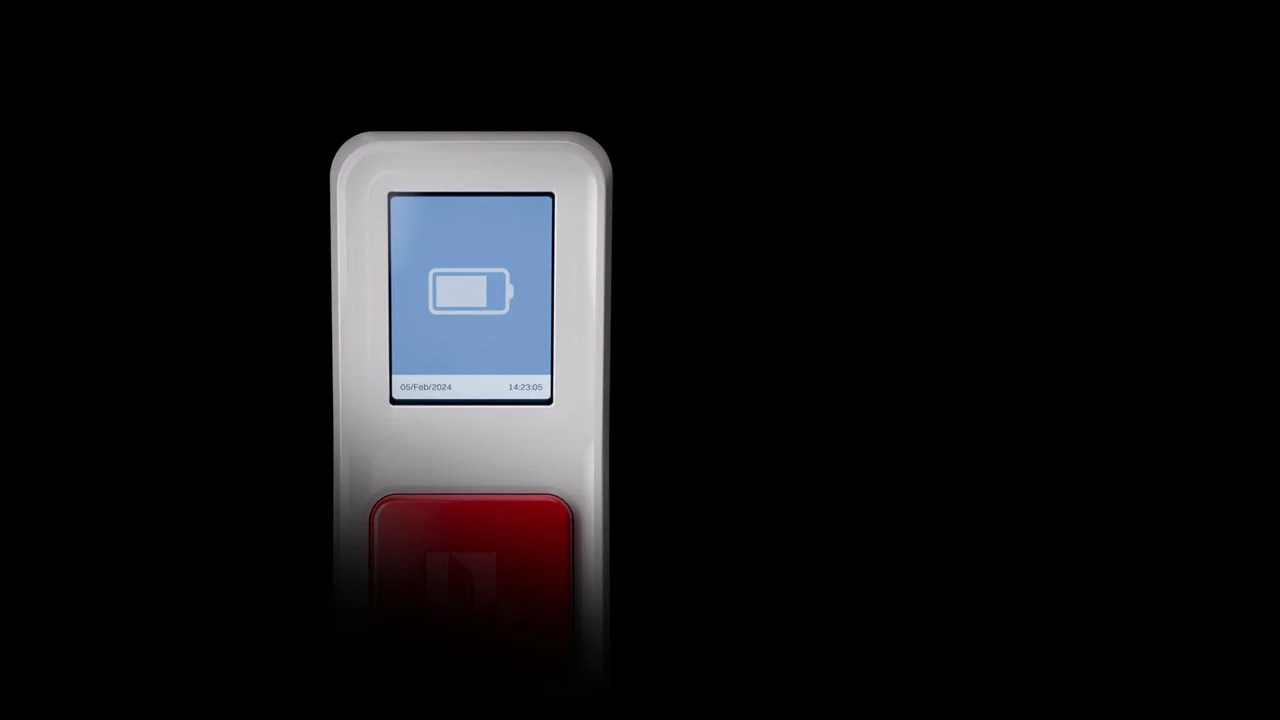
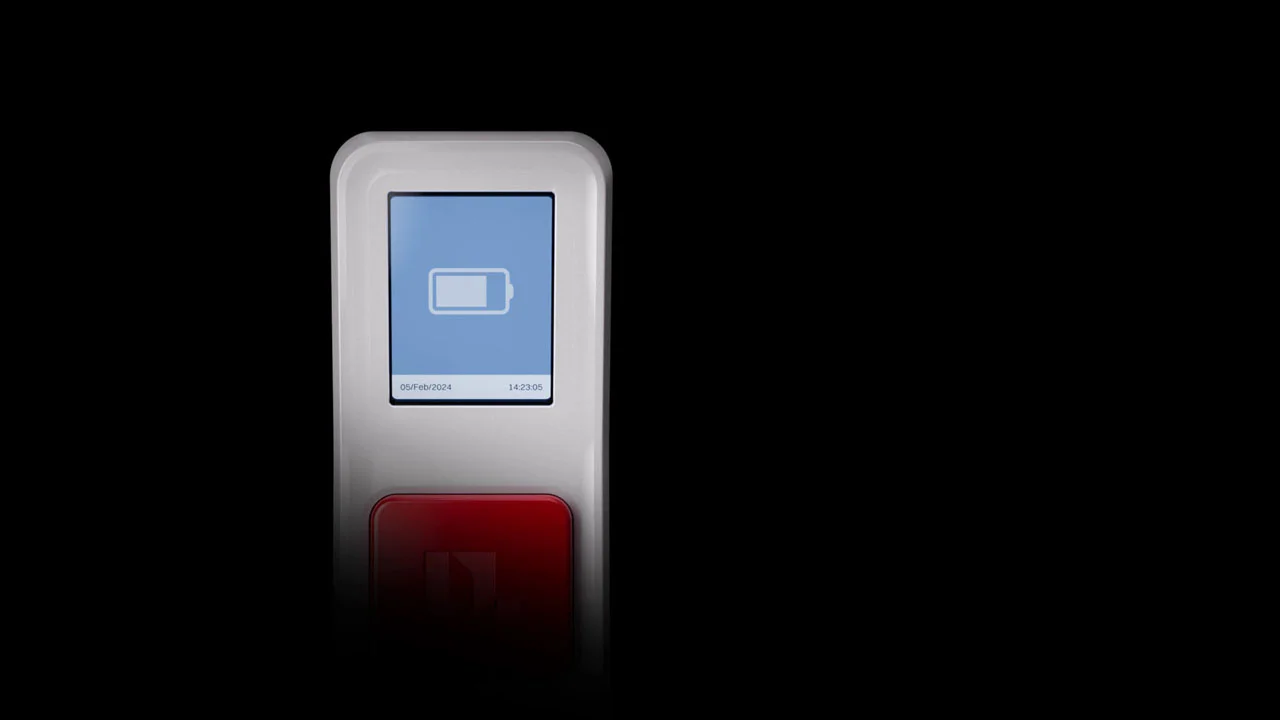
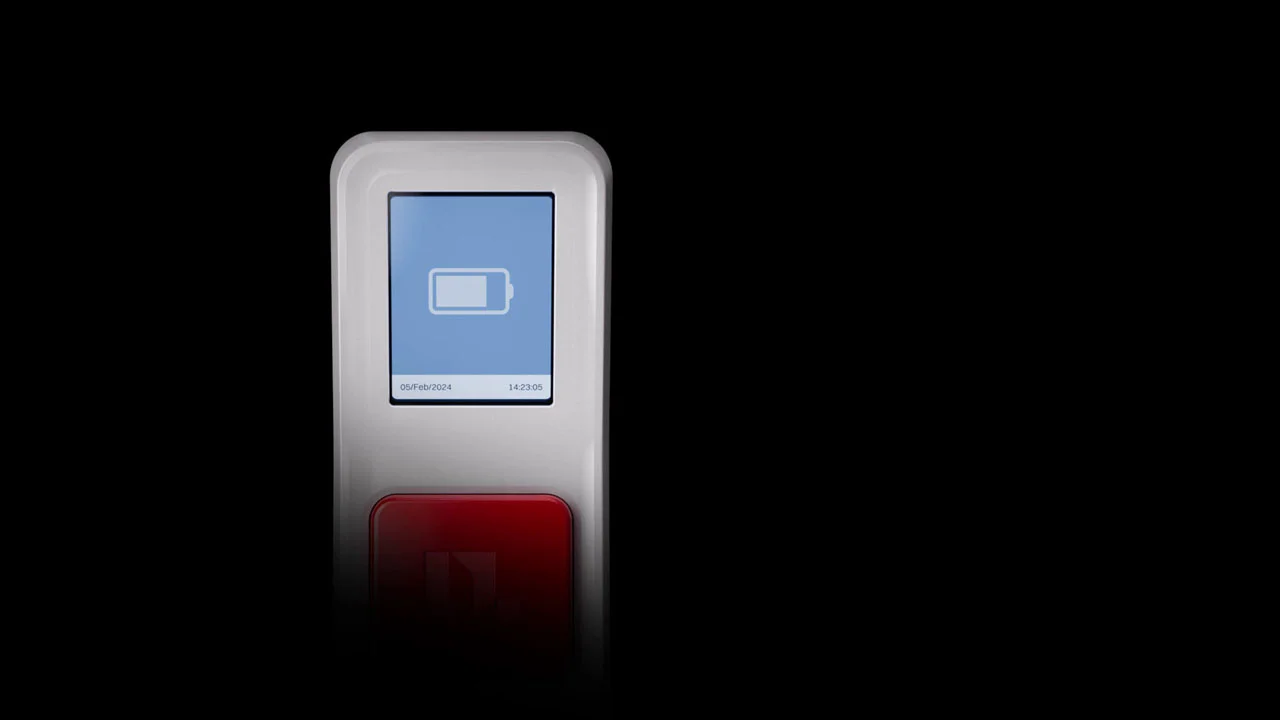
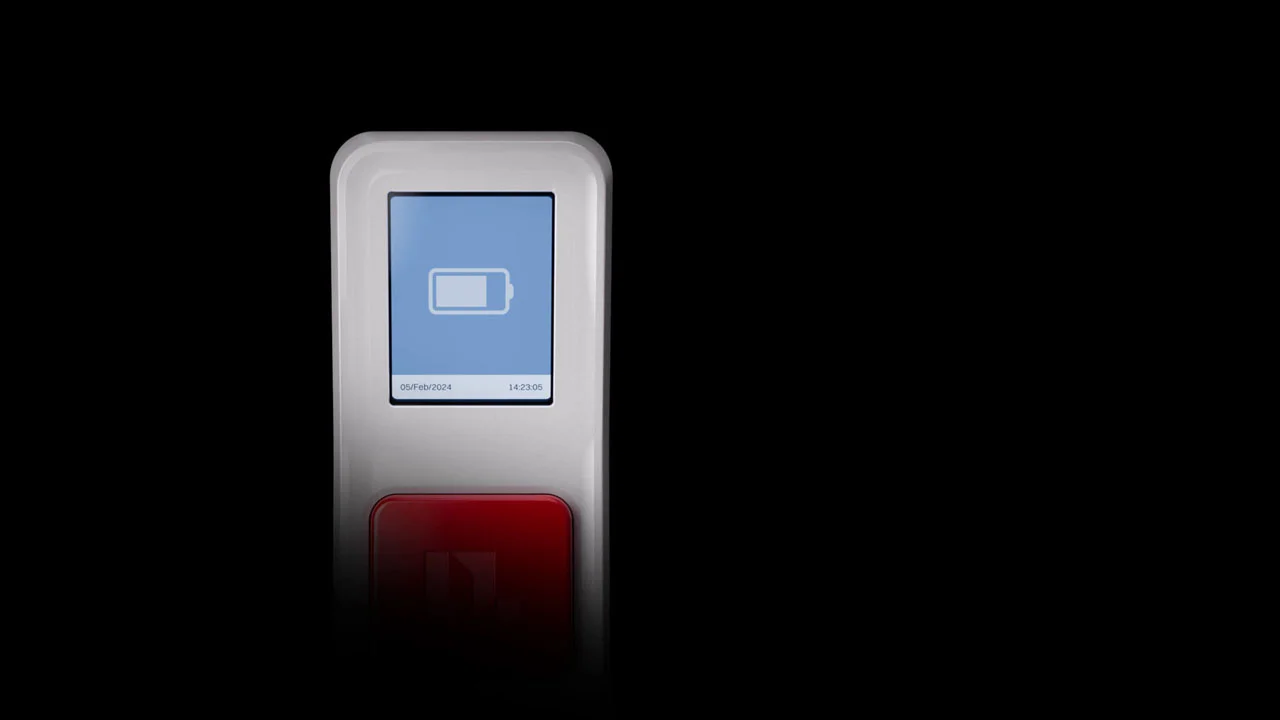
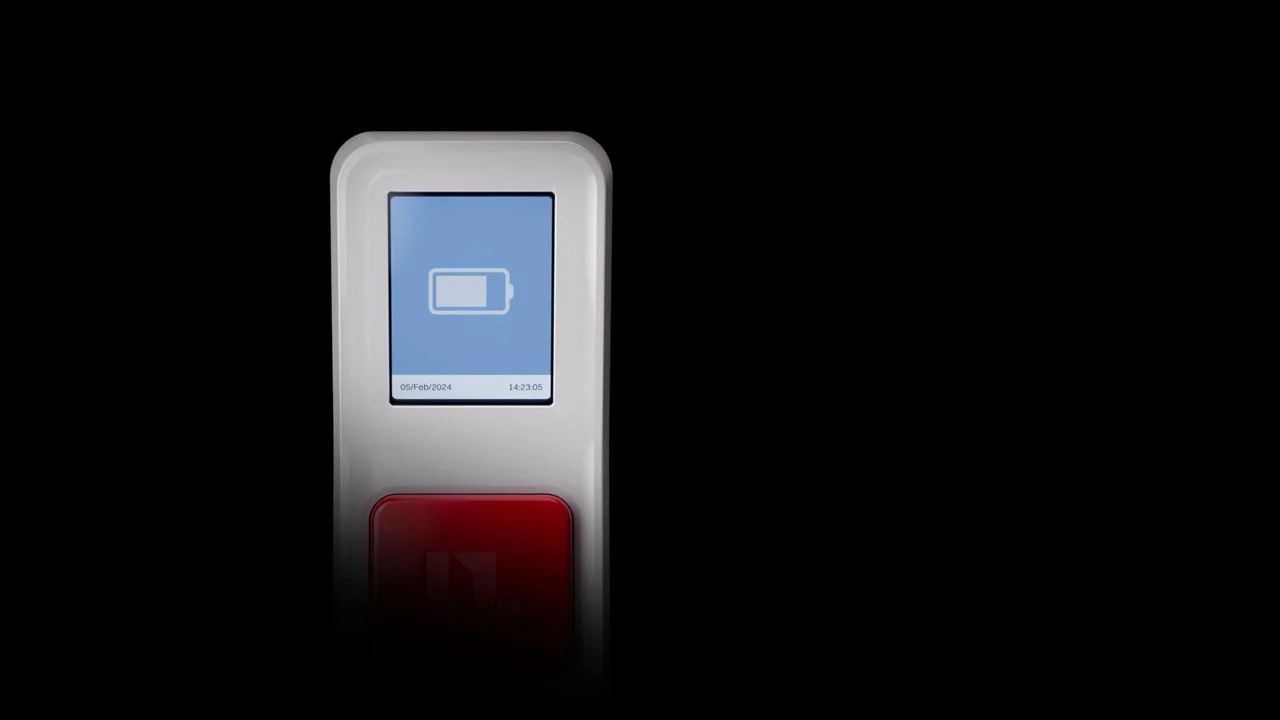
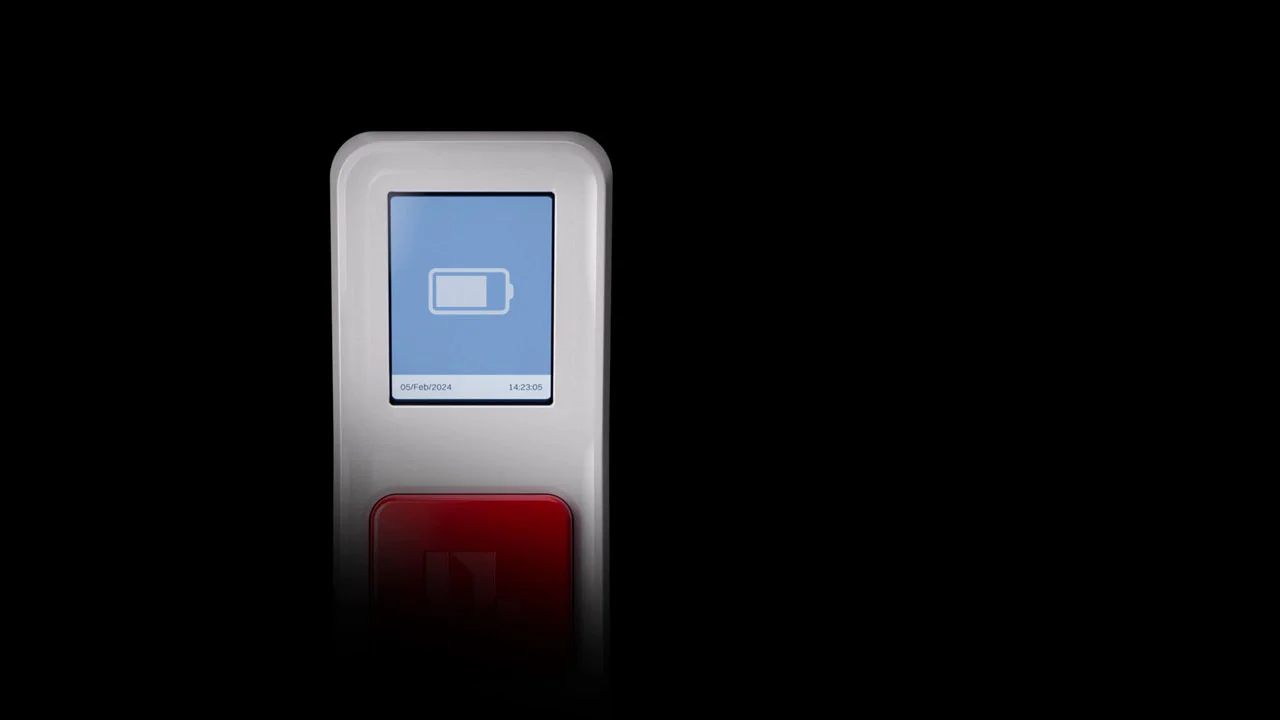
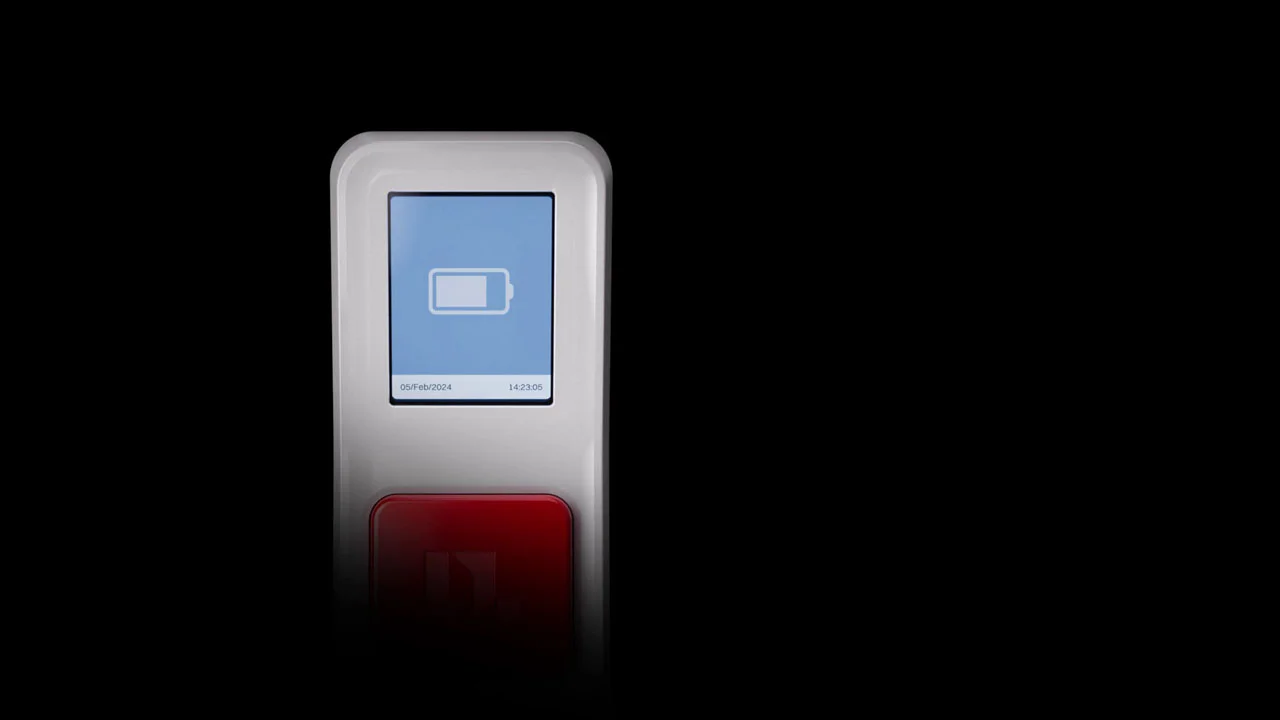
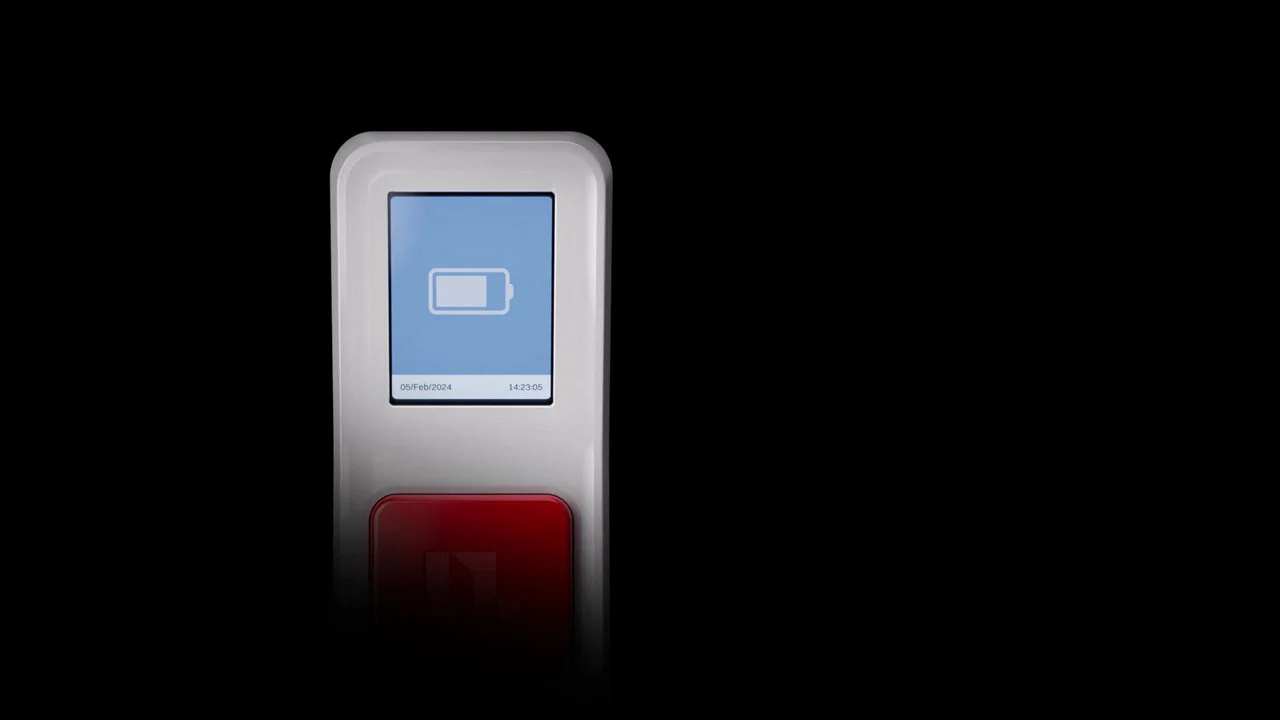
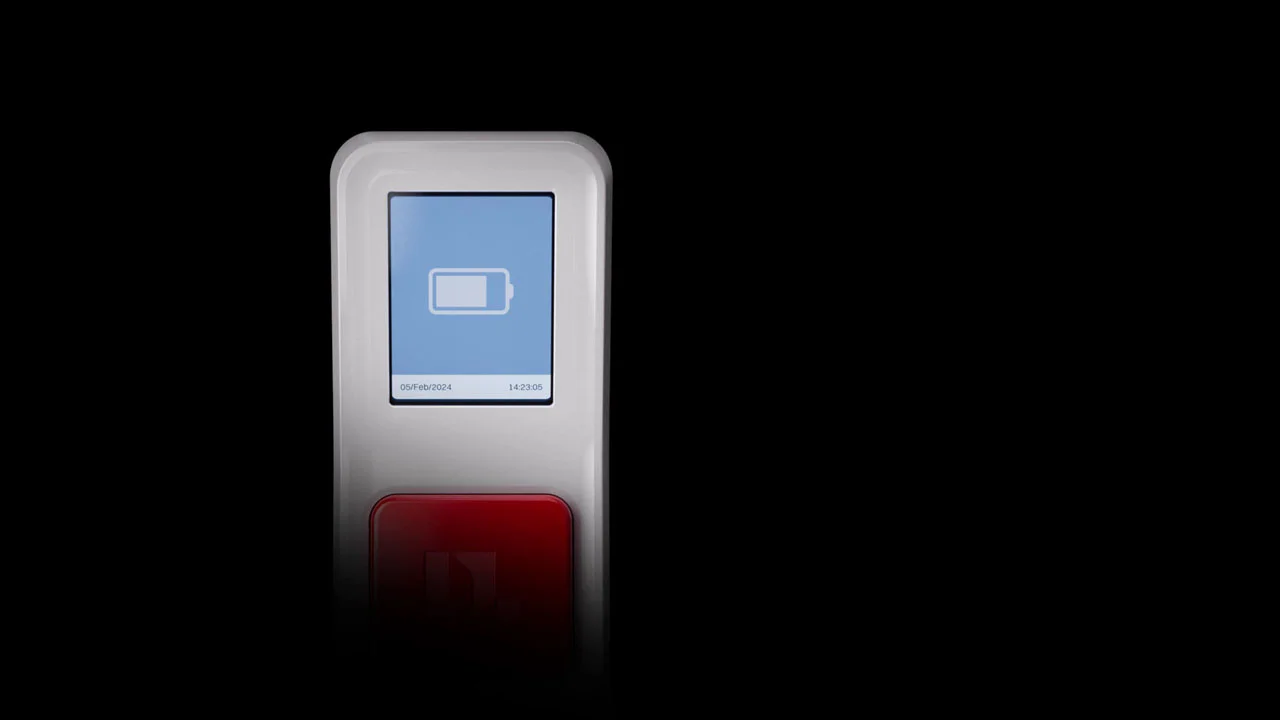
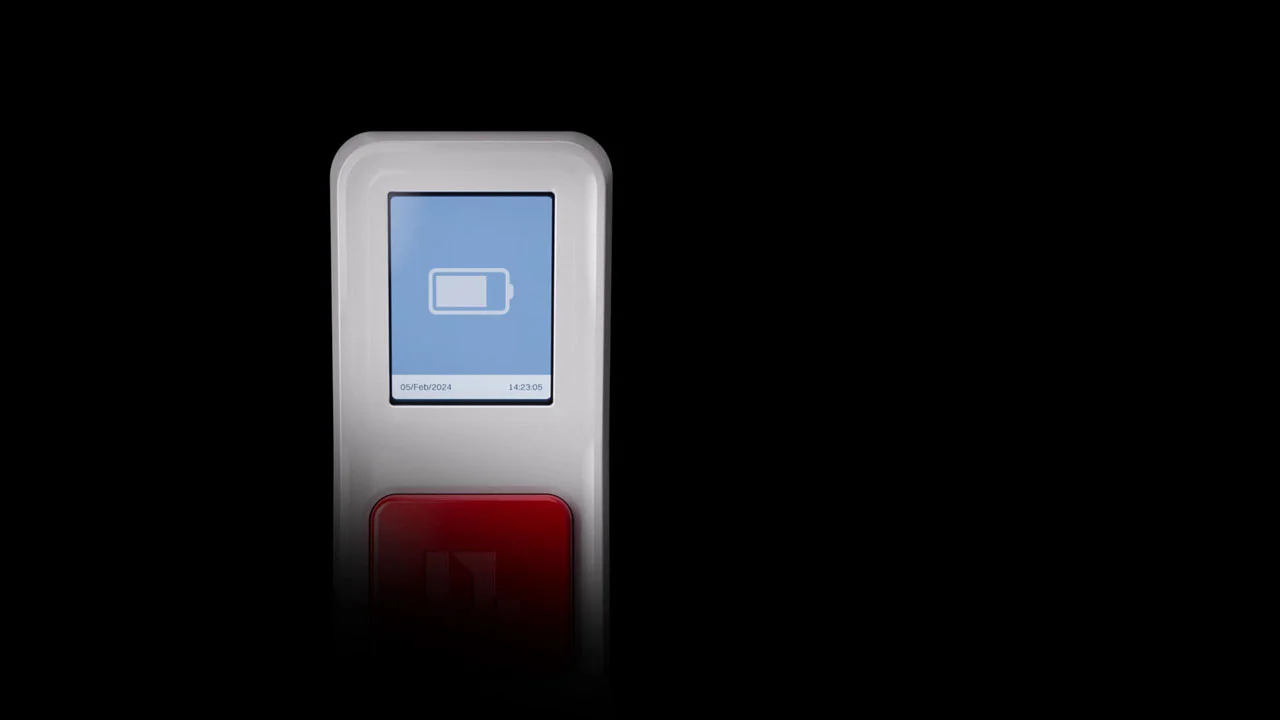
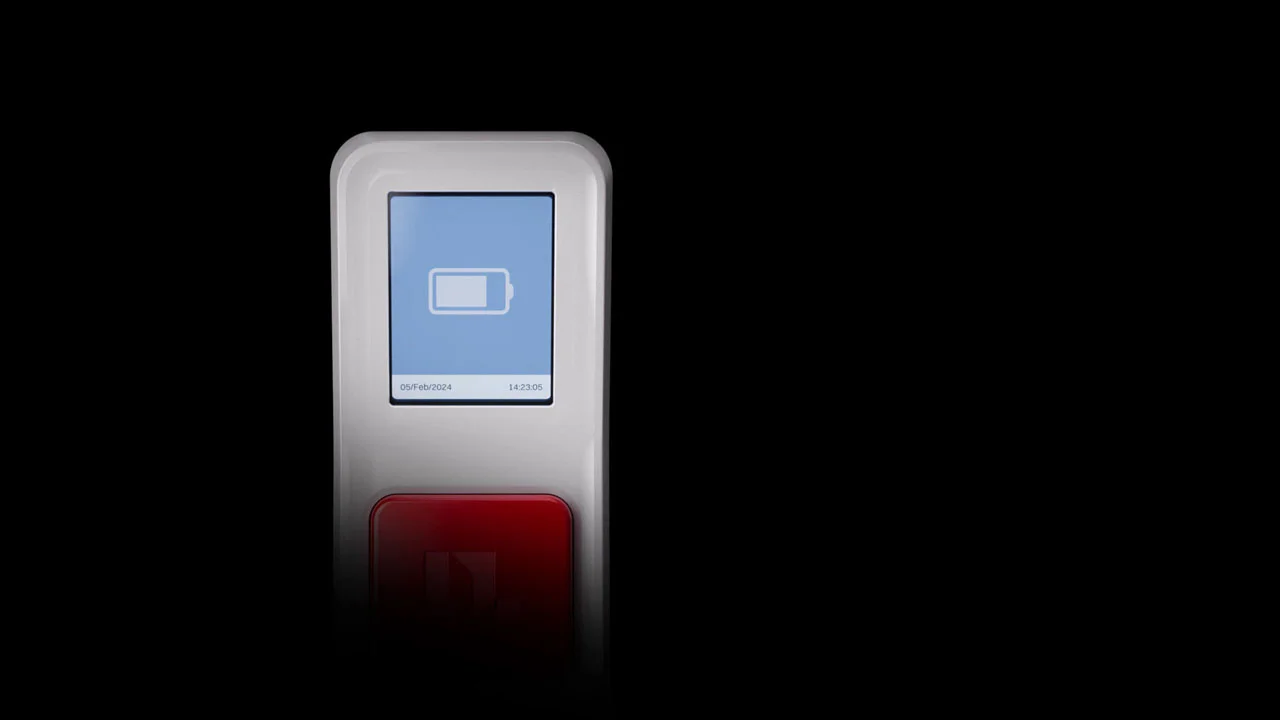
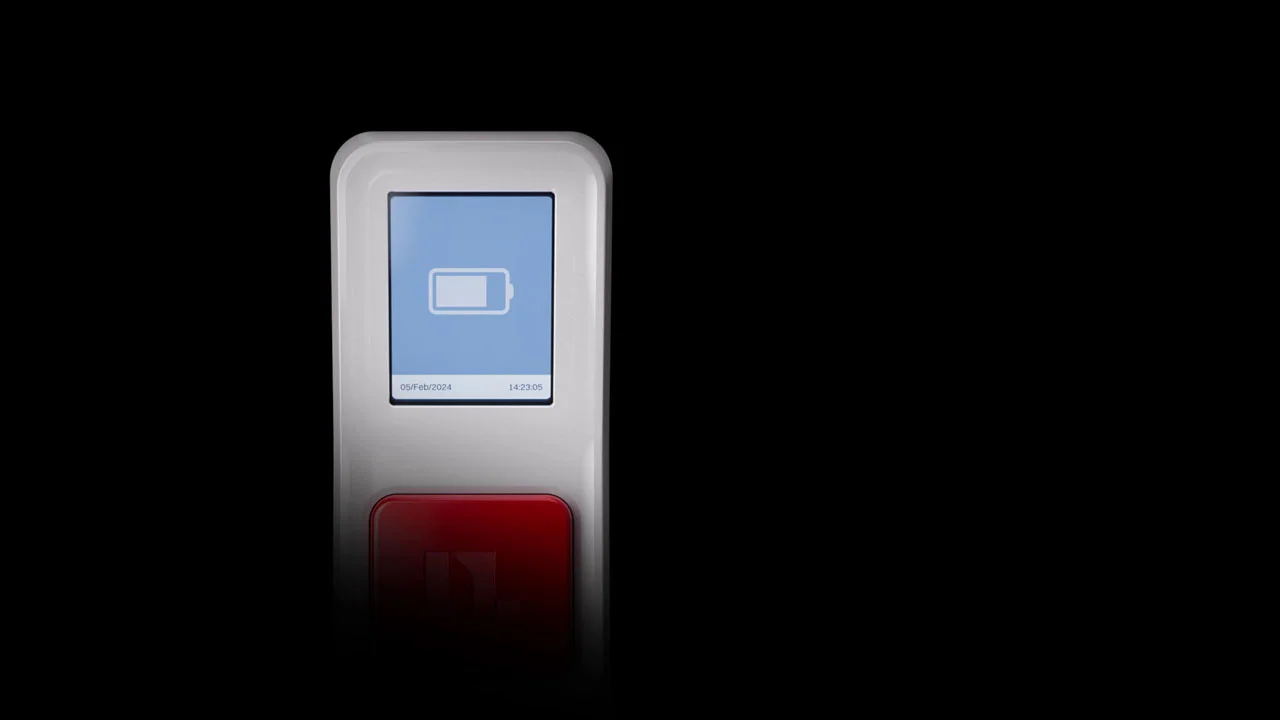
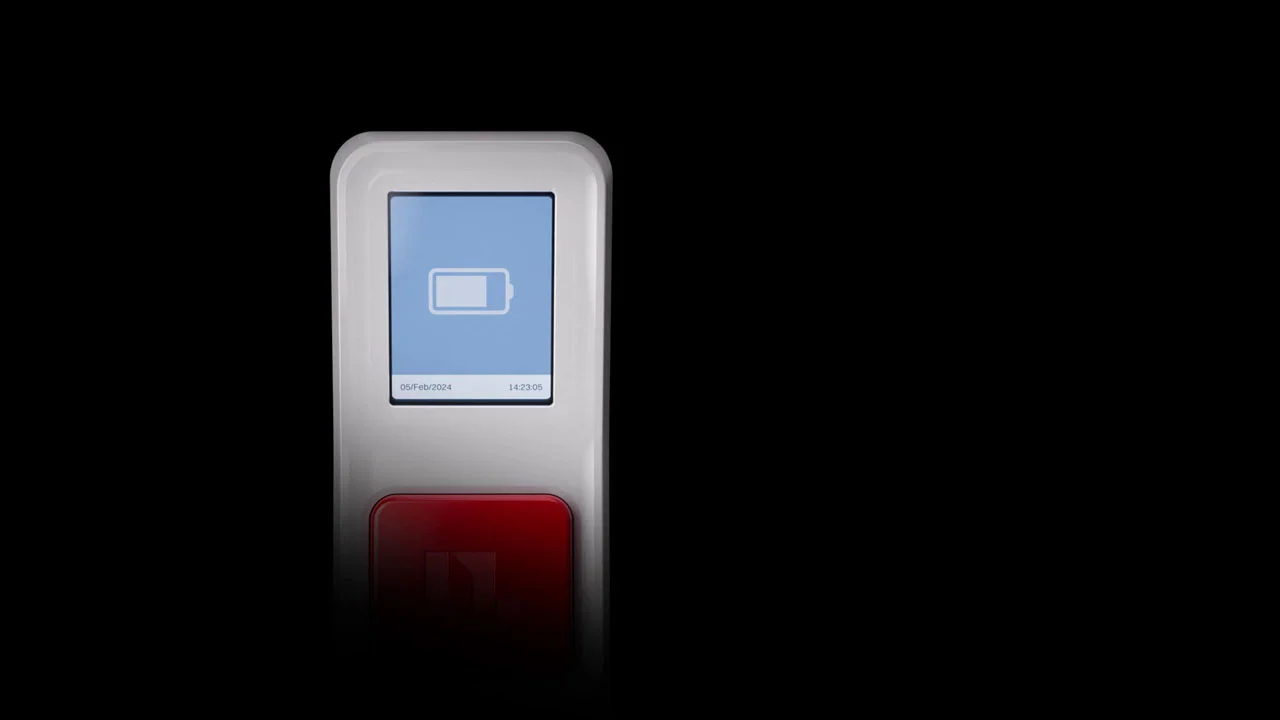
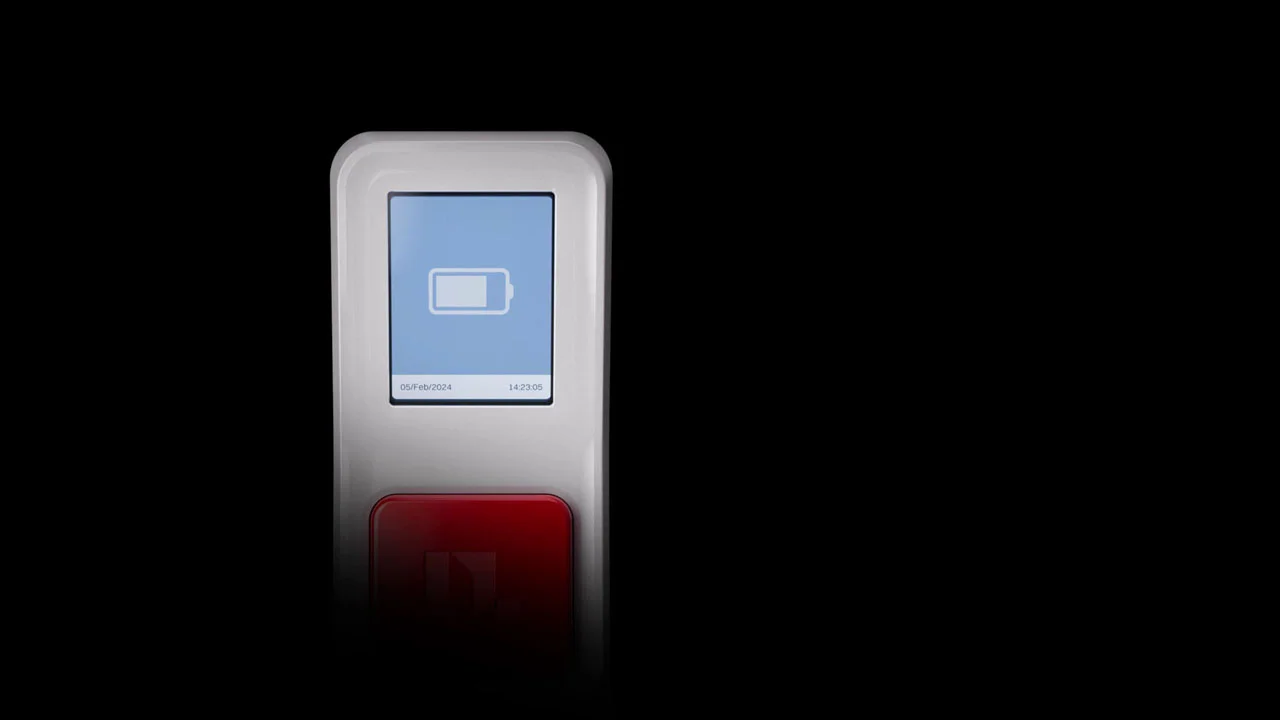
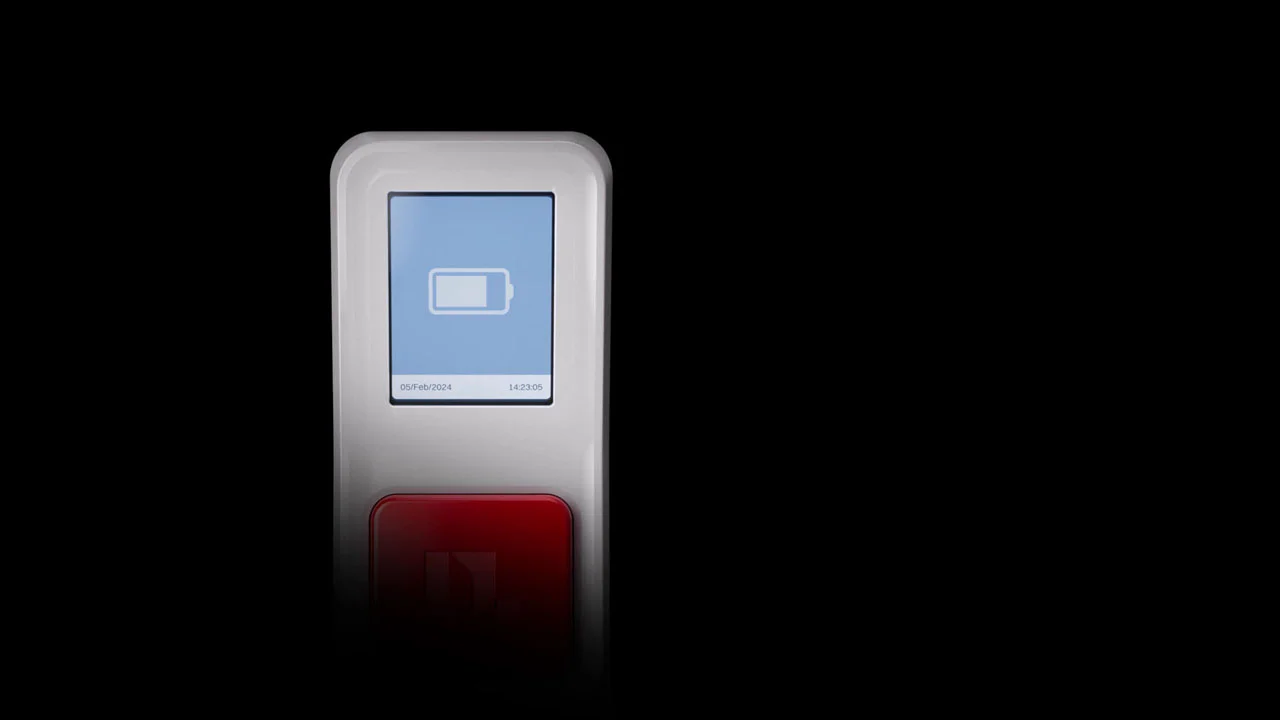
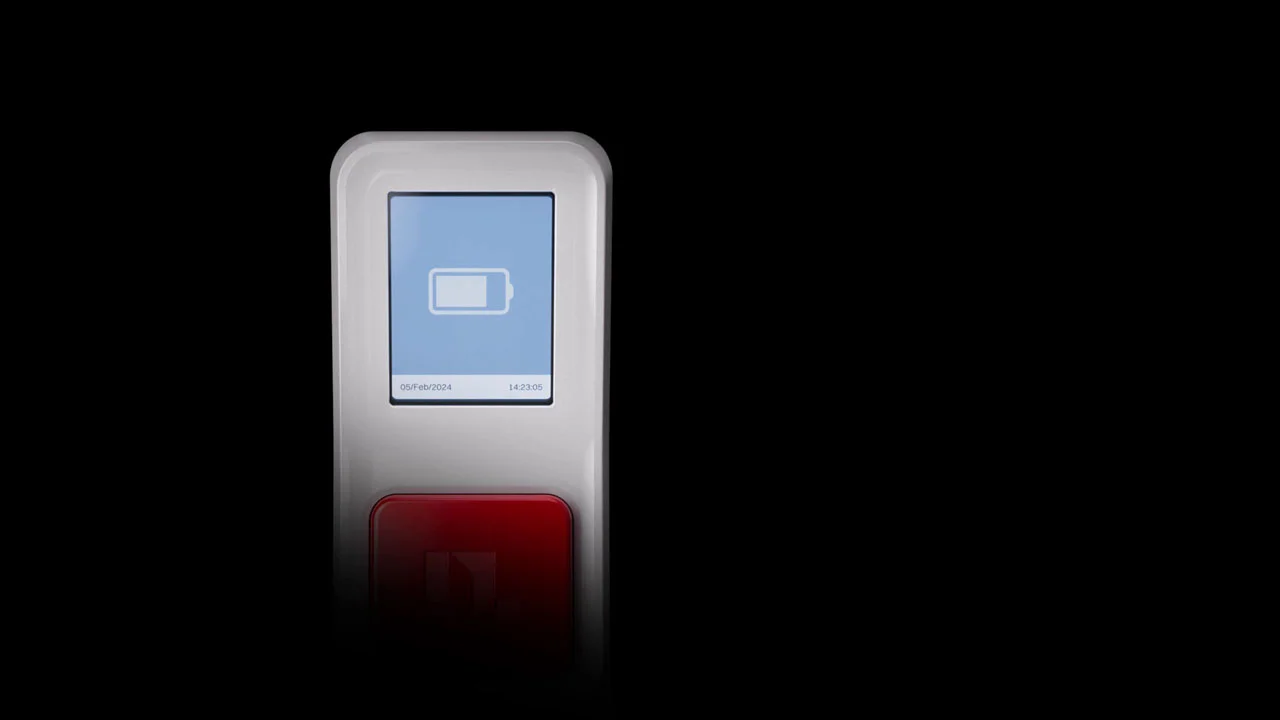
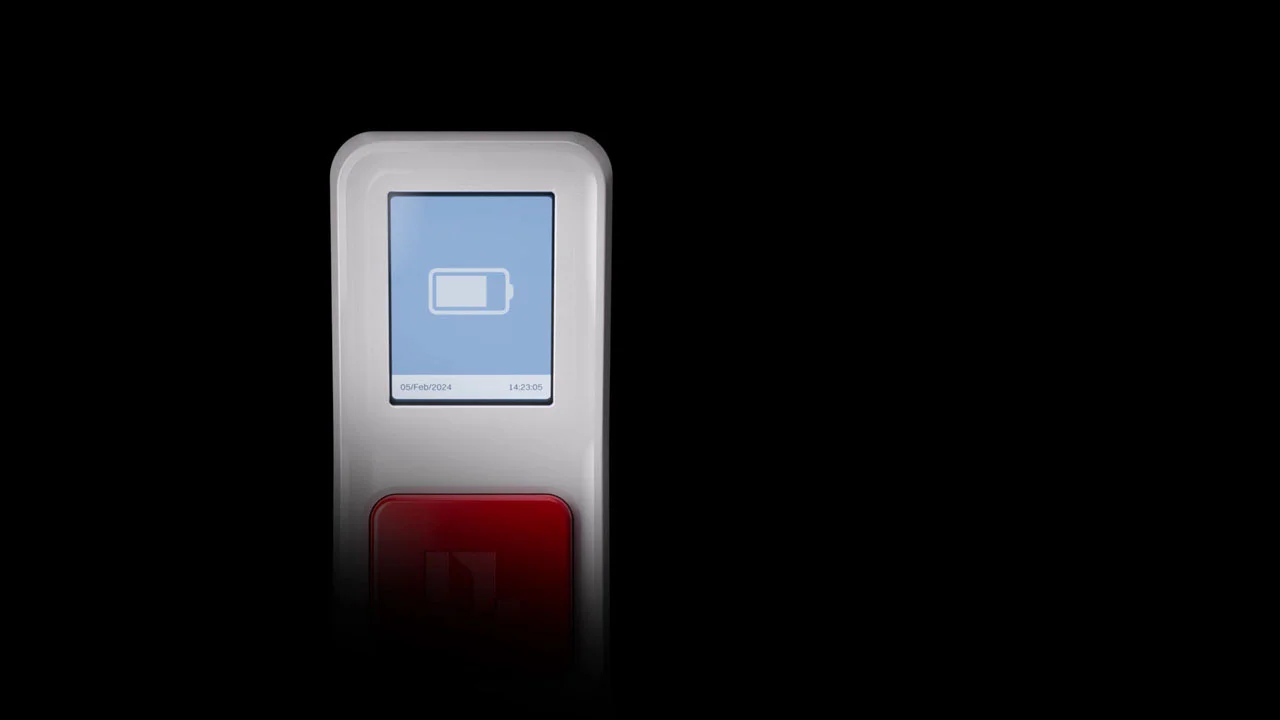
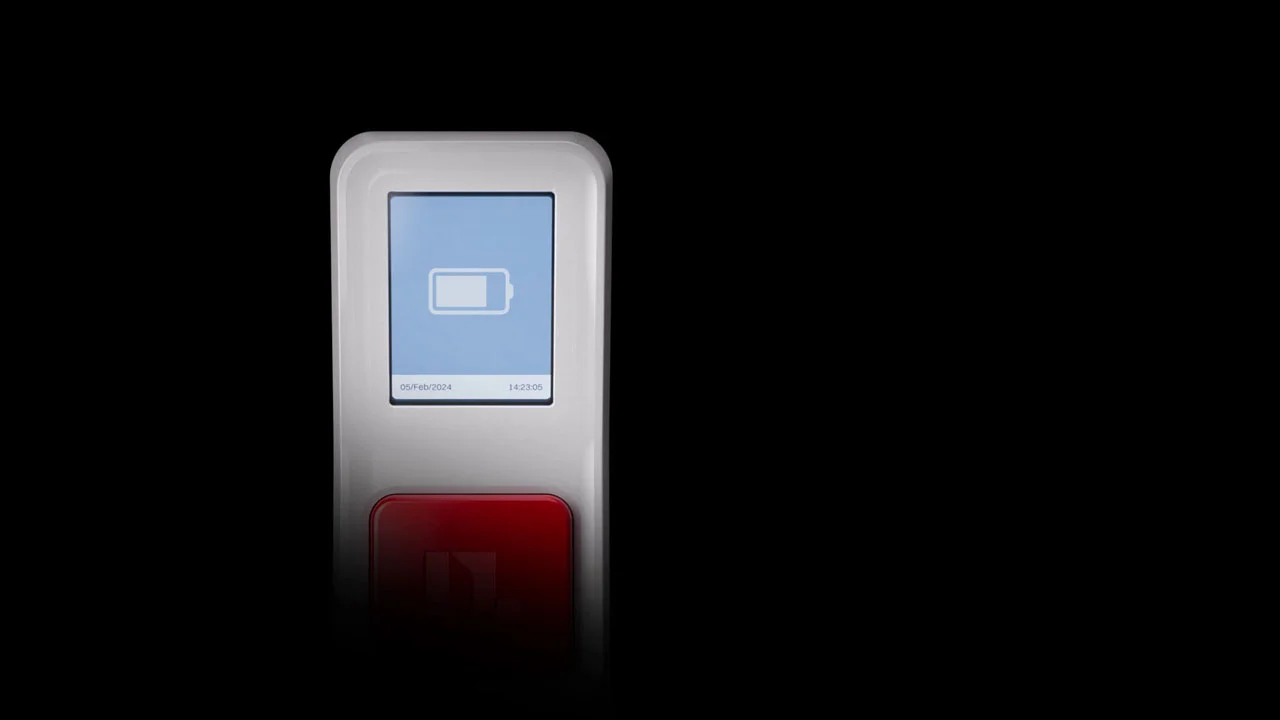
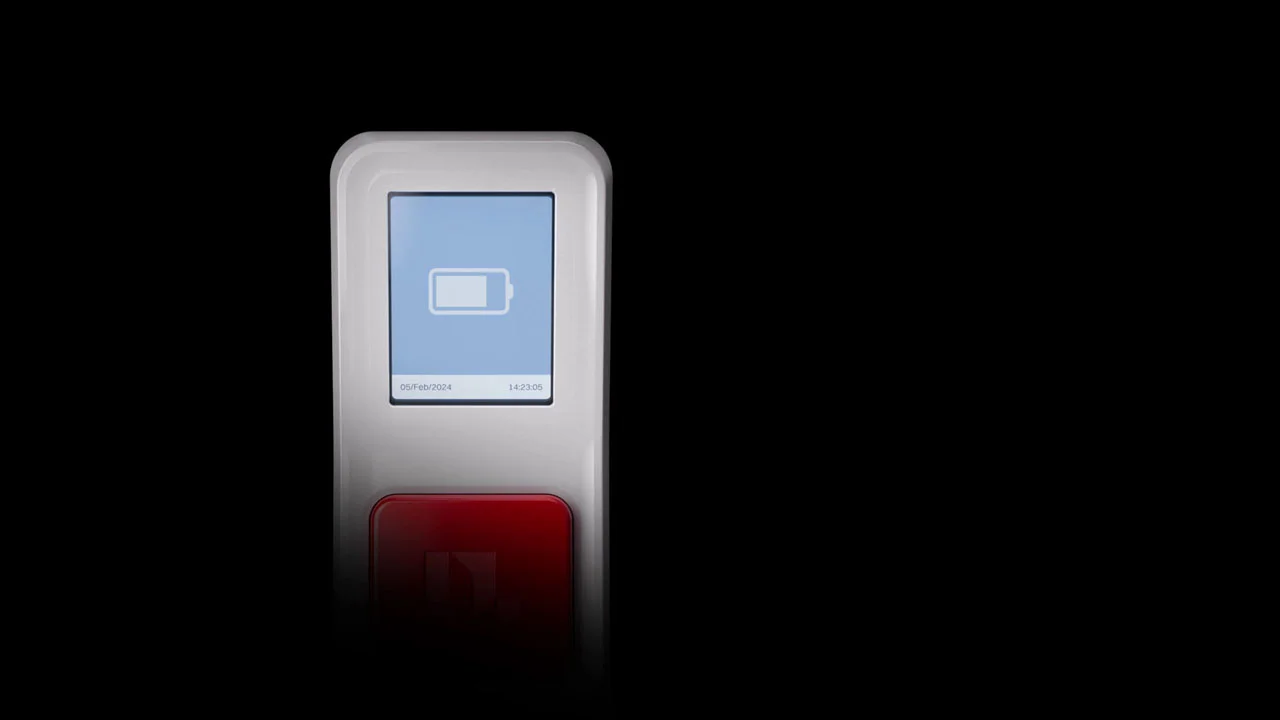
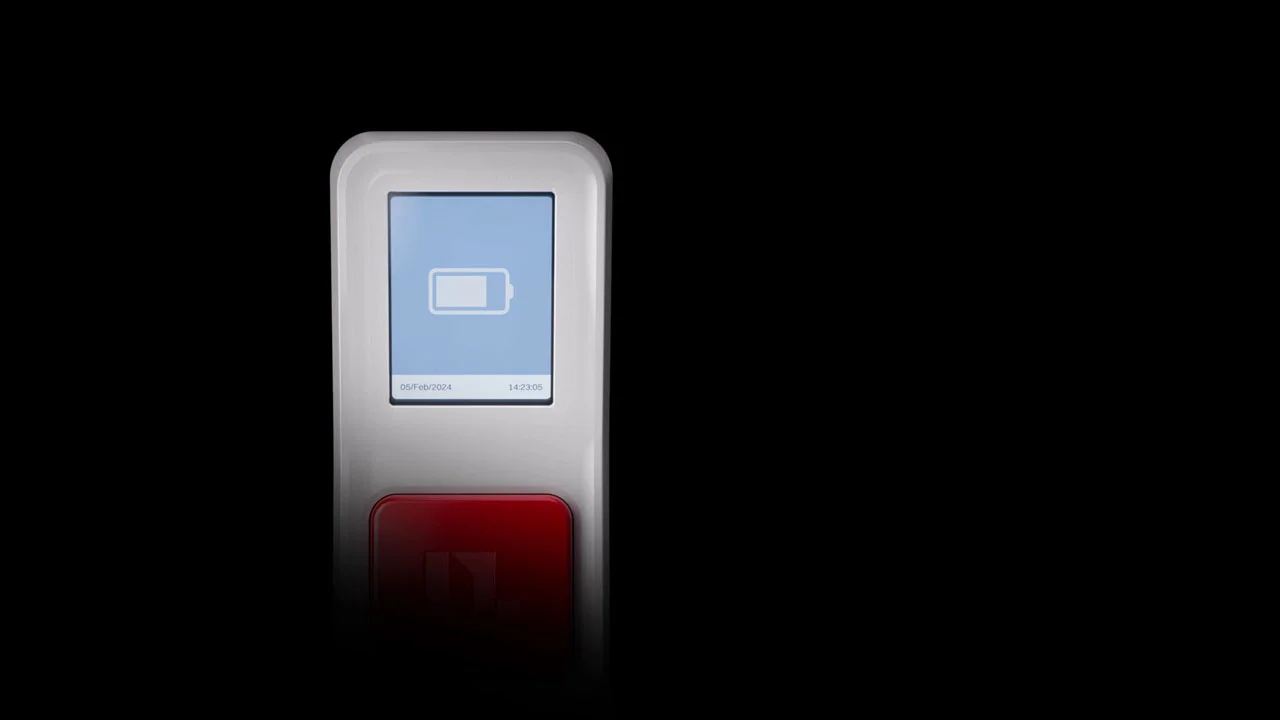
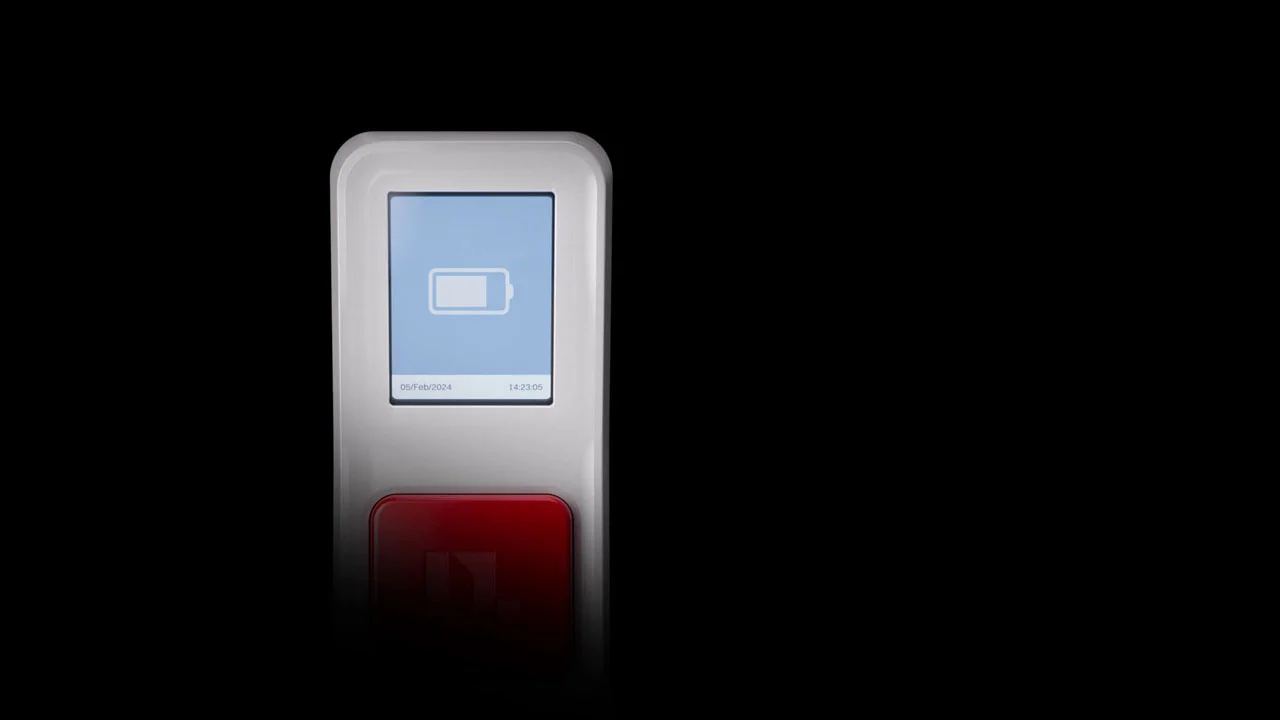
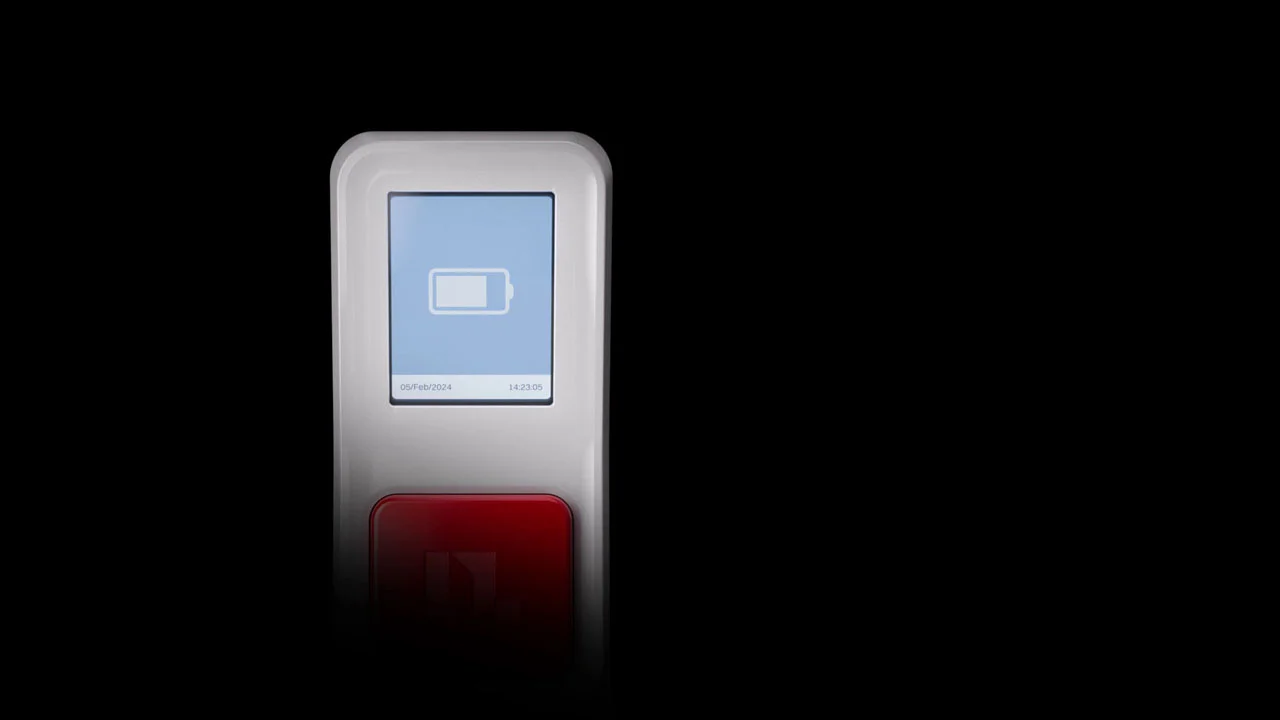
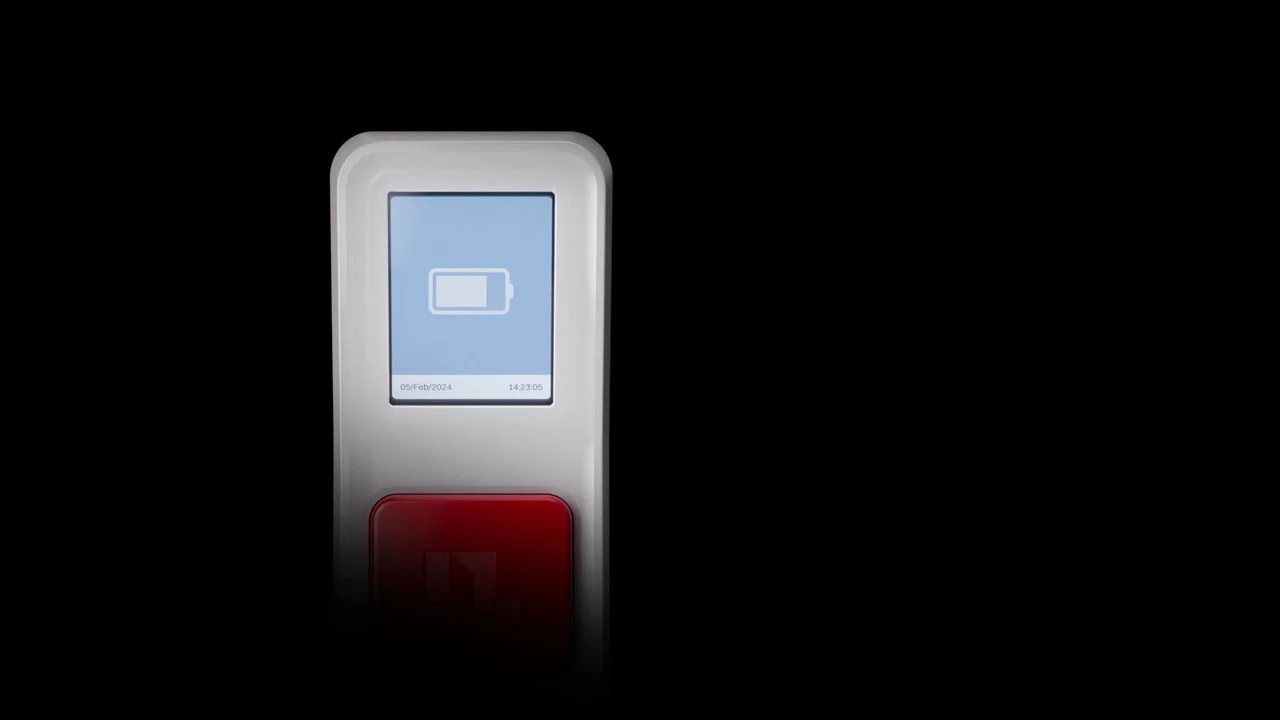
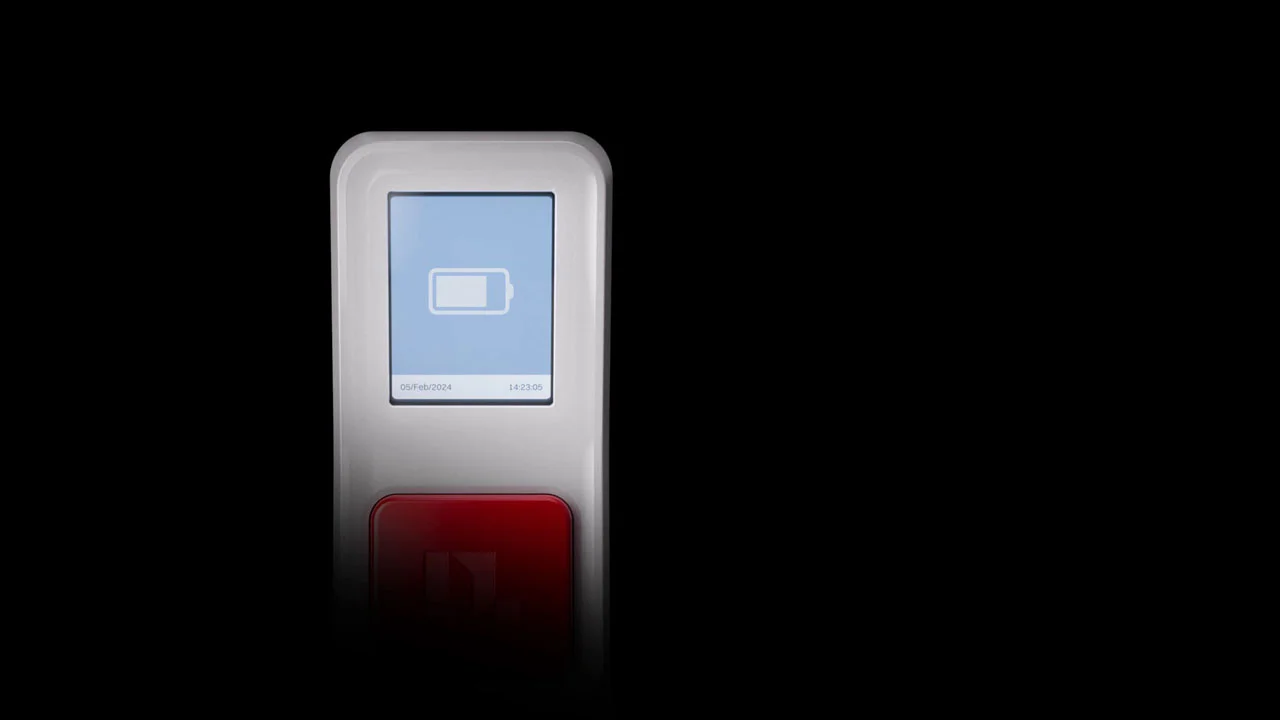
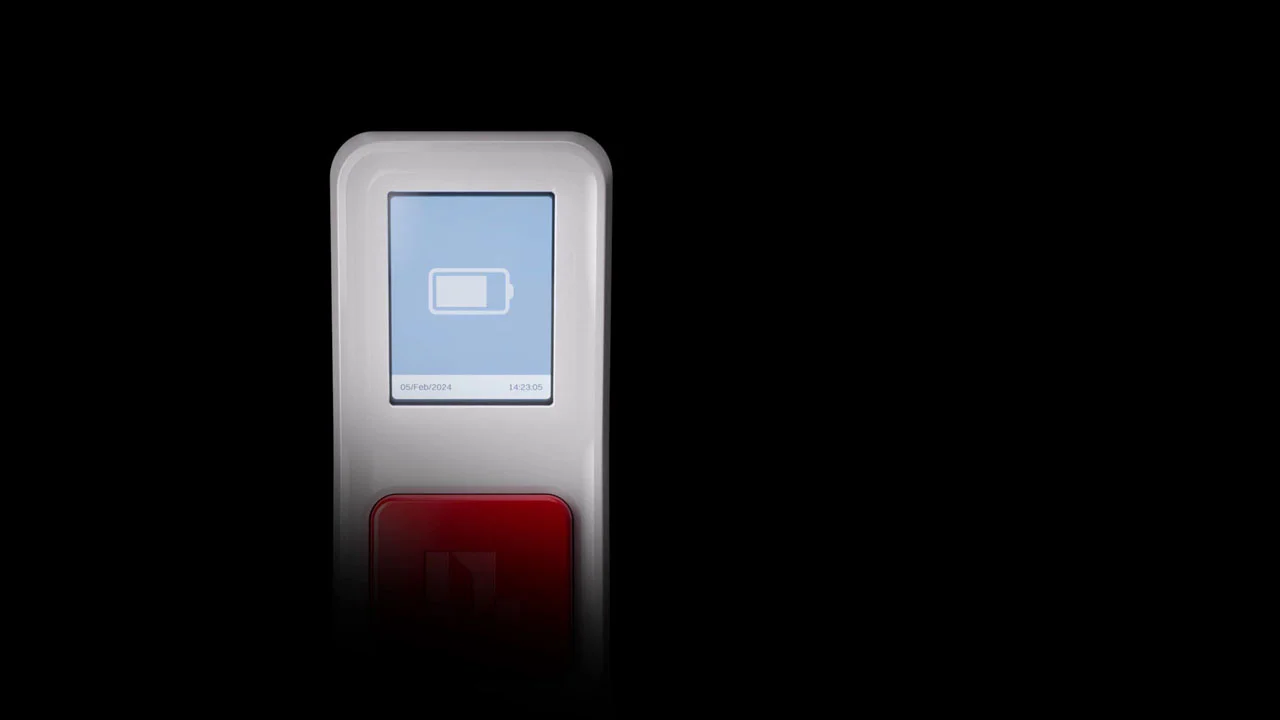
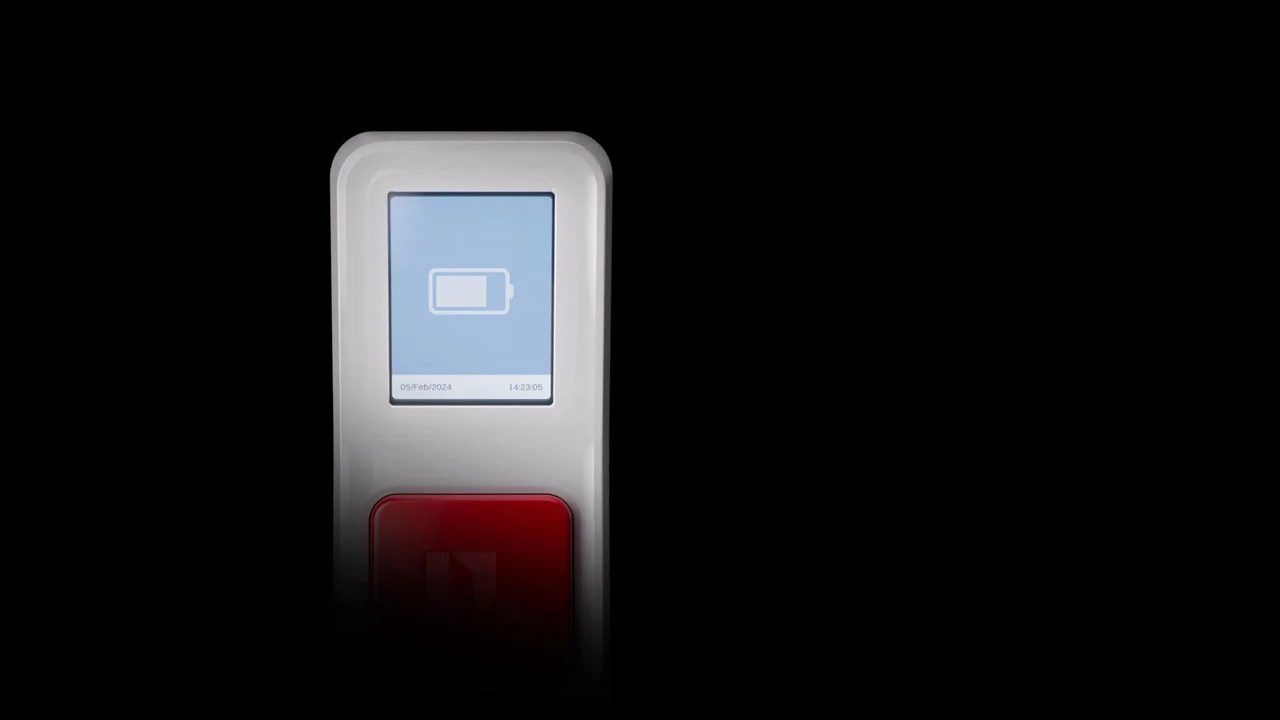
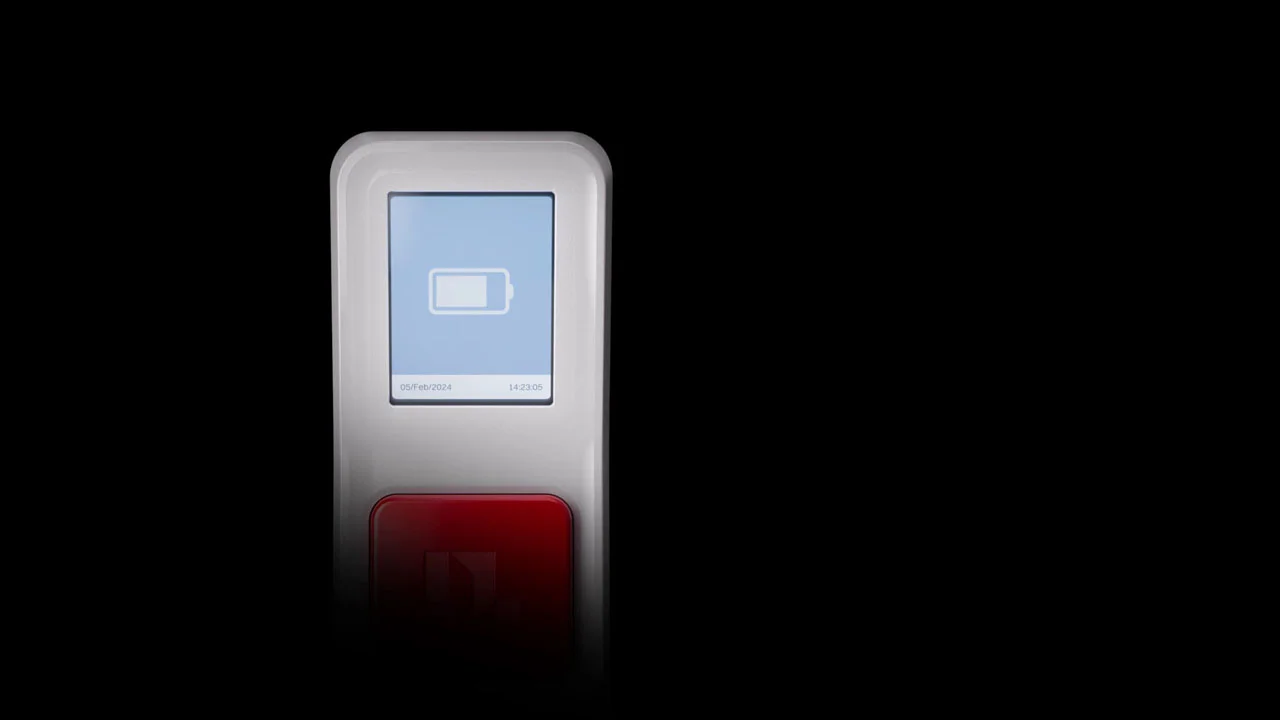
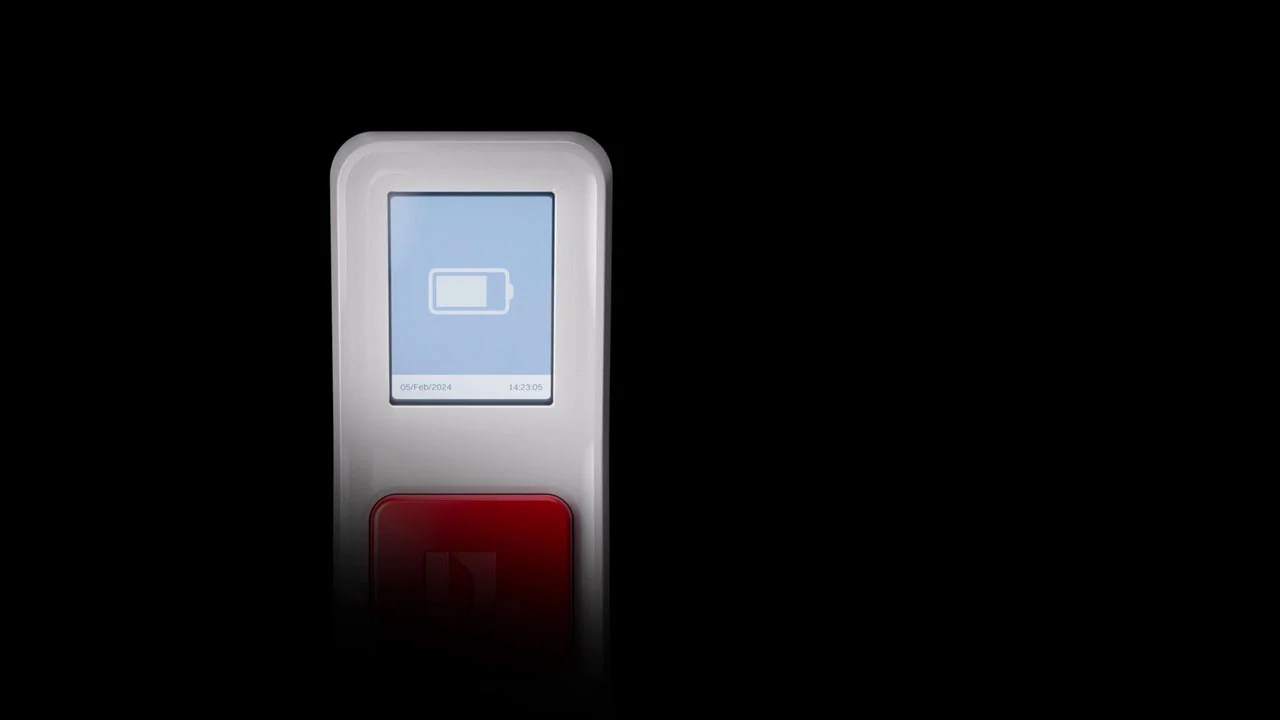
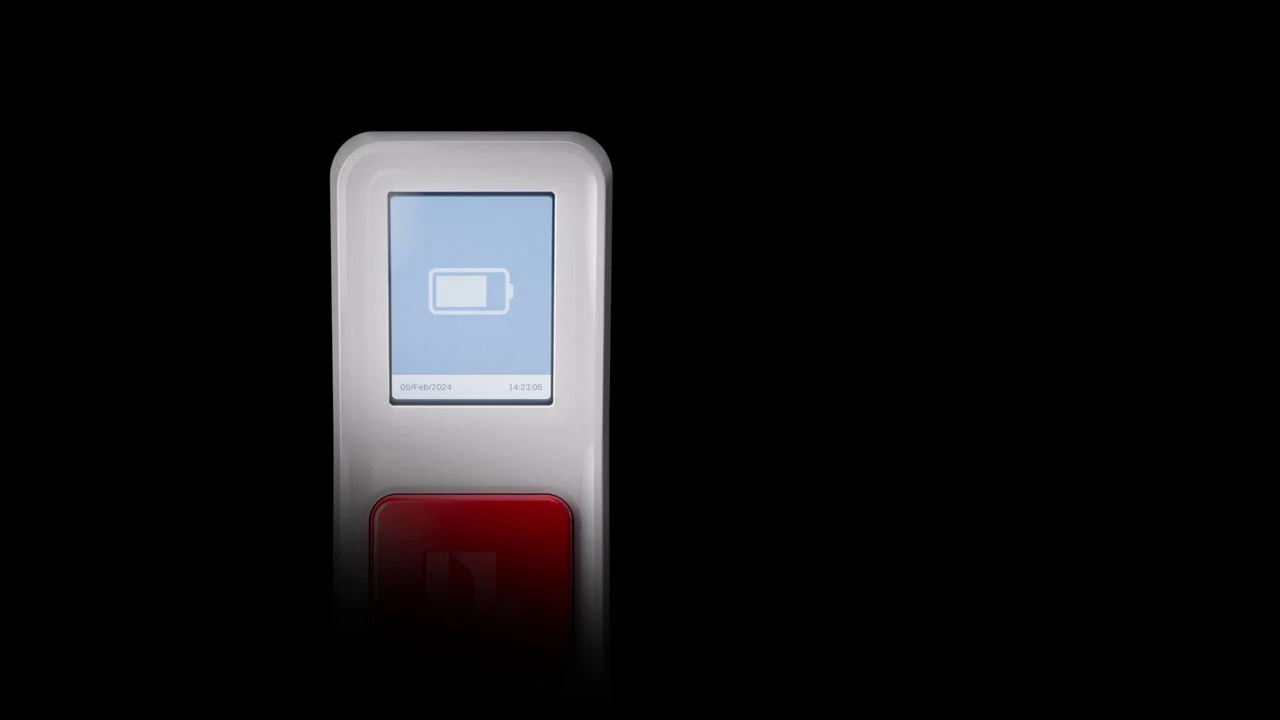
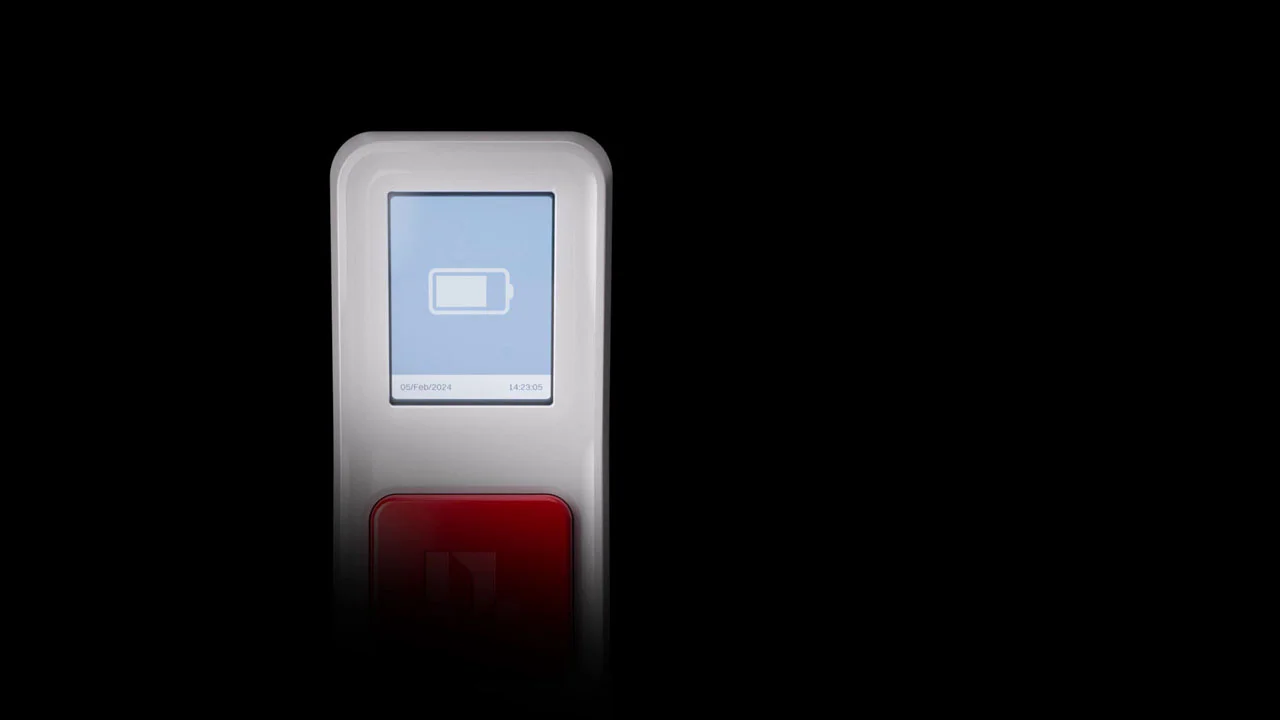
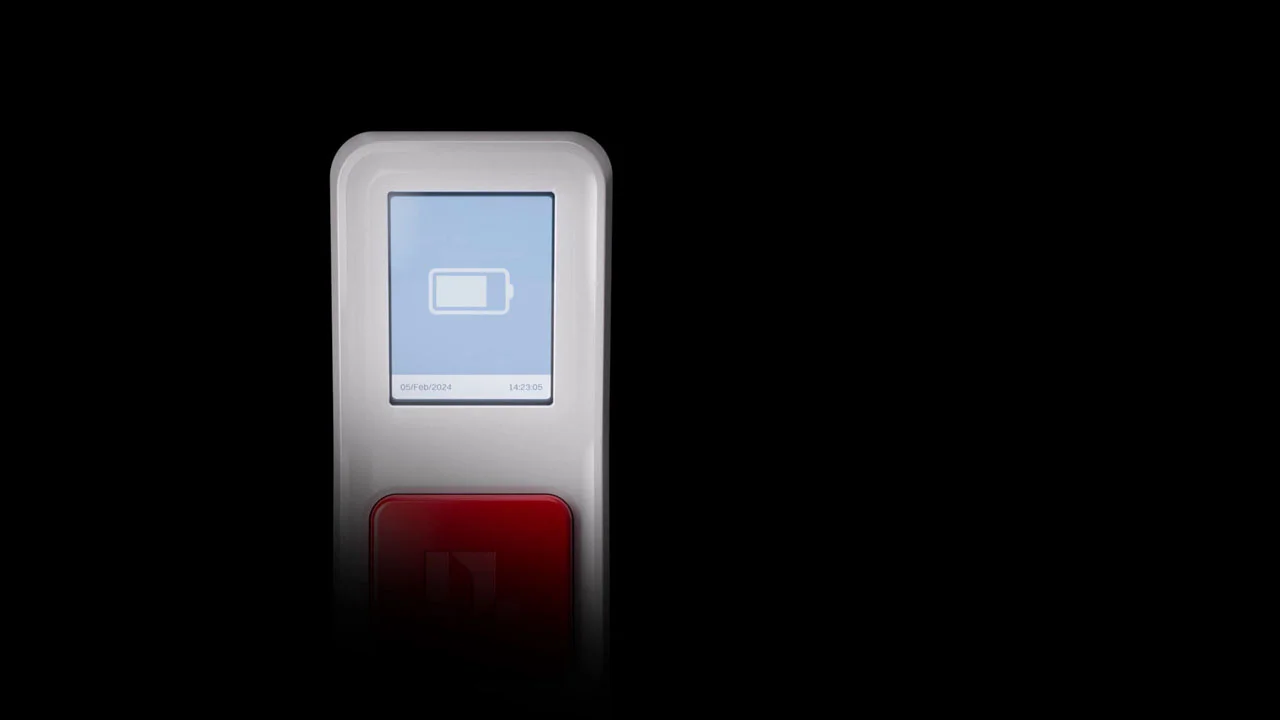
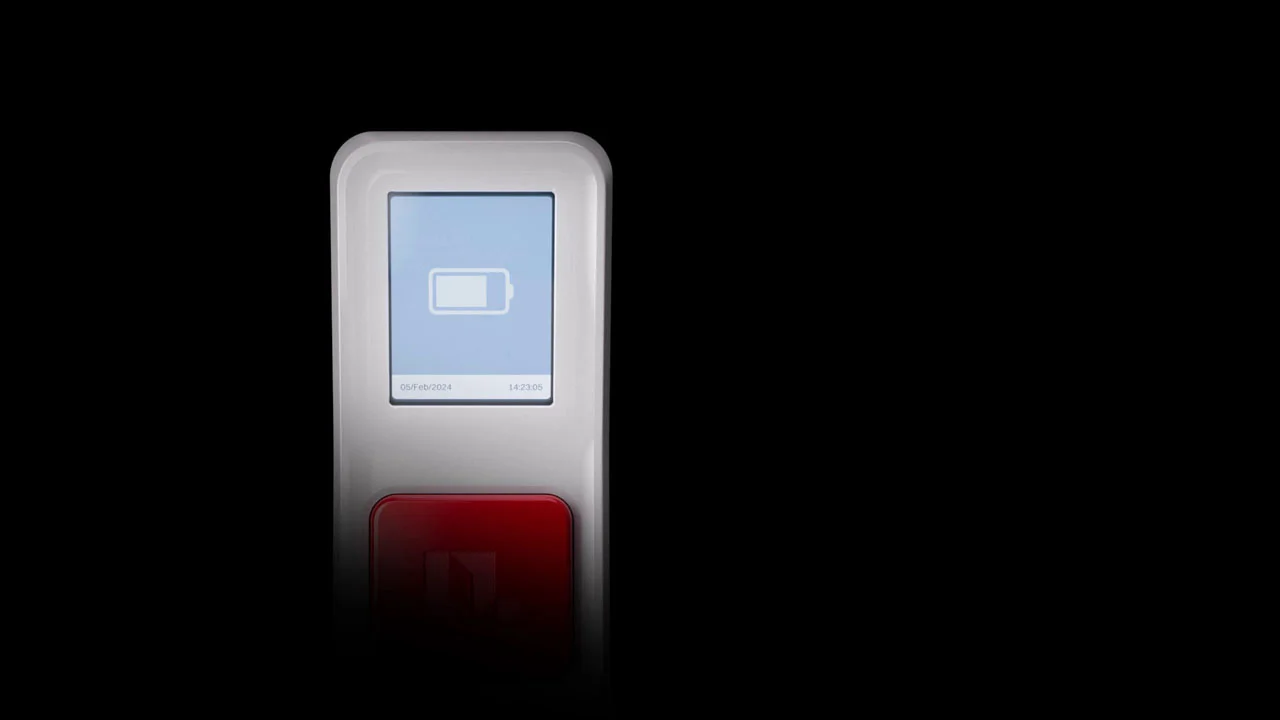
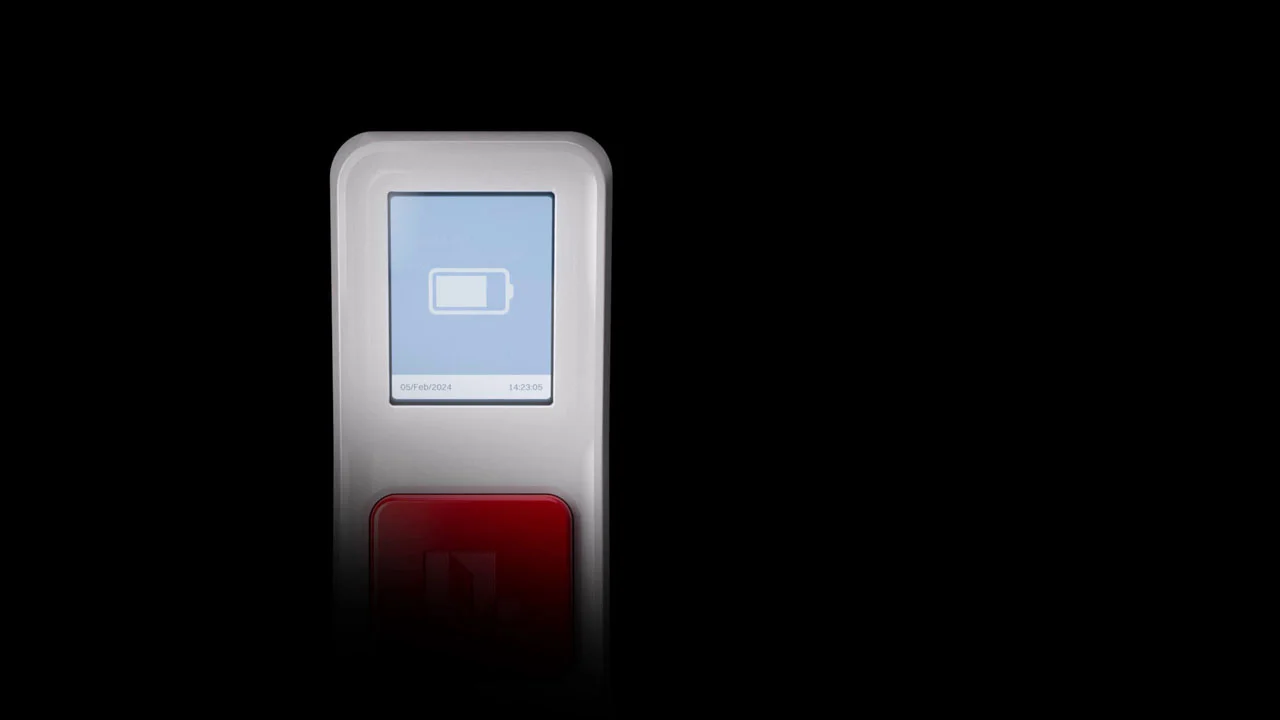
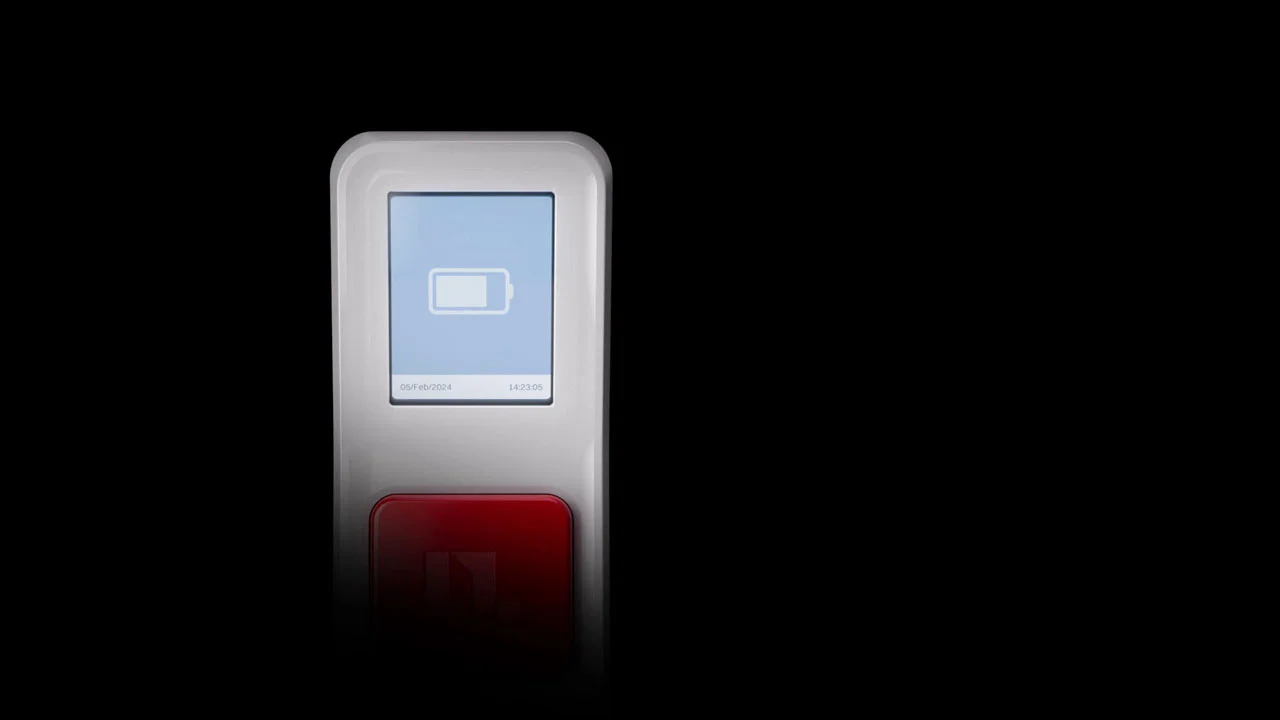
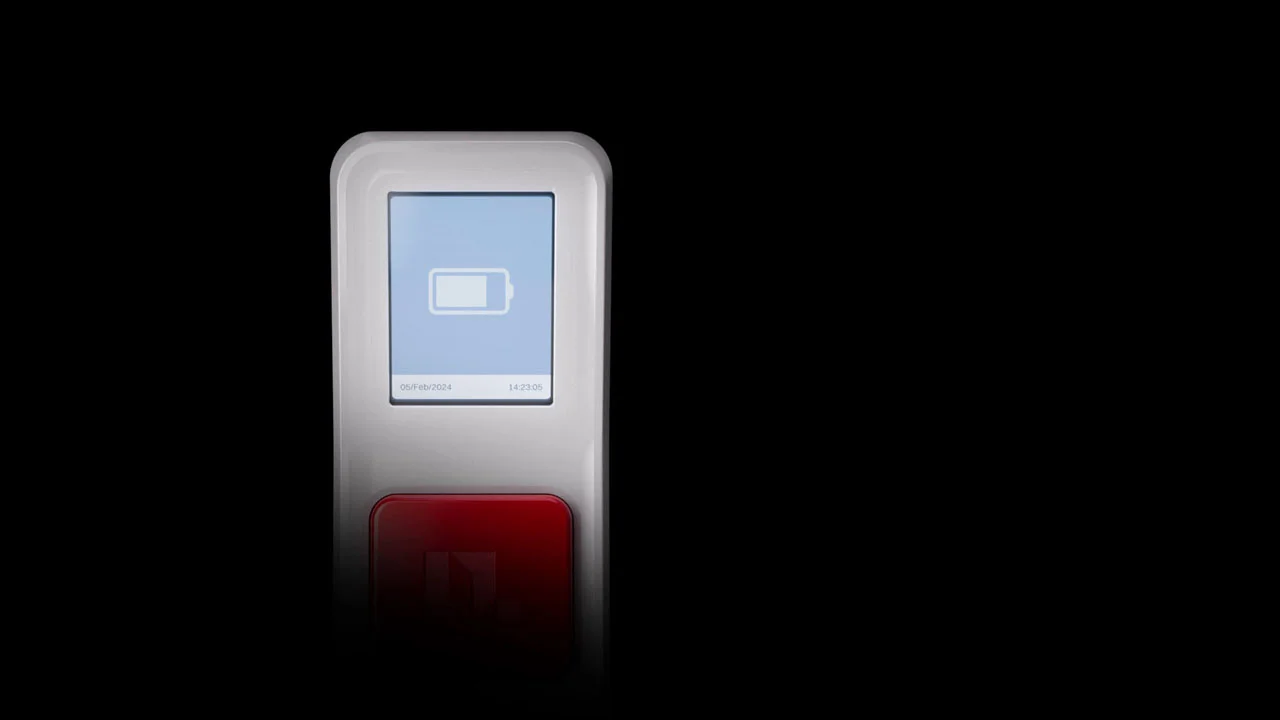
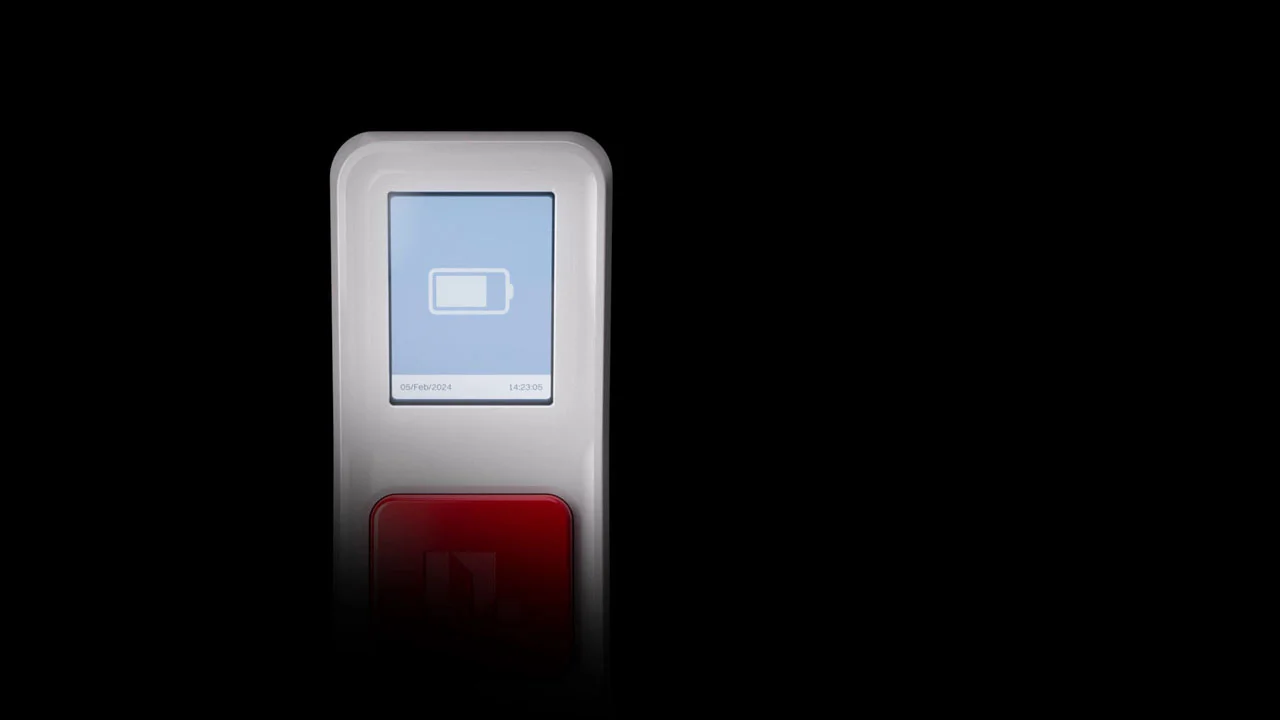
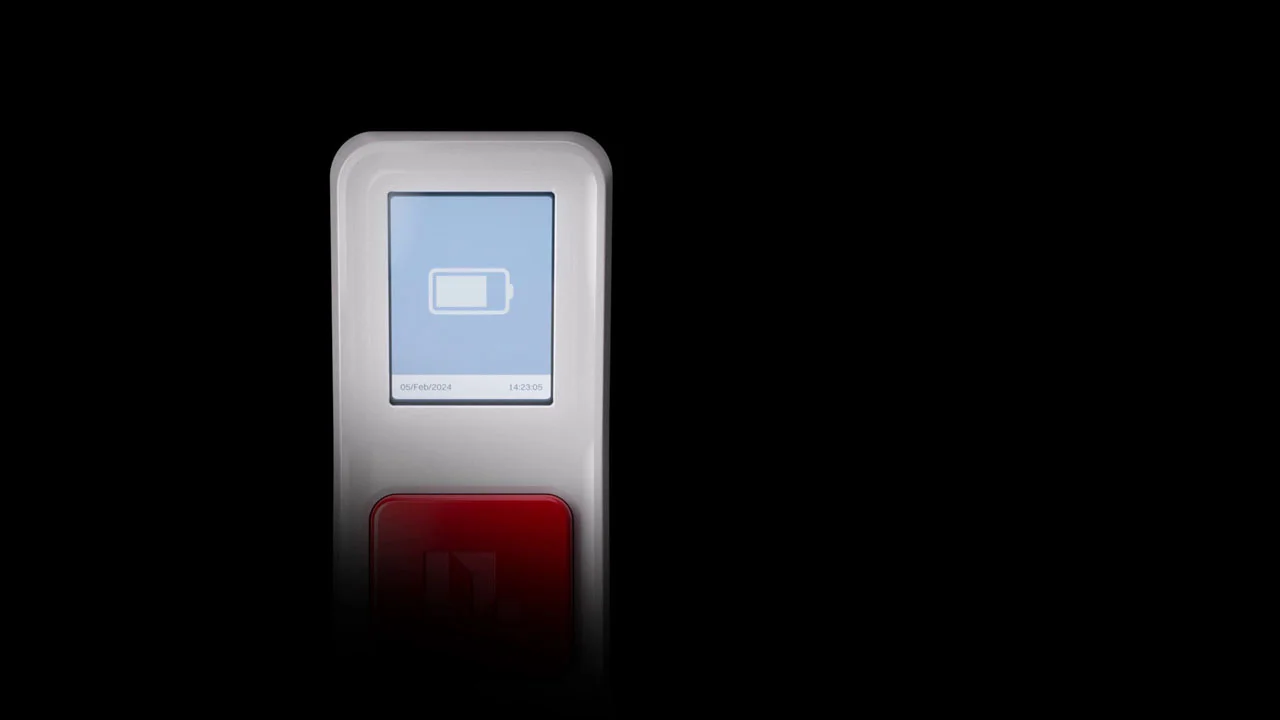
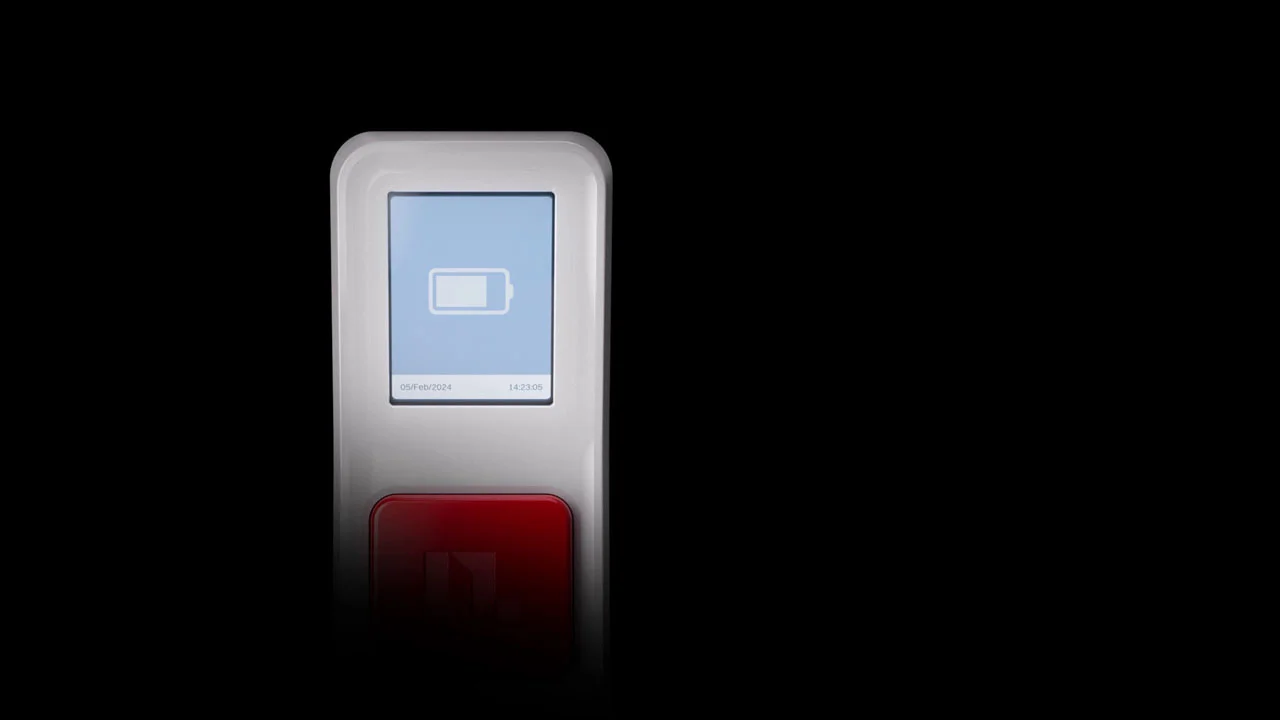
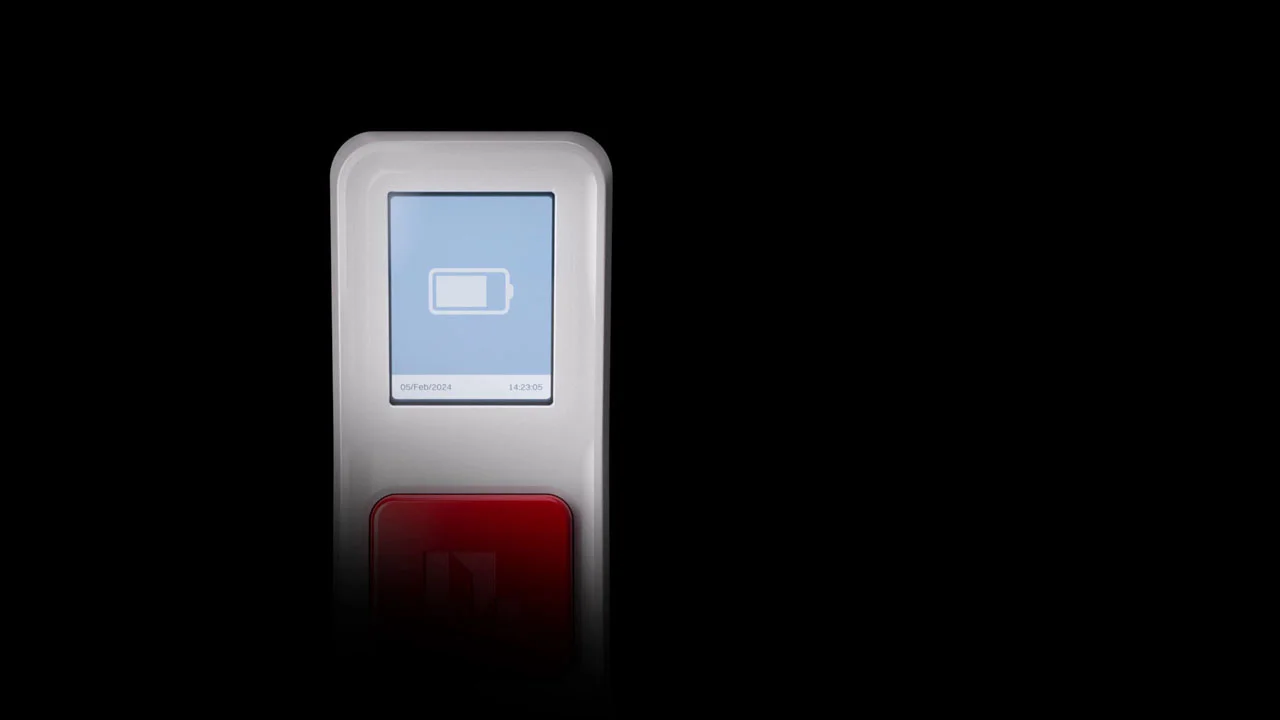
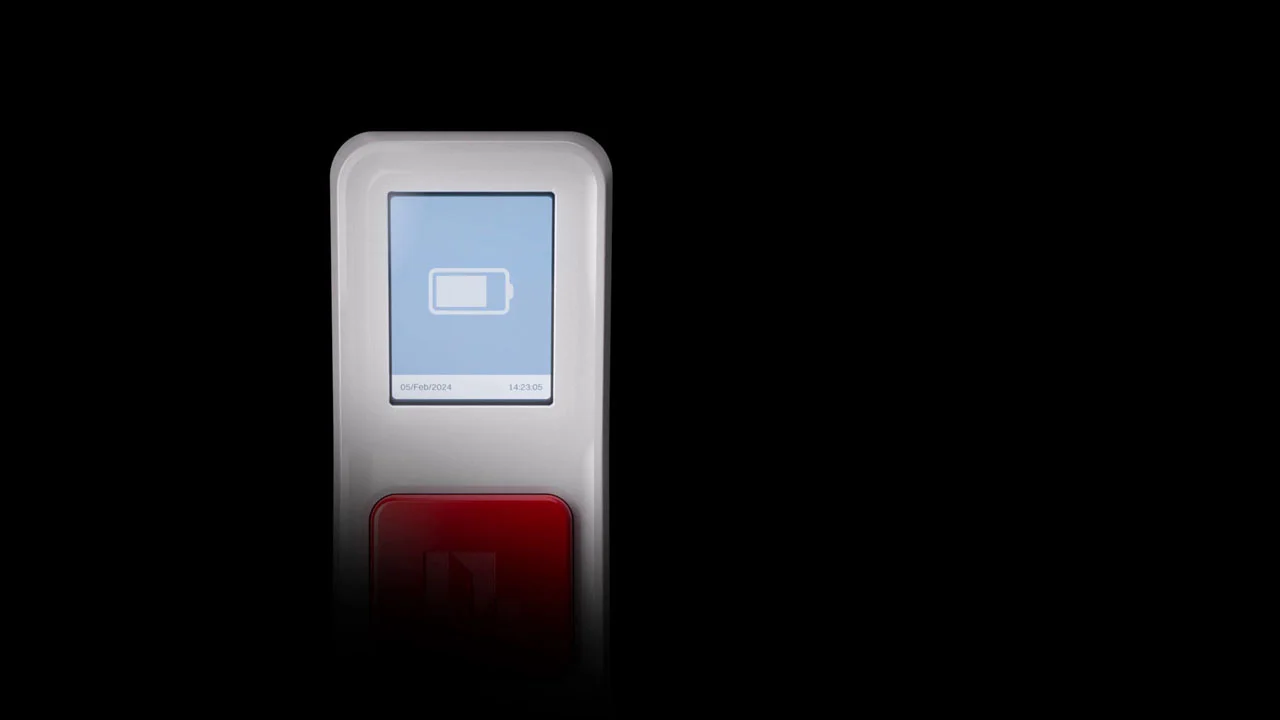
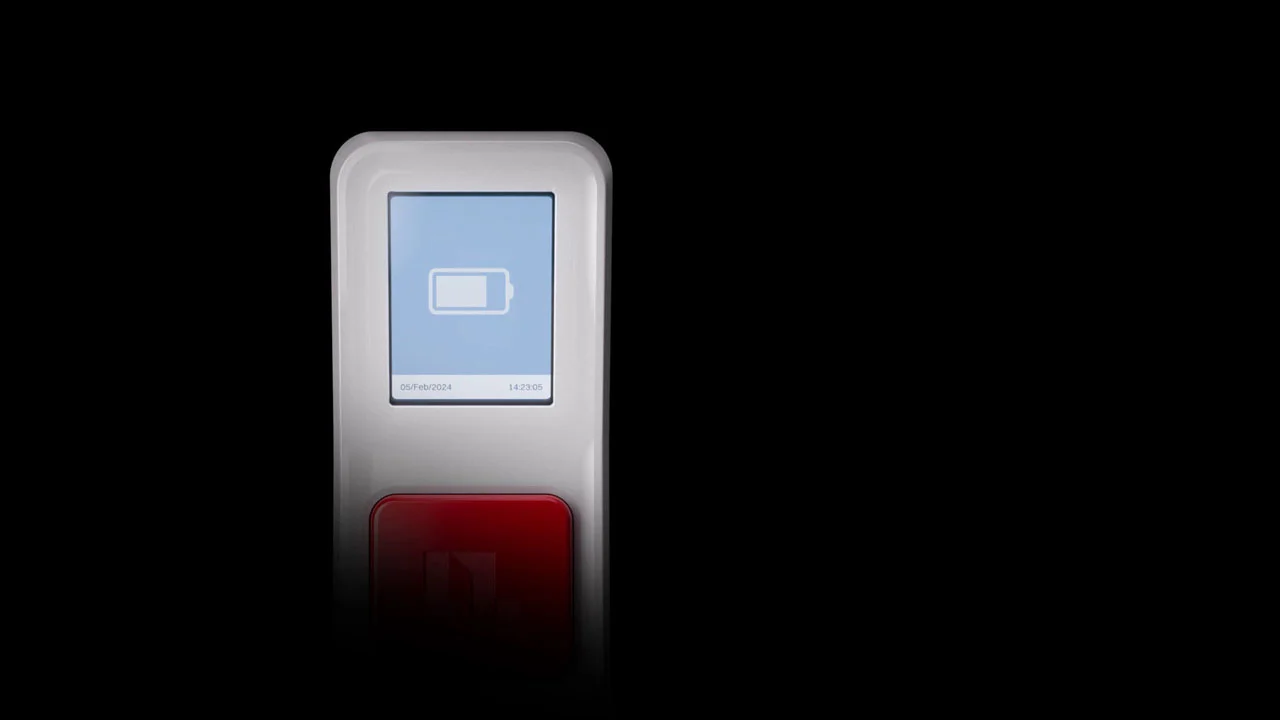
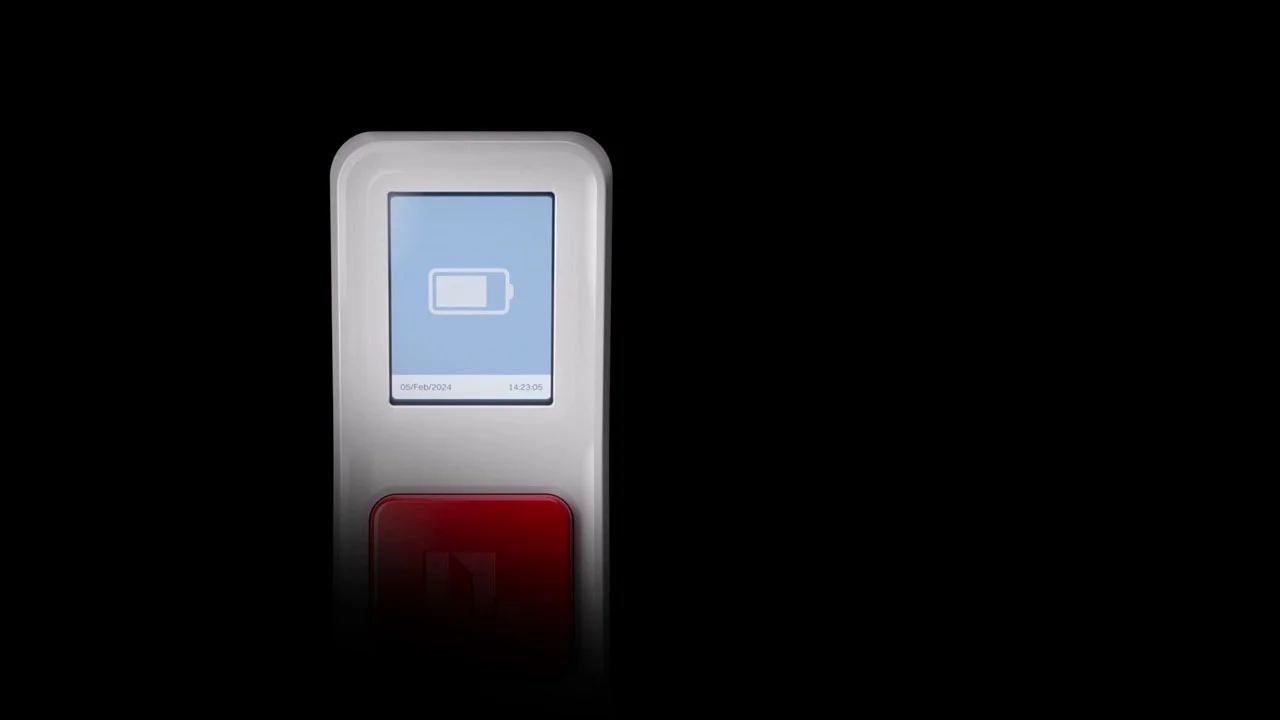
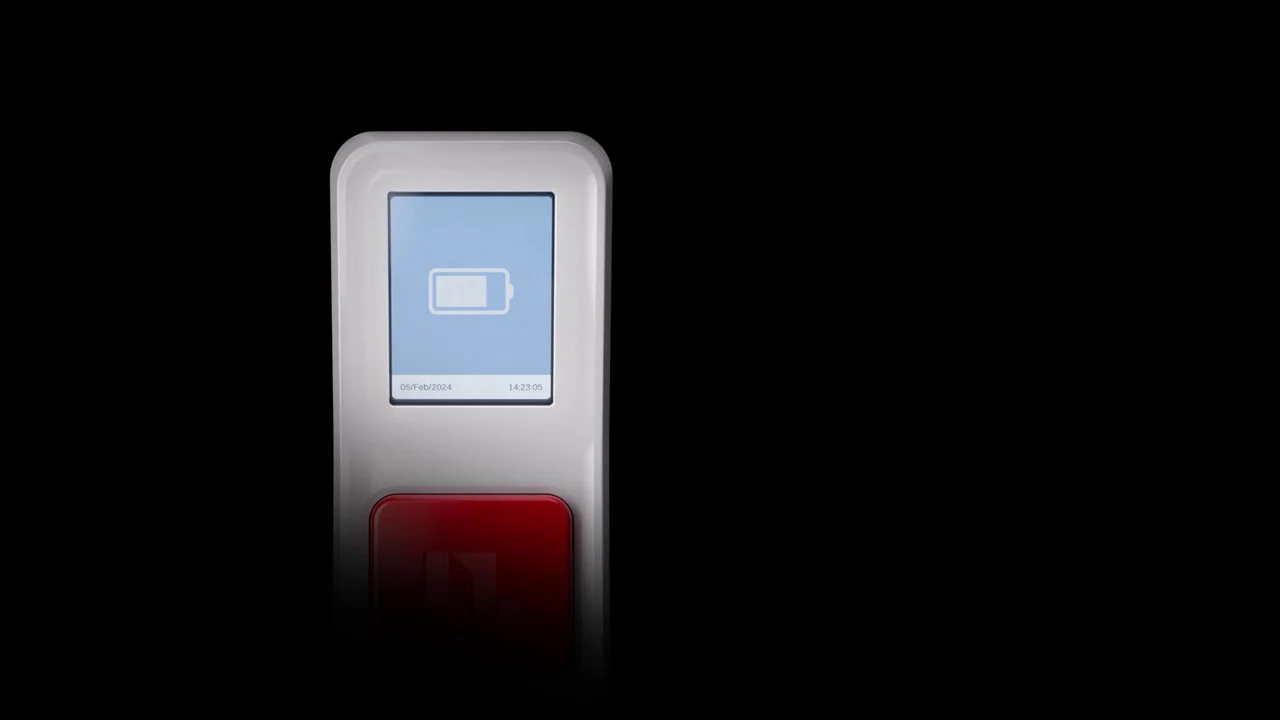
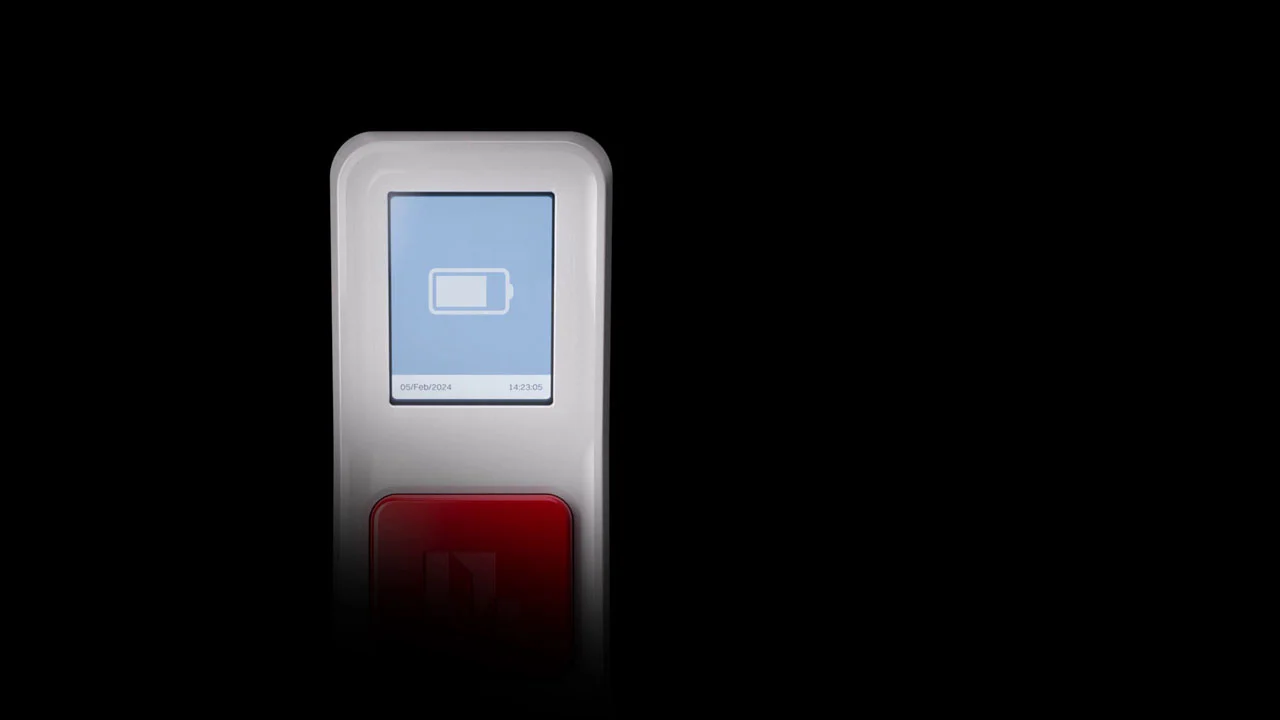
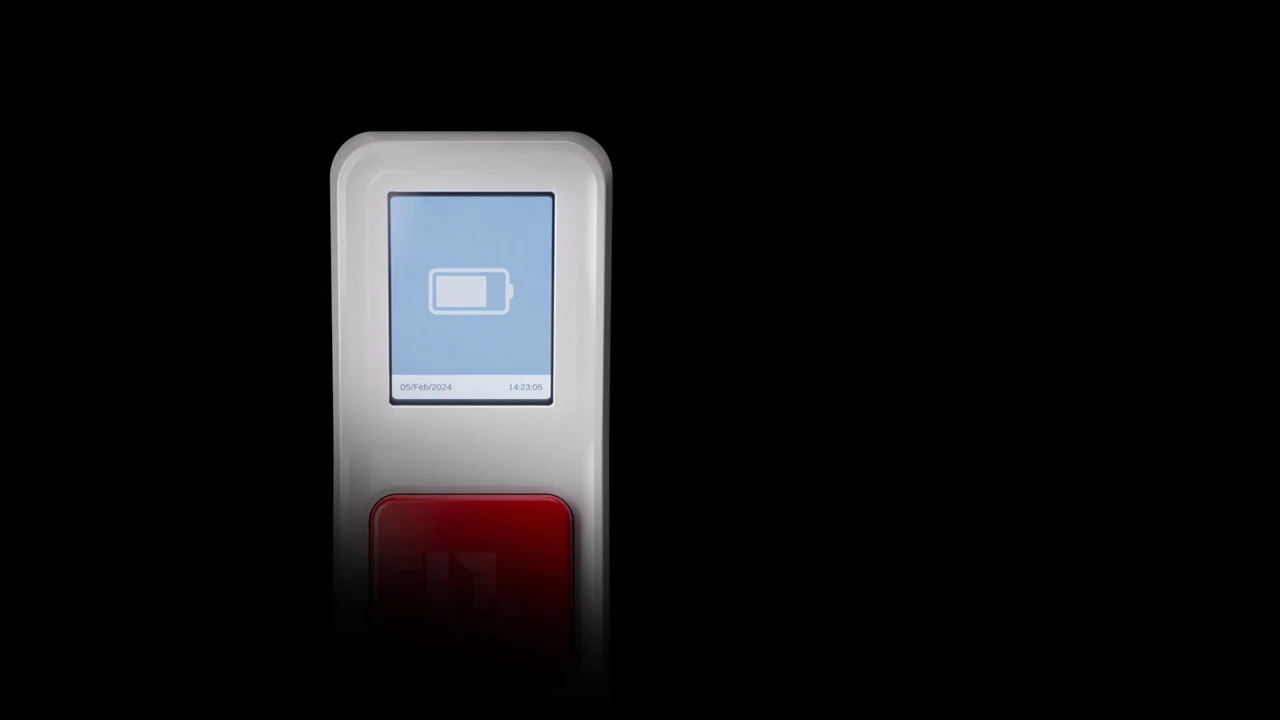
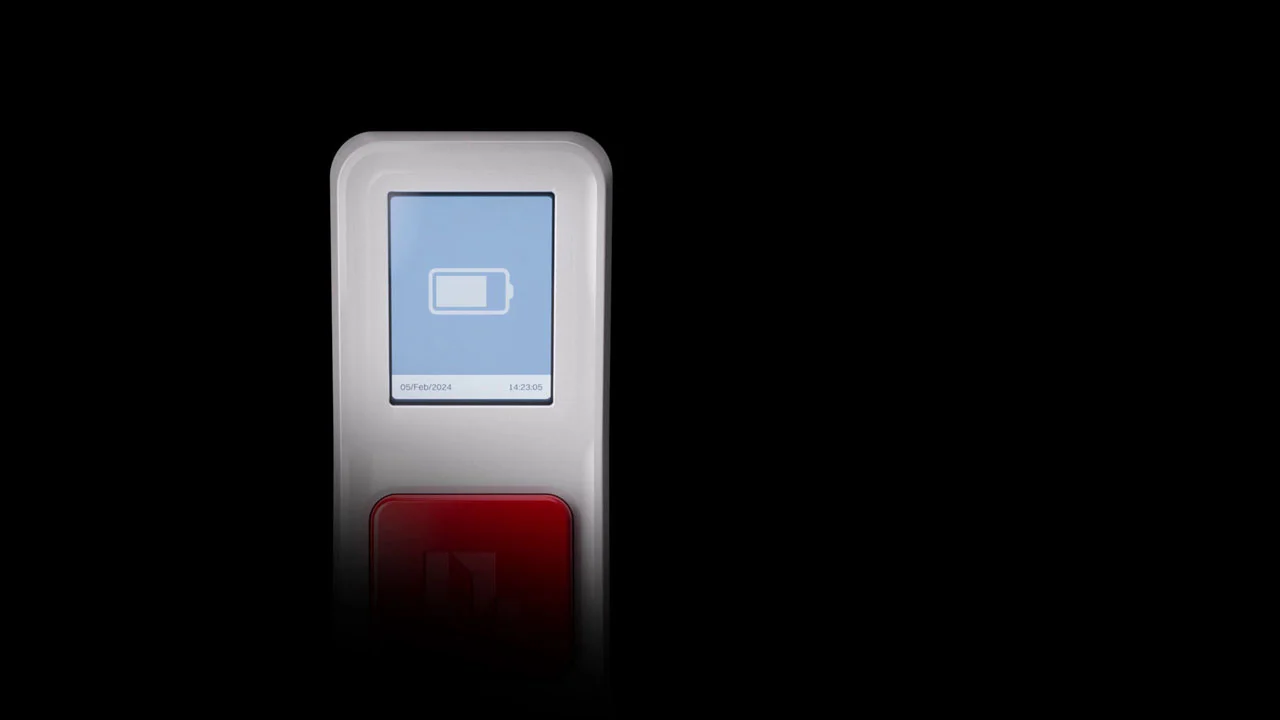
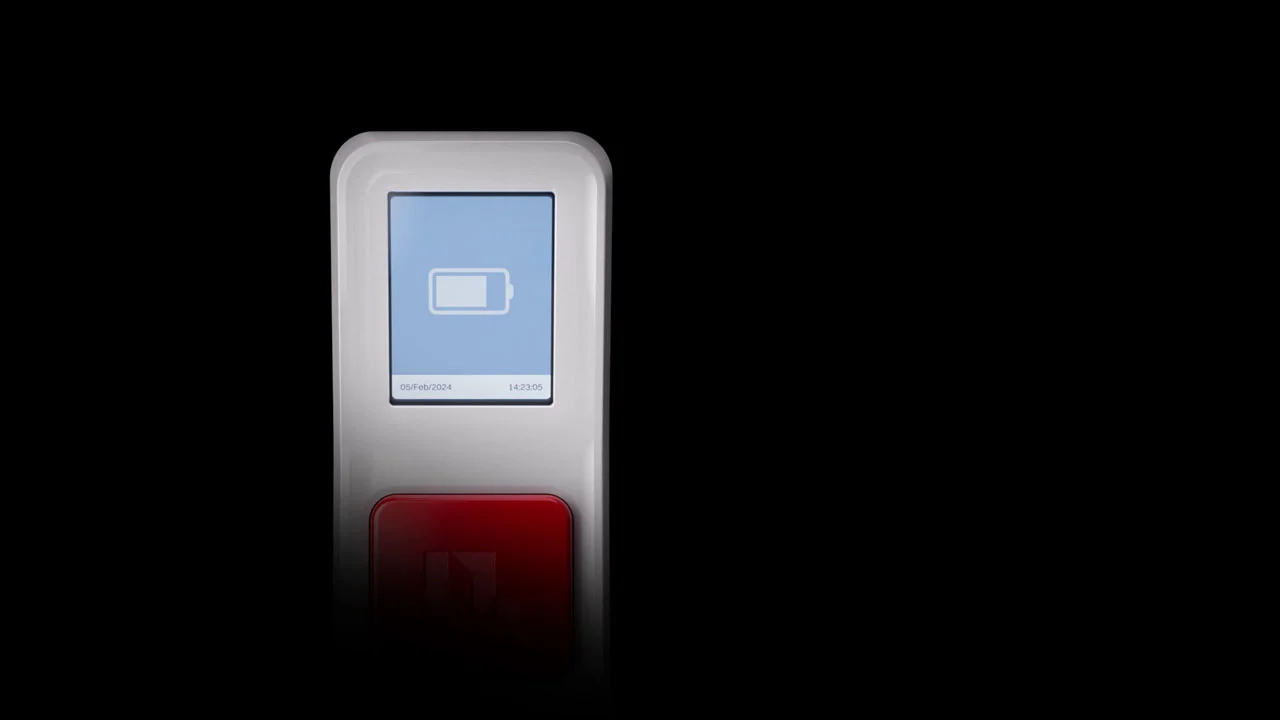
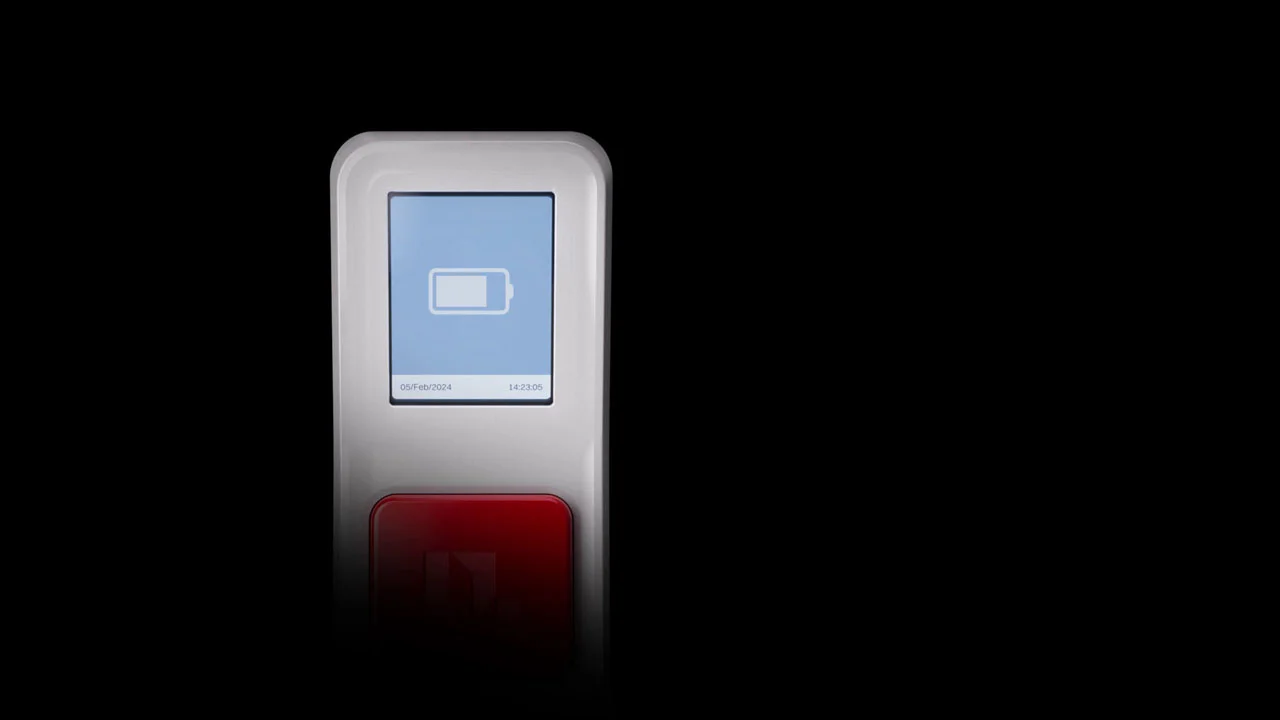
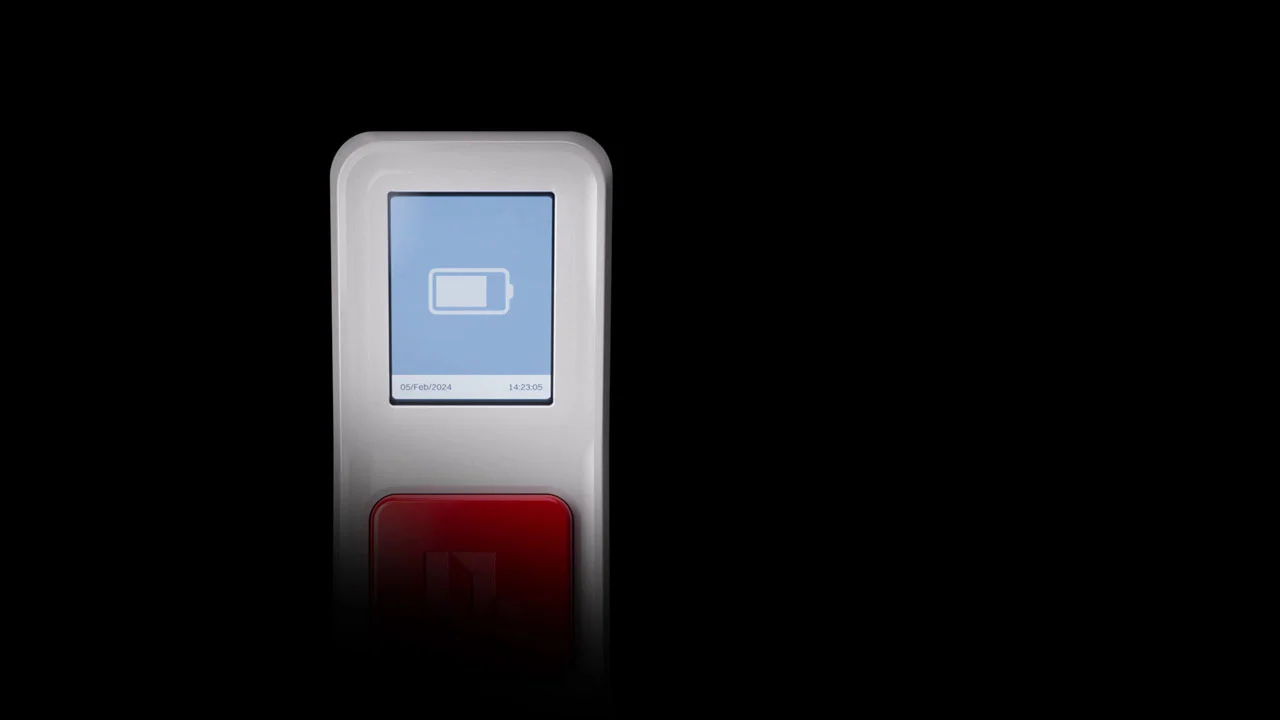
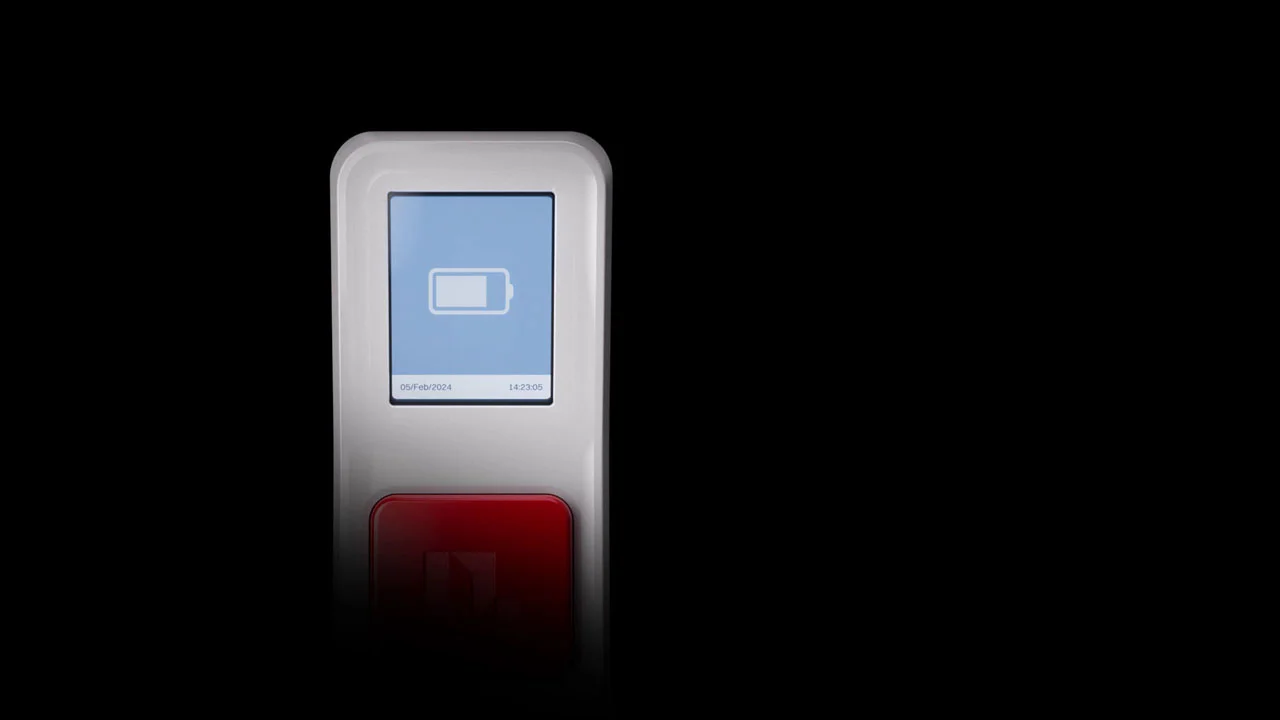
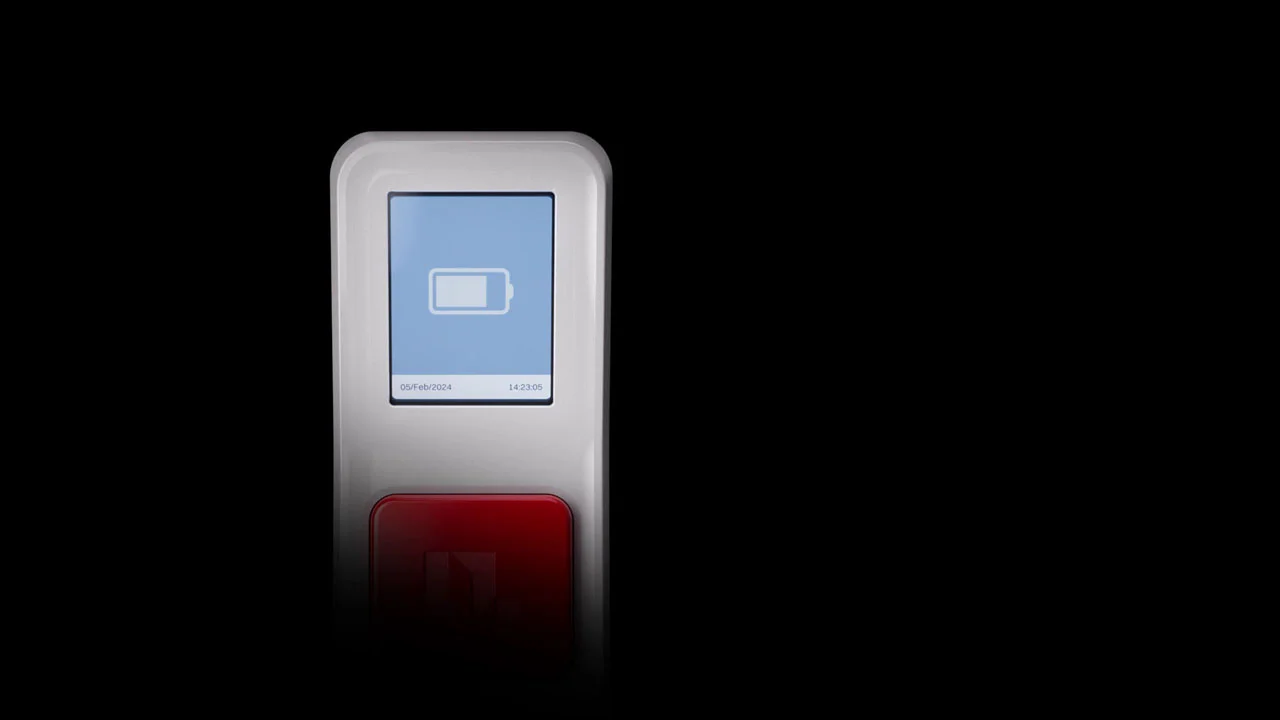
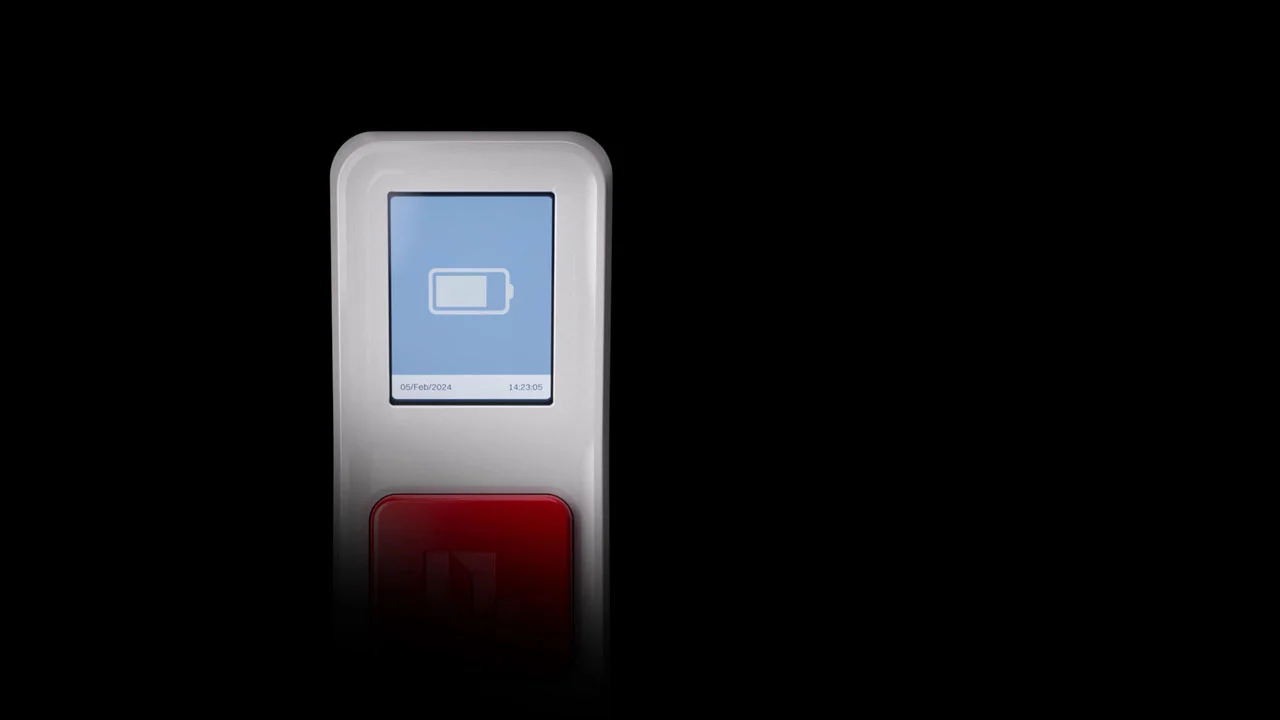
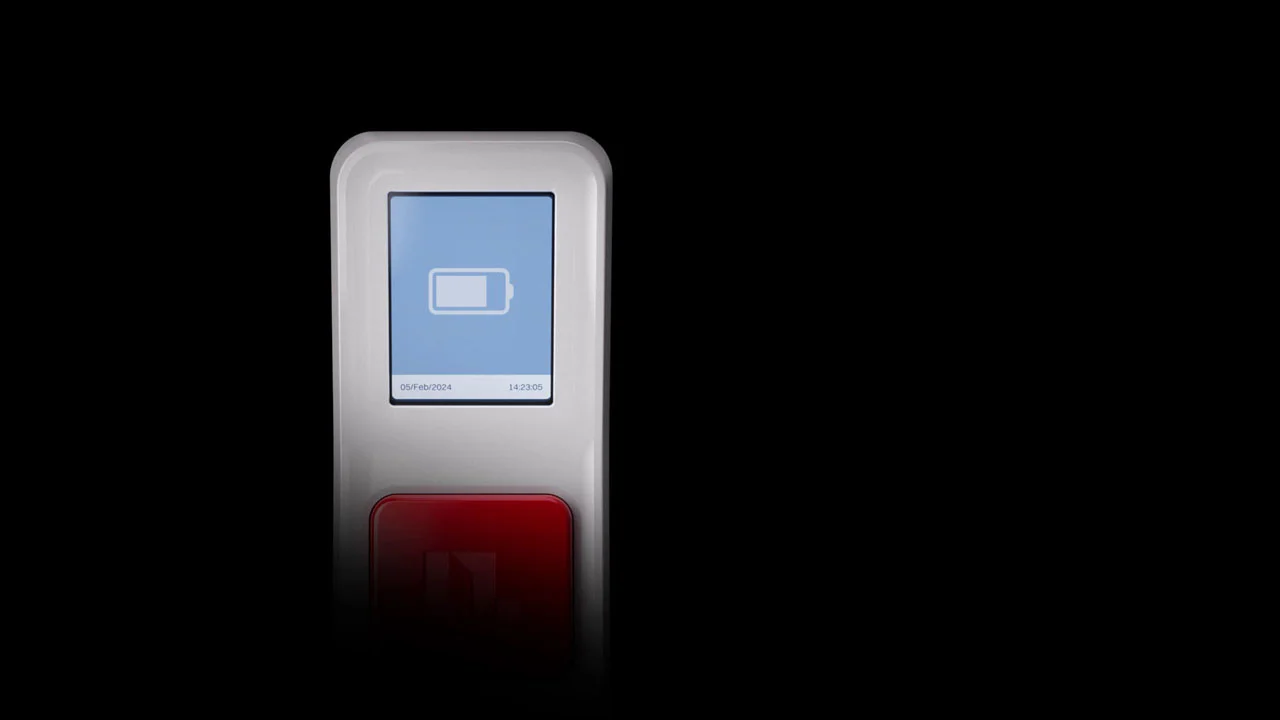
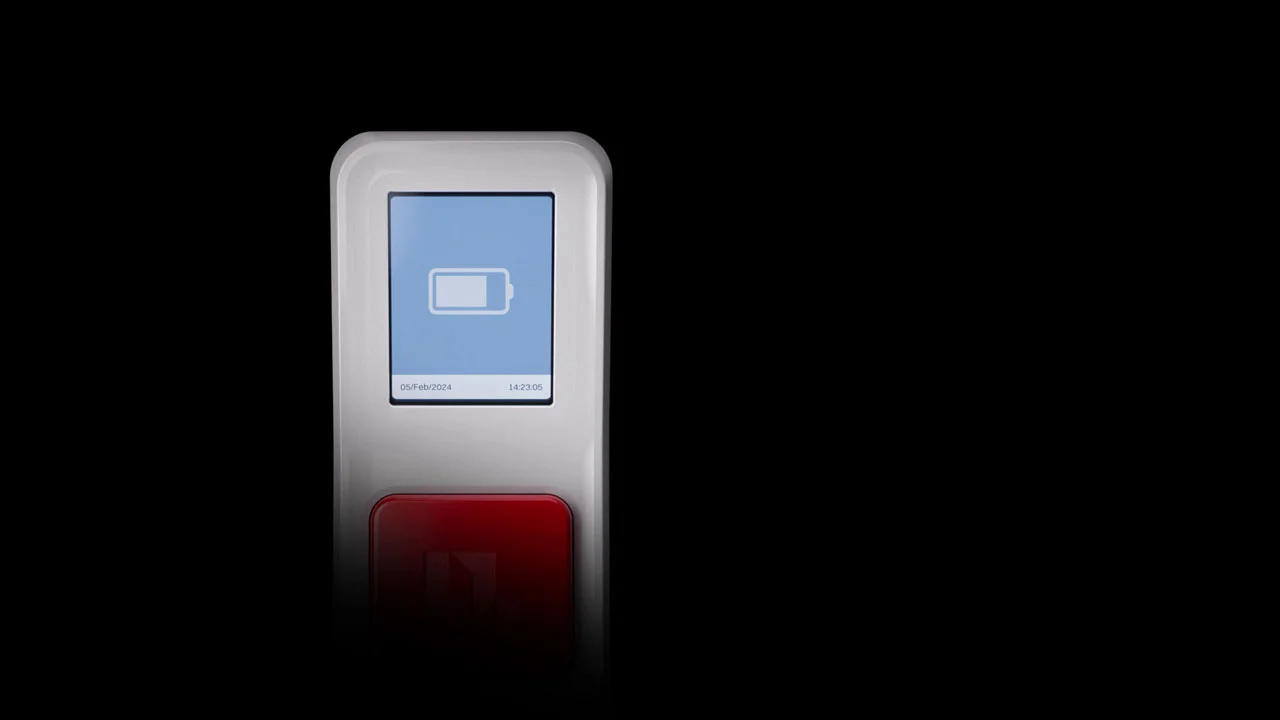
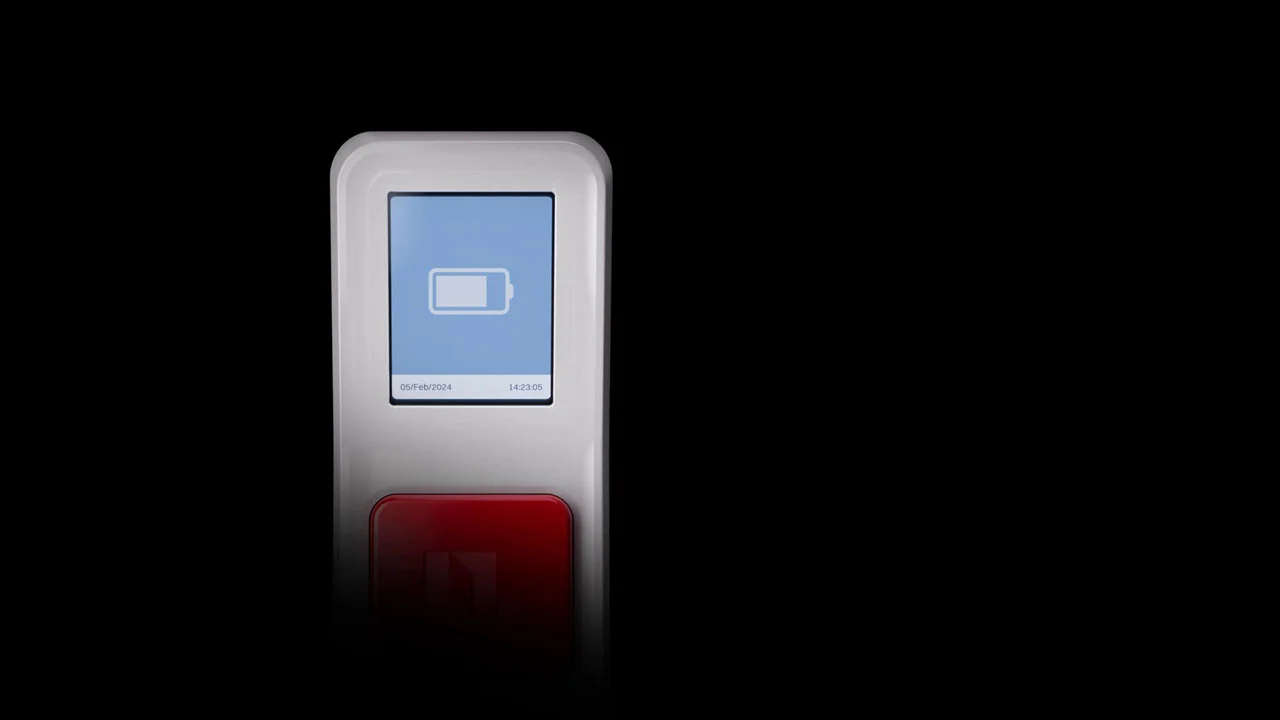
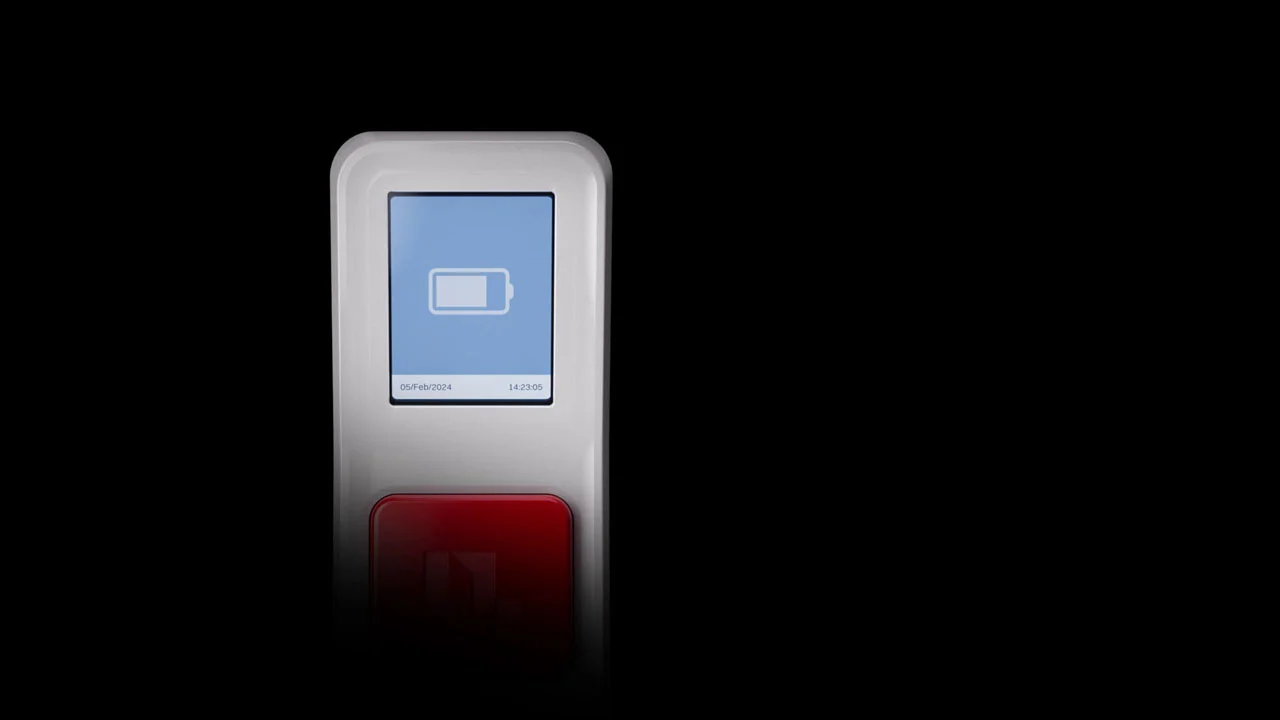
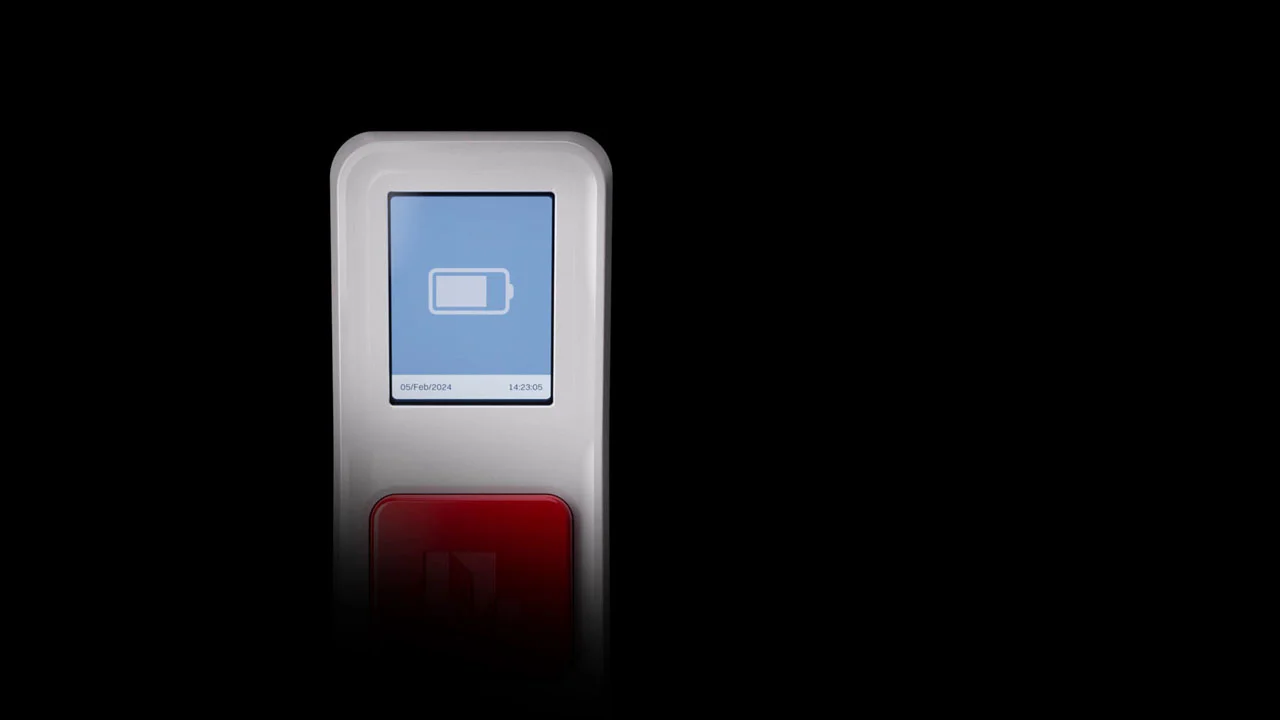
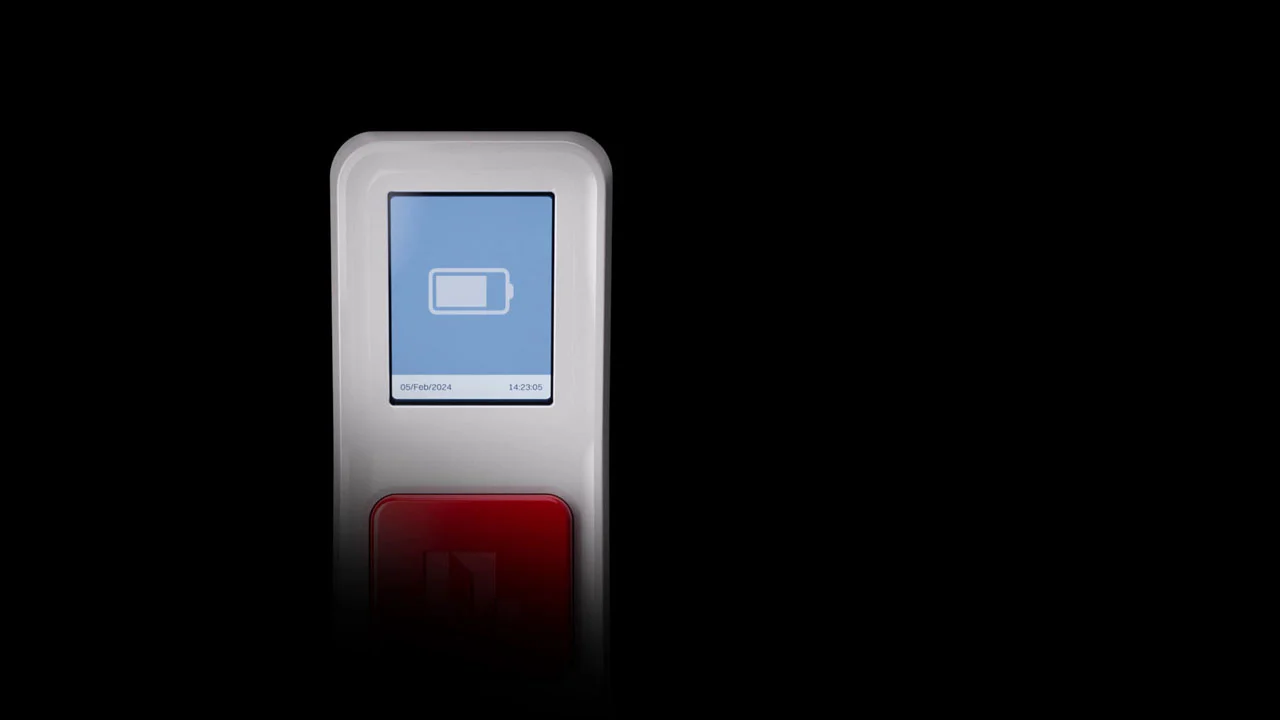
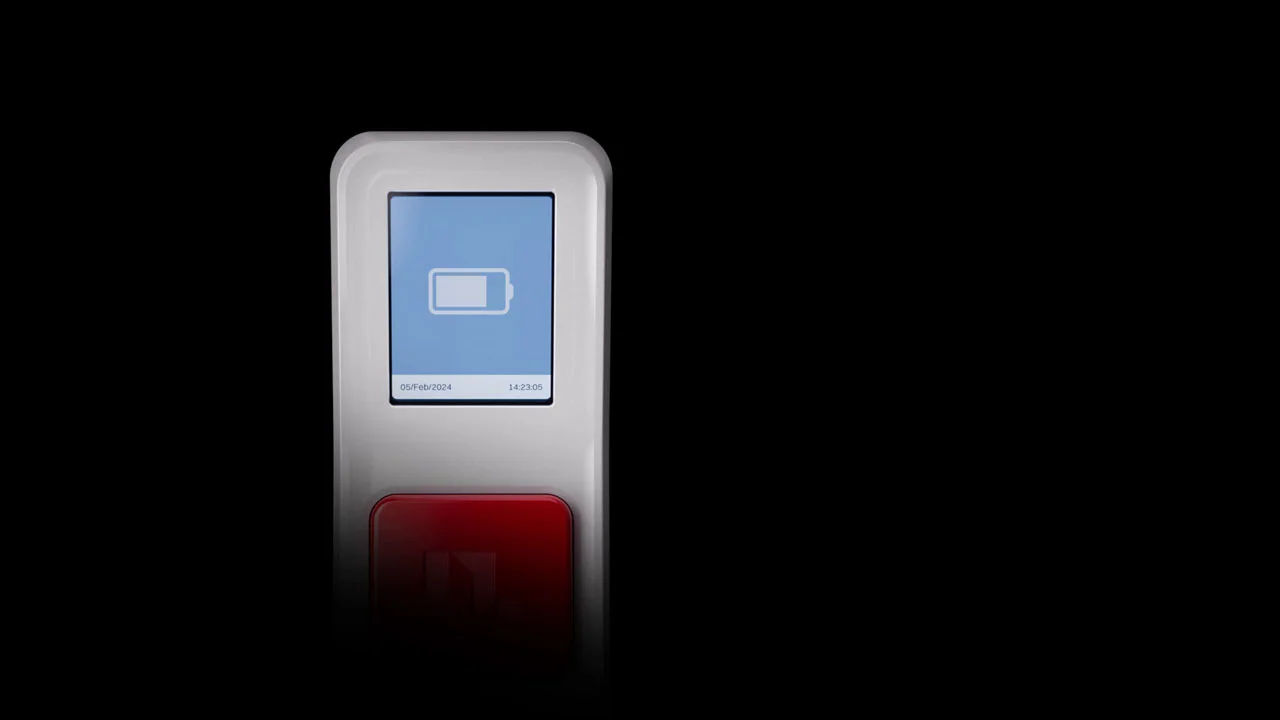
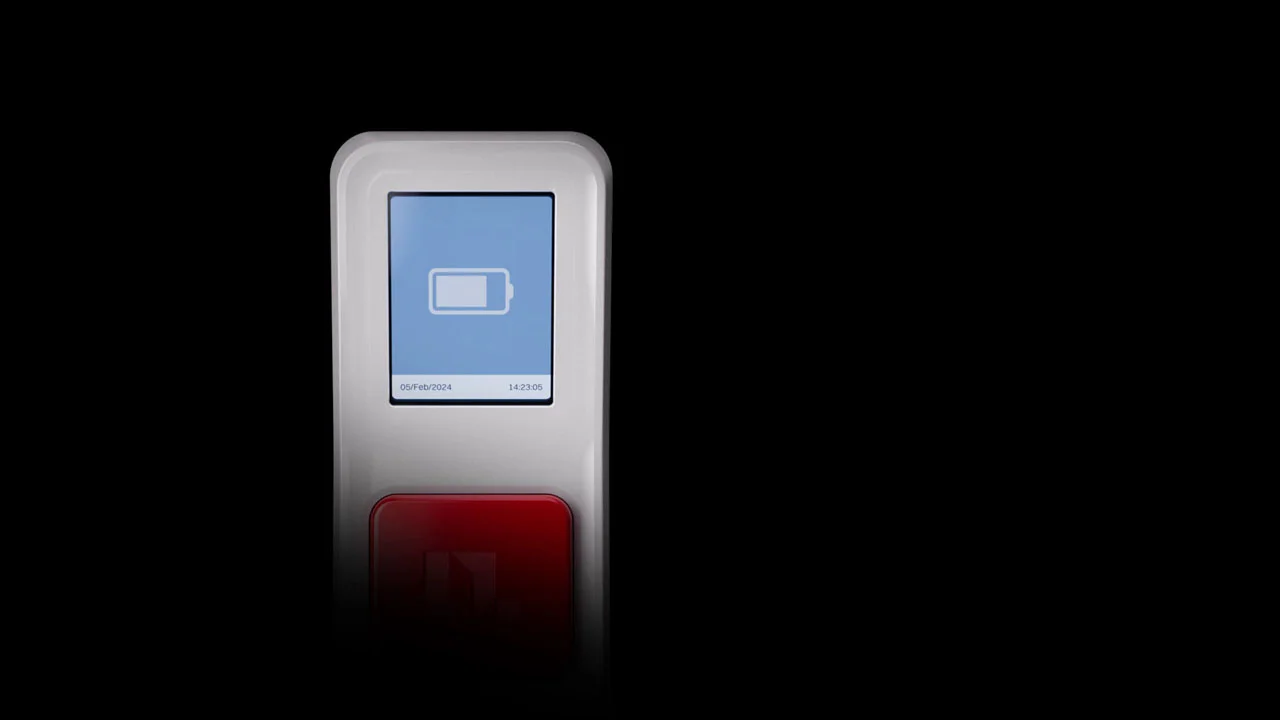
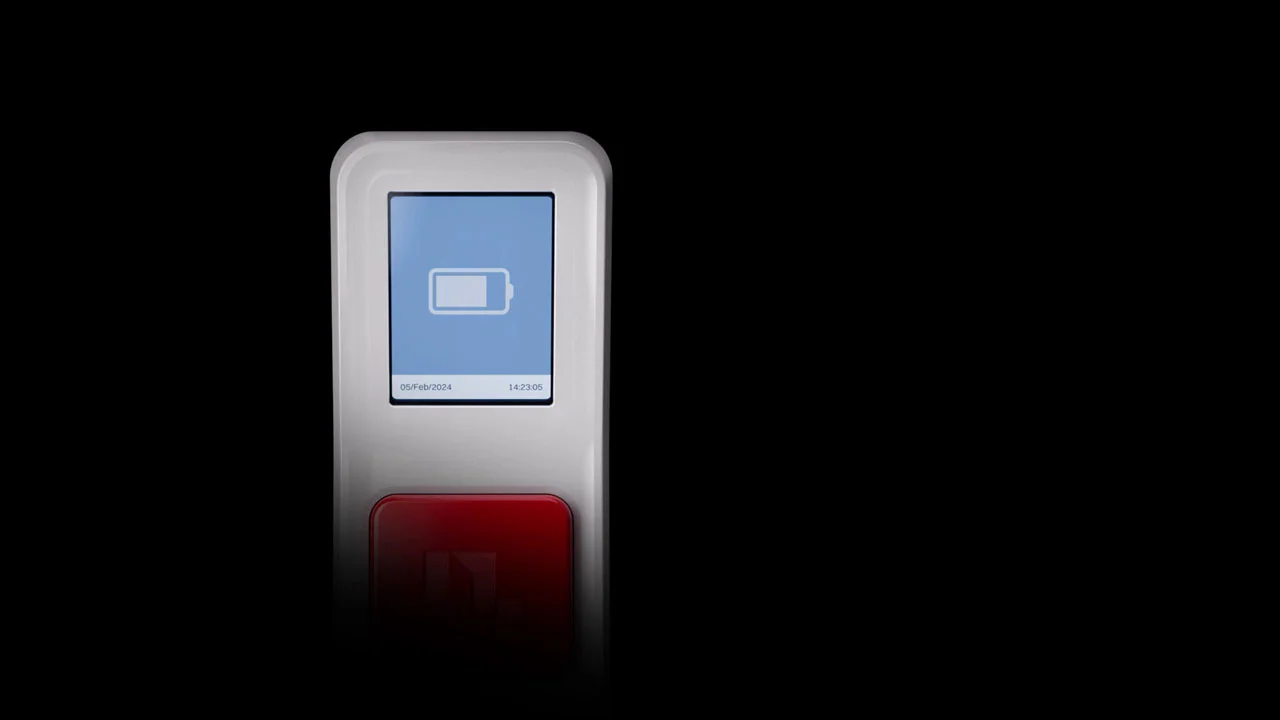
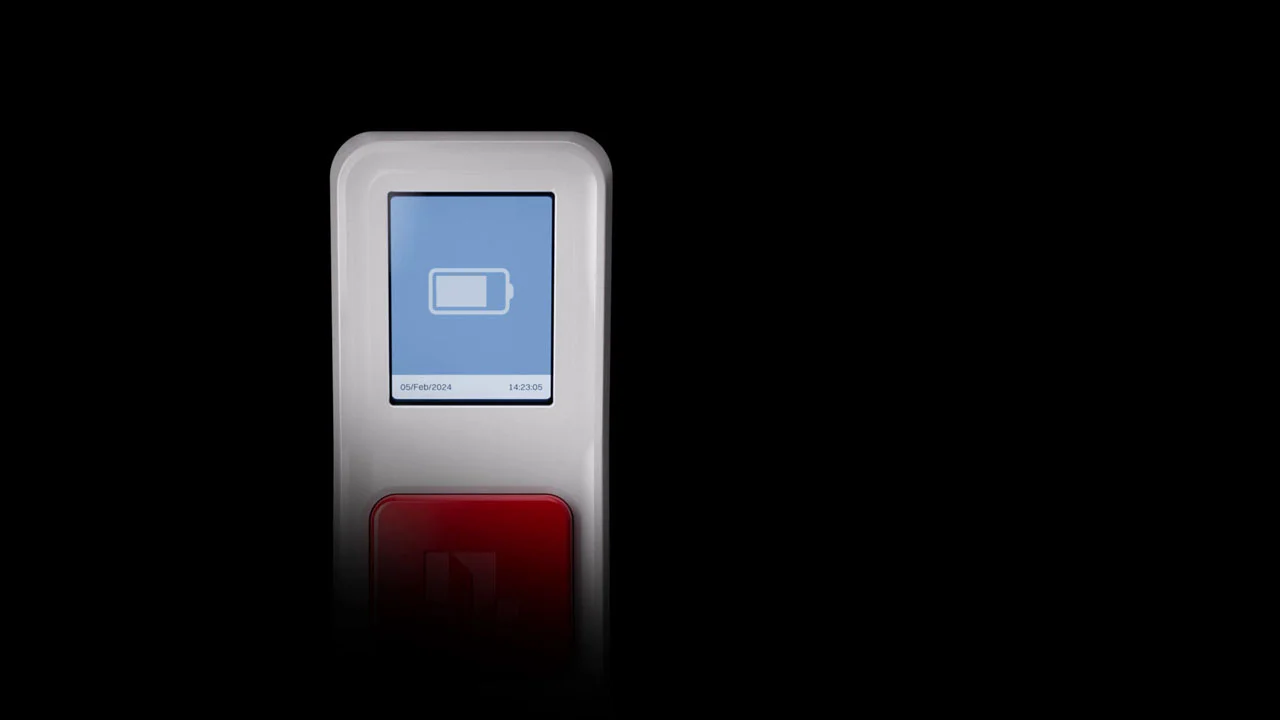
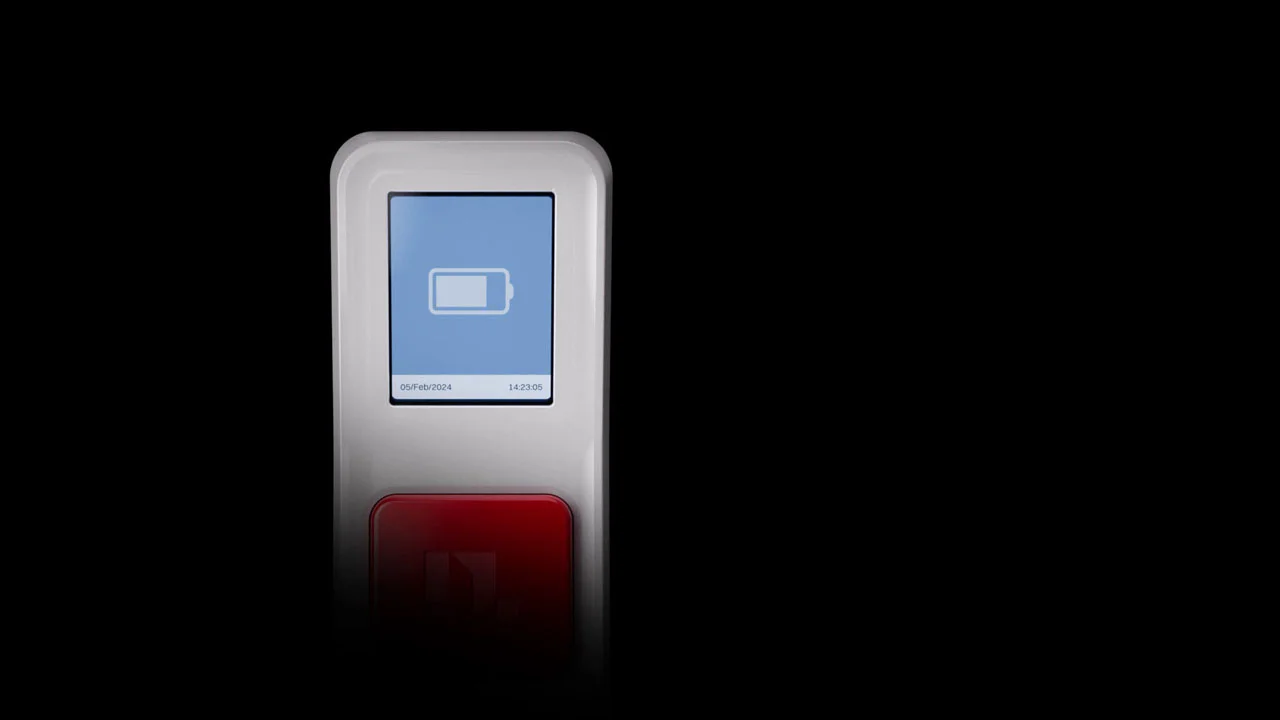
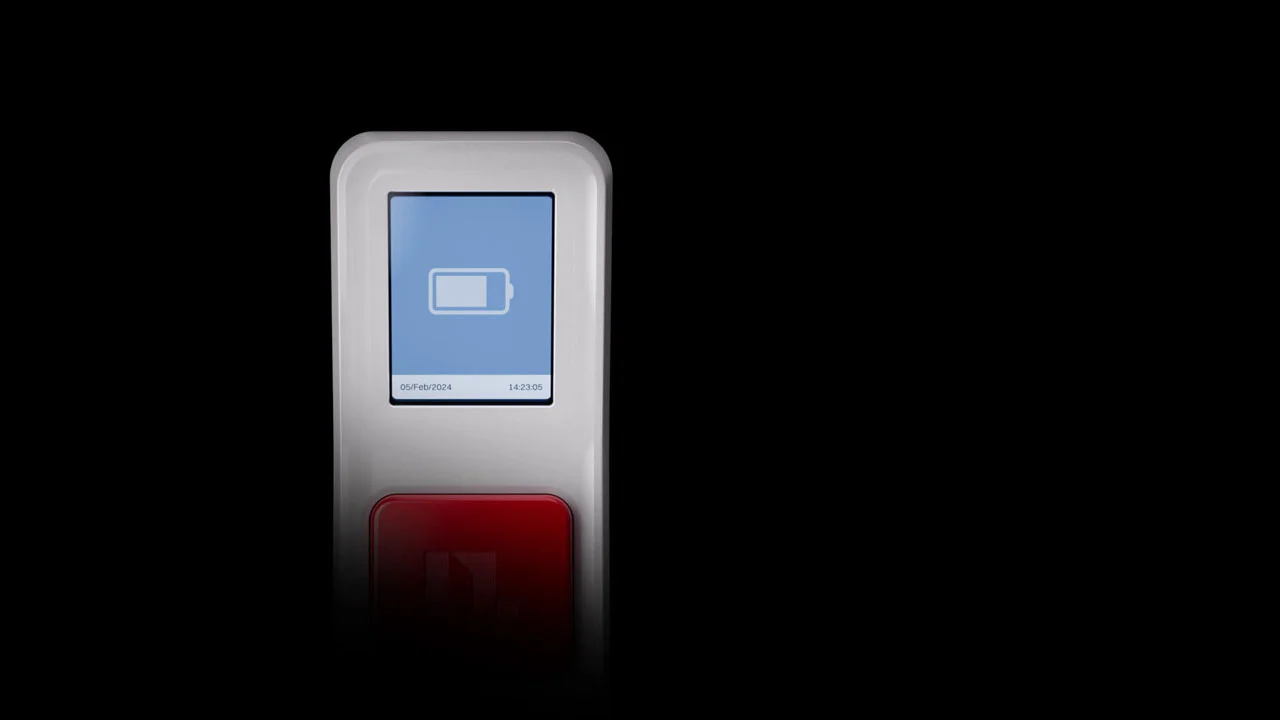
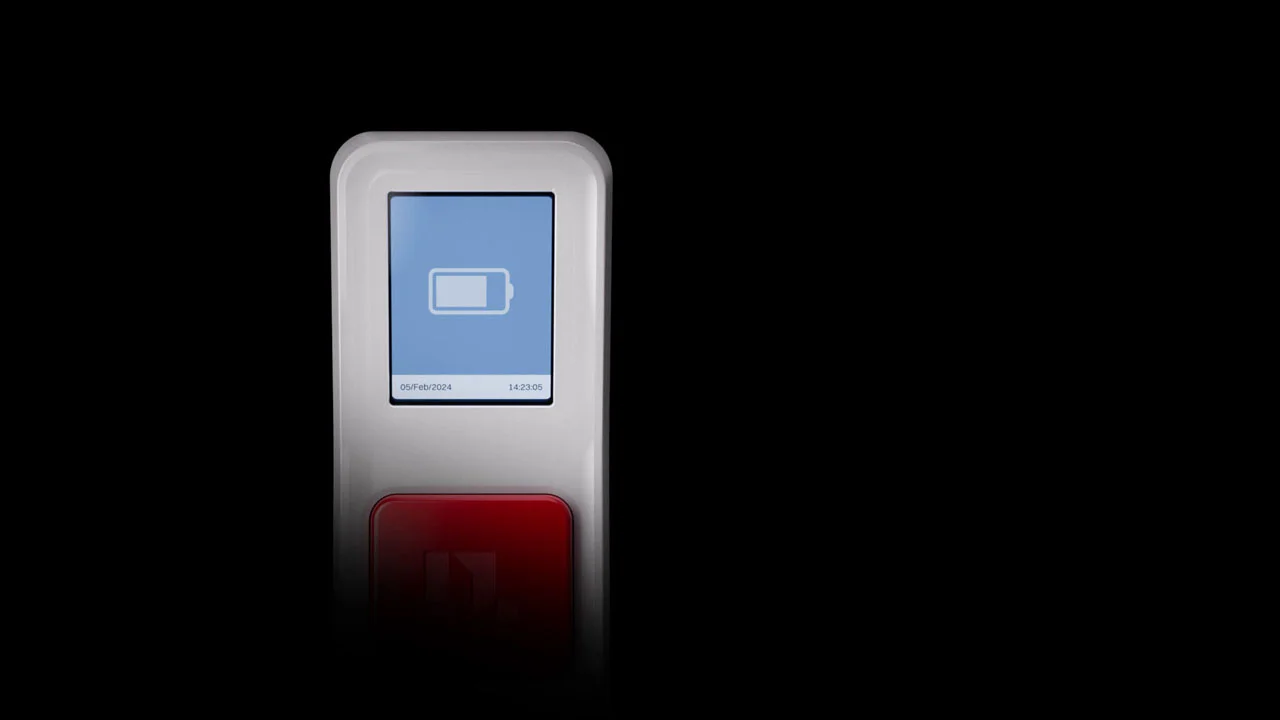
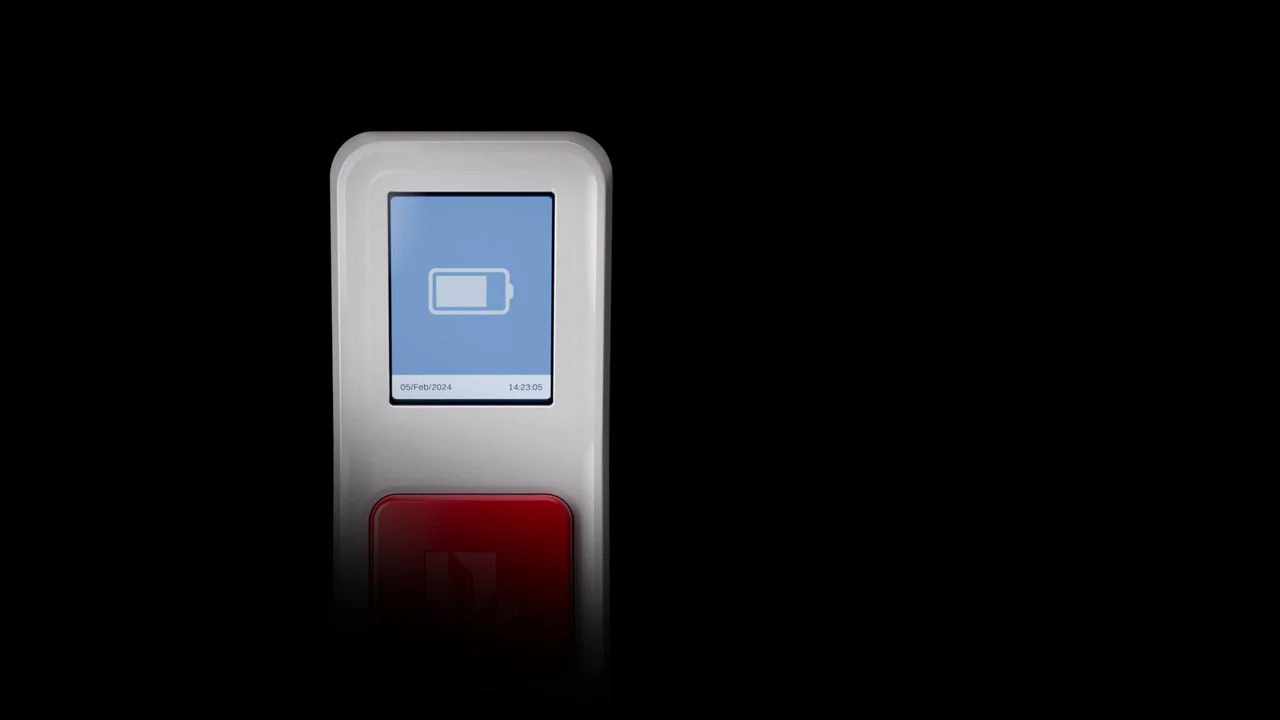
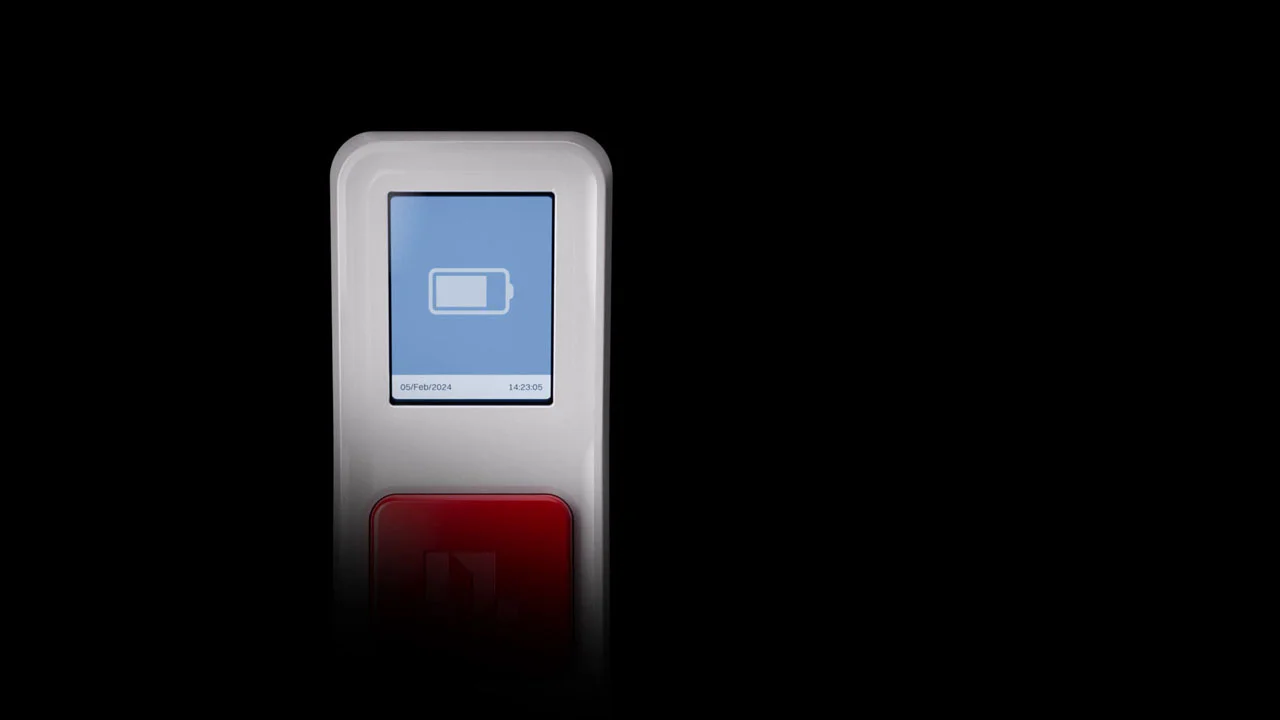
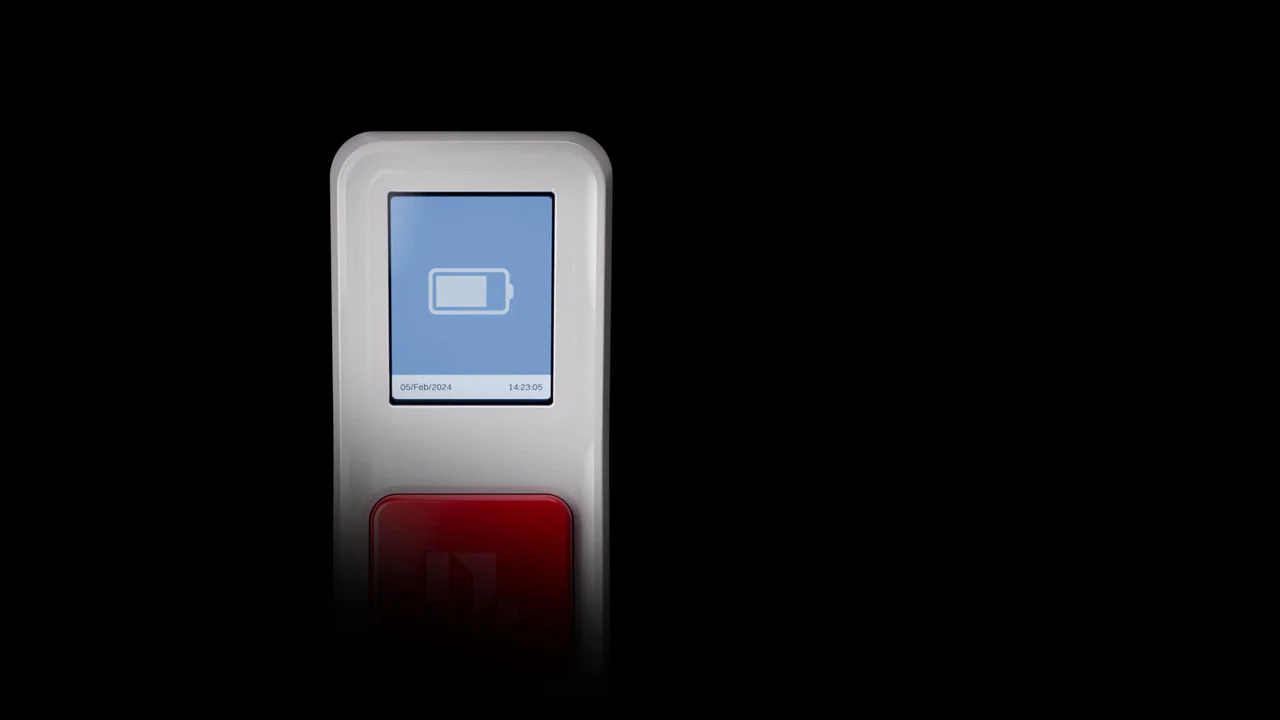
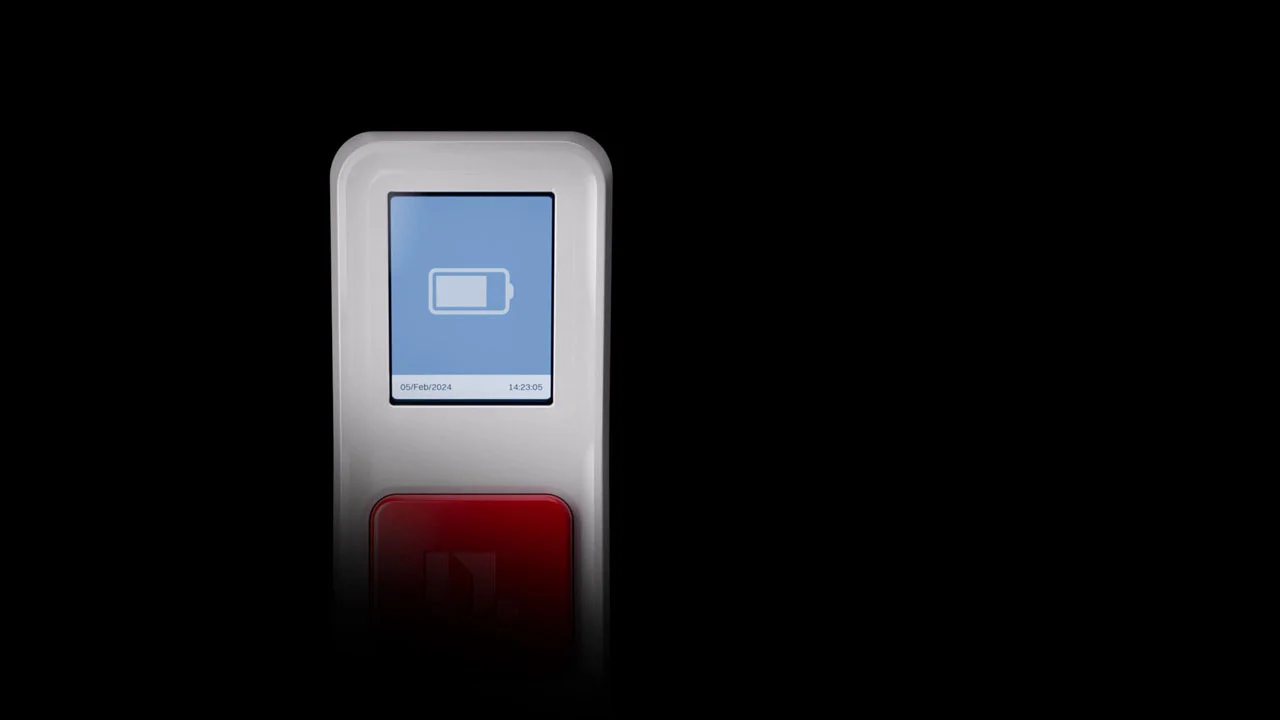
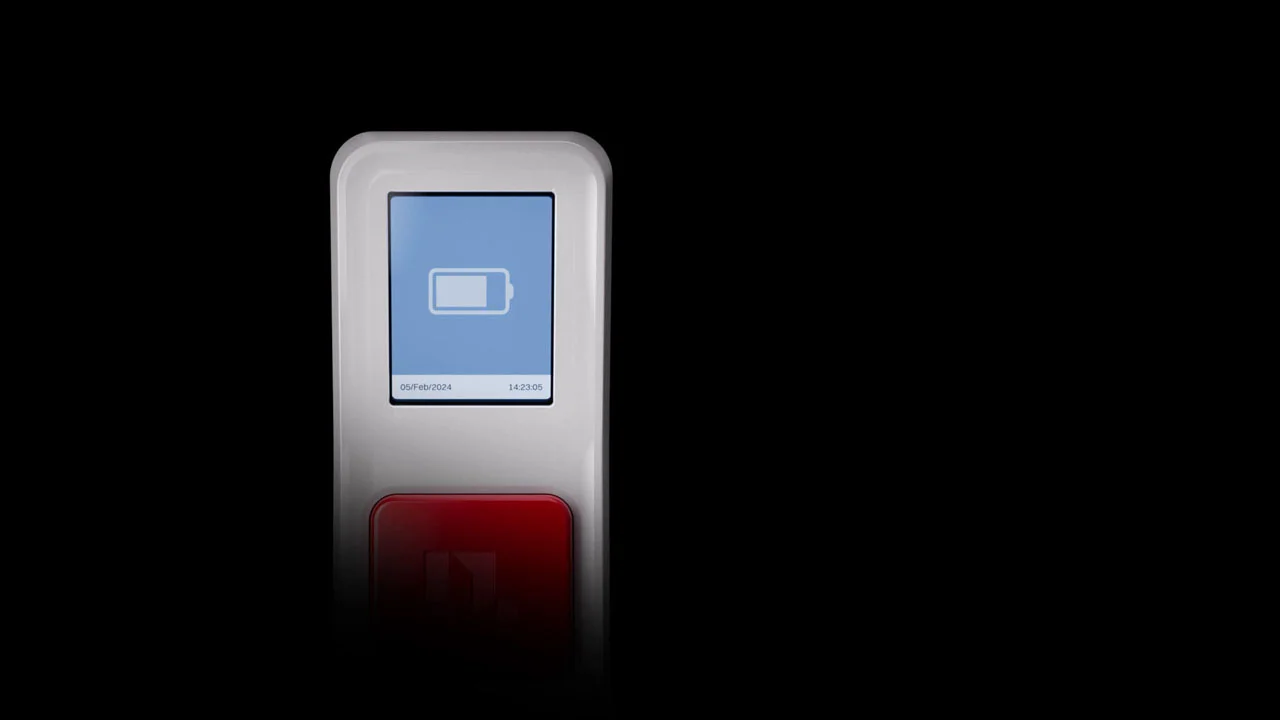
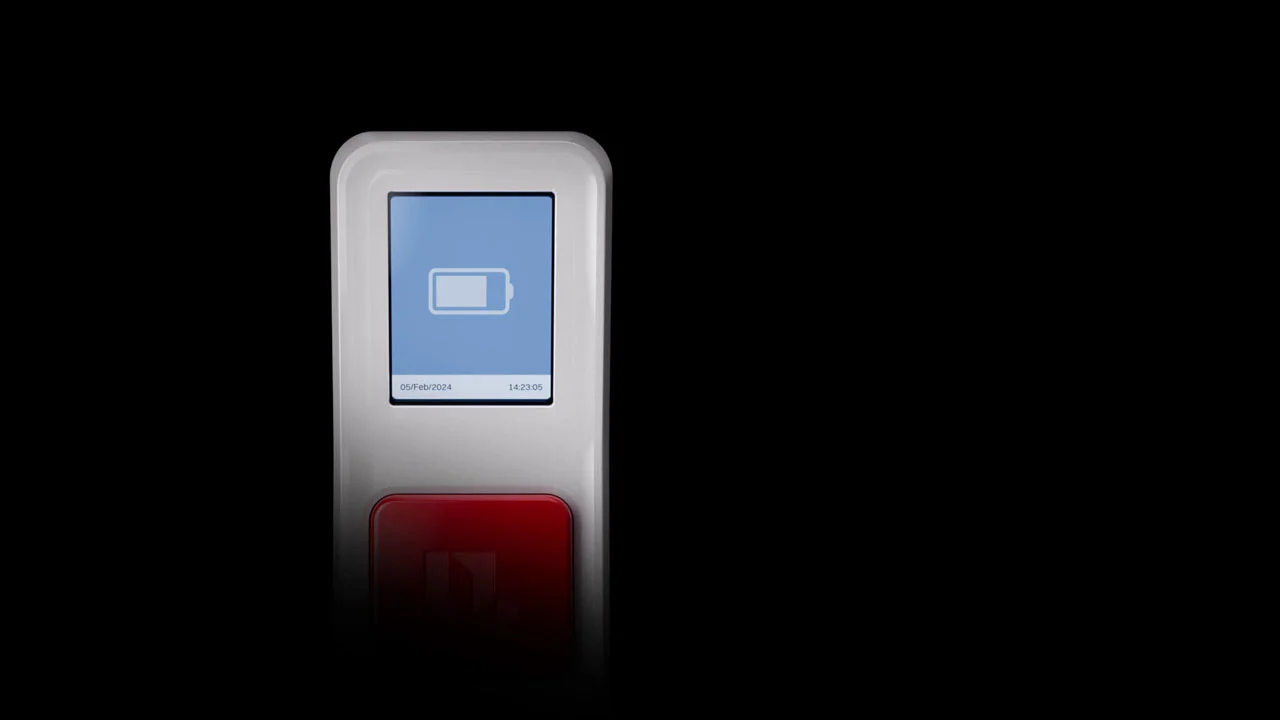
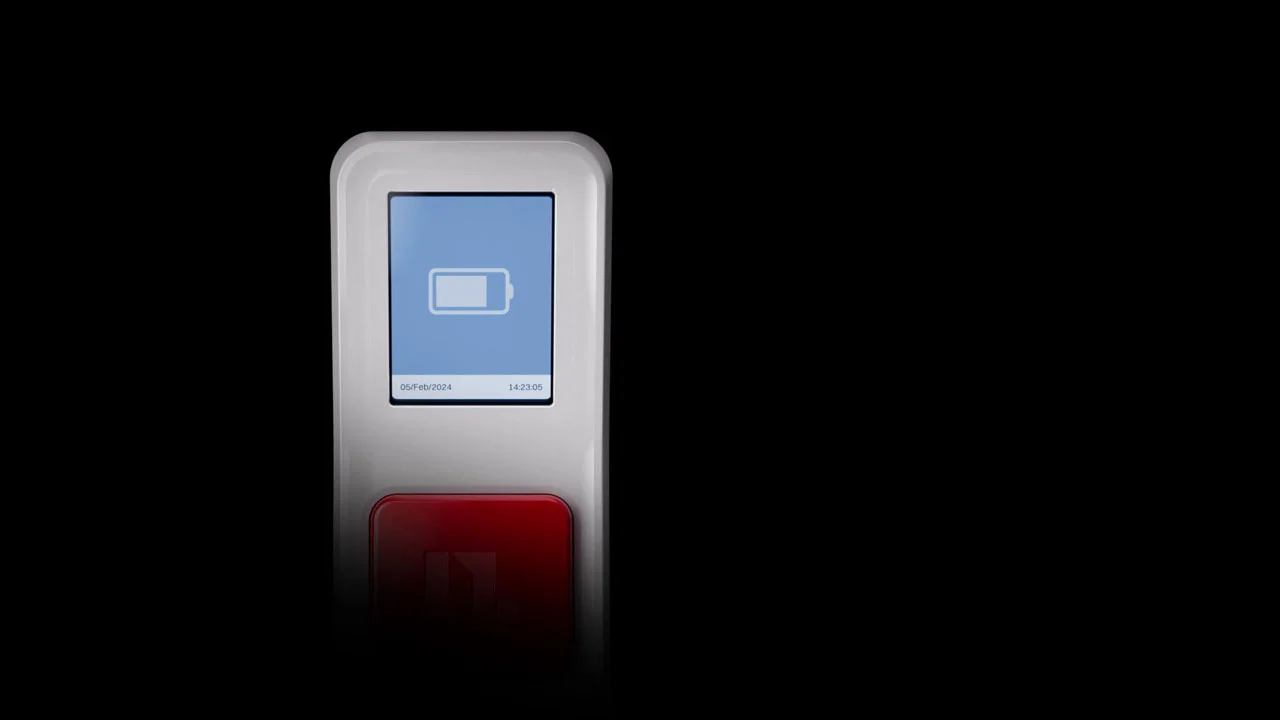
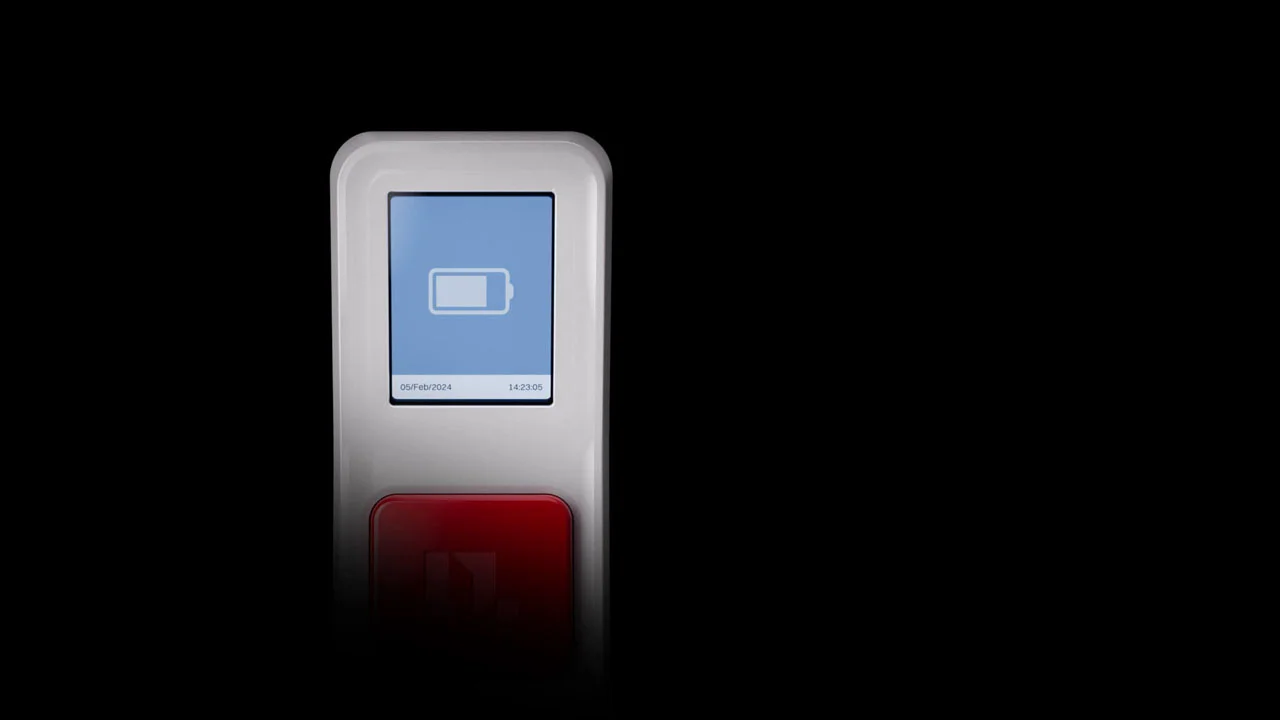
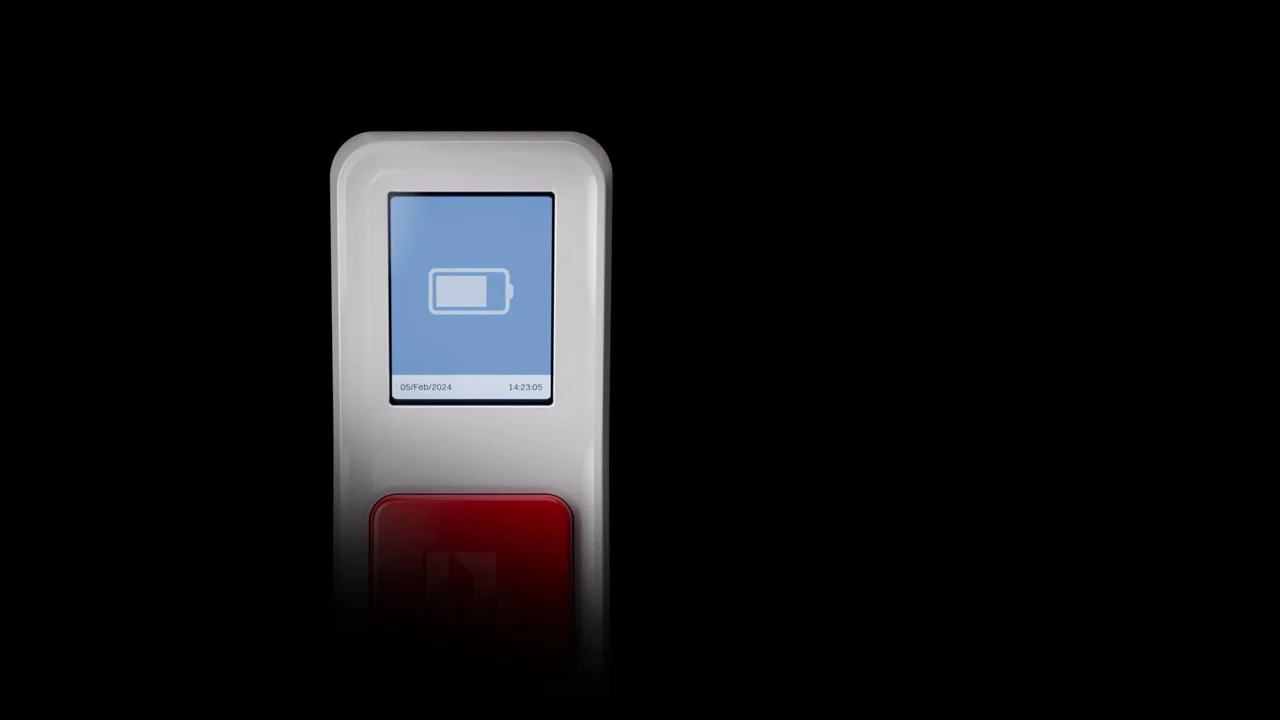
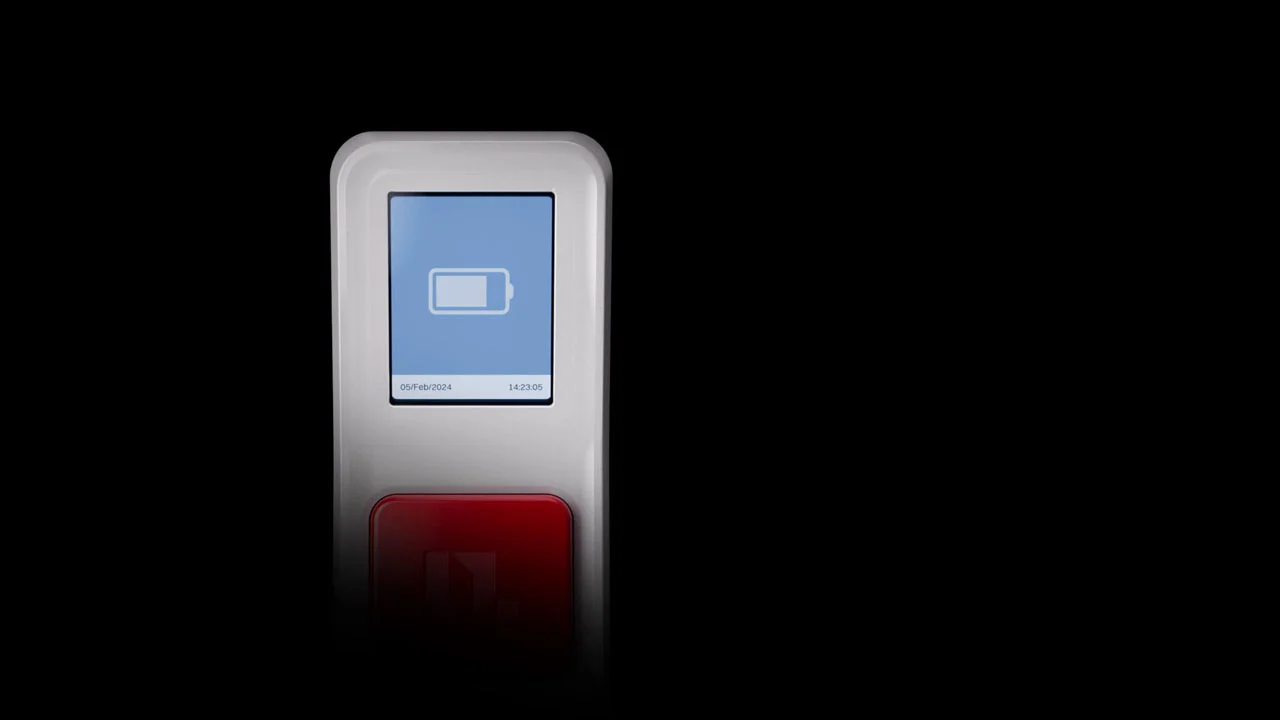
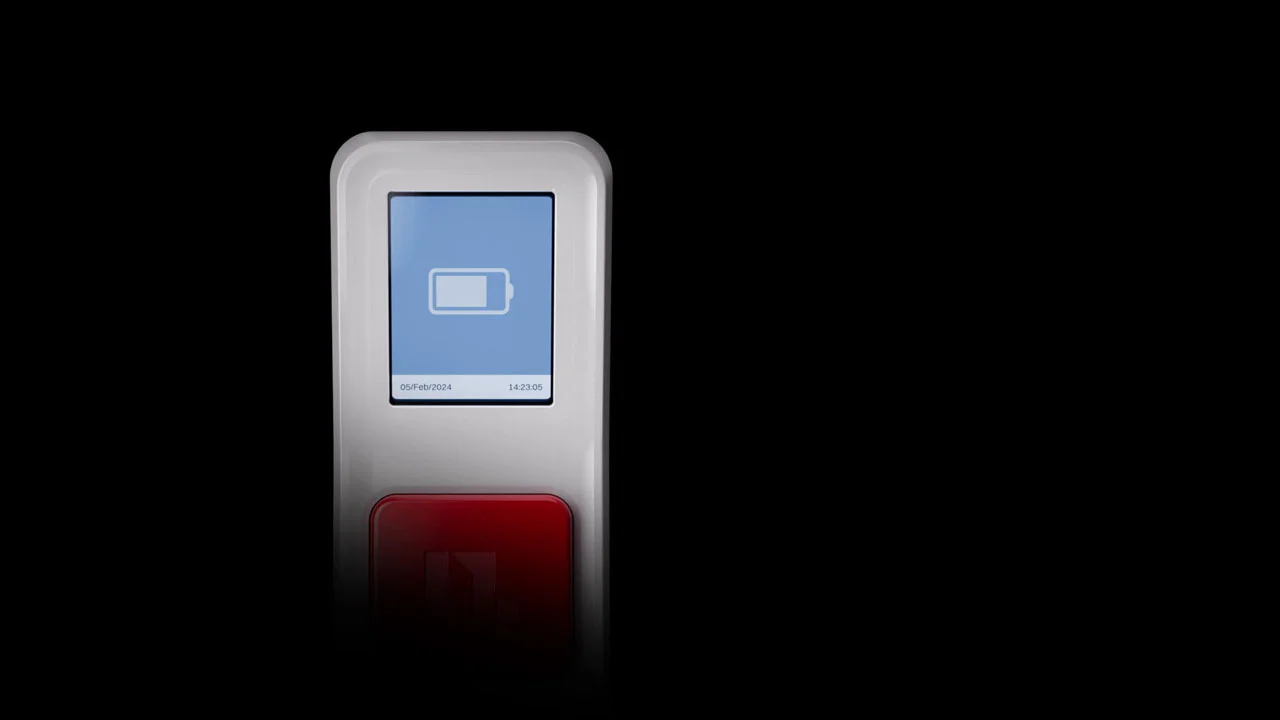
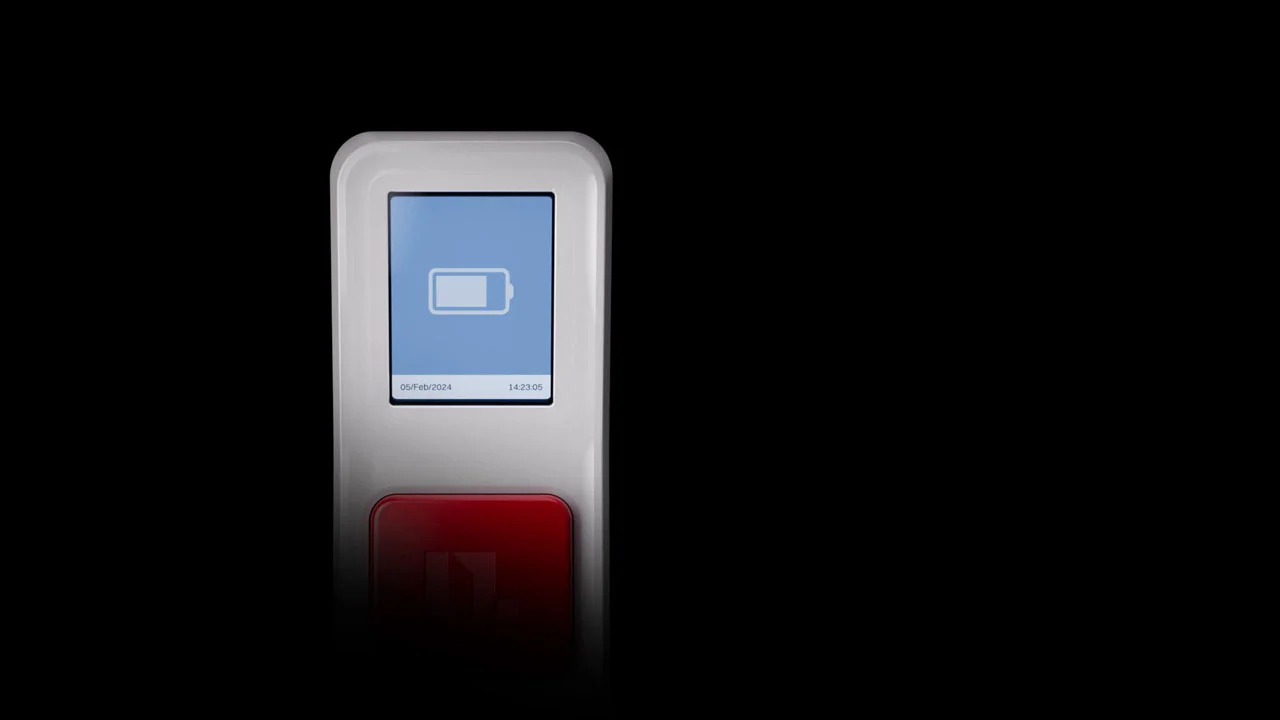
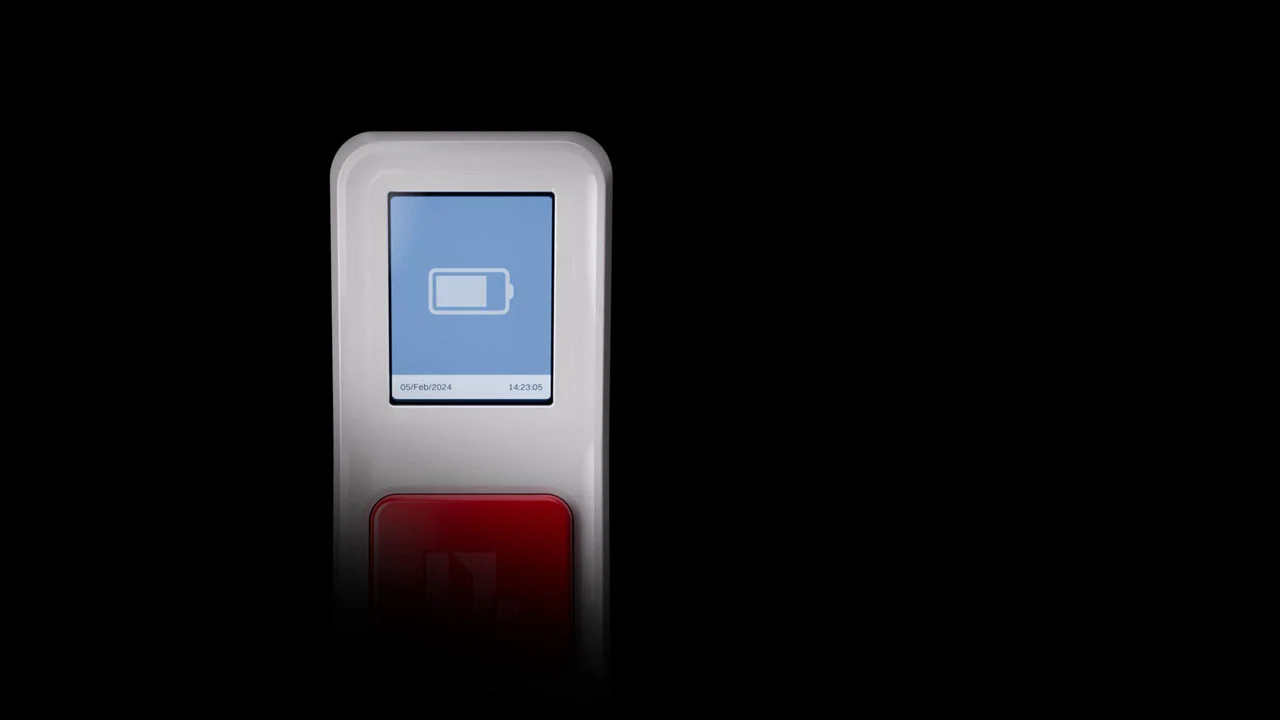
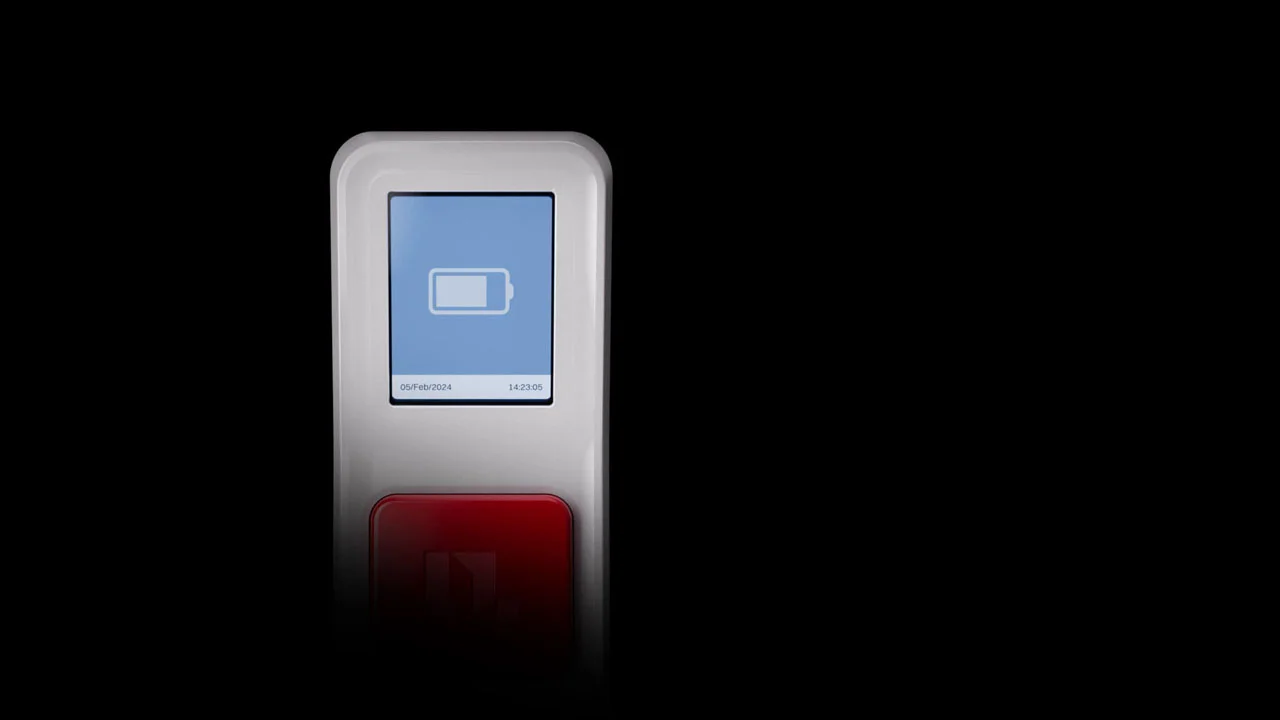
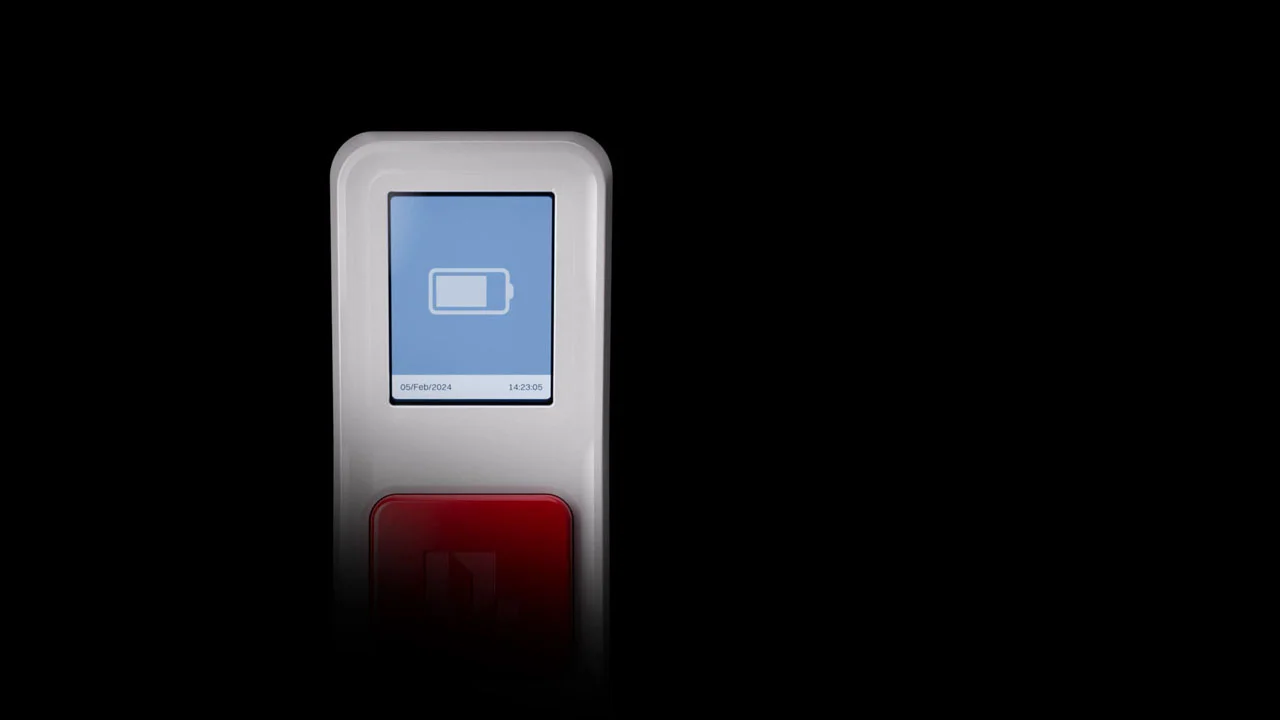
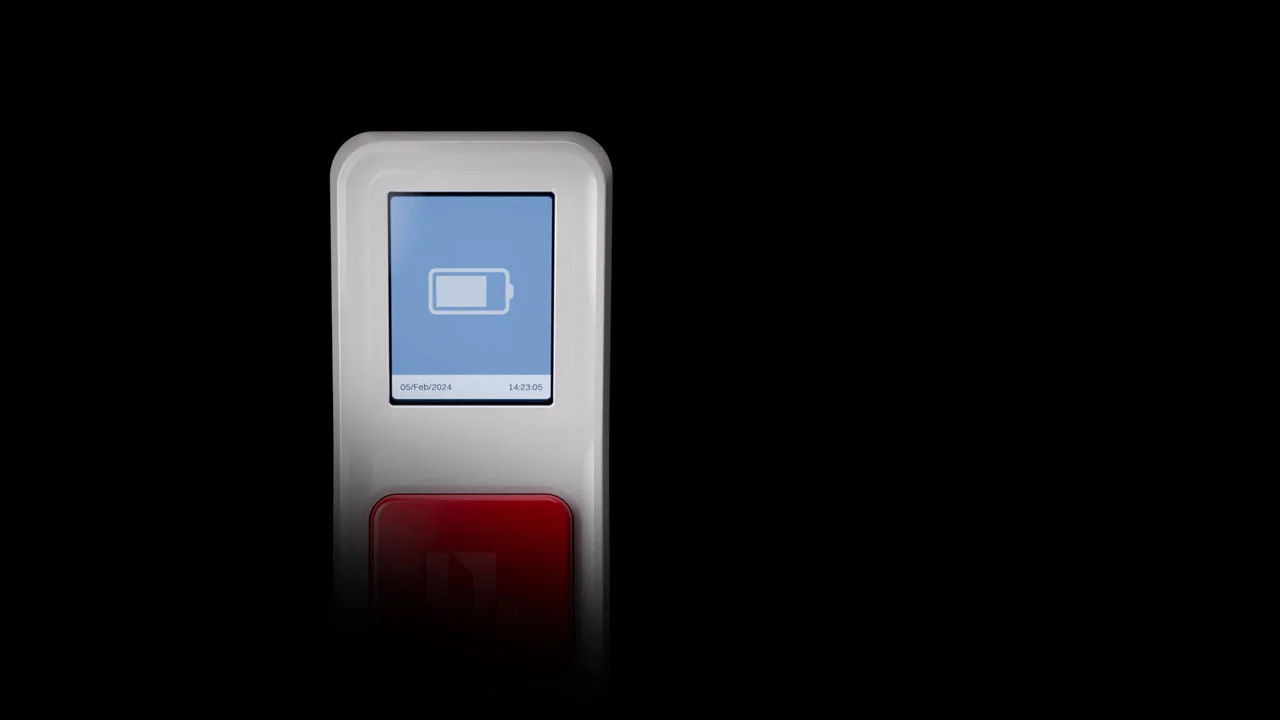
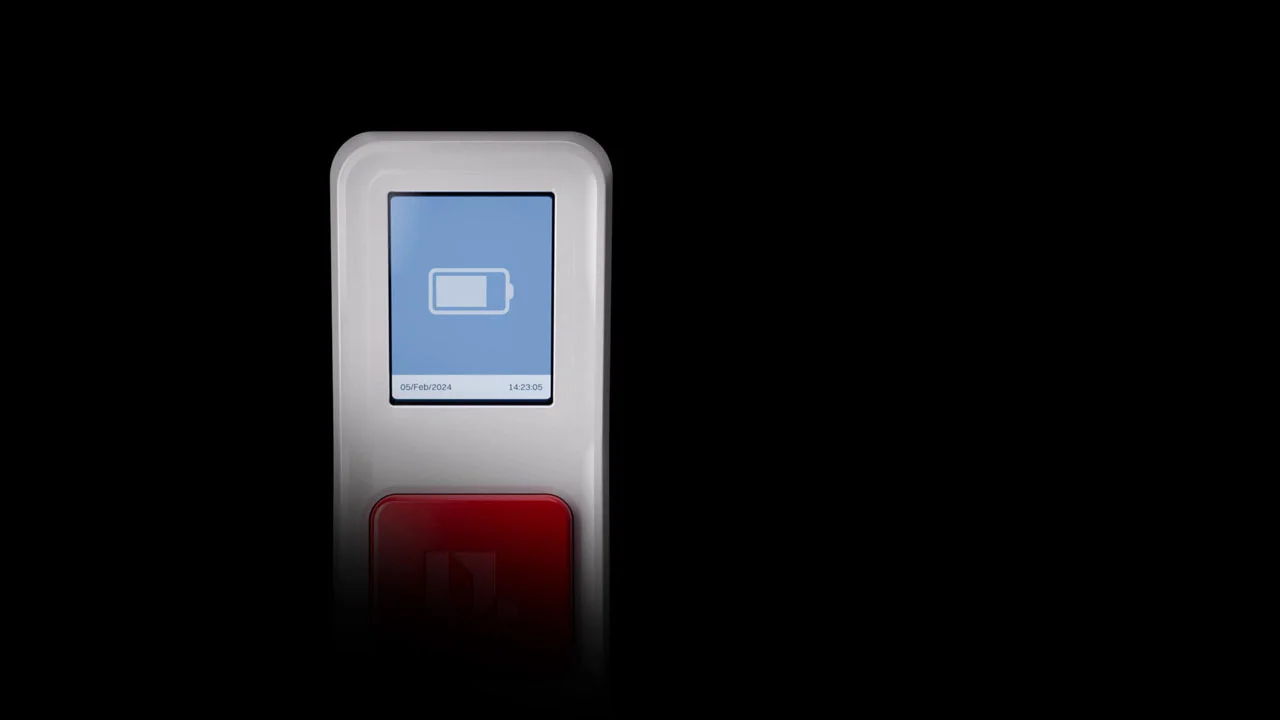
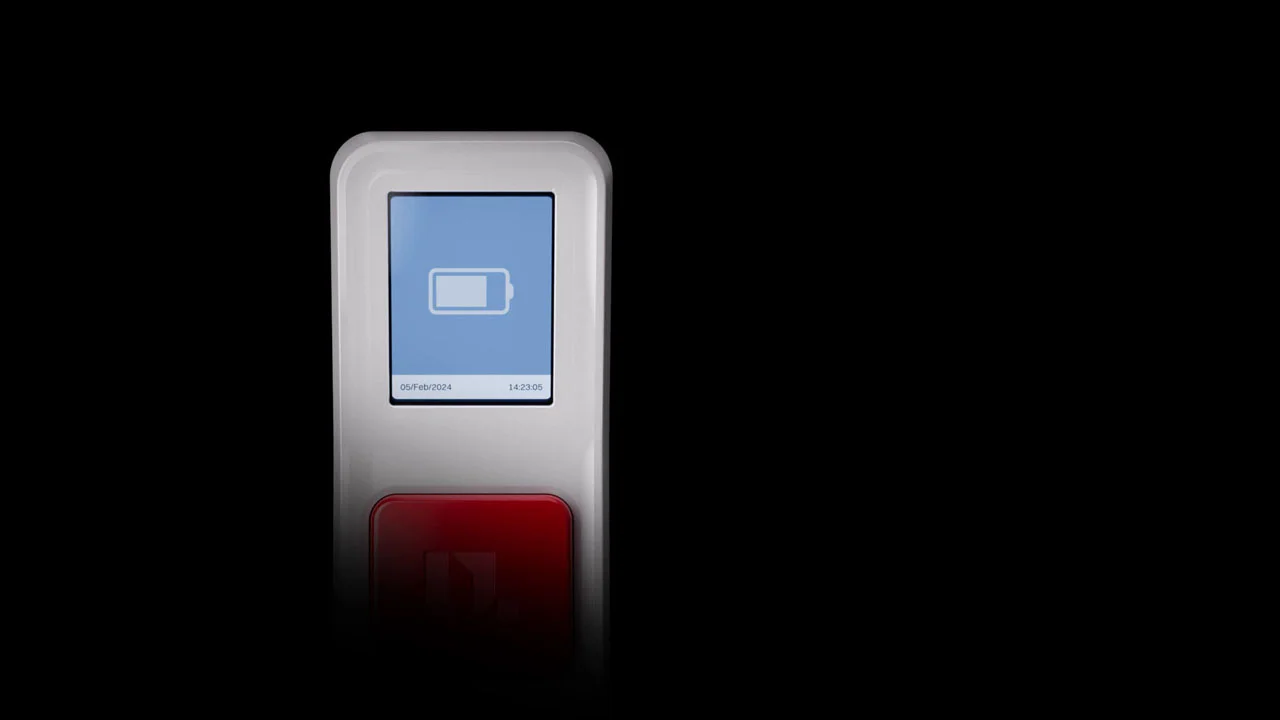
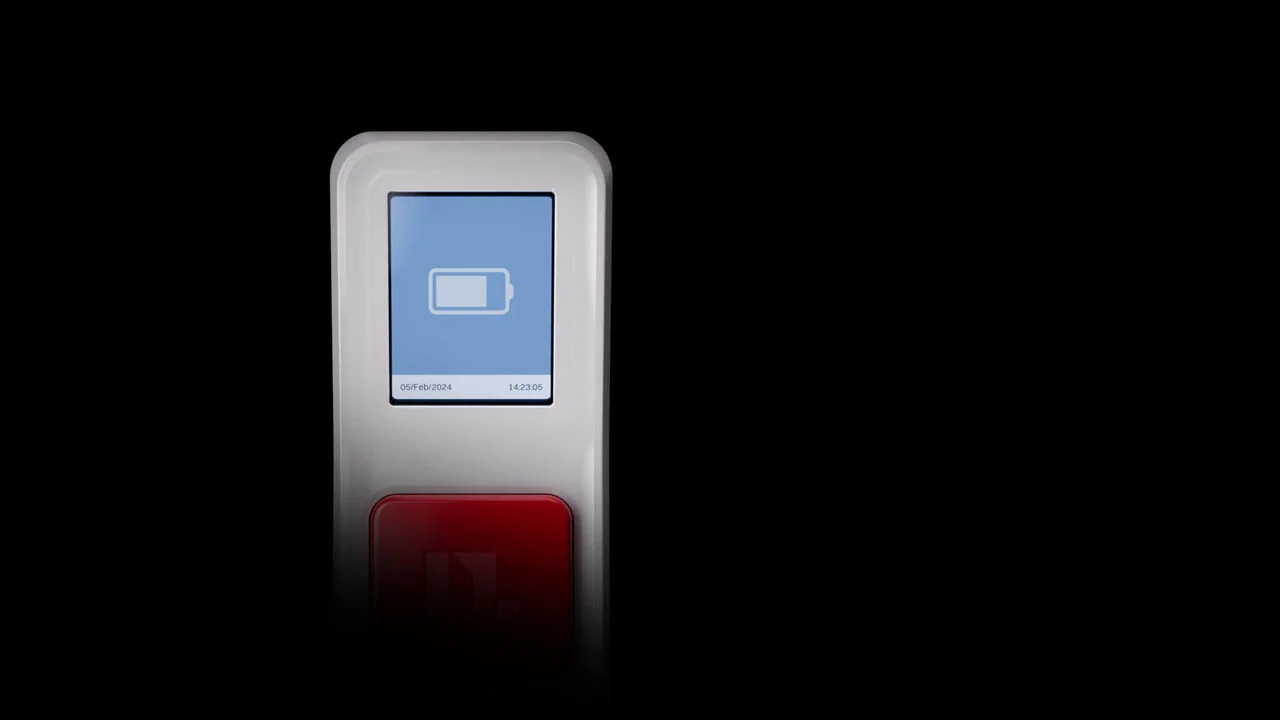
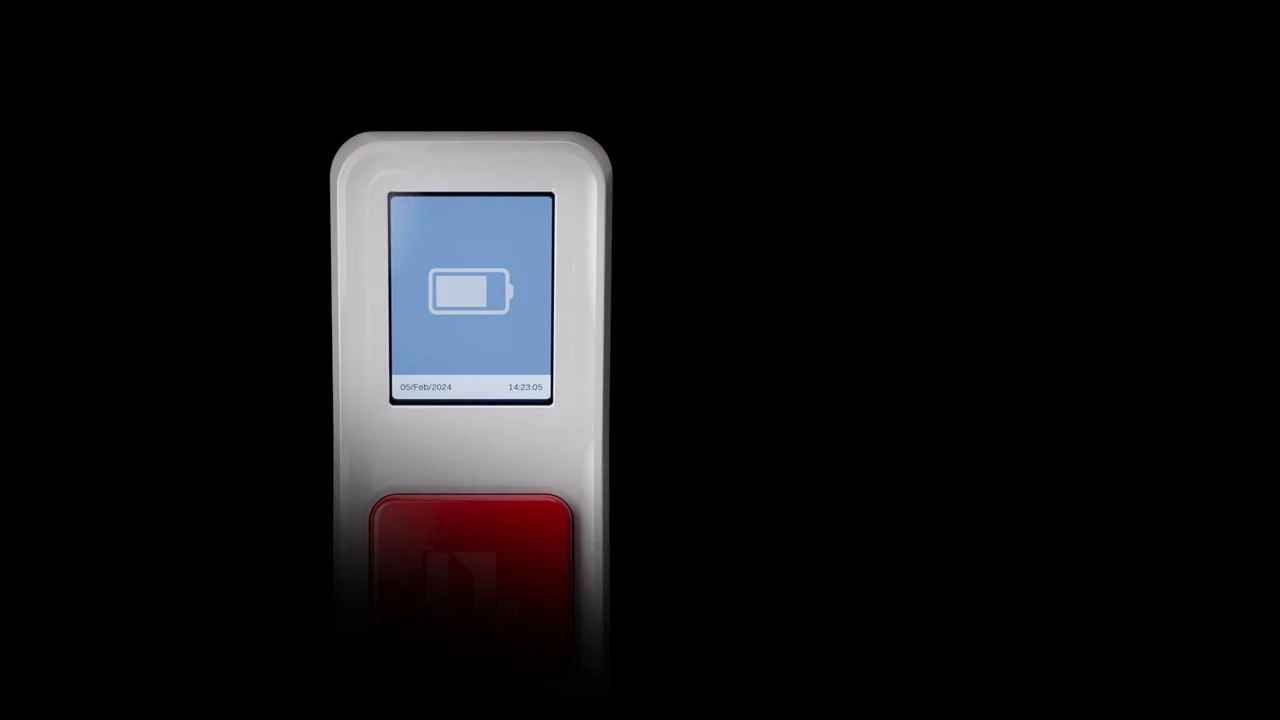
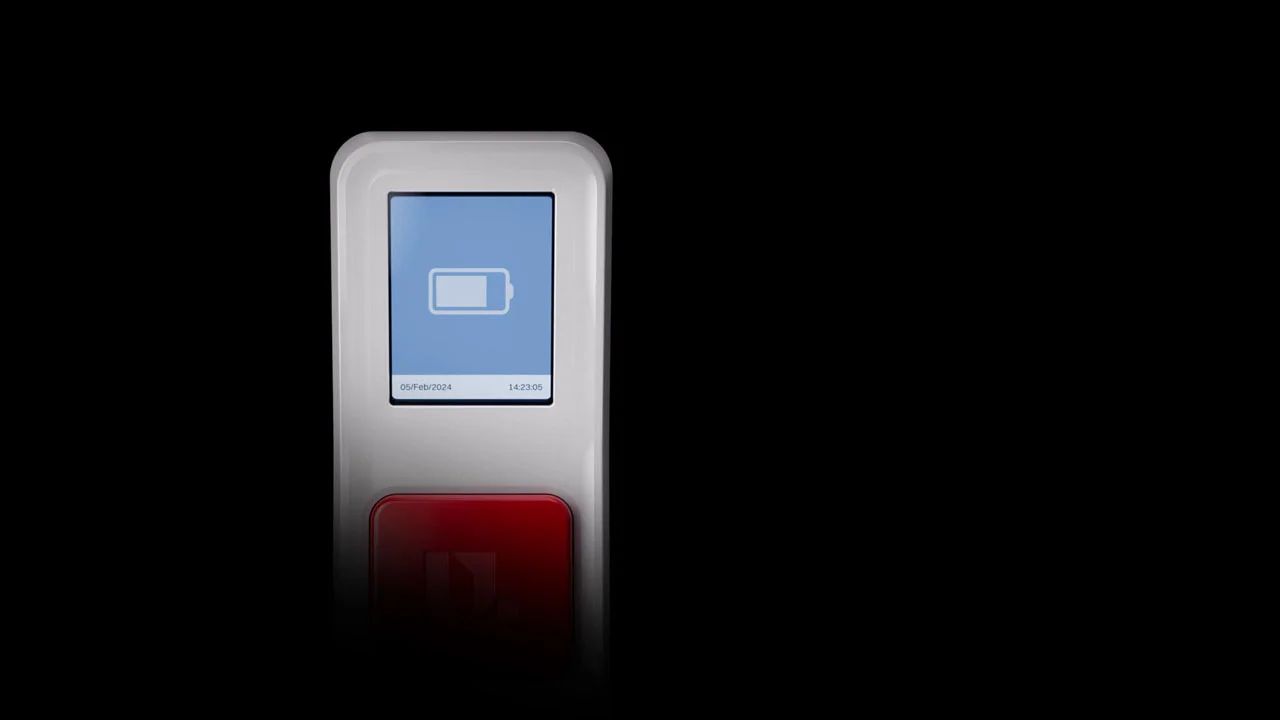
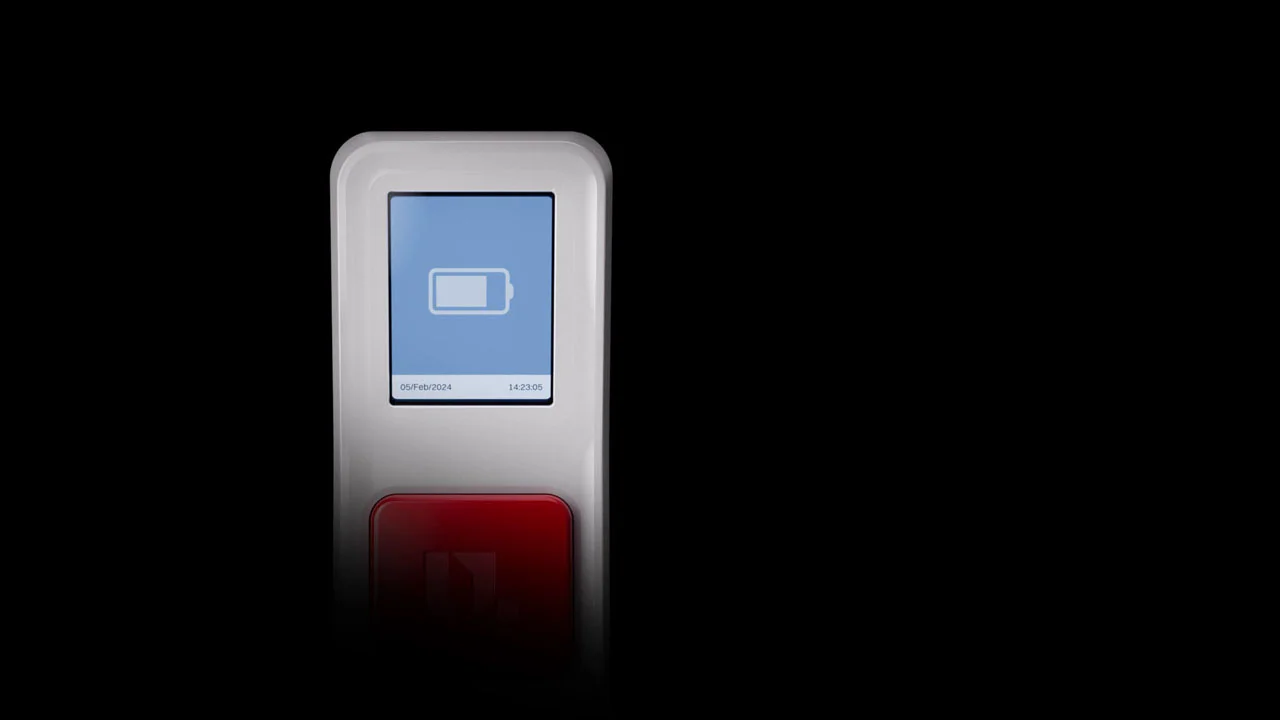
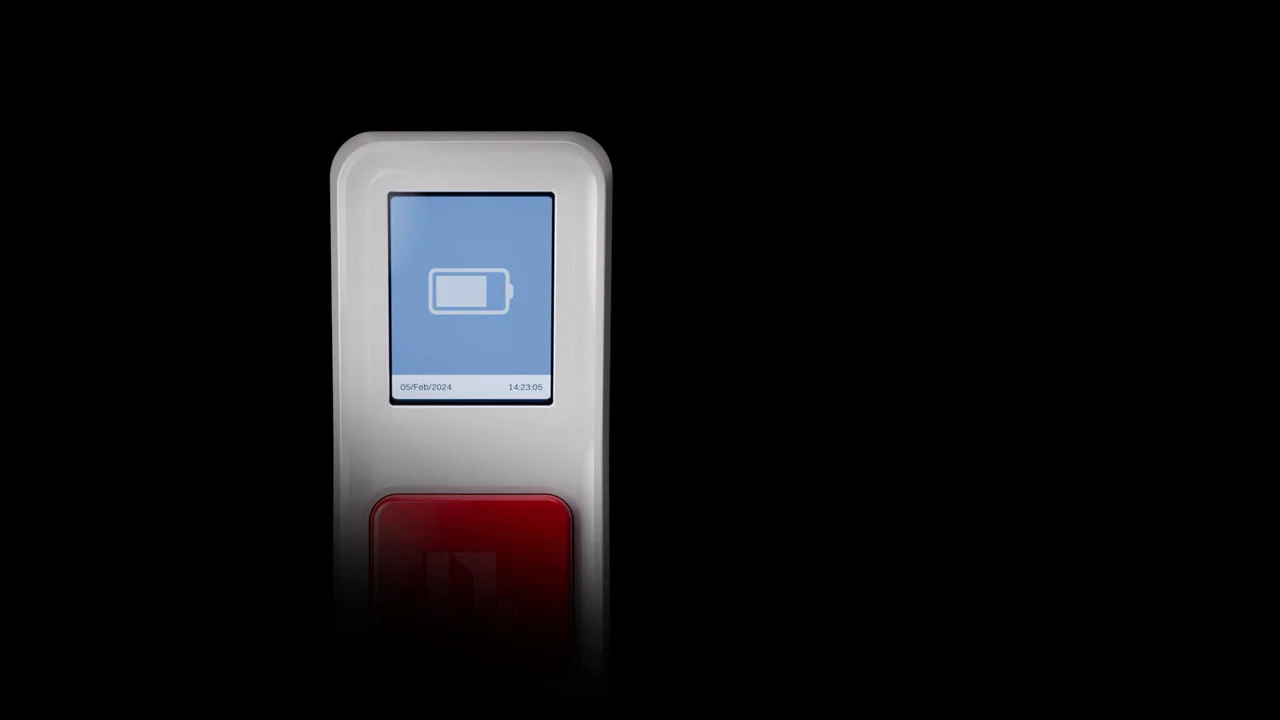
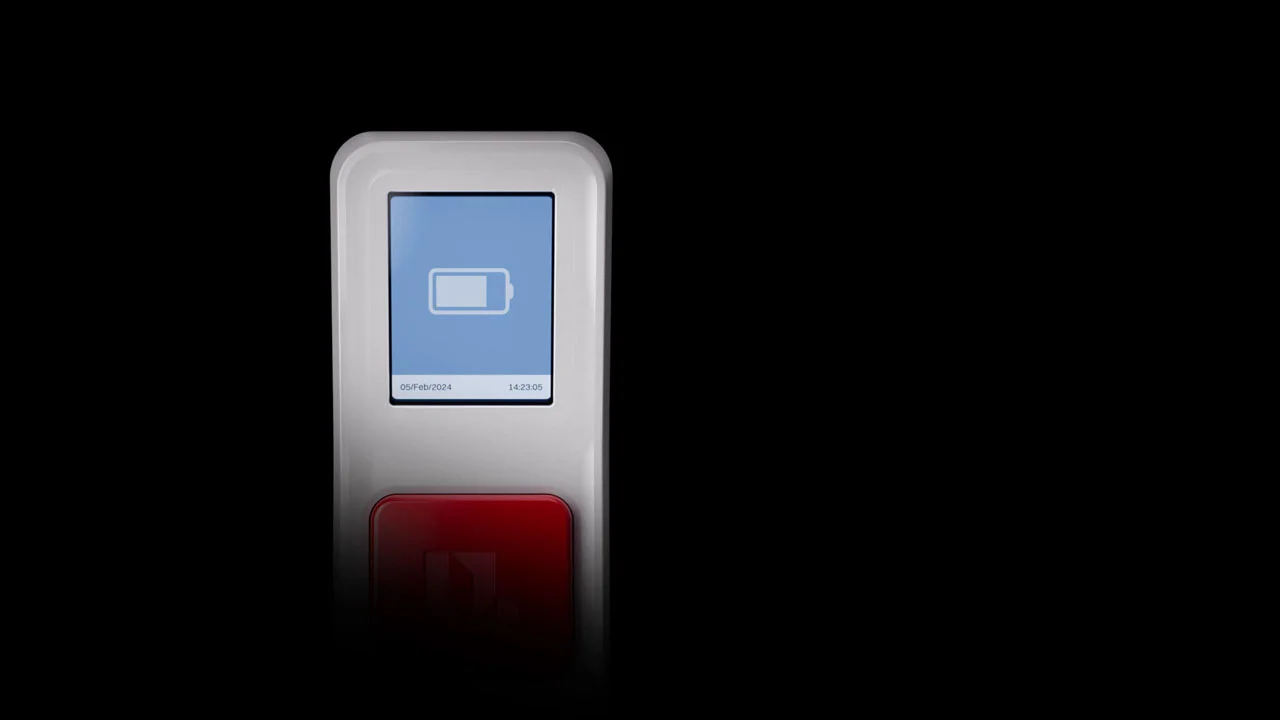
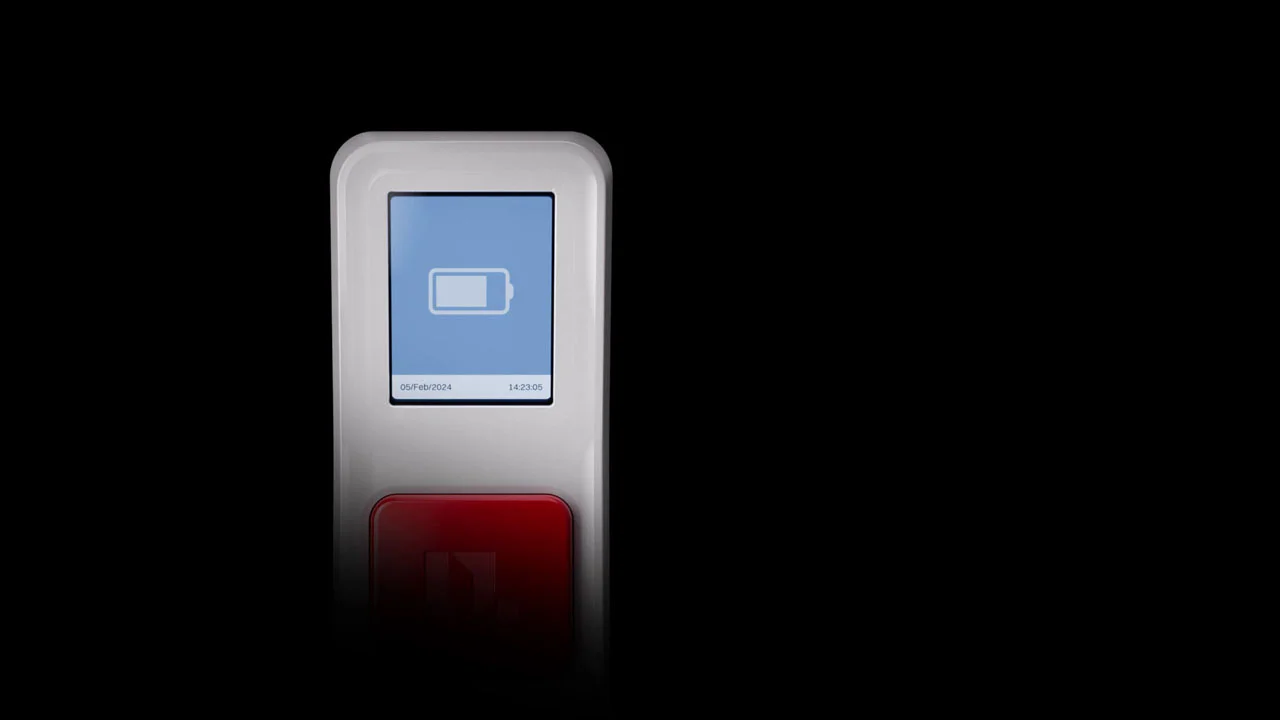
Pressing the red button in every time it's compeleted will update the production quantity and average SMV in real time.
Productivity can be measured accurately by collecting 30 vibration datas per second of sewing machines while production.
Even if there is a power outage at the factory, or the internet is disconnected, we operate stably through the battery to collect data without any issues.
How the vibration of sewing machines become data
Colleting of
microvibration on site
Colleting of microvibration on site
Production vibration collection of sewing machine
Fine Vibration
+ Production Vibration
Fine Vibration + Production Vibration
Colleting of microvibration on site
Production vibration collection of sewing machine
Fine Vibration + Production Vibration
Actual usage example

For industrial digital transformation,
a neural network connecting the physical and virtual area is required.
For industrial digital transformation, a neural network connecting the physical and virtual area is required.
For industrial digital transformation, a neural network connecting the physical and virtual area is required.

The system constructs a neural network of information
that can be utilized in MES, ERP (Levels 4 and 5)
for the current status of the factory (Level 1).
The system constructs a neural network of information
that can be utilized in MES, ERP (Levels 4 and 5)
for the current status of the factory (Level 1).
Digital
neural network
area
Digital
neural network area
Level 1
Level 1
Field Device Status in the factory
Field Device Status in the factory
Import, Inspection, Cutting, Production, QC, Trimming, Export
Level 2
Level 2
Control Network information collection
Control Network information collection
RTU, PLC, communication protocol, parameter control
RTU, PLC, communication protocol, parameter control
Level 3
Level 3
RTU, PLC, communication protocol, parameter control
Process Network Information Transmission
Process Network Information Transmission
Database Management (SCADA, KANBAN)

Level 4
Manage&Operate Production Execution
MES

Level 4
Manage&Operate Production Execution
MES

Level 4
Manage&Operate Production Execution
MES

Level 5
Corporation System Resource Management
ERP

Level 5
Corporation System Resource Management
ERP

Level 5
Corporation System Resource Management
ERP
SMV calculated through
vibration data, production efficiency, and loss rate
SMV calculated through vibration data, production efficiency, and loss rate

1
1
SMV
SMV
Standard Working Hours:
Time focused on production

2
2
Efficiency
Efficiency
Efficiency of production:
Allowable standard time

3
3
Loss Rate
Loss Rate
Non-Productive Time:
Wasted time during working hours
SMV calculated through vibration data, production efficiency, and loss rate
Through data parsing, quickly identify the SMV.
Derive SMV from numerous cycle times performed in a single process.
We compare the analyzed SMV with the actual production cycle time in real time.
Through data parsing, quickly identify the SMV.
Derive SMV from numerous cycle times performed in a single process.
We compare the analyzed SMV with the actual production cycle time in real time.
Through data parsing, quickly identify the SMV.
Derive SMV from numerous cycle times performed in a single process.
We compare the analyzed SMV with the actual production cycle time in real time.
Through data parsing, quickly identify the SMV.
Derive SMV from numerous cycle times performed in a single process.
We compare the analyzed SMV with the actual production cycle time in real time.
Through data parsing, quickly identify the SMV.
Derive SMV from numerous cycle times performed in a single process.
We compare the analyzed SMV with the actual production cycle time in real time.
Through data parsing, quickly identify the SMV.
Derive SMV from numerous cycle times performed in a single process.
We compare the analyzed SMV with the actual production cycle time in real time.
Through data parsing, quickly identify the SMV.
Derive SMV from numerous cycle times performed in a single process.
We compare the analyzed SMV with the actual production cycle time in real time.
Through data parsing, quickly identify the SMV.
Derive SMV from numerous cycle times performed in a single process.
We compare the analyzed SMV with the actual production cycle time in real time.
Carrying out effective improvement activities
to increase production efficiency with data.
By quantifying the roughly estimated efficiency,
you can establish efficiency enhancement strategies
for processes, lines, and plants.
Carrying out effective improvement activities
to increase production efficiency with data.
By quantifying the roughly estimated efficiency,
you can establish efficiency enhancement strategies
for processes, lines, and plants.
Analysis of work patterns
Analysis of work patterns
Notify when abnormal patterns occur
based on repetitive patterns
Notify when abnormal patterns occur
based on repetitive patterns
Line Balancing
Line Balancing
Indicate and address imbalances in workload
per process
Indicate and address imbalances in workload
per process

1
2
3
4
5
6

1
2
3
4
5
6

1
2
3
4
5
6

1
2
3
4
5
6




Individual management of worker
Individual management of worker
Human resources management
as a quantitative measure of workers' productivity skills
Human resources management
as a quantitative measure of workers' productivity skills





The transmitted data is provided as real-time indicators
so that problems can be immediately resolved
through on-site monitoring.

The transmitted data is provided as real-time indicators
so that problems can be immediately resolved
through on-site monitoring.
The transmitted data is provided as real-time indicators
so that problems can be immediately resolved
through on-site monitoring.


The data analyzing actual production time is stored in the DB,
and it becomes the process analysis data of Monolis.
Company name
Sije
Customer Service
+82(0)2-1644-4386
sije@sijecorp.com
Address
Main Office: 12, Gaetbeol-ro, Yeonsu-gu, Incheon, Republic of Korea
R&D Center: 14-6, Teheran-ro 78-gil, Gangnam-gu, Seoul, Republic of Korea
CEO: Shin In Jun Business Registration No: 444-88-02133
Copyright© 2024, Sije. All right reserved.

Company name
Sije
Customer Service
+82(0)2-1644-4386
sije@sijecorp.com
Address
Main Office: 12, Gaetbeol-ro, Yeonsu-gu, Incheon, Republic of Korea
R&D Center: 14-6, Teheran-ro 78-gil, Gangnam-gu, Seoul, Republic of Korea
CEO: Shin In Jun Business Registration No: 444-88-02133
Copyright© 2024, Sije. All right reserved.


+82(0)2-1644-4386
sije@sijecorp.com
Main Office
12, Gaetbeol-ro, Yeonsu-gu, Incheon, Republic of Korea
R&D Center
14-6, Teheran-ro 78-gil, Gangnam-gu, Seoul, Republic of Korea
CEO : Shin In Jun
Business Registration No : 444-88-02133
Copyright © 2024, Sije. All rights reserved.
The data analyzing actual production time is stored in the DB, and it becomes Monolis' process analysis data.

